Determinants of safety outcomes and performance: A systematic literature review of research in four high-risk industries
Affiliations.
- 1 Department of Communication Science, University of Twente, The Netherlands. Electronic address: [email protected].
- 2 Department of Communication Science, University of Twente, The Netherlands.
- PMID: 28882259
- DOI: 10.1016/j.jsr.2017.06.009
Introduction: In spite of increasing governmental and organizational efforts, organizations still struggle to improve the safety of their employees as evidenced by the yearly 2.3 million work-related deaths worldwide. Occupational safety research is scattered and inaccessible, especially for practitioners. Through systematically reviewing the safety literature, this study aims to provide a comprehensive overview of behavioral and circumstantial factors that endanger or support employee safety.
Method: A broad search on occupational safety literature using four online bibliographical databases yielded 27.527 articles. Through a systematic reviewing process 176 online articles were identified that met the inclusion criteria (e.g., original peer-reviewed research; conducted in selected high-risk industries; published between 1980-2016). Variables and the nature of their interrelationships (i.e., positive, negative, or nonsignificant) were extracted, and then grouped and classified through a process of bottom-up coding.
Results: The results indicate that safety outcomes and performance prevail as dependent research areas, dependent on variables related to management & colleagues, work(place) characteristics & circumstances, employee demographics, climate & culture, and external factors. Consensus was found for five variables related to safety outcomes and seven variables related to performance, while there is debate about 31 other relationships. Last, 21 variables related to safety outcomes and performance appear understudied.
Conclusions: The majority of safety research has focused on addressing negative safety outcomes and performance through variables related to others within the organization, the work(place) itself, employee demographics, and-to a lesser extent-climate & culture and external factors.
Practical applications: This systematic literature review provides both scientists and safety practitioners an overview of the (under)studied behavioral and circumstantial factors related to occupational safety behavior. Scientists could use this overview to study gaps, and validate or falsify relationships. Safety practitioners could use the insights to evaluate organizational safety policies, and to further development of safety interventions.
Keywords: Demographics; Employees; Occupational safety; Safety performance; Workplace circumstances.
Copyright © 2017 National Safety Council and Elsevier Ltd. All rights reserved.
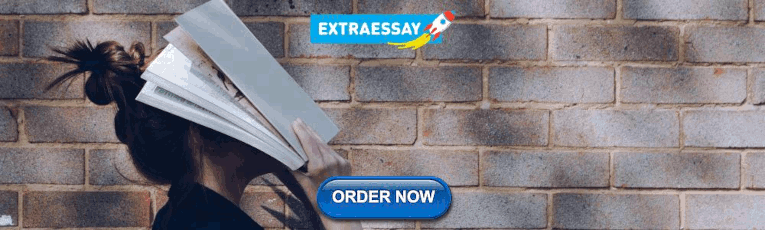
Publication types
- Industry / classification
- Industry / statistics & numerical data*
- Occupational Health / statistics & numerical data*
- Workplace / statistics & numerical data*
Academia.edu no longer supports Internet Explorer.
To browse Academia.edu and the wider internet faster and more securely, please take a few seconds to upgrade your browser .
Enter the email address you signed up with and we'll email you a reset link.
- We're Hiring!
- Help Center
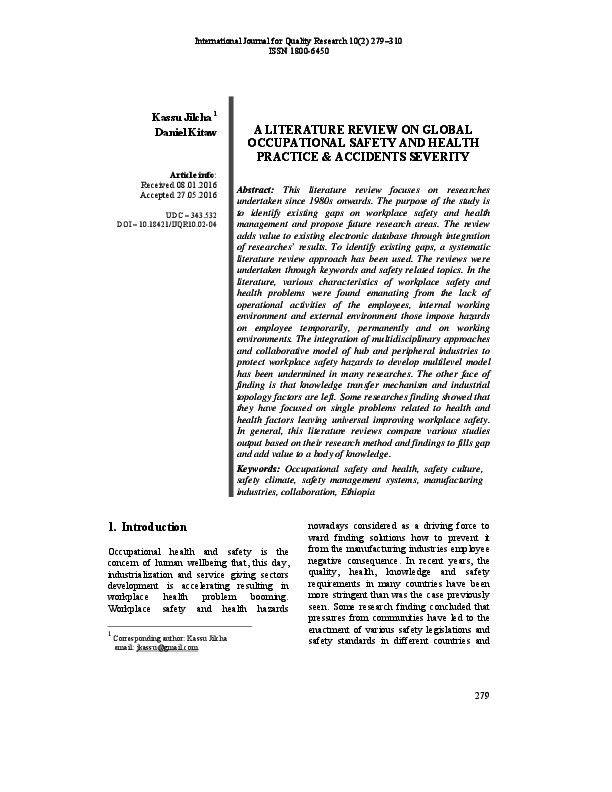
A LITERATURE REVIEW ON GLOBAL OCCUPATIONAL SAFETY AND HEALTH PRACTICE & ACCIDENTS SEVERITY

This literature review focuses on researches undertaken since 1980s onwards. The purpose of the study is to identify existing gaps on workplace safety and health management and propose future research areas. The review adds value to existing electronic database through integration of researches' results. To identify existing gaps, a systematic literature review approach has been used. The reviews were undertaken through keywords and safety related topics. In the literature, various characteristics of workplace safety and health problems were found emanating from the lack of operational activities of the employees, internal working environment and external environment those impose hazards on employee temporarily, permanently and on working environments. The integration of multidisciplinary approaches and collaborative model of hub and peripheral industries to protect workplace safety hazards to develop multilevel model has been undermined in many researches. The other face of finding is that knowledge transfer mechanism and industrial topology factors are left. Some researches finding showed that they have focused on single problems related to health and health factors leaving universal improving workplace safety. In general, this literature reviews compare various studies output based on their research method and findings to fills gap and add value to a body of knowledge.
Related Papers
kassu sileyew
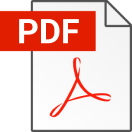
Kassu Jilcha
Sustainable development is not thought in a box without development pillars. Previous researchers put these pillars as economy, social and environment. Upon improving these three pillars, sustainable development becomes trustworthy in relation to workplace safety and health improvement. However, the researchers' findings have drawback in considering existing three pillars. Previous researches neglected to incorporate the other three pillars of sustainable development which are culture, political and technological factors. Having these pillars, sustainable development can also be guaranteed by considering workplace safety and health innovation for all internal and external entities engage at work. This is because of the implementation the pillars reduce the working environment accidents and disease. Hence, this research focuses on the workplace safety & health innovation, introducing new pillars for sustainable development, their impact on sustainable developments and indicating the three pillars future research areas. Methods like literature review, interviewing employees and observation of industries were used. There were few researches found on how sustainable development affected by workplace safety and health innovation approaches. However, this literature more focused on the relationship workplace innovation and sustainable development share in common. The other finding in this study showed that the innovation of workplace safety and health brings sustainable development through healthy people , safer workplace, reduced cost of accidents, controlled environment, managed workplace accidents and improved workplace safety knowledge. The researchers have also attempted to forward roads toward sustainable development through occupational safety and health innovation and improvement approaches.
This paper attempts to investigate the influence of lean thinking on occupational safety and health problems improvement in manufacturing industries and it also to show how occupational safety and health severely hurts manufacturing industries productivity. Nowadays, developing countries are focused on Technology Transfer and be engaged in developing their manufacturing industries so as to compete globally and for their economic growth. While expanding the development of their manufacturing industries, they are also importing new technologies with which they are not familiar and furthermore, neglecting workplace safety and health hazards impact on productivity and workers well-being. Due to these reasons, much productive working time is lost and high costs have been incurred. To highlight the impact of lean on workplace safety and health, this study was conducted by reviewing recent state-of-the-art literature and taking into consideration secondary data records from Ethiopian Ministry of Labor and Social Affair (MOLSA) for simple illustrative example. Findings from the literatures showed that there is less attention and consideration of lean workplace safety and health in manufacturing industries.
Kassu Sileyew , Kassu Jilcha
nnovation is an important science for the sustainable development of any country. Workplace innovation brings radical change in the workers’ environment, thereby enhancing the profitability of companies. However, most research and discussion of innovations are focussed on product development and/or process improvement, disregarding workplace and service innovation. Hence, this study aims to identify gaps that exist in previous studies to recommend further research areas related to workplace innovation influences on Occupational Safety and Health (OSH) interventions. The research was carried out through extensive literature reviews. It is evident that the influence of workplace innovation has been neglected in many studies despite the significance for workplace safety and health. Findings from the literature review revealed that workplace innovation has not been properly differentiated from new product development innovation and there are few cases of research carried out on the workplace innovation in particular. Thus, this research finding identified gaps in workplace innovation (non-technical innovation) such as workplace organisation, workplace layout, human resource management, top management changes, workers’ participation, policy and training. Hence, the workplace innovation influence on OSH indicates further research that can be conducted.
Ibne Saad Tarif Noor
The RMG industry is the core function of our country’s economy. So the extension of the further study is the assertion of time to put forward the significant changes for eliminating ceaseless suspicion in this sector. Currently, this industry is facing the hectic situation and it is necessary to conduct comprehensive study and analysis and to explore the accomplishment to flinch the situation. After the casualties of the building collapse of Rana Plaza and the fire accident of Tazreen Fashion Ltd., this industry is facing tremendous intimidation on the issue of health and safety of the workers. These accidents have added further vindicate to initiate and condiment research concentrating on health and safety issue. In addition, there is a deficiency of citable study on the effectiveness of occupational health and safety management of the RMG workers in the country. This study will perceive to be a valuable addition to the existing literature relating to the occupational health and safety management of the RMG sector.
Madhav Prasad Koirala
— Workers' health and safety is an important asset , it enhances the productivity, efficiency, quality of every industry if well maintained. Construction material producer are vital industries from where supplying the demand of emerging construction materials to infrastructure and habitat projects as per projects' need. These industries employing lot of workers but they are not being worry about health and safety. Different convention of ILO as well as WHO have emphasizes to respond the issues related workers health and safety. Nepal had not yet ratified ILO convention No. 155 on occupational safety and health. Therefore lot of death, fatalities are suffered due to health and safety. It is important asset of workers that must be taken seriously by all stake holders as well as the nation. To make civilize society, all should be aware about every one's health and safety that too, workers health of safety is very important issues in every industry.
RELATED PAPERS
Cogent Engineering
Eshetie Berhan
Yoseph Zelalem
Faslu aslam
Nicholas Petrovski
International Journal for Research in Applied Science and Engineering Technology IJRASET
IJRASET Publication
Seth Oppong, PhD
Sinah Seoke
Asian Social Science
aina amirah
The Academic Research Community Publication
IEREK press
zuriah abdul rahman
International Journal of Research in Business and Social Science (2147- 4478)
peter mwaura
Ibrahim Oluoch
Occupational Health And Safety
Anatoly Dufan
Accident Analysis & Prevention
Sidney Dekker
Esmael Seid Yimer
PATRICK A K W A S I A N A M U A H MENSAH
Journal of Safety Studies
Adel Zakaria
Proceedings of the 1st Aceh Global Conference (AGC 2018)
WAN IZATUL ASMA WAN TALAAT
International Journal of Occupational and Environmental Safety
Seppo Väyrynen
Emmanuel Adinyira
Michael Duah Obeng , Emmanuel Adinyira , Thomas Mills
Hanida Abdul Aziz
Inviolata Wesonga
Kwai Sum Ling
Hasan As' Adi
Arianto Wibowo
Iranian Journal of health, Safety and environment
Shuvo Hassan
Phineas Haluse
Gerard Zwetsloot
Dr Ciarán McAleenan
Marius Dumitrascu
American Journal of Health, Medicine and Nursing Practice
Dr. Kimeli M. Chirchir
Joydeep Majumder
IOP Conference Series: Materials Science and Engineering
Tijjani Abdullahi
RELATED TOPICS
- We're Hiring!
- Help Center
- Find new research papers in:
- Health Sciences
- Earth Sciences
- Cognitive Science
- Mathematics
- Computer Science
- Academia ©2024
- Open access
- Published: 09 May 2024
A systematic review of workplace triggers of emotions in the healthcare environment, the emotions experienced, and the impact on patient safety
- Raabia Sattar 1 ,
- Rebecca Lawton 1 ,
- Gillian Janes 2 ,
- Mai Elshehaly 3 ,
- Jane Heyhoe 1 ,
- Isabel Hague 1 &
- Chloe Grindey 1
BMC Health Services Research volume 24 , Article number: 603 ( 2024 ) Cite this article
311 Accesses
19 Altmetric
Metrics details
Healthcare staff deliver patient care in emotionally charged settings and experience a wide range of emotions as part of their work. These emotions and emotional contexts can impact the quality and safety of care. Despite the growing acknowledgement of the important role of emotion, we know very little about what triggers emotion within healthcare environments or the impact this has on patient safety.
To systematically review studies to explore the workplace triggers of emotions within the healthcare environment, the emotions experienced in response to these triggers, and the impact of triggers and emotions on patient safety.
Following the Preferred Reporting Items for Systematic Reviews and Meta-Analyses guidelines, four electronic databases were searched (MEDLINE, PsychInfo, Scopus, and CINAHL) to identify relevant literature. Studies were then selected and data synthesized in two stages. A quality assessment of the included studies at stage 2 was undertaken.
In stage 1 , 90 studies were included from which seven categories of triggers of emotions in the healthcare work environment were identified, namely: patient and family factors, patient safety events and their repercussions, workplace toxicity, traumatic events, work overload, team working and lack of supervisory support. Specific emotions experienced in response to these triggers (e.g., frustration, guilt, anxiety) were then categorised into four types: immediate, feeling states, reflective, and longer-term emotional sequelae. In stage 2 , 13 studies that explored the impact of triggers or emotions on patient safety processes/outcomes were included.
The various triggers of emotion and the types of emotion experienced that have been identified in this review can be used as a framework for further work examining the role of emotion in patient safety. The findings from this review suggest that certain types of emotions (including fear, anger, and guilt) were more frequently experienced in response to particular categories of triggers and that healthcare staff's experiences of negative emotions can have negative effects on patient care, and ultimately, patient safety. This provides a basis for developing and tailoring strategies, interventions, and support mechanisms for dealing with and regulating emotions in the healthcare work environment.
Peer Review reports
Healthcare is delivered in emotionally charged settings [ 1 ]. Worried patients present with complex health issues, and anxious relatives need information and support, and safe care is reliant on clinical judgement and effective multi-disciplinary teamwork within a time-pressured resource-limited, complex system. Working in this environment, healthcare staff experience a range of emotions (e.g., anxiety, anger, joy, sadness, pride, and guilt) which can impact the safety of the care delivered [ 1 , 2 , 3 , 4 , 5 ]. Clinical judgement often involves weighing up risk based on incomplete information and uncertain outcomes. Research outside healthcare [ 6 , 7 , 8 , 9 ] suggests that if, while making these decisions, healthcare staff experience strong emotions, this can influence their decisions and behavior.
Research focusing on the role of emotion in patient safety is still limited [ 1 , 2 , 10 ] and fragmented [ 11 ]. This may in part be because emotion research is complex. For example, the experience and influence of emotion can be approached and interpreted from a range of perspectives including cognitive and social psychology, cognitive neuroscience, and sociology. There is also a lack of consensus on what is meant by ‘emotion’. In decision-making research, ‘emotion’ has been distinguished from ‘affect’ [ 11 ]. In response to stimuli or situations, ‘emotion’ is viewed as a slower, more reflective process, whilst ‘affect’ is an instantaneous and automatic reaction. Other research has focused on identifying and examining different types of affect, such as ‘anticipatory affect’—an immediate, strong visceral state in response to stimuli e.g. anger (Knutson, 2008) and ‘anticipated affect’ – considering how current actions might make you feel in the future e.g. regret [ 12 ].
Research often lacks a clear distinction between the different types of feeling states being examined, and as such, it is difficult to build robust evidence of the processes involved and the role they each play in judgement and associated behaviour [ 11 ]. Furthermore, the emotions experienced by healthcare staff can be both positive and negative and can influence the delivery of safe care in positive and negative ways. Until more recently, the focus has tended to be on the impact of negative emotions, including their role in diagnostic accuracy [ 13 , 14 ], time spent on history taking, examinations, and treatment decisions [ 15 , 16 ], and the instigation of verbal checking during procedures [ 4 ]. More attention is now being given to the role of positive emotions in the workplace such as their effect on reasoning [ 17 ], and engagement and teamwork [ 18 ].
There are many potential triggers (e.g. physical, circumstantial, tangible, and psycho-social aspects of the immediate clinical work environment and the broader organisation) that generate a feeling state via reactions or interactions of emotion in the workplace. Research exploring some of the triggers of emotions within a healthcare environment has found that involvement in care that has gone wrong [ 4 ], and interactions with patients can elicit negative emotions [ 13 , 14 , 15 , 16 ] and that triggers of emotion can be at a clinical, hospital and system level [ 15 ]. Only a limited number of these studies have also explored how the emotions experienced by healthcare staff impact patient care [ 15 , 16 ]. While emotion has a direct effect on patient care, it can also indirectly influence patient safety. Burnout, sickness absence, and turnover are impacted by emotion [ 19 , 20 , 21 ] and, in turn, are associated with healthcare organisations’ ability to provide safe care [ 21 , 22 , 23 ]. Due to the multifaceted approaches to research in this area, it is currently unclear what contexts and settings elicit emotions in healthcare staff, how these make healthcare staff feel, and the influence these feelings may have on decisions and actions relevant to providing safe patient care. There is therefore a need to synthesise the current evidence to help develop an in-depth understanding of the triggers of emotions experienced by healthcare staff in the work environment, the emotions experienced and the impact these may have on patient safety.
The protocol was pre-registered on Prospero (ID: CRD42021298970).
This systematic review aimed to identify gaps in the evidence by answering these research questions:
What triggers emotions in the healthcare work environment?
What are the emotions experienced in response to these triggers?
Are certain emotions more often experienced in the context of particular triggers?
What impact do different triggers/ emotions have on patient safety processes and outcomes?
Search strategy and databases
Four electronic databases (MEDLINE, PsychInfo, Scopus, and CINAHL)( were systematically searched in March 2020 and updated in January 2022. Only studies published since 2000 were sought as this was when the Institute of Medicine’s seminal report, ‘To Err is Human’’ [ 24 ] was published promoting a widespread focus on patient safety. The search strategy had three main foci (patient safety, emotions, and healthcare staff). Previous systematic reviews examining any of these topics in combination; patient safety [ 25 ] and healthcare staff [ 26 ]were used to guide search strategy development. As a foundation to develop the search terms in relation to emotions, the six basic emotions (fear, anger, joy, sadness, disgust, and surprise) described by Ekman [ 27 ] were included, with synonyms for emotion. This resulted in a search strategy that combined all three concepts (Available in Appendix 1 ). The reference lists of all included studies were hand-searched.
Eligibility criteria
Studies were included if they were: published post-2000, original empirical research (either quantitative, qualitative, or mixed-methods), published in English, conducted in any healthcare environment, and included healthcare staff as participants. Studies were excluded if they; focused on healthcare staff’s non-work related emotions, included healthcare students/staff who were not involved in direct patient care (e.g. administrative staff), or if the primary focus was on longer-term emotional states (e.g. burnout and emotional exhaustion) with no reference to specific emotions.
This review had two stages:
Stage 1: The first stage addressed the first three research questions and identified studies focused on triggers of emotions in the healthcare work environment and the specific emotions experienced by healthcare staff in response to these.
Stage 2: The second stage examined the fourth research question and identified the impact of triggers and/or emotions on patient safety outcomes and processes. The studies included in stage 1 were further screened and considered at this stage if they included either (i.) triggers of emotions and their relationship with patient safety, (ii.) emotions experienced and their relationship with patient safety outcomes or processes (iii.) triggers, emotions and the relationship with patient safety outcomes or processes.
Study selection
PRISMA guidelines [ 28 ] for study selection were followed. The study selection process is described below. Throughout each stage, all decisions and any uncertainty or discrepancies were discussed by the review team to achieve consensus.
Stage 1: Title and abstract then full-text screening, was conducted by IH & CG independently and then discussed together. RL reviewed a random 10% at the abstract review stage and all included full-text articles.
Stage 2: RS independently conducted abstract and title screening for all included studies. A random 10% of these were each independently screened by two reviewers (JH&RL). Full texts were obtained for all studies deemed potentially eligible for inclusion. All full texts were screened by RS. JH &RL double-screened half each. A final set of studies meeting all the eligibility criteria was identified for data extraction.
Assessment of the methodological quality of included studies was carried out using the 16-item quality assessment tool (QuADS) [ 25 ] which is appropriate for studies using different methodological approaches. Quality assessment was undertaken independently for two studies by three reviewers (RS, GJ&JH) and scores were discussed to check for consistency. RS&GJ completed a quality assessment for the remaining studies and discussed scores to check for consistency. No studies were discarded based on low scoring.
Data extraction
Stage 1: A data extraction form developed in Microsoft Excel by IH&CG and agreed with the wider review team was used to extract: the study title, triggers of emotions in the healthcare work environment, and emotions experienced in response to these triggers. Two reviewers extracted these data (IH&CG), conferring at intervals throughout the extraction process to ensure consistency. Due to the large number of studies at this stage and our aim to take a broader approach to explore triggers and the associated emotions, we did not extract data related to study characteristics. We categorised both the types of triggers and emotions (drawing on existing theory and wider team expertise) to advance knowledge by providing an initial framework for further testing. The detailed process for categorisation of the emotions and triggers is described in supplementary Appendix 2 .
Stage 2: A data extraction form developed and agreed upon by authors was used to extract: information on the study population, setting, design and methods used, key findings, conclusions, recommendations, triggers of emotions, emotions experienced, and impact on patient safety. CG&IH completed data extraction for included studies. This was cross-checked by RS and discussed with all reviewers.
Categorising the patient safety outcomes and processes
The wide range of patient safety processes and outcomes ( n = 50) from the included studies, meant it was necessary to reduce the data. Therefore, categories of outcomes were developed to allow the relationship between triggers/emotions and patient safety to be explored. The first step in the categorisation process involved a team of 8 patient safety researchers using a sorting process in which they were provided with 50 cards each describing a patient safety process/outcome extracted from the studies Working independently they grouped these 50 cards and gave each group a title. A large group discussion with all 8 patient safety researchers followed this, resulting in 7 categories. We then presented these categories and the items each contained, to a large group of patient safety researchers, healthcare staff, and patients ( n = 16), at an inter-disciplinary meeting. This resulted in a final set of five categories representing patient safety processes: altered interaction with patients, disengagement with the job, negative consequences for work performance, defensive practice, being more cautious, negative impact on team relationships, and reduced staff confidence (see appendix 2 for further detail) and the sixth, patient safety outcomes.
Quality assessment
There was a very high level of agreement between RS & GJ regarding the quality assessment. The quality of studies was variable, with total scores ranging from 79 to 48% across the studies. There was limited discussion of relevant theories related to emotions and patient safety, and few studies provided a rationale for the choice of data collection tools. There was also limited evidence to suggest stakeholders had been considered in the research design and limited – or often no justification for analytical methods used. A detailed quality assessment table is available in Appendix 3 .
After duplicates were removed; the search resulted in 8,432 articles for initial review which were downloaded into the reference management software Endnote (see PRISMA flow diagram in (Fig. 1 ). Stage 1: 90 studies met the inclusion criteria, investigating triggers of emotions in the healthcare work environment and the emotions experienced by healthcare staff in response to these.
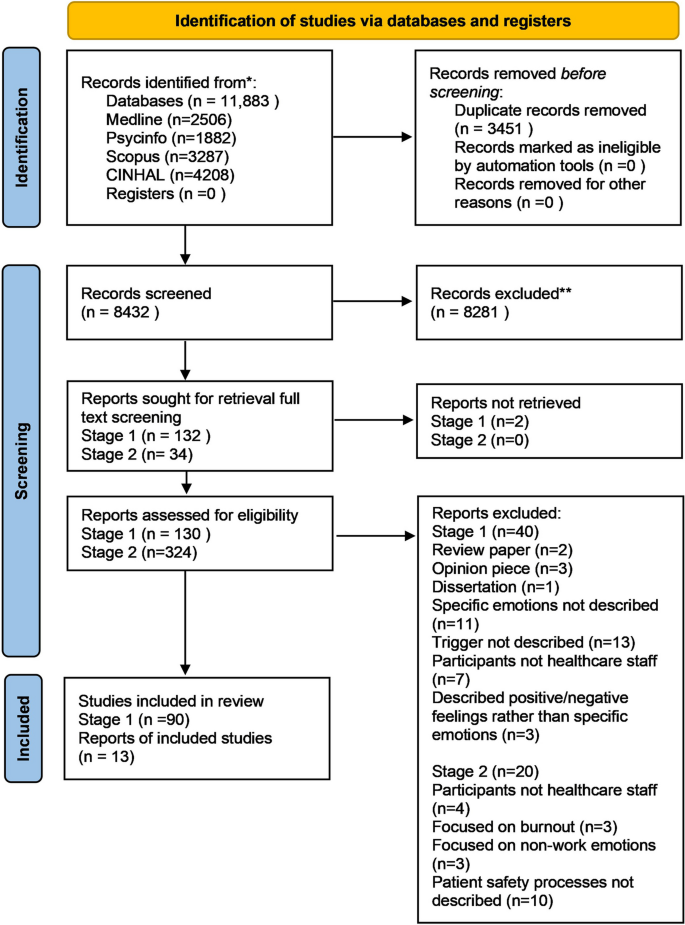
PRISMA flow diagram
Research question 1: What triggers emotions in the healthcare work environment?
The following categories of triggers were identified:
(1) Patient and family factors ( included patient aggression, challenging patient behaviours, patient violence, patient hostility, and interactions with patients family)*
(2) Patient safety events and their repercussions (including adverse events, errors, medical errors, and surgical complications)*
(3) Workplace toxicity (including workplace bullying, and staff hostility)*
(4) Traumatic events with negative outcomes for patients (including patient deaths/suicide, patient deterioration, and critical incidents).
(5) Work overload (including work pressures and poor staffing levels).
(6) Team working and lack of supervisory support (including teamwork and the lack of appropriate managerial support).
*The most common triggers investigated and reported in the literature
Research question 2: What are the emotions experienced in response to these triggers?
In response to the triggers described above, healthcare staff experienced four main types ofemotions:
1. Immediate: an instantaneous, visceral emotional response to a trigger ( e.g. fear, anxiety, anger, comfort, satisfaction, joy ).
2. Feeling states: short-lived, more mindful and conscious cognitive-based responses to a trigger (e.g. include feeling disoriented, confused, helpless, inadequate, alone).
3. Reflective/self-conscious: Mindful and conscious cognitive-based response after exposure to a trigger and following time to reflect on how others may perceive them (e.g. moral distress, guilt, pride).
4. Sequelae: chronic and longer-term mental health states that arise as a result of repeated exposure to a trigger and experiencing the emotions in response to that trigger over time (e.g. chronic depression, fatigue, distress, PTSD symptoms).
Research question 3: Are certain emotions more often experienced in the context of particular triggers?
The frequency of the emotions experienced across the studies in response to the categories of triggers is illustrated using a heat map (Fig. 2 ) developed by a data visualisation expert (MA). Below is a summary of the most frequently experienced emotions by healthcare staff in response to the categories of triggers across the studies.
Patient and family factors: Immediate emotional responses including most commonly anger, frustration, etc.
Patient safety events & their repercussions: Reflective/self–conscious emotions including guilt and regret
Workplace toxicity: Immediate emotional responses including fear and anxiety
Traumatic events with negative outcomes for patients: Reflective/self–conscious emotions including guilt and regret
Work overload: Immediate emotional responses including anxiety and worry
Team working & supervisory support: Immediate emotional responses including anxiety and worry
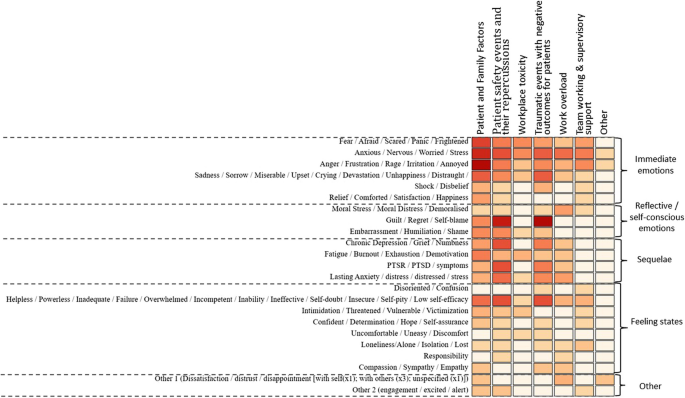
A heat map displaying the triggers of emotions experienced by healthcare staff and the emotions experienced in response to these. (The darker the colour on the heat map represents a higher frequency of that emotion being experienced across the studies)
Stage 2 Research question 4. What impact do different triggers/ emotions have on patient safety processes or outcomes?
Thirteen publications [ 15 , 16 , 30 , 31 , 32 , 33 , 34 , 35 , 36 , 37 , 38 , 39 , 40 ] addressed this research question and were included at this stage.
All 13 studies [ 15 , 16 , 30 , 31 , 32 , 33 , 34 , 35 , 36 , 37 , 38 , 39 , 40 ] described the following patient safety processes/outcomes as being impacted by either the triggers of emotions or the emotions experienced; altered interaction with patients, disengagement with the job, negative consequences for work performance, defensive practice, being more cautious, negative impact on team relationships and reduced staff confidence and patient safety outcomes.
Depending on the nature of the studies, some explored only one of the patient safety processes/outcomes, whereas others focused on several. Eight studies used quantitative methods [ 30 , 31 , 32 , 33 , 34 , 35 , 36 , 37 ], three qualitative methods [ 15 , 38 , 39 ], and two mixed-methods designs [ 16 , 40 ] to explore these relationships. Twelve studies were conducted in hospital settings [ 15 , 16 , 30 , 31 , 32 , 33 , 34 , 35 , 36 , 37 , 38 , 39 ], and one was conducted in hospital and community healthcare settings [ 40 ].
The impact of triggers of emotion on patient safety processes or outcomes
Of the 13 studies [ 15 , 16 , 30 , 31 , 32 , 33 , 34 , 35 , 36 , 37 , 38 , 39 , 40 ], 10 [ 30 , 31 , 32 , 33 , 34 , 35 , 36 , 38 , 39 , 40 ] included an exploration of or commented on the impact of triggers of emotion on patient safety processes/outcomes. None had a direct focus on exploring the relationship between the triggers and patient safety processes/outcomes; rather some stated this as one of multiple aims, whereas others reported any associations as part of the broader study findings. The relationship between specific triggers and patient safety processes/outcomes is displayed in Table 1 .
The most commonly described patient safety processes/outcomes following exposure to the triggers were 'disengagement with the job' [ 30 , 31 , 35 , 40 , 41 ] and ‘being more cautious’ [ 31 , 32 , 33 , 34 , 35 ]. There was increased disengagement with the job after experiencing workplace bullying, medical errors, and workplace violence. This included dissatisfaction and a desire to change jobs or leave healthcare practice. Involvement in medical errors, surgical complications, and workplace bullying also resulted in staff being more cautious. For example, they reported paying more attention to detail, keeping better patient records, and increased information-seeking from colleagues.
Within four studies, triggers were described as having a ‘negative impact on team relationships’ [ 30 , 34 , 36 , 39 ] where being exposed to workplace bullying resulted in communication problems amongst staff and conflicts with co-workers. In one case being involved in a patient safety incident resulted in staff feeling uncomfortable within their team [ 36 ]. Workplace bullying and being involved in a patient safety incident also resulted in ‘Negative consequences for work performance’ in four studies [ 30 , 34 , 36 , 40 ] which included delays in care delivery and being unable to provide quality care.
Exposure to triggers was also linked to ‘defensive practice’ [ 29 , 32 , 36 ]. There was an increase in defensive practice (e.g. ordering more tests, keeping errors to self, and avoiding risks) as a result of triggers such as workplace bullying and medical errors. 'Patient safety outcomes' were only described in one study, where it was perceived that there may be an increased risk of patient safety incidents such as increased medical errors, patient falls, adverse events, or patient mortality as a result of experiencing workplace bullying [ 30 ].
The impact of emotions on patient safety processes or outcomes
Only three studies [ 15 , 16 , 37 ] included an exploration of the impact of emotions on either patient safety processes [ 15 , 16 ] or outcomes [ 37 ]. These studies directly explored the relationship between the emotions experienced in response to triggers and patient safety processes/outcomes (see Table 2 ).
In response to the emotions experienced by healthcare staff, ‘negative consequences for work performance’ were described[15, 16} as staff feeling unable to provide quality care or a delay or failure to provide appropriate examination/treatment was reported. Emotions also influenced defensive practices [ 16 ] such as risk avoidance, and provision of unnecessary treatment, and emotions were described as an influencing factor for overprescribing. Emotions were also found to influence physical restraint in mental health settings, where a positive correlation was reported between staff experiencing anger (as a result of patient aggression) and the approval of physical restraint [ 37 ].
The type of patients also influenced the emotions experienced by staff, which in turn altered the interaction with patients [ 15 , 16 ]. Isbell et al. [ 16 ] found that encounters with angry and mental health patients elicited highly negative emotions such as fear and frustration, where staff spent less time with the patient and acted less compassionately. Increased interaction including expediting patient care and spending more time with the patient was associated with encounters with positive patients who elicited positive emotions (happiness, satisfaction)in staff. Isbell et al. [ 15 ] also found that patients with psychiatric conditions elicited negative emotions, which resulted in reduced patient interaction and potential for diagnostic error.
Whilst these studies [ 15 , 16 , 37 ] highlight that emotions may impact patient safety processes and/or outcomes, it was not always possible to ascertain the impact of specific emotions. Studies by Isbell et al. [ 15 , 16 ] illustrate how negative emotions elicited by patients have a negative impact on patient safety processes, whereas positive emotions resulting from patient behaviours have the potential to enhance patient care. However, it is difficult to disentangle the effect of specific emotions, due to a lack of evidence regarding the link between individual emotions and patient safety processes and/or outcomes as studies have not attempted to explore this. Only Jalil et al. [ 37 ] focused specifically on anger as an emotion and its impact on restraint practices, where higher levels of anger were correlated with greater approval of restraint of mental health patients.
Summary of main aims and findings
This review has identified and categorised the triggers of emotions in the healthcare work environment and the types of emotions experienced by healthcare staff in response to those triggers. It has also established the types of emotions more often experienced in the context of particular triggers, and the impact that different triggers and emotions may have on patient safety processes or outcomes. The most frequently reported triggers within the literature were 'patient and family factors', 'patient safety events and their repercussions', and ‘workplace toxicity’ , and the most frequently cited emotions were ‘ anger, frustration, rage, irritation, annoyance’ and ‘ guilt, regret and self-blame’ . These emotions were all negative in nature, which may reflect a bias in the research literature.
The studies that focused on the triggers did not directly set out to assess the impact of triggers of emotions on patient safety processes or outcomes, but the reporting of this link in study findings did enable knowledge to be gained about this. Studies that did focus on emotions and patient safety directly explored the relationship between the emotions experienced in response to triggers and patient safety processes and outcomes. Previous literature [ 41 , 42 , 43 ] supports the link between the categories of patient safety processes identified within this review ( including reduced staff confidence, disengagement with the job, and defensive practice ) and patient care and or/patient safety, suggesting these processes may serve as mechanisms to influence patient safety. Only three studies were identified that focused on the impact of emotions experienced by healthcare staff within the work environment on patient safety processes/outcomes [ 15 , 16 , 37 ]. These studies highlight that a majority of emotional responses experienced by healthcare staff are negative and have the potential to result in negative work performance ( including being unable to provide quality care ), increased defensive practice, and negative patient safety outcomes (increased approval of physical restraint ). In only one study [ 15 ], positive emotions were reported which resulted in positive outcomes including expediting patient care and spending more time with the patient.
The findings of this review support previous calls to acknowledge the importance of emotions and their impact on safe care [ 1 , 2 , 4 , 5 , 44 ], however, research in this area is still limited and fragmented [ 15 , 16 ]. Except for one study [ 41 ], it was not possible to ascertain the association between specific emotions and patient safety processes, and even for this study (a cross-sectional survey), causal relationships were not demonstrated. Nevertheless, the findings do suggest that negative emotions elicited by patients within healthcare staff have a negative impact on the described patient safety processes and positive emotions have a positive impact on these processes. Earlier work by Croskerry et al. [ 10 ] highlighted the importance of bringing attention to the notion that healthcare providers are not immune to emotional influences, and must therefore focus on not allowing their emotional experiences to negatively influence the care they provide.
Within this review, patients were described as the most common trigger eliciting emotions and subsequently influencing patient safety processes and outcomes. Although one study did also identify hospital and system-level factors as triggers [ 15 ], these were not explored in patient safety processes. As well as patients, many other factors within the healthcare work environment were identified in the first stage of this review as influencing the emotions staff experienced. However, how emotional responses to such triggers affect patient safety processes and outcomes is currently unclear and warrants further research. The studies reviewed here focused on the intrapersonal effects of emotion. Researchers have recently highlighted the need for further work to understand the social aspects of emotion [ 45 , 46 , 47 ]. The Emotions as Social Information (EASI) model [ 45 ] posits that many of our decisions and actions cannot be explained solely by individual thought processes, but are often due to social interaction which involves observing and responding to the emotional displays of others, providing a potentially useful framework for further exploration.
Workplace violence and patient aggression were identified in this review as triggers of emotions in the healthcare work environment. Research evidence suggests that gender plays a role in determining recipients who are subjected to workplace violence and the type of violence they may experience. Male healthcare staff report experiencing a higher prevalence of workplace violence compared to their female counterparts [ 48 , 49 ]. Gender influenced the types of violence experienced by healthcare staff, where in general, female healthcare staff experienced more verbal violence, and male healthcare staff experienced more physical violence [ 48 ]. Different risk factors for workplace violence have been reported for males and females. For male healthcare staff, lower income levels and managers were at a higher risk of workplace violence, whereas longer working hours were associated with a higher risk of workplace violence among female healthcare staff [ 49 ].
As experiencing workplace violence and patient aggression have been found to have a negative impact on the delivery of patient care, this is a topic area that warrants further research. The majority of emotions identified in response to the triggers in this review were negative in nature. Within the few studies where positive emotions were mentioned, experiencing these as a result of a positive patient encounter was associated with increased interaction with patients, where healthcare staff perceived they were more engaged and provided expedited care [ 15 , 16 ]. This finding is congruent with limited previous Research that suggests positive emotions may improve patient safety and patient care; positive affect led medical students to identify lung cancer in patients more quickly [ 50 ] and resulted in correctly diagnosing patients with liver disease sooner [ 51 ]. However, positive emotional responses may also have the opposite effect e.g. over-testing and over-treating patients, or reducing staff belief that the patient has a serious illness, resulting in adverse outcomes [ 16 ]. Greater understanding is required to articulate conditions and triggers of positive emotions and when these might support patient safety or cause harm [ 44 ].
Limitations
There was heterogeneity within the included studies and the primary aim of most studies was not to answer the research questions posed here. To answer our research questions, it was necessary to include articles where the study aims addressed only one of the concepts of interest or where only limited associations between triggers of emotion or the emotions experienced in response to triggers and patient safety processes or outcomes were made. Moreover, it is important to recognise that there is likely to be some bias in the research literature, meaning that the triggers of emotion we identified from the current published research and the emotions experienced in response to these cannot be assumed to accurately represent the routine experiences of healthcare staff. Also worthy of note is that the majority of studies focus on negative triggers of emotions or the negative emotions experienced which may also lead to reporting bias. We acknowledge that we did not search studies before the year 2000.
Implications for future research and practice
The triggers of emotion and types of emotion experienced that have been identified in this review can be used as a framework for further work examining the role of emotion in patient safety. Developing validated measures of the triggers of emotions, and the types of emotions experienced by healthcare staff in the work environment will facilitate this and is urgently needed. The findings also suggest that particular types of emotion were more frequently experienced in response to particular categories of triggers and that healthcare staff’s experiences of negative emotions have negative effects on patient care and ultimately, patient safety. This provides a basis for developing and tailoring strategies, interventions, and support mechanisms for dealing with either short-term or long-term consequences, and regulation of emotions in the healthcare work environment. For example, healthcare staff can be offered some time out from their clinical duties to take a brief pause when immediate and short-term emotional reactions are experienced. They may also be provided with one-to-one peer support to help healthcare staff experience a more reflective, self-conscious emotional response. It also highlights the possibility of preparing healthcare staff for likely emotional reactions in particular clinical situations to assist them in being more mindful of the possible impact on the safety of the care they provide. The limited research currently available suggests that emotions influence patient safety processes/outcomes. Further research is needed to explore this relationship further. For example, studies that focused exclusively on more amorphous emotional concepts like burnout were excluded. However, in some of the included studies, these longer-term emotional responses were identified in addition to the immediate, short-term, and reflective emotions. Further research needs to explore longer-term emotional responses such as PTSD, burnout, and work satisfaction, the associated triggers, and the impact on patient safety.
It is important to raise awareness of the potential impact of emotional triggers and the emotions experienced in response to these on patient safety through training and education for healthcare staff. As suggested by previous authors, we recommend that emotional awareness and regulation skills, both of which can be developed and enhanced using emotional intelligence training interventions [ 52 , 53 ] are included in healthcare staff training [ 44 , 54 , 55 , 56 ]. Future work should also distinguish between specific types of emotional responses rather than broadly classifying these as negative and positive, and explore how these influence patient safety. The findings also have potential implications for health equity given that the evidence indicates certain types of patients (e.g. angry and mental health patients) are more likely to provoke negative emotions, and such emotions can result in a negative impact on patient care and safety. This may suggest that such patient groups may receive poorer quality of care due to social factors beyond their control and is an area that requires further research.
Conclusions
Healthcare staff are exposed to many emotional triggers within their work environment including patient safety events, traumatic events, work overload, workplace toxicity, lack of supervisory support, and patient and family factors. In response, healthcare staff experience emotions ranging from anger and guilt to longer-term burnout and PTSD symptoms. Both triggers and the emotional responses to these are perceived to negatively impact patient care and safety, although robust empirical evidence is lacking.
Availability of data and materials
The datasets used and/or analysed during the current study are available from the corresponding author on reasonable request.
Heyhoe J, Birks Y, Harrison R, O’Hara JK, Cracknell A, Lawton R. The role of emotion in patient safety: are we brave enough to scratch beneath the surface? J R Soc Med. 2016;109(2):52–8. https://doi.org/10.1177/2F0141076815620614 .
Article PubMed Google Scholar
Croskerry P, Abbass A, Wu AW. Emotional influences in patient safety. J Patient Saf. 2010;6(4):199–205.
Ubel PA. Emotions, decisions, and the limits of rationality: symposium introduction. Med Decis Making. 2005;25(1):95–6. https://doi.org/10.1177/2F0272989X04273143 .
Iedema R, Jorm C, Lum M. Affect is central to patient safety: The horror stories of young anaesthetists. Soc Sci Med. 2009;69(12):1750–6. https://doi.org/10.1016/j.socscimed.2009.09.043 .
Heyhoe J, Lawton R. Social emotion and patient safety: an important and understudied intersection. BMJ Qual Saf. 2020;29(10):1–2. https://doi.org/10.1136/bmjqs-2019-010795 .
Finucane ML, Alhakami A, Slovic P, Johnson SM. The affect heuristic in judgments of risks and benefits. J Behav Decis Mak. 2000;13(1):1–17. https://doi.org/10.1002/(SICI)1099-0771(200001/03)13:1/3C1::AID-BDM333/3E3.0.CO;2-S .
Article Google Scholar
Finucane ML, Alhakami A, Slovic P, Johnson SM. The affect heuristic in judgments of risks and benefits. J Behav Decis Making. 2000;13(1):1–7.
Loewenstein G. Emotions in economic theory and economic behavior. Am Econ Rev. 2000;90(2):426–32.
Tversky A, Kahneman D. Judgment under Uncertainty: Heuristics and Biases: Biases in judgments reveal some heuristics of thinking under uncertainty. Science. 1974;185(4157):1124–31. https://doi.org/10.1126/science.185.4157.1124 .
Article CAS PubMed Google Scholar
Croskerry P, Abbass AA, Wu AW. How doctors feel: affective issues in patients’ safety. The Lancet. 2008;372(9645):1205–6.
Baumeister RF, Vohs KD, Nathan DeWall C, Zhang L. How emotion shapes behavior: Feedback, anticipation, and reflection, rather than direct causation. Pers Soc Psychol Rev. 2007;11(2):167–203. https://doi.org/10.1177/1088868307301033 .
Ferrer RA, Mendes WB. Emotion, health decision making, and health behaviour. Psychol Health. 2018;33(1):1–16. https://doi.org/10.1080/08870446.2017.1385787 .
Schmidt HG, Van Gog T, Schuit SC, Van den Berge K, Van Daele PL, Bueving H, Van der Zee T, Van den Broek WW, Van Saase JL, Mamede S. Do patients’ disruptive behaviours influence the accuracy of a doctor’s diagnosis? A randomised experiment. BMJ Qual Saf. 2017;26(1):19–23. https://doi.org/10.1136/bmjqs-2015-004109 .
Mamede S, Van Gog T, Schuit SC, Van den Berge K, Van Daele PL, Bueving H, Van der Zee T, Van den Broek WW, Van Saase JL, Schmidt HG. Why patients’ disruptive behaviours impair diagnostic reasoning: a randomised experiment. BMJ Qual Saf. 2017;26(1):13–8. https://doi.org/10.1136/bmjqs-2015-005065 .
Isbell LM, Boudreaux ED, Chimowitz H, Liu G, Cyr E, Kimball E. What do emergency department physicians and nurses feel? A qualitative study of emotions, triggers, regulation strategies, and effects on patient care. BMJ Qual Saf. 2020;29(10):1–2. https://doi.org/10.1136/bmjqs-2019-010179 .
Article PubMed Central PubMed Google Scholar
Isbell LM, Tager J, Beals K, Liu G. Emotionally evocative patients in the emergency department: a mixed methods investigation of providers’ reported emotions and implications for patient safety. BMJ Qual Saf. 2020;29(10):1–2. https://doi.org/10.1136/bmjqs-2019-010110 .
Craciun M. Emotions and knowledge in expert work: A comparison of two psychotherapies. Am J Sociol. 2018;123(4):959–1003. https://doi.org/10.1086/695682# .
Diener E, Thapa S, Tay L. Positive emotions at work. Annu Rev Organ Psych Organ Behav. 2020;7:451–77.
Sirriyeh R, Lawton R, Gardner P, Armitage G. Coping with medical error: a systematic review of papers to assess the effects of involvement in medical errors on healthcare professionals’ psychological well-being. Qual Saf Health Care. 2010;19(6):e43–e43. https://doi.org/10.1136/qshc.2009.035253 .
Seys D, Wu AW, Gerven EV, Vleugels A, Euwema M, Panella M, Scott SD, Conway J, Sermeus W, Vanhaecht K. Health care professionals as second victims after adverse events: a systematic review. Eval Health Prof. 2013;36(2):135–62. https://doi.org/10.1177/2F0163278712458918 .
Harrison R, Lawton R, Stewart K. Doctors’ experiences of adverse events in secondary care: the professional and personal impact. Clin Med. 2014;14(6):585. https://doi.org/10.7861/2Fclinmedicine.14-6-585 .
Lever I, Dyball D, Greenberg N, Stevelink SA. Health consequences of bullying in the healthcare workplace: a systematic review. J Adv Nurs. 2019;75(12):3195–209. https://doi.org/10.1111/jan.13986 .
Bailey, S. (2021). Parliamentary report on workforce burnout and resilience. bmj, 373. https://doi.org/10.1136/bmj.n1603
Kohn LT, Corrigan JM, Donaldson MS (Institute of Medicine). To err is human: building a safer health system. Washington, DC: National Academy Press, 2000
Hall LH, Johnson J, Watt I, Tsipa A, O’Connor DB. Healthcare staff wellbeing, burnout, and patient safety: a systematic review. PLoS ONE. 2016;11(7):e0159015. https://doi.org/10.1371/journal.pone.0159015 .
Article CAS PubMed Central PubMed Google Scholar
Gagnon MP, Ngangue P, Payne-Gagnon J, Desmartis M. m-Health adoption by healthcare professionals: a systematic review. J Am Med Inform Assoc. 2016;23(1):212–20. https://doi.org/10.1093/jamia/ocv052 .
Ekman P. An argument for basic emotions. Cogn Emot. 1992;6(3–4):169–200. https://doi.org/10.1080/02699939208411068 .
Moher D, Liberati A, Tetzlaff J, et al. Preferred reporting items for systematic reviews and meta-analyses: the PRISMA statement. Ann Intern Med. 2009;151:264–9. https://doi.org/10.7326/0003-4819-151-4-200908180-00135 .
Harrison R, Jones B, Gardner P, Lawton R. Quality assessment with diverse studies (QuADS): an appraisal tool for methodological and reporting quality in systematic reviews of mixed-or multi-method studies. BMC Health Serv Res. 2021;21(1):1–20.
Google Scholar
Al Omar M, Salam M, Al-Surimi K. Workplace bullying and its impact on the quality of healthcare and patient safety. Hum Resour Health. 2019;17(1):1–8.
Chard R. How perioperative nurses define, attribute causes of, and react to intraoperative nursing errors. AORN J. 2010;91(1):132–45. https://doi.org/10.1016/j.aorn.2009.06.028 .
Biggs S, Waggett HB, Shabbir J. Impact of surgical complications on the operating surgeon. Colorectal Dis. 2020;22(9):1169–74. https://doi.org/10.1111/codi.15021 .
Bari A, Khan RA, Rathore AW. Medical errors; causes, consequences, emotional response and resulting behavioral change. Pak J Med Sci. 2016;32(3):523. https://doi.org/10.12669/2Fpjms.323.9701 .
Yildirim A, Yildirim D. Mobbing in the workplace by peers and managers: mobbing experienced by nurses working in healthcare facilities in Turkey and its effect on nurses. J Clin Nurs. 2007;16(8):1444–53. https://doi.org/10.1111/j.1365-2702.2006.01814.x .
Karga M, Kiekkas P, Aretha D, Lemonidou C. Changes in nursing practice: associations with responses to and coping with errors. J Clin Nurs. 2011;20(21–22):3246–55. https://doi.org/10.1111/j.1365-2702.2011.03772.x .
Vanhaecht K, Seys D, Schouten L, Bruyneel L, CoeckelberghsPanella EM, Zeeman G. Duration of second victim symptoms in the aftermath of a patient safety incident and association with the level of patient harm: a cross-sectional study in the Netherlands. BMJ open. 2019;9(7):e029923. https://doi.org/10.1136/bmjopen-2019-029923 .
Jalil R, Huber JW, Sixsmith J, Dickens GL. Mental health nurses’ emotions, exposure to patient aggression, attitudes to and use of coercive measures: Cross sectional questionnaire survey. Int J Nurs Stud. 2017;75:130–8. https://doi.org/10.1016/j.ijnurstu.2017.07.018 .
Pinto A, Faiz O, Bicknell C, Vincent C. Surgical complications and their implications for surgeons’ well-being. J Br Surg. 2013;100(13):1748–55. https://doi.org/10.1002/bjs.9308 .
Article CAS Google Scholar
Hassankhani H, Parizad N, Gacki-Smith J, Rahmani A, Mohammadi E. The consequences of violence against nurses working in the emergency department: A qualitative study. Int Emerg Nurs. 2018;39:20–5. https://doi.org/10.1016/j.ienj.2017.07.007 .
Chambers, Charlotte NL, et al. "‘It feels like being trapped in an abusive relationship’: bullying prevalence and consequences in the New Zealand senior medical workforce: a cross-sectional study." BMJ open 8.3 (2018): e020158. https://doi.org/10.1136/bmjopen-2017-020158
Janes G, Mills T, Budworth L, Johnson J, Lawton R. The association between health care staff engagement and patient safety outcomes: a systematic review and meta-analysis. J Patient Saf. 2021;17(3):207. https://doi.org/10.1097/PTS.0000000000000807 .
Owens KM, Keller S. Exploring workforce confidence and patient experiences: A quantitative analysis. Patient Exp J. 2018;5(1):97–105. https://doi.org/10.35680/2372-0247.1210 .
White AA, Gallagher TH. After the apology: Coping and recovery after errors. AMA J Ethics. 2011;13(9):593–600.
Liu G, Chimowitz H, Isbell LM. Affective influences on clinical reasoning and diagnosis: insights from social psychology and new research opportunities. Diagnosis. 2022. https://doi.org/10.1515/dx-2021-0115 .
Van Kleef GA. How emotions regulate social life: The emotions as social information (EASI) model. Curr Dir Psychol Sci. 2009;18(3):184–8.
Fischer AH, Van Kleef GA. Where have all the people gone? A plea for including social interaction in emotion research. Emotion Rev. 2010;2(3):208–11. https://doi.org/10.1177/2F1754073910361980 .
Van Kleef GA, Cheshin A, Fischer AH, Schneider IK. The social nature of emotions. Front Psychol. 2016;7:896.
PubMed Central PubMed Google Scholar
Maran DA, Cortese CG, Pavanelli P, Fornero G, & Gianino MM. (2019). Gender differences in reporting workplace violence: a qualitative analysis of administrative records of violent episodes experienced by healthcare workers in a large public Italian hospital. BMJ open 9(11). https://doi.org/10.1136/bmjopen-2019-031546
Sun L, Zhang W, Qi F, Wang Y. Gender differences for the prevalence and risk factors of workplace violence among healthcare professionals in Shandong, China. Front Public Health. 2022;10:873–936. https://doi.org/10.3389/fpubh.2022.873936 .
Fischer AH, Manstead AS, Zaalberg R. Social influences on the emotion process. Eur Rev Soc Psychol. 2003;14(1):171–201. https://doi.org/10.1080/10463280340000054 .
Isen AM, Rosenzweig AS, Young MJ. The influence of positive affect on clinical problem solving. Med Decis Making. 1991;11(3):221–7. https://doi.org/10.1177/2F0272989X9101100313 .
Estrada CA, Isen AM, Young MJ. Positive affect facilitates integration of information and decreases anchoring in reasoning among physicians. Organ Behav Hum Decis Process. 1997;72(1):117–35. https://doi.org/10.1006/obhd.1997.2734 .
Hodzic S, Scharfen J, Ripoll P, Holling H, Zenasni F. How efficient are emotional intelligence trainings: a meta-analysis. Emot Rev. 2018;10:138–4869.
Mattingly V, Kraiger K. Can emotional intelligence be trained? A meta-analytical investigation. Hum Resour Manag Rev. 2019;29(2):140–55. https://doi.org/10.1016/j.hrmr.2018.03.002 .
Nightingale S, Spiby H, Sheen K, Slade P. The impact of emotional intelligence in health care professionals on caring behaviour towards patients in clinical and long-term care settings: Findings from an integrative review. Int J Nurs Stud. 2018;80:106–17. https://doi.org/10.1016/j.ijnurstu.2018.01.006 .
Bourgeon L, Bensalah M, Vacher A, Ardouin JC, Debien B. Role of emotional competence in residents’ simulated emergency care performance: a mixed-methods study. BMJ Qual Saf. 2016;25(5):364–71. https://doi.org/10.1136/bmjqs-2015-004032 .
Download references
Acknowledgements
The authors would like to thank all those were involved in supporting this review including the Workforce Engagement and Wellbeing Theme members within the Patient Safety Translational Research Centre.
This research is funded by the National Institute for Health Research (NIHR) Yorkshire and Humber Patient Safety Translational Research Centre (NIHR Yorkshire and Humber PSTRC) and Yorkshire and Humber ARC. This research has also been supported by the Yorkshire and Humber Patient Safety Research Collaboration (PSRC). The views expressed in this article are those of the author(s) and not necessarily those of the NIHR, or the Department of Health and Social Care.
Author information
Authors and affiliations.
Bradford Teaching Hospitals NHS Foundation Trust, Bradford, BD9 6RJ, UK
Raabia Sattar, Rebecca Lawton, Jane Heyhoe, Isabel Hague & Chloe Grindey
Anglia Ruskin University, Cambridge, CB1, 1PT, UK
Gillian Janes
University of London, London, EC1V 0HB, UK
Mai Elshehaly
You can also search for this author in PubMed Google Scholar
Contributions
RS, RL, GJ, and JH contributed towards the development of the ideas for this review. RS built the search strategy and this was checked by RL and JH. RS performed the searches in all databases. Data extraction for stage 1 was carried out by CG and IH and checked by RS, RL, JH and GJ. Data extraction for stage 2 was carried out by RS and checked by RL, JH, and JH. Data analysis was led by RS . ME developed the infographic for the results and supported with the interpretation of the data. RS wrote a first draft of the manuscript. All authors provided input and recommendations at all stages of the study and revised the draft manuscript. All authors read, contributed towards and approved the final manuscript.
Corresponding author
Correspondence to Raabia Sattar .
Ethics declarations
Ethics approval and consent to participate.
Ethics approvals and consent was not required for this systematic review.
Consent for publication
Not applicable.
Competing interests
The authors declare no competing interests.
Additional information
Publisher’s note.
Springer Nature remains neutral with regard to jurisdictional claims in published maps and institutional affiliations.
Supplementary Information
Supplementary material 1., supplementary material 2., supplementary material 3., rights and permissions.
Open Access This article is licensed under a Creative Commons Attribution 4.0 International License, which permits use, sharing, adaptation, distribution and reproduction in any medium or format, as long as you give appropriate credit to the original author(s) and the source, provide a link to the Creative Commons licence, and indicate if changes were made. The images or other third party material in this article are included in the article's Creative Commons licence, unless indicated otherwise in a credit line to the material. If material is not included in the article's Creative Commons licence and your intended use is not permitted by statutory regulation or exceeds the permitted use, you will need to obtain permission directly from the copyright holder. To view a copy of this licence, visit http://creativecommons.org/licenses/by/4.0/ . The Creative Commons Public Domain Dedication waiver ( http://creativecommons.org/publicdomain/zero/1.0/ ) applies to the data made available in this article, unless otherwise stated in a credit line to the data.
Reprints and permissions
About this article
Cite this article.
Sattar, R., Lawton, R., Janes, G. et al. A systematic review of workplace triggers of emotions in the healthcare environment, the emotions experienced, and the impact on patient safety. BMC Health Serv Res 24 , 603 (2024). https://doi.org/10.1186/s12913-024-11011-1
Download citation
Received : 31 August 2023
Accepted : 18 April 2024
Published : 09 May 2024
DOI : https://doi.org/10.1186/s12913-024-11011-1
Share this article
Anyone you share the following link with will be able to read this content:
Sorry, a shareable link is not currently available for this article.
Provided by the Springer Nature SharedIt content-sharing initiative
- Healthcare work environment
- Patient safety
- Systematic review
BMC Health Services Research
ISSN: 1472-6963
- General enquiries: [email protected]

Assessing Occupational Safety and Health Training – A Literature Review
Dhhs (niosh) publication number 98-145.
More than 100 Occupational Safety and Health Administration (OSHA) standards for hazard control in the workplace contain requirements for training aimed at reducing risk factors for injury or disease; others limit certain jobs to persons deemed competent by virtue of special training. A literature review was undertaken to assess the merits of such training rules to achieve this objective and to sort out factors of consequence. The review focused heavily on published reports, primarily drawn from the period 1980 through 1996, wherein training was used as an intervention effort to reduce risk of work-related injury and disease. Eighty (80) such reports were found and gave overwhelming evidence to show the merits of training in increasing worker knowledge of job hazards, and in effecting safer work practices and other positive actions in a wide array of worksites. Reports from select surveys and investigations of worker injuries and workplace fatalities were also accessed with many implicating lack of training as a contributing factor to the mishaps. In still other studies, workplace training devoted to first aid instruction showed linkage to reduced worker injury rates, suggesting that even this kind of training has benefits to job safety overall.
A critical analysis of the above findings found certain qualifications in viewing training impacts and successes with regard to current workplace standards. For example, most of the reported training intervention studies did not address OSHA training rules per se, and knowledge gain and safe behavior measures were used in many evaluations as opposed to actual injury/disease indicators. Also, in some instances, the training was coupled with other forms of intervention to make attribution difficult. Training deficits noted in some surveys of work injury cases lacked for confirmation and no information was available on the quality of the instruction if given at all.
Despite the above reservations and uncertainties, training’s role as a necessary element in developing and maintaining effective hazard control activities remained firmly supported by the available literature. What did emerge from this review and analysis was an appreciation of meaningful training procedures and the recognition of factors both within and beyond the training process that could greatly affect its impact. In this regard, the OSHA voluntary training guidelines were described along with illustrations from the reports to show how the various steps contained within them can be met in realistic ways and have merit in framing an effective program. In addition, factors both within and beyond the training process were assessed for their effects on training outcomes based on data found in the reviewed literature. Variables such as size of training group, length/frequency of training, manner of instruction, and trainer credentials were each shown to be significant determinants to the training process. Equally important were extra-training factors such as goal setting, feedback and motivational incentives along with managerial actions to promote the transfer of learning to the jobsite.
Based on the literature review, follow-on efforts to address outstanding issues and needs regarding effective occupational safety and health training were noted.
Assessing Occupational Safety and Health Training – A Literature Review
Follow NIOSH
Niosh homepage.
- Workplace Safety & Health Topics
- Publications and Products
- Contact NIOSH
Exit Notification / Disclaimer Policy
- The Centers for Disease Control and Prevention (CDC) cannot attest to the accuracy of a non-federal website.
- Linking to a non-federal website does not constitute an endorsement by CDC or any of its employees of the sponsors or the information and products presented on the website.
- You will be subject to the destination website's privacy policy when you follow the link.
- CDC is not responsible for Section 508 compliance (accessibility) on other federal or private website.
- Open access
- Published: 11 May 2024
The role of nurses for patients with Parkinson’s disease at home: a scoping review
- Takako Fujita 1 ,
- Miho Iwaki 1 &
- Yoko Hatono 1
BMC Nursing volume 23 , Article number: 318 ( 2024 ) Cite this article
51 Accesses
Metrics details
Parkinson’s disease is a neurodegenerative disease, and many patients are cared for at home by nurses. Parkinson’s disease nurse specialists have been certified in several countries. This study aimed to provide an overview of what is known about the role of nurses in the care of patients with Parkinson’s disease at home and to determine the differences between nurses and Parkinson’s disease nurse specialists.
A scoping review was conducted according to the Preferred Reporting Items for Systematic Reviews and Meta-Analyses Extension for Scoping Reviews guidelines. PubMed, Scopus, Web of Science, and Cumulative Index to Nursing and Allied Health Literature were searched (keywords: Parkinson’s disease AND nurse AND [community OR home]) for studies published in English up to September 2023 describing the nurse’s role in caring for patients with Parkinson’s disease at home. Studies without abstracts were removed, along with protocols, systematic reviews, and studies concerned with other diseases or including data that were difficult to distinguish from those of other diseases. Roles were described and organized by category.
A total of 26 studies were included. The nurses’ roles were categorized as overall assessment and support, treatment management, safety assessment regarding falls, care for non-motor symptoms, palliative care, support for caregivers, education for care home staff, multidisciplinary collaboration, and provision of information on social resources. Medication management and education of care home staff were identified as roles of nurse specialists.
Conclusions
This study revealed the role of nurses caring for patients with Parkinson’s disease at home. Because of the complexity of the patients’ medication regimens, nurse specialists provide assistance, especially with medication management and the provision of education to care staff. This study will facilitate the preparation of nurses to acquire the knowledge and skills necessary to help patients with Parkinson’s disease, even in countries where Parkinson’s disease nurse specialists are not officially certified, and will help patients feel comfortable with the care they receive.
Peer Review reports
Parkinson’s disease (PD) is a neurodegenerative disease affecting 8.5 million patients worldwide as of 2019 [ 1 ]. PD is more common in older people, and the risk increases over time [ 2 , 3 , 4 ]. There are prevalence differences by country even within the same ethnic group [ 4 ].
Patients with PD experience both motor and non-motor symptoms. Oral medication, levodopa–carbidopa intestinal gel (LCIG), apomorphine management, deep brain stimulation (DBS), and non-pharmacologic therapy, such as rehabilitation, can relieve motor symptoms. Oral medication is used as the first-line treatment based on previous evidence [ 5 , 6 ].
Nurses play an important role in supporting patients with PD, and nurses specializing in PD or related diseases (PD nurses) have been certified in several countries. The United Kingdom was the first nation to implement this system, and a competency framework that outlines the competencies for each level of nurse has also been developed [ 7 ]. In the United Kingdom, PD nurse specialists (PDNSs) run clinics in hospitals or the community, and they visit patients’ homes if the patients cannot attend clinics [ 7 ]. However, some countries have not officially approved such certification, even though the number of patients and treatment complexity has been increasing. Therefore, those countries may need to introduce certification in the future.
Many patients are cared for at home by nurses, and the basic treatment is oral medication. As stated previously, some countries have not introduced qualifications for PD nurses. Additionally, nurses in hospitals and clinics only provide care within their departments, and home-visiting nurses only provide care to patients in their own homes or in care homes. Some countries have guidelines for nurses specializing in PD, but these are not focused only on home-based care
This review aimed to provide an overview of what is known about the role of nurses in caring for patients with PD at home, and to determine the differences between nurses and PD nurses caring for these patients.
A scoping review was conducted according to the Preferred Reporting Items for Systematic Reviews and Meta-Analyses Extension for Scoping Reviews guidelines [ 8 ]. Because the focus of this study was on the role of nurses in caring for patients with PD at home, a scoping review was conducted. We employed the PCC framework to identify the review question [ 9 ], as follows:
P (Population): patients with Parkinson’s disease
C (Concept): the role of nurses
C (Context): the home environment
All types of studies about the role of nurses caring for patients with PD at home were considered. The review protocol was not registered, in accordance with the guidelines.
Search methods
The PubMed, Scopus, Web of Science, and Cumulative Index to Nursing and Allied Health Literature databases were searched with the keywords Parkinson’s disease AND nurse AND (community OR home). All types of studies published up to September 2023 were included, as the qualifications of PD nurses vary among countries and the role of nurses needs to be understood in broad terms.
Inclusion and/or exclusion criteria
All references of interest were imported into EndNote 21 (Clarivate Analytics, Philadelphia, PA, USA). Of the 1,190 references extracted during the search, 900 remained after removing duplicates (Fig. 1 ). Those without abstracts and those written in languages other than English were then removed, along with protocols, systematic reviews, studies concerned with other diseases or including data that were difficult to distinguish from those of other diseases, and studies that did not discuss the role of the nursing profession or home support. Two researchers determined whether studies should be excluded, and another researcher was added to the team to adjudicate for studies that caused a difference in opinion.
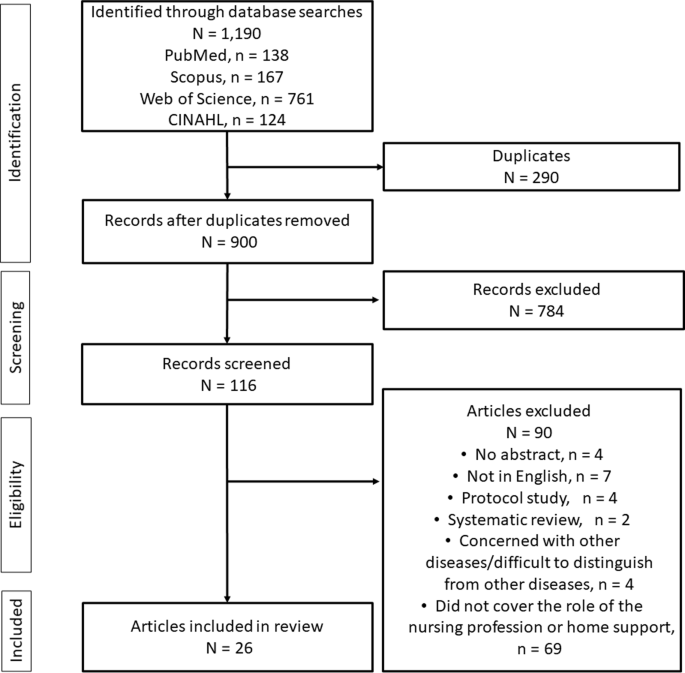
Flow diagram of the study
Search outcome
Only English-language literature describing the nurse’s role in caring for patients with PD at home was considered. Roles were described and organized by category.
Quality appraisal
Quality appraisal is optional according to the guidelines for a scoping review; therefore, quality assessment of each study was not performed [ 8 ].
Data abstraction
For each of the selected studies, we listed the author, year of publication, country in which the study was conducted, objective, study design, participants and sample size, type of nurse, and roles of the nurses. The roles of nurses were organized and described based on the thematic content.
All themes relating to the nurses’ roles were extracted from each study by three researchers. The researchers discussed and organized the roles based on similarities. The thematic content of the extracted roles was integrated into the narrative.
The included studies were published between 1999 and 2023 and performed in the United Kingdom ( n = 12), United States ( n = 7), Australia ( n = 2), Sweden ( n = 1), Germany ( n = 1), Italy ( n = 1), China ( n = 1), and Japan ( n = 1). PD nurses are referred to as PDNSs in the United Kingdom, Italy, and Germany; as community Parkinson’s nurse specialists (CPNSs) or community-based nurses specializing in PD in the United Kingdom; as registered PD nurses in China; as Parkinson’s nurse experts or movement disorder nurses in Australia; as neuroscience nurses in the United States; and as Duodopa nurse specialists in Sweden. A summary of the selected studies is presented in Table 1 .
In the following section, we describe the identified roles of nurses caring for patients with PD at home.
Comprehensive assessment and care
Comprehensive assessment is required of nurses not only for patients with PD but also for general patient support. Nurses need to plan appropriate care for patients with PD covering communication, personal hygiene, mobility, constipation, swallowing and diet, and psychological problems [ 10 ]. In addition, nurses provide education to the patients, including on therapeutic medications and fall prevention [ 11 ].
PDNSs are responsible for supporting patients by conducting regular in-person assessments, considering possible complications, and identifying changes in motor and non-motor symptoms to prevent worsening of the disease [ 12 , 13 ].
Because worsening idiopathic PD is likely to affect some aspects of a patient’s health and cause serious problems that can lead to hospitalization, such as dysphagia resulting in a chest infection or fractures following falls, an accurate assessment of symptoms by the nurse and an understanding of the concerns of patients with PD and their families and caregivers can help ensure timely responses to emerging problems [ 12 ]. In addition, since patients with PD are knowledgeable about their symptoms and the impact of idiopathic PD, PDNSs can consider patients’ concerns and involve them in decision-making at every stage [ 12 ].
Therapeutic management of motor symptoms
Support for medication adherence.
Medication management is one of the most common forms of support provided by nurses to patients with PD [ 11 ]. The support given by both PD nurses and other nurses includes discussing medication management, providing information and education about PD medications and side effects, and reviewing medication schedules [ 11 , 14 , 15 , 16 , 17 ]. In a study conducted in the United States, nurses performed a detailed medication reconciliation, reviewing multiple medication management methods (e.g., pillboxes, timers) and prescriptions, over-the-counter medications, and supplements, and comparing the actual regimen to that listed at the most recent outpatient visit [ 16 , 18 ]. Patients can also be supported by PD nurses in these respects through telenursing. Neuroscience nurses in the United States provided videophone and telephone guidance, which is helpful in confirming medications, side effects, and schedules [ 14 ]. The nurse–patient relationship was reportedly strengthened by this strong individualized support, and patients appreciated the guidance, especially the visual aspect provided by videophone [ 14 ].
Because oral medications for patients with PD are complex, it is necessary to be aware of a possible decline in medication adherence. A US medication error study involving registered nurses who made home visits to patients with PD reported that the most common medication errors were taking medications that were not known to their care providers, taking medications that patients were instructed to discontinue, taking an incorrect number of doses, and omitting or forgetting to take a dose [ 19 ]. Medication delays can have serious consequences, including impaired physical function and dysphagia, and neuroleptic malignant syndrome can be life-threatening. PDNSs need to be constantly vigilant to ensure that PD medications are not unexpectedly discontinued for any reason [ 12 ]. CPNSs may also consult with pharmacies about medication management and introduce dispensing aids, such as reminders and blister packaging [ 20 ]. One reason for poor medication adherence may be dysphagia. Crushing tablets or opening capsules to make swallowing easier for patients with PD should not be implemented without seeking advice from the dispenser [ 17 ]. Therefore, community nurses should consult with the pharmacist to discuss whether other forms of medications can be used when swallowing difficulties are affecting adherence [ 17 ].
Medication adjustment
Only studies regarding the adjustment of oral medication by PD nurses were extracted. In these studies, medications were adjusted based on knowledge of the association between diet and medications, including the time between meals and medications, and the timing of oral medications was adjusted to reduce drowsiness as a side effect [ 14 , 21 ]. PDNSs may also adjust medications. Studies in the United Kingdom and Italy have shown that PDNSs provide information about medical conditions and the purposes of medications, change medications via formal consultations or by telephone, aim to detect problems early, including side effects, when treatment is changed, and advise on medication adjustments [ 20 , 21 , 22 , 23 ].
A study that conducted semi-structured interviews reported that patients were satisfied with the information provided by PDNSs [ 21 ]. A study in which patients were questioned by community-based nurses specializing in PD, which included patients with and without regular interventions who had their clinical health status and response to treatment monitored (reporting back to their general practitioner or consultant as needed), reported that the intervention group had significantly better scores for overall health [ 24 ]. In a case study, a CPNS in the United Kingdom observed a patient’s hallucinations and discovered that they were a side effect of L-dopa, which led to an adjustment in dosage [ 20 ]. In Italy, a PDNS identified unpleasant hallucinations during telenursing, determined that they were possible side effects of cholinesterase, and provided this information to the physician, which led to modification of the prescription medication [ 23 ]. Similarly, in Australia, a movement disorder nurse conducted advocacy regarding medications, and medication was changed after physician–patient interactions [ 25 ].
LCIG care was reported in one study conducted in Sweden that implemented care at home using telehealth. In that study, Duodopa nurses provided training in the use of the equipment before and during the introduction of telemedicine, support through home visits, and instruction to patients using a video communication system; patients, neurologists, and nurses were all satisfied [ 26 ].
Apomorphine management
One study in the United Kingdom reported on apomorphine management. A community nursing team was responsible for the daily management of apomorphine, supported by guidance from PDNSs. The role of community nurses in apomorphine management was to supervise and support patients, caregivers, and family members, including by selecting appropriate sites for the infusion, setting up the pump, and siting and removing the needle. Apomorphine users, nurses, and caregivers should follow best practices to minimize severe reactions to apomorphine infusion, and better documentation of the rotation of the infusion site and nodule severity assures a high standard of care [ 27 ].
Postoperative management of DBS
In a US intervention study, home health nurses supported the postoperative management of patients who had undergone DBS. Postoperatively, trained nurses visited the patients at home to measure vital signs, implement an app-based program, and administer medications and clinical rating scales. The study reported no significant differences in motor symptoms, PD symptom severity, or quality of life compared with outpatients [ 28 ].
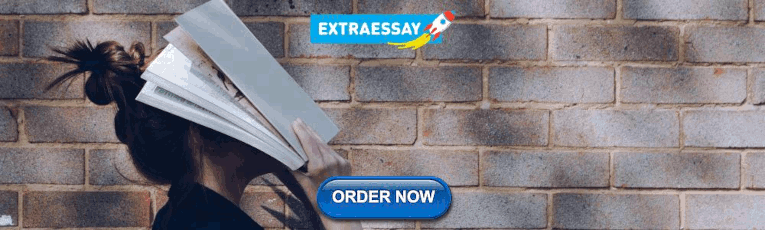
Safety assessment related to falls
As patients with PD are at increased risk of falling because of motor and non-motor symptoms, treatment, and other factors, they need to be assessed in terms of safety. Therefore, it is important to identify motor fluctuations [ 12 ]. Specifically, nurses use the Unified Parkinson’s Disease Rating scale (I and II) and fall assessments, paying particular attention to tremors; falls; frozen gait; dysphagia; independence level in dressing, toileting, and walking; and environmental safety assessments at home, such as in the bathroom and kitchen, including assessments of clutter and uneven floors [ 16 , 18 ]. In addition, nurses check orthostatic vital signs given the prevalence of orthostatic hypotension [ 16 ]. Because conditions change over time, PDNSs have a role in providing strategies for adapting to changes and advice for maintaining safety, such as by avoiding falls [ 12 ]. The appropriateness of mobility aids such as canes and walkers should also be assessed, and it is essential to educate family members about these aids [ 15 ].
In a study from the United Kingdom, it was reported that there were no significant differences in motor symptoms or quality of life between patients treated by community-based nurses specializing in PD and those treated by general physicians, and the nurse group had significantly better overall health scores [ 24 ]. An Italian telenursing service operated by PDNSs reported 13 telephone contacts during a 3-month intervention period and, at enrollment, 99 falls in the previous 3 months; this decreased to 3 falls in the 3 months after the intervention [ 23 ].
Support for non-motor symptoms
Support for sleep disorders.
Sleep disorders are also associated with quality of life and the risk of falls in patients with PD. One study from the United Kingdom found that after PDNSs received education on helping patients with PD and sleep disorders, patients reported a reduction in anxiety over sleep problems, feeling able to manage their sleep, having a sense of control over their sleep, and experiencing improved sleep efficiency (time to fall asleep) and quality of life [ 29 ]. This education included recognition of sleep problems in patients with PD, health education on sleep and insomnia, sleep assessment, sleep hygiene practices, use of stimulus control and sleep restriction procedures, implementation of relaxation methods, and cognitive approaches to manage insomnia symptoms [ 29 ].
Support for Dysphagia
Dysphagia is also associated with the previously mentioned oral medications. One role of community nurses for patients with PD in the United Kingdom is to be aware of the risks of dysphagia, to actively ask patients if they have experienced swallowing difficulties, and to consult with pharmacists about the shape and other aspects of the medication, given that difficulty swallowing can affect medication adherence [ 17 ]. In Italy, a case study reported that after 13 telenursing sessions over 3 months, in which a PDNS provided symptom assessment and individualized advice by telephone, the initial moderate dysphagia completely disappeared at the follow-up interview 3 months later [ 23 ].
Support for excretory problems
Urinary problems and constipation need to be incorporated into the nursing plan because of their impact on patient distress [ 10 , 15 ]. For urinary problems, a comprehensive assessment should be conducted before an appropriate treatment plan is implemented, including a continence assessment conducted with a trained professional and supporting families with tailored bladder training, such as a regular toileting routine, pelvic floor muscle training, and the supply of products for incontinence [ 30 ]. Regular clinical monitoring, medication management, and good communication with patients can help empower them to make decisions and choices related to their care [ 30 ]. It was reported that urinary problems were common in patients with Hoehn and Yahr stage V, and urinary and indwelling bladder catheters were commonly required [ 31 ].
Support for mental health
Mental health support and counseling are provided by nurses and PD nurses [ 11 , 22 ], and were among the most frequently performed nursing activities for patients with PD in a study of nurse care managers in the United States [ 11 ].
In terms of specific individualized support, in the United Kingdom, CPNSs found that patients continued to fall, lost weight because of anorexia, and felt increasingly depressed; the nurses discussed the best way forward with multidisciplinary staff and family members [ 20 ]. In Australia, a movement disorder nurse provided emotional support to her patient, with a reduction in illness uncertainty achieved through the sharing of information about future expectations [ 25 ]. In a case report about a telenursing service provided by a PDNS in Italy, 13 telephone support calls in 3 months led to a reduction in depression and anxiety [ 23 ].
Palliative care
Palliative care is designed to provide specialized disease management and physical, psychological, spiritual, and social support, to reduce suffering and improve quality of life for patients and their caregivers [ 32 ]. PDNSs play a role in symptom reduction and pain relief for both patients and caregivers, for example, by addressing dysuria, constipation, and sedation in patients and providing counseling, education, and advice for both patients and caregivers, including by facilitating referrals and collaborations with other agencies [ 22 ].
In China, a study of factors promoting and inhibiting palliative care provision by registered PD nurses was conducted, and the facilitatory factors included the desire for palliative care knowledge among healthcare professionals, the presence of social support, nurses acting as a bridge between the patient and physician, convenient community services, and the availability of hospital–community–family based services [ 32 ]. Factors inhibiting palliative care included misconceptions about this form of care among healthcare professionals, patients, and caregivers (e.g., believing that palliative care does not include surgical or conservative treatments and is similar to hospice care); lack of time for communication between patients and healthcare professionals; lack of specialized palliative care nurses; lack of palliative care referral criteria; lack of information on palliative care resources; and lack of maintenance of continuity of care [ 32 ].
Support for caregivers
Counseling and education about PD are provided to both patients and caregivers by PD nurses and other nurses, both in the home and over the phone, when patients are living at home [ 18 , 24 , 31 ]. In a US study, a program of home visits by several professionals, including nurses, to assess the psychosocial needs of patients and caregivers, along with follow-up by telephone to address any remaining needs, indicated that 98.1% of caregivers were satisfied with the program [ 18 ]. Another study reported that nurses mediated between physicians and patients or their families, such as by providing information to caregivers when the patient’s medication was changed [ 25 ]. When palliative care is provided, as noted above, PDNSs are responsible for providing counseling, education, and advice to both the patients and caregivers, as well as for facilitating referrals and coordination with other agencies [ 22 ].
Education for care home staff
Patients with PD may need to be admitted to a care home if they have difficulty living at home. Staff providing care to patients with PD find hallucinations, falls, and physical difficulties difficult to manage [ 33 ]. In the United Kingdom, 62% of PDNSs reported training care home staff, providing training to staff when a patient who had been in the care of a CPNS was admitted to a care home and providing training in the area of responsibility in collaboration with the Education and Training Officer of Parkinson’s UK [ 20 , 33 ]. Specifically, they provided materials and information related to the care of patients with PD, regular reviews of patients by PD nurses or general practitioners, and regular training for new employees [ 33 ]. An Australian study introducing an educational program (including video presentations, a 1-hour lecture, and a refresher program after 4–6 weeks) for staff in residential aged care facilities, developed primarily by Parkinson’s nurse experts and focusing specifically on therapeutic medication knowledge and management, reported that program implementation increased levels of knowledge [ 34 ].
Multidisciplinary approach
According to the included studies, PD nurses and nurses collaborated with neurologists, pharmacists, movement disorder specialists, social workers, psychologists, physiotherapists, occupational therapists, speech therapists, and other therapists specializing in neurology, as well as with nutritionists, care staff and, in the United Kingdom, other staff involved in caring for Parkinson’s patients, with contact maintained with hospital staff during admission and discharge [ 12 , 15 , 16 , 18 , 24 , 33 , 35 ]. Collaboration involved joint home visits, the exchange of information through conferences, and referrals to other professionals such as psychologists and therapists [ 12 , 20 , 33 ].
Nurses need to work as a care team to address both aspects of the clinical environment and the care provided in the home for patients with PD [ 13 ]. When working within a multidisciplinary team, nurses need to assess the patient’s abilities and plan appropriate care, including in relation to both written and verbal communication; personal hygiene; mobility (with consideration of problems related to moving in bed, transferring, and initiating and maintaining mobility); toileting, with special attention paid to the possibility of constipation; swallowing and eating; and psychological issues [ 10 ]. Nurses play a liaison role, working with the primary care team for ongoing assessment and treatment as needed [ 24 , 32 ]. The role also includes training nursing home staff and various other clinical and general staff, as well as educating the community [ 20 , 22 ].
The effects of multidisciplinary collaboration on patients have also been reported. A study conducted in Germany reported clear improvements in emotional well-being, stigma, communication, and physical discomfort through collaboration among PDNSs, community neurologists, and movement disorder specialists [ 35 ]. A study of palliative care in China stated that the registered PD nurse should serve as a coordinator between the physician and the patient, as the absence of this collaboration results in poor palliative care [ 32 ].
Provision of information on social resources
Nurses have a role in providing patients with PD, and their families and caregivers, with local and national resources and information, such as recommendations for respite and day hospitalization care, assessment of eligibility for social benefits, and special assistance at home [ 12 , 15 , 22 , 24 ]. For example, in an Australian study, movement disorder nurses provided extensive practical support through a variety of strategies, incorporating home modifications and housekeeping support to assist patients with daily living [ 25 ]. In a study of palliative care in China, a lack of information on social resources led to a lack of service utilization [ 32 ]. Finally, a case study conducted in the United Kingdom reported that a patient who had repeated falls, worsening depression, and difficulty living alone was referred to a nursing home [ 20 ].
This study was conducted to provide an overview of what is known about the role of nurses in caring for patients with PD at home, and to determine the differences between nurses and PDNSs caring for these patients. Nine main roles were identified: overall assessment and support, treatment management, safety assessment regarding falls, care for non-motor symptoms, palliative care, support for caregivers, education for care home staff, multidisciplinary collaboration, and provision of information on social resources. Most of the roles were similar to those associated with nursing care at home for patients in general [ 36 ] and were performed by both nurses and PD nurses. The results suggest that the main role of PD nurses at home is to manage medication and educate care staff. While these are also important roles for PD nurses in clinics, PD nurses in the home environment can provide superior support because they understand patients’ daily lives. The results of this study will help nurses prepare by providing them with the knowledge and skills needed to help patients with PD, leading to more reliable care for patients, especially in countries lacking a PD nurse system.
Because the basic treatment for PD is oral medication, medication management by nurses, subsuming medication adherence and dosage adjustment considering side effects and timing, is considered to be particularly important in supporting home care. Most patients with PD, particularly those with early stage disease, engage in medication non-adherence behaviors, often intentionally [ 21 , 37 ]. One common reason for hospitalization among patients with PD is falls or fractures caused by motor symptoms; in fact, this accounts for 65% of all falls [ 38 ]. For preventing hospitalization, nurses should be aware that patients are likely to show non-adherence behaviors, and they should aim to prevent missed or incorrectly taken medications by using medication boxes, building a relationship in which the patient feels free to ask for help regarding medication problems, and possibly providing guidance to family members and other support persons. The role of medication dosage adjustment was extracted only from the studies about PD nurses included in this review. It would be particularly meaningful for PD nurses to be involved in this aspect of care because they might spend more time with patients than other professionals; they can assess side effects and the need to add, subtract, or change medication doses at particular times, as well as explain the situation to the physician. In addition to oral medication, nurses are responsible for the daily management of apomorphine in homebound patients and are supported by guidance from PD nurses [ 27 ]. Novel non-oral medications may emerge in the future as alternatives to apomorphine, LCIG, and non-pharmacological treatments such as DBS. PD nurses may be required to advise nurses and to make suggestions to the patient’s physician regarding changes in administration methods and treatments, including implementing other non-pharmacologic therapies.
When patients with PD have difficulty living at home, they may be considered for admission to a care home, especially if they have advanced PD or comorbidities that significantly increase the possibility of admission, such as hip fracture or dementia [ 39 , 40 ]. Care needs for admitted patients with PD may not be met if staff knowledge of PD-related problems is insufficient [ 41 ]. In addition to the complexity of oral medications for PD, patients take medicines for the treatment of comorbidities as needed, which may make it difficult for care home staff without sufficient knowledge to assist patients in taking their medications. Therefore, education of care staff by PD nurses is necessary to ensure the safety of patients with PD.
Other roles of nurses related to motor and non-motor symptoms in patients with PD at home are generally applicable to patients with other diseases as well. Specific details of the nurse’s role regarding constipation, which is one of the non-motor symptoms of PD, were not extracted from the studies included in this review. However, the frequency of constipation varied among the studies, from 7 to 70%, and constipation is considered a common symptom in patients with PD because of the effects of the both the disease itself and oral medications [ 42 ]. In the management of constipation, dietary intervention with probiotics and prebiotics and the use of lubiprostone and macrogol have been suggested to be potentially effective, whereas the evidence for the efficacy of abdominal massage is considered insufficient [ 43 , 44 ]. A nurse intervention program for constipation in patients with PD has been created in China [ 45 ], and its results suggests that support for defecation is one of the forms of care that nurses should provide. Sleep medications are sometimes used to treat sleep problems, which are classified as non-motor symptoms. However, in patients with PD, only the dopamine agonist rotigotine has been shown to be effective; evidence for the efficacy of other drugs is insufficient [ 44 ]. Among older patients with PD, 18.6% were prescribed hypnotics, with benzodiazepines being reported to increase the risk of injury significantly, and melatonin receptor agonists reportedly significantly increasing the risk of femoral fractures [ 46 ]. Therefore, it is important to understand the treatments for non-motor symptoms and to provide nursing care while considering their side effects. This can be expected to improve the quality of life of patients with PD.
In addition to nursing care to address the symptoms of patients with PD at home, we found that nurses need to play roles in multidisciplinary collaborations and provide information on social resources. There are differences among countries and regions in terms of the granting of qualifications and the tasks performed in accordance therewith. This study found that there are PD nurses in the United Kingdom, Italy, Germany, Sweden, Australia, the United States, and China, and a competency framework for nurses working in PD management has been created in the United Kingdom [ 7 ]. In the Netherlands, which was not covered by this study, professional qualifications have been granted, and guidelines have been created [ 47 ]. Nurse-led community care is also provided in Singapore, particularly for patients with severe motor impairments and those without a caregiver; patients are visited by a PD nurse and, if necessary, referred to relevant community services [ 48 ]. In these countries, PD nurses take the lead in supporting patients, which is essential for patients to live at home. By contrast, in Japan, for example, nurses at hospitals and clinics only provide care within their departments, and home-visiting nurses only provide care to patients at home or in care homes. As such, some countries are not able to provide nursing care across all inpatient, outpatient, and home care settings. Instead, Japan has a national qualification for public health nurses, who are mainly affiliated with government agencies, playing a liaison role by providing information on available social services and communicating with various agencies. However, they do not provide patient support under the orders of a physician and cannot provide medical assistance. The introduction of PD nurses in countries such as Japan will require that they be given PD-specific roles in accordance with the national system. Clarifying the roles of each type of care provider in every country may increase the likelihood that patients with PD can continue to live at home.
This review has some limitations. First, we only included articles written in English; references written in other languages or included in databases from other countries were not extracted. On the basis of the distribution of the included studies by country, selection bias is plausible given that the countries with multiple references were all English-speaking countries. Second, no studies in this review described in detail the differences between the roles of PD nurses and other nurses in countries where PD nurses are qualified, and the relative impact on patients of interventions implemented by the different types of nurses was not evaluated. Interventions implemented by PD nurses may lead to greater improvements in patients’ symptom control and quality of life compared with those implemented by nurses. Therefore, further research focusing on the differences in nursing roles and outcomes is needed.
This study clarified the nine main roles of nurses caring for patients with PD at home, including overall assessment and support, treatment management, safety assessment regarding falls, care for non-motor symptoms, palliative care, support for caregivers, education for care home staff, multidisciplinary collaboration, and provision of information on social resources. Because PD medications are complex, medication management and education for care staff are particularly important roles for PD nurses. This study will help prepare nurses by providing them the knowledge and skills necessary to assist patients with PD, even in countries that do not have a PD nurse system, thereby leading to care that will improve patients’ sense of security.
Data availability
Not applicable.
Abbreviations
- Parkinson’s disease
levodopa–carbidopa intestinal gel
deep brain stimulation
Parkinson’s disease nurse specialist
community Parkinson’s nurse specialists
World Health Organization. Parkinson’s disease 2023. https://www.who.int/news-room/fact-sheets/detail/parkinson-disease . Accessed 28 Feb 2024.
Willis AW, Roberts E, Beck JC, Fiske B, Ross W, Savica R, et al. Incidence of Parkinson disease in North America. Npj Parkinson’s Disease. 2022;8(1):170. https://doi.org/10.1038/s41531-022-00410-y .
Article CAS PubMed PubMed Central Google Scholar
Brakedal B, Toker L, Haugarvoll K, Tzoulis C. A nationwide study of the incidence, prevalence and mortality of Parkinson’s disease in the Norwegian population. Npj Parkinson’s Disease. 2022;8(1):19. https://doi.org/10.1038/s41531-022-00280-4 .
Article PubMed PubMed Central Google Scholar
Abbas MM, Xu Z, Tan LCS. Epidemiology of Parkinson’s Disease—East Versus West. Mov Disorders Clin Pract. 2018;5(1):14–28. https://doi.org/10.1002/mdc3.12568 .
Article Google Scholar
NICE. Parkinson’s disease in adults NICE guideline [NG71] 2017. https://www.nice.org.uk/guidance/ng71 . Accessed 28 Feb 2024.
Fox SH, Katzenschlager R, Lim S-Y, Barton B, de Bie RMA, Seppi K, et al. International Parkinson and movement disorder society evidence-based medicine review: update on treatments for the motor symptoms of Parkinson’s disease. Mov Disord. 2018;33(8):1248–66. https://doi.org/10.1002/mds.27372 .
Article CAS PubMed Google Scholar
Parkinson’s UK, Competencies. A competency framework for nurses working in Parkinson’s disease management (3rd edition) 2016. https://www.parkinsons.org.uk/professionals/resources/competency-framework-nurses-working-parkinsons-disease-management-3rd . Accessed 28 Feb 2024.
Tricco AC, Lillie E, Zarin W, O’Brien KK, Colquhoun H, Levac D, et al. PRISMA Extension for scoping reviews (PRISMA-ScR): Checklist and Explanation. Ann Intern Med. 2018;169(7):467–73. https://doi.org/10.7326/M18-0850 .
Article PubMed Google Scholar
Peters MDJ, Marnie C, Tricco AC, Pollock D, Munn Z, Alexander L, et al. Updated methodological guidance for the conduct of scoping reviews. JBI Evid Synthesis. 2020;18(10):2119–26. https://doi.org/10.11124/jbies-20-00167 .
Thomas S, MacMahon D. Managing Parkinson’s disease in long-term care. Nurs Older People. 2002;14(9):23–30. https://doi.org/10.7748/nop2002.12.14.9.23.c2232 .
Connor KI, Siebens HC, Mittman BS, Ganz DA, Barry F, Ernst EJ, et al. Quality and extent of implementation of a nurse-led care management intervention: care coordination for health promotion and activities in Parkinson’s disease (CHAPS). BMC Health Serv Res. 2020;20(1):732. https://doi.org/10.1186/s12913-020-05594-8 .
Cotterell P. Parkinson’s disease: symptoms, treatment options and nursing care. Nurs Stand. 2018;33(7):53–8. https://doi.org/10.7748/ns.2018.e11207 .
Gardner R. Community and collaboration: a Parkinson’s nurse shares her leading lights. Pract Nurs. 2023;34(4):163. https://doi.org/10.12968/pnur.2023.34.4.163 .
Fincher L, Ward C, Dawkins V, Magee V, Willson P. Using telehealth to educate Parkinson’s disease patients about complicated medication regimens. J Gerontol Nurs. 2009;35(2):16–24. https://doi.org/10.3928/00989134-20090201-10 .
Fischer PP. Parkinson’s disease and the U.S. health care system. J Community Health Nurs. 1999;16(3):191–204. https://doi.org/10.1207/S15327655JCHN1603_5 .
Fleisher JE, Sweeney MM, Oyler S, Meisel T, Friede N, Di Rocco A, Chodosh J. Disease severity and quality of life in homebound people with advanced Parkinson disease: a pilot study. Neurol Clin Pract. 2020;10(4):277–86. https://doi.org/10.1212/CPJ.0000000000000716 .
Patel K. Optimising medication for Parkinson’s disease patients with dysphagia. Br J Community Nurs. 2015;20(7):322. https://doi.org/10.12968/bjcn.2015.20.7.322 .
Fleisher J, Barbosa W, Sweeney MM, Oyler SE, Lemen AC, Fazl A, et al. Interdisciplinary Home visits for individuals with Advanced Parkinson’s Disease and Related disorders. J Am Geriatr Soc. 2018;66(6):1226–32. https://doi.org/10.1111/jgs.15337 .
Oyler SE, Fleisher JE, Sweeney MM, Lemen AC, Fazl A, Dacpano G, et al. Medication errors: the role of the nurse in an interdisciplinary home visit program for advanced Parkinson’s disease patients. Mov Disord. 2016;31:S159–S.
Google Scholar
Soper C. Improving medicine concordance in a patient with Parkinson’s and dementia: a case study. Nurs Older People. 2021;33(4):20–6. https://doi.org/10.7748/nop.2021.e1301 .
Drey N, McKeown E, Kelly D, Gould D. Adherence to antiparkinsonian medication: an in-depth qualitative study. Int J Nurs Stud. 2012;49(7):863–71. https://doi.org/10.1016/j.ijnurstu.2012.01.012 .
MacMahon DG. Parkinson’s disease nurse specialists: an important role in disease management. Neurology. 1999;52(7 Suppl 3):S21–5.
CAS PubMed Google Scholar
Mancini F, van Halteren AD, Carta T, Thomas S, Bloem BR, Darweesh SKL. Personalized care management for persons with Parkinson’s disease: a telenursing solution. Clin Park Relat Disord. 2020;3:100070. https://doi.org/10.1016/j.prdoa.2020.100070 .
Jarman B, Hurwitz B, Cook A, Bajekal M, Lee A. Effects of community based nurses specialising in Parkinson’s disease on health outcome and costs: randomised controlled trial. BMJ. 2002;324(7345):1072–5. https://doi.org/10.1136/bmj.324.7345.1072 .
Coady V, Warren N, Bilkhu N, Ayton D. Preferences for rural specialist health care in the treatment of Parkinson’s disease: exploring the role of community-based nursing specialists. Aust J Prim Health. 2019;25(1):49–53. https://doi.org/10.1071/PY17119 .
Willows T, Dizdar N, Nyholm D, Widner H, Grenholm P, Schmiauke U, et al. Initiation of levodopa-carbidopa intestinal gel infusion using telemedicine (Video Communication System) facilitates efficient and well-accepted home Titration in patients with Advanced Parkinson’s Disease. J Parkinsons Dis. 2017;7(4):719–28. https://doi.org/10.3233/JPD-161048 .
Todd A, James CA. Apomorphine nodules in Parkinson’s disease: best practice considerations. Br J Community Nurs. 2008;13(10):457–63. https://doi.org/10.12968/bjcn.2008.13.10.31182 .
Duffley G, Lutz BJ, Szabo A, Wright A, Hess CW, Ramirez-Zamora A, et al. Home Health Management of Parkinson Disease Deep Brain Stimulation: a Randomized Clinical Trial. JAMA Neurol. 2021;78(8):972–81. https://doi.org/10.1001/jamaneurol.2021.1910 .
Gregory P, Morgan K, Lynall A. Improving sleep management in people with Parkinson’s. Br J Community Nurs. 2012;17(1):14–8. https://doi.org/10.12968/bjcn.2012.17.1.14 .
Duncan D. Role of the community nurse in Parkinson’s disease and lower urinary disorders. Br J Community Nurs. 2021;26(5):251–4. https://doi.org/10.12968/bjcn.2021.26.5.251 .
Iwasa Y, Saito I, Suzuki M. Differences in Home Health Nursing Care for patients with Parkinson’s Disease by Stage of Progress: patients in Hoehn and Yahr stages III, IV, and V. Parkinsons Dis. 2021;2021:8834998. https://doi.org/10.1155/2021/8834998 .
Chen Y, Zhang R, Lou Y, Li W, Yang H. Facilitators and barriers to the delivery of palliative care to patients with Parkinson’s disease: a qualitative study of the perceptions and experiences of stakeholders using the socio-ecological model. BMC Health Serv Res. 2023;23(1):215. https://doi.org/10.1186/s12913-023-09203-2 .
Brock P, Gray WK, Hand A, Oates LL, Walker RW. Improving and integrating care for Parkinson’s disease. Nurs Residential Care. 2018;20(12):615–20. https://doi.org/10.12968/nrec.2018.20.12.615 .
Chenoweth L, Sheriff J, McAnally L, Tait F. Impact of the Parkinson’s disease medication protocol program on nurses’ knowledge and management of Parkinson’s disease medicines in acute and aged care settings. Nurse Educ Today. 2013;33(5):458–64. https://doi.org/10.1016/j.nedt.2012.04.022 .
Eggers C, Dano R, Schill J, Fink GR, Hellmich M, Timmermann L, group CPNs. Patient-centered integrated healthcare improves quality of life in Parkinson’s disease patients: a randomized controlled trial. J Neurol. 2018;265(4):764–73. https://doi.org/10.1007/s00415-018-8761-7 .
Rusli KDB, Tan AJQ, Ong SF, Speed S, Lau Y, Liaw SY. Home-based nursing care competencies: a scoping review. J Clin Nurs. 2023;32(9–10):1723–37. https://doi.org/10.1111/jocn.16169 .
Radojević B, Dragašević-Mišković NT, Milovanović A, Svetel M, Petrović I, Pešić M, et al. Adherence to medication among Parkinson’s Disease patients using the adherence to refills and medications Scale. Int J Clin Pract. 2022;2022:6741280. https://doi.org/10.1155/2022/6741280 .
Chou KL, Zamudio J, Schmidt P, Price CC, Parashos SA, Bloem BR, et al. Hospitalization in Parkinson disease: a survey of National Parkinson Foundation Centers. Parkinsonism Relat Disord. 2011;17(6):440–5. https://doi.org/10.1016/j.parkreldis.2011.03.002 .
Shih TM, Sail KR, Jalundhwala YJ, Sullivan J, van Eijndhoven E, Zadikoff C, et al. The effect of functional status impairment on nursing home admission risk among patients with advanced Parkinson’s disease. J Med Econ. 2020;23(3):297–307. https://doi.org/10.1080/13696998.2019.1693383 .
Safarpour D, Thibault DP, DeSanto CL, Boyd CM, Dorsey ER, Racette BA, Willis AW. Nursing home and end-of-life care in Parkinson disease. Neurology. 2015;85(5):413–9. https://doi.org/10.1212/WNL.0000000000001715 .
van Rumund A, Weerkamp N, Tissingh G, Zuidema SU, Koopmans RT, Munneke M, et al. Perspectives on Parkinson disease care in Dutch nursing homes. J Am Med Dir Assoc. 2014;15(10):732–7. https://doi.org/10.1016/j.jamda.2014.05.009 .
Fasano A, Visanji NP, Liu LWC, Lang AE, Pfeiffer RF. Gastrointestinal dysfunction in Parkinson’s disease. Lancet Neurol. 2015;14(6):625–39. https://doi.org/10.1016/S1474-4422(15)00007-1 .
Pedrosa Carrasco AJ, Timmermann L, Pedrosa DJ. Management of constipation in patients with Parkinson’s disease. Npj Parkinson’s Disease. 2018;4(1):6. https://doi.org/10.1038/s41531-018-0042-8 .
Seppi K, Ray Chaudhuri K, Coelho M, Fox SH, Katzenschlager R, Perez Lloret S, et al. Update on treatments for nonmotor symptoms of Parkinson’s disease—an evidence-based medicine review. Mov Disord. 2019;34(2):180–98. https://doi.org/10.1002/mds.27602 .
Geng W, Ren M, Zhao F, Yang F, Liu H. Breaking ground: nursing-led approach to alleviating constipation in Parkinson’s disease. BMC Geriatr. 2023;23(1):657. https://doi.org/10.1186/s12877-023-04370-7 .
Fujita T, Babazono A, Li Y, Jamal A, Kim S-a. Hypnotics and injuries among older adults with Parkinson’s disease: a nested case–control design. BMC Geriatr. 2023;23(1):259. https://doi.org/10.1186/s12877-023-03944-9 .
Lennaerts H, Groot M, Rood B, Gilissen K, Tulp H, van Wensen E, et al. A Guideline for Parkinson’s Disease nurse specialists, with recommendations for clinical practice. J Parkinsons Dis. 2017;7:749–54. https://doi.org/10.3233/JPD-171195 .
Aye YM, Liew S, Neo SX, Li W, Ng HL, Chua ST, et al. Patient-centric care for Parkinson’s Disease: from hospital to the community. Front Neurol. 2020;11:502. https://doi.org/10.3389/fneur.2020.00502 .
Download references
Acknowledgements
We thank Michael Irvine, PhD, from Edanz (https://jp.edanz.com/ac) for editing a draft of this manuscript.
This work was supported by JSPS KAKENHI (grant numbers JP23H03231 and JP23K27921). The funding body did not participate in the design of the study; the collection, analysis, or interpretation of the data; or the writing of the manuscript.
Author information
Authors and affiliations.
Department of Health Sciences, Faculty of Medical Sciences, Kyushu University, 3-1-1 Maidashi, Higashi-ku, 812-8582, Fukuoka, Japan
Takako Fujita, Miho Iwaki & Yoko Hatono
You can also search for this author in PubMed Google Scholar
Contributions
TF, MI, and YH made substantial contributions to the study conception and design, the selection of and extraction of content from studies, and the drafting and revising of the manuscript; they also provided final approval of the manuscript.
Corresponding author
Correspondence to Takako Fujita .
Ethics declarations
Ethics approval and consent to participate, consent for publication, competing interests.
The authors declare that they have no conflict of interest.
Additional information
Publisher’s note.
Springer Nature remains neutral with regard to jurisdictional claims in published maps and institutional affiliations.
Rights and permissions
Open Access This article is licensed under a Creative Commons Attribution 4.0 International License, which permits use, sharing, adaptation, distribution and reproduction in any medium or format, as long as you give appropriate credit to the original author(s) and the source, provide a link to the Creative Commons licence, and indicate if changes were made. The images or other third party material in this article are included in the article’s Creative Commons licence, unless indicated otherwise in a credit line to the material. If material is not included in the article’s Creative Commons licence and your intended use is not permitted by statutory regulation or exceeds the permitted use, you will need to obtain permission directly from the copyright holder. To view a copy of this licence, visit http://creativecommons.org/licenses/by/4.0/ . The Creative Commons Public Domain Dedication waiver ( http://creativecommons.org/publicdomain/zero/1.0/ ) applies to the data made available in this article, unless otherwise stated in a credit line to the data.
Reprints and permissions
About this article
Cite this article.
Fujita, T., Iwaki, M. & Hatono, Y. The role of nurses for patients with Parkinson’s disease at home: a scoping review. BMC Nurs 23 , 318 (2024). https://doi.org/10.1186/s12912-024-01931-y
Download citation
Received : 28 February 2024
Accepted : 10 April 2024
Published : 11 May 2024
DOI : https://doi.org/10.1186/s12912-024-01931-y
Share this article
Anyone you share the following link with will be able to read this content:
Sorry, a shareable link is not currently available for this article.
Provided by the Springer Nature SharedIt content-sharing initiative
- Community care
BMC Nursing
ISSN: 1472-6955
- General enquiries: [email protected]
This paper is in the following e-collection/theme issue:
Published on 13.5.2024 in Vol 26 (2024)
Suitability of the Current Health Technology Assessment of Innovative Artificial Intelligence-Based Medical Devices: Scoping Literature Review
Authors of this article:

- Line Farah 1, 2 * , PharmD ;
- Isabelle Borget 2, 3, 4 , PharmB, PhD ;
- Nicolas Martelli 2, 5 , PharmD, PhD ;
- Alexandre Vallee 6 * , MD, PhD
1 Innovation Center for Medical Devices Department, Foch Hospital, Suresnes, France
2 Groupe de Recherche et d'accueil en Droit et Economie de la Santé Department, University Paris-Saclay, Orsay, France
3 Department of Biostatistics and Epidemiology, Gustave Roussy, University Paris-Saclay, Villejuif, France
4 Oncostat U1018, Inserm, Équipe Labellisée Ligue Contre le Cancer, University Paris-Saclay, Villejuif, France
5 Pharmacy Department, Georges Pompidou European Hospital, Paris, France
6 Department of Epidemiology and Public Health, Foch Hospital, Suresnes, France
*these authors contributed equally
Corresponding Author:
Line Farah, PharmD
Innovation Center for Medical Devices Department
Foch Hospital
40 rue Worth
Suresnes, 92150
Phone: 33 952329655
Email: [email protected]
Background: Artificial intelligence (AI)–based medical devices have garnered attention due to their ability to revolutionize medicine. Their health technology assessment framework is lacking.
Objective: This study aims to analyze the suitability of each health technology assessment (HTA) domain for the assessment of AI-based medical devices.
Methods: We conducted a scoping literature review following the PRISMA (Preferred Reporting Items for Systematic Reviews and Meta-Analyses) methodology. We searched databases (PubMed, Embase, and Cochrane Library), gray literature, and HTA agency websites.
Results: A total of 10.1% (78/775) of the references were included. Data quality and integration are vital aspects to consider when describing and assessing the technical characteristics of AI-based medical devices during an HTA process. When it comes to implementing specialized HTA for AI-based medical devices, several practical challenges and potential barriers could be highlighted and should be taken into account (AI technological evolution timeline, data requirements, complexity and transparency, clinical validation and safety requirements, regulatory and ethical considerations, and economic evaluation).
Conclusions: The adaptation of the HTA process through a methodological framework for AI-based medical devices enhances the comparability of results across different evaluations and jurisdictions. By defining the necessary expertise, the framework supports the development of a skilled workforce capable of conducting robust and reliable HTAs of AI-based medical devices. A comprehensive adapted HTA framework for AI-based medical devices can provide valuable insights into the effectiveness, cost-effectiveness, and societal impact of AI-based medical devices, guiding their responsible implementation and maximizing their benefits for patients and health care systems.
Introduction
Artificial intelligence (AI) has emerged as a transformative technology with vast potential across various sectors, including health care [ 1 , 2 ]. In this field, AI-based medical devices have garnered significant attention due to their ability to revolutionize diagnosis, treatment, and patient monitoring [ 3 ]. These devices use advanced algorithms and machine learning techniques to analyze complex medical data sets, thereby providing valuable insights and support to health care professionals in their decision-making. To meet the ever-increasing demand for integration of AI-based medical devices into clinical practice, their efficient evaluation through adapted health technology assessment (HTA) is now crucial [ 4 ]. Several frameworks have been published showing an adaptation of items for reporting clinical trials related to AI-based medical devices ( Table 1 ). However, there is still a lack of a much needed adaptation of the standard HTA framework to better suit the assessment of AI-based health care technologies.
a HTA: health technology assessment.
b CONSORT-AI: Consolidated Standards of Reporting Trials–Artificial Intelligence.
c CONSORT: Consolidated Standards of Reporting Trials.
d SPIRIT-AI: Standard Protocol Items: Recommendations for Interventional Trials–Artificial Intelligence.
e SPIRIT: Standard Protocol Items: Recommendations for Interventional Trials.
f TRIPOD-AI: Transparent Reporting of a Multivariable Prediction Model for Individual Prognosis or Diagnosis-Artificial Intelligence.
g TRIPOD: Transparent Reporting of a Multivariable Prediction Model for Individual Prognosis or Diagnosis.
An HTA involves a systematic evaluation of a medical technology’s clinical, economic, ethical, and social implications to determine its overall value and impact on health care delivery [ 9 ]. The European Network for Health Technology Assessment has designed an HTA Core Model that provides a methodological framework for production and sharing of HTA information [ 5 ]. It evaluates the following nine domains: (1) health problem and current use of technology, (2) description and technical characteristics of the technology, (3) safety, (4) clinical effectiveness, (5) costs and economic evaluation, (6) ethical analysis, (7) organizational aspects, (8) patients and social aspects, and (9) legal aspects ( Multimedia Appendix 1 ).
A full understanding of the capabilities, limitations, and specificities of AI-based medical devices is paramount to complete these HTA domain assessments and, thereby, inform evidence-based decision-making and allow for policy development and the responsible integration of these technologies into health care systems [ 10 , 11 ]. Much uncertainty remains with regard to the reliability of AI-based medical devices, data issues, and regulatory processes, resulting in multiple challenges faced by HTA agencies assessing new technologies and delivering their approval [ 4 ]. Nevertheless, AI-based medical devices require strict regulations and specific legislations [ 12 - 14 ]. Over the last few decades, the evaluation of AI-based medical devices through an HTA process has received growing interest, as shown by the increase from 1 published article in 1990 to 94 in December 2023, with 484 articles in total during the period 1990-2023 ( Table 2 ).
The objective of this review was to critically assess the comprehensive suitability of the current HTA process for AI-based medical devices. By evaluating the performance and capabilities of AI-based medical devices across multiple dimensions, this review aimed to inform health care professionals, policy makers, and researchers about the challenges and opportunities associated with these technologies. Ultimately, this review sought to facilitate evidence-based decision-making; promote responsible implementation; and maximize the potential benefits of AI-based medical devices in improving health care quality, accessibility, and outcomes.
To this end, we analyzed the suitability of each HTA domain for the assessment of AI-based medical devices and proposed an adapted HTA framework.
Search Strategies
A scoping literature review was conducted following the PRISMA (Preferred Reporting Items for Systematic Reviews and Meta-Analyses) methodology [ 15 , 16 ]. All articles related to HTA methods for AI-based medical devices were selected. Data extraction focused on assessment criteria, methodological evaluations, and results.
The search terms and strategy outlined in Textbox 1 were used for the scoping review.
Database and search strategy
- “technology assessment, biomedical” [MeSH term] OR (“technology” [all fields] AND “assessment” [all fields] AND “biomedical” [all fields]) OR “biomedical technology assessment” [all fields] OR (“health” [all fields] AND “technology” [all fields] AND “assessment” [all fields]) OR “health technology assessment” [all fields]
- “equipment and supplies” [MeSH term] OR (“equipment” [all fields] AND “supplies” [all fields]) OR “equipment and supplies” [all fields] OR (“medical” [all fields] AND “device” [all fields]) OR “medical device” [all fields]
- “artificial intelligence” [MeSH term] OR (“artificial” [all fields] AND “intelligence” [all fields]) OR “artificial intelligence” [all fields]
- (“artificial intelligence” OR “artificial intelligence-based medical device”) AND “technology assessment”
- (technology assessment) and (artificial intelligence)
We searched multiple databases: PubMed, Embase, and the Cochrane Library. Additional articles were retrieved manually from the gray literature and from HTA agency websites (Bundesinstitut für Arzneimittel und Medizinprodukte [Federal Institute for Drugs and Medical Devices in Germany], Haute Autorité de Santé [French Health Authority], National Health Service, National Institute for Health and Care Excellence, National Institute of Public Health, International Network of Agencies for Health Technology Assessment, and the European Network for Health Technology Assessment).
Study Selection Process
The studies were selected by 2 reviewers (LF and AV). After removing duplicates, both reviewers independently screened the abstracts to select eligible articles and then analyzed full-text reports for eligibility. A third party (NM) resolved the possible discrepancies highlighted during the selection process should a consensus not be reached. An extraction database was used to list the selected articles meeting the inclusion criteria to ensure that all eligible articles were included.
The inclusion and exclusion criteria were related to (1) language (this review was limited to the English and French languages), (2) article type (reviews and primary research were included, whereas other article types were excluded, such as abstracts, commentaries, editorials, letters to the editor, case reports, case series, animal studies, phase-1 and phase-2 studies, pilot studies, duplicate studies, irrelevant studies, studies with the wrong aim, and studies available in abstract form only), (3) type of technology (only AI-based medical devices were eligible), and (4) type of evaluation (HTA articles were included).
Data Extraction
In total, 2 analysts (LF and AV) independently extracted data items from the selected articles. A third party was involved to resolve any discrepancies highlighted during the selection process. The following data were extracted from each article: (1) general characteristics of the studies (authors, journal, and publication date), (2) study objective, and (3) HTA assessment domain for AI-based medical devices related to the article.
Methodological Quality Appraisal
Neither the methodological quality nor the risk of bias of the included articles were assessed, consistent with scoping review guidance [ 15 ].
Application Example of the HTA of an AI-Based Medical Device
Concerning each HTA domain, we added a case study of the HTA of several AI-based medical devices in diabetes to illustrate our recommendations.
Scoping Review Results
The literature search resulted in 775 citations summarized in the PRISMA flowchart ( Figure 1 ). After removal of 32.6% (253/775) of duplicates, we carried out an initial screening of the remaining 522 publications, resulting in 129 (24.7%) potentially relevant full-text papers. After further screening, we assessed 77 reports for eligibility, based on which we excluded 11 (14%) for not referring to AI-based medical devices and 2 (3%) for not being HTAs. We then included a further 14 records from 59 potential citations available from HTA agency websites and gray literature.

This gave a total of 78 included articles covering one or multiple HTA domains, the distribution of which is provided at the bottom of Figure 1 .
We then summarized the data collection for each selected article in Multimedia Appendix 2 [ 1 , 2 , 4 , 9 , 10 , 17 - 89 ].
Suitability of Each HTA Domain for the Assessment of AI-Based Medical Devices
As domain 1, health problem and current use of technology, is suitable for any type of medical technology and systematically addressed these technologies, we focused on the suitability of the HTA domains (from 1 to 9).
Domains 1 and 2 (Health Problem, Current Use, Description, and Technical Characteristics of the Technology)
Data quality and integration are vital aspects to consider when describing and assessing the technical characteristics of AI-based medical devices during an HTA process [ 17 ]. These devices often rely on accessing and analyzing diverse health care data sources, including electronic health records, medical images, genetic data, and wearable device data. Therefore, it is essential to evaluate their ability to seamlessly integrate and exchange data with existing health care systems [ 18 ].
First, interoperability refers to the ability of AI-based medical devices to interact and communicate with other health care technologies and systems [ 10 ]. This includes the ability to access and use data from different sources, such as laboratory systems, imaging archives, and patient health records. The assessment should consider whether the devices adhere to relevant data standards and protocols, ensuring efficient data exchange and compatibility with existing health care infrastructures [ 19 ].
Second, data integration involves the ability of AI-based medical devices to aggregate and analyze data from multiple sources to provide comprehensive and accurate insights [ 20 ]. The HTA should assess whether the devices can handle different data types, formats, and resolutions and whether they can effectively integrate and harmonize data from disparate sources.
In addition, data privacy and security considerations are crucial when evaluating AI-based medical devices [ 21 ]. The assessment should examine whether the devices comply with relevant data protection regulations, use appropriate data anonymization and encryption techniques, and have robust security measures in place to protect patient information [ 22 ].
Therefore, a comprehensive HTA should address both the interoperability and data integration capabilities of AI-based medical devices, ensuring that they can seamlessly interact with existing health care systems and integrate data from multiple sources and that they adhere to data privacy and security standards. By evaluating all these aspects, the HTA could determine the devices’ feasibility, scalability, and potential impact on health care delivery.
Domain 3 (Safety) and Domain 4 (Clinical Effectiveness)
The assessment of clinical effectiveness and the impact on patient outcomes is a crucial aspect when evaluating AI-based medical devices through a comprehensive HTA [ 4 ]. While accuracy and performance metrics are important, it is essential to determine how these devices translate into tangible benefits for patients and health care delivery [ 23 ].
Clinical utility refers to the extent to which the AI-based medical device improves clinical decision-making, patient outcomes, and health care processes [ 24 ]. The HTA should examine whether the device provides actionable and reliable information that potentially helps health care professionals make more accurate diagnoses or improve treatment plans or monitoring strategies [ 25 , 26 ]. It should also evaluate the potential impact of the device on patient outcomes by assessing, for example, improved survival rates, reduced complications, or enhanced quality of life [ 27 ].
To assess clinical utility, the HTA should consider the device’s performance in relevant clinical scenarios and its ability to address specific clinical questions or challenges. This may involve evaluating the device’s performance against established clinical guidelines or expert opinions as well as considering the device’s potential to fill gaps in clinical practice or enhance existing diagnostic or treatment methods.
Furthermore, the HTA should examine the broader impact of AI-based medical devices on health care systems and resource allocation [ 21 ]. This includes evaluating the devices’ potential to optimize resource use, reduce health care costs, or improve workflow efficiency. Economic evaluations such as cost-effectiveness analyses can provide insights into the value for money and long-term cost savings associated with the adoption of these devices [ 28 , 29 ].
A comprehensive HTA should thoroughly assess the clinical effectiveness and impact on patient outcomes of AI-based medical devices, examining their performance in relevant clinical scenarios, their alignment with clinical guidelines, and their potential to improve health care processes and resource allocation. By evaluating these aspects, the HTA can provide a holistic understanding of the AI devices’ effectiveness and their potential to positively transform health care delivery [ 21 ].
AI-based medical devices must undergo rigorous clinical validation to assess their performance and reliability in real-world health care settings [ 30 , 31 ]. Clinical validation involves evaluating the device’s accuracy, sensitivity, specificity, and overall diagnostic or prognostic performance. In addition, the devices should be tested across diverse patient populations and compared against gold-standard reference methods or expert opinions [ 32 ].
Furthermore, the use of real-world evidence (RWE) is crucial for a comprehensive HTA [ 33 ]. RWE involves gathering data from routine clinical practice, electronic health records, and other sources to evaluate the device’s effectiveness and safety in real-world settings [ 34 ]. These data can help assess the device’s performance in a broader patient population and identify any potential limitations or biases that may arise in specific clinical scenarios.
Robust clinical validation studies and the integration of RWE provide critical evidence for the evaluation of AI-based medical devices in an HTA process [ 4 ]. These studies should include a sufficient sample size, appropriate study design, and statistical analysis to ensure the validity and generalizability of the results [ 35 - 38 ]. By considering clinical validation and RWE adapted to AI-based medical devices, HTA can provide valuable insights into their clinical utility and impact in real-world health care settings, facilitating evidence-based decision-making for their adoption and use.
A lack of safety evaluation was highlighted in a systematic review, showing that only 9% of AI-based medical device studies evaluated safety criteria [ 4 ]. However, safety is crucial for the confidence in and adoption of AI-based technologies for both patients and health care professionals [ 39 ]. While these devices have the potential to improve diagnosis and treatment outcomes, their integration into clinical practice must be accompanied by robust safety evaluations [ 2 , 40 ]. Identifying and mitigating potential risks, such as algorithmic bias, data privacy breaches, and algorithm failures, is essential to protect patient well-being and maintain trust in these innovative technologies [ 41 ].
To ensure the safety of AI-based medical devices, it is crucial to establish standardized evaluation frameworks and guidelines [ 39 ]. These frameworks should encompass rigorous testing methodologies, validation procedures, and continuous monitoring of AI performance. Collaboration among stakeholders, including manufacturers, regulatory agencies, health care providers, and researchers, is essential to develop and implement comprehensive safety evaluation protocols. Addressing the safety gap in AI-based medical devices not only ensures patient welfare but also instills confidence in health care professionals to embrace and use these technologies effectively [ 40 ]. By prioritizing safety evaluation, we can unleash the full potential of AI-based medical devices, leading to transformative advancements in health care delivery [ 1 ].
Domain 5 (Costs and Economic Evaluation)
Costs and economic evaluation play a crucial role in the comprehensive assessment of health technology adoption, particularly in the context of the HTA of AI-based medical devices [ 4 , 9 , 42 ]. While AI technologies show huge potential to improve health care delivery, reduce time to diagnosis, and save money, their economic impact requires careful consideration to ensure their successful integration and sustainability [ 23 ].
When assessing AI-based medical devices through the HTA process, economic evaluations involve analyzing the costs and benefits associated with the adoption and use of the technology [ 4 ]. These evaluations go beyond the upfront costs of acquiring the AI-based medical device; they encompass various factors, such as training, infrastructure modifications, maintenance, and ongoing operational costs [ 43 ]. Cost analysis also includes improved patient outcomes, reduced hospital readmissions, and shortened hospital stays. By conducting economic evaluations, decision makers can gauge the cost-effectiveness and cost utility to weigh the affordability of AI-based medical devices for their particular use against the potential mid- and long-term cost savings considered [ 44 ].
HTA evaluates the suitability of AI-based medical devices by considering their potential economic benefits, risks, and ethical implications [ 45 , 46 ]. By incorporating economic evaluation into the HTA process, decision makers can make evidence-based choices about the allocation of limited health care resources and prioritize interventions that provide the greatest value for money [ 4 , 47 ].
Finally, considering cost savings and conducting economic evaluations as part of the HTA process helps facilitate the adoption of AI-based medical devices [ 37 ]. It provides decision makers with the necessary information to determine the financial feasibility and potential return on investment associated with implementing these technologies. HTA ensures that AI-based medical devices are suitable for integration into the health care system, fostering confidence in their effectiveness, efficiency, and cost utility [ 4 , 43 , 48 ].
Domain 6 (Ethical Analysis) and Domain 8 (Patients and Social Aspects)
Ethical and societal implications are critical aspects that must be considered when evaluating the suitability of AI-based medical devices for a comprehensive HTA [ 45 ]. The integration of AI technologies into health care raises important ethical concerns that need to be addressed to ensure responsible and equitable use [ 49 ].
Data privacy and patient consent are primary ethical considerations [ 50 ]. The HTA should evaluate whether AI-based medical devices adhere to strict data protection regulations, maintain patient confidentiality, and obtain appropriate informed consent for data use. It is essential to assess whether the devices have mechanisms in place to handle sensitive patient information securely and protect it against unauthorized access or data breaches.
Algorithmic fairness and bias are additional ethical concerns [ 51 ]. AI algorithms can inadvertently perpetuate biases present in the data used for training, resulting in unequal treatment or access to health care resources [ 52 ]. The HTA should assess whether the devices have been evaluated for fairness and bias and consider the steps taken to mitigate any identified biases [ 53 ].
Moreover, the HTA should examine the impact of AI-based medical devices on health care disparities and access to care [ 54 ]. It is crucial to assess whether the devices have the potential to exacerbate existing inequalities or whether they can contribute to reducing disparities by improving health care access, particularly for underserved populations.
Accountability and transparency in AI decision-making processes are also important ethical considerations [ 55 ]. The HTA should evaluate whether the AI devices provide clear explanations for their outputs and ensure that health care professionals and patients can understand and challenge the device’s recommendations [ 21 ].
A comprehensive HTA should thoroughly examine the ethical and societal implications of AI-based medical devices, ensuring that they prioritize patient privacy, fairness, and equitable access to care; fostering trust; and ensuring that these devices align with societal values and goals.
To ensure the adoption of AI-based medical devices through a truthfully AI concept, explainability, interpretability, and transparency are important considerations for their comprehensive HTA [ 56 ]. Indeed, these devices often use complex algorithms and machine and deep learning techniques, resulting in their operating as “black boxes” that reach decisions and recommendations that are challenging to understand [ 55 ]. However, explainability and transparency are essential to ensure trust, accountability, and acceptance of AI-based technologies in health care. First, Explainability refers to the ability to understand and interpret the reasoning behind the device’s outputs [ 56 ]. It involves providing clinicians and users with transparent explanations of how the AI algorithm processes input data and generates results. This enables health care professionals to trust the device’s recommendations and make informed decisions based on the provided information. The HTA should evaluate the extent to which AI-based medical devices can provide interpretable and understandable explanations of their decision-making processes [ 17 , 46 ]. Second, Transparency involves disclosing important information about the AI-based medical devices, including the data used for training, algorithmic methodologies used, and potential limitations or biases [ 57 ]. Transparency promotes trust and allows stakeholders to assess the device’s reliability and potential risks. The HTA should assess whether the device manufacturers provide clear documentation and information to health care professionals and patients about the device’s capabilities, limitations, and potential errors [ 21 , 58 , 59 ]. Transparency is closely linked to regulatory considerations [ 60 , 61 ]. The HTA should consider whether the device complies with relevant regulatory standards and whether the manufacturers have provided the necessary documentation and evidence to support their ethical claims [ 21 , 62 , 63 ].
A comprehensive HTA process should address concerns regarding the explainability and transparency of AI-based medical devices, trust, accountability, and ethical implications related to the use of AI in health care [ 45 , 46 ].
Domain 7 (Organizational Aspects)
Organizational aspects play a crucial role in the effective integration of AI-based medical devices into health care systems [ 64 ]. To ensure consistency, transparency, and comparability in the evaluation process, there is a growing need for a robust methodological framework that provides standardized guidelines for assessing the organizational impact of the implementation of AI-based medical devices [ 65 ]. According to a descriptive analysis led in German hospitals, the main barriers to AI-based medical device adoption were lack of resources (staff, knowledge, and financial) [ 66 ].
Clear indicators are needed to measure the organizational readiness for and impact of AI-based medical devices [ 4 , 10 , 67 ]. Several criteria have been highlighted, such as (1) health care workplace readiness and stakeholder acceptance, (2) AI-based medical device organization alignment assessment, and (3) business plan (financing and investments) [ 64 ].
Domain 9 (Legal Aspects)
The roles and responsibilities of health care professionals are also impacted by these AI solutions [ 68 ]. It is necessary to evaluate whether they complement or replace health care professionals’ expertise and whether additional training, supervision, or support is required for their optimal use [ 69 , 70 ]. As AI technologies could evolve and become more prevalent in health care, it is crucial to ensure that these devices comply with existing legal frameworks and regulations [ 49 , 71 ]. HTA plays a pivotal role in evaluating the legal implications of AI-based medical devices by assessing factors such as data privacy, security, liability, and regulatory compliance [ 72 ].
One key legal aspect to consider is data privacy and protection [ 73 , 74 ]. AI-based medical devices often rely on vast amounts of patient data for training and decision-making. Therefore, it is essential to evaluate whether these devices adhere to relevant data protection laws, such as the General Data Protection Regulation in the European Union [ 75 , 76 ]. HTA examines the measures taken by AI-based medical device manufacturers to safeguard patient privacy, including data anonymization, encryption, and secure data storage practices [ 37 ]. These issues are highlighted in the Artificial Intelligence Act published in June 2023 by the European Commission [ 77 , 78 ].
Cybersecurity is another critical consideration [ 21 , 79 ]. As AI-based medical devices handle sensitive patient data and make critical health care decisions, it is crucial to assess the security measures implemented to prevent unauthorized access, data breaches, or tampering [ 80 ]. HTA evaluates the robustness of the security protocols implemented by device manufacturers and their compliance with industry standards and regulations [ 21 , 81 ].
Liability is also a significant legal aspect to be addressed in the context of AI-based medical devices [ 82 , 83 ]. When errors or adverse events occur due to the use of these devices, determining liability can be complex [ 84 ]. HTA examines the legal frameworks and liability guidelines pertaining to AI technologies, including whether clear guidelines exist regarding the responsibility of manufacturers, health care providers, and users in case of device malfunctions or errors [ 85 ]. Assessing liability aspects within the HTA process helps establish accountability and ensures that legal frameworks adequately address potential risks [ 86 ].
Regulatory compliance is a crucial consideration when assessing the suitability of AI-based medical devices for HTA [ 87 ]. Depending on the jurisdiction, AI devices need to undergo regulatory approval processes before being introduced into the market [ 88 ]. HTA examines whether AI-based medical device manufacturers have obtained the necessary regulatory approvals, such as clearance from the relevant health authorities or certification from regulatory bodies such as the Food and Drug Administration (FDA) in the United States or notified bodies in Europe [ 89 ]. This evaluation ensures that AI-based medical devices comply with existing regulations and are fit for clinical use [ 61 ].
Recommendations to Adapt the 9 HTA Domains for AI-Based Medical Devices
Taking into account the previous considerations, some recommendations could be proposed to adapt and personalize these standard HTA domains to AI-based medical devices’ specificities. Therefore, we suggest 4 main recommendations by domain in Table 3 .
a ML: machine learning.
b DL: deep learning.
c DMS: diabetes management system.
d FDA: Food and Drug Administration.
The adaptation of the HTA process through a methodological framework for AI-based medical devices enhances the comparability of results across different evaluations and jurisdictions [ 90 - 92 ]. It promotes consistency in the assessment methodologies, reporting formats, and presentation of findings, enabling decision makers to make informed choices based on reliable and comparable evidence. This standardization contributes to the overall credibility and acceptance of HTA outcomes related to AI-based medical devices [ 93 ]. In addition, a methodological framework would address the need for appropriate expertise and skills to conduct the HTA of AI-based medical devices [ 94 - 99 ]. It would outline the qualifications and competencies required for the individuals involved in the assessment, including knowledge of AI technologies [ 46 ]. By defining the necessary expertise, the framework supports the development of a skilled workforce capable of conducting robust and reliable HTAs of AI-based medical devices ( Figure 2 ).

Evaluating the trade-offs and weighing different features of AI-based medical devices is indeed a complex and important task, especially in domains such as health care where the impact on human lives is significant. The acceptability of AI-based medical devices should be assessed on a case-by-case basis considering various factors, including, for instance, performance, accuracy, cost, explainability, and the specific context in which they are being used.
Trade-offs between accuracy and cost are common in AI. It may be acceptable for AI to increase follow-up care costs if it significantly improves accuracy and patient outcomes. For example, if an AI system can detect diseases at an earlier stage, it might lead to more effective treatment and, ultimately, lower overall health care costs in the long run.
The balance between explainability and performance is a critical consideration. While explainable algorithms are preferred for safety and transparency reasons, there may be cases in which a highly complex, unexplainable algorithm outperforms explainable ones. In such cases, the trade-off between transparency and performance should be carefully evaluated based on the specific use case and potential risks.
The minimum performance for adding this technology to the tools available to human clinicians depends on the specific task and the level of trust that patients, health care providers, and regulators have in the technology. Some key factors to consider include the following: (1) The complexity of the task—AI-based medical devices should excel in tasks that are well defined and data driven, but they may not replace human clinicians in tasks requiring complex decision-making, empathy, or ethical considerations. (2) Safety and reliability—AI-based medical devices should demonstrate a high level of safety and reliability, ideally surpassing the performance of human clinicians in terms of avoiding errors. (3) Ethical considerations—AI-based medical devices should adhere to ethical standards, including patient privacy, informed consent, and unbiased decision-making, which are often considered even more important than performance metrics. (4) Regulatory approval—regulatory bodies often establish performance thresholds for AI-based medical devices. Compliance with these thresholds is essential for market acceptance.
In general, AI should aim to complement and enhance the capabilities of human clinicians rather than completely replacing them. The specific threshold for acceptable performance will vary across applications and contexts, and it should be determined through a combination of rigorous testing, peer-reviewed studies, and input from health care professionals and patients.
It is important to note that ethical considerations, patient safety, and the potential for bias should always be at the forefront of these discussions, and AI-based medical devices should not be adopted solely for the sake of automation or cost reduction if they compromise these critical aspects of health care.
Use Case of the Application of the Aforementioned HTA Recommendations for AI: AI-Based Medical Devices in Pathways for Patients With Diabetes
Concerning the HTA domain 3 on the safety of an AI-based medical device, the potential risk of patient injury of an insulin delivery AI-based medical device system should be taken into account in the risk management process of algorithm development [ 96 ]. In the case of an evolutive deep learning–based medical device without continuous ongoing safety assessment, a wrong dosage administration due to an AI error, for instance, could provoke a serious adverse event such as an acid ketosis coma for a patient with diabetes.
A risk management plan should be available for users and regularly updated with the impact of the AI changes on the safety plan.
In relation to the HTA domain 4, which focuses on the effectiveness of an AI-based medical device, the FDA in the United States has proposed a regulatory framework for modifications to evolutive AI- and machine learning–based software as a medical device with modification guidance focused on the risk to users or patients resulting from the AI changes [ 100 ]. They have asked for an Algorithm Change Protocol with specific methods in place to achieve and control the risks of the anticipated types of modifications related to performance, use, or inputs. A continuous clinical effectiveness assessment could be an interesting approach for an AI-based medical device detection system for diabetes foot ulcer for patients needing adaptative treatment modifications to prevent ulcer development [ 101 ].
Concerning the HTA domain 5, to evaluate a diabetic retinopathy screening AI-based medical device, clinical effectiveness is not sufficient. The cost-effectiveness of different types of AI diabetic retinopathy screening should be compared with no screening and ophthalmologist screening. A recent study demonstrated that AI-based screening was the most cost-effective, not only saving costs but also improving the quality of life of patients with diabetes [ 44 ]. In this case, the long-term assessment of the economic impact of AI introduction in diabetic retinopathy screening highlighted the added value of this technology.
The HTA domain 7 on organizational impact has to assess how the AI-based medical device can be effectively integrated into the health care pathway and prevent wasteful spending. More thorough attention must be paid to the following aspects: (1) evaluating needs and determining the added value of the implementation of the AI-based medical device; (2) assessing workplace preparedness, including stakeholder acceptance of the introduction of the AI-based medical device and involvement; and (3) analyzing the alignment between AI technology and organizational structure [ 64 ]. Decision makers and technology advocates need to address the complexities of AI more comprehensively and understand the systemic challenges that its adoption poses to health care organizations and systems. As an example, consider an AI tool used for diagnosing diabetic retinopathy in a primary care setting, such as by a family physician or nurse [ 102 ]. In theory, this could lead to shorter waiting times for patients. However, if the health care organization faces challenges such as a shortage of specialized staff (eg, ophthalmologists), insufficient organization of care pathways, and lack of specialized facilities for proper management and follow-up after diagnosis, the introduction of AI might adversely affect the quality of care and patient experience. In such a scenario, the AI application might merely transfer the delay from primary to secondary care, failing to address the fundamental issue.
Finally, concerning the last domains (6, 8, and 9) about ethical, patient, social, and legal aspects, AI-based continuous glucose monitoring and insulin pumps should also be assessed on ethical and legal aspects [ 103 ]. While citizen juries have generally shown support for AI in research and treatment, concerns remain. The risks of data theft and privacy breaches necessitate careful consideration of ethical and legal issues for patients. Although AI can aid in decision-making, it cannot wholly substitute a physician’s expertise. Effective regulations and systems designed to ensure safety, reduce bias, and enhance transparency are essential.
By implementing these recommendations, stakeholders can foster the responsible integration, regulation, and evaluation of AI-based medical devices. These measures can enhance the evidence base, address ethical concerns, and maximize the potential benefits of these AI technologies in improving health care outcomes while protecting patient safety, privacy, and equity.
Practical Challenges and Potential Barriers to Implementing HTAs Specific for AI-Based Medical Devices
When it comes to implementing specialized HTAs for AI-based medical devices, several practical challenges and potential barriers could be encountered and should be taken into account: First, AI technological evolution timeline—AI technologies evolve at a much faster pace compared to traditional medical devices, making it challenging for HTA frameworks to keep up with the latest developments and assess their long-term impact effectively. AI-based medical devices have a short product lifetime, between 12 and 18 months, in contrast to drug products. This shorter life cycle highlights the need for evolutive and fast-track HTA processes for AI-based medical devices. Second, data requirements and quality—AI systems rely heavily on large data sets for training and validation. Ensuring the availability of high-quality, representative data is a significant challenge. There is also the issue of data privacy and security, which must be addressed. The availability and quality of data and evidence required for conducting HTAs on AI-based medical devices present a complex and evolving landscape. Assessing the feasibility and challenges associated with gathering such data is crucial for robust evaluations. First, the availability of data can vary significantly depending on the AI-based medical device. While some devices may have access to vast amounts of high-quality real-world patient data, others might face limitations due to the novelty of the technology or issues related to data privacy. Second, the quality of data is paramount as inaccurate or biased data can lead to flawed assessments. Ensuring data accuracy, representativeness, and relevance is a constant challenge in AI-based medical device evaluations [ 104 ]. The rapid pace of AI development can result in limited long-term data, making it difficult to assess the device’s sustained performance and safety [ 105 ]. Balancing the need for robust evidence with the dynamic nature of AI technologies is a significant challenge that HTA organizations must address to provide valuable insights for informed decision-making in health care. Third, complexity and transparency—the complex algorithms used in AI-based medical devices can be difficult to understand and assess, leading to issues with transparency and explainability [ 17 ]. This complexity can pose a challenge for regulators and assessors in HTA processes. Fourth, clinical validation and safety requirements—generating robust clinical evidence to demonstrate the safety, efficacy, and effectiveness of AI-based medical devices can be challenging. This includes proving that these devices perform consistently across diverse patient populations. Moreover, to account for a life cycle that would include updates that may improve performance, one solution to investigate could be the proposition of the FDA in the United States in 2019 to experiment with a “dynamic certification” [ 100 ]. It allows for the re-evaluation of the AI-based medical device in case of a substantial modification of the indication of the medical device or the way to deliver the diagnosis, for instance. Fifth, regulatory and ethical considerations—adapting existing regulatory frameworks to accommodate AI-based medical devices, addressing ethical concerns such as bias, and ensuring equitable access are critical challenges [ 106 ]. Sixth, economic evaluation—determining the cost-effectiveness of AI-based medical devices, especially when benefits might be indirect or long term, poses a unique challenge for HTA [ 93 ]. Seventh, stakeholder engagement and trust—building trust among health care providers, patients, and policy makers regarding the reliability, trustworthiness, and usefulness of AI-based medical devices is crucial but challenging [ 107 ]. Eighth, integration into health care systems and interoperability—the integration of AI-based medical devices into existing health care workflows and systems can be complex and resource intensive [ 18 ]. Ninth, global and local applicability—ensuring that AI-based medical devices are effective and appropriate for use in different global and local contexts, considering varying health care systems and population needs, is another significant barrier.
Practically, one suggestion to implement such frameworks could be to implement these recommendations in the future European clinical joint assessment guidelines. As they are being currently discussed in a European project on a common HTA process for connected medical devices that includes AI-based medical devices, it could be the opportunity to tackle these challenges at the European level [ 108 ].
Addressing these challenges requires a concerted effort from regulators, health care providers, technology developers, and other stakeholders in the health care ecosystem.
Conclusions
AI-based medical devices have the potential to transform health care delivery, but the suitability of the current comprehensive HTA requires careful adaptation of the evaluation across the 9 dimensions. While these AI devices show promise in improving accuracy, safety, and efficiency, there is a need for robust clinical validation, integration into workflows, economic evaluation, and addressing of ethical and legal implications. A comprehensive adapted HTA framework for AI-based medical devices can provide valuable insights into their effectiveness, cost-effectiveness, and societal impact, guiding their responsible implementation and maximizing their benefits for patients and health care systems.
Data Availability
The data sets generated during and analyzed during this study are available from the corresponding author on reasonable request.
Authors' Contributions
LF and AV contributed to the conceptualization. LF and AV contributed to the methodology. LF, AV, NM, and IB contributed to the article selection. LF and AV contributed to the writing and original draft preparation. NM and IB contributed to the writing and review and editing. LF and AV contributed to the validation. The authors have read and agreed to the published version of the manuscript.
Conflicts of Interest
None declared.
The 9 health technology assessment (HTA) domains for the assessment of artificial intelligence–based medical devices adapted from the HTA Core Model proposed by the European Network for Health Technology Assessment.
Summary results of the scoping review and general characteristics of the studies included in the scoping review.
- Bajwa J, Munir U, Nori A, Williams B. Artificial intelligence in healthcare: transforming the practice of medicine. Future Healthc J. Jul 2021;8(2):e188-e194. [ CrossRef ] [ Medline ]
- Bohr A, Memarzadeh K. The rise of artificial intelligence in healthcare applications. In: Artificial Intelligence in Healthcare. Cambridge, MA. Academic Press; 2020.
- Park CW, Seo SW, Kang N, Ko B, Choi BW, Park CM, et al. Artificial intelligence in health care: current applications and issues. J Korean Med Sci. Nov 02, 2020;35(42):e379. [ CrossRef ] [ Medline ]
- Farah L, Davaze-Schneider J, Martin T, Nguyen P, Borget I, Martelli N. Are current clinical studies on artificial intelligence-based medical devices comprehensive enough to support a full health technology assessment? A systematic review. Artif Intell Med. Jun 2023;140:102547. [ CrossRef ] [ Medline ]
- HTA core model. European Network for Health Technology Assessment. URL: https://www.eunethta.eu/hta-core-model/ [accessed 2021-10-20]
- Liu X, Cruz Rivera S, Moher D, Calvert MJ, Denniston AK, SPIRIT-AI and CONSORT-AI Working Group. Reporting guidelines for clinical trial reports for interventions involving artificial intelligence: the CONSORT-AI extension. Nat Med. Sep 2020;26(9):1364-1374. [ CrossRef ] [ Medline ]
- Rivera SC, Liu X, Chan AW, Denniston AK, Calvert MJ, SPIRIT-AICONSORT-AI Working Group. Guidelines for clinical trial protocols for interventions involving artificial intelligence: the SPIRIT-AI Extension. BMJ. Sep 09, 2020;370:m3210. [ CrossRef ] [ Medline ]
- Collins GS, Dhiman P, Andaur Navarro CL, Ma J, Hooft L, Reitsma JB, et al. Protocol for development of a reporting guideline (TRIPOD-AI) and risk of bias tool (PROBAST-AI) for diagnostic and prognostic prediction model studies based on artificial intelligence. BMJ Open. Jul 09, 2021;11(7):e048008. [ CrossRef ] [ Medline ]
- Chen Y. Health technology assessment and economic evaluation: is it applicable for the traditional medicine? Integr Med Res. Mar 2022;11(1):100756. [ CrossRef ] [ Medline ]
- He J, Baxter SL, Xu J, Xu J, Zhou X, Zhang K. The practical implementation of artificial intelligence technologies in medicine. Nat Med. Jan 2019;25(1):30-36. [ CrossRef ] [ Medline ]
- Giordano C, Brennan M, Mohamed B, Rashidi P, Modave F, Tighe P. Accessing artificial intelligence for clinical decision-making. Front Digit Health. Jun 25, 2021;3:645232. [ CrossRef ] [ Medline ]
- Vayena E, Blasimme A, Cohen IG. Machine learning in medicine: addressing ethical challenges. PLoS Med. Nov 6, 2018;15(11):e1002689. [ CrossRef ] [ Medline ]
- Dzobo K, Adotey S, Thomford NE, Dzobo W. Integrating artificial and human intelligence: a partnership for responsible innovation in biomedical engineering and medicine. OMICS. May 2020;24(5):247-263. [ CrossRef ] [ Medline ]
- Zawati M, Lang M. What's in the box?: uncertain accountability of machine learning applications in healthcare. Am J Bioeth. Nov 2020;20(11):37-40. [ CrossRef ] [ Medline ]
- Peters MD, Godfrey C, McInerney P, Khalil H, Larsen P, Marnie C, et al. Best practice guidance and reporting items for the development of scoping review protocols. JBI Evid Synth. Apr 01, 2022;20(4):953-968. [ CrossRef ] [ Medline ]
- Pollock D, Peters MD, Khalil H, McInerney P, Alexander L, Tricco AC, et al. Recommendations for the extraction, analysis, and presentation of results in scoping reviews. JBI Evid Synth. Mar 01, 2023;21(3):520-532. [ CrossRef ] [ Medline ]
- Farah L, Murris JM, Borget I, Guilloux A, Martelli NM, Katsahian SI. Assessment of performance, interpretability, and explainability in artificial intelligence–based health technologies: what healthcare stakeholders need to know. Mayo Clin Proc Digit Health. Jun 2023;1(2):120-138. [ CrossRef ]
- Lehne M, Sass J, Essenwanger A, Schepers J, Thun S. Why digital medicine depends on interoperability. NPJ Digit Med. Aug 20, 2019;2:79. [ CrossRef ] [ Medline ]
- Esmaeilzadeh P. Use of AI-based tools for healthcare purposes: a survey study from consumers' perspectives. BMC Med Inform Decis Mak. Jul 22, 2020;20(1):170. [ CrossRef ] [ Medline ]
- Zia A, Aziz M, Popa I, Khan SA, Hamedani AF, Asif AR. Artificial intelligence-based medical data mining. J Pers Med. Aug 24, 2022;12(9):1359. [ CrossRef ] [ Medline ]
- Ming J, He Y, Yang Y, Hu M, Zhao X, Liu J, et al. Health technology assessment of medical devices: current landscape, challenges, and a way forward. Cost Eff Resour Alloc. Oct 05, 2022;20(1):54. [ CrossRef ] [ Medline ]
- Filkins BL, Kim JY, Roberts B, Armstrong W, Miller MA, Hultner ML, et al. Privacy and security in the era of digital health: what should translational researchers know and do about it? Am J Transl Res. Mar 15, 2016;8(3):1560-1580. [ Medline ]
- Johnson KB, Wei WQ, Weeraratne D, Frisse ME, Misulis K, Rhee K, et al. Precision medicine, AI, and the future of personalized health care. Clin Transl Sci. Jan 2021;14(1):86-93. [ CrossRef ] [ Medline ]
- Sloane EJ, Silva RJ. Artificial intelligence in medical devices and clinical decision support systems. In: Clinical Engineering Handbook (Second Edition). Cambridge, MA. Academic Press; 2020.
- Steuten LM. Early stage health technology assessment for precision biomarkers in oral health and systems medicine. OMICS. Jan 2016;20(1):30-35. [ CrossRef ] [ Medline ]
- Javaid M, Haleem A, Pratap Singh R, Suman R, Rab S. Significance of machine learning in healthcare: features, pillars and applications. Int J Intell Networks. 2022;3:58-73. [ CrossRef ]
- Rowland SP, Fitzgerald JE, Holme T, Powell J, McGregor A. What is the clinical value of mHealth for patients? NPJ Digit Med. Jan 13, 2020;3:4. [ CrossRef ] [ Medline ]
- Voets MM, Veltman J, Slump CH, Siesling S, Koffijberg H. Systematic review of health economic evaluations focused on artificial intelligence in healthcare: the tortoise and the cheetah. Value Health. Mar 2022;25(3):340-349. [ CrossRef ] [ Medline ]
- Kirisits A, Redekop WK. The economic evaluation of medical devices: challenges ahead. Appl Health Econ Health Policy. Feb 2013;11(1):15-26. [ CrossRef ] [ Medline ]
- Park SH, Choi J, Byeon JS. Key principles of clinical validation, device approval, and insurance coverage decisions of artificial intelligence. Korean J Radiol. Mar 2021;22(3):442-453. [ CrossRef ] [ Medline ]
- Tsopra R, Fernandez X, Luchinat C, Alberghina L, Lehrach H, Vanoni M, et al. A framework for validating AI in precision medicine: considerations from the European ITFoC consortium. BMC Med Inform Decis Mak. Oct 02, 2021;21(1):274. [ CrossRef ] [ Medline ]
- Bolboacă SD. Medical diagnostic tests: a review of test anatomy, phases, and statistical treatment of data. Comput Math Methods Med. May 28, 2019;2019:1891569. [ CrossRef ] [ Medline ]
- Hogervorst MA, Pontén J, Vreman RA, Mantel-Teeuwisse AK, Goettsch WG. Real world data in health technology assessment of complex health technologies. Front Pharmacol. Feb 10, 2022;13:837302. [ CrossRef ] [ Medline ]
- Simon GE, Bindman AB, Dreyer NA, Platt R, Watanabe JH, Horberg M, et al. When can we trust real-world data to evaluate new medical treatments? Clin Pharmacol Ther. Jan 2022;111(1):24-29. [ CrossRef ] [ Medline ]
- Pongiglione B, Torbica A, Blommestein H, de Groot S, Ciani O, Walker S, et al. Do existing real-world data sources generate suitable evidence for the HTA of medical devices in Europe? Mapping and critical appraisal. Int J Technol Assess Health Care. Apr 26, 2021;37(1):e62. [ CrossRef ] [ Medline ]
- Pongiglione B, Torbica A. How real can we get in generating real world evidence? Exploring the opportunities of routinely collected administrative data for evaluation of medical devices. Health Econ. Sep 2022;31 Suppl 1(Suppl 1):25-43. [ CrossRef ] [ Medline ]
- Zemplényi A, Tachkov K, Balkanyi L, Németh B, Petykó ZI, Petrova G, et al. Recommendations to overcome barriers to the use of artificial intelligence-driven evidence in health technology assessment. Front Public Health. Apr 26, 2023;11:1088121. [ CrossRef ] [ Medline ]
- Daubner-Bendes R, Kovács S, Niewada M, Huic M, Drummond M, Ciani O, et al. Quo Vadis HTA for medical devices in central and eastern Europe? Recommendations to address methodological challenges. Front Public Health. Jan 08, 2021;8:612410. [ CrossRef ] [ Medline ]
- Larson DB, Harvey H, Rubin DL, Irani N, Tse JR, Langlotz CP. Regulatory frameworks for development and evaluation of artificial intelligence-based diagnostic imaging algorithms: summary and recommendations. J Am Coll Radiol. Mar 2021;18(3 Pt A):413-424. [ CrossRef ] [ Medline ]
- Choudhury A, Asan O. Role of artificial intelligence in patient safety outcomes: systematic literature review. JMIR Med Inform. Jul 24, 2020;8(7):e18599. [ CrossRef ] [ Medline ]
- Belenguer L. AI bias: exploring discriminatory algorithmic decision-making models and the application of possible machine-centric solutions adapted from the pharmaceutical industry. AI Ethics. 2022;2(4):771-787. [ CrossRef ] [ Medline ]
- Binder L, Ghadban M, Sit C, Barnard K. Health technology assessment process for oncology drugs: impact of CADTH changes on public payer reimbursement recommendations. Curr Oncol. Mar 01, 2022;29(3):1514-1526. [ CrossRef ] [ Medline ]
- Wolff J, Pauling J, Keck A, Baumbach J. The economic impact of artificial intelligence in health care: systematic review. J Med Internet Res. Feb 20, 2020;22(2):e16866. [ CrossRef ] [ Medline ]
- Abràmoff MD, Roehrenbeck C, Trujillo S, Goldstein J, Graves AS, Repka MX, et al. A reimbursement framework for artificial intelligence in healthcare. NPJ Digit Med. Jun 09, 2022;5(1):72. [ CrossRef ] [ Medline ]
- Bélisle-Pipon JC, Couture V, Roy MC, Ganache I, Goetghebeur M, Cohen IG. What makes artificial intelligence exceptional in health technology assessment? Front Artif Intell. Nov 02, 2021;4:736697. [ CrossRef ] [ Medline ]
- Alami H, Lehoux P, Auclair Y, de Guise M, Gagnon MP, Shaw J, et al. Artificial intelligence and health technology assessment: anticipating a new level of complexity. J Med Internet Res. Jul 07, 2020;22(7):e17707. [ CrossRef ] [ Medline ]
- Love-Koh J, Peel A, Rejon-Parrilla JC, Ennis K, Lovett R, Manca A, et al. The future of precision medicine: potential impacts for health technology assessment. Pharmacoeconomics. Dec 2018;36(12):1439-1451. [ CrossRef ] [ Medline ]
- Gomez Rossi J, Rojas-Perilla N, Krois J, Schwendicke F. Cost-effectiveness of artificial intelligence as a decision-support system applied to the detection and grading of melanoma, dental caries, and diabetic retinopathy. JAMA Netw Open. Mar 01, 2022;5(3):e220269. [ CrossRef ] [ Medline ]
- Naik N, Hameed BM, Shetty DK, Swain D, Shah M, Paul R, et al. Legal and ethical consideration in artificial intelligence in healthcare: who takes responsibility? Front Surg. Mar 14, 2022;9:862322. [ CrossRef ] [ Medline ]
- Haynes CL, Cook GA, Jones MA. Legal and ethical considerations in processing patient-identifiable data without patient consent: lessons learnt from developing a disease register. J Med Ethics. May 2007;33(5):302-307. [ CrossRef ] [ Medline ]
- McCradden MD, Joshi S, Mazwi M, Anderson JA. Ethical limitations of algorithmic fairness solutions in health care machine learning. Lancet Digit Health. May 2020;2(5):e221-e223. [ CrossRef ] [ Medline ]
- Gianfrancesco MA, Tamang S, Yazdany J, Schmajuk G. Potential biases in machine learning algorithms using electronic health record data. JAMA Intern Med. Nov 01, 2018;178(11):1544-1547. [ CrossRef ] [ Medline ]
- Fletcher RR, Nakeshimana A, Olubeko O. Addressing fairness, bias, and appropriate use of artificial intelligence and machine learning in global health. Front Artif Intell. Apr 15, 2021;3:561802. [ CrossRef ] [ Medline ]
- Tachkov K, Zemplenyi A, Kamusheva M, Dimitrova M, Siirtola P, Pontén J, et al. Barriers to use artificial intelligence methodologies in health technology assessment in central and east European countries. Front Public Health. Jul 14, 2022;10:921226. [ CrossRef ] [ Medline ]
- Durán JM, Jongsma KR. Who is afraid of black box algorithms? On the epistemological and ethical basis of trust in medical AI. J Med Ethics. Mar 18, 2021. (forthcoming). [ CrossRef ] [ Medline ]
- Amann J, Blasimme A, Vayena E, Frey D, Madai VI, Precise4Q consortium. Explainability for artificial intelligence in healthcare: a multidisciplinary perspective. BMC Med Inform Decis Mak. Nov 30, 2020;20(1):310. [ CrossRef ] [ Medline ]
- Kiseleva A, Kotzinos D, De Hert P. Transparency of AI in healthcare as a multilayered system of accountabilities: between legal requirements and technical limitations. Front Artif Intell. May 30, 2022;5:879603. [ CrossRef ] [ Medline ]
- Baltaxe E, Hsieh HW, Roca J, Cano I. The assessment of medical device software supporting health care services for chronic patients in a tertiary hospital: overarching study. J Med Internet Res. Jan 04, 2023;25:e40976. [ CrossRef ] [ Medline ]
- Garfield S, Polisena J, S Spinner D, Postulka A, Y Lu C, Tiwana SK, et al. Health technology assessment for molecular diagnostics: practices, challenges, and recommendations from the medical devices and diagnostics special interest group. Value Health. 2016;19(5):577-587. [ CrossRef ] [ Medline ]
- Fraser AG, Biasin E, Bijnens B, Bruining N, Caiani EG, Cobbaert K, et al. Artificial intelligence in medical device software and high-risk medical devices - a review of definitions, expert recommendations and regulatory initiatives. Expert Rev Med Devices. Jun 2023;20(6):467-491. [ CrossRef ] [ Medline ]
- Beckers R, Kwade Z, Zanca F. The EU medical device regulation: implications for artificial intelligence-based medical device software in medical physics. Phys Med. Mar 2021;83:1-8. [ CrossRef ] [ Medline ]
- Melvin T, Torre M. New medical device regulations: the regulator's view. EFORT Open Rev. Jun 03, 2019;4(6):351-356. [ CrossRef ] [ Medline ]
- Fleetcroft C, McCulloch P, Campbell B. IDEAL as a guide to designing clinical device studies consistent with the new European Medical Device Regulation. BMJ Surg Interv Health Technol. Mar 04, 2021;3(1):e000066. [ CrossRef ] [ Medline ]
- Alami H, Lehoux P, Denis JL, Motulsky A, Petitgand C, Savoldelli M, et al. Organizational readiness for artificial intelligence in health care: insights for decision-making and practice. J Health Organ Manag. Dec 03, 2020. (forthcoming). [ CrossRef ] [ Medline ]
- Segur-Ferrer J, Moltó-Puigmartí C, Pastells-Peiró R, Vivanco-Hidalgo RM. Methodological frameworks and dimensions to be taken into consideration in digital health technology assessment: protocol for a scoping review. JMIR Res Protoc. Oct 11, 2022;11(10):e39905. [ CrossRef ] [ Medline ]
- Weinert L, Müller J, Svensson L, Heinze O. Perspective of information technology decision makers on factors influencing adoption and implementation of artificial intelligence technologies in 40 German hospitals: descriptive analysis. JMIR Med Inform. Jun 15, 2022;10(6):e34678. [ CrossRef ] [ Medline ]
- de Hond AA, Leeuwenberg AM, Hooft L, Kant IM, Nijman SW, van Os HJ, et al. Guidelines and quality criteria for artificial intelligence-based prediction models in healthcare: a scoping review. NPJ Digit Med. Jan 10, 2022;5(1):2. [ CrossRef ] [ Medline ]
- Ahuja AS. The impact of artificial intelligence in medicine on the future role of the physician. PeerJ. 2019;7:e7702. [ CrossRef ] [ Medline ]
- Widrig D, Tag B. HTA and its legal issues: a framework for identifying legal issues in health technology assessment. Int J Technol Assess Health Care. Dec 2014;30(6):587-594. [ CrossRef ] [ Medline ]
- Vella Bonanno P, Bucsics A, Simoens S, Martin AP, Oortwijn W, Gulbinovič J, et al. Proposal for a regulation on health technology assessment in Europe - opinions of policy makers, payers and academics from the field of HTA. Expert Rev Pharmacoecon Outcomes Res. Jun 2019;19(3):251-261. [ CrossRef ] [ Medline ]
- McKee M, Wouters OJ. The challenges of regulating artificial intelligence in healthcare comment on "clinical decision support and new regulatory frameworks for medical devices: are we ready for it? - a viewpoint paper". Int J Health Policy Manag. 2023;12:7261. [ CrossRef ] [ Medline ]
- Hordern V. Data protection compliance in the age of digital health. Eur J Health Law. Jun 2016;23(3):248-264. [ CrossRef ] [ Medline ]
- Stanberry B. The legal and ethical aspects of telemedicine. 2: data protection, security and European law. J Telemed Telecare. 1998;4(1):18-24. [ CrossRef ] [ Medline ]
- Poullet Y. Legal aspects of data protection in medical informatics. Stud Health Technol Inform. 1991;1:138-160. [ Medline ]
- Dove ES, Chen J. To what extent does the EU general data protection regulation (GDPR) apply to citizen scientist-led health research with mobile devices? J Law Med Ethics. Mar 2020;48(1_suppl):187-195. [ CrossRef ] [ Medline ]
- Marovic B, Curcin V. Impact of the European general data protection regulation (GDPR) on health data management in a European Union candidate country: a case study of Serbia. JMIR Med Inform. Apr 17, 2020;8(4):e14604. [ CrossRef ] [ Medline ]
- EU Artificial Intelligence Act. URL: https://artificialintelligenceact.eu/ [accessed 2023-07-24]
- Proposal for a regulation of the European parliament and of the council laying down harmonised rules on artificial intelligence (Artificial Intelligence Act) and amending certain union legislative acts. European Union. 2021. URL: https://eur-lex.europa.eu/legal-content/EN/TXT/?uri=CELEX:52021PC0206 [accessed 2023-07-24]
- Camara C, Peris-Lopez P, Tapiador JE. Security and privacy issues in implantable medical devices: a comprehensive survey. J Biomed Inform. Jun 2015;55:272-289. [ CrossRef ] [ Medline ]
- Migliore A, Ratti M, Cerbo M, Jefferson T. Health Technology Assessment: managing the introduction and use of medical devices in clinical practice in Italy. Expert Rev Med Devices. May 2009;6(3):251-257. [ CrossRef ] [ Medline ]
- Pisapia A, Banfi G, Tomaiuolo R. The novelties of the regulation on health technology assessment, a key achievement for the European Union health policies. Clin Chem Lab Med. Jul 26, 2022;60(8):1160-1163. [ CrossRef ] [ Medline ]
- Maliha G, Gerke S, Cohen IG, Parikh RB. Artificial intelligence and liability in medicine: balancing safety and innovation. Milbank Q. Sep 2021;99(3):629-647. [ CrossRef ] [ Medline ]
- Jassar S, Adams SJ, Zarzeczny A, Burbridge BE. The future of artificial intelligence in medicine: medical-legal considerations for health leaders. Healthc Manage Forum. May 2022;35(3):185-189. [ CrossRef ] [ Medline ]
- Samore MH, Evans RS, Lassen A, Gould P, Lloyd J, Gardner RM, et al. Surveillance of medical device-related hazards and adverse events in hospitalized patients. JAMA. Jan 21, 2004;291(3):325-334. [ CrossRef ] [ Medline ]
- Bleher H, Braun M. Diffused responsibility: attributions of responsibility in the use of AI-driven clinical decision support systems. AI Ethics. 2022;2(4):747-761. [ CrossRef ] [ Medline ]
- Street J, Stafinski T, Lopes E, Menon D. Defining the role of the public in Health Technology Assessment (HTA) and HTA-informed decision-making processes. Int J Technol Assess Health Care. Apr 2020;36(2):87-95. [ CrossRef ] [ Medline ]
- Massella M, Dri DA, Gramaglia D. Regulatory considerations on the use of machine learning based tools in clinical trials. Health Technol (Berl). 2022;12(6):1085-1096. [ CrossRef ] [ Medline ]
- Marcus HJ, Payne CJ, Hughes-Hallett A, Marcus AP, Yang GZ, Darzi A, et al. Regulatory approval of new medical devices: cross sectional study. BMJ. May 20, 2016;353:i2587. [ CrossRef ] [ Medline ]
- Milam ME, Koo CW. The current status and future of FDA-approved artificial intelligence tools in chest radiology in the United States. Clin Radiol. Feb 2023;78(2):115-122. [ CrossRef ] [ Medline ]
- Vreman RA, Mantel-Teeuwisse AK, Hövels AM, Leufkens HG, Goettsch WG. Differences in health technology assessment recommendations among European jurisdictions: the role of practice variations. Value Health. Jan 2020;23(1):10-16. [ CrossRef ] [ Medline ]
- Allen N, Walker SR, Liberti L, Salek S. Health technology assessment (HTA) case studies: factors influencing divergent HTA reimbursement recommendations in Australia, Canada, England, and Scotland. Value Health. Mar 2017;20(3):320-328. [ CrossRef ] [ Medline ]
- Agboola F, Wright AC, Herron-Smith S, Mathur D, Rind D. Evaluation of diversity of clinical trials informing health technology assessments in the United States: a 5-year analysis of institute for clinical and economic review assessments. Value Health. Sep 2023;26(9):1345-1352. [ CrossRef ] [ Medline ]
- Hendrix N, Veenstra DL, Cheng M, Anderson NC, Verguet S. Assessing the economic value of clinical artificial intelligence: challenges and opportunities. Value Health. Mar 2022;25(3):331-339. [ CrossRef ] [ Medline ]
- Nielsen CP, Lauritsen SW, Kristensen FB, Bistrup ML, Cecchetti A, Turk E. Involving stakeholders and developing a policy for stakeholder involvement in the European network for health technology assessment, EUnetHTA. Int J Technol Assess Health Care. Dec 2009;25 Suppl 2:84-91. [ CrossRef ] [ Medline ]
- Scott AM, Wale JL, HTAi PatientCitizen Involvement in HTA Interest Group‚ Patient InvolvementEducation Working Group. Patient advocate perspectives on involvement in HTA: an international snapshot. Res Involv Engagem. Jan 10, 2017;3:2. [ CrossRef ] [ Medline ]
- Gagnon MP, Desmartis M, Gagnon J, St-Pierre M, Gauvin FP, Rhainds M, et al. Introducing the patient's perspective in hospital health technology assessment (HTA): the views of HTA producers, hospital managers and patients. Health Expect. Dec 2014;17(6):888-900. [ CrossRef ] [ Medline ]
- Gagnon M, Desmartis M, Gagnon J, St-Pierre M, Rhainds M, Coulombe M, et al. Framework for user involvement in health technology assessment at the local level: views of health managers, user representatives, and clinicians. Int J Technol Assess Health Care. Jan 2015;31(1-2):68-77. [ CrossRef ] [ Medline ]
- Angelis A, Kanavos P. Value-based assessment of new medical technologies: towards a robust methodological framework for the application of multiple criteria decision analysis in the context of health technology assessment. Pharmacoeconomics. May 2016;34(5):435-446. [ CrossRef ] [ Medline ]
- Wale JL, Thomas S, Hamerlijnck D, Hollander R. Patients and public are important stakeholders in health technology assessment but the level of involvement is low - a call to action. Res Involv Engagem. Jan 05, 2021;7(1):1. [ CrossRef ] [ Medline ]
- Artificial intelligence and machine learning (AI/ML)-enabled medical devices. U.S. Food & Drug Administration. Oct 19, 2023. URL: https://tinyurl.com/m8ba5aw4 [accessed 2023-11-25]
- Cassidy B, Hoon Yap M, Pappachan JM, Ahmad N, Haycocks S, O'Shea C, et al. Artificial intelligence for automated detection of diabetic foot ulcers: a real-world proof-of-concept clinical evaluation. Diabetes Res Clin Pract. Nov 2023;205:110951. [ CrossRef ] [ Medline ]
- Mackenzie SC, Sainsbury CA, Wake DJ. Diabetes and artificial intelligence beyond the closed loop: a review of the landscape, promise and challenges. Diabetologia. Feb 2024;67(2):223-235. [ CrossRef ] [ Medline ]
- Sridhar GR, Lakshmi G. Ethical issues of artificial intelligence in diabetes mellitus. Med Res Arch. Aug 2023;11(8):1-8. [ CrossRef ]
- Zhang L, Zhang L. Artificial intelligence for remote sensing data analysis: a review of challenges and opportunities. IEEE Geosci Remote Sens Mag. Apr 13, 2022;10(2):270-294. [ FREE Full text ] [ CrossRef ]
- Challen R, Denny J, Pitt M, Gompels L, Edwards T, Tsaneva-Atanasova K. Artificial intelligence, bias and clinical safety. BMJ Qual Saf. Mar 2019;28(3):231-237. [ CrossRef ] [ Medline ]
- Beil M, Proft I, van Heerden D, Sviri S, van Heerden PV. Ethical considerations about artificial intelligence for prognostication in intensive care. Intensive Care Med Exp. Dec 10, 2019;7(1):70. [ CrossRef ] [ Medline ]
- Bærøe K, Miyata-Sturm A, Henden E. How to achieve trustworthy artificial intelligence for health. Bull World Health Organ. Apr 01, 2020;98(4):257-262. [ CrossRef ] [ Medline ]
- Julian E, Gianfrate F, Sola-Morales O, Mol P, Bergmann JF, Salmonson T, et al. How can a joint European health technology assessment provide an 'additional benefit' over the current standard of national assessments? : insights generated from a multi-stakeholder survey in hematology/oncology. Health Econ Rev. Jun 02, 2022;12(1):30. [ CrossRef ] [ Medline ]
Abbreviations
Edited by Y Zhuang; submitted 02.08.23; peer-reviewed by A de Hond, E Vashishtha, I Cano, N Hendrix; comments to author 28.10.23; revised version received 17.12.23; accepted 28.12.23; published 13.05.24.
©Line Farah, Isabelle Borget, Nicolas Martelli, Alexandre Vallee. Originally published in the Journal of Medical Internet Research (https://www.jmir.org), 13.05.2024.
This is an open-access article distributed under the terms of the Creative Commons Attribution License (https://creativecommons.org/licenses/by/4.0/), which permits unrestricted use, distribution, and reproduction in any medium, provided the original work, first published in the Journal of Medical Internet Research, is properly cited. The complete bibliographic information, a link to the original publication on https://www.jmir.org/, as well as this copyright and license information must be included.
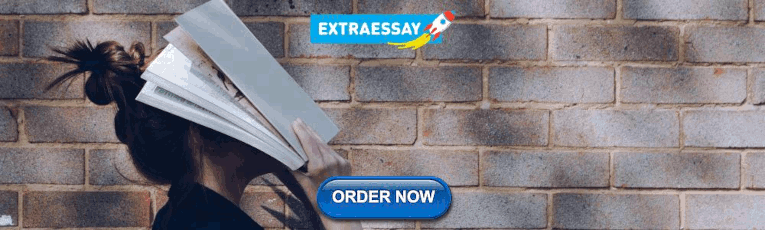
COMMENTS
This review aimed to identify, from the scholarly and grey literature, interventions used to address older worker health, safety and well-being and examine the evidence of their effectiveness (Bentley et al. Citation 2022). This paper provides a summary of those results, reflecting the current knowledge and research in this area.
The ILO estimates that poor occupational safety and health practices account for about 4% of global gross domestic product. According to Jilcha & Kitaw (2016), every 15 seconds of workers ...
Occupational health and safety (OHS) is a multidisciplinary activity that aims to identify, evaluate, and control hazards arising in or from the workplace that may impair the health and well-being of workers. ... This literature review study demonstrates that the OHSRA is gaining a higher recognition and a number of OHSRA models have been ...
First, the authors provide a formal definition of workplace safety and then create an integrated safety model (ISM) based on existing theory to summarize current theoretical expectations with regard to workplace safety. Second, the authors conduct a targeted review of the safety literature and compare extant empirical findings with the ISM.
This systematic literature review applied the matrix method to examine major literature in safety management practices and occupational health and safety performance.
Occupational health and safety (OHS) is a multidisciplinary activity that aims to identify, evaluate, and control hazards arising in or from the workplace that may impair the health and well-being of workers. ... This literature review study demonstrates that the OHSRA is gaining a higher recognition and a number of OHSRA models have been ...
The review concluded that occupational health and safety training positively affected worker behaviours, but there was insufficient evidence of the effect on health, attitudes and beliefs, and knowledge. The review was generally well conducted, and the authors' conclusions appropriately acknowledge the limitations in the evidence base and seem reliable.
Objectives The aim of this review was to assess the evidence that occupational safety and health (OSH) legislative and regulatory policy could improve the working environment in terms of reduced ...
They go on to review the literature of empowerment-oriented health and safety training. Jean Manoli, who provides training and compliance assistance as staff of the Massachusetts Department of Labor Standards, believes that OSHA 10-hour training is "becoming 'industry standard' and the 'way of doing business' in both construction and ...
INTRODUCTION. Efforts to protect and promote the safety, health, and wellbeing of workers have increasingly focused on integrating the complex and dynamic systems of the work organization and work environment. 1-3 The National Institute for Occupational Safety and Health (NIOSH) applies this integrated approach in the Total Worker Health ® (TWH) initiative by attending to "policies ...
The results of this literature review are based on the results of bibliometric data analysis through the context of previous research which shows that there are 6 definitions of occupational safety and health, 3 antecedents, 6 consequences, and 12 sectors of conflict management. The purpose of this literature review is to find and examine various definitions of conflict management based on the ...
Objectives The aim of this review was to assess the evidence that occupational safety and health (OSH) legislative and regulatory policy could improve the working environment in terms of reduced levels of industrial injuries and fatalities, musculoskeletal disorders, worker complaints, sick leave and adverse occupational exposures.
Writing literature reviews : a guide for students of the social and behavioral sciences by Jose L. Galvan; Melisa C. Galvan. ISBN: 9781138294219. Publication Date: 7th ed. 2017. Writing the Literature Review : a Practical Guide by by Sara Efrat Efron, Ruth Ravid. ISBN: 9781462536917.
Objective: The ability of occupational health and safety (OHS) legislation and regulatory enforcement to prevent workplace injuries and illnesses is contingent on political, economic, and organizational conditions. This systematic review of qualitative research articles considers how OHS legislation and regulatory enforcement are planned and implemented.
Introduction: In spite of increasing governmental and organizational efforts, organizations still struggle to improve the safety of their employees as evidenced by the yearly 2.3 million work-related deaths worldwide. Occupational safety research is scattered and inaccessible, especially for practitioners. Through systematically reviewing the safety literature, this study aims to provide a ...
5. Conclusions In conclusion, the authors have conducted a literature review on occupational safety and health state of the art in the globe considering previous and recent studies. The research focused on the global condition of safety in manufacturing industries both in developing and developed countries.
Introduction: To better understand what is known about issues affecting American Indian and Alaska Native (AI/AN) workers, authors conducted a literature review of publications specific to AI/AN and occupational safety and health.Methods: Search criteria included: (a) American Indian tribes and Alaska Native villages in the United States; (b) First Nations and aboriginals in Canada; and (c ...
Previous literature [41,42,43] supports the link between the categories of patient safety processes identified within this review (including reduced staff confidence, disengagement with the job, and defensive practice) and patient care and or/patient safety, suggesting these processes may serve as mechanisms to influence patient safety.
DOI: 10.1016/j.jsr.2024.04.009 Corpus ID: 269666440; The impact of Industry 4.0 on occupational health and safety: A systematic literature review @article{Bispo2024TheIO, title={The impact of Industry 4.0 on occupational health and safety: A systematic literature review}, author={Lucas Gomes Miranda Bispo and Fernando Gonçalves Amaral}, journal={Journal of Safety Research}, year={2024}, url ...
Assessing Occupational Safety and Health Training - A Literature Review. Print. June 1998. DHHS (NIOSH) Publication Number 98-145. More than 100 Occupational Safety and Health Administration (OSHA) standards for hazard control in the workplace contain requirements for training aimed at reducing risk factors for injury or disease; others limit ...
Its safety to workers who handle large amounts of CCP has been addressed in numerous studies and reports. This review encompasses the world's literature on CCP and provides a weight-of-evidence analysis of the safety of CCP to workers in the United States. Since 1987, has produced neither primary skin irritation nor skin sensitization under ...
Influence of communication determinants on safety commitment in a high-risk workplace: a systematic literature review of four communication dimensions. Health, safety, and environment (HSE) are ...
Mycotoxins contamination of cereals and other crops has only recently been recognized as an important food safety and public health issue (Bryła et al., 2016; Deligeorgakis et ... since the literature review identified less than three comparative datasets for emmer wheat, triticale, buckwheat, sorgum, millets, fonia, and quinoa, only data for ...
3.1 Literature search. After a complete search of the literature, 405 publications resulted, of these 261 publications were eligible for the title and abstract screening after removing duplicates. Of the 261, 247 were irrelevant and 14 studies were eligible for full-text screening.
The review protocol was not registered, in accordance with the guidelines. Search methods. The PubMed, Scopus, Web of Science, and Cumulative Index to Nursing and Allied Health Literature databases were searched with the keywords Parkinson's disease AND nurse AND (community OR home).
We provide an update with special consideration given to housing practices, safety, and access to care. A review of the literature shows that the World Professional Association for Transgender Health (WPATH) standards and Prison Rape Elimination Act (PREA) requirements are not uniformly implemented and enforced on a state level.
Objective: This study aims to analyze the suitability of each health technology assessment (HTA) domain for the assessment of AI-based medical devices. Methods: We conducted a scoping literature review following the PRISMA (Preferred Reporting Items for Systematic Reviews and Meta-Analyses) methodology.
Health literacy refers to the skills and abilities to access and utilize health information to make appropriate decisions about health-related issues (World Health Organization [WHO], 1998).Health literacy is a significant predictor of health knowledge (Baker, 2006; Quinlan et al., 2013; Speros, 2005).Based on a more comprehensive understanding of health literacy, health knowledge can also be ...
Among the various wearable devices, smart glasses have addressed the greatest scientific and development interest in recent years in the wake of the spread of Augmented reality (AR) and Virtual reality (VR), as Wearable devices for health and safety in production systems: a literature review Valentina Di Pasquale*, Valentina De Simone*, Martina ...