- How it works
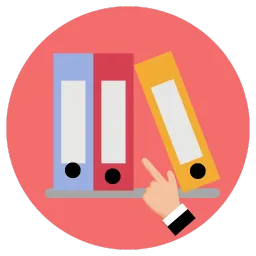
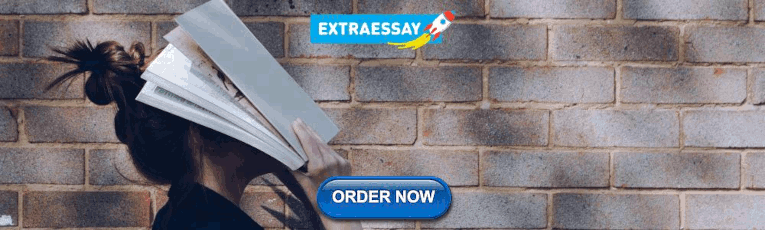
How to Write a Dissertation Discussion Chapter – A Quick Guide with Examples
Published by Alvin Nicolas at August 12th, 2021 , Revised On September 20, 2023
Dissertation discussion is the chapter where you explore the relevance, significance, and meanings of your findings – allowing you to showcase your talents in describing and analyzing the results of your study.
Here, you will be expected to demonstrate how your research findings answer the research questions established or test the hypothesis .
The arguments you assert in the dissertation analysis and discussions chapter lay the foundations of your conclusion . It is critically important to discuss the results in a precise manner.
To help you understand how to write a dissertation discussion chapter, here is the list of the main elements of this section so you stay on the right track when writing:
- Summary: Start by providing a summary of your key research findings
- Interpretations: What is the significance of your findings?
- Implications: Why are your findings important to academic and scientific communities, and what purpose would they serve?
- Limitations: When and where will your results have no implications?
- Future Recommendations : Advice for other researchers and scientists who explore the topic further in future.
The dissertation discussion chapter should be carefully drafted to ensure that the results mentioned in your research align with your research question, aims, and objectives.
Considering the importance of this chapter for all students working on their dissertations, we have comprehensive guidelines on how to write a dissertation discussion chapter.
The discussion and conclusion chapters often overlap. Depending on your university, you may be asked to group these two sections in one chapter – Discussion and Conclusion.
In some cases, the results and discussion are put together under the Results and Discussion chapter. Here are some dissertation examples of working out the best structure for your dissertation.
Alternatively, you can look for the required dissertation structure in your handbook or consult your supervisor.
Steps of How to Write Dissertation Discussion Chapter
1. provide a summary of your findings.
Start your discussion by summarising the key findings of your research questions. Avoid repeating the information you have already stated in the previous chapters.
You will be expected to clearly express your interpretation of results to answer the research questions established initially in one or two paragraphs.
Here are some examples of how to present the summary of your findings ;
- “The data suggests that”,
- “The results confirm that”,
- “The analysis indicates that”,
- “The research shows a relationship between”, etc.
2. Interpretations of Results
Your audience will expect you to provide meanings of the results, although they might seem obvious to you. The results and their interpretations should be linked to the research questions so the reader can understand the value your research has added to the literature.
There are many ways of interpreting the data, but your chosen approach to interpreting the data will depend on the type of research involved . Some of the most common strategies employed include;
- Describing how and why you ended up with unexpected findings and explaining their importance in detail
- Relating your findings with previous studies conducted
- Explaining your position with logical arguments when/if any alternative explanations are suggested
- An in-depth discussion around whether or not the findings answered your research questions and successfully tested the hypothesis
Examples of how you can start your interpretation in the Discussion chapter are –
- “Findings of this study contradict those of Allen et al. (2014) that”,
- “Contrary to the hypothesized association,” “Confirming the hypothesis…”,
- “The findings confirm that A is….. even though Allen et al. (2014) and Michael (2012) suggested B was …..”
3. Implications of your Study
What practical and theoretical implications will your study have for other researchers and the scientific community as a whole?
It is vital to relate your results to the knowledge in the existing literature so the readers can establish how your research will contribute to the existing data.
When thinking of the possible consequences of your findings, you should ask yourself these;
- Are your findings in line with previous studies? What contribution did your research make to them?
- Why are your results entirely different from other studies on the same topic?
- Did your findings approve or contradict existing knowledge?
- What are the practical implications of your study?
Remember that as the researcher, you should aim to let your readers know why your study will contribute to the existing literature. Possible ways of starting this particular section are;
- “The findings show that A….. whereas Lee (2017) and John (2013) suggested that B”, “The results of this study completely contradict the claims made in theories”,
- “These results are not in line with the theoretical perspectives”,
- “The statistical analysis provides a new understanding of the relationship between A and B”,
- “Future studies should take into consideration the findings of this study because”
“Improve your work’s language, structure, style, and overall quality. Get help from our experienced dissertation editors to improve the quality of your paper to First Class Standard. Click here to learn more about our Dissertation, Editing, and Improvement Services .
Looking for dissertation help?
Researchprospect to the rescue, then.
We have expert writers on our team who are skilled at helping students with dissertations across various disciplines. Guaranteeing 100% satisfaction!
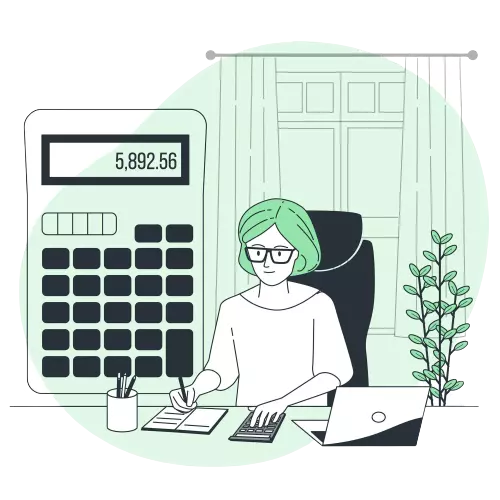
4. Recognise the Limitations of your Research
Almost every academic research has some limitations. Acknowledging them will only add to your credibility as a scientific researcher.
In addition to the possible human errors, it’s important to take into account other factors that might have influenced the results of your study, including but not limited to unexpected research obstacles, specific methodological choices , and the overall research design.
Avoid mentioning any limitations that may not be relevant to your research aim, but clearly state the limitations that may have affected your results.
For example, if you used a sample size that included a tiny population, you may not generalise your results.
Similarly, obstacles faced in collecting data from the participants can influence the findings of your study. Make a note of all such research limitations , but explain to the reader why your results are still authentic.
- The small sample size limited the generalisability of the results.
- The authenticity of the findings may have been influenced by….
- The obstacles in collecting data resulted in…
- It is beyond the framework of this research…
5. Provide Recommendations for Future Research
The limitations of your research work directly result in future recommendations. However, it should be noted that your recommendations for future research work should include the areas that your own work could not report so other researchers can build on them.
Sometimes the recommendations are a part of the conclusion chapter . Some examples;
- More research is needed to be performed….
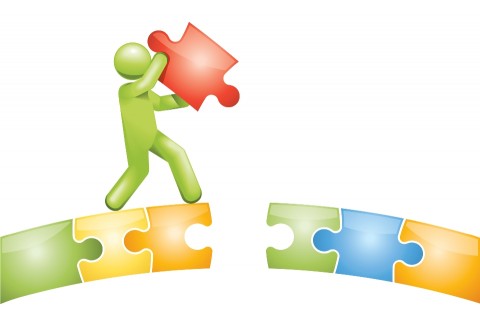
The Purpose of Dissertation Discussion Chapter
Remember that the discussion section of a dissertation is the heart of your research because a) it will indicate your stance on the topic of research, and b) it answers the research questions initially established in the Introduction chapter .
Every piece of information you present here will add value to the existing literature within your field of study. How you structured your findings in the preceding chapter will help you determine the best structure for your dissertation discussion section.
For example, it might be logical to structure your analysis/discussions by theme if you chose the pattern in your findings section.
But generally, discussion based on research questions is the more widely used structure in academia because this pattern clearly indicates how you have addressed the aim of your research.
Most UK universities require the supervisor or committee members to comment on the extent to which each research question has been answered. You will be doing them a great favour if you structure your discussion so that each research question is laid out separately.
Irrespective of whether you are writing an essay, dissertation, or chapter of a dissertation , all pieces of writing should start with an introduction .
Once your readers have read through your study results, you might want to highlight the contents of the subsequent discussion as an introduction paragraph (summary of your results – as explained above).
Likewise, the discussion chapter is expected to end with a concluding paragraph – allowing you the opportunity to summarise your interpretations.
The dissertation analysis & discussion chapter is usually very long, so it will make sense to emphasise the critical points in a concluding paragraph so the reader can grasp the essential information. This will also help to make sure the reader understands your analysis.
Also Read: Research Discussion Of Findings
Useful Tips
Presentation of graphs, tables, and figures.
In the 1990s and early 2000s, students spent days creating graphs and charts for their statistical analysis work . Thanks to technology, you can produce even more accurate graphs and figures today in a shorter period.
Using Microsoft Word, STATA, SPSS, Microsoft Excel and other statistical analysis software, we can now draw beautiful-looking figures, tables , and graphs with just a few clicks and make them appear in our document at the desired place. But there are downsides to being too dependent on technology.
Many students make the common mistake of using colours to represent variables when really they have to print their dissertation paper final copy in black and white.
Any colours on graphs and figures will eventually be viewed in the grayscale presentation. Recognizing different shades of grey on the same chart or graph can sometimes be a little confusing.
For example, green and purple appear as pretty much the same shade of grey on a line chat, meaning your chart will become unreadable to the marker.
Another trap you may fall into is the unintentional stuffing of the dissertation chapter with graphs and figures. Even though it is essential to show numbers and statistics, you don’t want to overwhelm your readers with too many.
It may not be necessary to have a graph/table under each sub-heading. Only you can best judge whether or not you need to have a graph/table under a particular sub-heading as the writer.
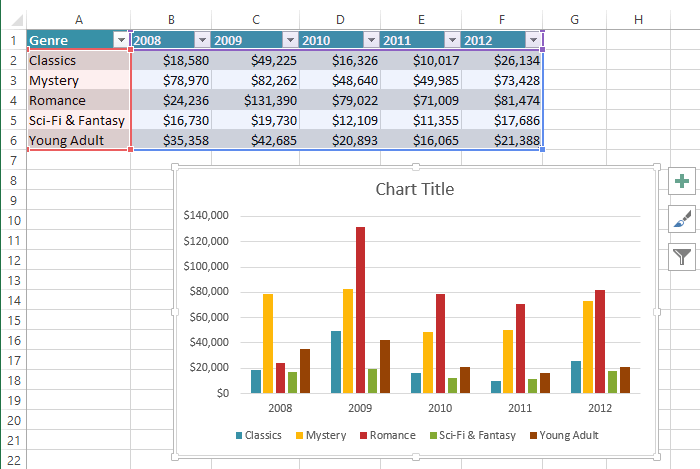
Relating to Previous Chapters
As a student, it can be challenging to develop your own analysis and discussion of results. One of the excellent discussion chapter requirements is to showcase your ability to relate previous research to your research results.
Avoid repeating the same information over and over. Many students fall into this trap which negatively affects the mark of their overall dissertation paper .
Concise and to-the-point information will help you effectively convey your point to the readers.
Although you must demonstrate how your findings relate to previous research, it is equally important to ensure you are not simply rewriting what has already been said in the introduction and literature review chapters.
The best strategy is to use examples from previous sections to postulate an argument.
Hyperlinks are recommended to take the reader from one section to another. This is especially important for submitting electronic documents as .word or .pdf files. Hyperlinking is tedious and time-consuming, so you should allow for this in your dissertation timeline to avoid rushing in the closing stages.
Also read: How to Write the Abstract for the Dissertation.
Using Subsections and Subheadings
You might want to reflect on the structure of the discussion in your organizstion of the dissertation discussion chapter, and for that, you will need to create sub-sections.
It is essential to keep subsections to the point and as short as possible. Use a layer of subheadings if possible.
For example
Subsection 4.1 of Chapter 4- Discussion can be further divided into sections 4.1.1 and 4.2.2. After three numerical layers (4.1.1, 4.2.2, and 4.2.3), any subheadings need not appear in the contents table.
The titles of all subsections will appear on your table of contents so choose the wordings carefully. A title too long or too short might confuse the reader. A one or two-word subheading will not give the reader enough information to understand the section.
Likewise, using a research question or long sentences in the subheading is not recommended. It might help to examine how other researchers and writers create these subheadings.
Critical Thinking
Your critical thinking skills are the crux of your dissertation discussion chapter. You will do yourself a great disservice if you fail to put the critical thinking element into the equation.
After all, this exercise aims to showcase clarity in your thoughts and arguments. Markers of the dissertation give more importance to the analysis and discussion chapter. But you could be marked negatively if this particular chapter lacks critical thinking.
Many students struggle to distinguish between fundamental descriptive analysis and critical thinking with their opinions on the research topic.
Critical thinking is a skill developed over time, and it might be daunting for you to come to terms with the idea of critical thinking and its use in your analysis. But even if you are no expert, you must try your best.

“Still unsure about how to write a dissertation discussion chapter ? Why not take advantage of our UK-based dissertation writing service ? Your dissertation is essential to your degree, so you cannot risk failing it.
With our custom writing service , you are guaranteed to have all your dissertation paper elements put into the right place. Our expert academics can help you with your full dissertation paper or a part of it. Click here to learn more about our dissertation services.
Duplication of Content
Another critical error students make reaffirming the point the graph/chart was supposed to make. Writing out the same information as presented in the graph defeats the whole purpose of having them in the first place.
You will be expected to form your opinions and arguments based on the findings (as presented by the graphs), so keep an eye on this mistake. Finally, avoid simply inserting a graph without any explanation whatsoever.
It should be noted that there is no correct or incorrect number of charts/figures one can use in the dissertation findings and discussion chapter. A balance must be struck.
Avoid Over Interpretation
This is a major no-no when writing a dissertation discussion. Do not make an argument that isn’t backed by your collected data.
The results and interpretations that cannot be supported should not be mentioned. Your research will be deemed unauthentic and will also be questioned by your supervisor if you do so. Results should be interpreted without any bias.
How to Write the Findings of a Dissertation.
Do not Speculate
Speculation in the discussion chapter of your dissertation is discouraged. Your dissertation’s discussion is based on your collected data and how it relates to your research questions. Thus, speculating here will undoubtedly undermine your research’s credibility.
Also, try not to generalise your findings. If your research is based on a specific population, do not state that the same findings might apply in every case. As indicated previously, it is essential to acknowledge the limitations of your research.
On the other hand, if you think your discussion needs to address other populations as well, start your sentence like this ‘We speculate that..’ or ‘It is speculated that..’ This will keep you from getting into any trouble.
What are the elements of the Dissertation Discussion?
The list of the main elements of the discussion chapter are:
- Implications : Why are your findings important to academic and scientific communities, and what purpose would they serve?
- Future Recommendations: Advice for other researchers and scientists who explore the topic further in future.
What are the steps of writing a Dissertation Discussion Chapter?
- Write a summary of the findings
- Provide a summary of your findings
- Interpretations of Results
- Recognise the Limitations of your research
- Provide Recommendations for Future Research.
Can we use graphs and charts in the Dissertation Discussion Chapter?
Yes, using graphs to aid your statistical results and enhance presentation is essential, but do not overwhelm it with a lot of graphs in multiple colours.
You May Also Like
Not sure how to write the findings of a dissertation. Here are some comprehensive guidelines for you to learn to write a flawless findings chapter.
This article is a step-by-step guide to how to write statement of a problem in research. The research problem will be half-solved by defining it correctly.
A list of glossary in a dissertation contains all the terms that were used in your dissertation but the meanings of which may not be obvious to the readers.
USEFUL LINKS
LEARNING RESOURCES
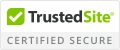
COMPANY DETAILS
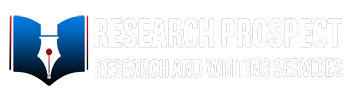
- How It Works
When you choose to publish with PLOS, your research makes an impact. Make your work accessible to all, without restrictions, and accelerate scientific discovery with options like preprints and published peer review that make your work more Open.
- PLOS Biology
- PLOS Climate
- PLOS Complex Systems
- PLOS Computational Biology
- PLOS Digital Health
- PLOS Genetics
- PLOS Global Public Health
- PLOS Medicine
- PLOS Mental Health
- PLOS Neglected Tropical Diseases
- PLOS Pathogens
- PLOS Sustainability and Transformation
- PLOS Collections
- How to Write Discussions and Conclusions

The discussion section contains the results and outcomes of a study. An effective discussion informs readers what can be learned from your experiment and provides context for the results.
What makes an effective discussion?
When you’re ready to write your discussion, you’ve already introduced the purpose of your study and provided an in-depth description of the methodology. The discussion informs readers about the larger implications of your study based on the results. Highlighting these implications while not overstating the findings can be challenging, especially when you’re submitting to a journal that selects articles based on novelty or potential impact. Regardless of what journal you are submitting to, the discussion section always serves the same purpose: concluding what your study results actually mean.
A successful discussion section puts your findings in context. It should include:
- the results of your research,
- a discussion of related research, and
- a comparison between your results and initial hypothesis.
Tip: Not all journals share the same naming conventions.
You can apply the advice in this article to the conclusion, results or discussion sections of your manuscript.
Our Early Career Researcher community tells us that the conclusion is often considered the most difficult aspect of a manuscript to write. To help, this guide provides questions to ask yourself, a basic structure to model your discussion off of and examples from published manuscripts.

Questions to ask yourself:
- Was my hypothesis correct?
- If my hypothesis is partially correct or entirely different, what can be learned from the results?
- How do the conclusions reshape or add onto the existing knowledge in the field? What does previous research say about the topic?
- Why are the results important or relevant to your audience? Do they add further evidence to a scientific consensus or disprove prior studies?
- How can future research build on these observations? What are the key experiments that must be done?
- What is the “take-home” message you want your reader to leave with?
How to structure a discussion
Trying to fit a complete discussion into a single paragraph can add unnecessary stress to the writing process. If possible, you’ll want to give yourself two or three paragraphs to give the reader a comprehensive understanding of your study as a whole. Here’s one way to structure an effective discussion:
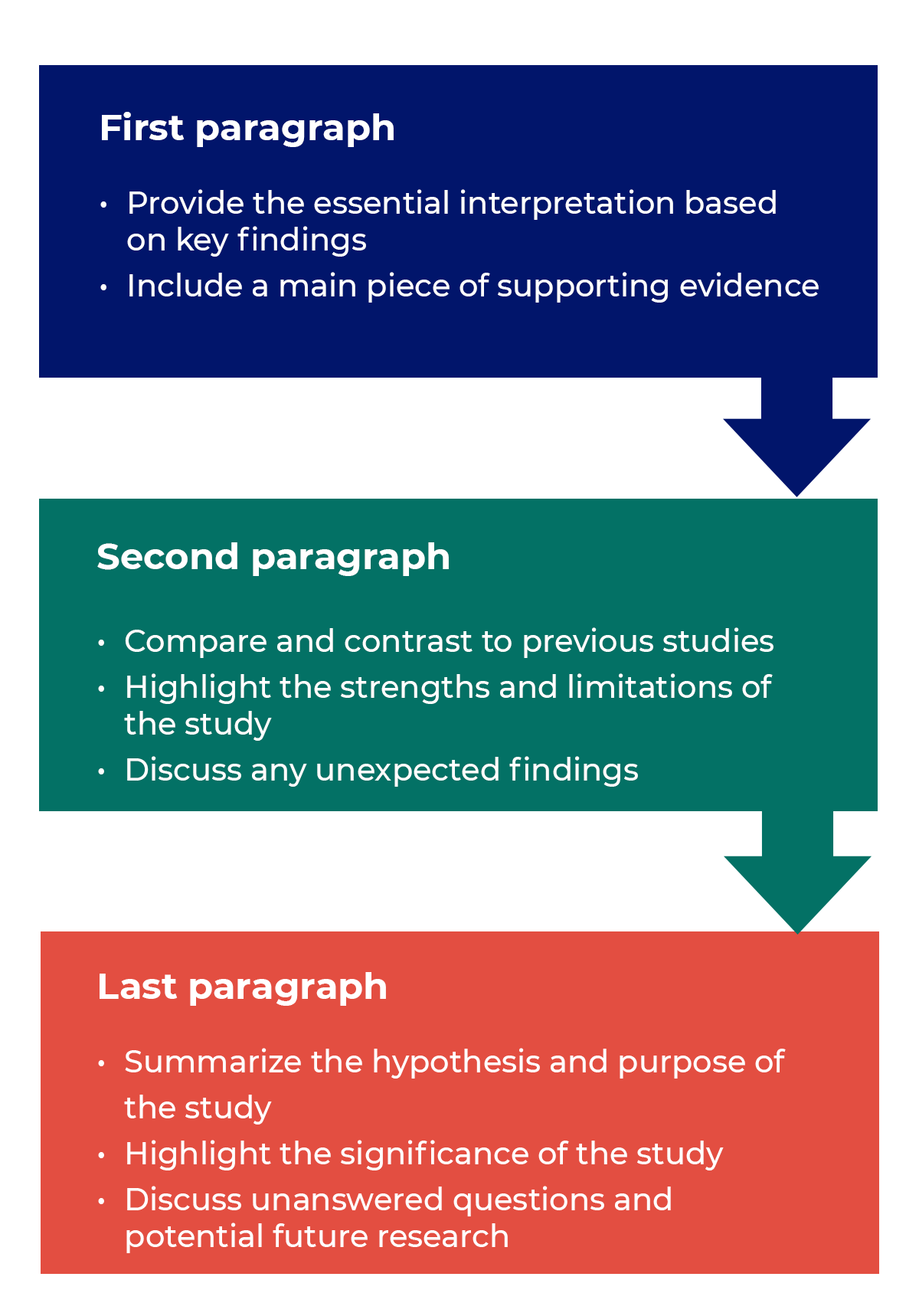
Writing Tips
While the above sections can help you brainstorm and structure your discussion, there are many common mistakes that writers revert to when having difficulties with their paper. Writing a discussion can be a delicate balance between summarizing your results, providing proper context for your research and avoiding introducing new information. Remember that your paper should be both confident and honest about the results!

- Read the journal’s guidelines on the discussion and conclusion sections. If possible, learn about the guidelines before writing the discussion to ensure you’re writing to meet their expectations.
- Begin with a clear statement of the principal findings. This will reinforce the main take-away for the reader and set up the rest of the discussion.
- Explain why the outcomes of your study are important to the reader. Discuss the implications of your findings realistically based on previous literature, highlighting both the strengths and limitations of the research.
- State whether the results prove or disprove your hypothesis. If your hypothesis was disproved, what might be the reasons?
- Introduce new or expanded ways to think about the research question. Indicate what next steps can be taken to further pursue any unresolved questions.
- If dealing with a contemporary or ongoing problem, such as climate change, discuss possible consequences if the problem is avoided.
- Be concise. Adding unnecessary detail can distract from the main findings.
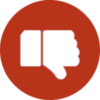
Don’t
- Rewrite your abstract. Statements with “we investigated” or “we studied” generally do not belong in the discussion.
- Include new arguments or evidence not previously discussed. Necessary information and evidence should be introduced in the main body of the paper.
- Apologize. Even if your research contains significant limitations, don’t undermine your authority by including statements that doubt your methodology or execution.
- Shy away from speaking on limitations or negative results. Including limitations and negative results will give readers a complete understanding of the presented research. Potential limitations include sources of potential bias, threats to internal or external validity, barriers to implementing an intervention and other issues inherent to the study design.
- Overstate the importance of your findings. Making grand statements about how a study will fully resolve large questions can lead readers to doubt the success of the research.
Snippets of Effective Discussions:
Consumer-based actions to reduce plastic pollution in rivers: A multi-criteria decision analysis approach
Identifying reliable indicators of fitness in polar bears
- How to Write a Great Title
- How to Write an Abstract
- How to Write Your Methods
- How to Report Statistics
- How to Edit Your Work
The contents of the Peer Review Center are also available as a live, interactive training session, complete with slides, talking points, and activities. …
The contents of the Writing Center are also available as a live, interactive training session, complete with slides, talking points, and activities. …
There’s a lot to consider when deciding where to submit your work. Learn how to choose a journal that will help your study reach its audience, while reflecting your values as a researcher…

- LEARNING SKILLS
- Writing a Dissertation or Thesis
- Results and Discussion
Search SkillsYouNeed:
Learning Skills:
- A - Z List of Learning Skills
- What is Learning?
- Learning Approaches
- Learning Styles
- 8 Types of Learning Styles
- Understanding Your Preferences to Aid Learning
- Lifelong Learning
- Decisions to Make Before Applying to University
- Top Tips for Surviving Student Life
- Living Online: Education and Learning
- 8 Ways to Embrace Technology-Based Learning Approaches
- Critical Thinking Skills
- Critical Thinking and Fake News
- Understanding and Addressing Conspiracy Theories
- Critical Analysis
- Study Skills
- Exam Skills
- How to Write a Research Proposal
- Ethical Issues in Research
- Dissertation: The Introduction
- Researching and Writing a Literature Review
- Writing your Methodology
- Dissertation: Results and Discussion
- Dissertation: Conclusions and Extras
Writing Your Dissertation or Thesis eBook

Part of the Skills You Need Guide for Students .
- Research Methods
- Teaching, Coaching, Mentoring and Counselling
- Employability Skills for Graduates
Subscribe to our FREE newsletter and start improving your life in just 5 minutes a day.
You'll get our 5 free 'One Minute Life Skills' and our weekly newsletter.
We'll never share your email address and you can unsubscribe at any time.
Writing your Dissertation: Results and Discussion
When writing a dissertation or thesis, the results and discussion sections can be both the most interesting as well as the most challenging sections to write.
You may choose to write these sections separately, or combine them into a single chapter, depending on your university’s guidelines and your own preferences.
There are advantages to both approaches.
Writing the results and discussion as separate sections allows you to focus first on what results you obtained and set out clearly what happened in your experiments and/or investigations without worrying about their implications.This can focus your mind on what the results actually show and help you to sort them in your head.
However, many people find it easier to combine the results with their implications as the two are closely connected.
Check your university’s requirements carefully before combining the results and discussions sections as some specify that they must be kept separate.
Results Section
The Results section should set out your key experimental results, including any statistical analysis and whether or not the results of these are significant.
You should cover any literature supporting your interpretation of significance. It does not have to include everything you did, particularly for a doctorate dissertation. However, for an undergraduate or master's thesis, you will probably find that you need to include most of your work.
You should write your results section in the past tense: you are describing what you have done in the past.
Every result included MUST have a method set out in the methods section. Check back to make sure that you have included all the relevant methods.
Conversely, every method should also have some results given so, if you choose to exclude certain experiments from the results, make sure that you remove mention of the method as well.
If you are unsure whether to include certain results, go back to your research questions and decide whether the results are relevant to them. It doesn’t matter whether they are supportive or not, it’s about relevance. If they are relevant, you should include them.
Having decided what to include, next decide what order to use. You could choose chronological, which should follow the methods, or in order from most to least important in the answering of your research questions, or by research question and/or hypothesis.
You also need to consider how best to present your results: tables, figures, graphs, or text. Try to use a variety of different methods of presentation, and consider your reader: 20 pages of dense tables are hard to understand, as are five pages of graphs, but a single table and well-chosen graph that illustrate your overall findings will make things much clearer.
Make sure that each table and figure has a number and a title. Number tables and figures in separate lists, but consecutively by the order in which you mention them in the text. If you have more than about two or three, it’s often helpful to provide lists of tables and figures alongside the table of contents at the start of your dissertation.
Summarise your results in the text, drawing on the figures and tables to illustrate your points.
The text and figures should be complementary, not repeat the same information. You should refer to every table or figure in the text. Any that you don’t feel the need to refer to can safely be moved to an appendix, or even removed.
Make sure that you including information about the size and direction of any changes, including percentage change if appropriate. Statistical tests should include details of p values or confidence intervals and limits.
While you don’t need to include all your primary evidence in this section, you should as a matter of good practice make it available in an appendix, to which you should refer at the relevant point.
For example:
Details of all the interview participants can be found in Appendix A, with transcripts of each interview in Appendix B.
You will, almost inevitably, find that you need to include some slight discussion of your results during this section. This discussion should evaluate the quality of the results and their reliability, but not stray too far into discussion of how far your results support your hypothesis and/or answer your research questions, as that is for the discussion section.
See our pages: Analysing Qualitative Data and Simple Statistical Analysis for more information on analysing your results.
Discussion Section
This section has four purposes, it should:
- Interpret and explain your results
- Answer your research question
- Justify your approach
- Critically evaluate your study
The discussion section therefore needs to review your findings in the context of the literature and the existing knowledge about the subject.
You also need to demonstrate that you understand the limitations of your research and the implications of your findings for policy and practice. This section should be written in the present tense.
The Discussion section needs to follow from your results and relate back to your literature review . Make sure that everything you discuss is covered in the results section.
Some universities require a separate section on recommendations for policy and practice and/or for future research, while others allow you to include this in your discussion, so check the guidelines carefully.
Starting the Task
Most people are likely to write this section best by preparing an outline, setting out the broad thrust of the argument, and how your results support it.
You may find techniques like mind mapping are helpful in making a first outline; check out our page: Creative Thinking for some ideas about how to think through your ideas. You should start by referring back to your research questions, discuss your results, then set them into the context of the literature, and then into broader theory.
This is likely to be one of the longest sections of your dissertation, and it’s a good idea to break it down into chunks with sub-headings to help your reader to navigate through the detail.
Fleshing Out the Detail
Once you have your outline in front of you, you can start to map out how your results fit into the outline.
This will help you to see whether your results are over-focused in one area, which is why writing up your research as you go along can be a helpful process. For each theme or area, you should discuss how the results help to answer your research question, and whether the results are consistent with your expectations and the literature.
The Importance of Understanding Differences
If your results are controversial and/or unexpected, you should set them fully in context and explain why you think that you obtained them.
Your explanations may include issues such as a non-representative sample for convenience purposes, a response rate skewed towards those with a particular experience, or your own involvement as a participant for sociological research.
You do not need to be apologetic about these, because you made a choice about them, which you should have justified in the methodology section. However, you do need to evaluate your own results against others’ findings, especially if they are different. A full understanding of the limitations of your research is part of a good discussion section.
At this stage, you may want to revisit your literature review, unless you submitted it as a separate submission earlier, and revise it to draw out those studies which have proven more relevant.
Conclude by summarising the implications of your findings in brief, and explain why they are important for researchers and in practice, and provide some suggestions for further work.
You may also wish to make some recommendations for practice. As before, this may be a separate section, or included in your discussion.
The results and discussion, including conclusion and recommendations, are probably the most substantial sections of your dissertation. Once completed, you can begin to relax slightly: you are on to the last stages of writing!
Continue to: Dissertation: Conclusion and Extras Writing your Methodology
See also: Writing a Literature Review Writing a Research Proposal Academic Referencing What Is the Importance of Using a Plagiarism Checker to Check Your Thesis?

Writing the Dissertation - Guides for Success: The Results and Discussion
- Writing the Dissertation Homepage
- Overview and Planning
- The Literature Review
- The Methodology
- The Results and Discussion
- The Conclusion
- The Abstract
- The Difference
- What to Avoid
Overview of writing the results and discussion
The results and discussion follow on from the methods or methodology chapter of the dissertation. This creates a natural transition from how you designed your study, to what your study reveals, highlighting your own contribution to the research area.
Disciplinary differences
Please note: this guide is not specific to any one discipline. The results and discussion can vary depending on the nature of the research and the expectations of the school or department, so please adapt the following advice to meet the demands of your project and department. Consult your supervisor for further guidance; you can also peruse our Writing Across Subjects guide .
Guide contents
As part of the Writing the Dissertation series, this guide covers the most common conventions of the results and discussion chapters, giving you the necessary knowledge, tips and guidance needed to impress your markers! The sections are organised as follows:
- The Difference - Breaks down the distinctions between the results and discussion chapters.
- Results - Provides a walk-through of common characteristics of the results chapter.
- Discussion - Provides a walk-through of how to approach writing your discussion chapter, including structure.
- What to Avoid - Covers a few frequent mistakes you'll want to...avoid!
- FAQs - Guidance on first- vs. third-person, limitations and more.
- Checklist - Includes a summary of key points and a self-evaluation checklist.
Training and tools
- The Academic Skills team has recorded a Writing the Dissertation workshop series to help you with each section of a standard dissertation, including a video on writing the results and discussion (embedded below).
- The dissertation planner tool can help you think through the timeline for planning, research, drafting and editing.
- iSolutions offers training and a Word template to help you digitally format and structure your dissertation.
Introduction
The results of your study are often followed by a separate chapter of discussion. This is certainly the case with scientific writing. Some dissertations, however, might incorporate both the results and discussion in one chapter. This depends on the nature of your dissertation and the conventions within your school or department. Always follow the guidelines given to you and ask your supervisor for further guidance.
As part of the Writing the Dissertation series, this guide covers the essentials of writing your results and discussion, giving you the necesary knowledge, tips and guidance needed to leave a positive impression on your markers! This guide covers the results and discussion as separate – although interrelated – chapters, as you'll see in the next two tabs. However, you can easily adapt the guidance to suit one single chapter – keep an eye out for some hints on how to do this throughout the guide.
Results or discussion - what's the difference?
To understand what the results and discussion sections are about, we need to clearly define the difference between the two.
The results should provide a clear account of the findings . This is written in a dry and direct manner, simply highlighting the findings as they appear once processed. It’s expected to have tables and graphics, where relevant, to contextualise and illustrate the data.
Rather than simply stating the findings of the study, the discussion interprets the findings to offer a more nuanced understanding of the research. The discussion is similar to the second half of the conclusion because it’s where you consider and formulate a response to the question, ‘what do we now know that we didn’t before?’ (see our Writing the Conclusion guide for more). The discussion achieves this by answering the research questions and responding to any hypotheses proposed. With this in mind, the discussion should be the most insightful chapter or section of your dissertation because it provides the most original insight.
Across the next two tabs of this guide, we will look at the results and discussion chapters separately in more detail.
Writing the results
The results chapter should provide a direct and factual account of the data collected without any interpretation or interrogation of the findings. As this might suggest, the results chapter can be slightly monotonous, particularly for quantitative data. Nevertheless, it’s crucial that you present your results in a clear and direct manner as it provides the necessary detail for your subsequent discussion.
Note: If you’re writing your results and discussion as one chapter, then you can either:
1) write them as distinctly separate sections in the same chapter, with the discussion following on from the results, or...
2) integrate the two throughout by presenting a subset of the results and then discussing that subset in further detail.
Next, we'll explore some of the most important factors to consider when writing your results chapter.
How you structure your results chapter depends on the design and purpose of your study. Here are some possible options for structuring your results chapter (adapted from Glatthorn and Joyner, 2005):
- Chronological – depending on the nature of the study, it might be important to present your results in order of how you collected the data, such as a pretest-posttest design.
- Research method – if you’ve used a mixed-methods approach, you could isolate each research method and instrument employed in the study.
- Research question and/or hypotheses – you could structure your results around your research questions and/or hypotheses, providing you have more than one. However, keep in mind that the results on their own don’t necessarily answer the questions or respond to the hypotheses in a definitive manner. You need to interpret the findings in the discussion chapter to gain a more rounded understanding.
- Variable – you could isolate each variable in your study (where relevant) and specify how and whether the results changed.
Tables and figures
For your results, you are expected to convert your data into tables and figures, particularly when dealing with quantitative data. Making use of tables and figures is a way of contextualising your results within the study. It also helps to visually reinforce your written account of the data. However, make sure you’re only using tables and figures to supplement , rather than replace, your written account of the results (see the 'What to avoid' tab for more on this).
Figures and tables need to be numbered in order of when they appear in the dissertation, and they should be capitalised. You also need to make direct reference to them in the text, which you can do (with some variation) in one of the following ways:
Figure 1 shows…
The results of the test (see Figure 1) demonstrate…
The actual figures and tables themselves also need to be accompanied by a caption that briefly outlines what is displayed. For example:
Table 1. Variables of the regression model
Table captions normally appear above the table, whilst figures or other such graphical forms appear below, although it’s worth confirming this with your supervisor as the formatting can change depending on the school or discipline. The style guide used for writing in your subject area (e.g., Harvard, MLA, APA, OSCOLA) often dictates correct formatting of tables, graphs and figures, so have a look at your style guide for additional support.
Using quotations
If your qualitative data comes from interviews and focus groups, your data will largely consist of quotations from participants. When presenting this data, you should identify and group the most common and interesting responses and then quote two or three relevant examples to illustrate this point. Here’s a brief example from a qualitative study on the habits of online food shoppers:
Regardless of whether or not participants regularly engage in online food shopping, all but two respondents commented, in some form, on the convenience of online food shopping:
"It’s about convenience for me. I’m at work all week and the weekend doesn’t allow much time for food shopping, so knowing it can be ordered and then delivered in 24 hours is great for me” (Participant A).
"It fits around my schedule, which is important for me and my family” (Participant D).
"In the past, I’ve always gone food shopping after work, which has always been a hassle. Online food shopping, however, frees up some of my time” (Participant E).
As shown in this example, each quotation is attributed to a particular participant, although their anonymity is protected. The details used to identify participants can depend on the relevance of certain factors to the research. For instance, age or gender could be included.
Writing the discussion
The discussion chapter is where “you critically examine your own results in the light of the previous state of the subject as outlined in the background, and make judgments as to what has been learnt in your work” (Evans et al., 2014: 12). Whilst the results chapter is strictly factual, reporting on the data on a surface level, the discussion is rooted in analysis and interpretation , allowing you and your reader to delve beneath the surface.
Next, we will review some of the most important factors to consider when writing your discussion chapter.
Like the results, there is no single way to structure your discussion chapter. As always, it depends on the nature of your dissertation and whether you’re dealing with qualitative, quantitative or mixed-methods research. It’s good to be consistent with the results chapter, so you could structure your discussion chapter, where possible, in the same way as your results.
When it comes to structure, it’s particularly important that you guide your reader through the various points, subtopics or themes of your discussion. You should do this by structuring sections of your discussion, which might incorporate three or four paragraphs around the same theme or issue, in a three-part way that mirrors the typical three-part essay structure of introduction, main body and conclusion.

Figure 1: The three-part cycle that embodies a typical essay structure and reflects how you structure themes or subtopics in your discussion.
This is your topic sentence where you clearly state the focus of this paragraph/section. It’s often a fairly short, declarative statement in order to grab the reader’s attention, and it should be clearly related to your research purpose, such as responding to a research question.
This constitutes your analysis where you explore the theme or focus, outlined in the topic sentence, in further detail by interrogating why this particular theme or finding emerged and the significance of this data. This is also where you bring in the relevant secondary literature.
This is the evaluative stage of the cycle where you explicitly return back to the topic sentence and tell the reader what this means in terms of answering the relevant research question and establishing new knowledge. It could be a single sentence, or a short paragraph, and it doesn’t strictly need to appear at the end of every section or theme. Instead, some prefer to bring the main themes together towards the end of the discussion in a single paragraph or two. Either way, it’s imperative that you evaluate the significance of your discussion and tell the reader what this means.
A note on the three-part structure
This is often how you’re taught to construct a paragraph, but the themes and ideas you engage with at dissertation level are going to extend beyond the confines of a short paragraph. Therefore, this is a structure to guide how you write about particular themes or patterns in your discussion. Think of this structure like a cycle that you can engage in its smallest form to shape a paragraph; in a slightly larger form to shape a subsection of a chapter; and in its largest form to shape the entire chapter. You can 'level up' the same basic structure to accommodate a deeper breadth of thinking and critical engagement.
Using secondary literature
Your discussion chapter should return to the relevant literature (previously identified in your literature review ) in order to contextualise and deepen your reader’s understanding of the findings. This might help to strengthen your findings, or you might find contradictory evidence that serves to counter your results. In the case of the latter, it’s important that you consider why this might be and the implications for this. It’s through your incorporation of secondary literature that you can consider the question, ‘What do we now know that we didn’t before?’
Limitations
You may have included a limitations section in your methodology chapter (see our Writing the Methodology guide ), but it’s also common to have one in your discussion chapter. The difference here is that your limitations are directly associated with your results and the capacity to interpret and analyse those results.
Think of it this way: the limitations in your methodology refer to the issues identified before conducting the research, whilst the limitations in your discussion refer to the issues that emerged after conducting the research. For example, you might only be able to identify a limitation about the external validity or generalisability of your research once you have processed and analysed the data. Try not to overstress the limitations of your work – doing so can undermine the work you’ve done – and try to contextualise them, perhaps by relating them to certain limitations of other studies.
Recommendations
It’s often good to follow your limitations with some recommendations for future research. This creates a neat linearity from what didn’t work, or what could be improved, to how other researchers could address these issues in the future. This helps to reposition your limitations in a positive way by offering an action-oriented response. Try to limit the amount of recommendations you discuss – too many can bring the end of your discussion to a rather negative end as you’re ultimately focusing on what should be done, rather than what you have done. You also don’t need to repeat the recommendations in your conclusion if you’ve included them here.
What to avoid
This portion of the guide will cover some common missteps you should try to avoid in writing your results and discussion.
Over-reliance on tables and figures
It’s very common to produce visual representations of data, such as graphs and tables, and to use these representations in your results chapter. However, the use of these figures should not entirely replace your written account of the data. You don’t need to specify every detail in the data set, but you should provide some written account of what the data shows, drawing your reader’s attention to the most important elements of the data. The figures should support your account and help to contextualise your results. Simply stating, ‘look at Table 1’, without any further detail is not sufficient. Writers often try to do this as a way of saving words, but your markers will know!
Ignoring unexpected or contradictory data
Research can be a complex process with ups and downs, surprises and anomalies. Don’t be tempted to ignore any data that doesn’t meet your expectations, or that perhaps you’re struggling to explain. Failing to report on data for these, and other such reasons, is a problem because it undermines your credibility as a researcher, which inevitably undermines your research in the process. You have to do your best to provide some reason to such data. For instance, there might be some methodological reason behind a particular trend in the data.
Including raw data
You don’t need to include any raw data in your results chapter – raw data meaning unprocessed data that hasn’t undergone any calculations or other such refinement. This can overwhelm your reader and obscure the clarity of the research. You can include raw data in an appendix, providing you feel it’s necessary.
Presenting new results in the discussion
You shouldn’t be stating original findings for the first time in the discussion chapter. The findings of your study should first appear in your results before elaborating on them in the discussion.
Overstressing the significance of your research
It’s important that you clarify what your research demonstrates so you can highlight your own contribution to the research field. However, don’t overstress or inflate the significance of your results. It’s always difficult to provide definitive answers in academic research, especially with qualitative data. You should be confident and authoritative where possible, but don’t claim to reach the absolute truth when perhaps other conclusions could be reached. Where necessary, you should use hedging (see definition) to slightly soften the tone and register of your language.
Definition: Hedging refers to 'the act of expressing your attitude or ideas in tentative or cautious ways' (Singh and Lukkarila, 2017: 101). It’s mostly achieved through a number of verbs or adverbs, such as ‘suggest’ or ‘seemingly.’
Q: What’s the difference between the results and discussion?
A: The results chapter is a factual account of the data collected, whilst the discussion considers the implications of these findings by relating them to relevant literature and answering your research question(s). See the tab 'The Differences' in this guide for more detail.
Q: Should the discussion include recommendations for future research?
A: Your dissertation should include some recommendations for future research, but it can vary where it appears. Recommendations are often featured towards the end of the discussion chapter, but they also regularly appear in the conclusion chapter (see our Writing the Conclusion guide for more). It simply depends on your dissertation and the conventions of your school or department. It’s worth consulting any specific guidance that you’ve been given, or asking your supervisor directly.
Q: Should the discussion include the limitations of the study?
A: Like the answer above, you should engage with the limitations of your study, but it might appear in the discussion of some dissertations, or the conclusion of others. Consider the narrative flow and whether it makes sense to include the limitations in your discussion chapter, or your conclusion. You should also consult any discipline-specific guidance you’ve been given, or ask your supervisor for more. Be mindful that this is slightly different to the limitations outlined in the methodology or methods chapter (see our Writing the Methodology guide vs. the 'Discussion' tab of this guide).
Q: Should the results and discussion be in the first-person or third?
A: It’s important to be consistent , so you should use whatever you’ve been using throughout your dissertation. Third-person is more commonly accepted, but certain disciplines are happy with the use of first-person. Just remember that the first-person pronoun can be a distracting, but powerful device, so use it sparingly. Consult your lecturer for discipline-specific guidance.
Q: Is there a difference between the discussion and the conclusion of a dissertation?
A: Yes, there is a difference. The discussion chapter is a detailed consideration of how your findings answer your research questions. This includes the use of secondary literature to help contextualise your discussion. Rather than considering the findings in detail, the conclusion briefly summarises and synthesises the main findings of your study before bringing the dissertation to a close. Both are similar, particularly in the way they ‘broaden out’ to consider the wider implications of the research. They are, however, their own distinct chapters, unless otherwise stated by your supervisor.
The results and discussion chapters (or chapter) constitute a large part of your dissertation as it’s here where your original contribution is foregrounded and discussed in detail. Remember, the results chapter simply reports on the data collected, whilst the discussion is where you consider your research questions and/or hypothesis in more detail by interpreting and interrogating the data. You can integrate both into a single chapter and weave the interpretation of your findings throughout the chapter, although it’s common for both the results and discussion to appear as separate chapters. Consult your supervisor for further guidance.
Here’s a final checklist for writing your results and discussion. Remember that not all of these points will be relevant for you, so make sure you cover whatever’s appropriate for your dissertation. The asterisk (*) indicates any content that might not be relevant for your dissertation. To download a copy of the checklist to save and edit, please use the Word document, below.
- Results and discussion self-evaluation checklist

- << Previous: The Methodology
- Next: The Conclusion >>
- Last Updated: Apr 19, 2024 12:38 PM
- URL: https://library.soton.ac.uk/writing_the_dissertation
Have a language expert improve your writing
Run a free plagiarism check in 10 minutes, automatically generate references for free.
- Knowledge Base
- Dissertation
- How to Write a Discussion Section | Tips & Examples
How to Write a Discussion Section | Tips & Examples
Published on 21 August 2022 by Shona McCombes . Revised on 25 October 2022.
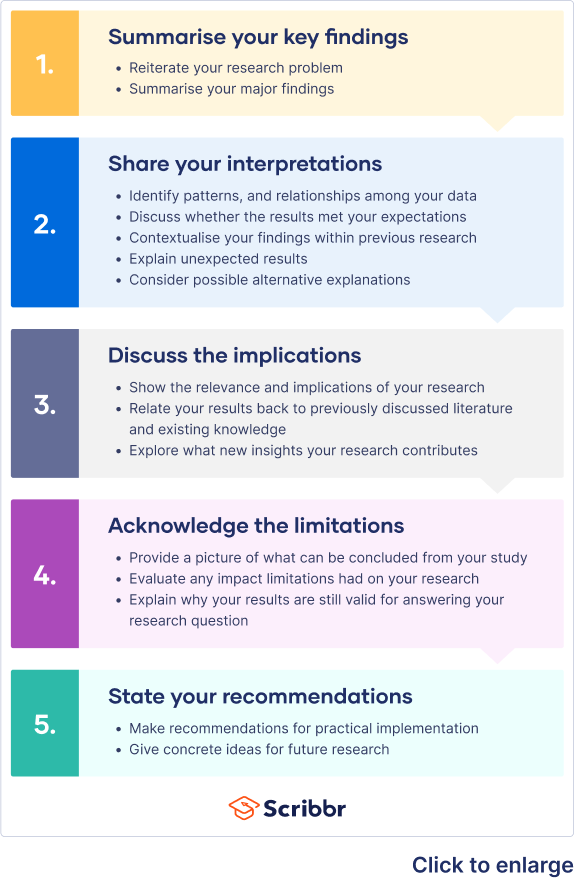
The discussion section is where you delve into the meaning, importance, and relevance of your results .
It should focus on explaining and evaluating what you found, showing how it relates to your literature review , and making an argument in support of your overall conclusion . It should not be a second results section .
There are different ways to write this section, but you can focus your writing around these key elements:
- Summary: A brief recap of your key results
- Interpretations: What do your results mean?
- Implications: Why do your results matter?
- Limitations: What can’t your results tell us?
- Recommendations: Avenues for further studies or analyses
Instantly correct all language mistakes in your text
Be assured that you'll submit flawless writing. Upload your document to correct all your mistakes.
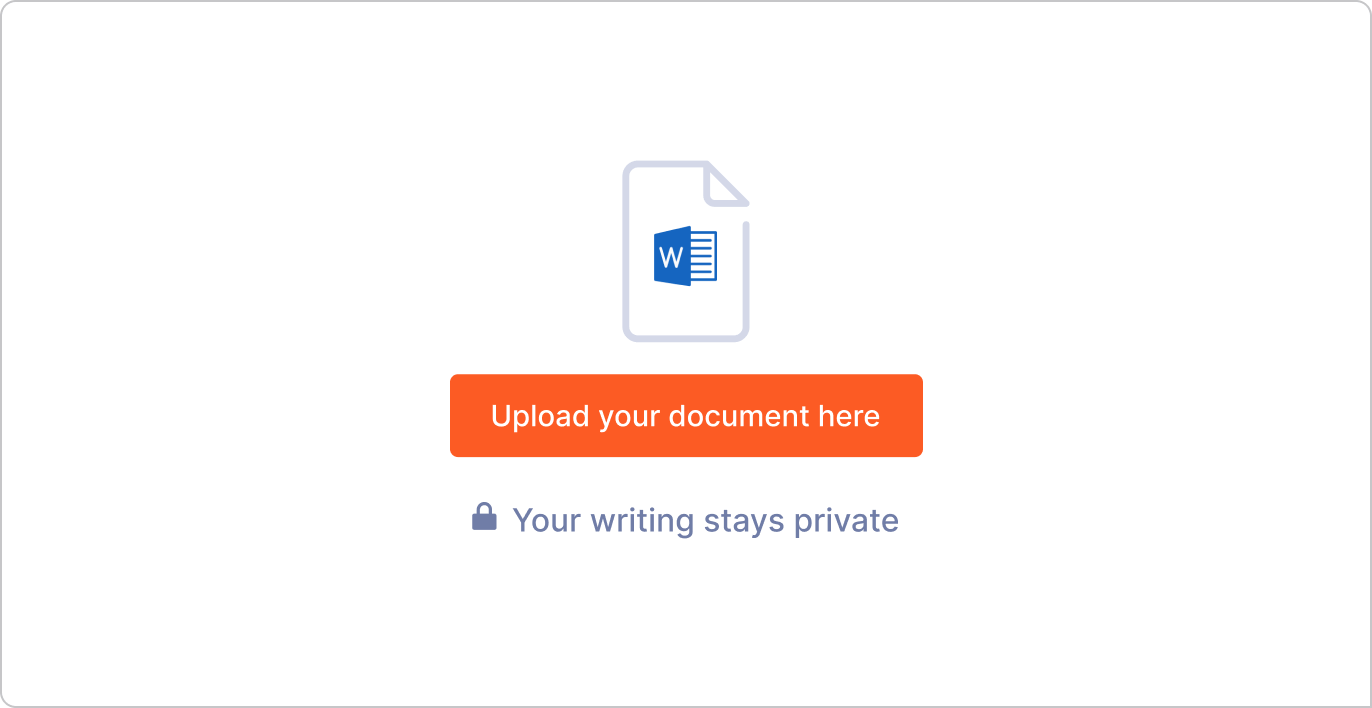
Table of contents
What not to include in your discussion section, step 1: summarise your key findings, step 2: give your interpretations, step 3: discuss the implications, step 4: acknowledge the limitations, step 5: share your recommendations, discussion section example.
There are a few common mistakes to avoid when writing the discussion section of your paper.
- Don’t introduce new results: You should only discuss the data that you have already reported in your results section .
- Don’t make inflated claims: Avoid overinterpretation and speculation that isn’t directly supported by your data.
- Don’t undermine your research: The discussion of limitations should aim to strengthen your credibility, not emphasise weaknesses or failures.
Prevent plagiarism, run a free check.
Start this section by reiterating your research problem and concisely summarising your major findings. Don’t just repeat all the data you have already reported – aim for a clear statement of the overall result that directly answers your main research question . This should be no more than one paragraph.
Many students struggle with the differences between a discussion section and a results section . The crux of the matter is that your results sections should present your results, and your discussion section should subjectively evaluate them. Try not to blend elements of these two sections, in order to keep your paper sharp.
- The results indicate that …
- The study demonstrates a correlation between …
- This analysis supports the theory that …
- The data suggest that …
The meaning of your results may seem obvious to you, but it’s important to spell out their significance for your reader, showing exactly how they answer your research question.
The form of your interpretations will depend on the type of research, but some typical approaches to interpreting the data include:
- Identifying correlations , patterns, and relationships among the data
- Discussing whether the results met your expectations or supported your hypotheses
- Contextualising your findings within previous research and theory
- Explaining unexpected results and evaluating their significance
- Considering possible alternative explanations and making an argument for your position
You can organise your discussion around key themes, hypotheses, or research questions, following the same structure as your results section. Alternatively, you can also begin by highlighting the most significant or unexpected results.
- In line with the hypothesis …
- Contrary to the hypothesised association …
- The results contradict the claims of Smith (2007) that …
- The results might suggest that x . However, based on the findings of similar studies, a more plausible explanation is x .
As well as giving your own interpretations, make sure to relate your results back to the scholarly work that you surveyed in the literature review . The discussion should show how your findings fit with existing knowledge, what new insights they contribute, and what consequences they have for theory or practice.
Ask yourself these questions:
- Do your results support or challenge existing theories? If they support existing theories, what new information do they contribute? If they challenge existing theories, why do you think that is?
- Are there any practical implications?
Your overall aim is to show the reader exactly what your research has contributed, and why they should care.
- These results build on existing evidence of …
- The results do not fit with the theory that …
- The experiment provides a new insight into the relationship between …
- These results should be taken into account when considering how to …
- The data contribute a clearer understanding of …
- While previous research has focused on x , these results demonstrate that y .
The only proofreading tool specialized in correcting academic writing
The academic proofreading tool has been trained on 1000s of academic texts and by native English editors. Making it the most accurate and reliable proofreading tool for students.
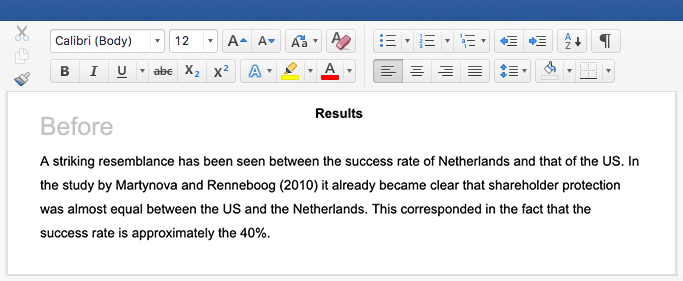
Correct my document today
Even the best research has its limitations. Acknowledging these is important to demonstrate your credibility. Limitations aren’t about listing your errors, but about providing an accurate picture of what can and cannot be concluded from your study.
Limitations might be due to your overall research design, specific methodological choices , or unanticipated obstacles that emerged during your research process.
Here are a few common possibilities:
- If your sample size was small or limited to a specific group of people, explain how generalisability is limited.
- If you encountered problems when gathering or analysing data, explain how these influenced the results.
- If there are potential confounding variables that you were unable to control, acknowledge the effect these may have had.
After noting the limitations, you can reiterate why the results are nonetheless valid for the purpose of answering your research question.
- The generalisability of the results is limited by …
- The reliability of these data is impacted by …
- Due to the lack of data on x , the results cannot confirm …
- The methodological choices were constrained by …
- It is beyond the scope of this study to …
Based on the discussion of your results, you can make recommendations for practical implementation or further research. Sometimes, the recommendations are saved for the conclusion .
Suggestions for further research can lead directly from the limitations. Don’t just state that more studies should be done – give concrete ideas for how future work can build on areas that your own research was unable to address.
- Further research is needed to establish …
- Future studies should take into account …
- Avenues for future research include …
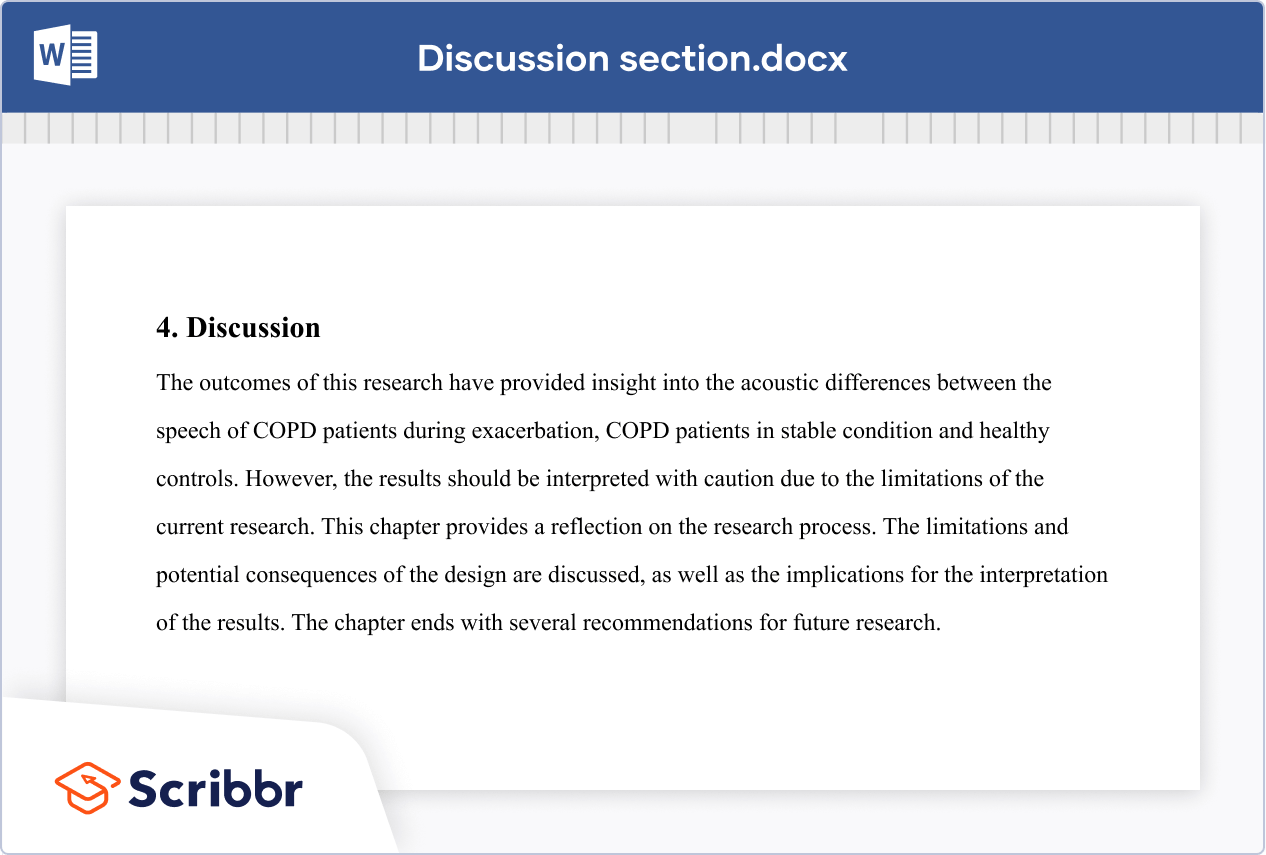
Cite this Scribbr article
If you want to cite this source, you can copy and paste the citation or click the ‘Cite this Scribbr article’ button to automatically add the citation to our free Reference Generator.
McCombes, S. (2022, October 25). How to Write a Discussion Section | Tips & Examples. Scribbr. Retrieved 14 May 2024, from https://www.scribbr.co.uk/thesis-dissertation/discussion/
Is this article helpful?
Shona McCombes
Other students also liked, how to write a results section | tips & examples, research paper appendix | example & templates, how to write a thesis or dissertation introduction.

- Dissertation & Thesis Guides
- Basics of Dissertation & Thesis Writing
- How to Write a Dissertation Discussion Chapter: Guide & Examples
- Speech Topics
- Basics of Essay Writing
- Essay Topics
- Other Essays
- Main Academic Essays
- Research Paper Topics
- Basics of Research Paper Writing
- Miscellaneous
- Chicago/ Turabian
- Data & Statistics
- Methodology
- Admission Writing Tips
- Admission Advice
- Other Guides
- Student Life
- Studying Tips
- Understanding Plagiarism
- Academic Writing Tips

- Essay Guides
- Research Paper Guides
- Formatting Guides
- Basics of Research Process
- Admission Guides
How to Write a Dissertation Discussion Chapter: Guide & Examples

Table of contents

Use our free Readability checker
Dissertation discussion section is a chapter that interprets the results obtained from research and offers an in-depth analysis of findings. In this section, students need to analyze the outcomes, evaluate their significance, and compare them to previous research. The discussion section may also explore the limitations of the study and suggest further research perspectives.
If you are stuck with your thesis or dissertation discussion chapter, you are in the right place to complete this section successfully. This article will outline our best solutions and methods on how to write the discussion of a dissertation or thesis. We also will share advanced dissertation discussion examples to help you finalize your PhD work. Feel like academic writing gives you hassles? Remember that you can always rely on academic experts qualified in your field to get professional dissertation help online .
What Is a Dissertation Discussion?
First and foremost, students need to have a clear understanding of what dissertation discussion is. This is not the same as your results section , where you share data from your research. You are going deeper into the explanation of the existing data in your thesis or dissertation discussion section. In other words, you illustrate practical implications of your research and how the data can be used, researched further, or limited. What will make your discussion section of a dissertation excellent:
- clear structure
- practical implication
- elaboration on future work on this topic.
This section should go after research methodology and before the dissertation conclusion . It should be directly relevant to questions posed in your introduction. The biggest mistake you can make is to rewrite your result chapter with other words and add some limitations and recommendation paragraphs. However, this is an entirely different type of writing you need to complete.
Purpose of a Dissertation Discussion Chapter
A dissertation discussion section is critical to explaining students’ findings and the application of data to real-life cases. As we mentioned before, this section will often be read right after the dissertation methods . It evaluates and elaborates on findings and helps to understand the importance of your performed thesis research. A dissertation discussion opens a new perspective on further research on the same field or topic. It also outlines critical data to consider in subsequent studies. In a nutshell, this is the section where you explain your work to a broad audience.
Structure of a Dissertation Discussion Section
Let’s start your writing journey of this research part with a clear delineation of what it should include and then briefly discuss each component. Here are some basic things you need to consider for an excellent discussion chapter of dissertation :
- Brief summary It does not mean copying an introduction section. However, the first few paragraphs will make an overview of your findings and topic.
- Interpretations This is a critical component of your work — elaborate on your results and explain possible ways of using them.
- Implication Research work is not just 100+ pages of text. Students should explain and illustrate how it could be used for solving practical problems.
- Constraints This is where you outline your limitations. For instance, your research was done only on students, and it may have different results with elderly people.
- Recommendations You can also define possible ways of future research on the exact topic when writing a discussion for your thesis or dissertation. Tell readers, for example, that it would be helpful to run similar research in other specific circumstances.
How to Write a Dissertation Discussion Chapter?
One of the most commonly asked questions for our experts is how to write the discussion section of a dissertation or thesis. We understand why it can be complicated to get a clear answer. Students often think that this section is similar to the result chapter and just retells it in other words. But it is not so. Let’s go through all steps to writing a discussion in a dissertation, and share our best examples from academic papers.
1. Remind Your Research Questions & Objectives
Writing the discussion chapter of a dissertation is not a big deal if you understand its aim and each component in a text structure. First of all, you need to evaluate how your results help to answer research questions you defined in the beginning. It is not about repeating the result, you did it in previous paragraphs. However, dissertation or thesis discussion should underline how your findings help to answer the research problem. Start writing from a brief intro by recalling research questions or hypotheses . Then, show how your results answer them or support a hypothesis in your work.
2. Sum Up Key Findings
Next part of your discussion for dissertation is to provide a short summary of previous data. But do not respite the same summary paragraphs from results or introduction of a dissertation . Here researchers should be more thoughtful and go deeper into the work’s aims. Try to explain in a few sentences what you get from running research. For instance, starters usually write the statement that “our data proves that…” or “survey results illustrate a clear correlation between a and b that is critical for proving our working hypothesis…”. A discussion chapter of your dissertation is not just a fixation on results but a more profound summary connected to research goals and purpose. Here is an example: Summary of Findings Example
According to the data, implementing the co-orientation theory was successful and can be used for the same circumstances in the future. As we found, most participants agreed with the importance of those theses on the five fundamental reforms. It means that the results identified a successful government work in choosing the messages to communicate about examined reforms. At the same time, the situation is not so favorable with implementing the principles of two-way symmetrical communications. According to the results, people did not feel that the government had a mutual, open, and equal dialogue with the public about the reforms.
3. Interpret the Results
The most critical part of a discussion section is to explain and enact the results you’ve got. It is the most significant part of any text. Students should be clear about what to include in these paragraphs. Here is some advice to make this elaboration structured:
- Identify correlations or patterns in the data for dissertation discussion.
- Underline how results can answer research questions or prove your hypothesis.
- Emphasize how your findings are connected to the previous topic studies.
- Point out essential statements you can use in future research.
- Evaluate the significance of your results and any unexpected data you have.
- What others can learn from your research and how this work contributes to the field.
- Consider any possible additional or unique explanation of your findings.
- Go deeper with options of how results can be applied in practice.
Writing a dissertation discussion chapter can be tough, but here is a great sample to learn from. Example of Interpretations in Disssertation Discussion
Our study underlines the importance of future research on using TikTok for political communication. As discussed above, TikTok is the most commonly used social media platform for many young voters. This means that political discussion will also move to this platform. Our research and typology of political communication content can be used in the future planning of effective political campaigns. For example, we can assume that “play videos” have enormous potential to facilitate complicated topics and provide specific agenda settings. We also identified additional affordances of TikTok used for political communication, such as built-in video editors, playlists for specific topics, a green screen for news explainers, and duets for reflection on news and discussion. It means that these features make TikTok suitable for efficient political communications.
4. Discuss How Your Findings Relate to the Literature
Here we came to the implications of your findings for the dissertation discussion. In other words, this is a few sentences on how your work is connected to other studies on the same research topic or what literature gap you are going to fill with the data and research you launched. Remember to mention how your study address the limitations you have discovered while writing a literature review . First, outline how your hypothesis relates to theories or previous works in the field. Maybe, you challenged some theories or tried to define your own. Be specific in this section. Second, define a practical implementation of your work. Maybe, it can support recommendations or change legislation. Discussion chapter of a thesis is a place where you explain your work, make it valuable, and incorporate additional meaning for some specific data. Example of Implications in Disssertation Discussion
As we pointed out in the literature review, there are few works on using TikTok affordances for political communications, and this topic can be expanded in the future. Government institutions have already understood the importance of this platform for efficient communication with younger audiences, and we will see more political projects on TikTok. That is why expanding research on using TikTok for political communication will be enormous in the following years. Our work is one of the first research on the role of emerging media in war communication and can be used as a practical guide for government's strategic planning in times of emergencies.
5. Mention Possible Limitations
It is pretty tricky to conduct research without limitations. You will always have some, which does not mean that your work is not good. When you write a discussion chapter in a thesis or dissertation, focus on what may influence your results and how changing independent variables can affect your data collection methods and final outcomes. Here are some points to consider when you structure your dissertation discussion limitation part:
- If results can change in case you change the reference group?
- What will happen with data if it changes circumstances?
- What could influence results?
Critical thinking and analysis can help you to outline possible limitations. It can be the age of the reference group, change of questionnaire in a survey, or specific use of data extraction equipment. Be transparent about what could affect your results. Example of Complications
Although this study has provided critical first insights into the effects of multimodal disinformation and rebuttals, there are some limitations. First and most importantly, the effects of multimodal disinformation and rebuttals partially depend on the topic of the message. Although fact-checkers reduce credibility of disinformation in both settings, and attitudinal congruence plays a consistent role in conditioning responses to multimodal disinformation, visuals do not have the same impact on affecting the credibility of news on school shootings and refugees.
6. Provide Recommendations for Further Research
Writing a dissertation discussion also makes a connection to possible future research. So, other scientists may complete that. While elaborating on possible implementations of your study, you may also estimate future approaches in topic research. Here are some points to consider while your discussion in thesis writing:
- Outline questions related to your topic that you did not answer in defined study or did not outline as research questions. There are other possible gaps to research.
- Suggest future research based on limitations. For example, if you define surveyed people’s age as a limitation, recommend running another survey for older or younger recipients.
Example of Recommendations
As we mentioned before, our study has some limitations, as the research was conducted based on data from United State citizens. However, for a better understanding of government communication practices, it would be productive to implement the same research in other countries. Some cultural differences can influence the communication strategies the government uses in times of emergency. Another possible way to examine this topic is to conduct research using a specific period of time. For future studies, it will be beneficial to expand the number of survey recipients.
7. Conclude Your Thesis/ Dissertation Discussion
You are almost done, the last step is to provide a brief summary of a section. It is not the same as a conclusion for whole research. However, you need to briefly outline key points from the dissertation discussion. To finalize writing the discussion section of a dissertation, go through the text and check if there is no unimportant information. Do not overload the text with relevant data you did not present in the result section. Be specific in your summary paragraphs. It is a holistic view of everything you pointed out. Provide a few sentences to systemize all you outlined in the text. Example of a Concluding Summary in a Dissertation Discussion Section
To summarize, Airbnb has expertise in communicating CSR and CSA campaigns. We defined their communication strategy about the program for Ukrainian refugees as quite successful. They applied all the principles of CSR communication best practices, used dialogic theory to engage with the public on social media, and created clear messaging on applying for the program. Airbnb examples of CSR communication can be used by other businesses to create a communication strategy for unplanned CSR campaigns. Moreover, it can be further researched how Airbnb's CSR campaign influenced the organizational reputation in the future.
Dissertation Discussion Example
If we need to share one piece of practical advice, it would be to use thesis or dissertation discussion examples when writing your own copy. StudyCrumb provides the best samples from real students' work to help you understand the stylistic and possible structure of this part. It does not mean you need to copy and paste them into your work. However, you can use a dissertation discussion example for inspiration and brainstorming ideas for breaking writing blocks. Here’s a doctoral thesis discussion chapter example.
Dissertation Discussion Writing Tips
Before reading this blog, you should already know how to write a thesis discussion. However, we would share some essential tips you need to have in mind while working on the document.
- Be consistent Your dissertation discussion chapter is a part of bigger research, and it should be in line with your whole work.
- Understand your reader You are writing an academic text that will be analyzed by professionals and experts in the same field. Be sure that you are not trying to simplify your discussion.
- Be logical Do not jump into a new line of discussion if you did not delineate it as a research question at the beginning.
- Be clear Do not include any data that was not presented in the result section.
- Consider word choice Use such terms as “our data indicate…” or “our data suggests…” instead of “the data proves.”
- Use proper format Follow the formatting rules specified by a specific paper style (e.g., APA style format , MLA format , or Chicago format ) or provided by your instructor.
Bottom Line on Writing a Dissertation Discussion Chapter
At this stage, it should not be a question for you on how to write a discussion chapter in a PhD thesis or dissertation. Let’s make it clear. It is not a result section but still a place to elaborate on data and go deeper with explanations. Dissertation discussion section includes some intro, result interpretations, limitations, and recommendations for future research. Our team encourages you to use examples before starting your own piece of writing. It will help you to realize the purpose and structure of this chapter and inspire better texts! If you have other questions regarding the PhD writing process, check our blog for more insights. From detailed instruction on how to write a dissertation or guide on formatting a dissertation appendix , we’ve got you covered.

Order dissertation discussion from our proficient writers. They will take a significant burden off of you. Instead, they will carry out high-level academic work in a short time.
FAQ About Dissertation Discussion Chapter
1. where does a discussion section go in a dissertation.
Dissertation discussion section is used to go right after the result chapter. The logic is simple — you share your data and then go to the elaboration and explanation of it. Check the sample thesis we provide to students for details on structure.
2. How long should a dissertation discussion chapter be?
It is not a surprise that dissertation discussion chapter is extremely significant for the research. Here you will go into the details of your study and interpret results to prove or not your hypothesis. It should take almost 25% of your work.
3. What tense should I use in a dissertation discussion?
Thesis or dissertation discussion used to have some rules on using tenses. You need to use the present tense when referring to established facts and use the past tense when referring to previous studies. And check your text before submission to ensure that you did not miss something.
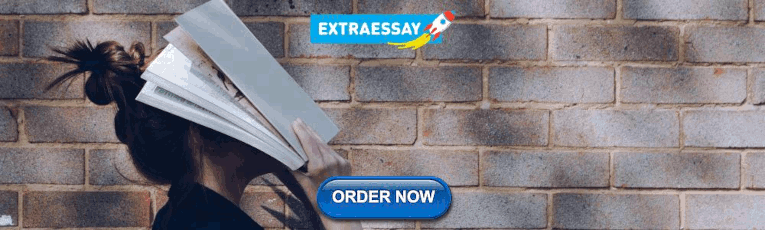
4. What not to include in a dissertation discussion section?
The answer is easy. Discussion section of a dissertation should not include any new findings or describe some unsupported claims. Also, do not try to feel all possible gaps with one research. It may be better to outline your ideas for future studies in recommendations.

Joe Eckel is an expert on Dissertations writing. He makes sure that each student gets precious insights on composing A-grade academic writing.
You may also like


Library Guides
Dissertations 5: findings, analysis and discussion: home.
- Results/Findings
Alternative Structures
The time has come to show and discuss the findings of your research. How to structure this part of your dissertation?
Dissertations can have different structures, as you can see in the dissertation structure guide.
Dissertations organised by sections
Many dissertations are organised by sections. In this case, we suggest three options. Note that, if within your course you have been instructed to use a specific structure, you should do that. Also note that sometimes there is considerable freedom on the structure, so you can come up with other structures too.
A) More common for scientific dissertations and quantitative methods:
- Results chapter
- Discussion chapter
Example:
- Introduction
- Literature review
- Methodology
- (Recommendations)
if you write a scientific dissertation, or anyway using quantitative methods, you will have some objective results that you will present in the Results chapter. You will then interpret the results in the Discussion chapter.
B) More common for qualitative methods
- Analysis chapter. This can have more descriptive/thematic subheadings.
- Discussion chapter. This can have more descriptive/thematic subheadings.
- Case study of Company X (fashion brand) environmental strategies
- Successful elements
- Lessons learnt
- Criticisms of Company X environmental strategies
- Possible alternatives
C) More common for qualitative methods
- Analysis and discussion chapter. This can have more descriptive/thematic titles.
- Case study of Company X (fashion brand) environmental strategies
If your dissertation uses qualitative methods, it is harder to identify and report objective data. Instead, it may be more productive and meaningful to present the findings in the same sections where you also analyse, and possibly discuss, them. You will probably have different sections dealing with different themes. The different themes can be subheadings of the Analysis and Discussion (together or separate) chapter(s).
Thematic dissertations
If the structure of your dissertation is thematic , you will have several chapters analysing and discussing the issues raised by your research. The chapters will have descriptive/thematic titles.
- Background on the conflict in Yemen (2004-present day)
- Classification of the conflict in international law
- International law violations
- Options for enforcement of international law
- Next: Results/Findings >>
- Last Updated: Aug 4, 2023 2:17 PM
- URL: https://libguides.westminster.ac.uk/c.php?g=696975
CONNECT WITH US
- Systematic Review
- Open access
- Published: 12 May 2024
Association between problematic social networking use and anxiety symptoms: a systematic review and meta-analysis
- Mingxuan Du 1 ,
- Chengjia Zhao 2 ,
- Haiyan Hu 1 ,
- Ningning Ding 1 ,
- Jiankang He 1 ,
- Wenwen Tian 1 ,
- Wenqian Zhao 1 ,
- Xiujian Lin 1 ,
- Gaoyang Liu 1 ,
- Wendan Chen 1 ,
- ShuangLiu Wang 1 ,
- Pengcheng Wang 3 ,
- Dongwu Xu 1 ,
- Xinhua Shen 4 &
- Guohua Zhang 1
BMC Psychology volume 12 , Article number: 263 ( 2024 ) Cite this article
186 Accesses
Metrics details
A growing number of studies have reported that problematic social networking use (PSNU) is strongly associated with anxiety symptoms. However, due to the presence of multiple anxiety subtypes, existing research findings on the extent of this association vary widely, leading to a lack of consensus. The current meta-analysis aimed to summarize studies exploring the relationship between PSNU levels and anxiety symptoms, including generalized anxiety, social anxiety, attachment anxiety, and fear of missing out. 209 studies with a total of 172 articles were included in the meta-analysis, involving 252,337 participants from 28 countries. The results showed a moderately positive association between PSNU and generalized anxiety (GA), social anxiety (SA), attachment anxiety (AA), and fear of missing out (FoMO) respectively (GA: r = 0.388, 95% CI [0.362, 0.413]; SA: r = 0.437, 95% CI [0.395, 0.478]; AA: r = 0.345, 95% CI [0.286, 0.402]; FoMO: r = 0.496, 95% CI [0.461, 0.529]), and there were different regulatory factors between PSNU and different anxiety subtypes. This study provides the first comprehensive estimate of the association of PSNU with multiple anxiety subtypes, which vary by time of measurement, region, gender, and measurement tool.
Peer Review reports
Introduction
Social network refers to online platforms that allow users to create, share, and exchange information, encompassing text, images, audio, and video [ 1 ]. The use of social network, a term encompassing various activities on these platforms, has been measured from angles such as frequency, duration, intensity, and addictive behavior, all indicative of the extent of social networking usage [ 2 ]. As of April 2023, there are 4.8 billion social network users globally, representing 59.9% of the world’s population [ 3 ]. The usage of social network is considered a normal behavior and a part of everyday life [ 4 , 5 ]. Although social network offers convenience in daily life, excessive use can lead to PSNU [ 6 , 7 ], posing potential threats to mental health, particularly anxiety symptoms (Rasmussen et al., 2020). Empirical research has shown that anxiety symptoms, including generalized anxiety (GA), social anxiety (SA), attachment anxiety (AA), and fear of missing out (FoMO), are closely related to PSNU [ 8 , 9 , 10 , 11 , 12 ]. While some empirical studies have explored the relationship between PSNU and anxiety symptoms, their conclusions are not consistent. Some studies have found a significant positive correlation [ 13 , 14 , 15 ], while others have found no significant correlation [ 16 , 17 , 18 , 19 ]. Furthermore, the degree of correlation varies widely in existing research, with reported r-values ranging from 0.12 to 0.80 [ 20 , 21 ]. Therefore, a systematic meta-analysis is necessary to clarify the impact of PSNU on individual anxiety symptoms.
Previous research lacks a unified concept of PSNU, primarily due to differing theoretical interpretations by various authors, and the use of varied standards and diagnostic tools. Currently, this phenomenon is referred to by several terms, including compulsive social networking use, problematic social networking use, excessive social networking use, social networking dependency, and social networking addiction [ 22 , 23 , 24 , 25 , 26 ]. These conceptual differences hinder the development of a cohesive and systematic research framework, as it remains unclear whether these definitions and tools capture the same underlying construct [ 27 ]. To address this lack of uniformity, this paper will use the term “problematic use” to encompass all the aforementioned nomenclatures (i.e., compulsive, excessive, dependent, and addictive use).
Regarding the relationship between PSNU and anxiety symptoms, two main perspectives exist: the first suggests a positive correlation, while the second proposes a U-shaped relationship. The former perspective, advocating a positive correlation, aligns with the social cognitive theory of mass communication. It posits that PSNU can reinforce certain cognitions, emotions, attitudes, and behaviors [ 28 , 29 ], potentially elevating individuals’ anxiety levels [ 30 ]. Additionally, the cognitive-behavioral model of pathological use, a primary framework for explaining factors related to internet-based addictions, indicates that psychiatric symptoms like depression or anxiety may precede internet addiction, implying that individuals experiencing anxiety may turn to social networking platforms as a coping mechanism [ 31 ]. Empirical research also suggests that highly anxious individuals prefer computer-mediated communication due to the control and social liberation it offers and are more likely to have maladaptive emotional regulation, potentially leading to problematic social network service use [ 32 ]. Turning to the alternate perspective, it proposes a U-shaped relationship as per the digital Goldilocks hypothesis. In this view, moderate social networking usage is considered beneficial for psychosocial adaptation, providing individuals with opportunities for social connection and support. Conversely, both excessive use and abstinence can negatively impact psychosocial adaptation [ 33 ]. In summary, both perspectives offer plausible explanations.
Incorporating findings from previous meta-analyses, we identified seven systematic reviews and two meta-analyses that investigated the association between PSNU and anxiety. The results of these meta-analyses indicated a significant positive correlation between PSNU and anxiety (ranging from 0.33 to 0.38). However, it is evident that these previous meta-analyses had certain limitations. Firstly, they focused only on specific subtypes of anxiety; secondly, they were limited to adolescents and emerging adults in terms of age. In summary, this systematic review aims to ascertain which theoretical perspective more effectively explains the relationship between PSNU and anxiety, addressing the gaps in previous meta-analyses. Additionally, the association between PSNU and anxiety could be moderated by various factors. Drawing from a broad research perspective, any individual study is influenced by researcher-specific designs and associated sample estimates. These may lead to bias compared to the broader population. Considering the selection criteria for moderating variables in empirical studies and meta-analyses [ 34 , 35 ], the heterogeneity of findings on problematic social network usage and anxiety symptoms could be driven by divergence in sample characteristics (e.g., gender, age, region) and research characteristics (measurement instrument of study variables). Since the 2019 coronavirus pandemic, heightened public anxiety may be attributed to the fear of the virus or heightened real life stress. The increased use of electronic devices, particularly smartphones during the pandemic, also instigates the prevalence of problematic social networking. Thus, our analysis focuses on three moderators: sample characteristics (participants’ gender, age, region), measurement tools (for PSNU and anxiety symptoms) and the time of measurement (before COVID-19 vs. during COVID-19).
The present study was conducted in accordance with the 2020 statement on Preferred Reporting Items for Systematic Reviews and Meta-Analyses (PRISMA) [ 36 ]. To facilitate transparency and to avoid unnecessary duplication of research, this study was registered on PROSPERO, and the number is CRD42022350902.
Literature search
Studies on the relationship between the PSNU and anxiety symptoms from 2000 to 2023 were retrieved from seven databases. These databases included China National Knowledge Infrastructure (CNKI), Wanfang Data, Chongqing VIP Information Co. Ltd. (VIP), Web of Science, ScienceDirect, PubMed, and PsycARTICLES. The search strings consisted of (a) anxiety symptoms, (b) social network, and (c) Problematic use. As shown in Table 1 , the keywords for anxiety are as follows: anxiety, generalized anxiety, social anxiety, attachment anxiety, fear of missing out, and FoMO. The keywords for social network are as follows: social network, social media, social networking site, Instagram, and Facebook. The keywords for addiction are as follows: addiction, dependence, problem/problematic use, excessive use. The search deadline was March 19, 2023. A total of 2078 studies were initially retrieved and all were identified ultimately.
Inclusion and exclusion criteria
Retrieved studies were eligible for the present meta-analysis if they met the following inclusion criteria: (a) the study provided Pearson correlation coefficients used to measure the relationship between PSNU and anxiety symptoms; (b) the study reported the sample size and the measurement instruments for the variables; (c) the study was written in English and Chinese; (d) the study provided sufficient statistics to calculate the effect sizes; (e) effect sizes were extracted from independent samples. If multiple independent samples were investigated in the same study, they were coded separately; if the study was a longitudinal study, they were coded by the first measurement. In addition, studies were excluded if they: (a) examined non-problematic social network use; (b) had an abnormal sample population; (c) the results of the same sample were included in another study and (d) were case reports or review articles. Two evaluators with master’s degrees independently assessed the eligibility of the articles. A third evaluator with a PhD examined the results and resolved dissenting views.
Data extraction and quality assessment
Two evaluators independently coded the selected articles according to the following characteristics: literature information, time of measurement (before the COVID-19 vs. during the COVID-19), sample source (developed country vs. developing country), sample size, proportion of males, mean age, type of anxiety, and measurement instruments for PSNU and anxiety symptoms. The following principles needed to be adhered to in the coding process: (a) effect sizes were extracted from independent samples. If multiple independent samples were investigated in the same study, they were coded separately; if the study was a longitudinal study, it was coded by the first measurement; (b) if multiple studies used the same data, the one with the most complete information was selected; (c) If studies reported t or F values rather than r , the following formula \( r=\sqrt{\frac{{t}^{2}}{{t}^{2}+df}}\) ; \( r=\sqrt{\frac{F}{F+d{f}_{e}}}\) was used to convert them into r values [ 37 , 38 ]. Additionally, if some studies only reported the correlation matrix between each dimension of PSNU and anxiety symptoms, the following formula \( {r}_{xy}=\frac{\sum {r}_{xi}{r}_{yj}}{\sqrt{n+n(n-1){r}_{xixj}}\sqrt{m+m(m-1){r}_{yiyj}}}\) was used to synthesize the r values [ 39 ], where n or m is the number of dimensions of variable x or variable y, respectively, and \( {r}_{xixj} \) or \( {r}_{yiyj}\) represents the mean of the correlation coefficients between the dimensions of variable x or variable y, respectively.
Literature quality was determined according to the meta-analysis quality evaluation scale developed [ 40 ]. The quality of the post-screening studies was assessed by five dimensions: sampling method, efficiency of sample collection, level of publication, and reliability of PSNU and anxiety symptom measurement instruments. The total score of the scale ranged from 0 to 10; higher scores indicated better quality of the literature.
Data analysis
All data were performed using Comprehensive Meta Analysis 3.3 (CMA 3.3). Pearson’s product-moment coefficient r was selected as the effect size index in this meta-analysis. Firstly, \( {\text{F}\text{i}\text{s}\text{h}\text{e}\text{r}}^{{\prime }}\text{s} Z=\frac{1}{2}\times \text{ln}\left(\frac{1+r}{1-r}\right)\) was used to convert the correlation coefficient to Fisher Z . Then the formula \( SE=\sqrt{\frac{1}{n-3}}\) was used to calculate the standard error ( SE ). Finally, the summary of r was obtained from the formula \( r=\frac{{e}^{2z}-1}{{e}^{2z}+1}\) for a comprehensive measure of the relationship between PSNU and anxiety symptoms [ 37 , 41 ].
Although the effect sizes estimated by the included studies may be similar, considering the actual differences between studies (e.g., region and gender), the random effects model was a better choice for data analysis for the current meta-analysis. The heterogeneity of the included study effect sizes was measured for significance by Cochran’s Q test and estimated quantitatively by the I 2 statistic [ 42 ]. If the results indicate there is a significant heterogeneity (the Q test: p -value < 0.05, I 2 > 75) and the results of different studies are significantly different from the overall effect size. Conversely, it indicates there are no differences between the studies and the overall effect size. And significant heterogeneity tends to indicate the possible presence of potential moderating variables. Subgroup analysis and meta-regression analysis were used to examine the moderating effect of categorical and continuous variables, respectively.
Funnel plots, fail-safe number (Nfs) and Egger linear regression were utilized to evaluate the publication bias [ 43 , 44 , 45 ]. The likelihood of publication bias was considered low if the intercept obtained from Egger linear regression was not significant. A larger Nfs indicated a lower risk of publication bias, and if Nfs < 5k + 10 (k representing the original number of studies), publication bias should be a concern [ 46 ]. When Egger’s linear regression was significant, the Duval and Tweedie’s trim-and-fill was performed to correct the effect size. If there was no significant change in the effect size, it was assumed that there was no serious publication bias [ 47 ].
A significance level of P < 0.05 was deemed applicable in this study.
Sample characteristics
The PRISMA search process is depicted in Fig. 1 . The database search yielded 2078 records. After removing duplicate records and screening the title and abstract, the full text was subject to further evaluation. Ultimately, 172 records fit the inclusion criteria, including 209 independent effect sizes. The present meta-analysis included 68 studies on generalized anxiety, 44 on social anxiety, 22 on attachment anxiety, and 75 on fear of missing out. The characteristics of the selected studies are summarized in Table 2 . The majority of the sample group were adults. Quality scores for selected studies ranged from 0 to 10, with only 34 effect sizes below the theoretical mean, indicating high quality for the included studies. The literature included utilized BSMAS as the primary tool to measure PSNU, DASS-21-A to measure GA, IAS to measure SA, ECR to measure AA, and FoMOS to measure FoMO.
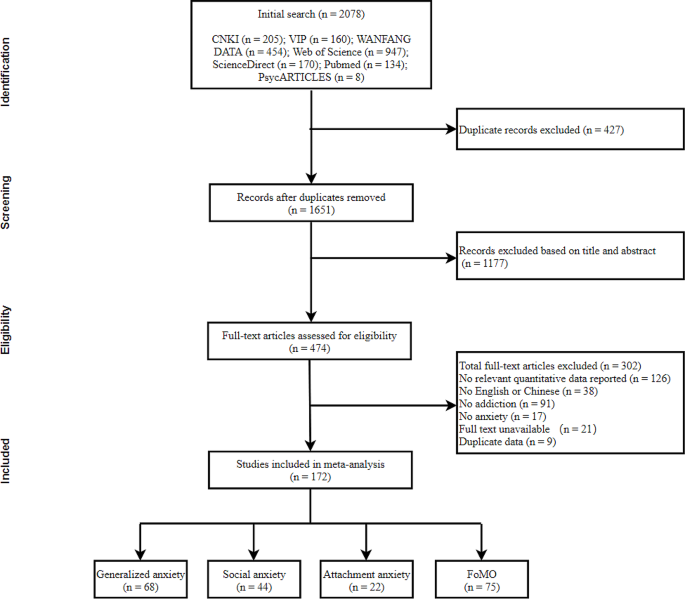
Flow chart of the search and selection strategy
Overall analysis, homogeneity tests and publication bias
As shown in Table 3 , there was significant heterogeneity between PSNU and all four anxiety symptoms (GA: Q = 1623.090, I 2 = 95.872%; SA: Q = 1396.828, I 2 = 96.922%; AA: Q = 264.899, I 2 = 92.072%; FoMO: Q = 1847.110, I 2 = 95.994%), so a random effects model was chosen. The results of the random effects model indicate a moderate positive correlation between PSNU and anxiety symptoms (GA: r = 0.350, 95% CI [0.323, 0.378]; SA: r = 0.390, 95% CI [0.347, 0.431]; AA: r = 0.345, 95% CI [0.286, 0.402]; FoMO: r = 0.496, 95% CI [0.461, 0.529]).
Figure 2 shows the funnel plot of the relationship between PSNU and anxiety symptoms. No significant symmetry was seen in the funnel plot of the relationship between PSNU and GA and between PSNU and SA. And the Egger’s regression results also indicated that there might be publication bias ( t = 3.775, p < 0.001; t = 2.309, p < 0.05). Therefore, it was necessary to use fail-safe number (Nfs) and the trim and fill method for further examination and correction. The Nfs for PSNU and GA as well as PSNU and SA are 4591 and 7568, respectively. Both Nfs were much larger than the standard 5 k + 10. After performing the trim and fill method, 14 effect sizes were added to the right side of the funnel plat (Fig. 2 .a), the correlation coefficient between PSNU and GA changed to ( r = 0.388, 95% CI [0.362, 0.413]); 10 effect sizes were added to the right side of the funnel plat (Fig. 2 .b), the correlation coefficient between PSNU and SA changed to ( r = 0.437, 95% CI [0.395, 0.478]). The correlation coefficients did not change significantly, indicating that there was no significant publication bias associated with the relationship between PSNU and these two anxiety symptoms (GA and SA).
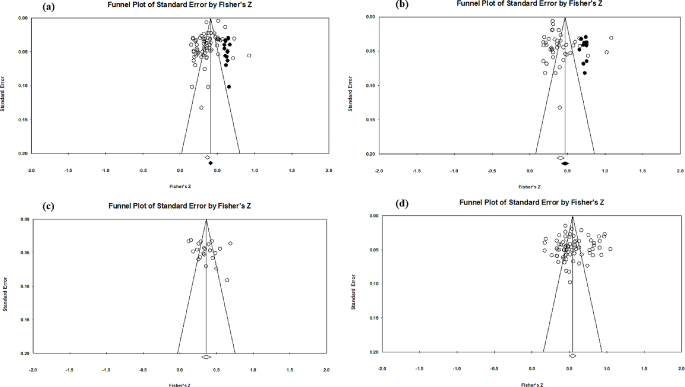
Funnel plot of the relationship between PSNU and anxiety symptoms. Note: Black dots indicated additional studies after using trim and fill method; ( a ) = Funnel plot of the PSNU and GA; ( b ) = Funnel plot of the PSNU and SA; ( c ) = Funnel plot of the PSNU and AA; ( d ) = Funnel plot of the PSNU and FoMO
Sensitivity analyses
Initially, the findings obtained through the one-study-removed approach indicated that the heterogeneities in the relationship between PSNU and anxiety symptoms were not attributed to any individual study. Nevertheless, it is important to note that sensitivity analysis should be performed based on literature quality [ 223 ] since low-quality literature could potentially impact result stability. In the relationship between PSNU and GA, the 10 effect sizes below the theoretical mean scores were excluded from analysis, and the sensitivity analysis results were recalculated ( r = 0.402, 95% CI [0.375, 0.428]); In the relationship between PSNU and SA, the 8 effect sizes below the theoretical mean scores were excluded from analysis, and the sensitivity analysis results were recalculated ( r = 0.431, 95% CI [0.387, 0.472]); In the relationship between PSNU and AA, the 5 effect sizes below the theoretical mean scores were excluded from analysis, and the sensitivity analysis results were recalculated ( r = 0.367, 95% CI [0.298, 0.433]); In the relationship between PSNU and FoMO, the 11 effect sizes below the theoretical mean scores were excluded from analysis, and the sensitivity analysis results were recalculated ( r = 0.508, 95% CI [0.470, 0.544]). The revised estimates indicate that meta-analysis results were stable.
Moderator analysis
The impact of moderator variables on the relation between psnu and ga.
The results of subgroup analysis and meta-regression are shown in Table 4 , the time of measurement significantly moderated the correlation between PSNU and GA ( Q between = 19.268, df = 2, p < 0.001). The relation between the two variables was significantly higher during the COVID-19 ( r = 0.392, 95% CI [0.357, 0.425]) than before the COVID-19 ( r = 0.270, 95% CI [0.227, 0.313]) or measurement time uncertain ( r = 0.352, 95% CI [0.285, 0.415]).
The moderating effect of the PSNU measurement was significant ( Q between = 6.852, df = 1, p = 0.009). The relation was significantly higher when PSNU was measured with the BSMAS ( r = 0.373, 95% CI [0.341, 0.404]) compared to others ( r = 0.301, 95% CI [0.256, 0.344]).
The moderating effect of the GA measurement was significant ( Q between = 60.061, df = 5, p < 0.001). Specifically, when GA measured by the GAD ( r = 0.398, 95% CI [0.356, 0.438]) and the DASS-21-A ( r = 0.433, 95% CI [0.389, 0.475]), a moderate positive correlation was observed. However, the correlation was less significant when measured using the STAI ( r = 0.232, 95% CI [0.187, 0.276]).
For the relation between PSNU and GA, the moderating effect of region, gender and age were not significant.
The impact of moderator variables on the relation between PSNU and SA
The effects of the moderating variables in the relation between PSNU and SA were shown in Table 5 . The results revealed a gender-moderated variances between the two variables (b = 0.601, 95% CI [ 0.041, 1.161], Q model (1, k = 41) = 4.705, p = 0.036).
For the relation between PSNU and SA, the moderating effects of time of measurement, region, measurement of PSNU and SA, and age were not significant.
The impact of moderator variables on the relation between PSNU and AA
The effects of the moderating variables in the relation between PSNU and AA were shown in Table 6 , region significantly moderated the correlation between PSNU and AA ( Q between = 6.410, df = 2, p = 0.041). The correlation between the two variables was significantly higher in developing country ( r = 0.378, 95% CI [0.304, 0.448]) than in developed country ( r = 0.242, 95% CI [0.162, 0.319]).
The moderating effect of the PSNU measurement was significant ( Q between = 6.852, df = 1, p = 0.009). Specifically, when AA was measured by the GPIUS-2 ( r = 0.484, 95% CI [0.200, 0.692]) and the PMSMUAQ ( r = 0.443, 95% CI [0.381, 0.501]), a moderate positive correlation was observed. However, the correlation was less significant when measured using the BSMAS ( r = 0.248, 95% CI [0.161, 0.331]) and others ( r = 0.313, 95% CI [0.250, 0.372]).
The moderating effect of the AA measurement was significant ( Q between = 17.283, df = 2, p < 0.001). The correlation was significantly higher when measured using the ECR ( r = 0.386, 95% CI [0.338, 0.432]) compared to the RQ ( r = 0.200, 95% CI [0.123, 0.275]).
For the relation between PSNU and AA, the moderating effects of time of measurement, region, gender, and age were not significant.
The impact of moderator variables on the relation between PSNU and FoMO
The effects of the moderating variables in the relation between PSNU and FoMO were shown in Table 7 , the moderating effect of the PSNU measurement was significant ( Q between = 8.170, df = 2, p = 0.017). Among the sub-dimensions, the others was excluded because there was only one sample. Specifically, when measured using the FoMOS-MSME ( r = 0.630, 95% CI [0.513, 0.725]), a moderate positive correlation was observed. However, the correlation was less significant when measured using the FoMOS ( r = 0.472, 95% CI [0.432, 0.509]) and the T-S FoMOS ( r = 0.557, 95% CI [0.463, 0.639]).
For the relationship between PSNU and FoMO, the moderating effects of time of measurement, region, measurement of PSNU, gender and age were not significant.
Through systematic review and meta-analysis, this study established a positive correlation between PSNU and anxiety symptoms (i.e., generalized anxiety, social anxiety, attachment anxiety, and fear of missing out), confirming a linear relationship and partially supporting the Social Cognitive Theory of Mass Communication [ 28 ] and the Cognitive Behavioral Model of Pathological Use [ 31 ]. Specifically, a significant positive correlation between PSNU and GA was observed, implying that GA sufferers might resort to social network for validation or as an escape from reality, potentially alleviating their anxiety. Similarly, the meta-analysis demonstrated a strong positive correlation between PSNU and SA, suggesting a preference for computer-mediated communication among those with high social anxiety due to perceived control and liberation offered by social network. This preference is often accompanied by maladaptive emotional regulation, predisposing them to problematic use. In AA, a robust positive correlation was found with PSNU, indicating a higher propensity for such use among individuals with attachment anxiety. Notably, the study identified the strongest correlation in the context of FoMO. FoMO’s significant association with PSNU is multifaceted, stemming from the real-time nature of social networks that engenders a continuous concern about missing crucial updates or events. This drives frequent engagement with social network, thereby establishing a direct link to problematic usage patterns. Additionally, social network’s feedback loops amplify this effect, intensifying FoMO. The culture of social comparison on these platforms further exacerbates FoMO, as users frequently compare their lives with others’ selectively curated portrayals, enhancing both their social networking usage frequency and the pursuit for social validation. Furthermore, the integral role of social network in modern life broadens FoMO’s scope, encompassing anxieties about staying informed and connected.
The notable correlation between FoMO and PSNU can be comprehensively understood through various perspectives. FoMO is inherently linked to the real-time nature of social networks, which cultivates an ongoing concern about missing significant updates or events in one’s social circle [ 221 ]. This anxiety prompts frequent engagement with social network, leading to patterns of problematic use. Moreover, the feedback loops in social network algorithms, designed to enhance user engagement, further intensify this fear [ 224 ]. Additionally, social comparison, a common phenomenon on these platforms, exacerbates FoMO as users continuously compare their lives with the idealized representations of others, amplifying feelings of missing out on key social experiences [ 225 ]. This behavior not only increases social networking usage but also is closely linked to the quest for social validation and identity construction on these platforms. The extensive role of social network in modern life further amplifies FoMO, as these platforms are crucial for information exchange and maintaining social ties. FoMO thus encompasses more than social concerns, extending to anxieties about staying informed with trends and dynamics within social networks [ 226 ]. The multifaceted nature of FoMO in relation to social network underscores its pronounced correlation with problematic social networking usage. In essence, the combination of social network’s intrinsic characteristics, psychological drivers of user behavior, the culture of social comparison, and the pervasiveness of social network in everyday life collectively make FoMO the most pronouncedly correlated anxiety type with PSNU.
Additionally, we conducted subgroup analyses on the timing of measurement (before COVID-19 vs. during COVID-19), measurement tools (for PSNU and anxiety symptoms), sample characteristics (participants’ region), and performed a meta-regression analysis on gender and age in the context of PSNU and anxiety symptoms. It was found that the timing of measurement, tools used for assessing PSNU and anxiety, region, and gender had a moderating effect, whereas age did not show a significant moderating impact.
Firstly, the relationship between PSNU and anxiety symptoms was significantly higher during the COVID-19 period than before, especially between PSNU and GA. However, the moderating effect of measurement timing was not significant in the relationship between PSNU and other types of anxiety. This could be attributed to the increased uncertainty and stress during the pandemic, leading to heightened levels of general anxiety [ 227 ]. The overuse of social network for information seeking and anxiety alleviation might have paradoxically exacerbated anxiety symptoms, particularly among individuals with broad future-related worries [ 228 ]. While the COVID-19 pandemic altered the relationship between PSNU and GA, its impact on other types of anxiety (such as SA and AA) may not have been significant, likely due to these anxiety types being more influenced by other factors like social skills and attachment styles, which were minimally impacted by the epidemic.
Secondly, the observed variance in the relationship between PSNU and AA across different economic contexts, notably between developing and developed countries, underscores the multifaceted influence of socio-economic, cultural, and technological factors on this dynamic. The amplified connection in developing countries may be attributed to greater socio-economic challenges, distinct cultural norms regarding social support and interaction, rising social network penetration, especially among younger demographics, and technological disparities influencing accessibility and user experience [ 229 , 230 ]. Moreover, the role of social network as a coping mechanism for emotional distress, potentially fostering insecure attachment patterns, is more pronounced in these settings [ 231 ]. These findings highlight the necessity of considering contextual variations in assessing the psychological impacts of social network, advocating for a nuanced understanding of how socio-economic and cultural backgrounds mediate the relationship between PSNU and mental health outcomes [ 232 ]. Additionally, the relationship between PSNU and other types of anxiety (such as GA and SA) presents uniform characteristics across different economic contexts.
Thirdly, the significant moderating effects of measurement tools in the context of PSNU and its correlation with various forms of anxiety, including GA, and AA, are crucial in interpreting the research findings. Specifically, the study reveals that the Bergen Social Media Addiction Scale (BSMAS) demonstrates a stronger correlation between PSNU and GA, compared to other tools. Similarly, for AA, the Griffiths’ Problematic Internet Use Scale 2 (GPIUS2) and the Problematic Media Social Media Use Assessment Questionnaire (PMSMUAQ) show a more pronounced correlation with AA than the BSMAS or other instruments, but for SA and FoMO, the PSNU instrument doesn’t significantly moderate the correlation. The PSNU measurement tool typically contains an emotional change dimension. SA and FoMO, due to their specific conditional stimuli triggers and correlation with social networks [ 233 , 234 ], are likely to yield more consistent scores in this dimension, while GA and AA may be less reliable due to their lesser sensitivity to specific conditional stimuli. Consequently, the adjustment effects of PSNU measurements vary across anxiety symptoms. Regarding the measurement tools for anxiety, different scales exhibit varying degrees of sensitivity in detecting the relationship with PSNU. The Generalized Anxiety Disorder Scale (GAD) and the Depression Anxiety Stress Scales 21 (DASS-21) are more effective in illustrating a strong relationship between GA and PSNU than the State-Trait Anxiety Inventory (STAI). In the case of AA, the Experiences in Close Relationships-21 (ECR-21) provides a more substantial correlation than the Relationship Questionnaire (RQ). Furthermore, for FoMO, the Fear of Missing Out Scale - Multi-Social Media Environment (FoMOS-MSME) is more indicative of a strong relationship with PSNU compared to the standard FoMOS or the T-S FoMOS. These findings underscore the importance of the selection of appropriate measurement tools in research. Different tools, due to their unique design, focus, and sensitivity, can reveal varying degrees of correlation between PSNU and anxiety disorders. This highlights the need for careful consideration of tool characteristics and their potential impact on research outcomes. It also cautions against drawing direct comparisons between studies without acknowledging the possible variances introduced by the use of different measurement instruments.
Fourthly, the significant moderating role of gender in the relationship between PSNU and SA, particularly pronounced in samples with a higher proportion of females. Women tend to engage more actively and emotionally with social network, potentially leading to an increased dependency on these platforms when confronting social anxiety [ 235 ]. This intensified use might amplify the association between PSNU and SA. Societal and cultural pressures, especially those related to appearance and social status, are known to disproportionately affect women, possibly exacerbating their experience of social anxiety and prompting a greater reliance on social network for validation and support [ 236 ]. Furthermore, women’s propensity to seek emotional support and express themselves on social network platforms [ 237 ] could strengthen this link, particularly in the context of managing social anxiety. Consequently, the observed gender differences in the relationship between PSNU and SA underscore the importance of considering gender-specific dynamics and cultural influences in psychological research related to social network use. In addition, gender consistency was observed in the association between PSNU and other types of anxiety, indicating no significant gender disparities.
Fifthly, the absence of a significant moderating effect of age on the relationship between PSNU and various forms of anxiety suggests a pervasive influence of social network across different age groups. This finding indicates that the impact of PSNU on anxiety is relatively consistent, irrespective of age, highlighting the universal nature of social network’s psychological implications [ 238 ]. Furthermore, this uniformity suggests that other factors, such as individual psychological traits or socio-cultural influences, might play a more crucial role in the development of anxiety related to social networking usage than age [ 239 ]. The non-significant role of age also points towards a potential generational overlap in social networking usage patterns and their psychological effects, challenging the notion that younger individuals are uniquely susceptible to the adverse effects of social network on mental health [ 240 ]. Therefore, this insight necessitates a broader perspective in understanding the dynamics of social network and mental health, one that transcends age-based assumptions.
Limitations
There are some limitations in this research. First, most of the studies were cross-sectional surveys, resulting in difficulties in inferring causality of variables, longitudinal study data will be needed to evaluate causal interactions in the future. Second, considerable heterogeneity was found in the estimated results, although heterogeneity can be partially explained by differences in study design (e.g., Time of measurement, region, gender, and measurement tools), but this can introduce some uncertainty in the aggregation and generalization of the estimated results. Third, most studies were based on Asian samples, which limits the generality of the results. Fourth, to minimize potential sources of heterogeneity, some less frequently used measurement tools were not included in the classification of measurement tools, which may have some impact on the results of heterogeneity interpretation. Finally, since most of the included studies used self-reported scales, it is possible to get results that deviate from the actual situation to some extent.
This meta-analysis aims to quantifies the correlations between PSNU and four specific types of anxiety symptoms (i.e., generalized anxiety, social anxiety, attachment anxiety, and fear of missing out). The results revealed a significant moderate positive association between PSNU and each of these anxiety symptoms. Furthermore, Subgroup analysis and meta-regression analysis indicated that gender, region, time of measurement, and instrument of measurement significantly influenced the relationship between PSNU and specific anxiety symptoms. Specifically, the measurement time and GA measurement tools significantly influenced the relationship between PSNU and GA. Gender significantly influenced the relationship between PSNU and SA. Region, PSNU measurement tools, and AA measurement tools all significantly influenced the relationship between PSNU and AA. The FoMO measurement tool significantly influenced the relationship between PSNU and FoMO. Regarding these findings, prevention interventions for PSNU and anxiety symptoms are important.
Data availability
The datasets are available from the corresponding author on reasonable request.
Abbreviations
- Problematic social networking use
- Generalized anxiety
- Social anxiety
- Attachment anxiety
Fear of miss out
Bergen Social Media Addiction Scale
Facebook Addiction Scale
Facebook Intrusion Questionnaire
Generalized Problematic Internet Use Scale 2
Problematic Mobile Social Media Usage Assessment Questionnaire
Social Network Addiction Tendency Scale
Brief Symptom Inventory
The anxiety subscale of the Depression Anxiety Stress Scales
Generalized Anxiety Disorder
The anxiety subscale of the Hospital Anxiety and Depression Scale
State-Trait Anxiety Inventory
Interaction Anxiousness Scale
Liebowitz Social Anxiety Scale
Social Anxiety Scale for Social Media Users
Social Anxiety for Adolescents
Social Anxiety Subscale of the Self-Consciousness Scale
Social Interaction Anxiety Scale
Experiences in Close Relationship Scale
Relationship questionnaire
Fear of Missing Out Scale
FoMO Measurement Scale in the Mobile Social Media Environment
Trait-State Fear of missing Out Scale
Rozgonjuk D, Sindermann C, Elhai JD, Montag C. Fear of missing out (FoMO) and social media’s impact on daily-life and productivity at work: do WhatsApp, Facebook, Instagram, and Snapchat Use disorders mediate that association? Addict Behav. 2020;110:106487.
Article PubMed Google Scholar
Mieczkowski H, Lee AY, Hancock JT. Priming effects of social media use scales on well-being outcomes: the influence of intensity and addiction scales on self-reported depression. Social Media + Soc. 2020;6(4):2056305120961784.
Article Google Scholar
Global digital population as of April. 2023 [ https://www.statista.com/statistics/617136/digital-population-worldwide/ ].
Marengo D, Settanni M, Fabris MA, Longobardi C. Alone, together: fear of missing out mediates the link between peer exclusion in WhatsApp classmate groups and psychological adjustment in early-adolescent teens. J Social Personal Relationships. 2021;38(4):1371–9.
Marengo D, Fabris MA, Longobardi C, Settanni M. Smartphone and social media use contributed to individual tendencies towards social media addiction in Italian adolescents during the COVID-19 pandemic. Addict Behav. 2022;126:107204.
Müller SM, Wegmann E, Stolze D, Brand M. Maximizing social outcomes? Social zapping and fear of missing out mediate the effects of maximization and procrastination on problematic social networks use. Comput Hum Behav. 2020;107:106296.
Sun Y, Zhang Y. A review of theories and models applied in studies of social media addiction and implications for future research. Addict Behav. 2021;114:106699.
Boustead R, Flack M. Moderated-mediation analysis of problematic social networking use: the role of anxious attachment orientation, fear of missing out and satisfaction with life. Addict Behav 2021, 119.
Hussain Z, Griffiths MD. The associations between problematic social networking Site Use and Sleep Quality, attention-deficit hyperactivity disorder, Depression, anxiety and stress. Int J Mental Health Addict. 2021;19(3):686–700.
Gori A, Topino E, Griffiths MD. The associations between attachment, self-esteem, fear of missing out, daily time expenditure, and problematic social media use: a path analysis model. Addict Behav. 2023;141:107633.
Marino C, Manari T, Vieno A, Imperato C, Spada MM, Franceschini C, Musetti A. Problematic social networking sites use and online social anxiety: the role of attachment, emotion dysregulation and motives. Addict Behav. 2023;138:107572.
Tobin SJ, Graham S. Feedback sensitivity as a mediator of the relationship between attachment anxiety and problematic Facebook Use. Cyberpsychology Behav Social Netw. 2020;23(8):562–6.
Brailovskaia J, Rohmann E, Bierhoff H-W, Margraf J. The anxious addictive narcissist: the relationship between grandiose and vulnerable narcissism, anxiety symptoms and Facebook Addiction. PLoS ONE 2020, 15(11).
Kim S-S, Bae S-M. Social Anxiety and Social Networking Service Addiction Proneness in University students: the Mediating effects of Experiential Avoidance and interpersonal problems. Psychiatry Invest. 2022;19(8):702–702.
Zhao J, Ye B, Yu L, Xia F. Effects of Stressors of COVID-19 on Chinese College Students’ Problematic Social Media Use: A Mediated Moderation Model. Front Psychiatry 2022, 13.
Astolfi Cury GS, Takannune DM, Prates Herrerias GS, Rivera-Sequeiros A, de Barros JR, Baima JP, Saad-Hossne R, Sassaki LY. Clinical and Psychological Factors Associated with Addiction and Compensatory Use of Facebook among patients with inflammatory bowel disease: a cross-sectional study. Int J Gen Med. 2022;15:1447–57.
Balta S, Emirtekin E, Kircaburun K, Griffiths MD. Neuroticism, trait fear of missing out, and Phubbing: the mediating role of state fear of missing out and problematic Instagram Use. Int J Mental Health Addict. 2020;18(3):628–39.
Boursier V, Gioia F, Griffiths MD. Do selfie-expectancies and social appearance anxiety predict adolescents’ problematic social media use? Comput Hum Behav. 2020;110:106395.
Worsley JD, McIntyre JC, Bentall RP, Corcoran R. Childhood maltreatment and problematic social media use: the role of attachment and depression. Psychiatry Res. 2018;267:88–93.
de Bérail P, Guillon M, Bungener C. The relations between YouTube addiction, social anxiety and parasocial relationships with YouTubers: a moderated-mediation model based on a cognitive-behavioral framework. Comput Hum Behav. 2019;99:190–204.
Naidu S, Chand A, Pandaram A, Patel A. Problematic internet and social network site use in young adults: the role of emotional intelligence and fear of negative evaluation. Pers Indiv Differ. 2023;200:111915.
Apaolaza V, Hartmann P, D’Souza C, Gilsanz A. Mindfulness, compulsive Mobile Social Media Use, and derived stress: the mediating roles of self-esteem and social anxiety. Cyberpsychology Behav Social Netw. 2019;22(6):388–96.
Demircioglu ZI, Goncu-Kose A. Antecedents of problematic social media use and cyberbullying among adolescents: attachment, the dark triad and rejection sensitivity. Curr Psychol (New Brunsw NJ) 2022:1–19.
Gao Q, Li Y, Zhu Z, Fu E, Bu X, Peng S, Xiang Y. What links to psychological needs satisfaction and excessive WeChat use? The mediating role of anxiety, depression and WeChat use intensity. BMC Psychol. 2021;9(1):105–105.
Article PubMed PubMed Central Google Scholar
Malak MZ, Shuhaiber AH, Al-amer RM, Abuadas MH, Aburoomi RJ. Correlation between psychological factors, academic performance and social media addiction: model-based testing. Behav Inform Technol. 2022;41(8):1583–95.
Song C. The effect of the need to belong on mobile phone social media dependence of middle school students: Chain mediating roles of fear of missing out and maladaptive cognition. Sichuan Normal University; 2022.
Tokunaga RS, Rains SA. A review and meta-analysis examining conceptual and operational definitions of problematic internet use. Hum Commun Res. 2016;42(2):165–99.
Bandura A. Social cognitive theory of mass communication. Media effects. edn.: Routledge; 2009. pp. 110–40.
Valkenburg PM, Peter J, Walther JB. Media effects: theory and research. Ann Rev Psychol. 2016;67:315–38.
Slater MD. Reinforcing spirals: the mutual influence of media selectivity and media effects and their impact on individual behavior and social identity. Communication Theory. 2007;17(3):281–303.
Ahmed E, Vaghefi I. Social media addiction: A systematic review through cognitive-behavior model of pathological use. 2021.
She R, han Mo PK, Li J, Liu X, Jiang H, Chen Y, Ma L, fai Lau JT. The double-edged sword effect of social networking use intensity on problematic social networking use among college students: the role of social skills and social anxiety. Comput Hum Behav. 2023;140:107555.
Przybylski AK, Weinstein N. A large-scale test of the goldilocks hypothesis: quantifying the relations between digital-screen use and the mental well-being of adolescents. Psychol Sci. 2017;28(2):204–15.
Ran G, Li J, Zhang Q, Niu X. The association between social anxiety and mobile phone addiction: a three-level meta-analysis. Comput Hum Behav. 2022;130:107198.
Fioravanti G, Casale S, Benucci SB, Prostamo A, Falone A, Ricca V, Rotella F. Fear of missing out and social networking sites use and abuse: a meta-analysis. Comput Hum Behav. 2021;122:106839.
Moher D, Liberati A, Tetzlaff J, Altman DG, Group* P. Preferred reporting items for systematic reviews and meta-analyses: the PRISMA statement. Ann Intern Med. 2009;151(4):264–9.
Card NA. Applied meta-analysis for social science research. Guilford; 2015.
Peterson RA, Brown SP. On the use of beta coefficients in meta-analysis. J Appl Psychol. 2005;90(1):175.
Hunter JE, Schmidt FL. Methods of meta-analysis: correcting error and bias in research findings. Sage; 2004.
Zhang Y, Li S, Yu G. The relationship between self-esteem and social anxiety: a meta-analysis with Chinese students. Adv Psychol Sci. 2019;27(6):1005–18.
Borenstein M, Hedges LV, Higgins JP, Rothstein HR. Introduction to meta-analysis. Wiley; 2021.
Higgins JP, Thompson SG. Quantifying heterogeneity in a meta-analysis. Stat Med. 2002;21(11):1539–58.
Egger M, Smith GD, Schneider M, Minder C. Bias in meta-analysis detected by a simple, graphical test. BMJ. 1997;315(7109):629–34.
Light RJ, Pillemer DB. Summing up: the science of reviewing research. Harvard University Press; 1984.
Rosenthal R. Meta-Analytic Procedures for Social Science Research Sage Publications: Beverly Hills, 1984, 148 pp. Educational Researcher 1986;15(8):18–20.
Rothstein HR, Sutton AJ, Borenstein M. Publication bias in meta-analysis. Publication bias meta‐analysis: Prev Assess Adjustments 2005:1–7.
Duval S, Tweedie R. Trim and fill: a simple funnel-plot–based method of testing and adjusting for publication bias in meta‐analysis. Biometrics. 2000;56(2):455–63.
Al-Mamun F, Hosen I, Griffiths MD, Mamun MA. Facebook use and its predictive factors among students: evidence from a lower- and middle-income country, Bangladesh. Front Psychiatry 2022, 13.
Schou Andreassen C, Billieux J, Griffiths MD, Kuss DJ, Demetrovics Z, Mazzoni E, Pallesen S. The relationship between addictive use of social media and video games and symptoms of psychiatric disorders: a large-scale cross-sectional study. Psychol Addict Behaviors: J Soc Psychologists Addict Behav. 2016;30(2):252–62.
Arikan G, Acar IH, Ustundag-Budak AM. A two-generation study: The transmission of attachment and young adults’ depression, anxiety, and social media addiction. Addict Behav 2022, 124.
Arpaci I, Karatas K, Kiran F, Kusci I, Topcu A. Mediating role of positivity in the relationship between state anxiety and problematic social media use during the COVID-19 pandemic. Death Stud. 2022;46(10):2287–97.
Brailovskaia J, Margraf J. Facebook Addiction Disorder (FAD) among German students-A longitudinal approach. PLoS ONE 2017, 12(12).
Brailovskaia J, Margraf J. The relationship between burden caused by coronavirus (Covid-19), addictive social media use, sense of control and anxiety. Comput Hum Behav. 2021;119:106720–106720.
Brailovskaia J, Margraf J. Addictive social media use during Covid-19 outbreak: validation of the Bergen Social Media Addiction Scale (BSMAS) and investigation of protective factors in nine countries. Curr Psychol (New Brunsw NJ) 2022:1–19.
Brailovskaia J, Krasavtseva Y, Kochetkov Y, Tour P, Margraf J. Social media use, mental health, and suicide-related outcomes in Russian women: a cross-sectional comparison between two age groups. Women’s Health (London England). 2022;18:17455057221141292–17455057221141292.
PubMed Google Scholar
Chang C-W, Huang R-Y, Strong C, Lin Y-C, Tsai M-C, Chen IH, Lin C-Y, Pakpour AHH, Griffiths MDD. Reciprocal relationships between Problematic Social Media Use, problematic gaming, and psychological distress among University students: a 9-Month Longitudinal Study. Front Public Health 2022, 10.
Charzynska E, Sussman S, Atroszko PA. Profiles of potential behavioral addictions’ severity and their associations with gender, personality, and well-being: A person-centered approach. Addict Behav 2021, 119.
Chen C-Y, Chen IH, Pakpour AH, Lin C-Y, Griffiths MD. Internet-related behaviors and psychological distress among Schoolchildren during the COVID-19 School Hiatus. Cyberpsychology Behav Social Netw. 2021;24(10):654–63.
Da Veiga GF, Sotero L, Pontes HM, Cunha D, Portugal A, Relvas AP. Emerging adults and Facebook Use: the validation of the Bergen Facebook Addiction Scale (BFAS). Int J Mental Health Addict. 2019;17(2):279–94.
Dadiotis A, Bacopoulou F, Kokka I, Vlachakis D, Chrousos GP, Darviri C, Roussos P. Validation of the Greek version of the Bergen Social Media Addiction Scale in Undergraduate Students. EMBnetjournal 2021, 26.
Fekih-Romdhane F, Jahrami H, Away R, Trabelsi K, Pandi-Perumal SR, Seeman MV, Hallit S, Cheour M. The relationship between technology addictions and schizotypal traits: mediating roles of depression, anxiety, and stress. BMC Psychiatry 2023, 23(1).
Flynn S, Noone C, Sarma KM. An exploration of the link between adult attachment and problematic Facebook use. BMC Psychol. 2018;6(1):34–34.
Fung XCC, Siu AMH, Potenza MN, O’Brien KS, Latner JD, Chen C-Y, Chen IH, Lin C-Y. Problematic use of internet-related activities and Perceived Weight Stigma in Schoolchildren: a longitudinal study across different epidemic periods of COVID-19 in China. Front Psychiatry 2021, 12.
Gonzalez-Nuevo C, Cuesta M, Muniz J, Postigo A, Menendez-Aller A, Kuss DJ. Problematic Use of Social Networks during the First Lockdown: User Profiles and the Protective Effect of Resilience and Optimism. Journal of Clinical Medicine 2022, 11(24).
Hou X-L, Wang H-Z, Hu T-Q, Gentile DA, Gaskin J, Wang J-L. The relationship between perceived stress and problematic social networking site use among Chinese college students. J Behav Addictions. 2019;8(2):306–17.
Hussain Z, Wegmann E. Problematic social networking site use and associations with anxiety, attention deficit hyperactivity disorder, and resilience. Computers Hum Behav Rep. 2021;4:100125.
Imani V, Ahorsu DK, Taghizadeh N, Parsapour Z, Nejati B, Chen H-P, Pakpour AH. The mediating roles of anxiety, Depression, Sleepiness, Insomnia, and Sleep Quality in the Association between Problematic Social Media Use and Quality of Life among patients with Cancer. Healthcare 2022, 10(9).
Islam MS, Sujan MSH, Tasnim R, Mohona RA, Ferdous MZ, Kamruzzaman S, Toma TY, Sakib MN, Pinky KN, Islam MR et al. Problematic smartphone and Social Media Use among Bangladeshi College and University students amid COVID-19: the role of Psychological Well-Being and Pandemic related factors. Front Psychiatry 2021, 12.
Islam MS, Jahan I, Dewan MAA, Pontes HM, Koly KN, Sikder MT, Rahman M. Psychometric properties of three online-related addictive behavior instruments among Bangladeshi school-going adolescents. PLoS ONE 2022, 17(12).
Jahan I, Hosen I, Al Mamun F, Kaggwa MM, Griffiths MD, Mamun MA. How has the COVID-19 pandemic impacted Internet Use behaviors and facilitated problematic internet use? A Bangladeshi study. Psychol Res Behav Manage. 2021;14:1127–38.
Jiang Y. Problematic social media usage and anxiety among University Students during the COVID-19 pandemic: the mediating role of Psychological Capital and the moderating role of academic burnout. Front Psychol. 2021;12:612007–612007.
Kim M-R, Oh J-W, Huh B-Y. Analysis of factors related to Social Network Service Addiction among Korean High School Students. J Addictions Nurs. 2020;31(3):203–12.
Koc M, Gulyagci S. Facebook addiction among Turkish college students: the role of psychological health, demographic, and usage characteristics. Cyberpsychology Behav Social Netw. 2013;16(4):279–84.
Lin C-Y, Namdar P, Griffiths MD, Pakpour AH. Mediated roles of generalized trust and perceived social support in the effects of problematic social media use on mental health: a cross-sectional study. Health Expect. 2021;24(1):165–73.
Lin C-Y, Imani V, Griffiths MD, Brostrom A, Nygardh A, Demetrovics Z, Pakpour AH. Temporal associations between morningness/eveningness, problematic social media use, psychological distress and daytime sleepiness: mediated roles of sleep quality and insomnia among young adults. J Sleep Res 2021, 30(1).
Lozano Blasco R, Latorre Cosculluela C, Quilez Robres A. Social Network Addiction and its impact on anxiety level among University students. Sustainability 2020, 12(13).
Marino C, Musetti A, Vieno A, Manari T, Franceschini C. Is psychological distress the key factor in the association between problematic social networking sites and poor sleep quality? Addict Behav 2022, 133.
Meshi D, Ellithorpe ME. Problematic social media use and social support received in real-life versus on social media: associations with depression, anxiety and social isolation. Addict Behav 2021, 119.
Mitropoulou EM, Karagianni M, Thomadakis C. Social Media Addiction, Self-Compassion, and Psychological Well-Being: a structural equation Model. Alpha Psychiatry. 2022;23(6):298–304.
Ozimek P, Brailovskaia J, Bierhoff H-W. Active and passive behavior in social media: validating the Social Media Activity Questionnaire (SMAQ). Telematics Inf Rep. 2023;10:100048.
Phillips WJ, Wisniewski AT. Self-compassion moderates the predictive effects of social media use profiles on depression and anxiety. Computers Hum Behav Rep. 2021;4:100128.
Reer F, Festl R, Quandt T. Investigating problematic social media and game use in a nationally representative sample of adolescents and younger adults. Behav Inform Technol. 2021;40(8):776–89.
Satici B, Kayis AR, Griffiths MD. Exploring the Association between Social Media Addiction and relationship satisfaction: psychological distress as a Mediator. Int J Mental Health Addict 2021.
Sediri S, Zgueb Y, Ouanes S, Ouali U, Bourgou S, Jomli R, Nacef F. Women’s mental health: acute impact of COVID-19 pandemic on domestic violence. Archives Womens Mental Health. 2020;23(6):749–56.
Shabahang R, Shim H, Aruguete MS, Zsila A. Oversharing on Social Media: anxiety, Attention-Seeking, and Social Media Addiction Predict the breadth and depth of sharing. Psychol Rep 2022:332941221122861–332941221122861.
Sotero L, Ferreira Da Veiga G, Carreira D, Portugal A, Relvas AP. Facebook Addiction and emerging adults: the influence of sociodemographic variables, family communication, and differentiation of self. Escritos De Psicología - Psychol Writings. 2019;12(2):81–92.
Stockdale LA, Coyne SM. Bored and online: reasons for using social media, problematic social networking site use, and behavioral outcomes across the transition from adolescence to emerging adulthood. J Adolesc. 2020;79:173–83.
Wang Z, Yang H, Elhai JD. Are there gender differences in comorbidity symptoms networks of problematic social media use, anxiety and depression symptoms? Evidence from network analysis. Pers Indiv Differ. 2022;195:111705.
White-Gosselin C-E, Poulin F. Associations Between Young Adults’ Social Media Addiction, Relationship Quality With Parents, and Internalizing Problems: A Path Analysis Model. 2022.
Wong HY, Mo HY, Potenza MN, Chan MNM, Lau WM, Chui TK, Pakpour AH, Lin C-Y. Relationships between Severity of Internet Gaming Disorder, Severity of Problematic Social Media Use, Sleep Quality and Psychological Distress. Int J Environ Res Public Health 2020, 17(6).
Yam C-W, Pakpour AH, Griffiths MD, Yau W-Y, Lo C-LM, Ng JMT, Lin C-Y, Leung H. Psychometric testing of three Chinese online-related addictive Behavior instruments among Hong Kong University students. Psychiatr Q. 2019;90(1):117–28.
Yuan Y, Zhong Y. A survey on the use of social networks and mental health of college students during the COVID-19 pandemic. J Campus Life Mental Health\. 2021;19(3):209–12.
Google Scholar
Yurdagul C, Kircaburun K, Emirtekin E, Wang P, Griffiths MD. Psychopathological consequences related to problematic Instagram Use among adolescents: the mediating role of body image dissatisfaction and moderating role of gender. Int J Mental Health Addict. 2021;19(5):1385–97.
Zhang W, Pu J, He R, Yu M, Xu L, He X, Chen Z, Gan Z, Liu K, Tan Y, et al. Demographic characteristics, family environment and psychosocial factors affecting internet addiction in Chinese adolescents. J Affect Disord. 2022;315:130–8.
Zhang L, Wu Y, Jin T, Jia Y. Revision and validation of the Chinese short version of social media disorder. Mod Prev Med. 2021;48(8):1350–3.
Zhang X, Fan L. The influence of anxiety on colleges’ life satisfaction. Chin J Health Educ. 2021;37(5):469–72.
Zhao M, Wang H, Dong Y, Niu Y, Fang Y. The relationship between self-esteem and wechat addiction among undergraduate students: the multiple mediating roles of state anxiety and online interpersonal trust. J Psychol Sci. 2021;44(1):104–10.
Zhao J, Zhou Z, Sun B, Zhang X, Zhang L, Fu S. Attentional Bias is Associated with negative emotions in problematic users of Social Media as measured by a dot-probe Task. Int J Environ Res Public Health 2022, 19(24).
Atroszko PA, Balcerowska JM, Bereznowski P, Biernatowska A, Pallesen S, Schou Andreassen C. Facebook addiction among Polish undergraduate students: validity of measurement and relationship with personality and well-being. Comput Hum Behav. 2018;85:329–38.
Chen Y, Li R, Zhang P, Liu X. The moderating role of state attachment anxiety and avoidance between social anxiety and social networking sites Addiction. Psychol Rep. 2020;123(3):633–47.
Chen B, Zheng X, Sun X. The relationship between problematic social media use and online social anxiety: the roles of social media cognitive overload and dispositional mindfulness. Psychol Dev Educ. 2023;39(5):743–51.
Chentsova VO, Bravo AJ, Mezquita L, Pilatti A, Hogarth L, Cross-Cultural AS. Internalizing symptoms, rumination, and problematic social networking site use: a cross national examination among young adults in seven countries. Addict Behav 2023, 136.
Chu X, Ji S, Wang X, Yu J, Chen Y, Lei L. Peer phubbing and social networking site addiction: the mediating role of social anxiety and the moderating role of Family Financial Difficulty. Front Psychol. 2021;12:670065–670065.
Dempsey AE, O’Brien KD, Tiamiyu MF, Elhai JD. Fear of missing out (FoMO) and rumination mediate relations between social anxiety and problematic Facebook use. Addict Behav Rep. 2019;9:100150–100150.
PubMed PubMed Central Google Scholar
Yildiz Durak H, Seferoglu SS. Modeling of variables related to problematic social media usage: Social desirability tendency example. Scand J Psychol. 2019;60(3):277–88.
Ekinci N, Akat M. The relationship between anxious-ambivalent attachment and social appearance anxiety in adolescents: the serial mediation of positive Youth Development and Instagram Addiction. Psychol Rep 2023:332941231159600–332941231159600.
Foroughi B, Griffiths MD, Iranmanesh M, Salamzadeh Y. Associations between Instagram Addiction, academic performance, social anxiety, Depression, and life satisfaction among University students. Int J Mental Health Addict. 2022;20(4):2221–42.
He L. Influence mechanism and intervention suggestions on addiction of social network addiction. Gannan Normal University; 2021.
Hu Y. The influencing mechanism of type D personality on problematic social networking sites use among adolescents and intervention research. Central China Normal University; 2020.
Jia L. A study of the relationship between neuroticism, perceived social support, social anxiety and problematic social network use in high school students. Harbin Normal University; 2022.
Lee-Won RJ, Herzog L, Park SG. Hooked on Facebook: the role of social anxiety and need for Social Assurance in Problematic Use of Facebook. Cyberpsychology Behav Social Netw. 2015;18(10):567–74.
Li H. Social anxiety and internet interpersonal addiction in adolescents and countermeasures. Central China Normal University; 2022.
Lin W-S, Chen H-R, Lee TS-H, Feng JY. Role of social anxiety on high engagement and addictive behavior in the context of social networking sites. Data Technol Appl. 2019;53(2):156–70.
Liu Y. The influence of family function on social media addiction in adolescents: the chain mediation effect of social anxiety and resilience. Hunan Normal University; 2021.
Lyvers M, Salviani A, Costan S, Thorberg FA. Alexithymia, narcissism and social anxiety in relation to social media and internet addiction symptoms. Int J Psychology: J Int De Psychologie. 2022;57(5):606–12.
Majid A, Yasir M, Javed A, Ali P. From envy to social anxiety and rumination: how social media site addiction triggers task distraction amongst nurses. J Nurs Adm Manag. 2020;28(3):504–13.
Mou Q, Zhuang J, Gao Y, Zhong Y, Lu Q, Gao F, Zhao M. The relationship between social anxiety and academic engagement among Chinese college students: a serial mediation model. J Affect Disord. 2022;311:247–53.
Ruggieri S, Santoro G, Pace U, Passanisi A, Schimmenti A. Problematic Facebook use and anxiety concerning use of social media in mothers and their offspring: an actor-partner interdependence model. Addict Behav Rep. 2020;11:100256–100256.
Ruiz MJ, Saez G, Villanueva-Moya L, Exposito F. Adolescent sexting: the role of body shame, Social Physique anxiety, and social networking site addiction. Cyberpsychology Behav Social Netw. 2021;24(12):799–805.
She R, Kit Han Mo P, Li J, Liu X, Jiang H, Chen Y, Ma L, Tak Fai Lau J. The double-edged sword effect of social networking use intensity on problematic social networking use among college students: the role of social skills and social anxiety. Comput Hum Behav. 2023;140:107555.
Stănculescu E. The Bergen Social Media Addiction Scale Validity in a Romanian sample using item response theory and network analysis. Int J Mental Health Addict 2022.
Teng X, Lei H, Li J, Wen Z. The influence of social anxiety on social network site addiction of college students: the moderator of intentional self-regulation. Chin J Clin Psychol. 2021;29(3):514–7.
Tong W. Influence of boredom on the problematic mobile social networks usage in adolescents: multiple chain mediator. Chin J Clin Psychol. 2019;27(5):932–6.
Tu W, Jiang H, Liu Q. Peer victimization and adolescent Mobile Social Addiction: mediation of social anxiety and gender differences. Int J Environ Res Public Health 2022, 19(17).
Wang S. The influence of college students self-esteem, social anxiety and fear of missing out on the problematic mobile social networks usage. Huaibei Normal University; 2021.
Wang X. The impact of peer relationship and social anxiety on secondary vocational school students’ problematic social network use and intervention study. Huaibei Normal University; 2022.
Wegmann E, Stodt B, Brand M. Addictive use of social networking sites can be explained by the interaction of internet use expectancies, internet literacy, and psychopathological symptoms. J Behav Addictions. 2015;4(3):155–62.
Yang W. The relationship between the type of internet addiction and the personality traits in college students. Huazhong University of Science and Technology; 2004.
Yang Z. The relationship between social variables and social networking usage among shanghai working population. East China Normal University; 2013.
Zhang C. The relationship between perceived social support and problematic social network use among junior high school students: a chain mediation model and an intervention study. Hebei University; 2022.
Zhang J, Chang F, Huang D, Wen X. The relationship between neuroticism and the problematic mobile social networks use in adolescents: the mediating role of anxiety and positive self-presentation. Chin J Clin Psychol. 2021;29(3):598–602.
Zhang Z. College students’ loneliness and problematic social networking use: Chain mediation of social self-efficacy and social anxiety. Shanghai Normal University; 2019.
Zhu B. Discussion on mechanism of social networking addiction——Social anxiety, craving and excitability. Liaoning Normal University; 2017.
Blackwell D, Leaman C, Tramposch R, Osborne C, Liss M. Extraversion, neuroticism, attachment style and fear of missing out as predictors of social media use and addiction. Pers Indiv Differ. 2017;116:69–72.
Chen A. From attachment to addiction: the mediating role of need satisfaction on social networking sites. Comput Hum Behav. 2019;98:80–92.
Chen Y, Zhong S, Dai L, Deng Y, Liu X. Attachment anxiety and social networking sites addiction in college students: a moderated mediating model. Chin J Clin Psychol. 2019;27(3):497–500.
Li J. The relations among problematic social networks usage behavior, Childhood Trauma and adult attachment in University students. Hunan Agricultural University; 2020.
Liu C, Ma J-L. Adult attachment orientations and social networking site addiction: the Mediating effects of Online Social Support and the fear of missing out. Front Psychol. 2019;10:2629–2629.
Mo S, Huang W, Xu Y, Tang Z, Nie G. The impact of medical students’ attachment anxiety on the use of problematic social networking sites during the epidemic. Psychol Monthly. 2022;17(9):1–4.
Teng X. The effect of attachment anxiety on problematic mobile social network use: the role of loneliness and self-control. Harbin Normal University; 2021.
Worsley JD, Mansfield R, Corcoran R. Attachment anxiety and problematic social media use: the Mediating Role of Well-Being. Cyberpsychology Behav Social Netw. 2018;21(9):563–8.
Wu Z. The effect of adult attachment on problematic social network use: the chain mediating effect of loneliness and fear of missing out. Jilin University; 2022.
Xia N. The impact of attachment anxiety on adolescent problem social networking site use: a moderated mediation model. Shihezi University; 2022.
Young L, Kolubinski DC, Frings D. Attachment style moderates the relationship between social media use and user mental health and wellbeing. Heliyon 2020, 6(6).
Bakioglu F, Deniz M, Griffiths MD, Pakpour AH. Adaptation and validation of the online-fear of missing out inventory into Turkish and the association with social media addiction, smartphone addiction, and life satisfaction. BMC Psychol. 2022;10(1):154–154.
Bendayan R, Blanca MJ. Spanish version of the Facebook Intrusion Questionnaire (FIQ-S). Psicothema. 2019;31(2):204–9.
Blachnio A, Przepiorka A. Facebook intrusion, fear of missing out, narcissism, and life satisfaction: a cross-sectional study. Psychiatry Res. 2018;259:514–9.
Casale S, Rugai L, Fioravanti G. Exploring the role of positive metacognitions in explaining the association between the fear of missing out and social media addiction. Addict Behav. 2018;85:83–7.
Chen Y, Zhang Y, Zhang S, Wang K. Effect of fear of’ missing out on college students negative social adaptation: Chain¬ - mediating effect of rumination and problematic social media use. China J Health Psychol. 2022;30(4):581–6.
Cheng S, Zhang X, Han Y. Relationship between fear of missing out and phubbing on college students: the chain intermediary effect of intolerance of uncertainty and problematic social media use. China J Health Psychol. 2022;30(9):1296–300.
Cui Q, Wang J, Zhang J, Li W, Li Q. The relationship between loneliness and negative emotion in college students: the chain-mediating role of fear of missing out and social network sites addiction. J Jining Med Univ. 2022;45(4):248–51.
Ding Q, Wang Z, Zhang Y, Zhou Z. The more gossip, the more addicted: the relationship between interpersonal curiosity and social networking sites addiction tendencies in college students. Psychol Dev Educ. 2022;38(1):118–25.
Fabris MA, Marengo D, Longobardi C, Settanni M. Investigating the links between fear of missing out, social media addiction, and emotional symptoms in adolescence: the role of stress associated with neglect and negative reactions on social media. Addict Behav. 2020;106:106364.
Fang J, Wang X, Wen Z, Zhou J. Fear of missing out and problematic social media use as mediators between emotional support from social media and phubbing behavior. Addict Behav. 2020;107:106430.
Gao Z. The study on the relationship and intervention among fear of missing out self-differentiation and problematic social media use of college students. Yunnan Normal University; 2021.
Gioia F, Fioravanti G, Casale S, Boursier V. The Effects of the Fear of Missing Out on People’s Social Networking Sites Use During the COVID-19 Pandemic: The Mediating Role of Online Relational Closeness and Individuals’ Online Communication Attitude. Front Psychiatry 2021, 12.
Gu X. Study on the Inhibitory Effect of Mindfulness Training on Social Media Addiction of College Students. Wuhan University; 2020.
Gugushvili N, Taht K, Schruff-Lim EM, Ruiter RA, Verduyn P. The Association between Neuroticism and problematic social networking sites Use: the role of fear of missing out and Self-Control. Psychol Rep 2022:332941221142003–332941221142003.
Hou J. The study on FoMO and content social media addiction among young people. Huazhong University of Science and Technology; 2021.
Hu R, Zhang B, Yang Y, Mao H, Peng Y, Xiong S. Relationship between college students’ fear of missing and wechat addiction: a cross-lagged analysis. J Bio-education. 2022;10(5):369–73.
Hu G. The relationship between basic psychological needs satisfaction and the use of problematic social networks by college students: a moderated mediation model and online intervention studies. Jiangxi Normal University; 2020.
Jiang Y, Jin T. The relationship between adolescents’ narcissistic personality and their use of problematic mobile social networks: the effects of fear of missing out and positive self-presentation. Chin J Special Educ 2018(11):64–70.
Li J. The effect of positive self-presentation on social networking sites on problematic use of social networking sites: a moderated mediation model. Henan University; 2020.
Li J, Zhang Y, Zhang X. The impact of Freshmen Social Exclusion on problematic Social Network Use: a Moderated Mediation Model. J Heilongjiang Vocat Inst Ecol Eng. 2023;36(1):118–22.
Li M. The relationship between fear of missing out and social media addiction among middle school students——The moderating role of self-control. Kashi University; 2022.
Li R, Dong X, Wang M, Wang R. A study on the relationship between fear of missing out and social network addiction. New Educ Era 2021(52):122–3.
Li Y. Fear of missing out or social avoidance? The influence of peer exclusion on problematic social media use among adolescents in Guangdong Province and Macao. Guangzhou University; 2020.
Ma J, Liu C. The effect of fear of missing out on social networking sites addiction among college students: the mediating roles of social networking site integration use and social support. Psychol Dev Educ. 2019;35(5):605–14.
Mao H. A follow-up study on the mechanism of the influence of university students’ Qi deficiency quality on WeChat addiction. Hunan University of Chinese Medicine; 2021.
Mao Y. The effect of dual filial piety to the college students ’internet social dependence: the mediation of maladaptive cognition and fear of missing out. Huazhong University of Science and Technology; 2021.
Moore K, Craciun G. Fear of missing out and personality as predictors of Social networking sites usage: the Instagram Case. Psychol Rep. 2021;124(4):1761–87.
Niu J. The relationship of college students’ basic psychological needs and social media dependence: the mediating role of fear of missing out. Huazhong University of Science and Technology; 2021.
Pi L, Li X. Research on the relationship between loneliness and problematic mobile social media usage: evidence from variable-oriented and person-oriented analyses. China J Health Psychol. 2023;31(6):936–42.
Pontes HM, Taylor M, Stavropoulos V. Beyond Facebook Addiction: the role of cognitive-related factors and Psychiatric Distress in Social networking site addiction. Cyberpsychol Behav Soc Netw. 2018;21(4):240–7.
Quaglieri A, Biondi S, Roma P, Varchetta M, Fraschetti A, Burrai J, Lausi G, Marti-Vilar M, Gonzalez-Sala F, Di Domenico A et al. From Emotional (Dys) Regulation to Internet Addiction: A Mediation Model of Problematic Social Media Use among Italian Young Adults. Journal of Clinical Medicine 2022, 11(1).
Servidio R, Koronczai B, Griffiths MD, Demetrovics Z. Problematic smartphone Use and Problematic Social Media Use: the predictive role of Self-Construal and the Mediating Effect of Fear Missing Out. Front Public Health 2022, 10.
Sheldon P, Antony MG, Sykes B. Predictors of problematic social media use: personality and life-position indicators. Psychol Rep. 2021;124(3):1110–33.
Sun C, Li Y, Kwok SYCL, Mu W. The relationship between intolerance of uncertainty and problematic Social Media Use during the COVID-19 pandemic: a serial mediation model. Int J Environ Res Public Health 2022, 19(22).
Tang Z. The relationship between loneliness and problematic social networks use among college students: the mediation of fear of missing out and the moderation of social support. Jilin University; 2022.
Tomczyk Ł, Selmanagic-Lizde E. Fear of missing out (FOMO) among youth in Bosnia and Herzegovina — Scale and selected mechanisms. Child Youth Serv Rev. 2018;88:541–9.
Unal-Aydin P, Ozkan Y, Ozturk M, Aydin O, Spada MM. The role of metacognitions in cyberbullying and cybervictimization among adolescents diagnosed with major depressive disorder and anxiety disorders: a case-control study. Clinical Psychology & Psychotherapy; 2023.
Uram P, Skalski S. Still logged in? The Link between Facebook Addiction, FoMO, Self-Esteem, Life satisfaction and loneliness in social media users. Psychol Rep. 2022;125(1):218–31.
Varchetta M, Fraschetti A, Mari E, Giannini AM. Social Media Addiction, fear of missing out (FoMO) and online vulnerability in university students. Revista Digit De Investigación en Docencia Universitaria. 2020;14(1):e1187.
Wang H. Study on the relationship and intervention between fear of missing and social network addiction in college students. Yunnan Normal University; 2021.
Wang M, Yin Z, Xu Q, Niu G. The relationship between shyness and adolescents’ social network sites addiction: Moderated mediation model. Chin J Clin Psychol. 2020;28(5):906–9.
Wegmann E, Oberst U, Stodt B, Brand M. Online-specific fear of missing out and internet-use expectancies contribute to symptoms of internet-communication disorder. Addict Behav Rep. 2017;5:33–42.
Wegmann E, Brandtner A, Brand M. Perceived strain due to COVID-19-Related restrictions mediates the Effect of Social needs and fear of missing out on the risk of a problematic use of Social Networks. Front Psychiatry 2021, 12.
Wei Q. Negative emotions and problematic social network sites usage: the mediating role of fear of missing out and the moderating role of gender. Central China Normal University; 2018.
Xiong L. Effect of social network site use on college students’ social network site addiction: A moderated mediation model and attention bias training intervention study. Jiangxi Normal University; 2022.
Yan H. The influence of college students’ basic psychological needs on social network addiction: The intermediary role of fear of missing out. Wuhan University; 2020.
Yan H. The status and factors associated with social media addiction among young people——Evidence from WeChat. Chongqing University; 2021.
Yang L. Research on the relationship of fear of missing out, excessive use of Wechat and life satisfaction. Beijing Forestry University; 2020.
Yin Y, Cai X, Ouyang M, Li S, Li X, Wang P. FoMO and the brain: loneliness and problematic social networking site use mediate the association between the topology of the resting-state EEG brain network and fear of missing out. Comput Hum Behav. 2023;141:107624.
Zhang C. The parental rejection and problematic social network sites with adolescents: the chain mediating effect of basic psychological needs and fear of missing out. Central China Normal University; 2022.
Zhang J. The influence of basic psychological needs on problematic mobile social networks usage of adolescent: a moderated mediation model. Liaocheng University; 2020.
Zhang Y, Chen Y, Jin J, Yu G. The relationship between fear of missing out and social media addiction: a cross-lagged analysis. Chin J Clin Psychol. 2021;29(5):1082–5.
Zhang Y, Jiang W, Ding Q, Hong M. Social comparison orientation and social network sites addiction in college students: the mediating role of fear of missing out. Chin J Clin Psychol. 2019;27(5):928–31.
Zhou J, Fang J. Social network sites support and addiction among college students: a moderated mediation model. Psychology: Techniques Appl. 2021;9(5):293–9.
Andreassen CS, Torsheim T, Brunborg GS, Pallesen S. Development of a Facebook addiction scale. Psychol Rep. 2012;110(2):501–17.
Andreassen CS, Billieux J, Griffiths MD, Kuss DJ, Demetrovics Z, Mazzoni E, Pallesen S. The relationship between addictive use of social media and video games and symptoms of psychiatric disorders: a large-scale cross-sectional study. Psychol Addict Behav. 2016;30(2):252.
Elphinston RA, Noller P. Time to face it! Facebook intrusion and the implications for romantic jealousy and relationship satisfaction. Cyberpsychology Behav Social Netw. 2011;14(11):631–5.
Caplan SE. Theory and measurement of generalized problematic internet use: a two-step approach. Comput Hum Behav. 2010;26(5):1089–97.
Jiang Y. Development of problematic mobile social media usage assessment questionnaire for adolescents. Psychology: Techniques Appl. 2018;6(10):613–21.
Wang X. College students’ social network addiction tendency: Questionnaire construction and correlation research. Master’s thesis Southwest University; 2016.
Derogatis LR. Brief symptom inventory 18. Johns Hopkins University Baltimore; 2001.
Lovibond PF, Lovibond SH. The structure of negative emotional states: comparison of the Depression anxiety stress scales (DASS) with the Beck Depression and anxiety inventories. Behav Res Ther. 1995;33(3):335–43.
Spitzer RL, Kroenke K, Williams JB, Löwe B. A brief measure for assessing generalized anxiety disorder: the GAD-7. Arch Intern Med. 2006;166(10):1092–7.
Zigmond AS, Snaith RP. The hospital anxiety and depression scale. Acta Psychiatrica Scandinavica. 1983;67(6):361–70.
Spielberger CD, Gonzalez-Reigosa F, Martinez-Urrutia A, Natalicio LF, Natalicio DS. The state-trait anxiety inventory. Revista Interamericana de Psicologia/Interamerican Journal of Psychology 1971, 5(3&4).
Marteau TM, Bekker H. The development of a six-item short‐form of the state scale of the Spielberger State—trait anxiety inventory (STAI). Br J Clin Psychol. 1992;31(3):301–6.
Leary MR. Social anxiousness: the construct and its measurement. J Pers Assess. 1983;47(1):66–75.
Liebowitz MR. Social phobia. Modern problems of pharmacopsychiatry 1987.
Alkis Y, Kadirhan Z, Sat M. Development and validation of social anxiety scale for social media users. Comput Hum Behav. 2017;72:296–303.
La Greca AM, Stone WL. Social anxiety scale for children-revised: factor structure and concurrent validity. J Clin Child Psychol. 1993;22(1):17–27.
Fenigstein A, Scheier MF, Buss AH. Public and private self-consciousness: Assessment and theory. J Consult Clin Psychol. 1975;43(4):522.
Mattick RP, Clarke JC. Development and validation of measures of social phobia scrutiny fear and social interaction anxiety. Behav Res Ther. 1998;36(4):455–70.
Peters L, Sunderland M, Andrews G, Rapee RM, Mattick RP. Development of a short form Social Interaction anxiety (SIAS) and Social Phobia Scale (SPS) using nonparametric item response theory: the SIAS-6 and the SPS-6. Psychol Assess. 2012;24(1):66.
Brennan KA, Clark CL, Shaver PR. Self-report measurement of adult attachment: an integrative overview. Attachment Theory Close Relationships. 1998;46:76.
Wei M, Russell DW, Mallinckrodt B, Vogel DL. The experiences in Close Relationship Scale (ECR)-short form: reliability, validity, and factor structure. J Pers Assess. 2007;88(2):187–204.
Bartholomew K, Horowitz LM. Attachment styles among young adults: a test of a four-category model. J Personal Soc Psychol. 1991;61(2):226.
Przybylski AK, Murayama K, DeHaan CR, Gladwell V. Motivational, emotional, and behavioral correlates of fear of missing out. Comput Hum Behav. 2013;29(4):1841–8.
Xiaokang S, Yuxiang Z, Xuanhui Z. Developing a fear of missing out (FoMO) measurement scale in the mobile social media environment. Libr Inform Service. 2017;61(11):96.
Bown M, Sutton A. Quality control in systematic reviews and meta-analyses. Eur J Vasc Endovasc Surg. 2010;40(5):669–77.
Turel O, Qahri-Saremi H. Problematic use of social networking sites: antecedents and consequence from a dual-system theory perspective. J Manage Inform Syst. 2016;33(4):1087–116.
Chou H-TG, Edge N. They are happier and having better lives than I am: the impact of using Facebook on perceptions of others’ lives. Cyberpsychology Behav Social Netw. 2012;15(2):117–21.
Beyens I, Frison E, Eggermont S. I don’t want to miss a thing: adolescents’ fear of missing out and its relationship to adolescents’ social needs, Facebook use, and Facebook related stress. Comput Hum Behav. 2016;64:1–8.
Di Blasi M, Gullo S, Mancinelli E, Freda MF, Esposito G, Gelo OCG, Lagetto G, Giordano C, Mazzeschi C, Pazzagli C. Psychological distress associated with the COVID-19 lockdown: a two-wave network analysis. J Affect Disord. 2021;284:18–26.
Yang X, Hu H, Zhao C, Xu H, Tu X, Zhang G. A longitudinal study of changes in smart phone addiction and depressive symptoms and potential risk factors among Chinese college students. BMC Psychiatry. 2021;21(1):252.
Kuss DJ, Griffiths MD. Social networking sites and addiction: ten lessons learned. Int J Environ Res Public Health. 2017;14(3):311.
Ryan T, Chester A, Reece J, Xenos S. The uses and abuses of Facebook: a review of Facebook addiction. J Behav Addictions. 2014;3(3):133–48.
Elhai JD, Levine JC, Dvorak RD, Hall BJ. Non-social features of smartphone use are most related to depression, anxiety and problematic smartphone use. Comput Hum Behav. 2017;69:75–82.
Jackson LA, Wang J-L. Cultural differences in social networking site use: a comparative study of China and the United States. Comput Hum Behav. 2013;29(3):910–21.
Ahrens LM, Mühlberger A, Pauli P, Wieser MJ. Impaired visuocortical discrimination learning of socially conditioned stimuli in social anxiety. Soc Cognit Affect Neurosci. 2014;10(7):929–37.
Elhai JD, Yang H, Montag C. Fear of missing out (FOMO): overview, theoretical underpinnings, and literature review on relations with severity of negative affectivity and problematic technology use. Brazilian J Psychiatry. 2020;43:203–9.
Barker V. Older adolescents’ motivations for social network site use: the influence of gender, group identity, and collective self-esteem. Cyberpsychology Behav. 2009;12(2):209–13.
Krasnova H, Veltri NF, Eling N, Buxmann P. Why men and women continue to use social networking sites: the role of gender differences. J Strateg Inf Syst. 2017;26(4):261–84.
Palmer J. The role of gender on social network websites. Stylus Knights Write Showc 2012:35–46.
Vannucci A, Flannery KM, Ohannessian CM. Social media use and anxiety in emerging adults. J Affect Disord. 2017;207:163–6.
Primack BA, Shensa A, Sidani JE, Whaite EO, yi Lin L, Rosen D, Colditz JB, Radovic A, Miller E. Social media use and perceived social isolation among young adults in the US. Am J Prev Med. 2017;53(1):1–8.
Twenge JM, Campbell WK. Associations between screen time and lower psychological well-being among children and adolescents: evidence from a population-based study. Prev Med Rep. 2018;12:271–83.
Download references
This research was supported by the Social Science Foundation of China (Grant Number: 23BSH135).
Author information
Authors and affiliations.
School of Mental Health, Wenzhou Medical University, 325035, Wenzhou, China
Mingxuan Du, Haiyan Hu, Ningning Ding, Jiankang He, Wenwen Tian, Wenqian Zhao, Xiujian Lin, Gaoyang Liu, Wendan Chen, ShuangLiu Wang, Dongwu Xu & Guohua Zhang
School of Education, Renmin University of China, 100872, Beijing, China
Chengjia Zhao
School of Media and Communication, Shanghai Jiao Tong University, Dongchuan Road 800, 200240, Shanghai, China
Pengcheng Wang
Department of Neurosis and Psychosomatic Diseases, Huzhou Third Municipal Hospital, 313002, Huzhou, China
Xinhua Shen
You can also search for this author in PubMed Google Scholar
Contributions
GZ, XS, XL and MD prepared the study design, writing - review and editing. MD and CZ wrote the main manuscript text. MD and HH analyzed data and edited the draft. ND, JH, WT, WZ, GL, WC, SW, PW and DX conducted resources and data curation. All authors have approved the final version of the manuscript.
Corresponding authors
Correspondence to Xinhua Shen or Guohua Zhang .
Ethics declarations
Ethics approval and consent to participate.
Not applicable.
Consent for publication
Competing interests.
The authors declare no competing interests.
Additional information
Publisher’s note.
Springer Nature remains neutral with regard to jurisdictional claims in published maps and institutional affiliations.
Rights and permissions
Open Access This article is licensed under a Creative Commons Attribution 4.0 International License, which permits use, sharing, adaptation, distribution and reproduction in any medium or format, as long as you give appropriate credit to the original author(s) and the source, provide a link to the Creative Commons licence, and indicate if changes were made. The images or other third party material in this article are included in the article’s Creative Commons licence, unless indicated otherwise in a credit line to the material. If material is not included in the article’s Creative Commons licence and your intended use is not permitted by statutory regulation or exceeds the permitted use, you will need to obtain permission directly from the copyright holder. To view a copy of this licence, visit http://creativecommons.org/licenses/by/4.0/ . The Creative Commons Public Domain Dedication waiver ( http://creativecommons.org/publicdomain/zero/1.0/ ) applies to the data made available in this article, unless otherwise stated in a credit line to the data.
Reprints and permissions
About this article
Cite this article.
Du, M., Zhao, C., Hu, H. et al. Association between problematic social networking use and anxiety symptoms: a systematic review and meta-analysis. BMC Psychol 12 , 263 (2024). https://doi.org/10.1186/s40359-024-01705-w
Download citation
Received : 25 January 2024
Accepted : 03 April 2024
Published : 12 May 2024
DOI : https://doi.org/10.1186/s40359-024-01705-w
Share this article
Anyone you share the following link with will be able to read this content:
Sorry, a shareable link is not currently available for this article.
Provided by the Springer Nature SharedIt content-sharing initiative
- Fear of missing out
- Meta-analysis
BMC Psychology
ISSN: 2050-7283
- General enquiries: [email protected]
Log in using your username and password
- Search More Search for this keyword Advanced search
- Latest content
- Current issue
- BMJ Journals More You are viewing from: Google Indexer
You are here
- Online First
- Socioeconomic gradients in 24-hour movement patterns across weekends and weekdays in a working-age sample: evidence from the 1970 British Cohort Study
- Article Text
- Article info
- Citation Tools
- Rapid Responses
- Article metrics

- http://orcid.org/0000-0001-7684-3571 Joanna M Blodgett 1 , 2 ,
- http://orcid.org/0000-0002-6454-626X David Bann 3 ,
- http://orcid.org/0000-0003-1421-9348 Sebastien F M Chastin 4 ,
- Matthew Ahmadi 5 , 6 ,
- Emmanuel Stamatakis 5 , 6 ,
- http://orcid.org/0000-0003-3370-5720 Rachel Cooper 7 , 8 ,
- Mark Hamer 1 , 2
- 1 Institute of Sport, Exercise and Health , UCL , London , UK
- 2 NIHR University College London Hospitals Biomedical Research Centre , London , England , UK
- 3 Centre for Longitudinal Studies, Social Research Institute , UCL , London , UK
- 4 School of Health and Life Sciences , Glasgow Caledonian University , Glasgow , UK
- 5 Mackenzie Wearables Research Hub, Charles Perkins Centre, Faculty of Medicine and Health, The University of Sydney , Sydney , New South Wales , Australia
- 6 School of Health Sciences, Faculty of Medicine and Health , The University of Sydney , Sydney , New South Wales , Australia
- 7 AGE Research Group , Newcastle University , Newcastle upon Tyne , UK
- 8 NIHR Newcastle Biomedical Research Centre , Newcastle upon Tyne , Newcastle upon Tyne , UK
- Correspondence to Dr Joanna M Blodgett, Institute of Sport, Exercise and Health, UCL, London WC1E 6BT, UK; joanna.blodgett{at}ucl.ac.uk
Background Socioeconomic differences in movement behaviours may contribute to health inequalities. The aim of this descriptive study was to investigate socioeconomic patterns in device-measured 24-hour movement and assess whether patterns differ between weekdays and weekends.
Methods 4894 individuals aged 46 years from the 1970 British Cohort Study were included. Participants wore thigh-worn accelerometers for 7 days. Movement behaviours were classified in two 24-hour compositions based on intensity and posture, respectively: (1) sleep, sedentary behaviour, light-intensity activity and moderate-vigorous activity; and (2) sleep, lying, sitting, standing, light movement, walking and combined exercise-like activity. Four socioeconomic measures were explored: education, occupation, income and deprivation index. Movement behaviours were considered compositional means on a 24-hour scale; isometric log ratios expressed per cent differences in daily time in each activity compared with the sample mean.
Results Associations were consistent across all socioeconomic measures. For example, those with a degree spent more time in exercise-like activities across weekdays (10.8%, 95% CI 7.3 to 14.7; ref: sample mean) and weekends (21.9%, 95% CI 17.2 to 26.9). Other patterns differed markedly by the day of the week. Those with no formal qualifications spent more time standing (5.1%, 95% CI 2.3 to 7.1), moving (10.8%, 95% CI 8.6 to 13.1) and walking(4.0%, 95% CI 2.2 to 6.1) during weekdays, with no differences on weekends. Conversely, those with no formal qualifications spent less time sitting during weekdays (−6.6%, 95% CI −7.8 to –4.8), yet more time lying on both weekends (8.8%, 95% CI 4.9 to 12.2) and weekdays (7.5%, 95% CI 4.0 to 11.5).
Conclusions There were strong socioeconomic gradients in 24-hour movement behaviours, with notable differences between weekdays/weekends and behaviour type/posture. These findings emphasise the need to consider socioeconomic position, behaviour type/posture and the day of the week when researching or designing interventions targeting working-age adults.
- SOCIAL CLASS
- COHORT STUDIES
- EPIDEMIOLOGY
Data availability statement
Data are available in a public, open access repository. The BCS70 datasets are publicly available in the UK Data Archive repository: BCS70 https://discover.ukdataservice.ac.uk/series/?sn=200001 .
This is an open access article distributed in accordance with the Creative Commons Attribution 4.0 Unported (CC BY 4.0) license, which permits others to copy, redistribute, remix, transform and build upon this work for any purpose, provided the original work is properly cited, a link to the licence is given, and indication of whether changes were made. See: https://creativecommons.org/licenses/by/4.0/ .
https://doi.org/10.1136/jech-2023-221726
Statistics from Altmetric.com
Request permissions.
If you wish to reuse any or all of this article please use the link below which will take you to the Copyright Clearance Center’s RightsLink service. You will be able to get a quick price and instant permission to reuse the content in many different ways.
WHAT IS ALREADY KNOWN ON THIS TOPIC
There is conflicting evidence of the direction of association between socioeconomic position (SEP) and sedentary behaviour (SB) and physical activity (PA).
Previous research has usually examined movement behaviours in isolation, often as total PA or SB time, without considering the day of the week or movement type.
WHAT THIS STUDY ADDS
Results indicate clear socioeconomic gradients in 24-hour movement patterns, with marked differences between weekdays and weekends and movement behaviour type.
Those with lower SEP spent more time standing, moving and walking and sat less during weekdays; conversely, these SEP differences were reversed on weekends.
Those with higher SEP spent more time in combined exercise-like activities and less time lying down regardless of the day of the week.
HOW THIS STUDY MIGHT AFFECT RESEARCH, PRACTICE OR POLICY
These findings provide novel insights into socioeconomic inequalities in movement behaviours and underscore the importance of considering both the day of the week and SEP when researching or designing interventions related to movement behaviours.
Introduction
Socioeconomic gradients in health behaviours, such as physical activity (PA) and sedentary behaviour (SB), may contribute to the higher morbidity and mortality rates observed in socioeconomically disadvantaged groups. 1 Consequently, it is important to understand how PA and SB patterns differ by socioeconomic position (SEP) 2 in order to develop effective policies and interventions. SEP disparities are typically associated with lower PA levels 3 4 ; both self-reported and device-measured data have repeatedly suggested that individuals with higher SEP engage in higher levels of leisure time PA. 5–8 However, there is varied evidence as others report null findings 9–11 or even inverse associations. 12 13 Conflicting evidence also exists regarding SB, with higher levels of TV watching in those with lower SEP, 14 15 while desk-based jobs are linked to higher SB in those from higher SEP. 16 17 Alongside sex differences in occupation, leisure and household-based PA, weekly timing of movement behaviours is likely to play a role in these discrepancies. Activity patterns in individuals of working age are naturally constrained by working schedules (eg, traditional office hours and shift work), 18 and therefore, socioeconomic patterning of SB and PA may differ by the day of the week.
Most research examining inequalities in PA has examined PA and SB behaviours in isolation or considered categorical phenotypes related to PA guidelines(eg, ‘active couch potato’ and ‘weekend warrior’ 19 20 ). This has provided an incomplete understanding of socioeconomic differences in movement behaviours, given the clear interdependence of behavioural and biological pathways underlying behaviours across the 24-hour day. 21 Increasingly, PA epidemiologists have applied compositional approaches to investigate 24-hour movement behaviours, instead of isolated exploration of PA or SB. 21 These approaches simultaneously consider the relative time spent in different movement types within a finite day, 21 accounting for shared behavioural considerations and exploring potential implications of changes between pairs of behaviours (ie, replacing SB with varying activities). 22
There is a need to better understand conflicting evidence in socioeconomic patterning of 24-hour movement behaviours to inform the development of new national and international guidelines using a 24-hour approach. Conflicting evidence could be due to differences in context (eg, occupation or leisure), movement or posture type (eg, sitting vs lying, walking vs running), or age (eg, children, working age and retired individuals). Thigh-worn accelerometers now provide improved postural identification, allowing greater sensitivity in detecting posture and activity types. 23 Finally, the separation of weekend and weekday data enables an improved understanding of potential contextual factors and aids in improving implementation frameworks needed for targeted PA and SB interventions.
The aim of this descriptive study was to investigate socioeconomic patterning in 24-hour movement behaviours—including sleep, movement intensity and posture/activity type—and assess whether these patterns differ between weekdays and weekends. We used an age-homogenous sample of working-age individuals to eliminate differences in age-related patterning of habits and behaviours and explored any differences by sex.
Study sample
The 1970 British Cohort Study (BCS70) is an ongoing birth cohort comprising over 17 000 individuals born within a week of each other in April 1970 in England, Scotland and Wales. 24 25 All births were eligible, including those in hospitals, homes and army facilities; the first questionnaire was completed by the midwife. At the most recent data collection wave at age 46 years, 8581 individuals participated; characteristics of those lost to follow-up have been described elsewhere. 24 Individuals completed a computer-assisted questionnaire and interview biomedical tests and were asked to wear an accelerometer device for 7 days. Of 6562 individuals who consented to wear an accelerometer, 693 (10.6%) accelerometers were not initiated or not returned, data could not be downloaded for 858 (13.1%) and 117 (1.8%) had insufficient valid wear time (defined below), leaving data on 4894 for inclusion in analyses. Those who declined to wear accelerometers were more likely to be male, to be smokers, to report poorer health and to be obese. 26
Socioeconomic position
At age 46 years, individuals reported any recent education or training they had completed since ages 38 and 42 years, which was compared with previous sweeps to determine participants’ highest academic qualification. Four categories were considered: none, up to General Certificate of Secondary Education (GCSE) level (typically age 16 years), up to A-levels or diploma (typically age 18 years) and degree or higher. Education is presented as the primary outcome throughout the paper as it is one of the more commonly used measures of SEP and is less susceptible to non-response bias and less likely to be affected by diseases that arise during adulthood. 2 Ascertainment of secondary outcomes (occupational class, income and index of multiple deprivation (IMD)) is provided in online supplemental text 1 .
Supplemental material
24-hour movement behaviours.
Participants were invited to wear an activPAL3 microaccelerometer for 7 days, including during sleeping, bathing or swimming activities. 26 Research nurses waterproofed and fitted the device on the midline anterior aspect of the upper thigh during the clinic visit. Data were processed using ActiPASS V.1.32, a validated software that can identify non-wear, sleep, and activity and posture types. 27 28 The average time spent in movement behaviours was classified in two 24-hour compositions. Composition 1 captured a traditional 4-part day and consisted of sleep, SB (lying and sitting), light-intensity PA (LIPA) and moderate-vigorous PA (MVPA). Composition 2 comprised sleep, lying, sitting, standing, moving, walking and combined exercise-like activities. 27–29 We combined running, cycling and inclined walking—previously categorised as high-intensity activities 22 —as a single category of exercise-like activities due to the small volumes of each component. Participants with 1+ weekday and 1+ weekend day (≥20 hours/day, ≥1 walking period and >0 min of sleep) were included in the analysis (n=4894). Although there is no established minimum wear time for 24-hour data, previous sensitivity analyses—including in this sample—suggest that results do not change when requirements were increased to 3+ days with 23+ hours/day of valid wear time. 22
Statistical analysis
Absolute time spent in each movement behaviour for weekdays and weekend days were described. We defined composition as the proportion of time spent in each behaviour (ie, 4-part or 7-part composition). For weekdays and weekend days, we calculated mean time (rescaled such that behaviours sum to 24 hours) for each educational group. Using sex-adjusted multivariate analysis of covariance (MANCOVA), we examined how movement behaviours differed across educational groups. Next, we described how time spent in each behaviour differs compared with the overall sample mean using isometric log ratio differences to express per cent differences; we derived bootstrap (n=1000) percentile confidence intervals for these differences. 30 We repeated the above analyses for occupational class, income and IMD. Finally, we carried out additional analyses of sex-stratified models (decided a priori 10 13 ) and investigated the characteristics of those who participated in the age of 46 years wave but had missing accelerometer data.
Sample characteristics
A total of 4894 individuals were included in analyses, of which 25.7% of individuals had no formal qualifications and 28.4% had a university degree or higher ( table 1 ). Nearly half of the sample (47%) reported having a lower managerial/intermediate occupation, with ~15% falling under each routine/semiroutine or small employer/lower supervisory. Median weekly income was £692 per week (Q1, £378, and Q3, £1036). Approximately a quarter of individuals were in the lowest four deciles of IMD (27.0%), 21% in deciles 5–6, 25% in deciles 7–8 and 26.9% in deciles 9–10. Men were more likely to have no qualifications than women (28.9% vs 22.3%) and be in a lower IMD decile (49.7% vs 46.6% deciles 1–6), yet they were more likely to have a professional/managerial role (22.5% vs 11.6%) and higher income (29.0% vs 24.6% in the highest quartile; online supplemental table 1 ).
- View inline
Characteristics of included sample (n=4894)
Average time spent in LIPA (4.7±1.8 vs 4.7±1.7 hours per day) or MVPA (1.3±0.56 vs 1.3±0.64 hours per day) was consistent across weekdays and weekend days; participants slept longer on weekends (7.7±1.6 vs 7.0±1.2 hours per day) and were more sedentary during weekdays (10.0±2.2 vs 9.2±2.1 hours per day; table 1 , Composition 1). There were no differences in standing, moving, walking or combined high-intensity activity; however, individuals spent more time lying (2.0±1.5 vs 1.6±1.2 hours per day) on the weekend and more time sitting during the weekday (8.5±2.4 vs 7.2±2.2 hours per day; table 1 , Composition 2).
Composition 1: sleep, SB, LIPA, MVPA
There was an overall association between each SEP indicator and Composition 1 for weekdays and weekend days (p<0.001). Figure 1 provides per cent differences in daily time spent in each behaviour for each education group compared with the overall sample mean. As patterns were similar across each outcome, education is primarily described in text; results are replicated in supplementary materials for occupational class, income and IMD ( online supplemental figures 1–3 ). There was no difference in time spent sleeping by educational group for either day type. During weekdays, there was a graded association between education level and sedentary time; for example, compared with the overall sample, individuals with a degree or higher spent 4.3% (95% CI 3.4% to 5.4%) more time in SB, while those with no qualifications spent 3.6% (−4.7% to –2.6%) less time. During weekends, the opposite pattern was observed as individuals with higher education spent less time sedentary (degree:−2.7% (−3.6% to –1.5%)) and those with no qualifications spent more (1.5% (0.4% to 2.7%)).
- Download figure
- Open in new tab
- Download powerpoint
Per cent differences in daily movement between education groups and the sample mean on (A) weekdays and (B) weekends; derived with the log ratio of geometric mean values for sleep, sedentary behaviour (SB), light-intensity physical activity (LIPA) and moderate-vigorous intensity physical activity (MVPA), with bootstrap 95% CIs. GCSE: General Certificate of Secondary Education.
Inequalities in activity patterns are also contrasted by the day of the week ( figure 1 ). During weekdays, those with no formal qualifications and those with GCSE levels or below spent 6.8% (95% CI 5.1% to 8.8%) and 4.1% (2.5% to 5.8%) more time in LIPA, while those with A-levels/diploma and those with a degree spent 3.5% (−5.9% to –1.0%) and 9.2% (–10.8% to –7.4%) less time in LIPA (ref: overall sample). There was a similar, although weaker association for MVPA. Conversely, during the weekends, those with greater educational attainment spent more time in LIPA (none, −1.9% (95% CI –2.7% to 0.1%); GCSE, −1.7% (−3.4% to –0.0%); A-levels, 2.8% (0.2% to 5.2%); and degree or higher, 2.0% (0.4% to 3.6%)) and in MVPA (none, −4.3% (−7.1% to –1.5%); GCSE, −4.8% (−7.0% to –2.6%); A-levels: 0.5% (–3.0% to 4.0%); degree or higher, 9.3% (7.0% to 11.6%)).
Near identical associations were observed across other SEP indicators ( online supplemental figures 2 and 3 ), although effect sizes were smaller for IMD and larger for occupation during weekdays. For example, there was little difference in SB time across IMD quartiles, despite clear trends for the other SEP indicators. Conversely, some per cent differences were twice the size for occupational class compared with education. For example, during weekdays, those in the lowest occupational classes engaged in ~20% more LIPA (vs ~7% in lowest educational classes), whereas the highest occupational class engaged in ~20% less (vs ~8% in highest educational class) ( online supplemental figure 2A ).
Composition 2: sleep, lying, sitting, standing, moving, walking, combined exercise-like activity
There were also associations between each SEP indicator and the 7-part composition (p<0.001). Time spent in movement behaviours differed by educational attainment for all behaviours except sleep during the weekdays and for three behaviours during the weekends (lying, walk and combined exercise-like activity).
During both weekdays and weekend days, those with lower education spent more time lying than those with higher educational attainment ( figure 2 ). For example, compared with the overall sample, those with no qualifications spent 8.8% (95% CI 4.9% to 12.2%) and 7.5% (4.0% to 11.5%) more time lying on weekdays and weekend days, respectively. This dose-response association was observed in the opposite direction for sitting during the week, with increasing levels of sitting at higher education levels (none, −6.6%(95% CI −7.8% to –4.8%); GCSE levels, −2.6% (−3.8% to –1.2%); A-levels (2.6% (0.7% to 4.6%); or degree or higher (6.4% (5.0% to 7.5%)). There were no differences in sitting time during the weekends ( figure 2B ).
Per cent differences in daily movement between education groups and the sample mean on (A) weekdays and (B) weekends; derived with the log ratio of geometric mean values for sleep, lying, sitting, standing, moving, walking and combined exercise-like activity, with bootstrap 95% CIs. GCSE: General Certificate of Secondary Education.
Standing, moving and walking exhibited similar patterns of association during the weekday; those with no formal qualifications spent more time (5.1% (95% CI 2.3% to 7.1%), 10.8% (8.6% to 13.1%) and 4.0% (2.2% to 6.1%), respectively), and those with a degree or higher spent less time(−8.2% (−9.9% to –6.3%), −13.0% (−14.7% to −11.0%) and −4.0% (−5.8% to –2.1%), respectively) in these activities. There were minimal differences between groups during the weekends, with evidence that those with more education spend more time walking (degree or higher: 6.9% (95% CI 4.9% to 9.0%)) than other groups. Finally, those with a degree or higher spent substantially more time in combined exercise-like activity than the rest of the sample across both weekdays (10.8% (95% CI 7.3% to 14.7%)) and weekends (21.9% (17.2% to 26.9%)).
As in Composition 1, similar patterns were observed for occupational class, income and IMD ( online supplemental figures 4–6 ). However, there was little difference in sitting, standing or walking during the week by IMD quartile, with more sitting, less moving or walking in those living in more deprived areas during the weekends.
Additional analyses
Associations were similar when stratified by sex ( online supplemental figures 7 and 8 ), with some indication of slightly larger effect sizes in men. Those with missing accelerometer data were more likely to be male and have lower educational attainment (eg, 33.2% vs 25.7% no qualifications), lower occupational class, lower income and living in more deprived areas ( online supplemental table 2 ).
We found clear socioeconomic gradients in 24-hour movement patterns in individuals aged 46 years, with marked differences between weekdays and weekends. Compared with higher SEP groups (ie, higher educational qualifications, occupational class, income or living in a lower deprivation area), those with lower SEP spent more time standing, moving and walking and sat less during weekdays; these SEP differences were reversed on weekends. Those with higher SEP spent more time in combined exercise-like activities and less time lying regardless of the day of the week. These findings provide novel insights into socioeconomic inequalities in movement behaviours and underscore the importance of considering SEP and weekdays when researching or designing interventions related to movement behaviours.
The novelty of this study is the consideration of the 24-hour composition and exploration of more nuanced posture (eg, sitting and lying) and activity (eg, standing, moving, walking and exercise) classifications. Findings address an important gap in the literature by reconciling conflicting evidence of associations between SEP and device-measured SB or PA. 4–7 We observed more lying time in those with lower SEP, regardless of the day of the week, and higher sitting time in those with higher SEP during the week. This is likely due to occupational sitting patterns, with individuals from higher SEP more likely to have desk jobs. 17 While context was not assessed in our study, we hypothesise that lying may be more consistent with television watching, video gaming or mobile phone use, 31 which as self-reported evidence suggests is frequent among lower SEP groups. 14 15 It may also reflect restricted opportunities to engage in leisure-time PA, due to economic or environmental factors. 32 33
Different patterns emerged depending on the activity type and intensity and the day of the week. Individuals from lower SEP spent more time standing, moving and walking during weekdays, reflecting the increased likelihood of manual-based work in lower socioeconomic groups. 34 Conversely, on weekends, differences in LIPA were negligible, with some evidence of less walking among those from lower socioeconomic groups. Lower walking time, combined with increased lying time on the weekends, might suggest that individuals in manual jobs prioritise rest instead of engaging in light leisure-time PA. Moreover, those from higher socioeconomic groups engaged in more high-intensity PA regardless of the day of the week. This may reflect greater participation in structured leisure-time PA and align with evidence suggesting that educational attainment is associated with greater engagement in health-promoting PA during leisure time. 6 These differences contrast with the negligible differences observed in MVPA during weekdays, which are likely due to both occupational-based moderate-intensity activity such as fast walking and leisure-time high-intensity combined activities being captured.
Opposing patterns of weekday and weekend activity may reflect differences in occupational and leisure-time PA. This is supported by the stronger associations observed when examining occupational or income differences, which are more strongly correlated with occupational activity, compared with educational differences. This is also consistent with several reviews suggesting assumed positive associations between SEP and PA are mainly driven by leisure-time PA. 6 35 36 We add to the evidence by suggesting an inverse association between occupational-based PA and SEP, although evidence combining self-reported data with objective assessments is needed.
Implications
Our results have implications for national and international PA guidelines, as well as those designing interventions targeting SB or PA. Universal movement recommendations may be inadequate due to socioeconomic disparities in behaviours and financial, health and personal circumstances. 34 Guidelines and interventions could instead be tailored to target samples and consider broader implications of how to effectively reach those in greatest need. There is a need to consider differences in SEP, occupation or leisure context, movement/posture (eg, lying vs sitting and exercise-like activities vs LIPA) and weekday. This may include interventions aimed to increase leisure-time PA on non-working days or standing-based interventions for those who spend most of their workday sitting.
The recommended 2.5 hours per week of MVPA may be challenging to achieve for working-age individuals with physically demanding jobs; occupational PA may not yield the same cardiovascular benefits as leisure-time PA. 37 Personalised activity guidance could consider SEP and occupational demands and target communities, employers and local government to address wider socioeconomic disparities. The largest per cent differences were observed for high-intensity activities; however, the absolute time spent in MVPA (eg, ~1–5 hours per day) or specific exercise-like activities (~10–15 min per day) is a relatively small fraction compared with sedentary time (~9–10 hours per day). However, high-intensity activity demonstrates the strongest associations with health outcomes 21 22 and therefore represents a feasible and efficient target for daily change. Evidence from an international consortium suggests that ~3–4 min per day of sedentary time replaced by MVPA could improve cardiometabolic health markers. 22
The opposing socioeconomic gradients across weekdays and weekends suggest a need to consider the day of the week when analysing device-measured movement data. Although there are some recommendations when deriving summary variables (eg, 2+ weekends and 3+ weekdays or 1+ weekend day 38 39 ), criteria can shift to 1+ or 4+ days due to competing desires to maximise sample size. Failure to consider the day may result in biased estimates of movement behaviour; this is applicable both to describing inequalities in these behaviours and to yielding accurate overall measures of behaviour, given established socioeconomic differences in wear time. 40 Opposing associations between SEP and PA/SB by the day of the week highlight that it may not be appropriate to give equal weight to weekend days and weekdays to derive a single summary indicator of mean daily time. Future studies could report on the distribution of activity captured across weekdays and weekends and explore how associations with disease and mortality outcomes are sensitive to this distribution.
Strengths and limitations
Key strengths include the large age-homogenous sample of working-age individuals, device-measured assessment of 24-hour behaviours and the ActiPASS algorithm to identify movement behaviours (ie, sitting, lying, standing, moving, walking, cycling and running). Limitations include the lack of movement behaviour context, potential underestimation of results due to missing accelerometer data in those from lower SEP, misclassification of some behaviours (eg, sleeping vs lying and LIPA vs MVPA) and activity types (eg, swimming and vigorous lifting). This study was descriptive in nature; future studies must extend findings to better investigate associations between SEP and movement behaviours. This includes further exploration of early life SEP, causal pathways between SEP, movement behaviours and health outcomes, and examining how SEP across life influences movement. Further research should also explore how these patterns may change across individuals with different working or personal circumstances (eg, shift work, retirement and childcare).
Conclusions
Our study provides insight into the complex relationships between SEP and PA/SB patterns, revealing opposing patterns between weekdays and weekends. Those from lower SEP groups had higher movement during the weekday and lower movement during the weekend, although socioeconomic inequalities in exercise-specific activities were observed regardless of the day of the week. Findings highlight the importance of considering SEP, movement or posture type, and the day of the week when investigating or intervening in PA and SB. By recognising this, researchers and practitioners can tailor approaches to address the specific needs of diverse populations, ultimately promoting healthier lifestyles and narrowing socioeconomic health disparities.
Ethics statements
Patient consent for publication.
Not applicable.
Ethics approval
This study involves human participants and was approved by the National Research Ethics Service Committee South East Coast–Brighton and Sussex (ref 15/LO/1446). Participants gave informed consent to participate in the study before taking part.
- Stringhini S ,
- Shipley M , et al
- Galobardes B ,
- Lawlor DA , et al
- Althoff T ,
- Hicks JL , et al
- Van Cauwenberg J ,
- Maenhout L , et al
- Johnston LH ,
- Crone D , et al
- Beenackers MA ,
- Kamphuis CBM ,
- Giskes K , et al
- Elhakeem A ,
- Bann D , et al
- Juneau CE ,
- Benmarhnia T ,
- Poulin AA , et al
- Mindell J ,
- Wennman H ,
- Pietilä A ,
- Rissanen H , et al
- Brage S , et al
- Mielke GI ,
- Silva ICM , et al
- Ferrari GL de M ,
- Kovalskys I ,
- Fisberg M , et al
- Sumimoto Y ,
- Yanagita M ,
- Miyamatsu N , et al
- Stamatakis E ,
- Hillsdon M ,
- Mishra G , et al
- Pedisic Z , et al
- Wareham N , et al
- Monnaatsie M ,
- Biddle SJH ,
- Khan S , et al
- Gubelmann C ,
- Vollenweider P ,
- Marques-Vidal P
- Gabriel KP , et al
- Chastin SFM ,
- Palarea-Albaladejo J ,
- Dontje ML , et al
- Blodgett JM ,
- Ahmadi MN ,
- Atkin AJ , et al
- Stevens ML ,
- Inan Eroglu E , et al
- Elliott J ,
- University of London
- Chastin S , et al
- Korshøj M ,
- Kristiansen J , et al
- Hettiarachchi P ,
- Holtermann A , et al
- Tudor-Locke C ,
- Aguiar EJ , et al
- Mathiassen SE ,
- Mateu-Figueras G , et al
- Heinrich S ,
- Kühnlein A , et al
- de Ridder DTD ,
- O’Donoghue G ,
- Kennedy A ,
- Puggina A , et al
- Holtermann A ,
- Rasmussen CL ,
- Hallman DM , et al
- Charity MJ ,
- Harvey JT , et al
- Stalsberg R ,
- Pedersen AV
- van der Beek AJ , et al
- Scheers T ,
- Philippaerts R ,
- Freedson PS , et al
- Pulsford RM ,
- Brocklebank L ,
- Fenton SAM , et al
Supplementary materials
Supplementary data.
This web only file has been produced by the BMJ Publishing Group from an electronic file supplied by the author(s) and has not been edited for content.
- Data supplement 1
X @blodgettjm
Contributors JMB, DB, SFMC, RC and MH contributed to the conception and design of the study. MA derived the device-measured movement behaviour data. JMB performed statistical analyses and wrote the first draft of the manuscript. All authors contributed to the article and approved the submitted version. JMB accepts full responsibility for the finished work and/or the conduct of the study, had access to the data, and controlled the decision to publish.
Funding JMB and MA are supported by a British Heart Foundation grant (SP/F/20/150002). DB is supported by the Economic and Social Research Council (ES/M001660/1) and Medical Research Council (MR/V002147/1). This work was supported by a CLOSER Innovation Fund work package which is funded by the Economic and Social Research Council (ES/K000357/1). ES is funded by an Investigator Grant Leadership Fellowship level 2 by the National Health and Medical Research Council (Australia; reference number not applicable). RC acknowledges support from the National Institute for Health and Care Research (NIHR) Newcastle Biomedical Research Centre based at Newcastle upon Tyne Hospitals NHS Foundation Trust, Cumbria, Northumberland, Tyne and Wear NHS Foundation Trust and Newcastle University (reference number not applicable). RC also receives support as part of a generous donation made by the McArdle family to the Newcastle University for research that will benefit the lives of older people in the UK (reference number not applicable).
Competing interests None declared.
Provenance and peer review Not commissioned; externally peer reviewed.
Supplemental material This content has been supplied by the author(s). It has not been vetted by BMJ Publishing Group Limited (BMJ) and may not have been peer-reviewed. Any opinions or recommendations discussed are solely those of the author(s) and are not endorsed by BMJ. BMJ disclaims all liability and responsibility arising from any reliance placed on the content. Where the content includes any translated material, BMJ does not warrant the accuracy and reliability of the translations (including but not limited to local regulations, clinical guidelines, terminology, drug names and drug dosages), and is not responsible for any error and/or omissions arising from translation and adaptation or otherwise.
Read the full text or download the PDF:
Artificial intelligence in strategy
Can machines automate strategy development? The short answer is no. However, there are numerous aspects of strategists’ work where AI and advanced analytics tools can already bring enormous value. Yuval Atsmon is a senior partner who leads the new McKinsey Center for Strategy Innovation, which studies ways new technologies can augment the timeless principles of strategy. In this episode of the Inside the Strategy Room podcast, he explains how artificial intelligence is already transforming strategy and what’s on the horizon. This is an edited transcript of the discussion. For more conversations on the strategy issues that matter, follow the series on your preferred podcast platform .
Joanna Pachner: What does artificial intelligence mean in the context of strategy?
Yuval Atsmon: When people talk about artificial intelligence, they include everything to do with analytics, automation, and data analysis. Marvin Minsky, the pioneer of artificial intelligence research in the 1960s, talked about AI as a “suitcase word”—a term into which you can stuff whatever you want—and that still seems to be the case. We are comfortable with that because we think companies should use all the capabilities of more traditional analysis while increasing automation in strategy that can free up management or analyst time and, gradually, introducing tools that can augment human thinking.
Joanna Pachner: AI has been embraced by many business functions, but strategy seems to be largely immune to its charms. Why do you think that is?
Subscribe to the Inside the Strategy Room podcast
Yuval Atsmon: You’re right about the limited adoption. Only 7 percent of respondents to our survey about the use of AI say they use it in strategy or even financial planning, whereas in areas like marketing, supply chain, and service operations, it’s 25 or 30 percent. One reason adoption is lagging is that strategy is one of the most integrative conceptual practices. When executives think about strategy automation, many are looking too far ahead—at AI capabilities that would decide, in place of the business leader, what the right strategy is. They are missing opportunities to use AI in the building blocks of strategy that could significantly improve outcomes.
I like to use the analogy to virtual assistants. Many of us use Alexa or Siri but very few people use these tools to do more than dictate a text message or shut off the lights. We don’t feel comfortable with the technology’s ability to understand the context in more sophisticated applications. AI in strategy is similar: it’s hard for AI to know everything an executive knows, but it can help executives with certain tasks.
When executives think about strategy automation, many are looking too far ahead—at AI deciding the right strategy. They are missing opportunities to use AI in the building blocks of strategy.
Joanna Pachner: What kind of tasks can AI help strategists execute today?
Yuval Atsmon: We talk about six stages of AI development. The earliest is simple analytics, which we refer to as descriptive intelligence. Companies use dashboards for competitive analysis or to study performance in different parts of the business that are automatically updated. Some have interactive capabilities for refinement and testing.
The second level is diagnostic intelligence, which is the ability to look backward at the business and understand root causes and drivers of performance. The level after that is predictive intelligence: being able to anticipate certain scenarios or options and the value of things in the future based on momentum from the past as well as signals picked in the market. Both diagnostics and prediction are areas that AI can greatly improve today. The tools can augment executives’ analysis and become areas where you develop capabilities. For example, on diagnostic intelligence, you can organize your portfolio into segments to understand granularly where performance is coming from and do it in a much more continuous way than analysts could. You can try 20 different ways in an hour versus deploying one hundred analysts to tackle the problem.
Predictive AI is both more difficult and more risky. Executives shouldn’t fully rely on predictive AI, but it provides another systematic viewpoint in the room. Because strategic decisions have significant consequences, a key consideration is to use AI transparently in the sense of understanding why it is making a certain prediction and what extrapolations it is making from which information. You can then assess if you trust the prediction or not. You can even use AI to track the evolution of the assumptions for that prediction.
Those are the levels available today. The next three levels will take time to develop. There are some early examples of AI advising actions for executives’ consideration that would be value-creating based on the analysis. From there, you go to delegating certain decision authority to AI, with constraints and supervision. Eventually, there is the point where fully autonomous AI analyzes and decides with no human interaction.
Because strategic decisions have significant consequences, you need to understand why AI is making a certain prediction and what extrapolations it’s making from which information.
Joanna Pachner: What kind of businesses or industries could gain the greatest benefits from embracing AI at its current level of sophistication?
Yuval Atsmon: Every business probably has some opportunity to use AI more than it does today. The first thing to look at is the availability of data. Do you have performance data that can be organized in a systematic way? Companies that have deep data on their portfolios down to business line, SKU, inventory, and raw ingredients have the biggest opportunities to use machines to gain granular insights that humans could not.
Companies whose strategies rely on a few big decisions with limited data would get less from AI. Likewise, those facing a lot of volatility and vulnerability to external events would benefit less than companies with controlled and systematic portfolios, although they could deploy AI to better predict those external events and identify what they can and cannot control.
Third, the velocity of decisions matters. Most companies develop strategies every three to five years, which then become annual budgets. If you think about strategy in that way, the role of AI is relatively limited other than potentially accelerating analyses that are inputs into the strategy. However, some companies regularly revisit big decisions they made based on assumptions about the world that may have since changed, affecting the projected ROI of initiatives. Such shifts would affect how you deploy talent and executive time, how you spend money and focus sales efforts, and AI can be valuable in guiding that. The value of AI is even bigger when you can make decisions close to the time of deploying resources, because AI can signal that your previous assumptions have changed from when you made your plan.
Joanna Pachner: Can you provide any examples of companies employing AI to address specific strategic challenges?
Yuval Atsmon: Some of the most innovative users of AI, not coincidentally, are AI- and digital-native companies. Some of these companies have seen massive benefits from AI and have increased its usage in other areas of the business. One mobility player adjusts its financial planning based on pricing patterns it observes in the market. Its business has relatively high flexibility to demand but less so to supply, so the company uses AI to continuously signal back when pricing dynamics are trending in a way that would affect profitability or where demand is rising. This allows the company to quickly react to create more capacity because its profitability is highly sensitive to keeping demand and supply in equilibrium.
Joanna Pachner: Given how quickly things change today, doesn’t AI seem to be more a tactical than a strategic tool, providing time-sensitive input on isolated elements of strategy?
Yuval Atsmon: It’s interesting that you make the distinction between strategic and tactical. Of course, every decision can be broken down into smaller ones, and where AI can be affordably used in strategy today is for building blocks of the strategy. It might feel tactical, but it can make a massive difference. One of the world’s leading investment firms, for example, has started to use AI to scan for certain patterns rather than scanning individual companies directly. AI looks for consumer mobile usage that suggests a company’s technology is catching on quickly, giving the firm an opportunity to invest in that company before others do. That created a significant strategic edge for them, even though the tool itself may be relatively tactical.
Joanna Pachner: McKinsey has written a lot about cognitive biases and social dynamics that can skew decision making. Can AI help with these challenges?
Yuval Atsmon: When we talk to executives about using AI in strategy development, the first reaction we get is, “Those are really big decisions; what if AI gets them wrong?” The first answer is that humans also get them wrong—a lot. [Amos] Tversky, [Daniel] Kahneman, and others have proven that some of those errors are systemic, observable, and predictable. The first thing AI can do is spot situations likely to give rise to biases. For example, imagine that AI is listening in on a strategy session where the CEO proposes something and everyone says “Aye” without debate and discussion. AI could inform the room, “We might have a sunflower bias here,” which could trigger more conversation and remind the CEO that it’s in their own interest to encourage some devil’s advocacy.
We also often see confirmation bias, where people focus their analysis on proving the wisdom of what they already want to do, as opposed to looking for a fact-based reality. Just having AI perform a default analysis that doesn’t aim to satisfy the boss is useful, and the team can then try to understand why that is different than the management hypothesis, triggering a much richer debate.
In terms of social dynamics, agency problems can create conflicts of interest. Every business unit [BU] leader thinks that their BU should get the most resources and will deliver the most value, or at least they feel they should advocate for their business. AI provides a neutral way based on systematic data to manage those debates. It’s also useful for executives with decision authority, since we all know that short-term pressures and the need to make the quarterly and annual numbers lead people to make different decisions on the 31st of December than they do on January 1st or October 1st. Like the story of Ulysses and the sirens, you can use AI to remind you that you wanted something different three months earlier. The CEO still decides; AI can just provide that extra nudge.
Joanna Pachner: It’s like you have Spock next to you, who is dispassionate and purely analytical.
Yuval Atsmon: That is not a bad analogy—for Star Trek fans anyway.
Joanna Pachner: Do you have a favorite application of AI in strategy?
Yuval Atsmon: I have worked a lot on resource allocation, and one of the challenges, which we call the hockey stick phenomenon, is that executives are always overly optimistic about what will happen. They know that resource allocation will inevitably be defined by what you believe about the future, not necessarily by past performance. AI can provide an objective prediction of performance starting from a default momentum case: based on everything that happened in the past and some indicators about the future, what is the forecast of performance if we do nothing? This is before we say, “But I will hire these people and develop this new product and improve my marketing”— things that every executive thinks will help them overdeliver relative to the past. The neutral momentum case, which AI can calculate in a cold, Spock-like manner, can change the dynamics of the resource allocation discussion. It’s a form of predictive intelligence accessible today and while it’s not meant to be definitive, it provides a basis for better decisions.
Joanna Pachner: Do you see access to technology talent as one of the obstacles to the adoption of AI in strategy, especially at large companies?
Yuval Atsmon: I would make a distinction. If you mean machine-learning and data science talent or software engineers who build the digital tools, they are definitely not easy to get. However, companies can increasingly use platforms that provide access to AI tools and require less from individual companies. Also, this domain of strategy is exciting—it’s cutting-edge, so it’s probably easier to get technology talent for that than it might be for manufacturing work.
The bigger challenge, ironically, is finding strategists or people with business expertise to contribute to the effort. You will not solve strategy problems with AI without the involvement of people who understand the customer experience and what you are trying to achieve. Those who know best, like senior executives, don’t have time to be product managers for the AI team. An even bigger constraint is that, in some cases, you are asking people to get involved in an initiative that may make their jobs less important. There could be plenty of opportunities for incorporating AI into existing jobs, but it’s something companies need to reflect on. The best approach may be to create a digital factory where a different team tests and builds AI applications, with oversight from senior stakeholders.
The big challenge is finding strategists to contribute to the AI effort. You are asking people to get involved in an initiative that may make their jobs less important.
Joanna Pachner: Do you think this worry about job security and the potential that AI will automate strategy is realistic?
Yuval Atsmon: The question of whether AI will replace human judgment and put humanity out of its job is a big one that I would leave for other experts.
The pertinent question is shorter-term automation. Because of its complexity, strategy would be one of the later domains to be affected by automation, but we are seeing it in many other domains. However, the trend for more than two hundred years has been that automation creates new jobs, although ones requiring different skills. That doesn’t take away the fear some people have of a machine exposing their mistakes or doing their job better than they do it.
Joanna Pachner: We recently published an article about strategic courage in an age of volatility that talked about three types of edge business leaders need to develop. One of them is an edge in insights. Do you think AI has a role to play in furnishing a proprietary insight edge?
Yuval Atsmon: One of the challenges most strategists face is the overwhelming complexity of the world we operate in—the number of unknowns, the information overload. At one level, it may seem that AI will provide another layer of complexity. In reality, it can be a sharp knife that cuts through some of the clutter. The question to ask is, Can AI simplify my life by giving me sharper, more timely insights more easily?
Joanna Pachner: You have been working in strategy for a long time. What sparked your interest in exploring this intersection of strategy and new technology?
Yuval Atsmon: I have always been intrigued by things at the boundaries of what seems possible. Science fiction writer Arthur C. Clarke’s second law is that to discover the limits of the possible, you have to venture a little past them into the impossible, and I find that particularly alluring in this arena.
AI in strategy is in very nascent stages but could be very consequential for companies and for the profession. For a top executive, strategic decisions are the biggest way to influence the business, other than maybe building the top team, and it is amazing how little technology is leveraged in that process today. It’s conceivable that competitive advantage will increasingly rest in having executives who know how to apply AI well. In some domains, like investment, that is already happening, and the difference in returns can be staggering. I find helping companies be part of that evolution very exciting.
Explore a career with us
Related articles.

Strategic courage in an age of volatility
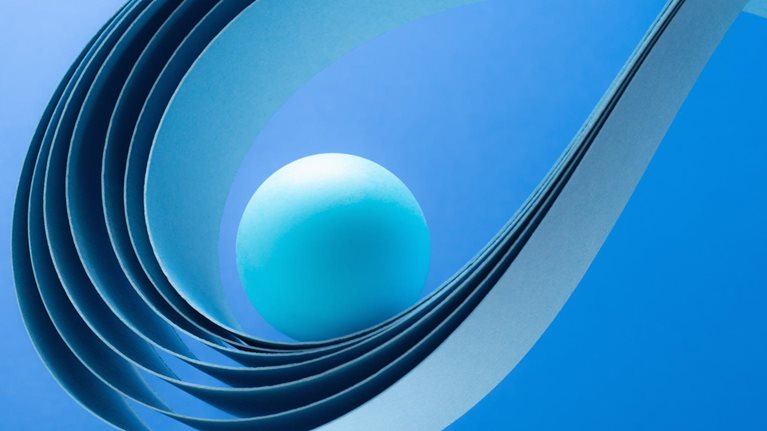
Bias Busters Collection

Subcortical alterations in newly diagnosed epilepsy and associated changes in brain connectivity and cognition
- Find this author on Google Scholar
- Find this author on PubMed
- Search for this author on this site
- For correspondence: [email protected]
- ORCID record for Simon S Keller
- Info/History
- Preview PDF
Objectives: Patients with chronic focal epilepsy commonly exhibit subcortical atrophy, particularly of the thalamus. The timing of these alterations remains uncertain, though preliminary evidence suggests that observable changes may already be present at diagnosis. It is also not yet known how these morphological changes are linked to the coherence of white matter pathways throughout the brain, or to neuropsychological function often compromised before anti-seizure medication treatment. This study investigates localised atrophy in subcortical regions using surface shape analysis in individuals with newly diagnosed focal epilepsy (NDfE) and assesses their implications on brain connectivity and cognitive function. Methods: We collected structural (T1w) and diffusion weighted MRI, as well as neuropsychological data from 104 patients with NDfE and 45 healthy controls (HC) matched for age, sex and education. A vertex-based shape analysis was performed on subcortical structures to compare patients with NDfE and HC, adjusting for age, sex and intracranial volume. The mean deformation of significance areas (pcor < 0.05) was used to identify white matter pathways associated with overall shape alterations in patients relative to controls using correlational tractography. Additionally, the relationship between significant subcortical shape values and neuropsychological outcomes was evaluated using a generalised canonical correlation approach. Results: Shape analysis revealed bilateral focal inward deformation (a proxy for localised atrophy) in anterior areas of the right and left thalamus and right pallidum in patients with NDfE compared to HC (FWE corrected). No structures showed areas of outward deformation in patients. The connectometry analysis revealed that fractional anisotropy (FA) was positively correlated with thalamic and pallidal shape deformation, i.e., reduced FA was associated with inward deformation in tracts proximal to and or connecting with the thalamus including the forix, frontal parahippocampal and corticothalamic pathways. Thalamic and pallidal shape changes were also related to with increased depression and anxiety, and reduced memory and cognitive function. Discussion: These findings suggest that atrophy of the thalamus, which has previously been associated with the generation and maintenance of focal seizures, may present at epilepsy diagnosis and relate to alterations in both white matter connectivity and cognitive performance. We suggest that at least some alterations in brain structure and consequent impact on cognitive and affective processes are the result of early epileptogenic processes rather than exclusively due to the chronicity of longstanding epilepsy, recurrent seizures, and treatment with anti-seizure medication.
Competing Interest Statement
The authors have declared no competing interest.
Funding Statement
A UK Medical Research Council (MRC) research grant (MR/S00355X/1)
Author Declarations
I confirm all relevant ethical guidelines have been followed, and any necessary IRB and/or ethics committee approvals have been obtained.
The details of the IRB/oversight body that provided approval or exemption for the research described are given below:
the study was approved by the North West, Liverpool East Research Ethics Committee (19/NW/0384) through the Integrated Research Application System (Project ID 260623). Health Research Authority (HRA) approval was provided and the project was sponsored by the UoL (UoL001449).
I confirm that all necessary patient/participant consent has been obtained and the appropriate institutional forms have been archived, and that any patient/participant/sample identifiers included were not known to anyone (e.g., hospital staff, patients or participants themselves) outside the research group so cannot be used to identify individuals.
I understand that all clinical trials and any other prospective interventional studies must be registered with an ICMJE-approved registry, such as ClinicalTrials.gov. I confirm that any such study reported in the manuscript has been registered and the trial registration ID is provided (note: if posting a prospective study registered retrospectively, please provide a statement in the trial ID field explaining why the study was not registered in advance).
I have followed all appropriate research reporting guidelines, such as any relevant EQUATOR Network research reporting checklist(s) and other pertinent material, if applicable.
Data Availability
All data produced in the present study are available upon reasonable request to the authors
View the discussion thread.
Thank you for your interest in spreading the word about medRxiv.
NOTE: Your email address is requested solely to identify you as the sender of this article.

Citation Manager Formats
- EndNote (tagged)
- EndNote 8 (xml)
- RefWorks Tagged
- Ref Manager
- Tweet Widget
- Facebook Like
- Google Plus One
- Addiction Medicine (323)
- Allergy and Immunology (627)
- Anesthesia (163)
- Cardiovascular Medicine (2367)
- Dentistry and Oral Medicine (288)
- Dermatology (206)
- Emergency Medicine (379)
- Endocrinology (including Diabetes Mellitus and Metabolic Disease) (835)
- Epidemiology (11765)
- Forensic Medicine (10)
- Gastroenterology (702)
- Genetic and Genomic Medicine (3731)
- Geriatric Medicine (348)
- Health Economics (633)
- Health Informatics (2392)
- Health Policy (929)
- Health Systems and Quality Improvement (896)
- Hematology (340)
- HIV/AIDS (780)
- Infectious Diseases (except HIV/AIDS) (13303)
- Intensive Care and Critical Care Medicine (767)
- Medical Education (365)
- Medical Ethics (104)
- Nephrology (398)
- Neurology (3493)
- Nursing (198)
- Nutrition (523)
- Obstetrics and Gynecology (673)
- Occupational and Environmental Health (662)
- Oncology (1819)
- Ophthalmology (535)
- Orthopedics (218)
- Otolaryngology (287)
- Pain Medicine (232)
- Palliative Medicine (66)
- Pathology (446)
- Pediatrics (1032)
- Pharmacology and Therapeutics (426)
- Primary Care Research (420)
- Psychiatry and Clinical Psychology (3172)
- Public and Global Health (6135)
- Radiology and Imaging (1279)
- Rehabilitation Medicine and Physical Therapy (746)
- Respiratory Medicine (825)
- Rheumatology (379)
- Sexual and Reproductive Health (372)
- Sports Medicine (322)
- Surgery (401)
- Toxicology (50)
- Transplantation (172)
- Urology (145)

Research Implications & Recommendations
A Plain-Language Explainer With Examples + FREE Template
By: Derek Jansen (MBA) | Reviewers: Dr Eunice Rautenbach | May 2024
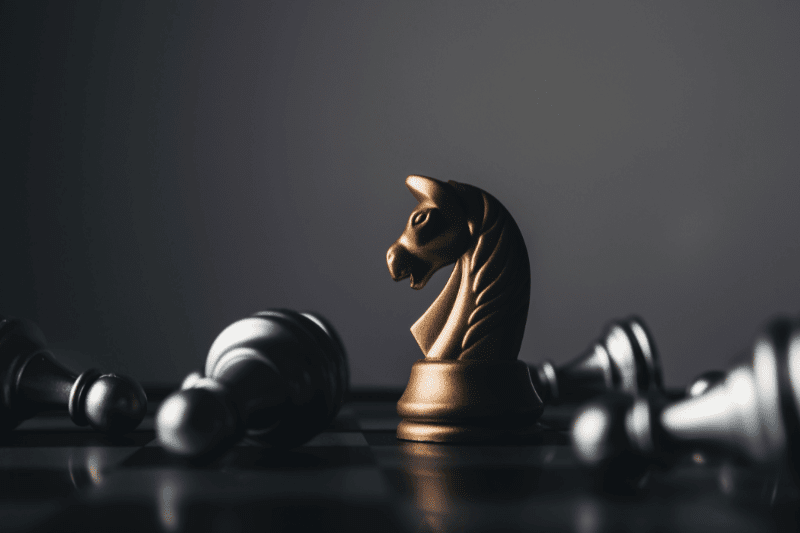
The research implications and recommendations are closely related but distinctly different concepts that often trip students up. Here, we’ll unpack them using plain language and loads of examples , so that you can approach your project with confidence.
Overview: Implications & Recommendations
- What are research implications ?
- What are research recommendations ?
- Examples of implications and recommendations
- The “ Big 3 ” categories
- How to write the implications and recommendations
- Template sentences for both sections
- Key takeaways
Implications & Recommendations 101
Let’s start with the basics and define our terms.
At the simplest level, research implications refer to the possible effects or outcomes of a study’s findings. More specifically, they answer the question, “ What do these findings mean?” . In other words, the implications section is where you discuss the broader impact of your study’s findings on theory, practice and future research.
This discussion leads us to the recommendations section , which is where you’ll propose specific actions based on your study’s findings and answer the question, “ What should be done next?” . In other words, the recommendations are practical steps that stakeholders can take to address the key issues identified by your study.
In a nutshell, then, the research implications discuss the broader impact and significance of a study’s findings, while recommendations provide specific actions to take, based on those findings. So, while both of these components are deeply rooted in the findings of the study, they serve different functions within the write up.
Need a helping hand?
Examples: Implications & Recommendations
The distinction between research implications and research recommendations might still feel a bit conceptual, so let’s look at one or two practical examples:
Let’s assume that your study finds that interactive learning methods significantly improve student engagement compared to traditional lectures. In this case, one of your recommendations could be that schools incorporate more interactive learning techniques into their curriculums to enhance student engagement.
Let’s imagine that your study finds that patients who receive personalised care plans have better health outcomes than those with standard care plans. One of your recommendations might be that healthcare providers develop and implement personalised care plans for their patients.
Now, these are admittedly quite simplistic examples, but they demonstrate the difference (and connection ) between the research implications and the recommendations. Simply put, the implications are about the impact of the findings, while the recommendations are about proposed actions, based on the findings.
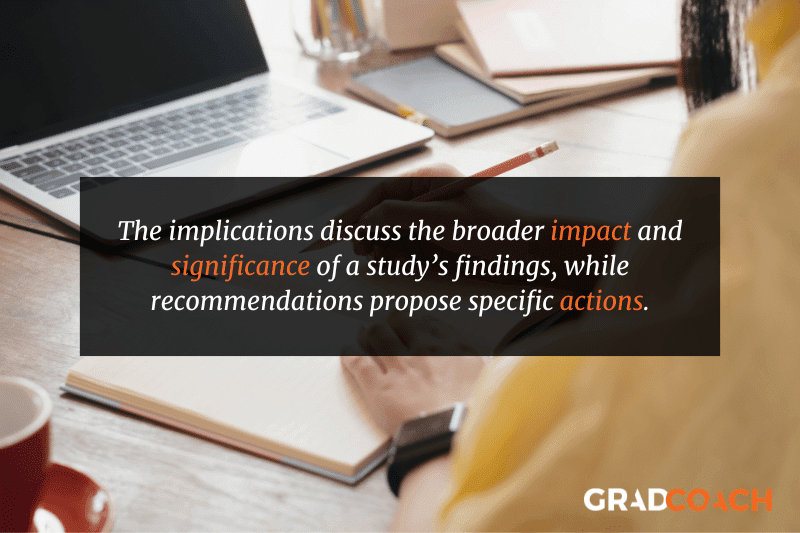
The “Big 3” Categories
Now that we’ve defined our terms, let’s dig a little deeper into the implications – specifically, the different types or categories of research implications that exist.
Broadly speaking, implications can be divided into three categories – theoretical implications, practical implications and implications for future research .
Theoretical implications relate to how your study’s findings contribute to or challenge existing theories. For example, if a study on social behaviour uncovers new patterns, it might suggest that modifications to current psychological theories are necessary.
Practical implications , on the other hand, focus on how your study’s findings can be applied in real-world settings. For example, if your study demonstrated the effectiveness of a new teaching method, this would imply that educators should consider adopting this method to improve learning outcomes.
Practical implications can also involve policy reconsiderations . For example, if a study reveals significant health benefits from a particular diet, an implication might be that public health guidelines be re-evaluated.
Last but not least, there are the implications for future research . As the name suggests, this category of implications highlights the research gaps or new questions raised by your study. For example, if your study finds mixed results regarding a relationship between two variables, it might imply the need for further investigation to clarify these findings.
To recap then, the three types of implications are the theoretical, the practical and the implications on future research. Regardless of the category, these implications feed into and shape the recommendations , laying the foundation for the actions you’ll propose.
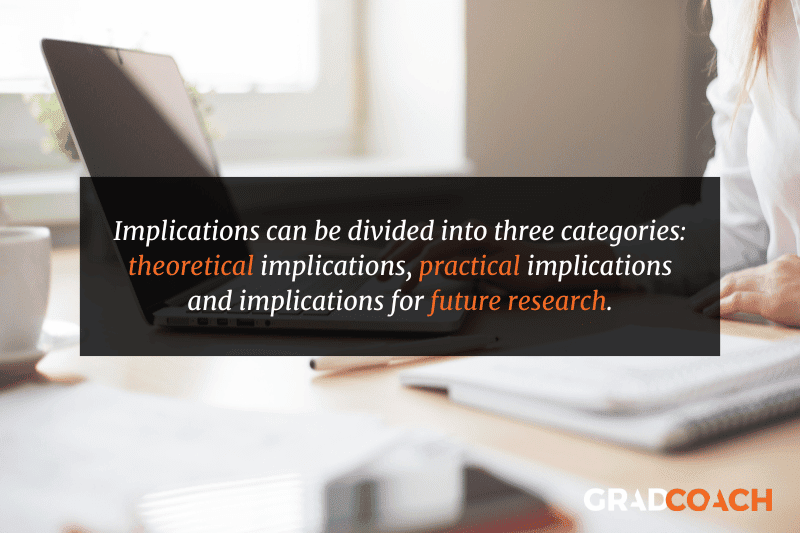
How To Write The Sections
Now that we’ve laid the foundations, it’s time to explore how to write up the implications and recommendations sections respectively.
Let’s start with the “ where ” before digging into the “ how ”. Typically, the implications will feature in the discussion section of your document, while the recommendations will be located in the conclusion . That said, layouts can vary between disciplines and institutions, so be sure to check with your university what their preferences are.
For the implications section, a common approach is to structure the write-up based on the three categories we looked at earlier – theoretical, practical and future research implications. In practical terms, this discussion will usually follow a fairly formulaic sentence structure – for example:
This research provides new insights into [theoretical aspect], indicating that…
The study’s outcomes highlight the potential benefits of adopting [specific practice] in..
This study raises several questions that warrant further investigation, such as…
Moving onto the recommendations section, you could again structure your recommendations using the three categories. Alternatively, you could structure the discussion per stakeholder group – for example, policymakers, organisations, researchers, etc.
Again, you’ll likely use a fairly formulaic sentence structure for this section. Here are some examples for your inspiration:
Based on the findings, [specific group] should consider adopting [new method] to improve…
To address the issues identified, it is recommended that legislation should be introduced to…
Researchers should consider examining [specific variable] to build on the current study’s findings.
Remember, you can grab a copy of our tried and tested templates for both the discussion and conclusion sections over on the Grad Coach blog. You can find the links to those, as well as loads of other free resources, in the description 🙂
FAQs: Implications & Recommendations
How do i determine the implications of my study.
To do this, you’ll need to consider how your findings address gaps in the existing literature, how they could influence theory, practice, or policy, and the potential societal or economic impacts.
When thinking about your findings, it’s also a good idea to revisit your introduction chapter, where you would have discussed the potential significance of your study more broadly. This section can help spark some additional ideas about what your findings mean in relation to your original research aims.
Should I discuss both positive and negative implications?
Absolutely. You’ll need to discuss both the positive and negative implications to provide a balanced view of how your findings affect the field and any limitations or potential downsides.
Can my research implications be speculative?
Yes and no. While implications are somewhat more speculative than recommendations and can suggest potential future outcomes, they should be grounded in your data and analysis. So, be careful to avoid overly speculative claims.
How do I formulate recommendations?
Ideally, you should base your recommendations on the limitations and implications of your study’s findings. So, consider what further research is needed, how policies could be adapted, or how practices could be improved – and make proposals in this respect.
How specific should my recommendations be?
Your recommendations should be as specific as possible, providing clear guidance on what actions or research should be taken next. As mentioned earlier, the implications can be relatively broad, but the recommendations should be very specific and actionable. Ideally, you should apply the SMART framework to your recommendations.
Can I recommend future research in my recommendations?
Absolutely. Highlighting areas where further research is needed is a key aspect of the recommendations section. Naturally, these recommendations should link to the respective section of your implications (i.e., implications for future research).
Wrapping Up: Key Takeaways
We’ve covered quite a bit of ground here, so let’s quickly recap.
- Research implications refer to the possible effects or outcomes of a study’s findings.
- The recommendations section, on the other hand, is where you’ll propose specific actions based on those findings.
- You can structure your implications section based on the three overarching categories – theoretical, practical and future research implications.
- You can carry this structure through to the recommendations as well, or you can group your recommendations by stakeholder.
Remember to grab a copy of our tried and tested free dissertation template, which covers both the implications and recommendations sections. If you’d like 1:1 help with your research project, be sure to check out our private coaching service, where we hold your hand throughout the research journey, step by step.
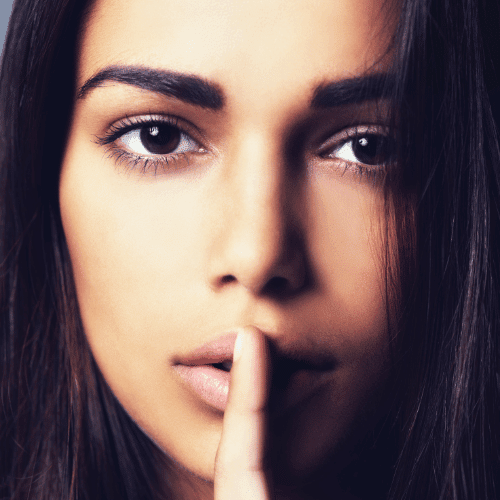
Psst... there’s more!
This post was based on one of our popular Research Bootcamps . If you're working on a research project, you'll definitely want to check this out ...
You Might Also Like:
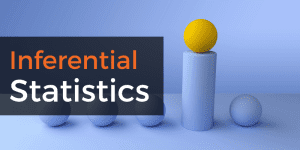
Submit a Comment Cancel reply
Your email address will not be published. Required fields are marked *
Save my name, email, and website in this browser for the next time I comment.
- Print Friendly
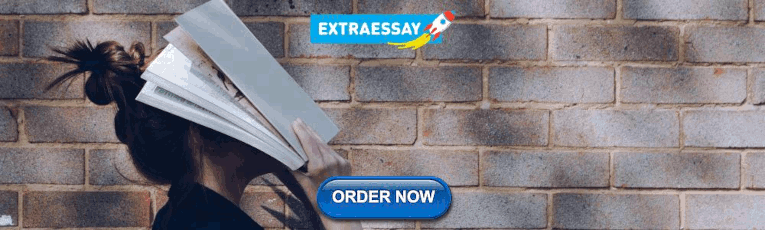
IMAGES
VIDEO
COMMENTS
The discussion section is where you delve into the meaning, importance, and relevance of your results.. It should focus on explaining and evaluating what you found, showing how it relates to your literature review and paper or dissertation topic, and making an argument in support of your overall conclusion.It should not be a second results section.. There are different ways to write this ...
The discussion chapter is where you interpret and explain your results within your thesis or dissertation. This contrasts with the results chapter, where you merely present and describe the analysis findings (whether qualitative or quantitative). In the discussion chapter, you elaborate on and evaluate your research findings, and discuss the ...
The results chapter (also referred to as the findings or analysis chapter) is one of the most important chapters of your dissertation or thesis because it shows the reader what you've found in terms of the quantitative data you've collected. It presents the data using a clear text narrative, supported by tables, graphs and charts.
Here are some examples of how to present the summary of your findings; "The data suggests that", "The results confirm that", "The analysis indicates that", "The research shows a relationship between", etc. 2. Interpretations of Results. Your audience will expect you to provide meanings of the results, although they might seem ...
Begin with a clear statement of the principal findings. This will reinforce the main take-away for the reader and set up the rest of the discussion. Explain why the outcomes of your study are important to the reader. Discuss the implications of your findings realistically based on previous literature, highlighting both the strengths and ...
Here are a few best practices: Your results should always be written in the past tense. While the length of this section depends on how much data you collected and analysed, it should be written as concisely as possible. Only include results that are directly relevant to answering your research questions.
This template covers all the core components required in the discussion/analysis chapter of a typical dissertation or thesis, including: The purpose of each section is explained in plain language, followed by an overview of the key elements that you need to cover. The template also includes practical examples to help you understand exactly what ...
When writing a dissertation or thesis, the results and discussion sections can be both the most interesting as well as the most challenging sections to write. ... Your explanations may include issues such as a non-representative sample for convenience purposes, a response rate skewed towards those with a particular experience, or your own ...
Tips to Write the Results Section. Direct the reader to the research data and explain the meaning of the data. Avoid using a repetitive sentence structure to explain a new set of data. Write and highlight important findings in your results. Use the same order as the subheadings of the methods section.
Guide contents. As part of the Writing the Dissertation series, this guide covers the most common conventions of the results and discussion chapters, giving you the necessary knowledge, tips and guidance needed to impress your markers! The sections are organised as follows: The Difference - Breaks down the distinctions between the results and discussion chapters.
Example dissertation #3: ... Read more about results sections. Discussion. Your discussion section is your opportunity to explore the meaning and implications of your results in relation to your research question. Here, interpret your results in detail, discussing whether they met your expectations and how well they fit with the framework that ...
A Complete Dissertation 5 in the third person (active voice without the personal pronouns I and we). Generally, the first sentence of an abstract describes the entire study; subsequent sentences expand on that description. Frequent Errors Inclusion of irrelevant material (i.e., examples, information extraneous to the dis-
Table of contents. What not to include in your discussion section. Step 1: Summarise your key findings. Step 2: Give your interpretations. Step 3: Discuss the implications. Step 4: Acknowledge the limitations. Step 5: Share your recommendations. Discussion section example.
A discussion chapter of your dissertation is not just a fixation on results but a more profound summary connected to research goals and purpose. Here is an example: Summary of Findings Example According to the data, implementing the co-orientation theory was successful and can be used for the same circumstances in the future.
if you write a scientific dissertation, or anyway using quantitative methods, you will have some objective results that you will present in the Results chapter. You will then interpret the results in the Discussion chapter. B) More common for qualitative methods. - Analysis chapter. This can have more descriptive/thematic subheadings.
Results and Discussion. 5.1 Modelling and joint negotiation of texts. This study set out to investigate the effects of extending the negotiation of text phase of the DSP teaching/learning cycle with English for Further Study students to incorporate student/teacher joint negotiation and construction of a marking instrument.
The results chapter in a dissertation or thesis (or any formal academic research piece) is where you objectively and neutrally present the findings of your qualitative analysis (or analyses if you used multiple qualitative analysis methods ). This chapter can sometimes be combined with the discussion chapter (where you interpret the data and ...
and 4) Smart Clothing's context. The results shown in this chapter have already been processed and analysed, and the raw findings are presented in Appendix B. Most quotes and examples are drawn from the interviews and the focus groups. Further results of the case studies are demonstrated in the last published paper in the Appendix.
Step 1: Craft a brief introduction section. As with all chapters in your dissertation or thesis, the conclusions chapter needs to start with a brief introduction. In this introductory section, you'll want to tell the reader what they can expect to find in the chapter, and in what order.
A growing number of studies have reported that problematic social networking use (PSNU) is strongly associated with anxiety symptoms. However, due to the presence of multiple anxiety subtypes, existing research findings on the extent of this association vary widely, leading to a lack of consensus. The current meta-analysis aimed to summarize studies exploring the relationship between PSNU ...
Results Associations were consistent across all socioeconomic measures. For example, those with a degree spent more time in exercise-like activities across weekdays (10.8%, 95% CI 7.3 to 14.7; ref: sample mean) and weekends (21.9%, 95% CI 17.2 to 26.9). Other patterns differed markedly by the day of the week.
Results; Discussion; Abstracts are usually around 100-300 words, but there's often a strict word limit, so make sure to check the relevant requirements. In a dissertation or thesis, include the abstract on a separate page, after the title page and acknowledgements but before the table of contents.
For example, imagine that AI is listening in on a strategy session where the CEO proposes something and everyone says "Aye" without debate and discussion. AI could inform the room, "We might have a sunflower bias here," which could trigger more conversation and remind the CEO that it's in their own interest to encourage some devil's ...
The methodology, results and discussion chapters go about undertaking new research about this question. The conclusion chapter (attempts to) answer the core research question . In other words, the dissertation structure and layout reflect the research process of asking a well-defined question(s), investigating, and then answering the question ...
Objectives: Patients with chronic focal epilepsy commonly exhibit subcortical atrophy, particularly of the thalamus. The timing of these alterations remains uncertain, though preliminary evidence suggests that observable changes may already be present at diagnosis. It is also not yet known how these morphological changes are linked to the coherence of white matter pathways throughout the brain ...
This template covers all the core components required in the results chapter of a typical dissertation, thesis or research project: The purpose of each section is explained in plain language, followed by an overview of the key elements that you need to cover. The template also includes practical examples to help you understand exactly what's ...
Learn how to write the research implications and recommendations sections for your dissertation or thesis. Simple explainer with examples. About Us; Services. 1-On-1 Coaching ... For example, if your study finds mixed results regarding a relationship between two variables, it might imply the need for further investigation to clarify these ...