- Research article
- Open access
- Published: 01 October 2021
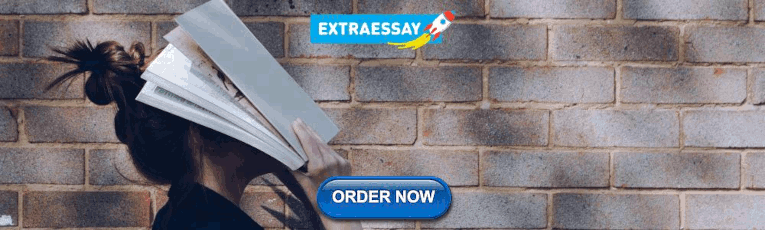
Adaptive e-learning environment based on learning styles and its impact on development students' engagement
- Hassan A. El-Sabagh ORCID: orcid.org/0000-0001-5463-5982 1 , 2
International Journal of Educational Technology in Higher Education volume 18 , Article number: 53 ( 2021 ) Cite this article
68 Citations
27 Altmetric
Metrics details
Adaptive e-learning is viewed as stimulation to support learning and improve student engagement, so designing appropriate adaptive e-learning environments contributes to personalizing instruction to reinforce learning outcomes. The purpose of this paper is to design an adaptive e-learning environment based on students' learning styles and study the impact of the adaptive e-learning environment on students’ engagement. This research attempts as well to outline and compare the proposed adaptive e-learning environment with a conventional e-learning approach. The paper is based on mixed research methods that were used to study the impact as follows: Development method is used in designing the adaptive e-learning environment, a quasi-experimental research design for conducting the research experiment. The student engagement scale is used to measure the following affective and behavioral factors of engagement (skills, participation/interaction, performance, emotional). The results revealed that the experimental group is statistically significantly higher than those in the control group. These experimental results imply the potential of an adaptive e-learning environment to engage students towards learning. Several practical recommendations forward from this paper: how to design a base for adaptive e-learning based on the learning styles and their implementation; how to increase the impact of adaptive e-learning in education; how to raise cost efficiency of education. The proposed adaptive e-learning approach and the results can help e-learning institutes in designing and developing more customized and adaptive e-learning environments to reinforce student engagement.
Introduction
In recent years, educational technology has advanced at a rapid rate. Once learning experiences are customized, e-learning content becomes richer and more diverse (El-Sabagh & Hamed, 2020 ; Yang et al., 2013 ). E-learning produces constructive learning outcomes, as it allows students to actively participate in learning at anytime and anyplace (Chen et al., 2010 ; Lee et al., 2019 ). Recently, adaptive e-learning has become an approach that is widely implemented by higher education institutions. The adaptive e-learning environment (ALE) is an emerging research field that deals with the development approach to fulfill students' learning styles by adapting the learning environment within the learning management system "LMS" to change the concept of delivering e-content. Adaptive e-learning is a learning process in which the content is taught or adapted based on the responses of the students' learning styles or preferences. (Normadhi et al., 2019 ; Oxman & Wong, 2014 ). By offering customized content, adaptive e-learning environments improve the quality of online learning. The customized environment should be adaptable based on the needs and learning styles of each student in the same course. (Franzoni & Assar, 2009 ; Kolekar et al., 2017 ). Adaptive e-learning changes the level of instruction dynamically based on student learning styles and personalizes instruction to enhance or accelerate a student's success. Directing instruction to each student's strengths and content needs can minimize course dropout rates, increase student outcomes and the speed at which they are accomplished. The personalized learning approach focuses on providing an effective, customized, and efficient path of learning so that every student can participate in the learning process (Hussein & Al-Chalabi, 2020 ). Learning styles, on the other hand, represent an important issue in learning in the twenty-first century, with students expected to participate actively in developing self-understanding as well as their environment engagement. (Klasnja-Milicevic et al., 2011 ; Nuankaew et al., 2019 ; Truong, 2016 ).
In current conventional e-learning environments, instruction has traditionally followed a “one style fits all” approach, which means that all students are exposed to the same learning procedures. This type of learning does not take into account the different learning styles and preferences of students. Currently, the development of e-learning systems has accommodated and supported personalized learning, in which instruction is fitted to a students’ individual needs and learning styles (Beldagli & Adiguzel, 2010 ; Benhamdi et al., 2017 ; Pashler et al., 2008 ). Some personalized approaches let students choose content that matches their personality (Hussein & Al-Chalabi, 2020 ). The delivery of course materials is an important issue of personalized learning. Moreover, designing a well-designed, effective, adaptive e-learning system represents a challenge due to complication of adapting to the different needs of learners (Alshammari, 2016 ). Regardless of using e-learning claims that shifting to adaptive e-learning environments to be able to reinforce students' engagement. However, a learning environment cannot be considered adaptive if it is not flexible enough to accommodate students' learning styles. (Ennouamani & Mahani, 2017 ).
On the other hand, while student engagement has become a central issue in learning, it is also an indicator of educational quality and whether active learning occurs in classes. (Lee et al., 2019 ; Nkomo et al., 2021 ; Robinson & Hullinger, 2008 ). Veiga et al. ( 2014 ) suggest that there is a need for further research in engagement because assessing students’ engagement is a predictor of learning and academic progress. It is important to clarify the distinction between causal factors such as learning environment and outcome factors such as achievement. Accordingly, student engagement is an important research topic because it affects a student's final grade, and course dropout rate (Staikopoulos et al., 2015 ).
The Umm Al-Qura University strategic plan through common first-year deanship has focused on best practices that increase students' higher-order skills. These skills include communication skills, problem-solving skills, research skills, and creative thinking skills. Although the UQU action plan involves improving these skills through common first-year academic programs, the student's learning skills need to be encouraged and engaged more (Umm Al-Qura University Agency, 2020 ). As a result of the author's experience, The conventional methods of instruction in the "learning skills" course were observed, in which the content is presented to all students in one style that is dependent on understanding the content regardless of the diversity of their learning styles.
According to some studies (Alshammari & Qtaish, 2019 ; Lee & Kim, 2012 ; Shih et al., 2008 ; Verdú, et al., 2008 ; Yalcinalp & Avc, 2019 ), there is little attention paid to the needs and preferences of individual learners, and as a result, all learners are treated in the same way. More research into the impact of educational technologies on developing skills and performance among different learners is recommended. This “one-style-fits-all” approach implies that all learners are expected to use the same learning style as prescribed by the e-learning environment. Subsequently, a review of the literature revealed that an adaptive e-learning environment can affect learning outcomes to fill the identified gap. In conclusion: Adaptive e-learning environments rely on the learner's preferences and learning style as a reference that supports to create adaptation.
To confirm the above: the author conducted an exploratory study via an open interview that included some questions with a sample of 50 students in the learning skills department of common first-year. Questions asked about the difficulties they face when learning a "learning skills" course, what is the preferred way of course content. Students (88%) agreed that the way students are presented does not differ according to their differences and that they suffer from a lack of personal learning that is compatible with their style of work. Students (82%) agreed that they lack adaptive educational content that helps them to be engaged in the learning process. Accordingly, the author handled the research problem.
This research supplements to the existing body of knowledge on the subject. It is considered significant because it improves understanding challenges involved in designing the adaptive environments based on learning styles parameter. Subsequently, this paper is structured as follows: The next section presents the related work cited in the literature, followed by research methodology, then data collection, results, discussion, and finally, some conclusions and future trends are discussed.
Theoretical framework
This section briefly provides a thorough review of the literature about the adaptive E-learning environments based on learning styles.
Adaptive e-learning environments based on learning styles
The adaptive e-learning employment in higher education has been slower to evolve, and challenges that led to the slow implementation still exist. The learning management system offers the same tools to all learners, although individual learners need different details based on learning style and preferences. (Beldagli & Adiguzel, 2010 ; Kolekar et al., 2017 ). The interactive e-learning environment requisite evaluating the learner's desired learning style, before the course delivery, such as an online quiz or during the course delivery, such as tracking student reactions (DeCapua & Marshall, 2015 ).
In e-learning environments, adaptation is constructed on a series of well-designed processes to fit the instructional materials. The adaptive e-learning framework attempt to match instructional content to the learners' needs and styles. According to Qazdar et al. ( 2015 ), adaptive e-learning (AEL) environments rely on constructing a model of each learner's needs, preferences, and styles. It is well recognized that such adaptive behavior can increase learners' development and performance, thus enriching learning experience quality. (Shi et al., 2013 ). The following features of adaptive e-learning environments can be identified through diversity, interactivity, adaptability, feedback, performance, and predictability. Although adaptive framework taxonomy and characteristics related to various elements, adaptive learning includes at least three elements: a model of the structure of the content to be learned with detailed learning outcomes (a content model). The student's expertise based on success, as well as a method of interpreting student strengths (a learner model), and a method of matching the instructional materials and how it is delivered in a customized way (an instructional model) (Ali et al., 2019 ). The number of adaptive e-learning studies has increased over the last few years. Adaptive e-learning is likely to increase at an accelerating pace at all levels of instruction (Hussein & Al-Chalabi, 2020 ; Oxman & Wong, 2014 ).
Many studies assured the power of adaptive e-learning in delivering e-content for learners in a way that fitting their needs, and learning styles, which helps improve the process of students' acquisition of knowledge, experiences and develop their higher thinking skills (Ali et al., 2019 ; Behaz & Djoudi, 2012 ; Chun-Hui et al., 2017 ; Daines et al., 2016 ; Dominic et al., 2015 ; Mahnane et al., 2013 ; Vassileva, 2012 ). Student characteristics of learning style are recognized as an important issue and a vital influence in learning and are frequently used as a foundation to generate personalized learning experiences (Alshammari & Qtaish, 2019 ; El-Sabagh & Hamed, 2020 ; Hussein & Al-Chalabi, 2020 ; Klasnja-Milicevic et al., 2011 ; Normadhi et al., 2019 ; Ozyurt & Ozyurt, 2015 ).
The learning style is a parameter of designing adaptive e-learning environments. Individuals differ in their learning styles when interacting with the content presented to them, as many studies emphasized the relationship between e-learning and learning styles to be motivated in learning situations, consequently improving the learning outcomes (Ali et al., 2019 ; Alshammari, 2016 ; Alzain et al., 2018a , b ; Liang, 2012 ; Mahnane et al., 2013 ; Nainie et al., 2010 ; Velázquez & Assar, 2009 ). The word "learning style" refers to the process by which the learner organizes, processes, represents, and combines this information and stores it in his cognitive source, then retrieves the information and experiences in the style that reflects his technique of communicating them. (Fleming & Baume, 2006 ; Jaleel & Thomas, 2019 ; Jonassen & Grabowski, 2012 ; Klasnja-Milicevic et al., 2011 ; Nuankaew et al., 2019 ; Pashler et al., 2008 ; Willingham et al., 2105 ; Zhang, 2017 ). The concept of learning style is founded based on the fact that students vary in their styles of receiving knowledge and thought, to help them recognizing and combining information in their mind, as well as acquire experiences and skills. (Naqeeb, 2011 ). The extensive scholarly literature on learning styles is distributed with few strong experimental findings (Truong, 2016 ), and a few findings on the effect of adapting instruction to learning style. There are many models of learning styles (Aldosarim et al., 2018 ; Alzain et al., 2018a , 2018b ; Cletus & Eneluwe, 2020 ; Franzoni & Assar, 2009 ; Willingham et al., 2015 ), including the VARK model, which is one of the most well-known models used to classify learning styles. The VARK questionnaire offers better thought about information processing preferences (Johnson, 2009 ). Fleming and Baume ( 2006 ) developed the VARK model, which consists of four students' preferred learning types. The letter "V" represents for visual and means the visual style, while the letter "A" represents for auditory and means the auditory style, and the letter "R/W" represents "write/read", means the reading/writing style, and the letter "K" represents the word "Kinesthetic" and means the practical style. Moreover, VARK distinguishes the visual category further into graphical and textual or visual and read/write learners (Murphy et al., 2004 ; Leung, et al., 2014 ; Willingham et al., 2015 ). The four categories of The VARK Learning Style Inventory are shown in the Fig. 1 below.
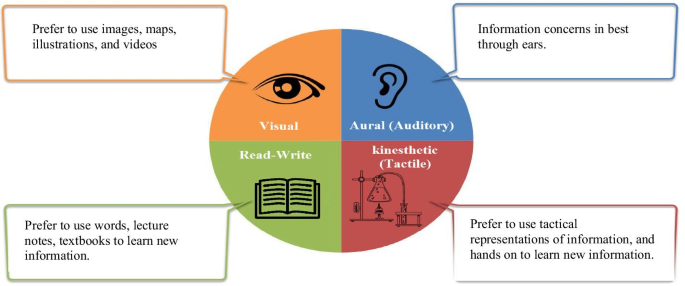
VARK learning styles
According to the VARK model, learners are classified into four groups representing basic learning styles based on their responses which have 16 questions, there are four potential responses to each question, where each answer agrees to one of the extremes of the dimension (Hussain, 2017 ; Silva, 2020 ; Zhang, 2017 ) to support instructors who use it to create effective courses for students. Visual learners prefer to take instructional materials and send assignments using tools such as maps, graphs, images, and other symbols, according to Fleming and Baume ( 2006 ). Learners who can read–write prefer to use written textual learning materials, they use glossaries, handouts, textbooks, and lecture notes. Aural learners, on the other hand, prefer to learn through spoken materials, dialogue, lectures, and discussions. Direct practice and learning by doing are preferred by kinesthetic learners (Becker et al., 2007 ; Fleming & Baume, 2006 ; Willingham et al., 2015 ). As a result, this research work aims to provide a comprehensive discussion about how these individual parameters can be applied in adaptive e-learning environment practices. Dominic et al., ( 2015 ) presented a framework for an adaptive educational system that personalized learning content based on student learning styles (Felder-Silverman learning model) and other factors such as learners' learning subject competency level. This framework allowed students to follow their adaptive learning content paths based on filling in "ils" questionnaire. Additionally, providing a customized framework that can automatically respond to students' learning styles and suggest online activities with complete personalization. Similarly, El Bachari et al. ( 2011 ) attempted to determine a student's unique learning style and then adapt instruction to that individual interests. Adaptive e-learning focused on learner experience and learning style has a higher degree of perceived usability than a non-adaptive e-learning system, according to Alshammari et al. ( 2015 ). This can also improve learners' satisfaction, engagement, and motivation, thus improving their learning.
According to the findings of (Akbulut & Cardak, 2012 ; Alshammari & Qtaish, 2019 ; Alzain et al., 2018a , b ; Shi et al., 2013 ; Truong, 2016 ), adaptation based on a combination of learning style, and information level yields significantly better learning gains. Researchers have recently initiated to focus on how to personalize e-learning experiences using personal characteristics such as the student's preferred learning style. Personal learning challenges are addressed by adaptive learning programs, which provide learners with courses that are fit to their specific needs, such as their learning styles.
- Student engagement
Previous research has emphasized that student participation is a key factor in overcoming academic problems such as poor academic performance, isolation, and high dropout rates (Fredricks et al., 2004 ). Student participation is vital to student learning, especially in an online environment where students may feel isolated and disconnected (Dixson, 2015 ). Student engagement is the degree to which students consciously engage with a course's materials, other students, and the instructor. Student engagement is significant for keeping students engaged in the course and, as a result, in their learning (Barkley & Major, 2020 ; Lee et al., 2019 ; Rogers-Stacy, et al, 2017 ). Extensive research was conducted to investigate the degree of student engagement in web-based learning systems and traditional education systems. For instance, using a variety of methods and input features to test the relationship between student data and student participation (Hussain et al., 2018 ). Guo et al. ( 2014 ) checked the participation of students when they watched videos. The input characteristics of the study were based on the time they watched it and how often students respond to the assessment.
Atherton et al. ( 2017 ) found a correlation between the use of course materials and student performance; course content is more expected to lead to better grades. Pardo et al., ( 2016 ) found that interactive students with interactive learning activities have a significant impact on student test scores. The course results are positively correlated with student participation according to previous research. For example, Atherton et al. ( 2017 ) explained that students accessed learning materials online and passed exams regularly to obtain higher test scores. Other studies have shown that students with higher levels of participation in questionnaires and course performance tend to perform well (Mutahi et al., 2017 ).
Skills, emotion, participation, and performance, according to Dixson ( 2015 ), were factors in online learning engagement. Skills are a type of learning that includes things like practicing on a daily foundation, paying attention while listening and reading, and taking notes. Emotion refers to how the learner feels about learning, such as how much you want to learn. Participation refers to how the learner act in a class, such as chat, discussion, or conversation. Performance is a result, such as a good grade or a good test score. In general, engagement indicated that students spend time, energy learning materials, and skills to interact constructively with others in the classroom, and at least participate in emotional learning in one way or another (that is, be motivated by an idea, willing to learn and interact). Student engagement is produced through personal attitudes, thoughts, behaviors, and communication with others. Thoughts, effort, and feelings to a certain level when studying. Therefore, the student engagement scale attempts to measure what students are doing (thinking actively), how they relate to their learning, and how they relate to content, faculty members, and other learners including the following factors as shown in Fig. 2 . (skills, participation/interaction, performance, and emotions). Hence, previous research has moved beyond comparing online and face-to-face classes to investigating ways to improve online learning (Dixson, 2015 ; Gaytan & McEwen, 2007 ; Lévy & Wakabayashi, 2008 ; Mutahi et al., 2017 ). Learning effort, involvement in activities, interaction, and learning satisfaction, according to reviews of previous research on student engagement, are significant measures of student engagement in learning environments (Dixson, 2015 ; Evans et al., 2017 ; Lee et al., 2019 ; Mutahi et al., 2017 ; Rogers-Stacy et al., 2017 ). These results point to several features of e-learning environments that can be used as measures of student participation. Successful and engaged online learners learn actively, have the psychological inspiration to learn, make good use of prior experience, and make successful use of online technology. Furthermore, they have excellent communication abilities and are adept at both cooperative and self-directed learning (Dixson, 2015 ; Hong, 2009 ; Nkomo et al., 2021 ).

Engagement factors
Overview of designing the adaptive e-learning environment
The paper follows the (ADDIE) Instructional Design Model: analysis, design, develop, implement, and evaluate to answer the first research question. The adaptive learning environment offers an interactive decentralized media environment that takes into account individual differences among students. Moreover, the environment can spread the culture of self-learning, attract students, and increase their engagement in learning.
Any learning environment that is intended to accomplish a specific goal should be consistent to increase students' motivation to learn. so that they have content that is personalized to their specific requirements, rather than one-size-fits-all content. As a result, a set of instructional design standards for designing an adaptive e-learning framework based on learning styles was developed according to the following diagram (Fig. 3 ).
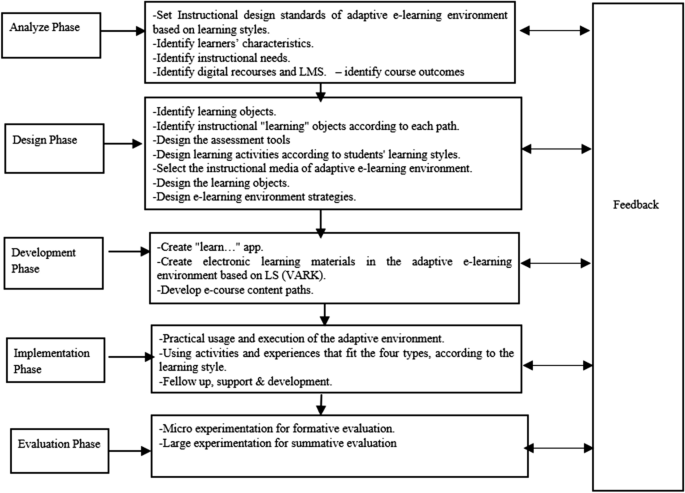
The ID (model) of the adaptive e-learning environment
According to the previous figure, The analysis phase included identifying the course materials and learning tools (syllabus and course plan modules) used for the study. The learning objectives were included in the high-level learning objectives (C4-C6: analysis, synthesis, evaluation).
The design phase included writing SMART objectives, the learning materials were written within the modules plan. To support adaptive learning, four content paths were identified, choosing learning models, processes, and evaluation. Course structure and navigation were planned. The adaptive structural design identified the relationships between the different components, such as introduction units, learning materials, quizzes. Determining the four path materials. The course instructional materials were identified according to the following Figure 4 .
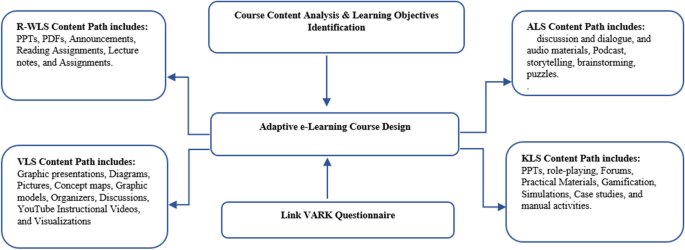
Adaptive e-course design
The development phase included: preparing and selecting the media for the e-course according to each content path in an adaptive e-learning environment. During this process, the author accomplished the storyboard and the media to be included on each page of the storyboard. A category was developed for the instructional media for each path (Fig. 5 )
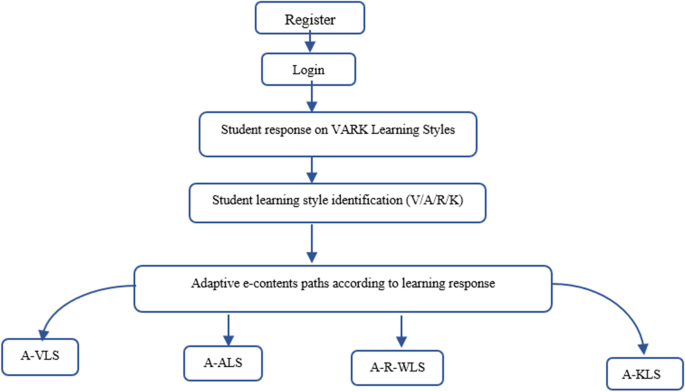
Roles and deployment diagram of the adaptive e-learning environment
The author developed a learning styles questionnaire via a mobile App. as follows: https://play.google.com/store/apps/details?id=com.pointability.vark . Then, the students accessed the adaptive e-course modules based on their learning styles.
The Implementation phase involved the following: The professional validation of the course instructional materials. Expert validation is used to evaluate the consistency of course materials (syllabi and modules). The validation was performed including the following: student learning activities, learning implementation capability, and student reactions to modules. The learner's behaviors, errors, navigation, and learning process are continuously geared toward improving the learner's modules based on the data the learner gathered about him.
The Evaluation phase included five e-learning specialists who reviewed the adaptive e-learning. After that, the framework was revised based on expert recommendations and feedback. Content assessment, media evaluation in three forms, instructional design, interface design, and usage design included in the evaluation. Adaptive learners checked the proposed framework. It was divided into two sections. Pilot testing where the proposed environment was tested by ten learners who represented the sample in the first phase. Each learner's behavior was observed, questions were answered, and learning control, media access, and time spent learning were all verified.
Research methodology
Research purpose and questions.
This research aims to investigate the impact of designing an adaptive e-learning environment on the development of students' engagement. The research conceptual framework is illustrated in Fig. 6 . Therefore, the articulated research questions are as follows: the main research question is "What is the impact of an adaptive e-learning environment based on (VARK) learning styles on developing students' engagement? Accordingly, there are two sub research questions a) "What is the instructional design of the adaptive e-learning environment?" b) "What is the impact of an adaptive e-learning based on (VARK) learning styles on development students' engagement (skills, participation, performance, emotional) in comparison with conventional e-learning?".
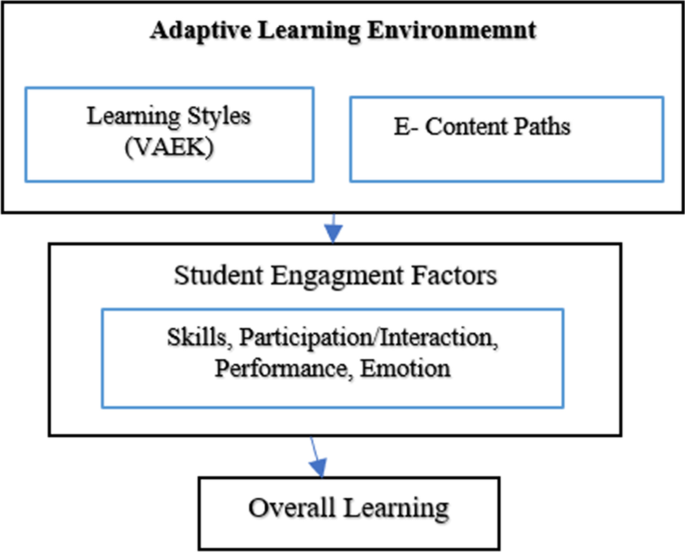
The conceptual framework (model) of the research questions
Research hypotheses
The research aims to verify the validity of the following hypothesis:
There is no statistically significant difference between the students' mean scores of the experimental group that exposed to the adaptive e-learning environment and the scores of the control group that was exposed to the conventional e-learning environment in pre-application of students' engagement scale.
There is a statistically significant difference at the level of (0.05) between the students' mean scores of the experimental group (adaptive e-learning) and the scores of the control group (conventional e-learning) in post-application of students' engagement factors in favor of the experimental group.
Research design
This research was a quasi-experimental research with the pretest-posttest. Research variables were independent and dependent as shown in the following Fig. 7 .
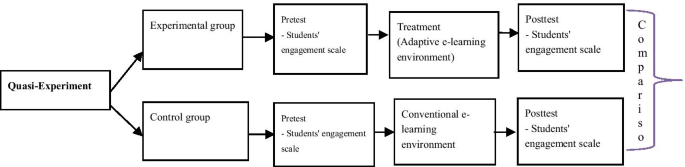
Research "Experimental" design
Both groups were informed with the learning activities tracks, the experimental group was instructed to use the adaptive learning environment to accomplish the learning goals; on the other hand, the control group was exposed to the conventional e-learning environment without the adaptive e-learning parameters.
Research participants
The sample consisted of students studying the "learning skills" course in the common first-year deanship aged between (17–18) years represented the population of the study. All participants were chosen in the academic year 2109–2020 at the first term which was taught by the same instructors. The research sample included two classes (118 students), selected randomly from the learning skills department. First-group was randomly assigned as the control group (N = 58, 31 males and 27 females), the other was assigned as experimental group (N = 60, 36 males and 24 females) was assigned to the other class. The following Table 1 shows the distribution of students' sample "Demographics data".
The instructional materials were not presented to the students before. The control group was expected to attend the conventional e-learning class, where they were provided with the learning environment without adaptive e-learning parameter based on the learning styles that introduced the "learning skills" course. The experimental group was exposed to the use of adaptive e-learning based on learning styles to learn the same course instructional materials within e-course. Moreover, all the student participants were required to read the guidelines to indicate their readiness to participate in the research experiment with permission.
Research instruments
In this research, the measuring tools included the VARK questionnaire and the students' engagement scale including the following factors (skills, participation/interaction, performance, emotional). To begin, the pre-post scale was designed to assess the level of student engagement related to the "learning skills" course before and after participating in the experiment.
VARK questionnaire
Questionnaires are a common method for collecting data in education research (McMillan & Schumacher, 2006 ). The VARK questionnaire had been organized electronically and distributed to the student through the developed mobile app and registered on the UQU system. The questionnaire consisted of 16 items within the scale as MCQ classified into four main factors (kinesthetic, auditory, visual, and R/W).
Reliability and Validity of The VARK questionnaire
For reliability analysis, Cronbach’s alpha is used for evaluating research internal consistency. Internal consistency was calculated through the calculation of correlation of each item with the factor to which it fits and correlation among other factors. The value of 0.70 and above are normally recognized as high-reliability values (Hinton et al., 2014 ). The Cronbach's Alpha correlation coefficient for the VARK questionnaire was 0.83, indicating that the questionnaire was accurate and suitable for further research.
Students' engagement scale
The engagement scale was developed after a review of the literature on the topic of student engagement. The Dixson scale was used to measure student engagement. The scale consisted of 4 major factors as follows (skills, participation/interaction, performance, emotional). The author adapted the original "Dixson scale" according to the following steps. The Dixson scale consisted of 48 statements was translated and accommodated into Arabic by the author. After consulting with experts, the instrument items were reduced to 27 items after adaptation according to the university learning environment. The scale is rated on a 5-point scale.
The final version of the engagement scale comprised 4 factors as follows: The skills engagement included (ten items) to determine keeping up with, reading instructional materials, and exerting effort. Participation/interaction engagement involved (five items) to measure having fun, as well as regularly engaging in group discussion. The performance engagement included (five items) to measure test performance and receiving a successful score. The emotional engagement involved (seven items) to decide whether or not the course was interesting. Students can access to respond engagement scale from the following link: http://bit.ly/2PXGvvD . Consequently, the objective of the scale is to measure the possession of common first-year students of the basic engagement factors before and after instruction with adaptive e-learning compared to conventional e-learning.
Reliability and validity of the engagement scale
The alpha coefficient of the scale factors scores was presented. All four subscales have a strong degree of internal accuracy (0.80–0.87), indicating strong reliability. The overall reliability of the instruments used in this study was calculated using Alfa-alpha, Cronbach's with an alpha value of 0.81 meaning that the instruments were accurate. The instruments used in this research demonstrated strong validity and reliability, allowing for an accurate assessment of students' engagement in learning. The scale was applied to a pilot sample of 20 students, not including the experimental sample. The instrument, on the other hand, had a correlation coefficient of (0.74–0.82), indicating a degree of validity that enables the instrument's use. Table 2 shows the correlation coefficient and Cronbach's alpha based on the interaction scale.
On the other hand, to verify the content validity; the scale was to specialists to take their views on the clarity of the linguistic formulation and its suitability to measure students' engagement, and to suggest what they deem appropriate in terms of modifications.
Research procedures
To calculate the homogeneity and group equivalence between both groups, the validity of the first hypothesis was examined which stated "There is no statistically significant difference between the students' mean scores of the experimental group that exposed to the adaptive e-learning environment and the scores of the control group that was exposed to the conventional e-learning environment in pre-application of students' engagement scale", the author applied the engagement scale to both groups beforehand, and the scores of the pre-application were examined to verify the equivalence of the two groups (experimental and control) in terms of students' engagement.
The t-test of independent samples was calculated for the engagement scale to confirm the homogeneity of the two classes before the experiment. The t-values were not significant at the level of significance = 0.05, meaning that the two groups were homogeneous in terms of students' engagement scale before the experiment.
Since there was no significant difference in the mean scores of both groups ( p > 0.05), the findings presented in Table 3 showed that there was no significant difference between both experimental and control groups in engagement as a whole, and each student engagement factor separately. The findings showed that the two classes were similar before start of research experiment.
Learner content path in adaptive e-learning environment
The previous well-designed processes are the foundation for adaptation in e-learning environments. There are identified entries for accommodating materials, including classification depending on learning style.: kinesthetic, auditory, visual, and R/W. The present study covered the 1st semester during the 2019/2020 academic year. The course was divided into modules that concentrated on various topics; eleven of the modules included the adaptive learning exercise. The exercises and quizzes were assigned to specific textbook modules. To reduce irrelevant variation, all objects of the course covered the same content, had equal learning results, and were taught by the same instructor.
The experimental group—in which students were asked to bring smartphones—was taught, where the how-to adaptive learning application for adaptive learning was downloaded, and a special account was created for each student, followed by access to the channel designed by the through the application, and the students were provided with instructions and training on how entering application with the appropriate default element of the developed learning objects, while the control group used the variety of instructional materials in the same course for the students.
In this adaptive e-course, students in the experimental group are presented with a questionnaire asked to answer that questions via a developed mobile App. They are provided with four choices. Students are allowed to answer the questions. The correct answer is shown in the students' responses to the results, but the learning module is marked as incomplete. If a student chooses to respond to a question, the correct answer is found immediately, regardless of the student's reaction.
Figure 8 illustrates a visual example from learning styles identification through responding VARK Questionnaire. The learning process experienced by the students in this adaptive Learning environment is as shown in Fig. 4 . Students opened the adaptive course link by tapping the following app " https://play.google.com/store/apps/details?id=com.pointability.vark ," which displayed the appropriate positioning of both the learning skills course and the current status of students. It directed students to the learning skills that they are interested in learning more. Once students reached a specific situation in the e-learning environment, they could access relevant digital instructional materials. Students were then able to progress through the various styles offered by the proposed method, giving them greater flexibility in their learning pace.
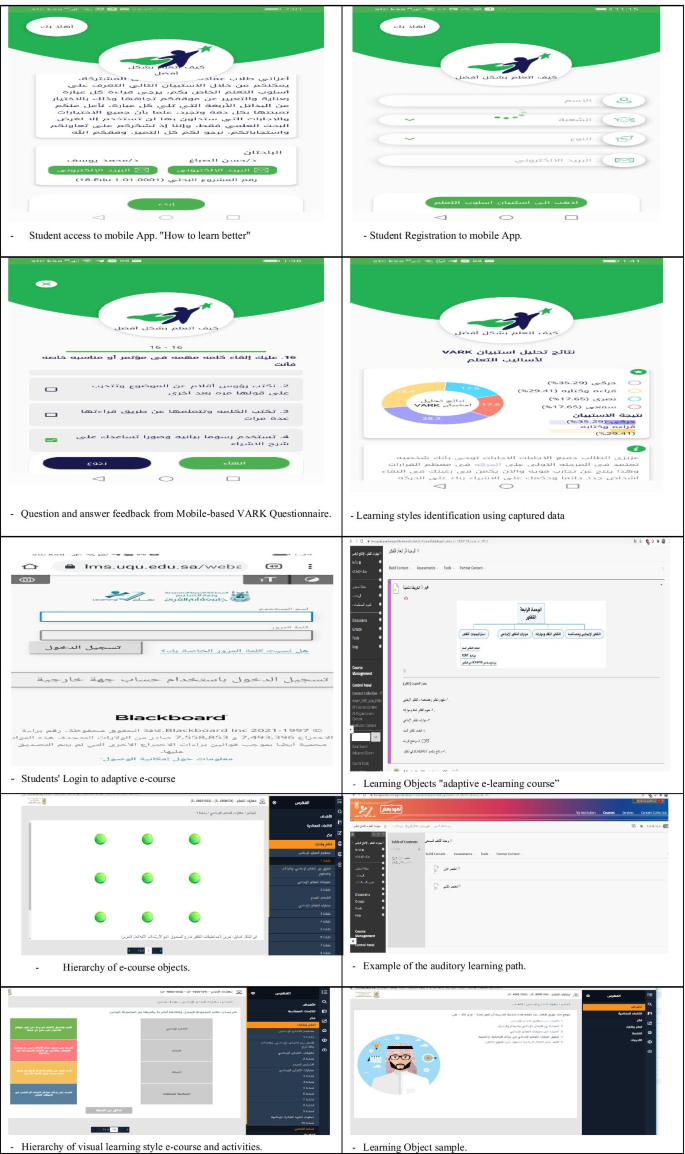
Visual example from "learning of the learning styles" identification and adaptive e-learning course process
The "flowchart" diagram below illustrates the learner's path in an adaptive e-learning environment, depending on the (VARK) learning styles (visual, auditory, kinesthetic, reading/writing) (Fig. 9 ).
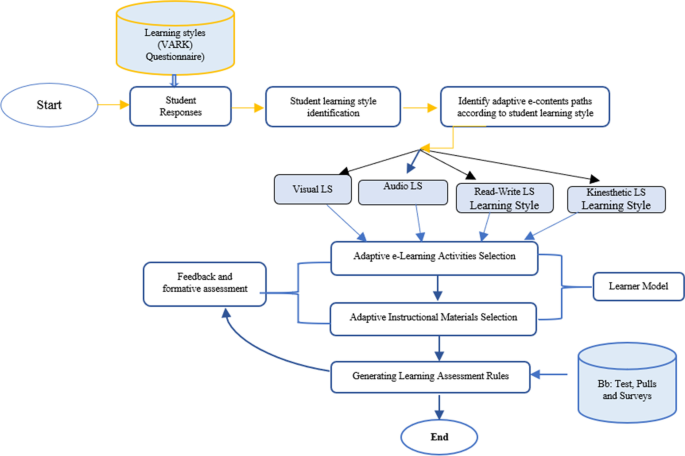
Student learning path
According to the previous design model of the adaptive framework, the students responded "Learning Styles" questionnaire. Based on each student's results, the orientation of students will direct to each of "Visual", "Aural", "Read-Write", and "Kinesthetic". The student took at the beginning the engagement scale online according to their own pace. When ready, they responded "engagement scale".
Based on the results, the system produced an individualized learning plan to fill in the gap based on the VARK questionnaire's first results. The learner model represents important learner characteristics such as personal information, knowledge level, and learning preferences. Pre and post measurements were performed for both experimental and control groups. The experimental group was exposed only to treatment (using the adaptive learning environment).
To address the second question, which states: “What is the impact "effect" of adaptive e-learning based on (VARK) learning styles on development students' engagement (skills, participation/interaction, performance, emotional) in comparison with conventional e-learning?
The validity of the second hypothesis of the research hypothesis was tested, which states " There is a statistically significant difference at the level of (0.05) between the students' mean scores of the experimental group (adaptive e-learning) and the scores of the control group (conventional e-learning) in post-application of students' engagement factors in favor of the experimental group". To test the hypothesis, the arithmetic means, standard deviations, and "T"-test values were calculated for the results of the two research groups in the application of engagement scale factors".
Table 4 . indicates that students in the experimental group had significantly higher mean of engagement post-test (engagement factors items) scores than students in the control group ( p < 0.05).
The experimental research was performed to evaluate the impact of the proposed adaptive e-learning. Independent sample t-tests were used to measure the previous behavioral engagement of the two groups related to topic of this research. Subsequently, the findings stated that the experimental group students had higher learning achievement than those who were taught using the conventional e-learning approach.
To verify the effect size of the independent variable in terms of the dependent variable, Cohen (d) was used to investigate that adaptive learning can significantly students' engagement. According to Cohen ( 1992 ), ES of 0.20 is small, 0.50 is medium, and 0.80 is high. In the post-test of the student engagement scale, however, the effect size between students' scores in the experimental and control groups was calculated using (d and r) using means and standard deviations. Cohen's d = 0.826, and Effect-size r = 0.401, according to the findings. The ES of 0.824 means that the treated group's mean is in the 79th percentile of the control group (Large effect). Effect sizes can also be described as the average percentile rank of the average treated learner compared to the average untreated learner in general. The mean of the treated group is at the 50th percentile of the untreated group, indicating an ES of 0.0. The mean of the treated group is at the 79th percentile of the untreated group, with an ES of 0.8. The results showed that the dependent variable was strongly influenced in the four behavioral engagement factors: skills: performance, participation/interaction, and emotional, based on the fact that effect size is a significant factor in determining the research's strength.
Discussions and limitations
This section discusses the impact of an adaptive e-learning environment on student engagement development. This paper aimed to design an adaptive e-learning environment based on learning style parameters. The findings revealed that factors correlated to student engagement in e-learning: skills, participation/interaction, performance, and emotional. The engagement factors are significant because they affect learning outcomes (Nkomo et al., 2021 ). Every factor's items correlate to cognitive process-related activities. The participation/interaction factor, for example, referred to, interactions with the content, peers, and instructors. As a result, student engagement in e-learning can be predicted by interactions with content, peers, and instructors. The results are in line with previous research, which found that customized learning materials are important for increasing students' engagement. Adaptive e-learning based on learning styles sets a strong emphasis on behavioral engagement, in which students manage their learning while actively participating in online classes to adapt instruction according to each learning style. This leads to improved learning outcomes (Al-Chalabi & Hussein, 2020 ; Chun-Hui et al., 2017 ; Hussein & Al-Chalabi, 2020 ; Pashler et al., 2008 ). The experimental findings of this research showed that students who learned through adaptive eLearning based on learning styles learned more; as learning styles are reflected in this research as one of the generally assumed concerns as a reference for adapting e-content path. Students in the experimental group reported that the adaptive eLearning environment was very interesting and able to attract their attention. Those students also indicated that the adaptive eLearning environment was particularly useful because it provided opportunities for them to recall the learning content, thus enhancing their overall learning impression. This may explain why students in the experimental group performed well in class and showed more enthusiasm than students in the control group. This research compared an adaptive e-learning environment to a conventional e-learning approach toward engagement in a learning skills course through instructional content delivery and assessment. It can also be noticed that the experimental group had higher participation than the control group, indicating that BB activities were better adapted to the students' learning styles. Previous studies have agreed on the effectiveness of adaptive learning; it provides students with quality opportunity that is adapted to their learning styles, and preferences (Alshammari, 2016 ; Hussein & Al-Chalabi, 2020 ; Roy & Roy, 2011 ; Surjono, 2014 ). However, it should be noted that this study is restricted to one aspect of content adaptation and its factors, which is learning materials adapting based on learning styles. Other considerations include content-dependent adaptation. These findings are consistent with other studies, such as (Alshammari & Qtaish, 2019 ; Chun-Hui et al., 2017 ), which have revealed the effectiveness of the adaptive e-learning environment. This research differs from others in that it reflects on the Umm Al-Qura University as a case study, VARK Learning styles selection, engagement factors, and the closed learning management framework (BB).
The findings of the study revealed that adaptive content has a positive impact on adaptive individuals' achievement and student engagement, based on their learning styles (kinesthetic; auditory; visual; read/write). Several factors have contributed to this: The design of adaptive e-content for learning skills depended on introducing an ideal learning environment for learners, and providing support for learning adaptation according to the learning style, encouraging them to learn directly, achieving knowledge building, and be enjoyable in the learning process. Ali et al. ( 2019 ) confirmed that, indicating that education is adapted according to each individual's learning style, needs, and characteristics. Adaptive e-content design that allows different learners to think about knowledge by presenting information and skills in a logical sequence based on the adaptive e-learning framework, taking into account its capabilities as well as the diversity of its sources across the web, and these are consistent with the findings of (Alshammari & Qtaish, 2019 ).
Accordingly, the previous results are due to the following: good design of the adaptive e-learning environment in light of the learning style and educational preferences according to its instructional design (ID) standards, and the provision of adaptive content that suits the learners' needs, characteristics, and learning style, in addition to the diversity of course content elements (texts, static images, animations, and video), variety of tests and activities, diversity of methods of reinforcement, return and support from the instructor and peers according to the learning style, as well as it allows ease of use, contains multiple and varied learning sources, and allows referring to the same point when leaving the environment.
Several studies have shown that using adaptive eLearning technologies allows students to improve their learning knowledge and further enhance their engagement in issues such as "skills, performance, interaction, and emotional" (Ali et al., 2019 ; Graf & Kinshuk, 2007 ; Murray & Pérez, 2015 ); nevertheless, Murray and Pérez ( 2015 ) revealed that adaptive learning environments have a limited impact on learning outcome.
The restricted empirical findings on the efficacy of adapting teaching to learning style are mixed. (Chun-Hui et al., 2017 ) demonstrated that adaptive eLearning technologies can be beneficial to students' learning and development. According to these findings, adaptive eLearning can be considered a valuable method for learning because it can attract students' attention and promote their participation in educational activities. (Ali et al., 2019 ); however, only a few recent studies have focused on how adaptive eLearning based on learning styles fits in diverse cultural programs. (Benhamdi et al., 2017 ; Pashler et al., 2008 ).
The experimental results revealed that the proposed environment significantly increased students' learning achievements as compared to the conventional e-learning classroom (without adaptive technology). This means that the proposed environment's adaptation could increase students' engagement in the learning process. There is also evidence that an adaptive environment positively impacts other aspects of quality such as student engagement (Murray & Pérez, 2015 ).
Conclusions and implications
Although this field of research has stimulated many interests in recent years, there are still some unanswered questions. Some research gaps are established and filled in this study by developing an active adaptive e-learning environment that has been shown to increase student engagement. This study aimed to design an adaptive e-learning environment for performing interactive learning activities in a learning skills course. The main findings of this study revealed a significant difference in learning outcomes as well as positive results for adaptive e-learning students, indicating that it may be a helpful learning method for higher education. It also contributed to the current adaptive e-learning literature. The findings revealed that adaptive e-learning based on learning styles could help students stay engaged. Consequently, adaptive e-learning based on learning styles increased student engagement significantly. According to research, each student's learning style is unique, and they prefer to use different types of instructional materials and activities. Furthermore, students' preferences have an impact on the effectiveness of learning. As a result, the most effective learning environment should adjust its output to the needs of the students. The development of high-quality instructional materials and activities that are adapted to students' learning styles will help them participate and be more motivated. In conclusion, learning styles are a good starting point for creating instructional materials based on learning theories.
This study's results have important educational implications for future studies on the effect of adaptive e-learning on student interaction. First, the findings may provide data to support the development and improvement of adaptive environments used in blended learning. Second, the results emphasize the need for more quasi-experimental and descriptive research to better understand the benefits and challenges of incorporating adaptive e-learning in higher education institutions. Third, the results of this study indicate that using an adaptive model in an adaptive e-learning environment will encourage, motivate, engage, and activate students' active learning, as well as facilitate their knowledge construction, rather than simply taking in information passively. Fourth, new research is needed to design effective environments in which adaptive learning can be used in higher education institutions to increase academic performance and motivation in the learning process. Finally, the study shows that adaptive e-learning allows students to learn individually, which improves their learning and knowledge of course content, such as increasing their knowledge of learning skills course topics beyond what they can learn in a conventional e-learning classroom.
Contribution to research
The study is intended to provide empirical evidence of adaptive e-learning on student engagement factors. This research, on the other hand, has practical implications for higher education stakeholders, as it is intended to provide university faculty members with learning approaches that will improve student engagement. It is also expected to offer faculty a framework for designing personalized learning environments based on learning styles in various learning situations and designing more adaptive e-learning environments.
Research implication
Students with their preferred learning styles are more likely to enjoy learning if they are provided with a variety of instructional materials such as references, interactive media, videos, podcasts, storytelling, simulation, animation, problem-solving, games, and accessible educational tools in an e-learning environment. Also, different learning strategies can be accommodated. Other researchers would be able to conduct future studies on the use of the "adaptive e-learning" approach throughout the instructional process, at different phases of learning, and in various e-courses as a result of the current study. Meanwhile, the proposed environment's positive impact on student engagement gained considerable interest for future educational applications. Further research on learning styles in different university colleges could contribute to a foundation for designing adaptive e-courses based on students' learning styles and directing more future research on learning styles.
Implications for practice or policy:
Adaptive e-learning focused on learning styles would help students become more engaged.
Proving the efficacy of an adaptive e-learning environment via comparison with conventional e-learning .
Availability of data and materials
The author confirms that the data supporting the findings of this study are based on the research tools which were prepared and explained by the author and available on the links stated in the research instruments sub-section. The data analysis that supports the findings of this study is available on request from the corresponding author.
Akbulut, Y., & Cardak, C. (2012). Adaptive educational hypermedia accommodating learning styles: A content analysis of publications from 2000 to 2011. Computers & Education . https://doi.org/10.1016/j.compedu.2011.10.008 .
Article Google Scholar
Al-Chalabi, H., & Hussein, A. (2020). Analysis & implementation of personalization parameters in the development of computer-based adaptive learning environment. SAR Journal Science and Research., 3 (1), 3–9. https://doi.org/10.18421//SAR31-01 .
Aldosari, M., Aljabaa, A., Al-Sehaibany, F., & Albarakati, S. (2018). Learning style preferences of dental students at a single institution in Riyadh Saudi Arabia, evaluated using the VARK questionnaire . Advances in Medical Education and Practice. https://doi.org/10.2147/AMEP.S157686 .
Ali, N., Eassa, F., & Hamed, E. (2019). Personalized Learning Style for Adaptive E-Learning System, International Journal of Advanced Trends in Computer Science and Engineering . 223-230. Retrieved June 26, 2020 from http://www.warse.org/IJATCSE/static/pdf/file/ijatcse4181.12019.pdf .
Alshammari, M., & Qtaish, A. (2019). Effective adaptive e-learning systems according to learning style and knowledge level. JITE Research, 18 , 529–547. https://doi.org/10.28945/4459 .
Alshammari, M. (2016). Adaptation based on learning style and knowledge level in e-learning systems, Ph.D. thesis , University of Birmingham. Retrieved April 18, 2019 from http://etheses.bham.ac.uk//id/eprint/6702/ .
Alshammari, M., Anane, R., & Hendley, R. (2015). Design and Usability Evaluation of Adaptive E-learning Systems based on Learner Knowledge and Learning Style. Human-Computer Interaction Conference- INTERACT , Vol. (9297), (pp. 157–186). https://doi.org/10.1007/978-3-319-22668-2_45 .
Alzain, A., Clack, S., Jwaid, A., & Ireson, G. (2018a). Adaptive education based on learning styles: Are learning style instruments precise enough. International Journal of Emerging Technologies in Learning (iJET), 13 (9), 41–52. https://doi.org/10.3991/ijet.v13i09.8554 .
Alzain, A., Clark, S., Ireson, G., & Jwaid, A. (2018b). Learning personalization based on learning style instruments. Advances in Science Technology and Engineering Systems Journal . https://doi.org/10.25046/aj030315 .
Atherton, M., Shah, M., Vazquez, J., Griffiths, Z., Jackson, B., & Burgess, C. (2017). Using learning analytics to assess student engagement and academic outcomes in open access enabling programs”. Journal of Open, Distance and e-Learning, 32 (2), 119–136.
Barkley, E., & Major, C. (2020). Student engagement techniques: A handbook for college faculty . Jossey-Bass . 10:047028191X.
Google Scholar
Becker, K., Kehoe, J., & Tennent, B. (2007). Impact of personalized learning styles on online delivery and assessment. Campus-Wide Information Systems . https://doi.org/10.1108/10650740710742718 .
Behaz, A., & Djoudi, M. (2012). Adaptation of learning resources based on the MBTI theory of psychological types. IJCSI International Journal of Computer Science, 9 (2), 135–141.
Beldagli, B., & Adiguzel, T. (2010). Illustrating an ideal adaptive e-learning: A conceptual framework. Procedia - Social and Behavioral Sciences, 2 , 5755–5761. https://doi.org/10.1016/j.sbspro.2010.03.939 .
Benhamdi, S., Babouri, A., & Chiky, R. (2017). Personalized recommender system for e-Learning environment. Education and Information Technologies, 22 , 1455–1477. https://doi.org/10.1007/s10639-016-9504-y .
Chen, P., Lambert, A., & Guidry, K. (2010). Engaging online learners: The impact of Web-based learning technology on college student engagement. Computers & Education, 54 , 1222–1232.
Chun-Hui, Wu., Chen, Y.-S., & Chen, T. C. (2017). An adaptive e-learning system for enhancing learning performance: based on dynamic scaffolding theory. Eurasia Journal of Mathematics, Science and Technology Education. https://doi.org/10.12973/ejmste/81061 .
Cletus, D., & Eneluwe, D. (2020). The impact of learning style on student performance: mediate by personality. International Journal of Education, Learning and Training. https://doi.org/10.24924/ijelt/2019.11/v4.iss2/22.47Desmond .
Cohen, J. (1992). Statistical power analysis. Current Directions in Psychological Science., 1 (3), 98–101. https://doi.org/10.1111/1467-8721.ep10768783 .
Daines, J., Troka, T. and Santiago, J. (2016). Improving performance in trigonometry and pre-calculus by incorporating adaptive learning technology into blended models on campus. https://doi.org/10.18260/p.25624 .
DeCapua, A. & Marshall, H. (2015). Implementing a Mutually Adaptive Learning Paradigm in a Community-Based Adult ESL Literacy Class. In M. Santos & A. Whiteside (Eds.). Low Educated Second Language and Literacy Acquisition. Proceedings of the Ninth Symposium (pps. 151-171). Retrieved Nov. 14, 2020 from https://www.researchgate.net/publication/301355138_Implementing_a_Mutually_Adaptive_Learning_Paradigm_in_a_Community-Based_Adult_ESL_Literacy_Class .
Dixson, M. (2015). Measuring student engagement in the online course: The online student engagement scale (OSE). Online Learning . https://doi.org/10.24059/olj.v19i4.561 .
Dominic, M., Xavier, B., & Francis, S. (2015). A Framework to Formulate Adaptivity for Adaptive e-Learning System Using User Response Theory. International Journal of Modern Education and Computer Science, 7 , 23. https://doi.org/10.5815/ijmecs.2015.01.04 .
El Bachari, E., Abdelwahed, E., & M., El. . (2011). E-Learning personalization based on Dynamic learners’ preference. International Journal of Computer Science and Information Technology., 3 , 200–216. https://doi.org/10.5121/ijcsit.2011.3314 .
El-Sabagh, H. A., & Hamed, E. (2020). The Relationship between Learning-Styles and Learning Motivation of Students at Umm Al-Qura University. Egyptian Association for Educational Computer Journal . https://doi.org/10.21608/EAEC.2020.25868.1015 ISSN-Online: 2682-2601.
Ennouamani, S., & Mahani, Z. (2017). An overview of adaptive e-learning systems. Eighth International ConfeRence on Intelligent Computing and Information Systems (ICICIS) . https://doi.org/10.1109/INTELCIS.2017.8260060 .
Evans, S., Steele, J., Robertson, S., & Dyer, D. (2017). Personalizing post titles in the online classroom: A best practice? Journal of Educators Online, 14 (2), 46–54.
Fleming, N., & Baume, D. (2006). Learning styles again: VARKing up the Right Tree! Educational Developments, 7 , 4–7.
Franzoni, A., & Assar, S. (2009). Student learning style adaptation method based on teaching strategies and electronic media. Journal of Educational Technology & Society , 12(4), 15–29. Retrieved March 21, 2020, from http://www.jstor.org/stable/jeductechsoci.12.4.15 .
Fredricks, J., Blumenfeld, P., & Paris, A. (2004). School Engagement: Potential of the Concept . State of the Evidence: Review of Educational Research. https://doi.org/10.3102/00346543074001059 .
Book Google Scholar
Gaytan, J., & McEwen, M. (2007). Effective Online Instructional and Assessment Strategies. American Journal of Distance Education, 21 (3), 117–132. https://doi.org/10.1080/08923640701341653 .
Graf, S. & Kinshuk. K. (2007). Providing Adaptive Courses in Learning Management Systems with respect to Learning Styles. Proceeding of the World Conference on eLearning in Corporate. Government. Healthcare. and Higher Education (2576–2583). Association for the Advancement of Computing in Education (AACE). Retrieved January 18, 2020 from https://www.learntechlib.org/primary/p/26739/ . ISBN 978-1-880094-63-1.
Guo, P., Kim, V., & Rubin, R. (2014). How video production affects student engagement: an empirical study of MOOC videos. Proceedings of First ACM Conference on Learning @ Scale Confernce . March 2014, (pp. 41-50). https://doi.org/10.1145/2556325.2566239 .
Hinton, P. R., Brownlow, C., McMurray, I., & Cozens, B. (2014). SPSS Explained (2nd ed., pp. 339–354). Routledge Taylor & Francis Group.
Hong, S. (2009). Developing competency model of learners in distance universities. Journal of Educational Technology., 25 , 157–186.
Hussain, I. (2017). Pedagogical implications of VARK model of learning. Journal of Literature, Languages and Linguistics, 38 , 33–37.
Hussain, M., Zhu, W., Zhang, W., & Abidi, S. (2018). Student engagement predictions in an e-learning system and their impact on student course assessment scores. Computational Intelligence, and Neuroscience. https://doi.org/10.1155/2018/6347186 .
Hussein, A., & Al-Chalabi, H. (2020). Pedagogical Agents in an Adaptive E-learning System. SAR Journal of Science and Research., 3 , 24–30. https://doi.org/10.18421/SAR31-04 .
Jaleel, S., & Thomas, A. (2019). Learning styles theories and implications for teaching learning . Horizon Research Publishing. 978-1-943484-25-6.
Johnson, M. (2009). Evaluation of Learning Style for First-Year Medical Students. Int J Schol Teach Learn . https://doi.org/10.20429/ijsotl.2009.030120 .
Jonassen, D. H., & Grabowski, B. L. (2012). Handbook of individual differences, learning, and instruction. Routledge . https://doi.org/10.1016/0022-4405(95)00013-C .
Klasnja-Milicevic, A., Vesin, B., Ivanovic, M., & Budimac, Z. (2011). E-Learning personalization based on hybrid recommendation strategy and learning style identification. Computers & Education, 56 (3), 885–899. https://doi.org/10.1016/j.compedu.2010.11.001 .
Kolekar, S. V., Pai, R. M., & Manohara Pai, M. M. (2017). Prediction of learner’s profile based on learning styles in adaptive e-learning system. International Journal of Emerging Technologies in Learning, 12 (6), 31–51. https://doi.org/10.3991/ijet.v12i06.6579 .
Lee, J., & Kim, D. (2012). Adaptive learning system applied bruner’ EIS theory. International Conference on Future Computer Supported Education, IERI Procedia, 2 , 794–801. https://doi.org/10.1016/j.ieri.2012.06.173 .
Lee, J., Song, H.-D., & Hong, A. (2019). Exploring factors, and indicators for measuring students’ sustainable engagement in e-learning. Sustainability, 11 , 985. https://doi.org/10.3390/su11040985 .
Leung, A., McGregor, M., Sabiston, D., & Vriliotis, S. (2014). VARK learning styles and student performance in principles of Micro-vs. Macro-Economics. Journal of Economics and Economic Education Research, 15 (3), 113.
Lévy, P. & Wakabayashi, N. (2008). User's appreciation of engagement in service design: The case of food service design. Proceedings of International Service Innovation Design Conference 2008 - ISIDC08 . Busan, Korea. Retrieved October 28, 2019 from https://www.researchgate.net/publication/230584075 .
Liang, J. S. (2012). The effects of learning styles and perceptions on application of interactive learning guides for web-based. Proceedings of Australasian Association for Engineering Education Conference AAEE . Melbourne, Australia. Retrieved October 22, 2019 from https://aaee.net.au/wpcontent/uploads/2018/10/AAEE2012-Liang.-Learning_styles_and_perceptions_effects_on_interactive_learning_guide_application.pdf .
Mahnane, L., Laskri, M. T., & Trigano, P. (2013). A model of adaptive e-learning hypermedia system based on thinking and learning styles. International Journal of Multimedia and Ubiquitous Engineering, 8 (3), 339–350.
Markey, M. K. & Schmit, K, J. (2008). Relationship between learning style Preference and instructional technology usage. Proceedings of American Society for Engineering Education Annual Conference & Expodition . Pittsburgh, Pennsylvania. Retrieved March 15, 2020 from https://peer.asee.org/3173 .
McMillan, J., & Schumacher, S. (2006). Research in education: Evidence-based inquiry . Pearson.
Murphy, R., Gray, S., Straja, S., & Bogert, M. (2004). Student learning preferences and teaching implications: Educational methodologies. Journal of Dental Education, 68 (8), 859–866.
Murray, M., & Pérez, J. (2015). Informing and performing: A study comparing adaptive learning to traditional learning. Informing Science. The International Journal of an Emerging Transdiscipline , 18, 111–125. Retrieved Febrauary 4, 2021 from http://www.inform.nu/Articles/Vol18/ISJv18p111-125Murray1572.pdf .
Mutahi, J., Kinai, A. , Bore, N. , Diriye, A. and Weldemariam, K. (2017). Studying engagement and performance with learning technology in an African classroom, Proceedings of Seventh International Learning Analytics & Knowledge Conference , (pp. 148–152), Canada: Vancouver.
Nainie, Z., Siraj, S., Abuzaiad, R. A., & Shagholi, R. (2010). Hypothesized learners’ technology preferences based on learning styles dimensions. The Turkish Online Journal of Educational Technology, 9 (4), 83–93.
Naqeeb, H. (2011). Learning Styles as Perceived by Learners of English as a Foreign Language in the English Language Center of The Arab American University—Jenin. Palestine. an Najah Journal of Research, 25 , 2232.
Nkomo, L. M., Daniel, B. K., & Butson, R. J. (2021). Synthesis of student engagement with digital technologies: a systematic review of the literature. International Journal of Educational Technology in Higher Education . https://doi.org/10.1186/s41239-021-00270-1 .
Normadhi, N. B., Shuib, L., Nasir, H. N. M., Bimba, A., Idris, N., & Balakrishnan, V. (2019). Identification of personal traits in adaptive learning environment: Systematic literature review. Computers & Education, 130 , 168–190. https://doi.org/10.1016/j.compedu.2018.11.005 .
Nuankaew, P., Nuankaew, W., Phanniphong, K., Imwut, S., & Bussaman, S. (2019). Students model in different learning styles of academic achievement at the University of Phayao, Thailand. International Journal of Emerging Technologies in Learning (iJET)., 14 , 133. https://doi.org/10.3991/ijet.v14i12.10352 .
Oxman, S. & Wong, W. (2014). White Paper: Adaptive Learning Systems. DV X Innovations DeVry Education Group. Retrieved December 14, 2020 from shorturl.at/hnsS8 .
Ozyurt, Ö., & Ozyurt, H. (2015). Learning style-based individualized adaptive e-learning environments: Content analysis of the articles published from 2005 to 2014. Computers in Human Behavior, 52 , 349–358. https://doi.org/10.1016/j.chb.2015.06.020 .
Pardo, A., Han, F., & Ellis, R. (2016). Exploring the relation between self-regulation, online activities, and academic performance: a case study. Proceedings of Sixth International Conference on Learning Analytics & Knowledge , (pp. 422-429). https://doi.org/10.1145/2883851.2883883 .
Pashler, H., McDaniel, M., Rohrer, D., & Bjork, R. (2008). Learning styles: concepts and evidence. Psychology Faculty Publications., 9 (3), 105–119. https://doi.org/10.1111/j.1539-6053.2009.01038.x .
Qazdar, A., Cherkaoui, C., Er-Raha, B., & Mammass, D. (2015). AeLF: Mixing adaptive learning system with learning management system. International Journal of Computer Applications., 119 , 1–8. https://doi.org/10.5120/21140-4171 .
Robinson, C., & Hullinger, H. (2008). New benchmarks in higher education: Student engagement in online learning. Journal of Education for Business, 84 , 101–109.
Rogers-Stacy, C., Weister, T., & Lauer, S. (2017). Nonverbal immediacy behaviors and online student engagement: Bringing past instructional research into the present virtual classroom. Communication Education, 66 (1), 37–53.
Roy, S., & Roy, D. (2011). Adaptive e-learning system: a review. International Journal of Computer Trends and Technology (IJCTT), 1 (1), 78–81. ISSN:2231-2803.
Shi, L., Cristea, A., Foss, J., Qudah, D., & Qaffas, A. (2013). A social personalized adaptive e-learning environment: a case study in topolor. IADIS International Journal on WWW/Internet., 11 , 13–34.
Shih, M., Feng, J., & Tsai, C. (2008). Research and trends in the field of e-learning from 2001 to 2005: A content analysis of cognitive studies in selected journals. Computers & Education, 51 (2), 955–967. https://doi.org/10.1016/j.compedu.2007.10.004 .
Silva, A. (2020). Towards a Fuzzy Questionnaire of Felder and Solomon for determining learning styles without dichotomic in the answers. Journal of Learning Styles, 13 (15), 146–166.
Staikopoulos, A., Keeffe, I., Yousuf, B. et al., (2015). Enhancing student engagement through personalized motivations. Proceedings of IEEE 15th International Conference on Advanced Learning Technologies , (pp. 340–344), Taiwan: Hualien. https://doi.org/10.1109/ICALT.2015.116 .
Surjono, H. D. (2014). The evaluation of Moodle-based adaptive e-learning system. International Journal of Information and Education Technology, 4 (1), 89–92. https://doi.org/10.7763/IJIET.2014.V4.375 .
Truong, H. (2016). Integrating learning styles and adaptive e-learning system: current developments, problems, and opportunities. Computers in Human Behavior, 55 (2016), 1185–1193. https://doi.org/10.1016/j.chb.2015.02.014 .
Umm Al-Qura University Agency for Educational Affairs (2020). Common first-year Deanship, at Umm Al-Qura University. Retrieved February 3, 2020 from https://uqu.edu.sa/en/pre-edu/70021 .
Vassileva, D. (2012). Adaptive e-learning content design and delivery based on learning style and knowledge level. Serdica Journal of Computing, 6 , 207–252.
Veiga, F., Robu, V., Appleton, J., Festas, I & Galvao, D. (2014). Students' engagement in school: Analysis according to self-concept and grade level. Proceedings of EDULEARN14 Conference 7th-9th July 2014 (pp. 7476-7484). Barcelona, Spain. Available Online at: http://hdl.handle.net/10451/12044 .
Velázquez, A., & Assar, S. (2009). Student learning styles adaptation method based on teaching strategies and electronic media. Educational Technology & SocieTy., 12 , 15–29.
Verdú, E., Regueras, L., & De Castro, J. (2008). An analysis of the research on adaptive Learning: The next generation of e-learning. WSEAS Transactions on Information Science and Applications, 6 (5), 859–868.
Willingham, D., Hughes, E., & Dobolyi, D. (2015). The scientific status of learning styles theories. Teaching of Psychology., 42 (3), 266–271. https://doi.org/10.1177/0098628315589505 .
Yalcinalp & Avcı. (2019). Creativity and emerging digital educational technologies: A systematic review. The Turkish Online Journal of Educational Technology, 18 (3), 25–45.
Yang, J., Huang, R., & Li, Y. (2013). Optimizing classroom environment to support technology enhanced learning. In A. Holzinger & G. Pasi (Eds.), Human-computer interaction and knowledge discovery in complex (pp. 275–284). Berlin: Springer.
Zhang, H. (2017). Accommodating different learning styles in the teaching of economics: with emphasis on fleming and mills¡¯s sensory-based learning style typology. Applied Economics and Finance, 4 (1), 72–78.
Download references
Acknowledgements
The author would like to thank the Deanship of Scientific Research at Umm Al-Qura University for the continuous support. This work was supported financially by the Deanship of Scientific Research at Umm Al-Qura University to Dr.: Hassan Abd El-Aziz El-Sabagh. (Grant Code: 18-EDU-1-01-0001).
Author information
Hassan A. El-Sabagh is an assistant professor in the E-Learning Deanship and head of the Instructional Programs Department, Umm Al-Qura University, Saudi Arabia, where he has worked since 2012. He has extensive experience in the field of e-learning and educational technologies, having served primarily at the Educational Technology Department of the Faculty of Specific Education, Mansoura University, Egypt since 1997. In 2011, he earned a Ph.D. in Educational Technology from Dresden University of Technology, Germany. He has over 14 papers published in international journals/conference proceedings, as well as serving as a peer reviewer in several international journals. His current research interests include eLearning Environments Design, Online Learning; LMS-based Interactive Tools, Augmented Reality, Design Personalized & Adaptive Learning Environments, and Digital Education, Quality & Online Courses Design, and Security issues of eLearning Environments. (E-mail: [email protected]; [email protected]).
Authors and Affiliations
E-Learning Deanship, Umm Al-Qura University, Mecca, Saudi Arabia
Hassan A. El-Sabagh
Faculty of Specific Education, Mansoura University, Mansoura, Egypt
You can also search for this author in PubMed Google Scholar
Contributions
The author read and approved the final manuscript.
Corresponding author
Correspondence to Hassan A. El-Sabagh .
Ethics declarations
Competing interests.
The author declares that there is no conflict of interest
Additional information
Publisher's note.
Springer Nature remains neutral with regard to jurisdictional claims in published maps and institutional affiliations.
Rights and permissions
Open Access This article is licensed under a Creative Commons Attribution 4.0 International License, which permits use, sharing, adaptation, distribution and reproduction in any medium or format, as long as you give appropriate credit to the original author(s) and the source, provide a link to the Creative Commons licence, and indicate if changes were made. The images or other third party material in this article are included in the article's Creative Commons licence, unless indicated otherwise in a credit line to the material. If material is not included in the article's Creative Commons licence and your intended use is not permitted by statutory regulation or exceeds the permitted use, you will need to obtain permission directly from the copyright holder. To view a copy of this licence, visit http://creativecommons.org/licenses/by/4.0/ .
Reprints and permissions
About this article
Cite this article.
El-Sabagh, H.A. Adaptive e-learning environment based on learning styles and its impact on development students' engagement. Int J Educ Technol High Educ 18 , 53 (2021). https://doi.org/10.1186/s41239-021-00289-4
Download citation
Received : 24 May 2021
Accepted : 19 July 2021
Published : 01 October 2021
DOI : https://doi.org/10.1186/s41239-021-00289-4
Share this article
Anyone you share the following link with will be able to read this content:
Sorry, a shareable link is not currently available for this article.
Provided by the Springer Nature SharedIt content-sharing initiative
- Adaptive e-Learning
- Learning style
- Learning impact
- Harvard Division of Continuing Education
- DCE Theses and Dissertations
- Communities & Collections
- By Issue Date
- FAS Department
- Quick submit
- Waiver Generator
- DASH Stories
- Accessibility
- COVID-related Research
Terms of Use
- Privacy Policy
- By Collections
- By Departments
A Study of Factors Influencing Teachers’ Usage of E-Learning for Teaching Math in the Public Secondary Schools in Makati City, Philippines

Citable link to this page
Collections.
- DCE Theses and Dissertations [1330]
Contact administrator regarding this item (to report mistakes or request changes)
An analysis of students' perspectives on e-learning participation – the case of COVID-19 pandemic
International Journal of Information and Learning Technology
ISSN : 2056-4880
Article publication date: 17 May 2021
Issue publication date: 24 June 2021
During the COVID-19 pandemic, educational institutions were forced to shut down, causing massive disruption of the education system. This paper aims to determine the critical factors for the intention to participate in e-learning during COVID-19.
Design/methodology/approach
Data were collected by surveying 131 university students and structural equation modelling technique using PLS-SEM was employed to analysis the data.
The results showed that the COVID-19 related factors such as perceived challenges and COVID-19 awareness not only directly impact students' intention but also such effects are mediated through perceived usefulness and perceived ease of use of e-learning systems. However, the results showed that the educational institution's preparedness does not directly impact the intention of students to participate in e-learning during COVID-19. The results also showed that the gender and length of the use of e-learning systems impact students' e-learning systems use.
Originality/value
These results demonstrated that, regardless of how well the educational institutions are prepared to promote the use of e-learning systems, other COVID-19-related challenges play a crucial role in forming the intention of students to participate in e-learning during the COVID-19 pandemic. Theoretical and practical implications are provided.
- Distance learning
- Higher education
- Online education
Nikou, S. and Maslov, I. (2021), "An analysis of students' perspectives on e-learning participation – the case of COVID-19 pandemic", International Journal of Information and Learning Technology , Vol. 38 No. 3, pp. 299-315. https://doi.org/10.1108/IJILT-12-2020-0220
Emerald Publishing Limited
Copyright © 2021, Shahrokh Nikou and Ilia Maslov
Published by Emerald Publishing Limited. This article is published under the Creative Commons Attribution (CC BY 4.0) licence. Anyone may reproduce, distribute, translate and create derivative works of this article (for both commercial and non-commercial purposes), subject to full attribution to the original publication and authors. The full terms of this licence may be seen at http://creativecommons.org/licences/by/4.0/legalcode
1. Introduction
The COVID-19 pandemic is the defining global health crisis of our time, and it is adding a fair amount of complexity in how different activities are being conducted ( Adnan and Anwar, 2020 ). Such effects are crucial on higher education, forcing all teaching and learning activities to face a sudden transition to wholly online learning contexts ( Toquero, 2020 ). While the educational environments are still struggling with the digitalisation and digital transformation challenges and finding optimal ways to adapt, the Coronavirus pandemic has fundamentally affected their core: staff and students ( Adedoyin and Soykan, 2020 ; Aristovnik et al. , 2020 ; Strauß and Rummel, 2020 ). For them, the period is inevitably very stressful as all learning and teaching activities – e.g. all classes, meetings, seminars, supervisions and exams were forced to move online within short notice ( Bao, 2020 ; Hodges et al. , 2020 ). Though such transformation is not entirely new for such institutions, they are all now forced to move away from traditional teaching and learning structures to a virtual environment as old education models are no longer adaptable to the challenges of rapidly changing educational environments ( Van Nuland et al. , 2020 ).
In the educational environments, information and communications technology (ICT) has been extensively used to deliver information for education and learning, and e-learning has been an emerging paradigm of modern education ( Sun et al. , 2008 ). E-learning relies on the use of multiple information systems, services and technologies. Information system encompasses information service and information technology (IT), where service is understood as the use of IT. Furthermore, the user experience (UX) and usability of information technology and services also affect e-learning process, not only the technical aspects, but also the social aspects ( Nakamura et al. , 2017 ). Given the relatively recent events in terms of COVID-19 and quarantine situation worldwide, e-learning has become increasingly important as one of the optimal solutions for education ( Radha et al. , 2020 ). We argue that in order to understand better factors influencing individual decision to participate in e-learning in a worldwide quarantine situation, comprehensive research with a holistic approach is needed. Hence, we aim to address this issue by assessing students' experience in their participation in e-learning. Based on this aim, the research question guides this study is What antecedent factors impact students ' intention to participate in e-learning during the COVID-19 quarantine? To answer the stated research question, we develop an integrated theoretical model that encompasses several antecedent factors (perceived challenges during COVID-19, school and teachers' perceived preparedness) and constructs from Technology Acceptance Model (TAM: Davis, 1989 ), perceived usefulness and perceived ease of use ( Yu, 2020 ). We conduct empirical research and collect data through an online survey questionnaire, focusing on university students as the target group. The data will be analysed through structural equation modelling (SEM) using SmartPLS v. 3.
The rest of this paper is structured as follows: Section 2 presents the literature review with the operationalisation of the required terminology and theoretical framework for the study. Section 3 provides the theoretical framework and hypotheses. Section 4 describes the methodology, research design, and data collection. Section 5 provides the results followed by Section 6 , providing discussions. Section 7 concludes the research and outlines the limitations and recommendations for future research.
2. Literature review
2.1 e-learning and participation in e-learning concepts.
To support e-learning, learning management systems (LMS) is increasingly being used, which are e-learning software that can be used to empower teachers to enrich students' learning ( Bansode and Kumbhar, 2012 , p. 415). LMS is a powerful software system enhancing learning and provides automated delivery of the course content and tracking of the learning progress of the students ( Dalsgaard, 2006 ). Sun et al. (2008 , p. 1183) defined e-learning as the use of telecommunication to deliver information for education and training. Garrison and Anderson (2003) defined e-learning participation as teaching and learning facilitated and supported by Internet technologies. In this research, e-learning is defined as the overall technological system to deliver teaching, whereas participation in e-learning is the act of use of telecommunication to deliver teaching and learning within such a system. Khan (2004) defined e-learning as an iterative process that goes from the planning stage through design, production and evaluation to delivery and maintenance stages. However, there are both advantages and disadvantages to e-learning. On a positive side, e-learning allows for a learner-centred, self-paced, cost-effective way of learning and on a negative side, there is a lack of social interactions, higher degrees of frustration and confusion, with higher preparation time for instructors ( Zhang et al. , 2012 ).
Sun et al. (2008) stated that personal perceptions about e-learning could influence attitudes and impact whether a user would intend to use to e-learning in the future. Uppal et al. (2018) and Kim and Frick (2011) mentioned that the supportiveness of the service, information quality and system quality are different aspects of e-learning quality, which could also impact the decision of the users. Moreover, Benigno and Trentin (2000) stated that e-learning is potentially affected by factors such as student characteristics, student-student interaction, learning materials, learning environment, and information technology (IT). Also, Selim (2007) mentioned that there are eight critical success factors of participation in e-learning (e.g. instructor’s attitude towards and control of the technology and student motivation and technical competency). Furthermore, Sun et al. (2008) suggested that perceived e-learning satisfaction is depended on the six dimensions: learner, instructor, course, technology, design and environmental. Sun et al. (2008) concluded that learner computer anxiety, instructor attitude toward e-learning, e-learning course flexibility, e-learning course quality, perceived usefulness, perceived ease of use, and diversity in assessments were the critical factors affecting learner's perceived satisfaction.
Garavan et al. (2010) conceptualised participation in e-learning and quantitatively validated the research model. In their model, the participation in e-learning is formed by the general-person characteristics (e.g. age and social class), motivation to learn and instructional design characteristics of e-learning (content quality and learner support, feedback and recognition). Additionally, the perceived barriers and enablers to e-learning are potentially affected by the proper instructional design of e-learning. Fleming et al. (2017) identified that predictors of future use and overall satisfaction from using e-learning are low perceived complexity of the e-learning system, the knowledge of e-learning, and available technical support for e-learning. Zhang et al. (2012) presented a research model that evaluates the impact of multiple factors on the intention to continue participation in the e-learning systems. Zhang et al. (2012) concluded that the intention to participate depends directly and indirectly on the psychological safety communication climate, on the perceived responsiveness of e-learning system and self-efficacy, as well as satisfaction from the previous use of the system. Furthermore, satisfaction and membership of the community were found to affect the intention to continue participation in e-learning.
2.2 Blended learning: boundaries between physical and virtual
Hrastinski (2008) stated that e-learning participation does not only occur online but also takes place offline. This is mainly due to the fact that e-learning requires time and energy to learn, communication, thinking and assessing what learners have obtained from e-learning communities in more traditional learning settings. Literature on e-learning is primarily on the so-called blended learning of physical and digital learning and Anthony et al. (2020) stated that blended learning (BL) has been increasing in popularity and demand. However, recent literature on the issue seems to be dominated with the factors of educator presence in online settings, interactions between students, teachers and content, and designed connections between online and offline activities as well as between campus-related and practice-related activities.
Wilson (2009 , p. 20) stated that “learning space continuum has two types of conditions at its extremities, wholly independent self-directed unstructured learning at one end and structured teacher-led didactic learning environments at the other”. Furthermore, Wilson (2009) identified different places for learning spectrums, ranging from unstructured that corresponds to home, bar, cafe or gym to lecture theatre and seminar places for holding workshops. The notion of learning space continuum may become necessary when we take into consideration e-learning. As Ellis and Goodyear (2016 , p. 150) identified, the “boundaries” between the physical and the virtual are become less transparent and more permeable, in addition to the greater need of students of being capable of using digital technologies to discover and construct knowledge that is meaningful to them.
Hence, we argue that e-learning participation cannot be defined narrowly as a specific activity in a specific context, but rather a range of activities, some of which may be even blended with the physical (more traditional) learning and interaction with teachers or other students in a more structured or unstructured manner. This could have a significant impact on the way not only e-learning, but the overall learning process is structured, including how the different technologies are used, how the instructional learning programs are structured, what are the social interrelationships between the students, instructors, organisations, and how the success of learning is measured.
2.3 COVID-19, quarantine and e-learning
Kaplan et al. (2020) stated that a third of the global population worldwide was on a quarantine lockdown in order to limit the spread of the COVID-19. This action led to the social distancing and thus fewer social connections, which also included closures of commercial enterprises and higher educations, resulting in limited physical presence and social interactions between the people. The impact of COVID-19 is also seen in the educational environments, with a potential to experience unparalleled transformations, just as many other human spheres of behaviour, which are facilitated by the advents in the development of IT, such as 5G ( Kaplan et al. , 2020 , p. 4). Paraschi (2020 , p. 19) stated that e-learning might even be an alternative activity that is to help communities previously relying on other activities, such as competitive educational and training e-learning programs blended with on-site summer schools in a Greek island as a replacement for tourism, which suffered greatly during the COVID-19 pandemic.
However, there are multiple challenges related to e-learning that come as a result of COVID-19. For instance, Almaiah et al. (2020) identified the critical challenges and factors of e-learning system usage during COVID-19 pandemic. In the research, the authors covered the topics of e-learning system quality, trust, culture, self-efficacy, and issues of financial support, change management and technical maintenance, all of which were mentioned as potentially influential factors of e-learning adoption. Moreover, we argue that COVID-19 pandemic is a challenge impacting the approach to e-learning, thus requiring adaptation and innovation in higher education to cope with the posed challenge. Alea et al. (2020) have evaluated the perceptions among the teachers about the impact of COVID-19 and the community quarantine on the distance learning and found multiple challenges related to it, as well as individual issues with preparedness for delivering distance learning. Also, Abbasi et al. (2020) stated that students did not prefer e-teaching over face-to-face teaching during the lockdown situation, and that administration and faculty members must take necessary measures to improve e-learning during the lockdown. Favale et al. (2020) stated that in the context of 80–90% of people in Italy staying at home during the quarantine, remote working and online collaboration exploded in an Italian university. Thus, the research on participation in e-learning in the context of COVID-19 is very relevant and timely.
2.4 Information service, information systems and information technology
In literature, information service is defined as “a component of an information system representing a well-defined business unit that offers capabilities to realise business activities and owns resources (data, rules, roles) to realise these capabilities” ( Ralyté et al. , 2015 , p. 39). Furthermore, Wijnhoven and Kraaijenbrink (2008 , p. 93) suggested that information services are “services that facilitate the exchange of information goods with or without transforming these goods”. The authors (2008, p. 114) stated that “information services have a lot in common with other types of information systems”, hence implying that the information services are distinct from the information systems. Importantly, it is necessary to outline that information system (IS) is defined as any combination of information technology (IT) and people's activities using that technology ( Gupta, 2000 ).
Accordingly, IT consists of telecommunications, computing, and content, whereby different types of IT are represented at the intersections (e.g. Internet being partly computing, and partly telecommunications). Hence, one may wonder about the exact definitions of an information service, an information system, an information technology and what is the interrelation between them. It is essential to underline that the terms are potentially having blurry boundaries and are hard to define. For the purposes of this particular study, information service is defined as the use of information technology by people. However, the information system of e-learning at large is not considered to be limited only to LMS such as Moodle as there are many other physical and virtual information services that could facilitate e-learning. This study will try to focus on the information services of e-learning that facilitate participation over IT.
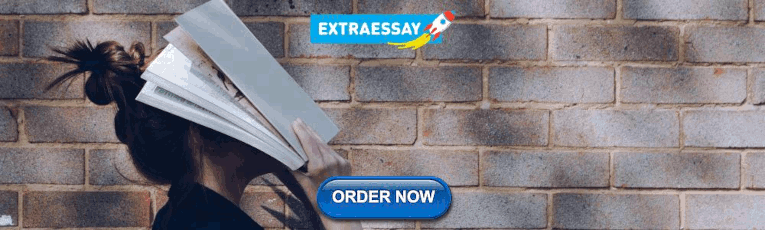
3. Theoretical framework and hypothesis development
Ke and Hoadley (2009) suggested that there is no “one size fits all frameworks” when evaluating online learning communities. From the literature on e-learning, there are a number of identified antecedent factors that could potentially influence participation in e-learning. Besides, factors related to the current situation of pandemic (COVID-19) may also impact the participation in e-learning. The research model for this study is developed based on the literature review outlined above. Firstly, several antecedent factors that may affect participation in e-learning are identified. Secondly, these factors are used to build a theoretical framework which will be evaluated and examined empirically.
3.1 COVID-19 related factors
At the time of writing the paper, the research on the COVID-19 is new, as it is a relatively recent event. Hence, the exploratory purpose of the paper is to identify potential factors that may impact e-learning participation in quarantine time. Therefore, we aim to review the most recently published studies on this topic. For example, Alea et al. (2020) have recently performed a research on the opinions of teachers concerning the preparedness and challenges that the university might face when adopting e-learning in the times of the quarantine. They empirically evaluated the (1) awareness of the COVID-related situation, (2) the teacher's readiness and school's preparedness to conduct distance learning, and (3) perceived challenges in distance learning education ( Musingafi et al. , 2015 ). In this study, nevertheless, as we plan to survey students instead of teachers, we adapt the same survey questions and modify them slightly to fit the context of our study. As such, we use (1) awareness of COVID-19, (2) perceived challenges to participate in e-learning during the quarantine, (3) perceived educational institutions preparedness [perceived teachers' preparedness and perceived school's preparedness] to conduct distance learning, as the COVID-19 related factors to examine the students' intention to e-learning participation.
Awareness of COVID-19 has a positive effect on the intention to e-learning participation.
Awareness of COVID-19 has a positive effect on perceived usefulness.
Awareness of COVID-19 has a positive effect on perceived ease of use.
Perceived challenges during COVID-19 has a negative effect on the intention to e-learning participation.
Perceived challenges during COVID-19 has a negative effect on perceived usefulness.
Perceived challenges during COVID-19 has a negative effect on perceived ease of use.
Perceived educational institutions preparedness during COVID-19 has a positive effect on the intention to e-learning participation.
Perceived educational institutions preparedness during COVID-19 has a positive effect on perceived usefulness.
Perceived educational institutions preparedness during COVID-19 has a positive effect on perceived ease of use.
3.2 Perceived usefulness of e-learning
Perceived usefulness has a significant effect on the intention to e-learning participation.
3.3 Perceived ease of use of e-learning
Perceived ease of use has a significant effect on the intention to e-learning participation.
Perceived ease of use has a significant effect on perceived usefulness.
3.4 Intention to participate in e-learning
In the current study, our dependent variable is e-learning participation, which is measured by the student's intention to participate. There may be multiple different factors that could affect the intention of students to participate in e-learning during the quarantine situation. Prior studies in e-learning research use intention to participate in e-learning ( Masrom, 2007 ; Tselios et al. , 2011 ; Zhang et al. , 2012 ; Park, 2009 ) as the outcome variable.
Moreover, we intend to examine several potential individual characteristics as control variables when assessing the model. We argue that the younger students are more accepting the use of IT for learning. Evidence is paradoxical in this aspect, as Fleming et al. (2017) stated that age does not impact the intention of using e-learning. Ong and Lai (2006) stated that gender might indirectly affect the acceptance of e-learning, as men and women had different perceptions of PU and PEOU of e-learning systems. The theoretical framework model is provided in Figure 1 .
4. Methodology
The data collection was done between 15 August to 15 October 2020 through an online survey when closure of all educational institution, specifically higher education was announced by the Finnish government started from March 2020. Prior to the primary data collection, survey items (instruments) to measure five factors predicting the use of e-learning during COVID-19 among higher education students were adopted from previously validated studies and based on the adaptation process, the items for the current study were slightly modified suit the contexts of the study, COVID-19 and e-learning.
The items for measuring COVID-19 awareness (three items), perceived teachers and school preparedness (six items) and perceived COVID-19 challenges (four items) all were derived from Alea et al. (2020 , pp. 134–136). Survey items for measure perceived usefulness (four items) and perceived ease of use (four items) were derived from Masrom (2007) and Davis (1989) . Finally, items for measuring intention to participate in e-learning during the COVID-19 were derived from Lee et al. (2009) and Davis (1989) . The model measurement and assessment of the constructs were done through the use of SmartPLS 3.2 that was guided by the procedures of Partial Least Squares Structural Equation Modelling (PLS-SEM).
4.1 Data collection
During the school closures, the survey instrument was distributed through an online survey application. The data were obtained only from those respondents who indicated they are currently university students. As mentioned, the data collection was formed in the course of two months, and over 350 invitations were sent. After the closure of the survey, 153 responses were received. Upon further examination of the completeness of the data and removing unengaged responses or those who indicated that they are not currently students, in total, 131 responses were included in the dataset for further analysis.
5.1 Descriptive statistics
Of the respondents, 73 (55.7%) were female, while 56 (42.7%) respondents were males, and two did not want to reveal their gender. The average age of respondents was 25 years old with (standard dev. = 6.1). Moreover, the highest degree of the respondents was as follow: high school diploma ( N = 63), bachelor's degree ( N = 40), master's degree ( N = 19), and PhD or other ( N = 9). We also asked respondents to indicate how long in total have they been using e-learning systems. The following information was retrieved; less than a year ( N = 61), between one to three years ( N = 37), more than three years ( N = 32) and only one respondent indicated has never used such learning systems. We also asked the respondent to indicate to what extent the instructor's teaching style would impact their decision to participate in e-learning. We asked, “the instructor encourages and motivates me to use e-learning”, or “the instructor's style of presentation holds my interest”. The results showed that 36 students thought the teaching style of the instructor would motivate and encourage them to use e-learning systems and interestingly 23 students mentioned it does not affect their intention or the effect is not considerable. Regarding the second question, we found 28 students who believed that the instructor's presentation style would have a substantial impact on their intention to use e-learning systems to participate in e-learning. The same number of ( N = 28) students believed that the instructor's presentation style does not at all play a role in their decision to use such systems for e-learning participation, or the effect is somewhat limited.
5.2 Measurement results
In the following, we report on the data analysis at the measurement model, which refers to the assessment of the measures' reliability and their validity. In doing so, we computed: (1) item (indicator) loadings and internal consistency reliability, (2) convergent validity, and (3) discriminant validity ( Hair et al. , 2019 ).
5.2.1 Item loadings and internal consistency reliability
PLS-SEM results were utilised for the item loadings in this study. Table 1 shows the detail of item loadings. As shown in Table 1 , all item loadings (except one item PCHA_2 with the slightly lower value) satisfied the recommended loading values of >0.70 ( Hair et al. , 2019 ). However, from the algorithm process in PLS-SEM, one item (indicator) from the COVID-19 awareness (CAWA_3) was dropped. Therefore, 24 items remained for the next step of the PLS-SEM analysis. Internal consistency reliability refers to the evaluation findings for the statistical consistency across survey items (indicators). According to Hair et al. (2019) , internal consistency reliability should be reported through Cronbach's alpha ( α ) and Composite Reliability (CR). Therefore, we computed these two tests and the values achieved were all above to the recommended threshold of 0.70 ( Hair et al. , 2019 ) providing good internal consistencies.
5.2.2 Convergent validity and discriminant validity
Convergent validity is a statistical measure that assesses the construct validity, and it suggests that assessments having similar or same constructs should be positively related. Regarding the convergent validity, the value s of average variance extracted (AVE) must be reported. As shown in Table 1 , all the AVE values were above the recommended threshold of 0.50.
Discriminant validity test examines the extent to which a construct is different from other constructs ( Hair et al. , 2019 ). In order to report the values, the Fornell Larcker criterion will be used, and the AVE scores of a construct should be lower than the shared variance for all model constructs. As shown in Table 2 , all the AVE scores satisfied this condition, and therefore, the discriminant validity was established based on the evaluation of the Fornell Larcker criterion ( Fornell and Larcker, 1981 ).
However, as we used the PLS-SEM approach to perform the data analysis, we also assessed the discriminant validity through the Heterotrait-Monotrait Ratio of Correlations (HTMT). Discriminant validity problems also appear when HTMT values are higher than 0.90. The construct can be similar if HTMT shows a value of >0.90, which in this case, it indicates the lack of discriminant validity. Table 3 shows the HTMT values, and as it is indicated, all values were lower than 0.90.
We also examined the collinearity by reporting Variance Inflation Factor (VIF) values. The collinearity will be an issue if the VIF value is above 3.00 ( Hair et al. , 2019 ). Perceived usefulness (VIF = 1.663) and perceived ease of use (VIF = 1.559) are the predictor of intention to participate in e-learning during the COVID-19. Moreover, COVID-19 awareness is the predictor of perceived usefulness (VIF = 1.064) and perceived ease of use (VIF = 1.064). Perceived educational institutions preparedness predict perceived usefulness (VIF = 1.087) and perceived ease of use (VIF = 1.087). Perceived COVID-19 challenges predict perceived usefulness (VIF = 1.088) and perceived ease of use (VIF = 1.088). Therefore, the collinearity test results show that collinearity does not emerge as an issue in this study ( Hair et al. , 2019 ).
5.3 Structural results
The structural model assessment was performed following Hair et al. (2019) recommendation. In order to assess the path coefficient between endogenous and exogenous constructs, the sample was bootstrapped through 5.000 sub-sampling. The results of the SRMR indicator estimating the goodness of fit of the structural model was 0.065. The structural results showed that most of the hypotheses were supported ( Table 4 and Figure 2 ). The outcome variable, i.e. intention to participate in e-learning was explained by variance of 69%. Moreover, the perceived usefulness and perceived ease of use were explained by variance of 21% and 15%, respectively. The SEM results showed that the path between COVID-19 awareness to intention to participate in e-learning was significant ( β = 0.192; t = 3.220; p = 0.001); therefore, H1 was supported by the model. The SEM results also showed that the path between COVID-19 awareness to perceived usefulness ( β = 0.243; t = 2.748; p = 0.005) was significant; thus H1a was supported by the model. However, the COVID-19 awareness to perceived ease of use was not significant; thus H1b was rejected by the model.
The SEM results showed that the path between perceived challenges, as expected, negatively impact intention to participate in e-learning ( β = −0.186; t = 2.789; p = 0.005); therefore, H2 was supported by the model. The SEM results also showed that the path between perceived challenges during the COVID-19, as expected, negatively impact both perceived usefulness ( β = −0.36; t = 4.599; p = 0.001) and ( β = −0.246; t = 3.167; p = 0.002), thus H2a and H2b were both supported by the model. In addition, the SEM results showed that the path between perceived educational institutions preparedness to intention to participate in e-learning was not significant; therefore, H3 was rejected by the model. This finding is similar to Zia (2020) who also found that the curriculum and technology have a negative impact on the online classes during the COVID-19 pandemic. Furthermore, the SEM results showed that the path between perceived educational institutions preparedness to PU was also not significant; thus H3a was rejected by the model. However, perceived educational institutions preparedness to PEOU was significant ( β = 0.235; t = 2.365; p = 0.02), thus H3b was supported by the model. Finally, the strongest relationship emerged between the path from perceived usefulness to participate in e-learning ( β = 0.623; t = 9.225; p = 0.001); therefore, H4 was supported by the model. However, the results showed that the path between perceived ease of use to participate in e-learning was significant was not significant; thus, H5 was rejected by the model. As per path between PEOU to PU, the SEM results showed a significant effect of PEOU to PU ( β = 0.484; t = 6.220; p = 0.001); thus H5a was supported by the model.
We also examined the mediating effect of perceived usefulness and ease of use between the COVID-19 related factors and intention to participate in e-learning. To do so, we first accounted for the results of total indirect effects and then examined the specific indirect effects values, as PLS-SEM procedures required. The mediation test results showed the total indirect effects for the paths between COVID-19 awareness ( β = 0.161; t = 2.618; p = 0.01), and perceived challenges ( β = −0.251; t = 4.630; p = 0.001) to intention to participate in e-learning were significant, indicating that there might be mediation effects in these path relationships. Therefore, we checked the specific indirect effects values and found that theses paths are mediated only through perceived usefulness. The result showed that the paths between COVID-19 awareness ( β = 0.152; t = 2.553; p = 0.01) and perceived challenges ( β = −0.224; t = 4.187; p = 0.001) to intention to participate in e-learning were partially mediated through perceived usefulness. Finally, the effect of perceived educational institutions preparedness to intention to participate in e-learning was only realised through the mediating effect of PEOU-PU ( β = 0.07; t = 2.218; p = 0.03).
5.4 Multigroup analysis (MGA)
The research model was further investigated to see if the demographic characteristics of the respondents impact the path relationships in the model. To do so, we used the gender, and the average time the participant used the e-learning system in their e-learning activities. These two variables were used as control variables, and then we ran multigroup analysis (MGA) with PLS-SEM. The MGA results showed that respondents are different in some paths (see Table 5 ). For example, the path between perceived teachers and school's preparedness to perceived usefulness was only significant for males ( β = 0.261; t = 1.995; p = 0.05). The MGA results also showed that the path relationships between perceived challenges to (1) intention to participate in e-learning, (2) PU and (3) PEOU, were significant only for females. Therefore, the perceived challenges of COVID-19 could be considered as an important and influential factor influencing directly the decision-making of the students in e-learning participation. Finally, the path between the COVID-19 awareness to PEOU was only significant for females ( β = 0.332; t = 3.406; p = 0.001).
We also divided respondents into two groups based on their use of e-learning systems; group 1 included those who indicated they have experienced and used such systems for less than a year ( N = 61), group two for those who indicated they have experienced and used such systems for more than one year ( N = 69). The MGA results showed that the path between perceived educational institutions preparedness and PEOU was only significant for Group 1, those who mentioned that they had used the e-learning system for less than one year. However, more differences were observed in paths between COVID-19 awareness and perceived challenges to intention to participate in e-learning, as well as the path between perceived challenges to PEOU, such that the effects of these two path relationships were only significant for respondents in Group 2 (see Table 5 ).
6. Discussion
The SEM analysis revealed that the students' intention to participate in e-learning is significantly affected by the COVID-19 awareness and perceived challenges of the pandemic. It may be because of the subjective nature of the studied phenomena, which relies on the factors that relate to the individual (i.e. awareness and perceived challenges of the pandemic). These finding are similar to Raza et al. (2020) who also stated that there is need for improving the e-learning experience among students and escalating their intention to use such learning systems. Moreover, the perceived educational institution's preparedness (i.e. teachers and schools) seems to affect the intention to participate in e-learning only through the mediating effect of PEOU-PU. It may suggest that students do not see educational institutions' preparedness by itself as a motivating factor to use the e-learning system. It may also suggest that educational institutions have not been appropriately prepared to fully utilise the functionalities of e-learning systems (e.g. usefulness) facilitating the students' learning.
Moreover, the structure results showed that the awareness of COVID-19 situation might affect the usefulness of e-learning systems, but not the extent to which the use of such systems is easy. Given the pandemic requirements for safety via the social distancing and distance learning, students might consider e-learning systems as a better and safer alternative towards conventional in campus education. In other words, students have no other alternative left other than adapting to the dynamic situation and accepting to use e-learning systems to cope with the changes in their learning modes. Interestingly and as expected, the perceived challenges of COVID-19 situation seem to be a very influential factor determining the perceived value of e-learning systems and the intention to use them, however, it should be noted that the effect is negative. It may suggest that emotional and stress management of students is highly crucial for e-learning in the quarantine times.
Ong and Lai (2006) found that gender might impact the participation in e-learning through the perceived usefulness and perceived ease of use of e-learning systems. In the current paper, it was found the gender of the students impact their decision in e-learning participation. We would suggest that the perceived challenges of COVID-19 situation are having a more pronounced negative effect on female students than on their male counterpart. Plausibly, this might be due to the females' perceptions of their computer self-efficacy, which is crucial for e-learning ( Zhang et al. , 2012 ). In a similar vein, we would argue that the personality variations across genders may affect the results of why COVID-19 awareness has a significant impact on PEOU and the effect is only for females and why perceived preparedness has a significant impact on PU and that the effect is realised only for males. However, the latter may also be explained by the fact that males are more things-oriented, whereas females are people-oriented ( Su et al. , 2009 ). Hence, suggesting that males could potentially see more connections between e-learning systems' functionality (usefulness) and how these were improved by the preparedness of educational institutions.
The fact that the path between perceived educational institutions preparedness and PEOU was significant for those who used e-learning systems for a year or less may indicate that the educational institution's preparedness is only able to help an inexperienced user of e-learning systems by providing sufficient support and relevant information in the times of the pandemic. More experienced users of e-learning systems may have learned how to use them; hence the preparedness did not affect their perception of ease-of-use of e-learning systems. Contrarily, for experienced users who have used e-learning systems longer than a year, it may be that they are able to put the perceived challenges in perspective to the times when e-learning was not the main and the only mode of learning. The experience of use of e-learning systems is logically expected to be highly correlated with the age and the education level; hence, it could be hard to pinpoint whether differences come from the experience or other demographic variables.
7. Conclusions
The education of university students has been interrupted due to COVID-19 pandemic. The current situation has imposed unique challenges of smoothly maintaining the process of teaching and learning, as such e-learning has become an immediate solution to cope with the disruption in higher education. The results of this research revealed several theoretical implications. The first being the extension of the Technology Acceptance Model (TAM: Davis, 1989 ) for making it relevant to the current COVID-19 situation, and its application in the context of higher education to assess students' intention to use e-learning systems. The core theoretical focus of this study was to develop a conceptual model to identify factors impacting the students' intention to e-learning participation during the COVID-19 pandemic. This paper theoretically contributes to the literature by showing that the awareness of and the perceived challenges of the COVID-19 pandemic situation were the most significant factors influencing e-learning participation during the COVID-19 pandemic. As students' awareness of COVID-19 pandemic is increased, they would be more willing to achieve their education goals through the use of e-learning systems, especially when they are socially isolated, campus education is restricted and have to perform their studies mostly online. Moreover, the findings showed that no matter how well prepared the educational institutions (teachers and schools) are, the usefulness of e-learning systems still plays the leading role in enhancing the students' intention to participate in e-learning. Surprisingly, we did not find any direct impact of ease of use of e-learning systems to the intention of e-learning participation. Perhaps, blended learning (offline and online education) could be still the most proffered modes of learning for the students. In other words, a blended approach, where traditional teaching is combined with online teaching, should have ushered the students to participate in e-learning.
Alea et al. (2020) have found that there are multiple challenges in terms of educational preparedness during the COVID-19. However, in this study, it was found that educational institutions preparedness has little to no effect on the intention to participate in e-learning. Thus, the educational institutions are advised to consider the findings of this study to review their approaches to address their politics regarding e-learning in the times of the quarantine. We also found that the effects of the perceived pandemic challenges and educational institutions preparedness are different for experienced and inexperienced users of e-learning systems as well as among female and male students. As such, gender should be considered as a crucial factor in e-learning initiative taken by the educational institutions. Perceived challenges seem to have the most negative impact on women in the pandemic situation and their participation in e-learning. Sun et al. (2008) suggested that personal perceptions about e-learning affect the intention to participate in e-learning. In our study, it seems that the intention to participate in e-learning is affected by the perceptions about the contextual situation, such as about the current pandemic situation, perceived challenges it creates, and how does the educational institution prepare itself to tackle the situation.
7.1 Limitations
One of the drawbacks of the current research is the sample size used that can be expanded to achieve more generalisable findings. The conceptual model was developed for the purpose of this research, and therefore, the structural results and findings should be interpreted carefully. The size of the dataset and the sampling strategy might be other sources of potential errors. Since the data were collected through an online survey and during the COVID-19 pandemic situation, it is very hard to evaluate and assess whether the respondents answered questions as accurate as possible. Finally, this study took place in Finland, and might not apply to other countries due to different COVID-19 situation, regulations and imposed restriction during the current situation.
7.2 Future research
This research has uncovered interesting manifold insights about the different COVID-19 related factors on e-learning at educational institutions. As such, future research may utilise the conceptual model developed in this research and aim to explore further findings in other contexts. For instance, by investigating what encourages students to participate in e-learning more and why education institutions preparedness (both teachers and schools) does not account for higher intention to participate in e-learning. Students' perceptions could also be explored qualitatively. For example, why and how exactly awareness about COVID-19 encourages more intention to use e-learning systems. Future research is also advised on exploring further how educational institutions should become better prepared for future events, if they may occur, such as one we are witnessing in the current pandemic situation.
Theoretical model
Structural model
Reflective indicator loadings and internal consistency reliability
Discriminant validity (HTMT)
Structural results
Multigroup analysis results
Abbasi , S. , Ayoob , T. , Malik , A. and Memon , S.I. ( 2020 ), “ Perceptions of students regarding E-learning during Covid-19 at a private medical college ”, Pakistan Journal of Medical Sciences , Vol. 36 , pp. S57 - S61 , doi: 10.12669/pjms.36.COVID19-S4.2766 .
Adedoyin , O.B. and Soykan , E. ( 2020 ), “ Covid-19 pandemic and online learning: the challenges and opportunities ”, Interactive Learning Environments , pp. 1 - 13 , doi: 10.1080/10494820.2020.1813180 .
Adnan , M. and Anwar , K. ( 2020 ), “ Online learning amid the COVID-19 pandemic: students' perspectives ”, Online Submission , Vol. 2 No. 1 , pp. 45 - 51 .
Al-Okaily , M. , Alqudah , H. , Matar , A. , Lutfi , A. and Taamneh , A. ( 2020a ), “ Dataset on the Acceptance of e-learning System among universities students' under the COVID-19 pandemic conditions ”, Data in Brief , Vol. 32 , 106176 .
Al-Okaily , M. , Alqudah , H.M. , Matar , A. , Lutfi , A. and Taamneh , A. ( 2020b ), “ Impact of covid-19 pandemic on acceptance of E-learning system in Jordan: a case of transforming the traditional education systems ”, Humanities and Social Sciences Reviews , Vol. 8 No. 4 , pp. 840 - 851 .
Alea , L.A. , Fabrea , M.F. , Roldan , R.D.A. and Farooqi , A.Z. ( 2020 ), “ Teachers' covid-19 awareness, distance learning education Experiences and Perceptions towards institutional Readiness and challenges ”, International Journal of Learning, Teaching and Educational Research , Vol. 19 No. 6 , pp. 127 - 144 .
Almaiah , M.A. , Al-Khasawneh , A. and Althunibat , A. ( 2020 ), “ Exploring the critical challenges and factors influencing the e-learning system usage during COVID-19 pandemic ”, Education and Information Technologies , Vol. 25 , pp. 5261 - 5280 .
Alsabawy , A.Y. , Cater-Steel , A. and Soar , J. ( 2016 ), “ Determinants of perceived usefulness of e-learning systems ”, Computers in Human Behaviour , Vol. 64 , pp. 843 - 858 .
Anthony Jnr , B. , Kamaludin , A. , Romli , A. , Mat Raffei , A.F. , A_L Eh Phon , D.N. , Abdullah , A. , Leong Ming , G. , A Shukor , N. , Shukri Nordin , M. and Baba , S. ( 2020 ), “ Predictors of blended learning deployment in institutions of higher learning: theory of planned behavior perspective ”, International Journal of Information and Learning Technology , Vol. 37 No. 4 , pp. 179 - 196 .
Aristovnik , A. , Keržič , D. , Ravšelj , D. , Tomaževič , N. and Umek , L. ( 2020 ), “ Impacts of the COVID-19 pandemic on life of higher education students: a global perspective ”, Sustainability , Vol. 12 No. 20 , p. 8438 .
Bansode , S.Y. and Kumbhar , R. ( 2012 ), “ E-learning experience using open-source software: Moodle ”, DESIDOC Journal of Library and Information Technology , Vol. 32 No. 5 , pp. 409 - 416 .
Bao , W. ( 2020 ), “ COVID‐19 and online teaching in higher education: a case study of Peking University ”, Human Behaviour and Emerging Technologies , Vol. 2 No. 2 , pp. 113 - 115 .
Benigno , V. and Trentin , G. ( 2000 ), “ The evaluation of online courses ”, Journal of Computer Assisted Learning , Vol. 16 No. 3 , pp. 259 - 270 .
Cheng , Y.M. ( 2012 ), “ Effects of quality antecedents on e‐learning acceptance ”, Internet Research , Vol. 22 No. 3 , pp. 361 - 390 .
Dalsgaard , C. ( 2006 ), “ Social software: E-learning beyond learning management systems ”, European Journal of Open, Distance and E-Learning , Vol. 9 No. 2 , pp. 1 - 7 .
Davis , F. ( 1989 ), “ Perceived usefulness, perceived ease of use and user acceptance of information technology ”, MIS Quarterly , Vol. 13 No. 3 , pp. 319 - 340 .
Ellis , R.A. and Goodyear , P. ( 2016 ), “ Models of learning space: integrating research on space, place and learning in higher education ”, Review of Education , Vol. 4 No. 2 , pp. 149 - 191 .
Favale , T. , Soro , F. , Trevisan , M. , Drago , I. and Mellia , M. ( 2020 ), “ Campus traffic and e-Learning during COVID-19 pandemic ”, Computer Networks , Vol. 176 , 107290 , doi: 10.1016/j.comnet.2020.107290 .
Fleming , J. , Becker , K. and Newton , C. ( 2017 ), “ Factors for successful e-learning: does age matter? ”, Education + Training , Vol. 59 No. 1 , pp. 76 - 89 .
Fornell , C.G. and Larcker , D.F. ( 1981 ), “ Evaluating structural equation models with unobservable variables and measurement error ”, Journal of Marketing Research , Vol. 18 No. 1 , pp. 39 - 50 .
Garavan , T.N. , Carbery , R. , O’Malley , G. and O’Donnell , D. ( 2010 ), “ Understanding participation in e‐learning in organisations: a large‐scale empirical study of employees ”, International Journal of Training and Development , Vol. 14 No. 3 , pp. 155 - 168 .
Garrison , D.R. and Anderson , T. ( 2003 ), E-learning in the 21 st Century: A Framework for Research and Practice , Routledge Falmer , London .
Gupta , A. ( 2000 ), “ Enterprise resource planning: the emerging organisational value systems ”, Industrial Management and Data Systems , Vol. 100 No. 3 , pp. 114 - 118 .
Habes , M. ( 2019 ), “ The influence of personal motivation on using social TV: a uses and gratifications approach ”, International Journal of Information Technology and Language Studies , Vol. 3 No. 1 , pp. 32 - 39 .
Hair , J.F. , Risher , J.J. , Sarstedt , M. and Ringle , C.M. ( 2019 ), “ When to use and how to report the results of PLS-SEM ”, European Business Review , Vol. 31 No. 1 , pp. 2 - 24 .
Hodges , C. , Moore , S. , Lockee , B. , Trust , T. and Bond , A. ( 2020 ), “ The difference between emergency remote teaching and online learning ”, Educause Review , Vol. 27 .
Hrastinski , S. ( 2008 ), “ What is online learner participation? A literature review ”, Computers and Education , Vol. 51 No. 4 , pp. 1755 - 1765 .
Kaplan , J. , Frias , L. and McFall-Johnsen , M. ( 2020 ), “ A third of the global population is on coronavirus lockdown — here's our constantly updated list of countries locking down and opening up ”, available at: https://www.businessinsider.com.au/countries-on-lockdown-coronavirus-italy-2020-3 ( accessed 12 December 2020 ).
Ke , F. and Hoadley , C. ( 2009 ), “ Evaluating online learning communities ”, Educational Technology Research and Development , Vol. 57 , pp. 487 - 510 .
Khan , B.H. ( 2004 ), “ The people—process—product Continuum in e-learning: the e-learning P3 model ”, Educational Technology , Vol. 44 No. 5 , pp. 33 - 40 .
Kim , K.J. and Frick , T.W. ( 2011 ), “ Changes in student motivation during online learning ”, Journal of Educational Computing Research , Vol. 44 No. 1 , pp. 1 - 23 .
Kraaijenbrink , J. and Wijnhoven , F. ( 2008 ), “ Managing heterogeneous knowledge: a theory of external knowledge integration ”, Knowledge Management Research and Practice , Vol. 6 No. 4 , pp. 274 - 286 .
Lee , B.C. , Yoon , J.O. and Lee , I. ( 2009 ), “ Learners' acceptance of e-learning in South Korea: Theories and results ”, Computers and Education , Vol. 53 No. 4 , pp. 1320 - 1329 .
Masrom , M. ( 2007 ), “ Technology acceptance model and E-learning ”, Proceedings of the 12th International Conference on Education , May 21-24 , University Brunei Darussalam , Brunei Darussalam , pp. 1 - 10 .
Musingafi , M.C. , Mapuranga , B. , Chiwanza , K. and Zebron , S. ( 2015 ), “ Challenges for open and distance learning (ODL) students: experiences from students of the Zimbabwe Open University ”, Journal of Education and Practice , Vol. 6 No. 18 , pp. 59 - 66 .
Nakamura , W.T. , de Oliveira , E.H.T. and Conte , T. ( 2017 ), “ Usability and user experience evaluation of learning management systems-A systematic mapping study ”, International Conference on Enterprise Information Systems , Scitepress , Vol. 2 , pp. 97 - 108 .
Ong , C.S. and Lai , J.Y. ( 2006 ), “ Gender differences in perceptions and relationships among dominants of e-learning acceptance ”, Computers in Human Behaviour , Vol. 22 No. 5 , pp. 816 - 829 .
Paraschi , E.P. ( 2020 ), “ Accessibility, tourism and social welfare: Covid19 and a new quality-of-life tourism model for the Greek islands ”, International Journal of Cultural and Digital Tourism , Vol. 6 , pp. 10 - 21 .
Park , S.Y. ( 2009 ), “ An analysis of the technology acceptance model in understanding university students' behavioural intention to use e-learning ”, Journal of Educational Technology and Society , Vol. 12 No. 3 , pp. 150 - 162 .
Radha , R. , Mahalakshmi , K. , Kumar , V.S. and Saravanakumar , A.R. ( 2020 ), “ E-learning during lockdown of Covid-19 pandemic: a global perspective ”, International Journal of Control and Automation , Vol. 13 No. 4 , pp. 1088 - 1099 .
Raza , S.A. , Qazi , W. , Khan , K.A. and Salam , J. ( 2020 ), “ Social isolation and acceptance of the learning management system (LMS) in the time of COVID-19 pandemic: an expansion of the UTAUT model ”, Journal of Educational Computing Research , Vol. 59 No. 2 , pp. 183 - 208 .
Ralyté , J. , Khadraoui , A. and Léonard , M. ( 2015 ), “ Designing the shift from information systems to information services systems ”, Business and Information Systems Engineering , Vol. 57 No. 1 , pp. 37 - 49 .
Selim , H.M. ( 2007 ), “ Critical success factors for e-learning acceptance: confirmatory factor models ”, Computers and Education , Vol. 49 No. 2 , pp. 396 - 413 .
Strauß , S. and Rummel , N. ( 2020 ), “ Promoting interaction in online distance education: designing, implementing and supporting collaborative learning ”, Information and Learning Sciences , Vol. 121 Nos 5/6 , pp. 251 - 260 .
Su , R. , Rounds , J. and Armstrong , P.I. ( 2009 ), “ Men and things, women and people: a meta-analysis of sex differences in interests ”, Psychological Bulletin , Vol. 135 No. 6 , p. 859 .
Sun , P.C. , Tsai , R.J. , Finger , G. , Chen , Y.Y. and Yeh , D. ( 2008 ), “ What drives a successful e-Learning? An empirical investigation of the critical factors influencing learner satisfaction ”, Computers and Education , Vol. 50 No. 4 , pp. 1183 - 1202 .
Toquero , C.M. ( 2020 ), “ Challenges and opportunities for higher education amid the COVID-19 pandemic: the philippine context ”, Pedagogical Research , Vol. 5 No. 4 , pp. 1 - 5 .
Tselios , N. , Daskalakis , S. and Papadopoulou , M. ( 2011 ), “ Assessing the acceptance of a blended learning university course ”, Journal of Educational Technology and Society , Vol. 14 No. 2 , pp. 224 - 235 .
Uppal , M.A. , Ali , S. and Gulliver , S.R. ( 2018 ), “ Factors determining e‐learning service quality ”, British Journal of Educational Technology , Vol. 49 No. 3 , pp. 412 - 426 .
Van Nuland , S. , Mandzuk , D. , Tucker Petrick , K. and Cooper , T. ( 2020 ), “ COVID-19 and its effects on teacher education in Ontario: a complex adaptive systems perspective ”, Journal of Education for Teaching , pp. 1 - 10 .
Wilson , H. ( 2009 ), “ The process of creating learning space ”, in Radcliffe , D. , Wilson , H. , Powell , D. and Tibbetts , B. (Eds), Designing Next Generation Places of Learning: Collaboration at the Pedagogy–Space– Technology Nexus , University of Queensland , Brisbane .
Yu , Z. ( 2020 ), “ Extending the learning technology acceptance model of WeChat by adding new psychological constructs ”, Journal of Educational Computing Research , Vol. 58 No. 6 , pp. 1121 - 1143 .
Zhang , Y. , Fang , Y. , Wei , K.K. and Wang , Z. ( 2012 ), “ Promoting the intention of students to continue their participation in e‐learning systems ”, Information Technology and People , Vol. 25 No. 4 , pp. 356 - 375 .
Zia , A. ( 2020 ), “ Exploring factors influencing online classes due to social distancing in COVID-19 pandemic: a business students' perspective ”, International Journal of Information and Learning Technology , Vol. 37 No. 4 , pp. 197 - 211 .
Corresponding author
Related articles, we’re listening — tell us what you think, something didn’t work….
Report bugs here
All feedback is valuable
Please share your general feedback
Join us on our journey
Platform update page.
Visit emeraldpublishing.com/platformupdate to discover the latest news and updates
Questions & More Information
Answers to the most commonly asked questions here
Introduction to E-Learning Systems
- First Online: 20 July 2016
Cite this chapter
- Aleksandra Klašnja-Milićević 8 ,
- Boban Vesin 9 ,
- Mirjana Ivanović 8 ,
- Zoran Budimac 8 &
- Lakhmi C. Jain 10 , 11
Part of the book series: Intelligent Systems Reference Library ((ISRL,volume 112))
4 Citations
Recently e-learning systems are experiencing rapid development. The advantages of learning through a global network are manifold and obvious: the independence of time and space, learners can learn at their own pace, learning materials can be organized in one place and used-processed all around the world. One of the most important segments in today’s development and use of the e-learning system is the personalization of content and building of user profiles based on the learning behaviour of each individual user. The personalization options increase efficiency of e-learning, thus justifying the higher initial cost of their construction. In order to personalize the learning process and adapt content to each learner, e-learning systems can use strategies that have the ability to meet the needs of learners. Also, these systems have to use different technologies to change the environment and perform the adaptation of teaching materials based on the needs of learners. The process of adaptation can be in the form of adaptation of content, learning process, feedback or navigation. This chapter introduces the motivation and objectives studied in the subsequently presented research, and presents major standards and specifications in e-learning.
This is a preview of subscription content, log in via an institution to check access.
Access this chapter
- Available as EPUB and PDF
- Read on any device
- Instant download
- Own it forever
- Compact, lightweight edition
- Dispatched in 3 to 5 business days
- Free shipping worldwide - see info
- Durable hardcover edition
Tax calculation will be finalised at checkout
Purchases are for personal use only
Institutional subscriptions
http://www.ieee.org/index.html .
http://adlnet.gov/ .
http://www.imsglobal.org/ .
http://www.ariadne-eu.org/ .
http://scorm.com/tincanoverview/ .
Aranda, A. D. (2012). Moodle for distance education. Distance Learning, 8 (2), 25–28.
MathSciNet Google Scholar
Barth, M., Adomßent, M., Fischer, D., Richter, S., & Rieckmann, M. (2014). Learning to change universities from within: A service-learning perspective on promoting sustainable consumption in higher education. Journal of Cleaner Production, 62 , 72–81.
Article Google Scholar
Bradford, P., Margaret Porciello, N., & Balkon, D. B. (2007). The blackboard learning system. The Journal of Educational Technology Systems, 35 , 301–314. http://doi.org/10.2190/X137-X73L-5261-5656
Brusilovsky, P. (2004). KnowledgeTree: A distributed architecture for adaptive e-learning. In WWW Alt. ’04: Proceedings of the 13th International World Wide Web Conference on Alternate Track Papers & Posters (pp. 104–113). http://doi.org/10.1145/1013367.1013386
Committee, L. T. S., et al. (2002). IEEE standard for learning object metadata. IEEE Standard, 1484 (1), 2004–2007.
Google Scholar
Dalziel, J. R. (2006). Lessons from LAMS for IMS learning design. In Sixth International Conference on Advanced Learning Technologies , (Vol. 3, 1101–1102). http://doi.org/10.1109/ICALT.2006.1652643
de Oliveira, F., & Gomes, A. S. (2015). A development model of units of learning for multiple platforms. In 2015 10th Iberian Conference on Information Systems and Technologies (CISTI) (pp. 1–6).
Devedzic, V. (2004). Education and the semantic web. International Journal of Artificial Intelligence in Education, 14 , 39–65.
Devedzic, V. (2006). Semantic web and education (Vol. 11). http://doi.org/10.1007/978-0-387-35417-0
Dutta, B. (2006). Semantic web based e-learning . Bangalore: Documentation Research and Training Centre Indian Statistical Institute.
Garrison, D. R., & Vaughan, N. D. (2008). Blended learning in higher education: Framework, principles, and guidelines . booksgooglecom (Vol. 1st).
González, L. A. G., & Ruggiero, W. V. (2009). Collaborative e-learning and learning objects. IEEE Latin America Transactions, 7 (5), 569–577. http://doi.org/10.1109/TLA.2009.5361195
Gourley, B., & Lane, A. (2009). Re-invigorating openness at The Open University: The role of open educational resources. Open Learning: The Journal of Open and Distance Learning , 37–41. http://doi.org/10.1080/02680510802627845
Harsh, O. K., & Sohail, M. S. (2002). Role of delivery, course design and teacher-student interaction: Observations of adult distance education and traditional on-campus education. The International Review of Research in Open and Distributed Learning , 3 (2).
Horvat, A., Dobrota, M., Krsmanovic, M., & Cudanov, M. (2015). Student perception of Moodle learning management system: A satisfaction and significance analysis. Interactive Learning Environments, 23 (4), 515–527.
Leo, D. H., Perez, J. I. A., & Dimitriadis, Y. A. (2004). IMS learning design support for the formalization of collaborative learning patterns. In IEEE International Conference on Advanced Learning Technologies, 2004. Proceedings. (pp. 350–355). http://doi.org/10.1109/ICALT.2004.1357434
Murphy, R. T., & Appeal, L. R. (1978). Evaluation of the PLATO IV computer-based education system in the community college. ACM SIGCUE Outlook, 12 (1), 12–28.
Pitkänen, S. H., & Silander, P. (2004). Criteria for pedagogical reusability of learning objects enabling adaptation and individualised learning processes. In IEEE International Conference on Advanced Learning Technologies, 2004. Proceedings (pp. 246–250).
Verdú, E., Regueras, L. M., Verdú, M. J., Leal, J. P., de Castro, J. P., & Queirós, R. (2012). A distributed system for learning programming online. Computers & Education, 58 (1), 1–10. http://doi.org/10.1016/j.compedu.2011.08.015
Vesin, B., & Ivanović, M. (2004). Modern educational tools. In Proceedings of PRIM2004, 16th Conference on Applied Mathematics, Budva, Montenegro (pp. 293–302).
Zdravkova, K., Ivanović, M., & Putnik, Z. (2012). Experience of integrating web 2.0 technologies. Educational Technology Research and Development . http://doi.org/10.1007/s11423-011-9228-z
Download references
Author information
Authors and affiliations.
Faculty of Sciences, Department of Mathematics and Informatics, University of Novi Sad, Novi Sad, Serbia
Aleksandra Klašnja-Milićević, Mirjana Ivanović & Zoran Budimac
Department of Computer Science and Engineering, University of Gothenburg/Chalmers, University of Technology, Gothenburg, Sweden
Boban Vesin
Bournemouth University, Fern Barrow, Poole, UK
Lakhmi C. Jain
University of Canberra, Canberra, Australia
You can also search for this author in PubMed Google Scholar
Corresponding author
Correspondence to Aleksandra Klašnja-Milićević .
Rights and permissions
Reprints and permissions
Copyright information
© 2017 Springer International Publishing Switzerland
About this chapter
Klašnja-Milićević, A., Vesin, B., Ivanović, M., Budimac, Z., Jain, L.C. (2017). Introduction to E-Learning Systems. In: E-Learning Systems. Intelligent Systems Reference Library, vol 112. Springer, Cham. https://doi.org/10.1007/978-3-319-41163-7_1
Download citation
DOI : https://doi.org/10.1007/978-3-319-41163-7_1
Published : 20 July 2016
Publisher Name : Springer, Cham
Print ISBN : 978-3-319-41161-3
Online ISBN : 978-3-319-41163-7
eBook Packages : Engineering Engineering (R0)
Share this chapter
Anyone you share the following link with will be able to read this content:
Sorry, a shareable link is not currently available for this article.
Provided by the Springer Nature SharedIt content-sharing initiative
- Publish with us
Policies and ethics
- Find a journal
- Track your research
Academia.edu no longer supports Internet Explorer.
To browse Academia.edu and the wider internet faster and more securely, please take a few seconds to upgrade your browser .
Enter the email address you signed up with and we'll email you a reset link.
- We're Hiring!
- Help Center
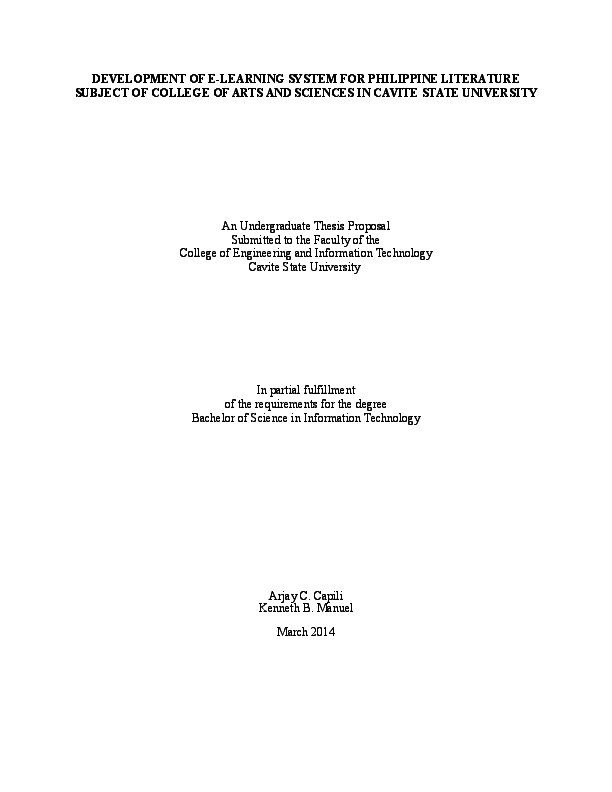
DEVELOPMENT OF E-LEARNING SYSTEM FOR PHILIPPINE LITERATURE SUBJECT OF COLLEGE OF ARTS AND SCIENCES IN CAVITE STATE UNIVERSITY

Related Papers
Pedagogy: Jurnal Pendidikan Matematika
Penelitian ini merupakan penelitian eksperimen dengan tujuan untuk mengetahui gambaran kemandirian belajar dan pemahaman konsep mahasiswa dalam penerapan E-Learning berbasis proyek dan mengetahui apakah terdapat peningkatan kemandirian belajar dan pemahaman konsep mahasiswa setelah penerapan E-Learning berbasis proyek. Penelitian ini bertempat di Universitas Cokroaminoto Palopo pada Program Studi Pendidikan Matematika dan program studi Teknik Informatika yang disetting sebagai kelas perkuliahan mata kuliah Struktur Data, Logika Informatika dan Media Pembelajaran Matematika. Hasil penelitian menunjukkan bahwa kemandirian belajar dan pemahaman konsep mahasiswa dengan penerapan E-Learning berbasis proyek jauh lebih baik jika dibandingkan dengan sebelum penerapan E-Learning. Terjadi peningkatan kemandirian belajar dan pemahaman konsep mahasiswa dengan penerapan E-Learning berbasis proyek.
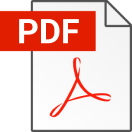
Jonathan C Javellana
Budapest International Research and Critics Institute (BIRCI-Journal) : Humanities and Social Sciences
Trisnawati Hutagalung
The challenge faced by teachers today is the lack of skills in developing technology-based media. In facing the demands of an increasingly developing curriculum, teachers need to be given reinforcement in the development of professionalism, including in making digital-based media. Based on the problem, MI Muhammadiyah 2 Kedungbanteng is a school that deserves instructional media training. The obstacles faced include the absence of assistance to develop technology-based media so that the digital literacy of teachers is still low, the absence of school literacy activities through teacher-made media, and the lack of activities that can hone creativity to optimize vocabulary learning. This service wants to make a meaningful contribution to teachers with training activities in developing Canva application-based big book media. The activity is carried out through preparation, implementation, and evaluation stage. In the preparatory stage, the team collected initial information about condi...
Mclean berania
Filipino culture places a high value on education. For the majority of the Filipinos, the only best thing for a child to acquire and secure a better future is through education. So, they want more effective education system. E-Learning is still an emerging market in the Philippines. Its use is still sporadic and most users represent only a small segment of the Philippines education and business communities. The study wants to correct analysis and right direction presentation for Philippine remote education environment.
tahrun tahrun
Saiyidi Hasbullah
The Covid-19 epidemic, which started in Wuhan, China in 2019, has spread to a number of countries, including Indonesia. Learning from Home is another term for online learning (BDR). To show support for government policies and to assist students in meeting their educational needs, SMKN 1 Bakung adopts an online learning strategy using e-learning. The aim of this article is to show many types of literature and the use of devices in e-learning as a source of literature. Qualitative research is used for this research. After analyzing the data, it could be concluded that in e-learning SMKN 1 Bakung, there are some types of works of literature. They are separated based on their characteristics and locations. Due to the characteristic, they are textual, non-textual, and mixed documents. While based on the location, they are general and references collections. The literature is mostly in form of pdf to make them easily accessed by students. The use of devices in e-learning is as follows: ac...
JTK (Jurnal Tadris Kimiya)
This study aims to develop a Schoology-based e-learning media and to find out the validity and response of users (teachers and students). The method used in this research was Research and Development (R&D) with the Analysis, Design, Development, Implementation, and Evaluation (ADDIE) model. The implementation stage was not carried out due to the limitations of the research. Validation tests carried out by material and media experts as well as limited trials on students and teachers were obtained using a questionnaire. The data analysis technique used was descriptive analysis. The validation results from material and media expert showed the percentage of 93.23% and 90.69% in the valid category. The results of trial by student and teacher responses showed the percentage of 94.40% and 93.12% in good category. The Schoology-based e-learning media on ion equilibrium and buffer solutions is valid and suitable for use in online learning.
Gómez Chova, L., Martí Belenguer, D. y Candel Torres, I. (eds.), International Technology, Education and Development Conference INTED2008 Proceedings (Valencia, 3th-5th March 2008)
F. Javier de Cos Ruiz
This work goes on a Project of Innovation in Education at the University of Cádiz, linked to the ETCS experiences. We are planning different work tools in the subject's programs of Spanish Language and Theory of Literature and Comparative Literature. We develop the e-learning trough a Virtual Classroom at the University of Cadiz, which takes its basis on the Moodle platform and finds its reason of being in the methodological change resulting from the introduction of ETCS. We use the new technologies having a support in a virtual classroom that helps the pupil to learn to work and to communicate with his companions. We think that the justification of using new Technologies are: in to diversify the supports through which the pupil accedes to the information; to foment the critical capacity before different types of speech and different supports of expression and presentation, to make the pupil learn by himself, to foment the communication and the cooperative work between the pupils. Resultado de la Acción de Innovación Docente "Programación centrada en el aprendizaje significativo: planificación y diseño de actividades" (cód. IN07-41). Coordinadora: Fátima Coca Ramírez. Con financiación de 1.800 euros. Universidad de Cádiz, Vicerrectorado de Ordenación Académica e Innovación Educativa, Dirección General para el Espacio Europeo de Educación Superior, Convocatoria de Proyectos de Innovación Docente para la Convergencia Europea. Curso 2006-07.
Ouda Teda Ena
RELATED PAPERS
Sizakele Mathba
2013 15th International Conference on Transparent Optical Networks (ICTON)
Tim Wilkinson
Maricel Jarencio
European journal of orthopaedic surgery & traumatology : orthopédie traumatologie
Hendrik Fuglesang
Bo Westerlund
Mathematical Researches
Hydrology Research
Lucio Pinotti
Roman Gancarz
Biological Chemistry
Biserka Kojić-prodić
ACTA ZOOLÓGICA MEXICANA (N.S.)
Erick Alexander contreras Ruiz
Earth and Planetary Science Letters
Laure Meynadier
Engineering Geology
Fadhil Ahmad
Mikola Kryvaltsevich
如何购买sfu毕业证 西蒙菲莎大学毕业证研究生文凭证书托业成绩单原版一模一样
Powder Technology
Eva Martin del Valle
Raul Gonzalez
Maria do Carmo Galiazzi
Sacred Civics
Michel Bauwens
Ginekologia Polska
Emin üstünyurt
- We're Hiring!
- Help Center
- Find new research papers in:
- Health Sciences
- Earth Sciences
- Cognitive Science
- Mathematics
- Computer Science
- Academia ©2024
Title: Human-Centered Explainable AI for Sequential Decision-Making Systems
Associated Organization(s)
Collections, supplementary to, permanent link, date issued, resource type, resource subtype, rights statement.
- MyU : For Students, Faculty, and Staff
CS&E Announces 2024-25 Doctoral Dissertation Fellowship (DDF) Award Winners

Seven Ph.D. students working with CS&E professors have been named Doctoral Dissertation Fellows for the 2024-25 school year. The Doctoral Dissertation Fellowship is a highly competitive fellowship that gives the University’s most accomplished Ph.D. candidates an opportunity to devote full-time effort to an outstanding research project by providing time to finalize and write a dissertation during the fellowship year. The award includes a stipend of $25,000, tuition for up to 14 thesis credits each semester, and subsidized health insurance through the Graduate Assistant Health Plan.
CS&E congratulates the following students on this outstanding accomplishment:
- Athanasios Bacharis (Advisor: Nikolaos Papanikolopoulos )
- Karin de Langis (Advisor: Dongyeop Kang )
- Arshia Zernab Hassan (Advisors: Chad Myers )
- Xinyue Hu (Advisors: Zhi-Li Zhang )
- Lucas Kramer (Advisors: Eric Van Wyk )
- Yijun Lin (Advisors: Yao-Yi Chiang )
- Mingzhou Yang (Advisors: Shashi Shekhar )
Athanasios Bacharis

Bacharis’ work centers around the robot-vision area, focusing on making autonomous robots act on visual information. His research includes active vision approaches, namely, view planning and next-best-view, to tackle the problem of 3D reconstruction via different optimization frameworks. The acquisition of 3D information is crucial for automating tasks, and active vision methods obtain it via optimal inference. Areas of impact include agriculture and healthcare, where 3D models can lead to reduced use of fertilizers via phenotype analysis of crops and effective management of cancer treatments. Bacharis has a strong publication record, with two peer-reviewed conference papers and one journal paper already published. He also has one conference paper under review and two journal papers in the submission process. His publications are featured in prestigious robotic and automation venues, further demonstrating his expertise and the relevance of his research in the field.
Karin de Langis

Karin's thesis works at the intersection of Natural Language Processing (NLP) and cognitive science. Her work uses eye-tracking and other cognitive signals to improve NLP systems in their performance and cognitive interpretability, and to create NLP systems that process language more similarly to humans. Her human-centric approach to NLP is motivated by the possibility of addressing the shortcomings of current statistics-based NLP systems, which often become stuck on explainability and interpretability, resulting in potential biases. This work has most recently been accepted and presented at SIGNLL Conference on Computational Natural Language Learning (CoNLL) conference which has a special focus on theoretically, cognitively and scientifically motivated approaches to computational linguistics.
Arshia Zernab Hassan
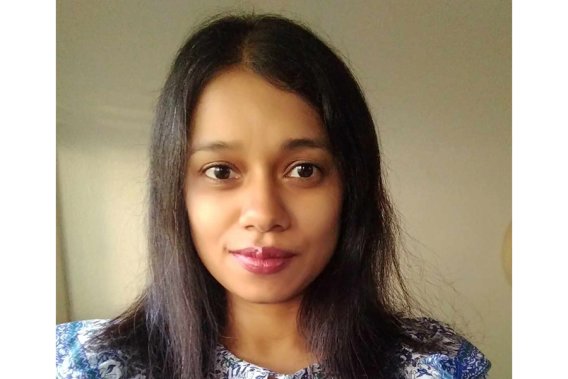
Hassan's thesis work delves into developing computational methods for interpreting data from genome wide CRISPR/Cas9 screens. CRISPR/Cas9 is a new approach for genome editing that enables precise, large-scale editing of genomes and construction of mutants in human cells. These are powerful data for inferring functional relationships among genes essential for cancer growth. Moreover, chemical-genetic CRISPR screens, where population of mutant cells are grown in the presence of chemical compounds, help us understand the effect the chemicals have on cancer cells and formulate precise drug solutions. Given the novelty of these experimental technologies, computational methods to process and interpret the resulting data and accurately quantify the various genetic interactions are still quite limited, and this is where Hassan’s dissertation is focused on. Her research extends to developing deep-learning based methods that leverage CRISPR chemical-genetic and other genomic datasets to predict cancer sensitivity to candidate drugs. Her methods on improving information content in CRISPR screens was published in the Molecular Systems Biology journal, a highly visible journal in the computational biology field.
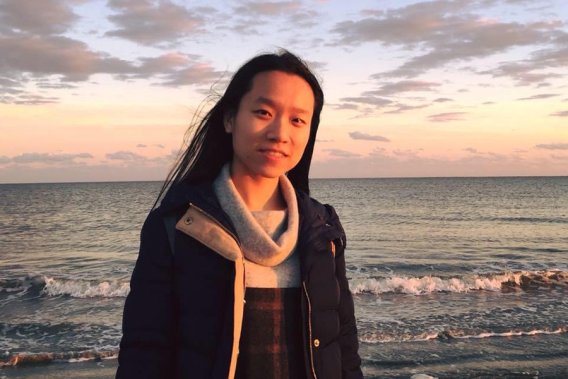
Hu's Ph.D. dissertation is concentrated on how to effectively leverage the power of artificial intelligence and machine learning (AI/ML) – especially deep learning – to tackle challenging and important problems in the design and development of reliable, effective and secure (independent) physical infrastructure networks. More specifically, her research focuses on two critical infrastructures: power grids and communication networks, in particular, emerging 5G networks, both of which not only play a critical role in our daily life but are also vital to the nation’s economic well-being and security. Due to the enormous complexity, diversity, and scale of these two infrastructures, traditional approaches based on (simplified) theoretical models and heuristics-based optimization are no longer sufficient in overcoming many technical challenges in the design and operations of these infrastructures: data-driven machine learning approaches have become increasingly essential. The key question now is: how does one leverage the power of AI/ML without abandoning the rich theory and practical expertise that have accumulated over the years? Hu’s research has pioneered a new paradigm – (domain) knowledge-guided machine learning (KGML) – in tackling challenging and important problems in power grid and communications (e.g., 5G) network infrastructures.
Lucas Kramer
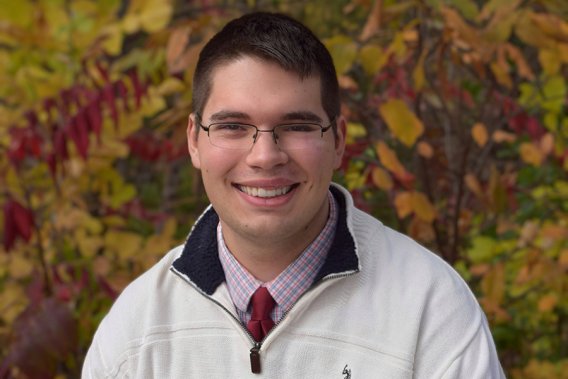
Kramer is now the driving force in designing tools and techniques for building extensible programming languages, with the Minnesota Extensible Language Tools (MELT) group. These are languages that start with a host language such as C or Java, but can then be extended with new syntax (notations) and new semantics (e.g. error-checking analyses or optimizations) over that new syntax and the original host language syntax. One extension that Kramer created was to embed the domain-specific language Halide in MELT's extensible specification of C, called ableC. This extension allows programmers to specify how code working on multi-dimensional matrices is transformed and optimized to make efficient use of hardware. Another embeds the logic-programming language Prolog into ableC; yet another provides a form of nondeterministic parallelism useful in some algorithms that search for a solution in a structured, but very large, search space. The goal of his research is to make building language extensions such as these more practical for non-expert developers. To this end he has made many significant contributions to the MELT group's Silver meta-language, making it easier for extension developers to correctly specify complex language features with minimal boilerplate. Kramer is the lead author of one journal and four conference papers on his work at the University of Minnesota, winning the distinguished paper award for his 2020 paper at the Software Language Engineering conference, "Strategic Tree Rewriting in Attribute Grammars".
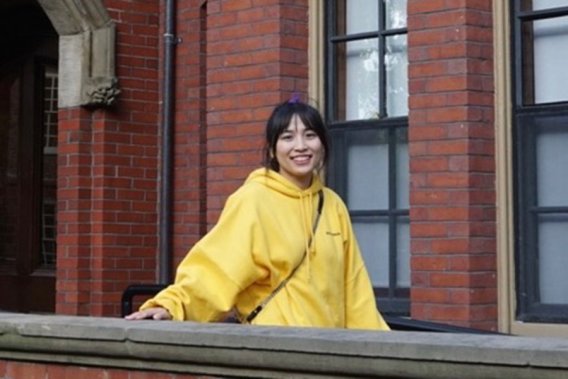
Lin’s doctoral dissertation focuses on a timely, important topic of spatiotemporal prediction and forecasting using multimodal and multiscale data. Spatiotemporal prediction and forecasting are important scientific problems applicable to diverse phenomena, such as air quality, ambient noise, traffic conditions, and meteorology. Her work also couples the resulting prediction and forecasting with multimodal (e.g., satellite imagery, street-view photos, census records, and human mobility data) and multiscale geographic information (e.g., census records focusing on small tracts vs. neighborhood surveys) to characterize the natural and built environment, facilitating our understanding of the interactions between and within human social systems and the ecosystem. Her work has a wide-reaching impact across multiple domains such as smart cities, urban planning, policymaking, and public health.
Mingzhou Yang

Yang is developing a thesis in the broad area of spatial data mining for problems in transportation. His thesis has both societal and theoretical significance. Societally, climate change is a grand challenge due to the increasing severity and frequency of climate-related disasters such as wildfires, floods, droughts, etc. Thus, many nations are aiming at carbon neutrality (also called net zero) by mid-century to avert the worst impacts of global warming. Improving energy efficiency and reducing toxic emissions in transportation is important because transportation accounts for the vast majority of U.S. petroleum consumption as well as over a third of GHG emissions and over a hundred thousand U.S. deaths annually via air pollution. To accurately quantify the expected environmental cost of vehicles during real-world driving, Yang's thesis explores ways to incorporate physics in the neural network architecture complementing other methods of integration: feature incorporation, and regularization. This approach imposes stringent physical constraints on the neural network model, guaranteeing that its outputs are consistently in accordance with established physical laws for vehicles. Extensive experiments including ablation studies demonstrated the efficacy of incorporating physics into the model.
Related news releases
- Brock Shamblin Wins 2024 Riedl TA Award
- Ph.D. Student Angel Sylvester Mentor’s High School Student
- 2024 John T. Riedl Memorial Graduate Teaching Assistant Award
- CS&E Earns Five Awards at 2023 SIAM SDM
- CS&E Announces 2023-24 Doctoral Dissertation Fellowship (DDF) Award Winners
- Future undergraduate students
- Future transfer students
- Future graduate students
- Future international students
- Diversity and Inclusion Opportunities
- Learn abroad
- Living Learning Communities
- Mentor programs
- Programs for women
- Student groups
- Visit, Apply & Next Steps
- Information for current students
- Departments and majors overview
- Departments
- Undergraduate majors
- Graduate programs
- Integrated Degree Programs
- Additional degree-granting programs
- Online learning
- Academic Advising overview
- Academic Advising FAQ
- Academic Advising Blog
- Appointments and drop-ins
- Academic support
- Commencement
- Four-year plans
- Honors advising
- Policies, procedures, and forms
- Career Services overview
- Resumes and cover letters
- Jobs and internships
- Interviews and job offers
- CSE Career Fair
- Major and career exploration
- Graduate school
- Collegiate Life overview
- Scholarships
- Diversity & Inclusivity Alliance
- Anderson Student Innovation Labs
- Information for alumni
- Get engaged with CSE
- Upcoming events
- CSE Alumni Society Board
- Alumni volunteer interest form
- Golden Medallion Society Reunion
- 50-Year Reunion
- Alumni honors and awards
- Outstanding Achievement
- Alumni Service
- Distinguished Leadership
- Honorary Doctorate Degrees
- Nobel Laureates
- Alumni resources
- Alumni career resources
- Alumni news outlets
- CSE branded clothing
- International alumni resources
- Inventing Tomorrow magazine
- Update your info
- CSE giving overview
- Why give to CSE?
- College priorities
- Give online now
- External relations
- Giving priorities
- CSE Dean's Club
- Donor stories
- Impact of giving
- Ways to give to CSE
- Matching gifts
- CSE directories
- Invest in your company and the future
- Recruit our students
- Connect with researchers
- K-12 initiatives
- Diversity initiatives
- Research news
- Give to CSE
- CSE priorities
- Corporate relations
- Information for faculty and staff
- Administrative offices overview
- Office of the Dean
- Academic affairs
- Finance and Operations
- Communications
- Human resources
- Undergraduate programs and student services
- CSE Committees
- CSE policies overview
- Academic policies
- Faculty hiring and tenure policies
- Finance policies and information
- Graduate education policies
- Human resources policies
- Research policies
- Research overview
- Research centers and facilities
- Research proposal submission process
- Research safety
- Award-winning CSE faculty
- National academies
- University awards
- Honorary professorships
- Collegiate awards
- Other CSE honors and awards
- Staff awards
- Performance Management Process
- Work. With Flexibility in CSE
- K-12 outreach overview
- Summer camps
- Outreach events
- Enrichment programs
- Field trips and tours
- CSE K-12 Virtual Classroom Resources
- Educator development
- Sponsor an event
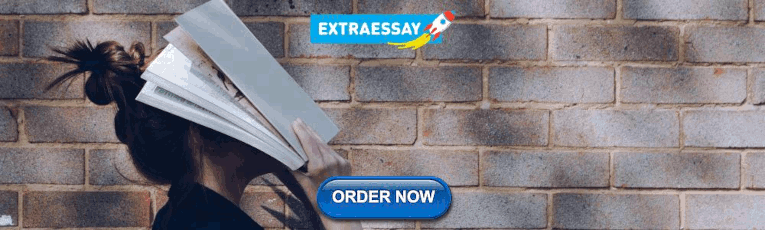
IMAGES
VIDEO
COMMENTS
A survey was conducted with the objective of discovering the need for eLearning in the education sector and how to avoid issues related to online learning. Other important subjects within the thesis include eLearning technologies, eLearning system, eLearning pros and cons and lastly eLearning design process.
Finally, this paper discusses whether e-Learning and traditional face-to-face learning should be measured according to the same definitions of and approaches to effectiveness, ending with a call for learning designers and researchers to target their measurement efforts to counting what counts for them and their stakeholders.
E-learning System. shakir abdirahman. 2022. In this thesis, we present a practical guide to developing and implementing successful e-learning management systems. We cover a range of topics, including: Planning and designing effective online courses and programs Utilizing technology and software to facilitate learning and engagement Managing and ...
Learning Management System (LMS), or e-learning platforms, are specialized online platforms that support e-learning, that is, online learning and training through content and communication sharing.
E-Learning was viewed favorably by teachers and students as an effective tool to enhance the delivery of instruction and develop knowledge acquisition skills through transfer of learning.
With the advancement of online-based learning systems, learner control over the instructional process has emerged as a decisive factor in technology-based forms of learning. However, conceptual work on the role of learner control in e-learning has not advanced sufficiently to predict how autonomous learning impacts e-learning effectiveness.
Thesis submitted for the degree of Doctor of Philosophy by Mubarak M Alkharang School of Information Systems Computing and Mathematics Brunel University 2014 . PhD Preface Mubarak M AlKharang II PhD Summary E-learning has emerged as a necessity to meet the challenges posed by the development of information technology and its potential for ...
A learning management system (LMS) is a software application or Web-based t echnology. used to plan, implement, and assess a specific learning process. The E-Learning come under. from application ...
E-learning is broadly defined as the use of electronic technologies in teaching and learning. But rivalrous views of e-learning as a technological artifact, a mode of delivery learning, a multidomain transformation of teaching and learning, or an apocalyptic "new wave" affect its use and success in higher education, because they define ...
E-learning systems are receiving ever increasing attention in academia, business and public administration. Major crises, like the pandemic, highlight the tremendous importance of the appropriate development of e-learning systems and its adoption and processes in organizations. Managers and employees who need efficient forms of training and learning flow within organizations do not have to ...
Research on information systems has identified a variety of factors across a range of adoption models that determine their acceptance. In this research, the unified theory of acceptance and use of technology (UTAUT), which integrates determinants across eight models, was utilised to analyse students' intentions to use and their actual usage of Moodle, an e-learning system at Hashemite ...
The purpose of this paper is to design an adaptive e-learning environment based on students' learning styles and study the impact of the adaptive e-learning environment on students' engagement. This research attempts as well to outline and compare the proposed adaptive e-learning environment with a conventional e-learning approach.
A review of the literature points out that a successful e-learning system should consider the personal, social, cultural, technological, organizational and environmental factors to offer academic ...
This thesis study examines the factors influencing teachers' use of e-learning to teach math. The study aims to identify those variables that have a significant correlation with the use of e-learning. The purpose of the study is to help improve schools' current and future implementation of e-learning by understanding and addressing the factors that have a significant correlation with the ...
A personal computer is usually used for delivering training or computer-enhanced learning related to e-learning (Samsuri, Nadzri and Rom, 2014). Other communication technologies deliver learning based on tutorials, learning support systems, and online lectures (Kattoua, Al-Lozi and Alrowwad, 2016).
The theoretical framework is a contribution for guiding e-learning studies. The article classifies the stakeholder groups and their relationship with e-learning systems. The framework shows a ...
With the emerging use of technological interventions in education, e-learning systems contribute immensely in educational delivery and with substantial efforts from the Rwandan Government, substantial efforts are being made to improve the quality of education. With the emerging use of technological interventions in education, e-learning systems contribute immensely in educational delivery ...
These results demonstrated that, regardless of how well the educational institutions are prepared to promote the use of e-learning systems, other COVID-19-related challenges play a crucial role in forming the intention of students to participate in e-learning during the COVID-19 pandemic. Theoretical and practical implications are provided.
Purpose of the Study. This study examined the integration of the E-learning system and determined the study habits and participation of Junior High School students, especially in the new normal education. This study thus describes and evaluates the integration of E-learning in technology-enhanced education.
This study aims to create an online E-Learning System that provides all in one tool for teachers to develop and administer courses online.
Recently e-learning systems are experiencing rapid development. The advantages of learning through a global network are manifold and obvious: the independence of time and space, learners can learn at their own pace, learning materials can be organized in one place...
DEVELOPMENT OF E-LEARNING SYSTEM FOR PHILIPPINE LITERATURE SUBJECT OF COLLEGE OF ARTS AND SCIENCES IN CAVITE STATE UNIVERSITY An Undergraduate Thesis Proposal Submitted to the Faculty of the College of Engineering and Information Technology Cavite State University In partial fulfillment of the requirements for the degree Bachelor of ...
This presents a need for human-centered explainability techniques that help increase everyday users' understanding of complex AI systems. This thesis examines computational methods for explaining sequential decision-making AI systems such that both end users and AI systems benefit in improved understanding and performance.
The presentation of online learning materials through these systems increases students' engagement in educational processes, motivation (Navimipour & Zareie, 2015), and success (Simonson, 2017).
Seven Ph.D. students working with CS&E professors have been named Doctoral Dissertation Fellows for the 2024-25 school year. The Doctoral Dissertation Fellowship is a highly competitive fellowship that gives the University's most accomplished Ph.D. candidates an opportunity to devote full-time effort to an outstanding research project by providing time to finalize and write a dissertation ...