Trends and new practical applications for warehouse allocation and layout design: a literature review
- Open access
- Published: 30 November 2023
- Volume 5 , article number 378 , ( 2023 )
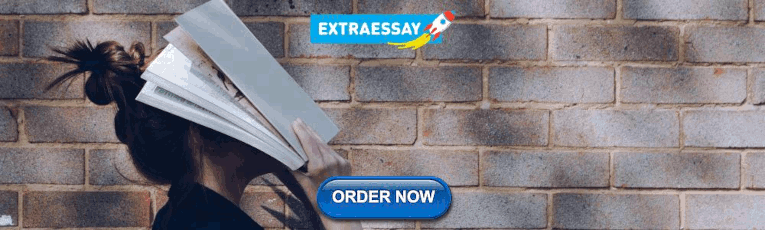
Cite this article
You have full access to this open access article
- Pierre-William Albert 1 ,
- Mikael Rönnqvist 1 &
- Nadia Lehoux 1
2016 Accesses
Explore all metrics
Companies’ productivity is critical in contemporary warehouse environment to maintain efficiency and competitiveness within their supply chain. Warehousing operations are well known for their impact on the overall supply chain and need to be wisely managed. Among those activities, allocation planning and layout design are some of the most important concepts in practice. Current research tends to emphasize homogeneous environment, which leaves heterogeneous and non-standard cases with less attention, even today. This paper aims to review the literature regarding warehouse allocation planning and layout design methods that could suit practical industry problems, with a focus on heterogeneous and non-standard spare parts. It also aims to describe the current trends in these fields. Following a literature review methodology, a total of fifty-seven articles were reviewed to identify the methods developed and used. The reviewed papers were also investigated in order to identify research gaps and future directions. The analysis concluded that more research is needed to better understand and optimize heterogeneous and non-standard spare parts environments in terms of allocation and layout design. More practical case applications also remain a gap to address.
Article highlights
This article evaluates 8 concepts/parameters related to warehouse allocation operation. This is to ensure a deep analysis of heterogeneous and non-standard parts presence in literature.
A research gap is identified regarding heterogeneous and non-standard parts in warehouse allocation and layout design methods in the scientific literature.
This article evaluates 3798 scientific papers over a 20-year timeframe. From this, we present 57 methods and 8 gaps in scientific literature.
Similar content being viewed by others
Special Optimization Process for Warehouse Layout Design
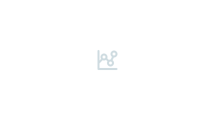
Warship spare parts configuration optimization for stock control: Investigating the gap between qualitative and quantitative constraints
Warehouse Design and Order Picking Algorithms for Apparel Industry
Avoid common mistakes on your manuscript.
1 Introduction
Faced with stiff economic and international competition, companies need to become truly efficient in warehouse operation planning to fulfill their mission and remain productive [ 59 ]. Nevertheless, in real-life situations, optimizing warehousing layout design and allocation is often a challenge to achieve a feasible solution [ 88 ], as different factors may be difficult to overcome [ 85 ]. Moreover, 90% of warehouses in the world are still managed manually or with low-level automation [ 50 ]. The recent changes in global uncertainty and risk on the supply chain that Covid-19 imposed is a good example of a factor which had a real impact on warehousing systems [ 76 ]. Furthermore, customer behavior and preferences can change at any moment and logistics should be able to keep pace [ 46 ]. Thus, a robust warehouse layout design and an efficient internal product allocation process enhance the probability of success [ 24 ]. [ 31 ] highlighted three priorities for optimal management systems, involving reduction of the use of sheets to keep track of the operations performed, to manage real-time inventory needs, and to maintain high productivity levels.
In this article, we perform a literature review that focuses on warehouse layout design and allocation planning methods. We focus on heterogeneous spare parts which suits practical industry problems. [ 6 , 44 , 75 , 88 ] all deplored the lack of literature on the practical effect of real-life features on warehousing operational planning activities. Many challenges arise with heterogeneous parts and real industrial constraints. [ 13 ] suggested an increase in the complexity of warehouse handling activities. [ 75 ] highlighted the difference in complexity for heterogeneous parts characteristics and their impact on warehousing and maintenance inventory. Specific interest in evaluating spare parts in such industrial realities is clear. In fact, the aftermarket business model and operational realities are completely different than in industrial production in relation to the uncertainty factor [ 75 ]. Thus, a focus on these aftermarket spare parts becomes evident to increase the understanding on this subject and determine storage needs and capacity. [ 110 ] From an economic perspective, spare parts play a critical role in the value for the customer and enterprise. This increases the need for further research on this matter since this subject still seems to best in its infancy.
The objective of this study is therefore to highlight the most promising methodologies and approaches that could suit such environment. To achieve this goal, concepts related to warehouse operations planning from the last 20 years (2000 to 2022) were analyzed to answer the following four research questions in relation to specific keyword sets. For each keyword iteration, one or more of those elements such as non-standard/heterogeneous, trends, practical case studies, and upcoming technologies were analyzed in the initial screening:
RQ1 Which methods were developed to improve productivity in warehouse allocation planning?
RQ2 Which methods were developed to improve productivity in warehouse layout design?
RQ3 Which methods were proposed to improve productivity in warehouse regarding allocation and layout design simultaneously?
RQ4 Which methods were used to increase productivity for practical case studies, especially for heterogeneous non-standard parts environment?
To answer these questions, we used a set of specific keywords. For each keyword iteration, one or more of those elements such as non-standard/heterogeneous, trends, practical case studies, and upcoming technologies were analyzed in the initial screening.
Trends and emerging practices were also examined during the analysis. The investigations showed that smaller and more frequent transactions occur in warehouses [ 31 ]. Also, product allocation planning regarding handling constraints for workforce security management seems to be on the rise [ 86 ]. Another concept in self-storage warehousing with customer choice appears as a contemporary issue [ 113 ].
The remainder of this paper is as follows. Section 2 summarizes the main concepts of warehousing and operational planning. Section 3 describes the methodology used in this paper. Section 4 presents the answers to the research questions and some recommendations. Section 5 concludes the paper.
2 Preliminary concepts
This section presents some preliminary concepts about warehouse layout design and allocation planning.
2.1 Layout desing
Warehouse layout design typically focuses on the positioning of docks, reception, racking location, racking height, and slots dimensioning. [ 46 ] described warehouse design activities as the physical layout, the storage equipment, the handling equipment, automation solutions, information systems, labor, and activities to determine. Physical layout can be described as the aisle and lane configuration, the stacking height, while storage, and handling equipment, including racking, forklift, and carousel. [ 93 ] tries to solve a warehouse design for a block stacked warehouse. They determined the lane depth, stages, and temporary additional capacity which is of dynamic nature. Thus, a lack of flexibility and capacity which evolve dynamically can have a major impact on the system [ 21 ]. [ 36 ] proposed a review of warehouse layout design methods and identified five decisions as follows: overall structure, department layout, operation strategy, equipment selection, sizing, and dimensioning. They also introduced the importance of benchmarking and analytical operations for a better performance evaluation. [ 96 ] investigated the warehouse layout problems and concluded on the utility of the cube-per-order index (COI). [ 63 ] presented a literature review that looks at designing warehousing systems with a focus on order picking. [ 107 ] presented a review for optimal warehouse designs with case study applications. They focused on average order picking and total traveled distance for determining the design.
Sometimes layout design needs to be reviewed to be more efficient, as redesigning might be more suitable and less expensive than building a new warehouse. [ 26 ] suggested how to redesign production layout for real case scenarios with a dedicated storage method. [ 5 ] followed the redesign layout planning for a systematic layout planning usable on real case studies. Another approach for real cases is considered by [ 81 ]. They evaluated different warehouse layouts by comparing a classic layout design with other general layout concepts like fishbone, class-based, shared storage, dedicated, and random. [ 43 ] utilized the warehouse design dedicated policy for a real case study. [ 67 ] followed the redesign by proposing layout changes and handling methods for a real case raw material storing process. [ 69 ] presented a case study with limited space situation and for warehouse layout design in a specific automobile material supplier. Their main objective was to design two warehouse layouts to fulfill the company storage assignment. They followed a class-based approach. [ 40 ] presented a design methodology for converting regular warehouses into different zones. [ 84 ] resolved a warehouse layout design for a real case problem while mixing allocation techniques such as ABC class-based storage. [ 51 ] worked on the design of a multi warehouse inventory model with the rain optimization algorithm. They also presented the concept of optimal replenishment policy (ORP). Figure 1 presents a dynamic cross-docking layout design for easy picking.
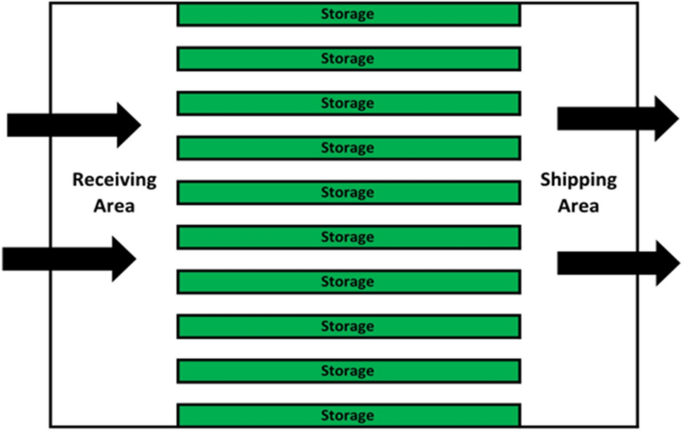
An illustration of a dynamic picking zone model
2.2 Storage, product allocation, and slotting
Storage, product allocation, and slotting all refer to the capacity and ability to put items correctly in a specific place. [ 37 ] specified that one of the most important things in warehouse operations planning relates to the storage and allocation of products to the right storage positions. [ 31 ] explained that SKU and storage zones, including strong correlation together, should be the closest possible to each other. [ 48 ] described slotting as the operation to find the best location to keep an SKU inside the storage zone. Thus, many problem-solving algorithms or methods related to allocation were developed over the years. Here is a non-exhaustive list of allocation problems or methods one can find in the literature: the multi-layer allocation problem (MLAP), the storage location allocation problem (SLAP), the class-based storage location allocation problem (CBSLAP), dedicated storage, random, closest, farthest, ABC class, forward reserve, and zoning. SLAP is derived from the more general location allocation problem (LAP). [ 14 ] studied storage and stacking in the marine transportation industry. The objective was to stack sea cans in a fixed stack with limited height while the stacks had to be at a workable distance from the cranes. [ 57 ] focused on stacking goods for a real case study in container stacking in ports which they defined as the stack loading problem (SLP). [ 64 ] described the MLAP problem as a variation of the SLAP problem, including multiple floor levels for bins in the warehouse. In other words, the MLAP differs from the general SLAP by adding another dimension in the storage with floors and height. [ 104 ] followed the class-based storage location but used a constrained clustering method as well. To achieve their results, the COI index was used. [ 33 ] also followed the class-based storage method for a real case study. [ 19 ] worked on order picking in a multi-level rack warehouse with a class-based storage policy. In their paper, they pointed out important factors to take into consideration like the material handling system, product characteristics, demand trends, and the turnover rate. They also regrouped the storage assignment policies in three main groups which are random storage, dedicated storage, and class-based storage. [ 3 ] wanted to optimize storage classes via a minimum travel cost. They ranked location with frequencies, arrivals, and demand. [ 108 ] also presented a class-based storage with a finite number of items. They concluded that using more classes is not always better. [ 11 ] investigated the CBSLAP in more depth with a class-based storage location assignment overview of policies and classification. Their classification for storage policies separated the SLAP problem between dedicated and shared storage, dedicated storage being linked to parts number, turnover rates, COI, and correlated items while shared policy being separated between class-based and haphazard. [ 32 ] tried to solve the general assignment problem by including worker capabilities. [ 45 ] used a genetic algorithm to solve a multi-objective storage allocation. [ 98 ] interacted with the intelligent storage allocation with multiple objectives. They used class-based with fixed location and worked with flood management. Forward reserve is another option for storage and allocation. [ 100 ] presented a forward reserve storage strategy for customer order mixed with class-based criteria. The objective was to measure customers’ order response time and the overall system capacity in an AS/RS situation. A forward pick area can be described as a warehouse within a warehouse. It can help to stock the most popular items in small amounts to reduce the main picking zone [ 9 ]. It can then be replenished with bigger reserve storage.
3 Research methodology
To find the most promising methods that could suit practical industry problems related to allocation planning and layout design, especially for heterogeneous spare parts, a literature review approach called a conservative search and screening methodology. This approach is a reliable methodology that could be easily repeated by any reader. In fact, the methodology is used in other papers. [ 23 , 36 , 90 ] Also, to the best of our knowledge, no other papers were evaluating heterogenous environment even at a low importance concept which represent a large gap between the literature and the practical world. Thus, a conservative and screening approach was more adequate to clearly address the research question. The process together with the number of articles identified in the steps is illustrated in Fig. 2 .
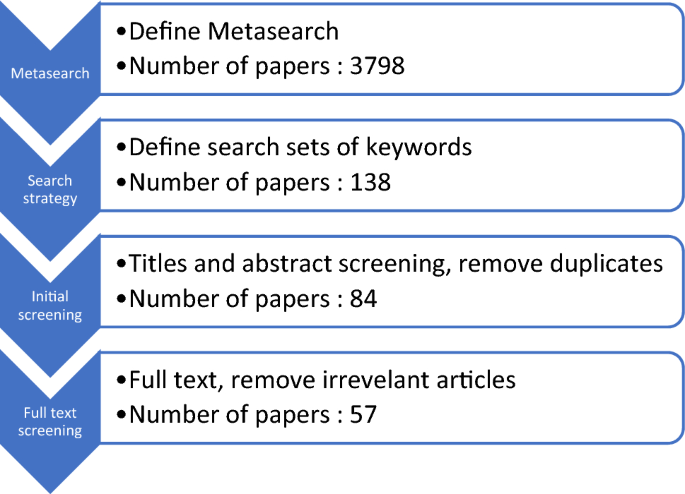
Conservative search and screening methodology
3.1 General Methodology
The first step of the method involved defining the research questions. To identify promising methods that would be useful in the real world, especially when dealing with non-standard space parts, the questions to investigate were defined. The objective was to find papers related to allocation planning, layout design or both concepts simultaneously. The last question is concerned about practical case studies and heterogeneous/non-standard environment.
The second step involved determining the keywords, the research databases, the source types, the language considered, and the years of publication. We investigated different combinations of keywords related to the variability of warehouse operations concepts. Thus, we first separated those concepts as follows: “warehouse allocation planning”, “warehouse layout planning”, “non-standard shelf layout or warehouse variable layout or warehouse adaptable layout”, “warehouse operational planning”, “warehouse limited storage capacity”, “non-standard or heterogeneous or diversified spare parts”, “warehouse capacity utilization”, “warehouse total storage capacity or warehouse storage capacity”, “warehouse storage management”. Then, a mix of keywords to identify previous literature reviews was searched: “literature review and warehouse allocation”, “literature review and warehouse layout design”, “literature review and warehouse operational planning”. All keywords set iterations evaluated the best articles related to the main concepts of this research which are non-standard/heterogeneous, trends, practical case studies, and upcoming technologies.
The research database “Compendex” and “Inspec” were the ones selected as being recognized as useful tools in the field of engineering and operations management. To limit the number of papers to examine, a focus on scientific journal papers was determined and English was the only language considered. Only papers published from 2000 to 2022 were considered from an evolutionary perspective of how the current subjects evolved through time while detecting the new trends emerging from such subjects. The reason for the diminution in the number of papers between the metasearch and the search strategy is related to the keywords sets which involved a focus on allocation or layout design concepts. All the papers with a focus on other main concepts such as picking, routing, automation technology or shipping were eliminated from the search. This reduced the number of papers from 3798 to 138.
The third step involved conducting an initial screening by looking at the titles and abstract to determine whether the articles were relevant for this study or not. Once again, articles focusing on concepts other than allocation and layout design were excluded, as well as purely theoretical studies considering a perfect homogeneous environment. This decreases the number of papers to be reviewed in the next step to 84.
The following step encompassed a full-text screening. A total of 57 papers were finally kept for the literature review.
4 Results and discussion
4.1 descriptive results.
Figure 3 presents the number of reviewed papers per year. We can see that research on warehouse allocation and layout design concepts has increased in recent decades and that 85% of the literature reviewed has become available since 2010.
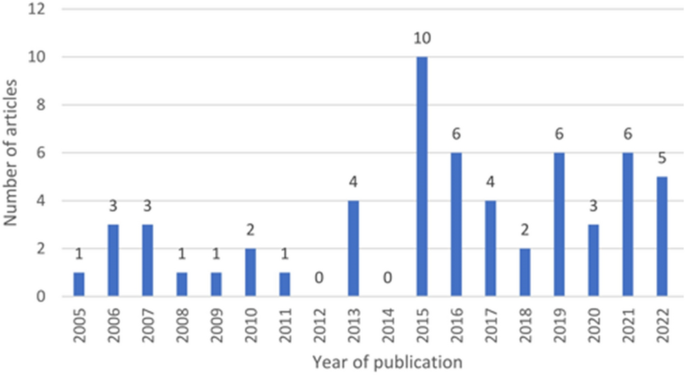
Statistics concerning the papers reviewed per year
Figure 4 shows the total number of papers based on the country of affiliation of the first author. All the countries with at least 3 papers reviewed are shown within this figure. Figure 5 describes the number of articles by journals in ascending order when at least 2 articles were published in them. The International Journal of Production Research, the International Journal of Advanced Manufacturing Technology, and the European Journal of Operational Research are the most frequent journals in the field. Figure 6 describes the number of papers looking at the two main concepts together (allocation and layout design) per year. Table 1 is related to the number of papers per methodology. Simulation methodology refers to research including a simulation model and/or the use of simulation software. A literature review methodology refers to a paper in which the research core is concerned with presenting the literature and papers related to the specific concepts of a scientific field. Analytical methodology refers to research papers including a mathematical model or a heuristic. Analytical represents mathematical models including algorithmic models that normally look for an exact solution and optimization model. Heuristics models are also included. A qualitative methodology refers to research papers describing conceptual frameworks, questionnaires, or factual data. Most of the reviewed papers used mathematical models to identify an optimal solution. Only a few articles exploiting heuristics were found. Table 2 presents the concepts studied in this research according to degree of popularity (number of papers and their overall percentage on the total numbers evaluated).
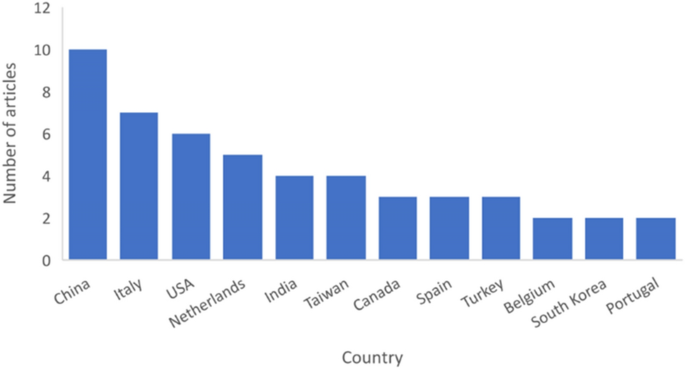
Papers distribution of publications based on first author’s country
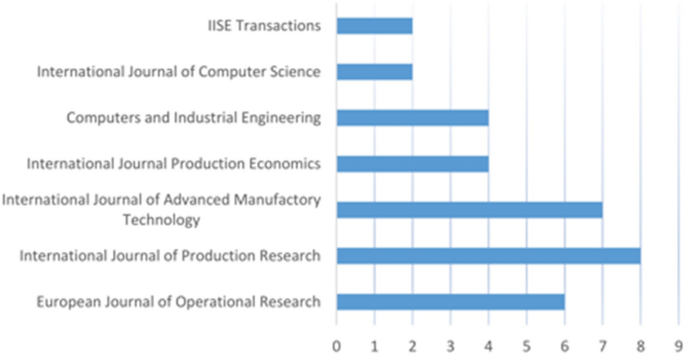
Papers reviewed according to the name of journals
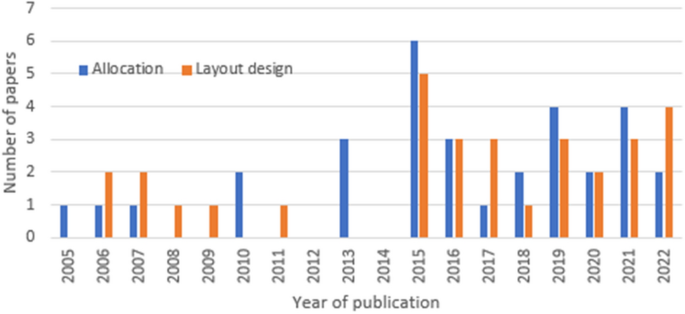
Papers reviewed per year based on the main concepts (allocation and layout design) considered in this study
4.2 Research questions
This part presents all relevant findings in relation to the research questions investigated.
4.2.1 Question #1
4.2.1.1 which methods were developed to improve productivity in warehouse allocation planning.
The first research question was related to warehouse allocation planning and the current approaches and methods available in the literature to solve this problem, especially regarding non-standard, heterogeneous environments. In [ 85 ] an architecture for product allocation planning with compatibility and safety constraints and with an IoT-based warehouse. A proposed multi-agent architecture for PAP with compatibility constraint was described. [ 86 ] focused on a product allocation planning (PAP) problem with handling constraints within a case study. The constraints evaluated were the weight and the shape. The model followed a zero–one quadratic assignment. They brought something new as the Covid-19 handling constraints which add additional challenges to operational planning. Their modeling led to 24% reduction in picking distance by implementing ABC clusters and odd shaped constraints. [ 98 ] described a dynamic programming approach for the SLAP problem. The main objective was to enhance order-picking with good storage location in a dynamic perspective. [ 111 ] mixed IoT, integrated production planning, and warehouse storage assignment for a practical case. A linear programming model minimizing the total production cost and warehouse operations was presented. [ 80 ] followed the same thinking with an integrated model for warehouse and inventory planning. They also evaluated the capacity restrictions in inventory planning. [ 61 ] proceeded in a separate direction with a class-based storage assignment over life cycle picking patterns. The model was based on a mixed-integer linear programming (MILP) pattern. They introduced product popularity (consumption level) as the main characteristic for an SKU storage level. The more popular it was, the closer to floor level it had to be. [ 55 ] focused on the optimization of warehouse storage capacity with a dedicated storage policy. Moreover, this paper aimed to determine the space requirements under a dedicated storage policy. Thus, they used a non-linear programming model to minimize the cost of space used in the warehouse. [ 58 ] evaluated beyond static scenarios with a dynamic storage assignment with product affinity and ABC classification. They proposed a case study to show the usefulness of such a scenario. Their method used a product affinity-based heuristic with a quadratic assignment problem. [ 41 ] created a model for storage arrangement and reallocation in storage operations. The model proposed a heuristic algorithm for storage reallocation. [ 70 ] aimed to maximize the storage space availability inside a chaotic warehouse via a heuristic for the SLAP problem in a multi-level case. Their method was also usable for determining the right timing for relocation. [ 3 ] checked the allocation problem for the unit-load warehouse and tried to optimize it via storage classes. The focus of the paper was on describing a potential solution for practical cases. The method was solved with linear programming. [ 38 ] evaluated the impact of storage space on storage policy performance in a unit-load warehouse. The study also found that class-based storage performs better than turnover-based policy. [ 99 ] pointed out that literature normally evaluates single-period instead of multiple-period for orders in warehouse. Thus, warehouses should rather be seen as a dynamic place which needs to evolve over time. The proposed innovative way for warehouse storage allocation evaluated the entry-item-quantity and then sorted all SKU. [ 104 ] followed a constrained clustering method for class-based SLAP in a warehouse. The originality of such method lies on the constrained cluster and in the practical storage constraints evaluated. They used the COI index for grouping and sub-grouping and then found results with a heuristic assignment model. Multiproduct slot allocation heuristic was the main object in [ 10 ]. The objective was to minimize storage space with such a heuristic. The model linked to a real industry case and showed good results with a dedicated storage policy. [ 108 ] discovered an interesting correlation that more classes in a class-based allocation strategy are not always better. Their research evaluated the class-based storage with a finite number of items in a SLAP problem. They found that 3 to 8 classes were normally sufficient to obtain near optimal solutions. [ 102 ] also evaluated class-based storage policies for the allocation problem. This time, the focus was on optimal dimensions for multi-deep storage systems instead of standard warehouse. This is related to AS/RS automated storage systems. Once again, the utilization of class-based strategies was relevant. [ 47 ] looked at large distribution centers for their storage location assignment heuristic based on slot selection and frequent itemset grouping. They evaluated a new dimension of SKU by integrating itemset grouping, which led to better agglomeration of parts in a smaller slot. [ 29 ] worked on the SLAP problem with order picking optimization in the automobile industry. They solved it with a class-based policy and batching and routing problems via an integer programming model. In [ 59 ] a linear programming and decision-making model was presented to improve warehouse utilization. The objectives were to find the best decision for storage racks with box placement.
4.2.2 Question #2
4.2.2.1 which methods were developed to improve productivity in warehouse layout design.
The second question focused on the warehouse layout design concept, with a focus on non-standard, heterogeneous environments. Layout design modeling for a real world just-in-time warehouse was presented in [ 42 ]. They proposed another perspective for layout design related to just-in-time warehouse instead of a traditional one. [ 66 ] used a particle swarm optimization algorithm for the multiple-level warehouse layout design problem. The paper proposed solutions such as orientation of storage racks and the overall configuration. For non-conventional warehouse design, [ 30 ] discussed a heuristic comparative assessment for unit-load warehouse. They used the FlexSim software to present a simulation of different designs. [ 16 ] proposed solutions to determine slot sizes in a unit-load warehouse. The purpose of this paper was to go beyond the equal height slot generally found in the literature and to present different slot size possibilities in one solution. [ 78 ] tried to enhance manual order picking areas via the design of the layout structure. Their model minimized the travel distances in the picking area with parallel structure. Another possibility with warehouse models is self-storage warehouses. Thus, [ 113 ] described a design for self-storage warehouse with customer choice. They used a customer choice model to evaluate the probability of purchase. This model was a mixed-integer model solved with the branch-and-price algorithm. A guideline was proposed by [ 83 ] for developing a design in a case-picking warehouse. The main objective was to determine guidelines for good design in manual case-picking warehouses. [ 79 ] explained how to estimate optimal ABC zone sizes in manual warehouses. They presented the benefits of ABC class-based storage policy for SLAP problems. The zoning experiments were compared between one to three zones (20/30/50) patterns. [ 103 ] investigated a fuzzy multi-level warehouse layout problem. A tabu search was utilized and presented a good steppingstone for the layout problem. [ 73 ] mixed the determination of warehouse layout and control policies in their paper. [ 39 ] studied the uncertainty theory method in relation to the multi-level warehouse layout problem. Uncertainty factors were included, and two different models’ scenarios were evaluated. [ 94 ] followed a different path with the imperfect staggering in product inflow with queuing theory. The paper explored the block stacking layout problem with a model of the imperfect staggering effects. [ 6 ] discussed a structured approach for warehouse design. The paper of [ 15 ] related to economic and ergonomic performance measures helped to understand the rack design for the warehouse layout design problem. [ 17 ] focused on the fishbone warehouse design with vertical travel. The paper presented a three-dimensional design with a fishbone layout. Another point in layout design is to manage the deep lane storage system layout. [ 1 ] proposed an iterative decision-support model for this problem with block stacking storage environment. A simulation and the analytic hierarchy process for decision support systems were presented in [ 76 ]. The focus was on air cargo warehouse capacity design. Thus, they tried to integrate a practical case study with a complex simulation. The question between automated or manual storage systems was the subject of [ 110 ]. The authors proposed insights for managers to select the most appropriate storage systems. [ 91 ] wanted to optimize layout for a three-dimensional order picking warehouse. Their work helped to determine layout warehouse while determining the depth and number of levels of such a warehouse. On a more global note, [ 23 ] presented the major changes in the last years in terms of warehousing. This paper encompassed the new trends and technologies and gave insights concerning what is happening nowadays. [ 92 ] evaluated the design of an order-picking warehouse with vertical travel and space sharing. The warehouse was a single block rack-based and the model integrated practical case data.
4.2.3 Question #3
4.2.3.1 which methods were proposed to improve productivity in warehouse regarding allocation and layout design simultaneously.
Regarding the study of both concepts simultaneously (allocation and layout design), only a few publications were found. Those articles mainly focused on reviews or general models. In [ 13 ], assembly kits were an important variable to determine warehouse layout design and assignment procedure. The main difference with previous research was based on the physical attributes of the parts evaluated and their occurrence in assembly kits. In [ 24 ], an overview of the problems found in layout design, storage assignment, and order concepts of warehouse operational planning such as zoning was proposed. They described many advantages of good practices in warehouse operational planning, such as transportation/production economies, reduced lead time uncertainty, increased customer service, and satisfied changing market conditions. Another review was found in [ 35 ]. The review based on warehouse operations enhanced the reader’s knowledge on various operations planning problems, including the layout design and allocation problem. [ 105 ] tried to minimize operating costs in a picker-to-part warehousing system. From this, an evaluation on multiple concepts from warehouse operational planning (WOP) was developed, which included allocation and layout design. The paper proposed an algorithm determining the number of zones, bins, and racks. [ 97 ] integrated an optimized fishbone warehouse layout with a SLAP problem and a picker routing. With those 3 concepts from WOP, they proposed a 3D layout design with a turnover-based assignment for allocation. In another spectrum, [ 34 ] incorporated human factors in order picking planning. [ 13 ] designed diagonal cross-aisle warehouses including a class-based storage assignment strategy. They worked on a non-traditional model such as diagonal cross-aisle with unit load and compared their results with a traditional and random one. In [ 90 ], the design of efficient order picking systems with a planning combination was studied. The main objective was to show which combination policies could be used for such problem.
4.2.4 Question #4
4.2.4.1 which methods were used to increase productivity for practical case studies, especially for heterogeneous non-standard parts environment.
For the last question, the literature related to practical case studies and heterogenous non-standard spare parts (SKU) was evaluated. However, a few numbers of articles on non-standard spare parts were found while practical case studies appeared to be a little more frequent. [ 30 ] worked on a heuristic for non-conventional warehouse designs. The fishbone design seemed to be the most effective method in terms of warehouse designs [ 13 ]. worked on assembly kits with variable parts and physical attributes. They looked for solutions to agglomerate the assembly kits parts in corridors and to create such a kit in a single warehouse corridor. Part categorization was the main procedure for such a solution. Then, one could determine the warehouse corridor and structure afterwards. [ 78 ] focused on the limited storage capacity problem found in some warehouses. They proposed an optimal policy for multi-item systems with stochastic demand and backlogged shortages. The solution was an inventory model for multiple products. [ 75 ] worked on maintenance inventory allocation with heterogeneous part characteristics. The paper helped to better understand dynamic demand in non-standard parts systems for specific maintenance with aeroengine. They used an ABC-VED matrix for the classification of distinct categories. [ 12 ] considered a non-traditional warehouse with a diagonal cross-aisle design.
In [ 86 ], the case study analytical model was related to a PAP problem with handling constraints in the retail sector and with manual order-picking operations. The model developed a shape parameter linked with data to distinguish non-conventional parts against standard ones. [ 42 ] evaluated the just-in-time warehouse real-world practical case with the layout design model problem. The case study was in the retail industry. [ 16 ] compared data from different distribution centers in the industry. A key part of their study was the evaluation of slot sizes in warehouses. From this, one could evaluate different slot sizes in a non-standard environment. For the automotive industry cases, [ 29 ] proposed a solution for the SLAP problem and order picking optimization. The solution showed that proximity for storage location is the main factor to minimize the total travel time in assembly warehouses. [ 113 ] evaluated a self-storage in Rotterdam for the design of self-storage warehouses with customer choices. The paper included layout design and a real case study for a specific non-standard warehousing aspect in self-storage. [ 111 ] proposed an IoT assisted case from a food company for warehouse storage assignment and integrated production planning. With the beverage industry, [ 1 ] from a block stacking warehouse presented a way to design and manage deep lane storage system layout. This helped in minimizing the storage space and time inefficiencies.
In their simulation, [ 76 ] worked in the air cargo warehouse capacity design. This case study gave insights concerning how to minimize the cost while enhancing the performance in a highly dynamic environment. [ 58 ] looked at a general case study on dynamic storage assignment including product affinity and ABC classification. [ 3 ] evaluated their method with a third-party logistics provider and a unit-load warehouse. They proposed an approach to optimize storage classes for this kind of environment. The method used the layout information and the product flow. They found that random allocation is the best choice in highly dynamic environment with few allocation bins available at any time. The busier the more random the allocation should be since emergency allocation tends to increase drastically traveling time inside the warehouse. [ 101 ] worked with the steel supply chain in a highly dynamic and look-like SKU environment. Their method was based on a managerial optimization with RFID warehouse. Thus, the allocation and retrieval process could easily enhance its productivity with proper RFID system implementation [ 10 ]. validated their multiproduct slot allocation heuristic with a real industrial case. Even if the main purpose of the paper did not directly involve a practical case implementation, their heuristic aiming at minimizing storage space using a dedicated storage policy meant it seemed to be possible to implement in real life. The constrained clustering method for class-based SLAP by [ 104 ] followed the same reality as in the previous paper. The main purpose of the paper was not directly related to a case study. However, this article tended to obtain satisfactory results for practical issues.
Figure 7 presents a resume of the analyzed paper related to the main criteria of this study.
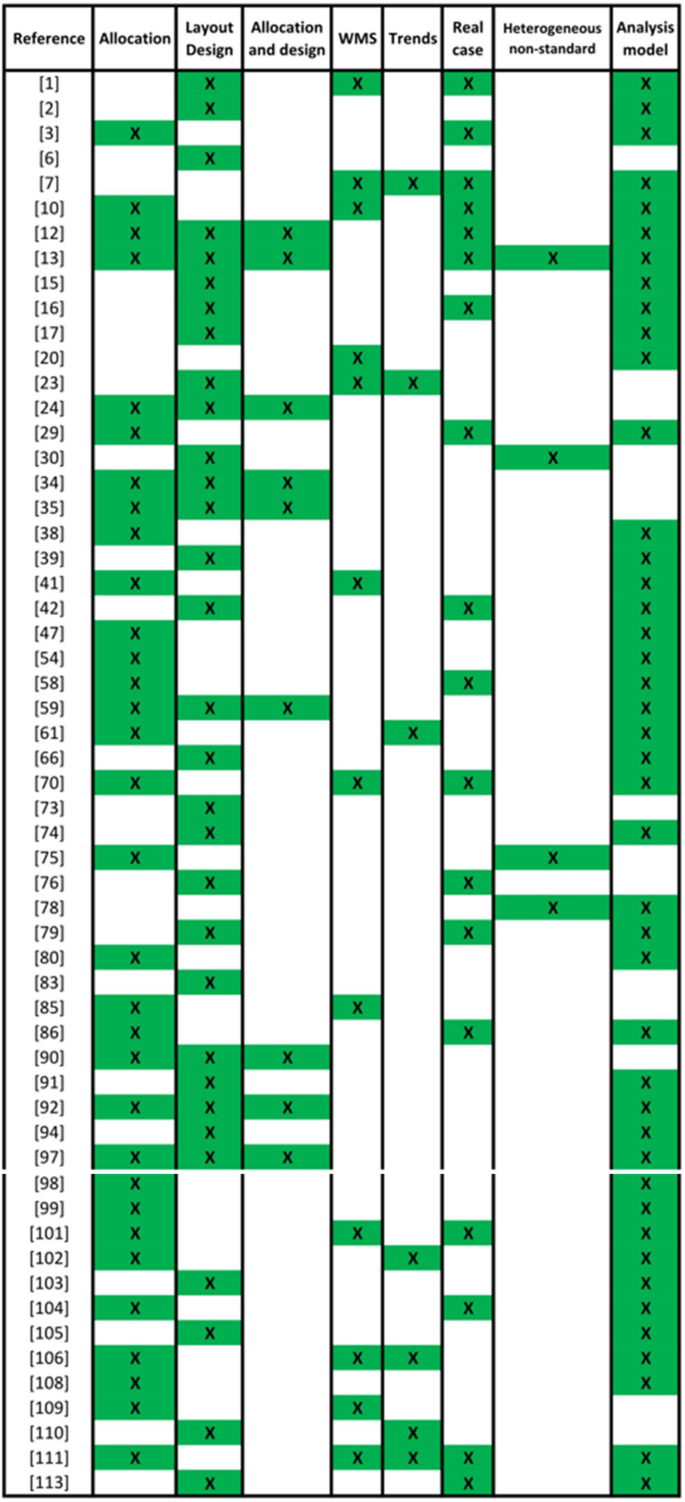
Summary categorization of the analyzed papers
4.3 Discussion
In this section, we discuss the approaches and methods found when conducting the review and their application to solve practical cases. A discussion focusing on the gaps identified as well as the opportunities and trends observed is also proposed.
4.3.1 Warehouse allocation methods
Based on the allocation methods reviewed in this paper, the most common elements considered by the authors were the following ones:
Determining an SKU classification
Considering ABC class within the SKU classification
Allocating block-unit warehouse (standard location)
Minimizing traveling time for SKU picking/slotting
Using the shortest path algorithm for allocation
One of the interesting methodologies proposed in the literature is the forward area where the warehouse storage location is split between a large warehouse containing all the SKUs and a smaller more convenient zone in the forward area. A main challenge identified when reviewing allocation solutions resides with the homogeneous standard evaluation. Most companies are not always standard in their operations and SKU dimensions. Thus, the literature should focus more on flexible warehouse storage solutions with different widths and sizes. More research should be done with methodologies suitable for unconventional items (heterogeneous) size problems.
4.3.2 Layout design discussion
The literature reviewed highlighted that designing storage zones and rack dimensions were the main elements studied by the authors. Nevertheless, the methods typically involved static solutions. There seems to be a lack of research on flexible layout design. Most of the layout design-oriented research also focused on classic rack layout problems with general, same-size SKUs. However, in practical case situations, SKUs of various sizes and dimensions can be handled. More research could certainly be conducted for evaluating the impact of SKUs characteristics with non-standard or variation in dimensions, evaluating the impact of dynamic factors over time, the impact of e-commerce/mass customization, as well as the impact and productivity of non-conventional layouts (e.g., cross-docking, JIT, heterogeneous parts).
Also, workforce behavior and management cannot be neglected to fully implement new designs, e.g., the impact of heavyweight SKUs on potential injury for a worker. Some possibilities for future research could include evaluating managerial and workforce variables in WOP research, including union or work restriction realities in algorithms and simulation models, and evaluating the impact of unconventional temperature environment on well-being and performance.
The Covid-19 epidemic showed how dramatically such events could impact the overall practice and realities in warehousing. The crisis has shaken the foundation of many concepts such as lead times and security stocks. [ 31 ] reported important changes over the years in warehousing activities. Among them, smaller and more frequent transactions [ 23 , 89 ]. This reality drastically increases the operations planning because of the volume increase of purchase orders and leads to more come and go for the pickers inside the warehouse. Another reality is an increase in item returns. This can be seen with e-commerce [ 23 ]. [ 89 ] dug into the emerging practice due to the recent change in order number and the increasing “just-in-time” demand from customers. In terms of time, [ 82 ] explained that companies should be able to complete customer orders in less than 24 h for more than 98% of overall order. [ 46 ] talked about the increasing complexity for inventory management where workforce seems to need to develop new capacities and understanding of warehousing activities. [ 51 ] made a three-decade literature review about warehouse research. Their results showed that the emerging keywords in terms of warehousing are related to automated & intelligent warehousing such as integrated WMS, application of industry 4.0, and AI sensors. [ 55 ] presented a case study to design and describe an application of Internet of Things (IOT) in the industry 4.0 era. [ 21 ] made a literature review presenting flexible automated warehouses. In terms of emerging technologies, dynamic/flexible automation is an important part of it. In this matter, flexibility can be interpreted as giving more opportunities to adjust to market changes such as e-commerce, customization, and just-in-time. Automation can be defined as the technology that processes tasks without human workforce or assistance. Emerging technologies presented in [ 21 ] are Internet of things (IOT), cyber-physical systems (CPS), Big data, artificial intelligence (AI), and cloud computing. The future of warehousing is slowly converging to fully automated systems and unmanned procedures. One should determine which level of automation is required to be effective and depending on the resources. There is an opportunity for future research to look at automation levels and the impact on productivity and cost, the minimum automation level to adopt in a specific warehouse, cross-automation on multilayer warehouses, including the impact of human workforce on floor levels and automatic picking on floor levels, dynamic and flexibility related to automation, as well as methodologies for automated implantation in practical case studies.
4.3.3 Mixed concepts
As stated in RQ3, some of the papers in this review integrated both concepts of warehouse allocation and layout design. However, most of the papers were literature reviews, explaining the concepts and presenting what has been done so far. Only one paper included an analytical model for a potential solution with a practical case and only one with a non-standard environment. There seems to be a necessity to conduct more research on both concepts supported by practical case studies. The reasons for such low interest in this matter are still unknown.
4.3.4 Practical cases and non-standard
During the analysis, practical case studies were found in the literature. However, most of the time, practical cases meant that some data from a real warehouse were integrated to a mathematical model to generate results. Those results do not necessarily confirm whether the implementation of such a solution is suitable for real-life practical cases. Moreover, some papers proposed high-level algorithms that probably only a few companies could use, and which would necessitate skilled workforce and/or a significant implementation cost. More research could thus look at practical case implementations involving allocation and layout design methods.
4.3.5 Future research directions and gaps
Based on the papers investigated, future research directions and gaps to fill in the literature could be summarized as follows.
Workforce satisfaction and well-being increase overall productivity in warehouses. New or adapted methods could therefore be proposed to consider this key element in heterogeneous environment and make the comparison with the standard one. This could increase the understanding of productivity related to specific environment [ 34 , 86 ].
Non-conventional environment such as cold storage and heated warehouses affects workers’ behavior inside warehouses. New management/human resources research mixed with allocation planning or layout design in those kinds of environments could increase productivity. To the best of our understanding, no research evaluates those impacts while mixing them with new innovative productivity solution [ 85 ].
Implementing solutions to practical real-life case studies increases the value for industry and enhances the research opportunity in the warehouse operations field. New adapted methods should be proposed to limit the gap between theoretical models and real-life applications that, most of the time, are stated in the literature [ 16 , 30 , 39 ].
Combined concepts solutions give a faster and more flexible way of solving problems for managerial teams. Most of the papers propose one concept without evaluating the overall warehouse activities for practical case scenarios. This could lead to improvement in one concept but cause problems for other ones. Thus, evaluating the impact of one warehouse operational concept on another could give a better understanding on operations correlation in real-life cases. As shown in the results, few papers really evaluate more than one concept and even fewer integrate them to practical situations, which presents an important gap in the literature.
Non-conventional or heterogeneous warehouse types such as cross-docking, heterogeneous, and JIT environment are not uncommon in the industry. However, as shown in the results, few studies are related to those. New methods and approaches could be proposed with those elements in further research.
The Covid-19 pandemic created drastic changes in terms of warehousing health and safety concerns regarding workforce protection in recent years. Research should investigate those impacts on real-life industry with new approaches regarding this topic [ 76 ].
Data-driven operations and automated solutions, enabled by Industry 4.0 technology, are an upcoming reality for warehousing systems [ 21 , 27 , 55 ]. They are expected to play a critical role in increasing warehousing efficiency. Thus, future research could study the impact of this evolution in modern warehousing methods using real case studies to facilitate their implementation. Future research could also include the effect of combining such technologies with sustainable practices on resources consumption and long-term warehouses productivity.
As warehouses may play a role in ensuring more resilient supply chains, some research could be conducted to identify the new warehouse allocation and layout design techniques to adopt so as to build resilience and increase the overall effectiveness in warehouse systems.
5 Conclusion
This paper presented a methodological literature review for warehouse allocation and layout design methods in general and for heterogeneous and non-standard spare parts in particular. The main objectives were to find relevant information regarding methodologies and concepts in that field of study and one of which could be implemented in practical cases.
The main findings were related to the lack of non-heterogeneous and non-standard environments concerning warehouse allocation and layout design methods. We find that very few papers integrated those concepts and even fewer with practical applications. At present, the literature is mainly composed of mathematical models looking at one specific concept such as layout design or allocation. A few papers try to integrate both concepts together and most of the time they do it from a literature review perspective.
The limitations of this paper reside in the specific keywords and sets involved. This could lead to different results if any other keywords were to be added or removed in subsequent research. The time frame can also limit the results. Another specification is the possibility that some research papers are not included in the final version due to initial screening keywords elimination.
We believe that future research might and will focus on emerging technologies and automatization in WOP related to allocation and layout design for productivity enhancement. It might be an interesting opportunity to mix emerging technologies and automation with management policies and workforce. More research on the impact of Covid-19 health issues with WOP is another opportunity for future research. As previously stated, the lack of practical case studies remains a challenge for future research. Also, it would be of great interest to include a bibliometric-based scientific approach for a better understanding of the evolution of this research field with spare parts and heterogeneous components. Finally, we also believe that more complex algorithms and mathematical models will evolve with emerging technologies and hopefully evaluate non-standard environments from a practical implementation perspective.
6 Conflict of interest
All of the authors have no relevant financial or non-financial interests to disclose. Also, none of the authors received support from any organization for the submitted work. Thus, no funding, no employment, no financial or non-financial interests have been received by any authors of this paper. The authors declare that they have no competing interests.
Data availability
All data generated or analyzed in this study are included in this published article [and its supplementary information files].
Code availability
All material and/or code generated or analyzed in this study are included in this published article [and its supplementary information files].
Accorsi R, Baruffaldi G, Manzini R (2017) Design and manage deep lane storage system layout. An iterative decision-support model. Int J Adv Manuf Technol 92:57–67. https://doi.org/10.1007/s00170-016-9962-9
Article Google Scholar
Al-Gwaiz M, Chao X, Romeijn HE (2016) Capacity expansion and cost efficiency improvement in the warehouse problem. Naval Res Log. https://doi.org/10.1002/nav.21703
Article MathSciNet MATH Google Scholar
Ang M, Lim YF (2019) How to optimize storage classes in a unit-load warehouse. Eur J Oper Res 278:186–201. https://doi.org/10.1016/j.ejor.2019.03.046
Azadeh K, De Koster R, Roy D (2019) Robotized and automoated warehouse systems: Review and recent developments. Transp Sci 53(4):917–945. https://doi.org/10.1287/trsc.2018.0873
Bagaskara B, Gozali L, Widodo L (2020) Redesign layout planning of raw material area and production are using systematic layout planning (SLP) methods (case study of CV Oto Boga Jaya). In: IOP Conf. Series: Materials science and engineering, 852. https://doi.org/10.1088/1757-899x/852/1/012122
Baker P, Canessa M (2009) Warehouse design: a structured approach. Eur J Oper Res 193:425–436. https://doi.org/10.1016/j.ejor.2007.11.045
Ballestin F, Perez A, Lino P, Quintanilla S, Valls V (2013) Static and dynamic policies with RFID for the scheduling of retrieval and storage warehouse operations. Comput Ind Eng 66:696–709. https://doi.org/10.1016/j.cie.2013.09.020
Bartholdi JJ, Hackman S, T., (2008) Warehouse and distribution science: release 089. Supply Chain Log Inst. https://doi.org/10.1201/9780849305801.ch1
Bartholdi JJ, Hackman S (2008) Allocating space in a forward pick area of a distribution center for small parts. IIE Trans 40(11):1046–1053. https://doi.org/10.1080/07408170802167662
Battista C, Fumi A, Laura L, Schiraldi MM (2014) Multiproduct slot allocation heuristic to minimize storage space. Int J Retail Distrib Manage 42(3):172–186. https://doi.org/10.1108/ijrdm-03-2012-0024
Behnam B, Hemen P, El-Houssaine A (2019) Class-based storage location assignment: an overview of the literature. In: Proceedings of the 16th international conference on informatics in control, automation and robotics, pp 390–397. https://doi.org/10.5220/0007952403900397
Bortolini M, Faccio M, Ferrari E, Gamberi M, Pilati F (2019) Design of diagonal cross-aisle warehouses with class-based storage assignment strategy. Int J Adv Manuf Technol. https://doi.org/10.1007/s00170-018-2833-9
Bortolini M, Faccio M, Gamberi M, Pilati F (2020) Assembly kits with variable part physical attributes: warehouse layout design and assignment procedure. Assem Autom 40(6):857–886. https://doi.org/10.1108/aa-10-2019-0173
Bruns F, Knust S, Shakhlevich NV (2016) Complexity results for storage loading problems with stacking constraints. Eur J Oper Res 249:1074–1081. https://doi.org/10.1016/j.ejor.2015.09.036
Calzavara M, Glock CH, Grosse EH, Persona A, Sgarbossa F (2017) Analysis of economic and ergonomic performance measures of different rack layouts in an order picking warehouse. Comput Ind Eng 111:527–536. https://doi.org/10.1016/j.cie.2016.07.001
Cardona LF, Gue KR (2019) How to determine slot sizes in a unit-load warehouse. IISE Transaction 51:355–367. https://doi.org/10.1080/24725854.2018.1509159
Cardona LF, Soto DF, Rivera L, Martinez HJ (2015) Detailed design of fishbone warehouse layouts with vertical travel. Int J Prod Econ 170:825–837. https://doi.org/10.1016/j.ijpe.2015.03.006
Caron F, Marchet G, Perego A (2000) Optimal layout in low-level picker-to-part systems. Int J Prod Res 38(1):101–117. https://doi.org/10.1080/002075400189608
Article MATH Google Scholar
Chan FTS, Chan HK (2011) Improving the productivity of order picking of a manual-pick and multi-level rack distribution warehouse through the implementation of class-based storage. Expert Syst Appl 38:2686–2700. https://doi.org/10.1016/j.eswa.2010.08.058
Chow KH, Choy KL, Lee WB (2006) On the design of a real-time knowledge-based system, for managing logistics operations, intelligent systems in accounting. Finance Manage 14:3–25. https://doi.org/10.1002/isaf.274
Custodio L, Machado R (2020) Flexible automated warehouse: a literature review ans innovative framework. Int J Adv Manuf Technol 160:533–558. https://doi.org/10.1007/s00170-019-04588-z
Davarzani H, Norrman A (2015) Toward a relevant agenda for warehousing research: literature review and practitioner’s input. Logist Res 8:1. https://doi.org/10.1007/s12159-014-0120-1
De Koster RBM, Johnson AL, Roy D (2017) Warehouse design and management. Int J Prod Res 55(21):6327–6330. https://doi.org/10.1080/00207543.2017.1371856
De Koster R, Le-Duc T, Roodbergen KJ (2007) Design and control of warehouse order picking: A literature review. Eur J Oper Res 182(2):481–501. https://doi.org/10.1016/j.ejor.2006.07.009
De Koster MBM, Smidts A (2012) Organizing warehouse management. Int J Oper Prod Manage 33(9):1230–1256. https://doi.org/10.1108/ijopm-12-2011-0471
Dianto C, Widiandoko F, Rahmanasari D, Yuniaristanto, Sutopo W (2020) Redesign production layout using dedicated storage method: case study of PT.Solo Grafika Utama, In: IOP conf. series: materials science and engineering, vol 943. https://doi.org/10.1088/1757-899x/943/1/012042
Duc ND, Huu TT, Nananukul N (2020) A dynamic route-planning system based on industry 4.0 technology. Algorithms 13:308. https://doi.org/10.3390/a13120308
Article MathSciNet Google Scholar
Elbert RM, Franzke T, Glock CH, Grosse EH (2017) The effects of human behavior on the efficiency of routing policies in order picking: the case of route deviations. Comput Ind Eng 111:537–551. https://doi.org/10.1016/j.cie.2016.11.033
Ene S, Öztürk N (2012) Storage location assignment and order picking optimization in the automotive industry. Int J Adv Manuf Technol 60:787–797. https://doi.org/10.1007/s00170-011-3593-y
Esmero A, Branzuela QR, Paypa J, Rojo SM (2021) Heuristic comparative assessment of non-conventional warehouse designs. Eng Manage Prod Serv 13:89–103. https://doi.org/10.2478/emj-2021-0007
Frazelle E (2016) World-class warehousing and material handling. McGraw Hill education, New York. https://doi.org/10.1002/9780470172506
Ganbold O, Kundu K, Li H, Zhang W (2020) A simulation-based optimization method for warehouse worker assignment. Algorithms 13:326. https://doi.org/10.3390/a13120326
Gozali L, Marie IA, Natalia, Kustandi GM, Adisurya E (2020) Suggestion of raw material warehouse layout improvement using class-based storage methode (case study of PT. XYZ). In: IOP conf. series: materials science and engineering, 1007. https://doi.org/10.1088/1757-899x/1007/1/012024
Grosse EH, Glock CH, Jaber MY, Neumann P (2015) Incorporating human factors in order picking planning models: framework and research opportunities. Int J Prod Res 53(3):695–717. https://doi.org/10.1080/00207543.2014.919424
Gu J, Goetschalckx M, McGinnins LF (2007) Research on warehouse operation: a comprehensive review. Eur J Oper Res 177:1–21. https://doi.org/10.1016/j.ejor.2006.02.025
Gu J, Goetschalckx M, McGinnins LF (2010) Research on warehouse design and performance evaluation: a comprenhensive review. Eur J Oper Res 203:539–549. https://doi.org/10.1016/j.ejor.2009.07.031
Guerriero F, Musmanno R, Pisacana O, Rende F (2013) A mathematical model for the multi-levels product allocation problem in a warehouse with compatibility constraints. Appl Math Model 37:4385–4398. https://doi.org/10.1016/j.apm.2012.09.015
Guo X, Yu Y, De Koster RBM (2016) Impact of required storage space on storage policy performance in a unit-load warehouse. Int J Prod Res 54(8):2405–2418. https://doi.org/10.1080/00207543.2015.1083624
He R, Li H, Zhang B, Chen M (2020) The multi-level warehouse layout problem with uncertain information: uncertainty theory method. Int J Gen Syst 49(5):497–520. https://doi.org/10.1080/03081079.2020.1778681
Ho Y-C, Liu C-F (2005) A design methodology for converting a regular warehouse into a zone-picking warehouse. J Chin Inst Indus Eng 22(4):332–345. https://doi.org/10.1080/10170660509509303
Hou J-L, Wu Y-J, Yang Y-J (2010) A model for storage arrangement and reallocation for storage management operations. Int J Comput Integr Manuf 23(4):369–390. https://doi.org/10.1080/09511921003642154
Horta M, Coelho F, Relvas S (2016) Layout design modelling for a real world just-in time warehouse. Comput Ind Eng 101:1–9. https://doi.org/10.1016/j.cie.2016.08.013
Irman A, Muharni Y, Yusuf A (2020) Design of warehouse model with dedicated policy to minimize total travel costs: a case study in a construction workshop. In: IOP conf. series: materials science and engineering. https://doi.org/10.1088/1757-899x/909/1/012088
Isler CA, Righetto GM, Morabito R (2016) Optimizing the order picking of a scholar and office supplies warehouse. Int J Adv Manuf Technol 87:2327–2336. https://doi.org/10.1007/s00170-016-8625-1
Jiao Y-L, Xing X-C, Zhang P, Xu L-C, Liu X-R (2018) Multi-objective storage location allocation optimization and simulation analysis of automated warehouse based on multi-population genetic algorithm. Concurr Eng Res Appl 26:367–377. https://doi.org/10.1177/1063293x18796365
Kembro JH, Norrman A, Eriksson E (2018) Adapting warehouse operations and design to omni-channel logistics a literature review and research agenda. Int J Phys Distrib Logist Manag 48(9):890–912. https://doi.org/10.1108/ijpdlm-01-2017-0052
Kim J, Mendez F, Jimenez J (2020) Storage location assignment heuristic based on slot selection and frequent itemset grouping for large distribution centers. IEEE Access. https://doi.org/10.1109/access.2020.3031585
Kofler M, Beham A, Wagner S, Affenzeller M, Reitinger C (2010) Reassigning storage locations in a warehouse to optimize the order picking process. In: 22 nd European modeling and simulation symposium, Morocco. https://doi.org/10.1109/lindi.2011.6031124
Kofler M, Beham A, Wagner S, Affenzeller M (2014) Affinity based slotting in warehouses with dynamic order patterns. Intell Eng Inf 6:123–143. https://doi.org/10.1007/978-3-319-01436-4_7
Kumar S, Mahapatra RP (2021) Design of multi-warehouse inventory model for an optimal replenishment policy using a rain optimization algorithm. Knowl-Based Syst. https://doi.org/10.1016/j.knosys.2021.107406
Kumar S, Narkhede BE, Jain K (2021) Revisiting the warehouse research through an evolutionary lens: a review from 1990 to 2019. Int J Prod Res 59(11):3470–3492. https://doi.org/10.1080/00207543.2020.1867923
Kusrini E, Asmarawati CI, Sari GM, Nurjanah A, Kisanjani A, Wibowo SA, Prakoso I (2018) Warehousing performance improvement using Frazelle model and per group benchmarking: a case study in retail warehouse in Yogyakarata and central Java, MATEX web of Conferences, . https://doi.org/10.1051/matecconf/201815401091
Larco JA, De Koster R, Roodbergen KJ, Dul J (2017) Managing warehouse efficiency and worker discomfort through enhanced storage assignment decisions. Int J Prod Res 55(21):6407–6422. https://doi.org/10.1080/00207543.2016.1165880
Lee M-K, Elsayed EA (2005) Optimization of warehouse storage capacity under a dedicated storage policy. Int J Prod Res 43(9):1785–1805. https://doi.org/10.1080/13528160412331326496
Lee CKM, Yaqiong L, Ho W, Choy KL (2018) Design and application of internet of things-based warehouse management system for smart logistics. Int J Prod Res 56(8):2753–2768. https://doi.org/10.1080/00207543.2017.1394592
Lenoble N, Frein Y, Hammami R (2018) Order batching in an automated warehouse with several vertical lift modules: optimization and experiments with real data. Eur J Oper Res 267:958–976. https://doi.org/10.1080/09537287.2020.1751326
Lersteau C, Nguyen TT, Le TT, Nguyen HN, Shen W (2021) Solving the problem of stacking goods: mathematical model, heuristics and a case study in container stacking in ports. IEEE Access. https://doi.org/10.1109/access.2021.3052945
Li J, Moghaddam M, Nof SY (2016) Dynamic storage assignment with product affinity and ABC classification- a case study. Int J Adv Manuge Technol 84(21):2179–2194. https://doi.org/10.1007/s00170-015-7806-7
Manoharan S, Stilling D, Kabir G, Sarker S (2022) Implementation of linear programming and decision-making model for the improvement of warehouse utilization. Appl Syst Innov 5:33. https://doi.org/10.3390/asi5020033
Manzini R, Gebennini E (2008) Optimization models for the dynamic facility location and allocation problem. Int J Prod Res 46(8):2061–2086. https://doi.org/10.1080/00207540600847418
Manzini R, Accorsi R, Gamberi M, Penazzi S (2015) Modeling class-based storage assignment over life cycle picking patterns. Int J Prod Econ 170:790–800. https://doi.org/10.1016/j.ijpe.2015.06.026
Mehmood E, Anees T (2020) Challenges and solutions for processing real-time big data stream: a systematic literature review. IEEE Access 8:119123–119143. https://doi.org/10.1109/access.2020.3005268
Melinda T, Nazaruddin, Ginting R (2020) Design of warehousing system in order picking process: literature review. In: IOP Conf. Series: materials science and engineering. https://doi.org/10.1088/1757-899x/801/1/012126
Mirabelli G, Pizzuti T, Macchione C, Lagana D (2013) Warehouse layout optimization: a case study based on the adaptation of the multi-layer allocation problem. XX Summer School “Francesco Turco” – Indust Syst Eng . https://doi.org/10.1109/idaacs.2013.6662663
Neamah AF (2021) Adoption of data warehouse in university management: Wasit University case study. J Conf, Ser Phys. https://doi.org/10.1088/1742-6596/1860/1/012027
Önut S, Tuzkaya U, Dogac B (2008) A particle swarm optimization algorithm for the multiple-level warehouse layout design problem. Comput Ind Eng 54:783–799. https://doi.org/10.1016/j.cie.2007.10.012
Parameswari Z, Pujawan IN (2019) Changes in layout and handling method for raw materials to reduce put away and picking time: a plastic packaging manufacturer case study. In: IOP conf. series: materials science and engineering, vol. 598. https://doi.org/10.1088/1757-899x/598/1/012125
Petersen CG, Siu C, Heiser DR (2005) Improving order picking performance utilizing slotting and golden zone storage. Int J Oper Prod Manage 25(10):997–1012. https://doi.org/10.1108/01443570510619491
Phumchusri N, Kitpipit P (2017) Warehouse layout design for an automotive raw material supplier. Eng J. https://doi.org/10.4186/ej.2017.21.7.361
Quintanilla S, Perez A, Ballestin F, Lino P (2015) Heuristic algorithms for a storage location assignment problem in a chaotic warehouse. Eng Optim 47(10):1405–1422. https://doi.org/10.1080/0305215x.2014.969727
Rogers WP, Kahraman MM, Dessureault S (2019) Exploring the value of using data: a case study of continuous improvement through data warehousing. Int J Min Reclam Environ 33(4):286–296. https://doi.org/10.1080/17480930.2017.1405473
Ronzoni C, Ferrara A, Grassi A (2015) A stochastic methodology for the optimal management of infrequent demand spare parts in the automotive industry. IFAC-PapersOnline 48–3:1405–1410. https://doi.org/10.1016/j.ifacol.2015.06.283
Roodbergen KJ, Vis I, Taylor GD Jr (2015) Simultaneous determination of warehouse layout and control policies. Int J Prod Res 53(11):3306–3326. https://doi.org/10.1080/00207543.2014.978029
Roodbergen KJ, Sharp GP, Vis I (2008) Designing the layout structure of manual order picking areas in warehouses. IIE Trans 40(11):1032–1045. https://doi.org/10.1080/07408170802167639
Samuel MP, Srivastava S (2013) Maintenance inventory allocation in presence of heterogeneous part characteristics. Int J Perform Eng 9(5):505–514. https://doi.org/10.1109/qr2mse.2013.6625815
Sencer A, Karaismailoglu A (2022) A simulation and analytic hierarchy process-based decision support system for air cargo warehouse capacity design. Simul Trans Soc Model Simul Int 98:235–255. https://doi.org/10.1177/00375497211029915
Shi Y, Guo X, Yu Y (2018) Dynamic warehouse size planning with demand forecast and contract flexibility. Int J Prod Res 56(3):1313–1325. https://doi.org/10.1080/00207543.2017.1336680
Sicilia J, San-josé LA, Alcaide D, Abdul-Jalbar B (2022) Optimal policy for multi-item systems with stochastic demands, backlogged shortages and limited storage capacity. Appl Math Model 108:236–257. https://doi.org/10.1016/j.apm.2022.03.025
Silva A, Roodbergen KJ, Coelho LC, Darvish M (2022) Estimating optimal ABC zone sizes in manual warehouses. Int J Prod Econ. https://doi.org/10.1016/j.ijpe.2022.108579
Strack G, Pochet Y (2010) An integrated model for warehouse and inventory planning. Eur J Oper Res 204:35–50. https://doi.org/10.1016/j.ejor.2009.09.006
Sudiarta N, Gozali L, Marie IA, Sukania IW (2020) Comparison study about warehouse layout from some paper case studies. In: IOP conf. series: materials science and engineering, vol. 852. https://doi.org/10.1088/1757-899x/852/1/012112
Suloriva I, Zavodska L (2017) State-of-the-art approaches to material transportation, handling and warehousing. Procedia Eng 192:857–862. https://doi.org/10.1016/j.proeng.2017.06.148
Thomas LM, Melle RD (2015) Developing design guidelines for a case-picking warehouse. Int J Prod Econ 170:741–762. https://doi.org/10.1016/j.ijpe.2015.02.011
Tippayawong KY, Sopadang A, Patitad P (2013) Improving warehouse layout design of a chicken slaughterhouse using combined ABC class based and optimized allocation techniques. In: Proceedings of the world congress on engineering, vol 1. https://doi.org/10.46254/an12.20220374
Trab S, Bajic E, Zouinkhi A, Abdelkrim MN, Chekir H, Ltaief RH (2015) Product allocation planning with safety compatibility constraints in IoT-based warehouse. Procedia Comput Sci 73:290–297. https://doi.org/10.1016/j.procs.2015.12.033
Trindade MAM, Sousa PSA, Moreira MRA (2021) Product allocation planning with handling constraints: a case study analysis. Int J Manag Sci Eng Manage 16(3):175–183. https://doi.org/10.1080/17509653.2021.1907812
Van Dinter R, Tekinerdogana B, Catal C (2021) Automation of systematic literature reviews: a systematic literature review. Inf Softw Technol. https://doi.org/10.1016/j.infsof.2021.106589
Van Gils T, Caris A, Ramaekers K, Braekers K, de Koster RBM (2019) Designing efficient order picking systems: the effect of real-life features on the relationship among planning problems. Transp Res Part E 125:47–73. https://doi.org/10.1016/j.tre.2019.02.010
Van Gils T, Ramaekers K, Braekers K, Depaire B, Caris A (2018) Increasing order picking efficiency by integrating storage, batching, zone picking, and routing policy decisions. Int J Prod Econ 197:243–261. https://doi.org/10.1016/j.ijpe.2017.11.021
Van Gils T, Ramaekers K, Caris A, De Koster RBM (2018) Designing efficient order picking systemns by combining planning problems: state-of-the-art classification and review. Eur J Oper Res 267:1–15. https://doi.org/10.1016/j.ejor.2017.09.002
Venkitasubramony R, Adil GK (2015) Layout optimization of a three-dimensional order picking warehouse. IFAC-PapersOnLine 48–3:1155–1160. https://doi.org/10.1016/j.ifacol.2015.06.240
Venkitasubramony R, Adil GK (2017) Design of an order-picking warehouse factoring vertical travel and space sharing. Int J Adv Manuf Technol 91:1921–1934. https://doi.org/10.1007/s00170-016-9879-3
Venkitasubramony R, Adil GK (2019) Designing a block stacked warehouse for dynamic and stochastic product flow: a scenario-based robust approach. Int J Prod Res 57(5):1345–1365. https://doi.org/10.1080/00207543.2018.1472402
Venkitasubramony R, Adil GK (2021) Modeling the effect of imperfect staggering in product inflow using queuing theory: revisiting block stacking layout. Flex Serv Manuf J 33:689–716. https://doi.org/10.1007/s10696-020-09390-8
Vieira A, Dias LS, Pereira G, Oliveira JA, Carvalho MS, Martins P (2015) Using Simio to automatically create 3D warehouse and compare different storage strategies. FME Trans 43:335–343. https://doi.org/10.5937/fmet1504335v
Vrysagotis V, Kontis PA (2011) warehouse layout problems: types of problems and solution algorithms. J Comput Model 1(1):131–152
Google Scholar
Wan Y, Liu Y (2022) Integrating optimized fishbone warehouse layout, storage location assignment and picker routing. Int J Comput Sci 49:3. https://doi.org/10.1061/9780784413753.154
Wang M, Zhang R-Q (2019) A dynamic programming approach for storage location assignment planning problem. Procedia CIRP 83:513–516. https://doi.org/10.1016/j.procir.2019.04.113
Wei N-C, Chang C-F, Lin S-K (2016) An innovative way for warehouse storage allocation. Int J Org Innov. https://doi.org/10.1109/wsc.2016.7822265
Wu W, de Koster RBM, Yu Y (2020) Forward-reserve storage strategies with order picking: When do they pay off? IISE Trans 52(9):961–976. https://doi.org/10.2139/ssrn.3243879
Xu Z, Ming XG, Zhou J, Song W, He L, Li M (2013) Management optimization based on dynamic SKU for RFID-enabled warehouse management in the steel supply chain. Int J Prod Res 51(10):2981–2996. https://doi.org/10.1080/00207543.2012.751513
Xu X, Zhao X, Zou B, Li M (2019) Optimal dimensions for multi-deep storage systems under class-based storage policies. Cluster Comput. https://doi.org/10.1007/s10586-018-2873-9
Yang L, Feng Y (2006) Fuzzy multi-level warehouse lyout problem: new model and algorithm. J Syst Sci Syst Eng 15(4):493–503. https://doi.org/10.1007/s11518-006-5017-3
Yang C-L, Nguyen TPQ (2015) Constrained clustering method for class-based storage location assignment in warehouse. Ìnd Manage Data Syst 166(4):667–689. https://doi.org/10.1108/imds-09-2015-0361
Yang M-F, Shih P-H, Pan JC-H, Li M-C (2022) The optimal layout design for minimizing operating costs in a picker-to-part warehousing system. Int J Adv Manuf Technol 118:2523–2537. https://doi.org/10.1007/s00170-021-08026-x
Yang D, Wu Y, Ma W (2021) Optimization of storage location assignment in automated warehouse. Microprocess Microsyst. https://doi.org/10.1016/j.micpro.2020.103356
Yener F, Yazgan HR (2019) Optimal warehouse design: literature review and case study application. Comput Ind Eng 129:1–13. https://doi.org/10.1016/j.cie.2019.01.006
Yu Y, de Koster RBM, Guo X (2015) Class-based storage with a finite number of items: using more classes is not always better. Prod Oper Manag 24(8):1235–1247. https://doi.org/10.1111/poms.12334
Yuan R, Cezik T, Graves SC (2018) Stowage decisions in multi-zone storage systems. Int J Prod Res 56:333–343. https://doi.org/10.2139/ssrn.2990432
Zaerpour N, Volbeda R, Gharehgozli A (2019) Automated or manual storage systems: Do throughout and storage capacity matter? INFOR: Inf Syst Oper Res 57(1):99–120. https://doi.org/10.1080/03155986.2018.1532765
Zhang G, Xiaoting S, Fawzat A, Yiqin Y, Tatsushi N (2021) Integrated production planning and warehouse storage assignment problem: An IoT assisted case. Int J Prod Econ. https://doi.org/10.1016/j.ijpe.2021.108058
Zhao Z, Zhang M, Yang C, Fang J, Huang GQ (2018) Distributed and collaborative proactive tandem location tracking of vehicle products for warehouse operations. Comput Ind Eng 125:637–648. https://doi.org/10.1016/j.cie.2018.05.005
Zhou S, Gong Y, De Koster R (2016) Designing self-storage warehouse with customer choice. Int J Prod Res 54(10):3080–3104. https://doi.org/10.1080/00207543.2016.1158880
Download references
All of the authors declare that no funds, grants, or other support were received during the preparation of this manuscript.
Author information
Authors and affiliations.
Département de Génie Mécanique, Faculté des Sciences et de Génie, Université Laval, Pavillon Adrien-Pouliot 1065, Av. de la Médecine, Local 1504, Québec, Canada
Pierre-William Albert, Mikael Rönnqvist & Nadia Lehoux
You can also search for this author in PubMed Google Scholar
Contributions
PWA contributed to the study conception and design. Material preparation, data collection and analysis were performed by PWA. The first draft of the manuscript was written by PWA. NL and MR commented on previous versions of the manuscript. All authors read and approved the final manuscript (PWA, NL and MR).
Corresponding author
Correspondence to Pierre-William Albert .
Ethics declarations
Conflicts of interest.
No authors have potential conflicts of interest in regard to this paper.
Consent for publication
Not applicable.
Informed consent
Human participants and/or animals, additional information, publisher's note.
Springer Nature remains neutral with regard to jurisdictional claims in published maps and institutional affiliations.
The following tables (Table 3 , 4 , 5 and 6 ) describe the main findings and method in relation to the previous questions. One can find the evaluated articles with their most important information.
Rights and permissions
Open Access This article is licensed under a Creative Commons Attribution 4.0 International License, which permits use, sharing, adaptation, distribution and reproduction in any medium or format, as long as you give appropriate credit to the original author(s) and the source, provide a link to the Creative Commons licence, and indicate if changes were made. The images or other third party material in this article are included in the article's Creative Commons licence, unless indicated otherwise in a credit line to the material. If material is not included in the article's Creative Commons licence and your intended use is not permitted by statutory regulation or exceeds the permitted use, you will need to obtain permission directly from the copyright holder. To view a copy of this licence, visit http://creativecommons.org/licenses/by/4.0/ .
Reprints and permissions
About this article
Albert, PW., Rönnqvist, M. & Lehoux, N. Trends and new practical applications for warehouse allocation and layout design: a literature review. SN Appl. Sci. 5 , 378 (2023). https://doi.org/10.1007/s42452-023-05608-0
Download citation
Received : 18 June 2023
Accepted : 15 November 2023
Published : 30 November 2023
DOI : https://doi.org/10.1007/s42452-023-05608-0
Share this article
Anyone you share the following link with will be able to read this content:
Sorry, a shareable link is not currently available for this article.
Provided by the Springer Nature SharedIt content-sharing initiative
- Warehouse allocation methods
- Warehouse layout design methods
- Literature review
- Non-standard shelf layout
- Non-standard spare parts
Advertisement
- Find a journal
- Publish with us
- Track your research
warehouse operations Recently Published Documents
Total documents.
- Latest Documents
- Most Cited Documents
- Contributed Authors
- Related Sources
- Related Keywords
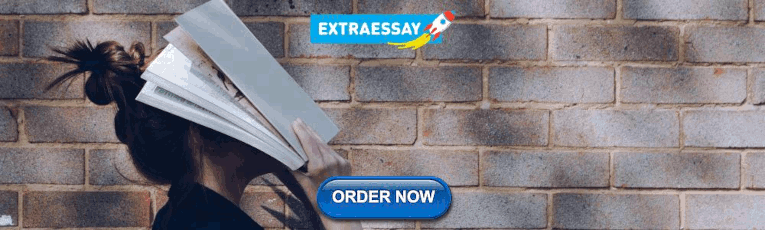
A literature review of smart warehouse operations management
AbstractE-commerce, new retail, and other changes have highlighted the requirement of high efficiency and accuracy in the logistics service. As an important section in logistics and supply chain management, warehouses need to respond positively to the increasing requirement. The “smart warehouse” system, which is equipped with emerging warehousing technologies, is increasingly attracting the attention of industry and technology giants as an efficient solution for the future of warehouse development. This study provides a holistic view of operations management problems within the context of smart warehouses. We provide a framework to review smart warehouse operations management based on the characteristics of smart warehouses, including the perspectives of information interconnection, equipment automation, process integration, and environmental sustainability. A comprehensive review of relevant literature is then carried out based on the framework with four perspectives. This study could provide future research directions on smart warehouses for academia and industry practitioners.
Towards an increased understanding of learning: a case study of a collaborative relationship between a retailer and a logistics service provider
PurposeThis study aims to explain how learning occurs in collaborative retailer–logistics service provider (LSP) relationships. The research is guided by two research questions, addressing absorptive and desorptive capacities and the interaction between these capacities.Design/methodology/approachThe study is based on a case study of a Swedish, collaborative retailer–LSP dyad. The empirical data are structured around five specific learning situations within the retailer–LSP dyad.FindingsThe findings provide an explanation for how learning occurs within a collaborative retailer–LSP relationship based on subprocesses of absorptive and desorptive capacities. The interaction between these processes is found to rely on two types of support: one-directional and bidirectional. The findings also indicate positive outcomes of learning, such as improved cost efficiencies in warehouse operations, better customer services and improved long-term strategic planning.Practical implicationsThis study shows how retailers and LSPs can learn from each other and together create an improved logistics system for end customers.Originality/valueThis research takes into account absorptive and desorptive capacities in a collaborative retailer–LSP relationship. This study enhances the understanding of inter-organisational learning processes in a retail logistics context.
WAREHOUSE OPERATIONS OF PASSENGER SERVICE CAR DEPOT WITH LOGISTICS ELEMENTS
The article discusses the issues of rational organization of the warehouse process of storing inventory, the sequential implementation of warehouse operations, the improvement of labor organization and technological solutions, the effective use of various equipment involved in the performance of technological operations at the warehouse of the carriage depot of the Joint Stock Company «Uzpasstrans». The issues of creating a database for storing goods, an effective system for organizing their account are considered. The ER-diagram of the database of warehouse inventory of spare parts and components has been developed. The functions and procedures for working with database data on components and spare parts located in the warehouse of the carriage depot have been determined.
A Survey of the Literature on Order-Picking Systems by Combining Planning Problems
Companies have been trying continuously to reduce their logistics costs in the current competitive markets. Warehouses are important components of the logistics systems and they must be managed effectively and efficiently to reduce the production cost as well as maintain customer satisfaction. Order-picking is the core of warehouse operations and an order-picking system (OPS) is essential to meet customer needs and orders. Failure to perform the OPS process properly results in high costs and customer dissatisfaction. This research aims to investigate the state of the art in the adoption of OPS and provide a broad systemic analysis on main operating strategies such as simultaneous consideration of order assignment, batching, sequencing, tardiness, and routing need. This study reviews 92 articles, classifies combinations of tactical and operational OPS problems, and provides guidelines on how warehouse managers can benefit from combining planning problems, in order to design efficient OPS and improve customer service. Combining multiple order-picking planning problems results in substantial efficiency benefits, which are required to face new market developments.
Determination of Performance Indicators for Warehouse Evaluation: A Case of Medium Sized Warehouses in Nakuru Town
Evaluation of warehouse processes is required for decision making purposes and the improvement of warehouse operations. To evaluate warehouse processes, it is essential to identify key indicators in the warehouse operations. This research was intended to identify the significant indicators of warehouse performance that would support management decision making on the improvement of warehouse operations. In this research 20 indicators were identified in four warehouse activities based on Frazelle model. The most important indicators in each warehouse were then determined. The study was conducted through a descriptive design using a survey of 3 medium warehouses in Nakuru. The study employed purposive sampling to select the sample and sample elements. The sample size of 10 warehouse management officers were used in the study. Structured questionnaire was adopted as data collection instrument. The research findings indicated that most important performance indicators for receiving is productivity, for storage is space utilization, for order picking is cycle time and for shipping is productivity. Process improvement steps were proposed based on benchmarking among warehouses handling similar goods. It was noted that different types of warehouses require different indicators. The study concluded that measurement of warehouse processes is important and enables operations improvements and cost reduction. Keywords: Warehouse procedures, warehouse performance, warehouse improvement, medium sized warehouses, Nakuru town, Kenya
Risk Assessment for the Use of Drones in Warehouse Operations in the First Phase of Introducing the Service to the Market
Services, unlike products, are intangible, and their production and consumption take place simultaneously. The latter feature plays a crucial role in mitigating the identified risk. This article presents the new approach to risk assessment, which considers the first phase of introducing the service to the market and the specificity of UAV systems in warehouse operations. The fuzzy logic concept was used in the risk analysis model. The described risk assessment method was developed based on a literature review, historical data of a service company, observations of development team members, and the knowledge and experience of experts’ teams. Thanks to this, the proposed approach considers the current knowledge in studies and practical experiences related to the implementation of drones in warehouse operations. The proposed methodology was verified on the example of the selected service for drones in the magazine inventory. The conducted risk analysis allowed us to identify ten scenarios of adverse events registered in the drone service in warehouse operations. Thanks to the proposed classification of events, priorities were assigned to activities requiring risk mitigation. The proposed method is universal. It can be implemented to analyze logistics services and support the decision-making process in the first service life phase.
Design and application of data analytics in an internet-of-things enabled warehouse
Purpose While several warehouses are now technologically equipped and smart, the implementation of real-time analytics in warehouse operations is scarcely reported in the literature. This study aims to develop a practical system for real-time analytics of process monitoring in an internet-of-things (IoT)-enabled smart warehouse environment. Design/methodology/approach A modified system development research process was used to carry out this research. A prototype system was developed that mimicked a case company’s actual warehouse operations in Indonesia’s manufacturing companies. The proposed system relied heavily on the utilization of IoT technologies, wireless internet connection and web services to keep track of the product movement to provide real-time access to critical warehousing activities, helping make better, faster and more informed decisions. Findings The proposed system in the presented case company increased real-time warehousing processes visibility for stakeholders at different management levels in their most convenient ways by developing visual representation to display crucial information. The numerical or textual data were converted into graphics for ease of understanding for stakeholders, including field operators. The key elements for the feasible implementation of the proposed model in an industrial area were discussed. They are strategic-level components, IoT-enabled warehouse environments, customized middleware settings, real-time processing software and visual dashboard configuration. Research limitations/implications While this study shows a prototype-based implementation of actual warehouse operations in one of Indonesia’s manufacturing companies, the architectural requirements are applicable and extensible by other companies. In this sense, the research offers significant economic advantages by using customized middleware to avoid unnecessary waste brought by the off-the-shelves generic middleware, which is not entirely suitable for system development. Originality/value This research’s finding contributes to filling the gap in the limited body of knowledge of real-time analytics implementation in warehousing operations. This should encourage other researchers to enhance and develop the devised elements to enrich smart warehousing’s theoretical knowledge. Besides, the successful proof-of-concept implementation reported in this research would allow other companies to gain valuable insights and experiences.
An Overview of Warehouse Operations for Cold Chain
An analytical design & optimization approach to enhance warehouse operations, genetic algorithm solution for transfer robot operation.
The proposed article presents the research of decision-making processes for control system of transport robot, acting inside warehouse. The analysis shows the growing importance of warehouse systems for flexible integrated system. Such warehouse operations, like loading/uploading pallets and sending goods to distribution center can be fully automated by applying robots and some examples are known. Simultaneously, the wide introduction of transport robots with extended possibilities is still limited. Application of intelligent robot can significantly improve properties of warehouse systems, making them closer to Industry 4.0 standards. The functioning of a transport robot can be described as a Travelling Salesmen Problem with numerous solutions that must be effectively limited. Genetic algorithms are proposed as a basis for path generation. The algorithms and software are implemented to model warehouse transfer robots using an Arduino-robot. The results of testing show the effectiveness of the developed algorithms, software and hardware system.
Export Citation Format
Share document.
The transformation from manual to smart warehousing: an exploratory study with Swedish retailers
The International Journal of Logistics Management
ISSN : 0957-4093
Article publication date: 22 June 2022
Issue publication date: 19 December 2022
To meet customers' expectations on shorter lead times, high product availability, flexibility, and variation in delivery and return options, retailers have turned their attention to warehousing and are making big investments in technology. Currently, technology providers are pushing for smart warehousing, a new and under-researched phenomenon. This study aims to conceptualize the term and examine pathways toward implementing smart warehousing.
Design/methodology/approach
An exploratory survey was administered to 50 leading Swedish retailers in varying segments. A two-tailed t -test for equality of means was used to detect significant differences between current and future states.
The study found that future smart warehouses will be automated, autonomous, digital, and connected, but that retailers will follow different paths along this journey, driven by contextual trends, e.g. sales growth, wider product assortment, shorter lead-time offerings, and integration of brick-and-mortar and online stores. Interestingly, the study revealed that many of the retailers that aim to create smart warehouses in five years are not the retailers with the most developed technology today.
Research limitations/implications
The paper operationalizes smart warehousing in two dimensions: degree of automation and degree of digitalization and connectivity of information platforms. Based on the findings, 16 theoretical propositions are put forth that, based on contextual factors, explain different pathways for retailers to implement smart warehousing.
Practical implications
The empirical insights and theoretical discussions provide practically useful guidance, including outlined trends, for selecting and benchmarking automation and complementary technologies in warehouse operations.
Originality/value
This paper conceptualizes and operationalizes smart warehousing – an original approach. It is also one of the first to investigate the technological transformation in retail warehousing empirically, explaining how and why retailers choose different pathways toward smart warehousing.
- Transformation
Kembro, J. and Norrman, A. (2022), "The transformation from manual to smart warehousing: an exploratory study with Swedish retailers", The International Journal of Logistics Management , Vol. 33 No. 5, pp. 107-135. https://doi.org/10.1108/IJLM-11-2021-0525
Emerald Publishing Limited
Copyright © 2022, Joakim Kembro and Andreas Norrman
Published by Emerald Publishing Limited. This article is published under the Creative Commons Attribution (CC BY 4.0) licence. Anyone may reproduce, distribute, translate and create derivative works of this article (for both commercial and non-commercial purposes), subject to full attribution to the original publication and authors. The full terms of this licence may be seen at http://creativecommons.org/licences/by/4.0/legalcode
1. Introduction
The retail industry has been undergoing a digital transformation coupled with customers' expectations of shorter lead times (i.e. demanding same-day delivery), high product availability, flexibility when and where to shop, and varying delivery (e.g. click-and-collect, pick-up points, home delivery) and return options ( Galipoglu et al. , 2018 ; Tokar et al. , 2020 ). To meet these demands, the logistics network, particularly the warehouse, has been highlighted as a critical component ( Kembro et al. , 2018 ). The warehouse, previously viewed as a “necessary evil” in the supply chain, now plays a key role in fulfilling customer orders and significantly influences both logistics costs and service levels ( Faber et al. , 2018 ). As Rouwenhorst et al. (2000 , p. 515) put it: “[T]he efficiency and effectiveness in any distribution network … is largely determined by the operation of the nodes in such a network, i.e. the warehouses.”
To improve warehouse operations, online global giants, e.g. Alibaba and Amazon have made large investments in automated material-handling technology ( Alibaba Cloud, 2019 ; Amazon, 2020 ). With increased competition and more mature and varied technologies, the automation trend also has spread among retailers and logistics service providers worldwide ( Reiser, 2020 ; MH&L, 2020 ). An important driver is that the latest generation of automation technologies offers flexibility to handle different types of products and adjust to demand variations, enabling effective and efficient storage, handling, and sorting of large product flows ( Azadeh et al. , 2019 ; Kembro et al. , 2022 ). To improve warehouse operations further, retailers couple automated material handling with digitalization and connectivity of information platforms. Examples of new technologies that are relevant for warehouse operations include artificial intelligence (AI), Internet of things (IoT), cyber-physical system (CPS), big data, 5G, and intelligent video analysis (IVA) ( Kembro et al. , 2017 ; Kamali, 2019 ; Taboada and Shee, 2021 ; Winkelhaus and Grosse, 2020 ; Chung, 2021 ).
This combination of technologies sometimes is termed smart warehousing ( Mahroof, 2019 ; Kamali, 2019 ), a term that is gaining increased attention, often in connection with Industry 4.0, Logistics 4.0, and IoT ( Lee et al. , 2018 ; Winkelhaus and Grosse, 2020 ; van Geest et al. , 2021 ; Issaoiu et al. , 2021 ). It is used in tech blogs, industry reports, conference proceedings, and scientific journal papers. However, extant smart-warehousing research is fragmented when it comes to its substance (see, e.g. Bolu and Korcak, 2019 ; Chung, 2021 ; Zhang et al. , 2021 ). Previous research has instead listed or developed sub-applications of smart technologies that could be used. This gap is important to address to bring research together, facilitate joint discussions, and enable analysis of patterns on a more holistic level, instead of focusing only on specific applications of certain technologies. Another important research gap is the lack of clarity on how to implement smart warehousing ( Azadeh et al. , 2019 ; Winkelhaus and Grosse, 2020 ; van Geest et al. , 2021 ). During the journey toward smart warehousing, companies operate in different contexts and, therefore, are likely to follow different paths ( Kembro and Norrman, 2021 ). However, extant research does not provide any guidance or explanation for different pathways on how and why retailers calibrate their timing, technology, and focus to suit certain operations during their transformation from manual to smart warehousing.
To address these gaps, this study aims to conceptualize smart warehousing and explain pathways on how to implement it. By administering an empirical exploratory survey to 50 Swedish retailers, we make several contributions. Theoretically, we contribute by operationalizing smart warehousing in two dimensions – degree of automation and degree of digitalization and connectivity of information platforms – and by conceptualizing future smart warehouses as automated, autonomous, digital, and connected. We also contribute by identifying different pathways to smart warehousing and explaining how retailers calibrate their timing, technology, and focus to suit certain operations using 16 theoretical propositions. For managers, our study outlines pioneering practices that can help other retailers understand critical issues earlier, as well as how to address them. Our findings provide insights into technologies that are expected to grow in use and criticality to support material handling in single warehouses and in increasingly complex and decentralized networks.
The paper is structured as follows. In Section 2 , we present a theoretical background on warehouse operations in retailing and technology's role in warehouse management. In Section 3 , we describe our empirical study's design. In Section 4 , the findings are presented and analyzed, describing how companies intend to transform from manual to smart warehousing. In Section 5 , based on our findings, we submit theoretical propositions, then conceptualize smart warehousing and explain pathways. Finally, in Section 6 , we outline conclusions and suggestions for future research.
2. Theoretical background
2.1 warehouse operations in retailing.
Warehouses represent “the points in the supply chain where [the] product pauses, however briefly, and is touched” ( Bartholdi and Hackman, 2016 , p. 3). In the retail context, warehouses primarily are used to consolidate and store a range of products to reduce transportation costs and lead times. Different types of retail warehouses include the distribution center (DC), online fulfilment center (OFC), and micro-fulfilment center (MFC). Warehouse operations include receiving, put-away, storage, picking, sorting, packing, and shipping. Along with managing increases in online orders, warehouses also might handle returns and cross-docking, in which goods move directly from receiving to shipping ( Kembro et al. , 2018 ).
In a warehouse, arriving products are checked for quality, registered, and potentially re-packed before being put away in assigned storage locations ( Frazelle, 2016 ). Storage, which includes a reserve and picking area, typically is divided into zones, depending on stock-keeping unit (SKU) characteristics (e.g. size and temperature requirements) and/or order characteristics (e.g. online orders vs store replenishment) ( Eriksson et al. , 2019 ). SKUs can be dedicated or randomly assigned to a location. A common approach is to combine the two (also termed class-based storage ), i.e. SKUs are placed randomly within a dedicated area, thereby reducing travel while avoiding congestion ( Gu et al. , 2007 ). Picking comprises most of the operational cost and has been by far the most-researched warehousing topic. Picking efficiency can be improved by putting the fastest-moving products in the most convenient locations ( Gu et al. , 2007 ) and by selecting appropriate picking methods. The four most common picking methods are single, batch, zone, and wave ( Bartholdi and Hackman, 2016 ). Kembro and Norrman (2019) described the SKU extraction method as one “which implies that a large number of customer orders are accumulated, and the picker only goes to one or a few storage locations and (manually) takes out hundreds of the same SKU to a trolley or pallet. These products are then moved to a sorting system” (p. 521). Eventually, orders are packed and shipped. If an order involves multiple flows (e.g. wave picking or cross-docking), the first step is to sort and merge the various order lines per customer and destination. SKUs are registered thereafter for departure and positioned based on the designated gate and time window ( Bartholdi and Hackman, 2016 ).
To improve warehouse operations' efficacy and efficiency, several configuration aspects are considered, including physical layout (e.g. placement of docks, aisle configuration, and lane depth) and storage and handling equipment (e.g. racks and forklifts for put-away and picking) ( Kembro et al. , 2018 ). Important resources also include labor management (e.g. scheduling, rotation, and shifts) ( De Leeuw and Wiers, 2015 ), information systems (e.g. warehouse management systems [WMS] and warehouse-control systems [WCS]) ( Kembro and Norrman, 2019 ), and automation technologies, e.g. conveyors and robots ( Baker and Halim, 2007 ; Azadeh et al. , 2019 ). These configurations' goal is to improve utilization of resources and capacities (labor, space, and equipment), increase throughput, reduce material-handling time, and increase operations and design flexibility ( Kembro and Norrman, 2019 ). However, which warehouse configuration to use depends on a range of contextual factors ( Hassan et al. , 2015 ; Faber et al. , 2018 ; Eriksson et al. , 2019 ; Kembro and Norrman, 2021 ), e.g. order-fulfillment time requirements, assortment range, number of transactions, and sizes of goods.
2.2 Technology's role in warehouse management
Just a decade ago, warehousing worldwide predominantly were manual operations, but increasing competition and expectations on shorter lead times (demand for same-day delivery), high product availability, and a variation of delivery and return options have necessitated improvements to transform from manual to smart warehousing (e.g. Chung, 2021 ; Kembro and Norrman, 2021 ; Zhang et al. , 2021 ). As described below, this transformation mainly involves technology, e.g. automated material handling and information systems.
2.2.1 Automated material handling
Warehouse automation has been around for many years. Defined as “the direct control of handling equipment producing movement and storage of loads without the need for operators or drivers” ( Rowley, 2000 , p. 38), it includes equipment, e.g. automated storage and retrieval systems (AS/RS), automated guided vehicles (AGVs), and conveyorized sorting systems. Positive aspects of warehouse automation include improved space utilization and service, as well as lower operating costs and reduced picking errors. However, it requires significant investments, and more static automation technologies may involve flexibility risks, e.g. during extreme demand peaks ( Baker and Halim, 2007 ; Kembro and Norrman, 2020 ). Therefore, these investments require careful analysis, considerable scale, and a long-term vision ( De Koster, 2018 ).
In recent years, retailers have increased the pace of implementing automation in warehouse operations, and various new technologies are being tested to make material handling more effective and efficient ( Kembro and Norrman, 2020 ). Azadeh et al. (2019) conducted a comprehensive review of recent developments in various automation technologies in warehousing, identifying the following categories: Crane/Automated Forklift (e.g. AS/RS, push-back rack); Carousels and Dispensers (e.g. horizontal carousel, A-frame); Shuttle (aisle-based, e.g. horizontal AVS/R system; grid-based, e.g. robotic compact storage and retrieval systems); and AGVs (e.g. movable racks in robotic mobile fulfillment systems). One interesting development is the increased flexibility in automation technologies, with different solutions available for different SKU characteristics, while it is also less difficult to adjust to demand variations. It is also relevant to consider whether humans will work in warehouses in the future and what their role will be. Azadeh et al. (2019 , p. 940) noted that: “Human picking in collaboration with AGVs is one of the most recent technologies that is becoming popular in practice because of its simplicity and flexibility.”
2.2.2 Digitalization and connectivity of information platforms
Simultaneously, retailers develop information systems to manage and control their warehouse operations. As Kembro and Norrman (2019) asserted, the three most common systems include enterprise resource planning (ERP) systems; WMS; and WCS. ERP connects information across a range of organizational functions, e.g. sourcing, inventory management, production planning, and financial matters. WMS is used for shorter-term planning and control of resources and order fulfilment for various warehouse operations. WCS is used to control automated systems, e.g. robots and advanced sorting algorithms. A related system is the warehouse execution system (WES), which synchronizes various automation technologies' operation with workers and could be viewed as an integration or combination involving WMS and WCS. Several other systems besides these also exist, e.g. for labelling, administering, and transport administration system (TAS). With the increasing importance of sharing real-time inventory and order information, both internally and externally, it becomes vital for all systems within each warehouse and across the logistics network to be integrated. For this purpose, Kembro and Norrman (2019) noted that retailers increasingly use a distributed order management (DOM) system.
2.3 The transformation toward smart warehousing
Smart warehousing is a term that is gaining increased attention, often in connection with Industry 4.0, Logistics 4.0, and IoT (see literature reviews in Winkelhaus and Grosse, 2020 ; Chung, 2021 ; Issaoui et al. , 2021 ). The term has not been defined precisely, but generally has been described as something more than using automated material handling and traditional information and communication technology (ICT), e.g. ERP, WMS, and WCS. Chung (2021 , p. 1) defined smart technologies as “applications of artificial intelligence and data science technologies, e.g. machine learning, big data, to create cognitive awareness (autonomous) of an object with the support of information and communication technologies, e.g. IoT and blockchain.” Previous research often either has listed different smart technology applications ( Winkelhaus and Grosse, 2020 ; Chung, 2021 ; Issaoui et al. , 2021 ), developed focused algorithms (e.g. Jiang et al. , 2021 ), proposed IoT-based WMS ( Lee et al. , 2018 ; Aamer and Sahara, 2021 ), or referred to architectures for information systems related to data collection and administration ( Jabbar et al. , 2018 ; van Geest et al. , 2021 ).
Several studies have used smart warehousing to describe a warehouse that includes a combination of automated material handling and AI. For example, Bolu and Korcak (2019) discussed smart warehouses in terms of automation technologies that make warehouse robots and systems smarter, including autonomous robots that perform most activities in a warehouse, as well as information systems that keep track of every object's movement in the warehouse. Mahroof (2019) noted that these robots carry out most warehouse work, have Wi-Fi capabilities, and use self-charging batteries and laser-detection technology. Mahroof (pp. 178–179) concluded that “the new automation age is here, whereby industrial robots and computers are being used beyond their traditional scope of performing highly accurate repetitive tasks, routine physical work tasks, through to more complex tasks that require cognitive capabilities, e.g. making tacit judgments, sensing emotion, and driving processes which previously seemed impossible.” Along the same lines, Zhang et al. (2021) investigated Alibaba's smart warehouses, which use a set of AI applications, along with collectively working robots and other related human and organizational resources, to improve key business processes' efficiency and efficacy. The researchers noted that while humans focus on higher-value tasks that require creativity, robots and AI are used to execute repetitive, time-consuming, and/or hazardous tasks. With WMS, WCS, and AGVs as foundational systems, AI uses algorithms as building blocks to automate, augment, or transform processes. Examples of algorithms include sales forecasting, location recommendation, 3D packing, order wave combination, route planning, robot scheduling, and robotic motion control.
Extending this perspective, Kamali (2019) described the smart warehouse as using a mix of technologies, including, e.g. robotics systems, IoT, radio-frequency identification (RFID), enterprise asset management (EAM), and digital twins with 3-D representations of objects and their components, e.g. sensors. Other relevant technologies include wide area network (WAN), cloud computing, automatic identification technology, CPS, and blockchain. Kamali argued that AI allows for gaining unprecedented insight into products, components, and even materials' life cycles, noting that smart warehouses make activities more efficient, save on labor costs, reduce errors, and generate higher productivity. Thus, the automation and AI focus is complemented (e.g. Lee et al. , 2018 ; Kamali, 2019 ; Issaoui et al. , 2021 ) by adding (inter)connectivity in terms of IoT and wide area networks/cloud for information management. Some studies have developed frameworks for WMS in the IoT context ( Lee et al. , 2018 ; Aamer and Sahara, 2021 ) or reference architectures ( Jabbar et al. , 2018 ; van Geest et al. , 2021 ). Another relevant technology is IVA, which enables advanced analytics and decision making in real time in the warehouse ( Kembro et al. , 2017 ). Using a mix of technologies is supported by Attaran (2020) , who pointed out that AI and robotics, cloud computing, 3D printing, advanced analytics, blockchain, AR, RFID, IoT, and cloud technology drive digital trends and elicit change in supply chain management. Similar reasoning can be found across tech blogs and suppliers. For example, Flytware (2021) stated: “Smart warehousing is essentially a set of interconnected and/or automated technologies for streamlining warehousing operations in an efficient manner.” The connectivity dimension links the warehouse as part of a digital network, pointing to the growing importance of Industry 4.0, digitalization, and a unified platform for multiple device connectivity in real time, which can be implemented through 5G networks ( Taboada and Shee, 2021 ). Another aspect sometimes stressed is robot autonomy ( Winkelhaus and Grosse, 2020 ; Chung, 2021 ; Jiang et al. , 2021 ).
To sum up, smart warehousing is receiving increased attention in both practice and academia. but extant research is fragmented, with no consensus reached on a definition of smart warehousing . Previous research instead has listed or developed sub-applications of smart technologies that could be used. This literature gap is important to address to facilitate discourse and create a common understanding of what smart warehousing is. As a starting point, our literature review revealed two smart warehousing dimensions: the development of automated material handling and development of digitalization and connectivity of information platforms. The review also revealed that pathways toward implementing smart warehousing are missing. Previous studies emphasized contextual factors' importance, suggesting that companies may follow different paths. However, extant research has not provided any guidance or explanation as to how and why retailers calibrate their timing, technology, and focus to suit certain operations during their transformation from manual to smart warehouse management.
3. Research design and method
This study aimed to grasp and generate practically relevant insights and theoretical propositions about a new phenomenon – smart warehousing – on which current knowledge is scarce concerning both the current situation and future developments. By illuminating this area for consideration, we seek to influence the definition of its problem domain, leading to contributions of the “theoretical pre-science” type ( Corley and Gioia, 2011 ).
For this, we used an exploratory survey (distinguished from explanatory and descriptive research). It is useful for becoming more familiar with a topic and identifying new possibilities and dimensions of interest ( Malhotra and Grover, 1998 ), as well as identifying interesting patterns during the early stages of the research-maturity cycle ( Malhotra and Grover, 1998 ; Edmondson and McManus, 2007 ), rather than testing a theory-driven hypothesis. The study is theory-elaborative and tries to adapt theory to contemporary practices and challenges ( Corley and Gioia, 2011 ; Ketokivi and Choi, 2014 ). Therefore, conducting an exploratory survey is appropriate for revealing trends, interesting patterns, and facets of the phenomenon with the purpose of developing propositions for future research studies. In this way, exploratory surveys (conducted with experts in the field) are similar to case studies and other qualitative methods, which also are useful for discovering new facets of phenomena under study ( Forza, 2002 ). Exploratory surveys have been used in supply chain and operations management research before, e.g. related to information systems: Themistocleous et al. (2001) examined problems related to ERP systems, and Kembro and Norrman (2019) investigated omnichannel logistics issues. These studies applied more open-ended research questions in combination with closed-ended survey questions using ranking or Likert scales.
3.1 Data collection
This study is the first part of a larger study, the Swedish Retail Logistics panel, in which Swedish retail companies (pure-play online retailers, henceforth referred to as e-tailers , as well as retailers with both online and brick-and-mortar stores) have been invited to participate in a series of exploratory surveys. The retailers were identified through addresses from different databases and listings of leading Swedish retailers (e.g. related to growth or turnover), e.g. the report “Who is who in Swedish retail 2020” ( Lindecrantz, 2020 ). Altogether, 50 retail companies provided input for this study (out of 300-plus companies who were sent invitations). The retailers represent a wide range of retail sectors and product types (see Table 1 ; respondents could provide multiple answers). The major retail sectors, currently at the forefront of online sales, are well-represented by their leading companies in the survey. Most of the retailers that are part of the panel are top-five (Sweden) in their segments based on turnover.
Our empirical data collection is based on an online survey. The respondents were senior supply chain/logistics managers divided between the following positions: 37 directors (or heads) of supply chain management/logistics/operations; one CEO; four warehouse managers; five managers responsible for development/design of logistics and/or warehouse solutions; one innovation lead; one head of e-business; and one head of an online store. The survey was pre-tested on five company representatives to test the questions' general appropriateness, functionality, and structure. The feedback was used to modify the survey instrument, mainly by increasing clarity in survey questions and explaining terminology. The respondents were asked to use a perceptual Likert scale ranging from 1 (“agree to a very low degree”) to 7 (“agree to a very high degree”) to assess their current focus and development in different areas. To address the limitations of scales in an exploratory study, most questions that included statements with fixed-scale alternatives were complemented with open-ended questions to explore alternative answers on the specific topic.
The main survey questions focused on the following issues: (1) company data and contextual factors, e.g. size, turnover, type of products, type of channel, assortment range, and lead-time offerings; (2) to what degree the companies invest in and use automation in warehouse operations – both in general and for different material-handling processes; (3) to what degree companies use technologies (other than automation) in the warehouse, e.g. regarding information platforms and connectivity. The respondents were asked to list and explain their three main focus areas related to the implementation of warehouse automation. Some questions included pre-determined answering alternatives, e.g. different types of technologies (with clarifying examples to reduce the risk of misunderstanding). We used many different sources to develop the survey questions, including scientific literature (discussing warehouse operations in retailing, warehouse automation and technology, and smart warehousing and technologies) and gray literature, e.g. business journals and tech blogs. We also scanned the market to understand more about automation technology and smart warehousing that either already exist or are being developed. As we initially looked for responses from both very small e-tailers (with large growth) and larger traditional and omnichannel (i.e. integrated store and online) retailers, we defined scales using a logarithmic approach to illustrate the large difference between potential answers regarding company data and contingency factors.
3.2 Data analysis
With an exploratory aim and too few respondents to conduct advanced statistical analysis, we described patterns in the participants' perceptions of current and future practice. We were inspired by multiple case study analyses (see, e.g. Miles and Huberman, 1994 ), with the aim of developing propositions for future research by pattern-matching data from open-ended and closed-ended questions. The same set of questions was used for both current and future states, and to detect significant differences in any context, we used a two-tailed t -test for equality of means.
To support the formulation of theoretical propositions about different pathways toward smart warehousing, we analyzed the data based on different contextual factors. First, we clustered the data based on channel strategy (with today's share of retailers stated): e-tailing (may include showrooms) (26%); partly integrated multichannel (both physical stores and online sales, but separated or only partly integrated channels) (50%); omnichannel (high degree of integration of store and online channels) (16%); and store focus, without (or locally store-driven) online sales (8%). Interestingly, our data indicate a strong trend (in five years) toward implementation of an omnichannel strategy among the panel retailers. Retailers selling only offline decreased (which is not a surprise), while store-driven online sales increased. Another observation is that e-tailers continued to focus on online sales and, with one exception, did not plan to open physical stores. The other contextual factors that we focused on in our analysis included turnover, assortment range, and sizes of goods.
4. Findings and analysis
To understand how Swedish retailers intend to transform from manual to smart warehouse management, we examined current trends and future intentions on several aspects. We present these findings in the following sub-sections and use them to develop 16 theoretical propositions.
4.1 Overall degree of automated warehouse operations
The study indicated an increased willingness to invest in automation, with retailers making large investments (>SEK 100 million annually), more than doubling such expenditures (8–22%), while those investing SEK 31–100 million annually increased such expenditures from 12 to 22%. Retailers not investing at all in automation decreased from 32 to 8%, indicating a statistically significant (see t -tests in Appendix ) and a strong increase in the overall degree of automation (in the warehouse with the most recent investment). On a scale from 1 (very low degree) to 7 (very high degree), the mean values increased from 2.07 (five years ago) via 3.15 (at present) to 5.13 (in five years). The retailers represented four clusters. The first group of retailers made large investments in recent years, but currently focuses on fine-tuning automation technology (e.g. by supplementing it with other technology). The second cluster has not yet automated to a large extent but intend to invest significantly in automated systems in the coming years. A third group represents retailers who already have made significant investments and continue to invest heavily in warehouse automation in their logistics networks. The fourth cluster, which is decreasing in number, represents retailers that have not and do not intend to automate their warehouses.
The degree of automation was analyzed on a deeper level, related to different warehouse operations ( Figure 1 ). The operations that have been automated to a greater extent represented outbound flows. Above all, “sorting outgoing goods” (average 3.30) stood out, while picking (2.89) and packing (2.80) also were higher, indicating that the most labor-intensive processes were automated first to justify return on investment. The focus on sorting also reflected the increased extent, variety, and complexity of sorting at the operational material-handling node level ( Kembro et al. , 2022 ). However, on the scale from 1 to 7, values below 4 still indicated a low degree of automation. The operations that currently are automated to a very low degree (thereby still requiring a greater share of manual work) are goods receipt (1.61), sorting of incoming goods (1.63), and handling returns (1.50). These flows included a wide variety of goods and requirements for quality control before storage. It is also important to determine the storage zone and balance incoming flows to avoid bottlenecks. Previous research (e.g. Kembro and Norrman, 2019 ) has indicated that retailers often position their most experienced staff in goods receipt.
Five years from now, a significant increase (see t -tests in Appendix ) in automation is expected, with several operations expected to average above 4.00 (storage 4.24, picking 4.98, packing 4.89, and outgoing sorting 5.32), indicating a clear trend toward a relatively high degree of automation (i.e. not only an increase, but also an increase to a relatively high level). Despite current low levels, the trend toward a higher degree of automation for incoming goods is interesting. Insights from a previous study ( Kembro and Norrman, 2019 ) indicate that automation of inbound processes requires greater work and coordination with suppliers, e.g. standardizing boxes/labels and balancing flows handled in warehouses (to avoid bottlenecks). It also seems that retailers want to try to make return handling more efficient, with some retailers stating that “automation of return flows is one of our top-three focus areas for technology in warehousing.”
Analyses of individual retailers and the number of warehouse operations that one has stated as highly automated (response alternatives 6–7 on a scale of 1–7) in five years provide additional insights ( Table 2 ). Twenty percent of the retailers answered that they will have automated five or more operations to a very high degree. Of these, many will have automated most of the warehouse operations to a high degree, and some even plan to use a fully automated warehouse. Altogether, 44% indicated that they will automate one to four operations significantly. Simultaneously, many retailers (38%) have not specified any of their operations as being highly automated, indicating that a high demand for manual work will remain in warehouses, but this differs between different retailers.
The more labor-intensive the processes, the higher the priority for retailers to automate. This implies that retailers begin by automating outbound picking, packing, and sorting (and related storage), followed by shipping and cross-docking to focus ultimately on inbound receipt, sorting, and handling returns.
4.2 Contextual factors' influence
To understand differences between the retailers, we first analyzed the data based on channel strategy. This analysis revealed that today's omnichannel retailers automated early (most indicated values 4–7 already five years ago), while e-tailers and retailers with partially integrated multichannels caught up by increasing the degree of automation sharply for the past five years. Retailers with a store focus remain at a low level. On a more detailed level, future omnichannel retailers, to some extent, will lead automation of incoming goods (receipt of goods, sorting of incoming goods, storage). E-tailers, to some extent, have less “cross-docking” compared with other retailers (which is logical considering that incoming pallets and cartons must be broken down for picking for e-customers). Instead, to a greater extent, they have invested in “sorting outgoing goods.” The greatest uncertainty (answer alternative “do not know”) applies to cross-docking and return handling, which, in itself, is an interesting observation.
Second, we analyzed the retailers' turnover. Most of the retailers in the panel (94%) currently have a turnover larger than 100 MSEK (∼10 MEuro), of which 36 (72%) sell more than one billion SEK (100 MEuro). Two of the retailers are global giants and sell over 100 billion SEK (∼10 billion Euro). Thus, relatively large retailers (rather than medium-size and small companies) dominate the panel. In five years, both the smaller and larger retailers plan quick growth. The analysis indicates that retailers with low turnover tend not to automate, which is in line with the large capital/investments required for automation. We noted a correlation between high turnover and high degree of automation ( Figure 2 ). An interesting observation is that medium-size companies presently have a relatively low degree of automation, but the tendency is that they are taking a big leap in five years.
Third, we analyzed assortment range, which generally continues to increase. Partially integrated multichannel retailers have driven this development (of which most aim to be highly integrated omnichannel retailers within five years). Altogether, 68% of these retailers will have an assortment range larger than 30,000 articles. The analysis indicates that retailers with a relatively small assortment tend to maintain a low degree of automation throughout their warehouses, while those with a larger assortment range (which are dominant in the study) increase their degree of automation the most ( Figure 3 ).
Fourth, we analyzed sizes of goods ( Figure 4 ). Presently, this mainly entails handling very small (0–1 liter) and small (1.1–50 liter) goods with automation. In five years, the trend is toward a significant increase in all sizes of goods (with the smallest for bulky, i.e. goods that do not fit on EUR pallets), with high averages for very small/small goods (5.60/5.39). Automated handling of medium-size goods (51–200 liters) also has been increasing (from 1.94 to 3.38). For large goods (>200 liters, which can fit on EUR pallets), a significant increase was found, but still at a generally low level. Among the answers were several “Do not knows,” which, in this case, also represent “not applicable,” a question that is not relevant to the retailer if it does not handle goods of this size in its warehouses.
Omnichannel retailers increased automated handling of large and complex order and goods flows early, but as other retailers catch up on their investments, channel strategy will have less explanatory value on the overall degree of automation.
The larger the turnover and assortment range, and the smaller the sizes of the goods, the more retailers invest in automation technology to improve material handling throughout the warehouse.
4.3 Choice of automation technology for material handling
Another interesting aspect is the choice of automation technology for material handling ( Figure 5 ). We observed generally rare use (Answer Option 1) of most examined automation technologies, but some stood out and are significantly more common than the others: stationary automated sorting systems (3.50); stationary automated storage and retrieval (AS/RS) (2.93); compact/grid-based storage (3.12); and automated packaging systems (2.88). Interestingly, for these technologies, the situation is dipolar , i.e. retailers did not use them at all or rarely. This is explained through our analysis: Some automation technologies tend to be chosen for a certain context (e.g. product characteristics), while others are used more generally among retailers. For storage and picking, companies mainly choose an automation technology depending on goods/flow characteristics: Omnichannel retailers with a mix of goods and order characteristics (e.g. both store replenishment and online) typically choose stationary AS/RS storage, while e-tailers typically choose compact/grid-based storage (e.g. AutoStore) to handle large assortments of relatively small goods (e.g. clothes) that individual online customers order. Retailers that handle piece-pick-intense online orders (i.e. large volumes of small goods) opt for A-frame automatic dispensers. Simultaneously, there tends to be a more general approach in terms of automation of packaging, weighing, dimensioning, sorting, and palletizing of outgoing goods (though mainly retailers with store networks use automatic palletizing).
In five years, use of most of these automation technologies will increase, a pattern in line with the current situation observed. We detected significant increases ( t -test; see Appendix ) in stationary automated sorting (5.06), automated packaging (5.06), compact/grid-based storage (4.56), cubing (4.91), robotic palletizing (4.41), stationary automated storage and retrieval (3.72), A-frame systems (2.58), robotized piece-picking (2.25), automated guided vehicles (2.34), and self-driving flexible forklifts (2.00). Several technologies reached over 4.00 (average), and most are dipolar , i.e. while some retailers are strong advocates (blue), others will not implement such technology at all (black/red). This implies that retailers will opt for different technologies as they automate material handling in their warehouses. However, the future is not as dipolar as the current situation (the color scale is more gradual). One possible explanation is that when retailers' warehouses (e.g. turnover and assortment ranges) grow, they must handle a larger mix of orders, flows, and goods, with more varied characteristics. Thus, retailers introduce multiple zones and may benefit from using a mix of different technologies (e.g. one technology designed to handle smaller goods in one zone and another technology tailored for larger goods in another zone).
The less a warehouse activity is influenced by contextual factors – which mainly applies to inbound and outbound due to more standardized processes and handling units – the more standardized automation technologies are used to improve material handling.
The more a warehouse's activity is influenced by contextual factors – which mainly applies to storage and picking adjusted to, for example, SKU and order characteristics – the more tailored the automation technologies used to improve material handling.
The larger the assortment of relatively small goods, the more retailers automate by using compact/grid-based storage and goods-to-person technology to improve space utilization and increase efficiency of storage and retrieval activities.
The higher the volume of small goods for individual customers, the more retailers automate by using A-frame technology to reduce costs and lead time for piece-picking activities.
The higher the volume of mixed goods and integrated channels, the more retailers automate by using stationary automated storage and retrieval technology to increase space utilization, and the greater the efficacy and efficiency in handling large throughputs of more varied order and product flows.
An interesting observation is that many retailers choose static, rather than flexible, automation technologies mainly for storage, picking, and sorting. This may mean less leeway to change and adapt automation technologies in the future – particularly considering that retailers are making very large investments now and that the future budget for major changes may be limited. Thus, this trend could mean that automation/warehousing, to a greater extent, will dictate conditions in the future (e.g. setting boundaries or creating opportunities) for changes in product assortment, logistics networks, and overall logistics strategy. If retailers invest in scalable and flexible solutions, it may be easier to grow with acquisitions, add more varied store formats, or create more flexible offerings and a wider variety of products. From a historical perspective, large investments in (static) automation technologies were made in the early 2000s, influencing later strategic decisions related to, for example, the designing of networks and warehouses to handle increasing numbers of online orders.
Investments in static automation technology for storage, picking, and sorting reduce a retailer's ability to adapt warehouse operations to future contextual changes, e.g. reduced customer order lead times and wider variety of product offerings.
Today's large investments in static automation technology for storage, picking, and sorting imply that warehouse operations to a greater extent may dictate conditions for changes in product assortment, logistics networks, and overall logistics strategy decisions.
4.4 Complementary technologies in warehouses
The survey also examined other technologies that complement automated material handling ( Figure 6 ), e.g. those related to digitalization and connectivity. Today, WMS is used frequently (average 5.98) and is viewed, more or less, as standard for controlling and managing daily operations in warehouses. Corresponding with WMS, WCS (3.71) and WES (3.07) are used to control and coordinate a variety of processes and automation. In addition to these, pick-by-voice (3.13) and pick-by-light/put-to-light (2.37) are used to some extent.
Digitalization and connectivity technologies, e.g. private networks (4G, 5G) and AI, are used to a small extent (2.74 and 2.25, respectively). However, some individual retailers have invested to a large extent and may be viewed as pioneers. Relatively speaking, more e-tailers than omnichannel retailers submitted high values (6, 7) for WMS, WCS, WCE, private networks, and AI. Today, other technologies are not used at all in principle, including RFID technology (1.08), pick-by-vision (1.29), and IVA (1.12), nor are other hyped technologies used, e.g. the IoT, digital twins, drone technology, or blockchain.
The trend in five years indicates that WMS (average 6.62), WCS (5.95), and WES (5.34) will become the backbone of warehouse management, with large significant increases in WCS and WES. Strong future trends also indicate significant increases for several technologies that humans use to strengthen workers' abilities, e.g. to pick faster or reduce picking errors (so-called “human augmentation”), including put-to-light/pick-by-light (4.45), pick-by-voice (3.16), and pick-by-vision (2.05). The increasing use of these technologies indicates that there still will be human workers in the future who conduct material-handling activities (particularly picking) in warehouses. The sharp rise in use of put-to-light/pick-by-light partly points to the importance of faster labor with lower error rates.
WMS, WCS, and WES represent the backbone of warehouse management, whereas hyped technologies – e.g. RFID, IoT, drone technology, and blockchain – will play a limited role in warehousing in the years to come.
The bigger the focus on online customers, the more retailers invest in technologies that support data management, connectivity, and real-time analysis.
5. The pathway toward smart warehousing
Building on our findings and analysis, we sought to understand to what degree smart warehousing was a tendency or trend among the panel's retailers. With support from the literature, we used survey data to operationalize two dimensions: (1) degree of automation and (2) degree of digitalization and connectivity of information platforms. Degree of automation focuses on automation of material handling, i.e. the handling of physical goods. Examples include stationary automated storage, compact/grid-based storage, A-frame systems, automated sorting systems, and automated weighing and dimensioning. Degree of digitalization and connectivity of information platforms focuses on technologies for handling, analysis, and coordination of information and includes, for example, WMS, WCS, WES, AI, IoT, and private networks.
For each dimension, we summarized the number of technologies (per dimension) for each retailer, in which a high degree of implementation was indicated (Values 5, 6, and 7). We then plotted this value for each retailer in Figure 7 , indicating each company's current position (yellow square) and its intentions in five years (red circle). Automation of material handling is illustrated on the Y -axis, with degree of digitalization and connectivity of information platforms on the X -axis. As previously described, several retailers increased automation of material handling (moving upward along the Y -axis). Simultaneously, many retailers are investing in information platforms and increased digitalization (moving to the right). As the gray arrows illustrate in Figure 7 , the overall trend is that the technology frontier moves diagonally upward to the right, implying that retailers to varying degrees are investing in both automation technologies and information platforms, representing a general tendency toward an intentional technological shift in retailers' warehouses over the next five years.
We conducted additional analyses of the individual retailers' movements. Interestingly, the retailers that perceive themselves as having smart warehouses in five years are not the retailers that are most automated or digitalized today. Instead, several retailers are planning major technological upgrades over the next five years, i.e. moving from having limited technology in warehousing to being at the forefront of development toward smart warehousing. We illustrated this technology shift with four retailers' movements (the blue dotted arrows in Figure 7 ).
Our analysis further indicates that retailers follow different paths toward smart warehousing. On one hand, some retailers follow an automation-focused path toward smart warehousing, i.e. while investing in a wide range of technologies to implement smart warehousing, these retailers emphasize technologies that automate material handling of their physical goods flows. This may arise from the need to manage more varied warehouse operations ( Kembro and Norrman, 2020 ). Examples include handling online customers vs store replenishment; a variation in flows, including handling returns and cross-docking; and large variations in SKU sizes (e.g. pieces, cartons, and pallets). The more varied the operations, the wider the range of automation technology needed for material handling. Another driver is increased sorting complexity (due to, e.g. multiple destinations, delivery modes, and transporters), creating a need for additional automation technology ( Kembro et al. , 2022 ). The historical footprint is also relevant, in which retailers that automated certain material flows early (e.g. store replenishment) add automation technologies dedicated to meeting continuously changing online customers' requirements ( Eriksson et al. , 2022 ).
On the other hand, our data indicate that some retailers, particularly e-tailers, will follow a more digitalization-focused path toward smart warehousing. While these retailers invest in automated material handling, they emphasize information platforms and technology that enable real-time data analysis (e.g. AI). These retailers have less variation in operations and, therefore, require a narrower range of automation technologies for material handling. For example, an e-tailer may have a high automation degree, but can handle warehouse operations with only one main form of automation technology (e.g. AutoStore). This enables a greater focus on other complementary technologies for digitalization and connectivity of information platforms. An important driver is online sales, in which e-tailers generally are more focused on virtual contact with customers, requiring a range of integrated IT systems. Another driver is the need to connect multiple material-handling nodes (e.g. DC, OFC, retail stores) in the logistics network ( Kembro and Norrman, 2019 ).
The advancement of the smart warehousing frontline is driven not by retailers with a current high degree of automated material handling, but rather by pioneering retailers that make a major technological shift from limited use of technology.
Retailers with different channel strategies take different implementation routes toward smart warehousing, in which more-integrated omnichannel retailers follow an automation-focused path, whereas less-integrated retailers and e-tailers follow a more digitalization-focused path.
5.1 Conceptualizing smart warehousing
At this stage, it is also relevant to define smart warehouse (which is currently missing in the literature). Based on extant literature ( Section 2 ) and on an analysis of retailers' current and intended technological implementation, we conceptualize future smart warehouses as: Automated , i.e. robots will handle a large part of physical material handling; Autonomous , in which robots make their own decisions regarding task distribution (e.g. order management) and movements in the warehouse – a combination of autonomous automation also can be called autonomization; Digital , i.e. integrated information platforms handle warehouse management (e.g. inventory levels, sequencing of order picks), including functionality for analysis of large amounts of data (AI/machine learning), e.g. for improved forecasting; and Connected , in which moving resources and products are monitored, controlled, and coordinated in real time. It also enables real-time analysis (e.g. via IVA) of in-store activity to allow for fast decision-making and further development of processes.
We summarize these insights in Figure 8 , which outlines both the two dimensions of smart warehousing, as well as the different stages and pathways toward this goal.
5.2 Connecting multiple smart warehouses in extended logistics networks
Moving beyond the single smart warehouse, retailers will use multiple material-handling nodes in their future logistics networks ( Hübner et al. , 2022 ; Kembro et al. , 2022 ). Our findings indicate that retailers will expand from zero or one to between two and five large distribution warehouses. Our study also found that retailers are adding more and varied material-handling nodes (e.g. DC, OFC, MFC) in their decentralized logistics networks. These are complemented by transformed physical stores, which are becoming the center of retail operations ( Hübner et al. , 2022 ).
Apart from market expansion, the main reason for expanding the number of material-handling nodes is the extremely short lead times from customer order to final delivery. Global giants, e.g. Alibaba and Amazon largely have driven this development, with their increasingly competitive promises to customers ( Kembro et al. , 2022 ). In our study, 52% of multichannel retailers (of which most aim to be highly integrated omnichannel retailers within five years) intend to offer standard lead times under 24 h. A similar pattern is visible for e-tailers. No matter how fast a central warehouse fulfills an order, the transportation times to final destination (e.g. home delivery, C&C) may result in lead times that exceed customer expectations. This development will require well-coordinated logistics networks (e.g. use of drop-shipment and small-scale warehouses, e.g. OFCs or MFCs in and around cities, i.e. closer to end customers). It also will drive the need for effective and efficient material handling across network nodes, which can be implemented, e.g. by investing in new automation technology, as well as advanced and integrated information systems. As Kembro and Norrman discussed ( 2019 ), future warehouses and stores, to a greater extent, will be interconnected, among other ways, through the use of a so-called DOM system. For example, inventory levels are coordinated between different material-handling nodes, and an online order can be routed to/managed in different nodes depending on several defined parameters/goals (e.g. reducing lead times or lowering handling costs).
With increasingly competitive lead-time promises to customers, retailers use more and varied (smaller, localized) material-handling nodes that need to be interconnected in smart warehouse networks.
With requirements on effective and efficient warehousing across logistics networks, retailers increasingly use automated material-handling technology in different types of decentralized material-handling nodes, e.g. micro-fulfillment centers.
6. Conclusions and future research
This study aimed to conceptualize the term smart warehousing and explain pathways on how to implement it. By empirically studying this novel phenomenon, our research influences the definition of its problem domain and offers multiple contributions of the “theoretical pre-science” type ( Corley and Gioia, 2011 ).
Contributing to recent and limited literature on smart warehousing ( Azadeh et al. , 2019 ; Mahroof, 2019 ; Chung, 2021 ; Zhang et al. , 2021 ), we put forth 16 propositions related to automation and complementary technology, as well as pathways toward smart warehousing. Our analysis indicates that the future smart warehouse will be automated, autonomous, digital, and connected, but that retailers will follow different paths along this journey. To support our analysis, we operationalized smart warehousing into two dimensions: degree of automation and degree of digitalization and connectivity of information platforms. This is an important contribution to the literature in different fields (e.g. logistics, operations research, and information systems) that mention smart warehousing without defining it. Our operationalization also could influence future conversion of smart warehousing, enabling analysis of patterns on a more holistic level and focusing not just on specific applications of certain technologies that characterize much of current research.
Interestingly, our study revealed that many of the retailers that aim to create smart warehouses in five years are not the retailers with the most developed technology today. In this transition, retailers followed different technological pathways driven by contextual trends, e.g. the growth of sales, wider product assortment, shorter lead-time offerings, and channel strategy. By demonstrating how the continuously evolving retail landscape influences back-end logistics, we contribute to the literature on retail logistics and warehousing (e.g. Galipoglu et al. , 2018 ; Kembro et al. , 2018 ), as well as related automation technology and information platforms ( Kembro and Norrman, 2019 ). Specifically, we explain why retailers calibrate their timing, technology, and focus to suit certain operations. The study found that retailers first automate labor-intensive, outbound warehousing operations, with an emphasis on small or very small goods. Next, many automate larger-size goods and expand their focus to include inbound operations. An important observation is that automation of outgoing flows is more non-contextual (i.e. similar across retailers), while storage and picking technologies seem more tailored to contextual factors (e.g. characteristics of goods). We also conclude that although new automation technology is available for a wider range of retail segments and sizes, it still requires a large investment. This may explain why retailers with larger turnovers and assortment ranges invest more in automation.
Our study proposes this and provides explanations as to why some retailers focus on advanced automation technology while others tend to pioneer digitalization and connectivity. Retailers generally have a solid understanding of information systems for automated material handling, but have limited knowledge about emerging smart warehousing technologies related to digitalization and connectivity. In five years, WMS, WCS, and WES will be the backbone of warehouse operations, complemented with technologies that support data management and real-time analysis, including AI, IoT, RFID, and IVA. This technological development also is important for connecting logistics networks with multiple, different, and decentralized material-handling nodes (e.g. automated MFCs) to meet growing demand for very short lead times from placed order to delivery.
This study provides practically useful guidance for managers by outlining what is trending now and five years down the road. In many companies and countries, the transformation toward smart warehousing has only just begun. Empirical insights from pioneering practice can help other retailers understand critical issues earlier, as well as how to address them. Our findings provide insights into technologies expected to grow in use and criticality to support both material handling in single warehouses and increasingly complex and decentralized networks. Managers also can use our 16 propositions to reflect on what the near future holds and use them as input for scenario analysis.
Pre-science studies' observations naturally elicit speculation that needs more research. We noted that, related to automation, retailers' current tendency to invest in static automation solutions could limit their future strategic options. Future research could investigate whether these kinds of technological investments follow existing strategy – or whether they instead are driving or delimiting future strategies (e.g. to be able to grow, we need to automate vs our current automation technology, which restricts/supports our scaling up). Explanations as to why some retailers seem to lead the digitalization and connectivity journey should be studied: Are fewer capital investments (compared with automation) required? Do they have fewer nodes and simpler flows on which to focus? Have they reached a higher maturity level regarding information technology?
The conceptualization and operationalization of smart warehousing can be developed further through additional empirical evidence collected in other markets. To build theory, in-depth case study research could be employed to better understand different contingency factors' influence. Of special interest would be research on implementation and transformation (using theoretical lenses, e.g. dynamic capabilities or technology adoption models), economic assessment of investment and performance, and an examination of barriers and opportunities related to the interaction between human and smart technologies in future warehouses. The literature presented mixed perspectives on humans' role in future warehousing. Some warehouse operations remain difficult to automate and may need to be carried out manually ( Azadeh et al. , 2019 ). While some researchers trumpet their unmanned warehouses as a defining characteristic or goal for smart warehouses ( Aamer and Sahara, 2021 ; Jiang et al. , 2021 ), others see robots and AI eventually replacing humans ( Jabber et al. , 2018 ). Some have argued that humans will not be replaced, but rather supported ( Winkelhaus and Grosse, 2020 ), with the intent to better connect people, objects, and physical systems ( Lee et al. , 2018 ). Thus, future research could study which factors explain to what extent future warehouses will be manual, automated, or smart.
Like most research designs, this study has limitations. The sample of retailers (50) that answered the survey was relatively small, not random, and only included retailers from one country. However, we argue that the sample is sufficient for developing propositions regarding the researched phenomenon ( Forza, 2002 ) because Sweden is among the leaders in online sales, and among the top-10 industrial digital transformation countries in 2020 ( Top 10 industrial digital transformation countries in 2020 | InfotechLead ). Furthermore, the most important product segments are covered, including leading retailers within each segment. To pinpoint theoretical and managerial implications further, our research needs to be complemented by and tested through more research. Specifically, the 16 propositions can be tested as hypotheses in future research with more empirical evidence by expanding testing to other markets, both in larger countries at similar stages of transformation toward omnichannels (e.g. the US, UK, and Germany) and in countries that are developed in terms of online sales. To understand smart warehousing technologies, logistics service providers and industrial companies also should be examined. Due to their deeper backgrounds with Industry 4.0 and their connection to smart production, industrial companies might make investments and implement smart warehousing differently than retailers.
In conclusion, the pace of development toward smart warehousing will increase in the coming years. Various systems and technologies will be developed and integrated within and across various material-handling nodes, providing many opportunities for researchers to examine and analyze new challenges and solutions, creating new knowledge in warehousing and retail logistics. Only the future can tell us how smart warehouses evolve and why.
Degree of automation per process, today and in five years ( n = 50)
Overall degree of warehouse automation over time, related to turnover
Overall degree of warehouse automation over time, related to assortment range
Automation of different sizes of goods, today and in five years
Choice of automation technology for material handling, today and in five years
Other complementary technology used in warehouses, today and in five years
Technology shifts in retailers' warehouses over the next five years; several retailers may hide behind the same point in the chart
Conceptualization of smart warehousing
Multiple, connected smart warehouses in expanding, decentralized logistics networks
Retail sectors and product categories in the panel
Number of highly automated processes per company five years ahead
T-tests to detect significant differences between current and future states
Funding : The Swedish Retail and Wholesale Council funded this research study.
Aamer , A.M. and Sahara , C.R. ( 2021 ), “ Real-time data integration of an internet-of-things-based smart warehouse: a case study ”, International Journal of Pervasive Computing and Communications , Vol. ahead-of-print No. ahead-of-print , doi: 10.1108/IJPCC-08-2020-0113 .
Alibaba Cloud ( 2019 ), “ Double 11 logistics: Cainiao's battle of ‘billions of parcels’ ”, available at: https://www.alibabacloud.com/blog/double-11-logistics-cainiaos-battle-of-billions-of-parcels_595648 ( accessed 17 September 2020 ).
Amazon ( 2020 ), “ Fulfilment in our buildings ”, available at: https://www.aboutamazon.co.uk/amazon-fulfilment/fulfilment-in-our-buildings ( accessed 15 September 2020 ).
Attaran , M. ( 2020 ), “ Digital technology enablers and their implications for supply chain management ”, Supply Chain Forum: An International Journal , Vol. 21 No. 3 , pp. 158 - 172 .
Azadeh , K. , De Koster , R. and Roy , D. ( 2019 ), “ Robotized and automated warehouse systems: review and recent developments ”, Transportation Science , Vol. 53 No. 4 , pp. 917 - 945 .
Baker , P. and Halim , Z. ( 2007 ), “ An exploration of warehouse automation implementations: cost, service, and flexibility issues ”, Supply Chain Management: An International Journal , Vol. 12 No. 2 , pp. 129 - 138 .
Bartholdi , J.J. III and Hackman , S.T. ( 2016 ), Warehouse and Distribution Science , Georgia Institute of Technology , Atlanta, Georgia .
Bolu , A. and Korcak , Ö. ( 2019 ), “ Path planning for multiple mobile robots in smart warehouse ”, 2019 IEEE 7th International Conference on Control, Mechatronics, and Automation , Delft .
Chung , S.-H. ( 2021 ), “ Applications of smart technologies in logistics and transport: a review ”, Transportation Research Part E: Logistics and Transportation Review , Vol. 153 , 102455 , pp. 1 - 17 .
Corley , K.G. and Gioia , D.A. ( 2011 ), “ Building theory about theory building: what constitutes a theoretical contribution? ”, Academy of Management Review , Vol. 36 No. 1 , pp. 12 - 32 .
De Koster , R. ( 2018 ), “ Automated and robotic warehouses: developments and research opportunities ”, Logistics and Transport , Vol. 38 , pp. 33 - 40 .
De Leeuw , S. and Wiers , V.C.S. ( 2015 ), “ Warehouse manpower planning strategies in times of financial crisis: evidence from logistics service providers and retailers in the Netherlands ”, Production Planning and Control , Vol. 26 , pp. 328 - 337 .
Edmondson , A.C. and McManus , S.E. ( 2007 ), “ Methodological fit in management field research ”, Academy of Management Review , Vol. 32 No. 4 , pp. 1155 - 1179 .
Eriksson , E. , Norrman , A. and Kembro , J. ( 2019 ), “ Contextual adaptation of omnichannel grocery retailers' online fulfilment centers ”, International Journal of Retail and Distribution Management , Vol. 47 No. 12 , pp. 1232 - 1250 .
Eriksson , E. , Norrman , A. and Kembro , J. ( 2022 ), “ Understanding the transformation toward omnichannel logistics in grocery retail: a dynamic capabilities perspective ”, International Journal of Retail and Distribution Management , Vol. ahead-of-print No. ahead-of-print , doi: 10.1108/IJRDM-10-2021-0508 .
Faber , N. , De Koster , R.B. and Smidts , A. ( 2018 ), “ Survival of the fittest: the impact of fit between warehouse management structure and warehouse context on warehouse performance ”, International Journal of Production Research , Vol. 56 Nos 1-2 , pp. 120 - 139 .
Flytware ( 2021 ), “ Smart warehouse: the present and the future of warehousing operations ”, available at: https://flytware.com/blog/smart-warehouse ( accessed 25 May 2021 ).
Forza , C. ( 2002 ), “ Survey research in operations management: a process-based perspective ”, International Journal of Operations and Production Management , Vol. 22 No. 2 , pp. 152 - 194 .
Frazelle ( 2016 ), World-Class Warehousing and Material Handling , 2nd ed. , McGraw-Hill Educational , New York .
Galipoglu , E. , Kotzab , H. , Teller , C. , Yumurtaci Hüseyinoglu , I.Ö. and Pöppelbuß , J. ( 2018 ), “ Omnichannel retailing research – state of the art and intellectual foundation ”, International Journal of Physical Distribution and Logistics Management , Vol. 48 No. 4 , pp. 365 - 390 .
Gu , J. , Goetschalckx , M. and McGinnis , L.F. ( 2007 ), “ Research on warehouse operation: a comprehensive review ”, European Journal of Operational Research , Vol. 177 No. 1 , pp. 1 - 21 .
Hassan , M. , Ali , M. , Aktas , E. and Alkayid , K. ( 2015 ), “ Factors affecting selection decision of auto-identification technology in warehouse management: an international Delphi study ”, Production Planning and Control , Vol. 26 No. 12 , pp. 1025 - 1049 .
Hübner , A. , Hense , J. and Dethlefs , C. ( 2022 ), “ The revival of retail stores via omnichannel operations: a literature review and research framework ”, European Journal of Operational Research , Vol. 302 No. 3 , pp. 799 - 818 , doi: 10.1016/j.ejor.2021.12.021 .
Issaoui , Y. , Khiat , A. , Bahnasse , A. and Ouajji , H. ( 2021 ), “ Toward smart logistics: engineering insights and emerging trends ”, Archives of Computational Methods in Engineering , Vol. 28 , pp. 3183 - 3210 .
Jabbar , S. , Khan , M. , Nathalia Silva , B. and Han , K. ( 2018 ), “ A REST-based industrial web of things' framework for smart warehousing ”, Journal of Supercomputing , Vol. 74 , pp. 4419 - 4433 .
Jiang , Z.-Z. , Wan , M. , Pei , Z. and Qin , X. ( 2021 ), “ Spatial and temporal optimization for smart warehouses with fast turnover ”, Computers and Operations Research , Vol. 125 , 105091 , pp. 1 - 17 .
Kamali , A. ( 2019 ), “ Smart warehouse vs. traditional warehouse – review ”, CiiT International Journal of Automation and Autonomous System , Vol. 11 No. 1 , pp. 9 - 16 .
Kembro , J. and Norrman , A. ( 2019 ), “ Exploring trends, implications, and challenges for logistics information systems in omnichannels: Swedish retailers' perception ”, International Journal of Retail and Distribution Management , Vol. 47 No. 4 , pp. 384 - 411 .
Kembro , J.H. and Norrman , A. ( 2020 ), “ Warehouse configuration in omnichannel retailing: a multiple case study ”, International Journal of Physical Distribution and Logistics Management , Vol. 50 No. 5 , pp. 509 - 533 .
Kembro , J.H. and Norrman , A. ( 2021 ), “ Which future path to pick? A contingency approach to omnichannel warehouse configuration ”, International Journal of Physical Distribution and Logistics Management , Vol. 51 No. 1 , pp. 48 - 75 .
Kembro , J. , Danielsson , V. and Smajli , G. ( 2017 ), “ Network video technology: exploring an innovative approach to improving warehouse operations ”, International Journal of Physical Distribution and Logistics Management , Vol. 47 No. 7 , pp. 623 - 645 .
Kembro , J.H. , Norrman , A. and Eriksson , E. ( 2018 ), “ Adapting warehouse operations and design to omnichannel logistics: a literature review and research agenda ”, International Journal of Physical Distribution and Logistics Management , Vol. 48 No. 9 , pp. 890 - 912 .
Kembro , J. , Eriksson , E. and Norrman , A. ( 2022 ), “ Sorting out the sorting in omnichannel retailing ”, Journal of Business Logistics . doi: 10.1111/jbl.12305 .
Ketokivi , M. and Choi , T. ( 2014 ), “ Renaissance of case research as a scientific method ”, Journal of Operations Management , Vol. 32 No. 5 , pp. 232 - 240 .
Lee , C.K.M. , Lv , Y. , Ng , K.K.H. , Ho , W. and Choy , K.L. ( 2018 ), “ Design and application of Internet of things-based warehouse management system for smart logistics ”, International Journal of Production Research , Vol. 5 No. 8 , pp. 2753 - 2768 .
Lindecrantz , A. ( 2020 ), Stora Guiden till Retail Vem är Vem 2020 , Hakon Media AB , Solna .
Mahroof , K. ( 2019 ), “ A human-centric perspective exploring the readiness toward smart warehousing: the case of a large retail distribution warehouse ”, International Journal of Information Management , Vol. 45 , pp. 176 - 190 .
Malhotra , M.K. and Grover , V. ( 1998 ), “ An assessment of survey research in POM: from constructs to theory ”, Journal of Operations Management , Vol. 16 , pp. 407 - 425 .
MH&L ( 2020 ), “ Warehouse automation investment up due to COVID-19 ”, available at: https://www.mhlnews.com/warehousing/article/21136484/warehouse-automation-invest-up-due-to-covid19 ( accessed 15 May 2021 ).
Miles , M.B. and Huberman , A.M. ( 1994 ), Qualitative Data Analysis: An Expanded Sourcebook , 2nd ed. , Sage Publications , Thousand Oaks, California .
Reiser , C. ( 2020 ), “ Investing in warehouse automation? Who isn't ”, available at: https://logisticsviewpoints.com/2020/07/08/warehouse-automation-investment ( accessed 15 May 2021 ).
Rouwenhorst , B. , Reuter , B. , Stockrahm , V. , van Houtum , G.J. , Mantel , R.J. and Zijm , W.H. ( 2000 ), “ Warehouse design and control: framework and literature review ”, European Journal of Operational Research , Vol. 122 No. 3 , pp. 515 - 533 .
Rowley , J. (Ed.) ( 2000 ), The Principles of Warehouse Design , 2nd ed., The Institute of Logistics & Transport , Corby .
Taboada , I. and Shee , H. ( 2021 ), “ Understanding 5G technology for future supply chain management ”, International Journal of Logistics Research and Applications , Vol. 24 No. 4 , pp. 392 - 406 , doi: 10.1080/13675567.2020.1762850 .
Themistocleous , M. , Irani , Z. and O'Keefe , R.M. ( 2001 ), “ ERP and application integration. Exploratory survey ”, Business Process Management Journal , Vol. 7 No. 3 , pp. 195 - 204 .
Tokar , T. , Williams , B.D. and Fugate , B.S. ( 2020 ), “ I heart logistics – just don't ask me to pay for it: online shopper behavior in response to a delivery carrier upgrade and subsequent shipping charge increase ”, Journal of Business Logistics , Vol. 41 No. 3 , pp. 182 - 205 .
van Geest , M. , Tekinerdogan , B. and Catal , C. ( 2021 ), “ Design of a reference architecture for developing smart warehouses in Industry 4.0 ”, Computers in Industry , Vol. 124 , 103343 , pp. 1 - 21 .
Winkelhaus , S. and Grosse , E.H. ( 2020 ), “ Logistics 4.0: a systematic review toward a new logistics system ”, International Journal of Production Research , Vol. 58 No. 1 , pp. 18 - 43 .
Zhang , D. , Pee , L.G. and Cui , L. ( 2021 ), “ Artificial intelligence in e-commerce fulfillment: a case study of resource orchestration at Alibaba's smart warehouse ”, International Journal of Information Management , Vol. 57 , 102304 .
Corresponding author
Related articles, we’re listening — tell us what you think, something didn’t work….
Report bugs here
All feedback is valuable
Please share your general feedback
Join us on our journey
Platform update page.
Visit emeraldpublishing.com/platformupdate to discover the latest news and updates
Questions & More Information
Answers to the most commonly asked questions here

- SUGGESTED TOPICS
- The Magazine
- Newsletters
- Managing Yourself
- Managing Teams
- Work-life Balance
- The Big Idea
- Data & Visuals
- Reading Lists
- Case Selections
- HBR Learning
- Topic Feeds
- Account Settings
- Email Preferences
Research: How Do Warehouse Workers Feel About Automation?
- Raghav Narsalay,
- Rushda Afzal,
- Ida Nair Sharma,

Interviews with workers from around the world shed light on their hopes and concerns.
Automated tools that help to lift, sort, and move goods around warehouses can substantially improve efficiency and quality. But how do the millions of workers employed in warehouses around the world feel about these changes? The authors conducted a series of interviews with on-the-ground workers and identified several common hopes and concerns. Based on these trends, they offer three strategies to help employers both address workers’ fears and build on their optimism: Emphasizing growth opportunities for entry-level workers, providing effective training to help workers learn to use automated tools, and investing in systems to ensure workers’ health and safety.
As of 2019, the global warehouse automation market — that is, programmable machines that pick, sort, and return goods to their shelves, as well as sensor- and AI-based tools that simplify tasks for warehouse workers — was worth about $15 billion . That number is expected to double within the next four years, with supply chain leaders in an internal Accenture survey citing warehouse automation as one of their top three priorities for digital investment. Clearly, the industry has huge growth potential. But what does this mean for the millions of workers who currently work in warehouses around the world?
- JL Joe Lui is the global robotics practice lead for Accenture and is based in Seattle.
- Raghav Narsalay is global research lead on Industry X for Accenture and is based in Mumbai.
- RA Rushda Afzal is a manager with Accenture Research and is based in Detroit.
- IS Ida Nair Sharma is an associate manager with Accenture Research and is based in Gurgaon, India.
- Dave Light is a director of Thought Leadership Development at Accenture Research and is based in Boston.
Partner Center
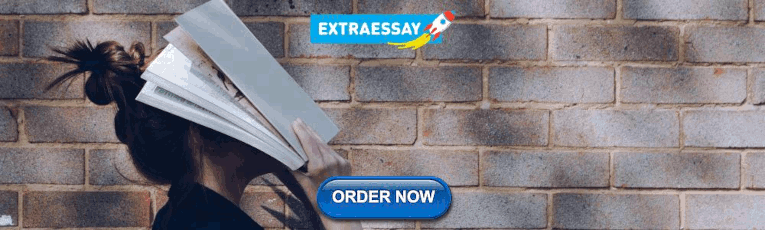
IMAGES
VIDEO
COMMENTS
This research paper provides an analysis of warehouse management systems, highlighting the benefits they offer, the challenges associated with their implementation, and the best practices for ...
The comparative analysis of the individual warehouse processes' duration is also visible in this chapter. Finally, everything is rounded off with the observed conclusions of the research. 2. Overview of warehouse process optimization methods Logistics costs take an important part of the overall production costs.
A scheme to classify warehouse design and operation planning problems and the corresponding literature is shown in Fig. 1 (the numbers in parentheses represent the numbers of papers reviewed in this document for each operation planning problem) and a more detailed description of each problem category identified is given in Table 1.This paper will focus on the operation planning problems, while ...
The "smart warehouse" system, which is equipped with emerging warehousing technologies, is increasingly attracting the attention of industry and technology giants as an efficient solution for the future of warehouse development. ... Research on warehouse operation: A comprehensive review. European Journal of Operational Research, 177(1): 1 ...
The reviewed papers were also investigated in order to identify research gaps and future directions. The analysis concluded that more research is needed to better understand and optimize heterogeneous and non-standard spare parts environments in terms of allocation and layout design. More practical case applications also remain a gap to address.
A warehouse covers a wide spectrum of operations for the distribution of goods in a supply chain network. The advancement of technology and the changing global business environment have compelled the transformation of a warehouse. The present study attempts to revisit the warehouse transformation from 1990 to 2019 through an evolutionary lens.
This survey and a companion paper ( Gu et al., 2007) present a comprehensive review of the state-of-art of warehouse research. Whereas the latter focuses on warehouse operation problems related to the four major warehouse functions, i.e., receiving, storage, order picking, and shipping, this paper concentrates on warehouse design, performance ...
Warehouse Operations. AbstractE-commerce, new retail, and other changes have highlighted the requirement of high efficiency and accuracy in the logistics service. As an important section in logistics and supply chain management, warehouses need to respond positively to the increasing requirement. The "smart warehouse" system, which is ...
Papers in the special issue. In their paper A Hierarchical Approach to Warehouse Design, Timothy Sprock, Anike Murrenhoff and Leon F. McGinnis propose a hierarchical design decision support methodology for warehouses, based on decomposing the design problem into a set of subproblems and using a formal model of the system to integrate the solutions to these subproblems.
1. Introduction. The retail industry has been undergoing a digital transformation coupled with customers' expectations of shorter lead times (i.e. demanding same-day delivery), high product availability, flexibility when and where to shop, and varying delivery (e.g. click-and-collect, pick-up points, home delivery) and return options (Galipoglu et al., 2018; Tokar et al., 2020).
This paper presents a comprehensive review of the state-of-the-art in research on warehouse operation planning. We first present a unifying framework to classify the research on different but related warehouse problems. Within this framework, historical progress and major results are summarized with an emphasis on how the research on these ...
The purpose of this study was examining the effect of warehousing management on warehouse performance in the case of Modjo dry port concerning the five main warehousing activities (receiving, put-away, storage, order-picking, and shipping). Both primary (questionnaires and interviews) and secondary sources of data were used.
As of 2019, the global warehouse automation market — that is, programmable machines that pick, sort, and return goods to their shelves, as well as sensor- and AI-based tools that simplify tasks ...
Automation and digitisation are the driving force of the Industrial Revolution 4.0. Industrial revolutions led to the mass production of goods, which increased the need for modern warehouses. Every year, the operation of warehouses becomes increasingly more complicated due to the increasing abundance of goods, thus the usual warehouse management strategies are no longer suitable. In order to ...
Warehouse Management enables us to analyze these components continually, so we can conserve effort, fill orders faster and more accurately, save space and reduce inventory. In this paper, a preliminary simplified layout of a warehouse using the quantities and sales data obtained from two frontrunners in shoe manufacturing is designed.
Warehouse management is an important part of supply chain management because warehouses can determine efficient logistics operations, and these logistics operations play a key role in maintaining ...
The different types of automated systems as well as a number of scientific results that are now known about such systems are discussed, followed by order picking systems from which individual packages can be picked. The first automated, high-bay, warehouses were introduced some 50 years ago. Since then, developments have continued at a rapid pace. Initially, automation was mainly focused on ...
The current study adds to the current body of knowledge in four different ways. First, the research adds to the body of knowledge on IoT technology by concentrating on technology adoption and its effect in the logistics and warehousing setting. The paper evaluates a comprehensive sample of 64 research publications from two independent databases.
Finance Research Letters, 58, 104687. 2 Saez, E., & Zucman, G. (2020). The rise of income and wealth inequality in America: Evidence from distributional ... warehouse workers from across the United States to complete a 98-question ... Paper 2005-17. Thanks to Brian Callaci for pointing us to this literature.