
- Table of Contents
- Random Entry
- Chronological
- Editorial Information
- About the SEP
- Editorial Board
- How to Cite the SEP
- Special Characters
- Advanced Tools
- Support the SEP
- PDFs for SEP Friends
- Make a Donation
- SEPIA for Libraries
- Entry Contents
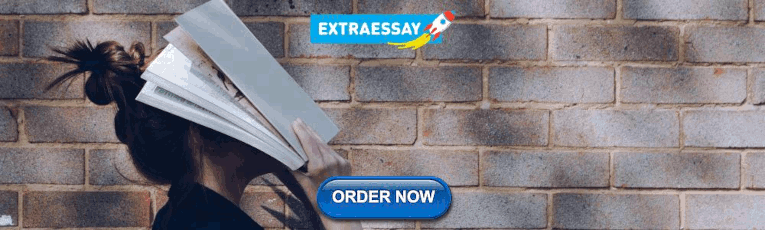
Bibliography
Academic tools.
- Friends PDF Preview
- Author and Citation Info
- Back to Top
Scientific Research and Big Data
Big Data promises to revolutionise the production of knowledge within and beyond science, by enabling novel, highly efficient ways to plan, conduct, disseminate and assess research. The last few decades have witnessed the creation of novel ways to produce, store, and analyse data, culminating in the emergence of the field of data science , which brings together computational, algorithmic, statistical and mathematical techniques towards extrapolating knowledge from big data. At the same time, the Open Data movement—emerging from policy trends such as the push for Open Government and Open Science—has encouraged the sharing and interlinking of heterogeneous research data via large digital infrastructures. The availability of vast amounts of data in machine-readable formats provides an incentive to create efficient procedures to collect, organise, visualise and model these data. These infrastructures, in turn, serve as platforms for the development of artificial intelligence, with an eye to increasing the reliability, speed and transparency of processes of knowledge creation. Researchers across all disciplines see the newfound ability to link and cross-reference data from diverse sources as improving the accuracy and predictive power of scientific findings and helping to identify future directions of inquiry, thus ultimately providing a novel starting point for empirical investigation. As exemplified by the rise of dedicated funding, training programmes and publication venues, big data are widely viewed as ushering in a new way of performing research and challenging existing understandings of what counts as scientific knowledge.
This entry explores these claims in relation to the use of big data within scientific research, and with an emphasis on the philosophical issues emerging from such use. To this aim, the entry discusses how the emergence of big data—and related technologies, institutions and norms—informs the analysis of the following themes:
- how statistics, formal and computational models help to extrapolate patterns from data, and with which consequences;
- the role of critical scrutiny (human intelligence) in machine learning, and its relation to the intelligibility of research processes;
- the nature of data as research components;
- the relation between data and evidence, and the role of data as source of empirical insight;
- the view of knowledge as theory-centric;
- understandings of the relation between prediction and causality;
- the separation of fact and value; and
- the risks and ethics of data science.
These are areas where attention to research practices revolving around big data can benefit philosophy, and particularly work in the epistemology and methodology of science. This entry doesn’t cover the vast scholarship in the history and social studies of science that has emerged in recent years on this topic, though references to some of that literature can be found when conceptually relevant. Complementing historical and social scientific work in data studies, the philosophical analysis of data practices can also elicit significant challenges to the hype surrounding data science and foster a critical understanding of the role of data-fuelled artificial intelligence in research.
1. What Are Big Data?
2. extrapolating data patterns: the role of statistics and software, 3. human and artificial intelligence, 4. the nature of (big) data, 5. big data and evidence, 6. big data, knowledge and inquiry, 7. big data between causation and prediction, 8. the fact/value distinction, 9. big data risks and the ethics of data science, 10. conclusion: big data and good science, other internet resources, related entries.
We are witnessing a progressive “datafication” of social life. Human activities and interactions with the environment are being monitored and recorded with increasing effectiveness, generating an enormous digital footprint. The resulting “big data” are a treasure trove for research, with ever more sophisticated computational tools being developed to extract knowledge from such data. One example is the use of various different types of data acquired from cancer patients, including genomic sequences, physiological measurements and individual responses to treatment, to improve diagnosis and treatment. Another example is the integration of data on traffic flow, environmental and geographical conditions, and human behaviour to produce safety measures for driverless vehicles, so that when confronted with unforeseen events (such as a child suddenly darting into the street on a very cold day), the data can be promptly analysed to identify and generate an appropriate response (the car swerving enough to avoid the child while also minimising the risk of skidding on ice and damaging to other vehicles). Yet another instance is the understanding of the nutritional status and needs of a particular population that can be extracted from combining data on food consumption generated by commercial services (e.g., supermarkets, social media and restaurants) with data coming from public health and social services, such as blood test results and hospital intakes linked to malnutrition. In each of these cases, the availability of data and related analytic tools is creating novel opportunities for research and for the development of new forms of inquiry, which are widely perceived as having a transformative effect on science as a whole.
A useful starting point in reflecting on the significance of such cases for a philosophical understanding of research is to consider what the term “big data” actually refers to within contemporary scientific discourse. There are multiple ways to define big data (Kitchin 2014, Kitchin & McArdle 2016). Perhaps the most straightforward characterisation is as large datasets that are produced in a digital form and can be analysed through computational tools. Hence the two features most commonly associated with Big Data are volume and velocity. Volume refers to the size of the files used to archive and spread data. Velocity refers to the pressing speed with which data is generated and processed. The body of digital data created by research is growing at breakneck pace and in ways that are arguably impossible for the human cognitive system to grasp and thus require some form of automated analysis.
Volume and velocity are also, however, the most disputed features of big data. What may be perceived as “large volume” or “high velocity” depends on rapidly evolving technologies to generate, store, disseminate and visualise the data. This is exemplified by the high-throughput production, storage and dissemination of genomic sequencing and gene expression data, where both data volume and velocity have dramatically increased within the last two decades. Similarly, current understandings of big data as “anything that cannot be easily captured in an Excel spreadsheet” are bound to shift rapidly as new analytic software becomes established, and the very idea of using spreadsheets to capture data becomes a thing of the past. Moreover, data size and speed do not take account of the diversity of data types used by researchers, which may include data that are not generated in digital formats or whose format is not computationally tractable, and which underscores the importance of data provenance (that is, the conditions under which data were generated and disseminated) to processes of inference and interpretation. And as discussed below, the emphasis on physical features of data obscures the continuing dependence of data interpretation on circumstances of data use, including specific queries, values, skills and research situations.
An alternative is to define big data not by reference to their physical attributes, but rather by virtue of what can and cannot be done with them. In this view, big data is a heterogeneous ensemble of data collected from a variety of different sources, typically (but not always) in digital formats suitable for algorithmic processing, in order to generate new knowledge. For example boyd and Crawford (2012: 663) identify big data with “the capacity to search, aggregate and cross-reference large datasets”, while O’Malley and Soyer (2012) focus on the ability to interrogate and interrelate diverse types of data, with the aim to be able to consult them as a single body of evidence. The examples of transformative “big data research” given above are all easily fitted into this view: it is not the mere fact that lots of data are available that makes a different in those cases, but rather the fact that lots of data can be mobilised from a wide variety of sources (medical records, environmental surveys, weather measurements, consumer behaviour). This account makes sense of other characteristic “v-words” that have been associated with big data, including:
- Variety in the formats and purposes of data, which may include objects as different as samples of animal tissue, free-text observations, humidity measurements, GPS coordinates, and the results of blood tests;
- Veracity , understood as the extent to which the quality and reliability of big data can be guaranteed. Data with high volume, velocity and variety are at significant risk of containing inaccuracies, errors and unaccounted-for bias. In the absence of appropriate validation and quality checks, this could result in a misleading or outright incorrect evidence base for knowledge claims (Floridi & Illari 2014; Cai & Zhu 2015; Leonelli 2017);
- Validity , which indicates the selection of appropriate data with respect to the intended use. The choice of a specific dataset as evidence base requires adequate and explicit justification, including recourse to relevant background knowledge to ground the identification of what counts as data in that context (e.g., Loettgers 2009, Bogen 2010);
- Volatility , i.e., the extent to which data can be relied upon to remain available, accessible and re-interpretable despite changes in archival technologies. This is significant given the tendency of formats and tools used to generate and analyse data to become obsolete, and the efforts required to update data infrastructures so as to guarantee data access in the long term (Bowker 2006; Edwards 2010; Lagoze 2014; Borgman 2015);
- Value , i.e., the multifaceted forms of significance attributed to big data by different sections of society, which depend as much on the intended use of the data as on historical, social and geographical circumstances (Leonelli 2016, D’Ignazio and Klein 2020). Alongside scientific value, researchers may impute financial, ethical, reputational and even affective value to data, depending on their intended use as well as the historical, social and geographical circumstances of their use. The institutions involved in governing and funding research also have ways of valuing data, which may not always overlap with the priorities of researchers (Tempini 2017).
This list of features, though not exhaustive, highlights how big data is not simply “a lot of data”. The epistemic power of big data lies in their capacity to bridge between different research communities, methodological approaches and theoretical frameworks that are difficult to link due to conceptual fragmentation, social barriers and technical difficulties (Leonelli 2019a). And indeed, appeals to big data often emerge from situations of inquiry that are at once technically, conceptually and socially challenging, and where existing methods and resources have proved insufficient or inadequate (Sterner & Franz 2017; Sterner, Franz, & Witteveen 2020).
This understanding of big data is rooted in a long history of researchers grappling with large and complex datasets, as exemplified by fields like astronomy, meteorology, taxonomy and demography (see the collections assembled by Daston 2017; Anorova et al. 2017; Porter & Chaderavian 2018; as well as Anorova et al. 2010, Sepkoski 2013, Stevens 2016, Strasser 2019 among others). Similarly, biomedical research—and particularly subfields such as epidemiology, pharmacology and public health—has an extensive tradition of tackling data of high volume, velocity, variety and volatility, and whose validity, veracity and value are regularly negotiated and contested by patients, governments, funders, pharmaceutical companies, insurances and public institutions (Bauer 2008). Throughout the twentieth century, these efforts spurred the development of techniques, institutions and instruments to collect, order, visualise and analyse data, such as: standard classification systems and formats; guidelines, tools and legislation for the management and security of sensitive data; and infrastructures to integrate and sustain data collections over long periods of time (Daston 2017).
This work culminated in the application of computational technologies, modelling tools and statistical methods to big data (Porter 1995; Humphreys 2004; Edwards 2010), increasingly pushing the boundaries of data analytics thanks to supervised learning, model fitting, deep neural networks, search and optimisation methods, complex data visualisations and various other tools now associated with artificial intelligence. Many of these tools are based on algorithms whose functioning and results are tested against specific data samples (a process called “training”). These algorithms are programmed to “learn” from each interaction with novel data: in other words, they have the capacity to change themselves in response to new information being inputted into the system, thus becoming more attuned to the phenomena they are analysing and improving their ability to predict future behaviour. The scope and extent of such changes is shaped by the assumptions used to build the algorithms and the capability of related software and hardware to identify, access and process information of relevance to the learning in question. There is however a degree of unpredictability and opacity to these systems, which can evolve to the point of defying human understanding (more on this below).
New institutions, communication platforms and regulatory frameworks also emerged to assemble, prepare and maintain data for such uses (Kitchin 2014), such as various forms of digital data infrastructures, organisations aiming to coordinate and improve the global data landscape (e.g., the Research Data Alliance), and novel measures for data protection, like the General Data Protection Regulation launched in 2017 by the European Union. Together, these techniques and institutions afford the opportunity to assemble and interpret data at a much broader scale, while also promising to deliver finer levels of granularity in data analysis. [ 1 ] They increase the scope of any investigation by making it possible for researchers to link their own findings to those of countless others across the world, both within and beyond the academic sphere. By enhancing the mobility of data, they facilitate their repurposing for a variety of goals that may have been unforeseeable when the data were originally generated. And by transforming the role of data within research, they heighten their status as valuable research outputs in and of themselves. These technological and methodological developments have significant implications for philosophical conceptualisations of data, inferential processes and scientific knowledge, as well as for how research is conducted, organised, governed and assessed. It is to these philosophical concerns that I now turn.
Big data are often associated to the idea of data-driven research, where learning happens through the accumulation of data and the application of methods to extract meaningful patterns from those data. Within data-driven inquiry, researchers are expected to use data as their starting point for inductive inference, without relying on theoretical preconceptions—a situation described by advocates as “the end of theory”, in contrast to theory-driven approaches where research consists of testing a hypothesis (Anderson 2008, Hey et al. 2009). In principle at least, big data constitute the largest pool of data ever assembled and thus a strong starting point to search for correlations (Mayer-Schönberger & Cukier 2013). Crucial to the credibility of the data-driven approach is the efficacy of the methods used to extrapolate patterns from data and evaluate whether or not such patterns are meaningful, and what “meaning” may involve in the first place. Hence, some philosophers and data scholars have argued that
the most important and distinctive characteristic of Big Data [is] its use of statistical methods and computational means of analysis, (Symons & Alvarado 2016: 4)
such as for instance machine learning tools, deep neural networks and other “intelligent” practices of data handling.
The emphasis on statistics as key adjudicator of validity and reliability of patterns extracted from data is not novel. Exponents of logical empiricism looked for logically watertight methods to secure and justify inference from data, and their efforts to develop a theory of probability proceeded in parallel with the entrenchment of statistical reasoning in the sciences in the first half of the twentieth century (Romeijn 2017). In the early 1960s, Patrick Suppes offered a seminal link between statistical methods and the philosophy of science through his work on the production and interpretation of data models. As a philosopher deeply embedded in experimental practice, Suppes was interested in the means and motivations of key statistical procedures for data analysis such as data reduction and curve fitting. He argued that once data are adequately prepared for statistical modelling, all the concerns and choices that motivated data processing become irrelevant to their analysis and interpretation. This inspired him to differentiate between models of theory, models of experiment and models of data, noting that such different components of inquiry are governed by different logics and cannot be compared in a straightforward way. For instance,
the precise definition of models of the data for any given experiment requires that there be a theory of the data in the sense of the experimental procedure, as well as in the ordinary sense of the empirical theory of the phenomena being studied. (Suppes 1962: 253)
Suppes viewed data models as necessarily statistical: that is, as objects
designed to incorporate all the information about the experiment which can be used in statistical tests of the adequacy of the theory. (Suppes 1962: 258)
His formal definition of data models reflects this decision, with statistical requirements such as homogeneity, stationarity and order identified as the ultimate criteria to identify a data model Z and evaluate its adequacy:
Z is an N-fold model of the data for experiment Y if and only if there is a set Y and a probability measure P on subsets of Y such that \(Y = \langle Y, P\rangle\) is a model of the theory of the experiment, Z is an N-tuple of elements of Y , and Z satisfies the statistical tests of homogeneity, stationarity and order. (1962: 259)
This analysis of data models portrayed statistical methods as key conduits between data and theory, and hence as crucial components of inferential reasoning.
The focus on statistics as entry point to discussions of inference from data was widely promoted in subsequent philosophical work. Prominent examples include Deborah Mayo, who in her book Error and the Growth of Experimental Knowledge asked:
What should be included in data models? The overriding constraint is the need for data models that permit the statistical assessment of fit (between prediction and actual data); (Mayo 1996: 136)
and Bas van Fraassen, who also embraced the idea of data models as “summarizing relative frequencies found in data” (Van Fraassen 2008: 167). Closely related is the emphasis on statistics as means to detect error within datasets in relation to specific hypotheses, most prominently endorsed by the error-statistical approach to inference championed by Mayo and Aris Spanos (Mayo & Spanos 2009a). This approach aligns with the emphasis on computational methods for data analysis within big data research, and supports the idea that the better the inferential tools and methods, the better the chance to extract reliable knowledge from data.
When it comes to addressing methodological challenges arising from the computational analysis of big data, however, statistical expertise needs to be complemented by computational savvy in the training and application of algorithms associated to artificial intelligence, including machine learning but also other mathematical procedures for operating upon data (Bringsjord & Govindarajulu 2018). Consider for instance the problem of overfitting, i.e., the mistaken identification of patterns in a dataset, which can be greatly amplified by the training techniques employed by machine learning algorithms. There is no guarantee that an algorithm trained to successfully extrapolate patterns from a given dataset will be as successful when applied to other data. Common approaches to this problem involve the re-ordering and partitioning of both data and training methods, so that it is possible to compare the application of the same algorithms to different subsets of the data (“cross-validation”), combine predictions arising from differently trained algorithms (“ensembling”) or use hyperparameters (parameters whose value is set prior to data training) to prepare the data for analysis.
Handling these issues, in turn, requires
familiarity with the mathematical operations in question, their implementations in code, and the hardware architectures underlying such implementations. (Lowrie 2017: 3)
For instance, machine learning
aims to build programs that develop their own analytic or descriptive approaches to a body of data, rather than employing ready-made solutions such as rule-based deduction or the regressions of more traditional statistics. (Lowrie 2017: 4)
In other words, statistics and mathematics need to be complemented by expertise in programming and computer engineering. The ensemble of skills thus construed results in a specific epistemological approach to research, which is broadly characterised by an emphasis on the means of inquiry as the most significant driver of research goals and outputs. This approach, which Sabina Leonelli characterised as data-centric , involves “focusing more on the processes through which research is carried out than on its ultimate outcomes” (Leonelli 2016: 170). In this view, procedures, techniques, methods, software and hardware are the prime motors of inquiry and the chief influence on its outcomes. Focusing more specifically on computational systems, John Symons and Jack Horner argued that much of big data research consists of software-intensive science rather than data-driven research: that is, science that depends on software for its design, development, deployment and use, and thus encompasses procedures, types of reasoning and errors that are unique to software, such as for example the problems generated by attempts to map real-world quantities to discrete-state machines, or approximating numerical operations (Symons & Horner 2014: 473). Software-intensive science is arguably supported by an algorithmic rationality focused on the feasibility, practicality and efficiency of algorithms, which is typically assessed by reference to concrete situations of inquiry (Lowrie 2017).
Algorithms are enormously varied in their mathematical structures and underpinning conceptual commitments, and more philosophical work needs to be carried out on the specifics of computational tools and software used in data science and related applications—with emerging work in philosophy of computer science providing an excellent way forward (Turner & Angius 2019). Nevertheless, it is clear that whether or not a given algorithm successfully applies to the data at hand depends on factors that cannot be controlled through statistical or even computational methods: for instance, the size, structure and format of the data, the nature of the classifiers used to partition the data, the complexity of decision boundaries and the very goals of the investigation.
In a forceful critique informed by the philosophy of mathematics, Christian Calude and Giuseppe Longo argued that there is a fundamental problem with the assumption that more data will necessarily yield more information:
very large databases have to contain arbitrary correlations. These correlations appear only due to the size, not the nature, of data. (Calude & Longo 2017: 595)
They conclude that big data analysis is by definition unable to distinguish spurious from meaningful correlations and is therefore a threat to scientific research. A related worry, sometimes dubbed “the curse of dimensionality” by data scientists, concerns the extent to which the analysis of a given dataset can be scaled up in complexity and in the number of variables being considered. It is well known that the more dimensions one considers in classifying samples, for example, the larger the dataset on which such dimensions can be accurately generalised. This demonstrates the continuing, tight dependence between the volume and quality of data on the one hand, and the type and breadth of research questions for which data need to serve as evidence on the other hand.
Determining the fit between inferential methods and data requires high levels of expertise and contextual judgement (a situation known within machine learning as the “no free lunch theorem”). Indeed, overreliance on software for inference and data modelling can yield highly problematic results. Symons and Horner note that the use of complex software in big data analysis makes margins of error unknowable, because there is no clear way to test them statistically (Symons & Horner 2014: 473). The path complexity of programs with high conditionality imposes limits on standard error correction techniques. As a consequence, there is no effective method for characterising the error distribution in the software except by testing all paths in the code, which is unrealistic and intractable in the vast majority of cases due to the complexity of the code.
Rather than acting as a substitute, the effective and responsible use of artificial intelligence tools in big data analysis requires the strategic exercise of human intelligence—but for this to happen, AI systems applied to big data need to be accessible to scrutiny and modification. Whether or not this is the case, and who is best qualified to exercise such scrutiny, is under dispute. Thomas Nickles argued that the increasingly complex and distributed algorithms used for data analysis follow in the footsteps of long-standing scientific attempts to transcend the limits of human cognition. The resulting epistemic systems may no longer be intelligible to humans: an “alien intelligence” within which “human abilities are no longer the ultimate criteria of epistemic success” (Nickles forthcoming). Such unbound cognition holds the promise of enabling powerful inferential reasoning from previously unimaginable volumes of data. The difficulties in contextualising and scrutinising such reasoning, however, sheds doubt on the reliability of the results. It is not only machine learning algorithms that are becoming increasingly inaccessible to evaluation: beyond the complexities of programming code, computational data analysis requires a whole ecosystem of classifications, models, networks and inference tools which typically have different histories and purposes, and whose relation to each other—and effects when they are used together—are far from understood and may well be untraceable.
This raises the question of whether the knowledge produced by such data analytic systems is at all intelligible to humans, and if so, what forms of intelligibility it yields. It is certainly the case that deriving knowledge from big data may not involve an increase in human understanding, especially if understanding is understood as an epistemic skill (de Regt 2017). This may not be a problem to those who await the rise of a new species of intelligent machines, who may master new cognitive tools in a way that humans cannot. But as Nickles, Nicholas Rescher (1984), Werner Callebaut (2012) and others pointed out, even in that case “we would not have arrived at perspective-free science” (Nickles forthcoming). While the human histories and assumptions interwoven into these systems may be hard to disentangle, they still affect their outcomes; and whether or not these processes of inquiry are open to critical scrutiny, their telos, implications and significance for life on the planet arguably should be. As argued by Dan McQuillan (2018), the increasing automation of big data analytics may foster acceptance of a Neoplatonist machinic metaphysics , within which mathematical structures “uncovered” by AI would trump any appeal to human experience. Luciano Floridi echoes this intuition in his analysis of what he calls the infosphere :
The great opportunities offered by Information and Communication Technologies come with a huge intellectual responsibility to understand them and take advantage of them in the right way. (2014: vii)
These considerations parallel Paul Humphreys’s long-standing critique of computer simulations as epistemically opaque (Humphreys 2004, 2009)—and particularly his definition of what he calls essential epistemic opacity:
A process is essentially epistemically opaque to X if and only if it is impossible , given the nature of X , for X to know all of the epistemically relevant elements of the process. (Humphreys 2009: 618)
Different facets of the general problem of epistemic opacity are stressed within the vast philosophical scholarship on the role of modelling, computing and simulations in the sciences: the implications of lacking experimental access to the concrete parts of the world being modelled, for instance (Morgan 2005; Parker 2009; Radder 2009); the difficulties in testing the reliability of computational methods used within simulations (Winsberg 2010; Morrison 2015); the relation between opacity and justification (Durán & Formanek 2018); the forms of black-boxing associated to mechanistic reasoning implemented in computational analysis (Craver and Darden 2013; Bechtel 2016); and the debate over the intrinsic limits of computational approaches and related expertise (Collins 1990; Dreyfus 1992). Roman Frigg and Julian Reiss argued that such issues do not constitute fundamental challenges to the nature of inquiry and modelling, and in fact exist in a continuum with traditional methodological issues well-known within the sciences (Frigg & Reiss 2009). Whether or not one agrees with this position (Humphreys 2009; Beisbart 2012), big data analysis is clearly pushing computational and statistical methods to their limit, thus highlighting the boundaries to what even technologically augmented human beings are capable of knowing and understanding.
Research on big data analysis thus sheds light on elements of the research process that cannot be fully controlled, rationalised or even considered through recourse to formal tools.
One such element is the work required to present empirical data in a machine-readable format that is compatible with the software and analytic tools at hand. Data need to be selected, cleaned and prepared to be subjected to statistical and computational analysis. The processes involved in separating data from noise, clustering data so that it is tractable, and integrating data of different formats turn out to be highly sophisticated and theoretically structured, as demonstrated for instance by James McAllister’s (1997, 2007, 2011) and Uljana Feest’s (2011) work on data patterns, Marcel Boumans’s and Leonelli’s comparison of clustering principles across fields (forthcoming), and James Griesemer’s (forthcoming) and Mary Morgan’s (forthcoming) analyses of the peculiarities of datasets. Suppes was so concerned by what he called the “bewildering complexity” of data production and processing activities, that he worried that philosophers would not appreciate the ways in which statistics can and does help scientists to abstract data away from such complexity. He described the large group of research components and activities used to prepare data for modelling as “pragmatic aspects” encompassing “every intuitive consideration of experimental design that involved no formal statistics” (Suppes 1962: 258), and positioned them as the lowest step of his hierarchy of models—at the opposite end of its pinnacle, which are models of theory. Despite recent efforts to rehabilitate the methodology of inductive-statistical modelling and inference (Mayo & Spanos 2009b), this approach has been shared by many philosophers who regard processes of data production and processing as so chaotic as to defy systematic analysis. This explains why data have received so little consideration in philosophy of science when compared to models and theory.
The question of how data are defined and identified, however, is crucial for understanding the role of big data in scientific research. Let us now consider two philosophical views—the representational view and the relational view —that are both compatible with the emergence of big data, and yet place emphasis on different aspects of that phenomenon, with significant implications for understanding the role of data within inferential reasoning and, as we shall see in the next section, as evidence. The representational view construes data as reliable representations of reality which are produced via the interaction between humans and the world. The interactions that generate data can take place in any social setting regardless of research purposes. Examples range from a biologist measuring the circumference of a cell in the lab and noting the result in an Excel file, to a teacher counting the number of students in her class and transcribing it in the class register. What counts as data in these interactions are the objects created in the process of description and/or measurement of the world. These objects can be digital (the Excel file) or physical (the class register) and form a footprint of a specific interaction with the natural world. This footprint—“trace” or “mark”, in the words of Ian Hacking (1992) and Hans-Jörg Rheinberger (2011), respectively—constitutes a crucial reference point for analytic study and for the extraction of new insights. This is the reason why data forms a legitimate foundation to empirical knowledge: the production of data is equivalent to “capturing” features of the world that can be used for systematic study. According to the representative approach, data are objects with fixed and unchangeable content, whose meaning, in virtue of being representations of reality, needs to be investigated and revealed step-by-step through adequate inferential methods. The data documenting cell shape can be modelled to test the relevance of shape to the elasticity, permeability and resilience of cells, producing an evidence base to understand cell-to-cell signalling and development. The data produced counting students in class can be aggregated with similar data collected in other schools, producing an evidence base to evaluate the density of students in the area and their school attendance frequency.
This reflects the intuition that data, especially when they come in the form of numerical measurements or images such as photographs, somehow mirror the phenomena that they are created to document, thus providing a snapshot of those phenomena that is amenable to study under the controlled conditions of research. It also reflects the idea of data as “raw” products of research, which are as close as it gets to unmediated knowledge of reality. This makes sense of the truth-value sometimes assigned to data as irrefutable sources of evidence—the Popperian idea that if data are found to support a given claim, then that claim is corroborated as true at least as long as no other data are found to disprove it. Data in this view represent an objective foundation for the acquisition of knowledge and this very objectivity—the ability to derive knowledge from human experience while transcending it—is what makes knowledge empirical. This position is well-aligned with the idea that big data is valuable to science because it facilitates the (broadly understood) inductive accumulation of knowledge: gathering data collected via reliable methods produces a mountain of facts ready to be analysed and, the more facts are produced and connected with each other, the more knowledge can be extracted.
Philosophers have long acknowledged that data do not speak for themselves and different types of data require different tools for analysis and preparation to be interpreted (Bogen 2009 [2013]). According to the representative view, there are correct and incorrect ways of interpreting data, which those responsible for data analysis need to uncover. But what is a “correct” interpretation in the realm of big data, where data are consistently treated as mobile entities that can, at least in principle, be reused in countless different ways and towards different objectives? Perhaps more than at any other time in the history of science, the current mobilisation and re-use of big data highlights the degree to which data interpretation—and with it, whatever data is taken to represent—may differ depending on the conceptual, material and social conditions of inquiry. The analysis of how big data travels across contexts shows that the expectations and abilities of those involved determine not only the way data are interpreted, but also what is regarded as “data” in the first place (Leonelli & Tempini forthcoming). The representative view of data as objects with fixed and contextually independent meaning is at odds with these observations.
An alternative approach is to embrace these findings and abandon the idea of data as fixed representations of reality altogether. Within the relational view , data are objects that are treated as potential or actual evidence for scientific claims in ways that can, at least in principle, be scrutinised and accounted for (Leonelli 2016). The meaning assigned to data depends on their provenance, their physical features and what these features are taken to represent, and the motivations and instruments used to visualise them and to defend specific interpretations. The reliability of data thus depends on the credibility and strictness of the processes used to produce and analyse them. The presentation of data; the way they are identified, selected, and included (or excluded) in databases; and the information provided to users to re-contextualise them are fundamental to producing knowledge and significantly influence its content. For instance, changes in data format—as most obviously involved in digitisation, data compression or archival procedures— can have a significant impact on where, when, and who uses the data as source of knowledge.
This framework acknowledges that any object can be used as a datum, or stop being used as such, depending on the circumstances—a consideration familiar to big data analysts used to pick and mix data coming from a vast variety of sources. The relational view also explains how, depending on the research perspective interpreting it, the same dataset may be used to represent different aspects of the world (“phenomena” as famously characterised by James Bogen and James Woodward, 1988). When considering the full cycle of scientific inquiry from the viewpoint of data production and analysis, it is at the stage of data modelling that a specific representational value is attributed to data (Leonelli 2019b).
The relational view of data encourages attention to the history of data, highlighting their continual evolution and sometimes radical alteration, and the impact of this feature on the power of data to confirm or refute hypotheses. It explains the critical importance of documenting data management and transformation processes, especially with big data that transit far and wide over digital channels and are grouped and interpreted in different ways and formats. It also explains the increasing recognition of the expertise of those who produce, curate, and analyse data as indispensable to the effective interpretation of big data within and beyond the sciences; and the inextricable link between social and ethical concerns around the potential impact of data sharing and scientific concerns around the quality, validity, and security of data (boyd & Crawford 2012; Tempini & Leonelli, 2018).
Depending on which view on data one takes, expectations around what big data can do for science will vary dramatically. The representational view accommodates the idea of big data as providing the most comprehensive, reliable and generative knowledge base ever witnessed in the history of science, by virtue of its sheer size and heterogeneity. The relational view makes no such commitment, focusing instead on what inferences are being drawn from such data at any given point, how and why.
One thing that the representational and relational views agree on is the key epistemic role of data as empirical evidence for knowledge claims or interventions. While there is a large philosophical literature on the nature of evidence (e.g., Achinstein 2001; Reiss 2015; Kelly 2016), however, the relation between data and evidence has received less attention. This is arguably due to an implicit acceptance, by many philosophers, of the representational view of data. Within the representational view, the identification of what counts as data is prior to the study of what those data can be evidence for: in other words, data are “givens”, as the etymology of the word indicates, and inferential methods are responsible for determining whether and how the data available to investigators can be used as evidence, and for what. The focus of philosophical attention is thus on formal methods to single out errors and misleading interpretations, and the probabilistic and/or explanatory relation between what is unproblematically taken to be a body of evidence and a given hypothesis. Hence much of the expansive philosophical work on evidence avoids the term “data” altogether. Peter Achinstein’s seminal work is a case in point: it discusses observed facts and experimental results, and whether and under which conditions scientists would have reasons to believe such facts, but it makes no mention of data and related processing practices (Achinstein 2001).
By contrast, within the relational view an object can only be identified as datum when it is viewed as having value as evidence. Evidence becomes a category of data identification, rather than a category of data use as in the representational view (Canali 2019). Evidence is thus constitutive of the very notion of data and cannot be disentangled from it. This involves accepting that the conditions under which a given object can serve as evidence—and thus be viewed as datum - may change; and that should this evidential role stop altogether, the object would revert back into an ordinary, non-datum item. For example, the photograph of a plant taken by a tourist in a remote region may become relevant as evidence for an inquiry into the morphology of plants from that particular locality; yet most photographs of plants are never considered as evidence for an inquiry into the features and functioning of the world, and of those who are, many may subsequently be discarded as uninteresting or no longer pertinent to the questions being asked.
This view accounts for the mobility and repurposing that characterises big data use, and for the possibility that objects that were not originally generated in order to serve as evidence may be subsequently adopted as such. Consider Mayo and Spanos’s “minimal scientific principle for evidence”, which they define as follows:
Data x 0 provide poor evidence for H if they result from a method or procedure that has little or no ability of finding flaws in H , even if H is false. (Mayo & Spanos 2009b)
This principle is compatible with the relational view of data since it incorporates cases where the methods used to generate and process data may not have been geared towards the testing of a hypothesis H: all it asks is that such methods can be made relevant to the testing of H, at the point in which data are used as evidence for H (I shall come back to the role of hypotheses in the handling of evidence in the next section).
The relational view also highlights the relevance of practices of data formatting and manipulation to the treatment of data as evidence, thus taking attention away from the characteristics of the data objects alone and focusing instead on the agency attached to and enabled by those characteristics. Nora Boyd has provided a way to conceptualise data processing as an integral part of inferential processes, and thus of how we should understand evidence. To this aim she introduced the notion of “line of evidence”, which she defines as:
a sequence of empirical results including the records of data collection and all subsequent products of data processing generated on the way to some final empirical constraint. (Boyd 2018:406)
She thus proposes a conception of evidence that embraces both data and the way in which data are handled, and indeed emphasises the importance of auxiliary information used when assessing data for interpretation, which includes
the metadata regarding the provenance of the data records and the processing workflow that transforms them. (2018: 407)
As she concludes,
together, a line of evidence and its associated metadata compose what I am calling an “enriched line of evidence”. The evidential corpus is then to be made up of many such enriched lines of evidence. (2018: 407)
The relational view thus fosters a functional and contextualist approach to evidence as the manner through which one or more objects are used as warrant for particular knowledge items (which can be propositional claims, but also actions such as specific decisions or modes of conduct/ways of operating). This chimes with the contextual view of evidence defended by Reiss (2015), John Norton’s work on the multiple, tangled lines of inferential reasoning underpinning appeals to induction (2003), and Hasok Chang’s emphasis on the epistemic activities required to ground evidential claims (2012). Building on these ideas and on Stephen Toulmin’s seminal work on research schemas (1958), Alison Wylie has gone one step further in evaluating the inferential scaffolding that researchers (and particularly archaeologists, who so often are called to re-evaluate the same data as evidence for new claims; Wylie 2017) need to make sense of their data, interpret them in ways that are robust to potential challenges, and modify interpretations in the face of new findings. This analysis enabled Wylie to formulate a set of conditions for robust evidential reasoning, which include epistemic security in the chain of evidence, causal anchoring and causal independence of the data used as evidence, as well as the explicit articulation of the grounds for calibration of the instruments and methods involved (Chapman & Wylie 2016; Wylie forthcoming). A similar conclusion is reached by Jessey Wright’s evaluation of the diverse data analysis techniques that neuroscientists use to make sense of functional magnetic resonance imaging of the brain (fMRI scans):
different data analysis techniques reveal different patterns in the data. Through the use of multiple data analysis techniques, researchers can produce results that are locally robust. (Wright 2017: 1179)
Wylie’s and Wright’s analyses exemplify how a relational approach to data fosters a normative understanding of “good evidence” which is anchored in situated judgement—the arguably human prerogative to contextualise and assess the significance of evidential claims. The advantages of this view of evidence are eloquently expressed by Nancy Cartwright’s critique of both philosophical theories and policy approaches that do not recognise the local and contextual nature of evidential reasoning. As she notes,
we need a concept that can give guidance about what is relevant to consider in deciding on the probability of the hypothesis, not one that requires that we already know significant facts about the probability of the hypothesis on various pieces of evidence. (Cartwright 2013: 6)
Thus she argues for a notion of evidence that is not too restrictive, takes account of the difficulties in combining and selecting evidence, and allows for contextual judgement on what types of evidence are best suited to the inquiry at hand (Cartwright 2013, 2019). Reiss’s proposal of a pragmatic theory of evidence similarly aims to
takes scientific practice [..] seriously, both in terms of its greater use of knowledge about the conditions under which science is practised and in terms of its goal to develop insights that are relevant to practising scientists. (Reiss 2015: 361)
A better characterisation of the relation between data and evidence, predicated on the study of how data are processed and aggregated, may go a long way towards addressing these demands. As aptly argued by James Woodward, the evidential relationship between data and claims is not a “a purely formal, logical, or a priori matter” (Woodward 2000: S172–173). This again sits uneasily with the expectation that big data analysis may automate scientific discovery and make human judgement redundant.
Let us now return to the idea of data-driven inquiry, often suggested as a counterpoint to hypothesis-driven science (e.g., Hey et al. 2009). Kevin Elliot and colleagues have offered a brief history of hypothesis-driven inquiry (Elliott et al. 2016), emphasising how scientific institutions (including funding programmes and publication venues) have pushed researchers towards a Popperian conceptualisation of inquiry as the formulation and testing of a strong hypothesis. Big data analysis clearly points to a different and arguably Baconian understanding of the role of hypothesis in science. Theoretical expectations are no longer seen as driving the process of inquiry and empirical input is recognised as primary in determining the direction of research and the phenomena—and related hypotheses—considered by researchers.
The emphasis on data as a central component of research poses a significant challenge to one of the best-established philosophical views on scientific knowledge. According to this view, which I shall label the theory-centric view of science, scientific knowledge consists of justified true beliefs about the world. These beliefs are obtained through empirical methods aiming to test the validity and reliability of statements that describe or explain aspects of reality. Hence scientific knowledge is conceptualised as inherently propositional: what counts as an output are claims published in books and journals, which are also typically presented as solutions to hypothesis-driven inquiry. This view acknowledges the significance of methods, data, models, instruments and materials within scientific investigations, but ultimately regards them as means towards one end: the achievement of true claims about the world. Reichenbach’s seminal distinction between contexts of discovery and justification exemplifies this position (Reichenbach 1938). Theory-centrism recognises research components such as data and related practical skills as essential to discovery, and more specifically to the messy, irrational part of scientific work that involves value judgements, trial-and-error, intuition and exploration and within which the very phenomena to be investigated may not have been stabilised. The justification of claims, by contrast, involves the rational reconstruction of the research that has been performed, so that it conforms to established norms of inferential reasoning. Importantly, within the context of justification, only data that support the claims of interest are explicitly reported and discussed: everything else—including the vast majority of data produced in the course of inquiry—is lost to the chaotic context of discovery. [ 2 ]
Much recent philosophy of science, and particularly modelling and experimentation, has challenged theory-centrism by highlighting the role of models, methods and modes of intervention as research outputs rather than simple tools, and stressing the importance of expanding philosophical understandings of scientific knowledge to include these elements alongside propositional claims. The rise of big data offers another opportunity to reframe understandings of scientific knowledge as not necessarily centred on theories and to include non-propositional components—thus, in Cartwright’s paraphrase of Gilbert Ryle’s famous distinction, refocusing on knowing-how over knowing-that (Cartwright 2019). One way to construe data-centric methods is indeed to embrace a conception of knowledge as ability, such as promoted by early pragmatists like John Dewey and more recently reprised by Chang, who specifically highlighted it as the broader category within which the understanding of knowledge-as-information needs to be placed (Chang 2017).
Another way to interpret the rise of big data is as a vindication of inductivism in the face of the barrage of philosophical criticism levelled against theory-free reasoning over the centuries. For instance, Jon Williamson (2004: 88) has argued that advances in automation, combined with the emergence of big data, lend plausibility to inductivist philosophy of science. Wolfgang Pietsch agrees with this view and provided a sophisticated framework to understand just what kind of inductive reasoning is instigated by big data and related machine learning methods such as decision trees (Pietsch 2015). Following John Stuart Mill, he calls this approach variational induction and presents it as common to both big data approaches and exploratory experimentation, though the former can handle a much larger number of variables (Pietsch 2015: 913). Pietsch concludes that the problem of theory-ladenness in machine learning can be addressed by determining under which theoretical assumptions variational induction works (2015: 910ff).
Others are less inclined to see theory-ladenness as a problem that can be mitigated by data-intensive methods, and rather see it as a constitutive part of the process of empirical inquiry. Arching back to the extensive literature on perspectivism and experimentation (Gooding 1990; Giere 2006; Radder 2006; Massimi 2012), Werner Callebaut has forcefully argued that the most sophisticated and standardised measurements embody a specific theoretical perspective, and this is no less true of big data (Callebaut 2012). Elliott and colleagues emphasise that conceptualising big data analysis as atheoretical risks encouraging unsophisticated attitudes to empirical investigation as a
“fishing expedition”, having a high probability of leading to nonsense results or spurious correlations, being reliant on scientists who do not have adequate expertise in data analysis, and yielding data biased by the mode of collection. (Elliott et al. 2016: 880)
To address related worries in genetic analysis, Ken Waters has provided the useful characterisation of “theory-informed” inquiry (Waters 2007), which can be invoked to stress how theory informs the methods used to extract meaningful patterns from big data, and yet does not necessarily determine either the starting point or the outcomes of data-intensive science. This does not resolve the question of what role theory actually plays. Rob Kitchin (2014) has proposed to see big data as linked to a new mode of hypothesis generation within a hypothetical-deductive framework. Leonelli is more sceptical of attempts to match big data approaches, which are many and diverse, with a specific type of inferential logic. She rather focused on the extent to which the theoretical apparatus at work within big data analysis rests on conceptual decisions about how to order and classify data—and proposed that such decisions can give rise to a particular form of theorization, which she calls classificatory theory (Leonelli 2016).
These disagreements point to big data as eliciting diverse understandings of the nature of knowledge and inquiry, and the complex iterations through which different inferential methods build on each other. Again, in the words of Elliot and colleagues,
attempting to draw a sharp distinction between hypothesis-driven and data-intensive science is misleading; these modes of research are not in fact orthogonal and often intertwine in actual scientific practice. (Elliott et al. 2016: 881, see also O’Malley et al. 2009, Elliott 2012)
Another epistemological debate strongly linked to reflection on big data concerns the specific kinds of knowledge emerging from data-centric forms of inquiry, and particularly the relation between predictive and causal knowledge.
Big data science is widely seen as revolutionary in the scale and power of predictions that it can support. Unsurprisingly perhaps, a philosophically sophisticated defence of this position comes from the philosophy of mathematics, where Marco Panza, Domenico Napoletani and Daniele Struppa argued for big data science as occasioning a momentous shift in the predictive knowledge that mathematical analysis can yield, and thus its role within broader processes of knowledge production. The whole point of big data analysis, they posit, is its disregard for causal knowledge:
answers are found through a process of automatic fitting of the data to models that do not carry any structural understanding beyond the actual solution of the problem itself. (Napoletani, Panza, & Struppa 2014: 486)
This view differs from simplistic popular discourse on “the death of theory” (Anderson 2008) and the “power of correlations” (Mayer-Schoenberg and Cukier 2013) insofar as it does not side-step the constraints associated with knowledge and generalisations that can be extracted from big data analysis. Napoletani, Panza and Struppa recognise that there are inescapable tensions around the ability of mathematical reasoning to overdetermine empirical input, to the point of providing a justification for any and every possible interpretation of the data. In their words,
the problem arises of how we can gain meaningful understanding of historical phenomena, given the tremendous potential variability of their developmental processes. (Napoletani et al. 2014: 487)
Their solution is to clarify that understanding phenomena is not the goal of predictive reasoning, which is rather a form of agnostic science : “the possibility of forecasting and analysing without a structured and general understanding” (Napoletani et al. 2011: 12). The opacity of algorithmic rationality thus becomes its key virtue and the reason for the extraordinary epistemic success of forecasting grounded on big data. While “the phenomenon may forever re-main hidden to our understanding”(ibid.: 5), the application of mathematical models and algorithms to big data can still provide meaningful and reliable answers to well-specified problems—similarly to what has been argued in the case of false models (Wimsatt 2007). Examples include the use of “forcing” methods such as regularisation or diffusion geometry to facilitate the extraction of useful insights from messy datasets.
This view is at odds with accounts that posit scientific understanding as a key aim of science (de Regt 2017), and the intuition that what researchers are ultimately interested in is
whether the opaque data-model generated by machine-learning technologies count as explanations for the relationships found between input and output. (Boon 2020: 44)
Within the philosophy of biology, for example, it is well recognised that big data facilitates effective extraction of patterns and trends, and that being able to model and predict how an organism or ecosystem may behave in the future is of great importance, particularly within more applied fields such as biomedicine or conservation science. At the same time, researchers are interested in understanding the reasons for observed correlations, and typically use predictive patterns as heuristics to explore, develop and verify causal claims about the structure and functioning of entities and processes. Emanuele Ratti (2015) has argued that big data mining within genome-wide association studies often used in cancer genomics can actually underpin mechanistic reasoning, for instance by supporting eliminative inference to develop mechanistic hypotheses and by helping to explore and evaluate generalisations used to analyse the data. In a similar vein, Pietsch (2016) proposed to use variational induction as a method to establish what counts as causal relationships among big data patterns, by focusing on which analytic strategies allow for reliable prediction and effective manipulation of a phenomenon.
Through the study of data sourcing and processing in epidemiology, Stefano Canali has instead highlighted the difficulties of deriving mechanistic claims from big data analysis, particularly where data are varied and embodying incompatible perspectives and methodological approaches (Canali 2016, 2019). Relatedly, the semantic and logistical challenges of organising big data give reason to doubt the reliability of causal claims extracted from such data. In terms of logistics, having a lot of data is not the same as having all of them, and cultivating illusions of comprehensiveness is a risky and potentially misleading strategy, particularly given the challenges encountered in developing and applying curatorial standards for data other than the high-throughput results of “omics” approaches (see also the next section). The constant worry about the partiality and reliability of data is reflected in the care put by database curators in enabling database users to assess such properties; and in the importance given by researchers themselves, particularly in the biological and environmental sciences, to evaluating the quality of data found on the internet (Leonelli 2014, Fleming et al. 2017). In terms of semantics, we are back to the role of data classifications as theoretical scaffolding for big data analysis that we discussed in the previous section. Taxonomic efforts to order and visualise data inform causal reasoning extracted from such data (Sterner & Franz 2017), and can themselves constitute a bottom-up method—grounded in comparative reasoning—for assigning meaning to data models, particularly in situation where a full-blown theory or explanation for the phenomenon under investigation is not available (Sterner 2014).
It is no coincidence that much philosophical work on the relation between causal and predictive knowledge extracted from big data comes from the philosophy of the life sciences, where the absence of axiomatized theories has elicited sophisticated views on the diversity of forms and functions of theory within inferential reasoning. Moreover, biological data are heterogeneous both in their content and in their format; are curated and re-purposed to address the needs of highly disparate and fragmented epistemic communities; and present curators with specific challenges to do with tracking complex, diverse and evolving organismal structures and behaviours, whose relation to an ever-changing environment is hard to pinpoint with any stability (e.g., Shavit & Griesemer 2009). Hence in this domain, some of the core methods and epistemic concerns of experimental research—including exploratory experimentation, sampling and the search for causal mechanisms—remain crucial parts of data-centric inquiry.
At the start of this entry I listed “value” as a major characteristic of big data and pointed to the crucial role of valuing procedures in identifying, processing, modelling and interpreting data as evidence. Identifying and negotiating different forms of data value is an unavoidable part of big data analysis, since these valuation practices determine which data is made available to whom, under which conditions and for which purposes. What researchers choose to consider as reliable data (and data sources) is closely intertwined not only with their research goals and interpretive methods, but also with their approach to data production, packaging, storage and sharing. Thus, researchers need to consider what value their data may have for future research by themselves and others, and how to enhance that value—such as through decisions around which data to make public, how, when and in which format; or, whenever dealing with data already in the public domain (such as personal data on social media), decisions around whether the data should be shared and used at all, and how.
No matter how one conceptualises value practices, it is clear that their key role in data management and analysis prevents facile distinctions between values and “facts” (understood as propositional claims for which data provide evidential warrant). For example, consider a researcher who values both openness —and related practices of widespread data sharing—and scientific rigour —which requires a strict monitoring of the credibility and validity of conditions under which data are interpreted. The scale and manner of big data mobilisation and analysis create tensions between these two values. While the commitment to openness may prompt interest in data sharing, the commitment to rigour may hamper it, since once data are freely circulated online it becomes very difficult to retain control over how they are interpreted, by whom and with which knowledge, skills and tools. How a researcher responds to this conflict affects which data are made available for big data analysis, and under which conditions. Similarly, the extent to which diverse datasets may be triangulated and compared depends on the intellectual property regimes under which the data—and related analytic tools—have been produced. Privately owned data are often unavailable to publicly funded researchers; and many algorithms, cloud systems and computing facilities used in big data analytics are only accessible to those with enough resources to buy relevant access and training. Whatever claims result from big data analysis are, therefore, strongly dependent on social, financial and cultural constraints that condition the data pool and its analysis.
This prominent role of values in shaping data-related epistemic practices is not surprising given existing philosophical critiques of the fact/value distinction (e.g., Douglas 2009), and the existing literature on values in science—such as Helen Longino’s seminal distinction between constitutive and contextual values, as presented in her 1990 book Science as Social Knowledge —may well apply in this case too. Similarly, it is well-established that the technological and social conditions of research strongly condition its design and outcomes. What is particularly worrying in the case of big data is the temptation, prompted by hyped expectations around the power of data analytics, to hide or side-line the valuing choices that underpin the methods, infrastructures and algorithms used for big data extraction.
Consider the use of high-throughput data production tools, which enable researchers to easily generate a large volume of data in formats already geared to computational analysis. Just as in the case of other technologies, researchers have a strong incentive to adopt such tools for data generation; and may do so even in cases where such tools are not good or even appropriate means to pursue the investigation. Ulrich Krohs uses the term convenience experimentation to refer to experimental designs that are adopted not because they are the most appropriate ways of pursuing a given investigation, but because they are easily and widely available and usable, and thus “convenient” means for researchers to pursue their goals (Krohs 2012).
Appeals to convenience can extend to other aspects of data-intensive analysis. Not all data are equally easy to digitally collect, disseminate and link through existing algorithms, which makes some data types and formats more convenient than others for computational analysis. For example, research databases often display the outputs of well-resourced labs within research traditions which deal with “tractable” data formats (such as “omics”). And indeed, the existing distribution of resources, infrastructure and skills determines high levels of inequality in the production, dissemination and use of big data for research. Big players with large financial and technical resources are leading the development and uptake of data analytics tools, leaving much publicly funded research around the world at the receiving end of innovation in this area. Contrary to popular depictions of the data revolution as harbinger of transparency, democracy and social equality, the digital divide between those who can access and use data technologies, and those who cannot, continues to widen. A result of such divides is the scarcity of data relating to certain subgroups and geographical locations, which again limits the comprehensiveness of available data resources.
In the vast ecosystem of big data infrastructures, it is difficult to keep track of such distortions and assess their significance for data interpretation, especially in situations where heterogeneous data sources structured through appeal to different values are mashed together. Thus, the systematic aggregation of convenient datasets and analytic tools over others often results in a big data pool where the relevant sources and forms of bias are impossible to locate and account for (Pasquale 2015; O’Neill 2016; Zuboff 2017; Leonelli 2019a). In such a landscape, arguments for a separation between fact and value—and even a clear distinction between the role of epistemic and non-epistemic values in knowledge production—become very difficult to maintain without discrediting the whole edifice of big data science. Given the extent to which this approach has penetrated research in all domains, it is arguably impossible, however, to critique the value-laden structure of big data science without calling into question the legitimacy of science itself. A more constructive approach is to embrace the extent to which big data science is anchored in human choices, interests and values, and ascertain how this affects philosophical views on knowledge, truth and method.
In closing, it is important to consider at least some of the risks and related ethical questions raised by research with big data. As already mentioned in the previous section, reliance on big data collected by powerful institutions or corporations risks raises significant social concerns. Contrary to the view that sees big and open data as harbingers of democratic social participation in research, the way that scientific research is governed and financed is not challenged by big data. Rather, the increasing commodification and large value attributed to certain kinds of data (e.g., personal data) is associated to an increase in inequality of power and visibility between different nations, segments of the population and scientific communities (O’Neill 2016; Zuboff 2017; D’Ignazio and Klein 2020). The digital gap between those who not only can access data, but can also use it, is widening, leading from a state of digital divide to a condition of “data divide” (Bezuidenout et al. 2017).
Moreover, the privatisation of data has serious implications for the world of research and the knowledge it produces. Firstly, it affects which data are disseminated, and with which expectations. Corporations usually only release data that they regard as having lesser commercial value and that they need public sector assistance to interpret. This introduces another distortion on the sources and types of data that are accessible online while more expensive and complex data are kept secret. Even many of the ways in which citizens -researchers included - are encouraged to interact with databases and data interpretation sites tend to encourage participation that generates further commercial value. Sociologists have recently described this type of social participation as a form of exploitation (Prainsack & Buyx 2017; Srnicek 2017). In turn, these ways of exploiting data strengthen their economic value over their scientific value. When it comes to the commerce of personal data between companies working in analysis, the value of the data as commercial products -which includes the evaluation of the speed and efficiency with which access to certain data can help develop new products - often has priority over scientific issues such as for example, representativity and reliability of the data and the ways they were analysed. This can result in decisions that pose a problem scientifically or that simply are not interested in investigating the consequences of the assumptions made and the processes used. This lack of interest easily translates into ignorance of discrimination, inequality and potential errors in the data considered. This type of ignorance is highly strategic and economically productive since it enables the use of data without concerns over social and scientific implications. In this scenario the evaluation on the quality of data shrinks to an evaluation of their usefulness towards short-term analyses or forecasting required by the client. There are no incentives in this system to encourage evaluation of the long-term implications of data analysis. The risk here is that the commerce of data is accompanied by an increasing divergence between data and their context. The interest in the history of the transit of data, the plurality of their emotional or scientific value and the re-evaluation of their origins tend to disappear over time, to be substituted by the increasing hold of the financial value of data.
The multiplicity of data sources and tools for aggregation also creates risks. The complexity of the data landscape is making it harder to identify which parts of the infrastructure require updating or have been put in doubt by new scientific developments. The situation worsens when considering the number of databases that populate every area of scientific research, each containing assumptions that influence the circulation and interoperability of data and that often are not updated in a reliable and regular way. Just to provide an idea of the numbers involved, the prestigious scientific publication Nucleic Acids Research publishes a special issue on new databases that are relevant to molecular biology every year and included: 56 new infrastructures in 2015, 62 in 2016, 54 in 2017 and 82 in 2018. These are just a small proportion of the hundreds of databases that are developed each year in the life sciences sector alone. The fact that these databases rely on short term funding means that a growing percentage of resources remain available to consult online although they are long dead. This is a condition that is not always visible to users of the database who trust them without checking whether they are actively maintained or not. At what point do these infrastructures become obsolete? What are the risks involved in weaving an ever more extensive tapestry of infrastructures that depend on each other, given the disparity in the ways they are managed and the challenges in identifying and comparing their prerequisite conditions, the theories and scaffolding used to build them? One of these risks is rampant conservativism: the insistence on recycling old data whose features and management elements become increasingly murky as time goes by, instead of encouraging the production of new data with features that specifically respond to the requirements and the circumstances of their users. In disciplines such as biology and medicine that study living beings and therefore are by definition continually evolving and developing, such trust in old data is particularly alarming. It is not the case, for example, that data collected on fungi ten, twenty or even a hundred years ago is reliable to explain the behaviour of the same species of fungi now or in the future (Leonelli 2018).
Researchers of what Luciano Floridi calls the infosphere —the way in which the introduction of digital technologies is changing the world - are becoming aware of the destructive potential of big data and the urgent need to focus efforts for management and use of data in active and thoughtful ways towards the improvement of the human condition. In Floridi’s own words:
ICT yields great opportunity which, however, entails the enormous intellectual responsibility of understanding this technology to use it in the most appropriate way. (Floridi 2014: vii; see also British Academy & Royal Society 2017)
In light of these findings, it is essential that ethical and social issues are seen as a core part of the technical and scientific requirements associated with data management and analysis. The ethical management of data is not obtained exclusively by regulating the commerce of research and management of personal data nor with the introduction of monitoring of research financing, even though these are important strategies. To guarantee that big data are used in the most scientifically and socially forward-thinking way it is necessary to transcend the concept of ethics as something external and alien to research. An analysis of the ethical implications of data science should become a basic component of the background and activity of those who take care of data and the methods used to view and analyse it. Ethical evaluations and choices are hidden in every aspect of data management, including those choices that may seem purely technical.
This entry stressed how the emerging emphasis on big data signals the rise of a data-centric approach to research, in which efforts to mobilise, integrate, disseminate and visualise data are viewed as central contributions to discovery. The emergence of data-centrism highlights the challenges involved in gathering, classifying and interpreting data, and the concepts, technologies and institutions that surround these processes. Tools such as high-throughput measurement instruments and apps for smartphones are fast generating large volumes of data in digital formats. In principle, these data are immediately available for dissemination through internet platforms, which can make them accessible to anybody with a broadband connection in a matter of seconds. In practice, however, access to data is fraught with conceptual, technical, legal and ethical implications; and even when access can be granted, it does not guarantee that the data can be fruitfully used to spur further research. Furthermore, the mathematical and computational tools developed to analyse big data are often opaque in their functioning and assumptions, leading to results whose scientific meaning and credibility may be difficult to assess. This increases the worry that big data science may be grounded upon, and ultimately supporting, the process of making human ingenuity hostage to an alien, artificial and ultimately unintelligible intelligence.
Perhaps the most confronting aspect of big data science as discussed in this entry is the extent to which it deviates from understandings of rationality grounded on individual agency and cognitive abilities (on which much of contemporary philosophy of science is predicated). The power of any one dataset to yield knowledge lies in the extent to which it can be linked with others: this is what lends high epistemic value to digital objects such as GPS locations or sequencing data, and what makes extensive data aggregation from a variety of sources into a highly effective surveillance tool. Data production and dissemination channels such as social media, governmental databases and research repositories operate in a globalised, interlinked and distributed network, whose functioning requires a wide variety of skills and expertise. The distributed nature of decision-making involved in developing big data infrastructures and analytics makes it impossible for any one individual to retain oversight over the quality, scientific significance and potential social impact of the knowledge being produced.
Big data analysis may therefore constitute the ultimate instance of a distributed cognitive system. Where does this leave accountability questions? Many individuals, groups and institutions end up sharing responsibility for the conceptual interpretation and social outcomes of specific data uses. A key challenge for big data governance is to find mechanisms for allocating responsibilities across this complex network, so that erroneous and unwarranted decisions—as well as outright fraudulent, unethical, abusive, discriminatory or misguided actions—can be singled out, corrected and appropriately sanctioned. Thinking about the complex history, processing and use of data can encourage philosophers to avoid ahistorical, uncontextualized approaches to questions of evidence, and instead consider the methods, skills, technologies and practices involved in handling data—and particularly big data—as crucial to understanding empirical knowledge-making.
- Achinstein, Peter, 2001, The Book of Evidence , Oxford: Oxford University Press. doi:10.1093/0195143892.001.0001
- Anderson, Chris, 2008, “The End of Theory: The Data Deluge Makes the Scientific Method Obsolete”, Wired Magazine , 23 June 2008.
- Aronova, Elena, Karen S. Baker, and Naomi Oreskes, 2010, “Big science and big data in biology: From the International Geophysical Year through the International Biological Program to the Long Term Ecological Research (LTER) Network, 1957–present”, Historical Studies in the Natural Sciences , 40: 183–224.
- Aronova, Elena, Christine von Oertzen, and David Sepkoski, 2017, “Introduction: Historicizing Big Data”, Osiris , 32(1): 1–17. doi:10.1086/693399
- Bauer, Susanne, 2008, “Mining Data, Gathering Variables and Recombining Information: The Flexible Architecture of Epidemiological Studies”, Studies in History and Philosophy of Science Part C: Studies in History and Philosophy of Biological and Biomedical Sciences , 39(4): 415–428. doi:10.1016/j.shpsc.2008.09.008
- Bechtel, William, 2016, “Using Computational Models to Discover and Understand Mechanisms”, Studies in History and Philosophy of Science Part A , 56: 113–121. doi:10.1016/j.shpsa.2015.10.004
- Beisbart, Claus, 2012, “How Can Computer Simulations Produce New Knowledge?”, European Journal for Philosophy of Science , 2(3): 395–434. doi:10.1007/s13194-012-0049-7
- Bezuidenhout, Louise, Leonelli, Sabina, Kelly, Ann and Rappert, Brian, 2017, “Beyond the Digital Divide: Towards a Situated Approach to Open Data”. Science and Public Policy , 44(4): 464–475. doi: 10.1093/scipol/scw036
- Bogen, Jim, 2009 [2013], “Theory and Observation in Science”, in The Stanford Encyclopedia of Philosophy (Spring 2013 Edition), Edward N. Zalta (ed.), URL = < https://plato.stanford.edu/archives/spr2013/entries/science-theory-observation/ >.
- –––, 2010, “Noise in the World”, Philosophy of Science , 77(5): 778–791. doi:10.1086/656006
- Bogen, James and James Woodward, 1988, “Saving the Phenomena”, The Philosophical Review , 97(3): 303. doi:10.2307/2185445
- Bokulich, Alisa, 2018, “Using Models to Correct Data: Paleodiversity and the Fossil Record”, in S.I.: Abstraction and Idealization in Scientific Modelling by Synthese , 29 May 2018. doi:10.1007/s11229-018-1820-x
- Boon, Mieke, 2020, “How Scientists Are Brought Back into Science—The Error of Empiricism”, in A Critical Reflection on Automated Science , Marta Bertolaso and Fabio Sterpetti (eds.), (Human Perspectives in Health Sciences and Technology 1), Cham: Springer International Publishing, 43–65. doi:10.1007/978-3-030-25001-0_4
- Borgman, Christine L., 2015, Big Data, Little Data, No Data , Cambridge, MA: MIT Press.
- Boumans, M.J. and Sabina Leonelli, forthcoming, “From Dirty Data to Tidy Facts: Practices of Clustering in Plant Phenomics and Business Cycles”, in Leonelli and Tempini forthcoming.
- Boyd, Danah and Kate Crawford, 2012, “Critical Questions for Big Data: Provocations for a Cultural, Technological, and Scholarly Phenomenon”, Information, Communication & Society , 15(5): 662–679. doi:10.1080/1369118X.2012.678878
- Boyd, Nora Mills, 2018, “Evidence Enriched”, Philosophy of Science , 85(3): 403–421. doi:10.1086/697747
- Bowker, Geoffrey C., 2006, Memory Practices in the Sciences , Cambridge, MA: The MIT Press.
- Bringsjord, Selmer and Naveen Sundar Govindarajulu, 2018, “Artificial Intelligence”, in The Stanford Encyclopedia of Philosophy (Fall 2018 edition), Edward N. Zalta (ed.), URL = < https://plato.stanford.edu/archives/fall2018/entries/artificial-intelligence/ >.
- British Academy & Royal Society, 2017, Data Management and Use: Governance in the 21st Century. A Joint Report of the Royal Society and the British Academy , British Academy & Royal Society 2017 available online (see Report).
- Cai, Li and Yangyong Zhu, 2015, “The Challenges of Data Quality and Data Quality Assessment in the Big Data Era”, Data Science Journal , 14: 2. doi:10.5334/dsj-2015-002
- Callebaut, Werner, 2012, “Scientific Perspectivism: A Philosopher of Science’s Response to the Challenge of Big Data Biology”, Studies in History and Philosophy of Science Part C: Studies in History and Philosophy of Biological and Biomedical Sciences , 43(1): 69–80. doi:10.1016/j.shpsc.2011.10.007
- Calude, Cristian S. and Giuseppe Longo, 2017, “The Deluge of Spurious Correlations in Big Data”, Foundations of Science , 22(3): 595–612. doi:10.1007/s10699-016-9489-4
- Canali, Stefano, 2016, “Big Data, Epistemology and Causality: Knowledge in and Knowledge out in EXPOsOMICS”, Big Data & Society , 3(2): 205395171666953. doi:10.1177/2053951716669530
- –––, 2019, “Evaluating Evidential Pluralism in Epidemiology: Mechanistic Evidence in Exposome Research”, History and Philosophy of the Life Sciences , 41(1): art. 4. doi:10.1007/s40656-019-0241-6
- Cartwright, Nancy D., 2013, Evidence: For Policy and Wheresoever Rigor Is a Must , London School of Economics and Political Science (LSE), Order Project Discussion Paper Series [Cartwright 2013 available online ].
- –––, 2019, Nature, the Artful Modeler: Lectures on Laws, Science, How Nature Arranges the World and How We Can Arrange It Better (The Paul Carus Lectures) , Chicago, IL: Open Court.
- Chang, Hasok, 2012, Is Water H2O? Evidence, Realism and Pluralism , (Boston Studies in the Philosophy of Science 293), Dordrecht: Springer Netherlands. doi:10.1007/978-94-007-3932-1
- –––, 2017, “VI—Operational Coherence as the Source of Truth”, Proceedings of the Aristotelian Society , 117(2): 103–122. doi:10.1093/arisoc/aox004
- Chapman, Robert and Alison Wylie, 2016, Evidential Reasoning in Archaeology , London: Bloomsbury Publishing Plc.
- Collins, Harry M., 1990, Artificial Experts: Social Knowledge and Intelligent Machines , Cambridge, MA: MIT Press.
- Craver, Carl F. and Lindley Darden, 2013, In Search of Mechanisms: Discoveries Across the Life Sciences , Chicago: University of Chicago Press.
- Daston, Lorraine, 2017, Science in the Archives: Pasts, Presents, Futures , Chicago: University of Chicago Press.
- De Regt, Henk W., 2017, Understanding Scientific Understanding , Oxford: Oxford University Press. doi:10.1093/oso/9780190652913.001.0001
- D’Ignazio, Catherine and Klein, Lauren F., 2020, Data Feminism , Cambridge, MA: The MIT Press.
- Douglas, Heather E., 2009, Science, Policy and the Value-Free Ideal , Pittsburgh, PA: University of Pittsburgh Press.
- Dreyfus, Hubert L., 1992, What Computers Still Can’t Do: A Critique of Artificial Reason , Cambridge, MA: MIT Press.
- Durán, Juan M. and Nico Formanek, 2018, “Grounds for Trust: Essential Epistemic Opacity and Computational Reliabilism”, Minds and Machines , 28(4): 645–666. doi:10.1007/s11023-018-9481-6
- Edwards, Paul N., 2010, A Vast Machine: Computer Models, Climate Data, and the Politics of Global Warming , Cambridge, MA: The MIT Press.
- Elliott, Kevin C., 2012, “Epistemic and methodological iteration in scientific research”. Studies in History and Philosophy of Science , 43: 376–382.
- Elliott, Kevin C., Kendra S. Cheruvelil, Georgina M. Montgomery, and Patricia A. Soranno, 2016, “Conceptions of Good Science in Our Data-Rich World”, BioScience , 66(10): 880–889. doi:10.1093/biosci/biw115
- Feest, Uljana, 2011, “What Exactly Is Stabilized When Phenomena Are Stabilized?”, Synthese , 182(1): 57–71. doi:10.1007/s11229-009-9616-7
- Fleming, Lora, Niccolò Tempini, Harriet Gordon-Brown, Gordon L. Nichols, Christophe Sarran, Paolo Vineis, Giovanni Leonardi, Brian Golding, Andy Haines, Anthony Kessel, Virginia Murray, Michael Depledge, and Sabina Leonelli, 2017, “Big Data in Environment and Human Health”, in Oxford Research Encyclopedia of Environmental Science , by Lora Fleming, Niccolò Tempini, Harriet Gordon-Brown, Gordon L. Nichols, Christophe Sarran, Paolo Vineis, Giovanni Leonardi, Brian Golding, Andy Haines, Anthony Kessel, Virginia Murray, Michael Depledge, and Sabina Leonelli, Oxford: Oxford University Press. doi:10.1093/acrefore/9780199389414.013.541
- Floridi, Luciano, 2014, The Fourth Revolution: How the Infosphere is Reshaping Human Reality , Oxford: Oxford University Press.
- Floridi, Luciano and Phyllis Illari (eds.), 2014, The Philosophy of Information Quality , (Synthese Library 358), Cham: Springer International Publishing. doi:10.1007/978-3-319-07121-3
- Frigg, Roman and Julian Reiss, 2009, “The Philosophy of Simulation: Hot New Issues or Same Old Stew?”, Synthese , 169(3): 593–613. doi:10.1007/s11229-008-9438-z
- Frigg, Roman and Stephan Hartmann, 2016, “Models in Science”, in The Stanford Encyclopedia of Philosophy (Winter 2016 edition), Edward N. Zalta (ed.), URL = < https://plato.stanford.edu/archives/win2016/entries/models-science/ >.
- Gooding, David C., 1990, Experiment and the Making of Meaning , Dordrecht & Boston: Kluwer.
- Giere, Ronald, 2006, Scientific Perspectivism , Chicago: University of Chicago Press.
- Griesemer, James R., forthcoming, “A Data Journey through Dataset-Centric Population Biology”, in Leonelli and Tempini forthcoming.
- Hacking, Ian, 1992, “The Self-Vindication of the Laboratory Sciences”, In Science as Practice and Culture , Andrew Pickering (ed.), Chicago, IL: The University of Chicago Press, 29–64.
- Harris, Todd, 2003, “Data Models and the Acquisition and Manipulation of Data”, Philosophy of Science , 70(5): 1508–1517. doi:10.1086/377426
- Hey Tony, Stewart Tansley, and Kristin Tolle, 2009, The Fourth Paradigm. Data-Intensive Scientific Discovery , Redmond, WA: Microsoft Research.
- Humphreys, Paul, 2004, Extending Ourselves: Computational Science, Empiricism, and Scientific Method , Oxford: Oxford University Press. doi:10.1093/0195158709.001.0001
- –––, 2009, “The Philosophical Novelty of Computer Simulation Methods”, Synthese , 169(3): 615–626. doi:10.1007/s11229-008-9435-2
- Karaca, Koray, 2018, “Lessons from the Large Hadron Collider for Model-Based Experimentation: The Concept of a Model of Data Acquisition and the Scope of the Hierarchy of Models”, Synthese , 195(12): 5431–5452. doi:10.1007/s11229-017-1453-5
- Kelly, Thomas, 2016, “Evidence”, in The Stanford Encyclopedia of Philosophy (Winter 2016 edition), Edward N. Zalta (ed.), URL = < https://plato.stanford.edu/archives/win2016/entries/evidence/ >.
- Kitchin, Rob, 2013, The Data Revolution: Big Data, Open Data, Data Infrastructures & Their Consequences , Los Angeles: Sage.
- –––, 2014, “Big Data, new epistemologies and paradigm shifts”, Big Data and Society , 1(1) April-June. doi: 10.1177/2053951714528481
- Kitchin, Rob and Gavin McArdle, 2016, “What Makes Big Data, Big Data? Exploring the Ontological Characteristics of 26 Datasets”, Big Data & Society , 3(1): 205395171663113. doi:10.1177/2053951716631130
- Krohs, Ulrich, 2012, “Convenience Experimentation”, Studies in History and Philosophy of Science Part C: Studies in History and Philosophy of Biological and Biomedical Sciences , 43(1): 52–57. doi:10.1016/j.shpsc.2011.10.005
- Lagoze, Carl, 2014, “Big Data, data integrity, and the fracturing of the control zone,” Big Data and Society , 1(2) July-December. doi: 10.1177/2053951714558281
- Leonelli, Sabina, 2014, “What Difference Does Quantity Make? On the Epistemology of Big Data in Biology”, Big Data & Society , 1(1): 205395171453439. doi:10.1177/2053951714534395
- –––, 2016, Data-Centric Biology: A Philosophical Study , Chicago: University of Chicago Press.
- –––, 2017, “Global Data Quality Assessment and the Situated Nature of ‘Best’ Research Practices in Biology”, Data Science Journal , 16: 32. doi:10.5334/dsj-2017-032
- –––, 2018, “The Time of Data: Timescales of Data Use in the Life Sciences”, Philosophy of Science , 85(5): 741–754. doi:10.1086/699699
- –––, 2019a, La Recherche Scientifique à l’Ère des Big Data: Cinq Façons Donc les Données Massive Nuisent à la Science, et Comment la Sauver , Milano: Éditions Mimésis.
- –––, 2019b, “What Distinguishes Data from Models?”, European Journal for Philosophy of Science , 9(2): 22. doi:10.1007/s13194-018-0246-0
- Leonelli, Sabina and Niccolò Tempini, 2018, “Where Health and Environment Meet: The Use of Invariant Parameters in Big Data Analysis”, Synthese , special issue on the Philosophy of Epidemiology , Sean Valles and Jonathan Kaplan (eds.). doi:10.1007/s11229-018-1844-2
- –––, forthcoming, Data Journeys in the Sciences , Cham: Springer International Publishing.
- Loettgers, Andrea, 2009, “Synthetic Biology and the Emergence of a Dual Meaning of Noise”, Biological Theory , 4(4): 340–356. doi:10.1162/BIOT_a_00009
- Longino, Helen E., 1990, Science as Social Knowledge: Values and Objectivity in Scientific Inquiry , Princeton, NJ: Princeton University Press.
- Lowrie, Ian, 2017, “Algorithmic Rationality: Epistemology and Efficiency in the Data Sciences”, Big Data & Society , 4(1): 1–13. doi:10.1177/2053951717700925
- MacLeod, Miles and Nancy J. Nersessian, 2013, “Building Simulations from the Ground Up: Modeling and Theory in Systems Biology”, Philosophy of Science , 80(4): 533–556. doi:10.1086/673209
- Massimi, Michela, 2011, “From Data to Phenomena: A Kantian Stance”, Synthese , 182(1): 101–116. doi:10.1007/s11229-009-9611-z
- –––, 2012, “ Scientific perspectivism and its foes”, Philosophica , 84: 25–52.
- –––, 2016, “Three Tales of Scientific Success”, Philosophy of Science , 83(5): 757–767. doi:10.1086/687861
- Mayer-Schönberger, Victor and Kenneth Cukier, 2013, Big Data: A Revolution that Will Transform How We Live, Work, and Think , New York: Eamon Dolan/Houghton Mifflin Harcourt.
- Mayo, Deborah G., 1996, Error and the Growth of Experimental Knowledge , Chicago: University of Chicago Press.
- Mayo, Deborah G. and Aris Spanos (eds.), 2009a, Error and Inference , Cambridge: Cambridge University Press.
- Mayo, Deborah G. and Aris Spanos, 2009b, “Introduction and Background”, in Mayo and Spanos (eds.) 2009a, pp. 1–27.
- McAllister, James W., 1997, “Phenomena and Patterns in Data Sets”, Erkenntnis , 47(2): 217–228. doi:10.1023/A:1005387021520
- –––, 2007, “Model Selection and the Multiplicity of Patterns in Empirical Data”, Philosophy of Science , 74(5): 884–894. doi:10.1086/525630
- –––, 2011, “What Do Patterns in Empirical Data Tell Us about the Structure of the World?”, Synthese , 182(1): 73–87. doi:10.1007/s11229-009-9613-x
- McQuillan, Dan, 2018, “Data Science as Machinic Neoplatonism”, Philosophy & Technology , 31(2): 253–272. doi:10.1007/s13347-017-0273-3
- Mitchell, Sandra D., 2003, Biological Complexity and Integrative Pluralism , Cambridge: Cambridge University Press. doi:10.1017/CBO9780511802683
- Morgan, Mary S., 2005, “Experiments versus Models: New Phenomena, Inference and Surprise”, Journal of Economic Methodology , 12(2): 317–329. doi:10.1080/13501780500086313
- –––, forthcoming, “The Datum in Context”, in Leonelli and Tempini forthcoming.
- Morrison, Margaret, 2015, Reconstructing Reality: Models, Mathematics, and Simulations , Oxford: Oxford University Press. doi:10.1093/acprof:oso/9780199380275.001.0001
- Müller-Wille, Staffan and Isabelle Charmantier, 2012, “Natural History and Information Overload: The Case of Linnaeus”, Studies in History and Philosophy of Science Part C: Studies in History and Philosophy of Biological and Biomedical Sciences , 43(1): 4–15. doi:10.1016/j.shpsc.2011.10.021
- Napoletani, Domenico, Marco Panza, and Daniele C. Struppa, 2011, “Agnostic Science. Towards a Philosophy of Data Analysis”, Foundations of Science , 16(1): 1–20. doi:10.1007/s10699-010-9186-7
- –––, 2014, “Is Big Data Enough? A Reflection on the Changing Role of Mathematics in Applications”, Notices of the American Mathematical Society , 61(5): 485–490. doi:10.1090/noti1102
- Nickles, Thomas, forthcoming, “Alien Reasoning: Is a Major Change in Scientific Research Underway?”, Topoi , first online: 20 March 2018. doi:10.1007/s11245-018-9557-1
- Norton, John D., 2003, “A Material Theory of Induction”, Philosophy of Science , 70(4): 647–670. doi:10.1086/378858
- O’Malley M, Maureen A., Kevin C. Elliott, Chris Haufe, and Richard Burian, 2009. “Philosophies of funding”. Cell , 138: 611–615. doi: 10.1016/j.cell.2009.08.008
- O’Malley, Maureen A. and Orkun S. Soyer, 2012, “The Roles of Integration in Molecular Systems Biology”, Studies in History and Philosophy of Science Part C: Studies in History and Philosophy of Biological and Biomedical Sciences , 43(1): 58–68. doi:10.1016/j.shpsc.2011.10.006
- O’Neill, Cathy, 2016, Weapons of Math Destruction: How Big Data Increases Inequality and Threatens Democracy , New York: Crown.
- Parker, Wendy S., 2009, “Does Matter Really Matter? Computer Simulations, Experiments, and Materiality”, Synthese , 169(3): 483–496. doi:10.1007/s11229-008-9434-3
- –––, 2017, “Computer Simulation, Measurement, and Data Assimilation”, The British Journal for the Philosophy of Science , 68(1): 273–304. doi:10.1093/bjps/axv037
- Pasquale, Frank, 2015, The Black Box Society: The Secret Algorithms That Control Money and Information , Cambridge, MA: Harvard University Press.
- Pietsch, Wolfgang, 2015, “Aspects of Theory-Ladenness in Data-Intensive Science”, Philosophy of Science , 82(5): 905–916. doi:10.1086/683328
- –––, 2016, “The Causal Nature of Modeling with Big Data”, Philosophy & Technology , 29(2): 137–171. doi:10.1007/s13347-015-0202-2
- –––, 2017, “Causation, probability and all that: Data science as a novel inductive paradigm”, in Frontiers in Data Science , Matthias Dehmer and Frank Emmert-Streib (eds.), Boca Raton, FL: CRC, 329–353.
- Porter, Theodore M., 1995, Trust in Numbers: The Pursuit of Objectivity in Science and Public Life , Princeton, NJ: Princeton University Press.
- Porter, Theodore M. and Soraya de Chadarevian, 2018, “Introduction: Scrutinizing the Data World”, Historical Studies in the Natural Sciences , 48(5): 549–556. doi:10.1525/hsns.2018.48.5.549
- Prainsack, Barbara and Buyx, Alena, 2017, Solidarity in Biomedicine and Beyond , Cambridge, UK: Cambridge University Press.
- Radder, Hans, 2009, “The Philosophy of Scientific Experimentation: A Review”, Automated Experimentation , 1(1): 2. doi:10.1186/1759-4499-1-2
- Ratti, Emanuele, 2015, “Big Data Biology: Between Eliminative Inferences and Exploratory Experiments”, Philosophy of Science , 82(2): 198–218. doi:10.1086/680332
- Reichenbach, Hans, 1938, Experience and Prediction: An Analysis of the Foundations and the Structure of Knowledge , Chicago, IL: The University of Chicago Press.
- Reiss, Julian, 2015, “A Pragmatist Theory of Evidence”, Philosophy of Science , 82(3): 341–362. doi:10.1086/681643
- Reiss, Julian, 2015, Causation, Evidence, and Inference , New York: Routledge.
- Rescher, Nicholas, 1984, The Limits of Science , Berkely, CA: University of California Press.
- Rheinberger, Hans-Jörg, 2011, “Infra-Experimentality: From Traces to Data, from Data to Patterning Facts”, History of Science , 49(3): 337–348. doi:10.1177/007327531104900306
- Romeijn, Jan-Willem, 2017, “Philosophy of Statistics”, in The Stanford Encyclopedia of Philosophy (Spring 2017), Edward N. Zalta (ed.), URL: https://plato.stanford.edu/archives/spr2017/entries/statistics/ .
- Sepkoski, David, 2013, “Toward ‘a natural history of data’: Evolving practices and epistemologies of data in paleontology, 1800–2000”, Journal of the History of Biology , 46: 401–444.
- Shavit, Ayelet and James Griesemer, 2009, “There and Back Again, or the Problem of Locality in Biodiversity Surveys*”, Philosophy of Science , 76(3): 273–294. doi:10.1086/649805
- Srnicek, Nick, 2017, Platform capitalism , Cambridge, UK and Malden, MA: Polity Press.
- Sterner, Beckett, 2014, “The Practical Value of Biological Information for Research”, Philosophy of Science , 81(2): 175–194. doi:10.1086/675679
- Sterner, Beckett and Nico M. Franz, 2017, “Taxonomy for Humans or Computers? Cognitive Pragmatics for Big Data”, Biological Theory , 12(2): 99–111. doi:10.1007/s13752-017-0259-5
- Sterner, Beckett W., Nico M. Franz, and J. Witteveen, 2020, “Coordinating dissent as an alternative to consensus classification: insights from systematics for bio-ontologies”, History and Philosophy of the Life Sciences , 42(1): 8. doi: 10.1007/s40656-020-0300-z
- Stevens, Hallam, 2016, “Hadooping the Genome: The Impact of Big Data Tools on Biology”, BioSocieties , 11: 352–371.
- Strasser, Bruno, 2019, Collecting Experiments: Making Big Data Biology , Chicago: University of Chicago Press.
- Suppes, Patrick, 1962, “Models of data”, in Logic, Methodology and Philosophy of Science , Ernest Nagel, Patrick Suppes, & Alfred Tarski (eds.), Stanford: Stanford University Press, 252–261.
- Symons, John and Ramón Alvarado, 2016, “Can We Trust Big Data? Applying Philosophy of Science to Software”, Big Data & Society , 3(2): 1-17. doi:10.1177/2053951716664747
- Symons, John and Jack Horner, 2014, “Software Intensive Science”, Philosophy & Technology , 27(3): 461–477. doi:10.1007/s13347-014-0163-x
- Tempini, Niccolò, 2017, “Till Data Do Us Part: Understanding Data-Based Value Creation in Data-Intensive Infrastructures”, Information and Organization , 27(4): 191–210. doi:10.1016/j.infoandorg.2017.08.001
- Tempini, Niccolò and Sabina Leonelli, 2018, “Concealment and Discovery: The Role of Information Security in Biomedical Data Re-Use”, Social Studies of Science , 48(5): 663–690. doi:10.1177/0306312718804875
- Toulmin, Stephen, 1958, The Uses of Arguments , Cambridge: Cambridge University Press.
- Turner, Raymond and Nicola Angius, 2019, “The Philosophy of Computer Science”, in The Stanford Encyclopedia of Philosophy (Spring 2019 edition), Edward N. Zalta (ed.), URL = < https://plato.stanford.edu/archives/spr2019/entries/computer-science/ >.
- Van Fraassen, Bas C., 2008, Scientific Representation: Paradoxes of Perspective , Oxford: Oxford University Press. doi:10.1093/acprof:oso/9780199278220.001.0001
- Waters, C. Kenneth, 2007, “The Nature and Context of Exploratory Experimentation: An Introduction to Three Case Studies of Exploratory Research”, History and Philosophy of the Life Sciences , 29(3): 275–284.
- Wilkinson, Mark D., Michel Dumontier, IJsbrand Jan Aalbersberg, Gabrielle Appleton, Myles Axton, Arie Baak, Niklas Blomberg, Jan-Willem Boiten, Luiz Bonino da Silva Santos, Philip E. Bourne, Jildau Bouwman, Anthony J. Brookes, Tim Clark, Mercè Crosas, Ingrid Dillo, Olivier Dumon, Scott Edmunds, Chris T. Evelo, Richard Finkers, Alejandra Gonzalez-Beltran, et al., 2016, “The FAIR Guiding Principles for Scientific Data Management and Stewardship”, Scientific Data , 3(1): 160018. doi:10.1038/sdata.2016.18
- Williamson, Jon, 2004 “A dynamic interaction between machine learning and the philosophy of science”, Minds and Machines , 14(4): 539–54. doi:10.1093/bjps/axx012
- Wimsatt, William C., 2007, Re-Engineering Philosophy for Limited Beings: Piecewise Approximations to Reality , Cambridge, MA: Harvard University Press.
- Winsberg, Eric, 2010, Science in the Age of Computer Simulation , Chicago: University of Chicago Press.
- Woodward, James, 2000, “Data, phenomena and reliability”, Philosophy of Science , 67(supplement): Proceedings of the 1998 Biennial Meetings of the Philosophy of Science Association. Part II: Symposia Papers (Sep., 2000), pp. S163–S179. https://www.jstor.org/stable/188666
- –––, 2010, “Data, Phenomena, Signal, and Noise”, Philosophy of Science , 77(5): 792–803. doi:10.1086/656554
- Wright, Jessey, 2017, “The Analysis of Data and the Evidential Scope of Neuroimaging Results”, The British Journal for the Philosophy of Science , 69(4): 1179–1203. doi:10.1093/bjps/axx012
- Wylie, Alison, 2017, “How Archaeological Evidence Bites Back: Strategies for Putting Old Data to Work in New Ways”, Science, Technology, & Human Values , 42(2): 203–225. doi:10.1177/0162243916671200
- –––, forthcoming, “Radiocarbon Dating in Archaeology: Triangulation and Traceability”, in Leonelli and Tempini forthcoming.
- Zuboff, Shoshana, 2017, The Age of Surveillance Capitalism: The Fight for the Future at the New Frontier of Power , New York: Public Affairs.
How to cite this entry . Preview the PDF version of this entry at the Friends of the SEP Society . Look up topics and thinkers related to this entry at the Internet Philosophy Ontology Project (InPhO). Enhanced bibliography for this entry at PhilPapers , with links to its database.
[Please contact the author with suggestions.]
artificial intelligence | Bacon, Francis | biology: experiment in | computer science, philosophy of | empiricism: logical | evidence | human genome project | models in science | Popper, Karl | science: theory and observation in | scientific explanation | scientific method | scientific theories: structure of | statistics, philosophy of
Acknowledgments
The research underpinning this entry was funded by the European Research Council (grant award 335925) and the Alan Turing Institute (EPSRC Grant EP/N510129/1).
Copyright © 2020 by Sabina Leonelli < s . leonelli @ exeter . ac . uk >
- Accessibility
Support SEP
Mirror sites.
View this site from another server:
- Info about mirror sites
The Stanford Encyclopedia of Philosophy is copyright © 2023 by The Metaphysics Research Lab , Department of Philosophy, Stanford University
Library of Congress Catalog Data: ISSN 1095-5054
- Español – América Latina
- Português – Brasil
What is Big Data?
Big data refers to extremely large and diverse collections of structured, unstructured, and semi-structured data that continues to grow exponentially over time. These datasets are so huge and complex in volume, velocity, and variety, that traditional data management systems cannot store, process, and analyze them.
The amount and availability of data is growing rapidly, spurred on by digital technology advancements, such as connectivity, mobility, the Internet of Things (IoT), and artificial intelligence (AI). As data continues to expand and proliferate, new big data tools are emerging to help companies collect, process, and analyze data at the speed needed to gain the most value from it.
Big data describes large and diverse datasets that are huge in volume and also rapidly grow in size over time. Big data is used in machine learning, predictive modeling, and other advanced analytics to solve business problems and make informed decisions.
Read on to learn the definition of big data, some of the advantages of big data solutions, common big data challenges, and how Google Cloud is helping organizations build their data clouds to get more value from their data.
Big data examples
Data can be a company’s most valuable asset. Using big data to reveal insights can help you understand the areas that affect your business—from market conditions and customer purchasing behaviors to your business processes.
Here are some big data examples that are helping transform organizations across every industry:
- Tracking consumer behavior and shopping habits to deliver hyper-personalized retail product recommendations tailored to individual customers
- Monitoring payment patterns and analyzing them against historical customer activity to detect fraud in real time
- Combining data and information from every stage of an order’s shipment journey with hyperlocal traffic insights to help fleet operators optimize last-mile delivery
- Using AI-powered technologies like natural language processing to analyze unstructured medical data (such as research reports, clinical notes, and lab results) to gain new insights for improved treatment development and enhanced patient care
- Using image data from cameras and sensors, as well as GPS data, to detect potholes and improve road maintenance in cities
- Analyzing public datasets of satellite imagery and geospatial datasets to visualize, monitor, measure, and predict the social and environmental impacts of supply chain operations
These are just a few ways organizations are using big data to become more data-driven so they can adapt better to the needs and expectations of their customers and the world around them.
The Vs of big data
Big data definitions may vary slightly, but it will always be described in terms of volume, velocity, and variety. These big data characteristics are often referred to as the “3 Vs of big data” and were first defined by Gartner in 2001.
In addition to these three original Vs, three others that are often mentioned in relation to harnessing the power of big data: veracity , variability , and value .
- Veracity : Big data can be messy, noisy, and error-prone, which makes it difficult to control the quality and accuracy of the data. Large datasets can be unwieldy and confusing, while smaller datasets could present an incomplete picture. The higher the veracity of the data, the more trustworthy it is.
- Variability: The meaning of collected data is constantly changing, which can lead to inconsistency over time. These shifts include not only changes in context and interpretation but also data collection methods based on the information that companies want to capture and analyze.
- Value: It’s essential to determine the business value of the data you collect. Big data must contain the right data and then be effectively analyzed in order to yield insights that can help drive decision-making.
How does big data work?
The central concept of big data is that the more visibility you have into anything, the more effectively you can gain insights to make better decisions, uncover growth opportunities, and improve your business model.
Making big data work requires three main actions:
- Integration: Big data collects terabytes, and sometimes even petabytes, of raw data from many sources that must be received, processed, and transformed into the format that business users and analysts need to start analyzing it.
- Management: Big data needs big storage, whether in the cloud, on-premises, or both. Data must also be stored in whatever form required. It also needs to be processed and made available in real time. Increasingly, companies are turning to cloud solutions to take advantage of the unlimited compute and scalability.
- Analysis: The final step is analyzing and acting on big data—otherwise, the investment won’t be worth it. Beyond exploring the data itself, it’s also critical to communicate and share insights across the business in a way that everyone can understand. This includes using tools to create data visualizations like charts, graphs, and dashboards.
Big data benefits
Improved decision-making.
Big data is the key element to becoming a data-driven organization. When you can manage and analyze your big data, you can discover patterns and unlock insights that improve and drive better operational and strategic decisions.
Increased agility and innovation
Big data allows you to collect and process real-time data points and analyze them to adapt quickly and gain a competitive advantage. These insights can guide and accelerate the planning, production, and launch of new products, features, and updates.
Better customer experiences
Combining and analyzing structured data sources together with unstructured ones provides you with more useful insights for consumer understanding, personalization, and ways to optimize experience to better meet consumer needs and expectations.
Continuous intelligence
Big data allows you to integrate automated, real-time data streaming with advanced data analytics to continuously collect data, find new insights, and discover new opportunities for growth and value.
More efficient operations
Using big data analytics tools and capabilities allows you to process data faster and generate insights that can help you determine areas where you can reduce costs, save time, and increase your overall efficiency.
Improved risk management
Analyzing vast amounts of data helps companies evaluate risk better—making it easier to identify and monitor all potential threats and report insights that lead to more robust control and mitigation strategies.
Challenges of implementing big data analytics
While big data has many advantages, it does present some challenges that organizations must be ready to tackle when collecting, managing, and taking action on such an enormous amount of data.
The most commonly reported big data challenges include:
- Lack of data talent and skills. Data scientists, data analysts, and data engineers are in short supply—and are some of the most highly sought after (and highly paid) professionals in the IT industry. Lack of big data skills and experience with advanced data tools is one of the primary barriers to realizing value from big data environments.
- Speed of data growth. Big data, by nature, is always rapidly changing and increasing. Without a solid infrastructure in place that can handle your processing, storage, network, and security needs, it can become extremely difficult to manage.
- Problems with data quality. Data quality directly impacts the quality of decision-making, data analytics, and planning strategies. Raw data is messy and can be difficult to curate. Having big data doesn’t guarantee results unless the data is accurate, relevant, and properly organized for analysis. This can slow down reporting, but if not addressed, you can end up with misleading results and worthless insights.
- Compliance violations. Big data contains a lot of sensitive data and information, making it a tricky task to continuously ensure data processing and storage meet data privacy and regulatory requirements, such as data localization and data residency laws.
- Integration complexity. Most companies work with data siloed across various systems and applications across the organization. Integrating disparate data sources and making data accessible for business users is complex, but vital, if you hope to realize any value from your big data.
- Security concerns. Big data contains valuable business and customer information, making big data stores high-value targets for attackers. Since these datasets are varied and complex, it can be harder to implement comprehensive strategies and policies to protect them.
How are data-driven businesses performing?
Some organizations remain wary of going all in on big data because of the time, effort, and commitment it requires to leverage it successfully. In particular, businesses struggle to rework established processes and facilitate the cultural change needed to put data at the heart of every decision.
But becoming a data-driven business is worth the work. Recent research shows:
- 58% of companies that make data-based decisions are more likely to beat revenue targets than those that don't
- Organizations with advanced insights-driven business capabilities are 2.8x more likely to report double-digit year-over-year growth
- Data-driven organizations generate, on average, more than 30% growth per year
The enterprises that take steps now and make significant progress toward implementing big data stand to come as winners in the future.
Big data strategies and solutions
Developing a solid data strategy starts with understanding what you want to achieve, identifying specific use cases, and the data you currently have available to use. You will also need to evaluate what additional data might be needed to meet your business goals and the new systems or tools you will need to support those.
Unlike traditional data management solutions, big data technologies and tools are made to help you deal with large and complex datasets to extract value from them. Tools for big data can help with the volume of the data collected, the speed at which that data becomes available to an organization for analysis, and the complexity or varieties of that data.
For example, data lakes ingest, process, and store structured, unstructured, and semi-structured data at any scale in its native format. Data lakes act as a foundation to run different types of smart analytics, including visualizations, real-time analytics, and machine learning .
It’s important to keep in mind that when it comes to big data—there is no one-size-fits-all strategy. What works for one company may not be the right approach for your organization’s specific needs.
Here are four key concepts that our Google Cloud customers have taught us about shaping a winning approach to big data:
Solve your business challenges with Google Cloud
How to get started with big data for your business.
Take the next step
Start building on Google Cloud with $300 in free credits and 20+ always free products.
Start your next project, explore interactive tutorials, and manage your account.
- Need help getting started? Contact sales
- Work with a trusted partner Find a partner
- Continue browsing See all products
- Get tips & best practices See tutorials

An official website of the United States government
The .gov means it’s official. Federal government websites often end in .gov or .mil. Before sharing sensitive information, make sure you’re on a federal government site.
The site is secure. The https:// ensures that you are connecting to the official website and that any information you provide is encrypted and transmitted securely.
- Publications
- Account settings
Preview improvements coming to the PMC website in October 2024. Learn More or Try it out now .
- Advanced Search
- Journal List
- SAGE Open Med
A review of big data and medical research
Universally, the volume of data has increased, with the collection rate doubling every 40 months, since the 1980s. “Big data” is a term that was introduced in the 1990s to include data sets too large to be used with common software. Medicine is a major field predicted to increase the use of big data in 2025. Big data in medicine may be used by commercial, academic, government, and public sectors. It includes biologic, biometric, and electronic health data. Examples of biologic data include biobanks; biometric data may have individual wellness data from devices; electronic health data include the medical record; and other data demographics and images. Big data has also contributed to the changes in the research methodology. Changes in the clinical research paradigm has been fueled by large-scale biological data harvesting (biobanks), which is developed, analyzed, and managed by cheaper computing technology (big data), supported by greater flexibility in study design (real-world data) and the relationships between industry, government regulators, and academics. Cultural changes along with easy access to information via the Internet facilitate ease of participation by more people. Current needs demand quick answers which may be supplied by big data, biobanks, and changes in flexibility in study design. Big data can reveal health patterns, and promises to provide solutions that have previously been out of society’s grasp; however, the murkiness of international laws, questions of data ownership, public ignorance, and privacy and security concerns are slowing down the progress that could otherwise be achieved by the use of big data. The goal of this descriptive review is to create awareness of the ramifications for big data and to encourage readers that this trend is positive and will likely lead to better clinical solutions, but, caution must be exercised to reduce harm.
Introduction
What is big data.
“Big data” is a term that was introduced in the 1990s to include data sets too large to be used with common software. In 2016, it was defined as information assets characterized by high volume, velocity, and variety that required specific technology and analytic methods for its transformation into use. 1 In addition to the three attributes of volume, velocity, and variety, some have suggested that for big data to be effective, nuances including quality, veracity, and value need to be added as well. 2 , 3 Big data reveals health patterns, and promises to provide solutions that have previously been out of society’s grasp; however, the murkiness of international laws, questions of data ownership, public ignorance, and privacy and security concerns are slowing down the progress that could otherwise be achieved by the use of big data. In this descriptive review, we highlight the roles of big data, the changing research paradigm, and easy access to research participation via the Internet fueled by the need for quick answers.
Universally, data volume has increased, with the collection rate doubling every 40 months, ever since the 1980s. 4 The big data age, starting in 2002, has generated increasing amounts of alphanumeric data; in addition, social media has generated large amounts of data in the form of audio and images. The use of Internet-based devices including smart phones and computers, wearable electronics, the Internet of things (IoT), electronic health records (EHRs), insurance websites, and mobile health all generate terabytes of data. Sources that are not obvious include clickstream data, machine to machine data processing, geo-spatial data, audio and video inputs, and unstructured text. In general, the total volume of data generated can only be estimated. For example, the usual personal computer in the year 2000 held 10 gigabytes of storage; recently, Facebook analyzed more than 105 terabytes of data every 30 min, including shared items and likes, which allows for optimization of product features for its advertising performance; additionally, in its first year Google images used up 13.7 petabytes of storage on users devices. 5 , 6 It is clear that all four domains of big data: acquisition, storage, analysis, and distribution have increased over the data life cycle. 7
Besides being statistically powerful and complex, data need to be available in real time, which allows it to be analyzed and used immediately. Big data has immense volume, dynamic and diverse characteristics, and requires special management technologies including software, infrastructure, and skills. Big data shows trends from shopping, crime statistics, weather patterns, disease outbreaks, and so on. Recognizing the power of big data to effect change, the United Nations (UN) Global Working Group on big data was created under the UN Statistical Commission in 2014. Its vision was to use big data technologies in the UN global platform to create a global statistical community for data sharing and economic benefit. 8
We aimed to write a descriptive review to inform physicians about use of big data (biological, biometric, and electronic health records) in both the commercial and research fields. Pubmed-based searches were performed, and in addition, since many of the topics were outside the scope of this data base, general Internet searches using Google search engine were performed. Searching for “Big data and volume and velocity and variety” in the Pubmed data base resulted in 45 articles in English. Papers were deemed to be appropriate by the consensus of at least two authors. Pubmed search for “artificial intelligence in clinical decision support” resulted in two relevant review articles, and the addition of “randomized control trials” resulted in 11 randomized control studies, of which only one was relevant. For non-Pubmed indexed scholarly articles, two authors determined relevance by the frequency of the paper being cited or accessed online. As some content was to be informative rather than conclusive, commercial websites, such as those dealing with DNA testing for ancestry, were accessed. The Food and Drug Administration (FDA) website was accessed when searching for the “oldest biobank,” which revealed the HIV registry. Landmark trials were selected for changes in research design and use of big data mining.
Big data in medicine
The major fields predicted to increasingly use big data by 2025 include astronomy, social media ( Twitter, YouTube , etc.) and medicine-Genomics, which will be measured in zetta-bytes/year (zetta = 10 21 ). Big data in medicine includes biologic, biometric, and electronic health data ( Figure 1 ).

Big data in medicine.
Biological banks , also called biobanks, may be present at the local, national, or international levels. Examples include local academic institutions, the National Cancer Institute, United Kingdom Biobank, China Kadoorie Biobank, and the European Bioinformatics Institute, among others. 9 Non-profit organizations may perform biological data collection during a health fair with screening of blood pressure, or urine and blood tests. Commercial biobanks include those that provide services like saliva testing for ancestry determination. 10
Before the data can be converted to digital form, biological specimens need to be processed and preserved. Biospecimen preservation standards in the past varied based on the organization. In 2005, in an effort to standardize biospecimen preservation, the National Cancer Institute contributed to the creation of the Office of Biobanking and Biospecimen Research (OBBR) and the annual symposium for Biospecimen Research Network Symposia. 11 In 2009, with international support, there was the publication of the first biobank-specific quality standard, which has since been applied to many biobanks. Biobanking has evolved with regulatory pressures, advances in medical and computational information technology, and is a crucial enterprise to biological sciences. One of the longest existing biobanks is the University of California at San Francisco AIDS specimen bank, which has functioned for the past 30 years. 12
One thing in common that all biobanks have is the need for significant resources to manage, analyze, and use the information in a timely manner. 13 Commercial biobanks include multinational companies that collect biological specimens from subjects for verification of ancestry. Subjects pay for the DNA analysis kit, which is collected by them and mailed to the companies where they are analyzed and stored. The company then can sell the data to third parties for research based on legislation.
The shifting paradigm in medical research
The clinical research paradigm has changed to match an increasingly older population’s needs. This has been fueled by large-scale biological data harvesting (biobanks), which is developed, analyzed, and managed by cheaper computing technology (big data), supported by greater flexibility in study design and the relationships between industry, government regulators, and academics. With easy access to information via the Internet, citizen science had allowed many non-scientists to participate in research. 14 Biological specimens collected via Internet-based projects may be sold to third parties for research; these may be as data of healthy controls or as part of a specific medical condition.
Historical precedent and its difficulties
In the past, drug development may have started in serendipity. 15 Subsequent to the Second World War, the therapeutic research approach became long and expensive. The initial step was the search into possible therapies, followed by in vitro and in vivo testing via multiple phases: the first phase for safety, the second for efficacy and the third to compare the treatment to the existing standard of care. In addition, hurdles for new drugs included FDA approval, randomized control trials (RCTs), and finally post-release studies. In some unfortunate cases, once the drug was released in the market, rare, but serious, adverse events would bankrupt the company and patients who needed the therapy would still not have effective treatment choices. This was particularly hard for patients suffering from rare diseases, where the small population needed a large investment of money and time, which was less attractive to industry to attempt a repeat study. In patients who had limited life spans, the long process precluded them from beneficial therapies. Understanding this need, when there was an urgency for rapid treatments, the FDA worked to expedite the release of new drugs, such as the release of new medications to treat HIV during its epidemic. 16 , 17
In the case of oncology, the historical approaches in research and development (R&D) of a new drug followed by the usual phases to RCTs have been expensive. In 2018, pharmaceutical companies invested approximately 50 billion dollars in R&D for a 3% probability of success from individual projects. A 3% probability of success, despite the investment of financial and human effort, is too low for patients who may not have any treatment options. 18
Changes in research
Changes in study design.
At present, a more purposeful and organized approach for determining the responsible cause as a starting point for subsequent therapy is being used.
After completion of the Human Genome Project, technology for pinpointing mutations increased. 19 Broad sweeps of the human genome with more than 3000 genome-wide association studies (GWAS) have examined about 1800 diseases. 20 Following GWAS or Quantitative trait locus (QTL) determination, microarray data allowed identification of candidate genes of interest. 21 For allelic variants to be correlated to disease, large biobanks that have both patient and control data are compared. If a mutated allelic frequency correlates at a significantly higher rate in those with the disease, that variant can be targeted for therapy.
In a tumor, once a driver mutation that promotes abnormal growth is identified, therapy targeting the specific genetic alteration can be attempted. 22 In the presence of multiple mutations, driver mutations are differentiated from bystander or passenger mutations, as tumors may have a heterogeneous molecular signature.
Pharmaco-genomics is the foundation for precision medicine, which is now being clinically practiced in oncology and is being adapted in other fields. The introduction of molecular pathological epidemiology (MPE) allows the identification of new biomarkers using big data to select therapy 23 , 24 ( Table 1 ). Based on an individual’s cellular genetics, drugs that target the desired mutation can be studied and effective doses determined, which can result in safe and efficient treatments.
Examples of big data and new research designs trials.
AI: artificial intelligence.
Big data technology allows large cohorts of biological specimens to be collected, and the data can be stored, managed, and analyzed. At the point of analysis, machine learning algorithms (a subset of artificial intelligence (AI)) can generate further output data that may be different from the initial input data. AI can create knowledge from big data 25 , 26 ( Table 1 ). For example, Beck et al., 25 using a computation pathology model in breast cancer specimens with AI, found prior unknown morphologic features to be predictive of negative outcomes.
Rapid learning health care (RLHC) models using AI may discover data that are of varying quality which need to be compared to validated data sets to be truly meaningful. 29 Subsequently, the information extracted can be processed into decision support systems (DSS), which are software applications that can eventually apply knowledge-driven healthcare into practice.
AI can be classified into knowledge-based or data-driven AI. Knowledge-based AI starts with information entered by humans to solve a query in a domain of expertise formalized by the software. Data-driven AI starts with large amounts of data generated by human activity to make a prediction. Data-driven AI needs big data and, with inexpensive computing, is a promising economic choice. 30 , 31
The combination of AI and DSS is a clinically powerful one to improve health care delivery. For example, in a small study of 12 patients with type one diabetes, using AI and DSS allowed for quicker changes in therapy rather than the patients waiting for their next caregiver appointment, without an increase in adverse events. 32
New study designs
With new technology for diagnosing, managing, and treating diseases, modifying the RCT design was essential. The development of master clinical trial protocols, platform trials, basket/bucket designs, and umbrella designs has been seen over the last decade. 33
Basket design : A basket trial is a clinical trial where enrollment eligibility is based on the presence of a specific genomic alteration, irrespective of histology or origin of cell type, and includes sub-trials of multiple tumor types. To qualify for the study, thousands of patients’ data need to be screened to find the suitable genomic alteration to get a small number of patients into a sub-trial.
Usually, sub-trials may be designed as early phase and single arm studies, with one or two stages having an option of stopping early if the study is considered futile. The study design is based on determining tumor pathophysiology/activity and matching the target mutation with a hypothesized treatment. Analogous to a screening test, a responsive sub-study would require a larger confirmatory study. For example, although rare cancers are uncommon on an individual basis, the total sum of these cases make “rare cancers” the fourth largest category of cancer in the United States and Europe. 34 These are challenging to diagnose and treat and have a worse 5-year survival rate as compared to common cancers. One option to help these patients would be to make them eligible for a clinical trial based on genetic dysregulation of the tumor rather than organ histology.
Drugs have been studied for a signature driver mutation rather than for an organ-specific disease. With enough information about the molecular definitions of the targets, the focus on the site of origin of the cancer is diminishing, for example, the study drug Larotrectinib was noted to have significant sustained antitumor activity in patients with 17 types of Tropomysin Receptor kinase fusion–positive cancers, regardless of the age of the patient or of the tumor site of origin. 35 , 36 This landmark drug was the first which was FDA approved for tumors with a specific mutation and not a disease.
Basket trials may also test off-label use of a drug in patients who have the same genomic alteration for which the drug was initially approved, or it could test a repurposed drug. 37
Umbrella design : The umbrella design looks at a single disease by testing various therapies on a variety of mutations, such as lung cancer. (Ferrarotto et al.; 28 Table 1 .)
Platform trials : Big data allows the pooling of resources. Data captured about biomarker status can allow patients to have access to various trials. Compared to a traditional RCT with a control and experimental arm, a platform trial uses a single control arm, which can be compared to many experimental arms, and which may not need to be randomized at the start of the trial; therefore, a platform trial may be seen as a prolonged screening process. 38
Even if the traditional RCT is planned, matching various data sets with AI to run various configurations can result in determining possible therapy choices, and can eliminate time and investment outlay. In the end, this could speed up the process of drug testing and result in a quicker arrival to the RCT stage.
Adverse Drug Events ( ADE ): ADE reporting is a continuous process. Big data in medicine includes literature searches for ADE; using data mining with AI can yield better results than traditional methods in regards to accuracy and precision. 39 In addition, big data can visualize ADE interactions between medications and can be updated on a daily basis.
Real-world evidence
Real-world evidence (RWE), is information obtained from routine clinical practice and it has increased with the use of the EHR. RWE in the digital format can be significantly furthered by big data. Clinical practice guidelines that have been using RWE-based insights include the National Comprehensive Cancer Network. In addition, the American Society of Clinical Oncology suggests using RWE in a complementary nature to randomized controlled trials. 40 Big data in RWE allows for more rapid evaluation of therapy in the clinical setting, which is a key element in the cost of R&D of drugs. The 21st Century Cures Act (signed into law 13 December 2016) resulted in the FDA creating a framework for evaluating the potential use of RWE to help support the approval of a new indication of a drug, or to help support post-approval study requirements. 41 Focusing on EHR data, industry is starting to generate interest in a new pathway to drug approvals. An example would be using natural language processing and machine learning systems to provide observational clinical studies with adequate quality to attempt justification of approval for the new indication of drugs. Another example includes using AI technology to identify the effect of comorbidities on therapy outcomes and subgroups in single disease entity all of which will enhance personalized medicine. RWE data that are collected include demographics, family history, lifestyle, and genetics, and can be used to predict probabilities of diseases in the future. Once marketed, RWE along with RCT could speed up the FDA requirements to get the therapy to the patient or to compare drugs. A recently published study that used RWE to compare cardiovascular outcomes between different therapies was the Cardiovascular Outcome Study of Linagliptin versus Glimepiride in Type 2 Diabetes (CAROLINA) trial. (Patorno et al.; 27 see Table 1 .)
Big data: technology and security
Computing technology has gotten cheaper which allows for the extensive use of big data. Examples of big data technology can be characterized by its function: either operational or analytic ( Table 2 ). Both systems have specific advantages, formats, data forms, and computer network capabilities ( Figure 2 ).
Big data technology with examples of systems in use.

Big data security.
Big data security should include measures and tools that guard big data at all points: data collection, transfer, analysis, storage, and processing. This includes the security needed to protective massive amounts of dynamic data and faster creative processing like massive parallel processing systems. The risk to data may be theft, loss, or corruption either through human error, inadequate technology (example crash of a server), or malicious intent. Loss of privacy with health-related information adds to the need for greater security and exposes involved organizations to financial losses, fines, and litigation.
Processes to prevent data loss and corruption at each access point needs to be in place, for example, during data collection, there needs to be interruption to incoming threats. Security measures include encrypting data at input and output points, allowing only partial data volume transfers and analysis to occur, separating storage compartments on cloud computing, limiting access with firewalls, and other filters. 45 For example, Block chain technology is a security device that can authenticate users, track data access, and, due to its decentralized nature, can limit data volume retrieval. 46 Standardizing big data security continues to be an area where further research and development is required. A review of 804 scholarly papers on big data analytics to identify challenges, found data security to be a major challenge while managing a large volume of sensitive personal health data. 47
With changes in the scientific method, difficulties are to be expected. Examples of big data with non-traditional research techniques and negative consequences are listed in Table 3 . These include preemptive release of drugs to the market as in the Bellini trial, loss of privacy of the relatives of criminals who underwent ancestry determination, and questions of ownership of data. Whether the developing research systems will justify the trust invested in it by altruistic participants, patients and physicians need to be seen. Government regulators are included in the struggle as a shifting legal framework could challenge everyone involved ( Table 3 ).
Weaknesses and consequences faced by big data in the changing research landscape.
RCTs: randomized control trials; FDA: Food and Drug Administration; AI: artificial intelligence.
Changing cultural context and the physician
All hospitals have collected biological specimens as part of their routine workflow, an example being routine blood tests. In the ideal world, many doctors would like to do some research; however, in the real world, research is performed by the minority of physicians. A survey of physicians across two hospitals in Australia found physicians interested in having biobanks in hospitals; 64 however, large biobanks may be more efficient and financially viable. Rather than discounting the routinely collected specimens, consideration to capture this potential resource should be explored. One option is to explore how to close the gap between those who routinely prepare the specimens, those who store it, and those who use the information for research. One such project, Polyethnic-1000 includes the collection of biological specimens from minority populations via community and academic hospitals in New York City. 65
Correlations between genetics and disease, and connections that were not obvious in the past, can become visible as the data set increases in size. Instead of starting with people who have the disease in whom the new drug is tested in a RCT and then waiting to determine post-marketing study outcomes, large data collections of genetic and demographic information (including family history, lifestyle, etc.) can be used to show the risk of disease in a population and predict if risk modification can prevent illness. The shift toward prevention rather than cure may get a big boost from big data. In those with the disease, cellular specifics (receptors, cytokines, along with gene variants) can predict what sites to target (increasing or decreasing effects) in order to develop therapies that are personalized in that subset of the same disease.
The growth of the Internet over the last 20 years and creation of open access to scientific literature has resulted in the availability of unlimited medical information to patients. 66 It has led to the direct use of products and practices by the general public, at times eliminating the need for the clinician’s input. Lack of transparency has created an inconsistently safe environment, and this is especially true among those who participate in social media research. Minimally invasive activities like mailing a saliva swab for genetic testing, while done for reasons of curiosity like determining one’s ancestry, contribute to the collection and sale of large amounts of genetic information to third parties. The loss of privacy is a clear risk outlined in the several pages of online consent that most subjects will probably not read. 67 , 68 There are collections of large data banks with more than a million biospecimens in many private organizations. In the past, medical big data may have seemed more aspirational than practical with both physicians and the general public unaware of its risks and benefits.
For physicians, researchers, and the general public, flexibility to find answers rapidly is vital for our well-being today more than ever before. For example, in the coronavirus disease of 2019 (COVID-19) pandemic, the FDA has engaged directly with more than 100 test developers since the end of January 2020. This unprecedented policy by the FDA is attempting to get rapid and widespread testing available. According to the policy update, responsibility for the tests, including those by commercial manufacturers, is being shared with state governments and these laboratories are not required to pursue emergency use authorization (EAU) with the FDA. 69
An example of big data with an alternate research paradigm using public participation in the COVID-19 pandemic could be as follows: direct-to-consumer marketing of a quantifiable antibody home test for COVID-19. The FDA is working with the Gates foundation to produce a self-test kit for COVID-19 as a nasopharyngeal swab. 70 If a biobank registry is subsequently created for COVID-19, it would provide us with tremendous information, including, but not limited to, an accurate mortality rate and identification of those who have high antibody levels. The identification of participants with high antibody levels may then allow them to donate antibodies to those at risk for worse outcomes.
Limitations of the article
The article is about the various aspects of data and medical research and is limited to being a relevant analysis of literature rather than an exhaustive review. The most cited or electronically accessed articles have been used as references. Changes in the many aspects of data collection to security are based on rapidly changing technology. Information which had physical restrictions and was located in controlled physical premises have migrated to the cloud with digital transformation. In addition, dynamic factors like enterprise mobility or even the current COVID-19 lock down has changed the way people work. A comprehensive review and in-depth analysis would be out of the scope of a review article.
Final thoughts
The increasing use of big data and AI with heterogeneous large data sets for analysis and predictive medicine may result in more contributions from physicians, patients, and citizen-scientists without having to go down the path of an expensive RCT. The formative pressures between altruistic public participants, government regulators, Internet-using patients in search of cures, clinicians who refer patients, and industries seeking to reduce cost, all supported by cheaper technology, will determine the direction of how new therapies are tried out for use. Increased government interest and funding in this aspect is noted with programs like the “All of Us initiative.” 71 At present, pressing needs in the COVID-19 pandemic force flexibility between all interested parties to conduct investigations and find answers quickly.
Personalized health care is expanding rapidly with more clues for cures than ever before. Each solution presented brings its own set of problems, which in turn needs new solutions. Collaboration across silos, like government agencies, commercial manufacturers, researchers, and the public needs to be flexible to help the greatest number of patients. Big data and biobanks are tools needed for basic research, which, if successful, may lead to new therapies and clinical trials, which will ultimately lead to new cures. Data that are collected, analyzed, and managed still needs to be converted into insight with the goal of “first do no harm.” All involved must have the common goal of data security and transparency to continue to build public trust.
Declaration of conflicting interests: The author(s) declared no potential conflicts of interest with respect to the research, authorship, and/or publication of this article.
Ethical approval: Ethical approval for this study was waived by “Institutional Review Board of State University of New York at Downstate” because “this is a review article and considered exempt.”
Funding: The author(s) received no financial support for the research, authorship, and/or publication of this article.
Informed consent: Written informed consent was obtained from all subjects before the study.

Big data: The next frontier for innovation, competition, and productivity
The amount of data in our world has been exploding, and analyzing large data sets—so-called big data—will become a key basis of competition, underpinning new waves of productivity growth, innovation, and consumer surplus, according to research by MGI and McKinsey's Business Technology Office. Leaders in every sector will have to grapple with the implications of big data, not just a few data-oriented managers. The increasing volume and detail of information captured by enterprises, the rise of multimedia, social media, and the Internet of Things will fuel exponential growth in data for the foreseeable future.
MGI studied big data in five domains—healthcare in the United States, the public sector in Europe, retail in the United States, and manufacturing and personal-location data globally. Big data can generate value in each. For example, a retailer using big data to the full could increase its operating margin by more than 60 percent. Harnessing big data in the public sector has enormous potential, too. If US healthcare were to use big data creatively and effectively to drive efficiency and quality, the sector could create more than $300 billion in value every year. Two-thirds of that would be in the form of reducing US healthcare expenditure by about 8 percent. In the developed economies of Europe, government administrators could save more than €100 billion ($149 billion) in operational efficiency improvements alone by using big data, not including using big data to reduce fraud and errors and boost the collection of tax revenues. And users of services enabled by personal-location data could capture $600 billion in consumer surplus. The research offers seven key insights.
1. Data have swept into every industry and business function and are now an important factor of production, alongside labor and capital. We estimate that, by 2009, nearly all sectors in the US economy had at least an average of 200 terabytes of stored data (twice the size of US retailer Wal-Mart's data warehouse in 1999) per company with more than 1,000 employees.
2. There are five broad ways in which using big data can create value. First, big data can unlock significant value by making information transparent and usable at much higher frequency. Second, as organizations create and store more transactional data in digital form, they can collect more accurate and detailed performance information on everything from product inventories to sick days, and therefore expose variability and boost performance. Leading companies are using data collection and analysis to conduct controlled experiments to make better management decisions; others are using data for basic low-frequency forecasting to high-frequency nowcasting to adjust their business levers just in time. Third, big data allows ever-narrower segmentation of customers and therefore much more precisely tailored products or services. Fourth, sophisticated analytics can substantially improve decision-making. Finally, big data can be used to improve the development of the next generation of products and services. For instance, manufacturers are using data obtained from sensors embedded in products to create innovative after-sales service offerings such as proactive maintenance (preventive measures that take place before a failure occurs or is even noticed).
3. The use of big data will become a key basis of competition and growth for individual firms. From the standpoint of competitiveness and the potential capture of value, all companies need to take big data seriously. In most industries, established competitors and new entrants alike will leverage data-driven strategies to innovate, compete, and capture value from deep and up-to-real-time information. Indeed, we found early examples of such use of data in every sector we examined.
4. The use of big data will underpin new waves of productivity growth and consumer surplus. For example, we estimate that a retailer using big data to the full has the potential to increase its operating margin by more than 60 percent. Big data offers considerable benefits to consumers as well as to companies and organizations. For instance, services enabled by personal-location data can allow consumers to capture $600 billion in economic surplus.
5. While the use of big data will matter across sectors, some sectors are set for greater gains. We compared the historical productivity of sectors in the United States with the potential of these sectors to capture value from big data (using an index that combines several quantitative metrics), and found that the opportunities and challenges vary from sector to sector. The computer and electronic products and information sectors, as well as finance and insurance, and government are poised to gain substantially from the use of big data.
6. There will be a shortage of talent necessary for organizations to take advantage of big data. By 2018, the United States alone could face a shortage of 140,000 to 190,000 people with deep analytical skills as well as 1.5 million managers and analysts with the know-how to use the analysis of big data to make effective decisions.
7. Several issues will have to be addressed to capture the full potential of big data. Policies related to privacy, security, intellectual property, and even liability will need to be addressed in a big data world. Organizations need not only to put the right talent and technology in place but also structure workflows and incentives to optimize the use of big data. Access to data is critical—companies will increasingly need to integrate information from multiple data sources, often from third parties, and the incentives have to be in place to enable this.
Related Articles

The social economy: Unlocking value and productivity through social technologies

Internet matters: The Net's sweeping impact on growth, jobs, and prosperity

The great transformer: The impact of the Internet on economic growth and prosperity
Thank you for visiting nature.com. You are using a browser version with limited support for CSS. To obtain the best experience, we recommend you use a more up to date browser (or turn off compatibility mode in Internet Explorer). In the meantime, to ensure continued support, we are displaying the site without styles and JavaScript.
- View all journals
- Explore content
- About the journal
- Publish with us
- Sign up for alerts
- Review Article
- Published: 05 September 2022
Big data in basic and translational cancer research
- Peng Jiang ORCID: orcid.org/0000-0002-7828-5486 1 ,
- Sanju Sinha 1 ,
- Kenneth Aldape 2 ,
- Sridhar Hannenhalli 1 ,
- Cenk Sahinalp ORCID: orcid.org/0000-0002-2170-2808 1 &
- Eytan Ruppin ORCID: orcid.org/0000-0002-7862-3940 1
Nature Reviews Cancer volume 22 , pages 625–639 ( 2022 ) Cite this article
42k Accesses
67 Citations
210 Altmetric
Metrics details
- Cancer epigenetics
- Cancer genomics
- Cancer therapy
- Computational biology and bioinformatics
Historically, the primary focus of cancer research has been molecular and clinical studies of a few essential pathways and genes. Recent years have seen the rapid accumulation of large-scale cancer omics data catalysed by breakthroughs in high-throughput technologies. This fast data growth has given rise to an evolving concept of ‘big data’ in cancer, whose analysis demands large computational resources and can potentially bring novel insights into essential questions. Indeed, the combination of big data, bioinformatics and artificial intelligence has led to notable advances in our basic understanding of cancer biology and to translational advancements. Further advances will require a concerted effort among data scientists, clinicians, biologists and policymakers. Here, we review the current state of the art and future challenges for harnessing big data to advance cancer research and treatment.
Similar content being viewed by others
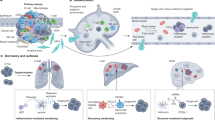
Decoding the interplay between genetic and non-genetic drivers of metastasis
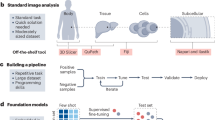
A guide to artificial intelligence for cancer researchers
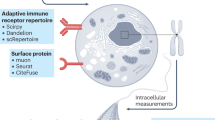
Best practices for single-cell analysis across modalities
Introduction.
Cancer is a complex process, and its progression involves diverse processes in the patient’s body 1 . Consequently, the cancer research community generates massive amounts of molecular and phenotypic data to study cancer hallmarks as comprehensively as possible. The rapid accumulation of omics data catalysed by breakthroughs in high-throughput technologies has given rise to the notion of ‘big data’ in cancer, which we define as a dataset with two basic properties; first, it contains abundant information that can give novel insights into essential questions, and second, its analysis demands a large computer infrastructure beyond equipment available to an individual researcher — an evolving concept as computational resources evolve exponentially following Moore’s law. A model example of such big data is the dataset collected by The Cancer Genome Atlas (TCGA) 2 . TCGA contains 2.5 petabytes of raw data — an amount 2,500 times greater than modern laptop storage in 2022 — and requires specialized computers for storage and analysis. Further, between its initial release in 2008 to March 2022, at least 10,242 articles and 11,054 NIH grants cited TCGA according to a PubMed search, demonstrating its transformative value as a community resource that has markedly driven cancer research forward.
Big data are not unique to the cancer field, and play an essential role in many scientific disciplines, notably cosmology, weather forecasting and image recognition. However, datasets in the cancer field differ from those in other fields in several key aspects. First, the size of cancer datasets is typically markedly smaller. For example, in March 2022, the US National Center for Biotechnology Information (NCBI) Gene Expression Omnibus (GEO) database 3 — the largest genomics data repository to our knowledge — contained approximately 1.1 million samples with ‘cancer’ as a keyword. However, ImageNet, the largest public repository for computer vision, contains 15 million images 4 . Second, cancer research data are typically heterogeneous and may contain many dimensions measuring distinct aspects of cellular systems and biological processes. Modern multi-omics workflows may generate genome-wide mRNA expression, chromatin accessibility and protein expression data on single cells 5 , together with a spatial molecular readout 6 . The comparatively limited data size in each modality and the high heterogeneity among them necessitate the development of innovative computational approaches for integrating data from different dimensions and cohorts.
The subject of big data in cancer is of immense scope, and it is impossible to cover everything in one review. We therefore focus on key big-data analyses that led to conceptual advances in our understanding of cancer biology and impacted disease diagnosis and treatment decisions. Further, we detail reviews in the pertaining sections to direct interested readers to relevant resources. We acknowledge that our limited selection of topics and examples may omit important work, for which we sincerely apologize.
In this Review, we begin by describing major data sources. Next, we review and discuss data analysis approaches designed to leverage big datasets for cancer discoveries. We then introduce ongoing efforts to harness big data in clinically oriented, translational studies, the primary focus of this Review. Finally, we discuss current challenges and future steps to push forward big data use in cancer.
Common data types
There are five basic data types in cancer research: molecular omics data, perturbation phenotypic data, molecular interaction data, imaging data, and textual data. Molecular omics data describe the abundance or status of molecules in cellular systems and tissue samples. Such data are the most abundant type generated in cancer research from patient or preclinical samples, and include information on DNA mutations (genomics), chromatin or DNA states (epigenomics), protein abundance (proteomics), transcript abundance (transcriptomics) and metabolite abundance (metabolomics) (Table 1 ). Early studies relied on data from bulk samples to provide insights into cancer progressions, tumour heterogeneity and tumour evolution, by using well-designed computational approaches 7 , 8 , 9 , 10 . Following the development of single-cell technologies and decreases in sequencing costs, current molecular data can be generated at multisample and single-cell levels 11 , 12 and reveal tumour heterogeneity and evolution at a much higher resolution. Furthermore, genomic and transcriptomic readouts can include spatial information 13 , revealing cancer clonal evolutions within distinct regions and gene expression changes associated with clone-specific aberrations. Although more limited in resolution, conventional bulk analyses are still useful for analysing large patient cohorts as the generation of single-cell and spatial data is costly and often feasible for only a few tumours per study.
Perturbation phenotypic data describe how cell phenotypes, such as cell proliferation or the abundance of marker proteins, are altered following the suppression or amplification of gene levels 14 or drug treatments 15 , 16 . Common phenotyping experiments include perturbation screens using CRISPR knockout 17 , interference or activation 18 ; RNA interference 19 ; overexpression of open reading frames 20 ; or treatment with a library of drugs 15 , 16 . As a limitation, the generation of perturbation phenotypic data from clinical samples is still challenging due to the requirement of genetically manipulable live cells.
Molecular interaction data describe the potential function of molecules through their interacting with diverse partners. Common molecular interaction data types include data on protein–DNA interactions 21 , protein–RNA interactions 22 , protein–protein intercations 23 and 3D chromosomal interactions 24 . Similar to perturbation phenotypic data, molecular interaction datasets are typically generated using cell lines as their generation requires a large quantity of material that often exceeds that available from clinical samples.
Clinical data such as health records 25 , histopathology images 26 and radiology images 27 , 28 can also be of considerable value. The boundary between molecular omics and image data is not absolute as both can include information of the other type, for example in datasets that contain imaging scans and information on protein expression from a tumour sample (Table 1 ).
Data repositories and analytic platforms
We provide an overview of key data resources for cancer research organized in three categories. The first category comprises resources from projects that systematically generate data (Table 2 ); for example, TCGA generated transcriptomic, proteomic, genomic and epigenomic data for more than 10,000 cancer genomes and matched normal samples, spanning 33 cancer types. The second category describes repositories presenting processed data from the aforementioned projects (Table 3 ), such as the Genomic Data Commons, which hosts TCGA data for downloading. The third category includes Web applications that systematically integrate data across diverse projects and provide interactive analysis modules (Table 4 ). For example, the TIDE framework systematically collected public data from immuno-oncology studies and provided interactive modules to study pathways and regulation mechanisms underlying tumour immune evasion and immunotherapy response 29 .
In addition to cancer-focused large-scale projects enumerated in Table 2 , many individual groups have deposited genomic datasets that are useful for cancer research in general databases such as GEO 3 and ArrayExpress 30 . Curation of these datasets could lead to new resources for cancer biology studies. For example, the PRECOG database contains 166 transcriptomic studies collected from GEO and ArrayExpress with patient survival information for querying the association between gene expression and prognostic outcome 31 .
Integrative analysis
Although data-intensive studies may generate omics data on hundreds of patients, the data scale in cancer research is still far behind that in other fields, such as computer vision. Cross-cohort aggregation and cross-modality integration can markedly enhance the robustness and depth of big data analysis (Fig. 1 ). We discuss these strategies in the following subsections.
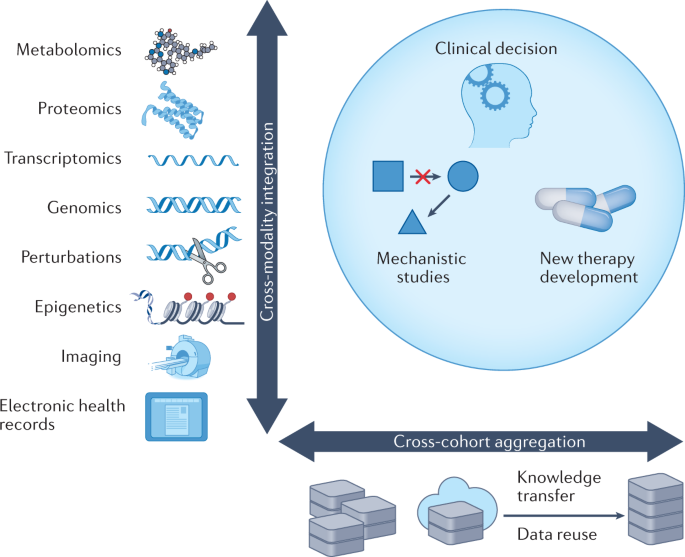
Clinical decisions, basic research and the development of new therapies should consider two orthogonal dimensions when leveraging big-data resources; integrating data across many data modalities and integrating data from different cohorts, which may include the transfer of knowledge from pre-existing datasets.
Cross-cohort data aggregation
Integration of datasets from multiple centres or studies can achieve more robust results and potentially new findings, especially where individual datasets are noisy, incomplete or biased with certain artefacts. A landmark of cross-cohort data aggregation is the discovery of the TMPRSS2 – ERG fusion and a less frequent TMPRSS2 – ETV1 fusion as oncogenic drivers in prostate cancer. A compendium analysis across 132 gene-expression datasets representing 10,486 microarray experiments first identified ERG and ETV1 as highly expressed genes in six independent prostate cancer cohorts 32 , further studies identified their fusions with TMPRSS2 as the cause of ERG and ETV1 overexpression. Another example is an integrative study of tumour immune evasion across many clinical datasets that revealed that SERPINB9 expression consistently correlates with intratumoural T cell dysfunction and resistance to immune checkpoint blockade 29 . Further studies found SERPINB9 activation to be an immune checkpoint blockade resistance mechanism in cancer cells 29 and immunosuppressive cells 33 .
A general approach for cross-cohort aggregation is to obtain public datasets that are related to a new research topic or have similar study designs to a new dataset. However, use of public data for a new analysis is challenging because the experimental design behind each published dataset is unique, requiring labour-intensive expert interpretation and manual standardization. A recent framework for data curation provides natural language processing and semi-automatic functions to unify datasets with heterogeneous meta-information into a format usable for algorithmic analysis 34 (Framework for Data Curation in Table 3 ).
Although data aggregation may generate robust hypotheses, batch effects caused by differences in laboratories, individual researcher’s techniques or platforms or other non-biological factors may mask or reduce the strength of signals uncovered 35 , and correcting for these effects is therefore a critical step in cross-cohort aggregations 36 , 37 . Popular batch effect correction approaches include the ComBat package, which uses empirical Bayes estimators to compute corrected data 36 , and the Seurat package, which creates integrated single-cell clusters anchored on similar cells between batches 38 . Despite the availability of batch correction methods, analysis of both original and corrected data is essential to draw reliable conclusions as batch correction can introduce false discoveries 39 .
Cross-modality data integration
Cross-modality integration of different data types is a promising and productive approach for maximizing the information gained from data as the information embedded in each data type is often complementary and synergistic 40 . Cross-modality data integration is exemplified by projects such as TCGA, which provides genomic, transcriptomic, epigenomic and proteomic data on the same set of tumours (Table 2 ). Cross-modality integration has led to many novel insights regarding factors associated with cancer progression. For example, the phosphorylation status of proteins in the EGFR signalling pathway — an indicator of EGFR signalling activity — is highly correlated with the expression of genes encoding EGFR ligands in head and neck cancers but not receptor expression, copy number alterations, protein levels or phosphorylations 41 , suggesting that patients should be stratified to receive anti-EGFR therapies on the basis of ligand abundance instead of receptor status.
A recent example of cross-modality data integration used single-cell multi-omics technologies that allowed genome-wide transcriptomics and chromatin accessibility data to be measured together with a handful of proteins of interest 42 . The advantages of using cross-modality data were clear as during cell lineage clustering, CD8 + T cell and CD4 + T cell populations could be clearly separated in the protein data but were blended when the transcriptome was analysed 42 . Conversely, dendritic cells formed distinct clusters when assessed on the basis of transcriptomic data, whereas they mixed with other cell types when assessed on the basis of cell-surface protein levels. Chromatin accessibility measured by assay for transposase-accessible chromatin using sequencing (ATAC-seq) further revealed T cell sublineages by capturing lineage-specific regulatory regions. For each cell, the study first identified neighbouring cells through similarities in each data modality. Then, the study defined the weights of the different data modalities in the lineage classification as their accuracy for predicting molecular profiles of the target cell from the profiles of neighbouring cells. The resulting cell clustering, using the weighted distance averaged across single-cell RNA, protein and chromatin accessibility data, was then shown to improve cell lineage separation 42 .
Another common type of multimodal data analysis involves integrating molecular omics data and data on physical interaction networks (typically those involving protein–protein or protein–DNA interactions) to understand how individual genes interact with each other to drive oncogenesis and metastasis 43 , 44 , 45 , 46 . For example, an integrative pan-cancer analysis of TCGA detected 407 master regulators organized into 24 modules, partly shared across cancer types, that appear to canalize heterogeneous sets of mutations 47 . In another study, an analysis of 2,583 whole-tumour genomes across 27 cancers by the Pan-Cancer Analysis of Whole Genomes Consortium revealed rare mutations in the promoters of genes with many interactions (such as TP53 , TLE4 and TCF4 ), and these mutations correlated with low downstream gene expression 45 . These examples of integrating networks and genomics data demonstrate a promising way to identify rare somatic mutations with a causal role in oncogenesis.
Knowledge transfer through data reuse
Existing data can be leveraged to make new discoveries. For example, cell-fraction deconvolution techniques can infer the composition of individual cell types in bulk-tumour transcriptomics profiles 48 . Such methods typically assemble gene expression profiles of diverse cell types from many existing datasets and perform regression or signature-enrichment analysis to deconvolve cell fractions 49 or lineage-specific expression 50 , 51 in a bulk-tumour expression profile.
Other data reuse examples come from single-cell transcriptomics data analysis. As single-cell RNA sequencing (scRNA-seq) has a high number of zero counts (dropout) 52 , analyses based on a limited number of genes may lead to unreliable results 53 , and genome-wide signatures from bulk data can therefore complement such analyses. For example, the transcriptomic data atlas collected from cytokine treatments in bulk cell cultures has enabled the reliable inference of signalling activities in scRNA-seq data 34 . Further, single-cell signalling activities inferred through bulk data have been used to reveal therapeutic targets, such as FIBP , to potentiate cellular therapies in solid tumours and molecular programmes of T cells that are resilient to immunosuppression in cancer 54 . In another example, the analysis of more than 50,000 scRNA-seq profiles from 35 pancreatic adenocarcinomas and control samples revealed edge cells among non-neoplastic acinar cells, whose transcriptomes have drifted towards malignant pancreatic adenocarcinoma cells 55 ; TCGA bulk pancreatic adenocarcinoma data were then used to validate the edge-cell signatures inferred from the single-cell data.
Data reuse can assist the development of new experimental tests. For example, existing tumour whole-exome sequencing data were used to optimize a circulating tumour DNA assay by maximizing the number of alterations detected per patient, while minimizing gene and region selection size 56 . The resulting circulating tumour DNA assay can provide a comprehensive view of therapy resistance and cancer relapse and metastasis by detecting alterations in DNA released from multiple tumour regions or different tumour sites 57 .
Although the data scale in cancer research is typically much smaller than in other fields, the number of input features, such as genes or imaging pixels, can be extremely high. Training a machine learning model with a high number of input dimensions (a large number of features) and small data size (a small number of training samples) is likely to lead to overfitting, in which the model learns noise from training data and cannot generalize on new data 58 . Transfer learning approaches are a promising way of addressing this disparity related to data reuse. These approaches involve training a neural network model on a large, related dataset, and then fine-tuning the model on the smaller, target dataset. For example, most cancer histopathology artificial intelligence (AI) frameworks start from pretrained architectures from ImageNet — an image database containing 15 million images with detailed hierarchical annotations 4 — and then fine-tune the framework on new imaging datasets of smaller sizes. As a further example of this approach, a few-shot learning framework enabled the prediction of drug response using data from only several patient-derived samples and a model pretrained using in vitro data from cell lines 59 . Despite these successful applications, transfer learning should be used with caution as it may produce mostly false predictions when data properties are markedly different between the pretraining set and the new dataset. Training a lightweight model 60 or augmenting the new dataset 61 are alternative solutions.
Data-rich translational studies
Many clinical diagnoses and decisions, such as histopathology interpretations, are inherently subjective and rely on interpreters’ experience or the availability of standardized diagnostic nomenclature and taxonomy. Such subjective factors may bring interpretive error 62 , 63 , 64 and diagnostic discrepancies, for example when senior stature can have an undue influence on diagnostic decisions — the so-called big-dog effect 65 . Big-data approaches can provide complementary options that are systematic and objective to guide diagnosis and clinical decisions.
Diagnostic biomarkers trained from data cohorts
A major focus of translational big-data studies in cancer has been the development of genomics tests for predicting disease risk, some of which have already been approved by the US Food and Drug Administration (FDA) and commercialized for clinical use 66 . Distinct from biomarker discoveries through biological mechanisms and empirical observations, big data-derived tests analyse genome-scale genomics data from many patients and cohorts to generate a gene signature for clinical assays 67 . Such predictors mainly help clinicians determine the minimal therapy aggressiveness needed to minimize unnecessary treatment and side effects. The success of such tests depends on their high negative predictive value — the proportion of negative tests that reflect true negative results — so as not to miss patients who need aggressive therapy options 66 .
Some early examples of diagnostic biomarker tests trained from big data include prognosis assays for patients with oestrogen receptor (ER)- or progesterone receptor (PR)-positive breast cancer, such as Oncotype DX 68 , 69 , MammaPrint 67 , 70 , EndoPredict 71 and Prosigna 72 . These tests are particularly useful as adjuvant endocrine therapy alone can bring sufficient clinical benefit to ER/PR-positive, HER2-negative patients with early-stage breast cancer 73 . Thus, patients stratified as being at low risk can avoid unnecessary additional chemotherapy. Predictors for other cancer types include Oncotype DX biomarkers for colon cancer 74 and prostate cancer 75 and Pervenio for early-stage lung cancer 76 .
In the early applications discussed above, large-scale data from genome-scale experiments served in the biomarker discovery stage but not in their clinical implementation. Owing to the high cost of genome-wide experiments and patent issues, the biomarker tests themselves still need to be performed through quantitative PCR or NanoString gene panels. However, the rapid decline of DNA sequencing costs in recent years could allow therapy decisions to be informed directly by genomics data and bring notable advantages over conventional approaches 77 . Gene alterations relevant to therapy decisions could involve diverse forms, including single-nucleotide mutations, DNA insertions, DNA deletions, copy number alterations, gene rearrangements, microsatellite instability and tumour mutational burden 78 , 79 , 80 . These alterations can be detected by combining hybridization-based capture and high-throughput sequencing. The MSK-IMPACT 81 and FoundationOne CDx 82 tests profile 300–500 genes and can use DNA from formalin-fixed, paraffin-embedded tumour specimens to detect oncogenic alterations and identify patients who may benefit from various therapies.
Variant interpretation in clinical decisions is still challenging as the oncogenic impact of each mutation depends on its clonality 83 , zygosity 84 and co-occurrences with other mutations 85 . Sequencing data can uncover tumorigenic processes (such as DNA repair defects, exogenous mutagen exposure and prior therapy histories 81 ) by identifying underlying mutational signatures, such as DNA substitution classes and sequence contexts 86 . Future computational frameworks for therapy decisions should therefore consider many dimensions of variants and inferred biological processes, together with other clinical data, such as histopathology data, radiology images and health records.
Data-rich assays that complement precision therapies currently focus on specific genomic aberrations. However, epigenetic therapies, such as inhibitors that target histone deacetylases 87 , have a genome-wide effect and are typically combined with other treatments, and therefore current genomics assays may not readily evaluate their therapeutic efficacy. We could not find any clinical datasets of histone deacetylase inhibitors deposited in the NCBI GEO database when writing this Review, indicating there are many unexplored territories of data-driven predictions for this broad category of anticancer therapies.
Clinical trials guided by molecular data
Genome-wide and multimodal data have begun to play a role in matching patients in prospective multi-arm clinical trials, particularly those investigating precision therapies. For example, the WINTHER trial prospectively matched patients with advanced cancer to therapy on the basis of DNA sequencing (arm A, through Foundation One assays) or RNA expression (arm B, comparing tumour tissue with normal tissue through Agilent oligonucleotide arrays) data from solid tumour biopsies 88 . Such therapy matches by omics data typically lead to off-label drug use. The WINTHER study concluded that both data types were of value for improving therapy recommendations and patient outcomes. Furthermore, there were no significant differences between DNA sequencing and RNA expression with regard to providing therapies with clinical benefits 88 , which was corroborated by a later study 89 .
Other, similar trials have demonstrated the utility of matching patients for off-label use of targeted therapies on the basis of genome-wide genomics or transcriptomics data 89 , 90 , 91 , 92 (Fig. 2 ). In these studies, the fraction of enrolled patients who had therapies matched by omics data ranged from 19% to 37% (WINTHER, 35% 88 ; POG, 37% 89 ; MASTER, 31.8% 92 ; MOSCATO 01, 19.2% 90 ; CoPPO, 20% 91 ). Among these matched patients, about one third demonstrated clinical benefits (WINTHER, 25% 88 ; POG, 46% 89 ; MASTER, 35.7% 92 ; MOSCATO 01, 33% 90 ; CoPPO, 32% 91 ). Except for the POG study, all studies used the end point defined by the Von Hoff model, which compares progression-free survival (PFS) for the trial (PFS2) with the PFS recorded for the therapy preceding enrolment (PFS1) and defines clinical benefit as a PFS2/PFS1 ratio of more than 1.3 (ref. 93 ).
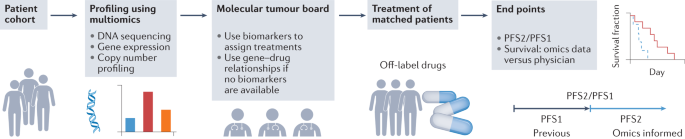
Recent umbrella clinical trials 88 , 89 , 90 , 91 , 92 have focused on multi-omics profiling of the tumours of enrolled patients by generating and analysing genome-wide data — including data from DNA sequencing, gene expression profiling, and copy number profiling — to prioritize treatments. After multi-omics profiling, a multidisciplinary molecular tumour board led by clinicians selects the best therapies on the basis of the current known relationships between drugs, genes and tumour vulnerabilities. For each therapy, the relevant altered vulnerabilities could include direct drug targets, genes in the same pathway, indirect drug targets upregulated or downregulated by drug treatment, or other genes interacting with the drug targets through physical or genetic interactions. This process then results in patients being treated with off-label targeted therapies. The end points for evaluating clinical efficacy include the ratio of the progression-free survival (PFS) associated with omics data-guided therapies (PFS2) and the PFS associated with previous therapy (PFS1), or differences in survival between patients treated with omics data-guided therapies and patients treated with therapies guided by physician’s choice alone.
A recent study demonstrated the feasibility and value of an N -of-one strategy that collected multimodal data, including immunohistochemistry data for multiple protein markers, RNA levels and genomics alterations in cell-free DNA from liquid biopsies 94 (Fig. 2 ). A broad multidisciplinary molecular tumour board (MTB) then made personalized decisions using these multimodal omics data. Overall, patients who received MTB-recommended treatments had significantly longer PFS and overall survival than those treated by independent physician choice. Similarly, another study also demonstrated overall survival benefits brought by MTB recommendations 95 .
With these initial successes, emerging clinical studies aim to collect additional data beyond bulk-sample sequencings — such as tumour cell death response following various drug treatments 96 or scRNA-seq data collected on longitudinal patient samples — to study therapy response and resistance mechanisms 97 . Besides omics data generated from tumour samples, cross-modality data integration is a potential strategy to improve therapy recommendations. One such promising direction involves the study and application of synthetic lethal interactions 98 , 99 , 100 , 101 , 102 , 103 , 104 , which, once integrated with tumour transcriptomic profiles, can accurately score drug target importance and predict clinical outcomes for many anticancer treatments, including targeted therapies and immunotherapies 98 . We foresee that new data modalities and assays will provide additional ways to design clinical trials.
Artificial intelligence for data-driven cancer diagnosis
Genomics datasets, such as gene expression levels or mutation status, can typically be aligned to each other on gene dimensions. However, data types in clinical diagnoses, such as imaging data or text reports, may not directly align across samples in any obvious way. AI approaches based on deep neural networks (Fig. 3a ) are an emerging method for integrating these data types for clinical applications 105 .
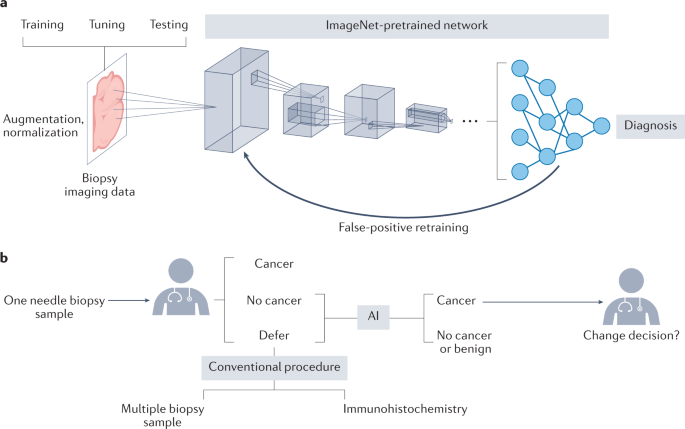
a | A common artificial intelligence (AI) framework in cancer detection uses a convolutional neural network (CNN) to detect the presence of cancer cells from a diagnostic image. CNNs use convolution (weighted sum of a region patch) and pooling (summarize values in a region to one value) to encode image regions into low-dimensional numerical vectors that can be analysed by machine learning models. The CNN architecture is typically pretrained with ImageNet data, which is much larger than any cancer biology imaging dataset. To increase the reliability of the AI framework, the input data can be augmented through rotation or blurring of tissue images to increase data size. The data are separated into non-overlapping training, tuning and test sets to train the AI model, tune hyperparameters and estimate the prediction accuracy on new inputs, respectively. False-positive predictions are typically essential data points for retraining the AI model. b | An example of the application of AI in informing clinical decisions, as per the US Food and Drug Administration-approved AI test Paige Prostate. From one needle biopsy sample, the pathologist can decide whether cancer cells are present. If the results are negative (‘no cancer’) or if the physician cannot make a firm diagnosis (‘defer’), the Paige Prostrate AI can analyse the image and prompt the pathologist with regard to potential cancer locations if any are detected. The alternative procedure involves evaluating multiple biopsy samples and performing immunohistochemistry tests on prostate cancer markers, independently from the AI test 185 .
The most popular application of AI for analysing imaging data involves clinical outcome prediction and tumour detection and grading from tissue stained with haematoxylin and eosin (H&E) 26 . In September 2021, the FDA approved the use of the AI software Paige Prostate 106 to assist pathologists in detecting cancer regions from prostate needle biopsy samples 107 (Fig. 3b ). This approval reflects the accelerating momentum of AI applications on histopathology images 108 to complement conventional pathologist practices and increase analysis throughput, particularly for less experienced pathologists. The CAMELYON challenge for identifying tumour regions provided 1,399 manually annotated whole-slide H&E-stained tissue images of sentinel lymph nodes from patients with breast cancer for training AI algorithms 109 . The top performers in the challenge used deep learning approaches, which achieved similar performance in detecting lymph node metastasis as expert pathologists 110 . Other studies have trained deep neural networks to predict patient survival outcomes 111 , gene mutations 112 or genomic alterations 113 , on the basis of analysing a large body of H&E-stained tissue images with clinical outcome labels or genomics profiles.
Besides histopathology, radiology is another application of AI imaging analysis. Deep convolutional neural networks that use 3D computed tomography volumes have been shown to predict the risk of lung cancer with an accuracy comparable to that of predictions by experienced radiologists 114 . Similarly, convolutional neural networks can use computed tomography data to stratify the survival duration of patients with lung cancer and highlight the importance of tumour-surrounding tissues in risk stratification 115 .
AI frameworks have started to play an important role in analysing electronic health records. A recent study evaluating the effect of different eligibility criteria on cancer trial outcomes using electronic health records of more than 60,000 patients with non-small-cell lung cancer revealed that many patient exclusion criteria commonly used in clinical trials had a minimal effect on trial hazard ratios 25 . Dropping these exclusion criteria would only marginally decrease the overall survival and result in more inclusive trials without compromising patient safety and overall trial success rates 25 . Besides images and health records, AI trained on other data types also has broad clinical applications, such as early cancer detection through liquid biopsies capturing cell-free DNA 116 , 117 or T cell receptor sequences 118 , or genomics-based cancer risk predictions 119 , 120 . Additional examples of AI applications in cancer are available in other reviews 40 , 121 .
New AI approaches have started to play a role in biological knowledge discovery. The saliency map 122 and class activation map 123 can highlight essential portions of input images that drive predicted outcomes. Also, in a multisample cohort, clustering data slices on the basis of deep learning-embedded similarities can reveal human-interpretable features associated with a clinical outcome. For example, clustering similar image patches related to colorectal cancer survival prediction revealed that high-risk survival predictions are associated with a tumour–adipose feature, characterized by poorly differentiated tumour cells adjacent to adipose tissue 124 . Although the molecular mechanisms underlying this association are unclear, this study provided an example of finding imaging features that could help cancer biologists pinpoint new disease mechanisms.
Despite the promising results described above, few AI-based algorithms have reached clinical deployment due to several limitations 26 . First, the performance of most AI predictors deteriorates when they are applied to test data generated in a setting different from that in which their training data are generated. For example, the performance of top algorithms from the CAMELYON challenge dropped by about 20% when they were evaluated on the basis of data from other centres 108 . Such a gap may arise from differences in image scanners (if imaging data are being evaluated), sample collection protocols or study design, emphasizing the need for reliable data homogenization. Second, supervised AI training requires a large amount of annotated data, and acquiring sufficient human-annotated data can be challenging. In imaging data, if a feature for a particular diagnosis is present in only a fraction of image regions, an algorithm will need many samples to learn the task. Furthermore, if features are not present in the training data, the AI will not make meaningful predictions; for example, the AI framework of AlphaFold2 can predict wild type protein structures with high accuracy, but it cannot predict the impact of cancer missense mutations on protein structures because the training data for AlphaFold2 do not contain altered structures of these mutated proteins 125 .
Many studies of AI applications that claim improvements lack comparisons with conventional clinical procedures. For example, the performance study of Paige Prostate evaluated cancer detection using an H&E-stained tissue image from one needle biopsy sample 126 . However, the pathologist may make decisions on the basis of multiple needle biopsy samples and immunohistochemistry stains for suspicious samples instead of relying on one H&E-stained tissue image (Fig. 3b ). Therefore, rigorous comparison with conventional clinical workflows is necessary for each application before the advantage of any AI framework is claimed.
New therapy development aided by big-data analysis
Developing a new drug is costly, is time-intensive and suffers from a high failure rate 127 . The development of new therapies is a promising direction for big-data applications. To our knowledge, no FDA-approved cancer drugs have been developed primarily through big-data approaches; however, some big data-driven preclinical studies have attracted the attention of the pharmaceutical industry for further development and may soon make impactful contributions to clinics 128 .
Big data have been used to aid the repurposing of existing drugs to treat new diseases 129 , 130 and the design of synergistic combinations 131 , 132 , 133 , 134 . By creating a network of 1.2 billion edges among diseases, tissues, genes, pathways and drugs by mining more than 40 million documents, one study revealed that the combination of vandetanib and everolimus could inhibit ACVR1, a drug efflux transporter, as a potential therapy for diffuse intrinsic pontine glioma 135 .
Recent studies have combined pharmacological data and AI to design new drugs (Fig. 4 ). A deep generative model was used to design new small molecules inhibiting the receptor tyrosine kinase DDR1 on the basis of information on existing DDR1 inhibitors and compound libraries, with the lead candidate demonstrating favourable pharmacokinetics in mice 136 . Deep generative models are neural networks with many layers that learn complex characteristics of specific datasets (such as high-dimensional probability distributions) and can use them to generate new data similar to the training data 137 . For each specific drug design application, such a framework can encode distinct data into the neural network parameters and thus naturally incorporate many data types. A network aiming to find novel kinase inhibitors, for example, may include data on the structure of existing kinase inhibitors, non-kinase inhibitors and patent-protected molecules that are to be avoided 136 .
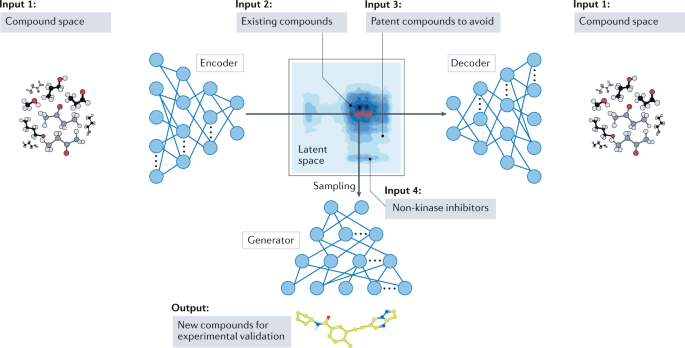
The variational autoencoder, trained with the structures of many compounds, can encode a molecular structure into a latent space of numerical vectors and decode this latent space back into the compound structure. For each target, such as the receptor tyrosine kinase DDR1, the variational autoencoder can create embeddings of compound categories, such as existing kinase inhibitors, patented compounds and non-kinase inhibitors. Sampling the latent space for compounds that are similar to existing on-target inhibitors and not patented compounds or non-kinase inhibitors can generate new candidate kinase inhibitors for downstream experimental validation. Adapted from ref. 136 , Springer Nature Limited.
AI can also be used for the virtual screening of bioactive ligands on target protein structures. Under the assumption that biochemical interactions are local among chemical groups, convolutional neural networks can comprehensively integrate training data from previous virtual screening studies to outperform previous docking methods based on minimizing empirical scores 138 . Similarly, a systematic evaluation revealed that deep neural networks trained using large and diverse datasets composed of molecular descriptors and drug biological activities could predict the activity of test-set molecules better than other approaches 139 .
Big data in front of narrow therapeutic bottlenecks
During dynamic tumour evolution, cancers generally become more heterogeneous and harbour a more diverse population of cells with different treatment sensitivities. Drug resistance can eventually evolve from a narrow bottleneck of a few cells 140 . Furthermore, the difference between a treatment dose with antitumour effects and toxicity leading to either clinical trial failure or treatment cessation is small 66 . These two challenges are common reasons for anticancer therapy failures as increasing drug combinations to target rare cancer cells will quickly lead to unacceptable toxic effects. An essential question is whether big data can bring solutions to overcome heterogeneous tumour evolution towards drug resistance while avoiding intolerable toxic effects.
Ideally, well-designed drug combinations should target various subsets of drug-tolerant cells in tumours and induce robust responses. Computational methods have been developed to design synergistic drug pairs 131 , 141 ; however, drug synergy may not be predictable for certain combinations even with comprehensive training data. A recent community effort assessed drug synergy prediction methods trained on AstraZeneca’s large drug combination dataset, consisting of 11,576 experiments from 910 combinations across 85 molecularly characterized cancer cell lines 134 . The results showed that none of the methods evaluated could make reliable predictions for approximately 20% of the drug pairs whose targets independently regulate downstream pathways.
There could be a theoretical limitation of the power of drug combinations in killing heterogeneous tumour cells while avoiding toxic effects on normal tissues. A recent study mining 15 single-cell transcriptomics datasets revealed that inhibition of four cell-surface targets is necessary to kill at least 80% of tumour cells while sparing at least 90% of normal cells in tumours 142 . However, a feasible drug-target combination may not exist to kill a higher fraction of tumour cells while sparing normal cells.
An important challenge accompanying therapy design efforts is the identification of genomic biomarkers that could predict toxicity. A community evaluation demonstrated that computational methods could predict the cytotoxicity of environmental chemicals on the basis of the genotype data of lymphoblastoid cell lines 143 . Further, a computational framework has been used to predict drug toxicity by integrating information on drug-target expression in tissues, gene network connectivity, chemical structures and toxicity annotations from clinical trials 144 . However, these studies were not explicitly designed for anticancer drugs, which are challenging with regard to toxicity prediction due to their extended cytotoxicity profiles.
Challenges and future perspectives
While many big-data advancements are encouraging and impressive, considerable challenges remain regarding big-data applications in cancer research and the clinic. Omics data often suffer from measurement inconsistencies between cohorts, marked batch effects and dependencies on specific experimental platforms. Such a lack of consistency is a major hurdle towards clinical translation. Consensus on the measurement, alignment and normalization of tumour omics data will be critical for each data type 35 . Besides these technical challenges, structural and societal challenges also exist and may impede the progress of the entire cancer data science field. We discuss these in the following subsections.
Less-than-desirable data availability
A key challenge of cancer data science is the insufficient availability of data and code. A recent study found that machine learning-based studies in the biomedical domain compare poorly with those in other areas regarding public data and source code availability 145 . Sometimes, the clinical information accompanying published cancer genomics data is not provided or complete, even when security and privacy issues are resolved. One possible reason for this bottleneck is related to data release policies and data stewardship costs. Although many journals require the public release of data, such requirements are often met by deposition of data into repositories that require author and institutional approval-of-access requests due to intellectual property and various other considerations. Furthermore, deposited data may be missing critical information, such as missing cell barcodes for single-cell sequencing data or low-resolution images in the case of histopathology data.
In our opinion, the mitigation of these issues will require the enforcement of policies regarding public data availability by funding agencies and additional community efforts to examine the fulfilment of open data access. For example, a funding agency may suspend a project if the community readers report any violations of data release agreements upon publication of articles. The allocation of budgets in grants for patient de-identification upon manuscript submission and financial incentives for checking data through independent data stewardship services upon paper acceptance could markedly help facilitate data and code availability. One notable advance in data availability through industry–academia alliances has come in the form of data-sharing initiatives; specifically, making large repositories of patient tumour sequencing and clinical data available for online queries to researchers in partner institutions 146 . Such initiatives typically involve query-only access (that is, without allowing downloads), but are an encouraging way to expand the collaborative network between academia and industry entities that generate massive amounts of data.
Data-scale gaps
As mentioned earlier, the datasets available for cancer therapeutics are substantially smaller than those available in other fields. One reason for such a gap is that the generation of medical data depends on professionally trained scientists. To close the data-scale gap, more investments will be required to automate the generation of at least some types of annotated medical data and patient omics data. Rare cancers especially suffer from a lack of preclinical models, clinical samples and dedicated funding 147 . Moreover, the usability of biomedical data is typically constrained by the genetic background of the population. For example, the frequency of actionable mutations may differ among East Asian, European and American populations 148 .
A further reason for the data-scale gap is a lack of data generation standards in cancer clinical and biology studies. For example, most clinical trials do not yet collect omics data from patients. With the exponential decrease in sequencing cost, collection of omics data in clinical trials should, in our opinion, be markedly expanded, and possibly be made mandatory as a standard requirement. Further, current data repositories, such as ClinicalTrials.gov and NCBI GEO, do not have common metalanguage standards, whose incorporation would markedly improve the development of algorithms applied to their analysis. Although semi-automated frameworks are becoming available to homogenize metadata 34 , the foundational solution should be establishing common vocabularies and systematic meta-information standards in critical fields.
Data science and AI are transforming our world through applications as diverse as self-driving cars, facial recognition and language translation, and in the medical world, the interpretation of images in radiology and pathology. We already have available tumour data to facilitate biomedical breakthroughs in cancer through cross-modality integration, cross-cohort aggregation and data reuse, and extraordinary advancements are being made in generating and analysing such data. However, the state of big data in the field is complex, and in our view, we should acknowledge that ‘big data’ in cancer are not yet so big. Future investments from the global research community to expand cancer datasets will be critical to allow better computational models to drive basic research, cancer diagnostics and the development of new therapies.
Hanahan, D. & Weinberg, R. A. Hallmarks of cancer: the next generation. Cell 144 , 646–674 (2011).
Article CAS PubMed Google Scholar
Weinstein, J. N. et al. The cancer genome atlas pan-cancer analysis project. Nat. Genet. 45 , 1113–110 (2013).
Article PubMed PubMed Central Google Scholar
Edgar, R., Domrachev, M. & Lash, A. E. Gene expression omnibus: NCBI gene expression and hybridization array data repository. Nucleic Acids Res. 30 , 207–210 (2002).
Article CAS PubMed PubMed Central Google Scholar
Deng, J. et al. ImageNet: a large-scale hierarchical image database. 2009 IEEE Conf. Computer Vis. Pattern Recognit. https://doi.org/10.1109/cvprw.2009.5206848 (2009).
Article Google Scholar
Stuart, T. & Satija, R. Integrative single-cell analysis. Nat. Rev. Genet. 20 , 257–272 (2019).
Ji, A. L. et al. Multimodal analysis of composition and spatial architecture in human squamous cell carcinoma. Cell 182 , 1661–1662 (2020).
Deshwar, A. G. et al. PhyloWGS: reconstructing subclonal composition and evolution from whole-genome sequencing of tumors. Genome Biol. 16 , 35 (2015).
Roth, A. et al. PyClone: statistical inference of clonal population structure in cancer. Nat. Methods 11 , 396–398 (2014).
Miller, C. A. et al. SciClone: inferring clonal architecture and tracking the spatial and temporal patterns of tumor evolution. PLoS Comput. Biol. 10 , e1003665 (2014).
Carter, S. L. et al. Absolute quantification of somatic DNA alterations in human cancer. Nat. Biotechnol. 30 , 413–421 (2012).
Minussi, D. C. et al. Breast tumours maintain a reservoir of subclonal diversity during expansion. Nature 592 , 302–308 (2021).
Laks, E. et al. Clonal decomposition and DNA replication states defined by scaled single-cell genome sequencing. Cell 179 , 1207–1221.e22 (2019).
Zhao, T. et al. Spatial genomics enables multi-modal study of clonal heterogeneity in tissues. Nature 601 , 85–91 (2022).
Przybyla, L. & Gilbert, L. A. A new era in functional genomics screens. Nat. Rev. Genet. 23 , 89–103 (2022).
Barretina, J. et al. The Cancer Cell Line Encyclopedia enables predictive modelling of anticancer drug sensitivity. Nature 483 , 603–607 (2012).
Subramanian, A. et al. A next generation connectivity map: L1000 platform and the first 1,000,000 profiles. Cell 171 , 1437–1452.e17 (2017).
Shalem, O., Sanjana, N. E. & Zhang, F. High-throughput functional genomics using CRISPR-Cas9. Nat. Rev. Genet. 16 , 299–311 (2015).
Gilbert, L. A. et al. Genome-scale CRISPR-mediated control of gene repression and activation. Cell 159 , 647–661 (2014).
Tsherniak, A. et al. Defining a cancer dependency map. Cell 170 , 564–576.e16 (2017).
Johannessen, C. M. et al. A melanocyte lineage program confers resistance to MAP kinase pathway inhibition. Nature 504 , 138–142 (2013).
Robertson, G. et al. Genome-wide profiles of STAT1 DNA association using chromatin immunoprecipitation and massively parallel sequencing. Nat. Methods 4 , 651–657 (2007).
Hafner, M. et al. CLIP and complementary methods. Nat. Rev. Methods Prim. 1 , 20 (2021).
Article CAS Google Scholar
Vidal, M., Cusick, M. E. & Barabási, A.-L. Interactome networks and human disease. Cell 144 , 986–998 (2011).
Kempfer, R. & Pombo, A. Methods for mapping 3D chromosome architecture. Nat. Rev. Genet. 21 , 207–226 (2020).
Liu, R. et al. Evaluating eligibility criteria of oncology trials using real-world data and AI. Nature 592 , 629–633 (2021).
van der Laak, J., Litjens, G. & Ciompi, F. Deep learning in histopathology: the path to the clinic. Nat. Med. 27 , 775–784 (2021).
Article PubMed Google Scholar
Hosny, A., Parmar, C., Quackenbush, J., Schwartz, L. H. & Hjwl, A. Artificial intelligence in radiology. Nat. Rev. Cancer 18 , 500–510 (2018).
Gillies, R. J., Kinahan, P. E. & Hricak, H. Radiomics: images are more than pictures, they are data. Radiology 278 , 563–577 (2016).
Jiang, P. et al. Signatures of T cell dysfunction and exclusion predict cancer immunotherapy response. Nat. Med. 24 , 1550–1558 (2018). This integrative study of tumour immune evasion across many clinical datasets reveals that SERPINB9 expression consistently correlates with intratumoural T cell dysfunction and resistance to immune checkpoint blockade .
Parkinson, H. et al. ArrayExpress — a public database of microarray experiments and gene expression profiles. Nucleic Acids Res. 35 , D747–D750 (2007).
Gentles, A. J. et al. The prognostic landscape of genes and infiltrating immune cells across human cancers. Nat. Med. 21 , 938–945 (2015).
Tomlins, S. A. et al. Recurrent fusion of TMPRSS2 and ETS transcription factor genes in prostate cancer. Science 310 , 644–648 (2005). This compendium analysis across 132 gene expression datasets representing 10,486 microarray experiments identifies ERG and ETV1 fused with TMPRSS2 as highly expressed genes in six independent prostate cancer cohorts .
Jiang, L. et al. Direct tumor killing and immunotherapy through anti-serpinB9 therapy. Cell 183 , 1219–1233.e18 (2020).
Jiang, P. et al. Systematic investigation of cytokine signaling activity at the tissue and single-cell levels. Nat. Methods 18 , 1181–1191 (2021). This study describes a transcriptomic data atlas collected from cytokine treatments in bulk cell cultures, which enables the inference of signalling activities in bulk and single-cell transcriptomics data to study human inflammatory diseases .
Leek, J. T. et al. Tackling the widespread and critical impact of batch effects in high-throughput data. Nat. Rev. Genet. 11 , 733–739 (2010).
Johnson, W. E., Li, C. & Rabinovic, A. Adjusting batch effects in microarray expression data using empirical Bayes methods. Biostatistics 8 , 118–127 (2007).
Butler, A., Hoffman, P., Smibert, P., Papalexi, E. & Satija, R. Integrating single-cell transcriptomic data across different conditions, technologies, and species. Nat. Biotechnol. 36 , 411–420 (2018).
Stuart, T. et al. Comprehensive integration of single-cell data. Cell 177 , 1888–1902.e21 (2019).
Nygaard, V., Rødland, E. A. & Hovig, E. Methods that remove batch effects while retaining group differences may lead to exaggerated confidence in downstream analyses. Biostatistics 17 , 29–39 (2016).
Boehm, K. M., Khosravi, P., Vanguri, R., Gao, J. & Shah, S. P. Harnessing multimodal data integration to advance precision oncology. Nat. Rev. Cancer 22 , 114–126 (2022).
Huang, C. et al. Proteogenomic insights into the biology and treatment of HPV-negative head and neck squamous cell carcinoma. Cancer Cell 39 , 361–379.e16 (2021).
Hao, Y. et al. Integrated analysis of multimodal single-cell data. Cell 184 , 3573–3587.e29 (2021). This study integrates multiple single-cell data modalities, such as gene expression, cell-surface protein levels and chromatin accessibilities, to increase the accuracy of cell lineage clustering .
Klein, M. I. et al. Identifying modules of cooperating cancer drivers. Mol. Syst. Biol. 17 , e9810 (2021).
Hofree, M., Shen, J. P., Carter, H., Gross, A. & Ideker, T. Network-based stratification of tumor mutations. Nat. Methods 10 , 1108–1115 (2013).
Reyna, M. A. et al. Pathway and network analysis of more than 2500 whole cancer genomes. Nat. Commun. 11 , 729 (2020).
Zheng, F. et al. Interpretation of cancer mutations using a multiscale map of protein systems. Science 374 , eabf3067 (2021).
Paull, E. O. et al. A modular master regulator landscape controls cancer transcriptional identity. Cell 184 , 334–351 (2021).
Avila Cobos, F., Alquicira-Hernandez, J., Powell, J. E., Mestdagh, P. & De Preter, K. Benchmarking of cell type deconvolution pipelines for transcriptomics data. Nat. Commun. 11 , 5650 (2020).
Newman, A. M. et al. Robust enumeration of cell subsets from tissue expression profiles. Nat. Methods 12 , 453–457 (2015).
Newman, A. M. et al. Determining cell type abundance and expression from bulk tissues with digital cytometry. Nat. Biotechnol. 37 , 773–782 (2019).
Wang, K. et al. Deconvolving clinically relevant cellular immune cross-talk from bulk gene expression using CODEFACS and LIRICS stratifies patients with melanoma to anti-PD-1 therapy. Cancer Discov. 12 , 1088–1105 (2022). Together with Newman et al. (2019), this study demonstrates that assembling gene expression profiles of diverse cell types from existing datasets can enable deconvolution of cell fractions and lineage-specific expression in a bulk-tumour expression profile .
Kharchenko, P. V., Silberstein, L. & Scadden, D. T. Bayesian approach to single-cell differential expression analysis. Nat. Methods 11 , 740–742 (2014).
Suvà, M. L. & Tirosh, I. Single-cell RNA sequencing in cancer: lessons learned and emerging challenges. Mol. Cell 75 , 7–12 (2019).
Zhang, Y. et al. A T cell resilience model associated with response to immunotherapy in multiple tumor types. Nat. Med. https://doi.org/10.1038/s41591-022-01799-y (2022). This study uses a computational model to repurpose a vast amount of single-cell transcriptomics data and identify biomarkers of tumour-resilient T cells and new therapeutic targets, such as FIBP , to potentiate cellular immunotherapies .
Gopalan, V. et al. A transcriptionally distinct subpopulation of healthy acinar cells exhibit features of pancreatic progenitors and PDAC. Cancer Res. 81 , 3958–3970 (2021).
Newman, A. M. et al. An ultrasensitive method for quantitating circulating tumor DNA with broad patient coverage. Nat. Med. 20 , 548–554 (2014).
Heitzer, E., Haque, I. S., Roberts, C. E. S. & Speicher, M. R. Current and future perspectives of liquid biopsies in genomics-driven oncology. Nat. Rev. Genet. 20 , 71–88 (2019).
Hastie, T., Friedman, J. & Tibshirani, R. The Elements of Statistical Learning (Springer, 2001).
Ma, J. et al. Few-shot learning creates predictive models of drug response that translate from high-throughput screens to individual patients. Nat. Cancer 2 , 233–244 (2021).
Raghu, M., Zhang, C., Kleinberg, J. & Bengio, S. Transfusion: understanding transfer learning for medical imaging. Adv. Neural Inf. Process. Syst . 33 , 3347–3357 (2019).
Google Scholar
Zoph, B. et al. Rethinking pre-training and self-training. Adv. Neural Inf. Process. Syst . 34 , 3833–3845 (2020).
Meier, F. A., Varney, R. C. & Zarbo, R. J. Study of amended reports to evaluate and improve surgical pathology processes. Adv. Anat. Pathol. 18 , 406–413 (2011).
Nakhleh, R. E. Error reduction in surgical pathology. Arch. Pathol. Lab. Med. 130 , 630–632 (2006).
Nakhleh, R. E. et al. Interpretive diagnostic error reduction in surgical pathology and cytology: guideline from the College of American Pathologists Pathology and Laboratory Quality Center and the Association of Directors of Anatomic and Surgical Pathology. Arch. Pathol. Lab. Med. 140 , 29–40 (2016).
Raab, S. S. et al. The ‘Big Dog’ effect: variability assessing the causes of error in diagnoses of patients with lung cancer. J. Clin. Oncol. 24 , 2808–2814 (2006).
Jiang, P., Sellers, W. R. & Liu, X. S. Big data approaches for modeling response and resistance to cancer drugs. Annu. Rev. Biomed. Data Sci. 1 , 1–27 (2018).
van’t Veer, L. J. et al. Gene expression profiling predicts clinical outcome of breast cancer. Nature 415 , 530–536 (2002).
Sparano, J. A. et al. Adjuvant chemotherapy guided by a 21-gene expression assay in breast cancer. N. Engl. J. Med. 379 , 111–121 (2018).
Kalinsky, K. et al. 21-gene assay to inform chemotherapy benefit in node-positive breast cancer. N. Engl. J. Med. 385 , 2336–2347 (2021).
Cardoso, F. et al. 70-gene signature as an aid to treatment decisions in early-stage breast cancer. N. Engl. J. Med. 375 , 717–729 (2016).
Filipits, M. et al. A new molecular predictor of distant recurrence in ER-positive, HER2-negative breast cancer adds independent information to conventional clinical risk factors. Clin. Cancer Res. 17 , 6012–6020 (2011).
Parker, J. S. et al. Supervised risk predictor of breast cancer based on intrinsic subtypes. J. Clin. Oncol. 27 , 1160–1167 (2009).
Early Breast Cancer Trialists’ Collaborative Group (EBCTCG). Effects of chemotherapy and hormonal therapy for early breast cancer on recurrence and 15-year survival: an overview of the randomised trials. Lancet 365 , 1687–1717 (2005).
You, Y. N., Rustin, R. B. & Sullivan, J. D. Onco type DX® colon cancer assay for prediction of recurrence risk in patients with stage II and III colon cancer: a review of the evidence. Surg. Oncol. 24 , 61–66 (2015).
Klein, E. A. et al. A 17-gene assay to predict prostate cancer aggressiveness in the context of Gleason grade heterogeneity, tumor multifocality, and biopsy undersampling. Eur. Urol. 66 , 550–560 (2014).
Kratz, J. R. et al. A practical molecular assay to predict survival in resected non-squamous, non-small-cell lung cancer: development and international validation studies. Lancet 379 , 823–832 (2012).
Beaubier, N. et al. Integrated genomic profiling expands clinical options for patients with cancer. Nat. Biotechnol. 37 , 1351–1360 (2019).
Snyder, A. et al. Genetic basis for clinical response to CTLA-4 blockade in melanoma. N. Engl. J. Med. 371 , 2189–2199 (2014).
Van Allen, E. M. et al. Genomic correlates of response to CTLA-4 blockade in metastatic melanoma. Science 350 , 207–211 (2015).
Rizvi, N. A. et al. Cancer immunology. Mutational landscape determines sensitivity to PD-1 blockade in non-small cell lung cancer. Science 348 , 124–128 (2015).
Zehir, A. et al. Mutational landscape of metastatic cancer revealed from prospective clinical sequencing of 10,000 patients. Nat. Med. 23 , 703–713 (2017).
Li, M. Statistical methods for clinical validation of follow-on companion diagnostic devices via an external concordance study. Stat. Biopharm. Res. 8 , 355–363 (2016).
Litchfield, K. et al. Meta-analysis of tumor- and T cell-intrinsic mechanisms of sensitization to checkpoint inhibition. Cell 184 , 596–614.e14 (2021).
Bielski, C. M. et al. Widespread selection for oncogenic mutant allele imbalance in cancer. Cancer Cell 34 , 852–862.e4 (2018).
El Tekle, G. et al. Co-occurrence and mutual exclusivity: what cross-cancer mutation patterns can tell us. Trends Cancer Res. 7 , 823–836 (2021).
Alexandrov, L. B. et al. Signatures of mutational processes in human cancer. Nature 500 , 415–421 (2013).
Cheng, Y. et al. Targeting epigenetic regulators for cancer therapy: mechanisms and advances in clinical trials. Signal Transduct. Target. Ther. 4 , 62 (2019).
Rodon, J. et al. Genomic and transcriptomic profiling expands precision cancer medicine: the WINTHER trial. Nat. Med. 25 , 751–758 (2019). This study describes the WINTHER trial, which prospectively matched patients with advanced cancer to therapy on the basis of DNA sequencing or RNA expression data from tumour biopsies and concluded that both data types were of value for improving therapy recommendations .
Pleasance, E. et al. Whole genome and transcriptome analysis enhances precision cancer treatment options. Ann. Oncol. https://doi.org/10.1016/j.annonc.2022.05.522 (2022).
Massard, C. et al. High-throughput genomics and clinical outcome in hard-to-treat advanced cancers: results of the MOSCATO 01 trial. Cancer Discov. 7 , 586–595 (2017).
Tuxen, I. V. et al. Copenhagen Prospective Personalized Oncology (CoPPO) — clinical utility of using molecular profiling to select patients to phase I trials. Clin. Cancer Res. 25 , 1239–1247 (2019).
Horak, P. et al. Comprehensive genomic and transcriptomic analysis for guiding therapeutic decisions in patients with rare cancers. Cancer Discov. 11 , 2780–2795 (2021).
Von Hoff, D. D. et al. Pilot study using molecular profiling of patients’ tumors to find potential targets and select treatments for their refractory cancers. J. Clin. Oncol. 28 , 4877–4883 (2010).
Kato, S. et al. Real-world data from a molecular tumor board demonstrates improved outcomes with a precision N-of-one strategy. Nat. Commun. 11 , 4965 (2020).
Hoefflin, R. et al. Personalized clinical decision making through implementation of a molecular tumor board: a German single-center experience. JCO Precis. Oncol . 1–16 https://doi.org/10.1200/po.18.00105 (2018).
Irmisch, A. et al. The Tumor Profiler Study: integrated, multi-omic, functional tumor profiling for clinical decision support. Cancer Cell 39 , 288–293 (2021).
Cohen, Y. C. et al. Identification of resistance pathways and therapeutic targets in relapsed multiple myeloma patients through single-cell sequencing. Nat. Med. 27 , 491–503 (2021).
Lee, J. S. et al. Synthetic lethality-mediated precision oncology via the tumor transcriptome. Cell 184 , 2487–2502.e13 (2021). This study demonstrates that integrating information regarding synthetic lethal interactions with tumour transcriptomics profiles can accurately score drug-target importance and predict clinical outcomes for a broad category of anticancer treatments .
Zhang, B. et al. The tumor therapy landscape of synthetic lethality. Nat. Commun. 12 , 1275 (2021).
Pathria, G. et al. Translational reprogramming marks adaptation to asparagine restriction in cancer. Nat. Cell Biol. 21 , 1590–1603 (2019).
Feng, X. et al. A platform of synthetic lethal gene interaction networks reveals that the GNAQ uveal melanoma oncogene controls the Hippo pathway through FAK. Cancer Cell 35 , (2019).
Lee, J. S. et al. Harnessing synthetic lethality to predict the response to cancer treatment. Nat. Commun. 9 , 2546 (2018).
Cheng, K., Nair, N. U., Lee, J. S. & Ruppin, E. Synthetic lethality across normal tissues is strongly associated with cancer risk, onset, and tumor suppressor specificity. Sci. Adv. 7 , eabc2100 (2021).
Sahu, A. D. et al. Genome-wide prediction of synthetic rescue mediators of resistance to targeted and immunotherapy. Mol. Syst. Biol. 15 , e8323 (2019).
Elemento, O., Leslie, C., Lundin, J. & Tourassi, G. Artificial intelligence in cancer research, diagnosis and therapy. Nat. Rev. Cancer 21 , 747–752 (2021).
Raciti, P. et al. Novel artificial intelligence system increases the detection of prostate cancer in whole slide images of core needle biopsies. Mod. Pathol. 33 , 2058–2066 (2020).
Office of the Commissioner. FDA authorizes software that can help identify prostate cancer. https://www.fda.gov/news-events/press-announcements/fda-authorizes-software-can-help-identify-prostate-cancer (2021).
Campanella, G. et al. Clinical-grade computational pathology using weakly supervised deep learning on whole slide images. Nat. Med. 25 , 1301–1309 (2019).
Litjens, G. et al. 1399 H&E-stained sentinel lymph node sections of breast cancer patients: the CAMELYON dataset. GigaScience 7 , giy065 (2018).
Article PubMed Central Google Scholar
Ehteshami Bejnordi, B. et al. Diagnostic assessment of deep learning algorithms for detection of lymph node metastases in women with breast cancer. JAMA 318 , 2199–2210 (2017).
Wulczyn, E. et al. Deep learning-based survival prediction for multiple cancer types using histopathology images. PLoS ONE 15 , e0233678 (2020).
Coudray, N. et al. Classification and mutation prediction from non-small cell lung cancer histopathology images using deep learning. Nat. Med. 24 , 1559–1567 (2018).
Kather, J. N. et al. Deep learning can predict microsatellite instability directly from histology in gastrointestinal cancer. Nat. Med. 25 , 1054–1056 (2019).
Ardila, D. et al. End-to-end lung cancer screening with three-dimensional deep learning on low-dose chest computed tomography. Nat. Med. 25 , 954–961 (2019).
Hosny, A. et al. Deep learning for lung cancer prognostication: a retrospective multi-cohort radiomics study. PLoS Med. 15 , e1002711 (2018).
Zviran, A. et al. Genome-wide cell-free DNA mutational integration enables ultra-sensitive cancer monitoring. Nat. Med. 26 , 1114–1124 (2020).
Mathios, D. et al. Detection and characterization of lung cancer using cell-free DNA fragmentomes. Nat. Commun. 12 , 5060 (2021).
Beshnova, D. et al. De novo prediction of cancer-associated T cell receptors for noninvasive cancer detection. Sci. Transl. Med. 12 , eaaz3738 (2020).
Katzman, J. L. et al. DeepSurv: personalized treatment recommender system using a Cox proportional hazards deep neural network. BMC Med. Res. Methodol. 18 , 24 (2018).
Ching, T., Zhu, X. & Garmire, L. X. Cox-nnet: an artificial neural network method for prognosis prediction of high-throughput omics data. PLoS Comput. Biol. 14 , e1006076 (2018).
Kann, B. H., Hosny, A. & Hjwl, A. Artificial intelligence for clinical oncology. Cancer Cell 39 , 916–927 (2021).
Kadir, T. & Brady, M. Saliency, scale and image description. Int. J. Comput. Vis. 45 , 83–105 (2001).
Zhou, B., Khosla, A., Lapedriza, A., Oliva, A. & Torralba, A. Learning deep features for discriminative localization. 2016 IEEE Conf. Comput. Vis. Pattern Recognit. (CVPR) https://doi.org/10.1109/cvpr.2016.319 https://www.computer.org/csdl/proceedings/cvpr/2016/12OmNqH9hnp (2016).
Wulczyn, E. et al. Interpretable survival prediction for colorectal cancer using deep learning. NPJ Digit. Med. 4 , 71 (2020). This study clusters similar image patches related to colorectal cancer survival prediction to reveal that high-risk survival predictions are associated with a tumour–adipose feature, characterized by poorly differentiated tumour cells adjacent to adipose tissue .
Buel, G. R. & Walters, K. J. Can AlphaFold2 predict the impact of missense mutations on structure? Nat. Struct. Mol. Biol. 29 , 1–2 (2022).
US Food and Drug Administration. Evaluation of automatic class III designation for Paige Prostate. https://www.accessdata.fda.gov/cdrh_docs/reviews/DEN200080.pdf (2021).
Calcoen, D., Elias, L. & Yu, X. What does it take to produce a breakthrough drug? Nat. Rev. Drug Discov. 14 , 161–162 (2015).
Jayatunga, M. K. P., Xie, W., Ruder, L., Schulze, U. & Meier, C. AI in small-molecule drug discovery: a coming wave? Nat. Rev. Drug Discov. 21 , 175–176 (2022).
Pushpakom, S. et al. Drug repurposing: progress, challenges and recommendations. Nat. Rev. Drug Discov. 18 , 41–58 (2019).
Jahchan, N. S. et al. A drug repositioning approach identifies tricyclic antidepressants as inhibitors of small cell lung cancer and other neuroendocrine tumors. Cancer Discov. 3 , 1364–1377 (2013).
Kuenzi, B. M. et al. Predicting drug response and synergy using a deep learning model of human cancer cells. Cancer Cell 38 , 672–684.e6 (2020).
Ling, A. & Huang, R. S. Computationally predicting clinical drug combination efficacy with cancer cell line screens and independent drug action. Nat. Commun. 11 , 5848 (2020).
Aissa, A. F. et al. Single-cell transcriptional changes associated with drug tolerance and response to combination therapies in cancer. Nat. Commun. 12 , 1628 (2021).
Menden, M. P. et al. Community assessment to advance computational prediction of cancer drug combinations in a pharmacogenomic screen. Nat. Commun. 10 , 2674 (2019).
Carvalho, D. M. et al. Repurposing vandetanib plus everolimus for the treatment of ACVR1-mutant diffuse intrinsic pontine glioma. Cancer Discov. https://doi.org/10.1158/2159-8290.CD-20-1201 (2021).
Zhavoronkov, A. et al. Deep learning enables rapid identification of potent DDR1 kinase inhibitors. Nat. Biotechnol. 37 , 1038–1040 (2019). This study describes a deep generative AI model, which enabled the design of new inhibitors of the receptor tyrosine kinase DDR1 by modelling molecule structures from a compound library, existing DDR1 inhibitors, non-kinase inhibitors and patented drugs .
Ruthotto, L. & Haber, E. An introduction to deep generative modeling. GAMM-Mitteilungen 44 , e202100008 (2021).
Wallach, I., Dzamba, M. & Heifets, A. AtomNet: a deep convolutional neural network for bioactivity prediction in structure-based drug discovery. Preprint at https://arxiv.org/abs/1510.02855 (2015).
Ma, J., Sheridan, R. P., Liaw, A., Dahl, G. E. & Svetnik, V. Deep neural nets as a method for quantitative structure–activity relationships. J. Chem. Inf. Model. 55 , 263–274 (2015).
Dagogo-Jack, I. & Shaw, A. T. Tumour heterogeneity and resistance to cancer therapies. Nat. Rev. Clin. Oncol. 15 , 81–94 (2018).
Bansal, M. et al. A community computational challenge to predict the activity of pairs of compounds. Nat. Biotechnol. 32 , 1213–1222 (2014).
Ahmadi, S. et al. The landscape of receptor-mediated precision cancer combination therapy via a single-cell perspective. Nat. Commun. 13 , 1613 (2022).
Eduati, F. et al. Prediction of human population responses to toxic compounds by a collaborative competition. Nat. Biotechnol. 33 , 933–940 (2015).
Gayvert, K. M., Madhukar, N. S. & Elemento, O. A data-driven approach to predicting successes and failures of clinical trials. Cell Chem. Biol. 23 , 1294–1301 (2016).
McDermott, M. B. A. et al. Reproducibility in machine learning for health research: still a ways to go. Sci. Transl. Med. 13 , eabb1655 (2021).
AP News. Caris Precision Oncology Alliance partners with the National Cancer Institute, part of the National Institutes of Health, to expand collaborative clinical research efforts. Associated Press https://apnews.com/press-release/pr-newswire/technology-science-business-health-cancer-221e9238956a7a4835be75cb65832573 (2021).
Alvi, M. A., Wilson, R. H. & Salto-Tellez, M. Rare cancers: the greatest inequality in cancer research and oncology treatment. Br. J. Cancer 117 , 1255–1257 (2017).
Park, K. H. et al. Genomic landscape and clinical utility in Korean advanced pan-cancer patients from prospective clinical sequencing: K-MASTER program. Cancer Discov. 12 , 938–948 (2022).
Bailey, M. H. et al. Retrospective evaluation of whole exome and genome mutation calls in 746 cancer samples. Nat. Commun. 11 , 4748 (2020).
Ellrott, K. et al. Scalable open science approach for mutation calling of tumor exomes using multiple genomic pipelines. Cell Syst. 6 , 271–281.e7 (2018).
Zare, F., Dow, M., Monteleone, N., Hosny, A. & Nabavi, S. An evaluation of copy number variation detection tools for cancer using whole exome sequencing data. BMC Bioinforma. 18 , 286 (2017).
Pan-cancer analysis of whole genomes. Nature 578 , 82–93 (2020).
Gawad, C., Koh, W. & Quake, S. R. Single-cell genome sequencing: current state of the science. Nat. Rev. Genet. 17 , 175–188 (2016).
Corces, M. R. et al. The chromatin accessibility landscape of primary human cancers. Science 362 , eaav1898 (2018).
Buenrostro, J. D. et al. Single-cell chromatin accessibility reveals principles of regulatory variation. Nature 523 , 486–490 (2015).
Furey, T. S. ChIP–seq and beyond: new and improved methodologies to detect and characterize protein–DNA interactions. Nat. Rev. Genet. 13 , 840–852 (2012).
Rotem, A. et al. Single-cell ChIP-seq reveals cell subpopulations defined by chromatin state. Nat. Biotechnol. 33 , 1165–1172 (2015).
Papanicolau-Sengos, A. & Aldape, K. DNA methylation profiling: an emerging paradigm for cancer diagnosis. Annu. Rev. Pathol. 17 , 295–321 (2022).
Smallwood, S. A. et al. Single-cell genome-wide bisulfite sequencing for assessing epigenetic heterogeneity. Nat. Methods 11 , 817–820 (2014).
Cieślik, M. & Chinnaiyan, A. M. Cancer transcriptome profiling at the juncture of clinical translation. Nat. Rev. Genet. 19 , 93–109 (2018).
Macosko, E. Z. et al. Highly parallel genome-wide expression profiling of individual cells using nanoliter droplets. Cell 161 , 1202–1214 (2015).
Zheng, G. X. Y. et al. Massively parallel digital transcriptional profiling of single cells. Nat. Commun. 8 , 14049 (2017).
Ramsköld, D. et al. Full-length mRNA-Seq from single-cell levels of RNA and individual circulating tumor cells. Nat. Biotechnol. 30 , 777–782 (2012).
Gierahn, T. M. et al. Seq-Well: portable, low-cost RNA sequencing of single cells at high throughput. Nat. Methods 14 , 395–398 (2017).
Rao, A., Barkley, D., França, G. S. & Yanai, I. Exploring tissue architecture using spatial transcriptomics. Nature 596 , 211–220 (2021).
Ståhl, P. L. et al. Visualization and analysis of gene expression in tissue sections by spatial transcriptomics. Science 353 , 78–82 (2016).
Rodriques, S. G. et al. Slide-seq: a scalable technology for measuring genome-wide expression at high spatial resolution. Science 363 , 1463–1467 (2019).
Lee, J. H. et al. Fluorescent in situ sequencing (FISSEQ) of RNA for gene expression profiling in intact cells and tissues. Nat. Protoc. 10 , 442–458 (2015).
Ellis, M. J. et al. Connecting genomic alterations to cancer biology with proteomics: the NCI Clinical Proteomic Tumor Analysis Consortium. Cancer Discov. 3 , 1108–1112 (2013).
Li, J. et al. TCPA: a resource for cancer functional proteomics data. Nat. Methods 10 , 1046–1047 (2013).
Stoeckius, M. et al. Simultaneous epitope and transcriptome measurement in single cells. Nat. Methods 14 , 865–868 (2017).
Bendall, S. C. et al. Single-cell mass cytometry of differential immune and drug responses across a human hematopoietic continuum. Science 332 , 687–696 (2011).
Jackson, H. W. et al. The single-cell pathology landscape of breast cancer. Nature 578 , 615–620 (2020).
Keren, L. et al. A structured tumor-immune microenvironment in triple negative breast cancer revealed by multiplexed ion beam imaging. Cell 174 , 1373–1387.e19 (2018).
Schürch, C. M. et al. Coordinated cellular neighborhoods orchestrate antitumoral immunity at the colorectal cancer invasive front. Cell 183 , 838 (2020).
Beckonert, O. et al. Metabolic profiling, metabolomic and metabonomic procedures for NMR spectroscopy of urine, plasma, serum and tissue extracts. Nat. Protoc. 2 , 2692–2703 (2007).
Jang, C., Chen, L. & Rabinowitz, J. D. Metabolomics and isotope tracing. Cell 173 , 822–837 (2018).
Ghandi, M. et al. Next-generation characterization of the Cancer Cell Line Encyclopedia. Nature 569 , 503–508 (2019).
Uhlén, M. et al. Tissue-based map of the human proteome. Science 347 , 1260419 (2015).
Fedorov, A. et al. NCI Imaging Data Commons. Cancer Res 81 , 4188–4193 (2021).
Cerami, E. et al. The cBio cancer genomics portal: an open platform for exploring multidimensional cancer genomics data. Cancer Discov. 2 , 401–404 (2012).
Goldman, M. J. et al. Visualizing and interpreting cancer genomics data via the Xena platform. Nat. Biotechnol. 38 , 675–678 (2020).
Jiang, P., Freedman, M. L., Liu, J. S. & Liu, X. S. Inference of transcriptional regulation in cancers. Proc. Natl Acad. Sci. USA 112 , 7731–7736 (2015).
Sun, D. et al. TISCH: a comprehensive web resource enabling interactive single-cell transcriptome visualization of tumor microenvironment. Nucleic Acids Res. 49 , D1420–D1430 (2021).
Kristiansen, G. Markers of clinical utility in the differential diagnosis and prognosis of prostate cancer. Mod. Pathol. 31 , S143–S155 (2018).
Download references
Acknowledgements
The authors are supported by the intramural research budget of the US National Cancer Institute.
Author information
Authors and affiliations.
Cancer Data Science Laboratory, Center for Cancer Research, National Cancer Institute, National Institutes of Health, Bethesda, MD, USA
Peng Jiang, Sanju Sinha, Sridhar Hannenhalli, Cenk Sahinalp & Eytan Ruppin
Laboratory of Pathology, Center for Cancer Research, National Cancer Institute, National Institutes of Health, Bethesda, MD, USA
Kenneth Aldape
You can also search for this author in PubMed Google Scholar
Contributions
P.J. and E.R. designed the scope and structure of the Review, assembled write-up components and finalized the manuscript. C.S. wrote the text on tumour evolution and heterogeneity. S.H. wrote the text on transcriptional dysregulation. P.J. wrote the sections related to spatial genomics and artificial intelligence. P.J., E.R. and K.A. wrote the section on cancer diagnosis and treatment decisions. S.S. and P.J. prepared Tables 1 – 4 .
Corresponding authors
Correspondence to Peng Jiang or Eytan Ruppin .
Ethics declarations
Competing interests.
The authors declare no competing interests.
Peer review
Peer review information.
Nature Reviews Cancer thanks Itai Yanai, Anjali Rao and the other, anonymous, reviewers for their contribution to the peer review of this work.
Additional information
Publisher’s note.
Springer Nature remains neutral with regard to jurisdictional claims in published maps and institutional affiliations.
Related links
Array Express: https://www.ebi.ac.uk/arrayexpress/
CAMELYON: https://camelyon17.grand-challenge.org/
cBioportal: https://www.cbioportal.org/
CCLE: https://depmap.org/portal/ccle/
CPTAC: https://proteomics.cancer.gov/data-portal
CytoSig: https://cytosig.ccr.cancer.gov/
DepMap: https://depmap.org/portal
DNA sequencing costs: https://www.genome.gov/about-genomics/fact-sheets/DNA-Sequencing-Costs-Data
DrugCombDB: http://drugcombdb.denglab.org/
FDC: https://curate.ccr.cancer.gov/
GDC: https://gdc.cancer.gov/
GENIE: https://www.aacr.org/professionals/research/aacr-project-genie
GEO: https://www.ncbi.nlm.nih.gov/geo
Human Protein Atlas: https://www.proteinatlas.org/humanproteome/pathology
ICGC: https://dcc.icgc.org/
IDC: https://datacommons.cancer.gov/repository/imaging-data-commons
LINCS: https://clue.io/
PCAWG: https://dcc.icgc.org/pcawg
PRECOG: https://precog.stanford.edu/
RABIT: http://rabit.dfci.harvard.edu/
TARGET: https://ocg.cancer.gov/programs/target/data-matrix
TCIA: https://www.cancerimagingarchive.net/
TCGA: https://gdc.cancer.gov/
TIDE: http://tide.dfci.harvard.edu/
TISCH: http://tisch.comp-genomics.org/
Tres: https://resilience.ccr.cancer.gov/
UCSC Xena: https://xena.ucsc.edu/
A machine learning method that classifies new data using only a few training samples by transferring knowledge from large, related datasets.
A map of important image locations that support machine learning outputs.
A coarse-resolution map of important image regions for predicting a specific class using activations and gradients in the final convolutional layer.
Rights and permissions
Springer Nature or its licensor holds exclusive rights to this article under a publishing agreement with the author(s) or other rightsholder(s); author self-archiving of the accepted manuscript version of this article is solely governed by the terms of such publishing agreement and applicable law.
Reprints and permissions
About this article
Cite this article.
Jiang, P., Sinha, S., Aldape, K. et al. Big data in basic and translational cancer research. Nat Rev Cancer 22 , 625–639 (2022). https://doi.org/10.1038/s41568-022-00502-0
Download citation
Accepted : 26 July 2022
Published : 05 September 2022
Issue Date : November 2022
DOI : https://doi.org/10.1038/s41568-022-00502-0
Share this article
Anyone you share the following link with will be able to read this content:
Sorry, a shareable link is not currently available for this article.
Provided by the Springer Nature SharedIt content-sharing initiative
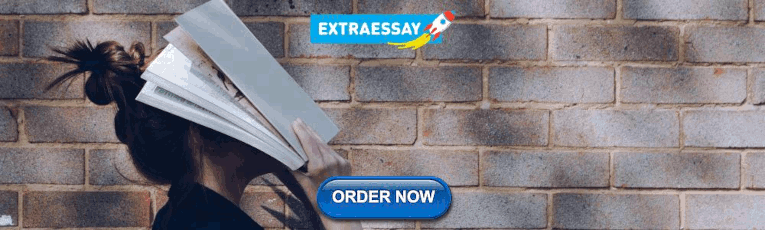
This article is cited by
Integration of pan-omics technologies and three-dimensional in vitro tumor models: an approach toward drug discovery and precision medicine.
- Pallavi Kulkarni
- Mahadev Rao
Molecular Cancer (2024)
DNMT3L inhibits hepatocellular carcinoma progression through DNA methylation of CDO1: insights from big data to basic research
- Xiaokai Yan
Journal of Translational Medicine (2024)
TIMM17A overexpression in lung adenocarcinoma and its association with prognosis
Scientific Reports (2024)
Roadmap for a European cancer data management and precision medicine infrastructure
- Macha Nikolski
- Eivind Hovig
- Gary Saunders
Nature Cancer (2024)
Multi-omics pan-cancer analyses identify MCM4 as a promising prognostic and diagnostic biomarker
Quick links.
- Explore articles by subject
- Guide to authors
- Editorial policies
Sign up for the Nature Briefing: Cancer newsletter — what matters in cancer research, free to your inbox weekly.

- Survey Paper
- Open access
- Published: 18 December 2021
A new theoretical understanding of big data analytics capabilities in organizations: a thematic analysis
- Renu Sabharwal 1 &
- Shah Jahan Miah ORCID: orcid.org/0000-0002-3783-8769 1
Journal of Big Data volume 8 , Article number: 159 ( 2021 ) Cite this article
17k Accesses
14 Citations
Metrics details
Big Data Analytics (BDA) usage in the industry has been increased markedly in recent years. As a data-driven tool to facilitate informed decision-making, the need for BDA capability in organizations is recognized, but few studies have communicated an understanding of BDA capabilities in a way that can enhance our theoretical knowledge of using BDA in the organizational domain. Big Data has been defined in various ways and, the past literature about the classification of BDA and its capabilities is explored in this research. We conducted a literature review using PRISMA methodology and integrated a thematic analysis using NVIVO12. By adopting five steps of the PRISMA framework—70 sample articles, we generate five themes, which are informed through organization development theory, and develop a novel empirical research model, which we submit for validity assessment. Our findings improve effectiveness and enhance the usage of BDA applications in various Organizations.
Introduction
Organizations today continuously harvest user data [e.g., data collections] to improve their business efficiencies and practices. Significant volumes of stored data or data regarding electronic transactions are used in support of decision making, with managers, policymakers, and executive officers now routinely embracing technology to transform these abundant raw data into useful, informative information. Data analysis is complex, but one data-handling method, “Big Data Analytics” (BDA)—the application of advanced analytic techniques, including data mining, statistical analysis, and predictive modeling on big datasets as new business intelligence practice [ 1 ]—is widely applied. BDA uses computational intelligence techniques to transform raw data into information that can be used to support decision-making.
Because decision-making in organizations has become increasingly reliant on Big Data, analytical applications have increased in importance for evidence-based decision making [ 2 ]. The need for a systematic review of Big Data stream analysis using rigorous and methodical approaches to identify trends in Big Data stream tools, analyze techniques, technologies, and methods is becoming increasingly important [ 3 ]. Organizational factors such as organizational resources adjustment, environmental acceptance, and organizational management relate to implement its BDA capability and enhancing its benefits through BDA technologies [ 4 ]. It is evident from past literature that BDA supports the organizational decision-making process by developing suitable theoretical understanding, but extending existing theories remains a significant challenge. The improved capability of BDA will ensure that the organizational products and services are continuously optimized to meet the evolving needs of consumers.
Previous systematic reviews have focused on future BDA adoption challenges [ 5 , 6 , 7 ] or technical innovation aspects of Big Data analytics [ 8 , 9 ]. This signifies those numerous studies have examined Big Data issues in different domains. These different domains are included: quality of Big Data in financial service organization [ 10 ]; organizational value creation because of BDA usage [ 11 ]; application of Big Data in health organizations [ 9 ]; decision improvement using Big Data in health [ 12 ]; application of Big Data in transport organizations [ 13 ]; relationships between Big Data in financial domains [ 14 ]; and quality of Big Data and its impact on government organizations [ 15 ].
While there has been a progressive increase in research on BDA, its capabilities and how organizations may exploit them are less well studied [ 16 ]. We apply a PRISMA framework [ 17 ]) and qualitative thematic analysis to create the model to define the relationship between BDAC and OD. The proposed research presents an overview of BDA capabilities and how they can be utilized by organizations. The implications of this research for future research development. Specifically, we (1) provide an observation into key themes regarding BDAC concerning state-of-the-art research in BDA, and (2) show an alignment to organizational development theory in terms of a new empirical research model which will be submitted for validity assessment for future research of BDAC in organizations.
According to [ 20 ], a systematic literature review first involves describing the key approach and establishing definitions for key concepts. We use a six-phase process to identify, analyze, and sequentially report themes using NVIVO 12.
Study background
Many forms of BDA exist to meet specific decision-support demands of different organizations. Three BDA analytical classes exist: (1) descriptive , dealing with straightforward questions regarding what is or has happened and why—with ‘opportunities and problems’ using descriptive statistics such as historical insights; (2) predictive , dealing with questions such as what will or is likely to happen, by exploring data patterns with relatively complex statistics, simulation, and machine-learning algorithms (e.g., to identify trends in sales activities, or forecast customer behavior and purchasing patterns); and (3) prescriptive , dealing with questions regarding what should be happening and how to influence it, using complex descriptive and predictive analytics with mathematical optimization, simulation, and machine-learning algorithms (e.g., many large-scale companies have adopted prescriptive analytics to optimize production or solve schedule and inventory management issues) [ 18 ]. Regardless of the type of BDA analysis performed, its application significantly impacts tangible and intangible resources within an organization.
Previous studies on BDA
BDA tools or techniques are used to analyze Big Data (such as social media or substantial transactional data) to support strategic decision-making [ 19 ] in different domains (e.g., tourism, supply chain, healthcare), and numerous studies have developed and evaluated BDA solutions to improve organizational decision support. We categorize previous studies into two main groups based on non-technical aspects: those which relate to the development of new BDA requirements and functionalities in a specific problem domain and those which focus on more intrinsic aspects such as BDAC development or value-adding because of their impact on particular aspects of the business. Examples of reviews focusing on technical or problem-solving aspects are detailed in Table 1 .
The second literature group examines BDA in an organizational context, such as improving firm performance using Big Data analytics in specific business domains [ 26 ]. Studies that support BDA lead to different aspects of organizational performance [ 20 , 24 , 25 , 27 , 28 , 29 ] (Table 2 ). Another research on BDA to improve data utilization and decision-support qualities. For example, [ 30 ] explained how BDAC might be developed to improve managerial decision-making processes, and [ 4 ] conducted a thematic analysis of 15 firms to identify the factors related to the success of BDA capability development in SCM.
Potential applications of BDA
Many retail organizations use analytical approaches to gain commercial advantage and organizational success [ 31 ]. Modern organizations increasingly invest in BDA projects to reduce costs, make accurate decision making, and future business planning. For example, Amazon was the first online retailer and maintained its innovative BDA improvement and use [ 31 ]. Examples of successful stories of BDA use in business sectors include.
Retail: business organizations using BDA for dynamic (surge) pricing [ 32 ] to adjust product or service prices based on demand and supply. For instance, Amazon uses dynamic pricing to surge prices by product demand.
Hospitality: Marriott hotels—the largest hospitality agent with a rapidly increasing number of hotels and serviced customers—uses BDA to improve sales [ 33 ].
Entertainment: Netflix uses BDA to retain clientele and increase sales and profits [ 34 , 35 ].
Transportation : Uber uses BDA [ 36 ] to capture Big Data from various consumers and identify the best routes to locations. ‘Uber eats,’ despite competing with other delivery companies, delivers foods in the shortest possible time.
Foodservice: McDonald's continuously updates information with BDA, following a recent shift in food quality, now sells healthy food to consumers [ 37 ], and has adopted a dynamic menu [ 38 ].
Finance: American Express has used BDA for a long time and was one of the first companies to understand the benefits of using BDA to improve business performance [ 39 ]. Big Data is collected on the ways consumers make on- and offline purchases, and predictions are made as to how they will shop in the future.
Manufacturing: General Electric manufactures and distributes products such as wind turbines, locomotives, airplane engines, and ship engines [ 40 ]. By dealing with a huge amount of data from electricity networks, meteorological information systems, geographical information systems, benefits can be brought to the existing power system, including improving customer service and social welfare in the era of big data.
Online business: music streaming websites are increasingly popular and continue to grow in size and scope because consumers want a customized streaming service [ 41 ]. Many streaming services (e.g., Apple Music, Spotify, Google Music) use various BDA applications to suggest new songs to consumers.
Organization value assessment with BDA
Specific performance measures must be established that rely on the number of organizational contextual factors such as the organization's goal, the external environment of the organization, and the organization itself. When looking at the above contexts regarding the use of BDA to strengthen process innovation skills, it is important to note that the approach required to achieve positive results depends on the different combinations along with the area in which BDA deployed [ 42 ].
Organizational development and BDA
To assist organization decision-making for growth, effective processes are required to perform operations such as continuous diagnosis, action planning, and the implementation and evaluation of BDA. Lewin’s Organizational Development (OD) theory regards processes as having a goal to transfer knowledge and skills to an organization, with the process being mainly to improve problem-solving capacity and to manage future change. Beckhard [ 43 ] defined OD as the internal dynamics of an organization, which involve a collection of individuals working as a group to improve organizational effectiveness, capability, work performance, and the ability to adjust culture, policies, practices, and procedure requirements.
OD is ‘a system-wide application and transfer of behavioral science knowledge to the planned development, improvement, and reinforcement of the strategies, structures, and processes that lead to organization effectiveness’ [ 44 ], and has three concepts: organizational climate, culture, and capability [ 45 ]. Organizational climate is ‘the mood or unique personality of an organization’ [ 45 ] which includes shared perceptions of policies, practices, and procedures; climate features also consist of leadership, communication, participative management, and role clarity. Organizational culture involves shared basic assumptions, values, norms, behavioral patterns, and artifacts, defined by [ 46 ] as a pattern of shared basic assumptions that a group learned by solving problems of external adaptation and internal integration (p. 38). Organizational capacity (OC) implies the organization's function, such as the production of services or products or maintenance of organizational operations, and has four components: resource acquisition, organization structure, production subsystem, and accomplishment [ 47 ]. Organizational culture and climate affect an organization’s capacity to operate adequately (Fig. 1 ).
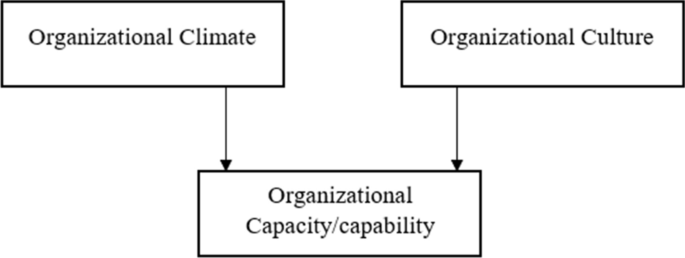
Framework of modified organizational development theory [ 45 ]
Research methodology
Our systematic literature review presents a research process for analyzing and examining research and gathering and evaluating it [ 48 ] In accordance with a PRISMA framework [ 49 ]. We use keywords to search for articles related to the BDA application, following a five-stage process.
Stage1: design development
We establish a research question to instruct the selection and search strategy and analysis and synthesis process, defining the aim, scope, and specific research goals following guidelines, procedures, and policies of the Cochrane Handbook for Systematic Reviews of Intervention [ 50 ]. The design review process is directed by the research question: what are the consistent definitions of BDA, unique attributes, objections, and business revolution, including improving the decision-making process and organization performance with BDA? The below table is created using the outcome of the search performed using Keywords- Organizational BDAC, Big Data, BDA (Table 3 ).
Stage 2: inclusion and elimination criteria
To maintain the nuances of a systematic review, we apply various inclusion and exclusion criteria to our search for research articles in four databases: Science Direct, Web of Science, IEEE (Institute of Electrical and Electronics Engineers), and Springer Link. Inclusion criteria include topics on ‘Big Data in Organization’ published between 2015 to 2021, in English. We use essential keywords to identify the most relevant articles, using truncation, wildcarding, and appropriate Boolean operators (Table 4 ).
Stage 3: literature sources and search approach
Research articles are excluded based on keywords and abstracts, after which 8062 are retained (Table 5 ). The articles only selected keywords such as Big Data, BDA, BDAC, and the Abstract only focused on the Organizational domain.
Stage 4: assess the quality of full papers
At this stage, for each of the 161 research articles that remained after stage 3 presented in Table 6 , which was assessed independently by authors in terms of several quality criteria such as credibility, to assess whether the articles were well presented, relevance which was assessed based on whether the articles were used in the organizational domain.
Stage 5: literature extraction and synthesis process
At this stage, only journal articles and conference papers are selected. Articles for which full texts were not open access were excluded, reducing our references to 70 papers Footnote 1 (Table 7 ).
Meta-analysis of selected papers
Of the 70 papers satisfying our selection criteria, publication year and type (journal or conference paper) reveal an increasing trend in big data analytics over the last 6 years (Table 6 ). Additionally, journals produced more BDA papers than Conference proceedings (Fig. 2 ), which may be affected during 2020–2021 because of COVID, and fewer conference proceedings or publications were canceled.
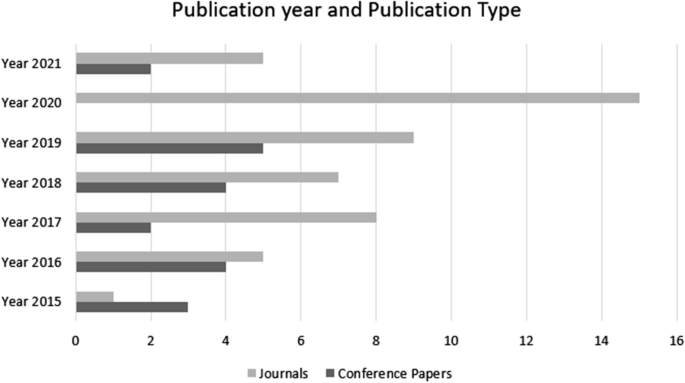
Distribution of publications by year and publication type
Of the 70 research articles, 6% were published in 2015, 13% (2016), 14% (2017), 16% (2018), 20% (2019), 21% (2020), and 10% (untill May 2021).
Thematic analysis is used to find the results which can identify, analyze and report patterns (themes) within data, and produce an insightful analysis to answer particular research questions [ 51 ].
The combination of NVIVO and Thematic analysis improves results. Judger [ 52 ] maintained that using computer-assisted data analysis coupled with manual checks improves findings' trustworthiness, credibility, and validity (p. 6).
Defining big data
Of 70 articles, 33 provide a clear replicable definition of Big Data, from which the five representative definitions are presented in Table 8 .
Defining BDA
Of 70 sample articles, 21 clearly define BDA. The four representative definitions are presented in Table 9 . Some definitions accentuate the tools and processes used to derive new insights from big data.
Defining Big Data analytics capability
Only 16% of articles focus on Big Data characteristics; one identifies challenges and issues with adopting and implementing the acquisition of Big Data in organizations [ 42 ]. The above study resulted that BDAC using the large volumes of data generated through different devices and people to increase efficiency and generate more profits. BDA capability and its potential value could be more than a business expects, which has been presented that the professional services, manufacturing, and retail have structural barriers and overcome these barriers with the use of Big Data [ 60 ]. We define BDAC as the combined ability to store, process, and analyze large amounts of data to provide meaningful information to users. Four dimensions of BDAC exist data integration, analytical, predictive, and data interpretation (Table 10 ).
It is feasible to identify outstanding issues of research that are of excessive relevance, which has termed in five themes using NVIVO12 (Fig. 3 ). Table 11 illustrates four units that combine NVIVO with thematic analysis for analysis: Big data, BDA, BDAC, and BDA themes. We manually classify five BDA themes to ensure accuracy with appropriate perception in detail and provide suggestions on how future researchers might approach these problems using a research model.
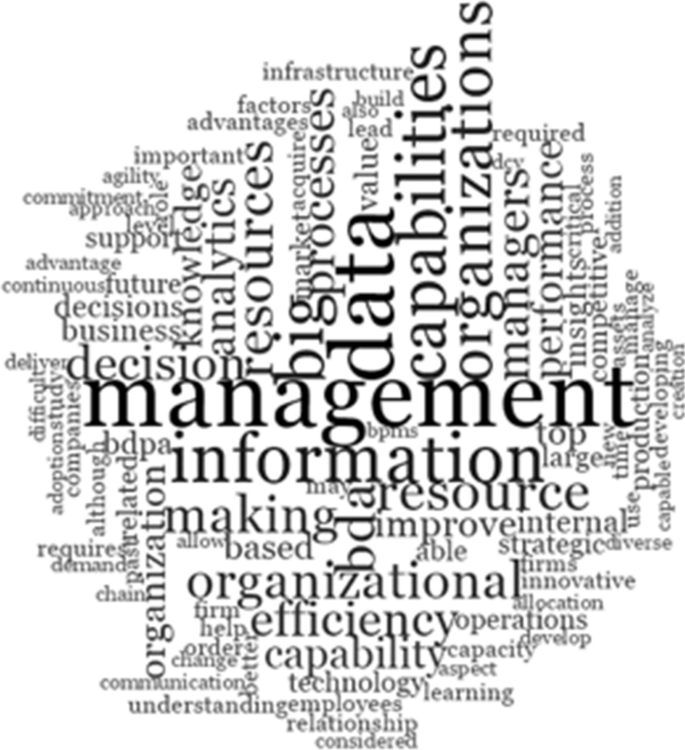
Thematic analysis using NVIVO 12
Manyika et al . [ 63 ] considered that BDA could assist an organization to improve its decision making, minimize risks, provide other valuable insights that would otherwise remain hidden, aid the creation of innovative business models, and improve performance.
The five themes presented in Table 11 identify limitations of existing literature, which are examined in our research model (Fig. 4 ) using four hypotheses. This theoretical model identifies organizational and individual levels as being influenced by organization climate, culture, and capacity. This model can assist in understanding how BDA can be used to improve organizational and individual performance.
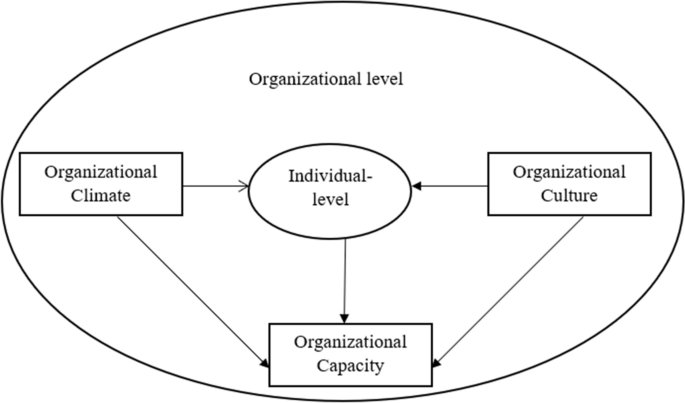
The framework of organizational development theory [ 64 ]
The Research model development process
We analyze literature using a new research method, driven by the connection between BDAC and resource-based views, which included three resources: tangible (financial and physical), human skills (employees’ knowledge and skills), and intangible (organizational culture and organizational learning) used in IS capacity literature [ 65 , 66 , 67 , 68 ]. Seven factors enable firms to create BDAC [ 16 ] (Fig. 5 ).
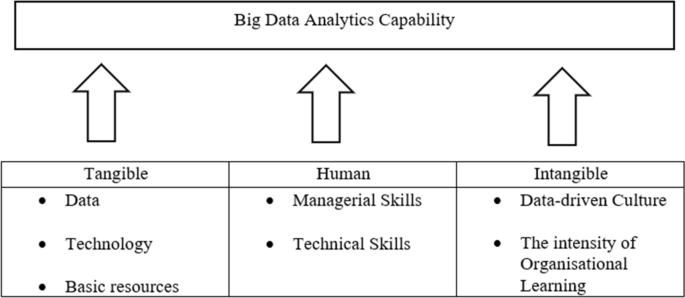
Classification of Big Data resources (adapted from [ 16 ])
To develop a robust model, tangible, intangible, and human resource types should be implemented in an organization and contribute to the emergence of the decision-making process. This research model recognizes BDAC to enhance OD, strengthening organizational strategies and the relationship between BD resources and OD. Figure 6 depicts a theoretical framework illustrating how BDA resources influence innovation sustainability and OD, where Innovation sustainability helps identify market opportunities, predict customer needs, and analyze customer purchase decisions [ 69 ].
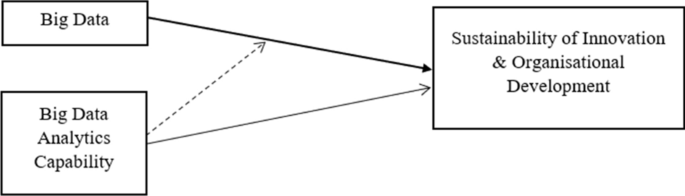
Theroretical framework illustrating how BDA resources influence innovation sustainability and organizational development (adapted from [ 68 ])
Miller [ 70 ] considered data a strategic business asset and recommended that businesses and academics collaborate to improve knowledge regarding BD skills and capability across an organization; [ 70 ] concluded that every profession, whether business or technology, will be impacted by big data and analytics. Gobble [ 71 ] proposed that an organization should develop new technologies to provide necessary supplements to enhance growth. Big Data represents a revolution in science and technology, and a data-rich smart city is the expected future that can be developed using Big Data [ 72 ]. Galbraith [ 73 ] reported how an organization attempting to develop BDAC might experience obstacles and opportunities. We found no literature that combined Big Data analytics capability and Organizational Development or discussed interaction between them.
Because little empirical evidence exists regarding the connection between OD and BDA or their characteristics and features, our model (Fig. 7 ) fills an important void, directly connecting BDAC and OD, and illustrates how it affects OD in the organizational concepts of capacity, culture, and climate, and their future resources. Because BDAC can assist OD through the implementation of new technologies [ 15 , 26 , 57 ], we hypothesize:
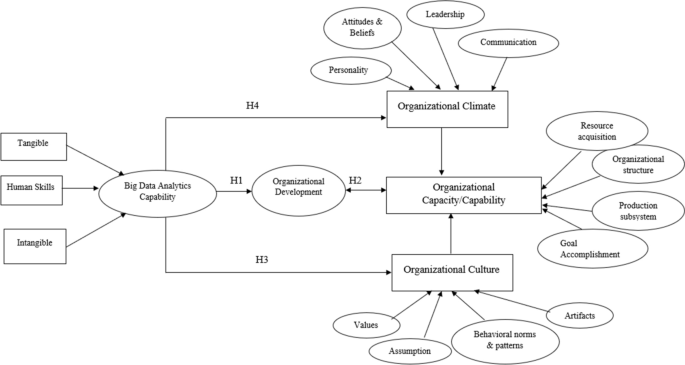
Proposed interpretation in the research model
H1: A positive relationship exists between Organizational Development and BDAC.
OC relies heavily on OD, with OC representing a resource requiring development in an organization. Because OD can improve OC [ 44 , 45 ], we hypothesize that:
H2: A positive relationship exists between Organizational Development and Organizational Capability.
With the implementation or adoption of BDAC, OC is impacted [ 46 ]. Big data enables an organization to improve inefficient practices, whether in marketing, retail, or media. We hypothesize that:
H3: A positive relationship exists between BDAC and Organizational Culture.
Because BDAC adoption can affect OC, the policies, practices, and measures associated with an organization's employee experience [ 74 ], and improve both the business climate and an individual’s performance, we hypothesize that:
H4: A positive relationship exists between BDAC and Organizational Climate.
Our research is based on a need to develop a framework model in relation to OD theory because modern organizations cannot ignore BDA or its future learning and association with theoretical understanding. Therefore, we aim to demonstrate current trends in capabilities and a framework to improve understanding of BDAC for future research.
Despite the hype that encompasses Big Data, the organizational development and structure through which it results in competitive gains have remained generally underexplored in empirical studies. It is feasible to distinguish the five prominent, highly relevant themes discussed in an earlier section by orchestrating a systematic literature review and recording what is known to date. By conducting those five thematic areas of the research, as depicted in the research model in Fig. 7 , provide relation how they are impacting each other’s performance and give some ideas on how researchers could approach these problems.
The number of published papers on Big Data is increasing. Between 2015 and May 2021, the highest proportion of journal articles for any given year (21%) occurred until May 2021 with the inclusion or exclusion criteria such as the article selection only opted using four databases: Science Direct, Web of Science, IEEE (Institute of Electrical and Electronics Engineers), and Springer Link and included only those articles which titled as 'Big Data in Organization' published, in the English language. We use essential keywords to identify the most relevant articles, using truncation, wildcarding, and appropriate Boolean operators. While BDAC can improve business-related outcomes, including more effective marketing, new revenue opportunities, customer personalization, and improved operational efficiency, existing literature has focused on only one or two aspects of BDAC. Our research model (Fig. 7 ) represents the relationship between BDAC and OD to better understand their impacts on OC. We explain that the proposed model education will enhance knowledge of BDAC and that it may better meet organizational requirements, ensuring improved products and services to optimize consumer outcomes.
Considerable research has been conducted in many different contexts such as the health sector, education about Big Data, but according to past literature, BDAC in an organization is still an open issue, how to utilize BDAC within the organization for development purposes. The full potential of BDA and what it can offer must be leveraged to gain a commercial advantage. Therefore, we focus on summarizing by creating the themes using past relevant literature and propose a research model based on literature [ 61 ] for business.
While we explored Springer Link, IEEE, Science Direct, and Web of Science (which index high-impact journal and conference papers), the possibility exists that some relevant journals were missed. Our research is constrained by our selection criteria, including year, language (English), and peer-reviewed journal articles (we omitted reports, grey journals, and web articles).
A steadily expanding number of organizations has been endeavored to utilize Big Data and organizational analytics to analyze available data and assist with decision-making. For these organizations, influence the full potential that Big Data and organizational analytics can present to acquire competitive advantage. In any case, since Big Data and organizational analytics are generally considered as new innovative in business worldview, there is a little exploration on how to handle them and leverage them adequately. While past literature has shown the advantages of utilizing Big Data in various settings, there is an absence of theoretically determined research on the most proficient method to use these solutions to acquire competitive advantage. This research recognizes the need to explore BDA through a comprehensive approach. Therefore, we focus on summarizing with the proposed development related to BDA themes on which we still have a restricted observational arrangement.
To this end, this research proposes a new research model that relates earlier studies regarding BDAC in organizational culture. The research model provides a reference to the more extensive implementation of Big Data technologies in an organizational context. While the hypothesis present in the research model is on a significant level and can be deciphered as addition to theoretical lens, they are depicted in such a way that they can be adapted for organizational development. This research poses an original point of view on Big Data literature since, by far majority focuses on tools, infrastructure, technical aspects, and network analytics. The proposed framework contributes to Big Data and its capability in organizational development by covering the gap which has not addressed in past literature. This research model also can be viewed as a value-adding knowledge for managers and executives to learn how to drive channels of creating benefit in their organization through the use of Big Data, BDA, and BDAC.
We identify five themes to leverage BDA in an organization and gain a competitive advantage. We present a research model and four hypotheses to bridge gaps in research between BDA and OD. The purpose of this model and these hypotheses is to guide research to improve our understanding of how BDA implementation can affect an organization. The model goes for the next phase of our study, in which we will test the model for its validity.
Availability of data and materials
Data will be supplied upon request.
Appendix A is submitted as a supplementary file for review.
Abbreviations
The Institute of Electrical and Electronics Engineers
- Big Data Analytics
Big Data Analytics Capabilities
Organizational Development
- Organizational Capacity
Russom P. Big data analytics. TDWI Best Practices Report, Fourth Quarter. 2011;19(4):1–34.
Google Scholar
Mikalef P, Boura M, Lekakos G, Krogstie J. Big data analytics and firm performance: findings from a mixed-method approach. J Bus Res. 2019;98:261–76.
Kojo T, Daramola O, Adebiyi A. Big data stream analysis: a systematic literature review. J Big Data. 2019;6(1):1–30.
Jha AK, Agi MA, Ngai EW. A note on big data analytics capability development in supply chain. Decis Support Syst. 2020;138:113382.
Posavec AB, Krajnović S. Challenges in adopting big data strategies and plans in organizations. In: 2016 39th international convention on information and communication technology, electronics and microelectronics (MIPRO). IEEE. 2016. p. 1229–34.
Madhlangobe W, Wang L. Assessment of factors influencing intent-to-use Big Data Analytics in an organization: pilot study. In: 2018 IEEE 20th International Conference on High-Performance Computing and Communications; IEEE 16th International Conference on Smart City; IEEE 4th International Conference on Data Science and Systems (HPCC/SmartCity/DSS). IEEE. 2018. p. 1710–1715.
Saetang W, Tangwannawit S, Jensuttiwetchakul T. The effect of technology-organization-environment on adoption decision of big data technology in Thailand. Int J Electr Comput. 2020;10(6):6412. https://doi.org/10.11591/ijece.v10i6.pp6412-6422 .
Article Google Scholar
Pei L. Application of Big Data technology in construction organization and management of engineering projects. J Phys Conf Ser. 2020. https://doi.org/10.1088/1742-6596/1616/1/012002 .
Marashi PS, Hamidi H. Business challenges of Big Data application in health organization. In: Khajeheian D, Friedrichsen M, Mödinger W, editors. Competitiveness in Emerging Markets. Springer, Cham; 2018. p. 569–584. doi: https://doi.org/10.1007/978-3-319-71722-7_28 .
Haryadi AF, Hulstijn J, Wahyudi A, Van Der Voort H, Janssen M. Antecedents of big data quality: an empirical examination in financial service organizations. In 2016 IEEE International Conference on Big Data (Big Data). IEEE. 2016. p. 116–121.
George JP, Chandra KS. Asset productivity in organisations at the intersection of Big Data Analytics and supply chain management. In: Chen JZ, Tavares J, Shakya S, Iliyasu A, editors. Image Processing and Capsule Networks. ICIPCN 2020. Advances in Intelligent Systems and Computing, vol 1200. Springer, Cham; 2020. p. 319–330.
Sousa MJ, Pesqueira AM, Lemos C, Sousa M, Rocha Á. Decision-making based on big data analytics for people management in healthcare organizations. J Med Syst. 2019;43(9):1–10.
Du G, Zhang X, Ni S. Discussion on the application of big data in rail transit organization. In: Wu TY, Ni S, Chu SC, Chen CH, Favorskaya M, editors. International conference on smart vehicular technology, transportation, communication and applications. Springer: Cham; 2018. p. 312–8.
Wahyudi A, Farhani A, Janssen M. Relating big data and data quality in financial service organizations. In: Al-Sharhan SA, Simintiras AC, Dwivedi YK, Janssen M, Mäntymäki M, Tahat L, Moughrabi I, Ali TM, Rana NP, editors. Conference on e-Business, e-Services and e-Society. Springer: Cham; 2018. p. 504–19.
Alkatheeri Y, Ameen A, Isaac O, Nusari M, Duraisamy B, Khalifa GS. The effect of big data on the quality of decision-making in Abu Dhabi Government organisations. In: Sharma N, Chakrabati A, Balas VE, editors. Data management, analytics and innovation. Springer: Singapore; 2020. p. 231–48.
Gupta M, George JF. Toward the development of a big data analytics capability. Inf Manag. 2016;53(8):1049–64.
Selçuk AA. A guide for systematic reviews: PRISMA. Turk Arch Otorhinolaryngol. 2019;57(1):57.
Tiwari S, Wee HM, Daryanto Y. Big data analytics in supply chain management between 2010 and 2016: insights to industries. Comput Ind Eng. 2018;115:319–30.
Miah SJ, Camilleri E, Vu HQ. Big Data in healthcare research: a survey study. J Comput Inform Syst. 2021;7:1–3.
Mikalef P, Pappas IO, Krogstie J, Giannakos M. Big data analytics capabilities: a systematic literature review and research agenda. Inf Syst e-Business Manage. 2018;16(3):547–78.
Nguyen T, Li ZHOU, Spiegler V, Ieromonachou P, Lin Y. Big data analytics in supply chain management: a state-of-the-art literature review. Comput Oper Res. 2018;98:254–64.
MathSciNet MATH Google Scholar
Günther WA, Mehrizi MHR, Huysman M, Feldberg F. Debating big data: a literature review on realizing value from big data. J Strateg Inf. 2017;26(3):191–209.
Rialti R, Marzi G, Ciappei C, Busso D. Big data and dynamic capabilities: a bibliometric analysis and systematic literature review. Manag Decis. 2019;57(8):2052–68.
Wamba SF, Gunasekaran A, Akter S, Ren SJ, Dubey R, Childe SJ. Big data analytics and firm performance: effects of dynamic capabilities. J Bus Res. 2017;70:356–65.
Wang Y, Hajli N. Exploring the path to big data analytics success in healthcare. J Bus Res. 2017;70:287–99.
Akter S, Wamba SF, Gunasekaran A, Dubey R, Childe SJ. How to improve firm performance using big data analytics capability and business strategy alignment? Int J Prod Econ. 2016;182:113–31.
Kwon O, Lee N, Shin B. Data quality management, data usage experience and acquisition intention of big data analytics. Int J Inf Manage. 2014;34(3):387–94.
Chen DQ, Preston DS, Swink M. How the use of big data analytics affects value creation in supply chain management. J Manag Info Syst. 2015;32(4):4–39.
Kim MK, Park JH. Identifying and prioritizing critical factors for promoting the implementation and usage of big data in healthcare. Inf Dev. 2017;33(3):257–69.
Popovič A, Hackney R, Tassabehji R, Castelli M. The impact of big data analytics on firms’ high value business performance. Inf Syst Front. 2018;20:209–22.
Hewage TN, Halgamuge MN, Syed A, Ekici G. Big data techniques of Google, Amazon, Facebook and Twitter. J Commun. 2018;13(2):94–100.
BenMark G, Klapdor S, Kullmann M, Sundararajan R. How retailers can drive profitable growth through dynamic pricing. McKinsey & Company. 2017. https://www.mckinsey.com/industries/retail/our-insights/howretailers-can-drive-profitable-growth-throughdynamic-pricing . Accessed 13 Mar 2021.
Richard B. Hotel chains: survival strategies for a dynamic future. J Tour Futures. 2017;3(1):56–65.
Fouladirad M, Neal J, Ituarte JV, Alexander J, Ghareeb A. Entertaining data: business analytics and Netflix. Int J Data Anal Inf Syst. 2018;10(1):13–22.
Hadida AL, Lampel J, Walls WD, Joshi A. Hollywood studio filmmaking in the age of Netflix: a tale of two institutional logics. J Cult Econ. 2020;45:1–26.
Harinen T, Li B. Using causal inference to improve the Uber user experience. Uber Engineering. 2019. https://eng.uber.com/causal-inference-at-uber/ . Accessed 10 Mar 2021.
Anaf J, Baum FE, Fisher M, Harris E, Friel S. Assessing the health impact of transnational corporations: a case study on McDonald’s Australia. Glob Health. 2017;13(1):7.
Wired. McDonald's Bites on Big Data; 2019. https://www.wired.com/story/mcdonalds-big-data-dynamic-yield-acquisition
Bernard M. & Co. American Express: how Big Data and machine learning Benefits Consumers And Merchants, 2018. https://www.bernardmarr.com/default.asp?contentID=1263
Zhang Y, Huang T, Bompard EF. Big data analytics in smart grids: a review. Energy Informatics. 2018;1(1):8.
HBS. Next Big Sound—moneyball for music? Digital Initiative. 2020. https://digital.hbs.edu/platform-digit/submission/next-big-sound-moneyball-for-music/ . Accessed 10 Apr 2021.
Mneney J, Van Belle JP. Big data capabilities and readiness of South African retail organisations. In: 2016 6th International Conference-Cloud System and Big Data Engineering (Confluence). IEEE. 2016. p. 279–86.
Beckhard R. Organizational issues in the team delivery of comprehensive health care. Milbank Mem Fund. 1972;50:287–316.
Cummings TG, Worley CG. Organization development and change. 8th ed. Mason: Thompson South-Western; 2009.
Glanz K, Rimer BK, Viswanath K, editors. Health behavior and health education: theory, research, and practice. San Francisco: Wiley; 2008.
Schein EH. Organizational culture and leadership. San Francisco: Jossey-Bass; 1985.
Prestby J, Wandersman A. An empirical exploration of a framework of organizational viability: maintaining block organizations. J Appl Behav Sci. 1985;21(3):287–305.
Liberati A, Altman DG, Tetzlaff J, Mulrow C, Gøtzsche PC, Ioannidis JP, Moher D. The PRISMA statement for reporting systematic reviews and meta-analyses of studies that evaluate health care interventions: explanation and elaboration. J Clin Epidemiol. 2009;62(10):e1–34.
Page MJ, McKenzie JE, Bossuyt PM, Boutron I, Hoffmann TC, Mulrow CD, Moher D. The PRISMA 2020 statement: an updated guideline for reporting systematic reviews. BMJ. 2021;372:n71.
Higgins JP, Green S, Scholten RJPM. Maintaining reviews: updates, amendments and feedback. Cochrane handbook for systematic reviews of interventions. 31; 2008.
Braun V, Clarke V. Using thematic analysis in psychology. Qual Res Psychol. 2006;3(2):77–101.
Judger N. The thematic analysis of interview data: an approach used to examine the influence of the market on curricular provision in Mongolian higher education institutions. Hillary Place Papers, University of Leeds. 2016;3:1–7
Khine P, Shun W. Big data for organizations: a review. J Comput Commun. 2017;5:40–8.
Zan KK. Prospects for using Big Data to improve the effectiveness of an education organization. In: 2019 IEEE Conference of Russian Young Researchers in Electrical and Electronic Engineering (EIConRus) . IEEE. 2019. p. 1777–9.
Ekambaram A, Sørensen AØ, Bull-Berg H, Olsson NO. The role of big data and knowledge management in improving projects and project-based organizations. Procedia Comput Sci. 2018;138:851–8.
Rialti R, Marzi G, Silic M, Ciappei C. Ambidextrous organization and agility in big data era: the role of business process management systems. Bus Process Manag. 2018;24(5):1091–109.
Wang Y, Kung L, Gupta S, Ozdemir S. Leveraging big data analytics to improve quality of care in healthcare organizations: a configurational perspective. Br J Manag. 2019;30(2):362–88.
De Mauro A, Greco M, Grimaldi M, Ritala P. In (Big) Data we trust: value creation in knowledge organizations—introduction to the special issue. Inf Proc Manag. 2018;54(5):755–7.
Batistič S, Van Der Laken P. History, evolution and future of big data and analytics: a bibliometric analysis of its relationship to performance in organizations. Br J Manag. 2019;30(2):229–51.
Jokonya O. Towards a conceptual framework for big data adoption in organizations. In: 2015 International Conference on Cloud Computing and Big Data (CCBD). IEEE. 2015. p. 153–160.
Mikalef P, Krogstie J, Pappas IO, Pavlou P. Exploring the relationship between big data analytics capability and competitive performance: the mediating roles of dynamic and operational capabilities. Inf Manag. 2020;57(2):103169.
Shuradze G, Wagner HT. Towards a conceptualization of data analytics capabilities. In: 2016 49th Hawaii International Conference on System Sciences (HICSS). IEEE. 2016. p. 5052–64.
Manyika J, Chui M, Brown B, Bughin J, Dobbs R, Roxburgh C, Hung Byers A. Big data: the next frontier for innovation, competition, and productivity. McKinsey Global Institute. 2011. https://www.mckinsey.com/business-functions/mckinsey-digital/our-insights/big-data-the-next-frontier-for-innovation . Accessed XX(day) XXX (month) XXXX (year).
Wu YK, Chu NF. Introduction of the transtheoretical model and organisational development theory in weight management: a narrative review. Obes Res Clin Pract. 2015;9(3):203–13.
Grant RM. Contemporary strategy analysis: Text and cases edition. Wiley; 2010.
Bharadwaj AS. A resource-based perspective on information technology capability and firm performance: an empirical investigation. MIS Q. 2000;24(1):169–96.
Chae HC, Koh CH, Prybutok VR. Information technology capability and firm performance: contradictory findings and their possible causes. MIS Q. 2014;38:305–26.
Santhanam R, Hartono E. Issues in linking information technology capability to firm performance. MIS Q. 2003;27(1):125–53.
Hao S, Zhang H, Song M. Big data, big data analytics capability, and sustainable innovation performance. Sustainability. 2019;11:7145. https://doi.org/10.3390/su11247145 .
Miller S. Collaborative approaches needed to close the big data skills gap. J Organ Des. 2014;3(1):26–30.
Gobble MM. Outsourcing innovation. Res Technol Manag. 2013;56(4):64–7.
Ann Keller S, Koonin SE, Shipp S. Big data and city living–what can it do for us? Signif (Oxf). 2012;9(4):4–7.
Galbraith JR. Organizational design challenges resulting from big data. J Organ Des. 2014;3(1):2–13.
Schneider B, Ehrhart MG, Macey WH. Organizational climate and culture. Annu Rev Psychol. 2013;64:361–88.
Download references
Acknowledgements
Not applicable
Not applicable.
Author information
Authors and affiliations.
Newcastle Business School, University of Newcastle, Newcastle, NSW, Australia
Renu Sabharwal & Shah Jahan Miah
You can also search for this author in PubMed Google Scholar
Contributions
The first author conducted the research, while the second author has ensured quality standards and rewritten the entire findings linking to underlying theories. All authors read and approved the final manuscript.
Corresponding author
Correspondence to Shah Jahan Miah .
Ethics declarations
Ethics approval and consent to participate, consent for publication, competing interests.
The authors declare that they have no competing interests.
Additional information
Publisher's note.
Springer Nature remains neutral with regard to jurisdictional claims in published maps and institutional affiliations.
Rights and permissions
Open Access This article is licensed under a Creative Commons Attribution 4.0 International License, which permits use, sharing, adaptation, distribution and reproduction in any medium or format, as long as you give appropriate credit to the original author(s) and the source, provide a link to the Creative Commons licence, and indicate if changes were made. The images or other third party material in this article are included in the article's Creative Commons licence, unless indicated otherwise in a credit line to the material. If material is not included in the article's Creative Commons licence and your intended use is not permitted by statutory regulation or exceeds the permitted use, you will need to obtain permission directly from the copyright holder. To view a copy of this licence, visit http://creativecommons.org/licenses/by/4.0/ .
Reprints and permissions
About this article
Cite this article.
Sabharwal, R., Miah, S.J. A new theoretical understanding of big data analytics capabilities in organizations: a thematic analysis. J Big Data 8 , 159 (2021). https://doi.org/10.1186/s40537-021-00543-6
Download citation
Received : 17 August 2021
Accepted : 16 November 2021
Published : 18 December 2021
DOI : https://doi.org/10.1186/s40537-021-00543-6
Share this article
Anyone you share the following link with will be able to read this content:
Sorry, a shareable link is not currently available for this article.
Provided by the Springer Nature SharedIt content-sharing initiative
- Organization
- Systematic literature review
- Big Data Analytics capabilities
- Organizational Development Theory
- Organizational Climate
- Organizational Culture
Not all data are created equal; some are structured, but most of them are unstructured. Structured and unstructured data are sourced, collected and scaled in different ways and each one resides in a different type of database.
In this article, we will take a deep dive into both types so that you can get the most out of your data.
Structured data—typically categorized as quantitative data—is highly organized and easily decipherable by machine learning algorithms . Developed by IBM® in 1974 , structured query language (SQL) is the programming language used to manage structured data. By using a relational (SQL) database , business users can quickly input, search and manipulate structured data.
Examples of structured data include dates, names, addresses, credit card numbers, among others. Their benefits are tied to ease of use and access, while liabilities revolve around data inflexibility:
- Easily used by machine learning (ML) algorithms: The specific and organized architecture of structured data eases the manipulation and querying of ML data.
- Easily used by business users: Structured data do not require an in-depth understanding of different types of data and how they function. With a basic understanding of the topic relative to the data, users can easily access and interpret the data.
- Accessible by more tools: Since structured data predates unstructured data, there are more tools available for using and analyzing structured data.
- Limited usage: Data with a predefined structure can only be used for its intended purpose, which limits its flexibility and usability.
- Limited storage options: Structured data are usually stored in data storage systems with rigid schemas (for example, “ data warehouses ”). Therefore, changes in data requirements necessitate an update of all structured data, which leads to a massive expenditure of time and resources.
- OLAP : Performs high-speed, multidimensional data analysis from unified, centralized data stores.
- SQLite : (link resides outside ibm.com) Implements a self-contained, serverless , zero-configuration, transactional relational database engine.
- MySQL : Embeds data into mass-deployed software, particularly mission-critical, heavy-load production system.
- PostgreSQL : Supports SQL and JSON querying as well as high-tier programming languages (C/C+, Java, Python , among others.).
- Customer relationship management (CRM): CRM software runs structured data through analytical tools to create datasets that reveal customer behavior patterns and trends.
- Online booking: Hotel and ticket reservation data (for example, dates, prices, destinations, among others.) fits the “rows and columns” format indicative of the pre-defined data model.
- Accounting: Accounting firms or departments use structured data to process and record financial transactions.
Unstructured data, typically categorized as qualitative data, cannot be processed and analyzed through conventional data tools and methods. Since unstructured data does not have a predefined data model, it is best managed in non-relational (NoSQL) databases . Another way to manage unstructured data is to use data lakes to preserve it in raw form.
The importance of unstructured data is rapidly increasing. Recent projections (link resides outside ibm.com) indicate that unstructured data is over 80% of all enterprise data, while 95% of businesses prioritize unstructured data management.
Examples of unstructured data include text, mobile activity, social media posts, Internet of Things (IoT) sensor data, among others. Their benefits involve advantages in format, speed and storage, while liabilities revolve around expertise and available resources:
- Native format: Unstructured data, stored in its native format, remains undefined until needed. Its adaptability increases file formats in the database, which widens the data pool and enables data scientists to prepare and analyze only the data they need.
- Fast accumulation rates: Since there is no need to predefine the data, it can be collected quickly and easily.
- Data lake storage: Allows for massive storage and pay-as-you-use pricing, which cuts costs and eases scalability.
- Requires expertise: Due to its undefined or non-formatted nature, data science expertise is required to prepare and analyze unstructured data. This is beneficial to data analysts but alienates unspecialized business users who might not fully understand specialized data topics or how to utilize their data.
- Specialized tools: Specialized tools are required to manipulate unstructured data, which limits product choices for data managers.
- MongoDB : Uses flexible documents to process data for cross-platform applications and services.
- DynamoDB : (link resides outside ibm.com) Delivers single-digit millisecond performance at any scale through built-in security, in-memory caching and backup and restore.
- Hadoop : Provides distributed processing of large data sets using simple programming models and no formatting requirements.
- Azure : Enables agile cloud computing for creating and managing apps through Microsoft’s data centers.
- Data mining : Enables businesses to use unstructured data to identify consumer behavior, product sentiment and purchasing patterns to better accommodate their customer base.
- Predictive data analytics : Alert businesses of important activity ahead of time so they can properly plan and accordingly adjust to significant market shifts.
- Chatbots : Perform text analysis to route customer questions to the appropriate answer sources.
While structured (quantitative) data gives a “birds-eye view” of customers, unstructured (qualitative) data provides a deeper understanding of customer behavior and intent. Let’s explore some of the key areas of difference and their implications:
- Sources: Structured data is sourced from GPS sensors, online forms, network logs, web server logs, OLTP systems , among others; whereas unstructured data sources include email messages, word-processing documents, PDF files, and others.
- Forms: Structured data consists of numbers and values, whereas unstructured data consists of sensors, text files, audio and video files, among others.
- Models: Structured data has a predefined data model and is formatted to a set data structure before being placed in data storage (for example, schema-on-write), whereas unstructured data is stored in its native format and not processed until it is used (for example, schema-on-read).
- Storage: Structured data is stored in tabular formats (for example, excel sheets or SQL databases) that require less storage space. It can be stored in data warehouses, which makes it highly scalable. Unstructured data, on the other hand, is stored as media files or NoSQL databases, which require more space. It can be stored in data lakes, which makes it difficult to scale.
- Uses: Structured data is used in machine learning (ML) and drives its algorithms, whereas unstructured data is used in natural language processing (NLP) and text mining.
Semi-structured data (for example, JSON, CSV, XML) is the “bridge” between structured and unstructured data. It does not have a predefined data model and is more complex than structured data, yet easier to store than unstructured data.
Semi-structured data uses “metadata” (for example, tags and semantic markers) to identify specific data characteristics and scale data into records and preset fields. Metadata ultimately enables semi-structured data to be better cataloged, searched and analyzed than unstructured data.
- Example of metadata usage: An online article displays a headline, a snippet, a featured image, image alt-text, slug, among others, which helps differentiate one piece of web content from similar pieces.
- Example of semi-structured data vs. structured data: A tab-delimited file containing customer data versus a database containing CRM tables.
- Example of semi-structured data vs. unstructured data: A tab-delimited file versus a list of comments from a customer’s Instagram.
Recent developments in artificial intelligence (AI) and machine learning (ML) are driving the future wave of data, which is enhancing business intelligence and advancing industrial innovation. In particular, the data formats and models that are covered in this article are helping business users to do the following:
- Analyze digital communications for compliance: Pattern recognition and email threading analysis software that can search email and chat data for potential noncompliance.
- Track high-volume customer conversations in social media: Text analytics and sentiment analysis that enables monitoring of marketing campaign results and identifying online threats.
- Gain new marketing intelligence: ML analytics tools that can quickly cover massive amounts of data to help businesses analyze customer behavior.
Furthermore, smart and efficient usage of data formats and models can help you with the following:
- Understand customer needs at a deeper level to better serve them
- Create more focused and targeted marketing campaigns
- Track current metrics and create new ones
- Create better product opportunities and offerings
- Reduce operational costs
Whether you are a seasoned data expert or a novice business owner, being able to handle all forms of data is conducive to your success. By using structured, semi-structured and unstructured data options, you can perform optimal data management that will ultimately benefit your mission.
Get the latest tech insights and expert thought leadership in your inbox.
To better understand data storage options for whatever kind of data best serves you, check out IBM Cloud Databases
Get our newsletters and topic updates that deliver the latest thought leadership and insights on emerging trends.
AI is poised to drive 160% increase in data center power demand
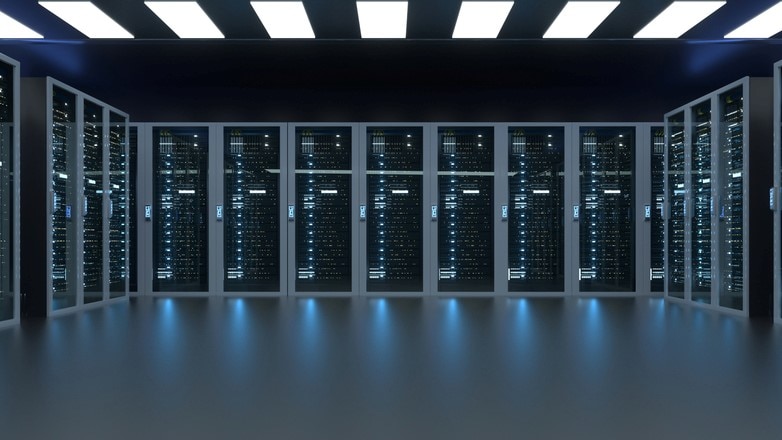
On average, a ChatGPT query needs nearly 10 times as much electricity to process as a Google search. In that difference lies a coming sea change in how the US, Europe, and the world at large will consume power — and how much that will cost.
For years, data centers displayed a remarkably stable appetite for power, even as their workloads mounted. Now, as the pace of efficiency gains in electricity use slows and the AI revolution gathers steam, Goldman Sachs Research estimates that data center power demand will grow 160% by 2030.
At present, data centers worldwide consume 1-2% of overall power, but this percentage will likely rise to 3-4% by the end of the decade. In the US and Europe, this increased demand will help drive the kind of electricity growth that hasn’t been seen in a generation. Along the way, the carbon dioxide emissions of data centers may more than double between 2022 and 2030.
How much power do data centers consume?
In a series of three reports, Goldman Sachs Research analysts lay out the US, European, and global implications of this spike in electricity demand. It isn’t that our demand for data has been meager in the recent past. In fact, data center workloads nearly tripled between 2015 and 2019. Through that period, though, data centers’ demand for power remained flattish, at about 200 terawatt-hours per year. In part, this was because data centers kept growing more efficient in how they used the power they drew, according to the Goldman Sachs Research reports, led by Carly Davenport, Alberto Gandolfi, and Brian Singer.
But since 2020, the efficiency gains appear to have dwindled, and the power consumed by data centers has risen. Some AI innovations will boost computing speed faster than they ramp up their electricity use, but the widening use of AI will still imply an increase in the technology’s consumption of power. A single ChatGPT query requires 2.9 watt-hours of electricity, compared with 0.3 watt-hours for a Google search, according to the International Energy Agency. Goldman Sachs Research estimates the overall increase in data center power consumption from AI to be on the order of 200 terawatt-hours per year between 2023 and 2030. By 2028, our analysts expect AI to represent about 19% of data center power demand.
In tandem, the expected rise of data center carbon dioxide emissions will represent a “social cost” of $125-140 billion (at present value), our analysts believe. “Conversations with technology companies indicate continued confidence in driving down energy intensity but less confidence in meeting absolute emissions forecasts on account of rising demand,” they write. They expect substantial investments by tech firms to underwrite new renewables and commercialize emerging nuclear generation capabilities. And AI may also provide benefits by accelerating innovation — for example, in health care, agriculture, education, or in emissions-reducing energy efficiencies.
US electricity demand is set to surge
Over the last decade, US power demand growth has been roughly zero, even though the population and its economic activity have increased. Efficiencies have helped; one example is the LED light, which drives lower power use. But that is set to change. Between 2022 and 2030, the demand for power will rise roughly 2.4%, Goldman Sachs Research estimates — and around 0.9 percent points of that figure will be tied to data centers.
That kind of spike in power demand hasn’t been seen in the US since the early years of this century. It will be stoked partly by electrification and industrial reshoring, but also by AI . Data centers will use 8% of US power by 2030, compared with 3% in 2022.
US utilities will need to invest around $50 billion in new generation capacity just to support data centers alone. In addition, our analysts expect incremental data center power consumption in the US will drive around 3.3 billion cubic feet per day of new natural gas demand by 2030, which will require new pipeline capacity to be built.
Europe needs $1 trillion-plus to prepare its power grid for AI
Over the past 15 years, Europe's power demand has been severely hit by a sequence of shocks: the global financial crisis, the covid pandemic, and the energy crisis triggered by the war in Ukraine. But it has also suffered due to a slower-than-expected pick up in electrification and the ongoing de-industrialization of the European economy. As a result, since a 2008 peak, electricity demand has cumulatively declined by nearly 10%.
Going forward, between 2023 and 2033, thanks to both the expansion of data centers and an acceleration of electrification, Europe’s power demand could grow by 40% and perhaps even 50%, according to Goldman Sachs Research. At the moment, around 15% of the world’s data centers are located in Europe. By 2030, the power needs of these data centers will match the current total consumption of Portugal, Greece, and the Netherlands combined.
Data center power demand will rise in two kinds of European countries, our analysts write. The first sort is those with cheap and abundant power from nuclear, hydro, wind, or solar sources, such as the Nordic nations, Spain and France. The second kind will include countries with large financial services and tech companies, which offer tax breaks or other incentives to attract data centers. The latter category includes Germany, the UK, and Ireland.
Europe has the oldest power grid in the world, so keeping new data centers electrified will require more investment. Our analysts expect nearly €800 billion ($861 billion) in spending on transmission and distribution over the coming decade, as well as nearly €850 billion in investment on solar, onshore wind, and offshore wind energy.
This article is being provided for educational purposes only. The information contained in this article does not constitute a recommendation from any Goldman Sachs entity to the recipient, and Goldman Sachs is not providing any financial, economic, legal, investment, accounting, or tax advice through this article or to its recipient. Neither Goldman Sachs nor any of its affiliates makes any representation or warranty, express or implied, as to the accuracy or completeness of the statements or any information contained in this article and any liability therefore (including in respect of direct, indirect, or consequential loss or damage) is expressly disclaimed.
Explore More Insights
Sign up for briefings, a newsletter from goldman sachs about trends shaping markets, industries and the global economy..
Thank you for subscribing to BRIEFINGS: a newsletter from Goldman Sachs about trends shaping markets, industries and the global economy.
Some error occurred. Please refresh the page and try again.
Invalid input parameters. Please refresh the page and try again.
Connect With Us
Language selection
- Français fr
WxT Search form
Sensitive technology research areas, pdf version.
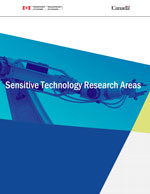
358 KB , 16 pages
Introduction
The list of Sensitive Technology Research Areas consists of advanced and emerging technologies that are important to Canadian research and development, but may also be of interest to foreign state, state-sponsored, and non-state actors, seeking to misappropriate Canada’s technological advantages to our detriment.
While advancement in each of these areas is crucial for Canadian innovation, it is equally important to ensure that open and collaborative research funded by the Government of Canada does not cause injury to Canada’s national security or defence.
The list covers research areas and includes technologies at various stages of development. Of specific concern is the advancement of a technology during the course of the research . This list is not intended to cover the use of any technology that may already be ubiquitous in the course of a research project. Each high-level technology category is complemented by sub-categories which provide researchers with further specificity regarding where the main concerns lie.
The list will be reviewed on a regular basis and updated as technology areas evolve and mature, and as new information and insights are provided by scientific and technical experts across the Government of Canada, allied countries, and the academic research community.
top of page
1. Advanced Digital Infrastructure Technology
Advanced digital infrastructure technology refers to the devices, systems and technologies which compute, process, store, transmit and secure a growing amount of information and data that support an increasingly digital and data-driven world.
Advanced communications technology
Technologies that enable fast, secure and reliable wireless communication to facilitate growing demand for connectivity and faster processing and transmission of data and information. These technologies could also enable communications in remote environments or adverse conditions where conventional methods are ineffective, or in spectrum-congested areas. Examples include: adaptive/cognitive/intelligent radios; massive multiple input/multiple output; millimeter-wave spectrum, open/virtualized radio access networks, optical/photonic communications and wideband high frequency communications.
Advanced computing technology
Computing systems with high computational power that enable the processing of complex calculations that are data- or compute-intensive. Examples include: context-aware computing, edge computing, high performance computing and neuromorphic computing.
Cryptography
Methods and technologies that enable secure communications by transforming, transmitting or storing data in a secure format that can only be deciphered by the intended recipient. Examples of emerging capabilities in cryptography that may replace or enhance current encryption methods include: biometric encryption, DNA-based encryption, post-quantum cryptography, homomorphic encryption and optical stealth encryption.
Cyber security technology
Technologies that protect the integrity, confidentiality and availability of internet-connected systems, including their hardware, software, as well as data from unauthorized access or malicious activities. Examples include: cyber defence tools, cross domain solutions and moving target defence technology.
Data storage technology
The methods, tools, platforms, and infrastructure for storing data or information securely in a digital format. Examples include: five-dimensional (5D) optical storage, DNA storage, single-molecule magnets.
Distributed ledger technology
Digital ledgers or databases that track assets or records transactions in multiple locations at the same time, with no centralized or single point of control or storage. Examples include: blockchain, cryptocurrencies, digital currencies and non-fungible tokens.
Microelectronics
Microelectronics encompasses the development and manufacturing of very small electronic designs on a substrate. It incorporates semiconductors as well as more conventional components such as surface mount technology with the goal of producing smaller and faster products. As microelectronics reach the limit for integration, photonic components are making their way into this field. Examples of semiconductor components include: memory-centric logic, multi-chip module, systems-on-chip and stacked memory on chip.
Next-generation network technology
Fifth and future generations of communications networks that use high frequency spectrums to enable significantly faster processing and transmission speeds for larger amounts of data. Advancements in networking could allow for integrated communication across air, land, space and sea using terrestrial and non-terrestrial networks, as well as increased data speed and capacity for network traffic. It could also pave the way for new AI- and big data-driven applications and services, and its massive data processing capabilities could enable the Internet of Everything.
2. Advanced Energy Technology
Advanced energy technology refers to technologies and processes that enable improved generation, storage and transmission of energy, as well as operating in remote or adverse environments where power sources may not be readily available, but are required to support permanent or temporary infrastructure and power vehicles, equipment and devices.
Advanced energy storage technology
Technologies that store energy, such as batteries, with new or enhanced properties, including improved energy density, compact size and low weight to enable portability, survivability in harsh conditions and the ability to recharge quickly. Examples include: fuel cells, novel batteries (biodegradable batteries; graphene aluminium-ion batteries; lithium-air batteries; room-temperature all-liquid-metal batteries; solid-state batteries; structural batteries) and supercapacitors (or ultracapacitors).
Advanced nuclear generation technology
New reactors and technologies that are smaller in size than conventional nuclear reactors and are developed to be less capital-intensive, therefore minimizing risks faced during construction. Examples include: nuclear fusion and small modular reactors.
Wireless power transfer technology
Enables the transmission of electricity without using wire over extended distances that vary greatly and could be up to several kilometres. Examples include recharging zones (analogous to Wi-Fi zones) that allow for electric devices, such as vehicles, to be recharged within a large radius, as well as for recharging space-based objects, such as satellites.
3. Advanced Materials and Manufacturing
Advanced materials.
Advanced materials refer to high-value products, components or materials with new or enhanced structural or functional properties. They may rely on advanced manufacturing processes or novel approaches for their production.
Augmented conventional materials
Conventional materials such as high strength steel or aluminum and magnesium alloys – products that are already widely used – which are augmented to have unconventional or extraordinary properties. Examples of these properties could include improved durability or high temperature strength, corrosion resistance, flexibility, weldability, or reduced weight, among others.
Auxetic materials
Materials that have a negative Poisson’s ratio, meaning that when stretched horizontally, they thicken or expand vertically (rather than thinning as most materials do when stretched), and do the opposite when compressed horizontally. These materials possess unique properties, such as energy-absorption, high rigidity, improved energy/impact absorption and resistance to fracture.
High-entropy materials
Special materials, including high-entropy alloys, high-entropy oxides or other high-entropy compounds, comprised of several elements or components. Depending on their composition, high-entropy materials can enhance fracture toughness, strength, conductivity, corrosion resistance, hardness and other desired properties. Due to the breadth of the theoretically available combinations and their respective properties, these materials can be used in several industries, including aerospace. Additionally, high-entropy oxides are being considered for applications in energy production and storage, as well as thermal barrier coatings.
Metamaterials
Structured materials that are not found or easily obtained in nature. Metamaterials often have unique interactions with electromagnetic radiation (i.e. light or microwaves) or sound waves.
Multifunctional/smart materials
Materials that can transform in response to external stimuli (e.g. heat, water, light, etc.) within a given amount of time. Examples include: magnetorheological fluid, shape memory alloys, shape memory polymers and self-assembled materials.
Nanomaterials
Nanomaterial materials have dimensions of less than 100 nanometers and exhibit certain properties or unique characteristics such as increased durability or self-repair. A subset of nanomaterials, nano-energetic materials are energetic materials synthesized and fabricated at the nano-level that have a small particle size and high surface area between particles, which enable faster or more efficient reaction pathways when exposed to other substances.
Powder materials for additive manufacturing
Powders that typically consist of metal, polymer, ceramic and composite materials. These powders enable additive manufacturing processes, also referred to as 3D printing. Research into novel powder materials can lead to manufactured parts with enhanced mechanical properties and other desired characteristics.
Superconducting materials
Materials that can transmit electricity with no resistance, ultimately eliminating power losses associated with electrical resistivity that normally occurs in conductors. Manufacturing of superconducting electronic circuits is one of the most promising approaches to implementing quantum computers.
Two-dimensional (2D) materials
Materials with a thickness of roughly one atomic layer. One of the most well-known 2D materials, for which there are currently production/fabrication technologies, is graphene. Other examples of 2D materials include: silicene, germanene, stantene, metal chalcogenides and others, which are currently being researched with potential applications in sensors, miniaturized electronic devices, semiconductors and more.
Advanced Manufacturing
Advanced manufacturing refers to enhanced or novel technologies, tools and processes used to develop and manufacture advanced materials or components. This could include using specialized software, artificial intelligence, sensors and high performance tools, among others, to facilitate process automation or closed-loop automated machining and create new materials or components.
Additive manufacturing (3D printing)
Various processes in which solid three-dimensional objects are constructed using computer-aided-design (CAD) software to build an object, ranging from simple geometric shapes to parts for commercial airplanes. 3D printing could be used to accelerate the development through rapid prototyping of customized equipment, spare tools or novel shapes or objects that are stronger and lighter. Approaches are also being developed for multi-material additive manufacturing and volumetric additive manufacturing, as well as additive manufacturing for repair and restoration.
Advanced semiconductor manufacturing
Methods, materials and processes related to the manufacturing of semiconductor devices. Examples of techniques include: advancements in deposition, coating, lithography, ionization/doping, and other core and supporting processes, such as thermal management techniques. Recent technological advancements include developments in Extreme Ultraviolet (EUV) lithography, which is an advanced method for fabricating intricate patterns on a substrate to produce a semiconductor device with extremely small features.
Critical materials manufacturing
Up and midstream technologies necessary to extract, process, upgrade, and recycle/recover critical materials (e.g. rare earth elements, scandium, lithium, etc.) and establish and maintain secure domestic and allied supply chains. More information about critical minerals can be found in Canada’s Critical Minerals List .
Four-dimensional (4D) printing
Production and manufacture of 3D products using multifunctional or “smart” materials that are programmed to transform in response to external stimuli (e.g. heat, water, light, etc.) within a given amount of time. Recent developments have also been made in creating reversible 4D printed objects, which can return to their original shape without human involvement.
Nano-manufacturing
Production and manufacture of nanoscale materials, structures, devices and systems in a scaled-up, reliable and cost-effective manner.
Two-dimensional (2D) materials manufacturing
Standardized, scalable and cost-effective large-scale production of 2D materials.
4. Advanced Sensing and Surveillance
Advanced sensing and surveillance refers to a large array of advanced technologies that detect, measure or monitor physical, chemical, biological or environmental conditions and generate data or information about them. Advanced surveillance technologies, in particular, are used to monitor and observe the activities and communications of specific individuals or groups for national security or law enforcement purposes, but have also been used for mass surveillance with increased accuracy and scale.
Advanced biometric recognition technologies
Technologies that identify individuals based on their distinctive physical identifiers (e.g. face, fingerprint or DNA) or behavioural identifiers (e.g. gait, keystroke pattern and voice). These technologies are becoming more advanced due to improving sensing capabilities, as well as integrating artificial intelligence to identify/verify an individual more quickly and accurately.
Advanced radar technologies
Radar is a system that uses radio waves to detect moving objects and measure their distance, speed and direction. Advancements in radar technology could enable improved detection and surveillance in different environments and over greater distances. Examples include: active electronically-scanned arrays, cognitive radars, high frequency skywave radar (or over-the-horizon radar), passive radar and synthetic aperture radar.
Atomic interferometer sensors
Sensors that perform sensitive interferometric measurements using the wave character of atomic particles and quantum gases. These sensors can detect small changes in inertial forces and can be used in gravimetry. They can also improve accuracy in navigation and provide position information in environments where the Global Positioning System (GPS) is unavailable.
Cross-cueing sensors
Systems that enable multiple sensors to cue one another. Cross cueing can be used in satellites for data validation, objection tracking, enhanced reliability (i.e. in the event of a sensor failure) and earth observations.
Electric field sensors
Sensors that detect variations in electric fields and use low amounts of power. They are useful for detecting power lines or lightning, as well as locating power grids or damaged components in the aftermath of a natural disaster.
Imaging and optical devices and sensors
Devices and sensors that provide a visual depiction of the physical structure of an object beyond the typical capabilities of consumer grade imaging techniques such as cameras, cellphones, and visible light-imaging. Such technologies typically make use of electromagnetic radiation beyond the visible spectrum, or use advanced techniques and materials to improve optical capabilities, such as enabling more precise imaging from a greater distance. This sensitive research area also includes sensitive infrared sensors.
Magnetic field sensors (or magnetometers)
Sensors that are used to detect or measure changes in a magnetic field, or its intensity or direction.
Micro (or nano) electro-mechanical systems (M/NEMS)
Miniaturized, lightweight electro-mechanical devices that integrate mechanical and electrical functionality at the microscopic or nano level. A potential use of M/NEMS could be as ‘smart dust’, or a group of M/NEMs, made up of various components, including sensors, circuits, communications technology and a power supply, that function as a single digital entity. Smart dust could be light enough to float in the air and detect vibrations, light, pressure and temperature, among other things, to capture a great deal of information about a particular environment.
Position, navigation and timing (PNT) technology
Systems, platforms or capabilities that enable accurate and timely calculation of positioning, navigation and timing. These technologies are critical to a wide-range of applications, most notably for enabling the Global Navigation Satellite System (GNSS), one of which is the widely-used Global Positioning System (GPS), but also for enabling navigation in areas where GPS or GNSS do not work. Examples include: chip-scale advanced atomic clocks, gravity-aided inertial navigation system, long-range underwater navigation system, magnetic anomaly navigation, precision inertial navigation system.
Side scan sonar
An active sonar system that uses a transducer array to send and receive acoustic pulses in swaths laterally from the tow-body or vessel, enabling it to quickly scan a large area in a body of water to produce an image of the sea floor beneath the tow-body or vessel.
Synthetic aperture sonar (SAS)
An active sonar system that produces high resolution images of the sea floor along the track of the vessel or tow body. SAS can send continuous sonar signals to capture images underwater at 30 times the resolution of traditional sonar systems, as well as up to 10 times the range and area coverage.
Underwater (wireless) sensor network
Network of sensors and autonomous/uncrewed underwater vehicles that use acoustic waves to communicate with each other, or with underwater sinks that collect and transmit data from deep ocean sensors, to enable remote sensing, surveillance and ocean exploration, observation and monitoring.
5. Advanced Weapons
Emerging or improved weapons used by military, and in some instances law enforcement, for defence and national security purposes. Advancements in materials, manufacturing, propulsion, energy and other technologies have brought weapons like directed energy weapons and hypersonic weapons closer to reality, while nanotechnology, synthetic biology, artificial intelligence and sensing technologies, among others, have provided enhancements to existing weapons, such as biological/chemical weapons and autonomous weapons.
6. Aerospace, Space and Satellite Technology
Aerospace technology refers to the technology that enables the design, production, testing, operation and maintenance of aircraft, spacecraft and their respective components, as well as other aeronautics. Space and satellite technology refers to technologies that enable travel, research and exploration in space, as well as weather-tracking, advanced PNT, communications, remote sensing and other capabilities using satellites and other space-based assets.
Advanced wind tunnels
Technological advancements in systems related to wind tunnel infrastructure. Existing facilities are used to simulate various flight conditions and speeds ranging from subsonic, transonic, supersonic and hypersonic.
On-orbit servicing, assembly and manufacturing systems
Systems and equipment that are used for space-based servicing, assembly and manufacturing. On-orbit servicing, assembly and manufacturing systems can be used to optimize space logistics, increase efficiencies, mitigate debris threats and to modernize space asset capabilities.
Lower cost satellite payloads with increased performance that can meet the needs of various markets. This will require several technology improvements, such as light weight apertures, antennas, panels, transceivers, control actuators, optical/infrared sensor and multi-spectral imagers, to meet the growing demand and ever-increasing technical requirements.
Propulsion technologies
Components and systems that produce a powerful thrust to push an object forward, which is essential to launching aircraft, spacecraft, rockets or missiles. Innovations could range from new designs or advanced materials to enable improved performance, speed, energy-efficiency and other enhanced properties, as well as reduced aircraft production times and emissions. Examples include: electrified aircraft propulsion, solar electric propulsion, pulse detonation engines, nuclear thermal propulsion systems, nuclear pulse propulsion systems and nuclear electric propulsion systems, among others.
Artificial or human-made, including (semi-)autonomous, objects placed into orbit. Depending on their specific function, satellites typically consist of an antenna, radio communications system, a power source and a computer, but their exact composition may vary. Continued developments have led to smaller satellites that are less costly to manufacture and deploy compared to large satellites, resulting in faster development times and increased accessibility to space. Examples include: remote sensing and communications satellites.
Space-based positioning, navigation and timing technology
Global Navigation Satellite System (GNSS)-based satellites and technologies that will improve the accuracy, agility and resilience of GNSS and the Global Positioning System (GPS).
Space stations
Space-based facility that can act as an orbital outpost while having the ability to support extended human operations. Space stations can be used as a hub to support other space-based activities including assembly, manufacturing, research, experimentations, training, space vehicle docking and storage. Examples of innovations in space stations could include the ability to extend further out into space or enhanced life support systems that can be used to prolong human missions.
Zero-emission/fuel aircraft
Aircraft powered by energy sources that do not emit polluting emissions that disrupt the environment or do not require fuel to fly. While still in early stages, these advances in powering aircraft could support cleaner air travel, as well as enable flight over greater distances and to remote areas without the need for refueling (for zero-fuel aircraft).
7. Artificial Intelligence and Big Data Technology
Artificial intelligence (AI) is a broad field encompassing the science of making computers behave in a manner that simulates human behaviour/intelligence using data and algorithms. Big data refers to information and data that is large and complex in volume, velocity and variety, and as such, requires specialized tools, techniques and technologies to process, analyze and visualize it. AI and big data technology may be considered cross-cutting given how important they are in enabling developments in other technology areas, including biotechnology, advanced materials and manufacturing, robotics and autonomous systems and others.
AI chipsets
Custom-designed chips meant to process large amounts of data and information that enable algorithms to perform calculations more efficiently, simultaneously and using less energy than general-purpose chips. AI chips have unique design features specialized for AI, which may make them more cost-effective to use for AI development.
Computer vision
Field of AI that allows computers to see and extract meaning from the content of digital images such as photos and videos. Examples of computer vision techniques include: image classification, object detection, depth perception and others.
Data science and big data technology
Enables the autonomous or semi-autonomous analysis of data, namely large and/or complex sets of data when it comes to big data technology. It also includes the extraction or generation of deeper insights, predictions or recommendations to inform decision-making. Examples include: AI-enabled data analytics, big data technology (i.e. data warehouse, data mining, data correlation) and predictive analytics.
Digital twin technology
Virtual representations of physical objects or systems that combine real-time sensor data, big data processing and artificial intelligence (namely machine learning) to create an interactive model and predict the object or system’s future behaviour or performance. Advancements in digital twin technology could enable the growth and integration of an immersive digital experience (e.g. the metaverse) into daily life.
Machine learning (ML)
Branch of AI where computer programs are trained using algorithms and data to improve their decisions when introduced to a new set of data without necessarily being programmed to do so. Types of ML include: deep learning, evolutionary computation and neural networks.
Natural language processing
An area of AI that allows computers to process and make sense of, or ‘translate’, natural human language using speech and audio recognition to identify, analyze and interpret human voices and other types of audio. Examples include: syntactic and semantic analysis, tokenization, text classification and others, which enable capabilities like virtual assistants, chatbots, machine translation, predictive text, sentiment analysis and automatic summarization.
8. Human-Machine Integration
Human-machine integration refers to the pairing of operators with technology to enhance or optimize human capability. The nature of the integration can vary widely, with an important dimension being the invasive nature of the pairing.
Brain-computer interfaces
Interfaces that allow a human to interact with a computer directly via input from the brain through a device that senses brain activity, allowing for research, mapping, assistance or augmentation of human brain functions that could enable improved cognitive performance or communication with digital devices.
Exoskeletons
External devices or ‘wearable robots’ that can assist or augment the physical and physiological performance/capabilities of an individual or a group.
Neuroprosthetic/cybernetic devices
Implanted and worn devices that interact with the nervous system to enhance or restore motor, sensory, cognitive, visual, auditory or communicative functions, often resulting from brain injury. This includes cybernetic limbs or devices that go beyond medical use to contribute to human performance enhancement.
Virtual/augmented/mixed reality
Immersive technologies that combine elements of the virtual world with the real world to create an interactive virtual experience. An application of these technologies that several companies are developing is the ‘metaverse’ which is an immersive digital experience that integrates the physical world with the digital one and allows users to interact and perform a variety of activities like shopping and gaming, seamlessly in one virtual ecosystem. While still being explored, this could potentially translate into a digital economy with its own currency, property and other goods.
Wearable neurotechnology
Brain-computer interfaces that are wearable and non-invasive (i.e. do not need to be implanted). These wearable brain devices can be used for medical uses, such as tracking brain health and sending data to a doctor to inform treatment, as well as for non-medical applications related to human optimization, augmentation or enhancement, such as user-drowsiness, cognitive load monitoring or early reaction detection, among others.
9. Life Science Technology
Life science technology is a broad term that encompasses a wide array of technologies that enhance living organisms, such as biotechnology and medical and healthcare technologies.
Biotechnology
Biotechnology uses living systems, processes and organisms, or parts of them, to develop new or improved products, processes or services. It often integrates other areas of technology, such as nanotechnology, artificial intelligence, computing and others, to create novel solutions to problems, including in the area of human performance enhancement.
Biomanufacturing
Methods and processes that enable the industrial production of biological products and materials through the modification of biological organisms or systems. Advances in biomanufacturing, such as automation and sensor-based production, has led to commercial-scale production of new biological products, such as biomaterials and biosensors.
Genomic sequencing and genetic engineering
Technologies that enable whole genome sequencing, the direct manipulation of an organism’s genome using DNA, or genetic engineering to produce new or modified organisms. Examples include: Clustered Regularly Interspaced Short Palindromic Repeats (CRISPR) and Next Generation Sequencing (NGS).
Large-scale and experimental analysis of protein, proteomes and proteome informatics. Proteomic applications can be used for the identification of unknown bacterial species and strains, as well as species level identifications of tissues, body fluids, and bones of unknown origin.
Synthetic biology
Combination of biology and engineering to create new biological entities, such as cells or enzymes, or redesign existing biological systems, with new functions like sensing or producing a specific substance. Synthetic biology is expected to enable advancements in many areas, such as antibiotic, drug and vaccine development, biocomputers, biofuel, novel drug delivery platforms, novel chemicals, synthetic food, and synthetic life.
Medical and Healthcare Technology
Medical and healthcare technology refers to tools, processes or services that support good health and prevent, or attempt to prevent, disease. Advances in biotechnology, nanotechnology and advanced materials are enabling new methods of delivering medicine or treating injuries, diseases or exposure to toxic substances.
Chemical, Biological, Radiological and Nuclear (CBRN) medical countermeasures
Various medical assets used to prevent, identify or treat injuries or illnesses caused by chemical, biological, radiological or nuclear (CBRN) threats, whether naturally-occurring or engineered. CBRN medical countermeasures include therapeutics to treat injuries and illnesses, such as biologic products or drugs, as well diagnostics to identify the threats.
Gene therapy
Use of gene manipulation or modification in humans to prevent, treat or cure disease, either by replacing or disabling disease-causing genes or inserting new or modified genes.
Nanomedicine
Use of nanomaterials to diagnose, monitor, prevent and/or treat disease. Examples of nanomedicine include nanoparticles for targeted drug delivery, smart imaging using nanomaterials, as well as nano-engineered implants to support tissue engineering and regenerative medicine.
Tissue engineering and regenerative medicine
Methods of regenerating or rebuilding cells, tissues or organs to allow normal, biological functions to be restored. Regenerative medicine includes self-healing, where the body is able to use its own tools or other biological materials to regrow tissues or cells, whereas tissue engineering largely focuses on the use of synthetic and biological materials, such as stem cells, to build function constructs or supports that help heal or restore damaged tissues or organs.
10. Quantum Science and Technology
Quantum science and technology refers to a new generation of devices that use quantum effects to significantly enhance the performance over those of existing, ‘classical’, technologies. This technology is expected to deliver sensing and imaging, communications, and computing capabilities that far exceed those of conventional technologies in certain cases, well as new materials with extraordinary properties and many useful applications. Quantum science and technology may be considered cross-cutting, given that quantum-enhanced technologies are expected to enable advancements or improvements in most other technology areas, including biotechnology, advanced materials, robotics and autonomous systems, aerospace, space and satellite technology and others.
Quantum communications
Use of quantum physics to enable secure communications and protect data using quantum cryptography, also know as quantum key distribution.
Quantum computing
Use of quantum bits, also known as qubits, to process information by capitalizing on quantum mechanical effects that allow for a large amount of information, such as calculations, to be processed at the same time. A quantum computer that can harness qubits in a controlled quantum state may be able to compute and solve certain problems significantly faster than the most powerful supercomputers.
Quantum materials
Materials with unusual magnetic and electrical properties. Examples include: superconductors, graphene, topological insulators, Weyl semimetals, metal chalcogenides and others. While many of these materials are still being explored and studied, they are promising contenders that could enable energy-efficient electrical systems, better batteries and the development of new types of electronic devices.
Quantum sensing
Broad of range of devices, at various stages of technological readiness, that use quantum systems, properties, or phenomena to measure a physical quantity with increased precision, stability and accuracy. Recent developments in applications of quantum physics identified the possibility of exploiting quantum phenomena as means to develop quantum radar technology.
Quantum software
Software and algorithms that run on quantum computers, enable the efficient operation and design of quantum computers, or software that enables the development and optimization of quantum computing applications.
11. Robotics and Autonomous Systems
Robotics and Autonomous Systems are machines or systems with a certain degree of autonomy (ranging from semi- to fully autonomous) that are able to carry out certain activities with little to no human control or intervention by gathering insights from their surroundings and making decisions based on them, including improving their overall task performance.
Molecular (or nano) robotics
Development of robots at the molecular or nano-scale level by programming molecules to carry out a particular task.
(Semi-)autonomous/uncrewed aerial/ground/marine vehicles
Vehicles that function without any onboard human intervention, and instead, are either controlled remotely by a human operator, or operate semi-autonomously or autonomously. Uncrewed vehicles rely on software, sensors and artificial intelligence technology to collect and analyze information about their environment, plan and alter their route (if semi- or fully autonomous), and interact with other vehicles (or human operator, if remotely-controlled).
Service robots
Robots that carry out tasks useful to humans that may be tedious, time-consuming, repetitive, dangerous or complement human behaviour when resources are not available, e.g. supporting elderly people. They are semi- or fully-autonomous, able to make decisions with some or no human interaction/intervention (depending on the degree of autonomy), and can be manually overridden by a human.
Space robotics
Devices, or ‘space robots’, that are able to perform various functions in orbit, such as assembling or servicing, to support astronauts, or replace human explorers in the exploration of remote planets.
Microsoft Research Blog
Microsoft at chi 2024: innovations in human-centered design.
Published May 15, 2024
Share this page
- Share on Facebook
- Share on Twitter
- Share on LinkedIn
- Share on Reddit
- Subscribe to our RSS feed

The ways people engage with technology, through its design and functionality, determine its utility and acceptance in everyday use, setting the stage for widespread adoption. When computing tools and services respect the diversity of people’s experiences and abilities, technology is not only functional but also universally accessible. Human-computer interaction (HCI) plays a crucial role in this process, examining how technology integrates into our daily lives and exploring ways digital tools can be shaped to meet individual needs and enhance our interactions with the world.
The ACM CHI Conference on Human Factors in Computing Systems is a premier forum that brings together researchers and experts in the field, and Microsoft is honored to support CHI 2024 as a returning sponsor. We’re pleased to announce that 33 papers by Microsoft researchers and their collaborators have been accepted this year, with four winning the Best Paper Award and seven receiving honorable mentions.
This research aims to redefine how people work, collaborate, and play using technology, with a focus on design innovation to create more personalized, engaging, and effective interactions. Several projects emphasize customizing the user experience to better meet individual needs, such as exploring the potential of large language models (LLMs) to help reduce procrastination. Others investigate ways to boost realism in virtual and mixed reality environments, using touch to create a more immersive experience. There are also studies that address the challenges of understanding how people interact with technology. These include applying psychology and cognitive science to examine the use of generative AI and social media, with the goal of using the insights to guide future research and design directions. This post highlights these projects.
Spotlight: On-demand video

AI Explainer: Foundation models and the next era of AI
Explore how the transformer architecture, larger models and more data, and in-context learning have helped advance AI from perception to creation.
Best Paper Award recipients
DynaVis: Dynamically Synthesized UI Widgets for Visualization Editing Priyan Vaithilingam, Elena L. Glassman, Jeevana Priya Inala , Chenglong Wang GUIs used for editing visualizations can overwhelm users or limit their interactions. To address this, the authors introduce DynaVis, which combines natural language interfaces with dynamically synthesized UI widgets, enabling people to initiate and refine edits using natural language.
Generative Echo Chamber? Effects of LLM-Powered Search Systems on Diverse Information Seeking Nikhil Sharma, Q. Vera Liao , Ziang Xiao Conversational search systems powered by LLMs potentially improve on traditional search methods, yet their influence on increasing selective exposure and fostering echo chambers remains underexplored. This research suggests that LLM-driven conversational search may enhance biased information querying, particularly when the LLM’s outputs reinforce user views, emphasizing significant implications for the development and regulation of these technologies.
Piet: Facilitating Color Authoring for Motion Graphics Video Xinyu Shi, Yinghou Wang, Yun Wang , Jian Zhao Motion graphic (MG) videos use animated visuals and color to effectively communicate complex ideas, yet existing color authoring tools are lacking. This work introduces Piet, a tool prototype that offers an interactive palette and support for quick theme changes and controlled focus, significantly streamlining the color design process.
The Metacognitive Demands and Opportunities of Generative AI Lev Tankelevitch , Viktor Kewenig, Auste Simkute, Ava Elizabeth Scott, Advait Sarkar , Abigail Sellen , Sean Rintel Generative AI systems offer unprecedented opportunities for transforming professional and personal work, yet they present challenges around prompting, evaluating and relying on outputs, and optimizing workflows. This paper shows that metacognition—the psychological ability to monitor and control one’s thoughts and behavior—offers a valuable lens through which to understand and design for these usability challenges.
Honorable Mentions
B ig or Small, It’s All in Your Head: Visuo-Haptic Illusion of Size-Change Using Finger-Repositioning Myung Jin Kim, Eyal Ofek, Michel Pahud , Mike J. Sinclair, Andrea Bianchi This research introduces a fixed-sized VR controller that uses finger repositioning to create a visuo-haptic illusion of dynamic size changes in handheld virtual objects, allowing users to perceive virtual objects as significantly smaller or larger than the actual device.
LLMR: Real-time Prompting of Interactive Worlds Using Large Language Models Fernanda De La Torre, Cathy Mengying Fang, Han Huang, Andrzej Banburski-Fahey, Judith Amores , Jaron Lanier Large Language Model for Mixed Reality (LLMR) is a framework for the real-time creation and modification of interactive mixed reality experiences using LLMs. It uses novel strategies to tackle difficult cases where ideal training data is scarce or where the design goal requires the synthesis of internal dynamics, intuitive analysis, or advanced interactivity.
Observer Effect in Social Media Use Koustuv Saha, Pranshu Gupta, Gloria Mark, Emre Kiciman , Munmun De Choudhury This work investigates the observer effect in behavioral assessments on social media use. The observer effect is a phenomenon in which individuals alter their behavior due to awareness of being monitored. Conducted over an average of 82 months (about 7 years) retrospectively and five months prospectively using Facebook data, the study found that deviations in expected behavior and language post-enrollment in the study reflected individual psychological traits. The authors recommend ways to mitigate the observer effect in these scenarios.
Reading Between the Lines: Modeling User Behavior and Costs in AI-Assisted Programming Hussein Mozannar, Gagan Bansal , Adam Fourney , Eric Horvitz By investigating how developers use GitHub Copilot, the authors created CUPS, a taxonomy of programmer activities during system interaction. This approach not only elucidates interaction patterns and inefficiencies but can also drive more effective metrics and UI design for code-recommendation systems with the goal of improving programmer productivity.
SharedNeRF: Leveraging Photorealistic and View-dependent Rendering for Real-time and Remote Collaboration Mose Sakashita, Bala Kumaravel, Nicolai Marquardt , Andrew D. Wilson SharedNeRF, a system for synchronous remote collaboration, utilizes neural radiance field (NeRF) technology to provide photorealistic, viewpoint-specific renderings that are seamlessly integrated with point clouds to capture dynamic movements and changes in a shared space. A preliminary study demonstrated its effectiveness, as participants used this high-fidelity, multi-perspective visualization to successfully complete a flower arrangement task.
Understanding the Role of Large Language Models in Personalizing and Scaffolding Strategies to Combat Academic Procrastination Ananya Bhattacharjee, Yuchen Zeng, Sarah Yi Xu, Dana Kulzhabayeva, Minyi Ma, Rachel Kornfield, Syed Ishtiaque Ahmed, Alex Mariakakis, Mary P. Czerwinski , Anastasia Kuzminykh, Michael Liut, Joseph Jay Williams In this study, the authors explore the potential of LLMs for customizing academic procrastination interventions, employing a technology probe to generate personalized advice. Their findings emphasize the need for LLMs to offer structured, deadline-oriented advice and adaptive questioning techniques, providing key design insights for LLM-based tools while highlighting cautions against their use for therapeutic guidance.
Where Are We So Far? Understanding Data Storytelling Tools from the Perspective of Human-AI Collaboration Haotian Li, Yun Wang , Huamin Qu This paper evaluates data storytelling tools using a dual framework to analyze the stages of the storytelling workflow—analysis, planning, implementation, communication—and the roles of humans and AI in each stage, such as creators, assistants, optimizers, and reviewers. The study identifies common collaboration patterns in existing tools, summarizes lessons from these patterns, and highlights future research opportunities for human-AI collaboration in data storytelling.
Learn more about our work and contributions to CHI 2024, including our full list of publications , on our conference webpage .
Related publications
Where are we so far understanding data storytelling tools from the perspective of human-ai collaboration, the metacognitive demands and opportunities of generative ai, piet: facilitating color authoring for motion graphics video, dynavis: dynamically synthesized ui widgets for visualization editing, generative echo chamber effects of llm-powered search systems on diverse information seeking, understanding the role of large language models in personalizing and scaffolding strategies to combat academic procrastination, sharednerf: leveraging photorealistic and view-dependent rendering for real-time and remote collaboration, big or small, it’s all in your head: visuo-haptic illusion of size-change using finger-repositioning, llmr: real-time prompting of interactive worlds using large language models, reading between the lines: modeling user behavior and costs in ai-assisted programming, observer effect in social media use, continue reading.
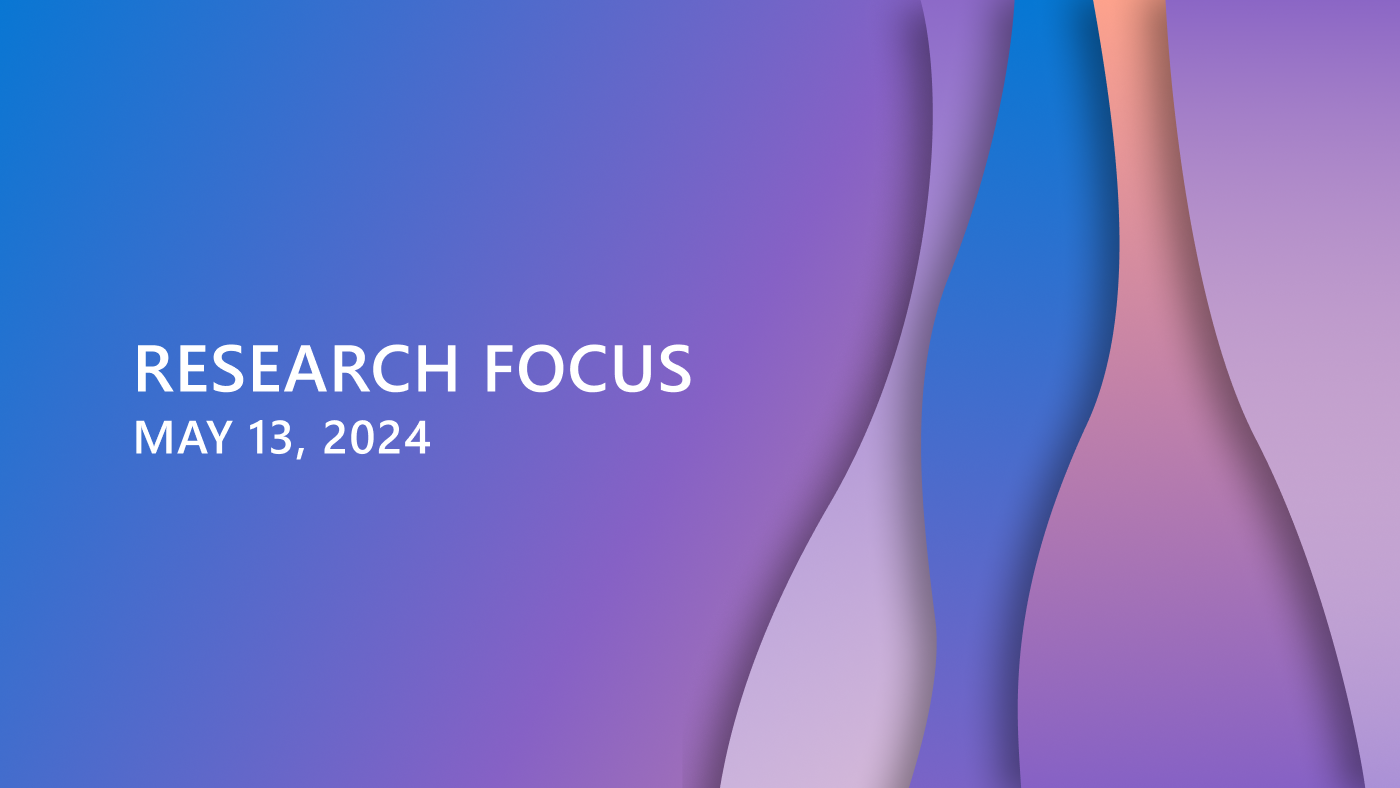
Research Focus: Week of May 13, 2024

Research Focus: Week of April 15, 2024

Research Focus: Week of March 18, 2024

Advancing human-centered AI: Updates on responsible AI research
Research areas.

Related events
- Microsoft at CHI 2024
Related labs
- Microsoft Research Lab - Redmond
- Microsoft Research Lab – Montréal
- AI Frontiers
- Microsoft Research Lab - Asia
- Microsoft Research Lab - Cambridge
- Follow on Twitter
- Like on Facebook
- Follow on LinkedIn
- Subscribe on Youtube
- Follow on Instagram
Share this page:
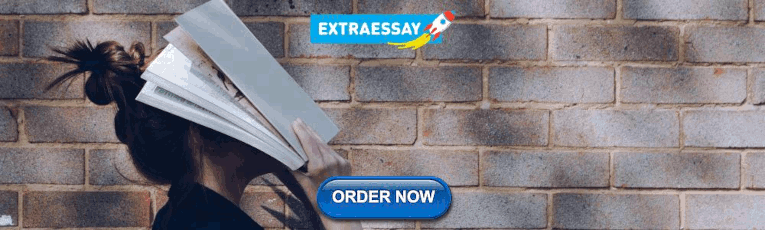
IMAGES
VIDEO
COMMENTS
About the journal. The journal aims to promote and communicate advances in big data research by providing a fast and high quality forum for researchers, practitioners and policy makers from the very many different communities working on, and with, this topic. The journal will accept papers on foundational aspects in dealing with big data, as ...
Research on big data analysis thus sheds light on elements of the research process that cannot be fully controlled, rationalised or even considered through recourse to formal tools. One such element is the work required to present empirical data in a machine-readable format that is compatible with the software and analytic tools at hand. Data ...
The Journal of Big Data publishes open-access original research on data science and data analytics. Deep learning algorithms and all applications of big data are welcomed. Survey papers and case studies are also considered. The journal examines the challenges facing big data today and going forward including, but not limited to: data capture ...
Research Topics. This innovative journal focuses on the power of big data - its role in machine learning, AI, and data mining, and its practical application from cybersecurity to climate science and public health.
Big Data reframes key questions about the constitution of knowledge, the processes of research, how we should engage with information, and the nature and the categorization of reality … Big Data stakes out new terrains of objects, methods of knowing, and definitions of social life. (boyd and Crawford, 2012)
Big Data is a large data set with increasing volume, variety and velocity. Learn more about the big data definition, examples and tools on Cloud. ... Using AI-powered technologies like natural language processing to analyze unstructured medical data (such as research reports, clinical notes, and lab ...
Today, sociological research faces a widely datafied world, where (big) data analytics are profoundly changing the paradigm of knowledge production, as Facebook, Twitter, Google and others produce ...
More recently, big data have been used to support research toward the UN Sustainable Development Goals (SDGs), such as climate action (SDG 13) and life below water (SDG 14) . Authors have provided a comprehensive review of the applications of big data in geophysics , biology , and for the use of big data and AI . The European ...
A method for skyline query of multidimensional incomplete data based on a classification tree has been proposed to address the problem of a large amount of useless data in existing skyline queries with multidi... Dengke Yuan, Liping Zhang, Song Li and Guanglu Sun. Journal of Big Data 2024 11 :72. Research Published on: 12 May 2024. Full Text.
Research methods are roadmaps, techniques, and procedures employed in a study to collect data, process data, analyze data, yield findings, and draw a conclusion to achieve the research aims. To a large degree the availability, nature, and size of a dataset can affect the selection of the research methods, even the research topics.
Huadong Guo. With data volumes expanding beyond the Petabyte and Exabyte levels across many scientific disciplines, the role of big data for scientific research is becoming increasingly apparent: the massive data processing has become valuable for scientific research. The term big data is not only a buzzword and a marketing tool, but also it ...
The Journal of Big Data publishes open-access original research on data science and data analytics. Deep learning algorithms and all applications of big data are welcomed. Survey papers and case studies are also considered. The journal examines the challenges facing big data today and going forward including, but not limited to: data capture ...
Big Data initiatives in the United Kingdom. The UK Biobank is a prospective cohort initiative that is composed of individuals between the ages of 40 and 69 before disease onset (Allen et al. 2012 ...
In this descriptive review, we highlight the roles of big data, the changing research paradigm, and easy access to research participation via the Internet fueled by the need for quick answers. Universally, data volume has increased, with the collection rate doubling every 40 months, ever since the 1980s. 4 The big data age, starting in 2002 ...
The amount of data in our world has been exploding, and analyzing large data sets—so-called big data—will become a key basis of competition, underpinning new waves of productivity growth, innovation, and consumer surplus, according to research by MGI and McKinsey's Business Technology Office. Leaders in every sector will have to grapple ...
While many big-data advancements are encouraging and impressive, considerable challenges remain regarding big-data applications in cancer research and the clinic.
Prior research observed several issues related to big data accumulated in healthcare, such as data quality (Sabharwal, Gupta, and Thirunavukkarasu Citation 2016) and data quantity (Gopal et al. Citation 2019). However, there is a lack of research into the types of problems that may occur during data accumulation processes in healthcare and how ...
Big data is a term used to describe data sources that are fast-changing, large in both size and breadth of information, and come from sources other than surveys. Examples include retail and payroll transactions, satellite images, and "smart" devices. Big data also includes administrative data from federal, state, and local governments, as well ...
A data scientist uses data to understand and explain the phenomena around them, and help organizations make better decisions. Working as a data scientist can be intellectually challenging, analytically satisfying, and put you at the forefront of new technological advances. Data scientists have become more common and in demand, as big data ...
They can be linked with Komodo's Healthcare Map, an anonymized dataset on more than 330 million Americans, to drive nuanced research inquiries. The latest partners to be announced are GeneDx ...
Big Data Analytics (BDA) usage in the industry has been increased markedly in recent years. As a data-driven tool to facilitate informed decision-making, the need for BDA capability in organizations is recognized, but few studies have communicated an understanding of BDA capabilities in a way that can enhance our theoretical knowledge of using BDA in the organizational domain.
The number of people experiencing poor health and early death caused by metabolism-related risk factors such as high blood pressure, high blood sugar, and high BMI has increased by 50% since 2000, reveals new global study. Last updated. May 16, 2024. News release.
Unstructured data, on the other hand, is stored as media files or NoSQL databases, which require more space. It can be stored in data lakes, which makes it difficult to scale. Uses: Structured data is used in machine learning (ML) and drives its algorithms, whereas unstructured data is used in natural language processing (NLP) and text mining.
Now, as the pace of efficiency gains in electricity use slows and the AI revolution gathers steam, Goldman Sachs Research estimates that data center power demand will grow 160% by 2030. At present, data centers worldwide consume 1-2% of overall power, but this percentage will likely rise to 3-4% by the end of the decade.
Artificial intelligence (AI) is a broad field encompassing the science of making computers behave in a manner that simulates human behaviour/intelligence using data and algorithms. Big data refers to information and data that is large and complex in volume, velocity and variety, and as such, requires specialized tools, techniques and ...
Honorable Mentions. B ig or Small, It's All in Your Head: Visuo-Haptic Illusion of Size-Change Using Finger-Repositioning Myung Jin Kim, Eyal Ofek, Michel Pahud, Mike J. Sinclair, Andrea Bianchi This research introduces a fixed-sized VR controller that uses finger repositioning to create a visuo-haptic illusion of dynamic size changes in handheld virtual objects, allowing users to perceive ...
09:13 AM ET 05/20/2024. Broadcom ( AVGO) on Monday announced a new portfolio of scalable, high-performance, low-power networking and switching products to resolve connectivity bottlenecks in data ...