Longitudinal Study Design
Julia Simkus
Editor at Simply Psychology
BA (Hons) Psychology, Princeton University
Julia Simkus is a graduate of Princeton University with a Bachelor of Arts in Psychology. She is currently studying for a Master's Degree in Counseling for Mental Health and Wellness in September 2023. Julia's research has been published in peer reviewed journals.
Learn about our Editorial Process
Saul Mcleod, PhD
Editor-in-Chief for Simply Psychology
BSc (Hons) Psychology, MRes, PhD, University of Manchester
Saul Mcleod, PhD., is a qualified psychology teacher with over 18 years of experience in further and higher education. He has been published in peer-reviewed journals, including the Journal of Clinical Psychology.
Olivia Guy-Evans, MSc
Associate Editor for Simply Psychology
BSc (Hons) Psychology, MSc Psychology of Education
Olivia Guy-Evans is a writer and associate editor for Simply Psychology. She has previously worked in healthcare and educational sectors.
A longitudinal study is a type of observational and correlational study that involves monitoring a population over an extended period of time. It allows researchers to track changes and developments in the subjects over time.
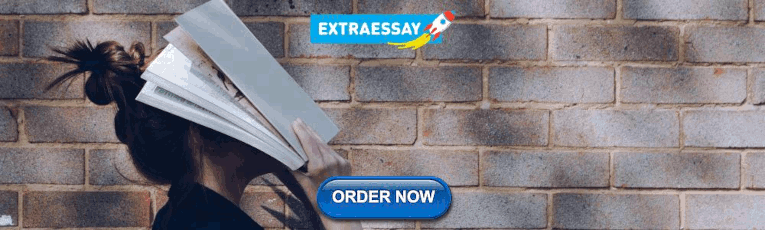
What is a Longitudinal Study?
In longitudinal studies, researchers do not manipulate any variables or interfere with the environment. Instead, they simply conduct observations on the same group of subjects over a period of time.
These research studies can last as short as a week or as long as multiple years or even decades. Unlike cross-sectional studies that measure a moment in time, longitudinal studies last beyond a single moment, enabling researchers to discover cause-and-effect relationships between variables.
They are beneficial for recognizing any changes, developments, or patterns in the characteristics of a target population. Longitudinal studies are often used in clinical and developmental psychology to study shifts in behaviors, thoughts, emotions, and trends throughout a lifetime.
For example, a longitudinal study could be used to examine the progress and well-being of children at critical age periods from birth to adulthood.
The Harvard Study of Adult Development is one of the longest longitudinal studies to date. Researchers in this study have followed the same men group for over 80 years, observing psychosocial variables and biological processes for healthy aging and well-being in late life (see Harvard Second Generation Study).
When designing longitudinal studies, researchers must consider issues like sample selection and generalizability, attrition and selectivity bias, effects of repeated exposure to measures, selection of appropriate statistical models, and coverage of the necessary timespan to capture the phenomena of interest.
Panel Study
- A panel study is a type of longitudinal study design in which the same set of participants are measured repeatedly over time.
- Data is gathered on the same variables of interest at each time point using consistent methods. This allows studying continuity and changes within individuals over time on the key measured constructs.
- Prominent examples include national panel surveys on topics like health, aging, employment, and economics. Panel studies are a type of prospective study .
Cohort Study
- A cohort study is a type of longitudinal study that samples a group of people sharing a common experience or demographic trait within a defined period, such as year of birth.
- Researchers observe a population based on the shared experience of a specific event, such as birth, geographic location, or historical experience. These studies are typically used among medical researchers.
- Cohorts are identified and selected at a starting point (e.g. birth, starting school, entering a job field) and followed forward in time.
- As they age, data is collected on cohort subgroups to determine their differing trajectories. For example, investigating how health outcomes diverge for groups born in 1950s, 1960s, and 1970s.
- Cohort studies do not require the same individuals to be assessed over time; they just require representation from the cohort.
Retrospective Study
- In a retrospective study , researchers either collect data on events that have already occurred or use existing data that already exists in databases, medical records, or interviews to gain insights about a population.
- Appropriate when prospectively following participants from the past starting point is infeasible or unethical. For example, studying early origins of diseases emerging later in life.
- Retrospective studies efficiently provide a “snapshot summary” of the past in relation to present status. However, quality concerns with retrospective data make careful interpretation necessary when inferring causality. Memory biases and selective retention influence quality of retrospective data.
Allows researchers to look at changes over time
Because longitudinal studies observe variables over extended periods of time, researchers can use their data to study developmental shifts and understand how certain things change as we age.
High validation
Since objectives and rules for long-term studies are established before data collection, these studies are authentic and have high levels of validity.
Eliminates recall bias
Recall bias occurs when participants do not remember past events accurately or omit details from previous experiences.
Flexibility
The variables in longitudinal studies can change throughout the study. Even if the study was created to study a specific pattern or characteristic, the data collection could show new data points or relationships that are unique and worth investigating further.
Limitations
Costly and time-consuming.
Longitudinal studies can take months or years to complete, rendering them expensive and time-consuming. Because of this, researchers tend to have difficulty recruiting participants, leading to smaller sample sizes.
Large sample size needed
Longitudinal studies tend to be challenging to conduct because large samples are needed for any relationships or patterns to be meaningful. Researchers are unable to generate results if there is not enough data.
Participants tend to drop out
Not only is it a struggle to recruit participants, but subjects also tend to leave or drop out of the study due to various reasons such as illness, relocation, or a lack of motivation to complete the full study.
This tendency is known as selective attrition and can threaten the validity of an experiment. For this reason, researchers using this approach typically recruit many participants, expecting a substantial number to drop out before the end.
Report bias is possible
Longitudinal studies will sometimes rely on surveys and questionnaires, which could result in inaccurate reporting as there is no way to verify the information presented.
- Data were collected for each child at three-time points: at 11 months after adoption, at 4.5 years of age and at 10.5 years of age. The first two sets of results showed that the adoptees were behind the non-institutionalised group however by 10.5 years old there was no difference between the two groups. The Romanian orphans had caught up with the children raised in normal Canadian families.
- The role of positive psychology constructs in predicting mental health and academic achievement in children and adolescents (Marques Pais-Ribeiro, & Lopez, 2011)
- The correlation between dieting behavior and the development of bulimia nervosa (Stice et al., 1998)
- The stress of educational bottlenecks negatively impacting students’ wellbeing (Cruwys, Greenaway, & Haslam, 2015)
- The effects of job insecurity on psychological health and withdrawal (Sidney & Schaufeli, 1995)
- The relationship between loneliness, health, and mortality in adults aged 50 years and over (Luo et al., 2012)
- The influence of parental attachment and parental control on early onset of alcohol consumption in adolescence (Van der Vorst et al., 2006)
- The relationship between religion and health outcomes in medical rehabilitation patients (Fitchett et al., 1999)
Goals of Longitudinal Data and Longitudinal Research
The objectives of longitudinal data collection and research as outlined by Baltes and Nesselroade (1979):
- Identify intraindividual change : Examine changes at the individual level over time, including long-term trends or short-term fluctuations. Requires multiple measurements and individual-level analysis.
- Identify interindividual differences in intraindividual change : Evaluate whether changes vary across individuals and relate that to other variables. Requires repeated measures for multiple individuals plus relevant covariates.
- Analyze interrelationships in change : Study how two or more processes unfold and influence each other over time. Requires longitudinal data on multiple variables and appropriate statistical models.
- Analyze causes of intraindividual change: This objective refers to identifying factors or mechanisms that explain changes within individuals over time. For example, a researcher might want to understand what drives a person’s mood fluctuations over days or weeks. Or what leads to systematic gains or losses in one’s cognitive abilities across the lifespan.
- Analyze causes of interindividual differences in intraindividual change : Identify mechanisms that explain within-person changes and differences in changes across people. Requires repeated data on outcomes and covariates for multiple individuals plus dynamic statistical models.
How to Perform a Longitudinal Study
When beginning to develop your longitudinal study, you must first decide if you want to collect your own data or use data that has already been gathered.
Using already collected data will save you time, but it will be more restricted and limited than collecting it yourself. When collecting your own data, you can choose to conduct either a retrospective or prospective study .
In a retrospective study, you are collecting data on events that have already occurred. You can examine historical information, such as medical records, in order to understand the past. In a prospective study, on the other hand, you are collecting data in real-time. Prospective studies are more common for psychology research.
Once you determine the type of longitudinal study you will conduct, you then must determine how, when, where, and on whom the data will be collected.
A standardized study design is vital for efficiently measuring a population. Once a study design is created, researchers must maintain the same study procedures over time to uphold the validity of the observation.
A schedule should be maintained, complete results should be recorded with each observation, and observer variability should be minimized.
Researchers must observe each subject under the same conditions to compare them. In this type of study design, each subject is the control.
Methodological Considerations
Important methodological considerations include testing measurement invariance of constructs across time, appropriately handling missing data, and using accelerated longitudinal designs that sample different age cohorts over overlapping time periods.
Testing measurement invariance
Testing measurement invariance involves evaluating whether the same construct is being measured in a consistent, comparable way across multiple time points in longitudinal research.
This includes assessing configural, metric, and scalar invariance through confirmatory factor analytic approaches. Ensuring invariance gives more confidence when drawing inferences about change over time.
Missing data
Missing data can occur during initial sampling if certain groups are underrepresented or fail to respond.
Attrition over time is the main source – participants dropping out for various reasons. The consequences of missing data are reduced statistical power and potential bias if dropout is nonrandom.
Handling missing data appropriately in longitudinal studies is critical to reducing bias and maintaining power.
It is important to minimize attrition by tracking participants, keeping contact info up to date, engaging them, and providing incentives over time.
Techniques like maximum likelihood estimation and multiple imputation are better alternatives to older methods like listwise deletion. Assumptions about missing data mechanisms (e.g., missing at random) shape the analytic approaches taken.
Accelerated longitudinal designs
Accelerated longitudinal designs purposefully create missing data across age groups.
Accelerated longitudinal designs strategically sample different age cohorts at overlapping periods. For example, assessing 6th, 7th, and 8th graders at yearly intervals would cover 6-8th grade development over a 3-year study rather than following a single cohort over that timespan.
This increases the speed and cost-efficiency of longitudinal data collection and enables the examination of age/cohort effects. Appropriate multilevel statistical models are required to analyze the resulting complex data structure.
In addition to those considerations, optimizing the time lags between measurements, maximizing participant retention, and thoughtfully selecting analysis models that align with the research questions and hypotheses are also vital in ensuring robust longitudinal research.
So, careful methodology is key throughout the design and analysis process when working with repeated-measures data.
Cohort effects
A cohort refers to a group born in the same year or time period. Cohort effects occur when different cohorts show differing trajectories over time.
Cohort effects can bias results if not accounted for, especially in accelerated longitudinal designs which assume cohort equivalence.
Detecting cohort effects is important but can be challenging as they are confounded with age and time of measurement effects.
Cohort effects can also interfere with estimating other effects like retest effects. This happens because comparing groups to estimate retest effects relies on cohort equivalence.
Overall, researchers need to test for and control cohort effects which could otherwise lead to invalid conclusions. Careful study design and analysis is required.
Retest effects
Retest effects refer to gains in performance that occur when the same or similar test is administered on multiple occasions.
For example, familiarity with test items and procedures may allow participants to improve their scores over repeated testing above and beyond any true change.
Specific examples include:
- Memory tests – Learning which items tend to be tested can artificially boost performance over time
- Cognitive tests – Becoming familiar with the testing format and particular test demands can inflate scores
- Survey measures – Remembering previous responses can bias future responses over multiple administrations
- Interviews – Comfort with the interviewer and process can lead to increased openness or recall
To estimate retest effects, performance of retested groups is compared to groups taking the test for the first time. Any divergence suggests inflated scores due to retesting rather than true change.
If unchecked in analysis, retest gains can be confused with genuine intraindividual change or interindividual differences.
This undermines the validity of longitudinal findings. Thus, testing and controlling for retest effects are important considerations in longitudinal research.
Data Analysis
Longitudinal data involves repeated assessments of variables over time, allowing researchers to study stability and change. A variety of statistical models can be used to analyze longitudinal data, including latent growth curve models, multilevel models, latent state-trait models, and more.
Latent growth curve models allow researchers to model intraindividual change over time. For example, one could estimate parameters related to individuals’ baseline levels on some measure, linear or nonlinear trajectory of change over time, and variability around those growth parameters. These models require multiple waves of longitudinal data to estimate.
Multilevel models are useful for hierarchically structured longitudinal data, with lower-level observations (e.g., repeated measures) nested within higher-level units (e.g., individuals). They can model variability both within and between individuals over time.
Latent state-trait models decompose the covariance between longitudinal measurements into time-invariant trait factors, time-specific state residuals, and error variance. This allows separating stable between-person differences from within-person fluctuations.
There are many other techniques like latent transition analysis, event history analysis, and time series models that have specialized uses for particular research questions with longitudinal data. The choice of model depends on the hypotheses, timescale of measurements, age range covered, and other factors.
In general, these various statistical models allow investigation of important questions about developmental processes, change and stability over time, causal sequencing, and both between- and within-person sources of variability. However, researchers must carefully consider the assumptions behind the models they choose.
Longitudinal vs. Cross-Sectional Studies
Longitudinal studies and cross-sectional studies are two different observational study designs where researchers analyze a target population without manipulating or altering the natural environment in which the participants exist.
Yet, there are apparent differences between these two forms of study. One key difference is that longitudinal studies follow the same sample of people over an extended period of time, while cross-sectional studies look at the characteristics of different populations at a given moment in time.
Longitudinal studies tend to require more time and resources, but they can be used to detect cause-and-effect relationships and establish patterns among subjects.
On the other hand, cross-sectional studies tend to be cheaper and quicker but can only provide a snapshot of a point in time and thus cannot identify cause-and-effect relationships.
Both studies are valuable for psychologists to observe a given group of subjects. Still, cross-sectional studies are more beneficial for establishing associations between variables, while longitudinal studies are necessary for examining a sequence of events.
1. Are longitudinal studies qualitative or quantitative?
Longitudinal studies are typically quantitative. They collect numerical data from the same subjects to track changes and identify trends or patterns.
However, they can also include qualitative elements, such as interviews or observations, to provide a more in-depth understanding of the studied phenomena.
2. What’s the difference between a longitudinal and case-control study?
Case-control studies compare groups retrospectively and cannot be used to calculate relative risk. Longitudinal studies, though, can compare groups either retrospectively or prospectively.
In case-control studies, researchers study one group of people who have developed a particular condition and compare them to a sample without the disease.
Case-control studies look at a single subject or a single case, whereas longitudinal studies are conducted on a large group of subjects.
3. Does a longitudinal study have a control group?
Yes, a longitudinal study can have a control group . In such a design, one group (the experimental group) would receive treatment or intervention, while the other group (the control group) would not.
Both groups would then be observed over time to see if there are differences in outcomes, which could suggest an effect of the treatment or intervention.
However, not all longitudinal studies have a control group, especially observational ones and not testing a specific intervention.
Baltes, P. B., & Nesselroade, J. R. (1979). History and rationale of longitudinal research. In J. R. Nesselroade & P. B. Baltes (Eds.), (pp. 1–39). Academic Press.
Cook, N. R., & Ware, J. H. (1983). Design and analysis methods for longitudinal research. Annual review of public health , 4, 1–23.
Fitchett, G., Rybarczyk, B., Demarco, G., & Nicholas, J.J. (1999). The role of religion in medical rehabilitation outcomes: A longitudinal study. Rehabilitation Psychology, 44, 333-353.
Harvard Second Generation Study. (n.d.). Harvard Second Generation Grant and Glueck Study. Harvard Study of Adult Development. Retrieved from https://www.adultdevelopmentstudy.org.
Le Mare, L., & Audet, K. (2006). A longitudinal study of the physical growth and health of postinstitutionalized Romanian adoptees. Pediatrics & child health, 11 (2), 85-91.
Luo, Y., Hawkley, L. C., Waite, L. J., & Cacioppo, J. T. (2012). Loneliness, health, and mortality in old age: a national longitudinal study. Social science & medicine (1982), 74 (6), 907–914.
Marques, S. C., Pais-Ribeiro, J. L., & Lopez, S. J. (2011). The role of positive psychology constructs in predicting mental health and academic achievement in children and adolescents: A two-year longitudinal study. Journal of Happiness Studies: An Interdisciplinary Forum on Subjective Well-Being, 12( 6), 1049–1062.
Sidney W.A. Dekker & Wilmar B. Schaufeli (1995) The effects of job insecurity on psychological health and withdrawal: A longitudinal study, Australian Psychologist, 30: 1,57-63.
Stice, E., Mazotti, L., Krebs, M., & Martin, S. (1998). Predictors of adolescent dieting behaviors: A longitudinal study. Psychology of Addictive Behaviors, 12 (3), 195–205.
Tegan Cruwys, Katharine H Greenaway & S Alexander Haslam (2015) The Stress of Passing Through an Educational Bottleneck: A Longitudinal Study of Psychology Honours Students, Australian Psychologist, 50:5, 372-381.
Thomas, L. (2020). What is a longitudinal study? Scribbr. Retrieved from https://www.scribbr.com/methodology/longitudinal-study/
Van der Vorst, H., Engels, R. C. M. E., Meeus, W., & Deković, M. (2006). Parental attachment, parental control, and early development of alcohol use: A longitudinal study. Psychology of Addictive Behaviors, 20 (2), 107–116.
Further Information
- Schaie, K. W. (2005). What can we learn from longitudinal studies of adult development?. Research in human development, 2 (3), 133-158.
- Caruana, E. J., Roman, M., Hernández-Sánchez, J., & Solli, P. (2015). Longitudinal studies. Journal of thoracic disease, 7 (11), E537.

Related Articles
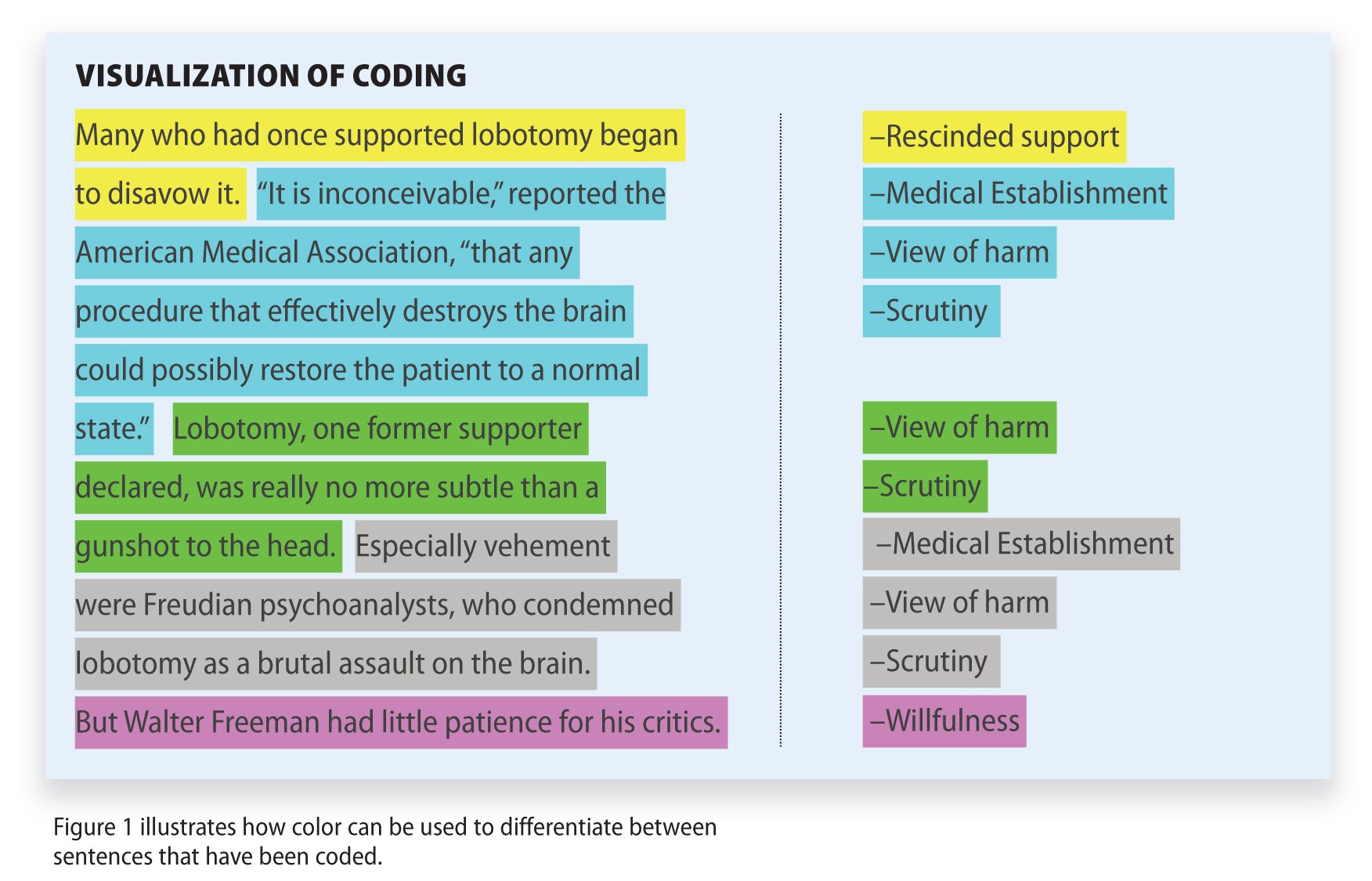
Research Methodology
Qualitative Data Coding
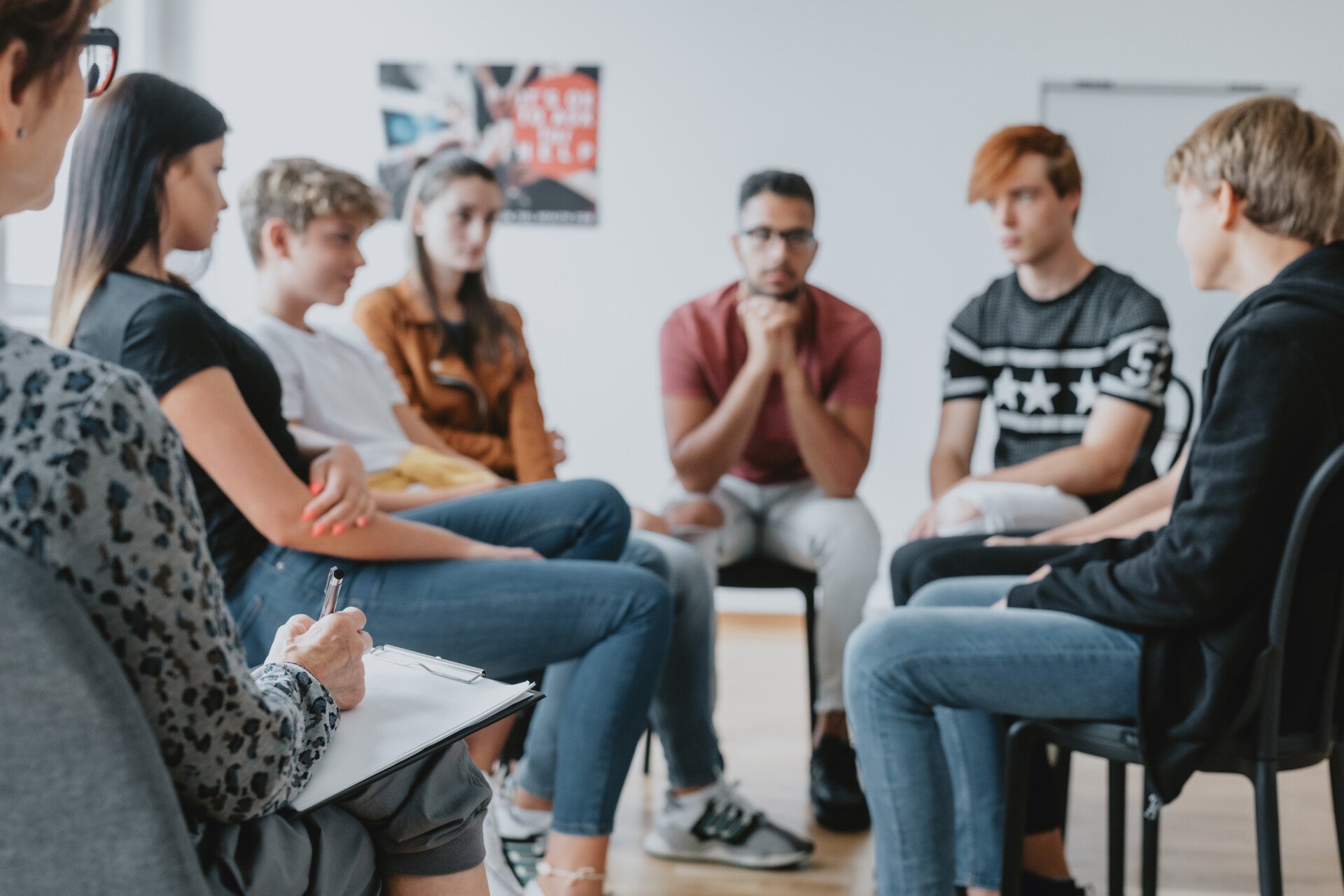
What Is a Focus Group?
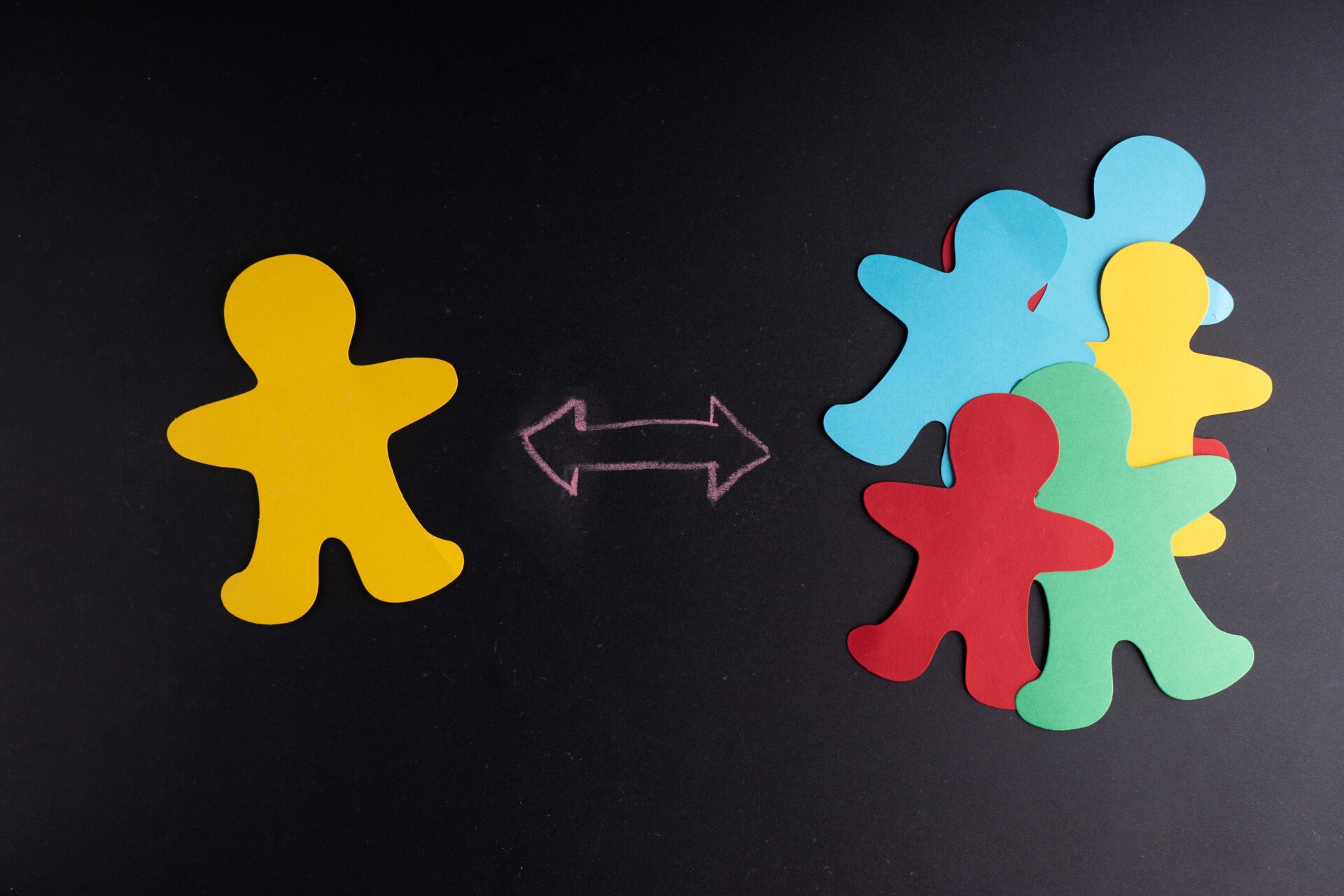
Cross-Cultural Research Methodology In Psychology
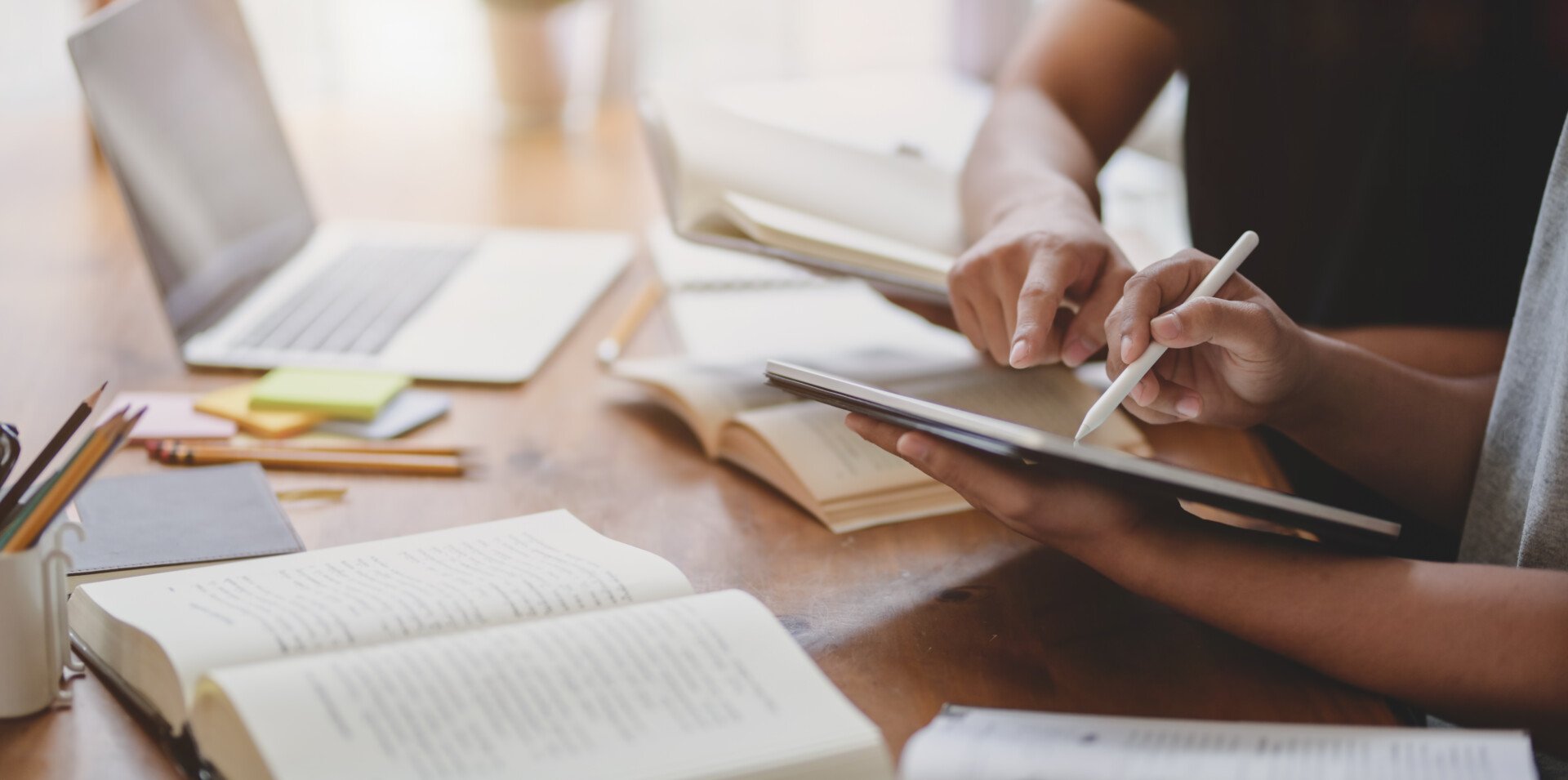
What Is Internal Validity In Research?
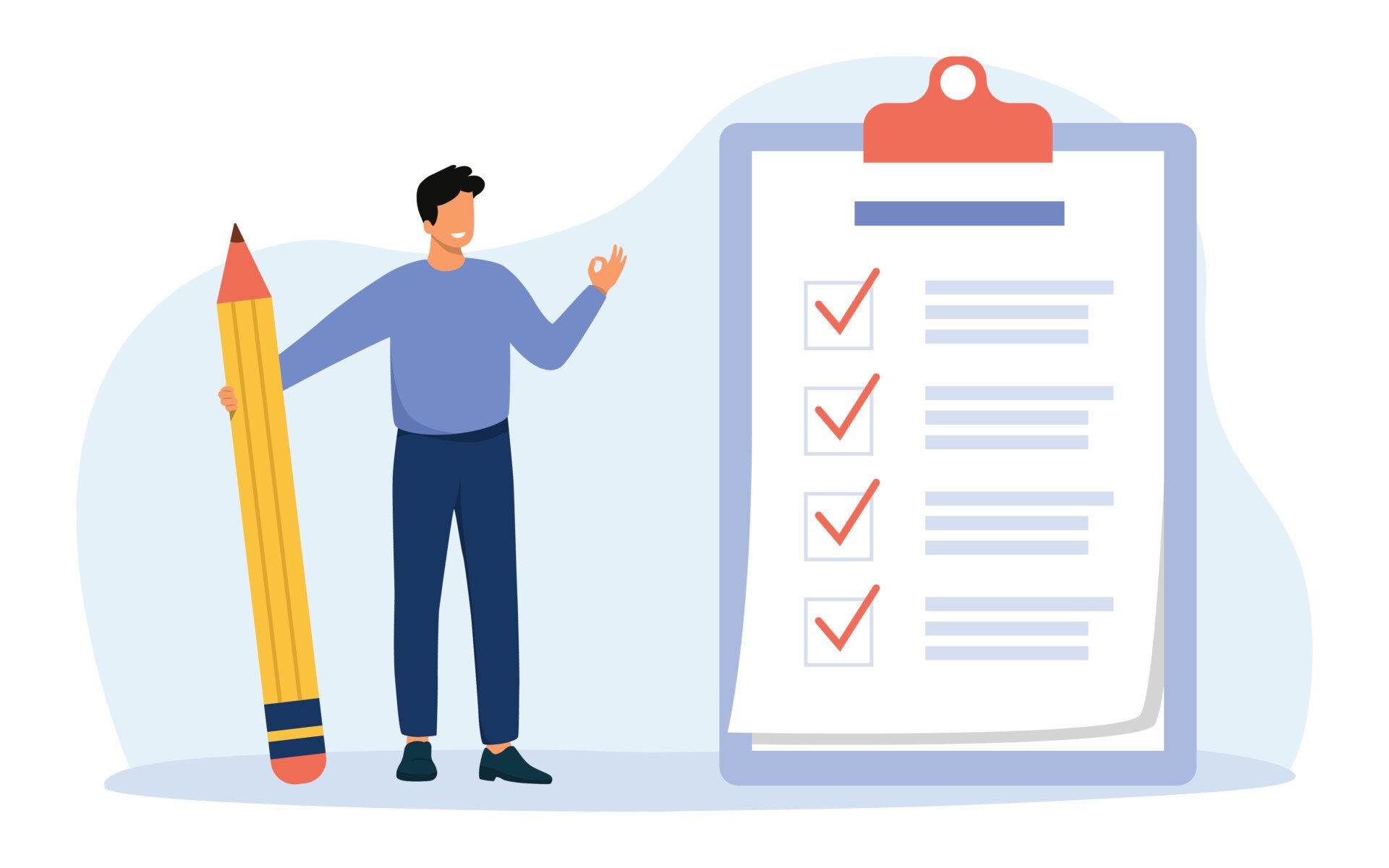
Research Methodology , Statistics
What Is Face Validity In Research? Importance & How To Measure
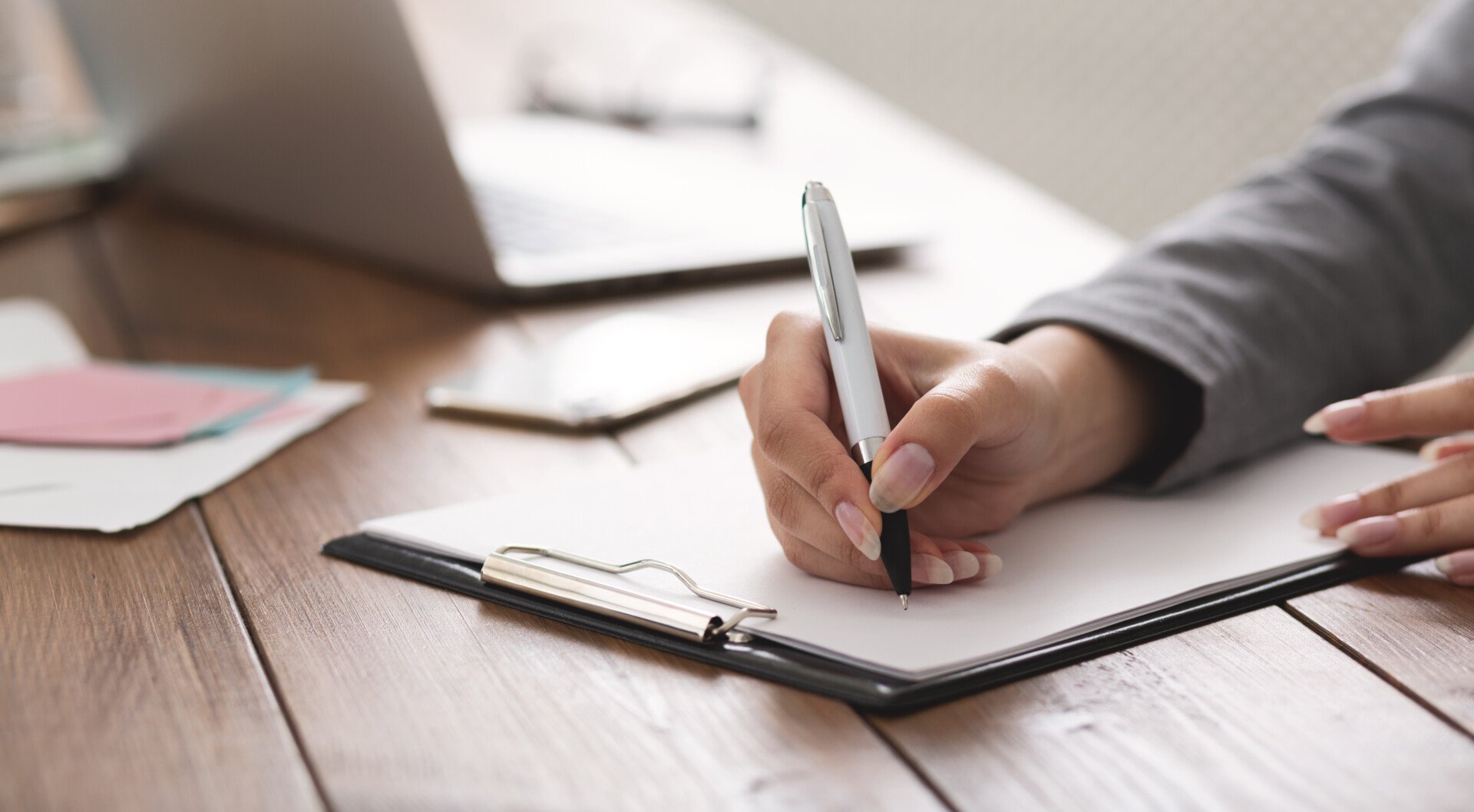
Criterion Validity: Definition & Examples
Have a language expert improve your writing
Run a free plagiarism check in 10 minutes, automatically generate references for free.
- Knowledge Base
- Methodology
- Longitudinal Study | Definition, Approaches & Examples
Longitudinal Study | Definition, Approaches & Examples
Published on 5 May 2022 by Lauren Thomas . Revised on 24 October 2022.
In a longitudinal study, researchers repeatedly examine the same individuals to detect any changes that might occur over a period of time.
Longitudinal studies are a type of correlational research in which researchers observe and collect data on a number of variables without trying to influence those variables.
While they are most commonly used in medicine, economics, and epidemiology, longitudinal studies can also be found in the other social or medical sciences.
Table of contents
How long is a longitudinal study, longitudinal vs cross-sectional studies, how to perform a longitudinal study, advantages and disadvantages of longitudinal studies, frequently asked questions about longitudinal studies.
No set amount of time is required for a longitudinal study, so long as the participants are repeatedly observed. They can range from as short as a few weeks to as long as several decades. However, they usually last at least a year, oftentimes several.
One of the longest longitudinal studies, the Harvard Study of Adult Development , has been collecting data on the physical and mental health of a group of men in Boston, in the US, for over 80 years.
Prevent plagiarism, run a free check.
The opposite of a longitudinal study is a cross-sectional study. While longitudinal studies repeatedly observe the same participants over a period of time, cross-sectional studies examine different samples (or a ‘cross-section’) of the population at one point in time. They can be used to provide a snapshot of a group or society at a specific moment.
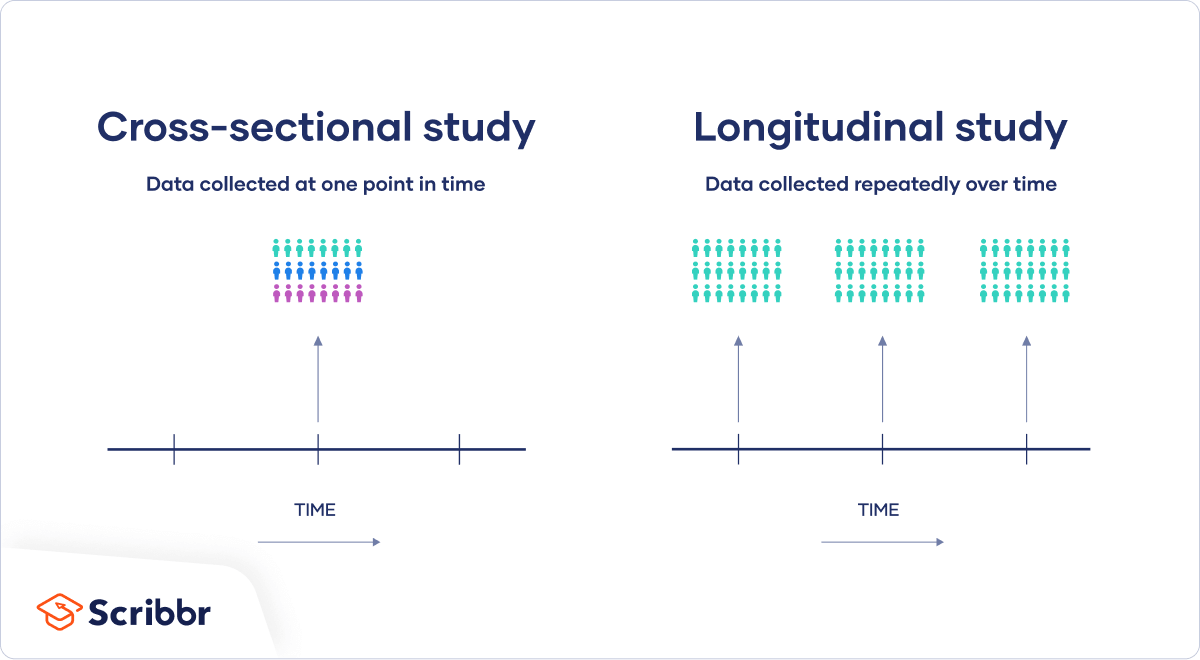
Both types of study can prove useful in research. Because cross-sectional studies are shorter and therefore cheaper to carry out, they can be used to discover correlations that can then be investigated in a longitudinal study.
If you want to implement a longitudinal study, you have two choices: collecting your own data or using data already gathered by somebody else.
Using data from other sources
Many governments or research centres carry out longitudinal studies and make the data freely available to the general public. For example, anyone can access data from the 1970 British Cohort Study, which has followed the lives of 17,000 Brits since their births in a single week in 1970, through the UK Data Service website .
These statistics are generally very trustworthy and allow you to investigate changes over a long period of time. However, they are more restrictive than data you collect yourself. To preserve the anonymity of the participants, the data collected is often aggregated so that it can only be analysed on a regional level. You will also be restricted to whichever variables the original researchers decided to investigate.
If you choose to go down this route, you should carefully examine the source of the dataset as well as what data are available to you.
Collecting your own data
If you choose to collect your own data, the way you go about it will be determined by the type of longitudinal study you choose to perform. You can choose to conduct a retrospective or a prospective study.
- In a retrospective study , you collect data on events that have already happened.
- In a prospective study , you choose a group of subjects and follow them over time, collecting data in real time.
Retrospective studies are generally less expensive and take less time than prospective studies, but they are more prone to measurement error.
Like any other research design , longitudinal studies have their trade-offs: they provide a unique set of benefits, but also come with some downsides.
Longitudinal studies allow researchers to follow their subjects in real time. This means you can better establish the real sequence of events, allowing you insight into cause-and-effect relationships.
Longitudinal studies also allow repeated observations of the same individual over time. This means any changes in the outcome variable cannot be attributed to differences between individuals.
Prospective longitudinal studies eliminate the risk of recall bias , or the inability to correctly recall past events.
Disadvantages
Longitudinal studies are time-consuming and often more expensive than other types of studies, so they require significant commitment and resources to be effective.
Since longitudinal studies repeatedly observe subjects over a period of time, any potential insights from the study can take a while to be discovered.
Attrition, which occurs when participants drop out of a study, is common in longitudinal studies and may result in invalid conclusions.
Longitudinal studies and cross-sectional studies are two different types of research design . In a cross-sectional study you collect data from a population at a specific point in time; in a longitudinal study you repeatedly collect data from the same sample over an extended period of time.
Longitudinal studies can last anywhere from weeks to decades, although they tend to be at least a year long.
The 1970 British Cohort Study , which has collected data on the lives of 17,000 Brits since their births in 1970, is one well-known example of a longitudinal study .
Longitudinal studies are better to establish the correct sequence of events, identify changes over time, and provide insight into cause-and-effect relationships, but they also tend to be more expensive and time-consuming than other types of studies.
Cite this Scribbr article
If you want to cite this source, you can copy and paste the citation or click the ‘Cite this Scribbr article’ button to automatically add the citation to our free Reference Generator.
Thomas, L. (2022, October 24). Longitudinal Study | Definition, Approaches & Examples. Scribbr. Retrieved 3 June 2024, from https://www.scribbr.co.uk/research-methods/longitudinal-study-design/
Is this article helpful?
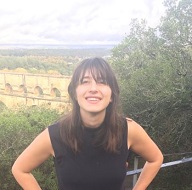
Lauren Thomas
Other students also liked, correlational research | guide, design & examples, a quick guide to experimental design | 5 steps & examples, descriptive research design | definition, methods & examples.
- Bipolar Disorder
- Therapy Center
- When To See a Therapist
- Types of Therapy
- Best Online Therapy
- Best Couples Therapy
- Best Family Therapy
- Managing Stress
- Sleep and Dreaming
- Understanding Emotions
- Self-Improvement
- Healthy Relationships
- Student Resources
- Personality Types
- Guided Meditations
- Verywell Mind Insights
- 2024 Verywell Mind 25
- Mental Health in the Classroom
- Editorial Process
- Meet Our Review Board
- Crisis Support
What Is a Longitudinal Study?
Tracking Variables Over Time
Kendra Cherry, MS, is a psychosocial rehabilitation specialist, psychology educator, and author of the "Everything Psychology Book."
:max_bytes(150000):strip_icc():format(webp)/IMG_9791-89504ab694d54b66bbd72cb84ffb860e.jpg)
Amanda Tust is a fact-checker, researcher, and writer with a Master of Science in Journalism from Northwestern University's Medill School of Journalism.
:max_bytes(150000):strip_icc():format(webp)/Amanda-Tust-1000-ffe096be0137462fbfba1f0759e07eb9.jpg)
Steve McAlister / The Image Bank / Getty Images
The Typical Longitudinal Study
Potential pitfalls, frequently asked questions.
A longitudinal study follows what happens to selected variables over an extended time. Psychologists use the longitudinal study design to explore possible relationships among variables in the same group of individuals over an extended period.
Once researchers have determined the study's scope, participants, and procedures, most longitudinal studies begin with baseline data collection. In the days, months, years, or even decades that follow, they continually gather more information so they can observe how variables change over time relative to the baseline.
For example, imagine that researchers are interested in the mental health benefits of exercise in middle age and how exercise affects cognitive health as people age. The researchers hypothesize that people who are more physically fit in their 40s and 50s will be less likely to experience cognitive declines in their 70s and 80s.
Longitudinal vs. Cross-Sectional Studies
Longitudinal studies, a type of correlational research , are usually observational, in contrast with cross-sectional research . Longitudinal research involves collecting data over an extended time, whereas cross-sectional research involves collecting data at a single point.
To test this hypothesis, the researchers recruit participants who are in their mid-40s to early 50s. They collect data related to current physical fitness, exercise habits, and performance on cognitive function tests. The researchers continue to track activity levels and test results for a certain number of years, look for trends in and relationships among the studied variables, and test the data against their hypothesis to form a conclusion.
Examples of Early Longitudinal Study Design
Examples of longitudinal studies extend back to the 17th century, when King Louis XIV periodically gathered information from his Canadian subjects, including their ages, marital statuses, occupations, and assets such as livestock and land. He used the data to spot trends over the years and understand his colonies' health and economic viability.
In the 18th century, Count Philibert Gueneau de Montbeillard conducted the first recorded longitudinal study when he measured his son every six months and published the information in "Histoire Naturelle."
The Genetic Studies of Genius (also known as the Terman Study of the Gifted), which began in 1921, is one of the first studies to follow participants from childhood into adulthood. Psychologist Lewis Terman's goal was to examine the similarities among gifted children and disprove the common assumption at the time that gifted children were "socially inept."
Types of Longitudinal Studies
Longitudinal studies fall into three main categories.
- Panel study : Sampling of a cross-section of individuals
- Cohort study : Sampling of a group based on a specific event, such as birth, geographic location, or experience
- Retrospective study : Review of historical information such as medical records
Benefits of Longitudinal Research
A longitudinal study can provide valuable insight that other studies can't. They're particularly useful when studying developmental and lifespan issues because they allow glimpses into changes and possible reasons for them.
For example, some longitudinal studies have explored differences and similarities among identical twins, some reared together and some apart. In these types of studies, researchers tracked participants from childhood into adulthood to see how environment influences personality , achievement, and other areas.
Because the participants share the same genetics , researchers chalked up any differences to environmental factors . Researchers can then look at what the participants have in common and where they differ to see which characteristics are more strongly influenced by either genetics or experience. Note that adoption agencies no longer separate twins, so such studies are unlikely today. Longitudinal studies on twins have shifted to those within the same household.
As with other types of psychology research, researchers must take into account some common challenges when considering, designing, and performing a longitudinal study.
Longitudinal studies require time and are often quite expensive. Because of this, these studies often have only a small group of subjects, which makes it difficult to apply the results to a larger population.
Selective Attrition
Participants sometimes drop out of a study for any number of reasons, like moving away from the area, illness, or simply losing motivation . This tendency, known as selective attrition , shrinks the sample size and decreases the amount of data collected.
If the final group no longer reflects the original representative sample , attrition can threaten the validity of the experiment. Validity refers to whether or not a test or experiment accurately measures what it claims to measure. If the final group of participants doesn't represent the larger group accurately, generalizing the study's conclusions is difficult.
The World’s Longest-Running Longitudinal Study
Lewis Terman aimed to investigate how highly intelligent children develop into adulthood with his "Genetic Studies of Genius." Results from this study were still being compiled into the 2000s. However, Terman was a proponent of eugenics and has been accused of letting his own sexism , racism , and economic prejudice influence his study and of drawing major conclusions from weak evidence. However, Terman's study remains influential in longitudinal studies. For example, a recent study found new information on the original Terman sample, which indicated that men who skipped a grade as children went on to have higher incomes than those who didn't.
A Word From Verywell
Longitudinal studies can provide a wealth of valuable information that would be difficult to gather any other way. Despite the typical expense and time involved, longitudinal studies from the past continue to influence and inspire researchers and students today.
A longitudinal study follows up with the same sample (i.e., group of people) over time, whereas a cross-sectional study examines one sample at a single point in time, like a snapshot.
A longitudinal study can occur over any length of time, from a few weeks to a few decades or even longer.
That depends on what researchers are investigating. A researcher can measure data on just one participant or thousands over time. The larger the sample size, of course, the more likely the study is to yield results that can be extrapolated.
Piccinin AM, Knight JE. History of longitudinal studies of psychological aging . Encyclopedia of Geropsychology. 2017:1103-1109. doi:10.1007/978-981-287-082-7_103
Terman L. Study of the gifted . In: The SAGE Encyclopedia of Educational Research, Measurement, and Evaluation. 2018. doi:10.4135/9781506326139.n691
Sahu M, Prasuna JG. Twin studies: A unique epidemiological tool . Indian J Community Med . 2016;41(3):177-182. doi:10.4103/0970-0218.183593
Almqvist C, Lichtenstein P. Pediatric twin studies . In: Twin Research for Everyone . Elsevier; 2022:431-438.
Warne RT. An evaluation (and vindication?) of Lewis Terman: What the father of gifted education can teach the 21st century . Gifted Child Q. 2018;63(1):3-21. doi:10.1177/0016986218799433
Warne RT, Liu JK. Income differences among grade skippers and non-grade skippers across genders in the Terman sample, 1936–1976 . Learning and Instruction. 2017;47:1-12. doi:10.1016/j.learninstruc.2016.10.004
Wang X, Cheng Z. Cross-sectional studies: Strengths, weaknesses, and recommendations . Chest . 2020;158(1S):S65-S71. doi:10.1016/j.chest.2020.03.012
Caruana EJ, Roman M, Hernández-Sánchez J, Solli P. Longitudinal studies . J Thorac Dis . 2015;7(11):E537-E540. doi:10.3978/j.issn.2072-1439.2015.10.63
By Kendra Cherry, MSEd Kendra Cherry, MS, is a psychosocial rehabilitation specialist, psychology educator, and author of the "Everything Psychology Book."
- Search Menu
- Sign in through your institution
- Advance articles
- Author Guidelines
- Submission Site
- Open Access
- Call for Papers
- Why publish with Work, Aging and Retirement?
- About Work, Aging and Retirement
- Editorial Board
- Advertising and Corporate Services
- Journals Career Network
- Self-Archiving Policy
- Dispatch Dates
- Journals on Oxford Academic
- Books on Oxford Academic

Article Contents
Questions on conceptual issues, questions on research design, questions on statistical techniques, acknowledgments, longitudinal research: a panel discussion on conceptual issues, research design, and statistical techniques.
All authors contributed equally to this article and the order of authorship is arranged arbitrarily. Correspondence concerning this article should be addressed to Mo Wang, Warrington College of Business, Department of Management, University of Florida, Gainesville, FL 32611. E-mail: [email protected]
Decision Editor: Donald Truxillo, PhD
- Article contents
- Figures & tables
- Supplementary Data
Mo Wang, Daniel J. Beal, David Chan, Daniel A. Newman, Jeffrey B. Vancouver, Robert J. Vandenberg, Longitudinal Research: A Panel Discussion on Conceptual Issues, Research Design, and Statistical Techniques, Work, Aging and Retirement , Volume 3, Issue 1, 1 January 2017, Pages 1–24, https://doi.org/10.1093/workar/waw033
- Permissions Icon Permissions
The goal of this article is to clarify the conceptual, methodological, and practical issues that frequently emerge when conducting longitudinal research, as well as in the journal review process. Using a panel discussion format, the current authors address 13 questions associated with 3 aspects of longitudinal research: conceptual issues, research design, and statistical techniques. These questions are intentionally framed at a general level so that the authors could address them from their diverse perspectives. The authors’ perspectives and recommendations provide a useful guide for conducting and reviewing longitudinal studies in work, aging, and retirement research.
An important meta-trend in work, aging, and retirement research is the heightened appreciation of the temporal nature of the phenomena under investigation and the important role that longitudinal study designs play in understanding them (e.g., Heybroek, Haynes, & Baxter, 2015 ; Madero-Cabib, Gauthier, & Le Goff, 2016 ; Wang, 2007 ; Warren, 2015 ; Weikamp & Göritz, 2015 ). This echoes the trend in more general research on work and organizational phenomena, where the discussion of time and longitudinal designs has evolved from explicating conceptual and methodological issues involved in the assessment of changes over time (e.g., McGrath & Rotchford, 1983 ) to the development and application of data analytic techniques (e.g., Chan, 1998 ; Chan & Schmitt, 2000 ; DeShon, 2012 ; Liu, Mo, Song, & Wang, 2016 ; Wang & Bodner, 2007 ; Wang & Chan, 2011 ; Wang, Zhou, & Zhang, 2016 ), theory rendering (e.g., Ancona et al. , 2001 ; Mitchell & James, 2001 ; Vancouver, Tamanini, & Yoder, 2010 ; Wang et al. , 2016 ), and methodological decisions in conducting longitudinal research (e.g., Beal, 2015 ; Bolger, Davis, & Rafaeli, 2003 ; Ployhart & Vandenberg, 2010 ). Given the importance of and the repeated call for longitudinal studies to investigate work, aging, and retirement-related phenomena (e.g., Fisher, Chaffee, & Sonnega, 2016 ; Wang, Henkens, & van Solinge, 2011 ), there is a need for more nontechnical discussions of the relevant conceptual and methodological issues. Such discussions would help researchers to make more informed decisions about longitudinal research and to conduct studies that would both strengthen the validity of inferences and avoid misleading interpretations.
In this article, using a panel discussion format, the authors address 13 questions associated with three aspects of longitudinal research: conceptual issues, research design, and statistical techniques. These questions, as summarized in Table 1 , are intentionally framed at a general level (i.e., not solely in aging-related research), so that the authors could address them from diverse perspectives. The goal of this article is to clarify the conceptual, methodological, and practical issues that frequently emerge in the process of conducting longitudinal research, as well as in the related journal review process. Thus, the authors’ perspectives and recommendations provide a useful guide for conducting and reviewing longitudinal studies—not only those dealing with aging and retirement, but also in the broader fields of work and organizational research.
Questions Regarding Longitudinal Research Addressed in This Article
Conceptual Issue Question 1: Conceptually, what is the essence of longitudinal research?
This is a fundamental question to ask given the confusion in the literature. It is common to see authors attribute their high confidence in their causal inferences to the longitudinal design they use. It is also common to see authors attribute greater confidence in their measurement because of using a longitudinal design. Less common, but with increasing frequency, authors claim to be examining the role of time in their theoretical models via the use of longitudinal designs. These different assumptions by authors illustrate the need for clarifying when specific attributions about longitudinal research are appropriate. Hence, a discussion of the essence of longitudinal research and what it provides is in order.
Oddly, definitions of longitudinal research are rare. One exception is a definition by Taris (2000) , who explained that longitudinal “data are collected for the same set of research units (which might differ from the sampling units/respondents) for (but not necessarily at) two or more occasions, in principle allowing for intra-individual comparison across time” (pp. 1–2). Perhaps more directly relevant for the current discussion of longitudinal research related to work and aging phenomena, Ployhart and Vandenberg (2010) defined “ longitudinal research as research emphasizing the study of change and containing at minimum three repeated observations (although more than three is better) on at least one of the substantive constructs of interest” (p. 97; italics in original). Compared to Taris (2000) , Ployhart and Vandenberg’s (2010) definition explicitly emphasizes change and encourages the collection of many waves of repeated measures. However, Ployhart and Vandenberg’s definition may be overly restrictive. For example, it precludes designs often classified as longitudinal such as the prospective design. In a prospective design, some criterion (i.e., presumed effect) is measured at Times 1 and 2, so that one can examine change in the criterion as a function of events (i.e., presumed causes) happening (or not) between the waves of data collection. For example, a researcher can use this design to assess the psychological and behavioral effects of retirement that occur before and after retirement. That is, psychological and behavioral variables are measured before and after retirement. Though not as internally valid as an experiment (which is not possible because we cannot randomly assign participants into retirement and non-retirement conditions), this prospective design is a substantial improvement over the typical design where the criteria are only measured at one time. This is because it allows one to more directly examine change in a criterion as a function of differences between events or person variables. Otherwise, one must draw inferences based on retrospective accounts of the change in criterion along with the retrospective accounts of the events; further, one may worry that the covariance between the criterion and person variables is due to changes in the criterion that are also changing the person. Of course, this design does not eliminate the possibility that changes in criterion may cause differences in events (e.g., changes observed in psychological and behavioral variables lead people to decide to retire).
In addition to longitudinal designs potentially having only two waves of data collection for a variable, there are certain kinds of criterion variables that need only one explicit measure at Time 2 in a 2-wave study. Retirement (or similarly, turnover) is an example. I say “explicit” because retirement is implicitly measured at Time 1. That is, if the units are in the working sample at Time 1, they have not retired. Thus, retirement at Time 2 represents change in working status. On the other hand, if retirement intentions is the criterion variable, repeated measures of this variable are important for assessing change. Repeated measures also enable the simultaneous assessment of change in retirement intentions and its alleged precursors; it could be that a variable like job satisfaction (a presumed cause of retirement intentions) is actually lowered after the retirement intentions are formed, perhaps in a rationalization process. That is, individuals first intend to retire and then evaluate over time their attitudes toward their present job. This kind of reverse causality process would not be detected in a design measuring job satisfaction at Time 1 and retirement intentions at Time 2.
Given the above, I opt for a much more straightforward definition of longitudinal research. Specifically, longitudinal research is simply research where data are collected over a meaningful span of time. A difference between this definition and the one by Taris (2000) is that this definition does not include the clause about examining intra-individual comparisons. Such designs can examine intra-individual comparisons, but again, this seems overly restrictive. That said, I do add a restriction to this definition, which is that the time span should be “meaningful.” This term is needed because time will always pass—that is, it takes time to complete questionnaires, do tasks, or observe behavior, even in cross-sectional designs. Yet, this passage of time likely provides no validity benefit. On the other hand, the measurement interval could last only a few seconds and still be meaningful. To be meaningful it has to support the inferences being made (i.e., improve the research’s validity). Thus, the essence of longitudinal research is to improve the validity of one’s inferences that cannot otherwise be achieved using cross-sectional research ( Shadish, Cook, & Campbell, 2002 ). The inferences that longitudinal research can potentially improve include those related to measurement (i.e., construct validity), causality (i.e., internal validity), generalizability (i.e., external validity), and quality of effect size estimates and hypothesis tests (i.e., statistical conclusion validity). However, the ability of longitudinal research to improve these inferences will depend heavily on many other factors, some of which might make the inferences less valid when using a longitudinal design. Increased inferential validity, particularly of any specific kind (e.g., internal validity), is not an inherent quality of the longitudinal design; it is a goal of the design. And it is important to know how some forms of the longitudinal design fall short of that goal for some inferences.
For example, consider a case where a measure of a presumed cause precedes a measure of a presumed effect, but over a time period across which one of the constructs in question does not likely change. Indeed, it is often questionable as to whether a gap of several months between the observations of many variables examined in research would change meaningfully over the interim, much less that the change in one preceded the change in the other (e.g., intention to retire is an example of this, as people can maintain a stable intention to retire for years). Thus, the design typically provides no real improvement in terms of internal validity. On the other hand, it does likely improve construct and statistical conclusion validity because it likely reduces common method bias effects found between the two variables ( Podsakoff et al., 2003 ).
Further, consider the case of the predictive validity design, where a selection instrument is measured from a sample of job applicants and performance is assessed some time later. In this case, common method bias is not generally the issue; external validity is. The longitudinal design improves external validity because the Time 1 measure is taken during the application process, which is the context in which the selection instrument will be used, and the Time 2 measure is taken after a meaningful time interval (i.e., after enough time has passed for performance to have stabilized for the new job holders). Again, however, internal validity is not much improved, which is fine given that prediction, not cause, is the primary concern in the selection context.
Another clear construct validity improvement gained by using longitudinal research is when one is interested in measuring change. A precise version of change measurement is assessing rate of change. When assessing the rate, time is a key variable in the analysis. To assess a rate one needs only two repeated measures of the variable of interest, though these measures should be taken from several units (e.g., individuals, groups, organizations) if measurement and sampling errors are present and perhaps under various conditions if systematic measurement error is possible (e.g., testing effect). Moreover, Ployhart and Vandenberg (2010) advocate at least three repeated measures because most change rates are not constant; thus, more than two observations will be needed to assess whether and how the rate changes (i.e., the shape of the growth curves). Indeed, three is hardly enough given noise in measurement and the commonality of complex processes (i.e., consider the opponent process example below).
Longitudinal research designs can, with certain precautions, improve one’s confidence in inferences about causality. When this is the purpose, time does not need to be measured or included as a variable in the analysis, though the interval between measurements should be reported because rate of change and cause are related. For example, intervals can be too short, such that given the rate of an effect, the cause might not have had sufficient time to register on the effect. Alternatively, if intervals are too long, an effect might have triggered a compensating process that overshoots the original level, inverting the sign of the cause’s effect. An example of this latter process is opponent process ( Solomon & Corbit, 1974 ). Figure 1 depicts this process, which refers to the response to an emotional stimulus. Specifically, the emotional response elicits an opponent process that, at its peak, returns the emotion back toward the baseline and beyond. If the emotional response is collected when peak opponent response occurs, it will look like the stimulus is having the opposite effect than it actually is having.

The opponent process effect demonstrated by Solomon and Corbit (1974) .
Most of the longitudinal research designs that improve internal validity are quasi-experimental ( Shadish et al. , 2002 ). For example, interrupted time series designs use repeated observations to assess trends before and after some manipulation or “natural experiment” to model possible maturation or maturation-by-selection effects ( Shadish et al. , 2002 ; Stone-Romero, 2010 ). Likewise, regression discontinuous designs (RDD) use a pre-test to assign participants to the conditions prior to the manipulation and thus can use the pre-test value to model selection effects ( Shadish et al. , 2002 ; Stone-Romero, 2010 ). Interestingly, the RDD design is not assessing change explicitly and thus is not susceptible to maturations threats, but it uses the timing of measurement in a meaningful way.
Panel (i.e., cohort) designs are also typically considered longitudinal. These designs measure all the variables of interest during each wave of data collection. I believe it was these kinds of designs that Ployhart and Vandenberg (2010) had in mind when they created their definition of longitudinal research. In particular, these designs can be used to assess rates of change and can improve causal inferences if done well. In particular, to improve causal inferences with panel designs, researchers nearly always need at least three repeated measures of the hypothesized causes and effects. Consider the case of job satisfaction and intent to retire. If a researcher measures job satisfaction and intent to retire at Times 1 and 2 and finds that the Time 2 measures of job satisfaction and intent to retire are negatively related when the Time 1 states of the variables are controlled, the researcher still cannot tell which changed first (or if some third variable causes both to change in the interim). Unfortunately, three observations of each variable is only a slight improvement because it might be a difficult thing to get enough variance in changing attitudes and changing intentions with just three waves to find anything significant. Indeed, the researcher might have better luck looking at actual retirement, which as mentioned, only needs one observation. Still, two observations of job satisfaction are needed prior to the retirement to determine if changes in job satisfaction influence the probability of retirement.
Finally, on this point I would add that meaningful variance in time will often mean case-intensive designs (i.e., lots of observations of lots of variables over time per case; Bolger & Laurenceau, 2013 ; Wang et al. , 2016 ) because we will be more and more interested in assessing feedback and other compensatory processes, reciprocal relationships, and how dynamic variables change. In these cases, within-unit covariance will be much more interesting than between-unit covariance.
It is important to point out that true experimental designs are also a type of longitudinal research design by nature. This is because in experimental design, an independent variable is manipulated before the measure of the dependent variable occurs. This time precedence (or lag) is critical for using experimental designs to achieve stronger causal inferences. Specifically, given that random assignment is used to generate experimental and control groups, researchers can assume that prior to the manipulation, the mean levels of the dependent variables are the same across experimental and control groups, as well as the mean levels of the independent variables. Thus, by measuring the dependent variable after manipulation, an experimental design reveals the change in the dependent variable as a function of change in the independent variable as a result of manipulation. As such, the time lag between the manipulation and the measure of the dependent variable is indeed meaningful in the sense of achieving causal inference.
Conceptual Issue Question 2: What is the status of “time” in longitudinal research? Is “time” a general notion of the temporal dynamics in phenomena, or is “time” a substantive variable similar to other focal variables in the longitudinal study?
In longitudinal research, we are concerned with conceptualizing and assessing the changes over time that may occur in one or more substantive variables. A substantive variable refers to a measure of an intended construct of interest in the study. For example, in a study of newcomer adaptation (e.g., Chan & Schmitt, 2000 ), the substantive variables, whose changes over time we are interested in tracking, could be frequency of information seeking, job performance, and social integration. We could examine the functional form of the substantive variable’s change trajectory (e.g., linear or quadratic). We could also examine the extent to which individual differences in a growth parameter of the trajectory (e.g., the individual slopes of a linear trajectory) could be predicted from the initial (i.e., at Time 1 of the repeated measurement) values on the substantive variable, the values on a time-invariant predictor (e.g., personality trait), or the values on another time-varying variable (e.g., individual slopes of the linear trajectory of a second substantive variable in the study). The substantive variables are measures used to represent the study constructs. As measures of constructs, they have specific substantive content. We can assess the construct validity of the measure by obtaining relevant validity evidence. The evidence could be the extent to which the measure’s content represents the conceptual content of the construct (i.e., content validity) or the extent to which the measure is correlated with another established criterion measure representing a criterion construct that, theoretically, is expected to be associated with the measure (i.e., criterion-related validity).
“Time,” on the other hand, has a different ontological status from the substantive variables in the longitudinal study. There are at least three ways to describe how time is not a substantive variable similar to other focal variables in the longitudinal study. First, when a substantive construct is tracked in a longitudinal study for changes over time, time is not a substantive measure of a study construct. In the above example of newcomer adaptation study by Chan and Schmitt, it is not meaningful to speak of assessing the construct validity of time, at least not in the same way we can speak of assessing the construct validity of job performance or social integration measures. Second, in a longitudinal study, a time point in the observation period represents one temporal instance of measurement. The time point per se, therefore, is simply the temporal marker of the state of the substantive variable at the point of measurement. The time point is not the state or value of the substantive variable that we are interested in for tracking changes over time. Changes over time occur when the state of a substantive variable changes over different points of measurement. Finally, in a longitudinal study of changes over time, “time” is distinct from the substantive process that underlies the change over time. Consider a hypothetical study that repeatedly measured the levels of job performance and social integration of a group of newcomers for six time points, at 1-month intervals between adjacent time points over a 6-month period. Let us assume that the study found that the observed change over time in their job performance levels was best described by a monotonically increasing trajectory at a decreasing rate of change. The observed functional form of the performance trajectory could serve as empirical evidence for the theory that a learning process underlies the performance level changes over time. Let us further assume that, for the same group of newcomers, the observed change over time in their social integration levels was best described by a positive linear trajectory. This observed functional form of the social integration trajectory could serve as empirical evidence for a theory of social adjustment process that underlies the integration level changes over time. In this example, there are two distinct substantive processes of change (learning and social adjustment) that may underlie the changes in levels on the two respective study constructs (performance and social integration). There are six time points at which each substantive variable was measured over the same time period. Time, in this longitudinal study, was simply the medium through which the two substantive processes occur. Time was not an explanation. Time did not cause the occurrence of the different substantive processes and there was nothing in the conceptual content of the time construct that could, nor was expected to, explain the functional form or nature of the two different substantive processes. The substantive processes occur or unfold through time but they did not cause time to exist.
The way that growth modeling techniques analyze longitudinal data is consistent with the above conceptualization of time. For example, in latent growth modeling, time per se is not represented as a substantive variable in the analysis. Instead, a specific time point is coded as a temporal marker of the substantive variable (e.g., as basis coefficients in a latent growth model to indicate the time points in the sequence of repeated measurement at which the substantive variable was measured). The time-varying nature of the substantive variable is represented either at the individual level as the individual slopes or at the group level as the variance of the slope factor. It is the slopes and variance of slopes of the substantive variable that are being analyzed, and not time per se. The nature of the trajectory of change in the substantive variable is descriptively represented by the specific functional form of the trajectory that is observed within the time period of study. We may also include in the latent growth model other substantive variables, such as time-invariant predictors or time-varying correlates, to assess the strength of their associations with variance of the individual slopes of trajectory. These associations serve as validation and explanation of the substantive process of change in the focal variable that is occurring over time.
Many theories of change require the articulation of a change construct (e.g., learning, social adjustment—inferred from a slope parameter in a growth model). When specifying a change construct, the “time” variable is only used as a marker to track a substantive growth or change process. For example, when we say, “Extraversion × time interaction effect” on newcomer social integration, we really mean that Extraversion relates to the change construct of social adjustment (i.e., where social adjustment is operationalized as the slope parameter from a growth model of individuals’ social integration over time). Likewise, when we say, “Conscientiousness × time2 quadratic interaction effect” on newcomer task performance, we really mean that Conscientiousness relates to the change construct of learning (where learning is operationalized as the nonlinear slope of task performance over time).
This view of time brings up a host of issues with scaling and calibration of the time variable to adequately assess the underlying substantive change construct. For example, should work experience be measured in number of years in the job versus number of assignments completed ( Tesluk & Jacobs, 1998 )? Should the change construct be thought of as a developmental age effect, historical period effect, or birth cohort effect ( Schaie, 1965 )? Should the study of time in teams reflect developmental time rather than clock time, and thus be calibrated to each team’s lifespan ( Gersick, 1988 )? As such, although time is not a substantive variable itself in longitudinal research, it is important to make sure that the measurement of time matches the theory that specifies the change construct that is under study (e.g., aging, learning, adaptation, social adjustment).
I agree that time is typically not a substantive variable, but that it can serve as a proxy for substantive variables if the process is well-known. The example about learning by Chan is a case in point. Of course, well-known temporal processes are rare and I have often seen substantive power mistakenly given to time: For example, it is the process of oxidation, not the passage of time that is responsible for rust. However, there are instances where time plays a substantive role. For example, temporal discounting ( Ainslie & Haslam, 1992 ) is a theory of behavior that is dependent on time. Likewise, Vancouver, Weinhardt, and Schmidt’s (2010) theory of multiple goal pursuit involves time as a key substantive variable. To be sure, in that latter case the perception of time is a key mediator between time and its hypothetical effects on behavior, but time has an explicit role in the theory and thus should be considered a substantive variable in tests of the theory.
I was referring to objective time when explaining that time is not a substantive variable in longitudinal research and that it is instead the temporal medium through which a substantive process unfolds or a substantive variable changes its state. When we discuss theories of substantive phenomena or processes involving temporal constructs, such as temporal discounting, time urgency, or polychronicity related to multitasking or multiple goal pursuits, we are in fact referring to subjective time, which is the individual’s psychological experience of time. Subjective time constructs are clearly substantive variables. The distinction between objective time and subjective time is important because it provides conceptual clarity to the nature of the temporal phenomena and guides methodological choices in the study of time (for details, see Chan, 2014 ).
Conceptual Issue Question 3: What are the procedures, if any, for developing a theory of changes over time in longitudinal research? Given that longitudinal research purportedly addresses the limitations of cross-sectional research, can findings from cross-sectional studies be useful for the development of a theory of change?
To address this question, what follows is largely an application of some of the ideas presented by Mitchell and James (2001) and by Ployhart and Vandenberg (2010) in their respective publications. Thus, credit for the following should be given to those authors, and consultation of their articles as to specifics is highly encouraged.
Before we specifically address this question, it is important to understand our motive for asking it. Namely, as most succinctly stated by Mitchell and James (2001) , and repeated by, among others, Bentein and colleagues (2005) , Chan (2002 , 2010 ), and Ployhart and Vandenberg (2010) , there is an abundance of published research in the major applied psychology and organizational science journals in which the authors are not operationalizing through their research designs the causal relations among their focal independent, dependent, moderator, and mediator variables even though the introduction and discussion sections imply such causality. Mitchell and James (2001) used the published pieces in the most recent issues (at that time) of the Academy of Management Journal and Administrative Science Quarterly to anchor this point. At the crux of the problem is using designs in which time is not a consideration. As they stated so succinctly:
“At the simplest level, in examining whether an X causes a Y, we need to know when X occurs and when Y occurs. Without theoretical or empirical guides about when to measure X and Y, we run the risk of inappropriate measurement, analysis, and, ultimately, inferences about the strength, order, and direction of causal relationships (italics added, Mitchell & James, 2001 , p. 530).”
When is key because it is at the heart of causality in its simplest form, as in the “cause must precede the effect” ( James, Mulaik, & Brett, 1982 ; Condition 3 of 10 for inferring causality, p. 36). Our casual glance at the published literature over the decade since Mitchell and James (2001) indicates that not much has changed in this respect. Thus, our motive for asking the current question is quite simple—“perhaps it’s ‘time’ to put these issues in front of us once more (pun intended), particularly given the increasing criticisms as to the meaningfulness of published findings from studies with weak methods and statistics” (e.g., statistical myths and urban legends, Lance & Vandenberg, 2009 ).
The first part of the question asks, “what are the procedures, if any, for developing a theory of change over time in longitudinal research?” Before addressing procedures per se, it is necessary first to understand some of the issues when incorporating change into research. Doing so provides a context for the procedures. Ployhart and Vandenberg (2010) noted four theoretical issues that should be addressed when incorporating change in the variables of interest across time. These were:
“To the extent possible, specify a theory of change by noting the specific form and duration of change and predictors of change.
Clearly articulate or graph the hypothesized form of change relative to the observed form of change.
Clarify the level of change of interest: group average change, intraunit change, or interunit differences in intraunit change.
Realize that cross-sectional theory and research may be insufficient for developing theory about change. You need to focus on explaining why the change occurs” (p. 103).
The interested reader is encouraged to consult Ployhart and Vandenberg (2010) as to the specifics underlying the four issues, but they were heavily informed by Mitchell and James (2001) . Please note that, as one means of operationalizing time, Mitchell and James (2001) focused on time very broadly in the context of strengthening causal inferences about change across time in the focal variables. Thus, Ployhart and Vandenberg’s (2010) argument, with its sole emphasis on change, is nested within the Mitchell and James (2001) perspective. I raise this point because it is in this vein that the four theoretical issues presented above have as their foundation the five theoretical issues addressed by Mitchell and James (2001) . Specifically, first, we need to know the time lag between X and Y . How long after X occurs does Y occur? Second, X and Y have durations. Not all variables occur instantaneously. Third, X and Y may change over time. We need to know the rate of change. Fourth, in some cases we have dynamic relationships in which X and Y both change. The rate of change for both variables should be known, as well as how the X – Y relationship changes. Fifth, in some cases we have reciprocal causation: X causes Y and Y causes X . This situation requires an understanding of two sets of lags, durations, and possibly rates. The major point of both sets of authors is that these theoretical issues need to be addressed first in that they should be the key determinants in designing the overall study; that is, deciding upon the procedures to use.
Although Mitchell and James (2001 , see p. 543) focused on informing procedures through theory in the broader context of time (e.g., draw upon studies and research that may not be in our specific area of interest; going to the workplace and actually observing the causal sequence, etc.), our specific question focuses on change across time. In this respect, Ployhart and Vandenberg (2010 , Table 1 in p. 103) identified five methodological and five analytical procedural issues that should be informed by the nature of the change. These are:
“Methodological issues
1. Determine the optimal number of measurement occasions and their intervals to appropriately model the hypothesized form of change.
2. Whenever possible, choose samples most likely to exhibit the hypothesized form of change, and try to avoid convenience samples.
3. Determine the optimal number of observations, which in turn means addressing the attrition issue before conducting the study. Prepare for the worst (e.g., up to a 50% drop from the first to the last measurement occasion). In addition, whenever possible, try to model the hypothesized “cause” of missing data (ideally theorized and measured a priori) and consider planned missingness approaches to data collection.
4. Introduce time lags between intervals to address issues of causality, but ensure the lags are neither too long nor too short.
5. Evaluate the measurement properties of the variable for invariance (e.g., configural, metric) before testing whether change has occurred.
Analytical issues
1. Be aware of potential violations in statistical assumptions inherent in longitudinal designs (e.g., correlated residuals, nonindependence).
2. Describe how time is coded (e.g., polynomials, orthogonal polynomials) and why.
3. Report why you use a particular analytical method and its strengths and weaknesses for the particular study.
4. Report all relevant effect sizes and fit indices to sufficiently evaluate the form of change.
5. It is easy to ‘overfit’ the data; strive to develop a parsimonious representation of change.”
In summary, the major point from the above is to encourage researchers to develop a thorough conceptual understanding of time as it relates to defining the causal relationships between the focal variables of interest. We acknowledge that researchers are generally good at conceptualizing why their x -variables cause some impact on their y -variables. What is called for here goes beyond just understanding why, but forcing ourselves to be very specific about the timing between the variables. Doing so will result in stronger studies and ones in which our inferences from the findings can confidently include statements about causality—a level of confidence that is sorely lacking in most published studies today. As succinctly stated by Mitchell and James (2001) , “With impoverished theory about issues such as when events occur, when they change, or how quickly they change, the empirical researcher is in a quandary. Decisions about when to measure and how frequently to measure critical variables are left to intuition, chance, convenience, or tradition. None of these are particularly reliable guides (p. 533).”
The latter quote serves as a segue to address the second part of our question, “Given that longitudinal research purportedly addresses the limitations of cross-sectional research, can findings from cross-sectional studies be useful for the development of a theory of change?” Obviously, the answer here is “it depends.” In particular, it depends on the design contexts around which the cross-sectional study was developed. For example, if the study was developed strictly following many of the principles for designing quasi-experiments in field settings spelled out by Shadish, Cook, and Campbell (2002) , then it would be very useful for developing a theory of change on the phenomenon of interest. Findings from such studies could inform decisions as to how much change needs to occur across time in the independent variable to see measurable change in the dependent variable. Similarly, it would help inform decisions as to what the baseline on the independent variable needs to be, and what amount of change from this baseline is required to impact the dependent variable. Another useful set of cross-sectional studies would be those developed for the purpose of verifying within field settings the findings from a series of well-designed laboratory experiments. Again, knowing issues such as thresholds, minimal/maximal values, and intervals or timing of the x -variable onset would be very useful for informing a theory of change. A design context that would be of little use for developing a theory of change is the case where a single cross-sectional study was completed to evaluate the conceptual premises of interest. The theory underlying the study may be useful, but the findings themselves would be of little use.
Few theories are not theories of change. Most, however, are not sufficiently specified. That is, they leave much to the imagination. Moreover, they often leave to the imagination the implications of the theory on behavior. My personal bias is that theories of change should generally be computationally rendered to reduce vagueness, provide a test of internal coherence, and support the development of predictions. One immediately obvious conclusion one will draw when attempting to create a formal computational theoretical model is that we have little empirical data on rates of change.
The procedures for developing a computational model are the following ( Vancouver & Weinhardt, 2012 ; also see Wang et al. , 2016 ). First, take variables from (a) existing theory (verbal or static mathematical theory), (b) qualitative studies, (c) deductive reasoning, or (d) some combination of these. Second, determine which variables are dynamic. Dynamic variables have “memory” in that they retain their value over time, changing only as a function of processes that move the value in one direction or another at some rate or some changing rate. Third, describe processes that would affect these dynamic variables (if using existing theory, this likely involves other variables in the theory) or the rates and direction of change to the dynamic variables if the processes that affect the rates are beyond the theory. Fourth, represent formally (e.g., mathematically) the effect of the variables on each other. Fifth, simulate the model to see if it (a) works (e.g., no out-of-bounds values generated), (b) produces phenomena the theory is presumed to explain, (c) produces patterns of data over time (trajectories; relationships) that match (or could be matched to) data, and (d) determine if variance in exogenous variables (i.e., ones not presumably affected by other variables in the model) affect trajectories/relationships (called sensitivity analysis). For example, if we build a computational model to understand retirement timing, it will be critical to simulate the model to make sure that it generates predictions in a realistic way (e.g., the simulation should not generate too many cases where retirement happens after the person is a 90-year old). It will also be important to see whether the predictions generated from the model match the actual empirical data (e.g., the average retirement age based on simulation should match the average retirement age in the target population) and whether the predictions are robust when the model’s input factors take on a wide range of values.
As mentioned above, many theories of change require the articulation of a change construct (e.g., learning, aging, social adjustment—inferred from a slope parameter in a growth model). A change construct must be specified in terms of its: (a) theoretical content (e.g., what is changing, when we say “learning” or “aging”?), (b) form of change (linear vs. quadratic vs. cyclical), and (c) rate of change (does the change process meaningfully occur over minutes vs. weeks?). One salient problem is how to develop theory about the form of change (linear vs. nonlinear/quadratic) and the rate of change (how fast?) For instance, a quadratic/nonlinear time effect can be due to a substantive process of diminishing returns to time (e.g., a learning curve), or to ceiling (or floor) effects (i.e., hitting the high end of a measurement instrument, past which it becomes impossible to see continued growth in the latent construct). Indeed, only a small fraction of the processes we study would turn out to be linear if we used more extended time frames in the longitudinal design. That is, most apparently linear processes result from the researcher zooming in on a nonlinear process in a way that truncates the time frame. This issue is directly linked to the presumed rate of change of a phenomenon (e.g., a process that looks nonlinear in a 3-month study might look linear in a 3-week study). So when we are called upon to theoretically justify why we hypothesize a linear effect instead of a nonlinear effect, we must derive a theory of what the passage of time means. This would involve three steps: (a) naming the substantive process for which time is a marker (e.g., see answers to Question #2 above), (b) theorizing the rate of this process (e.g., over weeks vs. months), which will be more fruitful if it hinges on related past empirical longitudinal research, than if it hinges on armchair speculation about time (i.e., the appropriate theory development sequence here is: “past data → theory → new data,” and not simply, “theory → new data”; the empirical origins of theory are an essential step), and (c) disavowing nonlinear forces (e.g., diminishing returns to time, periodicity), within the chosen time frame of the study.
Research Design Question 1: What are some of the major considerations that one should take into account before deciding to employ a longitudinal study design?
As with all research, the design needs to allow the researcher to address the research question. For example, if one is seeking to assess a change rate, one needs to ask if it is safe to assume that the form of change is linear. If not, one will need more than two waves or will need to use continuous sampling. One might also use a computational model to assess whether violations of the linearity assumption are important. The researcher needs to also have an understanding of the likely time frame across which the processes being examined occur. Alternatively, if the time frame is unclear, the researcher should sample continuously or use short intervals. If knowing the form of the change is desired, then one will need enough waves of data collection in which to comprehensively capture the changes.
If one is interested in assessing causal processes, more issues need to be considered. For example, what are the processes of interest? What are the factors affecting the processes or the rates of the processes? What is the form of the effect of these factors? And perhaps most important, what alternative process could be responsible for effects observed?
For example, consider proactive socialization ( Morrison, 2002 ). The processes of interest are those involved in determining proactive information seeking. One observation is that the rate of proactive information seeking drops with the tenure of an employee ( Chan & Schmitt, 2000 ). Moreover, the form of the drop is asymptotic to a floor (Vancouver, Tamanini et al. , 2010 ). The uncertainty reduction model predicts that proactive information seeking will drop over time because knowledge increases (i.e., uncertainty decreases). An alternative explanation is that ego costs grow over time: One feels that they will look more foolish asking for information the longer one’s tenure ( Ashford, 1986 ). To distinguish these explanations for a drop in information seeking over time, one might want to look at whether the transparency of the reason to seek information would moderate the negative change trend of information seeking. For the uncertainty reduction model, transparency should not matter, but for the ego-based model, transparency and legitimacy of reason should matter. Of course, it might be that both processes are at work. As such, the researcher may need a computational model or two to help think through the effects of the various processes and whether the forms of the relationships depend on the processes hypothesized (e.g., Vancouver, Tamanini et al. , 2010 ).
Research Design Question 2: Are there any design advantages of cross-sectional research that might make it preferable to longitudinal research? That is, what would be lost and what might be gained if a moratorium were placed on cross-sectional research?
Cross-sectional research is easier to conduct than longitudinal research, but it often estimates the wrong parameters. Interestingly, researchers typically overemphasize/talk too much about the first fact (ease of cross-sectional research), and underemphasize/talk too little about the latter fact (that cross-sectional studies estimate the wrong thing). Cross-sectional research has the advantages of allowing broader sampling of participants, due to faster and cheaper studies that involve less participant burden; and broader sampling of constructs, due to the possibility of participant anonymity in cross-sectional designs, which permits more honest and complete measurement of sensitive concepts, like counterproductive work behavior.
Also, when the theoretical process at hand has a very short time frame (e.g., minutes or seconds), then cross-sectional designs can be entirely appropriate (e.g., for factor analysis/measurement modeling, because it might only take a moment for a latent construct to be reflected in a survey response). Also, first-stage descriptive models of group differences (e.g., sex differences in pay; cross-cultural differences in attitudes; and other “black box” models that do not specify a psychological process) can be suggestive even with cross-sectional designs. Cross-sectional research can also be condoned in the case of a 2-study design wherein cross-sectional data are supplemented with lagged/longitudinal data.
But in the end, almost all psychological theories are theories of change (at least implicitly) [Contrary to Ployhart and Vandenberg (2010) , I tend to believe that “cross-sectional theory” does not actually exist— theories are inherently longitudinal, whereas models and evidence can be cross-sectional.]. Thus, longitudinal and time-lagged designs are indispensable, because they allow researchers to begin answering four types of questions: (a) causal priority, (b) future prediction, (c) change, and (d) temporal external validity. To define and compare cross-sectional against longitudinal and time-lagged designs, I refer to Figure 2 . Figure 2 displays three categories of discrete-time designs: cross-sectional ( X and Y measured at same time; Figure 2a ), lagged ( Y measured after X by a delay of duration t ; Figure 2b ), and longitudinal ( Y measured at three or more points in time; Figure 2c ) designs. First note that, across all time designs, a 1 denotes the cross-sectional parameter (i.e., the correlation between X 1 and Y 1 ) . In other words, if X is job satisfaction and Y is retirement intentions, a 1 denotes the cross-sectional correlation between these two variables at t 1 . To understand the value (and limitations) of cross-sectional research, we will look at the role of the cross-sectional parameter ( a 1 ) in each of the Figure 2 models.

Time-based designs for two constructs, X and Y . (a) cross-sectional design (b) lagged designs (c) longitudinal designs.
For assessing causal priority , the lagged models and panel model are most relevant. The time-lagged b 1 parameter (i.e., correlation between X 1 and Y 2 ; e.g., predictive validity) aids in future prediction, but tells us little about causal priority. In contrast, the panel regression b 1 ' parameter from the cross-lagged panel regression (in Figure 2b ) and the cross-lagged panel model (in Figure 2c ) tells us more about causal priority from X to Y ( Kessler & Greenberg, 1981 ; Shingles, 1985 ), and is a function of the b 1 parameter and the cross-sectional a 1 parameter [ b 1 ' = ( b 1 − a 1 r Y 1 , Y 2 ) / 1 − a 1 2 ] . For testing theories that X begets Y (i.e., X → Y ), the lagged parameter b 1 ' can be extremely useful, whereas the cross-sectional parameter a 1 is the wrong parameter (indeed, a 1 is often negatively related to b 1 ' ) . That is, a 1 does not estimate X → Y , but it is usually negatively related to that estimate (via the above formula for b 1 ' ) . Using the example of job satisfaction and retirement intentions, if we would like to know about the causal priority from job satisfaction to retirement intentions, we should at least measure both job satisfaction and retirement intentions at t 1 and then measure retirement intentions at t 2 . Deriving the estimate for b 1 ' involves regressing retirement intentions at t 2 on job satisfaction at t 1 , while controlling for the effect of retirement intentions at t 1 .
For future prediction , the autoregressive model and growth model in Figure 2c are most relevant. One illustrative empirical phenomenon is validity degradation, which means the X – Y correlation tends to shrink as the time interval between X and Y increases ( Keil & Cortina, 2001 ). Validity degradation and patterns of stability have been explained via simplex autoregressive models ( Hulin, Henry, & Noon, 1990 ; Humphreys, 1968 ; Fraley, 2002 ), which express the X – Y correlation as r X 1 , Y 1 + k = a 1 g k , where k is the number of time intervals separating X and Y . Notice the cross-sectional parameter a 1 in this formula serves as a multiplicative constant in the time-lagged X – Y correlation, but is typically quite different from the time-lagged X – Y correlation itself. Using the example of extraversion and retirement intentions, validity degradation means that the effect of extraversion at t 1 on the measure of retirement intentions is likely to decrease over time, depending on how stable retirement intentions are. Therefore, relying on a 1 to gauge how well extraversion can predict future retirement intentions is likely to overestimate the predictive effect of extraversion.
Another pertinent model is the latent growth model ( Chan, 1998 ; Ployhart & Hakel, 1998 ), which explains longitudinal data using a time intercept and slope. In the linear growth model in Figure 2 , the cross-sectional a 1 parameter is equal to the relationship between X 1 and the Y intercept, when t 1 = 0. I also note that from the perspective of the growth model, the validity degradation phenomenon (e.g., Hulin et al. , 1990 ) simply means that X 1 has a negative relationship with the Y slope. Thus, again, the cross-sectional a 1 parameter merely indicates the initial state of the X and Y relationship in a longitudinal system, and will only offer a reasonable estimate of future prediction of Y under the rare conditions when g ≈ 1.0 in the autoregressive model (i.e., Y is extremely stable), or when i ≈ 0 in the growth model (i.e., X does not predict the Y -slope; Figure 2c ).
For studying change , I refer to the growth model (where both X and the Y -intercept explain change in Y [or Y -slope]) and the coupled growth model (where X -intercept, Y -intercept, change in X , and change in Y all interrelate) in Figure 2c . Again, in these models the cross-sectional a 1 parameter is the relationship between the X and Y intercepts, when the slopes are specified with time centered at t 1 = 0 (where t 1 refers arbitrarily to any time point when the cross-sectional data were collected). In the same way that intercepts tell us very little about slopes (ceiling and floor effects notwithstanding), the cross-sectional X 1 parameter tells us almost nothing about change parameters. Again, using the example of the job satisfaction and retirement intentions relationship, to understand change in retirement intentions over time, it is important to gauge the effects of initial status of job satisfaction (i.e., job satisfaction intercept) and change in job satisfaction (i.e., job satisfaction slope) on change in retirement intentions (i.e., slope of retirement intentions).
Finally, temporal external validity refers to the extent to which an effect observed at one point in time generalizes across other occasions. This includes longitudinal measurement equivalence (e.g., whether the measurement metric of the concept or the meaning of the concept may change over time; Schmitt, 1982 ), stability of bivariate relationships over time (e.g., job satisfaction relates more weakly to turnover when the economy is bad; Carsten & Spector, 1987 ), the stationarity of cross-lagged parameters across measurement occasions ( b 1 ' = b 2 ' , see cross-lagged panel model in Figure 2c ; e.g., Cole & Maxwell, 2003 ), and the ability to identify change as an effect of participant age/tenure/development—not an effect of birth/hire cohort or historical period ( Schaie, 1965 ). Obviously, cross-sectional data have nothing to say about temporal external validity.
Should there be a moratorium on cross-sectional research? Because any single wave of a longitudinal design is itself cross-sectional data, a moratorium is not technically possible. However, there should be (a) an explicit acknowledgement of the different theoretical parameters in Figure 2 , and (b) a general moratorium on treating the cross-sectional a 1 parameter as though it implies causal priority (cf. panel regression parameter b 1 ' ) , future prediction (cf. panel regression, autoregressive, and growth models), change (cf. growth models), or temporal external validity. This recommendation is tantamount to a moratorium on cross-sectional research papers, because almost all theories imply the lagged and/or longitudinal parameters in Figure 2 . As noted earlier, cross-sectional data are easier to get, but they estimate the wrong parameter.
I agree with Newman that most theories are about change or should be (i.e., we are interested in understanding processes and, of course, processes occur over time). I am also in agreement that cross-sectional designs are of almost no value for assessing theories of change. Therefore, I am interested in getting to a place where most research is longitudinal, and where top journals rarely publish papers with only a cross-sectional design. However, as Newman points out, some research questions can still be addressed using cross-sectional designs. Therefore, I would not support a moratorium on cross-sectional research papers.
Research Design Question 3: In a longitudinal study, how do we decide on the length of the interval between two adjacent time points?
This question needs to be addressed together with the question on how many time points of measurement to administer in a longitudinal study. It is well established that intra-individual changes cannot be adequately assessed with only two time points because (a) a two-point measurement by necessity produces a linear trajectory and therefore is unable to empirically detect the functional form of the true change trajectory and (b) time-related (random or correlated) measurement error and true change over time are confounded in the observed change in a two-point measurement situation (for details, see Chan, 1998 ; Rogosa, 1995 ; Singer & Willett, 2003 ). Hence, the minimum number of time points for assessing intra-individual change is three, but more than three is better to obtain a more reliable and valid assessment of the change trajectory ( Chan, 1998 ). However, it does not mean that a larger number of time points is always better or more accurate than a smaller number of time points. Given that the total time period of study captures the change process of interest, the number of time points should be determined by the appropriate location of the time point. This then brings us to the current practical question on the choice regarding the appropriate length of the interval between adjacent time points.
The correct length of the time interval between adjacent time points in a longitudinal study is critical because it directly affects the observed functional form of the change trajectory and in turn the inference we make about the true pattern of change over time ( Chan, 1998 ). What then should be the correct length of the time interval between adjacent time points in a longitudinal study? Put simply, the correct or optimal length of the time interval will depend on the specific substantive change phenomenon of interest. This means it is dependent on the nature of the substantive construct, its underlying process of change over time, and the context in which the change process is occurring which includes the presence of variables that influence the nature and rate of the change. In theory, the time interval for data collection is optimal when the time points are appropriately spaced in such a way that it allows the true pattern of change over time to be observed during the period of study. When the observed time interval is too short or too long as compared to the optimal time interval, true patterns of change will get masked or false patterns of change will get observed.
The problem is we almost never know what this optimal time interval is, even if we have a relatively sound theory of the change phenomenon. This is because our theories of research phenomena are often static in nature. Even when our theories are dynamic and focus on change processes, they are almost always silent on the specific length of the temporal dimension through which the substantive processes occur over time ( Chan, 2014 ).
In practice, researchers determine their choice of the length of the time interval in conjunction with the choice of number of time points and the choice of the length of the total time period of study. Based on my experiences as an author, reviewer, and editor, I suspect that these three choices are influenced by the specific resource constraints and opportunities faced by the researchers when designing and conducting the longitudinal study. Deviation from optimal time intervals probably occurs more frequently than we would like, since decisions on time intervals between measures in a study are often pragmatic and atheoretical. When we interpret findings from longitudinal studies, we should consider the possibility that the study may have produced patterns of results that led to wrong inferences because the study did not reflect the true changes over time.
Given that our theories of phenomena are not at the stage where we could specify the optimal time intervals, the best we could do now is to explicate the nature of the change processes and the effects of the influencing factors to serve as guides for decisions on time intervals, number of time points, and total time period of study. For example, in research on sense-making processes in newcomer adaptation, the total period of study often ranged from 6 months to 1 year, with 6 to 12 time points, equally spaced at time intervals of 1 or 2 months between adjacent time points. A much longer time interval and total time period, ranging from several months to several years, would be more appropriate for a change process that should take a longer time to manifest itself, such as development of cognitive processes or skill acquisition requiring extensive practice or accumulation of experiences over time. On the other extreme, a much shorter time interval and total time period, ranging from several hours to several days, will be appropriate for a change process that should take a short time to manifest itself such as activation or inhibition of mood states primed by experimentally manipulated events.
Research Design Question 4: As events occur in our daily life, our mental representations of these events may change as time passes. How can we determine the point(s) in time at which the representation of an event is appropriate? How can these issues be addressed through design and measurement in a study?
In some cases, longitudinal researchers will wish to know the nature and dynamics of one’s immediate experiences. In these cases, the items included at each point in time will simply ask participants to report on states, events, or behaviors that are relatively immediate in nature. For example, one might be interested in an employee’s immediate affective experiences, task performance, or helping behavior. This approach is particularly common for intensive, short-term longitudinal designs such as experience sampling methods (ESM; Beal & Weiss, 2003 ). Indeed, the primary objective of ESM is to capture a representative sample of points within one’s day to help understand the dynamic nature of immediate experience ( Beal, 2015 ; Csikszentmihalyi & Larson, 1987 ). Longitudinal designs that have longer measurement intervals may also capture immediate experiences, but more often will ask participants to provide some form of summary of these experiences, typically across the entire interval between each measurement occasion. For example, a panel design with a 6-month interval may ask participants to report on affective states, but include a time frame such as “since the last survey” or “over the past 6 months”, requiring participants to mentally aggregate their own experiences.
As one might imagine, there also are various designs and approaches that range between the end points of immediate experience and experiences aggregated over the entire interval. For example, an ESM study might examine one’s experiences since the last survey. These intervals obviously are close together in time, and therefore are conceptually similar to one’s immediate state; nevertheless, they do require both increased levels of recall and some degree of mental aggregation. Similarly, studies with a longer time interval (e.g., 6-months) might nevertheless ask about one’s relatively recent experiences (e.g., affect over the past week), requiring less in terms of recall and mental aggregation, but only partially covering the events of the entire intervening interval. As a consequence, these two approaches and the many variations in between form a continuum of abstraction containing a number of differences that are worth considering.
Differences in Stability
Perhaps the most obvious difference across this continuum of abstraction is that different degrees of aggregation are captured. As a result, items will reflect more or less stable estimates of the phenomenon of interest. Consider the hypothetical temporal break-down of helping behavior depicted in Figure 3 . No matter how unstable the most disaggregated level of helping behavior may appear, aggregations of these behaviors will always produce greater stability. So, asking about helping behavior over the last hour will produce greater observed variability (i.e., over the entire scale) than averages of helping behavior over the last day, week, month, or one’s overall general level. Although it is well-known that individuals do not follow a strict averaging process when asked directly about a higher level of aggregation (e.g., helping this week; see below), it is very unlikely that such deviations from a straight average will result in less stability at higher levels of aggregation.

Hypothetical variability of helping behavior at different levels of aggregation.
The reason why this increase in stability is likely to occur regardless of the actual process of mental aggregation is that presumably, as you move from shorter to longer time frames, you are estimating either increasingly stable aspects of an individual’s dispositional level of the construct, or increasingly stable features of the context (e.g., a consistent workplace environment). As you move from longer to shorter time frames you are increasingly estimating immediate instances of the construct or context that are influenced not only by more stable predictors, but also dynamic trends, cycles, and intervening events ( Beal & Ghandour, 2011 ). Notably, this stabilizing effect exists independently of the differences in memory and mental aggregation that are described below.
Differences in Memory
Fundamental in determining how people will respond to these different forms of questions is the nature of memory. Robinson and Clore (2002) provided an in-depth discussion of how we rely on different forms of memory when answering questions over different time frames. Although these authors focus on reports of emotion experiences, their conclusions are likely applicable to a much wider variety of self-reports. At one end of the continuum, reports of immediate experiences are direct, requiring only one’s interpretation of what is occurring and minimizing mental processes of recall.
Moving slightly down the continuum, we encounter items that ask about very recent episodes (e.g., “since the last survey” or “in the past 2 hours” in ESM studies). Here, Robinson and Clore (2002) note that we rely on what cognitive psychologists refer to as episodic memory. Although recall is involved, specific details of the episode in question are easily recalled with a high degree of accuracy. As items move further down the continuum toward summaries of experiences over longer periods of time (e.g., “since the last survey” in a longitudinal panel design), the details of particular relevant episodes are harder to recall and so responses are tinged to an increasing degree by semantic memory. This form of memory is based on individual characteristics (e.g., neurotic individuals might offer more negative reports) as well as well-learned situation-based knowledge (e.g., “my coworkers are generally nice people, so I’m sure that I’ve been satisfied with my interactions over this period of time”). Consequently, as the time frame over which people report increases, the nature of the information provided changes. Specifically, it is increasingly informed by semantic memory (i.e., trait and situation-based knowledge) and decreasingly informed by episodic memory (i.e., particular details of one’s experiences). Thus, researchers should be aware of the memory-related implications when they choose the time frame for their measures.
Differences in the Process of Summarizing
Aside from the role of memory in determining the content of these reports, individuals also summarize their experiences in a complex manner. For example, psychologists have demonstrated that even over a single episode, people tend not to base subjective summaries of the episode on its typical or average features. Instead, we focus on particular notable moments during the experience, such as its peak or its end state, and pay little attention to some aspects of the experience, such as its duration ( Fredrickson, 2000 ; Redelmeier & Kahneman, 1996 ). The result is that a mental summary of a given episode is unlikely to reflect actual averages of the experiences and events that make up the episode. Furthermore, when considering reports that span multiple episodes (e.g., over the last month or the interval between two measurements in a longitudinal panel study), summaries become even more complex. For example, recent evidence suggests that people naturally organize ongoing streams of experience into more coherent episodes largely on the basis of goal relevance ( Beal, Weiss, Barros, & MacDermid, 2005 ; Beal & Weiss, 2013 ; Zacks, Speer, Swallow, Braver, & Reynolds, 2007 ). Thus, how we interpret and parse what is going on around us connects strongly to our goals at the time. Presumably, this process helps us to impart meaning to our experiences and predict what might happen next, but it also influences the type of information we take with us from the episode, thereby affecting how we might report on this period of time.
Practical Differences
What then, can researchers take away from this information to help in deciding what sorts of items to include in longitudinal studies? One theme that emerges from the above discussion is that summaries over longer periods of time will tend to reflect more about the individual and the meanings he or she may have imparted to the experiences, events, and behaviors that have occurred during this time period, whereas shorter-term summaries or reports of more immediate occurrences are less likely to have been processed through this sort of interpretive filter. Of course, this is not to say that the more immediate end of this continuum is completely objective, as immediate perceptions are still host to many potential biases (e.g., attributional biases typically occur immediately); rather, immediate reports are more likely to reflect one’s immediate interpretation of events rather than an interpretation that has been mulled over and considered in light of an individual’s short- and long-term goals, dispositions, and broader worldview.
The particular choice of item type (i.e., immediate vs. aggregated experiences) that will be of interest to a researcher designing a longitudinal study should of course be determined by the nature of the research question. For example, if a researcher is interested in what Weiss and Cropanzano (1996) referred to as judgment-driven behaviors (e.g., a calculated decision to leave the organization), then capturing the manner in which individuals make sense of relevant work events is likely more appropriate, and so items that ask one to aggregate experiences over time may provide a better conceptual match than items asking about immediate states. In contrast, affect-driven behaviors or other immediate reactions to an event will likely be better served by reports that ask participants for minimal mental aggregations of their experiences (e.g., immediate or over small spans of time).
The issue of mental representations of events at particular points in time should always be discussed and evaluated within the research context of the conceptual questions on the underlying substantive constructs and change processes that may account for patterns of responses over time. Many of these conceptual questions are likely to relate to construct-oriented issues such as the location of the substantive construct on the state-trait continuum and the timeframe through which short-term or long-term effects on the temporal changes in the substantive construct are likely to be manifested (e.g., effects of stressors on changes in health). On the issue of aggregation of observations across time, I see it as part of a more basic question on whether an individual’s subjective experience on a substantive construct (e.g., emotional well-being) should be assessed using momentary measures (e.g., assessing the individual’s current emotional state, measured daily over the past 1 week) or retrospective global reports (e.g., asking the individual to report an overall assessment of his or her emotional state over the past 1 week). Each of the two measurement perspectives (i.e., momentary and global retrospective) has both strengths and limitations. For example, momentary measures are less prone to recall biases compared to global retrospective measures ( Kahneman, 1999 ). Global retrospective measures, on the other hand, are widely used in diverse studies for the assessment of many subjective experience constructs with a large database of evidence concerning the measure’s reliability and validity ( Diener, Inglehart, & Tay, 2013 ). In a recent article ( Tay, Chan, & Diener, 2014 ), my colleagues and I reviewed the conceptual, methodological, and practical issues in the debate between the momentary and global retrospective perspectives as applied to the research on subjective well-being. We concluded that both perspectives could offer useful insights and suggested a multiple-method approach that is sensitive to the nature of the substantive construct and specific context of use, but also called for more research on the use of momentary measures to obtain more evidence for their psychometric properties and practical value.
Research Design Question 5: What are the biggest practical hurdles to conducting longitudinal research? What are the ways to overcome them?
As noted earlier, practical hurdles are perhaps one of the main reasons why researchers choose cross-sectional rather than longitudinal designs. Although we have already discussed a number of these issues that must be faced when conducting longitudinal research, the following discussion emphasizes two hurdles that are ubiquitous, often difficult to overcome, and are particularly relevant to longitudinal designs.
Encouraging Continued Participation
Encouraging participation is a practical issue that likely faces all studies, irrespective of design; however, longitudinal studies raise special considerations given that participants must complete measurements on multiple occasions. Although there is a small literature that has examined this issue specifically (e.g., Fumagalli, Laurie, & Lynn, 2013 ; Groves et al. , 2006 ; Laurie, Smith, & Scott, 1999 ), it appears that the relevant factors are fairly similar to those noted for cross-sectional surveys. In particular, providing monetary incentives prior to completing the survey is a recommended strategy (though nonmonetary gifts can also be effective), with increased amounts resulting in increased participation rates, particularly as the burden of the survey increases ( Laurie & Lynn, 2008 ).
The impact of participant burden relates directly to the special considerations of longitudinal designs, as they are generally more burdensome. In addition, with longitudinal designs, the nature of the incentives used can vary over time, and can be tailored toward reducing attrition rates across the entire span of the survey ( Fumagalli et al. , 2013 ). For example, if the total monetary incentive is distributed across survey waves such that later waves have greater incentive amounts, and if this information is provided to participants at the outset of the study, then attrition rates may be reduced more effectively ( Martin & Loes, 2010 ); however, some research suggests that a larger initial payment is particularly effective at reducing attrition throughout the study ( Singer & Kulka, 2002 ).
In addition, the fact that longitudinal designs reflect an implicit relationship between the participant and the researchers over time suggests that incentive strategies that are considered less effective in cross-sectional designs (e.g., incentive contingent on completion) may be more effective in longitudinal designs, as the repeated assessments reflect a continuing reciprocal relationship. Indeed, there is some evidence that contingent incentives are effective in longitudinal designs ( Castiglioni, Pforr, & Krieger, 2008 ). Taken together, one potential strategy for incentivizing participants in longitudinal surveys would be to divide payment such that there is an initial relatively large incentive delivered prior to completing the first wave, followed by smaller, but increasing amounts that are contingent upon completion of each successive panel. Although this strategy is consistent with theory and evidence just discussed, it has yet to be tested explicitly.
Continued contact
One thing that does appear certain, particularly in longitudinal designs, is that incentives are only part of the picture. An additional factor that many researchers have emphasized is the need to maintain contact with participants throughout the duration of a longitudinal survey ( Laurie, 2008 ). Strategies here include obtaining multiple forms of contact information at the outset of the study and continually updating this information. From this information, researchers should make efforts to keep in touch with participants in-between measurement occasions (for panel studies) or some form of ongoing basis (for ESM or other intensive designs). Laurie (2008) referred to these efforts as Keeping In Touch Exercises (KITEs) and suggested that they serve to increase belongingness and perhaps a sense of commitment to the survey effort, and have the additional benefit of obtaining updated contact and other relevant information (e.g., change of job).
Mode of Data Collection
General considerations.
In panel designs, relative to intensive designs discussed below, only a limited number of surveys are sought, and the interval between assessments is relatively large. Consequently, there is likely to be greater flexibility as to the particular methods chosen for presenting and recording responses. Although the benefits, costs, and deficiencies associated with traditional paper-and-pencil surveys are well-known, the use of internet-based surveys has evolved rapidly and so the implications of using this method have also changed. For example, early survey design technologies for internet administration were often complex and potentially costly. Simply adding items was sometimes a difficult task, and custom-formatted response options (e.g., sliding scales with specific end points, ranges, and tick marks) were often unattainable. Currently available web-based design tools often are relatively inexpensive and increasingly customizable, yet have maintained or even improved the level of user-friendliness. Furthermore, a number of studies have noted that data collected using paper-and-pencil versus internet-based applications are often comparable if not indistinguishable (e.g., Cole, Bedeian, & Feild, 2006 ; Gosling et al. , 2004 ), though notable exceptions can occur ( Meade, Michels, & Lautenschlager, 2007 ).
One issue related to the use of internet-based survey methods that is likely to be of increasing relevance in the years to come is collection of survey data using a smartphone. As of this writing (this area changes rapidly), smartphone options are in a developing phase where some reasonably good options exist, but have yet to match the flexibility and standardized appearance that comes with most desktop or laptop web-based options just described. For example, it is possible to implement repeated surveys for a particular mobile operating system (OS; e.g., Apple’s iOS, Google’s Android OS), but unless a member of the research team is proficient in programming, there will be a non-negligible up-front cost for a software engineer ( Uy, Foo, & Aguinis, 2010 ). Furthermore, as market share for smartphones is currently divided across multiple mobile OSs, a comprehensive approach will require software development for each OS that the sample might use.
There are a few other options, however, but some of these options are not quite complete solutions. For example, survey administration tools such as Qualtrics now allow for testing of smartphone compatibility when creating web-based surveys. So, one could conceivably create a survey using this tool and have people respond to it on their smartphone with little or no loss of fidelity. Unfortunately, these tools (again, at this moment in time) do not offer elegant or flexible signaling capabilities. For example, intensive repeated measures designs will often try to signal reasonably large (e.g., N = 50–100) number of participants multiple random signals every day for multiple weeks. Accomplishing this task without the use of a built-in signaling function (e.g., one that generates this pattern of randomized signals and alerts each person’s smartphone at the appropriate time), is no small feat.
There are, however, several efforts underway to provide free or low-cost survey development applications for mobile devices. For example, PACO is a (currently) free Google app that is in the beta-testing stage and allows great flexibility in the design and implementation of repeated surveys on both Android OS and iOS smartphones. Another example that is currently being developed for both Android and iOS platforms is Expimetrics ( Tay, 2015 ), which promises flexible design and signaling functions that is of low cost for researchers collecting ESM data. Such applications offer the promise of highly accessible survey administration and signaling and have the added benefit of transmitting data quickly to servers accessible to the research team. Ideally, such advances in accessibility of survey administration will allow increased response rates throughout the duration of the longitudinal study.
Issues specific to intensive designs
All of the issues just discussed with respect to the mode of data collection are particularly relevant for short-term intensive longitudinal designs such as ESM. As the number of measurement occasions increases, so too do the necessities of increasing accessibility and reducing participant burden wherever possible. Of particular relevance is the emphasis ESM places on obtaining in situ assessments to increase the ecological validity of the study ( Beal, 2015 ). To maximize this benefit of the method, it is important to reduce the interruption introduced by the survey administration. If measurement frequency is relatively sparse (e.g., once a day), it is likely that simple paper-and-pencil or web-based modes of collection will be sufficient without creating too much interference ( Green et al. , 2006 ). In contrast, as measurements become increasingly intensive (e.g., four or five times/day or more), reliance on more accessible survey modes will become important. Thus, a format that allows for desktop, laptop, or smartphone administration should be of greatest utility in such intensive designs.
Statistical Techniques Question 1: With respect to assessing changes over time in a latent growth modeling framework, how can a researcher address different conceptual questions by coding the slope variable differently?
As with many questions in this article, an in-depth answer to this particular question is not possible in the available space. Hence, only a general treatment of different coding schemes of the slope or change variable is provided. Excellent detailed treatments of this topic may be found in Bollen and Curran (2006 , particularly chapters 3 & 4), and in Singer and Willett (2003 , particularly chapter 6). As noted by Ployhart and Vandenberg (2010) , specifying the form of change should be an a priori conceptual endeavor, not a post hoc data driven effort. This stance was also stated earlier by Singer and Willett (2003) when distinguishing between empirical (data driven) versus rational (theory driven) strategies. “Under rational strategies, on the other hand, you use theory to hypothesize a substantively meaningful functional form for the individual change trajectory. Although rational strategies generally yield clearer interpretations, their dependence on good theory makes them somewhat more difficult to develop and apply ( Singer & Willett, 2003 , p. 190).” The last statement in the quote simply reinforces the main theme throughout this article; that is, researchers need to undertake the difficult task of bringing in time (change being one form) within their conceptual frameworks in order to more adequately examine the causal structure among the focal variables within those frameworks.
In general, there are three sets of functional forms for which the slope or change variable may be coded or specified: (a) linear; (b) discontinuous; and (c) nonlinear. Sets emphasize that within each form there are different types that must be considered. The most commonly seen form in our literature is linear change (e.g., Bentein et al. , 2005 ; Vandenberg & Lance, 2000 ). Linear change means there is an expectation that the variable of interest should increase or decrease in a straight-line function during the intervals of the study. The simplest form of linear change occurs when there are equal measurement intervals across time and the units of observations were obtained at the same time in those intervals. Assuming, for example, that there were four occasions of measurement, the coding of the slope variable would be 0 (Time 1), 1 (Time 2), 2 (Time 3) and 3 (Time 4). Such coding fixes the intercept (starting value of the line) at the Time 1 interval, and thus, the conceptual interpretation of the linear change is made relative to this starting point. Reinforcing the notion that there is a set of considerations, one may have a conceptual reason for wanting to fix the intercept to the last measurement occasion. For example, there may be an extensive training program anchored with a “final exam” on the last occasion, and one wants to study the developmental process resulting in the final score. In this case, the coding scheme may be −3, −2, −1, and 0 going from Time 1 to Time 4, respectively ( Bollen & Curran, 2006 , p. 116; Singer & Willett, 2003 , p. 182). One may also have a conceptual reason to use the middle of the time intervals to anchor the intercept and look at the change above and below this point. Thus, the coding scheme in the current example may be −1.5, −0.5, 0.5, and 1.5 for Time 1 to Time 4, respectively ( Bollen & Curran, 2006 ; Singer & Willett, 2003 ). There are other considerations in the “linear set” such as the specification of linear change in cohort designs or other cases where there are individually-varying times of observation (i.e., not everyone started at the same time, at the same age, at the same intervals, etc.). The latter may need to make use of missing data procedures, or the use of time varying covariates that account for the differences as to when observations were collected. For example, to examine how retirement influences life satisfaction, Pinquart and Schindler (2007) modeled life satisfaction data from a representative sample of German retirees who retired between 1985 and 2003. Due to the retirement timing differences among the participants (not everyone retired at the same time or at the same age), different numbers of life satisfaction observations were collected for different retirees. Therefore, the missing observations on a yearly basis were modeled as latent variables to ensure that the analyses were able to cover the entire studied time span.
Discontinuous change is the second set of functional form with which one could theoretically describe the change in one’s substantive focal variables. Discontinuities are precipitous events that may cause the focal variable to rapidly accelerate (change in slope) or to dramatically increase/decrease in value (change in elevation) or both change in slope and elevation (see Ployhart & Vandenberg, 2010 , Figure 1 in p. 100; Singer & Willett, 2003 , pp. 190–208, see Table 6.2 in particular). For example, according to the stage theory ( Wang et al. , 2011 ), retirement may be such a precipitous event, because it can create an immediate “honeymoon effect” on retirees, dramatically increasing their energy-level and satisfaction with life as they pursue new activities and roles.
This set of discontinuous functional form has also been referred to as piecewise growth ( Bollen & Curran, 2006 ; Muthén & Muthén, 1998–2012 ), but in general, represents situations where all units of observation are collected at the same time during the time intervals and the discontinuity happens to all units at the same time. It is actually a variant of the linear set, and therefore, could have been presented above as well. To illustrate, assume we are tracking individual performance metrics that had been rising steadily across time, and suddenly the employer announces an upcoming across-the-board bonus based on those metrics. A sudden rise (as in a change in slope) in those metrics could be expected based purely on reinforcement theory. Assume, for example, we had six intervals of measurement, and the bonus announcement was made just after the Time 3 data collection. We could specify two slope or change variables and code the first one as 0, 1, 2, 2, 2, and 2, and code the second slope variable as 0, 0, 0, 1, 2, and 3. The latter specification would then independently examine the linear change in each slope variable. Conceptually, the first slope variable brings the trajectory of change up to the transition point (i.e., the last measurement before the announcement) while the second one captures the change after the transition ( Bollen & Curran, 2006 ). Regardless of whether the variables are latent or observed only, if this is modeled using software such as Mplus ( Muthén & Muthén, 1998–2012 ), the difference between the means of the slope variables may be statistically tested to evaluate whether the post-announcement slope is indeed greater than the pre-announcement slope. One may also predict that the announcement would cause an immediate sudden elevation in the performance metric as well. This can be examined by including a dummy variable which is zero at all time points prior to the announcement and one at all time points after the announcement ( Singer & Willett, 2003 , pp. 194–195). If the coefficient for this dummy variable is statistically significant and positive, then it indicates that there was a sudden increase (upward elevation) in value post-transition.
Another form of discontinuous change is one in which the discontinuous event occurs at varying times for the units of observation (indeed it may not occur at all for some) and the intervals for collecting data may not be evenly spaced. For example, assume again that individual performance metrics are monitored across time for individuals in high-demand occupations with the first one collected on the date of hire. Assume as well that these individuals are required to report when an external recruiter approaches them; that is, they are not prohibited from speaking with a recruiter but need to just report when it occurred. Due to some cognitive dissonance process, individuals may start to discount the current employer and reduce their inputs. Thus, a change in slope, elevation, or both may be expected in performance. With respect to testing a potential change in elevation, one uses the same dummy-coded variable as described above ( Singer & Willett, 2003 ). With respect to whether the slopes of the performance metrics differ pre- versus post-recruiter contact, however, requires the use of a time-varying covariate. How this operates specifically is beyond the scope here. Excellent treatments on the topic, however, are provided by Bollen and Curran (2006 , pp. 192–218), and Singer and Willett (2003 , pp. 190–208). In general, a time-varying covariate captures the intervals of measurement. In the current example, this may be the number of days (weeks, months, etc.) from date of hire (when baseline performance was obtained) to the next interval of measurement and all subsequent intervals. Person 1, for example, may have the values 1, 22, 67, 95, 115, and 133, and was contacted after Time 3 on Day 72 from the date of hire. Person 2 may have the values 1, 31, 56, 101, 141, and 160, and was contacted after Time 2 on Day 40 from date of hire. Referring the reader to the specifics starting on page 195 of Singer and Willett (2003) , one would then create a new variable from the latter in which all of the values on this new variable before the recruiting contact are set to zero, and values after that to the difference in days when contact was made to the interval of measurement. Thus, for Person 1, this new variable would have the values 0, 0, 0, 23, 43, and 61, and for Person 2, the values would be 0, 0, 16, 61, 101, and 120. The slope of this new variable represents the increment (up or down) to what the slope would have been had the individuals not been contacted by a recruiter. If it is statistically nonsignificant, then there is no change in slope pre- versus post-recruiter contact. If it is statistically significant, then the slope after contact differed from that before the contact. Finally, while much of the above is based upon a multilevel approach to operationalizing change, Muthén and Muthén (1998–2012 ) offer an SEM approach to time-varying covariates through their Mplus software package.
The final functional form to which the slope or change variable may be coded or specified is nonlinear. As with the other forms, there is a set of nonlinear forms. The simplest in the set is when theory states that the change in the focal variable may be quadratic (curve upward or downward). As such, in addition to the linear slope/change variable, a second change variable is specified in which the values of its slope are fixed to the squared values of the first or linear change variable. Assuming five equally spaced intervals of measurement coded as 0, 1, 2, 3, and 4 on the linear change variable. The values of the second quadratic change variable would be 0, 1, 4, 9, and 16. Theory could state that there is cubic change as well. In that case, a third cubic change variable is introduced with the values of 0, 1, 8, 27, and 64. One problem with the use of quadratic (or even linear change variables) or other polynomial forms as described above is that the trajectories are unbounded functions ( Bollen & Curran, 2006 ); that is, there is an assumption that they tend toward infinity. It is unlikely that most, if any, of the theoretical processes in the social sciences are truly unbounded. If a nonlinear form is expected, operationalizing change using an exponential trajectory is probably the most realistic choice. This is because exponential trajectories are bounded functions in the sense that they approach an asymptote (either growing and/or decaying to asymptote). There are three forms of exponential trajectories: (a) simple where there is explosive growth from asymptote; (b) negative where there is growth to an asymptote; and (c) logistic where this is asymptote at both ends ( Singer & Willett, 2003 ). Obviously, the values of the slope or change variable would be fixed to the exponents most closely representing the form of the curve (see Bollen & Curren, 2006, p. 108; and Singer & Willett, 2003 , Table 6.7, p. 234).
There are other nonlinear considerations as well that belong to this. For example, Bollen and Curran (2006 , p. 109) address the issue of cycles (recurring ups and downs but that follow a general upward or downward trend.) Once more the values of the change variable would be coded to reflect those cycles. Similarly, Singer and Willett (2003 , p. 208) address recoding when one wants to remove through transformations the nonlinearity in the change function to make it more linear. They provide an excellent heuristic on page 211 to guide one’s thinking on this issue.
Statistical Techniques Question 2: In longitudinal research, are there additional issues of measurement error that we need to pay attention to, which are over and above those that are applicable to cross-sectional research?
Longitudinal research should pay special attention to the measurement invariance issue. Chan (1998) and Schmitt (1982) introduced Golembiewski and colleagues’ (1976) notion of alpha, beta, and gamma change to explain why measurement invariance is a concern in longitudinal research. When the measurement of a particular concept retains the same structure (i.e., same number of observed items and latent factors, same value and pattern of factor loadings), change in the absolute levels of the latent factor is called alpha change. Only for this type of change can we draw the conclusion that there is a specific form of growth in a given variable. When the measurement of a concept has to be adjusted over time (i.e., different values or patterns of factor loadings), beta change happens. Although the conceptual meaning of the factor remains the same over measurements, the subjective metric of the concept has changed. When the meaning of a concept changes over time (e.g., having different number of factors or different correlations between factors), gamma change happens. It is not possible to compare difference in absolute levels of a latent factor when beta and gamma changes happen, because there is no longer a stable measurement model for the construct. The notions of beta and gamma changes are particularly important to consider when conducting longitudinal research on aging-related phenomena, especially when long time intervals are used in data collection. In such situations, the risk for encountering beta and gamma changes is higher and can seriously jeopardize the internal and external validity of the research.
Longitudinal analysis is often conducted to examine how changes happen in the same variable over time. In other words, it operates on the “alpha change” assumption. Thus, it is often important to explicitly test measurement invariance before proceeding to model the growth parameters. Without establishing measurement invariance, it is unknown whether we are testing meaningful changes or comparing apples and oranges. A number of references have discussed the procedures for testing measurement invariance in latent variable analysis framework (e.g., Chan, 1998 ; McArdle, 2007 ; Ployhart & Vandenberg, 2010 ). The basic idea is to specify and include the measurement models in the longitudinal model, with either continuous or categorical indicators (see answers to Statistical Techniques #4 below on categorical indicators). With the latent factor invariance assumption, factor loadings across measurement points should be constrained to be equal. Errors from different measurement occasions might correlate, especially when the measurement contexts are very similar over time ( Tisak & Tisak, 2000 ). Thus, the error variances for the same item over time can also be correlated to account for common influences at the item-level (i.e., autocorrelation between items). With the specification of the measurement structure, the absolute changes in the latent variables can then be modeled by the mean structure. It should be noted that a more stringent definition of measurement invariance also requires equal variance in latent factors. However, in longitudinal data this requirement becomes extremely difficult to satisfy, and factor variances can be sample specific. Thus, this requirement is often eased when testing measurement invariance in longitudinal analysis. Moreover, this requirement may even be invalid when the nature of the true change over time involves changes in the latent variance ( Chan, 1998 ).
It is important to note that the mean structure approach not only applies to longitudinal models with three or more measurement points, but also applies to simple repeated measures designs (e.g., pre–post design). Traditional paired sample t tests and within-subject repeated measures ANOVAs do not take into account measurement equivalence, which simply uses the summed scores at two measurement points to conduct a hypothesis test. The mean structure approach provides a more powerful way to test the changes/differences in a latent variable by taking measurement errors into consideration ( McArdle, 2009 ).
However, sometimes it is not possible to achieve measurement equivalence through using the same scales over time. For example, in research on development of cognitive intelligence in individuals from birth to late adulthood, different tests of cognitive intelligence are administrated at different ages (e.g., Bayley, 1956 ). In applied settings, different domain-knowledge or skill tests may be administrated to evaluate employee competence at different stages of their career. Another possible reason for changing measures is poor psychometric properties of scales used in earlier data collection. Previously, researchers have used transformed scores (e.g., scores standardized within each measurement point) before modeling growth curves over time. In response to critiques of these scaling methods, new procedures have been developed to model longitudinal data using changed measurement (e.g., rescoring methods, over-time prediction, and structural equation modeling with convergent factor patterns). Recently, McArdle and colleagues (2009) proposed a joint model approach that estimated an item response theory (IRT) model and latent curve model simultaneously. They provided a demonstration of how to effectively handle changing measurement in longitudinal studies by using this new proposed approach.
I am not sure these issues of measurement error are “over and above” cross-sectional issues as much as that cross-sectional data provide no mechanisms for dealing with these issues, so they are simply ignored at the analysis stage. Unfortunately, this creates problems at the interpretation stage. In particular, issues of random walk variables ( Kuljanin, Braun, & DeShon, 2011 ) are a potential problem for longitudinal data analysis and the interpretation of either cross-sectional or longitudinal designs. Random walk variables are dynamic variables that I mentioned earlier when describing the computational modeling approach. These variables have some value and are moved from that value. The random walk expression comes from the image of a highly inebriated individual, who is in some position, but who staggers and sways from the position to neighboring positions because the alcohol has disrupted the nerve system’s stabilizers. This inebriated individual might have an intended direction (called “the trend” if the individual can make any real progress), but there may be a lot of noise in that path. In the aging and retirement literature, one’s retirement savings can be viewed as a random walk variable. Although the general trend of retirement savings should be positive (i.e., the amount of retirement savings should grow over time), at any given point, the exact amount added/gained into the saving (or withdrawn/loss from the saving) depends on a number of situational factors (e.g., stock market performance) and cannot be consistently predicted. The random walks (i.e., dynamic variables) have a nonindependence among observations over time. Indeed, one way to know if one is measuring a dynamic variable is if one observes a simplex pattern among inter-correlations of the variable with itself over time. In a simplex pattern, observations of the variable are more highly correlated when they are measured closer in time (e.g., Time 1 observations correlate more highly with Time 2 than Time 3). Of course, this pattern can also occur if its proximal causes (rather than itself) is a dynamic variable.
As noted, dynamic or random walk variables can create problems for poorly designed longitudinal research because one may not realize that the level of the criterion ( Y ), say measured at Time 3, was largely near its level at Time 2, when the presumed cause ( X ) was measured. Moreover, at Time 1 the criterion ( Y ) might have been busy moving the level of the “causal” variable ( X ) to the place it is observed at Time 2. That is, the criterion variable ( Y ) at Time 1 is actually causing the presumed causal variable ( X ) at Time 2. For example, performances might affect self-efficacy beliefs such that self-efficacy beliefs end up aligning with performance levels. If one measures self-efficacy after it has largely been aligned, and then later measures the largely stable performance, a positive correlation between the two variables might be thought of as reflecting self-efficacy’s influence on performance because of the timing of measurement (i.e., measuring self-efficacy before performance). This is why the multiple wave measurement practice is so important in passive observational panel studies.
However, the multiple waves of measurement might still create problems for random walk variables, particularly if there are trends and reverse causality. Consider the self-efficacy to performance example again. If performance is trending over time and self-efficacy is following along behind, a within-person positive correlation between self-efficacy and subsequent performance is likely be observed (even if there is no or a weak negative causal effect) because self-efficacy will be relatively high when performance is relatively high and low when performance is low. In this case, controlling for trend or past performance will generally solve the problem ( Sitzmann & Yeo, 2013 ), unless the random walk has no trend. Meanwhile, there are other issues that random walk variables may raise for both cross-sectional and longitudinal research, which Kuljanin et al. (2011) do a very good job of articulating.
A related issue for longitudinal research is nonindependence of observations as a function of nesting within clusters. This issue has received a great deal of attention in the multilevel literature (e.g., Bliese & Ployhart, 2002 ; Singer & Willett, 2003 ), so I will not belabor the point. However, there is one more nonindependence issue that has not received much attention. Specifically, the issue can be seen when a variable is a lagged predictor of itself ( Vancouver, Gullekson, & Bliese, 2007 ). With just three repeated measures or observations, the correlation of the variable on itself will average −.33 across three time points, even if the observations are randomly generated. This is because there is a one-third chance the repeated observations are changing monotonically over the three time points, which results in a correlation of 1, and a two-thirds chance they are not changing monotonically, which results in a correlation of −1, which averages to −.33. Thus, on average it will appear the variable is negatively causing itself. Fortunately, this problem is quickly mitigated by more waves of observations and more cases (i.e., the bias is largely removed with 60 pairs of observations).
Statistical Techniques Question 3: When analyzing longitudinal data, how should we handle missing values?
As reviewed by Newman (2014 ; see in-depth discussions by Enders, 2001 , 2010 ; Little & Rubin, 1987 ; Newman, 2003 , 2009 ; Schafer & Graham, 2002 ), there are three levels of missing data (item level missingness, variable/construct-level missingness, and person-level missingness), two problems caused by missing data (parameter estimation bias and low statistical power), three mechanisms of missing data (missing completely at random/MCAR, missing at random/MAR, and missing not at random/MNAR), and a handful of common missing data techniques (listwise deletion, pairwise deletion, single imputation techniques, maximum likelihood, and multiple imputation). State-of-the-art advice is to use maximum likelihood (ML: EM algorithm, Full Information ML) or multiple imputation (MI) techniques, which are particularly superior to other missing data techniques under the MAR missingness mechanism, and perform as well as—or better than—other missing data techniques under MCAR and MNAR missingness mechanisms (MAR missingness is a form of systematic missingness in which the probability that data are missing on one variable [ Y ] is related to the observed data on another variable [ X ]).
Most of the controversy surrounding missing data techniques involves two misconceptions: (a) the misconception that listwise and pairwise deletion are somehow more natural techniques that involve fewer or less tenuous assumptions than ML and MI techniques do, with the false belief that a data analyst can draw safer inferences by avoiding the newer techniques, and (b) the misconception that multiple imputation simply entails “fabricating data that were not observed.” First, because all missing data techniques are based upon particular assumptions, none is perfect. Also, when it comes to selecting a missing data technique to analyze incomplete data, one of the above techniques (e.g., listwise, pairwise, ML, MI) must be chosen. One cannot safely avoid the decision altogether—that is, abstinence is not an option. One must select the least among evils.
Because listwise and pairwise deletion make the exceedingly unrealistic assumption that missing data are missing completely at random/MCAR (cf. Rogelberg et al. , 2003 ), they will almost always produce worse bias than ML and MI techniques, on average ( Newman & Cottrell, 2015 ). Listwise deletion can further lead to extreme reductions in statistical power. Next, single imputation techniques (e.g., mean substitution, stochastic regression imputation)—in which the missing data are filled in only once, and the resulting data matrix is analyzed as if the data had been complete—are seriously flawed because they overestimate sample size and underestimate standard errors and p -values.
Unfortunately, researchers often get confused into thinking that multiple imputation suffers from the same problems as single imputation; it does not. In multiple imputation, missing data are filled in several different times, and the multiple resulting imputed datasets are then aggregated in a way that accounts for the uncertainty in each imputation ( Rubin, 1987 ). Multiple imputation is not an exercise in “making up data”; it is an exercise in tracing the uncertainty of one’s parameter estimates, by looking at the degree of variability across several imprecise guesses (given the available information). The operative word in multiple imputation is multiple , not imputation.
Longitudinal modeling tends to involve a lot of construct- or variable-level missing data (i.e., omitting answers from an entire scale, an entire construct, or an entire wave of observation—e.g., attrition). Such conditions create many partial nonrespondents, or participants for whom some variables have been observed and some other variables have not been observed. Thus a great deal of missing data in longitudinal designs tends to be MAR (e.g., because missing data at Time 2 is related to observed data at Time 1). Because variable-level missingness under the MAR mechanism is the ideal condition for which ML and MI techniques were designed ( Schafer & Graham, 2002 ), both ML and MI techniques (in comparison to listwise deletion, pairwise deletion, and single imputation techniques) will typically produce much less biased estimates and more accurate hypothesis tests when used on longitudinal designs ( Newman, 2003 ). Indeed, ML missing data techniques are now the default techniques in LISREL, Mplus, HLM, and SAS Proc Mixed. It is thus no longer excusable to perform discrete-time longitudinal analyses ( Figure 2 ) without using either ML or MI missing data techniques ( Enders, 2010 ; Graham, 2009 ; Schafer & Graham, 2002 ).
Lastly, because these newer missing data techniques incorporate all of the available data, it is now increasingly important for longitudinal researchers to not give up on early nonrespondents. Attrition need not be a permanent condition. If a would-be respondent chooses not to reply to a survey request at Time 1, the researcher should still attempt to collect data from that person at Time 2 and Time 3. More data = more useful information that can reduce bias and increase statistical power. Applying this advice to longitudinal research on aging and retirement, it means that even when a participant fails to provide responses at some measurement points, continuing to make an effort to collect more data from the participant in subsequent waves may still be worthwhile. It will certainly help combat the issue of attrition and allow more usable data to emerge from the longitudinal data collection.
Statistical Techniques Question 4: Most of existing longitudinal research focuses on studying quantitative change over time. What if the variable of interest is categorical or if the changes over time are qualitative in nature?
I think there are two questions here: How to model longitudinal data of categorical variables, and how to model discontinuous change patterns of variables over time. In terms of longitudinal categorical data, there are two types of data that researchers typically encounter. One type of data comes from measuring a sample of participants on a categorical variable at a few time points (i.e., panel data). The research question that drives the data analyses is to understand the change of status from one time point to the next. For example, researchers might be interested in whether a population of older workers would stay employed or switch between employed and unemployed statuses (e.g., Wang & Chan, 2011 ). To answer this question, employment status (employed or unemployed) of a sample of older workers might be measured five or six times over several years. When transition between qualitative statuses is of theoretical interest, this type of panel data can be modeled via Markov chain models. The simplest form of Markov chain models is a simple Markov model with a single chain, which assumes (a) the observed status at time t depends on the observed status at time t –1, (b) the observed categories are free from measurement error, and (c) the whole population can be described by a single chain. The first assumption is held by most if not all Markov chain models. The other two assumptions can be released by using latent Markov chain modeling (see Langeheine & Van de Pol, 2002 for detailed explanation).
The basic idea of latent Markov chains is that observed categories reflect the “true” status on latent categorical variables to a certain extent (i.e., the latent categorical variable is the cause of the observed categorical variable). In addition, because the observations may contain measurement error, a number of different observed patterns over time could reflect the same underlying latent transition pattern in qualitative status. This way, a large number of observed patterns (e.g., a maximum of 256 patterns of a categorical variable with four categories measured four times) can be reduced into reflecting a small number of theoretically coherent patterns (e.g., a maximum of 16 patterns of a latent categorical variable with two latent statuses over four time points). It is also important to note that subpopulations in a larger population can follow qualitatively different transition patterns. This heterogeneity in latent Markov chains can be modeled by mixture latent Markov modeling, a technique integrating latent Markov modeling and latent class analysis (see Wang & Chan, 2011 for technical details). Given that mixture latent Markov modeling is a part of the general latent variable analysis framework ( Muthén, 2001 ), mixture latent Markov models can include different types of covariates and outcomes (latent or observed, categorical or continuous) of the subpopulation membership as well as the transition parameters of each subpopulation.
Another type of longitudinal categorical data comes from measuring one or a few study units on many occasions separated by the same time interval (e.g., every hour, day, month, or year). Studies examining this type of data mostly aim to understand the temporal trend or periodic tendency in a phenomenon. For example, one can examine the cyclical trend of daily stressful events (occurred or not) over several months among a few employees. The research goal could be to reveal multiple cyclical patterns within the repeated occurrences in stressful events, such as daily, weekly, and/or monthly cycles. Another example is the study of performance of a particular player or a sports team (i.e., win, lost, or tie) over hundreds of games. The research question could be to find out time-varying factors that could account for the cyclical patterns of game performance. The statistical techniques typically used to analyze this type of data belong to the family of categorical time series analyses . A detailed technical review is beyond the current scope, but interested readers can refer to Fokianos and Kedem (2003) for an extended overview.
In terms of modeling discontinuous change patterns of variables, Singer and Willett (2003) and Bollen and Curran (2006) provided guidance on modeling procedures using either the multilevel modeling or structural equation modeling framework. Here I briefly discuss two additional modeling techniques that can achieve similar research goals: spline regression and catastrophe models.
Spline regression is used to model a continuous variable that changes its trajectory at a particular time point (see Marsh & Cormier, 2001 for technical details). For example, newcomers’ satisfaction with coworkers might increase steadily immediately after they enter the organization. Then due to a critical organizational event (e.g., the downsizing of the company, a newly introduced policy to weed out poor performers in the newcomer cohort), newcomers’ coworker satisfaction may start to drop. A spline model can be used to capture the dramatic change in the trend of newcomer attitude as a response to the event (see Figure 4 for an illustration of this example). The time points at which the variable changes its trajectory are called spline knots. At the spline knots, two regression lines connect. Location of the spline knots may be known ahead of time. However, sometimes the location and the number of spline knots are unknown before data collection. Different spline models and estimation techniques have been developed to account for these different explorations of spline knots ( Marsh & Cormier, 2001 ). In general, spline models can be considered as dummy-variable based models with continuity constraints. Some forms of spline models are equivalent to piecewise linear regression models and are quite easy to implement ( Pindyck & Rubinfeld, 1998 ).

Hypothetical illustration of spline regression: The discontinuous change in newcomers’ satisfaction with coworkers over time.
Catastrophe models can also be used to describe “sudden” (i.e., catastrophic) discontinuous change in a dynamic system. For example, some systems in organizations develop from one certain state to uncertainty, and then shift to another certain state (e.g., perception of performance; Hanges, Braverman, & Rentsch, 1991 ). This nonlinear dynamic change pattern can be described by a cusp model, one of the most popular catastrophe models in the social sciences. Researchers have applied catastrophe models to understand various types of behaviors at work and in organizations (see Guastello, 2013 for a summary). Estimation procedures are also readily available for fitting catastrophe models to empirical data (see technical introductions in Guastello, 2013 ).
Statistical Techniques Question 5: Could you speculate on the “next big thing” in conceptual or methodological advances in longitudinal research? Specifically, describe a novel idea or specific data analytic model that is rarely used in longitudinal studies in our literature, but could serve as a useful conceptual or methodological tool for future science in work, aging and retirement.
Generally, but mostly on the conceptual level, I think we will see an increased use of computational models to assess theory, design, and analysis. Indeed, I think this will be as big as multilevel analysis in future years, though the rate at which it will happen I cannot predict. The primary factors slowing the rate of adoption are knowledge of how to do it and ignorance of the cost of not doing it (cf. Vancouver, Tamanini et al. , 2010 ). Factors that will speed its adoption are easy-to-use modeling software and training opportunities. My coauthor and I recently published a tutorial on computational modeling ( Vancouver & Weinhardt, 2012 ), and we provide more details on how to use a specific, free, easy-to-use modeling platform on our web site ( https://sites.google.com/site/motivationmodeling/home ).
On the methodology level I think research simulations (i.e., virtual worlds) will increase in importance. They offer a great deal of control and the ability to measure many variables continuously or frequently. On the analysis level I anticipate an increased use of Bayesian and Hierarchical Bayesian analysis, particularly to assess computational model fits ( Kruschke, 2010 ; Rouder, & Lu, 2005 ; Wagenmakers, 2007 ).
I predict that significant advances in various areas will be made in the near future through the appropriate application of mixture latent modeling approaches. These approaches combine different latent variable techniques such as latent growth modeling, latent class modeling, latent profile analysis, and latent transition analysis into a unified analytical model ( Wang & Hanges, 2011 ). They could also integrate continuous variables and discrete variables, as either predictor or outcome variables, in a single analytical model to describe and explain simultaneous quantitative and qualitative changes over time. In a recent study, my coauthor and I applied an example of a mixture latent model to understand the retirement process ( Wang & Chan, 2011 ). Despite or rather because of the power and flexibility of these advanced mixture techniques to fit diverse models to longitudinal data, I will repeat the caution I made over a decade ago—that the application of these complex models to assess changes over time should be guided by adequate theories and relevant previous empirical findings ( Chan, 1998 ).
My hope or wish for the next big thing is the use of longitudinal methods to integrate the micro and macro domains of our literature on work-related phenomena. This will entail combining aspects of growth modeling with multi-level processes. Although I do not have a particular conceptual framework in mind to illustrate this, my reasoning is based on the simple notion that it is the people who make the place. Therefore, it seems logical that we could, for example, study change in some aspect of firm performance across time as a function of change in some aspect of individual behavior and/or attitudes. Another example could be that we can study change in household well-being throughout the retirement process as a function of change in the two partners’ individual well-being over time. The analytical tools exist for undertaking such analyses. What are lacking at this point are the conceptual frameworks.
I hope the next big thing for longitudinal research will be dynamic computational models ( Ilgen & Hulin, 2000 ; Miller & Page, 2007 ; Weinhardt & Vancouver, 2012 ), which encode theory in a manner that is appropriately longitudinal/dynamic. If most theories are indeed theories of change, then this advancement promises to revolutionize what passes for theory in the organizational sciences (i.e., a computational model is a formal theory, with much more specific, risky, and therefore more meaningful predictions about phenomena—in comparison to the informal verbal theories that currently dominate and are somewhat vague with respect to time). My preferred approach is iterative: (a) authors first collect longitudinal data, then (b) inductively build a parsimonious computational model that can reproduce the data, then (c) collect more longitudinal data and consider its goodness of fit with the model, then (d) suggest possible model modifications, and then repeat steps (c) and (d) iteratively until some convergence is reached (e.g., Stasser, 2000 , 1988 describes one such effort in the context of group discussion and decision making theory). Exactly how to implement all the above steps is not currently well known, but developments in this area can potentially change what we think good theory is.
I am uncertain whether my “next big thing” truly reflects the wave of the future, or if it instead simply reflects my own hopes for where longitudinal research should head in our field. I will play it safe and treat it as the latter. Consistent with several other responses to this question, I hope that researchers will soon begin to incorporate far more complex dynamics of processes into both their theorizing and their methods of analysis. Although process dynamics can (and do) occur at all levels of analysis, I am particularly excited by the prospect of linking them across at least adjacent levels. For example, basic researchers interested in the dynamic aspects of affect recently have begun theorizing and modeling emotional experiences using various forms of differential structural equation or state-space models (e.g. Chow et al. , 2005 ; Kuppens, Oravecz, & Tuerlinckx, 2010 ), and, as the resulting parameters that describe within-person dynamics can be aggregated to higher levels of analysis (e.g., Beal, 2014 ; Wang, Hamaker, & Bergeman, 2012 ), they are inherently multilevel.
Another example of models that capture this complexity and are increasingly used in both immediate and longer-term longitudinal research are multivariate latent change score models ( Ferrer & McArdle, 2010 ; McArdle, 2009 ; Liu et al. , 2016 ). These models extend LGMs to include a broader array of sources of change (e.g., autoregressive and cross-lagged factors) and consequently capture more of the complexity of changes that can occur in one or more variables measured over time. All of these models share a common interest in modeling the underlying dynamic patterns of a variable (e.g., linear, curvilinear, or exponential growth, cyclical components, feedback processes), while also taking into consideration the “shocks” to the underlying system (e.g., affective events, organizational changes, etc.), allowing them to better assess the complexity of dynamic processes with greater accuracy and flexibility ( Wang et al. , 2016 ).
I believe that applying a dynamical systems framework will greatly advance our research. Applying the dynamic systems framework (e.g., DeShon, 2012 ; Vancouver, Weinhardt, & Schmidt, 2010 ; Wang et al. , 2016 ) forces us to more explicitly conceptualize how changes unfold over time in a particular system. Dynamic systems models can also answer the why question better by specifying how elements of a system work together over time to bring about the observed change at the system level. Studies on dynamic systems models also tend to provide richer data and more detailed analyses on the processes (i.e., the black boxes not measured in traditional research) in a system. A number of research design and analysis methods relevant for dynamical systems frameworks are available, such as computational modeling, ESM, event history analyses, and time series analyses ( Wang et al. , 2016 ).
M. Wang’s work on this article was supported in part by the Netherlands Institute for Advanced Study in the Humanities and Social Sciences.
Ainslie G. , & Haslam N . ( 1992 ). Hyperbolic discounting . In G. Loewenstein J. Elster (Eds.), Choice over time (pp. 57 – 92 ). New York, NY : Russell Sage Foundation .
Google Scholar
Google Preview
Ancona D. G. Goodman P. S. Lawrence B. S. , & Tushman M. L . ( 2001 ). Time: A new research lens . Academy of Management Review , 26 , 645 – 563 . doi: 10.5465/AMR.2001.5393903
Ashford S. J . ( 1986 ). The role of feedback seeking in individual adaptation: A resource perspective . Academy of Management Journal , 29 , 465 – 487 . doi: 10.2307/256219
Bayley N . ( 1956 ). Individual patterns of development . Child Development , 27 , 45 – 74 . doi: 10.2307/1126330
Beal D. J . ( 2014 ). Time and emotions at work . In Shipp A. J. Fried Y. (Eds.), Time and work (Vol. 1 , pp. 40 – 62 ). New York, NY : Psychology Press .
Beal D. J . ( 2015 ). ESM 2.0: State of the art and future potential of experience sampling methods in organizational research . Annual Review of Organizational Psychology and Organizational Behavior , 2 , 383 – 407 .
Beal D. J. , & Ghandour L . ( 2011 ). Stability, change, and the stability of change in daily workplace affect . Journal of Organizational Behavior , 32 , 526 – 546 . doi: 10.1002/job.713
Beal D. J. , & Weiss H. M . ( 2013 ). The episodic structure of life at work . In Bakker A. B. Daniels K. (Eds.), A day in the life of a happy worker (pp. 8 – 24 ). London, UK : Psychology Press .
Beal D. J. , & Weiss H. M . ( 2003 ). Methods of ecological momentary assessment in organizational research . Organizational Research Methods , 6 , 440 – 464 . doi: 10.1177/1094428103257361
Beal D. J. Weiss H. M. Barros E. , & MacDermid S. M . ( 2005 ). An episodic process model of affective influences on performance . Journal of Applied Psychology , 90 , 1054 . doi: 10.1037/0021-9010.90.6.1054
Bentein K. Vandenberghe C. Vandenberg R. , & Stinglhamber F . ( 2005 ). The role of change in the relationship between commitment and turnover: a latent growth modeling approach . Journal of Applied Psychology , 90 , 468 – 482 . doi: 10.1037/0021-9010.90.3.468
Bliese P. D. , & Ployhart R. E . ( 2002 ). Growth modeling using random coefficient models: Model building, testing, and illustrations . Organizational Research Methods , 5 , 362 – 387 . doi: 10.1177/109442802237116
Bolger N. Davis A. , & Rafaeli E . ( 2003 ). Diary methods: Capturing life as it is lived . Annual Review of Psychology , 54 , 579 – 616 . doi: 10.1146/annurev.psych.54.101601.145030
Bolger N. , & Laurenceau J.-P . ( 2013 ). Intensive longitudinal methods: An introduction to diary and experience sampling research . New York, NY : Guilford .
Bollen K. A. , & Curran P. J . ( 2006 ). Latent curve models: A structural equation approach . Hoboken, NJ : Wiley .
Carsten J. M. , & Spector P. E . ( 1987 ). Unemployment, job satisfaction, and employee turnover: A meta-analytic test of the Muchinsky model . Journal of Applied Psychology , 72 , 374 . doi: 10.1037/0021-9010.72.3.374
Castiglioni L. Pforr K. , & Krieger U . ( 2008 ). The effect of incentives on response rates and panel attrition: Results of a controlled experiment . Survey Research Methods , 2 , 151 – 158 . doi: 10.18148/srm/2008.v2i3.599
Chan D . ( 1998 ). The conceptualization and analysis of change over time: An integrative approach incorporating longitudinal mean and covariance structures analysis (LMACS) and multiple indicator latent growth modeling (MLGM) . Organizational Research Methods , 1 , 421 – 483 . doi: 10.1177/109442819814004
Chan D . ( 2002 ). Longitudinal modeling . In Rogelberg S . Handbook of research methods in industrial and organizational psychology (pp. 412 – 430 ). Malden, MA : Blackwell Publishers, Inc .
Chan D . ( 2010 ). Advances in analytical strategies . In S. Zedeck (Ed.), APA handbook of industrial and organizational psychology (Vol. 1 ), Washington, DC : APA .
Chan D . ( 2014 ). Time and methodological choices . In In A. J. Shipp Y. Fried (Eds.), Time and work (Vol. 2): How time impacts groups, organizations, and methodological choices . New York, NY : Psychology Press .
Chan D. , & Schmitt N . ( 2000 ). Interindividual differences in intraindividual changes in proactivity during organizational entry: A latent growth modeling approach to understanding newcomer adaptation . Journal of Applied Psychology , 85 , 190 – 210 .
Chow S. M. Ram N. Boker S. M. Fujita F. , & Clore G . ( 2005 ). Emotion as a thermostat: representing emotion regulation using a damped oscillator model . Emotion , 5 , 208 – 225 . doi: 10.1037/1528-3542.5.2.208
Cole M. S. Bedeian A. G. , & Feild H. S . ( 2006 ). The measurement equivalence of web-based and paper-and-pencil measures of transformational leadership a multinational test . Organizational Research Methods , 9 , 339 – 368 . doi: 10.1177/1094428106287434
Cole D. A. , & Maxwell S. E . ( 2003 ). Testing mediational models with longitudinal data: Questions and tips in the use of structural equation modeling . Journal of Abnormal Psychology , 112 , 558 – 577 . doi: 10.1037/0021-843X.112.4.558
Csikszentmihalyi M. , & Larson R . ( 1987 ). Validity and reliability of the experience sampling method . Journal of Nervous and Mental Disease , 775 , 526 – 536 .
DeShon R. P . ( 2012 ). Multivariate dynamics in organizational science . In S. W. J. Kozlowski (Ed.), The Oxford Handbook of Organizational Psychology (pp. 117 – 142 ). New York, NY : Oxford University Press .
Diener E. Inglehart R. , & Tay L . ( 2013 ). Theory and validity of life satisfaction scales . Social Indicators Research , 112 , 497 – 527 . doi: 10.1007/s11205-012-0076-y
Enders C. K . ( 2001 ). . Structural Equation Modelling , 8 , 128 – 141 .
Enders C. K . ( 2010 ). Applied missing data analysis . New York City, NY : The Guilford Press .
Gersick C. J . ( 1988 ). Time and transition in work teams: Toward a new model of group development . Academy of Management Journal , 31 , 9 – 41 . doi: 10.2307/256496
Graham J. W . ( 2009 ). Missing data analysis: Making it work in the real world . Annual Review of Psychology , 60 , 549 – 576 . doi: 10.1146/annurev.psych.58.110405.085530
Ferrer E. , & McArdle J. J . ( 2010 ). Longitudinal modeling of developmental changes in psychological research . Current Directions in Psychological Science , 19 , 149 – 154 . doi: 10.1177/0963721410370300
Fisher G. G. Chaffee D. S. , & Sonnega A . ( 2016 ). Retirement timing: A review and recommendations for future research . Work, Aging and Retirement , 2 , 230 – 261 . doi: 10.1093/workar/waw001
Fokianos K. , & Kedem B . ( 2003 ). Regression theory for categorical time series . Statistical Science , 357 – 376 . doi: 10.1214/ss/1076102425
Fraley R. C . ( 2002 ). Attachment stability from infancy to adulthood: Meta-analysis and dynamic modeling of developmental mechanisms . Personality and Social Psychology Review , 6 , 123 – 151 . doi: 10.1207/S15327957PSPR0602_03
Fredrickson B. L . ( 2000 ). Extracting meaning from past affective experiences: The importance of peaks, ends, and specific emotions . Cognition and Emotion , 14 , 577 – 606 .
Fumagalli L. Laurie H. , & Lynn P . ( 2013 ). Experiments with methods to reduce attrition in longitudinal surveys . Journal of the Royal Statistical Society: Series A (Statistics in Society) , 176 , 499 – 519 . doi: 10.1111/j.1467-985X.2012.01051.x
Golembiewski R. T. Billingsley K. , & Yeager S . ( 1976 ). Measuring change and persistence in human affairs: Types of change generated by OD designs . Journal of Applied Behavioral Science , 12 , 133 – 157 . doi: 10.1177/002188637601200201
Gosling S. D. Vazire S. Srivastava S. , & John O. P . ( 2004 ). Should we trust web-based studies? A comparative analysis of six preconceptions about internet questionnaires . American Psychologist , 59 , 93 – 104 . doi: 10.1037/0003-066X.59.2.93
Green A. S. Rafaeli E. Bolger N. Shrout P. E. , & Reis H. T . ( 2006 ). Paper or plastic? Data equivalence in paper and electronic diaries . Psychological Methods , 11 , 87 – 105 . doi: 10.1037/1082-989X.11.1.87
Groves R. M. Couper M. P. Presser S. Singer E. Tourangeau R. Acosta G. P. , & Nelson L . ( 2006 ). Experiments in producing nonresponse bias . Public Opinion Quarterly , 70 , 720 – 736 . doi: 10.1093/poq/nfl036
Guastello S. J . ( 2013 ). Chaos, catastrophe, and human affairs: Applications of nonlinear dynamics to work, organizations, and social evolution . New York, NY : Psychology Press
Hanges P. J. Braverman E. P. , & Rentsch J. R . ( 1991 ). Changes in raters’ perceptions of subordinates: A catastrophe model . Journal of Applied Psychology , 76 , 878 – 888 . doi: 10.1037/0021-9010.76.6.878
Heybroek L. Haynes M. , & Baxter J . ( 2015 ). Life satisfaction and retirement in Australia: A longitudinal approach . Work, Aging and Retirement , 1 , 166 – 180 . doi: 10.1093/workar/wav006
Hulin C. L. Henry R. A. , & Noon S. L . ( 1990 ). Adding a dimension: Time as a factor in the generalizability of predictive relationships . Psychological Bulletin , 107 , 328 – 340 .
Humphreys L. G . ( 1968 ). The fleeting nature of the prediction of college academic success . Journal of Educational Psychology , 59 , 375 – 380 .
Ilgen D. R. , & Hulin C. L . (Eds.). ( 2000 ). Computational modeling of behavior in organizations: The third scientific discipline . Washington, DC : American Psychological Association .
James L. R. Mulaik S. A. , & Brett J. M . ( 1982 ). Causal analysis: Assumptions, models, and data . Beverly Hills, CA : Sage Publications .
Kahneman D . ( 1999 ). Objective happiness . In D. Kahneman E. Diener N. Schwarz (Eds.), Well-being: The foundations of hedonic psychology (pp. 3 – 25 ). New York, NY : Russell Sage Foundation .
Keil C. T. , & Cortina J. M . ( 2001 ). Degradation of validity over time: A test and extension of Ackerman’s model . Psychological Bulletin , 127 , 673 – 697 .
Kessler R. C. , & Greenberg D. F . ( 1981 ). Linear panel analysis: Models of quantitative change . New York, NY : Academic Press .
Kruschke J. K . ( 2010 ). What to believe: Bayesian methods for data analysis . Trends in Cognitive Science , 14 : 293 – 300 . doi: 10.1016/j.tics.2010.05.001
Kuljanin G. Braun M. T. , & DeShon R. P . ( 2011 ). A cautionary note on modeling growth trends in longitudinal data . Psychological Methods , 16 , 249 – 264 . doi: 10.1037/a0023348
Kuppens P. Oravecz Z. , & Tuerlinckx F . ( 2010 ). Feelings change: accounting for individual differences in the temporal dynamics of affect . Journal of Personality and Social Psychology , 99 , 1042 – 1060 . doi: 10.1037/a0020962
Lance C. E. , & Vandenberg R. J . (Eds.). ( 2009 ) Statistical and methodological myths and urban legends: Doctrine, verity and fable in the organizational and social sciences . New York, NY : Taylor & Francis .
Langeheine R. , & Van de Pol F . ( 2002 ). Latent Markov chains . In J. A. Hagenaars A. L. McCutcheon (Eds.), Applied latent class analysis (pp. 304 – 341 ). New York City, NY : Cambridge University Press .
Laurie H . ( 2008 ). Minimizing panel attrition . In S. Menard (Ed.), Handbook of longitudinal research: Design, measurement, and analysis . Burlington, MA : Academic Press .
Laurie H. , & Lynn P . ( 2008 ). The use of respondent incentives on longitudinal surveys (Working Paper No. 2008–42 ) . Retrieved from Institute of Social and Economic Research website: https://www.iser.essex.ac.uk/files/iser_working_papers/2008–42.pdf
Laurie H. Smith R. , & Scott L . ( 1999 ). Strategies for reducing nonresponse in a longitudinal panel survey . Journal of Official Statistics , 15 , 269 – 282 .
Little R. J. A. , & Rubin D. B . ( 1987 ). Statistical analysis with missing data . New York, NY : Wiley .
Liu Y. Mo S. Song Y. , & Wang M . ( 2016 ). Longitudinal analysis in occupational health psychology: A review and tutorial of three longitudinal modeling techniques . Applied Psychology: An International Review , 65 , 379 – 411 . doi: 10.1111/apps.12055
Madero-Cabib I Gauthier J. A. , & Le Goff J. M . ( 2016 ). The influence of interlocked employment-family trajectories on retirement timing . Work, Aging and Retirement , 2 , 38 – 53 . doi: 10.1093/workar/wav023
Marsh L. C. , & Cormier D. R . ( 2001 ). Spline regression models . Thousand Oaks, CA : Sage Publications .
Martin G. L. , & Loes C. N . ( 2010 ). What incentives can teach us about missing data in longitudinal assessment . New Directions for Institutional Research , S2 , 17 – 28 . doi: 10.1002/ir.369
Meade A. W. Michels L. C. , & Lautenschlager G. J . ( 2007 ). Are Internet and paper-and-pencil personality tests truly comparable? An experimental design measurement invariance study . Organizational Research Methods , 10 , 322 – 345 . doi: 10.1177/1094428106289393
McArdle JJ . ( 2007 ). Dynamic structural equation modeling in longitudinal experimental studies . In K.V. Montfort H. Oud and A. Satorra et al. (Eds.), Longitudinal Models in the Behavioural and Related Sciences (pp. 159 – 188 ). Mahwah, NJ : Lawrence Erlbaum .
McArdle J. J . ( 2009 ). Latent variable modeling of differences and changes with longitudinal data . Annual Review of Psychology , 60 , 577 – 605 . doi: 10.1146/annurev.psych.60.110707.163612
McArdle J. J. Grimm K. J. Hamagami F. Bowles R. P. , & Meredith W . ( 2009 ). Modeling life-span growth curves of cognition using longitudinal data with multiple samples and changing scales of measurement . Psychological methods , 14 , 126 – 149 .
McGrath J. E. , & Rotchford N. L . ( 1983 ). Time and behavior in organizations . Research in Organizational Behavior , 5 , 57 – 101 .
Miller J. H. , & Page S. E . ( 2007 ). Complex adaptive systems: An introduction to computational models of social life . Princeton, NJ, USA : Princeton University Press .
Mitchell T. R. , & James L. R . ( 2001 ). Building better theory: Time and the specification of when things happen . Academy of Management Review , 26 , 530 – 547 . doi: 10.5465/AMR.2001.5393889
Morrison E. W . ( 2002 ). Information seeking within organizations . Human Communication Research , 28 , 229 – 242 . doi: 10.1111/j.1468-2958.2002.tb00805.x
Muthén B . ( 2001 ). Second-generation structural equation modeling with a combination of categorical and continuous latent variables: New opportunities for latent class–latent growth modeling . In L. M. Collins A. G. Sayer (Eds.), New methods for the analysis of change. Decade of behavior (pp. 291 – 322 ). Washington, DC : American Psychological Association .
Muthén L. K. , & Muthén B. O . (1998– 2012 ). Mplus user’s guide . 7th ed. Los Angeles, CA : Muthén & Muthén .
Newman D. A . ( 2003 ). Longitudinal modeling with randomly and systematically missing data: A simulation of ad hoc, maximum likelihood, and multiple imputation techniques . Organizational Research Methods , 6 , 328 – 362 . doi: 10.1177/1094428103254673
Newman D. A . ( 2009 ). Missing data techniques and low response rates: The role of systematic nonresponse parameters . In C. E. Lance R. J. Vandenberg (Eds.), Statistical and methodological myths and urban legends: Doctrine, verity, and fable in the organizational and social sciences (pp. 7 – 36 ). New York, NY : Routledge .
Newman D. A. , & Cottrell J. M . ( 2015 ). Missing data bias: Exactly how bad is pairwise deletion? In C. E. Lance R. J. Vandenberg (Eds.), More statistical and methodological myths and urban legends , pp. 133 – 161 . New York, NY : Routledge .
Newman D. A . ( 2014 ). Missing data five practical guidelines . Organizational Research Methods , 17 , 372 – 411 . doi: 10.1177/1094428114548590
Pindyck R. S. , & Rubinfeld D. L . ( 1998 ). Econometric Models and Economic Forecasts . Auckland, New Zealand : McGraw-Hill .
Pinquart M. , & Schindler I . ( 2007 ). Changes of life satisfaction in the transition to retirement: A latent-class approach . Psychology and Aging , 22 , 442 – 455 . doi: 10.1037/0882-7974.22.3.442
Ployhart R. E. , & Hakel M. D . ( 1998 ). The substantive nature of performance variability: Predicting interindividual differences in intraindividual performance . Personnel Psychology , 51 , 859 – 901 . doi: 10.1111/j.1744-6570.1998.tb00744.x
Ployhart R. E. , & Vandenberg R. J . ( 2010 ). Longitudinal Research: The theory, design, and analysis of change . Journal of Management , 36 , 94 – 120 . doi: 10.1177/0149206309352110
Podsakoff P. M. MacKenzie S. B. Lee J. Y. , & Podsakoff N. P . ( 2003 ). Common method biases in behavioral research: a critical review of the literature and recommended remedies . Journal of Applied Psychology , 88 , 879 – 903 . doi: 10.1037/0021-9010.88.5.879
Redelmeier D. A. , & Kahneman D . ( 1996 ). Patients’ memories of painful medical treatments: real-time and retrospective evaluations of two minimally invasive procedures . Pain , 66 , 3 – 8 .
Robinson M. D. , & Clore G. L . ( 2002 ). Belief and feeling: evidence for an accessibility model of emotional self-report . Psychological Bulletin , 128 , 934 – 960 .
Rogelberg S. G. Conway J. M. Sederburg M. E. Spitzmuller C. Aziz S. , & Knight W. E . ( 2003 ). Profiling active and passive nonrespondents to an organizational survey . Journal of Applied Psychology , 88 , 1104 – 1114 . doi: 10.1037/0021-9010.88.6.1104
Rogosa D. R . ( 1995 ). Myths and methods: “Myths about longitudinal research” plus supplemental questions . In J. M. Gottman (Ed.), The analysis of change (pp. 3 – 66 ). Mahwah, NJ : Lawrence Erlbaum .
Rouder J. N. , & Lu J . ( 2005 ). An introduction to Bayesian hierarchical models with an application in the theory of signal detection . Psychonomic Bulletin & Review , 12 , 573 – 604 . doi: 10.3758/BF03196750
Rubin D. B . ( 1987 ). Multiple imputation for nonresponse in surveys . New York, NY : John Wiley .
Schafer J. L. , & Graham J. W . ( 2002 ). Missing data: Our view of the state of the art . Psychological Methods , 7 , 147 – 177 .
Schaie K. W . ( 1965 ). A general model for the study of developmental problems . Psychological bulletin , 64 , 92 – 107 . doi: 10.1037/h0022371
Schmitt N . ( 1982 ). The use of analysis of covariance structures to assess beta and gamma change . Multivariate Behavioral Research , 17 , 343 – 358 . doi: 10.1207/s15327906mbr1703_3
Shadish W. R. Cook T. D. , & Campbell D. T . ( 2002 ). Experimental and quasi-experimental designs for generalized causal inference . Boston, MA : Houghton Mifflin .
Shingles R . ( 1985 ). Causal inference in cross-lagged panel analysis . In H. M. Blalock (Ed.), Causal models in panel and experimental design (pp. 219 – 250 ). New York, NY : Aldine .
Singer E. , & Kulka R. A . ( 2002 ). Paying respondents for survey participation . In M. ver Ploeg R. A. Moffit , & C. F. Citro (Eds.), Studies of welfare populations: Data collection and research issues (pp. 105 – 128 ). Washington, DC : National Research Council .
Singer J. D. , & Willett J. B . ( 2003 ). Applied longitudinal data analysis: Modeling change and event occurrence . New York, NY : Oxford university press .
Sitzmann T. , & Yeo G . ( 2013 ). A meta-analytic investigation of the within-person self-efficacy domain: Is self-efficacy a product of past performance or a driver of future performance? Personnel Psychology , 66 , 531 – 568 . doi: 10.1111/peps.12035
Solomon R. L. , & Corbit J. D . ( 1974 ). An opponent-process theory of motivation: I. Temporal dynamics of affect . Psychological Review , 81 , 119 – 145 . doi: 10.1037/h0036128
Stasser G . ( 1988 ). Computer simulation as a research tool: The DISCUSS model of group decision making . Journal of Experimental Social Psychology , 24 , 393 – 422 . doi: 10.1016/ 0022-1031(88)90028-5
Stasser G . ( 2000 ). Information distribution, participation, and group decision: Explorations with the DISCUSS and SPEAK models . In D. R. Ilgen R. Daniel , & C. L. Hulin (Eds.), Computational modeling of behavior in organizations: The third scientific discipline (pp. 135 – 161 ). Washington, DC : American Psychological Association .
Stone-Romero E. F. , & Rosopa P. J . ( 2010 ). Research design options for testing mediation models and their implications for facets of validity . Journal of Managerial Psychology , 25 , 697 – 712 . doi: 10.1108/02683941011075256
Tay L . ( 2015 ). Expimetrics [Computer software] . Retrieved from http://www.expimetrics.com
Tay L. Chan D. , & Diener E . ( 2014 ). The metrics of societal happiness . Social Indicators Research , 117 , 577 – 600 . doi: 10.1007/s11205-013-0356-1
Taris T . ( 2000 ). Longitudinal data analysis . London, UK : Sage Publications .
Tesluk P. E. , & Jacobs R. R . ( 1998 ). Toward an integrated model of work experience . Personnel Psychology , 51 , 321 – 355 . doi: 10.1111/j.1744-6570.1998.tb00728.x
Tisak J. , & Tisak M. S . ( 2000 ). Permanency and ephemerality of psychological measures with application to organizational commitment . Psychological Methods , 5 , 175 – 198 .
Uy M. A. Foo M. D. , & Aguinis H . ( 2010 ). Using experience sampling methodology to advance entrepreneurship theory and research . Organizational Research Methods , 13 , 31 – 54 . doi: 10.1177/1094428109334977
Vancouver J. B. Gullekson N. , & Bliese P . ( 2007 ). Lagged Regression as a Method for Causal Analysis: Monte Carlo Analyses of Possible Artifacts . Poster submitted to the annual meeting of the Society for Industrial and Organizational Psychology, New York .
Vancouver J. B. Tamanini K. B. , & Yoder R. J . ( 2010 ). Using dynamic computational models to reconnect theory and research: Socialization by the proactive newcomer exemple . Journal of Management , 36 , 764 – 793 . doi: 10.1177/0149206308321550
Vancouver J. B. , & Weinhardt J. M . ( 2012 ). Modeling the mind and the milieu: Computational modeling for micro-level organizational researchers . Organizational Research Methods , 15 , 602 – 623 . doi: 10.1177/1094428112449655
Vancouver J. B. Weinhardt J. M. , & Schmidt A. M . ( 2010 ). A formal, computational theory of multiple-goal pursuit: integrating goal-choice and goal-striving processes . Journal of Applied Psychology , 95 , 985 – 1008 . doi: 10.1037/a0020628
Vandenberg R. J. , & Lance C. E . ( 2000 ). A review and synthesis of the measurement invariance literature: Suggestions, practices, and recommendations for organizational research . Organizational research methods , 3 , 4 – 70 . doi: 10.1177/109442810031002
Wagenmakers E. J . ( 2007 ). A practical solution to the pervasive problems of p values . Psychonomic Bulletin & Review , 14 , 779 – 804 . doi: 10.3758/BF03194105
Wang M . ( 2007 ). Profiling retirees in the retirement transition and adjustment process: Examining the longitudinal change patterns of retirees’ psychological well-being . Journal of Applied Psychology , 92 , 455 – 474 . doi: 10.1037/0021-9010.92.2.455
Wang M. , & Bodner T. E . ( 2007 ). Growth mixture modeling: Identifying and predicting unobserved subpopulations with longitudinal data . Organizational Research Methods , 10 , 635 – 656 . doi: 10.1177/1094428106289397
Wang M. , & Chan D . ( 2011 ). Mixture latent Markov modeling: Identifying and predicting unobserved heterogeneity in longitudinal qualitative status change . Organizational Research Methods , 14 , 411 – 431 . doi: 10.1177/1094428109357107
Wang M. , & Hanges P . ( 2011 ). Latent class procedures: Applications to organizational research . Organizational Research Methods , 14 , 24 – 31 . doi: 10.1177/1094428110383988
Wang M. Henkens K. , & van Solinge H . ( 2011 ). Retirement adjustment: A review of theoretical and empirical advancements . American Psychologist , 66 , 204 – 213 . doi: 10.1037/a0022414
Wang M. Zhou L. , & Zhang Z . ( 2016 ). Dynamic modeling . Annual Review of Organizational Psychology and Organizational Behavior , 3 , 241 – 266 .
Wang L. P. Hamaker E. , & Bergeman C. S . ( 2012 ). Investigating inter-individual differences in short-term intra-individual variability . Psychological Methods , 17 , 567 – 581 . doi: 10.1037/a0029317
Warren D. A . ( 2015 ). Pathways to retirement in Australia: Evidence from the HILDA survey . Work, Aging and Retirement , 1 , 144 – 165 . doi: 10.1093/workar/wau013
Weikamp J. G. , & Göritz A. S . ( 2015 ). How stable is occupational future time perspective over time? A six-wave study across 4 years . Work, Aging and Retirement , 1 , 369 – 381 . doi: 10.1093/workar/wav002
Weinhardt J. M. , & Vancouver J. B . ( 2012 ). Computational models and organizational psychology: Opportunities abound . Organizational Psychology Review , 2 , 267 – 292 . doi: 10.1177/2041386612450455
Weiss H. M. , & Cropanzano R . ( 1996 ). Affective Events Theory: A theoretical discussion of the structure, causes and consequences of affective experiences at work . Research in Organizational Behavior , 18 , 1 – 74 .
Zacks J. M. Speer N. K. Swallow K. M. Braver T. S. , & Reynolds J. R . ( 2007 ). Event perception: a mind-brain perspective . Psychological Bulletin , 133 , 273 – 293 . doi: 10.1037/0033-2909.133.2.273
Author notes
Email alerts, citing articles via.
- Recommend to your Library
Affiliations
- Online ISSN 2054-4650
- Copyright © 2024 Oxford University Press
- About Oxford Academic
- Publish journals with us
- University press partners
- What we publish
- New features
- Open access
- Institutional account management
- Rights and permissions
- Get help with access
- Accessibility
- Advertising
- Media enquiries
- Oxford University Press
- Oxford Languages
- University of Oxford
Oxford University Press is a department of the University of Oxford. It furthers the University's objective of excellence in research, scholarship, and education by publishing worldwide
- Copyright © 2024 Oxford University Press
- Cookie settings
- Cookie policy
- Privacy policy
- Legal notice
This Feature Is Available To Subscribers Only
Sign In or Create an Account
This PDF is available to Subscribers Only
For full access to this pdf, sign in to an existing account, or purchase an annual subscription.
- Open access
- Published: 01 October 2022
Qualitative longitudinal research in health research: a method study
- Åsa Audulv 1 ,
- Elisabeth O. C. Hall 2 , 3 ,
- Åsa Kneck 4 ,
- Thomas Westergren 5 , 6 ,
- Liv Fegran 5 ,
- Mona Kyndi Pedersen 7 , 8 ,
- Hanne Aagaard 9 ,
- Kristianna Lund Dam 3 &
- Mette Spliid Ludvigsen 10 , 11
BMC Medical Research Methodology volume 22 , Article number: 255 ( 2022 ) Cite this article
11k Accesses
21 Citations
2 Altmetric
Metrics details
Qualitative longitudinal research (QLR) comprises qualitative studies, with repeated data collection, that focus on the temporality (e.g., time and change) of a phenomenon. The use of QLR is increasing in health research since many topics within health involve change (e.g., progressive illness, rehabilitation). A method study can provide an insightful understanding of the use, trends and variations within this approach. The aim of this study was to map how QLR articles within the existing health research literature are designed to capture aspects of time and/or change.
This method study used an adapted scoping review design. Articles were eligible if they were written in English, published between 2017 and 2019, and reported results from qualitative data collected at different time points/time waves with the same sample or in the same setting. Articles were identified using EBSCOhost. Two independent reviewers performed the screening, selection and charting.
A total of 299 articles were included. There was great variation among the articles in the use of methodological traditions, type of data, length of data collection, and components of longitudinal data collection. However, the majority of articles represented large studies and were based on individual interview data. Approximately half of the articles self-identified as QLR studies or as following a QLR design, although slightly less than 20% of them included QLR method literature in their method sections.
Conclusions
QLR is often used in large complex studies. Some articles were thoroughly designed to capture time/change throughout the methodology, aim and data collection, while other articles included few elements of QLR. Longitudinal data collection includes several components, such as what entities are followed across time, the tempo of data collection, and to what extent the data collection is preplanned or adapted across time. Therefore, there are several practices and possibilities researchers should consider before starting a QLR project.
Peer Review reports
Health research is focused on areas and topics where time and change are relevant. For example, processes such as recovery or changes in health status. However, relating time and change can be complicated in research, as the representation of reality in research publications is often collected at one point in time and fixed in its presentation, although time and change are always present in human life and experiences. Qualitative longitudinal research (QLR; also called longitudinal qualitative research, LQR) has been developed to focus on subjective experiences of time or change using qualitative data materials (e.g., interviews, observations and/or text documents) collected across a time span with the same participants and/or in the same setting [ 1 , 2 ]. QLR within health research may have many benefits. Firstly, human experiences are not fixed and consistent, but changing and diverse, therefore people’s experiences in relation to a health phenomenon may be more comprehensively described by repeated interviews or observations over time. Secondly, experiences, behaviors, and social norms unfold over time. By using QLR, researchers can collect empirical data that represents not only recalled human conceptions but also serial and instant situations reflecting transitions, trajectories and changes in people’s health experiences, personal development or health care organizations [ 3 , 4 , 5 ].
Key features of QLR
Whether QLR is a methodological approach in its own right or a design element of a particular study within a traditional methodological approach (e.g., ethnography or grounded theory) is debated [ 1 , 6 ]. For example, Bennett et al. [ 7 ] describe QLR as untied to methodology, giving researchers the flexibility to develop a suitable design for each study. McCoy [ 6 ] suggests that epistemological and ontological standpoints from interpretative phenomenological analysis (IPA) align with QLR traditions, thus making longitudinal IPA a suitable methodology. Plano-Clark et al. [ 8 ] described how longitudinal qualitative elements can be used in mixed methods studies, thus creating longitudinal mixed methods. In contrast, several researchers have argued that QLR is an emerging methodology [ 1 , 5 , 9 , 10 ]. For example, Thomson et al. [ 9 ] have stated “What distinguishes longitudinal qualitative research is the deliberate way in which temporality is designed into the research process, making change a central focus of analytic attention” (p. 185). Tuthill et al. [ 5 ] concluded that some of the confusion might have arisen from the diversity of data collection methods and data materials used within QLR research. However, there are no investigations showing to what extent QLR studies use QLR as a distinct methodology versus using a longitudinal data collection as a more flexible design element in combination with other qualitative methodologies.
QLR research should focus on aspects of temporality, time and/or change [ 11 , 12 , 13 ]. The concepts of time and change are seen as inseparable since change is happening with the passing of time [ 13 ]. However, time can be conceptualized in different ways. Time is often understood from a chronological perspective, and is viewed as fixed, objective, continuous and measurable (e.g., clock time, duration of time). However, time can also be understood from within, as the experience of the passing of time and/or the perspective from the current moment into the constructed conception of a history or future. From this perspective, time is seen as fluid, meaning that events, contexts and understandings create a subjective experience of time and change. Both the chronological and fluid understanding of time influence QLR research [ 11 ]. Furthermore, there is a distinction between over-time, which constitutes a comparison of the difference between points in time, often with a focus on the latter point or destination, and through-time, which means following an aspect across time while trying to understand the change that occurs [ 11 ]. In this article, we will mostly use the concept of across time to include both perspectives.
Some authors assert that QLR studies should include a qualitative data collection with the same sample across time [ 11 , 13 ], whereas Thomson et al. [ 9 ] also suggest the possibility of returning to the same data collection site with the same or different participants. When a QLR study involves data collection in shorter engagements, such as serial interviews, these engagements are often referred to as data collection time points. Data collection in time waves relates to longer engagements, such as field work/observation periods. There is no clear-cut definition for the minimum time span of a QLR study; instead, the length of the data collection period must be decided based upon what processes or changes are the focus of the study [ 13 ].
Most literature describing QLR methods originates from the social sciences, where the approach has a long tradition [ 1 , 10 , 14 ]. In health research, one-time-data collection studies have been the norm within qualitative methods [ 15 ], although health research using QLR methods has increased in recent years [ 2 , 5 , 16 , 17 ]. However, collecting and managing longitudinal data has its own sets of challenges, especially regarding how to integrate perspectives of time and/or change in the data collection and subsequent analysis [ 1 ]. Therefore, a study of QLR articles from the health research literature can provide an insightful understanding of the use, trends and variations of how methods are used and how elements of time/change are integrated in QLR studies. This could, in turn, provide inspiration for using different possibilities of collecting data across time when using QLR in health research. The aim of this study was to map how QLR articles within the existing health research literature are designed to capture aspects of time and/or change.
More specifically, the research questions were:
What methodological approaches are described to inform QLR research?
What methodological references are used to inform QLR research?
How are longitudinal perspectives articulated in article aims?
How is longitudinal data collection conducted?
In this method study, we used an adapted scoping review method [ 18 , 19 , 20 ]. Method studies are research conducted on research studies to investigate how research design elements are applied across a field [ 21 ]. However, since there are no clear guidelines for method studies, they often use adapted versions of systematic reviews or scoping review methods [ 21 ]. The adaptations of the scoping review method consisted of 1) using a large subsample of studies (publications from a three-year period) instead of including all QLR articles published, and 2) not including grey literature. The reporting of this study was guided by the Preferred Reporting Items for Systematic reviews and Meta-Analyses extension for Scoping Reviews (PRISMA-ScR) checklist [ 20 , 22 ] (see Additional file 1 ). A (unpublished) protocol was developed by the research team during the spring of 2019.
Eligibility criteria
In line with method study recommendations [ 21 ], we decided to draw on a manageable subsample of published QLR research. Articles that were eligible for inclusion were health research primary studies written in English, published between 2017 and 2019, and with a longitudinal qualitative data collection. Our operating definition for qualitative longitudinal data collection was data collected at different time points (e.g., repeated interviews) or time waves (e.g., periods of field work) involving the same sample or conducted in the same setting(s). We intentionally selected a broad inclusion criterion for QLR since we wanted a wide variety of articles. The selected time period was chosen because the first QLR method article directed towards health research was published in 2013 [ 1 ] and during the following years the methodological resources for QLR increased [ 3 , 8 , 17 , 23 , 24 , 25 ], thus we could expect that researchers publishing QLR in 2017–2019 should be well-grounded in QLR methods. Further, we found that from 2012 to 2019 the rate of published QLR articles were steady at around 100 publications per year, so including those from a three-year period would give a sufficient number of articles (~ 300 articles) for providing an overview of the field. Published conference abstracts, protocols, articles describing methodological issues, review articles, and non-research articles (e.g., editorials) were excluded.
Search strategy
Relevant articles were identified through systematic searches in EBSCOhost, including biomedical and life science research and nursing and allied health literature. A librarian who specialized in systematic review searches developed and performed the searches, in collaboration with the author team (LF, TW & ÅA). In the search, the term “longitudinal” was combined with terms for qualitative research (for the search strategy see Additional file 2 ). The searches were conducted in the autumn of 2019 (last search 2019-09-10).
Study selection
All identified citations were imported into EndNote X9 ( www.endnote.com ) and further imported into Rayyan QCRI online software [ 26 ], and duplicates were removed. All titles and abstracts were screened against the eligibility criteria by two independent reviewers (ÅA & EH), and conflicting decisions were discussed until resolved. After discussions by the team, we decided to include articles published between 2017 and 2019, that selection alone included 350 records with diverse methods and designs. The full texts of articles that were eligible for inclusion were retrieved. In the next stage, two independent reviewers reviewed each full text article to make final decisions regarding inclusion (ÅA, EH, Julia Andersson). In total, disagreements occurred in 8% of the decisions, and were resolved through discussion. Critical appraisal was not assessed since the study aimed to describe the range of how QLR is applied and not aggregate research findings [ 21 , 22 ].
Data charting and analysis
A standardized charting form was developed in Excel (Excel 2016). The charting form was reviewed by the research team and pretested in two stages. The tests were performed to increase internal consistency and reduce the risk of bias. First, four articles were reviewed by all the reviewers, and modifications were made to the form and charting instructions. In the next stage, all reviewers used the charting form on four other articles, and the convergence in ratings was 88%. Since the convergence was under 90%, charting was performed in duplicate to reduce errors in the data. At the end of the charting process, the convergence among the reviewers was 95%. The charting was examined by the first author, who revised the charting in cases of differences.
Data items that were charted included 1) the article characteristics (e.g., authors, publication year, journal, country), 2) the aim and scope (e.g., phenomenon of interest, population, contexts), 3) the stated methodology and analysis method, 4) text describing the data collection (e.g., type of data material, number of participants, time frame of data collection, total amount of data material), and 5) the qualitative methodological references used in the methods section. Extracted text describing data collection could consist of a few sentences or several sections from the articles (and sometimes figures) concerning data collection practices, rational for time periods and research engagement in the field. This was later used to analyze how the longitudinal data collection was conducted and elements of longitudinal design. To categorize the qualitative methodology approaches, a framework from Cresswell [ 27 ] was used (including the categories for grounded theory, phenomenology, ethnography, case study and narrative research). Overall, data items needed to be explicitly stated in the articles in order to be charted. For example, an article was categorized as grounded theory if it explicitly stated “in this grounded theory study” but not if it referred to the literature by Glaser and Strauss without situating itself as a grounded theory study (See Additional file 3 for the full instructions for charting).
All charting forms were compiled into a single Microsoft Excel spreadsheet (see Supplementary files for an overview of the articles). Descriptive statistics with frequencies and percentages were calculated to summarize the data. Furthermore, an iterative coding process was used to group the articles and investigate patterns of, for example, research topics, words in the aims, or data collection practices. Alternative ways of grouping and presenting the data were discussed by the research team.
Search and selection
A total of 2179 titles and abstracts were screened against the eligibility criteria (see Fig. 1 ). The full text of one article could not be found and the article was excluded [ 28 ]. Fifty full text articles were excluded. Finally, 299 articles, representing 271 individual studies, were included in this study (see additional files 4 and 5 respectively for tables of excluded and included articles).
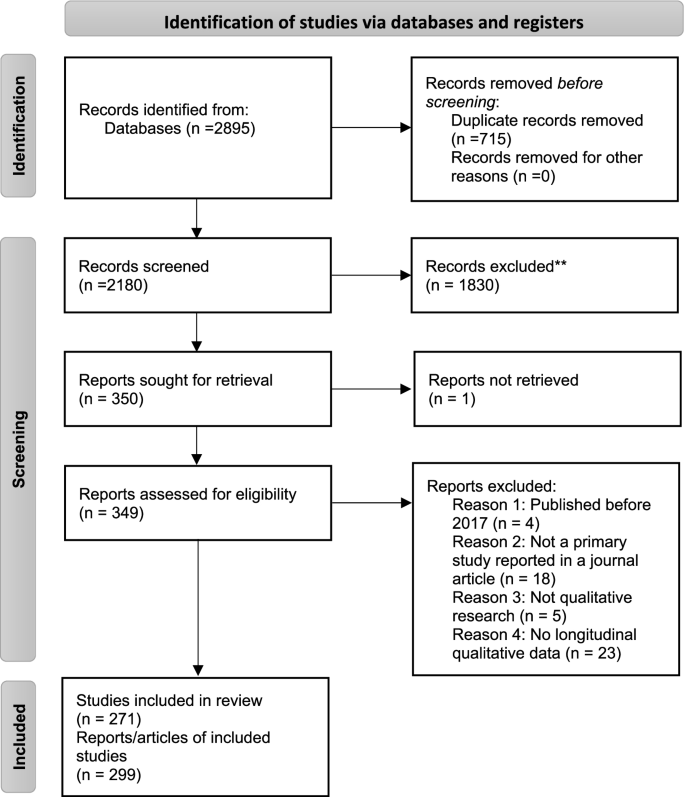
PRISMA diagram of study selection]
General characteristics and research areas of the included articles
The articles were published in many journals ( n = 193), and 138 of these journals were represented with one article each. BMJ Open was the most prevalent journal ( n = 11), followed by the Journal of Clinical Nursing ( n = 8). Similarly, the articles represented many countries ( n = 41) and all the continents; however, a large part of the studies originated from the US or UK ( n = 71, 23.7% and n = 70, 23.4%, respectively). The articles focused on the following types of populations: patients, families−/caregivers, health care providers, students, community members, or policy makers. Approximately 20% ( n = 63, 21.1%) of the articles collected data from two or more of these types of population(s) (see Table 1 ).
Approximately half of the articles ( n = 158, 52.8%) articulated being part of a larger research project. Of them, 95 described a project with both quantitative and qualitative methods. They represented either 1) a qualitative study embedded in an intervention, evaluation or implementation study ( n = 66, 22.1%), 2) a longitudinal cohort study collecting both quantitative and qualitative material ( n = 23, 7.7%), or 3) qualitative longitudinal material collected together with a cross sectional survey (n = 6, 2.0%). Forty-eight articles (16.1%) described belonging to a larger qualitative project presented in several research articles.
Methodological traditions
Approximately one-third ( n = 109, 36.5%) of the included articles self-identified with one of the qualitative traditions recognized by Cresswell [ 27 ] (case study: n = 36, 12.0%; phenomenology: n = 35, 11.7%; grounded theory: n = 22, 7.4%; ethnography: n = 13, 4.3%; narrative method: n = 3, 1.0%). In nine articles, the authors described using a mix of two or more of these qualitative traditions. In addition, 19 articles (6.4%) self-identified as mixed methods research.
Every second article self-identified as having a qualitative longitudinal design ( n = 156, 52.2%); either they self-identified as “a longitudinal qualitative study” or “using a longitudinal qualitative research design”. However, in some articles, this was stated in the title and/or abstract and nowhere else in the article. Fifty-two articles (17.4%) self-identified both as having a QLR design and following one of the methodological approaches (case study: n = 8; phenomenology: n = 23; grounded theory: n = 9; ethnography: n = 6; narrative method: n = 2; mixed methods: n = 4).
The other 143 articles used various terms to situate themselves in relation to a longitudinal design. Twenty-seven articles described themselves as a longitudinal study (9.0%) or a longitudinal study within a specific qualitative tradition (e.g., a longitudinal grounded theory study or a longitudinal mixed method study) ( n = 64, 21.4%). Furthermore, 36 articles (12.0%) referred to using longitudinal data materials (e.g., longitudinal data or longitudinal interviews). Nine of the articles (3.0%) used the term longitudinal in relation to the data analysis or aim (e.g., the aim was to longitudinally describe), used terms such as serial or repeated in relation to the data collection design ( n = 2, 0.7%), or did not use any term to address the longitudinal nature of their design ( n = 5, 1.7%).
Use of methodological references
The mean number of qualitative method references in the methods sections was 3.7 (range 0 to 16), and 20 articles did not have any qualitative method reference in their methods sections. Footnote 1 Commonly used method references were generic books on qualitative methods, seminal works within qualitative traditions, and references specializing in qualitative analysis methods (see Table 2 ). It should be noted that some references were comprehensive books and thus could include sections about QLR without being focused on the QLR method. For example, Miles et al. [ 31 ] is all about analysis and coding and includes a chapter regarding analyzing change.
Only approximately 20% ( n = 58) of the articles referred to the QLR method literature in their methods sections. Footnote 2 The mean number of QLR method references (counted for articles using such sources) was 1.7 (range 1 to 6). Most articles using the QLR method literature also used other qualitative methods literature (except two articles using one QLR literature reference each [ 39 , 40 ]). In total, 37 QLR method references were used, and 24 of the QLR method references were only referred to by one article each.
Longitudinal perspectives in article aims
In total, 231 (77.3%) articles had one or several terms related to time or change in their aims, whereas 68 articles (22.7%) had none. Over one hundred different words related to time or change were identified. Longitudinally oriented terms could focus on changes across time (process, trajectory, transition, pathway or journey), patterns of how something changed (maintenance, continuity, stability, shifts), or phenomena that by nature included change (learning or implementation). Other types of terms emphasized the data collection time period (e.g., over 6 months) or a specific changing situation (e.g., during pregnancy, through the intervention period, or moving into a nursing home). The most common terms used for the longitudinal perspective were change ( n = 63), over time ( n = 52), process ( n = 36), transition ( n = 24), implementation ( n = 14), development ( n = 13), and longitudinal (n = 13). Footnote 3
Furthermore, the articles varied in what ways their aims focused on time/change, e.g., the longitudinal perspectives in the aims (see Table 3 ). In 71 articles, the change across time was the phenomenon of interest of the article : for example, articles investigating the process of learning or trajectories of diseases. In contrast, 46 articles investigated change or factors impacting change in relation to a defined outcome : for example, articles investigating factors influencing participants continuing in a physical activity trial. The longitudinal perspective could also be embedded in an article’s context . In such cases, the focus of the article was on experiences that happened during a certain time frame or in a time-related context (e.g., described experiences of the patient-provider relationship during 6 months of rehabilitation).
Types of data and length of data collection
The QLR articles were often large and complex in their data collection methods. The median number of participants was 20 (range from one to 1366, the latter being an article with open-ended questions in questionnaires [ 46 ]). Most articles used individual interviews as the data material ( n = 167, 55.9%) or a combination of data materials ( n = 98, 32.8%) (e.g., interviews and observations, individual interviews and focus group interviews, or interviews and questionnaires). Forty-five articles (15.1%) presented quantitative and qualitative results. The median number of interviews was 46 (range three to 507), which is large in comparison to many qualitative studies. The observation materials were also comprehensive and could include several hundred hours of observations. Documents were often used as complementary material and included official documents, newspaper articles, diaries, and/or patient records.
The articles’ time spans Footnote 4 for data collection varied between a few days and over 20 years, with 60% of the articles’ time spans being 1 year or shorter ( n = 180) (see Fig. 2 ). The variation in time spans might be explained by the different kinds of phenomena that were investigated. For example, Jensen et al. [ 47 ] investigated hospital care delivery and followed each participant, with observations lasting between four and 14 days. Smithbattle [ 48 ] described the housing trajectories of teen mothers, and collected data in seven waves over 28 years.
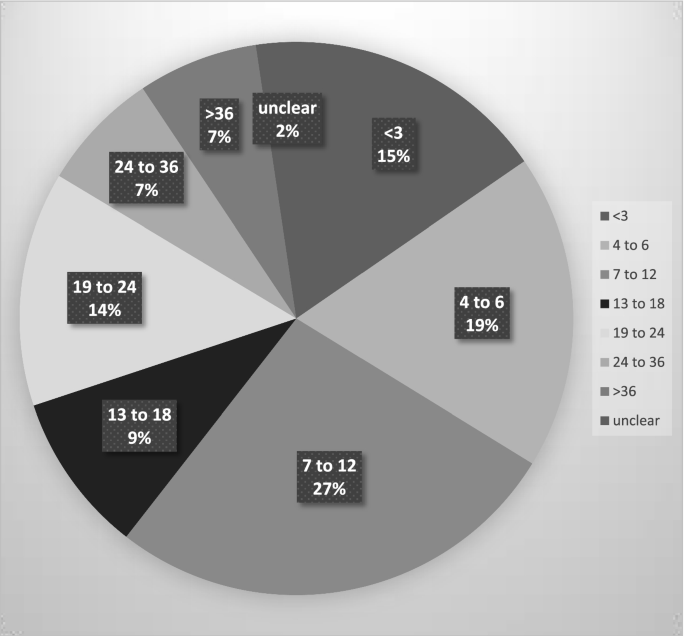
Number of articles in relation to the time span of data collection. The time span of data collection is given in months
Three components of longitudinal data collection
In the articles, the data collection was conducted in relation to three different longitudinal data collection components (see Table 4 ).
Entities followed across time
Four different types of entities were followed across time: 1) individuals, 2) individual cases or dyads, 3) groups, and 4) settings. Every second article ( n = 170, 56.9%) followed individuals across time, thus following the same participants through the whole data collection period. In contrast, when individual cases were followed across time, the data collection was centered on the primary participants (e.g., people with progressive neurological conditions) who were followed over time, and secondary participants (e.g., family caregivers) might provide complementary data at several time points or only at one-time point. When settings were followed over time, the participating individuals were sometimes the same, and sometimes changed across the data collection period. Typical settings were hospital wards, hospitals, smaller communities or intervention trials. The type of collected data corresponded with what kind of entities were followed longitudinally. Individuals were often followed with serial interviews, whereas groups were commonly followed with focus group interviews complemented with individual interviews, observations and/or questionnaires. Overall, the lengths of data collection periods seemed to be chosen based upon expected changes in the chosen entities. For example, the articles following an intervention setting were structured around the intervention timeline, collecting data before, after and sometimes during the intervention.
Tempo of data collection
The data collection tempo differed among the articles (e.g., the frequency and mode of the data collection). Approximately half ( n = 154, 51.5%) of the articles used serial time points, collecting data at several reoccurring but shorter sequences (e.g., through serial interviews or open-ended questions in questionnaires). When data were collected in time waves ( n = 50, 16.7%), the periods of data collection were longer, usually including both interviews and observations; often, time waves included observations of a setting and/or interviews at the same location over several days or weeks.
When comparing the tempo with the type of entities, some patterns were detected (see Fig. 3 ). When individuals were followed, data were often collected at time points, mirroring the use of individual interviews and/or short observations. For research in settings, data were commonly collected in time waves (e.g., observation periods over a few weeks or months). In studies exploring settings across time, time waves were commonly used and combined several types of data, particularly from interviews and observations. Groups were the least common studied entity ( n = 9, 3.0%), so the numbers should be interpreted with caution, but continuous data collection was used in five of the nine studies. The continuous data collection mode was, for example, collecting electronic diaries [ 62 ] or minutes from committee meetings during a time period [ 63 ].
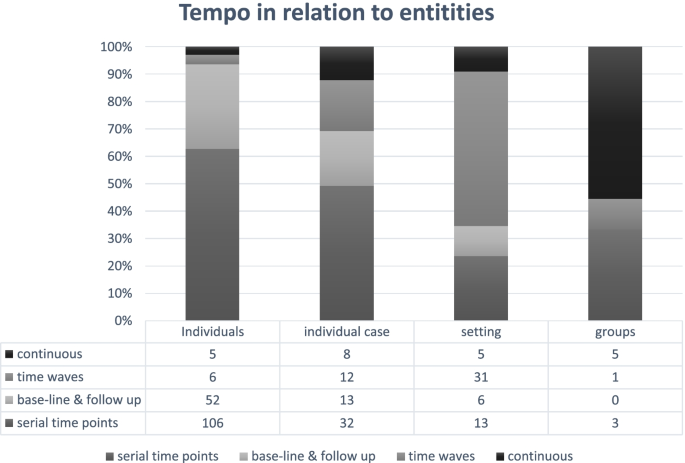
Tempo of data collection in relation to entities followed over time
Preplanned or adapted data collection
A large majority ( n = 224, 74.9%) of the articles used preplanned data collection (e.g., in preplanned data collection, all participants were followed across time according to the same data collection plan). For example, all participants were interviewed one, six and twelve months’ post-diagnosis. In contrast to the preplanned data collection approach, 44 articles had a participant-adapted data collection (14.7%), and participants were followed at different frequencies and/or over various lengths of time depending on each participant’s situation. Participant-adapted data collection was more common among articles following individuals or individual cases (see Fig. 4 ). To adapt the data collection to the participants, the researchers created strategies to reach participants when crucial events were happening. Eleven articles used a participant entry approach to data collection ( n = 11, 6.7%), and the whole or parts of the data were independently sent in by participants in the form of diaries, questionnaires, or blogs. Another approach to data collection was using theoretical or analysis-driven ideas to guide the data collection ( n = 19, 6.4%). In these articles, the analysis and data collection were conducted simultaneously, and ideas arising in the analysis could be followed up, for example, returning to some participants, recruiting participants with specific experiences, or collecting complementary types of data materials. This approach was most common in the articles following settings across time, which often included observations and interviews with different types of populations. Articles using theoretical or analysis driven data collection were not associated with grounded theory to a greater extent than the other articles in the sample (e.g., did not self-identify as grounded theory or referred to methodological literature within grounded theory traditions to a greater proportion).
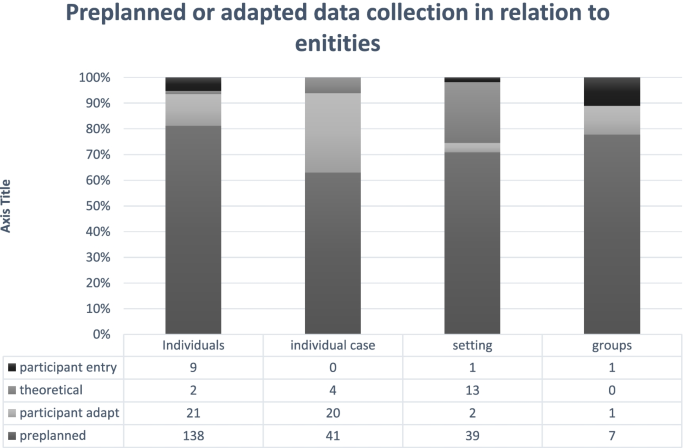
Preplanned or adapted data collection in relation to entities followed over time
According to our results, some researchers used QLR as a methodological approach and other researchers used a longitudinal qualitative data collection without aiming to investigate change. Adding to the debate on whether QLR is a methodological approach in its own right or a design element in a particular study we suggest that the use of QLR can be described as layered (see Fig. 5 ). Namely, articles must fulfill several criteria in order to use QLR as a methodological approach, and that is done in some articles. In those articles QLR method references were used, the aim was to investigate change of a phenomenon and the longitudinal elements of the data collection were thoroughly integrated into the method section. On the other hand, some articles using a longitudinal qualitative data collection were just collecting data over time, without addressing time and/or change in the aim. These articles can still be interesting research studies with valuable results, but they are not using the full potential of QLR as a methodological approach. In all, around 40% of the articles had an aim that focused on describing or understanding change (either as phenomenon or outcome); but only about 24% of the articles set out to investigate change across time as their phenomenon of interest.
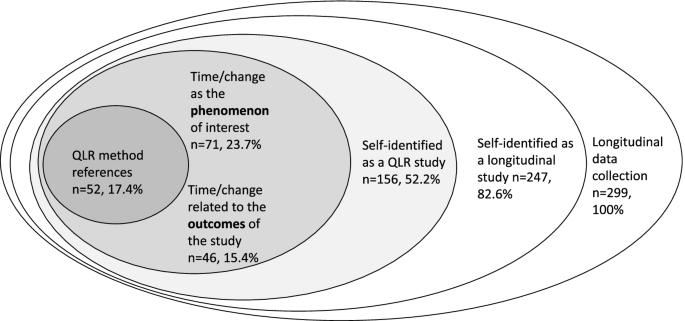
The QLR onion. The use of QLR design can be described as layered, where researchers use more or less elements of a QLR design. The two inmost layers represents articles using QLR as a methodological approach
Regarding methodological influences, about one-third of the articles self-identify with any of the traditional qualitative methodologies. Using a longitudinal qualitative data collection as an element integrated with another methodological tradition can therefore be seen as one way of working with longitudinal qualitative materials. In our results, the articles referring to methodologies other than QLR preferably used case study, phenomenology and grounded theory methodologies. This was surprising since Neale [ 10 ] identified ethnography, case studies and narrative methods as the main methodological influences on QLR. Our findings might mirror the profound impacts that phenomenology and grounded theory have had on the qualitative field of health research. Regarding phenomenology, the findings can also be influenced by more recent discussions of combining interpretative phenomenological analysis with QLR [ 6 ].
Half of the articles self-identified as QLR studies, but QLR method references were used in less than 20% of the identified articles. This is both surprising and troublesome since use of appropriate method literature might have supported researchers who were struggling with for example a large quantity of materials and complex analysis. A possible explanation for the lack of use of QLR method literature is that QLR as a methodological approach is not well known, and authors might not be aware that method literature exists. It is quite understandable that researchers can describe a qualitative project with longitudinal data collection as a qualitative longitudinal study, without being aware that QLR is a specific form of study. Balmer [ 64 ] described how their group conducted serial interviews with medical students over several years before they became aware of QLR as a method of study. Within our networks, we have met researchers with similar experiences. Likewise, peer reviewers and editorial boards might not be accustomed to evaluating QLR manuscripts. In our results, 138 journals published one article between 2017 and 2019, and that might not be enough for editorial boards and peer reviewers to develop knowledge to enable them to closely evaluate manuscripts with a QLR method.
In 2007, Holland and colleagues [ 65 ] mapped QLR in the UK and described the following four categories of QLR: 1) mixed methods approaches with a QLR component; 2) planned prospective longitudinal studies; 3) follow-up studies complementing a previous data collection with follow-up; and 4) evaluation studies. Examples of all these categories can be found among the articles in this method study; however, our results do paint a more complex picture. According to our results, Holland’s categories are not multi-exclusive. For example, studies with intentions to evaluate or implement practices often used a mixed methods design and were therefore eligible for both categories one and four described above. Additionally, regarding the follow-up studies, it was seldom clearly described if they were planned as a two-time-point study or if researchers had gained an opportunity to follow up on previous data collection. When we tried to categorize QLR articles according to the data collection design, we could not identify multi-exclusive categories. Instead, we identified the following three components of longitudinal data collection: 1) entities followed across time; 2) tempo; and 3) preplanned or adapted data collection approaches. However, the most common combination was preplanned studies that followed individuals longitudinally with three or more time points.
The use of QLR differs between disciplines [ 14 ]. Our results show some patterns for QLR within health research. Firstly, the QLR projects were large and complex; they often included several types of populations and various data materials, and were presented in several articles. Secondly, most studies focused upon the individual perspective, following individuals across time, and using individual interviews. Thirdly, the data collection periods varied, but 53% of the articles had a data collection period of 1 year or shorter. Finally, patients were the most prevalent population, even though topics varied greatly. Previously, two other reviews that focused on QLR in different parts of health research (e.g., nursing [ 4 ] and gerontology [ 66 ]) pointed in the same direction. For example, individual interviews or a combination of data materials were commonly used, and most studies were shorter than 1 year but a wide range existed [ 4 , 66 ].
Considerations when planning a QLR project
Based on our results, we argue that when health researchers plan a QLR study, they should reflect upon their perspective of time/change and decide what part change should play in their QLR study. If researchers decide that change should play the main role in their project, then they should aim to focus on change as the phenomenon of interest. However, in some research, change might be an important part of the plot, without having the main role, and change in relation to the outcomes might be a better perspective. In such studies, participants with change, no change or different kinds of change are compared to explore possible explanations for the change. In our results, change in relation to the outcomes was often used in relation to intervention studies where participants who reached a desired outcome were compared to individuals who did not. Furthermore, for some research studies, change is part of the context in which the research takes place. This can be the case when certain experiences happen during a period of change; for example, when the aim is to explore the experience of everyday life during rehabilitation after stroke. In such cases a longitudinal data collection could be advisable (e.g., repeated interviews often give a deep relationship between interviewer and participants as well as the possibility of gaining greater depth in interview answers during follow-up interviews [ 15 ]), but the study might not be called a QLR study since it does not focus upon change [ 13 ]. We suggest that researchers make informed decisions of what kind of longitudinal perspective they set out to investigate and are transparent with their sources of methodological inspiration.
We would argue that length of data collection period, type of entities, and data materials should be in accordance with the type of change/changing processes that a study focuses on. Individual change is important in health research, but researchers should also remember the possibility of investigating changes in families, working groups, organizations and wider communities. Using these types of entities were less common in our material and could probably grant new perspectives to many research topics within health. Similarly, using several types of data materials can complement the insights that individual interviews can give. A large majority of the articles in our results had a preplanned data collection. Participant-adapted data collection can be a way to work in alignment with a “time-as-fluid” conceptualization of time because the events of subjective importance to participants can be more in focus and participants (or other entities) change processes can differ substantially across cases. In studies with lengthy and spaced-out data collection periods and/or uncertainty in trajectories, researchers should consider participant-adapted or participant entry data collection. For example, some participants can be followed for longer periods and/or with more frequency.
Finally, researchers should consider how to best publish and disseminate their results. Many QLR projects are large, and the results are divided across several articles when they are published. In our results, 21 papers self-identified as a mixed methods project or as part of a larger mixed methods project, but most of these did not include quantitative data in the article. This raises the question of how to best divide a large research project into suitable pieces for publication. It is an evident risk that the more interesting aspects of a mixed methods project are lost when the qualitative and quantitative parts are analyzed and published separately. Similar risks occur, for example, when data have been collected from several types of populations but are then presented per population type (e.g., one article with patient data and another with caregiver data). During the work with our study, we also came across studies where data were collected longitudinally, but the results were divided into publications per time point. We do not argue that these examples are always wrong, there are situations when these practices are appropriate. However, it often appears that data have been divided without much consideration. Instead, we suggest a thematic approach to dividing projects into publications, crafting the individual publications around certain ideas or themes and thus using the data that is most suitable for the particular research question. Combining several types of data and/or several populations in an analysis across time is in fact what makes QLR an interesting approach.
Strengths and limitations
This method study intended to paint a broad picture regarding how longitudinal qualitative methods are used within the health research field by investigating 299 published articles. Method research is an emerging field, currently with limited methodological guidelines [ 21 ], therefore we used scoping review method to support this study. In accordance with scoping review method we did not use quality assessment as a criterion for inclusion [ 18 , 19 , 20 ]. This can be seen as a limitation because we made conclusions based upon a set of articles with varying quality. However, we believe that learning can be achieved by looking at both good and bad examples, and innovation may appear when looking beyond established knowledge, or assessing methods from different angles. It should also be noted that the results given in percentages hold no value for what procedures that are better or more in accordance with QLR, the percentages simply state how common a particular procedure was among the articles.
As described, the included articles showed much variation in the method descriptions. As the basis for our results, we have only charted explicitly written text from the articles, which might have led to an underestimation of some results. The researchers might have had a clearer rationale than described in the reports. Issues, such as word restrictions or the journal’s scope, could also have influenced the amount of detail that was provided. Similarly, when charting how articles drew on a traditional methodology, only data from the articles that clearly stated the methodologies they used (e.g., phenomenology) were charted. In some articles, literature choices or particular research strategies could implicitly indicate that the researchers had been inspired by certain methodologies (e.g., referring to grounded theory literature and describing the use of simultaneous data collection and analysis could indicate that the researchers were influenced by grounded theory), but these were not charted as using a particular methodological tradition. We used the articles’ aims and objectives/research questions to investigate their longitudinal perspectives. However, as researchers have different writing styles, information regarding the longitudinal perspectives could have been described in surrounding text rather than in the aim, which might have led to an underestimation of the longitudinal perspectives.
The experience and diversity of the research team in our study was a strength. The nine authors on the team represent ten universities and three countries, and have extensive experience in different types of qualitative research, QLR and review methods. The different level of experiences with QLR within the team (some authors have worked with QLR in several projects and others have qualitative experience but no experience in QLR) resulted in interesting discussions that helped drive the project forward. These experiences have been useful for understanding the field.
Based on a method study of 299 articles, we can conclude that QLR in health research articles published between 2017 and 2019 often contain comprehensive complex studies with a large variation in topics. Some research was thoroughly designed to capture time/change throughout the methodology, focus and data collection, while other articles included a few elements of QLR. Longitudinal data collection included several components, such as what entities were followed across time, the tempo of data collection, and to what extent the data collection was preplanned or adapted across time. In sum, health researchers need to be considerate and make informed choices when designing QLR projects. Further research should delve deeper into what kind of research questions go well with QLR and investigate the best practice examples of presenting QLR findings.
Availability of data and materials
The datasets used and analyzed in this current study are available in supplementary file 6 .
Qualitative method references were defined as a journal article or book with a title that indicated an aim to guide researchers in qualitative research methods and/or research theories. Primary studies, theoretical works related to the articles’ research topics, protocols, and quantitative method literature were excluded. References written in a language other than English was also excluded since the authors could not evaluate their content.
QLR method references were defined as a journal article or book that 1) focused on qualitative methodological questions, 2) used terms such as ‘longitudinal’ or ‘time’ in the title so it was evident that the focus was on longitudinal qualitative research. Referring to another original QLR study was not counted as using QLR method literature.
Words were charted depending on their word stem, e.g., change, changes and changing were all charted as change.
It should be noted that here time span refers to the data collection related to each participant or case. Researchers could collect data for 2 years but follow each participant for 6 months.
Calman L, Brunton L, Molassiotis A. Developing longitudinal qualitative designs: lessons learned and recommendations for health services research. BMC Med Res Methodol. 2013;13:14.
Article PubMed PubMed Central Google Scholar
Solomon P, Nixon S, Bond V, Cameron C, Gervais N. Two approaches to longitudinal qualitative analyses in rehabilitation and disability research. Disabil Rehabil. 2020;42:3566–72.
Article PubMed Google Scholar
Grossoehme D, Lipstein E. Analyzing longitudinal qualitative data: the application of trajectory and recurrent cross-sectional approaches. BMC Res Notes. 2016;9:136.
SmithBattle L, Lorenz R, Reangsing C, Palmer JL, Pitroff G. A methodological review of qualitative longitudinal research in nursing. Nurs Inq. 2018;25:e12248.
Tuthill EL, Maltby AE, DiClemente K, Pellowski JA. Longitudinal qualitative methods in health behavior and nursing research: assumptions, design, analysis and lessons learned. Int J Qual Methods. 2020;19:10.
Article PubMed Central Google Scholar
McCoy LK. Longitudinal qualitative research and interpretative phenomenological analysis: philosophical connections and practical considerations. Qual Res Psychol. 2017;14:442–58.
Article Google Scholar
Bennett D, Kajamaa A, Johnston J. How to... Do longitudinal qualitative research. Clin Teach. 2020;17:489–92.
Plano Clark V, Anderson N, Wertz JA, Zhou Y, Schumacher K, Miaskowski C. Conceptualizing longitudinal mixed methods designs: a methodological review of health sciences research. J Mix Methods Res. 2014;23:1–23.
Google Scholar
Thomson R, Plumridge L, Holland J. Longitudinal qualitative research: a developing methodology. Int J Soc Res Methodol. 2003;6:185–7.
Neale B. The craft of qualitative longitudinal research: thousand oaks. Sage. 2021.
Balmer DF, Varpio L, Bennett D, Teunissen PW. Longitudinal qualitative research in medical education: time to conceptualise time. Med Educ. 2021;55:1253–60.
Smith N. Cross-sectional profiling and longitudinal analysis: research notes on analysis in the longitudinal qualitative study, 'Negotiating transitions to Citizenship'. Int J Soc Res Methodol. 2003;6:273–7.
Saldaña J. Longitudinal qualitative research - analyzing change through time. Walnut Creek: AltaMira Press; 2003.
Corden A, Millar J. Time and change: a review of the qualitative longitudinal research literature for social policy. Soc Policy Soc. 2007;6:583–92.
Thorne S. Interpretive description: qualitative research for applied practice (2nd ed): Routledge; 2016.
Book Google Scholar
Kneck Å, Audulv Å. Analyzing variations in changes over time: development of the pattern-oriented longitudinal analysis approach. Nurs Inq. 2019;26:e12288.
Whiffin CJ, Bailey C, Ellis-Hill C, Jarrett N. Challenges and solutions during analysis in a longitudinal narrative case study. Nurse Res. 2014;21:20–62.
Arksey H, O'Malley L. Scoping studies: towards a methodological framework. Int J Soc Res Methodol. 2005;8:19–32.
Levac D, Colquhoun H, O’Brien KK. Scoping studies: advancing the methodology. Implement Sci. 2010;5:69.
Peters MDJ, Marnie C, Tricco AC, Pollock D, Munn Z, Alexander L, et al. Updated methodological guidance for the conduct of scoping reviews. JBI Evidence Implementation. 2021;19:3–10.
Mbuagbaw L, Lawson DO, Puljak L, Allison DB, Thabane L. A tutorial on methodological studies: the what, when, how and why. BMC Med Res Methodol. 2020;20:226.
Tricco AC, Lillie E, Zarin W, O’Brien KK, Colquhoun H, Levac D, et al. PRISMA extension for scoping reviews (PRISMA-ScR): checklist and explanation. Ann Intern. 2018;169:467–73.
Neale B. Adding time into the mix: stakeholder ethics in qualitative longitudinal research. Methodological Innovations Online. 2013;8:6–20.
Henderson S, Holland J, McGrellis S, Sharpe S, Thomson R. Storying qualitative longitudinal research: sequence, voice and motif. Qual Res. 2016;12:16–34.
Balmer DF, Richards BF. Longitudinal qualitative research in medical education. Perspect Med Educ. 2017;6:306–10.
Ouzzani M, Hammady H, Fedorowicz Z, Elmagarmid A. Rayyan-a web and mobile app for systematic reviews. Syst Rev. 2016;5:210.
Creswell JW. Qualitative inquiry and research design: choosing among five approaches. 3rd ed: SAGE Publications; 2012.
McIntyre H, Fraser D. 'Hands-off' breastfeeding skill development in a UK, UNICEF baby friendly initiative pre-registration midwifery programme. MIDIRS Midwifery Digest. 2018;28:98–102.
Braun V, Clarke V. Using thematic analysis in psychology. Qual Res Psychol. 2006;3:77–101.
Patton MQ. Qualitative research and evaluation methods: integrating theory and practice. Sage. 2015.
Miles M, Huberman A, Saldaña J. Qualitative data analysis: a methods sourcebook. 4th ed: Sage Publications; 2020.
Miles MB, Huberman AM. Qualitative data analysis: an expanded sourcebook (2nd ed): Sage Publications; 1994.
Smith JA, Flowers P, Larkin M. Interpretative phenomenological analysis: theory, method and research. Sage. 2009.
Hsieh HF, Shannon SE. Three approaches to qualitative content analysis. Qual Health Res. 2005;15:1277–88.
Glaser B, Strauss A. The discovery of grounded theory: strategies for qualitative research. Aldine De Gruyter. 1967.
Tong A, Sainsbury P, Craig J. Consolidated criteria for reporting qualitative research (COREQ): a 32-item checklist for interviews and focus groups. Int J Qual Health Care. 2007;19:349–57.
Murray SA, Kendall M, Carduff E, Worth A, Harris FM, Lloyd A, et al. Use of serial qualitative interviews to understand patients' evolving experiences and needs. BMJ. 2009;339:b3702.
Thomson R, Holland J. Hindsight, foresight and insight: the challenges of longitudinal qualitative research. Int J Soc Res Methodol. 2003;6:233–44.
Morrow V, Tafere Y, Chuta N, Zharkevich I. "I started working because I was hungry": the consequences of food insecurity for children's well-being in rural Ethiopia. Soc Sci Med. 2017;182:1–9.
Solomon P, O'Brien KK, Nixon S, Letts L, Baxter L, Gervais N. Qualitative longitudinal study of episodic disability experiences of older women living with HIV in Ontario, Canada. BMJ Open. 2018;8:e021507.
Coombs MA, Parker R, de Vries K. Managing risk during care transitions when approaching end of life: a qualitative study of patients’ and health care professionals’ decision making. Palliat Med. 2017;31:617–24.
Vaghefi I, Tulu B. The continued use of mobile health apps: insights from a longitudinal study. JMIR Mhealth And Uhealth. 2019;7:e12983.
Andersen IC, Thomsen TG, Bruun P, Bødtger U, Hounsgaard L. Patients' and their family members' experiences of participation in care following an acute exacerbation in chronic obstructive pulmonary disease: a phenomenological-hermeneutic study. J Clin Nurs. 2017;26:4877–89.
Albrecht TA, Keim-Malpass J, Boyiadzis M, Rosenzweig M. Psychosocial experiences of young adults diagnosed with acute leukemia during hospitalization for induction chemotherapy treatment. JHPN. 2019;21:167–73.
PubMed Google Scholar
Corepal R, Best P, O'Neill R, Tully MA, Edwards M, Jago R, et al. Exploring the use of a gamified intervention for encouraging physical activity in adolescents: a qualitative longitudinal study in Northern Ireland. BMJ Open. 2018;8:e019663.
PubMed PubMed Central Google Scholar
Malin H, Liauw I, Damon W. Purpose and character development in early adolescence. J Youth Adolesc. 2017;46:1200–15.
Jensen AM, Pedersen BD, Olsen RB, Hounsgaard L. Medication and care in Alzheimer's patients in the acute care setting: a qualitative analysis. Dementia. 2019;18:2173–88.
SmithBattle L. Housing trajectories of teen mothers and their families over 28 years. Am J Orthop. 2019;89:258–67.
Denney-Koelsch EM, Côté-Arsenault D, Jenkins Hall W. Feeling cared for versus experiencing added burden: Parents' interactions with health-care providers in pregnancy with a lethal fetal diagnosis. Illn Crisis Loss. 2018;26:293–315.
Pyörälä E, Mäenpää S, Heinonen L, Folger D, Masalin T, Hervonen H. The art of note taking with mobile devices in medical education. BMC Med Educ. 2019;19:96.
Lindberg K, Mørk BE, Walter L. Emergent coordination and situated learning in a hybrid OR: the mixed blessing of using radiation. Soc Science Med. 2019;228:232–9.
Frost J, Wingham J, Britten N, Greaves C, Abraham C, Warren FC, et al. Home-based rehabilitation for heart failure with reduced ejection fraction: mixed methods process evaluation of the REACH-HF multicentre randomised controlled trial. BMJ Open. 2019;9:e026039.
Young JL, Werner-Lin A, Mueller R, Hoskins L, Epstein N, Greene MH. Longitudinal cancer risk management trajectories of BRCA1/2 mutation-positive reproductive-age women. J Psych Oncology. 2017;35:393–408.
Lewis M, Jones A, Hunter B. Women's experience of trust within the midwife-mother relationship. Int J Childbirth. 2017;7:40–52.
Mozaffar H, Cresswell KM, Williams R, Bates DW, Sheikh A. Exploring the roots of unintended safety threats associated with the introduction of hospital ePrescribing systems and candidate avoidance and/or mitigation strategies: a qualitative study. BMJ Qual Saf. 2017;26:722–33.
Castro A, Andrews G. Nursing lives in the blogosphere: a thematic analysis of anonymous online nursing narratives. J Adv Nurs. 2018;74:329–38.
Jensen AM, Pedersen BD, Olsen RB, Wilson RL, Hounsgaard L. "if only they could understand me!" acute hospital care experiences of patients with Alzheimer's disease. Dementia. 2018;19:2332–53.
Nash BH, Mitchell AW. Longitudinal study of changes in occupational therapy students' perspectives on frames of reference. Am J Occup Ther. 2017;71:7105230010p1–7.
Bright FAS, Kayes NM, McPherson KM, Worrall LE. Engaging people experiencing communication disability in stroke rehabilitation: a qualitative study. Int J Lang Commun Disord. 2018;53:981–94.
Superdock AK, Barfield RC, Brandon DH, Docherty SL. Exploring the vagueness of religion & spirituality in complex pediatric decision-making: a qualitative study. BMC Palliat. 2018;17:107.
Gordon L, Jindal-Snape D, Morrison J, Muldoon J, Needham G, Siebert S, et al. Multiple and multidimensional transitions from trainee to trained doctor: a qualitative longitudinal study in the UK. BMJ Open. 2017;7:e018583.
Cain CL, Frazer M, Kilaberia TR. Identity work within attempts to transform healthcare: invisible team processes. Hum Relat. 2019;72:370–96.
Klinga C, Hasson H, Andreen Sachs M, Hansson J. Understanding the dynamics of sustainable change: a 20-year case study of integrated health and social care. BMC Health Serv Res. 2018;18:400.
Balmer DF, Richards BF. Conducting qualitative research through time: how might theory be useful in longitudinal qualitative research? Adv Health Sci Educ Theory Pract. 2021;27:277–88.
Holland J. Qualitative longitudinal research: exploring ways of researching lives through time. ESRC National Centre for Research Methods Workshop; London: London South Bank University; 2007.
Nevedal AL, Ayalon L, Briller SH. A qualitative evidence synthesis review of longitudinal qualitative research in gerontology. Gerontologist. 2019;59:e791–801.
Download references
Acknowledgments
The authors wish to acknowledge Ellen Sejersted, librarian at the University of Agder, Kristiansand, Norway, who conducted the literature searches and Julia Andersson, research assistant at the Department of Nursing, Umeå University, Sweden, who supported the data management and took part in the initial screening phases of the project.
Open access funding provided by Umea University. This project was conducted within the authors’ positions and did not receive any specific funding.
Author information
Authors and affiliations.
Department of Nursing, Umeå University, Umeå, Sweden
Faculty of Health, Aarhus University, Aarhus, Denmark
Elisabeth O. C. Hall
Faculty of Health Sciences, University of Faroe Islands, Thorshavn, Faroe Islands, Denmark
Elisabeth O. C. Hall & Kristianna Lund Dam
Department of Health Care Sciences, Ersta Sköndal Bräcke University College, Stockholm, Sweden
Department of Health and Nursing Science, University of Agder, Kristiansand, Norway
Thomas Westergren & Liv Fegran
Department of Public Health, University of Stavanger, Stavanger, Norway
Thomas Westergren
Center for Clinical Research, North Denmark Regional Hospital, Hjørring, Denmark
Mona Kyndi Pedersen
Department of Clinical Medicine, Aalborg University, Aalborg, Denmark
Lovisenberg Diaconale Univeristy of College, Oslo, Norway
Hanne Aagaard
Department of Clinical Medicine-Randers Regional Hospital, Aarhus University, Aarhus, Denmark
Mette Spliid Ludvigsen
Faculty of Nursing and Health Sciences, Nord University, Bodø, Norway
You can also search for this author in PubMed Google Scholar
Contributions
ÅA conceived the study. ÅA, EH, TW, LF, MKP, HA, and MSL designed the study. ÅA, TW, and LF were involved in literature searches together with the librarian. ÅA and EH performed the screening of the articles. All authors (ÅA, EH, TW, LF, ÅK, MKP, KLD, HA, MSL) took part in the data charting. ÅA performed the data analysis and discussed the preliminary results with the rest of the team. ÅA wrote the 1st manuscript draft, and ÅK, MSL and EH edited. All authors (ÅA, EH, TW, LF, ÅK, MKP, KLD, HA, MSL) contributed to editing the 2nd draft. MSL and LF provided overall supervision. All authors read and approved the final manuscript.
Authors’ information
All authors represent the nursing discipline, but their research topics differ. ÅA and ÅK have previously worked together with QLR method development. ÅA, EH, TW, LF, MKP, HA, KLD and MSL work together in the Nordic research group PRANSIT, focusing on nursing topics connected to transition theory using a systematic review method, preferably meta synthesis. All authors have extensive experience with qualitative research but various experience with QLR.
Corresponding author
Correspondence to Åsa Audulv .
Ethics declarations
Ethics approval and consent to participate.
Not applicable.
Consent for publication
Competing interests.
The authors declare that they have no competing interests.
Additional information
Publisher’s note.
Springer Nature remains neutral with regard to jurisdictional claims in published maps and institutional affiliations.
Supplementary Information
Additional file 1..
PRISMA-ScR checklist.
Additional file 2.
Data base searches.
Additional file 3.
Guidelines for data charting
Additional file 4.
List of excluded articles
Additional file 5.
Table of included articles (author(s), year of publication, reference, country, aims and research questions, methodology, type of data material, length of data collection period, number of participants)
Additional file 6.
Rights and permissions.
Open Access This article is licensed under a Creative Commons Attribution 4.0 International License, which permits use, sharing, adaptation, distribution and reproduction in any medium or format, as long as you give appropriate credit to the original author(s) and the source, provide a link to the Creative Commons licence, and indicate if changes were made. The images or other third party material in this article are included in the article's Creative Commons licence, unless indicated otherwise in a credit line to the material. If material is not included in the article's Creative Commons licence and your intended use is not permitted by statutory regulation or exceeds the permitted use, you will need to obtain permission directly from the copyright holder. To view a copy of this licence, visit http://creativecommons.org/licenses/by/4.0/ . The Creative Commons Public Domain Dedication waiver ( http://creativecommons.org/publicdomain/zero/1.0/ ) applies to the data made available in this article, unless otherwise stated in a credit line to the data.
Reprints and permissions
About this article
Cite this article.
Audulv, Å., Hall, E.O.C., Kneck, Å. et al. Qualitative longitudinal research in health research: a method study. BMC Med Res Methodol 22 , 255 (2022). https://doi.org/10.1186/s12874-022-01732-4
Download citation
Received : 13 January 2022
Accepted : 22 September 2022
Published : 01 October 2022
DOI : https://doi.org/10.1186/s12874-022-01732-4
Share this article
Anyone you share the following link with will be able to read this content:
Sorry, a shareable link is not currently available for this article.
Provided by the Springer Nature SharedIt content-sharing initiative
- Qualitative longitudinal research
- Method development
- Repeated data collection
BMC Medical Research Methodology
ISSN: 1471-2288
- General enquiries: [email protected]
- Chapter 7. Longitudinal studies
Clinical follow up studies
- Chapter 1. What is epidemiology?
- Chapter 2. Quantifying disease in populations
- Chapter 3. Comparing disease rates
- Chapter 4. Measurement error and bias
- Chapter 5. Planning and conducting a survey
- Chapter 6. Ecological studies
- Chapter 8. Case-control and cross sectional studies
- Chapter 9. Experimental studies
- Chapter 10. Screening
- Chapter 11. Outbreaks of disease
- Chapter 12. Reading epidemiological reports
- Chapter 13. Further reading
Follow us on
Content links.
- Collections
- Health in South Asia
- Women’s, children’s & adolescents’ health
- News and views
- BMJ Opinion
- Rapid responses
- Editorial staff
- BMJ in the USA
- BMJ in South Asia
- Submit your paper
- BMA members
- Subscribers
- Advertisers and sponsors
Explore BMJ
- Our company
- BMJ Careers
- BMJ Learning
- BMJ Masterclasses
- BMJ Journals
- BMJ Student
- Academic edition of The BMJ
- BMJ Best Practice
- The BMJ Awards
- Email alerts
- Activate subscription
Information
Longitudinal Studies in HCI Research: A Review of CHI Publications From 1982–2019
- First Online: 12 August 2021
Cite this chapter
- Maria Kjærup 7 ,
- Mikael B. Skov 7 ,
- Peter Axel Nielsen 7 ,
- Jesper Kjeldskov 7 ,
- Jens Gerken 8 &
- Harald Reiterer 9
Part of the book series: Human–Computer Interaction Series ((HCIS))
760 Accesses
8 Citations
Longitudinal studies in HCI research have the potential to increase our understanding of how human–technology interactions evolve over time. Potentially, longitudinal studies eliminate learning or novelty effects by considering change through repeated measurements of interaction and use. However, there seems to exist no agreement of how longitudinal HCI study designs are characterized. We conducted an analysis of 106 HCI papers published at the CHI conference from 1982 to 2019 where longitudinal studies were explicitly reported. We analysed these papers using classical longitudinal study metrics, e.g. duration, metrics, methods, change or stability. We illustrate that longitudinal studies in HCI research are highly diverse in terms of duration lasting from few days to several years and different metrics are applied. It appears that the paper contribution type highly influences study design, while only a little more than half of the papers discuss or illustrate change/stability during their studies. We further underline considerations of durations versus saturation, identifying points of measurements and matching contribution types with research questions. Finally, we urge researchers to extend implications presented on perceiving duration as a singular attribute, as well as longitudinal systematic approaches to ‘in situ’ studies and ethnography in HCI.
This is a preview of subscription content, log in via an institution to check access.
Access this chapter
- Available as PDF
- Read on any device
- Instant download
- Own it forever
- Available as EPUB and PDF
- Compact, lightweight edition
- Dispatched in 3 to 5 business days
- Free shipping worldwide - see info
- Durable hardcover edition
Tax calculation will be finalised at checkout
Purchases are for personal use only
Institutional subscriptions
Bargas-Avila JA, Hornbæk K (2011) Old wine in new bottles or novel challenges. In: Proceedings of the 2011 annual conference on Human factors in computing systems—CHI’11. ACM Press: New York, USA, p 2689
Google Scholar
Baxter KK, Avrekh A, Evans B (2015) Using experience sampling methodology to collect deep data about your users. In: Proceedings of the 33rd annual ACM conference extended abstracts on human factors in computing systems—CHI EA ’15. ACM Press: New York, USA, pp 2489–2490
Bickmore TW, Consolvo S, Intille SS (2009) Engagement by design. In: Proceedings of the 27th international conference extended abstracts on human factors in computing systems—CHI EA ’09. ACM Press: New York, USA, p 4807
Courage C, Jain J, Rosenbaum S (2009) Best practices in longitudinal research. In: Proceedings of the 27th international conference extended abstracts on human factors in computing systems—CHI EA ’09. ACM Press: New York, USA, p 4791
Gaver W, Michael M, Kerridge T, Wilkie A, Boucher A, Ovalle L, Plummer-Fernandez M (2015) Energy babble: mixing environmentally-oriented internet content to engage community groups. In: Conference on human factors in computing systems—proceedings. pp 1115–1124
Gerken J (2011) Longitudinal research in human—computer interaction. Universität Konstanz
Jain J, Rosenbaum S, Courage C (2010) Best practices in longitudinal research. In: Proceedings of the 28th of the international conference extended abstracts on human factors in computing systems—CHI EA ’10. ACM Press: New York, USA, p 3167
Karapanos E, Jain J, Hassenzahl M (2012) Theories, methods and case studies of longitudinal HCI research. In: Proceedings of the 2012 ACM annual conference extended abstracts on human factors in computing systems extended abstracts—CHI EA ’12. ACM Press: New York, USA, p 2727
Karapanos E, Martens J, Hassenzahl M (2010) On the retrospective assessment of users’ experiences over time. In: Proceedings of the 28th of the international conference extended abstracts on human factors in computing systems—CHI EA ’10. ACM Press: New York, USA, p 4075
Karapanos E, Zimmerman J, Forlizzi J (2009) User experience over time: an initial framework. Chi2009 Proceedings of the 27Th annual CHI conference hum factors computer system vol 1–4. pp 729–738
Kjeldskov J, Skov MB, Stage J (2010) A longitudinal study of usability in health care: does time heal? Int J Med Inform 79:e135–e143. https://doi.org/10.1016/j.ijmedinf.2008.07.008
Article Google Scholar
Lazar J, Feng J, Hochheiser H (2017) Research methods in human-computer interaction, 2nd ed. Morgan Kaufmann
Menard S (2002) Longitudinal research, 2nd ed. SAGE Publications
Odom W, Wakkary R, Hol J, Naus B, Verburg P, Amram T, Chen AYS (2019) Investigating slowness as a frame to design longer-term experiences with personal data. In: Proceedings of the 2019 CHI conference on human factors in computing systems—CHI’19. ACM Press: New York, USA, pp 1–16
Odom WT, Sellen AJ, Banks R, Kirk DS, Regan T, Selby M, Forlizzi JL, Zimmerman J (2014) Designing for slowness, anticipation and re-visitation. In: Proceedings of the 32nd annual ACM conference on human factors in computing systems—CHI’14. ACM Press: New York, USA, pp 1961–1970
Pettigrew AM (1990) Longitudinal field research on change: theory and practice. Organ Sci 1:267–292. https://doi.org/10.1287/orsc.1.3.267
Pettigrew AM (1997) What is a processual analysis? Scand J Manag 13:337–348. https://doi.org/10.1016/S0956-5221(97)00020-1
Ployhart RE, Vandenberg RJ (2010) Longitudinal research: the theory, design, and analysis of change. J Manage 36:94–120. https://doi.org/10.1177/0149206309352110
Rogers Y (2011) Interaction design gone wild. Interactions 18:58
Rogers Y, Paul M (2017) Research in the wild. Morgan & Claypool, London
Book Google Scholar
Taris T (2000) A primer in longitudinal data analysis. SAGE Publications
Vaughan M, Courage C (2007) SIG: capturing longitudinal usability. In: CHI’07 extended abstracts on human factors in computing systems—CHI’07. ACM Press: New York, USA, p 2149
Vaughan M, Courage C, Rosenbaum S, Jain J, Hammontree M, Beale R, Welsh D (2008) Longitudinal usability data collection. In: Proceeding of the twenty-sixth annual CHI conference extended abstracts on human factors in computing systems—CHI’08. ACM Press: New York, USA, p 2261
Appendix References CHI 1982–2019
Alcaidinho J (2016) Canine behavior and working dog suitability from quantimetric data. In: Proceedings of the 2016 CHI conference extended abstracts on human factors in computing systems—CHI EA ’16. ACM Press: New York, USA, pp 193–197
Aragon CR, Williams A (2011) Collaborative creativity. In: Proceedings of the 2011 annual conference on Human factors in computing systems—CHI’11. ACM Press: New York, USA, p 1875
Archambault A, Grudin J (2012) A longitudinal study of facebook, linkedin, & twitter use. In: Proceedings of the 2012 ACM annual conference on human factors in computing systems—CHI’12. ACM Press: New York, USA, p 2741
Arguello J, Butler BS, Joyce L, Kraut R, Ling KS, Wang X (2006) Talk to me: foundations for successful individual-group interactions in online communities. In: Proceedings of the 2006 SIGCHI conference on human factors in computing systems—CHI’06. ACM Press: New York, USA, p 959
Beale R, Vaughan M, Courage C, Rosenbaum S, Jain J, Hammontree M, Welsh D (2008) Longitudinal usability data collection. In: Proceeding of the twenty-sixth annual CHI conference extended abstracts on human factors in computing systems—CHI’08. ACM Press: New York, USA, p 2261
Bickmore TW, Caruso L, Clough-Gorr K (2005) Acceptance and usability of a relational agent interface by urban older adults. In: CHI’05 extended abstracts on human factors in computing systems—CHI’05. ACM Press: New York, USA, p 1212
Bickmore TW, Picard RW (2004) Towards caring machines. In: Extended abstracts of the 2004 conference on Human factors and computing systems—CHI’04. ACM Press: New York, USA, p 1489
Boardman R, Sasse MA (2004) “Stuff goes into the computer and doesn’t come out”: a cross-tool study of peronal information management. In: Proceedings of the 2004 conference on human factors in computing systems—CHI’04. ACM Press: New York, USA, pp 583–590
Bruun A, Gull P, Hofmeister L, Stage J (2009) Let your users do the testing: a comparison of three remote asynchronous usability testing methods. In: Proceedings of the 27th international conference on human factors in computing systems—CHI 09. ACM Press: New York, USA, p 1619
Burke M, Kraut RE (2014) Growing closer on facebook: changes in tie strength through social network site use. In: Proceedings of the 32nd annual ACM conference on human factors in computing systems—CHI’14. ACM Press: New York, USA, pp 4187–4196
Burke M, Kraut R, Marlow C (2011) Social capital on facebook: differentiating uses and users. In: Proceedings of the 2011 annual conference on human factors in computing systems—CHI’11. ACM Press: New York, USA, p 571
Campbell RL (1990) Developmental scenario analysis of smalltalk programming. In: Proceedings of the 1990 SIGCHI conference on human factors in computing systems empowering people—CHI’90. ACM Press: New York, USA, pp 269–276
Castellucci SJ, MacKenzie IS (2008) Graffiti versus unistrokes: an empirical comparison. In: Proceeding of the twenty-sixth annual CHI conference on human factors in computing systems—CHI’08. ACM Press: New York, USA, p 305
Cataldo M, Ehrlich K (2012) The impact of communication structure on new product development outcomes. In: Proceedings of the 2012 ACM annual conference on human factors in computing systems—CHI’12. ACM Press: New York, USA, p 3081
Chang JS, Doucette AF, Yeboah G, Welsh T, Nitsche M, Mazalek A (2018) A tangible VR game designed for spatial penetrative thinking ability. Extended abstracts of the 2018 CHI conference on human factors in computing systems. ACM, New York, USA, pp 1–4
Chattopadhyay D, O’Hara K, Rintel S, Rädle R (2016) Office social: presentation interactivity for nearby devices. In: Proceedings of the 2016 CHI conference on human factors in computing systems. ACM: New York, USA, pp 2487–2491
Chu SL, Schlegel R, Quek F, Christy A, Chen K (2017) “I make, therefore i am”: The effects of curriculum-aligned making on children’s self-identity. In: Proceedings of the 2017 CHI conference on human factors in computing systems. ACM: New York, USA, pp 109–120
Clarkson E, Clawson J, Lyons K, Starner T (2005) An empirical study of typing rates on mini-QWERTY keyboards. In: CHI’05 extended abstracts on human factors in computing systems—CHI’05. ACM Press: New York, USA, p 1288
Clarkson E, Lyons K, Clawson J, Starner T (2007) Revisiting and validating a model of two-thumb text entry. In: Proceedings of the SIGCHI conference on human factors in computing systems—CHI’07. ACM Press: New York, USA, pp 163–166
Clawson J, Lyons K, Rudnick A, Iannucci RA, Starner T (2008) Automatic whiteout++: correcting mini-QWERTY typing errors using keypress timing. In: Proceeding of the twenty-sixth annual CHI conference on human factors in computing systems—CHI’08. ACM Press: New York, USA, p 573
Constantinides M (2015) Apps with habits: adaptive interfaces for news apps. In: Proceedings of the 33rd annual ACM conference extended abstracts on human factors in computing systems—CHI EA ’15. ACM Press: New York, USA, pp 191–194
Cook GJ, Grabski SV (1992) An empirical examination of software-mediated information exchange and communication richness. In: Posters and short talks of the 1992 SIGCHI conference on human factors in computing systems—CHI’92. ACM Press: New York, USA, p 46
Courage C, Jain J, Rosenbaum S (2010) Best practices in longitudinal research. In: Proceedings of the 28th of the international conference extended abstracts on human factors in computing systems—CHI EA ’10. ACM Press: New York, USA, p 3167
Dantec CA Le, Farrell RG, Christensen JE, Bailey M, Ellis JB, Kellogg WA, Edwards WK (2011) Publics in practice: ubiquitous computing at a shelter for homeless mothers. In: Proceedings of the 2011 annual conference on human factors in computing systems—CHI’11. ACM Press: New York, USA, p 1687
Dantec C Le (2012) Participation and publics: supporting community engagement. In: Proceedings of the 2012 ACM annual conference on human factors in computing systems—CHI’12. ACM Press: New York, USA, p 1351
Day J, Foley J (2006) Evaluating web lectures: a case study from HCI. In: CHI’06 extended abstracts on human factors in computing systems—CHI EA ’06. ACM Press: New York, USA, p 195
Ducheneaut N, Yee N, Nickell E, Moore RJ (2006) “Alone together?”: exploring the social dynamics of massively multiplayer online games. In: Proceedings of the 2006 SIGCHI conference on human factors in computing systems—CHI’06. ACM Press: New York, USA, p 407
Dudley C, Jones SL (2018) Fitbit for the mind?: An exploratory study of “cognitive personal informatics.” Extended abstracts of the 2018 CHI conference on human factors in computing systems. ACM, New York, USA, pp 1–6
Erete S, Burrell JO (2017) Empowered participation: how citizens use technology in local governance. In: Proceedings of the 2017 CHI conference on human factors in computing systems. ACM: New York, USA, pp 2307–2319
Erickson T (1996) The design and long-term use of a personal electronic notebook: a reflective analysis. In: Proceedings of the 1996 SIGCHI conference on human factors in computing systems common ground—CHI’96. ACM Press: New York, USA, pp 11–18
Fan X, Luo W, Wang J (2017) Mastery learning of second language through asynchronous modeling of native speakers in a collaborative mobile game. In: Proceedings of the 2017 CHI conference on human factors in computing systems. ACM: New York, USA, pp 4887–4898
Fiorani M, Mariani M, Minin L, Montanari R (2008) Monitoring time-headway in car-following task. In: Proceeding of the twenty-sixth annual CHI conference extended abstracts on human factors in computing systems—CHI’08. ACM Press: New York, USA, p 2143
Fiore AT, Cheshire C, Shaw Taylor L, Mendelsohn GA (2014) Incentives to participate in online research: an experimental examination of “surprise” incentives. In: Proceedings of the 32nd annual ACM conference on human factors in computing systems—CHI’14. ACM Press: New York, USA, pp 3433–3442
Fitchett S, Cockburn A, Gutwin C (2014) Finder highlights: field evaluation and design of an augmented file browser. In: Proceedings of the 32nd annual ACM conference on human factors in computing systems—CHI’14. ACM Press: New York, USA, pp 3685–3694
Flounders C (2001) “Are you there Margaret? It’s me, Margaret”: speech recognition as a mirror. In: CHI’01 extended abstracts on Human factors in computing systems—CHI’01. ACM Press: New York, USA, p 459
Friess E (2008) Defending design decisions with usability evidence: a case study. In: Proceeding of the twenty-sixth annual CHI conference extended abstracts on human factors in computing systems—CHI’08. ACM Press: New York, USA, p 2009
Garzonis S, Jones S, Jay T, O’Neill E (2009) Auditory icon and earcon mobile service notifications: intuitiveness, learnability, memorability and preference. In: Proceedings of the 27th international conference on human factors in computing systems—CHI 09. ACM Press: New York, USA, p 1513
Gerken J, Bieg H-J, Dierdorf S, Reiterer H (2009) Enhancing input device evaluation: longitudinal approaches. In: Proceedings of the 27th international conference extended abstracts on human factors in computing systems—CHI EA ’09. ACM Press: New York, USA, p 4351
Gerken J, Jetter H, Reiterer H (2010) Using concept maps to evaluate the usability of APIs. In: Proceedings of the 28th of the international conference extended abstracts on human factors in computing systems—CHI EA ’10. ACM Press: New York, USA, p 3937
Gerken J, Jetter H, Zöllner M, Mader M, Reiterer H (2011) The concept maps method as a tool to evaluate the usability of APIs. In: Proceedings of the 2011 annual conference on human factors in computing systems—CHI’11. ACM Press: New York, USA, p 3373
Ghosh S, Joshi A, Joshi M, Emmadi N, Dalvi G, Ahire S, Rangale S (2017) Shift+Tap or Tap+LongPress?: The upper bound of typing speed on InScript. In: Proceedings of the 2017 CHI conference on human factors in computing systems. ACM: New York, USA, pp 2059–2063
Gray CM (2014) Evolution of design competence in UX practice. In: Proceedings of the 32nd annual ACM conference on human factors in computing systems—CHI’14. ACM Press: New York, USA, pp 1645–1654
Gupta A, Balakrishnan R (2016) DualKey: miniature screen text entry via finger identification. In: Proceedings of the 2016 CHI conference on human factors in computing systems. ACM: New York, USA, pp 59–70
Harada S, Takagi H, Asakawa C (2011) On the audio representation of radial direction. In: Proceedings of the 2011 annual conference on human factors in computing systems—CHI’11. ACM Press: New York, USA, p 2779
Harada S, Wobbrock JO, Malkin J, Bilmes JA, Landay JA (2009) Longitudinal study of people learning to use continuous voice-based cursor control. In: Proceedings of the 27th international conference on human factors in computing systems—CHI 09. ACM Press: New York, USA, p 347
Harrison J, Chamberlain A, McPherson AP (2019) Accessible instruments in the wild: engaging with a community of learning-disabled musicians. Extended abstracts of the 2019 CHI conference on human factors in computing systems. ACM, New York USA, pp 1–6
Hass C, Rosenzweig E (2017) Rivet counting and ocean crossing: case examples illuminating the fracticality of the theory-practice cycle and the importance of horizon expansion. In: Proceedings of the 2017 CHI conference extended abstracts on human factors in computing systems. ACM: New York, USA, pp 1012–1017
Hoggan E, Brewster SA (2010) Crosstrainer: testing the use of multimodal interfaces in situ. In: Proceedings of the 28th international conference on human factors in computing systems—CHI’10. ACM Press: New York, USA, p 333
Houben S, Weichel C (2013) Overcoming interaction blindness through curiosity objects. In: CHI’13 extended abstracts on human factors in computing systems on—CHI EA ’13. ACM Press: New York, USA, p 1539
Howard S, Kjeldskov J, Skov MB, Garnæs K, Grünberger O (2006) Negotiating presence-in-absence: contact, content and context. In: Proceedings of the 2006 SIGCHI conference on human factors in computing systems—CHI’06. ACM Press: New York, USA, p 909
Hsu C-Y, Hristov R, Lee G-H, Zhao M, Katabi D (2019) Enabling identification and behavioral sensing in homes using radio reflections. In: Proceedings of the 2019 CHI conference on human factors in computing systems—CHI’19. ACM Press: New York, USA, pp 1–13
Hutto CJ, Yardi S, Gilbert E (2013) A longitudinal study of follow predictors on twitter. In: Proceedings of the 2013 CHI conference on human factors in computing systems. ACM: New York, USA, pp 821–830
Irons DM (1982) Cognitive correlates of programming tasks in novice programmers. In: Proceedings of the 1982 conference on human factors in computing systems—CHI’82. ACM Press: New York, USA, pp 219–222
Jain J, Boyce S (2012) Case study: longitudinal comparative analysis for analyzing user behavior. In: Proceedings of the 2012 ACM annual conference extended abstracts on human factors in computing systems extended abstracts—CHI EA ’12. ACM Press: New York, USA, p 793
Jain J, Ghosh R, Dekhil M (2008) Multimodal capture of consumer intent in retail. In: Proceeding of the twenty-sixth annual CHI conference extended abstracts on human factors in computing systems—CHI’08. ACM Press: New York, USA, p 3207
Jain M, Balakrishnan R (2012) User learning and performance with bezel menus. In: Proceedings of the 2012 ACM annual conference on human factors in computing systems—CHI’12. ACM Press: New York, USA, p 2221
Jensen C, Lonsdale H, Wynn E, Cao J, Slater M, Dietterich TG (2010) The life and times of files and information: a study of desktop provenance. In: Proceedings of the 28th international conference on human factors in computing systems—CHI’10. ACM Press: New York, USA, p 767
Jones W, Bellotti V, Capra R, Dinneen JD, Mark G, Marshall C, Moffatt K, Teevan J, Van Kleek M (2016) For richer, for poorer, in sickness or in health...: the long-term management of personal information. In: Proceedings of the 2016 CHI conference extended abstracts on human factors in computing systems—CHI EA ’16. ACM Press: New York, USA, pp 3508–3515
Ju WG, Lee BA, Klemmer SR (2007) Range: exploring proxemics in collaborative whiteboard interaction. In: CHI’07 extended abstracts on human factors in computing systems—CHI’07. ACM Press: New York, USA, p 2483
Kantner L, Goold SD, Danis M, Nowak M, Monroe-Gatrell L (2006) Web tool for ealth insurance design by small groups: usability study. In: CHI’06 extended abstracts on human factors in computing systems—CHI EA ’06. ACM Press: New York, USA, p 141
Karapanos E, Hassenzahl M, Martens J-B (2008) User experience over time. In: Proceeding of the twenty-sixth annual CHI conference extended abstracts on human factors in computing systems—CHI’08. ACM Press: New York, USA, p 3561
Khan MT, Hyun M, Kanich C, Ur B (2018) Forgotten but not gone: identifying the need for longitudinal data management in cloud storage. In: Proceedings of the 2018 CHI conference on human factors in computing systems—CHI’18. ACM Press: New York, USA, pp 1–12
Kim S, Paulos E, Mankoff J (2013) inAir: a longitudinal study of indoor air quality measurements and visualizations. In: Proceedings of the 2013 CHI conference on human factors in computing systems. ACM: New York, USA, pp 2745–2754
Kirman B, Linehan C, Lawson S (2012) Get lost: facilitating serendipitous exploration in location-sharing services. In: Proceedings of the 2012 ACM annual conference extended abstracts on human factors in computing systems extended abstracts—CHI EA ’12. ACM Press: New York, USA, p 2303
Kleek MG Van, Bernstein M, Panovich K, Vargas GG, Karger DR, Schraefel M (2009) Note to self: examining personal information keeping in a lightweight note-taking tool. In: Proceedings of the 27th international conference on human factors in computing systems—CHI 09. ACM Press: New York, USA, p 1477
Kleek MG Van, Styke W, Schraefel M c., Karger D (2011) Finders/keepers: a longitudinal study of people managing information scraps in a micro-note tool. In: Proceedings of the 2011 annual conference on human factors in computing systems—CHI’11. ACM Press: New York, USA, p 2907
Költringer T, Van MN, Grechenig T (2007) Game controller text entry with alphabetic and multi-tap selection keyboards. In: CHI’07 extended abstracts on human factors in computing systems—CHI’07. ACM Press: New York, USA, p 2513
Kristensson PO, Denby LC (2009) Text entry performance of state of the art unconstrained handwriting recognition: a longitudinal user study. In: Proceedings of the 27th international conference on human factors in computing systems—CHI 09. ACM Press: New York, USA, p 567
Larsen SB, Bardram JE (2008) Competence articulation: alignment of competences and responsibilities in synchronous telemedical collaboration. In: Proceeding of the twenty-sixth annual CHI conference on human factors in computing systems—CHI’08. ACM Press: New York, USA, p 553
Latulipe C, Carroll EA, Lottridge D (2011) Evaluating longitudinal projects combining technology with temporal arts. In: Proceedings of the 2011 annual conference on human factors in computing systems—CHI’11. ACM Press: New York, USA, p 1835
Lee K, Kim S, Myaeng S-H (2013) Measuring touch bias of one thumb posture on direct touch-based mobile devices. In: CHI’13 extended abstracts on human factors in computing systems on—CHI EA ’13. ACM Press: New York, USA, p 241
Lee MK, Kiesler S, Forlizzi J, Rybski P (2012) Ripple effects of an embedded social agent: a field study of a social robot in the workplace. In: Proceedings of the 2012 ACM annual conference on human factors in computing systems—CHI’12. ACM Press: New York, USA, p 695
Lee U, Kim J, Yi E, Sung J, Gerla M (2013) Analyzing crowd workers in mobile pay-for-answer q&a. In: Proceedings of the 2013 CHI conference on human factors in computing systems. ACM: New York, USA, pp 533–542
Lyons K, Starner T, Plaisted D, Fusia J, Lyons A, Drew A, Looney EW (2004) Twiddler typing: one-hand chording text entry for mobile phones. In: Proceedings of the 2004 conference on human factors in computing systems—CHI’04. ACM Press: New York, USA, pp 671–678
MacKenzie IS, Zhang SX (1999) The design and evaluation of a high-performance soft keyboard. In: Proceedings of the 1999 SIGCHI conference on human factors in computing systems the CHI is the limit—CHI’99. ACM Press: New York, USA, pp 25–31
Macvean A, Robertson J (2013) Understanding exergame users’ physical activity, motivation and behavior over time. In: Proceedings of the 2013 CHI conference on human factors in computing systems. ACM: New York, USA, pp 1251–1260
Majaranta P, Ahola U, Špakov O (2009) Fast gaze typing with an adjustable dwell time. In: Proceedings of the 27th international conference on human factors in computing systems—CHI 09. ACM Press: New York, USA, p 357
Maldonado H, Lee B, Klemmer S (2006) Technology for design education: a case study. In: CHI’06 extended abstracts on human factors in computing systems—CHI EA ’06. ACM Press: New York, USA, p 1067
Mann A-M, Hinrichs U, Read JC, Quigley A (2016) Facilitator, functionary, friend or foe?: Studying the role of iPads within learning activities across a school year. In: Proceedings of the 2016 CHI conference on human factors in computing systems—CHI’16. pp 1833–1845
Mariakakis A, Parsi S, Patel SN, Wobbrock JO (2018) Drunk user interfaces: determining blood alcohol level through everyday smartphone tasks. Proc 2018 CHI conference human factors computing system—CHI’18, 1–13 April 2018. https://doi.org/10.1145/3173574.3173808
Marquardt N, Greenberg S (2015) Sketching user experiences: the hands-on course. In: Proceedings of the 33rd annual ACM conference extended abstracts on human factors in computing systems—CHI EA ’15. ACM Press: New York, USA, pp 2479–2480
Masliah MR, Milgram P (2000) Measuring the allocation of control in a 6 degree-of-freedom docking experiment. In: Proceedings of the 2000 SIGCHI conference on human factors in computing systems—CHI’00. ACM Press: New York, USA, pp 25–32
Mattingly SM, Gregg JM, Audia P, Bayraktaroglu AE, Campbell AT, Chawla N V., Das Swain V, De Choudhury M, D’Mello SK, Dey AK, Gao G, Jagannath K, Jiang K, Lin S, Liu Q, Mark G, Martinez GJ, Masaba K, Mirjafari S, Moskal E, Mulukutla R, Nies K, Reddy MD, Robles-Granda P, Saha K, Sirigiri A, Striegel A (2019) The tesserae project: large-scale. longitudinal, in situ, multimodal sensing of information workers. In: Extended abstracts of the 2019 CHI conference on human factors in computing systems. ACM: New York, USA, pp 1–8
McLachlan P, Munzner T, Koutsofios E, North S (2008) LiveRAC: interactive visual exploration of system management time-series data. In: Proceeding of the twenty-sixth annual CHI conference on human factors in computing systems—CHI’08. ACM Press: New York, USA, p 1483
Meyer J, Wasmann M, Heuten W, El Ali A, Boll SCJ (2017) Identification and classification of usage patterns in long-term activity tracking. In: Proceedings of the 2017 CHI conference on human factors in computing systems. ACM: New York, USA, pp 667–678
Millen DR, Yang M, Warner M (2013) Best practices for enterprise social software adoption. In: CHI’13 extended abstracts on human factors in computing systems on—CHI EA ’13. ACM Press: New York, USA, p 2349
Molapo M, Densmore M, DeRenzi B (2017) Video consumption patterns for first time smartphone users: community health workers in Lesotho. In: Proceedings of the 2017 CHI conference on human factors in computing systems. ACM: New York, USA, pp 6159–6170
Mott ME, Williams S, Wobbrock JO, Morris MR (2017) Improving dwell-based gaze typing with dynamic, cascading dwell times. In: Proceedings of the 2017 CHI conference on human factors in computing systems. ACM: New York, USA, pp 2558–2570
Ni T, Bowman D, North C (2011) AirStroke: bringing unistroke text entry to freehand gesture interfaces. In: Proceedings of the 2011 annual conference on human factors in computing systems—CHI’11. ACM Press: New York, USA, p 2473
Niemantsverdriet K, van de Werff T, van Essen H, Eggen B (2018) Share and share alike? Social information and interaction style in coordination of shared use. In: Proceedings of the 2018 CHI conference on human factors in computing systems—CHI’18. ACM Press: New York, USA, pp 1–14
Nilsen E, Jong H, Olson JS, Biolsi K, Rueter H, Mutter S (1993) The growth of software skill: a longitudinal look at learning & performance. In: Proceedings of the 1993 SIGCHI conference on human factors in computing systems—CHI’93. ACM Press: New York, USA, pp 149–156
Oviatt S, Lunsford R, Coulston R (2005) Individual differences in multimodal integration patterns: what are they and why do they exist? In: Proceedings of the 2005 SIGCHI conference on human factors in computing systems—CHI’05. ACM Press New York, USA, p 241
Parkes AJ, Raffle HS, Ishii H (2008) Topobo in the wild: longitudinal evaluations of educators appropriating a tangible interface. In: Proceeding of the twenty-sixth annual CHI conference on human factors in computing systems—CHI’08. ACM Press: New York, USA, p 1129
Pasquetto I V, Sands AE, Darch PT, Borgman CL (2016) Open data in scientific settings: from policy to practice. In: Proceedings of the 2016 CHI conference on human factors in computing systems. ACM: New York, USA, pp 1585–1596
Pfeifer LM, Bickmore T (2011) Is the media equation a flash in the pan?: the durability and longevity of social responses to computers. In: Proceedings of the 2011 annual conference on human factors in computing systems—CHI’11. ACM Press: New York, USA, p 777
Rector K (2014) The development of novel eyes-free exercise technologies using participatory design. In: Proceedings of the extended abstracts of the 32nd annual ACM conference on human factors in computing systems—CHI EA ’14. ACM Press: New York, USA, pp 327–330
Ren J, Schulman D, Jack B, Bickmore TW (2014) Supporting longitudinal change in many health behaviors. In: Proceedings of the extended abstracts of the 32nd annual ACM conference on human factors in computing systems—CHI EA ’14. ACM Press: New York, USA, pp 1657–1662
Richter H (2002) Understanding meeting capture and access. In: CHI’02 extended abstracts on human factors in computing systems—CHI’02. ACM Press: New York, USA, p 558
Robinson S, Rajput N, Jones M, Jain A, Sahay S, Nanavati A (2011) TapBack: towards richer mobile interfaces in impoverished contexts. In: Proceedings of the 2011 annual conference on human factors in computing systems—CHI’11. ACM Press: New York, USA, p 2733
Saha K, Bayraktaroglu AE, Campbell AT, Chawla NV, De Choudhury M, D’Mello SK, Dey AK, Gao G, Gregg JM, Jagannath K, Mark G, Martinez GJ, Mattingly SM, Moskal E, Sirigiri A, Striegel A, Yoo DW (2019) Social media as a passive sensor in longitudinal studies of human behavior and wellbeing. Extended abstracts of the 2019 chi conference on human factors in computing systems. ACM, New York, USA, pp 1–8
Sato M, Puri RS, Olwal A, Ushigome Y, Franciszkiewicz L, Chandra D, Poupyrev I, Raskar R (2017) Zensei: embedded, multi-electrode bioimpedance sensing for implicit, ubiquitous user recognition. In: Proceedings of the 2017 CHI conference on human factors in computing systems. ACM: New York, USA, pp 3972–3985
Schwartz T, Denef S, Stevens G, Ramirez L, Wulf V (2013) Cultivating energy literacy: results from a longitudinal living lab study of a home energy management system. In: Proceedings of the 2013 CHI conference on human factors in computing systems. ACM: New York, USA, pp 1193–1202
Seay AF, Jerome WJ, Lee KS, Kraut RE (2004) Project massive: a study of online gamin communities. In: Extended abstracts of the 2004 conference on human factors and computing systems—CHI’04. ACM Press: New York, USA, p 1421
Seay AF, Kraut RE (2007) Project massive: self-regulation and problematic use of online gaming. In: Proceedings of the SIGCHI conference on human factors in computing systems—CHI’07. ACM Press: New York, USA, pp 829–838
Settles B, Dow S (2013) Let’s get together: the formation and success of online creative collaborations. In: Proceedings of the 2013 CHI conference on human factors in computing systems. ACM: New York, USA, pp 2009–2018
Shami NS, Nichols J, Chen J (2014) Social media participation and performance at work: a longitudinal study. In: Proceedings of the 32nd annual ACM conference on human factors in computing systems—CHI’14. ACM Press: New York, USA, pp 115–118
Sillence E, Briggs P, Harris P, Fishwick L (2006) Changes in online health usage over the last 5 years. In: CHI’06 extended abstracts on human factors in computing systems—CHI EA ’06. ACM Press: New York, USA, p 1331
Son J, Ahn S, Kim S, Lee G (2019) Improving two-thumb touchpad typing in virtual reality. Extended abstracts of the 2019 CHI conference on human factors in computing systems. ACM, New York, USA, pp 1–6
Sporka AJ, Felzer T, Kurniawan SH, Poláček O, Haiduk P, MacKenzie IS (2011) CHANTI: predictive text entry using non-verbal vocal input. In: Proceedings of the 2011 annual conference on Human factors in computing systems—CHI’11. ACM Press: New York, USA, p 2463
Sporka AJ, Kurniawan SH, Mahmud M, Slavik P (2007) Longitudinal study of continuous non-speech operated mouse pointer. In: CHI’07 extended abstracts on human factors in computing systems—CHI’07. ACM Press: New York, USA, p 2669
Tak S, Cockburn A (2010) Improved window switching interfaces. In: Proceedings of the 28th of the international conference extended abstracts on human factors in computing systems—CHI EA ’10. ACM Press: New York, USA, p 2915
Tan A, Kondoz AM (2008) Barriers to virtual collaboration. In: Proceeding of the twenty-sixth annual CHI conference extended abstracts on human factors in computing systems—CHI’08. ACM Press, New York, USA, p 2045
Taylor N, Cheverst K, Wright P, Olivier P (2013) Leaving the wild: lessons from community technology handovers. In: Proceedings of the 2013 CHI conference on human factors in computing systems. ACM: New York, USA, pp 1549–1558
Teevan J, Dumais ST, Liebling DJ (2010) A longitudinal study of how highlighting web content change affects people’s web interactions. In: Proceedings of the 28th international conference on human factors in computing systems—CHI’10. ACM Press: New York, USA, p 1353
Tossell C, Kortum P, Rahmati A, Shepard C, Zhong L (2012) Characterizing web use on smartphones. In: Proceedings of the 2012 ACM annual conference on human factors in computing systems—CHI’12. ACM Press: New York, USA, p 2769
Tullis TS, Tedesco DP, McCaffrey KE (2011) Can users remember their pictorial passwords six years later. In: Proceedings of the 2011 annual conference extended abstracts on human factors in computing systems—CHI EA ’11. ACM Press: New York, USA, p 1789
Vance A, Kirwan B, Bjornn D, Jenkins J, Anderson BB (2017) What do we really know about how habituation to warnings occurs over time?: A longitudinal fMRI study of habituation and polymorphic warnings. In: Proceedings of the 2017 CHI conference on human factors in computing systems. ACM: New York, USA, pp 2215–2227
Vaughn LJ, Bortnick MJ, Carey J, Orgovan VR, Munko J (2018) Measuring response rate and increasing satisfaction in innovative environments: the impact of feedback. Extended abstracts of the 2018 CHI conference on human factors in computing systems. ACM, New York, USA, pp 1–7
Voida S, Mynatt ED (2009) It feels better than filing: everyday work experiences in an activity-based computing system. In: Proceedings of the 27th international conference on human factors in computing systems—CHI 09. ACM Press: New York, USA, p 259
Wang EJ, Zhu J, Jain M, Lee T-J, Saba E, Nachman L, Patel SN (2018) Seismo: blood pressure monitoring using built-in smartphone accelerometer and camera. In: Proceedings of the 2018 CHI conference on human factors in computing systems—CHI’18. ACM Press: New York, USA, pp 1–9
Wang Y, Kraut R (2012) Twitter and the development of an audience: those who stay on topic thrive! In: Proceedings of the 2012 ACM annual conference on human factors in computing systems—CHI’12. ACM Press: New York, USA, p 1515
White RW, Richardson M, Liu Y (2011) Effects of community size and contact rate in synchronous social q&a. In: Proceedings of the 2011 annual conference on human factors in computing systems—CHI’11. ACM Press: New York, USA, p 2837
Williamson JR, Williamson J, Kostakos V, Hamilton K, Green J (2016) Mobile phone usage cycles: a torus topology for spherical visualisation. In: Proceedings of the 2016 CHI conference extended abstracts on human factors in computing systems—CHI EA ’16. ACM Press: New York, USA, pp 2751–2757
Wilson G, Carter T, Subramanian S, Brewster SA (2014) Perception of ultrasonic haptic feedback on the hand: localisation and apparent motion. In: Proceedings of the 32nd annual ACM conference on human factors in computing systems—CHI’14. ACM Press: New York, USA, pp 1133–1142
Wobbrock J, Myers B, Rothrock B (2006) Few-key text entry revisited: mnemonic gestures on four keys. In: Proceedings of the 2006 SIGCHI conference on human factors in computing systems—CHI’06. ACM Press: New York, USA, p 489
Xu Q, Casiez G (2010) Push-and-pull switching: window switching based on window overlapping. In: Proceedings of the 28th international conference on human factors in computing systems—CHI’10. ACM Press: New York, USA, p 1335
Yee N, Ducheneaut N, Yao M, Nelson L (2011) Do men heal more when in drag?: conflicting identity cues between user and avatar. In: Proceedings of the 2011 annual conference on human factors in computing systems—CHI’11. ACM Press: New York, USA, p 773
Yeo H, Phang X, Castellucci SJ, Kristensson PO, Quigley A (2017) Investigating tilt-based gesture keyboard entry for single-handed text entry on large devices. In: Proceedings of the 2017 CHI conference on human factors in computing systems. ACM: New York, USA, pp 4194–4202
Yu B (2016) Adaptive biofeedback for mind-body practices. In: Proceedings of the 2016 CHI conference extended abstracts on human factors in computing systems—CHI EA ’16. ACM Press: New York, USA, pp 260–264
Yürüten O, Zhang J, Pu PHZ (2014) Predictors of life satisfaction based on daily activities from mobile sensor data. In: Proceedings of the 32nd annual ACM conference on human factors in computing systems—CHI’14. ACM Press: New York, USA, pp 497–500
Zhang LH, Bucci P, Cang XL, MacLean K (2018) Infusing cuddlebits with emotion: build your own and tell us about it. Extended abstracts of the 2018 CHI conference on human factors in computing systems. ACM, New York, USA, pp 1–4
Download references
Author information
Authors and affiliations.
Aalborg University, Aalborg, Denmark
Maria Kjærup, Mikael B. Skov, Peter Axel Nielsen & Jesper Kjeldskov
Westphalian University of Applied Sciences, Gelsenkirchen, Germany
Jens Gerken
University of Konstanz, Konstanz, Germany
Harald Reiterer
You can also search for this author in PubMed Google Scholar
Corresponding author
Correspondence to Maria Kjærup .
Editor information
Editors and affiliations.
Cyprus University of Technology, Limassol, Cyprus
Evangelos Karapanos
Westphalian University of Applied Scienc, Gelsenkirchen, Nordrhein-Westfalen, Germany
Aalborg University, Aalborg East, Denmark
Jesper Kjeldskov
Mikael B. Skov
Rights and permissions
Reprints and permissions
Copyright information
© 2021 Springer Nature Switzerland AG
About this chapter
Kjærup, M., Skov, M.B., Nielsen, P.A., Kjeldskov, J., Gerken, J., Reiterer, H. (2021). Longitudinal Studies in HCI Research: A Review of CHI Publications From 1982–2019. In: Karapanos, E., Gerken, J., Kjeldskov, J., Skov, M.B. (eds) Advances in Longitudinal HCI Research. Human–Computer Interaction Series. Springer, Cham. https://doi.org/10.1007/978-3-030-67322-2_2
Download citation
DOI : https://doi.org/10.1007/978-3-030-67322-2_2
Published : 12 August 2021
Publisher Name : Springer, Cham
Print ISBN : 978-3-030-67321-5
Online ISBN : 978-3-030-67322-2
eBook Packages : Computer Science Computer Science (R0)
Share this chapter
Anyone you share the following link with will be able to read this content:
Sorry, a shareable link is not currently available for this article.
Provided by the Springer Nature SharedIt content-sharing initiative
- Publish with us
Policies and ethics
- Find a journal
- Track your research
Thank you for visiting nature.com. You are using a browser version with limited support for CSS. To obtain the best experience, we recommend you use a more up to date browser (or turn off compatibility mode in Internet Explorer). In the meantime, to ensure continued support, we are displaying the site without styles and JavaScript.
- View all journals
- My Account Login
- Explore content
- About the journal
- Publish with us
- Sign up for alerts
- Data Descriptor
- Open access
- Published: 28 May 2024
A 2-year longitudinal study examining the change in psychosocial factors under the COVID-19 pandemic in Japan
- Nagisa Sugaya 1 ,
- Tetsuya Yamamoto ORCID: orcid.org/0000-0003-4241-532X 2 &
- Chigusa Uchiumi 2
Scientific Data volume 11 , Article number: 544 ( 2024 ) Cite this article
304 Accesses
1 Altmetric
Metrics details
- Human behaviour
- Psychology and behaviour
To examine changes in individuals’ psychosocial variables (e.g., psychological distress, social isolation, and alcohol use) during the prolonged COVID-19 pandemic, a two-year longitudinal survey was conducted at approximately one-year intervals between May 2020 and May 2022, after the first COVID-19-related state of emergency was announced in Japan. The online survey was conducted on May 11-12, 2020 (Phase 1), June 14–20, 2021 (Phase 2), and May 13–30, 2022 (Phase 3). The survey in Phase 1 was conducted during the first emergency declaration period, the survey in Phase 2 was conducted during the third emergency declaration period, and the survey in Phase 3 was conducted at a time when there was no state of emergency but many COVID-19 positive cases. Notably, 3,892 participants responded to all three surveys. In addition to psychosocial inventories often used worldwide, survey items included lifestyle and stress management indicators related to COVID-19 and various sociodemographic items including occupation (e.g., healthcare workers) or income, history of medical treatment for mental problems, severe physical illnesses, and COVID-19.
Similar content being viewed by others
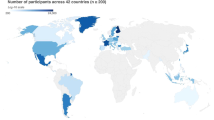
COVIDiSTRESS diverse dataset on psychological and behavioural outcomes one year into the COVID-19 pandemic
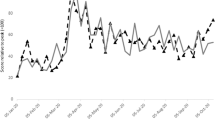
COVID-19 restrictions and age-specific mental health—U.S. probability-based panel evidence
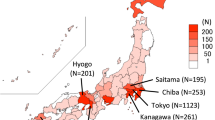
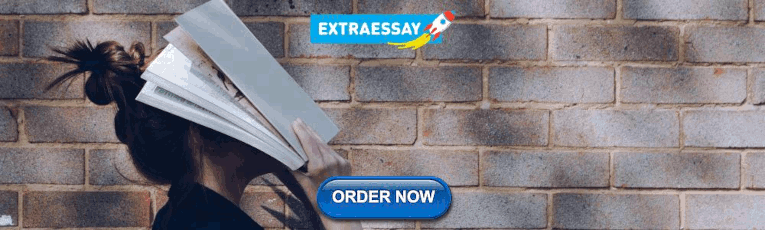
A real-time survey on the psychological impact of mild lockdown for COVID-19 in the Japanese population
Background & summary.
Coronavirus disease 2019 (COVID-19) broke out in December 2019 and spread rapidly worldwide. Although the World Health Organization (WHO) announced the end of the emergency in May 2023, as of August 2023, there were still cases of infection in many parts of the world 1 . To deter its spread, many countries have repeatedly implemented lockdowns, restricting people’s movements and temporarily closing services. However, while these lockdowns have been effective in preventing the spread of the disease, they have also caused significant economic hardships and emotional distress 2 , 3 .
In Japan, four states of emergency were declared between 2020 and 2021 to combat the COVID-19 outbreak. While many countries were in lockdown with penalties for violations, Japan’s COVID-19 measures were characterized by the government’s request to refrain from leaving the house, except in an emergency, temporary closure of some businesses, and no penalties for violations. As the declaration of the states of emergency in Japan was a “request” by the government, it did not prohibit people from going out or meeting with others. Despite this, even the mild lockdown 4 in Japan affected people’s lives in various ways, including lifestyle changes due to teleworking and online classes, as well as economic hardship due to reduced income and unemployment. We have previously reported how Japanese citizens experienced severe psychological stress, depression, anxiety, loneliness, and social isolation during the state of emergency 4 , 5 , 6 , 7 , 8 . In addition, several previous studies have suggested that not all psychosocial variables may have changed uniformly during the pandemic. For example, no improvements were observed in severe social isolation or loneliness among the Japanese population 7 between the two survey phases, although psychological distress improved significantly and depression decreased slightly.
Therefore, long-term studies are needed to identify the actual changes in psychosocial conditions during prolonged pandemics. To examine changes in psychosocial variables, we conducted a longitudinal survey at approximately one-year intervals during the two years after the first state of emergency for COVID-19 in individuals who experienced repeated states of emergency.
Participants and data collection
The online survey was conducted on May 11-12, 2020 (Phase 1), June 14–20, 2021 (Phase 2), and May 13–30, 2022 (Phase 3). Phase 1 was undertaken during the first state of emergency, while Phase 2 was carried out during the third state of emergency. The online survey in Phase 1 was conducted among residents in seven prefectures in which the state of emergency was declared relatively early (Tokyo, Kanagawa, Osaka, Saitama, Chiba, Hyogo, and Fukuoka prefectures) to accurately assess its impact. The inclusion criteria were as follows: (a) residents of the seven prefectures, and (b) aged 18 years or older. The exclusion criteria were as follows: (a) under 18 years of age, (b) high school students, and (c) living outside the seven prefectures. Phase 1 had 11,333 participants, with the number of participants from each prefecture based on the ratio of the number of people residing in each prefecture (Tokyo: n = 2783, 24.6%; Kanagawa: n = 1863, 16.4%; Osaka: n = 1794, 15.8%; Saitama: n = 1484, 13.1%; Chiba: n = 1263, 11.1%; Hyogo: n = 1119, 9.9%; Fukuoka: n = 1027, 9.1%). In Phase 2, residents living in Kanagawa, Saitama, and Chiba prefectures, where the emergency declaration did not apply, were excluded from the survey, and 4,592 residents of Tokyo, Osaka, Hyogo, and Fukuoka participated in the follow-up survey. In Phase 3, an additional follow-up survey was conducted with some participants who had participated in Phase 2 (N = 3892).
Study participants were recruited through Macromill Inc. (Tokyo, Japan), a global marketing research company. The company has more than 1.3 million registered members from all prefectures in Japan, with diverse characteristics (e.g., both genders, a wide range of age groups, and occupational statuses). The online survey system automatically eliminated duplicate responses from single respondents. A recruitment invitation was sent via e-mail to approximately 80,000 registered respondents living in the target area, and data were collected online. Upon receiving the link, participants completed an online survey voluntarily and anonymously, after providing informed consent online. Participants were given a clear explanation of the survey procedures and the release of data in a form that did not identify individuals and had the option to discontinue or terminate the survey at any time without providing a reason. Except for the default items provided by Macromill (gender, age, occupation, household income, marital status, and presence of children), the survey format did not allow participants to proceed to the next page if there were unanswered items. In addition, all participants were awarded Macromill points that they could exchange for prizes or cash.
This study was approved by the Research Ethics Committee of the Graduate School of Social and Industrial Science and Technology at Tokushima University (Approval No. 212). This study was conducted in accordance with the ethical standards of the 1964 Declaration of Helsinki and its amendments.
Measurements
Sociodemographic data.
Sociodemographic information, including age, gender, employment status, marital status, and household income, was collected from participants. To discuss groups assumed to be vulnerable to lockdown in previous studies in the early stages of the pandemic 9 , 10 , 11 , 12 , information was collected on whether the individual or family member was a healthcare worker and whether the individual was currently or had previously been treated for mental health problems, severe physical illnesses, or COVID-19.
Psychological distress
The Japanese version of the Kessler Psychological Distress Scale-6 (K6) 13 , a nonspecific psychological stress scale comprising six items, was used to measure psychological distress over the past 30 days. Each question was rated on a scale of 0 (never) to 4 (always), with total scores ranging from 0 to 24. The K6 is regarded as an ideal instrument for screening mental disorders in population-based health surveys because of its high accuracy and brevity 13 , 14 , 15 .
We adopted a threshold of five points commonly used to screen for mild-to-moderate mood/anxiety disorders 16 . Scores ranging from 5 to 12 indicated mild-to-moderate psychological distress. This is the optimal lower threshold for screening for moderate psychological distress 16 . Mild-to-moderate psychological distress is considered because of the associated risk of progression to more severe disability, as well as current distress and disability 17 . A threshold score of 13 has been traditionally used in previous studies 14 , 18 . A score of ≥13 was defined as serious psychological distress. Additionally, a score of ≤4 was defined as no or low psychological distress.
Depressive symptoms
The Japanese version of the Patient Health Questionnaire-9 (PHQ-9) 19 was used to assess depression. Participants reported depressive symptoms for the past 4 weeks on a scale of 0 (not at all) to 3 (almost daily) 20 .
A cutoff score of 10 or higher, as recommended by previous research, indicates a high probability of major depression 19 . The PHQ-9 has been used internationally as a screening scale for depression 21 , with high reliability and validity 19 .
Loneliness was measured using the Japanese version of the UCLA Loneliness Scale version 3 (UCLA-LS3) 22 , consisting of 10 items ranging from 1 (never) to 4 (always). The total score ranged from 10 to 40, with higher scores indicating greater loneliness 23 . The UCLA-LS3 has high reliability and validity and is used internationally as a scale to measure loneliness 24 , 25 , 26 .
Social network
Social networks were assessed by using the Japanese version of the Lubben Social Network Scale (LSNS-6) 27 . The LSNS-6 is a shortened version of the Lubben Social Network Scale 28 , and it includes items on the network size of relatives and friends who provide emotional and instrumental support. The LSNS-6 consists of three items related to family networks and three items related to friendship networks. The number of people in the network was calculated on a 6-point scale (0 = none; 1 = 1 person; 2 = 2 persons; 3 = 3–4 persons; 4 = 5–8 persons; 5 = 9 or more persons) for each item 29 . Total scores ranged from 0 to 30, with higher scores indicating greater social networks and scores below 12 indicating social isolation.
Subjective happiness
Subjective happiness was assessed using the Japanese version of the Subjective Happiness Scale (SHS) 30 , a 4-item global subjective happiness scale. The response format is a 7-point Likert scale. One composite score is computed by averaging the responses to the four items, according to the reverse coding of the fourth item. The scores range from 1 to 7, with higher scores indicating greater well-being 31 .
Physical symptoms
The Japanese version of the Somatic Symptom Scale-8 (SSS-8) was used to assess the burden of physical symptoms 32 . The SSS-8 consists of eight items that assess the following physical symptoms: stomach or bowel problems; back pain; pain in the arms, legs, or joints; headache; chest pain or shortness of breath; dizziness; fatigue or low energy; and sleep disturbances. These items comprise four symptom domains: gastrointestinal, pain, cardiopulmonary, and fatigue. Participants reported the extent to which each symptom had bothered them in the past 7 days on a scale of 0 to 4 (0 = not at all; 1 = a little bit; 2 = somewhat; 3 = quite a bit; 4 = very much) 33 .
Alcohol use
Alcohol use was assessed using the Japanese version of the Alcohol Use Disorders Identification Test (AUDIT) 34 . As the AUDIT identifies the presence or absence of alcohol-related problems based on the past one year of alcohol use, the adoption of the AUDIT in a survey conducted every other year is optimal. The test consists of 10 items across three domains: hazardous alcohol use, dependent symptoms, and harmful alcohol use (three, three, and four items, respectively). Each item is scored on a scale of 0 to 4. The lowest AUDIT score was 0, and the highest score was 40. Higher scores indicated a higher likelihood and severity of hazardous drinking, harmful drinking, and alcohol dependence. Based on the WHO AUDIT cutoff criteria and the Japanese Ministry of Health, Labour and Welfare health guidance 35 , 36 , scores of 8–14 and 15 or higher were categorized into the hazardous drinking group and potential alcohol dependence group, respectively. Participants who scored 7 or less were placed in the no alcohol problem group.
Lifestyle, coping behavior, and stressors related to the COVID-19 pandemic
With extensive reference to the literature on the COVID-19 pandemic 9 , 10 , 12 , 37 , 38 , we developed eight lifestyle and coping behavior items, and seven stressors were assumed to be associated with the COVID-19 pandemic (refer to Yamamoto et al . 39 ). We asked participants to rate the frequency of implementation and their experience of these items from the start of the state of emergency (Phases 1 and 2) or the last 30 days (Phase 3), to the time of the survey, on a scale of 1 (not at all) to 7 (extremely). The details of these items have been described in our previously published articles.
Data Records
Data records are available in XLSX format from the Open Science Framework platform, together with the questionnaire description file 39 . The datasets were anonymized to remove personal information. The abbreviation guidelines for variable names are also included in the questionnaire description file.
Technical Validation
Verification of the suitability of the timing of the survey.
We examined the suitability of the timing of the survey to investigate the impact of a prolonged pandemic considering the spread of infection and social conditions.
The first survey (Phase 1, May 11-12, 2020) was conducted during the first emergency declaration period, the second survey (Phase 2, June 14-20, 2021) was conducted during the third emergency declaration period, and the third survey (Phase 3, May 13–30, 2022) was conducted during a period of no emergency and relatively few severe cases, but with the presence of many COVID-19-positive cases (Fig. 1 ) 40 . The emergency declaration in Japan was the government’s request to refrain from leaving the house except in an emergency and temporary closure of some businesses (e.g., restrictions on the use of facilities that attract large numbers of people), and there were no penalties for violations. The investigation dates of Phases 1 and 2 were toward the ending of the states of emergency, when the effects of lifestyle changes might have been amplified. This study will enable us to observe changes in psychosocial variables across long-term periods, as well as changes in these variables due to changes in social conditions, such as the declaration of a state of emergency. As such, it will provide useful information in considering when and which factors to intervene therewith during a prolonged pandemic.
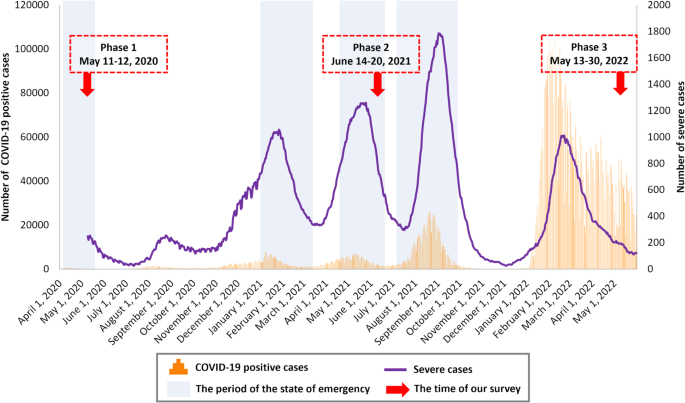
Change in the numbers of newly confirmed COVID-19-positive cases and severe cases in Japan. Newly confirmed COVID-19 positive cases: The number of newly confirmed cases is calculated by summing the number of cases published through press releases, including recurrent positive cases, by each jurisdiction. Severe cases: As a rule, severe cases are defined as those meeting one of the following conditions: (1) connected to a mechanical ventilator, (2) on ECMO, or (3) treated in the ICU (or a similar facility). However, certain jurisdictions may use other definitions.
Verification of the suitability of exposure factors
We verified the suitability of the assumption that the prolonged COVID-19 pandemic was an exposure factor that placed a long-term burden on mental health compared to studies conducted before the pandemic.
In our dataset (N = 3892), the annual household incomes of 813 participants in Phase 1, 742 participants in Phase 2, and 686 participants in Phase 3, as well as the AUDIT scores of 4 participants (all under 20 years of age) in Phase 2 were not provided; there were no missing data for other variables. Regarding the AUDIT, the survey company’s rules prevent participants under 20 years of age from responding to the AUDIT items, given that drinking alcohol under the age of 20 is legally prohibited in Japan. Table 1 shows the survey participants’ sociodemographic characteristics.
In the comparisons of psychosocial and physical variables between phases by the repeated measures analysis of variance and paired-samples t -test (only the AUDIT), there were significant differences between phases in all variables except “Healthy sleep habits” and the AUDIT score (Table 2 ). Regarding the UCLA-LS3, the SHS, “Healthy eating habits,” “Altruistic preventive behavior,” “Deterioration of household economy,” “Frustration,” and “COVID-19-related sleeplessness,” results did not exceed the lower limit of “small effect size” ( η 2 ≥ 0.010), although there were statistically significant effects of phase in these variables. While many indicators showed improvement from Phase 1 to Phase 3, there were increases in the UCLA-LS3 scores and “Deterioration of relationship with familiar people,” as well as decreases in the LSNS-6 scores, “Exercise,” “Favorite activity,” and “Online interaction with familiar people.”
Figure 2 shows the percentage of psychosocial problems based on the cutoff values for the K6, PHQ-9, LSNS-6, and AUDIT. For the K6, the prevalence of mild-to-moderate psychological distress (K6 score = 5–12) decreased significantly from Phase 1 to Phase 2 (Phase 1: 34.4%, Phase 2: 24.9%), whereas the prevalence of severe psychological distress (K6 score ≥ 13) changed only slightly over the 2-year period (Phase 1: 9.7%; Phase 2: 7.9%). According to data published by the Ministry of Health, Labour and Welfare in 2019 concerning the K6 in the Japanese population, 26.9% of participants had mild-to-moderate or severe psychological stress (i.e., K6 score ≥ 5) 41 while our data showed that 44.1% and 32.8% of participants had it in Phases 1 and 2, respectively. For the PHQ-9, the prevalence of depression (PHQ-9 score ≥ 10) decreased slightly from Phase 1 to Phase 2 (Phase 1: 15.8%, Phase 2: 13.6%). In a previous survey of the general Japanese population conducted in 2013, 7.9% of participants reported a PHQ-9 score of ≥10 42 . Thus, we could conclude that psychological distress and depression did not improve sufficiently in our survey, even in Phase 3, compared with before the pandemic. For the LSNS-6, the prevalence of social isolation increased slightly from Phase 1 to Phase 2. In a previous study conducted before the pandemic 27 , the prevalence of social isolation (less than 12 points) in Japan was 19.4%, and our survey showed a much higher prevalence. For the AUDIT, there was a slight increase in hazardous users, but little change in the prevalence of potential alcoholism. Based on gender, the prevalence of potential alcoholism in men was 10.4% in Phase 2 and 10.1% in Phase 3, whereas that of hazardous alcohol use was 16.0% in Phase 2 and 17.5% in Phase 3. The prevalence of potential alcoholism in women was 4.7% in Phase 2 and 4.6% in Phase 3, and that of hazardous alcohol use was 6.2% in Phase 2 and 6.6% in Phase 3. Prior research before the pandemic showed that the prevalence of potential alcoholism in Japan was 5.2% for men and 0.7% for women, and that of hazardous alcohol use was 16.2% for men and 3.8% for women 43 . In men, the prevalence of potential alcoholism increased compared to a previous study 43 , and in women, both the prevalence of hazardous use and potential alcoholism increased, with the latter being particularly prominent.
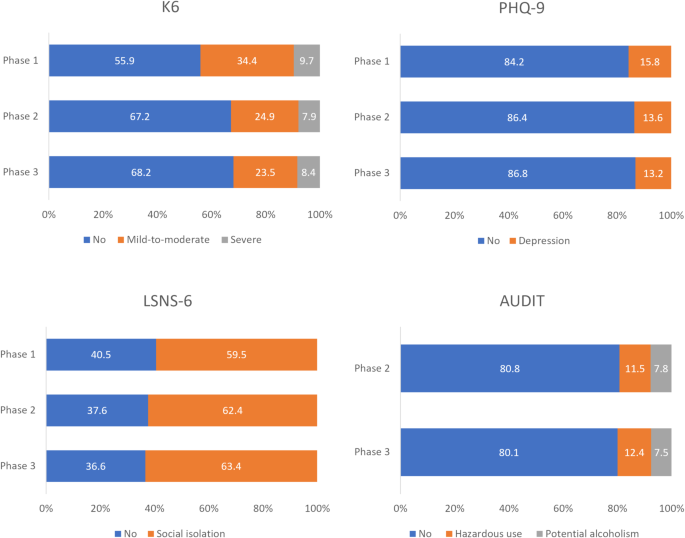
Percentage of psychosocial problems based on cutoffs for each measure.
Usage Notes
This dataset was obtained through a large-scale survey conducted annually over a two-year period, allowing for time series analysis stratified by demographic characteristics and other factors. Two of the three time points surveyed were at the final stages of the state of emergency, a period of particular stress, and thus may be useful for detecting the effects of the emergency. In addition, several globally used measures were employed in the dataset, which facilitated comparisons with other studies.
However, this study has several limitations. First, as the data were collected through an online survey, random sampling was not conducted. Therefore, we cannot guarantee the representativeness of the sample, as it cannot be matched to the percentages of each age group or gender in each region. Compared with the actual age ratio, the data are more skewed toward the middle-aged and less toward the elderly 44 , and accordingly, the household income is higher 45 , and a higher percentage of participants were workers in terms of their employment status 46 . Therefore, it may be desirable to consider age when analyzing the data, if necessary. However, when people are encouraged to avoid unnecessary outings, online surveys can be a valuable way to assess people’s health status. Second, although it is natural that the number of past treatments for mental problems and severe physical illnesses should increase over time, it decreased in Phases 2 and 3. This may indicate that the definitions of mental problems and severe physical illnesses differed for participants in each phase, and the data on these items should be treated with caution. Third, the dropout rate of participants in this study was high; 2,831 (42.1%) of the 6,723 individuals who participated in Phase 1 did not respond in Phase 2 or 3. In addition, there were significantly more females than males among individuals who did not participate in Phase 2 or 3 ( p < 0.001). Individuals who did not participate in Phase 2 or 3 were younger and had lower UCLA-LS3 scores and higher LSNS-6, K6, PHQ-9, and SSS-8 scores than those who participated in the three phases ( p < 0.001). Thus, many participants with mental and physical problems may have been excluded from the dataset.
Code availability
No custom codes were used in this study. Microsoft Excel was used to tabulate and distribute the data.
World Health Organization. COVID-19 weekly epidemiological update , edition 156, 17 August 2023. World Health Organization. https://apps.who.int/iris/handle/10665/372386 (2023).
Liu, X. et al . Public mental health problems during COVID-19 pandemic: A large-scale meta-analysis of the evidence. Transl. Psychiatry 11 , 384 (2021).
Article CAS PubMed PubMed Central Google Scholar
Prati, G. & Mancini, A. D. The psychological impact of COVID-19 pandemic lockdowns: A review and meta-analysis of longitudinal studies and natural experiments. Psychol. Med. 51 , 201–211 (2021).
Article PubMed Google Scholar
Yamamoto, T., Uchiumi, C., Suzuki, N., Yoshimoto, J. & Murillo-Rodriguez, E. The psychological impact of ‘mild lockdown’ in Japan during the COVID-19 pandemic: A nationwide survey under a declared state of emergency. Int. J. Environ. Res. Public Health 17 , 9382 (2020).
Yamamoto, T. et al . Mental health and social isolation under repeated mild lockdowns in Japan. Sci. Rep. 12 , 8452 (2022).
Article ADS CAS PubMed PubMed Central Google Scholar
Sugaya, N., Yamamoto, T., Suzuki, N. & Uchiumi, C. Social isolation and its psychosocial factors in mild lockdown for the COVID-19 pandemic: a cross-sectional survey of the Japanese population. BMJ Open 11 , e048380 (2021).
Sugaya, N., Yamamoto, T., Suzuki, N. & Uchiumi, C. The transition of social isolation and related psychological factors in 2 mild lockdown periods during the COVID-19 pandemic in Japan: longitudinal survey study. JMIR Public Health Surveill. 8 , e32694 (2022).
Article PubMed PubMed Central Google Scholar
Sugaya, N., Yamamoto, T., Suzuki, N. & Uchiumi, C. Change in alcohol use during the prolonged COVID-19 pandemic and its psychosocial factors: a one-year longitudinal study in Japan. Int. J. Environ. Res. Public Health 20 , 3871 (2023).
Brooks, S. K. et al . The psychological impact of quarantine and how to reduce it: Rapid review of the evidence. Lancet 395 , 912–920 (2020).
Mazza, M. et al . A nationwide survey of psychological distress among Italian people during the COVID-19 pandemic: Immediate psychological responses and associated factors. Int. J. Environ. Res. Public Health 17 , 3165 (2020).
Holmes, E. A. et al . Multidisciplinary research priorities for the COVID-19 pandemic: a call for action for mental health science. Lancet Psychiatry 7 , 547–560 (2020).
Kisely, S. et al . Occurrence, prevention, and management of the psychological effects of emerging virus outbreaks on healthcare workers: A rapid review and meta-analysis. BMJ 369 , m1642 (2020).
Furukawa, T. A., Kessler, R. C., Slade, T. & Andrews, G. The performance of the K6 and K10 screening scales for psychological distress in the Australian National Survey of Mental Health and Well-Being. Psychol. Med. 33 , 357–362 (2003).
Article CAS PubMed Google Scholar
Kessler, R. C. et al . Screening for serious mental illness in the general population. Arch. Gen. Psychiatry 60 , 184–189 (2003).
Veldhuizen, S., Cairney, J., Kurdyak, P. & Streiner, D. L. The sensitivity of the K6 as a screen for any disorder in community mental health surveys: A cautionary note. Can. J. Psychiatry 52 , 256–259 (2007).
Prochaska, J. J., Sung, H. Y., Max, W., Shi, Y. & Ong, M. Validity study of the K6 scale as a measure of moderate mental distress based on mental health treatment need and utilization. Int. J. Methods Psychiatr. Res. 21 , 88–97 (2012).
Kessler, R. C. et al . Mild Disorders Should Not Be Eliminated from the DSM-V. Arch. Gen. Psychiatry. 60 , 1117–1122 (2003).
Kessler, R. C. et al . Trends in mental illness and suicidality after Hurricane Katrina. Mol. Psychiatry. 13 , 374–384 (2008).
Muramatsu, K. et al . Performance of the Japanese version of the Patient Health Questionnaire-9 (J-PHQ-9) for depression in primary care. Gen. Hosp. Psychiatry 52 , 64–69 (2018).
Kroenke, K., Spitzer, R. L. & Williams, J. B. W. The PHQ-9: Validity of a brief depression severity measure. J. Gen. Intern. Med. 16 , 606–613 (2001).
Siu, A. L. et al . Screening for depression in adults: US preventive services task force recommendation statement. J. Am. Med. Assoc. 315 , 380–387 (2016).
Article CAS Google Scholar
Arimoto, A. & Tadaka, E. Reliability and validity of Japanese versions of the UCLA loneliness scale version 3 for use among mothers with infants and toddlers: A cross-sectional study. BMC Women’s Health 19 , 105 (2019).
Russell, D. W. UCLA Loneliness Scale (Version 3): reliability, validity, and factor structure. J. Pers. Assess. 66 , 20–40 (1996).
Durak, M. & Senol-Durak, E. Psychometric qualities of the UCLA loneliness scale-version 3 as applied in a Turkish culture. Educ. Gerontol. 36 , 988–1007 (2010).
Article Google Scholar
Shevlin, M., Murphy, S. & Murphy, J. The Latent Structure of Loneliness: Testing Competing Factor Models of the UCLA Loneliness Scale in a Large Adolescent Sample. Assessment 22 , 208–215 (2015).
Zarei, S., Memari, A. H., Moshayedi, P. & Shayestehfar, M. Validity and reliability of the UCLA loneliness scale version 3 in Farsi. Educ. Gerontol. 42 , 49–57 (2016).
Kurimoto, A. et al . Reliability and validity of the Japanese version of the abbreviated Lubben Social Network Scale. Jpn. J. Geriatr. 48 , 149–157 (2011).
Lubben, J. E. Assessing social networks among elderly populations. Fam. Commun. Heal. 11 , 42–52 (1988).
Lubben, J. E. et al . Performance of an abbreviated version of the Lubben Social Network Scale among three European community-dwelling older adult populations. Gerontologist 46 , 503–513 (2006).
Shimai, S., Otake, K., Utsuki, N., Ikemi, A. & Lyubomirsky, S. Development of a Japanese version of the Subjective Happiness Scale (SHS), and examination of its validity and reliability. Jpn. J. Public Health 51 , 845–853 (2004).
Google Scholar
Lyubomirsky, S. & Lepper, H. S. A measure of subjective happiness: Preliminary reliability and construct validation. Soc. Indic . Res. 46 , 137–155 (1999).
Matsudaira, K. et al . Development of a Japanese version of the Somatic Symptom Scale-8: Psychometric validity and internal consistency. Gen. Hosp. Psychiatry 45 , 7–11 (2017).
Gierk, B. et al . The somatic symptom scale-8 (SSS-8): a brief measure of somatic symptom burden. JAMA Intern. Med. 174 , 399–407 (2014).
Hiro, H. & Shima, S. Availability of the Alcohol Use Disorders Identification Test (AUDIT) for a complete health examination in Japan. Nihon Arukoru Yakubutsu Igakkai Zasshi 31 , 437–450 (1996).
CAS PubMed Google Scholar
World Health Organization. AUDIT: The Alcohol Use Disorders Identification Test: Guidelines for Use in Primary Healthcare . World Health Organization: Geneva, Switzerland (2001).
Japanese Ministry of Health, Labour and Welfare. Standard Health Checkups and Health Guidance Program . Available online: https://www.mhlw.go.jp/file/06-Seisakujouhou-10900000-Kenkoukyoku/00_3.pdf (2018).
Ahorsu, D. K. et al . The fear of COVID-19 scale: Development and initial validation. Int. J. Ment. Health Addict. 27 , 1–9 (2020).
Tang, W. et al . Prevalence and correlates of PTSD and depressive symptoms one month after the outbreak of the COVID-19 epidemic in a sample of home-quarantined Chinese university students. J. Affect. Disord. 274 , 1–7 (2020).
Yamamoto, T., Sugaya, N. & Uchiumi, C. A two-year longitudinal study examining the change in psychosocial factors under the prolonged COVID-19 pandemic in Japan. Open Science Framework https://doi.org/10.17605/OSF.IO/NGW6D (2023).
Ministry of Health Labour and Welfare. Visualizing the data: information on COVID-19 infections . https://covid19.mhlw.go.jp/extensions/public/en/index.html (2023).
Ministry of Health Labour and Welfare. Comprehensive Survey of Living Conditions . https://www.mhlw.go.jp/english/database/db-hss/cslc-index.html (2020).
Hoshino, E. et al . Variation in somatic symptoms by patient health questionnaire-9 depression scores in a representative Japanese sample. BMC Public Health 18 , 1406 (2018).
Kinjo, A. et al . Different socioeconomic backgrounds between hazardous drinking and heavy episodic drinking: Prevalence by sociodemographic factors in a Japanese general sample. Drug Alcohol Depend. 193 , 55–62 (2018).
Statistics Bureau of Japan. Current Population Estimates as of October 1, 2022 . https://www.stat.go.jp/english/data/jinsui/2022np/index.html (2022).
Ministry of Health, Labour and Welfare. Comprehensive Survey of Living Conditions, 2022 . https://www.mhlw.go.jp/toukei/saikin/hw/k-tyosa/k-tyosa22/dl/03.pdf (2022).
Statistics Bureau of Japan. Labour Force Survey in 2022 . https://www.stat.go.jp/data/roudou/sokuhou/nen/ft/pdf/index1.pdf (2022).
Download references
Acknowledgements
This work was supported by a Grant-in-Aid for Scientific Research from the Japan Society for the Promotion of Science (JSPS KAKENHI; Grants 18K13323, 20K10883, 21H00949, and 22K10586); the FY 2021 Discretionary Funds for the Research Director of Tokushima University; the Project for Creative Research of the Faculty of Integrated Science, Tokushima University, Research-Aid; Meiji Yasuda Mental Health Foundation; and the JPA Research Grant for the COVID-19 Pandemic. The funders had no role in the study design, data collection and analysis, decision to publish, or manuscript preparation. We would like to thank Ms. Naho Suzuki for checking the survey form and assistance with data organization.
Author information
Authors and affiliations.
Occupational Stress and Health Management Research Group, National Institute of Occupational Safety and Health, Kawasaki, Japan
Nagisa Sugaya
Graduate School of Technology, Industrial and Social Sciences, Tokushima University, Tokushima, Japan
Tetsuya Yamamoto & Chigusa Uchiumi
You can also search for this author in PubMed Google Scholar
Contributions
N.S., T.Y., and C.U. conceived, designed, and performed the study; contributed to and wrote the paper; and approved the final manuscript. N.S. analyzed the data.
Corresponding author
Correspondence to Tetsuya Yamamoto .
Ethics declarations
Competing interests.
The authors declare no competing interests.
Additional information
Publisher’s note Springer Nature remains neutral with regard to jurisdictional claims in published maps and institutional affiliations.
Rights and permissions
Open Access This article is licensed under a Creative Commons Attribution 4.0 International License, which permits use, sharing, adaptation, distribution and reproduction in any medium or format, as long as you give appropriate credit to the original author(s) and the source, provide a link to the Creative Commons licence, and indicate if changes were made. The images or other third party material in this article are included in the article’s Creative Commons licence, unless indicated otherwise in a credit line to the material. If material is not included in the article’s Creative Commons licence and your intended use is not permitted by statutory regulation or exceeds the permitted use, you will need to obtain permission directly from the copyright holder. To view a copy of this licence, visit http://creativecommons.org/licenses/by/4.0/ .
Reprints and permissions
About this article
Cite this article.
Sugaya, N., Yamamoto, T. & Uchiumi, C. A 2-year longitudinal study examining the change in psychosocial factors under the COVID-19 pandemic in Japan. Sci Data 11 , 544 (2024). https://doi.org/10.1038/s41597-024-03125-2
Download citation
Received : 13 November 2023
Accepted : 04 March 2024
Published : 28 May 2024
DOI : https://doi.org/10.1038/s41597-024-03125-2
Share this article
Anyone you share the following link with will be able to read this content:
Sorry, a shareable link is not currently available for this article.
Provided by the Springer Nature SharedIt content-sharing initiative
Quick links
- Explore articles by subject
- Guide to authors
- Editorial policies
Sign up for the Nature Briefing newsletter — what matters in science, free to your inbox daily.

- Introduction
- Conclusions
- Article Information
CT indicates computed tomography; ED, emergency department; HRS, Health and Retirement Study; ICD-9 or ICD-10 , International Classification of Diseases, Ninth or Tenth Revision; MRI, magnetic resonance imaging; and TBI, traumatic brain injury.
Data were adjusted for demographic and health characteristics. ADI score range is 1 to 100, with higher scores indicating higher levels of deprivation. GED indicates General Educational Development; HS, high school; and TBI, traumatic brain injury.
eTable 1. Baseline characteristics of HRS enrollees with and without TBI (diagnosis code only) during study follow-up
eTable 2. Multivariate models showing time to incident TBI (diagnosis code only)
eTable 3. Multivariate models showing time to incident TBI (diagnosis code only), stratified by hospital admission (inpatient vs outpatient)
eTable 4. Multivariate models showing time to incident TBI (diagnosis code only), stratified by hospital cluster: private vs public or other
Data Sharing Statement
See More About
Sign up for emails based on your interests, select your interests.
Customize your JAMA Network experience by selecting one or more topics from the list below.
- Academic Medicine
- Acid Base, Electrolytes, Fluids
- Allergy and Clinical Immunology
- American Indian or Alaska Natives
- Anesthesiology
- Anticoagulation
- Art and Images in Psychiatry
- Artificial Intelligence
- Assisted Reproduction
- Bleeding and Transfusion
- Caring for the Critically Ill Patient
- Challenges in Clinical Electrocardiography
- Climate and Health
- Climate Change
- Clinical Challenge
- Clinical Decision Support
- Clinical Implications of Basic Neuroscience
- Clinical Pharmacy and Pharmacology
- Complementary and Alternative Medicine
- Consensus Statements
- Coronavirus (COVID-19)
- Critical Care Medicine
- Cultural Competency
- Dental Medicine
- Dermatology
- Diabetes and Endocrinology
- Diagnostic Test Interpretation
- Drug Development
- Electronic Health Records
- Emergency Medicine
- End of Life, Hospice, Palliative Care
- Environmental Health
- Equity, Diversity, and Inclusion
- Facial Plastic Surgery
- Gastroenterology and Hepatology
- Genetics and Genomics
- Genomics and Precision Health
- Global Health
- Guide to Statistics and Methods
- Hair Disorders
- Health Care Delivery Models
- Health Care Economics, Insurance, Payment
- Health Care Quality
- Health Care Reform
- Health Care Safety
- Health Care Workforce
- Health Disparities
- Health Inequities
- Health Policy
- Health Systems Science
- History of Medicine
- Hypertension
- Images in Neurology
- Implementation Science
- Infectious Diseases
- Innovations in Health Care Delivery
- JAMA Infographic
- Law and Medicine
- Leading Change
- Less is More
- LGBTQIA Medicine
- Lifestyle Behaviors
- Medical Coding
- Medical Devices and Equipment
- Medical Education
- Medical Education and Training
- Medical Journals and Publishing
- Mobile Health and Telemedicine
- Narrative Medicine
- Neuroscience and Psychiatry
- Notable Notes
- Nutrition, Obesity, Exercise
- Obstetrics and Gynecology
- Occupational Health
- Ophthalmology
- Orthopedics
- Otolaryngology
- Pain Medicine
- Palliative Care
- Pathology and Laboratory Medicine
- Patient Care
- Patient Information
- Performance Improvement
- Performance Measures
- Perioperative Care and Consultation
- Pharmacoeconomics
- Pharmacoepidemiology
- Pharmacogenetics
- Pharmacy and Clinical Pharmacology
- Physical Medicine and Rehabilitation
- Physical Therapy
- Physician Leadership
- Population Health
- Primary Care
- Professional Well-being
- Professionalism
- Psychiatry and Behavioral Health
- Public Health
- Pulmonary Medicine
- Regulatory Agencies
- Reproductive Health
- Research, Methods, Statistics
- Resuscitation
- Rheumatology
- Risk Management
- Scientific Discovery and the Future of Medicine
- Shared Decision Making and Communication
- Sleep Medicine
- Sports Medicine
- Stem Cell Transplantation
- Substance Use and Addiction Medicine
- Surgical Innovation
- Surgical Pearls
- Teachable Moment
- Technology and Finance
- The Art of JAMA
- The Arts and Medicine
- The Rational Clinical Examination
- Tobacco and e-Cigarettes
- Translational Medicine
- Trauma and Injury
- Treatment Adherence
- Ultrasonography
- Users' Guide to the Medical Literature
- Vaccination
- Venous Thromboembolism
- Veterans Health
- Women's Health
- Workflow and Process
- Wound Care, Infection, Healing
Get the latest research based on your areas of interest.
Others also liked.
- Download PDF
- X Facebook More LinkedIn
Kornblith E , Diaz-Ramirez LG , Yaffe K , Boscardin WJ , Gardner RC. Incidence of Traumatic Brain Injury in a Longitudinal Cohort of Older Adults. JAMA Netw Open. 2024;7(5):e2414223. doi:10.1001/jamanetworkopen.2024.14223
Manage citations:
© 2024
- Permissions
Incidence of Traumatic Brain Injury in a Longitudinal Cohort of Older Adults
- 1 San Francisco Veterans Affairs Health Care System, San Francisco, California
- 2 Department of Psychiatry, University of California San Francisco, San Francisco
- 3 Department of Medicine, University of California, San Francisco, San Francisco
- 4 Northern California Institute for Research and Education, San Francisco, California
- 5 Department of Neurology, University of California, San Francisco, San Francisco
- 6 Department of Epidemiology and Biostatistics, University of California, San Francisco, San Francisco
- 7 Joseph Sagol Neuroscience Center, Sheba Medical Center, Ramat Gan, Israel
Question How common is traumatic brain injury (TBI) among older adults in the US?
Findings Over an 18-year study period, this cohort study found that 12.9% of 9239 study respondents experienced TBI. Race and ethnicity, sex, cognition, educational level, and medical conditions were associated with TBI status.
Meaning Findings of this study suggest that incident TBI is common among older adults and may be associated with demographic or social factors.
Importance Traumatic brain injury (TBI) occurs at the highest rate in older adulthood and increases risk for cognitive impairment and dementia.
Objectives To update existing TBI surveillance data to capture nonhospital settings and to explore how social determinants of health (SDOH) are associated with TBI incidence among older adults.
Design, Setting, and Participants This nationally representative longitudinal cohort study assessed participants for 18 years, from August 2000 through December 2018, using data from the Health and Retirement Study (HRS) and linked Medicare claims dates. Analyses were completed August 9 through December 12, 2022. Participants were 65 years of age or older in the HRS with survey data linked to Medicare without a TBI prior to HRS enrollment. They were community dwelling at enrollment but were retained in HRS if they were later institutionalized.
Exposures Baseline demographic, cognitive, medical, and SDOH information from HRS.
Main Outcomes and Measures Incident TBI was defined using inpatient and outpatient International Classification of Disease s, Ninth or Tenth Revision, diagnosis codes received the same day or within 1 day as the emergency department (ED) visit code and the computed tomography (CT) or magnetic resonance imaging (MRI) code, after baseline HRS interview. A cohort with TBI codes but no ED visit or CT or MRI scan was derived to capture diagnoses in nonhospital settings. Descriptive statistics and bivariate associations of TBI with demographic and SDOH characteristics used sample weights. Fine-Gray regression models estimated associations between covariates and TBI, with death as a competing risk. Imputation considering outcome and complex survey design was performed by race and ethnicity, sex, education level, and Area Deprivation Index percentiles 1, 50, and 100. Other exposure variables were fixed at their weighted means.
Results Among 9239 eligible respondents, 5258 (57.7%) were female and 1210 (9.1%) were Black, 574 (4.7%) were Hispanic, and 7297 (84.4%) were White. Mean (SD) baseline age was 75.2 (8.0) years. During follow-up (18 years), 797 (8.9%) of respondents received an incident TBI diagnosis with an ED visit and a CT code within 1 day, 964 (10.2%) received an incident TBI diagnosis and an ED code, and 1148 (12.9%) received a TBI code with or without an ED visit and CT scan code. Compared with respondents without incident TBI, respondents with TBI were more likely to be female (absolute difference, 7.0 [95% CI, 3.3-10.8]; P < .001) and White (absolute difference, 5.1 [95% CI, 2.8-7.4]; P < .001), have normal cognition (vs cognitive impairment or dementia; absolute difference, 6.1 [95% CI, 2.8-9.3]; P = .001), higher education (absolute difference, 3.8 [95% CI, 0.9-6.7]; P < .001), and wealth (absolute difference, 6.5 [95% CI, 2.3-10.7]; P = .01), and be without baseline lung disease (absolute difference, 5.1 [95% CI, 3.0-7.2]; P < .001) or functional impairment (absolute difference, 3.3 [95% CI, 0.4-6.1]; P = .03). In adjusted multivariate models, lower education (subdistribution hazard ratio [SHR], 0.73 [95% CI, 0.57-0.94]; P = .01), Black race (SHR, 0.61 [95% CI, 0.46-0.80]; P < .001), area deprivation index national rank (SHR 1.00 [95% CI 0.99-1.00]; P = .009), and male sex (SHR, 0.73 [95% CI, 0.56-0.94]; P = .02) were associated with membership in the group without TBI. Sensitivity analyses using a broader definition of TBI yielded similar results.
Conclusions and Relevance In this longitudinal cohort study of older adults, almost 13% experienced incident TBI during the 18-year study period. For older adults who seek care for TBI, race and ethnicity, sex, and SDOH factors may be associated with incidence of TBI, seeking medical attention for TBI in older adulthood, or both.
Traumatic brain injury (TBI) is common in the US and occurs at the highest rate in older adulthood. 1 , 2 It is associated with several negative cognitive and functional outcomes and staggering health care costs. 3 Vulnerability to TBI may differ by sociodemographic factors and presence of cognitive impairment or dementia, 3 yet TBI incidence among older adults and their subgroups is poorly characterized. The most recent comprehensive numbers, from 2014, come from a study that included only hospital codes and as such do not capture older adults who receive treatment for a TBI in an outpatient (ie, primary care, urgent care) setting. 4
Social determinants of health (SDOH) are defined as the conditions in which individuals exist in their daily lives, which are shaped by the distribution of resources and presence of obstacles at both the global and local levels. 5 These social, demographic, and contextual factors may include education and employment, socioeconomic status, region, and community or neighborhood variables. Because they reflect the day-to-day outcomes of historical legacies of systemic oppression, SDOH are highly correlated with race and ethnicity and may underly race- and ethnicity-based health disparities. Social determinants of health impact risk for multiple medical conditions and are thought to underly disparities in conditions such as diabetes and cardiovascular disease, 6 which are associated with increased risk of TBI 7 as well as with accelerated aging and dementia. 8 However, the impact of SDOH on risk of TBI is unknown. Social determinants of health also impact how individuals interact with the medical system and whether and how they access care for a particular injury or illness.
By accessing linked Medicare claims data to obtain incident TBI diagnoses, we were able to leverage the detailed cognitive, demographic, and SDOH information available in the nationally representative Health and Retirement Study (HRS) to explore the association of cognitive status, demographics, and SDOH with the incidence of TBI in a diverse cohort of older adults. We were also able to further understanding of the epidemiology of TBI among older adults by including data up to 2018 (compared with the most recent comprehensive data, from 2014). Moreover, we included codes received in nonhospital settings (ie, outpatient, primary care, urgent care, and specialty care), thereby adding granularity to our understanding of risk for TBI among older adults. Although the use of diagnostic codes has limitations, the current work provides opportunities to examine both the scale of TBI as a medical issue for older adults as well as to document potential biases in access to care or documentation of TBI diagnoses.
Our goal was to investigate the association of demographics and SDOH with TBI incidence to inform both targeted intervention (eg, primary TBI prevention) for groups most at risk and to explore identification and mollification of relevant structural and contextual factors to reduce risk of TBI for older adults.
This cohort study follows the Strengthening The Reporting of Observational Studies in Epidemiology ( STROBE ) reporting guideline. All study procedures were approved by institutional review boards and the University of California, San Francisco, and San Francisco Veterans Affairs Medical Center. The requirement to obtain informed consent was waived by these entities because of the use of deidentified archival data.
From 34 409 HRS community-dwelling age-eligible respondents with a core interview in 2000 or later, we created a nationally representative cohort of 9239 community-dwelling seniors enrolled in the HRS in survey waves of 2000 through 2018 who had Medicare-linked data from 2000 through 2018 (latest year available as of December 1, 2023). Respondents were community dwelling at enrollment but were retained in HRS if they were later institutionalized. See Figure 1 for details of exclusion and cohort derivation. The baseline date was the date of the first age-eligible HRS core interview in the community in 2000 or later. The HRS is sponsored by the National Institute on Aging and is conducted by the University of Michigan. 9 , 10
We identified incident TBI in inpatient and outpatient Medicare 2000 through 2018 claims. To ascertain TBI status, we used an existing, comprehensive, and updated list of International Classification of Diseases, Ninth Revision ( ICD-9 ) and Tenth Revision ( ICD-10 ) diagnosis codes developed by the Defense and Veterans Brain Injury Center and the Armed Forces Health Surveillance Branch for TBI surveillance (2016 criteria) to identify incident TBI in Medicare data, received the same day or 1 day before or after an emergency department (ED) visit code and a computed tomography (CT) or magnetic resonance imaging (MRI) scan code occurring after the enrollee’s baseline HRS interview. To conduct a sensitivity analysis, we also derived a cohort of older adults who received a TBI code but no ED visit or CT or MRI scan to capture individuals receiving diagnoses in nonhospital settings. The time to first event was computed as the time difference between the baseline date and “claim admission date” (inpatient file) or “claim from date” (outpatient file) from the first claim with a TBI diagnosis code.
We examined the following 2 exposures. The first exposure was cognitive status as assessed using the Langa-Weir dementia probability, a predictive model, frequently used in dementia research and well-validated, 11 which classifies each HRS participant as having Alzheimer disease or related dementia, cognitive impairment with no dementia, or normal cognition. The second exposure was demographic and SDOH variables. The HRS includes self-reported data on demographics (race and ethnicity and sex), detailed information about educational level, employment, and individual- and neighborhood-level socioeconomic status as well as data on rurality. The categories for race and ethnicity included Black, Hispanic, White, or other. Education was defined as a 4-level variable: less than high school or General Educational Development, high school, some college, or college and above. Employment was defined as a dichotomous variable based on self-report of whether the respondent was currently employed for pay at the time of baseline survey. Neighborhood socioeconomic status was defined by the national percentile of the block group area deprivation index national rank 12 (ADI) score (range 1-100, with higher scores indicating higher levels of deprivation). Individual socioeconomic status was defined by weighted quartiles of total assets: $46 879 or lower, more than $46 879 to $154 969, more than $154 969 to $387 837, more than $387 837. Rurality was a harmonized, dichotomous variable reflecting urban vs rural residence at the time of entry into HRS.
We adjusted analyses for splines of age (defined at Harrell default quantiles of age with 4 knots at 65.7, 70.9, 77.6, and 88.5) as well as important medical and behavioral factors (cardiovascular disease, splines of body mass index [BMI], alcohol use, stroke, diabetes, and lung disease); any activity of daily living difficulty in bathing, dressing, toileting, transferring, and eating; marital status; veteran status; physical activity (a dichotomous variable, with participants coded as 1 [yes] if they reported performing vigorous physical activity more than once per week); ADI score; and continuous format psychiatric symptom severity (ie, Center for Epidemiologic Studies Depression Scale score) variables associated with, and that may confound, the association between various exposures and incident TBI. All covariates were ascertained at the time of entry into the study, that is, the date of the respondent’s first age-eligible HRS core interview in the community in 2000 or later.
We accounted for the HRS complex survey design. For continuous variables, the assumption of normality was checked by visual examination using histograms with a normal-density curve and quantile-quantile plots. We used Fine-Gray competing risks regression 13 to estimate the associations between exposures and TBI in the presence of death as a competing risk. We used HRS survey weights and the robust sandwich variance estimation with a linear combination of the number of clusters and strata.
We checked the proportional hazards assumption by visual examination. We also tested the interaction terms with age, BMI, and ADI. Overall, the plotted curves for each level of the categorical exposure variables in the model were approximately parallel. Moreover, the inclusion of the interaction terms with time of age, BMI, and ADI did not meaningfully affect the results. Thus, the proportional hazards assumption was not violated.
We did not impute ADI since the algorithm to compute this variable includes several variables that were not present in our dataset. For the rest of the exposure variables, we imputed missing values using the fully conditional specification method. For most of the exposure variables, except for race and ethnicity and BMI, we used the logistic regression method with the default binary logit model for 8 binary predictors and the cumulative logit function for the ordered categorical variable education. For race and ethnicity, we used the discriminant function method, and for BMI we used the predictive mean matching method. Imputation was performed considering the outcome and complex survey design variables, including survey weights, clusters, and strata.
We computed the mean cumulative incidence across 50 multiple imputed datasets by race and ethnicity, sex, educational level, and ADI percentiles 1, 50, and 100. We fixed the rest of the exposure variables at their weighted means.
All analyses were performed with SAS/STAT, version 15.2 (SAS Institute Inc), Stata, version 17.0 (StataCorp LLC), and R, version 4.2.1 (R Project for Statistical Computing). A 2-sided value of P < .05 was considered statistically significant.
The final analytic cohort included 9239 older adults (5258 [57.7%] female, 3981 [42.3%] male; 1210 [9.1%] Black, 574 [4.7%] Hispanic, 7197 [84.4%] White, and 152 [1.8%] self-identified as American Indian or Alaskan Native; Asian, Native Hawaiian, or Other Pacific Islander; or other, more than one, or unknown race). Mean (SD) baseline age was 75.2 (8.0) years. Bivariate associations between exposures and TBI incidence are shown in Table 1 . Respondents were most likely to have less than a high school education and to reside in urban areas of the southern US. During the study follow-up period of approximately 18 years, 797 (8.9%) received an incident TBI diagnosis with an ED and a CT code, 964 (10.2%) received ED treatment for an incident TBI, and 1148 (12.9%) received an incident TBI diagnosis code either with or without ED and CT. The percentage of respondents missing at least 1 exposure variable in the primary model was 14.3%, with 10.4% missing Center for Epidemiologic Studies Depression Scale score, 2.7% missing ADI score, and 1.3% missing BMI. The remaining covariates had less than 1% missing.
Table 1 shows medical, demographic, and SDOH characteristics of the sample with (n = 797) or without (n = 8442) TBI. Older adults who experienced incident TBI during the study period were more likely to be female (absolute difference, 7.0 [95% CI, 3.3-10.8]; P < .001) and White (absolute difference, 5.1 [95% CI, 2.8-7.4]; P < .001), have normal cognition (vs cognitive impairment or dementia; absolute difference, 6.1 [95% CI, 2.8-9.3]; P = .001), higher education (absolute difference, 3.8 [95% CI, 0.9-6.7]; P < .001), and wealth (absolute difference, 6.5 [95% CI, 2.3-10.7]; P = .01), and be without baseline lung disease (absolute difference, 5.1 [95% CI, 3.0-7.2]; P < .001) or functional impairment (absolute difference, 3.3 [95% CI, 0.4-6.1]; P = .03). Respondents who endorsed US military veteran status were less likely to experience an incident TBI (absolute difference, 3.5 [95% CI, 0.1-7.0]; P = .06) as well.
Sensitivity analyses including elders who received an incident TBI code but no ED visit and CT or MRI scan (n = 1148) showed similar results (eTables 1 and 2 in Supplement 1 ). Other sensitivity analyses using codes only (ie, TBI diagnosis but no ED visit or imaging scan) showed similar results when stratified by hospital admission status, with the exception that female sex was no longer associated with incident TBI (HR, 0.81 [95% CI, 0.52-1.27]; P = .36) (eTable 3 in Supplement 1 ). In additional sensitivity analyses stratified by public vs private or other hospital cluster, the findings for sex (HR, 0.82 [95% CI, 0.55-1.23]; P = .35), race and ethnicity (HR, 0.67 [95% CI, 0.33-1.37]; P = .27) and educational level (HR, 0.59 [95% CI, 0.34-1.03]; P = .06) no longer reached statistical significance. However, the sample number was much smaller due to missing data on hospital cluster 2000 through 2009, resulting in the exclusion of several covariates from the model. Thus, these results should be interpreted with caution (eTable 4 in Supplement 1 ).
Figure 2 shows imputed cumulative incidence of TBI over time by race or ethnicity, sex, educational level, and neighborhood characteristics (ie, ADI 12 ). Imputation considering outcome and complex survey design was performed by race and ethnicity, sex, education level, and ADI percentiles 1, 50, and 100. Other exposure variables were fixed at their weighted means. Female sex, higher education, and residence in higher resource areas were associated with higher incidence of TBI. Examining the plots for race and ethnicity, we found that cumulative incidence of TBI was highest for respondents who categorize their race and ethnicity as other . White race was associated with higher cumulative incidence compared with Black and Hispanic race and ethnicity. Notably, the change in trajectory around 2015 is most likely due to the change from using ICD-9 to ICD-10 codes and not representative of a true change in TBI incidence. The increase in trajectory slope around 2018 may be associated with providers experiencing increased comfort in the use of the ICD-10 codes.
In multivariate models with incident TBI as the outcome and adjusted for demographics and medical and SDOH factors, lower educational level (subdistribution HR [SHR], 0.73 [95% CI, 0.57-0.94]; P = .01), Black race (SHR, 0.61 [95% CI, 0.46-0.80]; P < .001), and male sex (SHR, 0.73 [95% CI, 0.56-0.94]; P = .02) were associated with lower rates of incident TBI ( Table 2 ). Specifically, White race was associated with elevated incidence of TBI compared with respondents identifying as Black or Hispanic race and ethnicity, and Black race was associated with a 40% decrease in the subdistribution hazard of TBI (SHR, 0.61 [95% CI, 0.44-0.85]; P < .01).
The results of this population-based cohort study assessing the incidence of TBI and factors associated with incident TBI among older adults in the US indicated an extremely high rate of TBI in this group. This finding is consistent with other recent work documenting the scope of the problem of TBI in older adults. 2 As the US population rapidly ages, the epidemiology of TBI is changing, and older adults are the age group most likely to be hospitalized or die from TBI. 2 However, little is known about which older adults are most vulnerable to incident TBI. This information is important from a public health perspective because TBI increases risk of multiple negative outcomes associated with aging, including multisystem (neurologic, cardiovascular, and endocrine) medical comorbidity, 14 loss of functional independence 15 and reduced quality of life. 16 Our work suggests associations of TBI incidence with race and ethnicity, sex, and demographic factors, with highest incidence possibly associated with healthy, active, high-socioeconomic status White women.
Whereas strong evidence for race-based and regional disparities in TBI-related deaths exist, 16 incidence of TBI is harder to quantify because of issues with reporting and differences in public health messaging around treatment seeking, as well as differences in access to care, which vary by demographics and SDOH. Our work used Medicare claims data to identify incident TBI. Although evidence does exist that claims data have excellent criterion validity, 17 it is also true that limitations around confidence in the ascertainment of TBI diagnoses that may be related to access to health care, diagnostic bias, or other factors are unavoidable. Prospective studies featuring a comprehensive diagnostic evaluation are the gold standard in TBI research. However, even existing prospective studies have similar problems with respect to being able to enroll only individuals who seek care and consent to participation, which may limit the diversity of cohorts. Self-reported data may capture more of the true breadth and differential prevalence of TBI diagnoses but also can be unreliable regarding presence, severity, and outcomes of the condition being studied. 18 Given these methodological challenges, documentation and awareness of detection bias in TBI research and care are important for designing future studies and increasing both the accuracy of scientific knowledge and the quality and equity of health care.
We aimed to circumvent some of these methodological challenges in the current work by utilizing a large, nationally representative survey dataset in which most participants agreed to have their responses linked to Medicare claims data. Thus, we were able to examine incidence of TBI adjusted for multiple important demographic and structural variables, such as the ADI, an objective measure of neighborhood resource deprivation that has been associated with a wide array of medical and psychosocial outcomes. 12 Our results also update the existing information available on nationwide TBI incidence (ED visits, hospitalizations, and deaths; more recent data up to 2020 exist on hospital admissions and deaths 16 ) in older adults, which covers up to 2014 19 ; our work covers 2000 through 2018 and also includes both ICD-9 and ICD-10 diagnosis codes. We were also able to show similar results for definitions of TBI with or without hospital treatment (ie, ED code and CT or MRI scan), thereby addressing a limitation of existing older adult TBI surveillance by showing differential incidence of TBI that includes nonhospital settings, such as primary care, urgent care, and specialty care. 20
Our results suggest that almost 13% of US older adults received treatment for an incident TBI experienced during the 18-year study period, and that race, sex, and SDOH factors may be associated with incident TBI. In this diverse sample of older Americans, our also work suggested that an increased rate of TBI was associated with healthy, wealthy, White female individuals. This finding stands in contrast to existing understanding of factors associated with incident TBI, specifically, male sex has long been considered a risk factor for TBI, 21 but our work suggests this may not hold for the older adult population. Prior work documenting that although older men are at higher risk for head injury, women have worse outcomes 22 suggests that our results showing increased incidence of TBI in older women may reflect more immediate negative outcomes leading to higher rates of care seeking. Since the most recent surveillance data available show higher rates of hospitalization and death associated with TBI for older men, as well as higher rates of the most common mechanisms of injury (falls, motor vehicle crashes), 2 , 19 perhaps older women are more likely to experience a less serious head injury and not be admitted to a hospital. Interestingly, however, these results are consistent with prior work from our group suggesting the possibility of a greater prevalence of TBI in older female veterans compared with male veterans in a nationwide Veterans Affairs Health Care System dataset 23 and with a recent study by Yashkin and colleagues 24 using the HRS cohort to show higher incidence of TBI for older women compared with men. Sex differences in geriatric TBI is an area with interesting opportunities for further study.
Many medical conditions are more common in individuals and populations with lower education and financial resources. Our findings suggesting an increased rate of TBI in wealthier respondents, therefore, run counter to expectations and raise important questions about whether this finding reflects a real difference in TBI incidence or, because we were able to study only incident head injuries for which individuals received care, a difference in access to or willingness to seek care. An older study, published in 2007, 25 found that 42% of respondents to an online survey did not seek medical care after experiencing a TBI, and older respondents were less likely to seek care as well as those experiencing a mild TBI and those who were injured at home. Of the 1381 survey respondents with TBI, 584 (42%) did not seek medical care. A similar study found that 50% of adults who experience what they suspect is a TBI do not seek medical care, and most of these injuries were related to falls. 26 Thus, older adults who experience falls, the largest segment of US citizens experiencing incident TBI, are also the least likely to seek care. In addition, lower resourced individuals may be even less likely to seek care due to multiple factors, including but not limited to the racial and ethnic microaggressions that commonly occur in the medical setting. 27 Thus, our estimates of TBI incidence among US older adults are likely to be lower than the true burden of TBI in this population and may not reflect true differential incidence of TBI based on race and ethnicity and other demographics.
In contrast, it is possible that our findings reflect that older adults who are healthier, wealthier, and more active are more able or likely to engage in activities, such as skiing or horseback riding, that carry risk for TBI. An additional alternative explanation is bias (intentional or unintentional) on the part of health care providers who may be more likely to diagnose a TBI in healthy, wealthy, white women who present to the ED after a fall. Indeed, recent work by Tsoy and colleagues 28 using a large representative sample of Medicare beneficiaries in California shows Black-, Asian-, and Hispanic-identified older adults have delayed and less comprehensive dementia diagnoses in claims data. A similar association (ie, reduced comprehensiveness of assessment and diagnosis) may also be true for TBI and may bias our results. However, we preformed sensitivity analyses showing that respondents with a TBI code but no brain imaging or ED visit were similarly distributed across race and ethnic groups compared with the sample as a whole. Other sensitivity analyses showed a similar pattern of results in participants who were admitted to the hospital vs those who were not and in public vs private hospitals, although because of limitations in our available data, the impact of hospital admission and cluster type may still bear further study.
We also identified differences in incident TBI based on cognitive status when first joining the HRS cohort: respondents who had cognitive problems may be less likely to experience incident TBI. Although this association was only found in bivariate analyses, this finding nevertheless runs counter to findings suggesting that individuals with cognitive impairment are more likely to fall and sustain a head injury. It is possible that respondents with cognitive impairment are more limited in their activity and therefore experience less opportunity to fall. But respondents with both milder cognitive impairment and frank dementia had lower incidence of TBI, and those with milder cognitive problems would not be suspected to experience significant activity limitations. Further work is needed to understand the impact of cognitive status on incident TBI over time.
A recent analysis of a large community-based cohort of older adults followed up for more than a mean of 20 years shows that older adults with a previous head injury are much more likely to fall again. 29 Although we did not measure lifetime history of TBI, and respondents with a prevalent TBI at baseline were excluded, there were only 32 respondents excluded for this reason. While our current study does not address the impact of multiple TBIs, our work defining a cohort of respondents experiencing incident TBI allows for future follow-up study of respondents who experienced incident TBI to examine rates of subsequent falls and head injuries and their impact on outcomes.
In our population-based cohort, we showed that older veterans had lower rates of incident TBI during the study period, a finding consistent with our group’s earlier work documenting that nonveterans in the HRS sample were more likely to have a lifetime history of TBI. 30 Although younger veterans are generally understood to be at higher risk of head injury compared with nonveterans because of combat and training-related exposures, the impact of age on TBI incidence for veterans remains unclear. Further research is needed to elucidate TBI rates in older veterans and nonveterans, incident TBI risk for aging veterans, and prevention strategies.
There are some important limitations to our study that may affect the interpretation and generalizability of our results. Receiving neuroimaging is the current standard of care for older adults who present to the ED after a possible TBI. However, this definition of TBI is conservative and may have caused us to miss cases. Still, sensitivity analyses in which we identified TBI cases without this requirement yielded similar results (eTables 1 and 2 in Supplement 1 ). Moreover, most sex, race and ethnicity, and other important SDOH factors, such as wealth and educational level, were based on self-report, and sex was coded as a binary variable only (ie, transgender individuals were not captured), likely excluding some of the true complexity of these variables. We also did not examine TBI severity in the current analysis. Furthermore, our sample had some limitations: we were unable to examine race and ethnicity with the granularity we would have preferred due to small sample sizes. Specifically, work examining incidence of TBI among older Asian and Native American individuals and other minoritized groups is urgently needed, and further research focused on minoritized older adult samples and those that identify their race as other would be helpful and provide insights for TBI treatment planning and prevention as this growing cohort of adults ages. Also, we used ICD-9 and ICD-10 codes in existing medical records to ascertain incident TBI. Although evidence does exist that claims data have excellent criterion validity, 17 this method may result in less accurate categorization of participants compared with studies in which participants were given a comprehensive TBI screening.
This novel cohort study assessing the association of demographics and SDOH with incident TBI in a nationwide sample includes updated data on incidence of TBI, including TBI treated outside a hospital setting, and suggests that older adults may experience differential incidence of TBI. However, it remains unclear to what extent demographics or access to care may affect who accesses treatment for TBI. Further research may lead to clarification of this question as well as to opportunities for both targeted intervention (eg, primary TBI prevention) for groups most at risk and identification and mollification of the most relevant structural and contextual factors (eg, access to care) to reduce incidence of TBI among older adults.
Accepted for Publication: March 29, 2024.
Published: May 31, 2024. doi:10.1001/jamanetworkopen.2024.14223
Open Access: This is an open access article distributed under the terms of the CC-BY License . © 2024 Kornblith E et al. JAMA Network Open .
Corresponding Author: Erica Kornblith, PhD, University of California, San Francisco, San Francisco Veterans Affairs Health Care System, 4150 Clement St, San Francisco, CA 94121 ( [email protected] ).
Author Contributions: Ms Diaz-Ramirez had full access to all of the data in the study and takes responsibility for the integrity of the data and the accuracy of the data analysis.
Concept and design: Kornblith, Diaz-Ramirez, Gardner.
Acquisition, analysis, or interpretation of data: All authors.
Drafting of the manuscript: Kornblith, Yaffe.
Critical review of the manuscript for important intellectual content: All authors.
Statistical analysis: Kornblith, Diaz-Ramirez, Boscardin.
Obtained funding: Kornblith, Yaffe.
Supervision: Boscardin, Gardner.
Conflict of Interest Disclosures: Dr Yaffe reported receiving grants from the US Department of Defense during the conduct of the study. No other disclosures were reported.
Funding/Support: This work was supported by Alzheimer’s Association Research Grant 21-851520 and US Department of Veterans Affairs Career Development Award 1 IK2 RX003073-01A2 (both to Dr Kornblith), grant R35 AG071916 from the National Institute on Aging (NIA) to Dr Yaffe, grant W81XWH-18-PH/TBIRP-LIMBIC I01CX002096 from VA/Department of Defense to Drs Yaffe and Boscardin, and grant R01 NS110944 from the National Institute on Aging to Dr Gardner. Support for Veterans Affairs/Centers for Medicare and Medicaid Services data is provided by the Department of Veterans Affairs, VA Health Services Research and Development Service, VA Information Resource Center (Project Numbers SDR 02-237 and 98-004).
Role of the Funder/Sponsor: The funders had no role in the design and conduct of the study; collection, management, analysis, and interpretation of the data; preparation, review, or approval of the manuscript; and decision to submit the manuscript for publication.
Data Sharing Statement: See Supplement 2 .
Additional Information: This analysis uses data or information from the Harmonized HRS dataset and Codebook, Version C, as of January 2022 developed by the Gateway to Global Aging Data. The development of the Harmonized HRS was funded by the NIA (grants R01 AG030153, RC2 AG036619, and 1R03AG043052).
- Register for email alerts with links to free full-text articles
- Access PDFs of free articles
- Manage your interests
- Save searches and receive search alerts
- Open access
- Published: 26 May 2024
Effect of standardized patient simulation-based pedagogics embedded with lecture in enhancing mental status evaluation cognition among nursing students in Tanzania: A longitudinal quasi-experimental study
- Violeth E. Singano 1 ,
- Walter C. Millanzi 1 &
- Fabiola Moshi 1
BMC Medical Education volume 24 , Article number: 577 ( 2024 ) Cite this article
758 Accesses
1 Altmetric
Metrics details
Nurses around the world are expected to demonstrate competence in performing mental status evaluation. However, there is a gap between what is taught in class and what is practiced for patients with mental illness among nursing students during MSE performance. It is believed that proper pedagogics may enhance this competence. A longitudinal controlled quasi-experimental study design was used to evaluate the effect of using standardized patient simulation-based pedagogics embedded with a lecture in enhancing mental status evaluation cognition among nursing students in Tanzania.
A longitudinal controlled quasi-experimental study design with pre-and post-test design studied 311 nursing students in the Tanga and Dodoma regions. The Standardized Patient Simulation-Based Pedagogy (SPSP) package was administered to the intervention group. Both groups underwent baseline and post-test assessments using a Interviewer-adminstered structured questionnaire as the primary data collection tool, which was benchmarked from previous studies. The effectiveness of the intervention was assessed using both descriptive and inferential statistics, specifically the Difference in Difference linear mixed model, and the t-test was carried out using IBM Statistical Package for Social Science (SPSS) software, version 25.
The participant’s mean age was 21 years ± 2.69 with 68.81% of the students being female. Following the training Students in the intervention group demonstrated a significant increase in MSE cognition post-test, with an overall mean score of ( M ± SD = 22.15 ± 4.42;p = < 0.0001), against ( M ± SD = 16.52 ± 6.30) for the control group.
A significant difference exists in the levels of cognition, among nursing students exposed to Mental Status Evaluation (MSE) materials through Standardized Patient Simulation-Based Pedagogy (SPSP) embeded with lectures. When MSE materials are delivered through SPSP along with lectures, the results are significantly superior to using lectures pedagogy alone.
Peer Review reports
Introduction
The most prominent and dominant strategy used to diagnose a mental health problem in a clinical setting is Mental status evaluation MSE [ 1 ]. The type of diagnosis is based on the chief signs and symptoms, and treatment is agreed upon accordingly. The MSE is data received using information gathered by the psychiatrist, clinician, and nurse from direct inquiries and passive assessment during the interview to determine the patient’s actual mental state. The purpose of evaluating the range of mental functions and behaviors at a particular moment gives crucial information for diagnosis and determining the disease’s severity, trajectory, and responsiveness to treatment. Countries such as the U.S. practice mental status evaluation as a diagnostic tool for the diagnosis of mental illness, and the rest of the world uses similar cataloging from the American Psychiatric Association [ 2 ]. Nursing students are expected to demonstrate competence in performing mental status evaluation. However, there is a gap between what is taught in class and what is practiced for patients with mental illness among nursing students during MSE performance. Classroom and clinical pedagogies, such as lecture role play and demonstrations, are implemented to facilitate MSE competencies among nursing students [ 3 ]. Scholars have reported conventional pedagogics such as lectures, demonstrations, and portfolios to be dominantly used in facilitating MSE learning among nursing students [ 4 ]. The predominant use of conventional pedagogy has been linked to anxiety, frustration, stress, and fear in nursing students when they encounter mentally ill patients during their clinical rotation [ 5 , 6 ].
Educators and health workers argue that these abilities are inadequate to provide evidence-based mental health nursing care. They may thus lead to prolonged hospital stays, remissions, drug-resistant and long-term adverse drug effects in mentally ill patients [ 7 ]. The study was conducted on the practices of the nursing students throughout clinical teaching in mental health hospitals and stated that there is a mismatch between theory and practice, insufficient instruction approaches, and an absence of person-mode nurses and coaching staff to facilitate MSE learning for nursing students appropriately [ 8 ]. A study by [ 9 ] on designing instruction to teach MSE reported that nursing students who taught MSE using conventional clinical pedagogics demonstrated inabilities to diagnose patient conditions plan patient care, prevent injury to patients and others, and provide specific management. Moreover, findings from [ 10 ] on the discrepancy between what occurs internally and externally in student mental health nursing showed a significant mismatch between theoretical mental health content knowledge and practical skills when nursing students are developed using conventional clinical pedagogy.
International and national organizations respond to Sustainable Development Goal number four, target number four (SDG), by emphasizing training institutions and teaching hospitals to adopt and implement innovative pedagogics in facilitating MSE learning for learners [ 11 ]. The incorporation of standardized patient simulation-based pedagogy (SPSP) as suggested by other scholars [ 12 , 13 ], appears to demonstrate academic potential, such as enhancing learners’ cognitive and empowering them with self-efficacy when performing MSE. Simulation offers a chance to make cases more challenging without endangering clients, families, or students, as nursing students in clinical practice are frequently tasked with working with amicable and amenable clients and families [ 14 ]. The SP comes to life in front of the learners in the state-of-the-art lab. Students can practice their diagnosis and develop therapeutic clinical expertise in the laboratories, which are offered in a friendly environment [ 15 ].
Similarly, a pilot study using a mixed method was done in Baccalaureate nursing education in the US to examine the use of SPSP compared with the traditional hours used for learning mental health, showing nursing students who received SPSP showed increased confidence and cognition about mental health by 25% compared to traditional hours [ 16 ]. Good MSE cognition among nursing students may ultimately lead to timely and appropriate diagnosis and, thus, positive mental health outcomes for mental illness patients. While the adoption and implementation of SPSP are popular in other countries, published scholarly works are scarce about it in clinical nursing education for MSE cognition among nursing students in Tanzania. It may be time to invest in research about the effect of SPSP embedded with lecture on enhancing MSE cognition among nursing students in this country.
Method and materials
The methodology of this study complied with national and international research ethics. Moreover, the study was conducted by the University of Dodoma’s institutional postgraduate guidelines and standards.
The purpose of the current research was to evaluate the impact of standardized patient simulation-based pedagogy (SPSP) linked with lectures on mental status evaluation cognition among nursing students in Tanzania. To accomplish this, a longitudinal quasi-experimental study design was implemented.
Study population
The target demographic was made up of students enrolled in diploma nursing programs in the regions of Tanga and Dodoma. The study involved 311, diploma nursing students (the age between 16 and 32 years). The reason for selecting middle college nursing students is that they constitute a big population of the future nursing force, which is expected to deliver nursing mental health services in the peripheral community. This study believed that skills provided to the nursing students were beneficial for them since it is targeted to be delivered to the large population for timely diagnosis of mental illness disorders, which most of the population are living in remote areas with inadequate mental health services.
Sampling procedure and technique
The purposive sampling technique was used to sample nursing schools from two regions, 5 nursing schools from Dodoma Central zone and 2 nursing schools from Tanga in the Northern zone, where 311 nursing students sampled and (109) were in the intervention group and (202) nursing students were in the control group then the proportional calculation was done to get the required number of participant’s in each nursing school whereby the simple random sampling were done to select the requires number of participants in each class. After being explained the purpose, and benefit of this study, nursing students who were willing to participate in this study and signed the written informed consent form were included in this study.
Proportional for the intervention group
Whereby n = Total number of sample sizes for each group whether interventional Control croup.
N = Total number of students in both classes.
Nh = Total number of students in each class.
Two nursing schools from Tanga were Tanga College of Health and Allied Sciences (TACOHAS) with a total number of students of 97, college A, and Korogwe Nursing Training Center (KNTC) with a total number of students of 71, college B.
The proportion for college A;
Nh = 97 and 71.
nA = (109/168) 97 = 63.
Therefore, the number of participants in College A was 63.
nB = (109/168) 71 = 46.
Therefore, the number of participants in College B was 46 (making a total sample size of 109 for the intervention group).
The proportion for the control group in the Dodoma region
Five colleges offering Diplomas in nursing from Dodoma are DECCA College of Health and Allied Sciences (DECCA COHAS) with a total number of nursing students 60; Dodoma Institute of Health and Allied Sciences (DIHAS) with a total number of nursing students 81; Saint John’s University with a total number of nursing students of 45; Mvumi Institute of Health and Allied Sciences (MIHAS) with a total number of students of 19; Kondoa School of Nursing with a total number of 47;
Total number of students = 252.
nC = (217/252) 60 = 52.
nD = (217/252) 81 = 70.
nE = (217/252) 45 = 39.
nF = (217/252) 19 = 16.
nG = (217/252) 47 = 40.
Which makes a total sample size of 217.
The required number of participants was obtained through proportional calculation. To select participants, a simple random sampling method was employed by listing the names of students on pieces of paper. The selection was made by choosing participants for every 10th number on the list until the required number was reached, Fig. 1 illustrates this. To prevent contamination, interventional and control groups were assigned to different regions, and participants were not informed about the other study sites. Additionally, the researcher’s assistant was kept unaware of whether participants were part of the control or intervention groups.
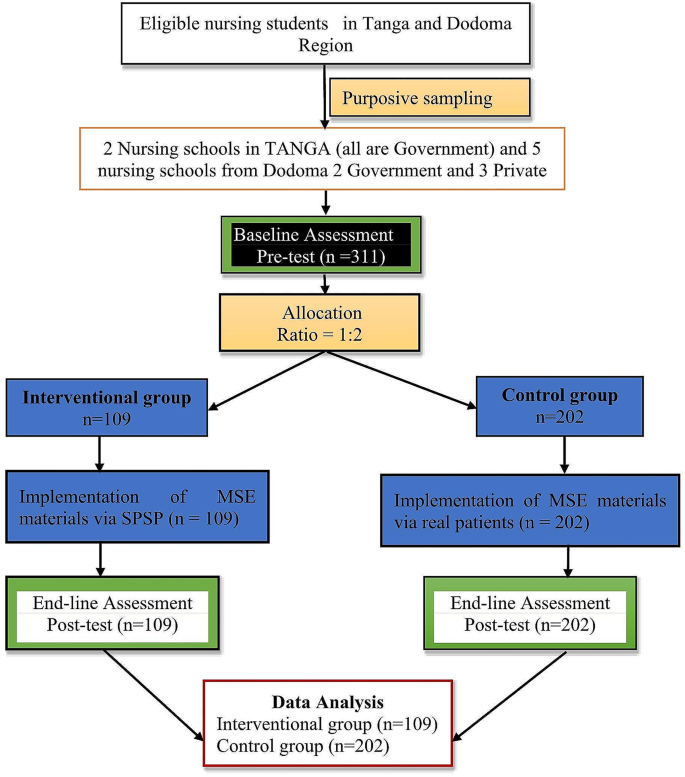
Study design flow diagram Source: Study plan (2022)
Sample size estimation
The sample size n for this study was determined using WinPepi software version 11.65 [ 17 ]. Findings from the study on simulation-based learning in psychiatry for undergraduates at the University of Zimbabwe Medical School [ 18 ] showed a pre-session mean score of 15.90 and a post-session mean score of 20.05. With a sample size of the effect size of 2, a significance of 95% confidence interval of 5% significance level, and a power of the study of 80%, the ratio of the sample size B: A is a ratio of 1:2.As shown in Fig. 2 , therefore, sample size (n) = 326 Participants (109 in A and 217 in B). This program has been used by different scholars and reported to have statistical validity and reliability in studies [ 19 , 20 , 21 ].
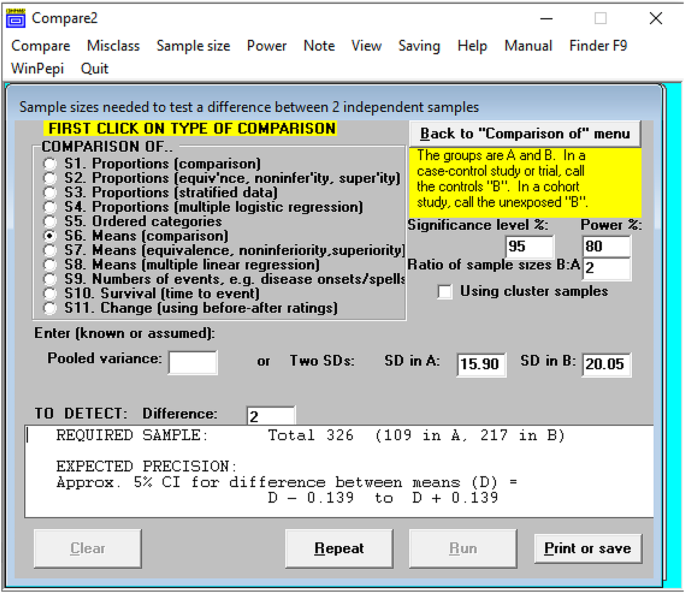
Source: Study plan (2022)
WinPepi program for sample size calculation.
Data collection procedure
After obtaining the necessary permissions, an available classroom was designated for the study. The Principal Investigator then introduced the study’s objectives to the participants. Once informed consent was obtained, the students were seated in separate chairs to prevent any potential copying or sharing of responses. Data collection was carried out by interviewer-administered structured questionnaire, with the trained researcher’s trainer. The Principal Investigator was present to provide clarifications when necessary. Once completed, the questionnaires were collected by the trained researcher trainer and securely stored in a locked cupboard by the Principal Investigator.
Data collection tool
This study employed a standardized structured questionnaire benchmarked from previous studies [ 22 ] with 33 items modified from a literature review. The adopted questionnaire for cognition has a test re-test approach which is used to assess the dependability of the study instrument (alpha reliability = 0.770, test-re-test reliability = 0.880). Therefore, the questionnaires used for data collection in this study consisted of two parts: Part “A” collected demographic characteristics profiles of the study participants, Part “B” assessed participants’ MSE cognition (28 items),
Nursing professionals were given the first draft of the instrument, and they were asked to reply to the open-ended questions, propose any changes they believed should be made, and suggest any additional items they thought should be added. Items having a relevancy score of less than 0.7 were removed, and adjustments to the wording were made to the expert’s suggestions. For face and construct validity, a preliminary draft was examined by a second nursing expert from a nursing faculty. Students in their second year of nursing ( n = 33) provided comments on the tool’s usefulness. After comments from the experts and the nursing students, there were 5 questions from cognition questions that lacked face validity or content validity were removed. A total of 28 questions on cognition remain.
Reliability
To verify the tool’s capabilities for producing the expected results, a pilot study of 10% of the sample size was conducted. The statistical program for the Social Solution (SPSS) software version 25 was used to scale the results from the pilot study. The overall Cronbach’s alpha of cognition was 0.736. As recommended by previous scholars, a Cronbach’s Alpha (α) of ≥ 0.7 was considered a significantly reliable tool for the actual field data collection.
Variable measurement
A structured questionnaire benchmarked from previous studies was used to measure the variable pre- and post-intervention to test cognition. Cognition of MSE was measured using multiple-choice open-ended questions for baseline assessment and immediate 1-week post-intervention, the test had 28 questions for assessing MSE cognition with three domains including (2-questions) on the concept of MSE, (4-questions) on the content of MSE and (22-questions) on MSE implementation. Scores per each correct response ranged from ֞ 0 ֞ point for a wrong response to.
֞ 1 ֞ point for a correct response, and the highest score of MSE cognition were computed as a sum of each item, then cognition was explained as ֞ adequate cognition ֞ for participants who scored 50% and above, and the lowest score was explained as ֞ inadequate cognition ֞ for participants who scored < 50%. The domain of MSE cognition was also measured separately based on the total score that the nursing students scored out of the total score assigned to each domain then, the mean difference between the two groups was measured using a paired t-test.
The SPSP intervention
Table 1 shows the prescription of the intervention training. The intervention took 4 weeks to facilitate both MSE theory and practice. Topics of the MSE materials included a definition of MSE and steps in performing MSE to identify a client with mental illness. Two sessions were conducted in a week, lasting 120 min each. They were facilitated during the morning hours and were negotiated with the principles of the respective colleges. Two sessions were implemented to cover the MSE theoretical and practical sessions, respectively. Both English and Swahili were used alternatively at the convenience of research trainers and participants. The intervention group learned MSE using an SPSP embedded with a lecture compared to the control group, which learned the same MSE materials using lectures and real patient pedagogies. The rationale behind choosing these two approaches was to assess the impact of the intervention on two groups : those who were exposed to the MSE materials via SPSP embedded with lecture and went on to the skills laboratory to interview the SP who is trained and coached to portray sign and symptoms of mental illness, and those who were exposed through MSE lecture methods and actual patients in general medical wards without symptoms of mental illness. Upon completion of the data analysis, a comparison was made between the subjects who were exposed to the MSE materials through SP and the subjects who were exposed to the actual patient who did not exhibit any symptoms of mental illness. Before the intervention, participants in both groups were matched in their sociodemographic profiles, such as age, sex, education level, entry qualification, and marital status, to ensure their similarities before intervention. Pre-tests were then administered to participants to establish their baseline MSE cognition.
The MSE intervention focused on the area where nursing students struggled with technique questions to assess and determine if the patient exhibited the characteristics of hallucination, illusion, delusion, derealization, depersonalization, and insight, terms that can used commonly. To help nursing students understand that what the patient demonstrated or explained reflected the question asked, that failing to probe precisely what the patient was experiencing may lead to the wrong MSE conclusion, and that the SP was trained to answer the questions asked to reflect the reality of what the patient was suffering from, how these questions were asked was given more consideration.
Recruitment and training of SP and research trainers
Training of sp.
Professional actors who know mental health, work at a mental health facility, or have a family relative who has a mental illness, or encountered a person with a mental health problem and who were willing to help the student learn and be able to retain the script of the scenario was recruited as SP. Principles for preparing SPSP were found in the association of Standardized patient education standards, and practice [ 23 ] was applied to ensure SPSP is a safe work environment and training for role portray and feedback to students during debriefing. The agreed-upon formula, primary goals, duties, materials, and structure of the mental health scenario were covered during a weekly 2-hour training class. This class included instruction on scenario reading, guidance in verbal interaction techniques, input on the scenario, debriefing strategies, and discussions on how to reduce learner anxiety during the simulation experiences.
Before the rehearsal, each SP was provided with a scenario that outlined the signs and symptoms of a mentally ill patient. This scenario encompassed the various domains of MSE, with specific questions and answers to which the SP was required to respond in each domain. Emphasis was placed on the domains that nursing students commonly encountered difficulties with during mental status evaluation and clinical practice. For instance, they were trained on how to assess mood and affect, illusions and hallucinations, depersonalization and derealization, orientation, memory, intelligence, insight, and judgment. However, not all SPs were required to portray all domains of symptoms. This is because it’s uncommon for one patient to exhibit all possible symptoms simultaneously. Additionally, having all the symptoms portrayed by the SPs might lead to an exaggeration of the true symptoms of a real patient.
The SPs were thoroughly rehearsed using scenario scripts, and the research team, mental health experts, and nurse tutors who specialize in teaching mental health subjects reviewed their performances. The portrayal of the client’s character was observed, and the experts addressed any areas that required clarification or correction. Out of the four SPs who were willing to participate in this study, two were able to effectively portray the signs and symptoms of a mentally ill patient and were selected for the actual fieldwork implementation.
Implementation of MSE materials in an SPSP
Nursing students were assigned to the interventional group (typical education plus SPSP), which first completed both pre-tests before getting intervention. The MSE lecture method was taught to the students on the first day of the training by the researcher trainers focused on the definition of MSE, steps on performing MSE, and how to perform MSE to identify patient with mental illness disordes. The nursing students were then introduced to the simulation on the following day, and they were informed that the simulation would take place in a skills laboratory, nursing students were invited to the prepared skills laboratory, Students were seated on the semi-cycle sitting plan for easy visualization of the simulation, and then SP together with the nursing student who acted as a nurse were seated at the center and the researcher trainer was there to provide any assistance needed by students during simulation. Interventional students participated in two-hour simulation sessions, with a break in between to prevent student fatigue. Each group consisted of 5 to 8 students. Following the simulation pre-briefing on the scenario was done by the researcher trainer to make sure that they understood the whole simulation process, and SPSP orientation was included in each simulation. Thereafter each nursing student was provided with a checklist of the MSE categories to make a follow-up to what had been assessed during the simulation. SP was brought to the skills laboratory by his relatives dressed in dirty loose- jogging tracksuits and his hair was messy with a history of abnormal behavior characterized by abusive language, over-talkative, threatening his mother and others, reduced sleep during the night, grandiose delusion, persecutory delusion and hearing unknown voices, one nursing student was chosen from the class for each simulation to play the nurse role on how to perform MSE to the patient with abnormal behavior by using the technique and procedures learned during the lecture methods, and the other was designated as an observer. The positions of nurse and observer were available to all students. The duration of each simulation was 15 min, followed by a 10-minutes structural debriefing.
Evaluation of MSE materials in an SPSP
The three-part debrief paradigm, which entails defusing, identifying, and developing [ 24 ], served as the framework for the debriefing sessions. The trained researcher trainer was offered SPSP one-on-one organized time for debriefing immediately following each simulation exercise to examine psychological problems in role acting and how students’ emotional states influence their conduct and communication. To encourage cooperative learning, SP and nursing student observers discussed what they had noticed about communication and evaluation methods. Students playing nurses’ roles were encouraged to speak about their experiences. SP provided feedback via formative and summative methods that involved face-to-face engagement. The trained researcher trainer commented on the student’s responses.
Data analysis
The IBM statistical package of Social Science (SPSS) computer software program version 25 was used to analyze data. The frequency distribution table was used for data cleaning to ensure that all data was recorded accurately. To go through the data, labels had to be applied, value had to be checked and re-assigned for the open-ended questions, noise had to be checked, and the erroneous spellings verification for nominal response had to be rewritten. Additionally, the baseline and end-line data were combined and added during the procedure of the calculation of the important outcome A descriptive and inferential analysis was conducted based on the study’s goal. To calculate the frequencies and the percentage of each participant’s distribution between the two groups, a descriptive analysis was performed to examine participant characteristics Bar chats, mean values, and averages as well as tabular data, were all included in the descriptive evaluation. The pre-post mean score, and post-test mean score, for both the interventional and control groups were compared using the independent samples t-test. To evaluate the effect of the SPSP embedded with a lecture on MSE cognition, among nursing students from baseline to end line, the inferential analysis involved the differences in difference (DID) analysis using a Linear mixed model. A 95% Confidence interval set at a 5% (≤ 0.05) significance level was used to reject the null hypothesis. Results from the parameter multiple measurements were taken into consideration by models, and the groups were considered as fixed influences.
Difference–in–difference (DID) analysis for inferential analysis
By eliminating the confounding variables, difference-in-difference (D-I-D) analysis enables the comparison of changes over time in the results between interventions. The DID design examines the difference between the treatment groups by measuring the change in results between two-time intervals (pre and post) for the intervention and control groups, then subtracting one from another. In this research, the impact of the intervention on cognition change score was evaluated using difference-in-difference analysis using a linear mixed method. The outcomes of the variables’ repeated measurements were taken into account by the model. Interventions were regarded as having fixed effects in this analysis. The following formula is used to present the general fixed-effect DID mixed model
Time is an empty variable for the period, denoted as 1 when the outcome analysis was completed in the final stage and 0 for benchmark evaluation. Here, Y it is the final result for participant i at time t . This variable acts as a substitute variable for the intervention group. The combined parameter Time* Treatment is the relationship between time and the intervention, this ε it is also the amount of error for the participant i outcome measurements at the time t. The value of the intercept in the equation given parameter β 0 , represents the mean outcome value for the group receiving the intervention at the baseline measurement. β 1 is the change in an intervention group’s mean outcome variable between the baseline and the end line Parameter β 2 represents the variation in the mean result variable across individual interventions. The estimate and inference of the difference-in-difference between the two groups are provided by the coefficient of the interaction between groups.
Social demographic characteristics among nursing students
Distribution of the similarity of demographic characteristics among nursing students between intervention and control groups at the baseline. Table 2 reported that among the participants ( n = 311), who indicated their age 34.86% ( n = 38) for intervention and 54.46% ( n = 110) for control were ranged between 21 and 32 years old with their age distribution ( p = 0.0510) between groups, for those who indicated their gender 57.80% ( n = 63) were female in intervention group and 68.81% (= 139) were from control group with ( p = 0.0521) of their gender distribution between groups. However, for those who are single were many in both groups compared to those who are married 95.41% ( n = 104) for intervention and 97.45% ( n = 191) with ( p = 0.3373*) of their distribution between marital status. The distribution of form four education entrance was higher compared to others with 76.15%( n = 83) for control and 59.90% ( n = 121) for intervention with ( p = 0.0540) of their education level distribution between groups. Among all participants, 99.07% ( n = 107) in intervention and 84.69% ( n = 166) in control showed interest in nursing with ( p = 0.0601) of their interest distribution between groups.
The effect of standardized patient simulation-based pedagogics embedded with lecture on MSE cognition among nursing students in Tanzania
As shown in Table 3 . below, the cognition pretest score of the concepts of MSE in the intervention group was ( M ± SD = 0.87 ± 0.84) and the control group was M ± SD = 0.81 ± 0.79, p = 0.5341, the post-test results were M ± SD = 1.33 ± 0.73 for the intervention group, and the control was M ± SD = 1.17 ± 0.76; p = 0.0785, so there is a marked change of MSE content from both groups. However, the MSE content on the baseline was ( M ± SD = 2.63 ± 1.06) for the intervention and ( M ± SD = 2.42 ± 0.95; p = 0.0700) for the control group, the end line score for the intervention group was ( M ± SD = 3.39 ± 0.80) and control group was ( M ± SD = 2.85 ± 0.98; P < 0.0001), baseline findings for implementation of MSE Intervention group scored ( M ± SD = 11.4 ± 3.00) and control group scored ( M ± SD = 9.85 ± 3.96; p = 0.0076 for the pre-test intervention group scored ( M ± SD = 17.42 ± 3.90) control group scored ( M ± SD = 13.29 ± 4.58; p = < 0.0001).
The overall pretest was (M = 13.05, SD = 4.63) for intervention group, ( M ± SD = 12.11 ± 5.21), p = 0.1189 from control group posttest cognition was ( M ± SD = 22.15 ± 4.42) for intervention and ( M ± SD = 16.52 ± 6.30; p = < 0.0001) for control group. There is a significant change in cognition for the intervention and control group for the post-test. According to the substantial mean changes between the pre-test and post-test scores in all categories (Concepts, Content, Implementation, and Overall cognition) for the intervention group, it appears that the intervention has had a significant change effect on the cognition of nursing students in general. A small amount of progress is also seen in the control group, but overall, the intervention group exhibits more development.
Findings of nursing student’s cognition mean score between baseline and end line ( n = 311)
The finding shows that the mean cognition increased from base to end line between the interventional group and the control group. As shown in Fig. 3 mean score of nursing student cognition increased by ( M ± SD = 22.15 ± 4.42) for the intervention group, whereas cognition in the control group increased by ( M ± SD = 16.52 ± 6.30). This implies that the change in cognition mean score from baseline to end line was higher in the intervention group than in the control group.
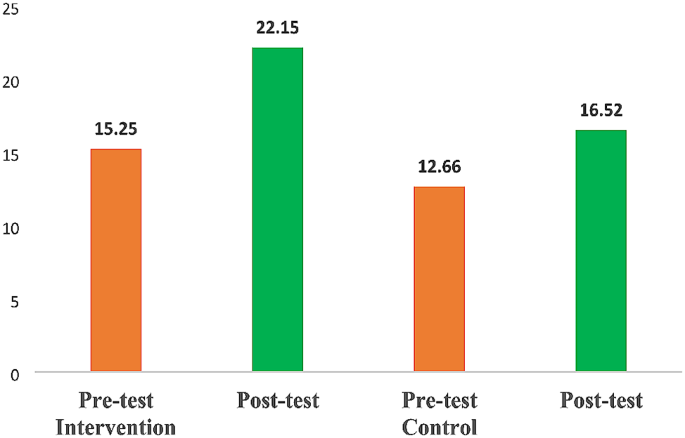
Source: Field data (2023)
Findings of nursing student’s cognition mean score between baseline and end-line.
DID analysis for MSE cognition among nursing students in Tanzania
The fitted model results are presented in Table 4 . The findings indicate that there was a significant improvement in cognition from the baseline to the end line, as indicated by a p-value of < 0.0001. The coefficient for the Difference-in-Differences (D-I-D) analysis, comparing the intervention group to the control arm, was 4.6950. This suggests that the change in cognition from baseline to end line was significantly higher in the intervention group compared to the control group.
The study’s results establish a strong correlation between the impact of Standardized Patient Simulation-Based Pedagogy embedded with lecture (SPSP) and the cognition scores of nursing students. In the final analysis, nursing students exposed to standardized patient-based simulation materials displayed a significantly higher level of cognition regarding Mental Status Evaluation (MSE) when compared to the control group. This outcome aligns with a study conducted at university of Queens Canada on the impact of SPSP in psychiatric nursing on mental health education, which demonstrated a significant cognition improvement [ 25 ].Additionally, nursing students who interacted with standardized patients (SP) during interviews were able to relate what they had learned from the designed teaching pedagogy during the simulation, the simulation’s method of delivery provided nursing students with ample time to interview the SP. The study done by [ 26 ] on the use of SPS to train new nurses supporting this findings that new nursing students cognition improved higher compared to control group whose not exposed to the SPS.
During simulation process in case where clarification was required or certain behaviors were not well understood by the learners, students could request the SP to repeat the behavior, but also the skills of the trained researcher on the delivering of the content contributed to the increasing nursing students’ cognition. This aligns with the findings of a study conducted in Australia to explore the effect of SPSP on mental health education. The study reported that students who used SPSP for teaching scored higher, felt safer, and experienced reduced anxiety levels during examinations, as demonstrated in research [ 27 , 28 ]. Given the challenge of exposing nursing students directly to realistic patients without prior practice in a skills laboratory, exposing nursing students in SPS demonstrated a significant higher level of cognition this changes are due to the fact that students were able to control the learning environment during the simulation, a similar study was conducted in Baccalaureate nursing education in the US to examine the use of SPSP compared to traditional hours dedicated to learning mental health. The study found that student nurses who received SPSP demonstrated a 25% increase in confidence and cognition about mental health compared to traditional instructional hours, as highlighted in the research by [ 16 ]. Observing others successfully perform Mental Status Evaluation (MSE) using Standardized Patients (SP) and receiving encouraging feedback from colleagues and facilitators played a pivotal role in boosting nursing students’ cognition. This research aligns with study done to compare SPS versus mannequins in mental health simulation, which posits that cognition is influenced by positive simulation modalities, guidance through observational learning, approval, and inspiration [ 29 ]. The training program encouraged students to focus on acquiring the necessary knowledge, and the briefing provided during simulation on how MSE should be conducted contributed to building students’ MSE cognition.
However, This outcome aligns with a study conducted by [ 30 ] on the use of SPSP in psychiatric nursing, which demonstrated a substantial improvement in nursing students’ understanding compared to traditional teaching methods. Specifically, the study reported an 80% increase in cognition acquisition when utilizing SPSP as opposed to conventional approaches. These findings are consistent with a study conducted by [ 31 ], which implemented various active teaching methods during simulation to enhance nursing students’ knowledge. Additionally, the manner in which SPs were trained to accurately portray signs and symptoms of patients was instrumental in this process. Furthermore, the design of the SP teaching materials fostered collaboration among nursing students, encouraging each student to actively participate in classroom activities. This collaborative approach played a vital role in enhancing their MSE cognition. These findings are consistent with the work of several scholars, such as [ 32 ] and [ 33 ], who have emphasized the significant contribution of peer-to-peer education in boosting nursing students’ sense of cognition.
The findings of this study suggested that using SPSP embedded with lectures will help increase nursing student MSE cognition among nursing students in Tanzania. This is because there is no skills laboratory for nursing students to practice before encountering a real patient, and the practicum sites for nursing students to practice mental health services, especially MSE, are few. For this reason, nursing students are required to travel far from their institution to practice. This is contrary to the Tanzania curriculum, which states that nursing students should practice in the skills laboratory before going to the clinical. Standardized patients in teaching mental status evaluation is a useful pedagogical method and increases the cognition of the nursing students, while it’s difficult to use real patients because it may cause inconveniences to the patient and the learner. MSE is challenging to assess because it cannot be directly assessed as a physical disease. Nursing students require the technique of performing MSE to get the real symptom from the patient.
Strength of the study
To improve the performance of nursing students, the study addressed clinical pedagogical deficiencies in clinical mental health nursing education on Mental status evaluation to better manage and diagnose people with mental diseases promptly. However, the study has managed to use a control group and enough sample to increase the validity of results and power of the study on the effect of SPSP and their outcome.
Suggestion for further studies
Future researchers should include this training among nursing students at higher institutions. Future studies should address the problems with the study’s design and expand on some of the topics that were not fully explored in this one. Based on the study’s shortcomings, there were several implications that another study might take into account.
Limitations of the study
The generalization of the study findings among nursing students in Tanzania will be difficult since the calculated sample size was 326 and the participants who were willing to participate in this study during actual data collection was 311, even though the response rate was 95%. The results of the study cannot be used to determine whether they apply to all Tanzanian nursing students this is because study participants were the nursing students from the middle college who are pursuing diplomas in nursing from Dodoma and Tanga Regions, and excluded the university students who are also learning MSE and are expected to deliver MSE service within the community, and they also suffer from a lack of stimulation of MSE in a skill-based environment. Consequently, results must be examined and analyzed carefully while considering them. The study employed purposive sampling that cannot tell exactly that the selected participants present the sample of nursing students in Tanzania. However, the Study did not show the separate effect of lecture as embedded in the SPSP training materials and how much contributed to the outcome of interest.
Data availability
The datasets that are used or analyzed in the current study are available from the corresponding author on reasonable request via [email protected] or [email protected].
Abbreviations
Mental Status Evaluation
- Standardized patient
Statistical Package of Social Sciences
Standardized patient Simulation Pedagogics
University of Dodoma
United states
World Health Organization
Rocha Neto HG, Estellita-Lins CE, Lessa JLM, Cavalcanti MT. Mental State Examination and Its Procedures—Narrative Review of Brazilian Descriptive Psychopathology. Front Psychiatry [Internet]. 2019;10. https://www.frontiersin.org/article/ https://doi.org/10.3389/fpsyt.2019.00077/full .
Ma F. Diagnostic and Statistical Manual of Mental Disorders-5 (DSM-5). In: Encyclopedia of Gerontology and Population Aging [Internet]. Cham: Springer International Publishing; 2021. pp. 1414–25. https://link.springer.com/ https://doi.org/10.1007/978-3-030-22009-9_419 .
García-Mayor S, Quemada-González C, León-Campos Á, Kaknani-Uttumchandani S, Gutiérrez-Rodríguez L, del Mar, Carmona-Segovia A et al. Nursing students’ perceptions on the use of clinical simulation in psychiatric and mental health nursing by means of objective structured clinical examination (OSCE). Nurse Educ Today. 2021;100.
Thomas SP. Thoughts about Teaching Psychiatric-Mental Health Nursing. Issues Ment Health Nurs [Internet]. 2019;40(11):931–931. https://www.tandfonline.com/doi/full/10.1080/01612840.2019.1653729 .
Abraham SP, Cramer C, Palleschi H. Walking on Eggshells: Addressing Nursing Students’ Fear of the Psychiatric Clinical Setting. J Psychosoc Nurs Ment Health Serv [Internet]. 2018;56(9):5–8. https://journals.healio.com/doi/ https://doi.org/10.3928/02793695-20180322-01 .
Wedgeworth ML, Ford CD, Tice JR. I’m scared: Journaling Uncovers Student Perceptions Prior to a Psychiatric Clinical Rotation. J Am Psychiatr Nurses Assoc [Internet]. 2020;26(2):189–95. http://journals.sagepub.com/doi/ https://doi.org/10.1177/1078390319844002 .
Moges S, Belete T, Mekonen T, Menberu M. Lifetime relapse and its associated factors among people with schizophrenia spectrum disorders who are on follow up at Comprehensive Specialized Hospitals in Amhara region, Ethiopia: a cross-sectional study. Int J Ment Health Syst [Internet]. 2021;15(1):42. https://ijmhs.biomedcentral.com/articles/ https://doi.org/10.1186/s13033-021-00464-0 .
Roy K, Nagalla M, Riba MB. Education in Psychiatry for Medical Specialists. In 2019. pp. 119–40. http://link.springer.com/ https://doi.org/10.1007/978-981-10-2350-7_8 .
Lenouvel E, Chivu C, Mattson J, Young JQ, Klöppel S, Pinilla S. Instructional Design Strategies for Teaching the Mental Status Examination and Psychiatric Interview: a Scoping Review. Acad Psychiatry [Internet]. 2022; https://link.springer.com/ https://doi.org/10.1007/s40596-022-01617-0 .
Marszalek MA, Faksvåg H, Frøystadvåg TH, Ness O, Veseth M. A mismatch between what is happening on the inside and going on, on the outside: a qualitative study of therapists’ perspectives on student mental health. Int J Ment Health Syst [Internet]. 2021;15(1):87. https://ijmhs.biomedcentral.com/articles/ https://doi.org/10.1186/s13033-021-00508-5 .
Silva M, De, Roland J. Mental Health Sustainable Dev. 2014;1–32.
Johnson KV, Scott AL, Franks L. Impact of standardized patients on first semester nursing students Self-Confidence, satisfaction, and communication in a simulated clinical case. SAGE Open Nurs. 2020;6(June).
Witt MA, McGaughan K, Smaldone A. Standardized Patient Simulation Experiences Improves Mental Health Assessment and Communication. Clin Simul Nurs [Internet]. 2018;23:16–20. https://doi.org/10.1016/j.ecns.2018.08.002 .
Oudshoorn A, Sinclair B. Using Unfolding Simulations to Teach Mental Health Concepts in Undergraduate Nursing Education. Clin Simul Nurs [Internet]. 2015;11(9):396–401. https://linkinghub.elsevier.com/retrieve/pii/S187613991500050X .
Edward K, Hercelinskyj J, Warelow P, Munro I. Simulation to Practice: Developing Nursing Skills in Mental Health–An Australian Perspective. Int Electron J Health Educ [Internet]. 2007;10(February 2014):60–4. http://search.ebscohost.com/login.aspx?direct=true&db=eric&AN=EJ794196&login.asp&site=ehost-live%5Cnhttp://www.aahperd.org/iejhe/template.cfm?template=currentIssue.cfm#volume10
Soccio DA. Effectiveness of Mental Health Simulation in Replacing Traditional Clinical Hours in Baccalaureate Nursing Education. J Psychosoc Nurs Ment Health Serv [Internet]. 2017;55(11):36–43. https://journals.healio.com/doi/ https://doi.org/10.3928/02793695-20170905-03 .
Abramson JH. WINPEPI updated: Computer programs for epidemiologists, and their teaching potential. Epidemiol Perspect Innov [Internet]. 2011;8(1):1. http://www.epi-perspectives.com/content/8/1/1 .
Piette A, Service NH, Muchirahondo F, Mangezi W, Cowan FM. ‘ Simulation-based learning in psychiatry for undergraduates at the University of Zimbabwe medical school. ’ 2015;(March).
Ganz JB, Earles-Vollrath TL, Heath AK, Parker RI, Rispoli MJ, Duran JB. A meta-analysis of single case research studies on aided augmentative and alternative communication systems with individuals with autism spectrum disorders. J Autism Dev Disord. 2012;42(1):60–74.
Article Google Scholar
Millanzi WC, Kibusi SM. Exploring the effect of problem based facilitatory teaching approach on motivation to learn: a quasi-experimental study of nursing students in Tanzania. BMC Nurs [Internet]. 2021;20(1):3. https://bmcnurs.biomedcentral.com/articles/ https://doi.org/10.1186/s12912-020-00509-8 .
Parker RI, Vannest KJ, Davis JL. Effect size in single-case research: a review of nine nonoverlap techniques. Behav Modif. 2011;35(4):303–22.
Gabriel A, Violato C. The development of a knowledge test of depression and its treatment for patients suffering from non-psychotic depression: a psychometric assessment. BMC Psychiatry [Internet]. 2009;9(1):56. https://bmcpsychiatry.biomedcentral.com/articles/ https://doi.org/10.1186/1471-244X-9-56 .
Lewis KL, Bohnert CA, Gammon WL, Hölzer H, Lyman L, Smith C et al. The Association of Standardized Patient Educators (ASPE) Standards of Best Practice (SOBP). Adv Simul [Internet]. 2017;2(1):10. http://advancesinsimulation.biomedcentral.com/articles/ https://doi.org/10.1186/s41077-017-0043-4 .
Zigmont JJ, Kappus LJ, Sudikoff SN. The 3D Model of Debriefing: Defusing, Discovering, and Deepening. Semin Perinatol [Internet]. 2011;35(2):52–8. https://linkinghub.elsevier.com/retrieve/pii/S0146000511000048 .
Rabie A, Hakami A. Impact of standardised patient Simulation Training on clinical competence, knowledge, and attitudes in Mental. Health Nurs Educ. 2023;15(9).
Liu Y, Qie D, Wang M, Li Y, Guo D, Chen X, et al. Application of role reversal and standardized patient simulation (SPS) in the training of new nurses. BMC Med Educ. 2023;23(1):1–6.
Alexander L, Sheen J, Rinehart N, Hay M, Boyd L. Mental Health Simulation With Student Nurses: A Qualitative Review. Clin Simul Nurs [Internet]. 2018;14:8–14. https://linkinghub.elsevier.com/retrieve/pii/S1876139917301664 .
Skinner D, Kendall H, Skinner HM, Campbell C. Mental Health Simulation: Effects on Students’ Anxiety and Examination Scores. Clin Simul Nurs [Internet]. 2019;35:33–7. https://linkinghub.elsevier.com/retrieve/pii/S1876139919300222 .
Luebbert R, Perez A, Andrews A, Webster-Cooley T. Standardized Patients Versus Mannequins in Mental Health Simulation. J Am Psychiatr Nurses Assoc [Internet]. 2023;29(4):283–9. http://journals.sagepub.com/doi/10.1177/10783903231183322 .
Conway KA, Scoloveno RL. The Use of Standardized Patients as an Educational Strategy in Baccalaureate Psychiatric Nursing Simulation: A Mixed Method Pilot Study. J Am Psychiatr Nurses Assoc [Internet]. 2022;107839032211010. http://journals.sagepub.com/doi/ https://doi.org/10.1177/10783903221101049 .
Horntvedt M-ET, Nordsteien A, Fermann T, Severinsson E. Strategies for teaching evidence-based practice in nursing education: a thematic literature review. BMC Med Educ [Internet]. 2018;18(1):172. https://bmcmededuc.biomedcentral.com/articles/ https://doi.org/10.1186/s12909-018-1278-z .
Kamali M, Hasanvand S, Kordestani-Moghadam P, Ebrahimzadeh F, Amini M. Impact of dyadic practice on the clinical self-efficacy and empathy of nursing students. BMC Nurs [Internet]. 2023;22(1):8. https://bmcnurs.biomedcentral.com/articles/ https://doi.org/10.1186/s12912-022-01171-y .
Riley J, Mandi DG, Bamouni J, Yaméogo RA, Naïbé DT, Kaboré E et al. No Title. Dasgupta K, editor. PLoS One [Internet]. 2021;4(1):e0205326. https://bmcresnotes.biomedcentral.com/articles/ https://doi.org/10.1186/s13104-018-3275-z .
Download references
Acknowledgements
Dr. W.C. Millanzi (PhD) and Dr. F. Moshi (PhD) are supervisors. This study was conducted by adhering to the international and national guidelines and the University of Dodoma postgraduate guidelines.
No source of funds.
Author information
Authors and affiliations.
Department of Nursing Management and Education, The University of Dodoma, Dodoma, Tanzania
Violeth E. Singano, Walter C. Millanzi & Fabiola Moshi
You can also search for this author in PubMed Google Scholar
Contributions
V.E.S.: Conceptualization, data collection, data analysis, and writing the manuscript W.C.M.: Conceptualization, supervision, data interpretation, draft and reviewed the manuscript. F.M.: Conceptualization, supervision, data interpretation, draft and reviewed the manuscript. All authors approved the manuscript.
Corresponding author
Correspondence to Violeth E. Singano .
Ethics declarations
Ethical approval and consent to participate in the study.
It is imperative to carry out tasks properly for any research project to be considered, so all protocol processes, including ethical Clearance obtained by the UDOM Institutional Research Review Ethics Committee (IRREC) with research proposal ethical clearance number MA.84/261/61/37 and research permit number MA.84/261/02/35 for Dodoma Region and MA.84/261/02/36 for Tanga Region, Tanzania. Written informed consent was obtained from the participants; respondents participated in the study after being informed and understanding all information concerning the research process. Confidentiality is assured by ensuring that the names of the participants or the training institution are not shown on the data collection instruments or the data collected from them for research purposes. Respondents’ privacy was safeguarded by providing them with separate, unoccupied rooms. The principal investigator maintained a high level of focus throughout the investigation. Data were meticulously managed using a designated key folder exclusively by the Principal Investigator and were not shared externally without the express authorization of both the Principal Investigator and UDOM. In cases where students chose to discontinue their participation in the study, permission was granted after they provided a reason to the principal investigator. Additionally, the respective authorities in the sampled study settings were readily available to manage unforeseen events such as student fainting, asthma attacks, or collapses, as the researcher may not have been able to address these situations adequately.
Consent for publication
Not applicable.
Competing interests
The authors declare no competing interests.
Additional information
Publisher’s note.
Springer Nature remains neutral with regard to jurisdictional claims in published maps and institutional affiliations.
Rights and permissions
Open Access This article is licensed under a Creative Commons Attribution 4.0 International License, which permits use, sharing, adaptation, distribution and reproduction in any medium or format, as long as you give appropriate credit to the original author(s) and the source, provide a link to the Creative Commons licence, and indicate if changes were made. The images or other third party material in this article are included in the article’s Creative Commons licence, unless indicated otherwise in a credit line to the material. If material is not included in the article’s Creative Commons licence and your intended use is not permitted by statutory regulation or exceeds the permitted use, you will need to obtain permission directly from the copyright holder. To view a copy of this licence, visit http://creativecommons.org/licenses/by/4.0/ . The Creative Commons Public Domain Dedication waiver ( http://creativecommons.org/publicdomain/zero/1.0/ ) applies to the data made available in this article, unless otherwise stated in a credit line to the data.
Reprints and permissions
About this article
Cite this article.
Singano, V.E., Millanzi, W.C. & Moshi, F. Effect of standardized patient simulation-based pedagogics embedded with lecture in enhancing mental status evaluation cognition among nursing students in Tanzania: A longitudinal quasi-experimental study. BMC Med Educ 24 , 577 (2024). https://doi.org/10.1186/s12909-024-05562-4
Download citation
Received : 16 October 2023
Accepted : 16 May 2024
Published : 26 May 2024
DOI : https://doi.org/10.1186/s12909-024-05562-4
Share this article
Anyone you share the following link with will be able to read this content:
Sorry, a shareable link is not currently available for this article.
Provided by the Springer Nature SharedIt content-sharing initiative
- Simulation pedagogics
BMC Medical Education
ISSN: 1472-6920
- Submission enquiries: [email protected]
- General enquiries: [email protected]

An official website of the United States government
The .gov means it’s official. Federal government websites often end in .gov or .mil. Before sharing sensitive information, make sure you’re on a federal government site.
The site is secure. The https:// ensures that you are connecting to the official website and that any information you provide is encrypted and transmitted securely.
- Publications
- Account settings
Preview improvements coming to the PMC website in October 2024. Learn More or Try it out now .
- Advanced Search
- Journal List
- BMC Med Res Methodol

Qualitative longitudinal research in health research: a method study
Åsa audulv.
1 Department of Nursing, Umeå University, Umeå, Sweden
Elisabeth O. C. Hall
2 Faculty of Health, Aarhus University, Aarhus, Denmark
3 Faculty of Health Sciences, University of Faroe Islands, Thorshavn, Faroe Islands Denmark
Åsa Kneck
4 Department of Health Care Sciences, Ersta Sköndal Bräcke University College, Stockholm, Sweden
Thomas Westergren
5 Department of Health and Nursing Science, University of Agder, Kristiansand, Norway
6 Department of Public Health, University of Stavanger, Stavanger, Norway
Mona Kyndi Pedersen
7 Center for Clinical Research, North Denmark Regional Hospital, Hjørring, Denmark
8 Department of Clinical Medicine, Aalborg University, Aalborg, Denmark
Hanne Aagaard
9 Lovisenberg Diaconale Univeristy of College, Oslo, Norway
Kristianna Lund Dam
Mette spliid ludvigsen.
10 Department of Clinical Medicine-Randers Regional Hospital, Aarhus University, Aarhus, Denmark
11 Faculty of Nursing and Health Sciences, Nord University, Bodø, Norway
Associated Data
The datasets used and analyzed in this current study are available in supplementary file 6 .
Qualitative longitudinal research (QLR) comprises qualitative studies, with repeated data collection, that focus on the temporality (e.g., time and change) of a phenomenon. The use of QLR is increasing in health research since many topics within health involve change (e.g., progressive illness, rehabilitation). A method study can provide an insightful understanding of the use, trends and variations within this approach. The aim of this study was to map how QLR articles within the existing health research literature are designed to capture aspects of time and/or change.
This method study used an adapted scoping review design. Articles were eligible if they were written in English, published between 2017 and 2019, and reported results from qualitative data collected at different time points/time waves with the same sample or in the same setting. Articles were identified using EBSCOhost. Two independent reviewers performed the screening, selection and charting.
A total of 299 articles were included. There was great variation among the articles in the use of methodological traditions, type of data, length of data collection, and components of longitudinal data collection. However, the majority of articles represented large studies and were based on individual interview data. Approximately half of the articles self-identified as QLR studies or as following a QLR design, although slightly less than 20% of them included QLR method literature in their method sections.
Conclusions
QLR is often used in large complex studies. Some articles were thoroughly designed to capture time/change throughout the methodology, aim and data collection, while other articles included few elements of QLR. Longitudinal data collection includes several components, such as what entities are followed across time, the tempo of data collection, and to what extent the data collection is preplanned or adapted across time. Therefore, there are several practices and possibilities researchers should consider before starting a QLR project.
Supplementary Information
The online version contains supplementary material available at 10.1186/s12874-022-01732-4.
Health research is focused on areas and topics where time and change are relevant. For example, processes such as recovery or changes in health status. However, relating time and change can be complicated in research, as the representation of reality in research publications is often collected at one point in time and fixed in its presentation, although time and change are always present in human life and experiences. Qualitative longitudinal research (QLR; also called longitudinal qualitative research, LQR) has been developed to focus on subjective experiences of time or change using qualitative data materials (e.g., interviews, observations and/or text documents) collected across a time span with the same participants and/or in the same setting [ 1 , 2 ]. QLR within health research may have many benefits. Firstly, human experiences are not fixed and consistent, but changing and diverse, therefore people’s experiences in relation to a health phenomenon may be more comprehensively described by repeated interviews or observations over time. Secondly, experiences, behaviors, and social norms unfold over time. By using QLR, researchers can collect empirical data that represents not only recalled human conceptions but also serial and instant situations reflecting transitions, trajectories and changes in people’s health experiences, personal development or health care organizations [ 3 – 5 ].
Key features of QLR
Whether QLR is a methodological approach in its own right or a design element of a particular study within a traditional methodological approach (e.g., ethnography or grounded theory) is debated [ 1 , 6 ]. For example, Bennett et al. [ 7 ] describe QLR as untied to methodology, giving researchers the flexibility to develop a suitable design for each study. McCoy [ 6 ] suggests that epistemological and ontological standpoints from interpretative phenomenological analysis (IPA) align with QLR traditions, thus making longitudinal IPA a suitable methodology. Plano-Clark et al. [ 8 ] described how longitudinal qualitative elements can be used in mixed methods studies, thus creating longitudinal mixed methods. In contrast, several researchers have argued that QLR is an emerging methodology [ 1 , 5 , 9 , 10 ]. For example, Thomson et al. [ 9 ] have stated “What distinguishes longitudinal qualitative research is the deliberate way in which temporality is designed into the research process, making change a central focus of analytic attention” (p. 185). Tuthill et al. [ 5 ] concluded that some of the confusion might have arisen from the diversity of data collection methods and data materials used within QLR research. However, there are no investigations showing to what extent QLR studies use QLR as a distinct methodology versus using a longitudinal data collection as a more flexible design element in combination with other qualitative methodologies.
QLR research should focus on aspects of temporality, time and/or change [ 11 – 13 ]. The concepts of time and change are seen as inseparable since change is happening with the passing of time [ 13 ]. However, time can be conceptualized in different ways. Time is often understood from a chronological perspective, and is viewed as fixed, objective, continuous and measurable (e.g., clock time, duration of time). However, time can also be understood from within, as the experience of the passing of time and/or the perspective from the current moment into the constructed conception of a history or future. From this perspective, time is seen as fluid, meaning that events, contexts and understandings create a subjective experience of time and change. Both the chronological and fluid understanding of time influence QLR research [ 11 ]. Furthermore, there is a distinction between over-time, which constitutes a comparison of the difference between points in time, often with a focus on the latter point or destination, and through-time, which means following an aspect across time while trying to understand the change that occurs [ 11 ]. In this article, we will mostly use the concept of across time to include both perspectives.
Some authors assert that QLR studies should include a qualitative data collection with the same sample across time [ 11 , 13 ], whereas Thomson et al. [ 9 ] also suggest the possibility of returning to the same data collection site with the same or different participants. When a QLR study involves data collection in shorter engagements, such as serial interviews, these engagements are often referred to as data collection time points. Data collection in time waves relates to longer engagements, such as field work/observation periods. There is no clear-cut definition for the minimum time span of a QLR study; instead, the length of the data collection period must be decided based upon what processes or changes are the focus of the study [ 13 ].
Most literature describing QLR methods originates from the social sciences, where the approach has a long tradition [ 1 , 10 , 14 ]. In health research, one-time-data collection studies have been the norm within qualitative methods [ 15 ], although health research using QLR methods has increased in recent years [ 2 , 5 , 16 , 17 ]. However, collecting and managing longitudinal data has its own sets of challenges, especially regarding how to integrate perspectives of time and/or change in the data collection and subsequent analysis [ 1 ]. Therefore, a study of QLR articles from the health research literature can provide an insightful understanding of the use, trends and variations of how methods are used and how elements of time/change are integrated in QLR studies. This could, in turn, provide inspiration for using different possibilities of collecting data across time when using QLR in health research. The aim of this study was to map how QLR articles within the existing health research literature are designed to capture aspects of time and/or change.
More specifically, the research questions were:
- What methodological approaches are described to inform QLR research?
- What methodological references are used to inform QLR research?
- How are longitudinal perspectives articulated in article aims?
- How is longitudinal data collection conducted?
In this method study, we used an adapted scoping review method [ 18 – 20 ]. Method studies are research conducted on research studies to investigate how research design elements are applied across a field [ 21 ]. However, since there are no clear guidelines for method studies, they often use adapted versions of systematic reviews or scoping review methods [ 21 ]. The adaptations of the scoping review method consisted of 1) using a large subsample of studies (publications from a three-year period) instead of including all QLR articles published, and 2) not including grey literature. The reporting of this study was guided by the Preferred Reporting Items for Systematic reviews and Meta-Analyses extension for Scoping Reviews (PRISMA-ScR) checklist [ 20 , 22 ] (see Additional file 1 ). A (unpublished) protocol was developed by the research team during the spring of 2019.
Eligibility criteria
In line with method study recommendations [ 21 ], we decided to draw on a manageable subsample of published QLR research. Articles that were eligible for inclusion were health research primary studies written in English, published between 2017 and 2019, and with a longitudinal qualitative data collection. Our operating definition for qualitative longitudinal data collection was data collected at different time points (e.g., repeated interviews) or time waves (e.g., periods of field work) involving the same sample or conducted in the same setting(s). We intentionally selected a broad inclusion criterion for QLR since we wanted a wide variety of articles. The selected time period was chosen because the first QLR method article directed towards health research was published in 2013 [ 1 ] and during the following years the methodological resources for QLR increased [ 3 , 8 , 17 , 23 – 25 ], thus we could expect that researchers publishing QLR in 2017–2019 should be well-grounded in QLR methods. Further, we found that from 2012 to 2019 the rate of published QLR articles were steady at around 100 publications per year, so including those from a three-year period would give a sufficient number of articles (~ 300 articles) for providing an overview of the field. Published conference abstracts, protocols, articles describing methodological issues, review articles, and non-research articles (e.g., editorials) were excluded.
Search strategy
Relevant articles were identified through systematic searches in EBSCOhost, including biomedical and life science research and nursing and allied health literature. A librarian who specialized in systematic review searches developed and performed the searches, in collaboration with the author team (LF, TW & ÅA). In the search, the term “longitudinal” was combined with terms for qualitative research (for the search strategy see Additional file 2 ). The searches were conducted in the autumn of 2019 (last search 2019-09-10).
Study selection
All identified citations were imported into EndNote X9 ( www.endnote.com ) and further imported into Rayyan QCRI online software [ 26 ], and duplicates were removed. All titles and abstracts were screened against the eligibility criteria by two independent reviewers (ÅA & EH), and conflicting decisions were discussed until resolved. After discussions by the team, we decided to include articles published between 2017 and 2019, that selection alone included 350 records with diverse methods and designs. The full texts of articles that were eligible for inclusion were retrieved. In the next stage, two independent reviewers reviewed each full text article to make final decisions regarding inclusion (ÅA, EH, Julia Andersson). In total, disagreements occurred in 8% of the decisions, and were resolved through discussion. Critical appraisal was not assessed since the study aimed to describe the range of how QLR is applied and not aggregate research findings [ 21 , 22 ].
Data charting and analysis
A standardized charting form was developed in Excel (Excel 2016). The charting form was reviewed by the research team and pretested in two stages. The tests were performed to increase internal consistency and reduce the risk of bias. First, four articles were reviewed by all the reviewers, and modifications were made to the form and charting instructions. In the next stage, all reviewers used the charting form on four other articles, and the convergence in ratings was 88%. Since the convergence was under 90%, charting was performed in duplicate to reduce errors in the data. At the end of the charting process, the convergence among the reviewers was 95%. The charting was examined by the first author, who revised the charting in cases of differences.
Data items that were charted included 1) the article characteristics (e.g., authors, publication year, journal, country), 2) the aim and scope (e.g., phenomenon of interest, population, contexts), 3) the stated methodology and analysis method, 4) text describing the data collection (e.g., type of data material, number of participants, time frame of data collection, total amount of data material), and 5) the qualitative methodological references used in the methods section. Extracted text describing data collection could consist of a few sentences or several sections from the articles (and sometimes figures) concerning data collection practices, rational for time periods and research engagement in the field. This was later used to analyze how the longitudinal data collection was conducted and elements of longitudinal design. To categorize the qualitative methodology approaches, a framework from Cresswell [ 27 ] was used (including the categories for grounded theory, phenomenology, ethnography, case study and narrative research). Overall, data items needed to be explicitly stated in the articles in order to be charted. For example, an article was categorized as grounded theory if it explicitly stated “in this grounded theory study” but not if it referred to the literature by Glaser and Strauss without situating itself as a grounded theory study (See Additional file 3 for the full instructions for charting).
All charting forms were compiled into a single Microsoft Excel spreadsheet (see Supplementary files for an overview of the articles). Descriptive statistics with frequencies and percentages were calculated to summarize the data. Furthermore, an iterative coding process was used to group the articles and investigate patterns of, for example, research topics, words in the aims, or data collection practices. Alternative ways of grouping and presenting the data were discussed by the research team.
Search and selection
A total of 2179 titles and abstracts were screened against the eligibility criteria (see Fig. 1 ). The full text of one article could not be found and the article was excluded [ 28 ]. Fifty full text articles were excluded. Finally, 299 articles, representing 271 individual studies, were included in this study (see additional files 4 and 5 respectively for tables of excluded and included articles).

PRISMA diagram of study selection]
General characteristics and research areas of the included articles
The articles were published in many journals ( n = 193), and 138 of these journals were represented with one article each. BMJ Open was the most prevalent journal ( n = 11), followed by the Journal of Clinical Nursing ( n = 8). Similarly, the articles represented many countries ( n = 41) and all the continents; however, a large part of the studies originated from the US or UK ( n = 71, 23.7% and n = 70, 23.4%, respectively). The articles focused on the following types of populations: patients, families−/caregivers, health care providers, students, community members, or policy makers. Approximately 20% ( n = 63, 21.1%) of the articles collected data from two or more of these types of population(s) (see Table 1 ).
Characteristics of the included QLR articles
Approximately half of the articles ( n = 158, 52.8%) articulated being part of a larger research project. Of them, 95 described a project with both quantitative and qualitative methods. They represented either 1) a qualitative study embedded in an intervention, evaluation or implementation study ( n = 66, 22.1%), 2) a longitudinal cohort study collecting both quantitative and qualitative material ( n = 23, 7.7%), or 3) qualitative longitudinal material collected together with a cross sectional survey (n = 6, 2.0%). Forty-eight articles (16.1%) described belonging to a larger qualitative project presented in several research articles.
Methodological traditions
Approximately one-third ( n = 109, 36.5%) of the included articles self-identified with one of the qualitative traditions recognized by Cresswell [ 27 ] (case study: n = 36, 12.0%; phenomenology: n = 35, 11.7%; grounded theory: n = 22, 7.4%; ethnography: n = 13, 4.3%; narrative method: n = 3, 1.0%). In nine articles, the authors described using a mix of two or more of these qualitative traditions. In addition, 19 articles (6.4%) self-identified as mixed methods research.
Every second article self-identified as having a qualitative longitudinal design ( n = 156, 52.2%); either they self-identified as “a longitudinal qualitative study” or “using a longitudinal qualitative research design”. However, in some articles, this was stated in the title and/or abstract and nowhere else in the article. Fifty-two articles (17.4%) self-identified both as having a QLR design and following one of the methodological approaches (case study: n = 8; phenomenology: n = 23; grounded theory: n = 9; ethnography: n = 6; narrative method: n = 2; mixed methods: n = 4).
The other 143 articles used various terms to situate themselves in relation to a longitudinal design. Twenty-seven articles described themselves as a longitudinal study (9.0%) or a longitudinal study within a specific qualitative tradition (e.g., a longitudinal grounded theory study or a longitudinal mixed method study) ( n = 64, 21.4%). Furthermore, 36 articles (12.0%) referred to using longitudinal data materials (e.g., longitudinal data or longitudinal interviews). Nine of the articles (3.0%) used the term longitudinal in relation to the data analysis or aim (e.g., the aim was to longitudinally describe), used terms such as serial or repeated in relation to the data collection design ( n = 2, 0.7%), or did not use any term to address the longitudinal nature of their design ( n = 5, 1.7%).
Use of methodological references
The mean number of qualitative method references in the methods sections was 3.7 (range 0 to 16), and 20 articles did not have any qualitative method reference in their methods sections. 1 Commonly used method references were generic books on qualitative methods, seminal works within qualitative traditions, and references specializing in qualitative analysis methods (see Table 2 ). It should be noted that some references were comprehensive books and thus could include sections about QLR without being focused on the QLR method. For example, Miles et al. [ 31 ] is all about analysis and coding and includes a chapter regarding analyzing change.
Most frequently used method references (8 most used) and QLR method references (5 most used). Citations in Google Scholar were used as an indication of how widely used the references are; searches conducted in Google Scholar 2022-01-02
Only approximately 20% ( n = 58) of the articles referred to the QLR method literature in their methods sections. 2 The mean number of QLR method references (counted for articles using such sources) was 1.7 (range 1 to 6). Most articles using the QLR method literature also used other qualitative methods literature (except two articles using one QLR literature reference each [ 39 , 40 ]). In total, 37 QLR method references were used, and 24 of the QLR method references were only referred to by one article each.
Longitudinal perspectives in article aims
In total, 231 (77.3%) articles had one or several terms related to time or change in their aims, whereas 68 articles (22.7%) had none. Over one hundred different words related to time or change were identified. Longitudinally oriented terms could focus on changes across time (process, trajectory, transition, pathway or journey), patterns of how something changed (maintenance, continuity, stability, shifts), or phenomena that by nature included change (learning or implementation). Other types of terms emphasized the data collection time period (e.g., over 6 months) or a specific changing situation (e.g., during pregnancy, through the intervention period, or moving into a nursing home). The most common terms used for the longitudinal perspective were change ( n = 63), over time ( n = 52), process ( n = 36), transition ( n = 24), implementation ( n = 14), development ( n = 13), and longitudinal (n = 13). 3
Furthermore, the articles varied in what ways their aims focused on time/change, e.g., the longitudinal perspectives in the aims (see Table 3 ). In 71 articles, the change across time was the phenomenon of interest of the article : for example, articles investigating the process of learning or trajectories of diseases. In contrast, 46 articles investigated change or factors impacting change in relation to a defined outcome : for example, articles investigating factors influencing participants continuing in a physical activity trial. The longitudinal perspective could also be embedded in an article’s context . In such cases, the focus of the article was on experiences that happened during a certain time frame or in a time-related context (e.g., described experiences of the patient-provider relationship during 6 months of rehabilitation).
Different longitudinal perspectives in the articles’ aims and objectives
Types of data and length of data collection
The QLR articles were often large and complex in their data collection methods. The median number of participants was 20 (range from one to 1366, the latter being an article with open-ended questions in questionnaires [ 46 ]). Most articles used individual interviews as the data material ( n = 167, 55.9%) or a combination of data materials ( n = 98, 32.8%) (e.g., interviews and observations, individual interviews and focus group interviews, or interviews and questionnaires). Forty-five articles (15.1%) presented quantitative and qualitative results. The median number of interviews was 46 (range three to 507), which is large in comparison to many qualitative studies. The observation materials were also comprehensive and could include several hundred hours of observations. Documents were often used as complementary material and included official documents, newspaper articles, diaries, and/or patient records.
The articles’ time spans 4 for data collection varied between a few days and over 20 years, with 60% of the articles’ time spans being 1 year or shorter ( n = 180) (see Fig. 2 ). The variation in time spans might be explained by the different kinds of phenomena that were investigated. For example, Jensen et al. [ 47 ] investigated hospital care delivery and followed each participant, with observations lasting between four and 14 days. Smithbattle [ 48 ] described the housing trajectories of teen mothers, and collected data in seven waves over 28 years.

Number of articles in relation to the time span of data collection. The time span of data collection is given in months
Three components of longitudinal data collection
In the articles, the data collection was conducted in relation to three different longitudinal data collection components (see Table 4 ).
Components of longitudinal data collection
Entities followed across time
Four different types of entities were followed across time: 1) individuals, 2) individual cases or dyads, 3) groups, and 4) settings. Every second article ( n = 170, 56.9%) followed individuals across time, thus following the same participants through the whole data collection period. In contrast, when individual cases were followed across time, the data collection was centered on the primary participants (e.g., people with progressive neurological conditions) who were followed over time, and secondary participants (e.g., family caregivers) might provide complementary data at several time points or only at one-time point. When settings were followed over time, the participating individuals were sometimes the same, and sometimes changed across the data collection period. Typical settings were hospital wards, hospitals, smaller communities or intervention trials. The type of collected data corresponded with what kind of entities were followed longitudinally. Individuals were often followed with serial interviews, whereas groups were commonly followed with focus group interviews complemented with individual interviews, observations and/or questionnaires. Overall, the lengths of data collection periods seemed to be chosen based upon expected changes in the chosen entities. For example, the articles following an intervention setting were structured around the intervention timeline, collecting data before, after and sometimes during the intervention.
Tempo of data collection
The data collection tempo differed among the articles (e.g., the frequency and mode of the data collection). Approximately half ( n = 154, 51.5%) of the articles used serial time points, collecting data at several reoccurring but shorter sequences (e.g., through serial interviews or open-ended questions in questionnaires). When data were collected in time waves ( n = 50, 16.7%), the periods of data collection were longer, usually including both interviews and observations; often, time waves included observations of a setting and/or interviews at the same location over several days or weeks.
When comparing the tempo with the type of entities, some patterns were detected (see Fig. 3 ). When individuals were followed, data were often collected at time points, mirroring the use of individual interviews and/or short observations. For research in settings, data were commonly collected in time waves (e.g., observation periods over a few weeks or months). In studies exploring settings across time, time waves were commonly used and combined several types of data, particularly from interviews and observations. Groups were the least common studied entity ( n = 9, 3.0%), so the numbers should be interpreted with caution, but continuous data collection was used in five of the nine studies. The continuous data collection mode was, for example, collecting electronic diaries [ 62 ] or minutes from committee meetings during a time period [ 63 ].

Tempo of data collection in relation to entities followed over time
Preplanned or adapted data collection
A large majority ( n = 224, 74.9%) of the articles used preplanned data collection (e.g., in preplanned data collection, all participants were followed across time according to the same data collection plan). For example, all participants were interviewed one, six and twelve months’ post-diagnosis. In contrast to the preplanned data collection approach, 44 articles had a participant-adapted data collection (14.7%), and participants were followed at different frequencies and/or over various lengths of time depending on each participant’s situation. Participant-adapted data collection was more common among articles following individuals or individual cases (see Fig. 4 ). To adapt the data collection to the participants, the researchers created strategies to reach participants when crucial events were happening. Eleven articles used a participant entry approach to data collection ( n = 11, 6.7%), and the whole or parts of the data were independently sent in by participants in the form of diaries, questionnaires, or blogs. Another approach to data collection was using theoretical or analysis-driven ideas to guide the data collection ( n = 19, 6.4%). In these articles, the analysis and data collection were conducted simultaneously, and ideas arising in the analysis could be followed up, for example, returning to some participants, recruiting participants with specific experiences, or collecting complementary types of data materials. This approach was most common in the articles following settings across time, which often included observations and interviews with different types of populations. Articles using theoretical or analysis driven data collection were not associated with grounded theory to a greater extent than the other articles in the sample (e.g., did not self-identify as grounded theory or referred to methodological literature within grounded theory traditions to a greater proportion).

Preplanned or adapted data collection in relation to entities followed over time
According to our results, some researchers used QLR as a methodological approach and other researchers used a longitudinal qualitative data collection without aiming to investigate change. Adding to the debate on whether QLR is a methodological approach in its own right or a design element in a particular study we suggest that the use of QLR can be described as layered (see Fig. 5 ). Namely, articles must fulfill several criteria in order to use QLR as a methodological approach, and that is done in some articles. In those articles QLR method references were used, the aim was to investigate change of a phenomenon and the longitudinal elements of the data collection were thoroughly integrated into the method section. On the other hand, some articles using a longitudinal qualitative data collection were just collecting data over time, without addressing time and/or change in the aim. These articles can still be interesting research studies with valuable results, but they are not using the full potential of QLR as a methodological approach. In all, around 40% of the articles had an aim that focused on describing or understanding change (either as phenomenon or outcome); but only about 24% of the articles set out to investigate change across time as their phenomenon of interest.

The QLR onion. The use of QLR design can be described as layered, where researchers use more or less elements of a QLR design. The two inmost layers represents articles using QLR as a methodological approach
Regarding methodological influences, about one-third of the articles self-identify with any of the traditional qualitative methodologies. Using a longitudinal qualitative data collection as an element integrated with another methodological tradition can therefore be seen as one way of working with longitudinal qualitative materials. In our results, the articles referring to methodologies other than QLR preferably used case study, phenomenology and grounded theory methodologies. This was surprising since Neale [ 10 ] identified ethnography, case studies and narrative methods as the main methodological influences on QLR. Our findings might mirror the profound impacts that phenomenology and grounded theory have had on the qualitative field of health research. Regarding phenomenology, the findings can also be influenced by more recent discussions of combining interpretative phenomenological analysis with QLR [ 6 ].
Half of the articles self-identified as QLR studies, but QLR method references were used in less than 20% of the identified articles. This is both surprising and troublesome since use of appropriate method literature might have supported researchers who were struggling with for example a large quantity of materials and complex analysis. A possible explanation for the lack of use of QLR method literature is that QLR as a methodological approach is not well known, and authors might not be aware that method literature exists. It is quite understandable that researchers can describe a qualitative project with longitudinal data collection as a qualitative longitudinal study, without being aware that QLR is a specific form of study. Balmer [ 64 ] described how their group conducted serial interviews with medical students over several years before they became aware of QLR as a method of study. Within our networks, we have met researchers with similar experiences. Likewise, peer reviewers and editorial boards might not be accustomed to evaluating QLR manuscripts. In our results, 138 journals published one article between 2017 and 2019, and that might not be enough for editorial boards and peer reviewers to develop knowledge to enable them to closely evaluate manuscripts with a QLR method.
In 2007, Holland and colleagues [ 65 ] mapped QLR in the UK and described the following four categories of QLR: 1) mixed methods approaches with a QLR component; 2) planned prospective longitudinal studies; 3) follow-up studies complementing a previous data collection with follow-up; and 4) evaluation studies. Examples of all these categories can be found among the articles in this method study; however, our results do paint a more complex picture. According to our results, Holland’s categories are not multi-exclusive. For example, studies with intentions to evaluate or implement practices often used a mixed methods design and were therefore eligible for both categories one and four described above. Additionally, regarding the follow-up studies, it was seldom clearly described if they were planned as a two-time-point study or if researchers had gained an opportunity to follow up on previous data collection. When we tried to categorize QLR articles according to the data collection design, we could not identify multi-exclusive categories. Instead, we identified the following three components of longitudinal data collection: 1) entities followed across time; 2) tempo; and 3) preplanned or adapted data collection approaches. However, the most common combination was preplanned studies that followed individuals longitudinally with three or more time points.
The use of QLR differs between disciplines [ 14 ]. Our results show some patterns for QLR within health research. Firstly, the QLR projects were large and complex; they often included several types of populations and various data materials, and were presented in several articles. Secondly, most studies focused upon the individual perspective, following individuals across time, and using individual interviews. Thirdly, the data collection periods varied, but 53% of the articles had a data collection period of 1 year or shorter. Finally, patients were the most prevalent population, even though topics varied greatly. Previously, two other reviews that focused on QLR in different parts of health research (e.g., nursing [ 4 ] and gerontology [ 66 ]) pointed in the same direction. For example, individual interviews or a combination of data materials were commonly used, and most studies were shorter than 1 year but a wide range existed [ 4 , 66 ].
Considerations when planning a QLR project
Based on our results, we argue that when health researchers plan a QLR study, they should reflect upon their perspective of time/change and decide what part change should play in their QLR study. If researchers decide that change should play the main role in their project, then they should aim to focus on change as the phenomenon of interest. However, in some research, change might be an important part of the plot, without having the main role, and change in relation to the outcomes might be a better perspective. In such studies, participants with change, no change or different kinds of change are compared to explore possible explanations for the change. In our results, change in relation to the outcomes was often used in relation to intervention studies where participants who reached a desired outcome were compared to individuals who did not. Furthermore, for some research studies, change is part of the context in which the research takes place. This can be the case when certain experiences happen during a period of change; for example, when the aim is to explore the experience of everyday life during rehabilitation after stroke. In such cases a longitudinal data collection could be advisable (e.g., repeated interviews often give a deep relationship between interviewer and participants as well as the possibility of gaining greater depth in interview answers during follow-up interviews [ 15 ]), but the study might not be called a QLR study since it does not focus upon change [ 13 ]. We suggest that researchers make informed decisions of what kind of longitudinal perspective they set out to investigate and are transparent with their sources of methodological inspiration.
We would argue that length of data collection period, type of entities, and data materials should be in accordance with the type of change/changing processes that a study focuses on. Individual change is important in health research, but researchers should also remember the possibility of investigating changes in families, working groups, organizations and wider communities. Using these types of entities were less common in our material and could probably grant new perspectives to many research topics within health. Similarly, using several types of data materials can complement the insights that individual interviews can give. A large majority of the articles in our results had a preplanned data collection. Participant-adapted data collection can be a way to work in alignment with a “time-as-fluid” conceptualization of time because the events of subjective importance to participants can be more in focus and participants (or other entities) change processes can differ substantially across cases. In studies with lengthy and spaced-out data collection periods and/or uncertainty in trajectories, researchers should consider participant-adapted or participant entry data collection. For example, some participants can be followed for longer periods and/or with more frequency.
Finally, researchers should consider how to best publish and disseminate their results. Many QLR projects are large, and the results are divided across several articles when they are published. In our results, 21 papers self-identified as a mixed methods project or as part of a larger mixed methods project, but most of these did not include quantitative data in the article. This raises the question of how to best divide a large research project into suitable pieces for publication. It is an evident risk that the more interesting aspects of a mixed methods project are lost when the qualitative and quantitative parts are analyzed and published separately. Similar risks occur, for example, when data have been collected from several types of populations but are then presented per population type (e.g., one article with patient data and another with caregiver data). During the work with our study, we also came across studies where data were collected longitudinally, but the results were divided into publications per time point. We do not argue that these examples are always wrong, there are situations when these practices are appropriate. However, it often appears that data have been divided without much consideration. Instead, we suggest a thematic approach to dividing projects into publications, crafting the individual publications around certain ideas or themes and thus using the data that is most suitable for the particular research question. Combining several types of data and/or several populations in an analysis across time is in fact what makes QLR an interesting approach.
Strengths and limitations
This method study intended to paint a broad picture regarding how longitudinal qualitative methods are used within the health research field by investigating 299 published articles. Method research is an emerging field, currently with limited methodological guidelines [ 21 ], therefore we used scoping review method to support this study. In accordance with scoping review method we did not use quality assessment as a criterion for inclusion [ 18 – 20 ]. This can be seen as a limitation because we made conclusions based upon a set of articles with varying quality. However, we believe that learning can be achieved by looking at both good and bad examples, and innovation may appear when looking beyond established knowledge, or assessing methods from different angles. It should also be noted that the results given in percentages hold no value for what procedures that are better or more in accordance with QLR, the percentages simply state how common a particular procedure was among the articles.
As described, the included articles showed much variation in the method descriptions. As the basis for our results, we have only charted explicitly written text from the articles, which might have led to an underestimation of some results. The researchers might have had a clearer rationale than described in the reports. Issues, such as word restrictions or the journal’s scope, could also have influenced the amount of detail that was provided. Similarly, when charting how articles drew on a traditional methodology, only data from the articles that clearly stated the methodologies they used (e.g., phenomenology) were charted. In some articles, literature choices or particular research strategies could implicitly indicate that the researchers had been inspired by certain methodologies (e.g., referring to grounded theory literature and describing the use of simultaneous data collection and analysis could indicate that the researchers were influenced by grounded theory), but these were not charted as using a particular methodological tradition. We used the articles’ aims and objectives/research questions to investigate their longitudinal perspectives. However, as researchers have different writing styles, information regarding the longitudinal perspectives could have been described in surrounding text rather than in the aim, which might have led to an underestimation of the longitudinal perspectives.
The experience and diversity of the research team in our study was a strength. The nine authors on the team represent ten universities and three countries, and have extensive experience in different types of qualitative research, QLR and review methods. The different level of experiences with QLR within the team (some authors have worked with QLR in several projects and others have qualitative experience but no experience in QLR) resulted in interesting discussions that helped drive the project forward. These experiences have been useful for understanding the field.
Based on a method study of 299 articles, we can conclude that QLR in health research articles published between 2017 and 2019 often contain comprehensive complex studies with a large variation in topics. Some research was thoroughly designed to capture time/change throughout the methodology, focus and data collection, while other articles included a few elements of QLR. Longitudinal data collection included several components, such as what entities were followed across time, the tempo of data collection, and to what extent the data collection was preplanned or adapted across time. In sum, health researchers need to be considerate and make informed choices when designing QLR projects. Further research should delve deeper into what kind of research questions go well with QLR and investigate the best practice examples of presenting QLR findings.
Acknowledgments
The authors wish to acknowledge Ellen Sejersted, librarian at the University of Agder, Kristiansand, Norway, who conducted the literature searches and Julia Andersson, research assistant at the Department of Nursing, Umeå University, Sweden, who supported the data management and took part in the initial screening phases of the project.
Authors’ contributions
ÅA conceived the study. ÅA, EH, TW, LF, MKP, HA, and MSL designed the study. ÅA, TW, and LF were involved in literature searches together with the librarian. ÅA and EH performed the screening of the articles. All authors (ÅA, EH, TW, LF, ÅK, MKP, KLD, HA, MSL) took part in the data charting. ÅA performed the data analysis and discussed the preliminary results with the rest of the team. ÅA wrote the 1st manuscript draft, and ÅK, MSL and EH edited. All authors (ÅA, EH, TW, LF, ÅK, MKP, KLD, HA, MSL) contributed to editing the 2nd draft. MSL and LF provided overall supervision. All authors read and approved the final manuscript.
Authors’ information
All authors represent the nursing discipline, but their research topics differ. ÅA and ÅK have previously worked together with QLR method development. ÅA, EH, TW, LF, MKP, HA, KLD and MSL work together in the Nordic research group PRANSIT, focusing on nursing topics connected to transition theory using a systematic review method, preferably meta synthesis. All authors have extensive experience with qualitative research but various experience with QLR.
Open access funding provided by Umea University. This project was conducted within the authors’ positions and did not receive any specific funding.
Availability of data and materials
Declarations.
Not applicable.
The authors declare that they have no competing interests.
1 Qualitative method references were defined as a journal article or book with a title that indicated an aim to guide researchers in qualitative research methods and/or research theories. Primary studies, theoretical works related to the articles’ research topics, protocols, and quantitative method literature were excluded. References written in a language other than English was also excluded since the authors could not evaluate their content.
2 QLR method references were defined as a journal article or book that 1) focused on qualitative methodological questions, 2) used terms such as ‘longitudinal’ or ‘time’ in the title so it was evident that the focus was on longitudinal qualitative research. Referring to another original QLR study was not counted as using QLR method literature.
3 Words were charted depending on their word stem, e.g., change, changes and changing were all charted as change.
4 It should be noted that here time span refers to the data collection related to each participant or case. Researchers could collect data for 2 years but follow each participant for 6 months.
Publisher’s Note
Springer Nature remains neutral with regard to jurisdictional claims in published maps and institutional affiliations.

Longitudinal Study Looks at Risk of Cardiovascular Disease With Long-Term ADHD Medication Use
- Mark Mullen, MD
- Rajesh R. Tampi, MD, MS, DFAPA, DFAAGP
A study assessed the associations between the use of ADHD medications and CVD over the course of 14 years. Here's what the investigators found.
TRANSLATING RESEARCH INTO PRACTICE
Rajesh R. Tampi, MD, MS, DFAPA, DFAAGP, Column Editor
A monthly column dedicated to reviewing the literature and sharing clinical implications.

vegefox.com_AdobeStock
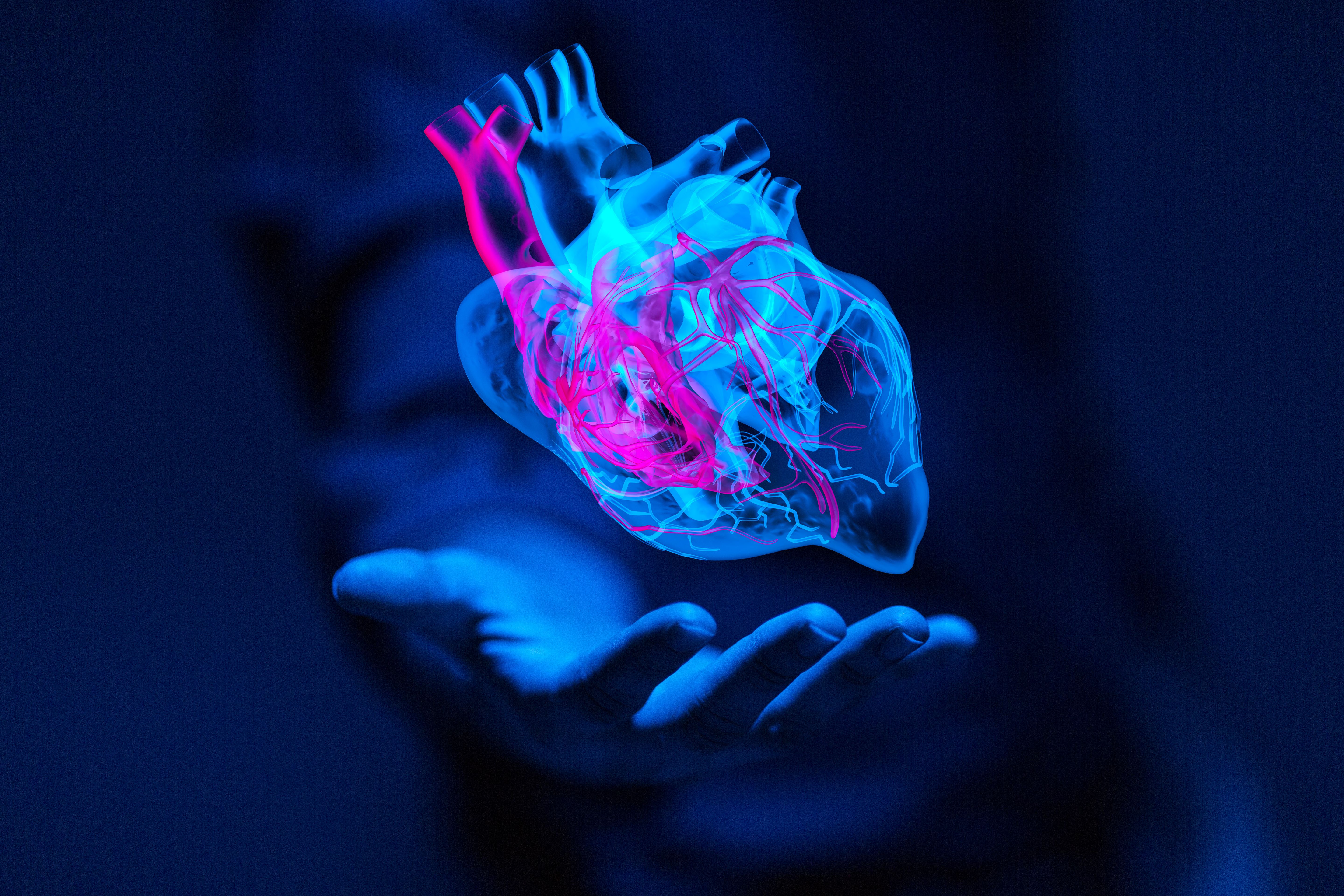
Recent decades have seen increased medication use for attention-deficit/hyperactivity disorder (ADHD), including both stimulants and nonstimulants. However, long-term effects of ADHD medications on the cardiovascular system are not fully understood.
There is limited evidence on whether long-term use is associated with an increased risk of cardiovascular disease (CVD), with most prior studies having an average follow-up time of no more than 2 years.
This study used the nationwide health registers in Sweden to assess the associations between the use of ADHD medications and CVD over the course of 14 years. 1
Zhang L, Li L, Andell P, et al. Attention-deficit/hyperactivity disorder medications and long-term risk of cardiovascular diseases . JAMA Psychiatry . 2024;81(2):178-187.
Study Funding
L. Zhang: Grants from Swedish Research Council for Health, Working Life, and Welfare
H. Larsson: European Union’s Horizon 2020 research and innovation program under grant agreement
Study Objectives
To assess the association between long-term use of ADHD medication and the risk of CVD.
Methodology
This was a population-based case-control study looking at all individuals in Sweden aged 6 to 64 years who either had an incident diagnosis of ADHD or had been prescribed ADHD medication between January 1, 2007 and December 31, 2020. Excluded from the study were patients with a previous CVD diagnosis, those who used ADHD medication for other indications, and those who had emigrated or died before the baseline.
In this study, the investigators defined baseline (cohort entry) as the date of incident ADHD diagnosis or ADHD medication dispensation, whichever event came earlier. Patient demographics including diagnosis, medication dispensation history, socioeconomic factors, and death information were obtained from multiple Swedish nationwide registries: the Swedish National Inpatient Register, the Swedish Prescribed Drug Register, the Longitudinal Integrated Database for Health Insurance and Labor Market Studies, and the National Cause of Death Register.
CVD diagnosis was defined by the International Statistical Classification of Diseases and Related Health Problems, Tenth Revision (ICD-10) and included ischemic heart diseases, cerebrovascular diseases, hypertension, heart failure, arrhythmias, thromboembolic disease, arterial disease, and other forms of heart disease.
Each patient case—that is, a patient with ADHD who received a CVD diagnosis after initiation of ADHD medication—was matched with up to 5 controls who did not receive a CVD diagnosis. Patient cases and controls were matched based on age, sex, and calendar time to ensure similar lengths of follow-up. Each patient was followed for up to 14 years. The primary exposure was cumulative duration of ADHD medication use, and the primary outcome was incident CVD.
Study Strengths
1. This is a large study that included 278,027 individuals aged 6 to 64 years with ADHD.
2. The follow-up for this study was significantly longer than that of previous studies assessing long-term cardiovascular risk with ADHD medication use.
3. Data on ADHD medication prescriptions and CVD diagnoses were recorded prospectively, which mitigates the risk of recall bias.
4. Many confounding variables were accounted for, including age, sex, calendar time, country of birth, educational level, and psychiatric comorbidities.
5. Funders had no role in the study’s design, collection or interpretation of the data, or preparation or publication of the manuscript.
Study Limitations
1. Patients with CVD were identified based on diagnoses recorded in medical records, rather than assessment of symptomatology by investigators, which could lead to underreporting of CVD diagnoses.
2. As there was no way to ensure adherence with ADHD medications, it is possible that patients were misclassified due to discrepancies in adherence.
3. This study’s data cannot prove causality, as the study is observational.
4. Other time-varying confounders that were not accounted for, such as use of other psychotropic medications and lifestyle factors, could have impacted the risk for CVD.
5. Severity of ADHD is a potential confounding variable because patients with more severe ADHD symptoms tend to have more comorbidities and less healthy lifestyles, increasing the risk for CVD.
6. This is a Swedish study; it is possible that cultural differences regarding ADHD medication use may limit generalizability of the findings in the United States and in other countries.
Conditional logistic regression analyses were used to estimate odds ratios for the associations between cumulative durations of ADHD medication use and incident CVD. The crude odds ratios were adjusted for all matching variables, which included age, sex, and calendar time. The adjusted odds ratios (AORs) were additionally controlled for country of birth (Sweden vs another country), highest educational level, and diagnoses of somatic and psychiatric comorbidities before baseline.
Study Results
The study’s treatment effect showed that longer use of ADHD medications was associated with increased CVD risk compared with nonuse (1 to ≤ 2 years: AOR, 1.09 [95% CI, 1.01-1.18]; 2 to ≤ 3 years: AOR, 1.15 [95% CI, 1.05-1.25]; 3 to ≤ 5 years: AOR, 1.27 [95% CI, 1.17-1.39]; and > 5 years: AOR, 1.23 [95% CI, 1.12-1.36]).
Specifically, there was a significant association of long-term ADHD medication use with hypertension (AOR, 1.72 [95% CI, 1.51-1.97] for 3 to ≤ 5 years) and arterial disease (AOR, 1.65 [95% CI, 1.11-2.45] for 3 to ≤ 5 years). There were no statistically significant associations for arrhythmias, heart failure, ischemic heart disease, thromboembolic disease, or cerebrovascular disease.
Throughout the study’s follow-up, each 1-year increase in the use of ADHD medications correlated with a 4% increased association of CVD (95% CI, 1.03-1.05) and an 8% increased association in the first 3 years (95% CI, 1.04-1.11). There was a rapid increase in association for the first 3 cumulative years, but the association stabilized thereafter.
The association between CVD and ADHD medications increased with higher average defined daily doses (DDDs; eg, 30 mg methylphenidate or 80 mg atomoxetine), with a statistically significant risk found only among individuals with a mean dose of at least 1.5 times the DDD. Researchers found a 4% increased risk for individuals receiving 1.5 to 2 times the DDD, whereas they found a 5% increased risk for individuals receiving more than 2 times the DDD.
Looking at specific classes of ADHD medications, atomoxetine’s association with CVD was significant only for the first year of use, with an AOR of 1.07 (95% CI, 1.01-1.13). For methylphenidate use vs no use, the AOR was 1.20 (95% CI, 1.10-1.31) for 3 to 5 or fewer years.
For greater than 5 years, the AOR was 1.19 (95% CI, 1.08-1.31). The data for lisdexamfetamine use vs no use showed an AOR of 1.23 (95% CI, 1.05-1.44) for 2 to 3 or fewer years. Across groups, researchers observed similar associations in females and males.
Conclusions
The results of this population-based case control study suggest that long-term ADHD medication use was associated with an increased risk of CVD, specifically hypertension and arterial diseases. The observed risk of CVD was greater when stimulant medications were used in comparison with nonstimulant medications to treat ADHD. There was also a significant association between the cumulative duration of ADHD medication use and increased risk of CVD.
Practical Applications
Long-term use of ADHD medications is associated with CVD. It is important to weigh the risks and benefits of this treatment modality with every patient. It is also important to closely monitor patients for the signs and symptoms of CVD in those who are taking these medications.
Bottom Line
This nested case-control study followed patients for 14 years and found that long-term ADHD medication use was associated with CVD, specifically hypertension and arterial diseases.
Dr Impallaria is a first-year psychiatry resident at Creighton University in Omaha, Nebraska. Dr Schuster is a third-year psychiatry resident at Creighton University. Dr Mullen is a fourth-year psychiatry resident at Creighton University. Dr Tampi is a professor and the chairman of the Department of Psychiatry at Creighton University School of Medicine and Catholic Health Initiatives (CHI) Health Behavioral Health Services. He is also an adjunct professor of psychiatry at Yale School of Medicine and a member of the Psychiatric Times editorial board.
1. Zhang L, Li L, Andell P, et al. Attention-deficit/hyperactivity disorder medications and long-term risk of cardiovascular diseases. JAMA Psychiatry . 2024;81(2):178-187.
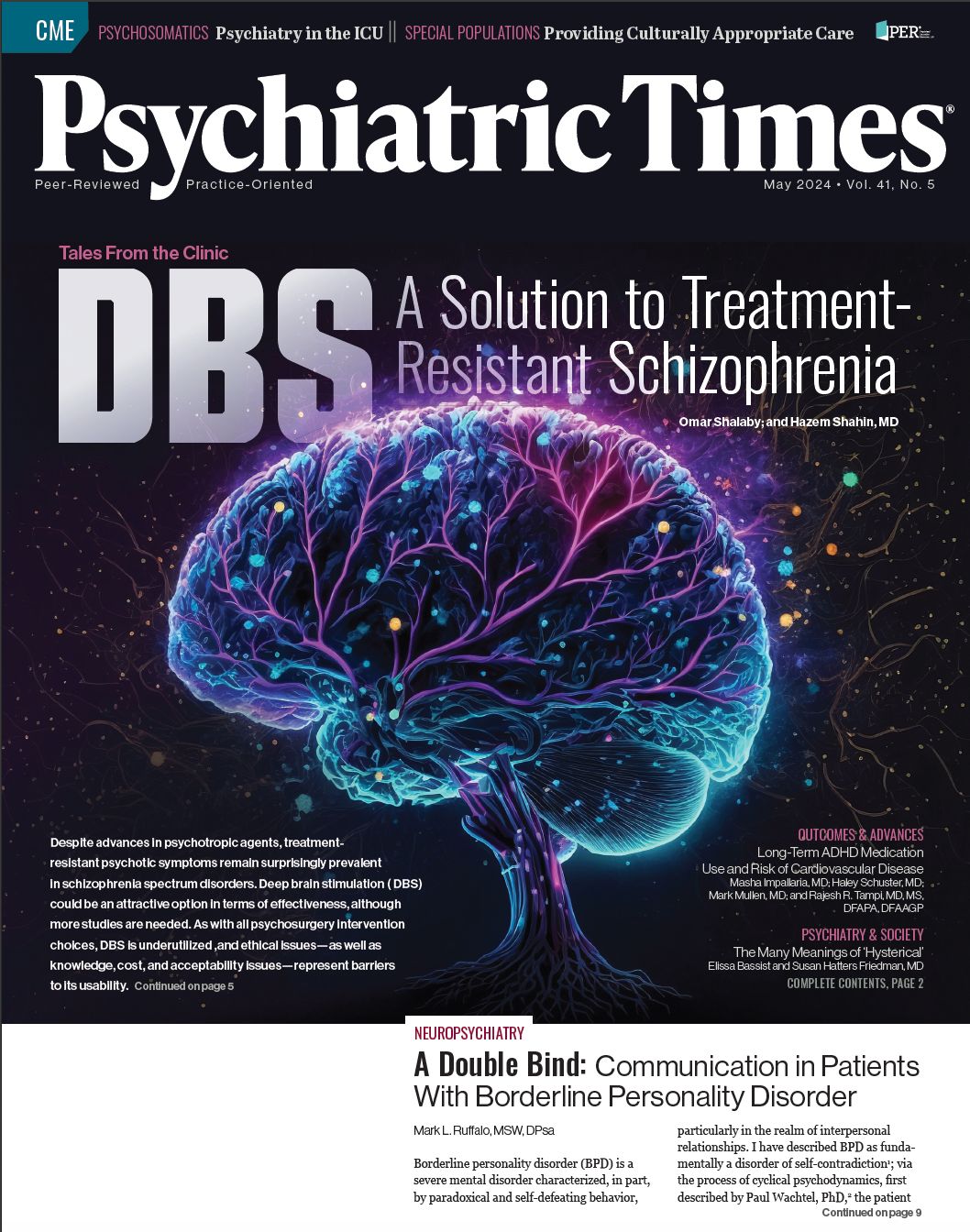
An Update on GLP-1 Receptor Agonists as Pharmacotherapies for AUD
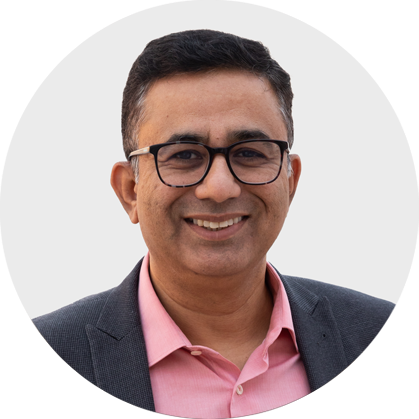
Here's to a Psychedelic Revolution
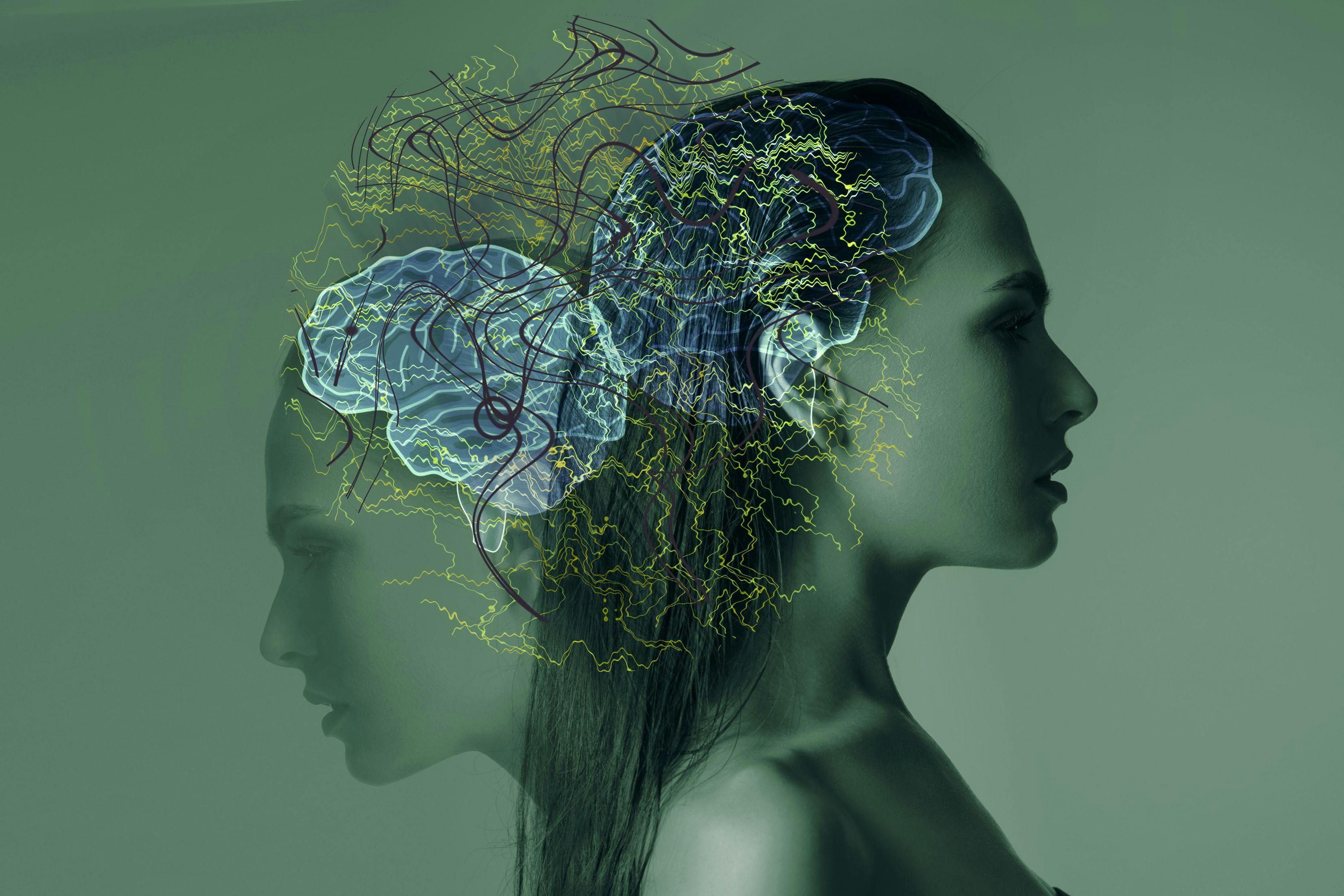
Evaluating the Efficacy of Lumateperone for MDD and Bipolar Depression With Mixed Features
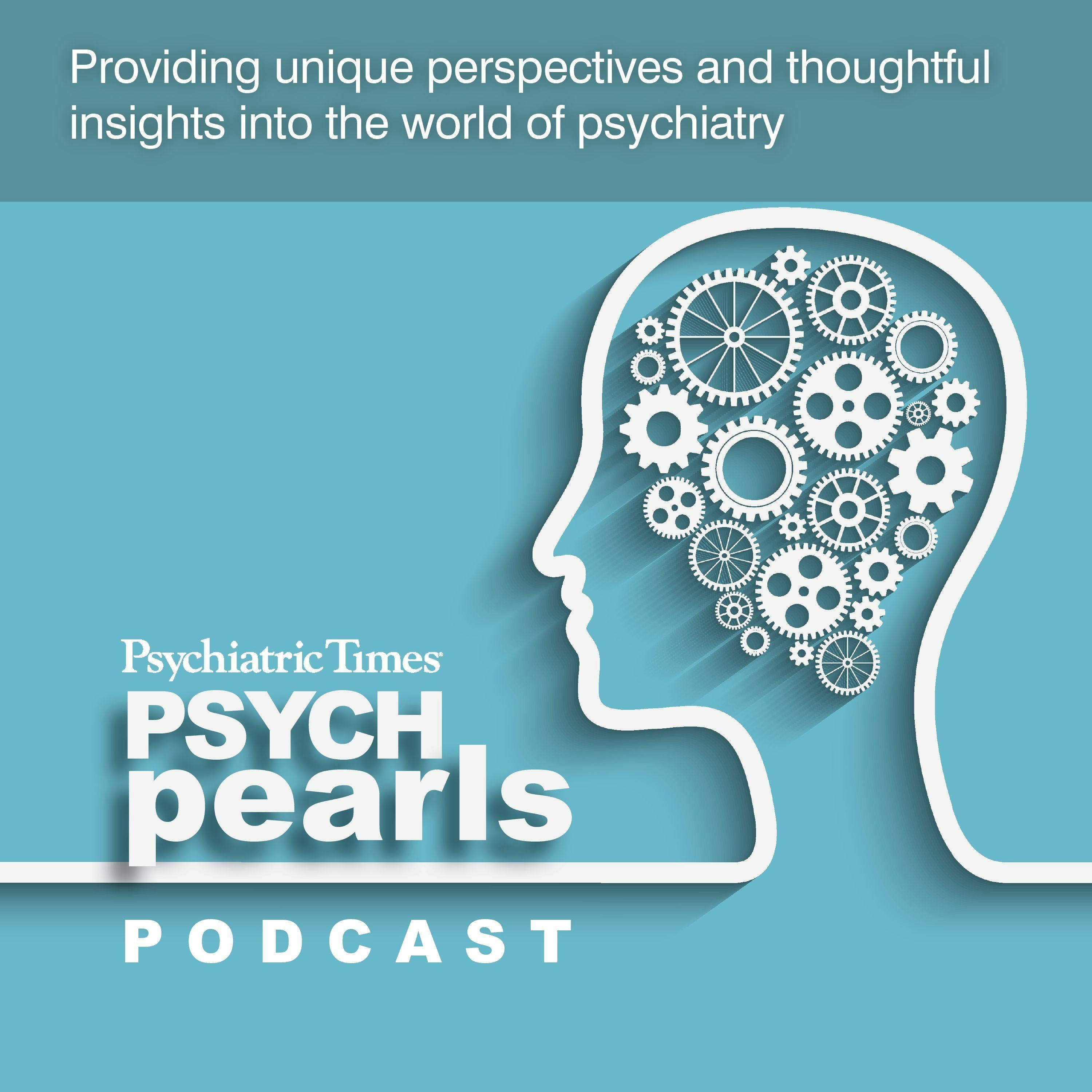
Four Myths About Lamotrigine
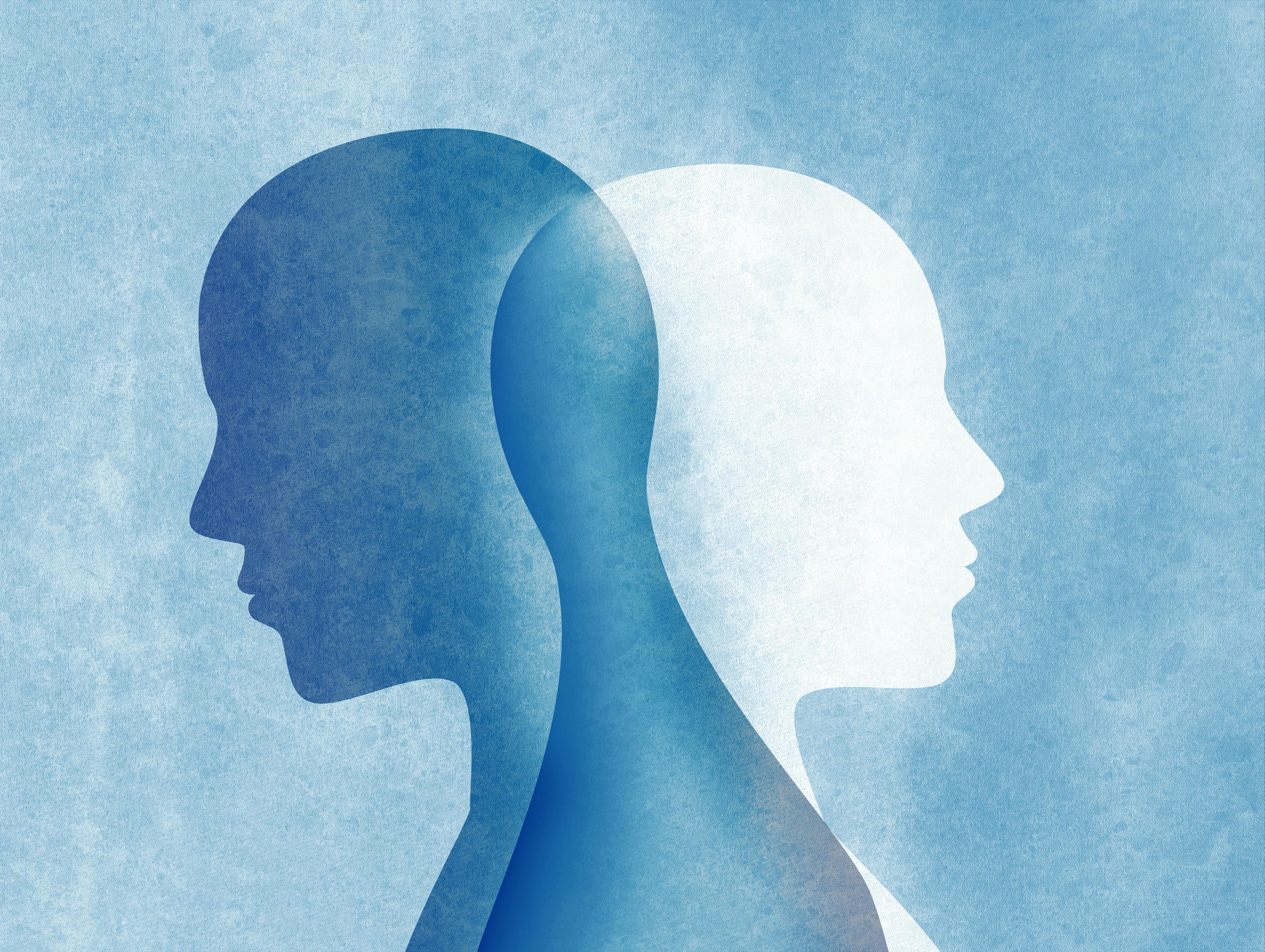
Early Improvement of Symptoms in Bipolar 1 Depression
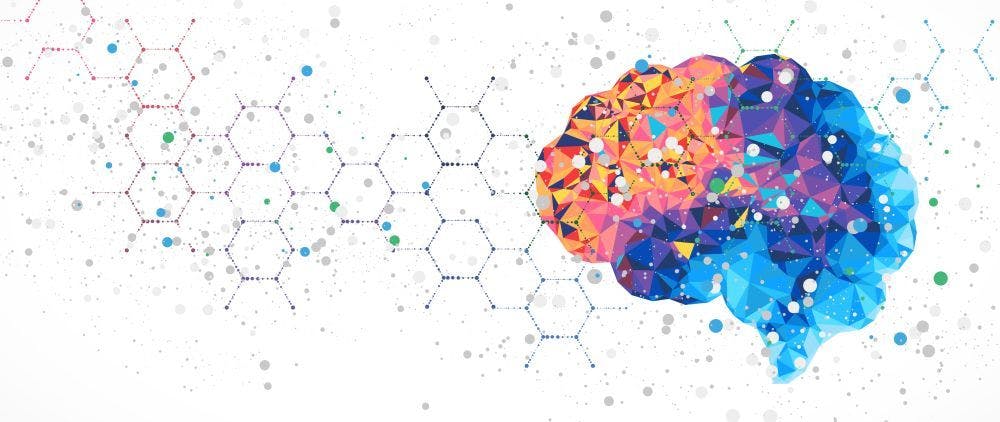
Pseudospecific and Transdiagnostic Symptom Targeting in Clinical Trials
2 Commerce Drive Cranbury, NJ 08512
609-716-7777

- Open access
- Published: 03 June 2024
Temporal composition of the cervicovaginal microbiome associates with hrHPV infection outcomes in a longitudinal study
- Mariano A. Molina 1 , 2 ,
- William P. J. Leenders 3 ,
- Martijn A. Huynen 4 ,
- Willem J. G. Melchers 1 &
- Karolina M. Andralojc 1
BMC Infectious Diseases volume 24 , Article number: 552 ( 2024 ) Cite this article
51 Accesses
2 Altmetric
Metrics details
Persistent infections with high-risk human papillomavirus (hrHPV) can cause cervical squamous intraepithelial lesions (SIL) that may progress to cancer. The cervicovaginal microbiome (CVM) correlates with SIL, but the temporal composition of the CVM after hrHPV infections has not been fully clarified.
To determine the association between the CVM composition and infection outcome, we applied high-resolution microbiome profiling using the circular probe-based RNA sequencing technology on a longitudinal cohort of cervical smears obtained from 141 hrHPV DNA-positive women with normal cytology at first visit, of whom 51 were diagnosed by cytology with SIL six months later.
Here we show that women with a microbial community characterized by low diversity and high Lactobacillus crispatus abundance at both visits exhibit low risk to SIL development, while women with a microbial community characterized by high diversity and Lactobacillus depletion at first visit have a higher risk of developing SIL. At the level of individual species, we observed that a high abundance for Gardnerella vaginalis and Atopobium vaginae at both visits associate with SIL outcomes. These species together with Dialister micraerophilus showed a moderate discriminatory power for hrHPV infection progression.
Conclusions
Our results suggest that the CVM can potentially be used as a biomarker for cervical disease and SIL development after hrHPV infection diagnosis with implications on cervical cancer prevention strategies and treatment of SIL.
Peer Review reports
High-risk human papillomavirus (hrHPV) infections are associated with premalignant cervical lesions that may progress to cervical cancer [ 1 ]. Around 80% of all sexually active women will acquire an HPV infection during their lives and in most of the cases the virus is spontaneously cleared by the host immune system [ 2 , 3 ]. In some women, however, hrHPV evades the immune response and the infection becomes persistent, promoting the development of squamous intraepithelial lesions (SIL) that eventually can progress to invasive cervical cancer [ 4 , 5 ]. Despite increased use of HPV-vaccines to prevent hrHPV infection, cervical cancer represents a huge public health burden worldwide with over 500,000 diagnoses and over 300,000 deaths yearly [ 6 ]. Current screening programs include hrHPV DNA testing followed by cytology triage (Pap test). Overall, the clinical specificity of screening is low, resulting in high rates of overdiagnosis and overtreatment, and stratification of women who are at risk of hrHPV-induced cancer remains a challenge [ 7 ]. Thus, there is a remaining need to better understand the cervicovaginal ecosystem, and to discover and apply effective predictive biomarkers for early detection and treatment of SIL.
The cervicovaginal microbiome (CVM) is a promising candidate biomarker for cervical disease behavior [ 8 , 9 ]. Changes in the composition of the cervicovaginal microbiota have been associated with bacterial vaginosis (BV), pre-term birth, and viral infections caused by HIV and hrHPV [ 10 , 11 , 12 ]. The CVM is structured in microbial community state types (CSTs) in which specific bacterial species dominate the microbiome or assemble in a diverse microbial population. In a healthy cervix, the CVM is characterized by dominance of Lactobacillus species such as Lactobacillus crispatus (CST I), while depletion of Lactobacillus species and colonization by Gardnerella vaginalis , Atopobium vaginae , and Megasphaera genomosp type 1 (CST IV) is typical of dysbiosis [ 13 , 14 ]. CST IV has been associated with hrHPV infection, viral persistence, viral-induced cervical lesions, and cervical cancer [ 15 ]. In contrast, Lactobacillus -dominated microbiomes have been correlated with hrHPV clearance and disease regression [ 15 ]. Most of these observations have been described in cross-sectional studies, and since the CVM is a highly dynamic ecological environment [ 16 ], a thorough understanding of how the microbiome changes in the course of hrHPV infection to SIL requires longitudinal microbiome profiling studies.
Evaluating the cervicovaginal microbiota’s role in health and disease mainly relies on 16S rRNA gene sequencing (16S RNA-seq) methods [ 17 , 18 ]. Using 16S RNA-seq, Oh HY et al. described an association of microbial communities and species with SIL development in hrHPV-positive women [ 19 ]. Nevertheless, 16S RNA-seq yields only genus-resolution microbiome profiling for many taxa and provides limited species information due to the complexity of the variable regions (VRs) in the 16S rRNA gene [ 18 ]. Species-level microbiome profiling can be achieved by shotgun metagenomics or circular probe-based RNA sequencing (ciRNAseq) techniques [ 20 ]. Using shotgun metagenomics, Yan Q et al. found high abundance of G. vaginalis in HPV16-positive women [ 21 ]. However, shotgun metagenomics is relatively expensive, and it requires specialized resources for data analyses [ 22 ]. The ciRNAseq technology employs single-molecule molecular inversion probes (smMIPs) to target conserved DNA and RNA sequences in the 16S and 23S rRNA genes of microbial species within the CVM. ciRNAseq exhibits high specificity and sensitivity in identifying microbial species in mock community samples and women’s cervical smears [ 20 ]. Likewise, ciRNAseq provides improved taxonomic resolution compared to 16S RNA-seq, which is critical for the study of the CVM in hrHPV infections. Furthermore, by employing unique molecule identifiers (UMIs) the technique yields quantitative information irrespective of PCR-amplification bias [ 20 ]. Through ciRNAseq profiling of the CVM, our group has previously defined associations of the CVM with hrHPV-negative conditions and hrHPV-induced high-grade squamous intraepithelial lesions (HSIL) [ 20 ]. More recently, we identified subgroups of CSTs based on the abundance of bacterial species commonly overlooked by conventional sequencing methods due to their high level of sequence identity with other species [ 14 , 23 , 24 ]. Nonetheless, the temporal associations of these microbial communities and species with hrHPV infection outcomes are unknown.
In this longitudinal study, we investigate the composition of the CVM in a cohort of Dutch women participating in the population-based cervical cancer screening program, with proven hrHPV infection but normal cytology at baseline, who were diagnosed with SIL six-months later or did not develop cervical abnormalities. Our study aimed to evaluate the composition and temporal changes in the microbiome in relation to hrHPV progression in a 6-month period to identify potential early microbiome signatures associated cervical disease development after an hrHPV infection diagnosis. We show that an initial CST IV-A and high G. vaginalis or A. vaginae abundance associate with a progressive infection outcome at six-months, while L. crispatus dominance at both visits associates with non-progression. In addition to CSTs, we describe a combination of microbial species associated with hrHPV outcomes at both visits and relationships between bacteria occurring in the CVM. Our results suggest that the CVM is a valuable biomarker for hrHPV infection progression.
Study subjects and inclusion criteria
A total of 141 women participating in the Dutch population-based cervical cancer screening program and diagnosed with hrHPV infection and cytologically characterized as negative for intraepithelial lesion or malignancy (NILM) were included in the study. Women participating in the screening program were informed that residual material could be used for anonymous research and had the opportunity to opt out. Exclusion criteria included an hrHPV negative test result or diagnosis of squamous intraepithelial lesions (SIL) at first visit. Women without a follow-up sample were also excluded. Women were included irrespective of their ethnicity, parity, smoking habits, phase in their cycle, and use of contraception. At first visit (V1, time = 0 months) and second visit (V2, time = 6 months), 141 cervical smears in PreservCyt were included in the study and were processed and sequenced for microbiome profiling [ 20 ]. Five milliliters of each cervical cell suspension were centrifuged for 5 min at 2500 × g, and the pellet dissolved in 1 ml of TRIzol reagent (Thermo Scientific). RNA was isolated through standard procedures and dissolved in 20 μl nuclease-free water. We routinely processed a maximum of 2 μg of RNA for DNase treatment and cDNA generation, using SuperscriptII (Thermo). At V1, all women had sufficient RNA material for further processing. From the women with microbiome profiling at V2, a total of 83 cervical smears (58.8%) had sufficient material available for hrHPV DNA testing. The cytological follow-up outcomes at V2 were obtained for all participating women from the nationwide network and registry of histo- and cytopathology in the Netherlands (PALGA; Houten, The Netherlands). In this study we used liquid-based cytology (LBC) data according to the Bethesda coding system with categories NILM, low-grade squamous intraepithelial lesion (LSIL), and high-grade squamous intraepithelial lesion (HSIL).
HrHPV identification and genotyping
HrHPV testing was performed with the Roche Cobas 4800 test, according to the manufacturer’s recommendations in the Department of Medical Microbiology at Radboudumc [ 25 ].
CiRNAseq microbiome profiling and output analyses
High-resolution microbiome profiling was performed on ~ 50 ng of cDNA using the ciRNAseq technology [ 20 , 26 ]. Probes (smMIPs) designed and selected to bind to framework regions flanking VRs in the 16S and 23S rRNA genes of microbial species [ 20 ] in the CVM were mixed with cDNA in a capture hybridization reaction and were circularized via a combined primer extension and ligation reaction. Circularized probes were subjected to PCR with barcoded Illumina primers. After purification of correct-size amplicons, quality control, and quantification [ 27 ], a 4 nM library was sequenced on the Illumina Nextseq500 platform (Illumina, San Diego, CA) at the Radboudumc sequencing facility to produce 2 × 151 bp paired-end reads.
Reads were mapped against reference regions of interest (ROIs) in our Cervicovaginal Microbiome Panel containing 321 microbial species[ 20 ] using the SeqNext module of JSI Sequence Pilot version 4.2.2 build 502 (JSI Medical Systems, Ettenheim, Germany). Our microbiome panel and ROIs were designed based on the most relevant species in the CVM and validated as previously described [ 20 ]. The settings for read processing were a minimum of 50% matching bases, a maximum of 15% mismatches, and a minimum of 50% consecutive bases without a mismatch between them; for read assignment, the threshold was a minimum of 95% of identical bases within the ROIs [ 20 ]. All identical PCR products were reduced to one consensus read (unique read counts, URC) using unique molecular identifiers (UMI), which consisted of a random 8-nucleotide sequence flanking the ligation probe in the smMIP and which is co-amplified during PCR. All FASTQs with identical (UMI) sequences therefore originate from the same circularized smMIP, allowing decomplexing of these sequences and making the assay insensitive of amplification bias. We set an arbitrary threshold of at least 1000 unique read counts (URC) from all smMIPs combined in an individual sample, below which we considered an output non-interpretable. Using a custom R script, microbial species were annotated when at least two reactive smMIPs for that species had URC. To define relative abundances, microbial species URC was divided by the total URC of all microbes annotated in the sample [ 20 ].
Microbiome assessment and analyses
Hierarchical clustering (HC) and Partial least-squares discriminant analysis (PLSDA) were performed using ClustVis and MetaboAnalyst, respectively [ 28 , 29 ]. The settings for HC were as follows: clustering distance for columns: Manhattan; clustering method: Ward. CSTs designation was performed through unsupervised clustering analyses [ 24 ]. CSTs were classified into five major groups (I to V) and the subgroups of CSTs I, III, and IV [ 14 , 24 ] based on microbiome composition.
The predictive diagnostic potential of A. vaginae , G. vaginalis , D. micraerophilus , and L. crispatus for distinguishing non-progressive and progressive women at V1 were evaluated by a Random Forest analysis followed by receiver operating characteristic (ROC) curves of the bacterial species markers, and results were quantified by the area under the curve (AUC) using the randomForest [ 30 ] and pROC [ 31 ] R packages.
SankeyMATIC software was utilized to visualize the temporal changes in microbiomes. Pearson’s r partial correlations between microbial species were determined and generated with the ppcor R package [ 32 ]. The microbiome variation in the six-month period within a woman was obtained through a Jensen-Shannon distance (JSD) calculation in the philentropy R package [ 33 ]. JSD values give a measure of similarity between samples (i.e., by calculating the distance between samples) from the same woman. Low JSD values indicate similar microbial communities between samples, and conversely, large values indicate less similar communities.
Statistical analysis
GraphPad Prism v9.4.0 (GraphPad Software, Inc., USA) was used to analyze datasets and determine the Shannon’s diversity indices and odds ratios. The statistical significance of differences was calculated using the Kruskal–Wallis test for multiple comparisons followed by a Benjamini–Hochberg test correction. Mann–Whitney U and Wilcoxon rank tests were employed for single and paired analyses, respectively. A McNemar’s test with a continuity correction was applied for matched-pairs analyses between both visits.
Study design and hrHPV infection outcomes
Cervical smears from 141 women with DNA-confirmed hrHPV infection and a cytological diagnosis of negative for intraepithelial lesion or malignancy (NILM) were profiled for CVM at first visit (V1). Of these, 90 women also had a diagnosis NILM at 6 months (63.8%) (non-progression group, NP) while 51 women (36.2%) were diagnosed with low-grade squamous intraepithelial lesions (LSIL) (41/51, 80.4%) or HSIL (10/51, 19.6%) (progression group, P) (Fig. 1 ).
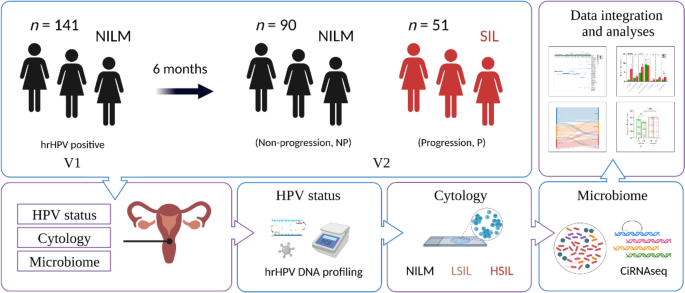
Study design. All 141 women entered the study at baseline with DNA confirmed hrHPV infection, no cervical abnormalities and CVM profiling. The results of follow-up cytology were assessed at 6 months to determine whether the individual had progressed to intraepithelial lesion or malignancy (LSIL, HSIL) or not (NILM). By 6 months, 90 women were confirmed for NILM, and 51 women had a LSIL ( n = 41) or HSIL ( n = 10) diagnosis. Experimental procedures, analysis, and integration were carried out as described in Methods
Early microbiome composition and hrHPV infection outcomes
Through unsupervised cluster analysis, we characterized the composition of the CVM in our longitudinal cohort at baseline ( n = 141) and determined their association with cytological outcomes at six-months. Microbiomes clustered in CSTs dominated by Lactobacillus species: clusters I, III, and V (Fig. 2 a, left clusters), and CSTs with a high diversity: clusters II and IV (Fig. 2 a, right clusters, and Additional File 1 : Supplementary Figure 1), including the subgroups of CSTs I (I-A, I-B), III (III-A, III-B) and IV (IV-A, IV-B, and IV-C) [ 24 ]. We did not observe a significant association between the overall baseline Lactobacillus -dominated (LDO, CSTs I, II, III, and V combined) and Lactobacillus -depleted (LDE, CSTs IV combined) microbiomes with hrHPV infection outcomes at V2 (Fig. 2 a-b). Nevertheless, we see a clear trend where, of the CST types, CST I-A at baseline was most strongly associated with NILM at six-months (26/32, 81.2%, OR 0.32, 95% CI 0.12–0.82, p = 0.03, q = 0.15, Fisher’s exact test), while CST IV-A at baseline was most strongly associated with SIL outcomes at six-months (9/15, 60%, OR 3.07, 95% CI 1.03–9.40, p = 0.04, q = 0.16), however, the associations were only moderate when corrected for multiple testing (FDR < 0.2) (Fig. 2 a-b).
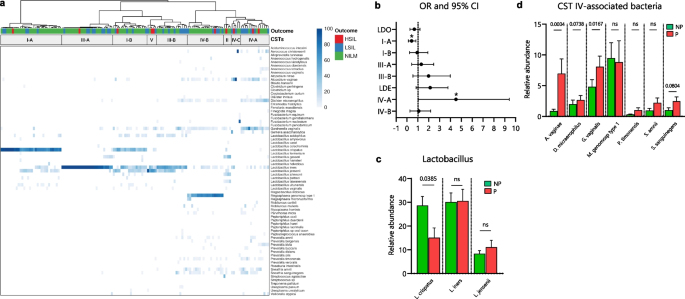
Early cervicovaginal microbiome composition is associated with hrHPV infection outcomes at six-months. a Cluster analysis of species-level profiling of the cervicovaginal microbiota at first collection visit (V1). Visualization of the distribution of hrHPV infection outcomes based on clusters show enrichment of NILM and SIL outcomes in specific communities. b Odd ratios (OR) and 95% confidence intervals comparing baseline CST groups (LDO: I, II, III, and V; LDE: IV) and individual CST subgroups for hrHPV infection progression at six-months. c Analyses of the relative abundances of Lactobacillus species in the CVM at V1 demonstrate association of L. crispatus with non-progression. d Analyses of the relative abundances of pathogenic anaerobes and the infection outcomes at six-months. OR in b were analyzed through a Fisher’s exact test, * p < 0.05. Differences in relative abundances were analyzed by using a Kruskal–Wallis test followed by the Benjamini–Hochberg test correction for multiple comparisons. q values are shown in c and d and error bars represent standard error of the mean ± s.e.m. q values < 0.10 are considered significant, ns = not significant. NP = non-progression group; P = progression group; LDO = Lactobacillus -dominated; LDE = Lactobacillus -depleted
To further explore the association of the CVM with progression to SIL, we examined it at level of individual bacterial species. We observed a significantly increased abundance of L. crispatus ( q = 0.03, Kruskal Wallis test) in the NP group when compared to the P group (Fig. 2 c). Moreover, we noticed that A. vaginae ( q = 0.0004, Kruskal Wallis test), G. vaginalis ( q = 0.01), D. micraerophilus ( q = 0.07) and S. sanguinegens ( q = 0.08), which are typical species found in CST IV, were more abundant in the P group than in the NP group (Fig. 2 d).
Dynamics of the microbiome and hrHPV infection outcomes
Six months after the initial diagnosis of hrHPV infection (V2), we again performed microbiome profiling on the cervical smears from all participating women ( n = 141). We then established their CVM composition and examined the microbial changes between both visits and their association with hrHPV infection outcomes (Additional File 2 : Supplementary Figure 2). Although we did not observe a significant association between CST subgroups and hrHPV infection outcomes at V2 like we observed at V1 (Fig. 2 , Additional File 2 : Supplementary Figure 2), we found that, LDE CSTs (IV) correlated with SIL (OR 2.21, 95% CI 1.02–4.45, p = 0.03, Fisher’s exact test), while LDO (I, II, III, and V) were associated with NILM (OR 0.45, 95% CI 0.22–0.97) (Fig. 3 b).
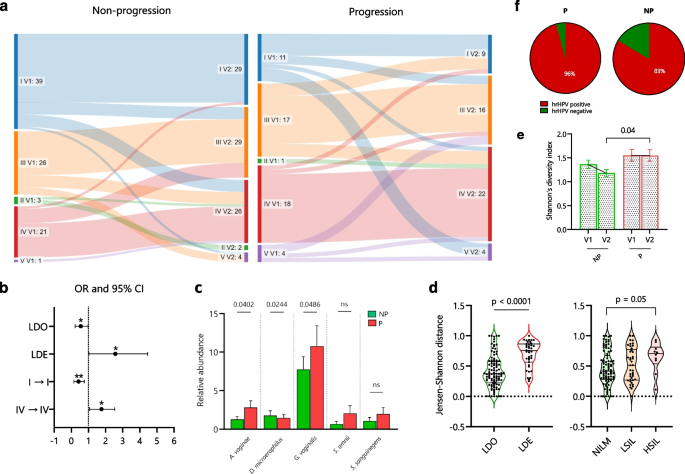
Dynamics of the microbiome and hrHPV infection outcomes. a The microbial shifts between both visits and groups. b Odd ratios (OR) and 95% confidence intervals comparing CST groups (LDO: I, II, III, and V; LDE: IV) at V2 and the six-months stability of CSTs I and IV with hrHPV infection outcomes. c Similarity of the CVM composition per microbial community and infection outcome through the Jensen-Shannon distance (JSD). d Comparison of relative abundances of the most abundant bacterial species associated with LDE microbiomes between NP and P groups. e Comparison of Shannon’s diversity indices for all microbiomes in NP and P groups at both visits. f Analysis on the hrHPV status in a subcohort of 83 women with hrHPV DNA testing at V2. OR in b were analyzed through a Fisher’s exact test, * p < 0.05, ** p < 0.01. Differences in relative abundances, JSD per outcomes, and Shannon indices per group, were analyzed by using a Kruskal–Wallis test followed by the Benjamini–Hochberg test correction for multiple comparisons. q values are shown in d and e and error bars represent standard error of the mean ± s.e.m. q values < 0.10 are considered significant, ns = not significant. Differences in JSD by Lactobacillus composition and paired Shannon indices were analyzed by using a Mann–Whitney U test and Wilcoxon matched-pairs test, respectively. NP = non-progression group; P = progression group; LDO = Lactobacillus -dominated; LDE = Lactobacillus -depleted
We did not find a significant association between the CSTs II, III, IV, and V with microbiome stability in both groups (Fig. 3 a). Nonetheless, compared to these CSTs in the NP group, CST I was significantly associated with a stable composition between both visits ( p = 0.01, McNemar’s test) (Fig. 3 a). Moreover, when analyzing the association between the six-months stability of CSTs with the hrHPV outcomes, we found that compared to other CSTs, stable CST I (OR 0.24, 95% CI 0.09–0.64, p = 0.003, Fisher’s exact test) and CST IV (OR 2.48, 95% CI 1.12–5.66, p = 0.03) were significantly associated with non-progression and progression, respectively (Fig. 3 b).
Next, we examined the association of the CVM composition at V2 with the infection outcomes by comparing the relative abundances of pathogenic anaerobic species between both groups. Interestingly, we observed that the species D. micraerophilus ( q = 0.02, Kruskal Wallis test) was more abundant in women with NILM than in women with SIL (Fig. 3 c). Alternatively, we found that A. vaginae ( q = 0.04), G. vaginalis ( q = 0.04), Prevotella bivia ( q = 0.008) and Prevotella buccalis ( q = 0.008) were more abundant in the SIL group than in the NILM group (Fig. 3 c and Additional File 3 : Supplementary Figure 3).
To further evaluate the association of the temporal CVM composition with hrHPV infection outcomes, we calculated the Jensen-Shannon distance (JSD) [ 33 ] of the microbiome composition between both visits. A low JSD indicates a high similarity in microbial composition between the timepoints. We observed that women with LDO at baseline had a significantly more similar microbiome at V2 than women with LDE at baseline ( p < 0.0001, Mann–Whitney U test) (Fig. 3 d). Conversely, women with NILM outcomes had a more similar microbiome composition between both visits than those who developed HSIL ( p = 0.05, q = 0.17, Kruskal Wallis test) (Fig. 3 d).
We then assessed the Shannon’s diversity index and observed that women in both NP and P groups did not exhibit a significant change in microbial diversity from V1 to V2 (Wilcoxon matched-pairs test) (Fig. 3 e). Nevertheless, women in the NP group had a significantly lower microbial diversity than the P group at V2 ( q = 0.04, Kruskal Wallis test) (Fig. 3 e). To test whether these microbial dynamics were associated with hrHPV persistence, we analyzed the hrHPV status for a subcohort of cervical smears with available hrHPV DNA testing at V2 ( n = 83) and noticed that there were more hrHPV positive cervical smears in women with SIL (23/24, 96%) than women with NILM (49/59, 83%) (OR 4.69, 95% CI 0.68–53.04, p = 0.16, Fisher’s exact test) (Fig. 3 f). Altogether, these findings demonstrate that prolonged Lactobacillus depletion, high microbial diversity, and increased abundance of CST IV-associated bacteria correlate with SIL. Conversely, a stable microbiome composition characterized by Lactobacillus dominance and low microbial diversity over a six-months period correlates with non-progression.
Microbiome-based prediction of hrHPV infection outcomes
Aside from estimating species that most significantly associate with the NP and P groups, we aimed to determine to what extent a combination of species associated with each group at both visits and whether such combination at V1 could be used to predict hrHPV infection outcomes at V2. To this purpose, we first performed a Partial least-squares discriminant analysis (PLSDA) with all microbiomes collected at V1 and V2 ( n = 141) (Fig. 4 a). We determined that L. crispatus , A. vaginae , D. micraerophilus , and G. vaginalis showed the strongest correlations with PLSDA Component 1 at both visits (Additional Files 4–5). Analyses of the Variable Importance in Projection (VIP) scores, a weighted sum of squares of the PLS loadings, showed consistent microbial species separating both groups in PLSDA C1 and the relative abundance associated with each group (Additional File 6 : Supplementary Figure 4).
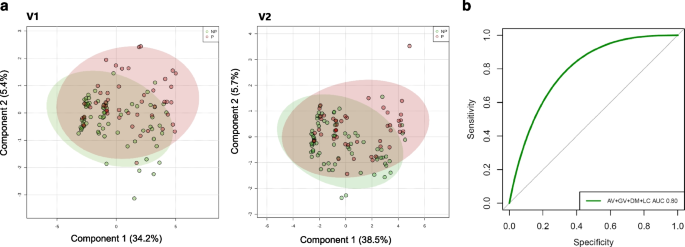
Cervicovaginal microbial species associated with hrHPV infection outcomes. a Partial least-squares discriminant analysis (PLSDA) of women’s CVM ( n = 141) shows a similar separation between NP and P groups at both V1 and V2. b Receiver operating characteristics (ROC) curve and AUC of A. vaginae (AV), G. vaginalis (GV), D. micraerophilus (DM), and L. crispatus (LC) abundances in the microbiome from 141 hrHPV-positive women at V1 were calculated to generate a predictive model for the infection outcomes at six-months. These species combined exhibit a model with an AUC of 0.80 (95% CI 0.64–0.96)
Next, we performed a Random Forest analysis and ROC to assess the performance of the early abundances in the CVM of the species L. crispatus , A. vaginae , D. micraerophilus , and G. vaginalis , which exhibited the strongest associations in our PLSDA (Fig. 4 a), in predicting hrHPV outcomes at six-months. We found that these species together had a moderate discriminatory power at baseline for hrHPV infection progression at V2 with an AUC of 0.80 (95% CI 0.64–0.96) (Fig. 4 b).
Correlations among the cervicovaginal microbiota in hrHPV infections
Lastly, to assess whether relationships between bacterial species were observed and persisted in non-progressive and progressive microbiomes, we performed Pearson’s partial r correlation analyses considering the most abundant species in both NP and P groups. We analyzed the microbial species abundances by integrating the two timepoints datasets to establish the correlations that persisted throughout both visits. In the NP group, there was a positive correlation between A. vaginae and D. micraerophilus and G. vaginalis . In the P group, there were inverse relationships between Lactobacillus and A. vaginae , between M. genomosp type 1 and other pathogenic bacteria, and between Prevotella species (Fig. 5 ). Alternatively, we observed significant positive correlations between D. micraerophilus and Prevotella species and between Prevotella and Sneathia species in the P group (Fig. 5 ). In both groups, there was a negative correlation between Lactobacillus and G. vaginalis and M. genomosp type 1 (Fig. 5 ). Likewise, both groups exhibited a significantly positive correlation between D. micraerophilus and P. bivia (Fig. 5 ). In conclusion, associations between Lactobacillus and pathogenic anaerobes, and between pathogenic anaerobes themselves, appear typical in the CVM during hrHPV infections.
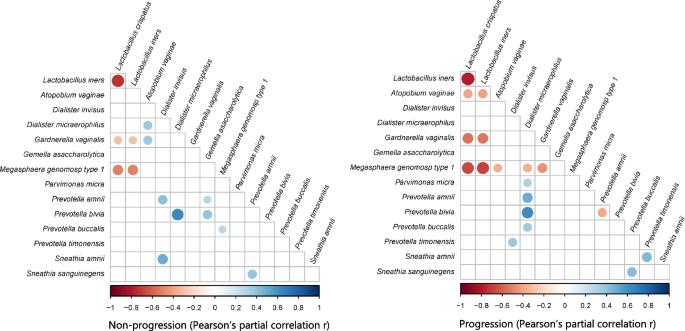
Interdependent relationships between the cervicovaginal microbiota in hrHPV infection. Pearson’s r partial correlations for multiple comparisons were estimated with the most abundant bacterial species in the microbiome of women in NP and P groups and integrating V1 and V2 datasets ( n = 141). Color, size, and shade indicate the extent of positive and negative correlations. Correlation significance: p < 0.001
The composition of the CVM not only correlates with hrHPV infections and cervical disease, but it may also predict the infection outcome. In this study, we observed that following hrHPV infection diagnosis, women with the microbial community IV-A [ 14 , 24 ], characterized by G. vaginalis dominance and co-occurrence with D. micraerophilus , A. vaginae , S. amnii , and S. sanguinegens associate with progression to SIL six-months later. Pathogenic anaerobes such as G. vaginalis have been associated with viral persistence and cervical lesions [ 14 , 34 , 35 ]. Our findings are in line with previous longitudinal studies that established an association between bacterial vaginosis (BV) and the species G. vaginalis with cervical neoplastic lesions [ 34 , 36 ]. Notably, we found that A. vaginae abundance at both visits is associated with infection progression and SIL at second visit. Increased A. vaginae abundance in the microbiome has been reported as a hallmark of SIL development [ 37 , 38 ]. A. vaginae induces cytotoxic immune responses in cervicovaginal epithelial cells that reduces the protective mucosal layer, which might facilitate hrHPV persistence and integration into host cells [ 39 , 40 ]. Thus, since these bacteria are highly abundant in hrHPV progressive infections and SIL, they could potentially be applied as biomarkers for cervical carcinogenesis. Moreover, these pathogenic species may represent promising targets for microbiome-based therapies against the development of cervical cancer.
Microbiomes abundant in Lactobacillus are associated with cervical health and their depletion results in cervical disorders [ 9 , 37 , 41 , 42 ]. Lactobacillus species create a favorable microenvironment that allows sustained presence of lactate-producing bacteria and prevent outgrowth of harmful bacteria such as G. vaginalis [ 43 , 44 ]. By this mechanism, Lactobacillus species may therefore prevent dysbiosis and persistent hrHPV infections [ 14 , 45 ]. Additionally, microbial dominance by L. crispatus (CST I), L. gasseri (CST II), or L. jensenii (CST V) has been associated with hrHPV negative conditions, viral clearance, and regression of cervical lesions [ 46 ]. Although we did not assess this relationship in CSTs II and V due to the low prevalence of these CSTs in this study, these Lactobacillus species are known to stimulate a non-inflammatory state in the cervical epithelium, which facilitates effective immune responses against hrHPV infections and carcinogenesis [ 47 ]. Similarly, we described that LDO microbiomes have a more stable composition than LDE microbiomes. Since non-progressive women have microbial communities richer in Lactobacillus species than progressive women, this may explain the protection against hrHPV progression observed in our study [ 16 , 48 ].
Aside from disease development, the microbiome dynamics also rely in the interactions of the cervicovaginal microbiota with the virus and the microenvironment [ 36 , 49 ]. In our study, we describe that Lactobacillus species exhibit a strong negative relationship with CST IV-associated bacteria, which can be explained by the ecological conditions where they grow and their antimicrobial activities against pathogenic bacteria [ 50 , 51 ]. Interestingly, D. micraerophilus showed strong positive associations with several Prevotella species in women with SIL outcomes, and in particular, a strong relationship with P. bivia was observed in both NP and P groups. Prevotella species often co-occur with D. micraerophilus and other pathogenic anaerobes in the CVM, and it is a clear example of how microbial species can associate with each other synergistically in the cervicovaginal environment [ 52 ]. P. bivia is an important source of ammonia and sialidase in the vaginal mucus and has been associated with cervical disease, which may explain its occurrence during infection [ 52 ]. Similarly, we observed that P. timonensis positively correlated with S. amnii in women with SIL outcomes (Fig. 5 ). P. timonensis interacts with vaginal dendritic cells, which are involved in mucosal inflammation [ 53 , 54 ], and both species have been associated with viral persistence, slower regression of SIL, and cervical cancer [ 46 , 55 ]. Sneathia and Prevotella species also express several homologous genes that are enriched in CST IV and that allow them to consume glycogen and mucins from cervical cells [ 56 ]. It could be hypothesized that S. amnii or P. timonensis may facilitate each other’s colonization, contributing to the risk of neoplastic lesions in hrHPV infection. This hypothesis is also consistent with the hrHPV downregulation of immune peptides that act as amino acid sources for Lactobacillus species, which leads to the growth of CST IV-associated bacteria in the CVM [ 57 ]. In general, the microbial dynamics during hrHPV infections remain interesting markers for infection behavior, but further studies are clearly needed to validate these associations in vitro and in vivo.
The strengths of the study are the use of the ciRNAseq technology for targeted sequencing of the microbiome and the application of longitudinal profiling in our study cohort. Potential limitations may include a short study period (six months) and a relatively small cohort size. Whereas the short study period may not be sufficient to capture the full spectrum of microbiome dynamics and their potential impact on disease progression, the cohort size may limit the statistical power of the study, making it challenging to detect subtle but potentially important associations. Furthermore, high-grade cases might have been missed during the visits due to the relatively low sensitivity of cervical cytology and the lack of histopathological data to further support the cytological analysis. Of note, although we included women with LSIL outcomes in our progression group, LSIL are considered non-progressive lesions [ 58 ], and therefore the microbiome associations described here should be considered carefully when investigating outcomes beyond six-months. Women with LSIL, however, developed these cervical abnormalities at V2 from diagnosed NILM at V1, which is defined as hrHPV infection progression. We were also unable to control for other cofounders, such as lifesytle, phase of the menstrual cycle, and antibiotic use during the study, which are known co-factors that impact on the CVM composition [ 59 ]. For instance, variations in hormone levels throughout the menstrual cycle can alter the CVM, and antibiotic use can disrupt microbial communities, potentially masking or exaggerating associations with disease outcomes. Therefore, a larger cohort and longitudinal clinical studies will be needed to validate our findings.
In summary, we have shown how bacterial species, communities, dynamics, and relationships are relevant for assessing the role of the CVM in hrHPV carcinogenesis. This way, the CVM could be employed to support current cervical cancer prevention strategies and therapies against cervical lesions. Nonetheless, more studies and clinical trials are needed to properly assess and translate these findings in the clinic. Additionally, even though CSTs correlate with the infection outcome, their usefulness as biomarkers for cervical disease is not clear yet. Our in-depth analyses suggest species like A. vaginae , G. vaginalis , L. crispatus , and D. micraerophilus exhibiting strong associations with cervical conditions, and clustering species into CSTs does not necessarily result in better biomarkers than just examining the presence of a few species [ 60 ]. Further supervised analyses like Random Forest integrating host cell gene expression [ 61 ] to the microbiome data would be valuable to obtain a combination of biomarkers for disease progression, and such studies are on the way. Since ciRNAseq can provide bacterial information on DNA and RNA levels [ 20 ], while simultaneously perform transcriptome profiling [ 61 ], it could be applied to better understand the relationship of the microbiome with hrHPV infections.
Availability of data and materials
The sequence read data generated in this study are available at NCBI in the Sequencing Read Archive, projects PRJNA856437 (174 files) [ 62 ] and PRJNA888791 (108 files) [ 63 ] (Sample Accession Numbers are shown in Additional File 7 ).
Abbreviations
Area under the curve
Bacterial vaginosis
Circular probe-based RNA sequencing
Community state types
Cervicovaginal microbiome
Hierarchical clustering
High-risk human papillomavirus
High-grade squamous intraepithelial lesions
Jensen-Shannon distance
Lactobacillus -Dominated
Lactobacillus -Depleted
Low-grade squamous intraepithelial lesions
Negative for intraepithelial lesions or malignancy
Non-progression
Progression
Partial least squares discriminant analysis
Receiver operating characteristic
Squamous intraepithelial lesions
Unique read counts
Hausen HZ. Papillomaviruses causing cancer: evasion from host-cell control in early events in carcinogenesis. J Natl Cancer Inst. 2000;92(9):690–8.
Article PubMed Google Scholar
Doorbar J, Quint W, Banks L, Bravo IG, Stoler M, Broker TR, et al. The biology and life-cycle of human papillomaviruses. Vaccine. 2012;30(Suppl 5):F55–70.
Article CAS PubMed Google Scholar
Sasagawa T, Takagi H, Makinoda S. Immune responses against human papillomavirus (HPV) infection and evasion of host defense in cervical cancer. J Infect Chemother. 2012;18(6):807–15.
Steinbach A, Riemer AB. Immune evasion mechanisms of human papillomavirus: an update. Int J Cancer. 2018;142(2):224–9.
Koshiol J, Lindsay L, Pimenta JM, Poole C, Jenkins D, Smith JS. Persistent human papillomavirus infection and cervical neoplasia: a systematic review and meta-analysis. Am J Epidemiol. 2008;168(2):123–37.
Article PubMed PubMed Central Google Scholar
Molina MA, Carosi Diatricch L, Castany Quintana M, Melchers WJG, Andralojc KM. Cervical cancer risk profiling: molecular biomarkers predicting the outcome of hrHPV infection. Expert Rev Mol Diagn. 2020;20(11):1–22.
Wang R, Pan W, Jin L, Huang W, Li Y, Wu D, et al. Human papillomavirus vaccine against cervical cancer: opportunity and challenge. Cancer Lett. 2020;471:88–102.
Curty G, de Carvalho PS, Soares MA. The role of the cervicovaginal microbiome on the genesis and as a biomarker of premalignant cervical intraepithelial neoplasia and invasive cervical cancer. Int J Mol Sci. 2019;21(1):222.
Ventolini G, Vieira-Baptista P, De Seta F, Verstraelen H, Lonnee-Hoffmann R, Lev-Sagie A. The vaginal microbiome: IV. The role of vaginal microbiome in reproduction and in gynecologic cancers. J Low Genit Tract Dis. 2022;26(1):93–8.
Feehily C, Crosby D, Walsh CJ, Lawton EM, Higgins S, McAuliffe FM, et al. Shotgun sequencing of the vaginal microbiome reveals both a species and functional potential signature of preterm birth. NPJ Biofilms Microbiomes. 2020;6(1):50.
Article CAS PubMed PubMed Central Google Scholar
Kyrgiou M, Mitra A, Moscicki A-B. Does the vaginal microbiota play a role in the development of cervical cancer? Transl Res. 2017;179:168–82.
dos Anjos Borges LG, Pastuschek J, Heimann Y, Dawczynski K, Bergner M, Haase R, et al. Vaginal and neonatal microbiota in pregnant women with preterm premature rupture of membranes and consecutive early onset neonatal sepsis. BMC Med. 2023;21(1):92.
Ravel J, Gajer P, Abdo Z, Schneider GM, Koenig SSK, McCulle SL, et al. Vaginal microbiome of reproductive-age women. Proc Natl Acad Sci. 2011;108(Supplement 1):4680.
Molina Mariano A, Coenen Britt A, Leenders William PJ, Andralojc Karolina M, HuynenMartijn A, Melchers Willem JG. Assessing the cervicovaginal microbiota in the context of hrHPV infections: temporal dynamics and therapeutic strategies. mBio. 2022;13(5):e01619–22.
PubMed PubMed Central Google Scholar
Norenhag J, Du J, Olovsson M, Verstraelen H, Engstrand L, Brusselaers N. The vaginal microbiota, human papillomavirus and cervical dysplasia: a systematic review and network meta-analysis. BJOG. 2020;127(2):171–80.
Ravel J, Brotman RM, Gajer P, Ma B, Nandy M, Fadrosh DW, et al. Daily temporal dynamics of vaginal microbiota before, during and after episodes of bacterial vaginosis. Microbiome. 2013;1(1):29.
Clarridge JE. Impact of 16s rRNA gene sequence analysis for identification of bacteria on clinical microbiology and infectious diseases. Clin Microbiol Rev. 2004;17(4):840.
Graspeuntner S, Loeper N, Künzel S, Baines JF, Rupp J. Selection of validated hypervariable regions is crucial in 16S-based microbiota studies of the female genital tract. Sci Rep. 2018;8(1):9678.
Oh HY, Kim BS, Seo SS, Kong JS, Lee JK, Park SY, et al. The association of uterine cervical microbiota with an increased risk for cervical intraepithelial neoplasia in Korea. Clin Microbiol Infect. 2015;21(7):674.e1-.e9.
Article Google Scholar
Andralojc KM, Molina MA, Qiu M, Spruijtenburg B, Rasing M, Pater B, et al. Novel high-resolution targeted sequencing of the cervicovaginal microbiome. BMC Biol. 2021;19(1):267.
Yang Q, Wang Y, Wei X, Zhu J, Wang X, Xie X, et al. The alterations of vaginal microbiome in hpv16 infection as identified by shotgun metagenomic sequencing. Front Cell Infect Microbiol. 2020;10:286.
Quince C, Walker AW, Simpson JT, Loman NJ, Segata N. Shotgun metagenomics, from sampling to analysis. Nat Biotechnol. 2017;35(9):833–44.
Kullen MJ, Sanozky-Dawes RB, Crowell DC, Klaenhammer TR. Use of the DNA sequence of variable regions of the 16S rRNA gene for rapid and accurate identification of bacteria in the Lactobacillus acidophilus complex. J Appl Microbiol. 2000;89(3):511–6.
Molina MA, Andralojc KM, Huynen MA, Leenders WPJ, Melchers WJG. In-depth insights into cervicovaginal microbial communities and hrHPV infections using high-resolution microbiome profiling. NPJ Biofilms Microbiomes. 2022;8(1):75.
Heideman DAM, Hesselink AT, Berkhof J, van Kemenade F, Melchers WJG, Daalmeijer NF, et al. Clinical validation of the cobas 4800 HPV test for cervical screening purposes. J Clin Microbiol. 2011;49(11):3983–5.
de Bitter T, van de Water C, van den Heuvel C, Zeelen C, Eijkelenboom A, Tops B, et al. Profiling of the metabolic transcriptome via single molecule molecular inversion probes. Sci Rep. 2017;7(1):11402.
van den Heuvel CNAM, Loopik DL, Ebisch RMF, Elmelik D, Andralojc KM, Huynen M, et al. RNA-based high-risk HPV genotyping and identification of high-risk HPV transcriptional activity in cervical tissues. Mod Pathol. 2020;33(4):748–57.
Metsalu T, Vilo J. ClustVis: a web tool for visualizing clustering of multivariate data using Principal Component Analysis and heatmap. Nucleic Acids Res. 2015;43(W1):W566–70.
Xia J, Psychogios N, Young N, Wishart DS. MetaboAnalyst: a web server for metabolomic data analysis and interpretation. Nucleic Acids Res. 2009;37(suppl_2):W652–60.
Breiman L. Random forests. Mach Learn. 2001;45(1):5–32.
Robin X, Turck N, Hainard A, Tiberti N, Lisacek F, Sanchez J-C, et al. pROC: an open-source package for R and S+ to analyze and compare ROC curves. BMC Bioinformatics. 2011;12(1):77.
Kim S. ppcor: an R package for a fast calculation to semi-partial correlation coefficients. CSAM. 2015;22(6):665–74.
Drost H-G. Philentropy: information theory and distance quantification with R. J Open Source Softw. 2018;3(26):765.
Brotman RM, Shardell MD, Gajer P, Tracy JK, Zenilman JM, Ravel J, et al. Interplay between the temporal dynamics of the vaginal microbiota and human papillomavirus detection. J Infect Dis. 2014;210(11):1723–33.
Di Paola M, Sani C, Clemente AM, Iossa A, Perissi E, Castronovo G, et al. Characterization of cervico-vaginal microbiota in women developing persistent high-risk Human Papillomavirus infection. Sci Rep. 2017;7(1):10200.
Arokiyaraj S, Seo SS, Kwon M, Lee JK, Kim MK. Association of cervical microbial community with persistence, clearance and negativity of Human Papillomavirus in Korean women: a longitudinal study. Sci Rep. 2018;8(1):15479.
So KA, Yang EJ, Kim NR, Hong SR, Lee J-H, Hwang C-S, et al. Changes of vaginal microbiota during cervical carcinogenesis in women with human papillomavirus infection. PLoS ONE. 2020;15(9):e0238705.
Zhou F-Y, Zhou Q, Zhu Z-Y, Hua K-Q, Chen L-M, Ding J-X. Types and viral load of human papillomavirus, and vaginal microbiota in vaginal intraepithelial neoplasia: a cross-sectional study. Ann Transl Med. 2020;8(21):1408.
Libby EK, Pascal KE, Mordechai E, Adelson ME, Trama JP. Atopobium vaginae triggers an innate immune response in an in vitro model of bacterial vaginosis. Microbes Infect. 2008;10(4):439–46.
Borgdorff H, Gautam R, Armstrong SD, Xia D, Ndayisaba GF, van Teijlingen NH, et al. Cervicovaginal microbiome dysbiosis is associated with proteome changes related to alterations of the cervicovaginal mucosal barrier. Mucosal Immunol. 2016;9(3):621–33.
Mei L, Wang T, Chen Y, Wei D, Zhang Y, Cui T, et al. Dysbiosis of vaginal microbiota associated with persistent high-risk human papilloma virus infection. J Transl Med. 2022;20(1):12.
Bowden SJ, Doulgeraki T, Bouras E, Markozannes G, Athanasiou A, Grout-Smith H, et al. Risk factors for human papillomavirus infection, cervical intraepithelial neoplasia and cervical cancer: an umbrella review and follow-up Mendelian randomisation studies. BMC Med. 2023;21(1):274.
Witkin SS, Mendes-Soares H, Linhares IM, Jayaram A, Ledger WJ, Forney LJ, et al. Influence of vaginal bacteria and d- and l- lactic acid isomers on vaginal extracellular matrix metalloproteinase inducer: implications for protection against upper genital tract infections. mBio. 2013;4(4):e00460–13.
Stoyancheva G, Marzotto M, Dellaglio F, Torriani S. Bacteriocin production and gene sequencing analysis from vaginal Lactobacillus strains. Arch Microbiol. 2014;196(9):645–53.
Amabebe E, Anumba DOC. The vaginal microenvironment: the physiologic role of lactobacilli. Front Med. 2018;5:181.
Mitra A, MacIntyre DA, Ntritsos G, Smith A, Tsilidis KK, Marchesi JR, et al. The vaginal microbiota associates with the regression of untreated cervical intraepithelial neoplasia 2 lesions. Nat Commun. 2020;11(1):1999.
Morais IMC, Cordeiro AL, Teixeira GS, Domingues VS, Nardi RMD, Monteiro AS, et al. Biological and physicochemical properties of biosurfactants produced by Lactobacillus jensenii P6A and Lactobacillus gasseri P65 . Microb Cell Fact. 2017;16(1):155.
Romero R, Hassan SS, Gajer P, Tarca AL, Fadrosh DW, Nikita L, et al. The composition and stability of the vaginal microbiota of normal pregnant women is different from that of non-pregnant women. Microbiome. 2014;2(1):4.
Moscicki A-B, Shi B, Huang H, Barnard E, Li H. Cervical-vaginal microbiome and associated cytokine profiles in a prospective study of HPV 16 acquisition, persistence, and clearance. Front Cell Infect Microbiol. 2020;10:528.
dos Santos Santiago Lopes G, Tency I, Verstraelen H, Verhelst R, Trog M, Temmerman M, et al. Longitudinal qPCR study of the dynamics of L . crispatus , L . iners , A . vaginae , (Sialidase Positive) G . vaginalis , and P . bivia in the vagina. PLOS ONE. 2012;7(9):e45281.
Verstraelen H, Verhelst R, Claeys G, De Backer E, Temmerman M, Vaneechoutte M. Longitudinal analysis of the vaginal microflora in pregnancy suggests that L . crispatus promotes the stability of the normal vaginal microflora and that L . gasseri and/or L. iners are more conducive to the occurrence of abnormal vaginal microflora. BMC Microbiol. 2009;9(1):116.
Tett A, Pasolli E, Masetti G, Ercolini D, Segata N. Prevotella diversity, niches and interactions with the human host. Nat Rev Microbiol. 2021;19(9):585–99.
van Teijlingen NH, Helgers LC, Zijlstra-Willems EM, van Hamme JL, Ribeiro CMS, Strijbis K, et al. Vaginal dysbiosis associated-bacteria Megasphaera elsdenii and Prevotella timonensis induce immune activation via dendritic cells. J Reprod Immunol. 2020;138:103085.
van Teijlingen NH, Helgers LC, Sarrami-Forooshani R, Zijlstra-Willems EM, van Hamme JL, Segui-Perez C, et al. Vaginal bacterium Prevotella timonensis turns protective Langerhans cells into HIV-1 reservoirs for virus dissemination. EMBO J. 2022;41(19):e110629.
López-Filloy M, Cortez FJ, Gheit T, Cruz y Cruz O, Cruz-Talonia F, Chávez-Torres M, et al. Altered vaginal microbiota composition correlates with human papillomavirus and mucosal immune responses in women with symptomatic cervical ectopy. Front Cell Infect Microbiol. 2022;12:884272.
France MT, Fu L, Rutt L, Yang H, Humphrys MS, Narina S, et al. Insight into the ecology of vaginal bacteria through integrative analyses of metagenomic and metatranscriptomic data. Genome Biol. 2022;23(1):66.
Lebeau A, Bruyere D, Roncarati P, Peixoto P, Hervouet E, Cobraiville G, et al. HPV infection alters vaginal microbiome through down-regulating host mucosal innate peptides used by Lactobacilli as amino acid sources. Nat Commun. 2022;13(1):1076.
Chen EY, Tran A, Raho CJ, Birch CM, Crum CP, Hirsch MS. Histological ‘progression’ from low (LSIL) to high (HSIL) squamous intraepithelial lesion is an uncommon event and an indication for quality assurance review. Mod Pathol. 2010;23(8):1045–51.
dos Santos Santiago Lopes G, Cools P, Verstraelen H, Trog M, Missine G, Aila NE, et al. Longitudinal study of the dynamics of vaginal microflora during two consecutive menstrual cycles. PLOS ONE. 2011;6(11):e28180.
Usyk M, Schlecht NF, Pickering S, Williams L, Sollecito CC, Gradissimo A, et al. molBV reveals immune landscape of bacterial vaginosis and predicts human papillomavirus infection natural history. Nat Commun. 2022;13(1):233.
Andralojc KM, Elmelik D, Rasing M, Pater B, Siebers AG, Bekkers R, et al. Targeted RNA next generation sequencing analysis of cervical smears can predict the presence of hrHPV-induced cervical lesions. BMC Med. 2022;20(1):206.
Molina MA, Andralojc KM, Leenders WPJ, Huynen MA, Melchers WJG. Cervicovaginal microbial communities and hrHPV infections Sequencing Read Archive: NCBI; 2022. BioProject: PRJNA856437]. Available from: https://www.ncbi.nlm.nih.gov/bioproject/PRJNA856437 . [cited 2022 October 9].
Molina MA, Leenders WPJ, Huynen MA, Melchers WJG, Andralojc KM. Longitudinal profiling of the cervicovaginal microbiome Sequencing Read Archive: NCBI; 2022. BioProject: PRJNA888791]. Available from: https://www.ncbi.nlm.nih.gov/bioproject/PRJNA888791 . [cited 2022 October 9].
Download references
Acknowledgements
BioRender.com was used to design figures for the manuscript.
This work was supported by a research grant from the Ruby and Rose Foundation.
Author information
Authors and affiliations.
Department of Medical Microbiology, Radboud University Medical Center, Nijmegen, 6500 HB, The Netherlands
Mariano A. Molina, Willem J. G. Melchers & Karolina M. Andralojc
Department of Medical Microbiology, Radboud Institute for Molecular Life Sciences, Nijmegen, The Netherlands
Mariano A. Molina
Predica Diagnostics, Toernooiveld 1, Nijmegen, 6525 ED, The Netherlands
William P. J. Leenders
Center for Molecular and Biomolecular Informatics, Radboud University Medical Center, Nijmegen, 6525 GA, The Netherlands
Martijn A. Huynen
You can also search for this author in PubMed Google Scholar
Contributions
KA, WM, and WL conceptualized the study. MM performed the data analyses for the manuscript, supervised by KA, MH, WM, and WL. MM drafted the manuscript and was revised by all authors (KA, MH, WL, and WM). All authors approved the manuscript and contributed to the final version for publication.
Corresponding author
Correspondence to Willem J. G. Melchers .
Ethics declarations
Ethics approval and consent to participate.
The Central Committee on Research Involving Human Subjects (CCMO) and the National Institute for Public Health and Environment (RIVM) reviewed and granted approval before the start of the study (No. 2014–1295). All methods were performed in accordance with the Radboudumc ethical guidelines for using human samples, including the Declaration of Helsinki.
Consent for publication
Not applicable.
Competing interests
The authors declare no competing non-financial interests but the following competing financial interests: WL is CSO and shareholder of Predica Diagnostics.
Additional information
Publisher’s note.
Springer Nature remains neutral with regard to jurisdictional claims in published maps and institutional affiliations.
Supplementary Information
Additional file 1: supplementary figure 1. microbial diversity of microbiomes at visit 1., additional file 2: supplementary figure 2. composition of the microbiomes at visit 2., additional file 3: supplementary figure 3. abundances of prevotella species at visit 2., additional file 4. plsda loadings at v1., additional file 5. plsda loadings at v2., additional file 6: supplementary figure 4. identification of relevant microbial species in the plsda., additional file 7. sample accession numbers in ncbi database. sample id, hrhpv status, and accession numbers per visit., rights and permissions.
Open Access This article is licensed under a Creative Commons Attribution 4.0 International License, which permits use, sharing, adaptation, distribution and reproduction in any medium or format, as long as you give appropriate credit to the original author(s) and the source, provide a link to the Creative Commons licence, and indicate if changes were made. The images or other third party material in this article are included in the article's Creative Commons licence, unless indicated otherwise in a credit line to the material. If material is not included in the article's Creative Commons licence and your intended use is not permitted by statutory regulation or exceeds the permitted use, you will need to obtain permission directly from the copyright holder. To view a copy of this licence, visit http://creativecommons.org/licenses/by/4.0/ . The Creative Commons Public Domain Dedication waiver ( http://creativecommons.org/publicdomain/zero/1.0/ ) applies to the data made available in this article, unless otherwise stated in a credit line to the data.
Reprints and permissions
About this article
Cite this article.
Molina, M.A., Leenders, W.P.J., Huynen, M.A. et al. Temporal composition of the cervicovaginal microbiome associates with hrHPV infection outcomes in a longitudinal study. BMC Infect Dis 24 , 552 (2024). https://doi.org/10.1186/s12879-024-09455-1
Download citation
Received : 07 November 2023
Accepted : 30 May 2024
Published : 03 June 2024
DOI : https://doi.org/10.1186/s12879-024-09455-1
Share this article
Anyone you share the following link with will be able to read this content:
Sorry, a shareable link is not currently available for this article.
Provided by the Springer Nature SharedIt content-sharing initiative
- Longitudinal study
BMC Infectious Diseases
ISSN: 1471-2334
- General enquiries: [email protected]
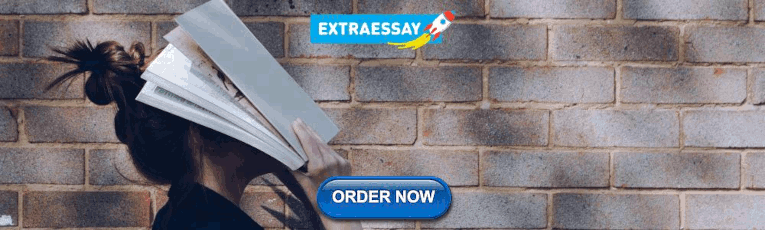
COMMENTS
Revised on June 22, 2023. In a longitudinal study, researchers repeatedly examine the same individuals to detect any changes that might occur over a period of time. Longitudinal studies are a type of correlational research in which researchers observe and collect data on a number of variables without trying to influence those variables.
Panel Study. A panel study is a type of longitudinal study design in which the same set of participants are measured repeatedly over time. Data is gathered on the same variables of interest at each time point using consistent methods. This allows studying continuity and changes within individuals over time on the key measured constructs.
The Framingham study is widely recognised as the quintessential longitudinal study in the history of medical research. An original cohort of 5,209 subjects from Framingham, Massachusetts between the ages of 30 and 62 years of age was recruited and followed up for 20 years.
A longitudinal study (or longitudinal survey, or panel study) is a research design that involves repeated observations of the same variables (e.g., people) over long periods of time (i.e., uses longitudinal data).It is often a type of observational study, although it can also be structured as longitudinal randomized experiment.. Longitudinal studies are often used in social-personality and ...
CRD and LRD. Based on the number of time periods for which the same variable is measured, the research designs in social sciences are broadly classified into two types: CRD and LRD. In CRD, the researcher collects the data on one or more than one variable for a single time period for each case in the study. The researcher measures the variables ...
The opposite of a longitudinal study is a cross-sectional study. While longitudinal studies repeatedly observe the same participants over a period of time, cross-sectional studies examine different samples (or a 'cross-section') of the population at one point in time. They can be used to provide a snapshot of a group or society at a ...
A longitudinal study is an experimental design that takes repeated measurements of the same subjects over time. These studies can span years or even decades. Unlike cross-sectional studies, which analyze data at a single point, longitudinal studies track changes and developments, producing a more dynamic assessment.
A longitudinal study is observational and involves the continuous and repeated measurements of selected individuals followed over a period of time. Quantitative and qualitative data is gathered on "any combination of exposures and outcome." For instance, longitudinal studies are useful for observing relationships between the risk factors, development, and treatment outcomes of disease for ...
Key Research Findings. Both cross-sectional and longitudinal studies are observational in nature, meaning that researchers measure variables of interest without manipulating them. Cross-sectional studies gather information and compare multiple population groups at a single point in time. They offer snapshots of the important current social ...
Introduction. Longitudinal qualitative research (LQR) is an emerging methodology in health behavior and nursing research—fields focused on generating evidence to support nursing practices as well as programs, and policies promoting healthy behaviors (Glanz et al., 2008; Polit & Beck, 2017).Because human experiences are rarely comprised of concrete, time-limited events, but evolve and change ...
Longitudinal studies, a type of correlational research, are usually observational, in contrast with cross-sectional research. Longitudinal research involves collecting data over an extended time, whereas cross-sectional research involves collecting data at a single point. To test this hypothesis, the researchers recruit participants who are in ...
A longitudinal study is a study that repeatedly measures observations (collects data) over time. It often involves following up with patients for a prolonged period, such as years, and measuring both explanatory and outcome variables at multiple points, usually more than two, of follow-up. Longitudinal studies are most commonly observational ...
As the name implies, longitudinal studies follow subjects over time (Fig. 42.1).There are three main types of studies that fall under the umbrella of the longitudinal study: cohort studies, panel studies, and retrospective studies. 1 The cohort study is one of the most common types of longitudinal studies. It involves following a cohort (a group of individuals with a shared characteristic(s ...
LONGITUDINAL STUDY DESIGN. The design of longitudinal studies on aging should focus on a set of primary questions and hypotheses while taking into account the important contributions of function, comorbid health conditions, and behavioral and environmental factors. By focusing on primary questions and hypotheses, other methodological concerns ...
An important meta-trend in work, aging, and retirement research is the heightened appreciation of the temporal nature of the phenomena under investigation and the important role that longitudinal study designs play in understanding them (e.g., Heybroek, Haynes, & Baxter, 2015; Madero-Cabib, Gauthier, & Le Goff, 2016; Wang, 2007; Warren, 2015; Weikamp & Göritz, 2015).
This chapter outlines critical design decisions for longitudinal research and provides practical tips for managing such studies. It emphasizes that generative longitudinal studies are driven by conceptual and theoretical insights and describes four foundational design issues including questions about time lags and sample sizes.
Qualitative longitudinal research (QLR) comprises qualitative studies, with repeated data collection, that focus on the temporality (e.g., time and change) of a phenomenon. The use of QLR is increasing in health research since many topics within health involve change (e.g., progressive illness, rehabilitation). A method study can provide an insightful understanding of the use, trends and ...
In the special issue of Qualitative Research, different researchers apply discrete approaches to make sense of narrative stability and change in a set of case-studies from the Foley Longitudinal Study (Dunlop, 2019; Fivush et al., 2019; McLean et al., 2019; Pasupathi & Wainryb, 2019; Singer, 2019). Researchers and articles with separate ...
Longitudinal studies. Chapter 7. Longitudinal studies. More chapters in Epidemiology for the uninitiated. In a longitudinal study subjects are followed over time with continuous or repeated monitoring of risk factors or health outcomes, or both. Such investigations vary enormously in their size and complexity.
Longitudinal studies in human-computer interaction (HCI) research have been applied and discussed for several years, and the potential of conducting studies that are longitudinal by nature is almost quite evident, e.g. the opportunity to measure or observe changes over time [].Longitudinal studies or longitudinal research are commonly applied and used in other research disciplines.
To examine changes in individuals' psychosocial variables (e.g., psychological distress, social isolation, and alcohol use) during the prolonged COVID-19 pandemic, a two-year longitudinal survey ...
Design, Setting, and Participants This nationally representative longitudinal cohort study assessed participants for 18 years, from August 2000 through December 2018, using data from the Health and Retirement Study (HRS) and linked Medicare claims dates. Analyses were completed August 9 through December 12, 2022.
Nurses around the world are expected to demonstrate competence in performing mental status evaluation. However, there is a gap between what is taught in class and what is practiced for patients with mental illness among nursing students during MSE performance. It is believed that proper pedagogics may enhance this competence. A longitudinal controlled quasi-experimental study design was used ...
This multicentre, longitudinal cohort study included 40 091 consecutive patients undergoing clinically indicated CCTA in eight UK hospitals, who were followed up for MACE (ie, myocardial infarction, new onset heart failure, or cardiac death) for a median of 2·7 years (IQR 1·4-5·3).
Background. Qualitative longitudinal research (QLR) comprises qualitative studies, with repeated data collection, that focus on the temporality (e.g., time and change) of a phenomenon. The use of QLR is increasing in health research since many topics within health involve change (e.g., progressive illness, rehabilitation).
A topic of debate in business press and academic research is whether metrics such as customer likelihood to recommend predict revenue or market share growth. ... most likelihood-to-recommend or NPS studies have relied on customer samples. ... Ali M. (2019). Investigating relationship between net promoter score and company performance: A ...
Study Funding. L. Zhang: Grants from Swedish Research Council for Health, Working Life, and Welfare. H. Larsson: European Union's Horizon 2020 research and innovation program under grant agreement. Study Objectives. To assess the association between long-term use of ADHD medication and the risk of CVD. Methodology
In this longitudinal study, we investigate the composition of the CVM in a cohort of Dutch women participating in the population-based cervical cancer screening program, with proven hrHPV infection but normal cytology at baseline, who were diagnosed with SIL six-months later or did not develop cervical abnormalities.
Aims This study aims to assess the dynamics of in-school adolescents' mental health problems in Harari regional state, eastern Ethiopia for a year. Materials and methods Using multistage sampling technique, we conducted a year-long longitudinal study at three public high schools between March 2020 and 2021. Three hundred fifty-eight in-school adolescents were chosen by systematic random ...
presented in this study, the longitudinal stability of the PCCT builds higher confidence in the development and translation of these solutions. This study has several limitations: (i) We only evaluated a single protocol designed for abdominal imaging. Further studies will be necessary to assess the long-term performance of