- Business Essentials
- Leadership & Management
- Credential of Leadership, Impact, and Management in Business (CLIMB)
- Entrepreneurship & Innovation
- Digital Transformation
- Finance & Accounting
- Business in Society
- For Organizations
- Support Portal
- Media Coverage
- Founding Donors
- Leadership Team
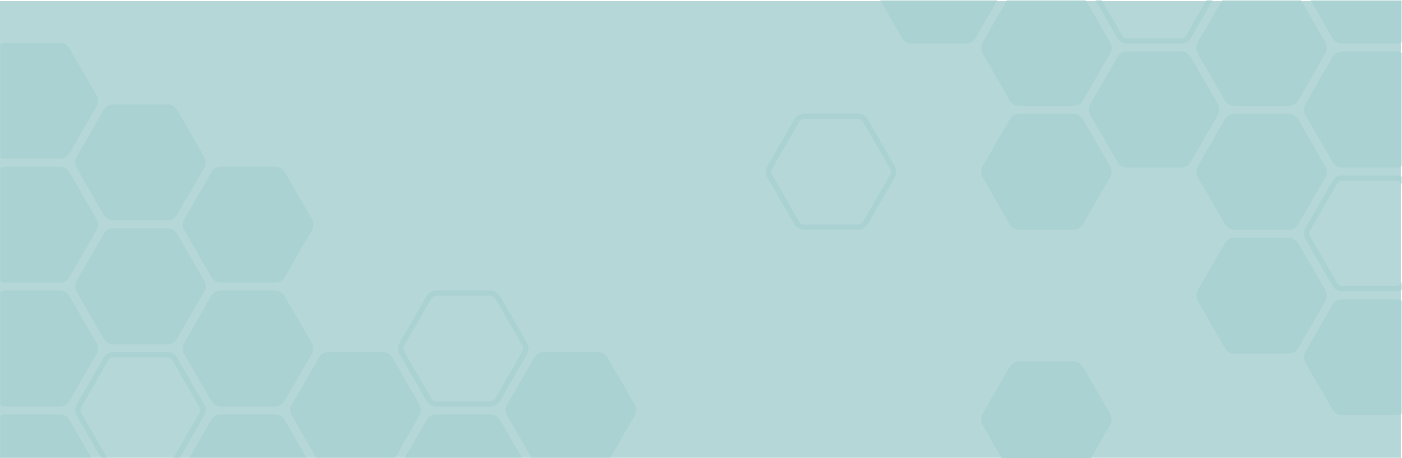
- Harvard Business School →
- HBS Online →
- Business Insights →
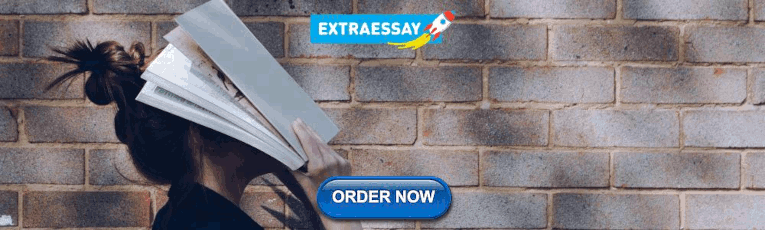
Business Insights
Harvard Business School Online's Business Insights Blog provides the career insights you need to achieve your goals and gain confidence in your business skills.
- Career Development
- Communication
- Decision-Making
- Earning Your MBA
- Negotiation
- News & Events
- Productivity
- Staff Spotlight
- Student Profiles
- Work-Life Balance
- AI Essentials for Business
- Alternative Investments
- Business Analytics
- Business Strategy
- Business and Climate Change
- Design Thinking and Innovation
- Digital Marketing Strategy
- Disruptive Strategy
- Economics for Managers
- Entrepreneurship Essentials
- Financial Accounting
- Global Business
- Launching Tech Ventures
- Leadership Principles
- Leadership, Ethics, and Corporate Accountability
- Leading with Finance
- Management Essentials
- Negotiation Mastery
- Organizational Leadership
- Power and Influence for Positive Impact
- Strategy Execution
- Sustainable Business Strategy
- Sustainable Investing
- Winning with Digital Platforms
What Is Creative Problem-Solving & Why Is It Important?
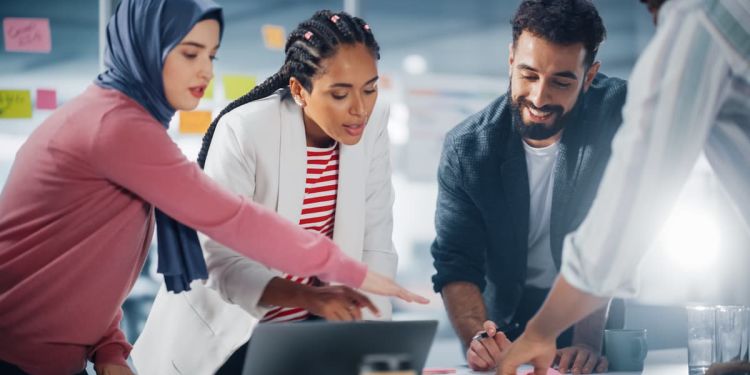
- 01 Feb 2022
One of the biggest hindrances to innovation is complacency—it can be more comfortable to do what you know than venture into the unknown. Business leaders can overcome this barrier by mobilizing creative team members and providing space to innovate.
There are several tools you can use to encourage creativity in the workplace. Creative problem-solving is one of them, which facilitates the development of innovative solutions to difficult problems.
Here’s an overview of creative problem-solving and why it’s important in business.
Access your free e-book today.
What Is Creative Problem-Solving?
Research is necessary when solving a problem. But there are situations where a problem’s specific cause is difficult to pinpoint. This can occur when there’s not enough time to narrow down the problem’s source or there are differing opinions about its root cause.
In such cases, you can use creative problem-solving , which allows you to explore potential solutions regardless of whether a problem has been defined.
Creative problem-solving is less structured than other innovation processes and encourages exploring open-ended solutions. It also focuses on developing new perspectives and fostering creativity in the workplace . Its benefits include:
- Finding creative solutions to complex problems : User research can insufficiently illustrate a situation’s complexity. While other innovation processes rely on this information, creative problem-solving can yield solutions without it.
- Adapting to change : Business is constantly changing, and business leaders need to adapt. Creative problem-solving helps overcome unforeseen challenges and find solutions to unconventional problems.
- Fueling innovation and growth : In addition to solutions, creative problem-solving can spark innovative ideas that drive company growth. These ideas can lead to new product lines, services, or a modified operations structure that improves efficiency.

Creative problem-solving is traditionally based on the following key principles :
1. Balance Divergent and Convergent Thinking
Creative problem-solving uses two primary tools to find solutions: divergence and convergence. Divergence generates ideas in response to a problem, while convergence narrows them down to a shortlist. It balances these two practices and turns ideas into concrete solutions.
2. Reframe Problems as Questions
By framing problems as questions, you shift from focusing on obstacles to solutions. This provides the freedom to brainstorm potential ideas.
3. Defer Judgment of Ideas
When brainstorming, it can be natural to reject or accept ideas right away. Yet, immediate judgments interfere with the idea generation process. Even ideas that seem implausible can turn into outstanding innovations upon further exploration and development.
4. Focus on "Yes, And" Instead of "No, But"
Using negative words like "no" discourages creative thinking. Instead, use positive language to build and maintain an environment that fosters the development of creative and innovative ideas.
Creative Problem-Solving and Design Thinking
Whereas creative problem-solving facilitates developing innovative ideas through a less structured workflow, design thinking takes a far more organized approach.
Design thinking is a human-centered, solutions-based process that fosters the ideation and development of solutions. In the online course Design Thinking and Innovation , Harvard Business School Dean Srikant Datar leverages a four-phase framework to explain design thinking.
The four stages are:

- Clarify: The clarification stage allows you to empathize with the user and identify problems. Observations and insights are informed by thorough research. Findings are then reframed as problem statements or questions.
- Ideate: Ideation is the process of coming up with innovative ideas. The divergence of ideas involved with creative problem-solving is a major focus.
- Develop: In the development stage, ideas evolve into experiments and tests. Ideas converge and are explored through prototyping and open critique.
- Implement: Implementation involves continuing to test and experiment to refine the solution and encourage its adoption.
Creative problem-solving primarily operates in the ideate phase of design thinking but can be applied to others. This is because design thinking is an iterative process that moves between the stages as ideas are generated and pursued. This is normal and encouraged, as innovation requires exploring multiple ideas.
Creative Problem-Solving Tools
While there are many useful tools in the creative problem-solving process, here are three you should know:
Creating a Problem Story
One way to innovate is by creating a story about a problem to understand how it affects users and what solutions best fit their needs. Here are the steps you need to take to use this tool properly.
1. Identify a UDP
Create a problem story to identify the undesired phenomena (UDP). For example, consider a company that produces printers that overheat. In this case, the UDP is "our printers overheat."
2. Move Forward in Time
To move forward in time, ask: “Why is this a problem?” For example, minor damage could be one result of the machines overheating. In more extreme cases, printers may catch fire. Don't be afraid to create multiple problem stories if you think of more than one UDP.
3. Move Backward in Time
To move backward in time, ask: “What caused this UDP?” If you can't identify the root problem, think about what typically causes the UDP to occur. For the overheating printers, overuse could be a cause.
Following the three-step framework above helps illustrate a clear problem story:
- The printer is overused.
- The printer overheats.
- The printer breaks down.
You can extend the problem story in either direction if you think of additional cause-and-effect relationships.
4. Break the Chains
By this point, you’ll have multiple UDP storylines. Take two that are similar and focus on breaking the chains connecting them. This can be accomplished through inversion or neutralization.
- Inversion: Inversion changes the relationship between two UDPs so the cause is the same but the effect is the opposite. For example, if the UDP is "the more X happens, the more likely Y is to happen," inversion changes the equation to "the more X happens, the less likely Y is to happen." Using the printer example, inversion would consider: "What if the more a printer is used, the less likely it’s going to overheat?" Innovation requires an open mind. Just because a solution initially seems unlikely doesn't mean it can't be pursued further or spark additional ideas.
- Neutralization: Neutralization completely eliminates the cause-and-effect relationship between X and Y. This changes the above equation to "the more or less X happens has no effect on Y." In the case of the printers, neutralization would rephrase the relationship to "the more or less a printer is used has no effect on whether it overheats."
Even if creating a problem story doesn't provide a solution, it can offer useful context to users’ problems and additional ideas to be explored. Given that divergence is one of the fundamental practices of creative problem-solving, it’s a good idea to incorporate it into each tool you use.
Brainstorming
Brainstorming is a tool that can be highly effective when guided by the iterative qualities of the design thinking process. It involves openly discussing and debating ideas and topics in a group setting. This facilitates idea generation and exploration as different team members consider the same concept from multiple perspectives.
Hosting brainstorming sessions can result in problems, such as groupthink or social loafing. To combat this, leverage a three-step brainstorming method involving divergence and convergence :
- Have each group member come up with as many ideas as possible and write them down to ensure the brainstorming session is productive.
- Continue the divergence of ideas by collectively sharing and exploring each idea as a group. The goal is to create a setting where new ideas are inspired by open discussion.
- Begin the convergence of ideas by narrowing them down to a few explorable options. There’s no "right number of ideas." Don't be afraid to consider exploring all of them, as long as you have the resources to do so.
Alternate Worlds
The alternate worlds tool is an empathetic approach to creative problem-solving. It encourages you to consider how someone in another world would approach your situation.
For example, if you’re concerned that the printers you produce overheat and catch fire, consider how a different industry would approach the problem. How would an automotive expert solve it? How would a firefighter?
Be creative as you consider and research alternate worlds. The purpose is not to nail down a solution right away but to continue the ideation process through diverging and exploring ideas.

Continue Developing Your Skills
Whether you’re an entrepreneur, marketer, or business leader, learning the ropes of design thinking can be an effective way to build your skills and foster creativity and innovation in any setting.
If you're ready to develop your design thinking and creative problem-solving skills, explore Design Thinking and Innovation , one of our online entrepreneurship and innovation courses. If you aren't sure which course is the right fit, download our free course flowchart to determine which best aligns with your goals.

About the Author
- Meet the OEB Speakers
- Industry Insights
- Community Resources
- The Conference
- Subscribe to Newsletter

Why Creativity Is the Most Important Skill in the World
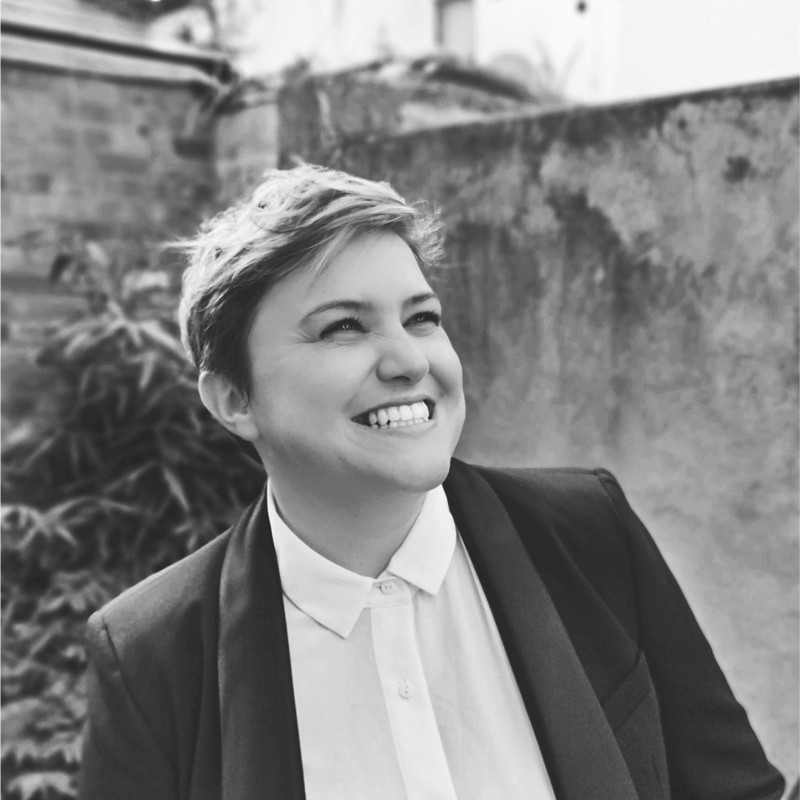
Recently at LinkedIn Learning , using the power of our Economic Graph , we determined the skills companies need most .
And there was a bit of a surprise that at the very top of the list for a second year was: creativity.
Technically, creativity is the most in-demand soft skill in the world, while Blockchain is the top hard skill. Hard skills, are those which apply to what people are working on (I.e. Blockchain) and only apply to that section of the workforce and doesn’t have the staying power a soft skill has.
Conversely, learning how to think more creatively will benefit you the rest of your career. And, macroeconomic trends suggest creativity will only become more important moving forward. Hence, it’s no stretch to say creativity is the single-most important skill in the world for all business professionals today to master. Why?
Let’s start with the what.
What it Means to be Creative: The Ability to Solve Problems with Relevance and Novelty
When many people think of creativity, they think of artists, graphic designers, writers, painters, etc.
But that’s not what it means to be creative, because creativity doesn’t mean artistry. Yes, an artist could be creative, but so could a software engineer, a mathematician, a salesperson or a CEO.
What does it actually mean to be creative? LinkedIn Learning Instructor Stefan Mumaw , who has authored six books on creativity, has this definition: “Creativity is problem-solving with relevance and novelty.”
Let’s break that definition down into its two parts:
- Relevancy : Relevancy means actually solving the problem. As in, the degree by which a problem is solved, as often we are not solving the problem itself.
- Novelty: Novelty is harder to judge, but it’s when you are able to solve a problem in an original way. A way that isn’t what’s expected or has been done before.
Putting it together, creativity is really just solving problems in original ways.
Mumaw also believes creativity isn’t strictly innate. Yes, like anything, some people are naturally more creative than others. But, by putting the time in, you can learn how to be more creative.
“Creativity is a skill and any skill that you can undertake, the byproduct to it being a skill, is that you can get better at it,” Mumaw said in his course, Creativity Bootcamp . “And we’ve never really thought of creativity as being something that we can get better at. But you can.”
Why Creativity Is So Important Today: Process-Driven Jobs Are Going Away
Using that definition of what creativity is, why is it the most important skill for professionals today?
It really comes down to commoditisation. Today, basically anything that can be automated has been automated or soon will be automated, which cuts down on a lot of process-orientated tasks.
Use the example of the news industry. In the old days of newspapers, it took teams of people to set the layout of the paper, create the printing press, print the paper and then deliver it to subscribers. Today, with most news dispersed electronically, virtually all of that process-driven work is automated.
What’s left? Journalists who can tell news stories that cut through the noise and connect with people. That takes creativity; i.e. a relevant and novel solution – what are the stories that aren’t being told already that people want to hear?
That same phenomenon is happening across every industry and every function. Software companies don’t just want someone who can write code, they want someone who can dream up new softwares to fix old problems. Companies don’t want business analysts who just crunch numbers; they want analysts who can think of creative solutions based off what the numbers are telling them.
What This All Means to You: There’s No Better Investment You Can Make Today Than Strengthening Your Creative Skills
2020 has been a year of change as people adapted to new ways of working or saw their employment circumstances change due to the global pandemic. Many have invested time developing new skills to help get them through, particularly soft skills which are highly valued by companies today and have grown in importance in recent years. Skills like creativity are in high demand. Learning new skills or developing existing ones can help people be great at their job today, and open doors to new opportunities in the future. It can also help people improve their confidence which is particularly beneficial to anyone who has been out of the workplace for a while.
Are you looking to strengthen your creativity skills? These LinkedIn Learning courses can help:
- Creativity Bootcamp with Stefan Mumaw
- Creative Exercises to Spark Original Thinking with Amy Wynne
- Banish Your Inner Critic to Unleash Creativity with Denise Jacobs
Written for OEB20 by Nicola M. Doherty , LinkedIn Learning
Leave a Reply Cancel Reply
Your email address will not be published.
Save my name, email, and website in this browser for the next time I comment.
An exploration of how creativity, functionality, and aesthetics are related in design
- Original Paper
- Open access
- Published: 05 May 2021
- Volume 32 , pages 289–307, ( 2021 )
Cite this article
You have full access to this open access article
- Ji Han ORCID: orcid.org/0000-0003-3240-4942 1 ,
- Hannah Forbes 1 &
- Dirk Schaefer 1
13k Accesses
43 Citations
Explore all metrics
Creativity is considered to have a significant impact on the design process and its outcomes, while aesthetics and functionality are considered key characteristics of products. A relationship between creativity, aesthetics and functionality is, therefore, often assumed, however, researchers view the relations between creativity, functionality and aesthetics differently. In this paper, the authors present first evidence that novelty, usefulness and surprise are the core elements of design creativity. The aim of this research is the exploration of the relations between functionality, aesthetics, novelty, usefulness, surprise, and overall creativity, by means of an experimental case study involving design experts evaluating forty-five design samples. Statistical analysis has been conducted to investigate and understand these relations. The results obtained indicate that aesthetics has a significant positive relationship with creativity but that functionality does not have a statistically significant relationship with creativity in general. Further analysis confirms that design creativity is strongly and positively related to novelty and surprise, but not significantly related to usefulness. In addition, high correlation coefficient values have revealed that creativity, novelty and surprise are perceived as the same dimension as are functionality and usefulness. This paper may be of interest to researchers, practitioners, and educators in the broader realm of design, including industrial design, creativity in design, engineering design, design innovation, product design and new product development. It provides new insights into how creativity is perceived within the field and offers a new point of view on creativity and its dimensions for the community to meditate and to debate.
Similar content being viewed by others
Introduction to Design Science Research
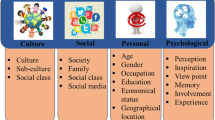
Package design as a branding tool in the cosmetic industry: consumers’ perception vs. reality
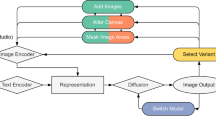
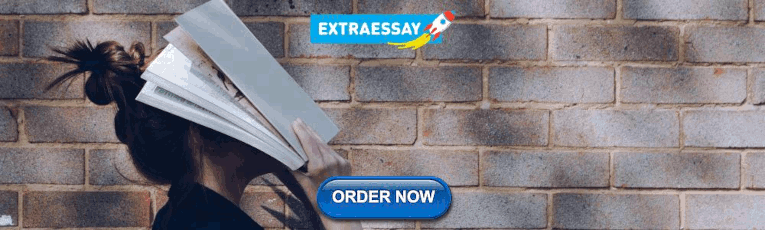
AI art in architecture
Avoid common mistakes on your manuscript.
1 Introduction
Creativity is considered an essential element of human intelligence (Cross 2011 ). The human capacity for creativity has evolved over thousands of years driven by biological and social factors (Pringle 2013 ). The outputs of creativity, emerging from combinations of essential mental capabilities, are the results from long periods of work with several mini-breakthroughs (Childs 2018 ). These outputs, in forms of ideas, objects and actions, are conceived to be novel, useful, and of good quality (Carruthers 2011 ). In this study, the authors focus on creative products and the outcomes of creative processes or actions.
Creativity plays a significant role in the early phases of design (also known as the fuzzy front end) and benefits business performance in the long run (Sarkar and Chakrabarti 2011 ; Han et al. 2018a ). It has been widely considered a fundamental part of the ideation phase of the design process, as innovative and successful designs often originate from creative concepts (Thompson and Lordan 1999 ; Chiu and Shu 2012 ; Toh and Miller 2015 ; Crilly and Moroşanu Firth 2019 ). Creative conceptual design is therefore considered central to innovative product development (Shai et al. 2009 ) and while creativity is a crucial measure of design effectiveness, customers may not explicitly indicate creativity as a requirement while seeking creative designs (Chiu and Shu, 2012 ).
Functionality and aesthetics are considered the core characteristics and success factors of products (Rahman et al. 2010 ; Cropley and Kaufman 2019 ). Functionality refers to the opportunities for action(s) which are afforded by a product, which enables consumer engagements (Ziamou and Ratneshwar 2003 ), and is considered the performance of a product in this paper (Cropley and Kaufman 2019 ). Design researchers generally agree that aesthetics refers to two concepts: the senses, particularly vision, represented by a product; and the specific cognitive reaction or response to a product (Crilly et al. 2004 ; Sonderegger and Sauer 2010 ). In this paper, aesthetics refers to the objective design features presented by a product in general, such as form and colour. In other words, it involves the visual and ergonomic appeal of a product to a user (Cropley and Kaufman 2019 ).
Several studies have investigated how aesthetics and functionality are related to creativity . Christensen and Ball ( 2016 ) claim that aesthetics, functionality and originality are the core dimensions of design creativity, but Sarkar and Chakrabarti ( 2011 ) conclude that novelty and usefulness are the core creativity components. Cropley and Cropley ( 2011 ) indicate that creativity involves aesthetic properties but also includes novelty and effectiveness. However, Christensen et al. ( 2015 ) indicate that aesthetics and creativity are distinct factors in product evaluation, but they both positively affect consumer willingness-to-pay. Acar et al. ( 2017 ) suggests aesthetics have little influence in creative product evaluation. Furthermore, in a study conducted by Kreitler and Casakin ( 2009 ), aesthetics and functionality are found to have no significant correlations with creativity. Bao et al. ( 2018 ) also show that aesthetics and functionality are not significantly correlated to creativity, but that the two dimensions themselves are highly correlated.
Innovation and creativity are often simultaneously explored by the engineering design community. Studies include those conducted by Chakrabarti ( 2013 ), Yannou ( 2013 ), and Crilly and Moroşanu Firth ( 2019 ). Innovation is defined as the development and intentional implementation of new and useful ideas (Bledow et al. 2009 ; Shalley et al. 2015 ), while innovative product design is significant in ensuring product success (Moon et al. 2015 ). Creativity, which signals the generation of ideas, is considered a prerequisite for innovation in design (Sarkar and Chakrabarti 2011 ; Shalley et al. 2015 ; Crilly and Moroşanu Firth 2019 ). Successful innovation depends on the generation of creative ideas, but merely generating creative ideas is insufficient for innovation (Starkey et al. 2016 ). However, a creative product is often considered the starting point for product innovation (Cropley et al. 2011 ). Aesthetics and functionality have been identified as the core dimensions of product design (Homburg et al. 2015 ), and the main aspects used by designers for product innovation (Shiu 2017 ). Goode et al. ( 2013 ) indicate that a product’s visual appearance (aesthetics) is the first thing noticed by consumers in an innovation. Moon et al. ( 2015 ) claim that improving the functionality of a product leads to innovative product design. This shows that creativity , aesthetics and functionality all relate to innovation in some way.
Aesthetics , functionality , and creativity relate to one another to some extent in product design, but it appears there exist various different views on this relationship. Furthermore, few studies have investigated how the core dimensions of creativity , such as novelty and usefulness , relate to aesthetics and functionality , which could provide further insights. This study is an extension and new exploration of prior research conducted by Han et al. ( 2019a ). It involves a more comprehensive literature review, a new case study with more types of samples, a larger sample size and more expert evaluators aiming to yield more general findings. The authors of this paper aim to explore how aesthetics , functionality , creativity and the core components of creativity relate to one another in the product design context, to gain new insights into their relationships. A case study is conducted by means of expert evaluation, and followed by statistical analysis. The results obtained provide new insights into design creativity and its interpretation, allowing to reconsider its definition and assessment. Design researchers, educators, and practitioners alike may benefit from this, both in their endeavours to develop new and innovative products that succeed in the market, and in the context of a scholarly debate.
In the following section, relevant work on aesthetics and functionality is reviewed. In Sects. 3 and 4 , commonly used definitions and assessments of design creativity are investigated, respectively. A case study exploring the relations between aesthetics , functionality and design creativity , including its core dimensions, is provided Sect. 5 , and followed by discussion in Sect. 6 . The paper ends with a presentation of the key findings and conclusions drawn from this work.
2 Aesthetics and functionality in the design context
In real design, aesthetics play a major role (Reich 1993 ). The term aesthetics commonly refers to product aesthetics, which relates to ‘what the product presents to the senses (especially vision)’, and aesthetic experience, which relates to ‘the perception of how pleasing (or otherwise) the process of regarding an object is’ (Crilly et al. 2004 ). A perception of aesthetics is one of the first responses from consumers to a product, which is closely associated with visual information (Ulrich 2011 ). It, therefore, has a significant impact on the perception of a product as a whole (Mata et al. 2018 ). Aesthetics are particularly vital for a product that only slightly differs in functionality and price from its competitors (Moon et al. 2015 ; Lugo et al. 2016 ; Mata et al. 2018 ). It is often used to provide customers with an additional value proposition, in a competitive market of products possessing similar functionality (Perez Mata et al. 2017 ). Thus, aesthetics are considered a key factor in customers’ buying decision and satisfaction (Orsborn et al. 2009 ). Furthermore, it facilitates the acceptance of new technology and its success in society (Haug 2016 ; Eggink and Snippert 2017 ). For example, the success of technological products produced by Apple and Dyson are considered to be a result of their aesthetic appeal to customers. To clarify the discussion to this point, in this paper, aesthetics refers to product aesthetics in general and not aesthetic experience.
Functionality reflects the consumers’ perceptions of the ability of a product for fulfilling its purpose (Homburg et al. 2015 ). Srinivasan et al. ( 2012 ) suggest that functionality is more important than aesthetics as a product design element for consumers, and has a stronger impact on consumer satisfaction. It arises from product features and delivers specific benefits through usage (Srinivasan et al. 2012 ). Functional attributes are described as the actions required by a design problem, or the actions provided by the problem’s solutions (Chakrabarti and Bligh 2001 ). As explained in Sect. 1 , in this paper functionality refers to the performance of a product. The usefulness of a product refers to what it can do according to its functionality and how well users can explore the functionality offered (Rabiser et al. 2012 ). Usefulness can be recognized easily in functional products, but is more challenging to see in non-functional products (such as movies and arts) (Moldovan et al. 2011 ). As a consequence, functionality and usefulness are considered independent dimensions in this paper.
A product’s functionality fulfils customer needs by problem solving and prevention, while aesthetics produce experiential pleasures by viewing, interaction, consumption, and sense of ownership (Rahman et al. 2010 ). Aesthetics and functionality are often used as assessment aspects in benchmarking activities for investigating competitors’ products (Toh and Miller 2013 ). Sylcott et al. ( 2013 ) indicate that aesthetics are weighted less heavily than functionality in evaluating a design, while Haug ( 2016 ) argues that aesthetics are more important than functionality for noticing and appreciating a product. Hagtvedt and Patrick ( 2014 ) suggest a balance between aesthetics and functionality is needed in product design. Reich ( 1993 ) points out that functionality and aesthetic appeal may have conflicts. Alipour et al. ( 2017 ) indicate that function is a constructive goal for designers, whereas aesthetics is a detrimental goal. In addition, many researchers have argued that functionality is not assessable without usage or consumption, but Hoegg and Alba ( 2011 ) and Radford and Bloch ( 2011 ) hold the view that consumers often assess functionalities of products from just seeing them (Homburg et al. 2015 ). This usually occurs while consumers are purchasing new products, especially when doing so online. For example, a product with a highly machined appearance provides a strong cue indicating the technical robustness of the product (Radford and Bloch 2011 ). This indicates that aesthetics and functionality are correlated in design, which is in line with the findings of Bao et al. ( 2018 ).
3 Definitions of design creativity
Creativity is considered a crucial element in new product design and development. It is an integral part of design, contributing to problem-solving and innovative product development, occurring between the problem and solution space (Demirkan and Afacan 2012 ; Hsiao and MacDonald 2013 ). It is also claimed that creativity is a prerequisite for the generation of ideas for breakthrough products (Taura and Nagai 2017 ).
In addition to the design context, creativity is also a significant topic in psychology and cognitive science. Researchers from these areas have provided many definitions of creativity at various levels of scope and complexity. For example, ‘the act of making new relationships from old ideas’ (Koestler 1964 ); ‘the process by which something so judged (to be creative) is produced’ (Amabile 1983 ); ‘the ability to produce work that is both novel (i.e. original, unexpected) and appropriate (i.e., useful, adaptive concerning task constraints)’ (Sternberg and Lubart 1998 ); ‘the production of novel, useful products’ (Mumford 2003 ); ‘a response to the continual innovation and resourcefulness that have become necessary for economic survival’ (Craft 2003 ); ‘the ability to come up with ideas or artefacts that are new, surprising, and valuable’ (Boden 2004 ); ‘creativity requires both originality and effectiveness’ (Runco and Jaeger 2012 ); ‘one that is novel and produced intentionally’ (Weisberg 2015 ); and ‘discovery of new possibility and bringing it into being’ (Martin and Wilson 2017 ). The examples demonstrate the fierce and long-term debate surrounding the definition of creativity , but also consistently present novelty and usefulness as core elements of creativity .
To explore and understand how design researchers describe and define creativity , specifically in the context of design research, the authors reviewed more than twenty-five relevant articles published over the course of the past fifteen years in top-tier design research journals, including Research in Engineering Design , Design Studies , Journal of Engineering Design , Journal of Mechanical Design , and International Journal of Design Creativity and Innovation . An overview of the results is depicted in Table 1 .
Similar to researchers from psychology and cognitive science, design researchers have used various definitions of creativity . Demirkan and Afacan ( 2012 ), who conduct research in design creativity, claim that the nature of creativity is so complex that no single definition could encompass and identify this concept. Rodgers and Jones ( 2017 ) show that it is challenging for design students and tutors to define and conceptualise creativity. As indicated in Table 1 , novelty and usefulness are considered to be the two key elements of defining creativity in design. This is in line with the common definition of design creativity provided by Sarkar and Chakrabarti ( 2008 ).
Surprise is commonly defined as the violation of expectations, it is often interchangeable with the term unexpectedness or unexpected (Becattini et al. 2017 ). It is considered to be a game-changer in creative product design, triggering attention and curiosity (Becattini et al. 2020 ). Aiming to design products that (positively) surprise the customer could significantly increase individually perceived creativity (Gotzsch 2017 ). Although many researchers use novelty and usefulness to describe design creativity, others argue that it is necessary to augment these two criteria by adding surprise to measure the unexpectedness of a design (Gero et al. 2019 ). A number of researchers have claimed surprise to be a nuance or an element of novelty, while many others indicate that surprise might be an independent dimension of creativity (Becattini et al. 2017 ). For example, Chiu and Shu ( 2012 ) consider surprise as a degree of novelty; Zheng and Miller ( 2020 ) describe surprise as an indicator of novelty; and Koronis et al. ( 2019 ) define novelty as the ‘surprisingness’ and originality of a concept. However, Boden ( 2004 ) considers surprise as the essence of creativity, along with newness and value. Nguyen and Shanks ( 2009 ) indicate surprise, novelty and value are the core characteristics of creativity. Simonton ( 2012 ) claim that a creative idea is novel, useful and surprising. Moreover, Maher et al. ( 2013 ) indicate that surprise stems from violating expectations, but novelty does not necessarily imply a violation of expectations in a space of expected designs. Grace et al. ( 2015 ) describe surprise as the notion of evoking astonishment and unexpectedness that is not included in novelty. Acar et al. ( 2017 ) even argue that surprise should be the second factor of creativity, which is more important than usefulness, besides novelty. As shown in Table 1 , surprise or surprising (alternatively unexpectedness or unexpected ) have been used increasingly as the third element to describe creativity in design research in recent years. In this study, surprise is considered a separate dimension that does not relate to novelty . Therefore, we suggest that novelty , usefulness and surprise may be the three key elements for defining design creativity based on the research conducted.
As depicted in Table 1 , not one of the many creativity definitions reviewed directly include the term aesthetics . Only Valgeirsdottir et al. ( 2015 ) have employed ‘functionality’ in describing creativity , where creativity is described as ‘the process of developing new and original ideas that are somehow appropriate for a specific function, or occasion, thus bringing value to prospective users or adopters.’ However, a few researchers have involved aesthetics and functionality in describing design creativity . For example, Cropley and Cropley ( 2005 ) propose a four-dimensional model to define creativity for engineering products, which involves relevance and effectiveness, novelty, elegance (aesthetics), and generalizability; and Acuna and Sosa ( 2011 ) suggest the two basic elements for defining creativity are novelty and functionality. To provide more insights from another perspective, criteria used for creativity assessments are investigated in the next section. To be more specific, whether functionality and aesthetics are included in assessing creativity in design is explored.
4 Assessments of design creativity
Creativity assessment plays a vital role in selecting creative ideas for products, as well as identifying better designers and inventors (Sarkar and Chakrabarti 2011 ). It also ensures an understanding of creativity and its measures (Jagtap 2019 ). Human judgement-based criteria for creativity assessment are amongst those most often used in existing literature. This section reviews several popular criteria-based creativity assessment methods used in design research.
Creative Product Semantic Scale (CPSS) is a design creativity measurement approach for individual products proposed by O'Quin and Besemer ( 1989 ). It involves three conceptual dimensions which are resolution, novelty, and elaboration and synthesis. Resolution refers to valuable, useful and logical. Novelty includes original, germinal and surprising. Elaboration and synthesis represent complex, understandable, well-crafted, organic, and elegant. Chulvi et al. ( 2012 ) presents the use of an adapted questionnaire-based CPSS, which mainly focuses on novelty and utility (resolution), for the particular needs of the research. In the same line, García-García et al. ( 2017 ) employ novelty and style for their adapted CPSS questionnaire.
Novelty, quality, quantity and variety, proposed by Shah et al. ( 2003 ), are often used for evaluating the effectiveness of an idea generation method. Novelty refers to the newness of an idea to an individual or the history. Quality indicates the feasibility of an idea. Quantity represents the total number of ideas generated by an individual or a group. Variety shows the number of categories of the ideas generated. Novelty and quality are associated with the assessment of the degree of creativity of an idea generated. Similarly, Plucker and Makel ( 2010 ) employ originality, flexibility, fluency and elaborations, and Lopez et al. ( 2011 ) use novelty, feasibility, quantity and variety for creativity assessments.
Horn and Salvendy ( 2009 ) employ novelty, affect and importance to measure product design creativity. Novelty is defined as the newness and uniqueness of a product. Affect is described as the product’s ability to attract, delight and stimulate the product’s evaluator or user. Importance refers to the suitability and criticality of the product. The three dimensions of product design creativity have shown a connection with consumer satisfaction.
Novelty and usefulness are used in a creativity assessment method, proposed by Sarkar and Chakrabarti ( 2011 ), for evaluating a product or an idea. In this method, novelty refers to something new and original, which is assessed by employing the Function-Behaviour-Structure (FBS) model and the SAPPhIRE model (Chakrabarti et al. 2005 ). Function plays an important role in this novelty assessment, as it is the core element used to differentiate novel products from others. Usefulness refers to social value, which is measured by utilizing Eq. ( 1 ). The overall creativity of a product can then be calculated by Eq. ( 2 ). It has been suggested that this assessment method may better reflect designers’ collective and intuitive notion of design creativity.
Novelty, usefulness and cohesiveness are used by Chiu and Shu ( 2012 ) to measure design creativity of individual concepts. Novelty involves originality, newness and surprise; usefulness includes value and appropriateness; and cohesiveness involves wholeness, elaboration, detail, clarity and style.
Demirkan and Afacan ( 2012 ) propose three factors, composed of 31 items, for evaluating creativity of artefacts, particularly in the context of design education. Factor 1 is the novelty and affective characteristics of the artefact. It is associated with the shape of design, involving items such as novel, unusual, different, new, and shape. Factor 2 is the elaboration characteristics of the artefacts, which is related to harmony of design elements as well as geometric and figure-ground relations. It involves items such as geometric, harmony, balanced, and integrated. Factor 3 involves items such as order, number, repetition, unity and rhythm, which is known as design principles.
Novelty, usefulness, aesthetics, and complexity are the four criteria used by Lee et al. ( 2015 ) for measuring design creativity. Novelty and usefulness refer to the creativity measurement dimensions often used in the design domain. Aesthetics indicates the degree of how aesthetically appealing a design is. Complexity refers to the level of difficulty presented by a product, which is specifically related to the complex forms generated in parametric design.
Originality, functionality and aesthetics are proposed by Christensen and Ball ( 2016 ) for creativity assessment. Originality assessment is tied closely to the birth of ideas, while functionality assessment focuses on the life of ideas. Assessments of aesthetics rest on affective and cognitive aspects relating to object perceptions.
Novelty and quality are used by Srinivasan et al. ( 2018 ) for evaluating creative design concepts. In their approach, novelty refers to the exploration of new solution spaces, while quality measures the fulfilment of the requirement.
Starkey et al. ( 2019 ) employ usefulness and uniqueness for evaluating design creativity. Uniqueness is based on perceptions of surprise and originality, while usefulness is founded on perceptions of utility, logic, value and understandable of the idea.
A summary of the design creativity assessment criteria discussed is shown in Table 2 . Several sets of human judgement-based criteria have been proposed to assess design creativity, of which novelty and usefulness are often used. This is in line with definitions of creativity in the design context illustrated in Sec. 3 Novelty generally refers to originality and newness. Many researchers have included quality as another element in evaluating design creativity. Girotra et al. ( 2010 ) have proposed four variables to govern the quality of ideas generated. These involve the average quality, the number, the variance in the quality, and the capability to discern the quality of ideas produced. A product, which is considered useful, delivers quality, value, and feasibility by fulfilling requirements. Usefulness therefore refers to quality, feasibility, and value. He and Luo ( 2017 ) have theoretically reasoned and empirically found that the novelty profile of an inventive design can influence its potential usefulness value in a non-intuitive manner through investigating 3.9 million patents. However, in this paper, novelty and usefulness are considered independent variables, which echoes the view presented in most of design creativity studies.
Functionality and aesthetics are used more frequently in design creativity assessment than in defining creativity . For instance, Christensen and Ball ( 2016 ) have included both functionality and aesthetics as the core assessment dimensions. ‘Function’ has been considered a significant element in assessing novelty in the approach proposed by Sarkar and Chakrabarti ( 2011 ). The CPSS approach proposed by O'Quin and Besemer ( 1989 ) involves aspects of aesthetics, such as elegance. The criteria used by Lee et al. ( 2015 ) and García-García et al. ( 2017 ) includes aesthetics and style, respectively. The set of factors considered by Demirkan and Afacan ( 2012 ) also involves items associated with aesthetics, such as appeal and delight, but these factors are used to assess paintings rather than products or processes. However, surprise is used less frequently for assessing rather than describing creativity in a direct manner. Some researchers, such as O'Quin and Besemer ( 1989 ), Chiu and Shu ( 2012 ), and Starkey et al. ( 2019 ) have included surprise in novelty . This is in contrary to the findings in Sec. 3 of which novelty and surprise are considered independent variables.
Throughout Sects. 3 , 4 , the authors investigated how creativity is defined and assessed in the context of design. Although novelty and usefulness are the core dimensions used in both assessing and defining creativity , surprise has been used more often as the third dimension of creativity in recent years. Therefore, it can be deduced that novelty , usefulness , and surprise are the three core elements of design creativity . Functionality and aesthetics are also employed in both defining and assessing design creativity , but less frequently. However, some researchers, such as Kreitler and Casakin ( 2009 ) and Bao et al. ( 2018 ) have indicated that aesthetics and functionality have no significant relations with creativity . Furthermore, few studies have explored how aesthetics and functionality are related to the core dimensions of creativity , such as novelty and usefulness . A case study addressing these points is provided in the following section.
5 Case study
A case study has been designed to explore the relationships, in the product design context, between aesthetics , functionality , and creativity and its core dimensions. This section first describes the methodology used to conduct the case study, and then presents the results with associated analyses and interpretations.
5.1 Methodology of the case study
Three types of products were selected to be observation samples: vases, chairs and lamps, which represent different degrees of functional and aesthetic attributes. In this study, vases are considered highly aesthetic products, which are essentially decorative objects that have clear design constraints (Reed 2013 ). They are often associated with aesthetics and aesthetic measures in design research. For example, vases are used by Perez Mata et al. ( 2017 ) to study the relationships between aesthetic features, perceptions, ownership, and consumer background. Conversely, chairs are ideal tangible products representing both functional and aesthetic values in design (Cropley and Kaufman 2019 ). They are often employed in design research, such as by Hung and Chen ( 2012 ) and Cropley and Kaufman ( 2019 ). Finally, lamps are regarded as products with high functionality, which involves broad technological-focused problem-solving processes. Gupta et al. ( 2017 ) indicate that lighting (lamp) industries have shifted their focus from aesthetics to functionality. Lamps are becoming more and more so-called ‘smart’ products, alternatively functional products, that involve functions such as intelligent control, speaker, wireless charger, and flexible structure.
Chairs and lamps are frequently used in design creativity research, of which chairs are used more extensively. For example, Besemer ( 1998 ) has employed three chairs to demonstrate the empirical use of Creative Product Semantic Scale, Yu and Nickerson ( 2011 ) have used chairs to investigate crowd creativity, Christensen et al. ( 2015 ) have used lamps to investigate how creativity and beauty affect consumer willingness-to-pay for a product, and Horn and Salvendy ( 2009 ) have employed both chairs and lamps to measure consumers’ perceptions of product creativity. In comparison, vases are used less frequently in design creativity studies, as they may have a relatively simple functionality in general.
In this case study, novelty , usefulness , surprise , aesthetics and functionality are the five factors to be investigated, of which novelty , usefulness and surprise are the three key elements of design creativity . The five factors are employed to measure the relationships among design creativity , aesthetics and functionality , using novelty , usefulness and surprise to represent design creativity . In addition, overall creativity is considered the sixth factor, which is employed to explore its relationship with novelty , usefulness and surprise , as well as its direct relationship with aesthetics and functionality . According to the preceding, the definitions of the six factors used in this study are presented in Table 3 , where the definitions of aesthetics and functionality are in line with the descriptions in the study conducted by Cropley and Kaufman ( 2019 ).
The vase, chair and lamp design samples used in this case study were chosen from the winners of international design competitions, such as the iF and Red Dot design awards. For each type of product, fifteen specific samples were selected: fifteen vases, fifteen chairs, and fifteen lamps. Random purposive sampling was used to select the samples. The use of design competition winners in design research has shown positive results in several recent studies. For example, Wang ( 2016 ) has proposed a set of winning formulas for metaphor design; Yilmaz et al. ( 2016 ) have developed 77 evidence-based design heuristics for supporting early conceptual design; Hölttä-Otto et al. ( 2018 ) have explored the success rates of innovative products launched by new ventures and established firms; and Han et al. ( 2019b ) have identified three approaches for producing combinational creative ideas, by employing and analysing design competition-winning products in their studies. Furthermore, aesthetics and functionality are the core evaluation and judging criteria of these design competitions or awards. Wang and Chan ( 2010 ) have indicated that these design competitions are often creativity-oriented. It is therefore proven to be reliable to use products selected from the winners of the design competitions as samples.
Expert evaluation (please refer to Sec. 5.2 for details) is used in this case study as the method to investigate the relationships among aesthetics , functionality , and creativity ( novelty , usefulness , and surprise ). Employing experts for evaluation in design creativity research has become a dominant approach, for example, the studies conducted by Sarkar and Chakrabarti ( 2011 ), Han et al. ( 2018b ), and Cropley and Kaufman ( 2019 ). As a consequence, it is suitable and reliable to employ experts for evaluating the design samples. Details of the design samples, including names, descriptions and images, are provided to the expert for evaluation, as shown in Fig. 1 . According to the information provided, the experts are asked to evaluate the design samples using the six factors discussed in this paper: aesthetics , functionality , novelty , usefulness , surprise and overall creativity . Instructions of the interpretations of the six factors are provided to the experts prior to starting the evaluation. However, the experts are not informed of the source of the samples used in the case study to avoid biased evaluations. The evaluation involves a 7-point Likert rating scale, ranging from 1 (‘poor’) through 4 (‘moderate’) and up to 7 (‘excellent’). The experts rated the six factors using the 7-point rating scale respectively for each design sample in the evaluation.
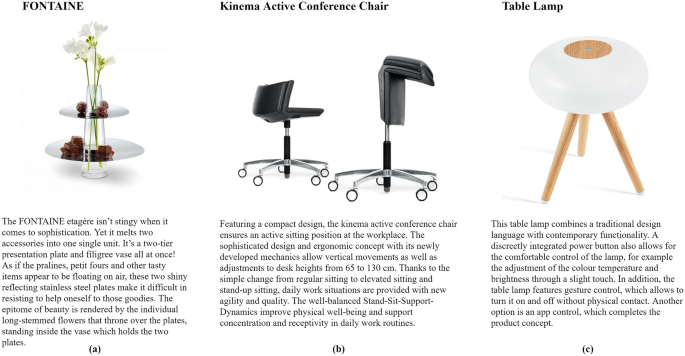
Representative examples of designs employed in the case study: ( a ) vase, ( b ) chair, ( c ) lamp
Further examples of vases used are provided in Fig. 2 , demonstrating a glimpse of the samples selected for this case study. In Fig. 2 , Two Way Watering Pot (d) is a decorative vase that can be used as a watering pot offering two kinds of watering approaches for plant leaves and roots, respectively; Segment (e) involves two portions that can be attached to form a complete vase, as well as used separately as two vases; and Rosenthal Squall (f) is a vase reminisces about a whirlwind. Besides, FONTAINE, as shown in Fig. 1 a, is a combination of a two-tier plate and a filigree vase. It shows that the samples selected have differentiations in both aesthetics and functionality , even in products with simple functional attributes.
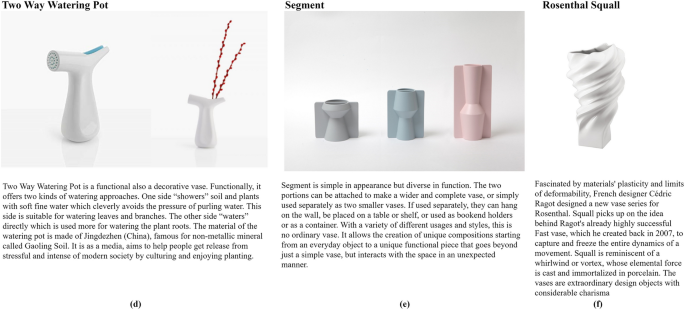
Further examples of vases employed in the case study
5.2 Case study results
Twenty design experts volunteered to participate in the evaluation, mainly for intrinsic motivations, such as personal interest, enjoyment, or inherent value of and learning from the activity. Nineteen provided valid results, which include ten males and nine females. Their mean age is 32.58 years [Standard Deviation (SD) = 7.69] and their mean years of design experience is 10.26 (SD = 6.88). They signed up with standard ethical protocols concerning the use of data. Although at a first glance the number of experts involved may appear to be low, Lai et al. ( 2006 ) indicate that there is no common agreement as to the minimum number of experts required for such evaluations. Furthermore, Achiche et al. ( 2013 ) indicate that the number of experts needed in an evaluation is far less compared with employing general people. Many design studies involving expert evaluations have employed a low number of experts, for example, less than six experts have participated in the studies conducted by Doré et al. ( 2007 ), Charyton and Merrill ( 2009 ), and Achiche et al. ( 2013 ), respectively. Therefore, the nineteen experts employed in this case study can be considered a sufficient number.
The forty-five design samples (fifteen vases, fifteen chairs, and fifteen lamps) were provided to the experts for evaluation using the evaluation approach discussed in the previous section. The samples were evaluated individually by each of experts, based on their experience and knowledge, measuring the following six factors: functionality , aesthetics , novelty , usefulness , surprise and overall creativity .
A Cronbach’s alpha test was conducted to indicate the internal consistency of the rating scores for the conducted case study. As shown in Table 4 , the Cronbach’s alpha of the ratings of the vase, chair and lamp examples are 0.964, 0.968 and 0.966 respectively, which suggests excellent internal consistency. The overall Cronbach’s alpha is 0.986, which also indicates excellent overall internal consistency. The results of the Cronbach’s alpha test therefore suggest that the expert evaluation conducted possesses good reliability. The mean values of the six factors of each sample for vases, chairs, and lamps, rated by the design experts, are calculated for further analysis, as shown in Tables 5 , 6 , 7 , respectively. For instance, vase sample 1 has a mean creativity value of 4.63 with a standard deviation (SD) of 1.35, as shown in Table 5 . Its mean functionality , aesthetics , novelty , usefulness and surprise values are 3.53 (SD = 1.39), 4.79 (SD = 1.20), 4.95 (SD = 1.39), 3.79 (SD = 1.15), and 4.32 (SD = 1.22), respectively.
Likert scales are often considered ordinal data, and therefore Spearman correlation tests are employed to explore the directions of relations and the strengths existing among the six factors ( functionality , aesthetics , novelty , usefulness , surprise and creativity ). The tests are conducted employing the mean factor scores of the vase, chair, and lamp samples, respectively, as described in the preceding section. The results of the Spearman correlation tests for vases, chairs and lamps are shown in Tables 8 , 9 , 10 respectively. In the tables, a positive Spearman correlation coefficient ( r s ) indicates a positive monotonic correlation where the dependent parameter tends to increase while the independent parameter increases. A negative Spearman correlation coefficient ( r s ) suggests a negative monotonic correlation where the dependent parameter tends to decrease when the independent parameter increases. A zero Spearman correlation coefficient ( r s ) indicates a non-monotonic correlation, where there are no tendencies for the dependant parameter to increase or decrease while the independent parameter increases. A higher magnitude of the Spearman correlation coefficient ( r s ) suggests a stronger correlation between the dependant and independent parameters, and vice versa. There is a statistically significant correlation between two parameters while the p value *p < 0.05 , and a statistically highly significant when **p < 0.01 . The interpretation of the Spearman correlation coefficients ( r s ) is based on the guidance provided by Dancey and Reidy ( 2014 ), of which 0 < │ r s │ < 0.3 indicates a weak correlation, 0.3 ≤ │ r s │ < 0.7 suggests a moderate correlation, and 0.7 ≤ │ r s │ < 1 refers to a strong correlation.
For the vase samples, the correlation coefficient between functionality and aesthetics is − 0.182, which indicates a negative and weak correlation with no statistical significances, as shown in Table 8 . In addition, there are no statistically significant correlations between functionality and creativity ( r s = 0.320), as well as aesthetics and creativity ( r s = 0.419). However, there is a statistically significant positive and strong correlation between functionality and usefulness ( r s = 0.918**). With regards to the relations among design creativity and its three core elements ( novelty , usefulness , and surprise ); novelty is strongly correlated to surprise ( r s = 0.920**) and creativity ( r s = 0.777**) with positive statistical high significance, while surprise is also strongly positively correlated to creativity ( r s = 0.857**) with statistical high significance.
For the evaluation of chair samples, there is shown to be a statistically highly significant negative and moderate correlation between functionality and aesthetics ( r s = − 0.650**), while there is a statistically significant positive and moderate correlation between aesthetics and creativity ( r s = 0.619*), as shown in Table 9 . Functionality is positively and strongly correlated to usefulness ( r s = 0.874**) with statistical high significance. For creativity and its core elements, there are statistically highly significant positive and strong correlations between novelty and surprise ( r s = 0.936**), between novelty and creativity ( r s = 0.955**), and between surprise and creativity ( r s = 0.921**), which is also in line with the vase samples.
With regards to the lamp samples, there are no statistically significant correlations among functionality , aesthetics and creativity , which is in line with the vase examples, as shown in Table 10 . Similar to the vase and chair samples, functionality is positively and strongly correlated to usefulness ( r s = 0.752**), but aesthetics is negatively and moderately correlated to usefulness ( r s = − 0.620*), with statistical significance. With regards to the vase and chair samples, there are also statistically highly significant strong and positive correlations between novelty and surprise ( r s = 0.958**), novelty and creativity ( r s = 0.953**), and surprise and creativity ( r s = 0.911**).
For all three types of products, no significant correlations were identified between functionality and creativity . Only the chair samples showed a moderate and positive relation between aesthetics and creativity , while the other two types of product have shown no significant correlations. Furthermore, only the chair samples evaluations indicated a moderate and negative relation between aesthetics and functionality .
In terms of the relations between functionality , aesthetics , and the core elements of creativity , it is evident that there is a strong and positive correlation between functionality and usefulness for all three types of products. However, functionality was found to have no significant correlations with novelty and surprise . Furthermore, no significant correlations have been shown between aesthetics and the three core elements of creativity , except the lamp samples which indicate a moderate and negative relation to usefulness .
For creativity and its three core elements, there are strong and positive relations between novelty and creativity , surprise and creativity , as well as novelty and surprise , across all three types of products. However, no significant correlations are shown between usefulness and creativity , usefulness and surprise , as well as usefulness and novelty .
6 Discussion
As shown in the preceding section, Spearman correlation tests were conducted to analyse the relations among the six factors: functionality , aesthetics , novelty , usefulness , surprise , and creativity , for samples of vases, chairs and lamps. Several similar correlation results are shown in all three types of products, especially the relations between creativity and its three core elements ( novelty , usefulness , and surprise ). Some correlations, however, are only depicted in particular types of products, which may be related to the products’ functional and aesthetic attributes. Therefore, the forty-five samples, including the fifteen vases, fifteen chairs and fifteen lamps, are analysed as a whole to yield a more general result. The Spearman correlation test results for the overall product samples are provided in Table 11 .
In consideration of all three types of products as a whole, there are no statistically significant correlations between functionality and creativity , while there is a positive, moderate statistically and highly significant correlation between aesthetics and creativity ( r s = 0.528**). Furthermore, aesthetics is negatively and moderately correlated to functionality ( r s = − 0.501**) with a statistical high significance.
This indicates that creativity is positively and moderately related to aesthetics , but not significantly correlated to functionality in general, for the samples concerned. It shows a different result against the studies conducted by Kreitler and Casakin ( 2009 ) and Bao et al. ( 2018 ) who hold the view that functionality and aesthetics have no significant relations to creativity . Furthermore, these results also suggest that designs perceived to be less functionally appealing are more likely to be considered to have better aesthetics.
In terms of the relations between functionality and the three core elements of creativity, functionality is not statistically significantly correlated to novelty or surprise , but it is positively and strongly correlated to usefulness ( r s = 0.888**) with statistically high significance. According to Cropley and Kaufman ( 2019 ), a correlation coefficient of greater than 0.8 suggests redundancy in general. It therefore implies functionality and usefulness are measuring the same construct of a product. This shows that the two factors refer to the same dimension in assessing creative designs, which is in contrary to the findings in the review Sect. 2 .
For aesthetics and the three core elements, aesthetics is positively and moderately correlated to novelty ( r s = 0.463**) and surprise ( r s = 0.439**), but negatively and moderately correlated to usefulness ( r s = − 0.449**), with statistical high significance. This indicates that a design perceived to be more aesthetically appealing is likely to be perceived as more novel and surprising, but less useful. It also suggests that increasing the usefulness of a product will lead to a decrease in its aesthetics , which is in line with the preceding result that shows a negative correlation between functionality and aesthetics .
The analysis of how functionality and aesthetics relate to design creativity and its three core elements show that aesthetics is more important than functionality in creative product assessment, of which aesthetics is identified to have a positive relationship with creativity , novelty and surprise . The results also suggest that functionality and usefulness represent the same dimension, but has no significant relations with creativity and a negative relation with aesthetics . The relations between creativity and its three core elements ( novelty , usefulness , and surprise ) are therefore analysed to provide more insights.
As depicted in Table 11 , creativity is found to have positive and strong relations with both novelty ( r s = 0.925**) and surprise ( r s = 0.908**). Furthermore, it is shown that novelty is positively and strongly correlated to surprise ( r s = 0.945**), which has implied that novelty and surprise are measuring the same construct of a product based on the perceptions of the design experts involved in the case study. This is in contrary to the findings of the review section that novelty and surprise are claimed as independent factors. Moreover, the result has indicated that novelty , surprise and creativity refer to the same dimension perceived by the design experts, for the case study concerned.
The result also shows that there are no significant correlations between usefulness and creativity , while there are negative correlations between usefulness and novelty ( r s = − 0.336*) and surprise ( r s = − 0.336*). This confirms the findings in the preceding that functionality and usefulness are perceived as the same factor which is not significantly related to creativity . Furthermore, r s = − 0.336* is a low correlation coefficient, which is close to the values of suggesting weak correlations (0 < │ r s │ < 0.3). We could consider the correlation between usefulness and novelty is ‘weak’, as well as between usefulness and surprise .
The main findings of this study indicate that design creativity is only related to aesthetics with positive and moderate effects in general considering the vase, chair and lamp samples as a whole, but it is not significantly related to functionality . However, this may not be a generalised result that could be applied to all types of products, due to the specificity of the product types involved in the study. For example, the vase and lamp samples have shown no significant direct relations between creativity and aesthetics , while the chair samples have indicated a significant relation. Further explorations have shown that creativity , novelty and surprise are perceived as the same dimension by the experts involved in the case study, as well as usefulness and functionality , for all the results concerned. While the case study results might be specific to the types of products involved and the design experts who participated, general implications of the relationships are discussed below. This provides design researchers and practitioners with more insights into generating creative concepts and assessing creative products.
Comparing with functionality , aesthetics is shown to have a stronger relation with design creativity in general, especially for classical products that have rather stable functionalities, such as chairs. Thereby, it implies that a design with better aesthetic attributes are perceived more creative. The result is also reflected in terms of the relations between functionality , aesthetics and the core elements of creativity . Aesthetics is more likely to have a significant relation with novelty and surprise , while functionality and usefulness are perceived as the same factor. This suggest that, for a classical product (such as chairs), improving its aesthetic rather than functional attributes could increase its creativity , novelty , and surprise perceived.
Although many researchers have involved novelty and usefulness in defining and assessing design creativity , the findings above have indicated that usefulness is not significantly related to creativity for the case study concerned. Furthermore, some researchers also considered surprise as the third dimension of design creativity , which is independent from novelty . However, the case study conducted has shown that novelty and surprise refer to the same dimension. In addition, the results show that creativity , novelty and surprise are perceived as the same dimension by the design experts involved. It thereby indicates that, even though creativity involves usefulness , novelty and surprise , individuals perceive creative designs as the ones that are novel or surprising .
However, the results of the case study might be influenced by the limitations of using the images and text descriptions of the product samples rather than the physical objects in the case study. Although researchers, such as Hoegg and Alba ( 2011 ) and Radford and Bloch ( 2011 ), claim the functionality of a product is often assessed from just seeing it, it might still be challenging for individuals to perceive the full scope of the functionality of a product without hands-on experience. The results might also be determined by the three types of products, vases, chairs and lamps, selected. These types of products are classic and have rather stable and maturely defined functionality in general. In terms of radically new and path-breaking products with first-of-its-kind functions, functionality or usefulness may contribute more to design creativity , novelty and surprise .
In summary, the outcomes of this research lead to a new point of view for the community to reflect upon, allowing for a scholarly debate of creativity and its dimensions. As illustrated in the preceding, Kreitler and Casakin ( 2009 ) and Bao et al. ( 2018 ) claim that creativity is not related to aesthetics and functionality . However, the results of this research reveal that design creativity is related to aesthetics , which is supported by studies of other researchers, including (O'Quin and Besemer 1989 ; Cropley and Cropley 2005 ; Lee et al. 2015 ; Christensen and Ball 2016 ; García-García et al. 2017 ). Our results further suggest that there is no direct relationship between creativity and functionality , which is contrary to other studies, for example (Acuna and Sosa 2011 ; Sarkar and Chakrabarti 2011 ; Valgeirsdottir et al. 2015 ), that conclude functionality should be considered a significant dimension of creativity . Our research also shows that novelty and surprise are actually measuring the same construct, which is in agreement with work conducted by other researchers including O'Quin and Besemer ( 1989 ), Chiu and Shu ( 2012 ), Starkey et al. ( 2019 ), and Zheng and Miller ( 2020 ), whereas Boden ( 2004 ), Simonton ( 2012 ), Maher et al. ( 2013 ), Grace et al. ( 2015 ), and Acar et al. ( 2017 ) argue the opposite point, indicating that novelty and surprise are independent dimensions. Moreover, our research results indicate that creativity is not directly related to usefulness , while creativity , novelty and surprise are perceived as the same dimension. This is in opposition to most of the current studies in design, for example (Jeffries 2007 ; Kim et al. 2007 ; Robertson et al. 2007 ; Sarkar and Chakrabarti 2011 ; Chiu and Shu 2012 ; Chulvi et al. 2012 ; Sosa and Marle 2013 ; Crilly 2015 ; Grace et al. 2015 ; Mahdizadeh Hakak et al. 2016 ; Kelly and Gero 2017 ; Crilly and Moroşanu Firth 2019 ; Starkey et al. 2019 ), and psychology and cognitive science, such as (Sternberg and Lubart 1998 ; Mumford 2003 ; Boden 2004 ; Runco and Jaeger 2012 ), which claim that usefulness , novelty and/or surprise are the core dimensions of creativity . Therefore, elusive and debatable positions regarding the relations between creativity and its dimensions remain, requiring further explorations.
7 Conclusions
The research presented throughout this paper revealed that novelty , usefulness and surprise are the three core elements of design creativity . Functionality and aesthetics are often considered the core of design, however, existing literature presents inconsistent and conflicting opinions on the relationships between functionality , aesthetics and creativity . To date few studies have considered such core creativity elements into the relationship explorations. A case study was conducted to address the issues and provide new insights. Through an experimental approach, the design experts involved evaluated the creativity , novelty , surprise , usefulness , functionality and aesthetics of forty-five design samples. The samples include three types of products for investigation, vases, chairs and lamps, representing typical products that focus on different functional and aesthetical attributes. Statistical correlation analysis was performed to explore the relationships.
Overall, the case study conducted revealed that design creativity is positively related (directly and indirectly through its core elements) to aesthetics , but not significantly related to functionality in general. This shows that the ergonomic and visual appeals ( aesthetics ) tend to be more important than the performance ( functionality ) of a product, while regarding the product’s design creativity. However, it is shown that the relations might varies from product to product, according to the product’s aesthetic and functional attributes. The study has also indicated creativity is not significantly related to usefulness which represents the same dimension as functionality . Furthermore, it is shown that the design experts, involved in the case study, perceive creativity , novelty and surprise as the same dimension. This is against the common understanding of design creativity which involves usefulness and novelty as the core elements, as well as the recent findings that consider surprise as the third element which is distinct from novelty . This might also affect how we normally assess creative products, which is mainly based on the product’s novel and useful features.
The outcomes of the research conducted represent a contribution to the body of knowledge in research on design, creativity, innovation, engineering design, product design, and new product development. The results obtained provide evidence for how design creativity is perceived by design experts while considering functionality and aesthetics . In practice, it is suggested that designers could moderately increase the degree of design creativity of a product by improving its ergonomic and visual appeals, especially for classical products that have maturely defined functionality. This may be of importance to new product design and development, where creativity is considered a prerequisite for generating innovative products that ensures product success. Most importantly, the study allows for new meditations and debates on how creativity should be defined, assessed, and interpreted, as well as the role that creativity plays in design-related research areas and professional practice.
Data availability
Not applicable.
Code availability
Acar S, Burnett C, Cabra JF (2017) Ingredients of creativity: originality and more. Creat Res J 29(2):133–144. https://doi.org/10.1080/10400419.2017.1302776
Article Google Scholar
Achiche S, Appio FP, McAloone TC, Di Minin A (2013) Fuzzy decision support for tools selection in the core front end activities of new product development. Res Eng Design 24(1):1–18. https://doi.org/10.1007/s00163-012-0130-4
Acuna A, Sosa R (2011) The complementary role of representations in design creativity: sketches and models. Springer. pp 265–270
Google Scholar
Alipour L, Faizi M, Moradi AM, Akrami G (2017) The impact of designers’ goals on design-by-analogy. Des Stud 51:1–24. https://doi.org/10.1016/j.destud.2017.04.001
Amabile TM (1983) The social psychology of creativity. Springer-Verlag
Book Google Scholar
Bao Q, Faas D, Yang M (2018) Interplay of sketching & prototyping in early stage product design. Internat J Design Creat Innov 6(3–4):146–168. https://doi.org/10.1080/21650349.2018.1429318
Becattini N, Borgianni Y, Cascini G, Rotini F (2017) Surprise and design creativity: investigating the drivers of unexpectedness. Internat J Design Creat Innov 5(1–2):29–47. https://doi.org/10.1080/21650349.2015.1090913
Becattini N, Borgianni Y, Cascini G, Rotini F (2020) Investigating users’ reactions to surprising products. Des Stud 69:100946. https://doi.org/10.1016/j.destud.2020.05.003
Besemer SP (1998) Creative product analysis matrix: testing the model structure and a comparison among products-three novel chairs. Creat Res J 11(4):333–346. https://doi.org/10.1207/s15326934crj1104_7
Bledow R, Frese M, Anderson N, Erez M, Farr J (2009) A dialectic perspective on innovation: conflicting demands, multiple pathways, and ambidexterity, industrial and organizational. Psychology 2(3):305–337. https://doi.org/10.1111/j.1754-9434.2009.01154.x
Boden MA (2004) The creative mind: Myths and mechanisms, 2nd edn. Routledge
Carruthers P (2011) Creative action in mind. Philos Psychol 24(4):437–461. https://doi.org/10.1080/09515089.2011.556609
Chakrabarti A (2013) Understanding influences on engineering creativity and innovation: a biographical study of 12 outstanding engineering designers and innovators. Internat J Design Creat Innov 1(1):56–68. https://doi.org/10.1080/21650349.2013.754643
Chakrabarti A, Bligh TP (2001) A scheme for functional reasoning in conceptual design. Des Stud 22(6):493–517. https://doi.org/10.1016/S0142-694X(01)00008-4
Chakrabarti A, Sarkar P, Leelavathamma B, Nataraju BS (2005) A functional representation for aiding biomimetic and artificial inspiration of new ideas. Artif Intell Eng Des Anal Manuf 19(2):113–132. https://doi.org/10.1017/S0890060405050109
Charyton C, Merrill JA (2009) Assessing general creativity and creative engineering design in first year engineering students. J Eng Educ 98(2):145–156. https://doi.org/10.1002/j.2168-9830.2009.tb01013.x
Childs PRN (2018) Mechanical design engineering handbook, 2nd edn. Butterworth-Heinemann
Chiu I, Shu LH (2012) Investigating effects of oppositely related semantic stimuli on design concept creativity. J Eng Des 23(4):271–296. https://doi.org/10.1080/09544828.2011.603298
Christensen BT, Ball LJ (2016) Dimensions of creative evaluation: distinct design and reasoning strategies for aesthetic, functional and originality judgments. Des Stud 45:116–136. https://doi.org/10.1016/j.destud.2015.12.005
Christensen BT, Kristensen T, Reber R (2015) Contributions of consumer-perceived creativity and beauty to willingness-to-pay for design products. Internat J Design Creat Innov 3(3–4):164–176. https://doi.org/10.1080/21650349.2014.981216
Chulvi V, Sonseca Á, Mulet E, Chakrabarti A (2012) Assessment of the relationships among design methods, design activities, and creativity. J Mech Design 134(11):111004–1110011. https://doi.org/10.1115/1.4007362
Collado-Ruiz D, Ostad-Ahmad-Ghorabi H (2010) Influence of environmental information on creativity. Des Stud 31(5):479–498. https://doi.org/10.1016/j.destud.2010.06.005
Craft A (2003) The limits to creativity in education: dilemmas for the educator. Br J Educ Studies 51(2):113–127. https://doi.org/10.1111/1467-8527.t01-1-00229
Crilly N (2015) Fixation and creativity in concept development: the attitudes and practices of expert designers. Des Stud 38:54–91. https://doi.org/10.1016/j.destud.2015.01.002
Crilly N, Moroşanu Firth R (2019) Creativity and fixation in the real world: three case studies of invention, design and innovation. Des Stud 64:169–212. https://doi.org/10.1016/j.destud.2019.07.003
Crilly N, Moultrie J, Clarkson PJ (2004) Seeing things: consumer response to the visual domain in product design. Des Stud 25(6):547–577. https://doi.org/10.1016/j.destud.2004.03.001
Cropley D, Cropley A (2005) “Engineering creativity: a systems concept of functional creativity” in creativity across domains: faces of the muse. Lawrence Erlbaum Associates Publishers, pp 169–185
Cropley DH, Cropley AJ (2011) Aesthetics and creativity. In: Runco MA, Pritzker SR (eds) Encyclopedia of creativity (Second Edition). Academic Press, pp 24–28
Chapter Google Scholar
Cropley DH, Kaufman JC (2019) The siren song of aesthetics? Domain differences and creativity in engineering and design, Proceedings of the Institution of Mechanical Engineers. J Mech Eng Sci 233(2):451–464. https://doi.org/10.1177/0954406218778311
Cropley DH, Kaufman JC, Cropley AJ (2011) Measuring creativity for innovation management. J Technol Manag Innov 6:13–30
Cross N (2011) Design thinking: understanding how designers think and work. Berg Publishers
Dancey CP, Reidy J (2014) Statistics without maths for psychology. Pearson Education M.U.A
Demirkan H, Afacan Y (2012) Assessing creativity in design education: analysis of creativity factors in the first-year design studio. Des Stud 33(3):262–278. https://doi.org/10.1016/j.destud.2011.11.005
Doboli A, Umbarkar A (2014) The role of precedents in increasing creativity during iterative design of electronic embedded systems. Des Stud 35(3):298–326. https://doi.org/10.1016/j.destud.2014.01.001
Doré R, Pailhes J, Fischer X, Nadeau J-P (2007) Identification of sensory variables towards the integration of user requirements into preliminary design. Int J Ind Ergon 37(1):1–11. https://doi.org/10.1016/j.ergon.2006.08.006
D’Souza N, Dastmalchi MR (2016) Creativity on the move: exploring little-c (p) and big-C (p) creative events within a multidisciplinary design team process. Des Stud 46:6–37. https://doi.org/10.1016/j.destud.2016.07.003
Eckert CM, Stacey M, Wyatt D, Garthwaite P (2012) Change as little as possible: creativity in design by modification. J Eng Des 23(4):337–360. https://doi.org/10.1080/09544828.2011.639299
Eggink W, Snippert J (2017) Future Aesthetics of Technology; context specific theories from design and philosophy of technology. Des J 20(sup1):S196–S208. https://doi.org/10.1080/14606925.2017.1352748
García-García C, Chulvi V, Royo M (2017) Knowledge generation for enhancing design creativity through co-creative virtual learning communities. Think Skills Creativity 24:12–19. https://doi.org/10.1016/j.tsc.2017.02.009
Gero J, Yu R, Wells J (2019) The effect of design education on creative design cognition of high school students. Internat J Design Creat Innov 7(4):196–212. https://doi.org/10.1080/21650349.2019.1628664
Girotra K, Terwiesch C, Ulrich KT (2010) Idea generation and the quality of the best idea. Manage Sci 56(4):591–605
Goode MR, Dahl DW, Moreau CP (2013) Innovation aesthetics: the relationship between category cues, categorization certainty, and newness perceptions. J Prod Innov Manag 30(2):192–208. https://doi.org/10.1111/j.1540-5885.2012.00995.x
Gotzsch J (2017) Getting creative again awaking your inner creative self. Design J 20(sup1):S1072–S1079. https://doi.org/10.1080/14606925.2017.1353051
Grace K, Maher ML, Fisher D, Brady K (2015) Data-intensive evaluation of design creativity using novelty, value, and surprise. Internat J Design Creativity Innov 3(3–4):125–147. https://doi.org/10.1080/21650349.2014.943295
Gupta H, Noshin L, Sultana N (2017) Multipurpose table lamp: a functional improvement of a table lamp. Internat J Design Creativity Innov 4:138–148
Hagtvedt H, Patrick VM (2014) Consumer response to overstyling: balancing aesthetics and functionality in product design. Psychol Marke 31(7):518–525. https://doi.org/10.1002/mar.20713
Han J, Shi F, Chen L, Childs PRN (2018a) A computational tool for creative idea generation based on analogical reasoning and ontology. Artif Intell Eng Des Anal Manuf 32(4):462–477. https://doi.org/10.1017/S0890060418000082
Han J, Shi F, Park D, Chen L, Childs P (2018b) The conceptual distances between ideas in combinational creativity, in DS92 : proceedings of the DESIGN 2018 15th international design conference, 1857–1866. https://doi.org/10.21278/idc.2018.0264 .
Han J, Forbes H, Schaefer D (2019a) An exploration of the relations between functionality, aesthetics and creativity in design. Proc Design Soc Internat Conf Eng Design 1(1):259–268. https://doi.org/10.1017/dsi.2019.29
Han J, Park D, Shi F, Chen L, Hua M, Childs PR (2019b) Three driven approaches to combinational creativity: problem-, similarity- and inspiration-driven. Proc Inst Mech Eng C J Mech Eng Sci 233(2):373–384. https://doi.org/10.1177/0954406217750189
Haug A (2016) A framework for the experience of product aesthetics. Des J 19(5):809–826. https://doi.org/10.1080/14606925.2016.1200342
He Y, Luo J (2017) The novelty ‘sweet spot’ of invention. Design Sci 3:e21. https://doi.org/10.1017/dsj.2017.23
Hoegg J, Alba JW (2011) Seeing is believing (Too Much): the influence of product form on perceptions of functional performance. J Prod Innov Manag 28(3):346–359. https://doi.org/10.1111/j.1540-5885.2011.00802.x
Hölttä-Otto K, Otto K, Song C, Luo J, Li T, Seepersad CC, Seering W (2018) The characteristics of innovative, mechanical products—10 years later. J Mech Design 140(8):12. https://doi.org/10.1115/1.4039851
Homburg C, Schwemmle M, Kuehnl C (2015) New product design: concept, measurement, and consequences. J Mark 79(3):41–56. https://doi.org/10.1509/jm.14.0199
Horn D, Salvendy G (2009) Measuring consumer perception of product creativity: Impact on satisfaction and purchasability. Hum Factors Ergonomics Manuf Service Indust 19(3):223–240. https://doi.org/10.1002/hfm.20150
Hsiao Y, MacDonald MC (2013) Experience and generalization in a connectionist model of Mandarin Chinese relative clause processing. Front Psychol 4:767. https://doi.org/10.3389/fpsyg.2013.00767
Hung W-K, Chen L-L (2012) Effects of novelty and its dimensions on aesthetic preference in product design. Internat J Design Creativity Innov 6(2):81–90
Jagtap S (2019) Design creativity: refined method for novelty assessment. Internat J Design Creativity Innov 7(1–2):99–115. https://doi.org/10.1080/21650349.2018.1463176
Jeffries KK (2007) Diagnosing the creativity of designers: individual feedback within mass higher education. Des Stud 28(5):485–497. https://doi.org/10.1016/j.destud.2007.04.002
Kazerounian K, Foley S (2007) Barriers to creativity in engineering education: a study of instructors and students perceptions. J Mech Des 129(7):761–768. https://doi.org/10.1115/1.2739569
Kelly N, Gero JS (2017) Generate and situated transformation as a paradigm for models of computational creativity. Internat J Design Creativity Innov 5(3–4):149–167. https://doi.org/10.1080/21650349.2016.1203821
Keshwani S, Lenau TA, Ahmed-Kristensen S, Chakrabarti A (2017) Comparing novelty of designs from biological-inspiration with those from brainstorming. J Eng Des 28(10–12):654–680. https://doi.org/10.1080/09544828.2017.1393504
Kim MH, Kim YS, Lee HS, Park JA (2007) An underlying cognitive aspect of design creativity: Limited Commitment Mode control strategy. Des Stud 28(6):585–604. https://doi.org/10.1016/j.destud.2007.04.006
Koestler A (1964) The act of creation. Hutchinson
Koronis G, Chia PZ, Kang Kai Siang J, Silva A, Yogiaman C, Raghunath N (2019) An empirical study on the impact of design brief information on the creativity of design outcomes with consideration of gender and gender diversity. J Mech Design 141(7):8. https://doi.org/10.1115/1.4043207
Kreitler S, Casakin H (2009) Self-perceived creativity: the perspective of design. Eur J Psychol Assess 25(3):194–203. https://doi.org/10.1027/1015-5759.25.3.194
Lai H-H, Lin Y-C, Yeh C-H, Wei C-H (2006) User-oriented design for the optimal combination on product design. Int J Prod Econ 100(2):253–267. https://doi.org/10.1016/j.ijpe.2004.11.005
Lee JH, Gu N, Ostwald MJ (2015) Creativity and parametric design? Comparing designer’s cognitive approaches with assessed levels of creativity. Internat J Design Creativity Innov 3(2):78–94. https://doi.org/10.1080/21650349.2014.931826
Lopez R, Linsey JS, Smith SM (2011) Characterizing the effect of domain distance in design-by-analogy, 5:141–151. https://doi.org/10.1115/DETC2011-48428 .
Lugo JE, Schmiedeler JP, Batill SM, Carlson L (2016) Relationship between product aesthetic subject preference and quantified gestalt principles in automobile wheel rims. J Mech Design 138(5):9. https://doi.org/10.1115/1.4032775
Mahdizadeh Hakak A, Bhattacharya J, Biloria N, Ahmadi Venhari A (2016) The Proto-Fuse project: methods to boost creativity for architects. Internat J Design Creativity Innov 4(3–4):206–221. https://doi.org/10.1080/21650349.2015.1021838
Maher ML, Brady KA, Fisher DH (2013) Computational models of surprise in evaluating creative design. Proc Fourth Internat Conf Comput Creativity (ICCC) 2013:147–151
Martin L, Wilson N (2017) Defining creativity with discovery. Creat Res J 29(4):417–425. https://doi.org/10.1080/10400419.2017.1376543
Mata MP, Ahmed-Kristensen S, Shea K (2018) Implementation of design rules for perception into a tool for three-dimensional shape generation using a shape grammar and a parametric model. J Mech Design 141(1):2. https://doi.org/10.1115/1.4040169
Moldovan S, Goldenberg J, Chattopadhyay A (2011) The different roles of product originality and usefulness in generating word-of-mouth. Int J Res Mark 28(2):109–119. https://doi.org/10.1016/j.ijresmar.2010.11.003
Moon H, Park J, Kim S (2015) The importance of an innovative product design on customer behavior: development and validation of a scale. J Prod Innov Manag 32(2):224–232. https://doi.org/10.1111/jpim.12172
Mumford MD (2003) Taking stock in taking stock. Creat Res J 15:147–151
Nguyen L, Shanks G (2009) A framework for understanding creativity in requirements engineering. Inf Softw Technol 51(3):655–662. https://doi.org/10.1016/j.infsof.2008.09.002
Oman SK, Tumer IY, Wood K, Seepersad C (2013) A comparison of creativity and innovation metrics and sample validation through in-class design projects. Res Eng Design 24(1):65–92. https://doi.org/10.1007/s00163-012-0138-9
Onarheim B (2012) Creativity from constraints in engineering design: lessons learned at Coloplast. J Eng Des 23(4):323–336. https://doi.org/10.1080/09544828.2011.631904
O’Quin K, Besemer SP (1989) The development, reliability, and validity of the revised creative product semantic scale. Creat Res J 2(4):267–278. https://doi.org/10.1080/10400418909534323
Orsborn S, Cagan J, Boatwright P (2009) Quantifying aesthetic form preference in a utility function. J Mech Design 131(6):061001–0610010. https://doi.org/10.1115/1.3116260
Perez Mata M, Ahmed-Kristensen S, Brockhoff PB, Yanagisawa H (2017) Investigating the influence of product perception and geometric features. Res Eng Design 28(3):357–379. https://doi.org/10.1007/s00163-016-0244-1
Plucker JA, Makel MC (2010) Assessment of creativity. In: Kaufman JC, Sternberg RJ (eds) The Cambridge handbook of creativity. The Cambridge University Press, pp 48–73
Pringle H (2013) The origins of creativity. Sci Am 23:4–11. https://doi.org/10.1038/scientificamericancreativity1213-4
Rabiser R, Grünbacher P, Lehofer M (2012) A qualitative study on user guidance capabilities in product configuration tools, in 2012. Proceedings of the 27th IEEE/ACM international conference on automated software engineering. 3–7:110–119.
Radford SK, Bloch PH (2011) Linking innovation to design: consumer responses to visual product newness. J Prod Innov Manag 28(s1):208–220. https://doi.org/10.1111/j.1540-5885.2011.00871.x
Rahman O, Jiang Y, Liu W-S (2010) Evaluative criteria of denim jeans: a cross-national study of functional and aesthetic aspects. Des J 13(3):291–311. https://doi.org/10.2752/146069210X12766130824894
Redelinghuys C, Bahill AT (2006) A framework for the assessment of the creativity of product design teams. J Eng Des 17(2):121–141. https://doi.org/10.1080/09544820500273136
Reed K (2013) Aesthetic measures for evolutionary vase design. Berlin. Springer, pp 59–71
Reich Y (1993) A model of aesthetic judgment in design. Artif Intell Eng 8(2):141–153. https://doi.org/10.1016/0954-1810(93)90023-9
Robertson BF, Walther J, Radcliffe DF (2007) Creativity and the use of CAD tools: lessons for engineering design education from industry. J Mech Des 129(7):753–760. https://doi.org/10.1115/1.2722329
Rodgers PA, Jones P (2017) Comparing university design students’ and tutors’ perceptions of creativity. Des J 20(4):435–457. https://doi.org/10.1080/14606925.2017.1323503
Runco MA, Jaeger GJ (2012) The standard definition of creativity. Creat Res J 24(1):92–96. https://doi.org/10.1080/10400419.2012.650092
Sarkar P, Chakrabarti A (2008) Studying engineering design creativity-developing a common definition and associated measures. In: Gero J (ed) Proceedings of the NSF workshop on studying design creativity.
Sarkar P, Chakrabarti A (2011) Assessing design creativity. Des Stud 32(4):348–383. https://doi.org/10.1016/j.destud.2011.01.002
Shah JJ, Smith SM, Vargas-Hernandez N (2003) Metrics for measuring ideation effectiveness. Des Stud 24(2):111–134. https://doi.org/10.1016/s0142-694x(02)00034-0
Shai O, Reich Y, Rubin D (2009) Creative conceptual design: extending the scope by infused design. Comput Aided Des 41(3):117–135. https://doi.org/10.1016/j.cad.2007.11.004
Shalley CE, Hitt MA, Zhou J (2015) Introduction: Integrating creativity, innovation, and entrepreneurship to enhance the organization’s capability to navigate in the new competitive landscape. The Oxford handbook of creativity innovation and entrepreneurship
Shiu E (2017) Product design innovation: trade-off decisions on functionality, aesthetics and sustainability from the consumer perspective in research handbook of innovation and creativity for marketing management. Edward Elgar Publishing
Simonton DK (2012) Taking the US patent office criteria seriously: a quantitative three-criterion creativity definition and its implications. Creativity Res J 24(2–3):97–106. https://doi.org/10.1080/10400419.2012.676974
Snider C, Dekoninck E, Culley S (2016) Beyond the concept: characterisations of later-stage creative behaviour in design. Res Eng Design 27(3):265–289. https://doi.org/10.1007/s00163-016-0218-3
Sonderegger A, Sauer J (2010) The influence of design aesthetics in usability testing: effects on user performance and perceived usability. Appl Ergon 41(3):403–410. https://doi.org/10.1016/j.apergo.2009.09.002
Sosa ME, Marle F (2013) Assembling creative teams in new product development using creative team familiarity. J Mech Design 135(8):81009–810013. https://doi.org/10.1115/1.4024763
Srinivasan R, Lilien GL, Rangaswamy A, Pingitore GM, Seldin D (2012) The total product design concept and an application to the auto market. J Prod Innov Manag 29(S1):3–20. https://doi.org/10.1111/j.1540-5885.2012.00958.x
Srinivasan V, Song B, Luo J, Subburaj K, Elara MR, Blessing L, Wood K (2018) Does analogical distance affect performance of ideation? J Mech Design 140(7):9. https://doi.org/10.1115/1.4040165
Starkey E, Toh CA, Miller SR (2016) Abandoning creativity: the evolution of creative ideas in engineering design course projects. Des Stud 47:47–72. https://doi.org/10.1016/j.destud.2016.08.003
Starkey EM, Menold J, Miller SR (2019) When are designers willing to take risks? How concept creativity and prototype fidelity influence perceived risk. J Mech Design 141(3):8. https://doi.org/10.1115/1.4042339
Sternberg RJ, Lubart TI (1998) The concept of creativity: prospects and paradigms. In: Sternberg RJ (ed) Handbook of creativity. Cambridge University Press, pp 3–15
Sylcott B, Cagan J, Tabibnia G (2013) Understanding consumer tradeoffs between form and function through metaconjoint and cognitive neuroscience analyses. J Mech Design 135(10):101002–1010013. https://doi.org/10.1115/1.4024975
Tan C (2016) Understanding creativity in East Asia: insights from Confucius’ concept of junzi. Internat J Design Creativity Innov 4(1):51–61. https://doi.org/10.1080/21650349.2015.1026943
Taura T, Nagai Y (2017) Creativity in Innovation Design: the roles of intuition, synthesis, and hypothesis. Internat J Design Creativity Innov 5(3–4):131–148. https://doi.org/10.1080/21650349.2017.1313132
Thompson G, Lordan M (1999) A review of creativity principles applied to engineering design. Proc Instit Mech Eng Part E J Proc Mech Eng 213(1):17–31. https://doi.org/10.1243/0954408991529960
Toh CA, Miller SR (2013) Visual inspection or product dissection? the impact of designer-product interactions on engineering design creativity, in international design engineering technical conferences and computers and information in engineering conference, american society of mechanical engineers. V005T06A011.
Toh CA, Miller SR (2015) How engineering teams select design concepts: a view through the lens of creativity. Des Stud 38:111–138. https://doi.org/10.1016/j.destud.2015.03.001
Toh C, Miller SR (2019) Does the preferences for creativity scale predict engineering students’ ability to generate and select creative design alternatives? J Mech Design 141(6):9. https://doi.org/10.1115/1.4042154
Ulrich KT (2011) Design: creation of artifacts in society. SSRN
Valgeirsdottir D, Onarheim B, Gabrielsen G (2015) Product creativity assessment of innovations: considering the creative process. Internat J Design Creativity Innov 3(2):95–106. https://doi.org/10.1080/21650349.2014.954626
Wang H-H (2016) Winning formulas for metaphor design: A case study of design competitions, in DS 84: proceedings of the DESIGN 2016 14th international design conference.
Wang H-H, Chan J-H (2010) An approach to measuring metaphoricity of creative design. In: Taura T, Nagai Y (eds) Design creativity 2010. Springer, pp 89–96
Weisberg RW (2015) On the usefulness of “value” in the definition of creativity. Creat Res J 27(2):111–124. https://doi.org/10.1080/10400419.2015.1030320
Yannou B (2013) Which research in design creativity and innovation? Let us not forget the reality of companies. Internat J Design Creativity Innov 1(2):72–92. https://doi.org/10.1080/21650349.2013.754647
Yilmaz S, Daly SR, Seifert CM, Gonzalez R (2016) Evidence-based design heuristics for idea generation. Des Stud 46(Suppl C):95–124. https://doi.org/10.1016/j.destud.2016.05.001
Yu L, Nickerson JV (2011) Cooks or cobblers? crowd creativity through combination, in Proceedings of the SIGCHI conference on human factors in computing systems Association for computing machinery, Canada.
Zheng X, Miller SR (2019) Is ownership bias bad? the influence of idea goodness and creativity on design professionals concept selection practices. J Mech Design 141(2):9. https://doi.org/10.1115/1.4042081
Zheng X, Miller SR (2020) Out in the field versus inside in the lab: a comparison of design professionals’ concept screening practices. J Mech Des. https://doi.org/10.1115/1.4047904
Ziamou P, Ratneshwar S (2003) Innovations in product functionality: when and why are explicit comparisons effective? J Mark 67(2):49–61
Download references
Author information
Authors and affiliations.
Systems Realization Laboratory, School of Engineering, University of Liverpool, Liverpool, L69 3BX, UK
Ji Han, Hannah Forbes & Dirk Schaefer
You can also search for this author in PubMed Google Scholar
Contributions
JH: The research idea, research method, case study design, data analysis and writing the paper. HF: Case study design, data collection, and proof reading. DS: Overall oversight and guidance, proof reading, editing, feedback.
Corresponding author
Correspondence to Ji Han .
Ethics declarations
Conflicts of interest, additional information, publisher's note.
Springer Nature remains neutral with regard to jurisdictional claims in published maps and institutional affiliations.
Rights and permissions
Open Access This article is licensed under a Creative Commons Attribution 4.0 International License, which permits use, sharing, adaptation, distribution and reproduction in any medium or format, as long as you give appropriate credit to the original author(s) and the source, provide a link to the Creative Commons licence, and indicate if changes were made. The images or other third party material in this article are included in the article's Creative Commons licence, unless indicated otherwise in a credit line to the material. If material is not included in the article's Creative Commons licence and your intended use is not permitted by statutory regulation or exceeds the permitted use, you will need to obtain permission directly from the copyright holder. To view a copy of this licence, visit http://creativecommons.org/licenses/by/4.0/ .
Reprints and permissions
About this article
Han, J., Forbes, H. & Schaefer, D. An exploration of how creativity, functionality, and aesthetics are related in design. Res Eng Design 32 , 289–307 (2021). https://doi.org/10.1007/s00163-021-00366-9
Download citation
Received : 01 May 2020
Revised : 11 April 2021
Accepted : 19 April 2021
Published : 05 May 2021
Issue Date : July 2021
DOI : https://doi.org/10.1007/s00163-021-00366-9
Share this article
Anyone you share the following link with will be able to read this content:
Sorry, a shareable link is not currently available for this article.
Provided by the Springer Nature SharedIt content-sharing initiative
- Functionality
- Find a journal
- Publish with us
- Track your research
- Search Menu
- Advance articles
- Browse content in Biological, Health, and Medical Sciences
- Administration Of Health Services, Education, and Research
- Agricultural Sciences
- Allied Health Professions
- Anesthesiology
- Anthropology
- Anthropology (Biological, Health, and Medical Sciences)
- Applied Biological Sciences
- Biochemistry
- Biophysics and Computational Biology (Biological, Health, and Medical Sciences)
- Biostatistics
- Cell Biology
- Dermatology
- Developmental Biology
- Environmental Sciences (Biological, Health, and Medical Sciences)
- Immunology and Inflammation
- Internal Medicine
- Medical Microbiology
- Medical Sciences
- Microbiology
- Neuroscience
- Obstetrics and Gynecology
- Ophthalmology
- Pharmacology
- Physical Medicine
- Plant Biology
- Population Biology
- Psychological and Cognitive Sciences (Biological, Health, and Medical Sciences)
- Public Health and Epidemiology
- Radiation Oncology
- Rehabilitation
- Sustainability Science (Biological, Health, and Medical Sciences)
- Systems Biology
- Browse content in Physical Sciences and Engineering
- Aerospace Engineering
- Applied Mathematics
- Applied Physical Sciences
- Bioengineering
- Biophysics and Computational Biology (Physical Sciences and Engineering)
- Chemical Engineering
- Civil and Environmental Engineering
- Computer Sciences
- Computer Science and Engineering
- Earth Resources Engineering
- Earth, Atmospheric, and Planetary Sciences
- Electric Power and Energy Systems Engineering
- Electronics, Communications and Information Systems Engineering
- Engineering
- Environmental Sciences (Physical Sciences and Engineering)
- Materials Engineering
- Mathematics
- Mechanical Engineering
- Sustainability Science (Physical Sciences and Engineering)
- Browse content in Social and Political Sciences
- Anthropology (Social and Political Sciences)
- Economic Sciences
- Environmental Sciences (Social and Political Sciences)
- Political Sciences
- Psychological and Cognitive Sciences (Social and Political Sciences)
- Social Sciences
- Sustainability Science (Social and Political Sciences)
- Author guidelines
- Submission site
- Open access policy
- Self-archiving policy
- Why submit to PNAS Nexus
- The PNAS portfolio
- For reviewers
- About PNAS Nexus
- About National Academy of Sciences
- Editorial Board
- Journals on Oxford Academic
- Books on Oxford Academic

Article Contents
Introduction, semantic networks organization, networks percolation, distributional semantics, computational modeling, materials and methods, acknowledgements, authors' contributions, data availability.
- < Previous
Balancing novelty and appropriateness leads to creative associations in children

- Article contents
- Figures & tables
- Supplementary Data
Clara Rastelli, Antonino Greco, Nicola De Pisapia, Chiara Finocchiaro, Balancing novelty and appropriateness leads to creative associations in children, PNAS Nexus , Volume 1, Issue 5, November 2022, pgac273, https://doi.org/10.1093/pnasnexus/pgac273
- Permissions Icon Permissions
Creative problem solving is a fundamental skill of human cognition and is conceived as a search process whereby a novel and appropriate solution is generated. However, it is unclear whether children are able to balance novelty and appropriateness to generate creative solutions and what are the underlying computational mechanisms. Here, we asked children, ranging from 10 to 11 years old, to perform a word association task according to three instructions, which triggered a more appropriate (ordinary), novel (random), or balanced (creative) response. Results revealed that children exhibited greater cognitive flexibility in the creative condition compared to the control conditions, as revealed by the structure and resiliency of the semantic networks. Moreover, responses’ word embeddings extracted from pretrained deep neural networks showed that semantic distance and category switching index increased in the creative condition with respect to the ordinary condition and decreased compared to the random condition. Critically, we showed how children efficiently solved the exploration/exploitation trade-off to generate creative associations by fitting a computational reinforcement learning (RL) model that simulates semantic search strategies. Our findings provide compelling evidence that children balance novelty and appropriateness to generate creative associations by optimally regulating the level of exploration in the semantic search. This corroborates previous findings on the adult population and highlights the crucial contribution of both components to the overall creative process. In conclusion, these results shed light on the connections between theoretical concepts such as bottom-up/top-down modes of thinking in creativity research and the exploration/exploitation trade-off in human RL research.
The ability to generate creative associations between concepts is notoriously increasing with age, reaching its peak during adulthood and decreasing later. However, there is limited evidence in children about the stage of development of these creative problem-solving skills and what are the underlying computational mechanisms. Here, we found 10 years old children are able to solve the tension between novelty and appropriateness when searching for creative associations in a semantic space by optimally regulating the level of exploration in the semantic search. These results corroborate previous findings on the adult population and highlight the relevant contribution of novelty and appropriateness to the overall creative process, shedding light on the connections between theoretical concepts in creativity and human reinforcement learning research.
Children constantly face complex problems in their environment, even though, compared to adults, they have limited knowledge of the world ( 1 ) and tend to adopt a more exploratory search strategy when looking for solutions ( 2–6 ). The ability to tackle problems by finding an optimal solution has been associated with creative problem solving (CPS) skills ( 7 , 8 ), which require not exclusively cognitive flexibility but also higher cognitive resources and a broad understanding of facts and constraints ( 9–11 ). Indeed, achieving creative solutions rely on the reorganization of existing knowledge, balancing the dual objectives of exploring unknown options and exploiting common ones, as in other cognitive processes ( 5 , 10 , 12 , 13 ). Throughout the last decades, researchers have been exploring creative cognition in development and education ( 14 ), acknowledging it among the major skills of the 21st century. While CPS is pivotal to human development, its scientific understanding is fundamentally challenging due to its open-ended and internally driven nature. Additionally, whereas many studies have explored the creative process (i.e., CPS) in adults (e.g., 15 to 20), less is known about the developmental processes shaping CPS during childhood. Investigating how children use search strategies when looking for optimal solutions is critically important for developmental and educational scientists, as it can provide us with a crucial understanding of the behavioral principles that lead to creative cognition and healthy development overall.
CPS is semantically imbued ( 15 , 16 ), involving the search for connections between apparently conflicting or distant concepts and recombining them into new meaningful patterns ( 15 , 16 , 17 ). By definition, creative ideas are distinguished from more routine solutions by their novelty (i.e., originality, unexpectedness), while they are set apart from bizarre and fanciful ideas by their appropriateness, as they must relate to a problem or conform to the requirements of a relevant domain ( 18 , 19 ). However, the contribution of novelty and appropriateness to CPS is far from being understood ( 11 ), especially during developmental age. More than 50 years have been dedicated to studying these processes in children within the field of education ( 14 ) albeit empirical results in the literature are inconsistent ( 20 ), probably due to measurement and methodological differences across studies.
To overcome this limitation, researchers proposed a reconceptualization of CPS under the computationally informed semantic cognition framework, which relies on two interconnected systems ( 21 , 22 ): the first is a system of knowledge representation, which enables the storing of semantic concepts; in the second system, stored representations are activated by manipulating them in a goal-oriented and task-dependent manner using semantic control. Notably, such endeavor already has benefited researchers shedding new light on a theoretical and empirical understanding of the CPS ( 15 ), especially in terms of semantic memory search strategies ( 23–28 ) and toward a robust assessment of CPS potential, refining computational methods to quantify a broad range of verbal tasks ( 29 , 30 , 31 , 28, 32–34 ).
A well-established approach to model knowledge representation, inspired by associative theory, is the spreading activation model by Collins and Loftus ( 35 ). In this model, knowledge is represented as a network in which concepts are treated as nodes and relations between them are determined by a principle of semantic similarity, the degree to which concepts share essential properties or features. As a result of this prior model, computational models of semantic representation were developed based on the “distributional hypothesis” ( 36 ), best captured by Firth ( 37 ) sentence “you shall know a word by the company it keeps.” In this model, the distributional properties of concepts are examined in large samples of language data, following the assumption that the more the similarity between two words the greater their occurrence in similar linguistic contexts (statistical redundancy). Concerning differences in knowledge representations over the lifespan, researchers found that children's semantic networks tend to have fewer nodes, connections, clusters, and a greater distance between concepts compared to adults ( 1 , 38 , 39 ). These findings have significant implications for understanding the cognitive processes involved in CPS since how a knowledge system is organized greatly influences information retrieval mechanisms and efficiency ( 21 , 40 , 41 ).
The stored representations in the semantic memory space, on the one hand, can be easily activated. From an early age ( 42 ), the passive retrieving of one concept in memory diffusely triggers other connected concepts, from the closest (e.g., “dog” and “cat”) to the most distant (e.g., “dog” and “turtle”) until the patch is exhausted ( 12 , 35 ). Coherently, studies found that during divergent thinking, many thoughts spontaneously arise (fluency) but manifest highly variable transitions (flexibility) ( 9 , 43 , 44 ), thus facilitating free association through decreased latent inhibition ( 45 ). Accordingly, several scholars nowadays have explored individual differences in CPS ability by probing the bottom-up conceptualizations of creative cognition, which heavily rely on associative theories ( 46 , 16 ) arguing that semantic memory structure mediates CPS performances ( 15 , 47 ). For instance, recent works found that, throughout the lifespan ( 48 , 49 ), creative conceptual associations emerge from a flexible ( 31 , 48 ), small-world semantic network organization which is characterized by highly connected and closer concepts ( 15 , 33 , 48 , 49 ); offering a plausible explanation to why creative individuals can easily reach weaker and remote concepts while searching their memory.
On the other hand, within the semantic control system, executive semantic processing has been proposed to aid or constrain the propagation of semantic activation ( 21 , 35 ). Assuming no differences in associative hierarchy, when creative individuals are prompted for common associations, they are expected to exhibit similar associations while when asked explicitly for uncommon associations, they should show a higher distance between associations due to differences in control strategies ( 50 ), as largely demonstrated within adulthood ( 29 , 15 , 30 , 24 , 34 ). Executive theory of CPS indeed emphasizes a major role of top-down control over attention, ensuring a more efficient memory retrieval through the online monitoring of the search process (e.g. avoiding intrusions and repetitions), leading to the inhibition of ordinary associations and shifting towards meaningful solutions ( 46 , 31 , 50 ). Yet, verbal tasks, such as the verbal fluency or word association tasks (WATs), are frequently used in clinical assessments whose executive functions are impaired ( 1 , 51 , 52 ).
Far from being antagonistic, scholars mainly agree in seeing bottom-up and top-down cognitive processing in a synergistic relationship during the semantic search for creative solutions ( 46 ), positing this as a balance between the search for novelty and appropriateness during semantic processing—respectively, alternating between a broad exploratory search process and a process of exploiting nearby concepts to ensure a meaningful solution ( 8 , 12 ). Likewise, the trade-off between bottom-up/top-down functioning in CPS parallels another trade-off that appears in the reinforcement learning (RL) algorithms literature (exploration/exploitation) ( 13 ).
Such literature similarly claims that in many cases, when searching for an optimal solution through a high-dimensional space, the most efficient strategy is to begin with wider exploration and then move to narrower exploitation. Remarkably, researchers suggested that children are more likely to explore the vast space of possibilities when compared to adults ( 2–6 ). Unlike adults, children might originate more disparate ideas, switching between ideas less systematically while exploring widely the space of possibilities with a gradual reduction over the lifespan ( 3–5 , 53 ). Through this behavior, children can pick up information, and gain an understanding of unexpected causal relationships, that adults overlook ( 2 , 3 ). Even though children's exploratory behavior is well known, their creative outcome is unlikely to be highly valued since the greater variability of the search process might also lead to a higher deviation from meaningful and appropriate solutions ( 5 , 54 ).
Taken together, previous research highlights differences in children's semantic knowledge size and organization compared to that of adults ( 1 , 38 , 39 ), as well as differences in their search process ( 2–6 , 55 ), and especially in cognitive control abilities ( 56 , 57 ); suggesting that children's behavior may differ from that of adults when searching for an optimal solution. What remains to be investigated is how children solve the tension in the context of CPS semantic decisions. Here, we investigate how children navigate the vast space of knowledge representations in order to reach creative associations. To achieve this goal, during a WAT, we varied the demands of cognitive control on associative responses according to CPS criteria. In this way, two control conditions that trigger either a more appropriate (ordinary) or novel (random) response were set, together with the experimental condition that balances the two criteria (creative). We investigated associative responses by modeling them as semantic networks and measured their efficiency ( 15 , 31 , 33 ). Complementary, we quantified the semantic distance between the target word and the associative responses among conditions ( 30 , 34 ) and extracted a switching index between the selected semantic categories. Ultimately, in order to gain insight into the relationship between bottom-up/top-down and the exploration/exploitation trade-off and create a bridge between creative problem solving and RL scientific community ( 12 ), we leveraged the RL framework ( 58 , 59 ) to model the semantic navigation strategies participants implemented to solve the task.
Hence, consistent with the associative and executive theories of CPS ( 46 , 31 , 50 ) and empirical results on the adult population, we expect that children's creative associations are characterized by more flexibility as compared to the control conditions. Flexibility can thus be considered as a means of both small-world reconfiguration of the creative semantic network ( 26 , 27 , 33 ) and network robustness when undergoing to targeted attack ( 31 , 27 , 48 ). Crucially, by leveraging distributional analysis, we expected the semantic distance of the creative condition to be in the middle of a continuum ( 29 ) that has its lowest extreme (low semantic distance) in the ordinary condition and the highest one (high semantic distance) in the random condition ( 29 , 15 , 30 , 24 , 34 ). In a similar vein, we quantify a category switching index expecting to find the creative condition in the balance between control conditions ( 29 , 43 ). Finally, by fitting a computational model to estimate the degree of exploration/exploitation for each individual and condition, we expected to find that the creative condition stood in between the ordinary and random condition in terms of the amount of exploration adopted by participants to solve the task.
We collected data from 56 participants (age |$M\ = $| 10.05, |$SD$| = 0.23) by assessing the WAT. Participants were asked to generate as many related responses as possible to a given target word. We manipulated the nature of these associative responses by asking participants to generate responses according to a set of three instructions, which triggered either a more ordinary (OR), random (RA), or a balanced (CR) response (Fig. 1a and b ). By presenting 40 target words per condition, taken equally from 10 semantic categories (Fig. 1c ), responses across all the participants and conditions were collected and preprocessed. Because of the open-ended nature of the WAT (i.e., multiple answers were possible for each target word), we checked whether the fluency of responses diverged across conditions by comparing the number of responses generated to the target words between conditions. Across all participants, we collected a total of 21,388 responses, 7734 were generated after the OR condition, 4393 responses after the CR condition, and 9661 after the RA condition. Using a permutation t test, we found that participants produced more responses in RA condition ( |$M\ = $| 172.52, |$SD\ = $| 57.36) compared to both the CR ( |$M\ = $| 78.45, |$SD$| = 46.84, Bonferroni-corrected |$\mathit{ P}\ = $| <0.001) and the OR conditions ( |$M\ = $| 130.96, |$SD$| = 46.84, |$\mathit{ P}\ = $| <0.001). Notably, also the comparison between responses given in OR and CR leads to a significant difference ( |$\mathit{ P}\ = $| <0.001). Thus, we controlled for this confounding fluency factor in the subsequent analyses appropriately. We also collected human ratings for testing whether the responses in the creative condition were generated by following a balanced strategy between novelty and appropriateness (Fig. 1d ). Here, we asked eight raters to indicate a score on three scales, namely novelty, appropriateness, and creativity. There was a substantial agreement among the raters, measured by the intra class correlation coefficient (ICC) with the two-way fixed-effects model ( |$IC{C}_{novelty} = \ 0.93,\ \ IC{C}_{appropriateness} = \ 0.96,\ \ IC{C}_{creativity} = \ 0.61$| ). Thus, we tested whether participants’ responses in each condition were judged by the raters as aligning to the instruction set. Indeed, we found that the appropriateness was higher in the OR condition compared to both CR and RA conditions (all |$\mathit{ P}$| <0.001). We also found that novelty was higher in the RA condition with respect to CR and OR (all |$\mathit{ P}$| <0.001) and that the creativity score was higher in CR compared to OR and RA conditions (all |$\mathit{ P}$| <0.001, for all statistics see Table S2 ).

Experimental design, procedure, and selected word stimuli. (a) Participants were instructed to employ different semantic navigation strategies during the WAT, depending on the OR, CR, and RA conditions (top). We represent the possible search processes in a hypothetical knowledge space where the nodes represent the unique concepts, and the edges represent the similarity between these concepts. Nodes with colored borders represent possible answers, those colored inside indicate the answer under consideration. Each condition varied on the inclusion of appropriateness (OR), novelty (RA) search strategies and a balance between these two (CR) (bottom). (b) Participants performed the WAT for approximately 40 minutes, with a 1-week interval between conditions; the order of conditions was fixed while target words were presented in a randomized order. (c) Scatterplot visualizing the selected target words (cross) and their categories (triangles). The 2D coordinates are taken from t-SNE dimensionality-reduced word embeddings (see the "Methods" section). (d) Barplots representing subjective human ratings on appropriateness, creativity, and novelty using a 5-point likert scale. Responses were found to be more creative in the creative condition, which also ranks in the middle range for both novelty and appropriateness with respect to the other conditions. Responses in the ordinary condition were judged more appropriate while those in the random condition more novel. Error bars indicate the SEM.
Data were first analyzed using a network science-based approach ( 33 , 60 ), which enables us to explore the organization of the conceptual network depending on how often the responses co-occur across the sample in each condition. We constructed a group-level semantic network for each condition structuring the data into a matrix in which columns represent the target words, rows indicate the preprocessed unique association responses, and cells contain the amount of participants generating a response to a target word. Then, we computed the cosine similarity between the vectors associated with each target word in a pairwise fashion. The higher the number of similar associations generated by participants between pairs of target words and the greater the number of participants generating these associations, the stronger the association between them (link weight). This procedure resulted in an undirected weighted semantic network for each condition, with the target words as nodes and the cosine similarity as links. After filtering the networks ( 61 ), their organization was analyzed using the following network quantifiers: clustering coefficient (CC) ( 62 , 63 ), average shortest path length (ASPL) ( 62 ), modularity index (Q) ( 64 ), and small-worldness measure (S) ( 62 ). Results from the networks’ comparisons revealed qualitative (Fig. 2a ) and quantitative (Fig. 2b and c ) differences in their structures between conditions. The semantic graph of the CR condition showed lower level of organization (ASPL = 2.71 and Q = 0.51) and higher flexibility (S = 2.72) compared to the network of the OR condition (ASPL = 2.84, Q = 0.55, and S = 2.70). Similarly, RA showed a lower level of organization (ASPL = 2.16 and Q = 0.38) as well as a lower S value (2.58) compared to the CR condition. The CC measure was higher in the OR network (0.49) than in both the CR (0.47) and RA (0.35) networks.

Topological quantifiers of the semantic networks. (a) A 2D visualization of the undirected, unweighted semantic networks of the OR, CR, and RA conditions. The nodes represent the target WAT words, and the edges represent the cosine similarity between unique responses given by participants. (b) Barplots depicting the topological quantifiers of the full networks. (c) Raincloud plots represent the results from the leave-one-node-out (LONO) and leave-one-subject-out (LOSO) procedures on the topological quantifiers. All P < 0.001 (Bonferroni corrected).
We statistically examined the validity of our findings by applying two complementary approaches, the LONO and LOSO. In the LONO procedure, we iteratively computed the topological quantifiers on the partial networks resulting from the exclusion of one node at each iteration, for each node. The LOSO procedure iteratively excludes one participant and then repeats the entire process of building semantic networks for each subject. Thus, we computed the topological quantifiers on the resulting partial networks. A permutation t test was computed on each measure for comparing the conditions in both the LONO and LOSO procedures. Results indicated that CR had a significantly lower ASPL, CC, Q, and higher S compared to OR, whereas significantly higher ASPL, CC, Q, and S were found in CR in comparison to the RA condition (all |$\mathit{ P}$| <0.0001). Overall, these findings confirm the analyses on the full networks, all with very large effect sizes measured by Cohen's d (Fig. 2c ; for statistics see Table 1 ).
Semantic networks topology quantifiers and results from the paired two-tailed permutation t test of the partial networks comparing the CR, OR, and RA conditions.
ASPL; CC; Q; and S. LONO; LOSO. The t-statistics (from a parametric t test student) and Cohen's |${\boldsymbol{d}}$| ( 65 ) values are presented. Cohen's d effect sizes: 0.20, small; 0.50, moderate; 0.80, large; 1.10, very large. All P < 0.0001, corrected for multiple comparisons using Bonferroni (0.017).
To get rid of possible associative fluency confounds on network structure, we also repeated the above network analysis considering only the first two associative responses given by all participants to a target word ( 33 ). Results from this subset are in line with the results relying on the full dataset (see Table S3 in Supplementary Materials for statistics), corroborating the validity of the findings.
Next, we performed a percolation analysis ( 31 ) to investigate the flexibility of the semantic networks, probing the network's resilience under targeted attacks. Here, we assumed that the higher the network robustness is, the higher its cognitive flexibility ( 31 ). Thus, we used the weighted networks previously constructed from the preprocessed data (we controlled for possible associative bias in response fluency ( 31 ), see the "Method" section). To avoid possible confounders and retain more information, we used in this analysis the unfiltered similarity matrices. Throughout the percolation analysis, networks are “attacked” by removing all the edges whose weight strength falls below a threshold, called the percolation step. Each time a percolation step occurs, we measure the size of the largest connected component (LCCS), namely the largest cluster of nodes connected only to each other. Once the percolation procedure was completed, we computed the percolation integral ( |$\phi $| ), which is the area under the curve representing the LCCS across the percolation steps. Our analysis revealed that the CR network was more robust to network percolation, as exhibited by a higher percolation integral ( |$\phi $| = 7.09), with respect to both OR ( |$\phi $| = 5.84) and RA ( |$\phi $| = 4.83), as shown in Fig. 3a . We illustrated in Fig. 3b the network appearance throughout the percolation process, focusing on how the LCCS differed across conditions at various percolation steps, and indicating that the OR and RA networks broke apart faster than the CR networks.

Network percolation. (a) Line plot representing the percolation process of the CR (pink), OR (green), and RA (blue) full networks. The x-axis represents the weight threshold, starting from the smallest weight in a network (0.01) to a weight strength in which the giant component is smaller than three nodes (0.5). (b) OR, CR, and RA semantic networks undergoing the percolation process, visualized at different weight thresholds. (c) On the left, line plots of the LONO, LOSO, link shuffling (LS), and link noise procedures. Each line is an iteration, colors encode the conditions. On the right, barplots show the percolation integral |$\phi $| between conditions and across the three procedures. Error bars represent the SEM.
To determine the statistical significance of our findings, we implemented four approaches: LOSO, LONO, LS, and noise analyses (NA). As mentioned above, when computing the LONO and LOSO procedures, we iteratively excluded each node or participant and ran the percolation process on the resulted networks computing |$\phi $| . In order to control for the possibility that differences between networks may stem from the differences in the link weights, the LS analysis was conducted by randomly exchanging pairs of links in the network, running the percolation process, and computing |$\phi $| . Lastly, NA was computed by adding gaussian noise to the link weights and then analyzing the |$\phi $| . Overall, these analyses indicated consistent results, meaning that the average percolation integral |$\phi {\rm{\ }}$| of the CR network was significantly larger than the OR and RA networks (all |$\mathit{ p}$| <0.0001) with effect sizes ranging from moderate to very large (see Fig. 3c and Table 2 for statistics).
Results from the two-tailed paired-sample permutation t-test on percolation integral comparing conditions.
Results from the paired two-tailed permutation t test on percolation integral comparing the CR, OR, and RA conditions. LONO; LOSO. FullNet . L; T-statistic estimated from a parametric t test. Cohen's |${\boldsymbol{d}}$| effect sizes: 0.20, small; 0.50, moderate; 0.80, large; 1.10, and very large. All P < 0.0001 corrected for multiple comparison using Bonferroni (0.017).
Then, we also analyzed the data using a distributional semantics approach. According to this model, semantically similar words tend to occur in similar contexts ( 36 ). For methodological robustness, in this study, we used three pretrained word2vec models ( 66 ) trained with different methods (CBOW or Skip-gram) and different Italian text corpora. To ensure a reduced influence of specific models, while increasing the reliability of the results ( 67 ), we averaged the embeddings of the target words, category, and participant responses across all models. In Fig. 4a , we depicted the associative responses’ word embeddings of each condition in a 3D space using the t-distributed stochastic neighbor embedding (t-SNE) algorithm. We found that, qualitatively, CR responses tend to be more clustered around target words with respect to RA but less clustered compared to the OR condition. To quantify this intuition, for each target-response pair of word embedding vectors, we computed the semantic similarity as the cosine similarity, subtracted it from 1 to obtain a semantic distance score. Then for each participant, we averaged the semantic distances of all target words for each condition. Permutation t tests were computed on the resulted semantic distance score to compare the conditions. Results indicated that the semantic distance in the CR condition ( |$M\ = $| 0.66, |$SD\ = $| 0.02) was significantly lower than the RA condition ( |$M\ = $| 0.77, |$SD\ = $| 0.02, Bonferroni-corrected |$\mathit{ P }< $| 0.0001, |$d\ = $| 4.61, 95% CI [3.9, 5.32]) and significantly higher compared to the OR condition ( |$M\ = $| 0.65, |$SD\ = $| 0.03, |$p\ = {\rm{\ }}$| 0.003, |$d\ = {\rm{\ }}$| 0.49, 95% CI [0.12, 0.87]) (as illustrated in Fig. 4b and reported in Table 3 and 4 ). Additionally, we calculated a category switching index by computing the semantic distance between a target word and all the selected category words as well as between the response of that target and the categories. We assign a category to the target and the response words as the one that has the minimum semantic distance. For statistical comparison, we averaged category switching values across targets for each participant and condition. Permutation t tests were computed on category switching scores to compare the conditions. As a result of this analysis (Fig. 4c and Table 3 ), during the CR condition, the category switching score ( |$M\ = $| 0.68, |$SD\ = $| 0.07) was significantly higher compared to the OR condition ( |$M\ = $| 0.65, |$SD\ = $| 0.08), whereas both CR and OR showed significantly lower category switching scores in comparison with the RA condition ( |$M\ = $| 0.88, |$SD\ = $| 0.05). Statistically significant differences in category switching scores between conditions were observed among all comparisons, with effect sizes measured by Cohen's d , ranging from small when comparing CR vs. OR for category switching ( p = 0.013, d = 0.39, 95% CI [0.02, 0.77]), to very high when comparing CR vs. RA and OR vs. RA (see Table 4 for statistics). With the aim to control for possible associative fluency confounds on semantic fluency and category switching indexes, we also repeated the above pipelines considering only the first two responses of the WAT. In line with full dataset findings, results showed significant differences for both semantic distance and category switching, placing the CR condition values in between the OR (lower score) and RA (higher score) conditions (see Tables S4 and S5 in Supplementary Materials for statistics).

Semantic distance and category switching. (a) t-SNE scatter plots representing the preprocessed data among the conditions in the embedding space. Responses are coded as dots, target words as cross and category words as triangles. (b) Raincloud plots representing the semantic distance between conditions. (c) Raincloud plots representing the category switching between conditions.
Descriptive statistics for semantic distance and category switching.
Results from comparisons across conditions using the two-tailed paired-sample permutation t test on semantic distance and category switching.
Results from the paired two-tailed permutation t test on SD and CS comparing the CR, OR, and RA conditions. T-statistic estimated from a parametric t test. Cohen's |${\boldsymbol{d}}$| effect sizes: 0.20, small; 0.50, moderate; 0.80, large; >1.10,and very large. All P values corrected for three paired comparison using Bonferroni (0.017).
Finally, we leveraged the RL framework ( 58 , 59 ) to analyze the semantic navigation strategies implemented by children to generate an associative response to a given target word. Therefore, we propose a computational model that is able to capture the amount of exploration that children adopted in a semantic space (Fig. 5a ). The computational model, which we called the Semantic Explorer model (SemExp), consists of two components. The first one is a similarity matrix between target words and all the unique responses given by the whole sample. This matrix is created from the averaged word embeddings previously used for the distributional semantic analysis (Fig. 5a ) and can be seen as an approximation of a value function operating in a semantic state space ( 59 ). The second component is an action selection rule that generate the response word given a target word. This selection is performed by using the softmax function on the vector of similarity values of a given target word. To control the amount of exploration versus exploitation, there is an inverse temperature parameter, which we called beta ( β ), that determines the degree to which the value function influences choice. In other words, it controls the level of stochasticity in the response generation process, where lower values of β induce the model to select responses more randomly (i.e., β <1 means more exploratory behavior) and a higher β allows the model to deterministically generate the highest value response word (i.e,. β >1 means more exploitative behavior). Model fitting was performed using the negative log-likelihood (NLL) as loss function and gradient-based methods for the parameter estimation procedure (Fig. 5a , see methods for further information). Before fitting the SemExp model to the participants’ choices, we checked whether the amount of data collected was enough for reliably estimating the β parameter by performing a parameter recovery analysis (Fig. 5b ). We found that the correlation of real and fitted β parameters was very high ( r = 0.97 p < |${10}^{ - 109}$| ). Next, we performed model selection by comparing three models (Fig. 5c ), a null model with always a uniform distribution for generating responses, a fixed model (WordEmb) relying only on the first component of the SemExp model (the similarity matrix built on the word embeddings shown in Fig. 1a ) and the SemExp model with the free parameter β fitted for each participant and condition. Results showed how the SemExp model significantly performed better at explaining children's response data compared to both the null model ( p < 0.0001, d = 5.44, 95% CI [4.63, 6.24]) and the WordEmb model ( p < 0.0001, d = 2.33, 95% CI [1.85, 2.81]). Notably, the WordEmb model significantly outperformed the null model ( p < 0.0001, d = 7.65, 95% CI [6.58, 8.72]), providing evidence that our methodological choice of averaging the word2vec models was reliable (Fig. 5c ). After confirming that SemExp was the best model among candidates, we visually inspected the loss landscape as a function of the beta parameter using a grid search procedure (Fig. 5d ). We observed how the RA condition substantially diverged from the other two, confirming the previous results on the distributional semantics analysis. Finally, we extracted the β parameters for each participant and condition and tested their differences. We found that the beta in the CR condition (mean |${\beta }_{CR} = \ 2.34$| ) was significantly lower compared to OR (mean |${\beta }_{OR} = \ 2.47$| , p = 0.0058, d = 0.41, 95% CI [0.04, 0.79]) and significantly higher compared to RA (mean |${\beta }_{RA} = \ 0.76$| , p < 0.0001, d = 3.44, 95% CI [2.86, 4.03]). We also observed OR to be significantly higher compared to RA ( p < 0.0001, d = 3.73, 95% CI [3.11, 4.34]).

Computational modeling. (a) Description of the computational modeling analysis pipeline. From left, we took word2vec distributional semantics models and get from them word embeddings vectors of target and unique responses words. Then, we computed a similarity matrix having the target words as rows and responses as columns. We used this matrix, together with an action selection rule (softmax function), to build the semantic explorer model and fit it to the children data using the NLL loss function. (b) Scatterplot of the parameter recovery analysis, showing on the x and y axis the real and fitted parameters, respectively. (c) Barplots showing the loss values of the three models. The null model does not have variability since it has a fixed uniform distribution over the response selection. Errorbars indicate SEM. (d) Lineplots depicting the loss landscape, computed using a grid search procedure as a function of the beta parameter. Each line represents a single participant on each condition. (e) Raincloud plots representing the beta parameter between conditions.
In the present study, we investigated efficient information search during childhood from the semantic cognition perspective. To achieve this goal, we used a within-subject design, administering a WAT while varying the demands of cognitive control on associative responses. Our paradigm involves three conditions that differ for the inclusion of the CPS’ defining criteria ( 29 ): i.e., appropriateness (OR condition), novelty (RA condition), and a balance between these two (CR condition). Our task provided us to address several related questions.
We first adopted a network science approach to explore the nature of associative responses by modeling them as conceptual networks for each condition. Within this framework, we measured and compared the networks’ efficiency in terms of both structural properties and resilience when undergoing percolation analysis ( 15 , 31 , 33 ). We found that children's associative performance in the creative condition significantly leads to increased small-worldness, compared to both the OR and RA conditions. Our results are consistent with previous studies demonstrating that high levels of small-worldness mark a maximization of network efficiency ( 68 ), associated with higher levels of CPS and cognitive flexibility, in both adults ( 69 , 33 , 48 ) and children ( 49 ). More in-depth evidence of the efficient structure of the CR network is provided by the comparison of all remaining topological quantifiers, whose values consistently lie in between the OR and RA networks.
At one end of this continuum, the OR network shows the most rigid organization ( 51 ), characterized by a better local organization of associations but also by less-interconnected nodes and greater modularity (i.e,. highest CC, ASPL, and modularity, respectively). This is in line with our expectation since the network configuration during the OR condition was guided by the inclusion of only the appropriateness criteria, which increased the likelihood of activating nearby nodes while constraining the search and inhibiting the spreading of activation. The rigidity of such network configuration has been well-documented in the literature ( 41 ). For example, some studies found this pattern to be advantageous for information search, predominantly encountered in individuals with high deductive reasoning scores both in children ( 49 ) and adults ( 69 , 70 , 71 ). More often, networks with higher ASPL and Q tend to reflect an impairment in the efficiency of the search process ( 1 , 51 ). For instance, individuals with high-functioning autism have been found to have a more modular semantic network than neurotypical controls ( 51 ). A possible explanation proposed by the authors is that higher levels of segregation could make it more difficult to integrate information since the network breaks apart into smaller subparts.
When compared to OR, the CR network showed lower ASPL and modularity, which in turn has been linked with higher network flexibility. The CR semantic network configuration has already been linked to both higher levels of CPS abilities ( 15 , 33 ) and cognitive flexibility ( 27 , 48 ). As compared to CR, the RA network significantly decreased in all topological quantifiers.
Indeed, at the other end of the continuum, the RA network was the most chaotic, having locally poorly connected nodes, a shorter average distance between nodes, and the lowest modularity (i.e., lowest CC, ASPL, and modularity, respectively). Although a chaotic semantic network state allows for more flexible creative processing, here we interpret the findings on the RA network as representative of an over-flexible semantic memory state ( 72 ). Chaotic networks have been described as random networks, i.e., networks that are highly connected but poorly organized ( 72 ), assuming that all connections between two nodes are random and uniform ( 73 ). A similar network topology and language disorganization is a distinctive feature in psychosis ( 52 ).
Consistently, the percolation analysis revealed that the CR semantic network was significantly more resilient to the percolation process as compared to both control conditions. When undergoing a targeted attack, the CR network breaks apart more slowly than the OR and, in turn, the OR showed more robustness than RA, as indicated by a larger percolation integral. Again, these results corroborate those already found in the literature, linking this behavior in semantic networks with cognitive flexibility and CPS ( 31 , 27 , 48 ).
Another goal of this study was to clarify the role of semantic distance in light of the novelty-appropriateness trade-off during childhood. Since CPS relies on the notion that farther associations are more creative ( 16 ), we leveraged the distributional semantics approach using different pretrained word2vec models, to probe how semantic distance can disentangle the different search strategies adopted between conditions. Additionally, with the aim to quantify the number of deviations in categories tapped by responses, we proposed a new approach to quantify category switching based on distributional models.
Here, results from the distributional analysis showed that CR responses increased in both semantic distance and category switching rate with respect to OR responses. These findings acknowledge results from other studies regarding the role played by divergent thinking in achieving farther associations and reaching diverse categories among adults ( 30 , 43 , 44 ). However, when compared to RA, the CR associations were less distant, also showing less category switching. This is due to the more exploratory nature of the RA condition, which accounts solely for the search for novelty in the association. Critically, in contrast to the conventional assumption that novelty characterizes CPS, we showed the crucial prerequisite of the appropriateness criterion in understanding CPS, as a few previous studies have already highlighted ( 11 , 29 ).
Finally, we adopted a computational modeling approach to gain insights on the computational mechanisms operated by children for navigating the semantic space and generate responses given the target words and instruction set. We leveraged the RL framework ( 58 , 59 ) and proposed a semantic explorer model able to generate linguistic responses for the WAT. To the best of our knowledge, our model is the first in the literature aiming to provide a computational generative model for this task, exploiting state-of-the-art computational frameworks such as deep learning ( 66 ), for building the first component (semantic similarity matrix) of the SemExp model, and RL ( 59 ), for implementing the second component (action selection rule). Critically, our results provided insights on the semantic navigation strategies adopted by children, since in the RA condition the observed mean sample value of β was 0.76. This indicates that children's response generation policy was indeed dominated by an exploration factor, given that a value of β < 1 is commonly considered as indicating exploratory behavior ( 59 ). Crucially, we observed that in the CR condition the observed mean sample value of β was 2.34, indicating that children were exploiting their semantic knowledge to generate creative associations, but were more exploratory when compared to the OR condition (mean β = 2.47). Importantly, these findings help to create a bridge between different communities we believe being tightly connected, namely the creative problem-solving and the RL communities. We hope these results will shed more light on the connections between theoretical concepts such as bottom-up/top-down modes of thinking in creativity research and the exploration/exploitation trade-off in human RL research.
In summary, our study provides evidence for different kinds of results supporting optimal memory search during developmental age. The associations in the CR condition led to a more flexible and robust semantic network, as well as a balanced semantic distance and category switching compared to control conditions. Notably, in the CR condition, children's performance was closer to the OR than to RA (in terms of both networks and distributional semantics measures). This pattern is opposite to what Heinen and colleagues ( 29 ) found in the adult population, where the semantic distance in their creative condition was closer to the random condition. A possible explanation for this could be the fact that in children of the age of our sample, the vocabulary size is poorer than in adults, therefore it is generally more difficult for them to move away very far in the semantic space from the target word. In addition, viewed in the light of the recent developmental literature based on optimality search, newfound research also demonstrated that, even at the youngest age range, children do not solely rely on random exploration instead they are able to efficiently adapt their search behavior ( 5 , 74–76 ).
Several limitations need to be taken into consideration. The first relied on observed differences in the fluency of word association, probably due to either difference in cognitive load between conditions, or individual differences in verbal fluency. Despite we dealt with this issue throughout the analyses, further studies are encouraged to control for this factor, for instance using a fixed range of responses ( 29 ). Also, a possible confounding factor could be the order of presentation of the conditions. Although we followed the logic of previous studies, highlighting the importance of not presenting first the creative condition, we encourage future studies to investigate the importance of counterbalancing the conditions’ order. Next, as already pointed out in the literature ( 30 ), when cued to be random, participants’ word associations may be artificially modulated by using different strategies (e.g., finding inspiration around the room, following category-based or phonological-based strategies), affecting the final score. A possible intervention in this regard could be to check the used strategy by asking participants at the end of the task. Another limitation is that we do not directly compare our data to those of adults, nor between different ages during development. Finally, a possible improvement could be to increase the number of measures used. Given the parallels with other trade-offs, such as the exploration-exploitation, deliberate/controlled cognitive processes, or more broadly, the crucial role of executive functions in CPS, future studies could replicate and extend our results by implementing other measures and tasks.
In conclusion, our findings provide evidence that 10-years-old children implement CPS similarly to adults, efficiently solving the tension between a conventional and a chaotic semantic search strategy. Furthermore, we corroborated the notion that CPS can be fully investigated only when both novelty and appropriateness components are considered. Overall, our findings lay a bridge between developmental science, and the creative and semantic cognition communities, promoting a multidisciplinary approach to the investigation of how CPS skills develop in humans and providing a critical understanding of the behavioral principles underlying creative cognition during childhood.
Participants
We recruited 61 participants from a primary school in Rovereto (Italy). Children were thoroughly screened to exclude individuals with a history of neurological diseases and learning disabilities affecting language. Two volunteers were excluded from the analysis because gave incomplete fulfillment of the data collections. The final pool results of 56 healthy children (32 male), Italian language speakers, aged between 10 and 11 years ( |$M\ = $| 10.05, |$SD$| = 0.23). No statistical methods were used to predetermine sample sizes, but our sample size is comparatively similar to experimental studies adopting a within-subject design ( 77 ). A parent or legal guardian provided informed consent for their children's participation. The study protocol was approved by the Human Research Ethics Committee of the University of Trento (prot. 2018–027).
The experiment consisted of three conditions where participants performed the WAT following different instructions (see ( 29 ) for similar procedures). Following previous studies ( 29 , 78 ), conditions were always administered in the following order: OR, RA, and CR. The target words ( n = 40), within each condition, were randomized across participants. The WAT was administered as a paper and pencil task. The study was conducted in the classroom, during usual class hours, from 2019 January to March (completed in groups of ∼20 participants). During the experiment, volunteers were spaced apart and supervised by two of the authors. Each condition lasted 1 hour and was collected on different days (∼1-week interval between conditions); with a total duration of ∼3 hours.
In the WAT, participants had one minute to generate as many related responses as possible to a given target word. Participants were asked to respond using a single or a compound word and avoiding proper names. In this task, 40 target words were presented in each condition (Fig. 1c ), belonging to 10 categories, and counterbalanced for the domain in natural and artifact concepts ( 79 , 80 ). Semantic categories were selected a priori from a list of 32 Italian production norms gathered by ( 81 ) and accordingly to the most commonly used categories in the cognitive neuroscience literature ( 80 , 82 , 83 ). Target words were extracted from the Lexvar database ( 84 ) and controlled for concreteness, familiarity, and imageability. See the " Supplementary Materials " section for a detailed description of the stimuli selection procedure ( Table S1 ). Before analyzing the data, we preprocessed the raw responses to the WAT by excluding idiosyncratic answers and nonwords and controlling for other possible confounds (i.e., spell-checked, converted plural words into singular, transformed to lowercase, cleared of nonalphabetic tokens and stop-words). Additionally, responses that contain the target words were not included in the analysis.
Human rating analysis
We collected human ratings from eight raters on a subsample of our data. We presented the unique responses of 10 out of 40 target words (one for each category) to each rater and ask them to rate with a 5-point Likert scale (ranging from not at all as 1 to very much as 5) each target-response pair on three scales, namely novelty, appropriateness and creativity. To estimate the inter-raters reliability, we computed the ICC among all the raters on each scale across all the target-response pairs. Then, we averaged the raters’ scores on each scale for each participant and condition. We used a two-tailed paired-samples permutation t test analysis ( |$\alpha $| = 0.05 adjusted using Bonferroni correction for three pairwise comparisons |$\alpha $| = 0.017; 10,000 iterations) to investigate the statistical differences between conditions for each scale.
Semantic network structure analysis
First, we modeled the WAT responses as a group-based network using a recently developed ( 60 ) yet applied framework ( 15 , 33 , 48 , 49 ). In each network, nodes represent target words, and edges represent relations between two vertices. This link is based on the extent to which participants generated similar associative responses to a pair of target words and the number of participants who generated similar associative responses ( 33 ). Accordingly, the greater the number of similar associations generated between a pair of target words and the larger the number of participants who generated these associations, the higher the strength between the pair of words. In order to construct the semantic network, we structured the data into a n × m matrix for each condition, in which each column m represents the target word (i.e., nodes), and each row n represents a unique response given by the entire sample. On each cell, responses were encoded using the number of participants which provided the response n to the target word m and 0 otherwise.
Thus, we constructed a word-similarity matrix by computing the cosine similarity between the vectors associated with each target word in a pairwise fashion. We obtained an adjacency matrix of a fully connected, weighted network, with target words as columns and rows, and cells as the weights of the links between the words. To ensure that only relevant information is retained in the network, we removed spurious associations (i.e., weak similarity) by filtering the adjacency matrices with the Triangulated Maximally Filtered Graph (TMFG) method ( 61 ). The result was an undirected weighted semantic network containing the target words as nodes and the cosine similarity as links, for each condition.
We performed thorough descriptive analyses of the structure of the conditions’ semantic networks. We calculated the following topological measures on each network: the CC ( 63 ), ASPL( 85 ), Q ( 64 ), and S ( 62 ). The CC measures how closely the nodes in a network cluster together, and it is considered a measure of connectivity. The higher the CC, the better the local organization, and the stronger the connection within the network. Here, network clustering is based on transitivity ( 63 ). The ASPL determines the average number of steps required for all possible pairs of network nodes. A lower ASPL value may help reach relatively remote nodes faster ( 85 ). The Q evaluates how a network is split into subnetworks ( 64 ). Finally, we computed the small-worldness of the conditions’ semantic networks to provide additional context to the CC and ASPL results. Indeed, since small-world networks are highly clustered (e.g., lattice graphs), with an averaged short path lengths (e.g., random networks), a network G with n nodes and m edges is defined as a small-world network ( 85 ) if it has a similar path length but greater clustering of nodes than an equivalent Erdös-Rényi random graph with the same m and n ( 62 ). In this way, the S indicates the extent to which nodes that are not directly connected can be reached through connections between their neighbors.
Two complementary methods of statistical analysis were used, LONO and LOSO. Implementing the LONO approach, we constructed several partial networks resulting from the exclusion of one node at each iteration, for every node ( n = 40). Then we calculated the before mentioned network measures on the partial networks. In a similar vein, applying the LOSO procedure, we excluded one participant at each iteration and repeat the entire pipeline for building the semantic networks and computing the network measures, for every participant ( n = 56). We used a two-tailed paired-samples permutation t test analysis ( |$\alpha $| = 0.05 adjusted using Bonferroni correction for three pairwise comparisons |$\alpha $| = 0.017; 10,000 iterations) to investigate the statistical differences between conditions for each measure in both LONO and LOSO. We also used Cohen's d as a measure of effect size. Network analyses were conducted in R and Python using the NetworkToolbox package ( 86 ) and the NetworkX library ( 87 ), respectively; inferential statistics and data visualization were implemented in Python.
Additionally, to control for associative fluency bias, we performed the above network analysis only based on the first two associative responses given by each participant to a target word ( 33 ). We first selected a subset of the raw response's dataset, including the first two responses given by a participant to each target word and for each condition. Afterward, we analyzed differences between conditions in semantic networks only considering the first two association responses from the sample.
Network percolation analysis
Network percolation analysis enables us to estimate networks’ robustness and their structure flexibility ( 88 ), inferred by how fast the network breaks apart under a targeted “attack” ( 31 ). In the percolation analysis, networks are “attacked” by removing edges that fall beneath a certain threshold, named the percolation step ( 31 ). Here, the smallest weight in the network was used as the initial threshold, and the threshold resolution was computed as the lowest difference between the sorted weights. At each percolation step, we measure the LCCS, which is the size of the LCCS, or the cluster of nodes connected only to each other. The percolation process ended when the LCCS had fewer than three nodes. Once the percolation process terminated, we calculated the percolation integral |$\phi $| indicative of the area under the curve, representing the LCCS across all the percolation steps. Indeed, the LCCS is similarly defined as the sum of all LCCS weighted by their weight threshold value ( 31 ). In this study, we implemented percolation analysis using the weighted unfiltered networks previously constructed from the WAT data. According to Kenett and colleagues ( 31 ), we control for the fluency bias (i.e., numerous answers were possible for each target word) estimating the ratio between the average amount of responses for each condition. Therefore, we normalized the weights of the RA network by a factor of 2.20 (the ratio between the average amount of responses to RA and CR conditions) and the weights of OR network by a factor of 1.67 (the ratio between the average amount of responses of OR and CR conditions). See Fig. S1 in Supplementary Materials for a comparison of the distribution of link strengths in both networks.
We then used four approaches to examine the statistical significance of our findings, namely LOSO, LONO, the link shuffling, and the effect of noise analyses. In accordance with the previous analysis, to conduct the LONO and LOSO procedures, we iteratively excluded one node or one participant, respectively. Then the percolation procedure was run on the resulted partial networks, computing the percolation integral |$\phi $| . Next, the Link Shuffling analysis was conducted in order to run out the case that differences between networks were due to a difference in link weights. This was done by randomly selecting two pairs of nodes and exchanging their links in the network. For example, given a pair of nodes a and b with link strength 0.5 and a pair of nodes c and d with link strength 0.7 we exchanged edge weight such that a and b are connected with 0.7 and c and d with 0.5. For each link in each network, this process was repeated 10 times, aiming to exchange the majority of the links (∼ 1140 shuffles). This procedure was repeated with 500 iterations, computing |$\phi $| on the link-shuffled network at each iteration. Lastly, we implemented the noise analysis by adding noise to network link weights, using 500 iterations, and then computing the |$\phi $| . According to ( 31 ), in each iteration, Gaussian noise was added to the network links with a mean value of zero and a varied standard variation, of 10 to 4 to 10 to 3.
We then conducted permutation t tests ( |$\alpha $| = 0.05,10,000 iterations) between the |$\phi $| of the CR, OR, and RA conditions for each procedure described above. We corrected for multiple comparisons using Bonferroni correction ( |$\alpha $| = 0.017).
Distributional semantics analysis
Computational modeling analysis.
This manuscript was posted on a preprint: https://psyarxiv.com/2tjhd . Importantly, we would like to extend our appreciation for the availability and assistance of the headmaster and teachers at the “F.lli Filzi” Primary School in Rovereto (Italy). We thank especially the children who made this study possible.
This study was supported by a grant from the Italian Ministry of University and Research (Excellence Department Grant awarded to the Department of Psychology and Cognitive Science, University of Trento, Italy).
C.R. and C.F. conceived and designed the study. C.R. and A.G. performed the analysis. C.R. and C.F. carried out the experiment. C.R. leads in writing the manuscript. A.G. and N.D.P. provided critical feedback and helped shape the research and manuscript. C.F. supervised the project.
The datasets generated and analyzed during the current study are not publicly available to comply with the EU's General Data Protection Regulations 2018 (GPDR), since participants did not provide explicit written consent regarding the sharing of their data on public repositories. Researchers interested in the data need to contact the corresponding authors and provide a research plan for using the data. This plan will be submitted to the ethical committee to obtain access to the data. Source data regarding all the results and statistics displayed in the tables and figures are provided at the following link: https://osf.io/vumf3/ . Regarding the code, all the analyses were carried out using R (version 4.1.1) and Python (version 3.7).
Semantic network analyses were carried out using the following publicly available libraries:
- The SemNeT package (1.4.4). https://github.com/AlexChristensen/SemNeT
- The NetworkToolbox package (1.4.2). https://cran.r-project.org/web/packages/NetworkToolbox/index.html
- The NetworkX package (2.8.6). https://networkx.org/
Distributional semantics and computational modeling analyses were carried out using the following publicly available libraries:
- The Scipy package (1.9.0). https://scipy.org/
- The Tensorflow package (2.5). https://www.tensorflow.org/
The word2vec deep learning models utilized in this study are available at the following links:
- The first model was trained with the CBOW on italian Common Crawl and Wikipedia using fastText. https://fasttext.cc./docs/en/crawl-vectors.html
- The second model was trained with the Skip-gram method on italian Wikipedia. https://wikipedia2vec.github.io/wikipedia2vec/pretrained/
- The third model was trained with the Skip-gram method using fastText on the Italian OpenSubtiles corpus. https://github.com/jvparidon/subs2vec
Classification: Psychological and Cognitive Sciences
Competing Interest: Authors declare no competing interests.
Wulff DU , De Deyne S , Jones MN , Mata R . 2019 . New Perspectives on the aging Lexicon . Trends Cogn Sci . 23 : 686 – 698 .
Google Scholar
Sumner E , et al. 2019 . The exploration advantage: children's instinct to explore allows them to find information that adults miss . PsyArXiv. https://doi.org/10.31234/osf.io/h437v .
Gopnik A , et al. 2017 . Changes in cognitive flexibility and hypothesis search across human life history from childhood to adolescence to adulthood . Proc Natl Acad Sci . 114 : 7892 – 7899 .
Giron AP , et al. 2022 . Developmental changes in learning resemble stochastic optimization . PsyArXiv. https://doi.org/10.31234/osf.io/9f4k3 .
Hart Y , et al. 2022 . The development of creative search strategies . Cognition . 225 : 105102 .
Blanco NJ , Sloutsky VM . 2021 . Systematic exploration and uncertainty dominate young children's choices . Dev Sci . 24 : e13026 .
Osborn AF . 1953 . Applied imagination . New York : Scribner .
Hart Y , et al. 2018 . Creative exploration as a scale-invariant search on a meaning landscape . Nat Commun . 9 : 5411 .
Uddin LQ . 2021 . Cognitive and behavioural flexibility: neural mechanisms and clinical considerations . Nat Rev Neurosci . 22 : 167 – 179 .
Campbell DT . 1960 . Blind variation and selective retentions in creative thought as in other knowledge processes . Psychol Rev . 67 : 380 .
Harvey S , Berry J . 2022 . Toward a meta-theory of creativity forms: how novelty and usefulness shape creativity . AMR . https://doi.org/10.5465/amr.2020.0110 (March 28, 2022) .
Hills TT , Todd PM , Lazer D , Redish AD , Couzin ID . 2015 . Exploration versus exploitation in space, mind, and society . Trends Cogn Sci . 19 : 46 – 54 .
Sripada CS . 2018 . An exploration/exploitation trade-off between mind wandering and goal-directed thinking . Oxf Handb Spontaneous Thought Mind-Wander Creat Dreaming . 34 : 23 – 34 .
Hernández-Torrano D , Ibrayeva L . 2020 . Creativity and education: a bibliometric mapping of the research literature (1975–2019) . Think Ski Creat . 35 : 100625 .
Kenett YN , Faust M . 2019 . A semantic network cartography of the creative mind . Trends Cogn Sci . 23 : 271 – 274 .
Mednick S . 1962 . The associative basis of the creative process . Psychol Rev . 69 : 220 – 232 .
Stein MI . 1953 . Creativity and culture . J Psychol . 36 : 311 – 322 .
JP G . 1950 . Creativity . Am Psychol . 5 : 444 – 454 .
Runco MA , Jaeger GJ . 2012 . The standard definition of creativity . Creat Res J . 24 : 92 – 96 .
Said-Metwaly S , Fernández-Castilla B , Kyndt E , Van den Noortgate W , Barbot B . 2020 . Does the fourth-Grade slump in creativity actually exist? A meta-analysis of the development of divergent thinking in school-Age children and adolescents . Educ Psychol Rev . 1 – 24 .. https://doi.org/10.1007/s10648-020-09547-9 .
Ralph MAL , Jefferies E , Patterson K , Rogers TT . 2017 . The neural and computational bases of semantic cognition . Nat Rev Neurosci . 18 : 42 – 55 .
Lake BM , Murphy GL . 2021 . Word meaning in minds and machines . Psychol Rev . https://doi.org/10.1037/rev0000297 .
Hass RW . 2017 . Semantic search during divergent thinking . Cognition . 166 : 344 – 357 .
Gray K , et al. 2019 . “Forward flow”: a new measure to quantify free thought and predict creativity . Am Psychol . 74 : 539 – 554 .
Kenett YN , Austerweil JL . 2016 . Examining search processes in low and high creative individuals with random . CogSci . 8 : 313 – 318 .
Kenett Y , Thompson-Schill SL . 2020 . Novel conceptual combination can dynamically reconfigure semantic memory networks . PsyArXiv . https://doi.org/10.31234/osf.io/crp47 .
Rastelli C , Greco A , Kenett YN , Finocchiaro C , De Pisapia N . 2022 . Simulated visual hallucinations in virtual reality enhance cognitive flexibility . Sci Rep . 12 : 4027 .
Hills TT , Jones MN , Todd PM . 2012 . Optimal foraging in semantic memory . Psychol Rev . 119 : 431 – 440 .
Heinen DJP , Johnson DR . 2018 . Semantic distance: an automated measure of creativity that is novel and appropriate . Psychol Aesthet . 12 : 144 – 156 .
Olson JA , Nahas J , Chmoulevitch D , Cropper SJ , Webb ME . 2021 . Naming unrelated words predicts creativity . Proc Natl Acad Sci . 118 .
Kenett YN , et al. 2018 . Flexibility of thought in high creative individuals represented by percolation analysis . Proc Natl Acad Sci . 115 : 867 – 872 .
Beaty RE , Johnson DR . 2021 . Automating creativity assessment with SemDis: an open platform for computing semantic distance . Behav Res Methods . 53 : 757 – 780 .. Springer .
Kenett YN , Anaki D , Faust M . 2014 . Investigating the structure of semantic networks in low and high creative persons . Front Hum Neurosci . 8 : 407 .
Kenett YN . 2019 . What can quantitative measures of semantic distance tell us about creativity? . Curr Opin Behav Sci . 27 : 11 – 16 .
Collins AM , Loftus EF . 1975 . A spreading-activation theory of semantic processing . Psychol Rev . 82 : 407 – 428 .
Harris ZS . 1954 . Distributional structure . Word . 10 : 146 – 162 .
Firth JR . 1957 . A synopsis of linguistic theory, 1930-1955 . Studies in linguistic analysis . Oxford, UK : Blackwell . p. 1 – 32 .
Google Preview
Dubossarsky H , De Deyne S , Hills TT . 2017 . Quantifying the structure of free association networks across the life span . Dev Psychol . 53 : 1560 .
Zortea M , Menegola B , Villavicencio A , de Salles JF . 2014 . Graph analysis of semantic word association among children, adults, and the elderly . Psicol Reflex Crit . 27 : 90 – 99 .
Noh JD , Rieger H . 2004 . Random Walks on complex networks . Phys Rev Lett . 92 : 118701 .
Siew CS , Wulff DU , Beckage NM , Kenett YN . 2019 . Cognitive network science: a review of research on cognition through the lens of network representations, processes, and dynamics . complex . 2019 : 1 – 24 .
Vales C , Fisher AV . 2019 . When stronger knowledge slows you down: semantic relatedness predicts children's co-activation of related items in a visual search paradigm . Cogn Sci . 43 : e12746 .
Acar S , Runco MA . 2014 . Assessing associative distance among ideas elicited by tests of divergent thinking . Creat Res J . 26 : 229 – 238 .
Johnson DR , Hass RW . 2022 . Semantic context search in creative idea generation . J Creat Behav . 56 ( 3 ): 362 – 381 .
Carson SH , Peterson JB , Higgins DM . 2003 . Decreased latent inhibition is associated with increased creative achievement in high-functioning individuals . J Pers Soc Psychol . 85 : 499 – 506 .
Beaty RE , Silvia PJ , Nusbaum EC , Jauk E , Benedek M . 2014 . The roles of associative and executive processes in creative cognition . Mem Cognit . 42 : 1186 – 1197 .
Ovando-Tellez M , et al. Brain connectivity–based prediction of real-life creativity is mediated by semantic memory structure . Sci Adv . 8 : eabl4294 .
Cosgrove AL , Kenett YN , Beaty RE , Diaz MT . 2021 . Quantifying flexibility in thought: the resiliency of semantic networks differs across the lifespan . Cognition . 211 : 104631 .
Rastelli C , Greco A , Finocchiaro C . 2020 . Revealing the role of divergent thinking and fluid intelligence in children's semantic Memory organization . J Intell . 8 : 43 .
Benedek M , Neubauer AC . 2013 . Revisiting mednick's model on creativity-related differences in associative hierarchies. Evidence for a common path to uncommon thought . J Creat Behav . 47 : 273 – 289 .
Kenett YN , Gold R , Faust M . 2016 . The hyper-modular associative mind: a computational analysis of associative responses of persons with Asperger syndrome . Lang Speech . 59 : 297 – 317 .
Alonso-Sánchez MF et al. 2022 . Progressive changes in descriptive discourse in first Episode Schizophrenia: a longitudinal computational semantics study . Schizophr . 8 : 1 – 9 .
Runco MA , Okuda SM . 1991 . The instructional enhancement of the flexibility and originality scores of divergent thinking tests . Appl Cogn Psychol . 5 : 435 – 441 .
Nussenbaum K , Hartley CA . 2019 . Reinforcement learning across development: what insights can we draw from a decade of research? . Dev Cogn Neurosci . 40 : 100733 .
Schulz E , Wu CM , Ruggeri A , Meder B . 2019 . Searching for rewards like a child means less generalization and more directed exploration . Psychol Sci . 30 : 1561 – 1572 .
Diamond A . 2013 . Executive functions . Annu Rev Psychol . 64 : 135 – 168 .
Davidson MC , Amso D , Anderson LC , Diamond A . 2006 . Development of cognitive control and executive functions from 4 to 13 years: evidence from manipulations of memory, inhibition, and task switching . Neuropsychologia . 44 : 2037 – 2078 .
Sutton RS , Barto AG . 1981 . Toward a modern theory of adaptive networks: expectation and prediction . Psychol Rev . 88 : 135 – 170 .
Sutton RS , Bart. AG . 2018 . Reinforcement learning: An introduction . 2nd ed. Cambridge, MA : The MIT Press .
Kenett Y et al. 2013 . Semantic organization in children with cochlear implants: computational analysis of verbal fluency . Front Psychol . 4 : 543 .
Massara GP , Di Matteo T , Aste T . 2017 . Network filtering for big data: triangulated maximally filtered graph . J Complex Netw . 5 : 161 – 178 .
Humphries MD , Gurney K . 2008 . Network ‘small-World-Ness’: a quantitative method for determining canonical Network equivalence . PLoS One . 3 : e0002051 .
Newman ME , Moore C , Watts DJ . 2000 . Mean-field solution of the small-world network model . Phys Rev Lett . 84 : 3201 .
Newman MEJ . 2006 . Modularity and community structure in networks . Proc Natl Acad Sci . 103 : 8577 – 8582 .
Cohen J . 1992 . A power primer . Psychol Bull . 112 : 155 .
Mikolov T , Sutskever I , Chen K , Corrado GS , Dean J . 2013 . Distributed representations of words and phrases and their compositionality . Adv Neural Inf Process Syst . 26 : 3111 – 3119 .. Curran Associates Inc., Red Hook, NY, USA .
Mandera P , Keuleers E , Brysbaert M . 2017 . Explaining human performance in psycholinguistic tasks with models of semantic similarity based on prediction and counting: a review and empirical validation . J Mem Lang . 92 : 57 – 78 .
Motter AE , de Moura APS , Lai Y-C , Dasgupta P . 2002 . Topology of the conceptual network of language . Phys Rev E . 65 : 065102 .
Benedek M , et al. 2017 . How semantic memory structure and intelligence contribute to creative thought: a network science approach . Think Reason . 23 : 158 – 183 .
Borodkin K , Kenett YN , Faust M , Mashal N . 2016 . When pumpkin is closer to onion than to squash: the structure of the second language lexicon . Cognition . 156 : 60 – 70 .
Kenett YN , Beaty RE , Silvia PJ , Anaki D , Faust M . 2016 . Structure and flexibility: investigating the relation between the structure of the mental lexicon, fluid intelligence, and creative achievement . Psychol Aesthet . 10 : 377 – 388 .
Faust M , Kenett YN . 2014 . Rigidity, chaos and integration: hemispheric interaction and individual differences in metaphor comprehension . Front Hum Neurosci . 8 : 511 .
Barabási A-L , Albert R , Jeong H . 2000 . Scale-free characteristics of random networks: the topology of the world-wide web . Physica A . 281 : 69 – 77 .
Ruggeri A , Lombrozo T . 2015 . Children adapt their questions to achieve efficient search . Cognition . 143 : 203 – 216 .
Bonawitz EB , van Schijndel TJ , Friel D , Schulz L . 2012 . Children balance theories and evidence in exploration, explanation, and learning . Cognit Psychol . 64 : 215 – 234 .
Ruggeri A , Lombrozo T , Griffiths TL , Xu F . 2016 . Sources of developmental change in the efficiency of information search . Dev Psychol . 52 : 2159 – 2173 .
Brysbaert M . 2019 . How many participants do we have to include in properly powered experiments? A tutorial of power analysis with reference tables . J Cogn . 2 ( 1 ). Article 16 .
Harrington DM . 1975 . Effects of explicit instructions to “be creative” on the psychological meaning of divergent thinking test scores 1 . J Pers . 43 : 434 – 454 .
Garrard P , Lambon Ralph MA , Hodges JR , Patterson K . 2001 . Prototypicality, distinctiveness, and intercorrelation: analyses of the semantic attributes of living and nonliving concepts . Cogn Neuropsychol . 18 : 125 – 174 .
McRae K , Cree GS , Seidenberg MS , Mcnorgan C . 2005 . Semantic feature production norms for a large set of living and nonliving things . Behav Res Methods . 37 : 547 – 559 .
Montefinese M , Ambrosini E , Fairfield B , Mammarella N . 2013 . Semantic memory: a feature-based analysis and new norms for Italian . Behav Res Methods . 45 : 440 – 461 .
De Deyne S , Navarro DJ , Perfors A , Brysbaert M , Storms G . 2016 . Measuring the associative structure of English: the “Small World of Words” norms for word association . Behav Res Methods . 51 ( 3 ): 987 – 1006 .
Kremer G , Baroni M . 2011 . A set of semantic norms for German and italian . Behav Res Methods . 43 : 97 – 109 .
Barca L , Burani C , Arduino LS . 2011 . Lexical and Sublexical Variables: Norms for 626 Italian Nouns . Available online at: http://www.istc.cnr.it/it/grouppage/lexvar .
Watts DJ , Strogatz SH . 1998 . Collective dynamics of ‘small-world’ networks . Nature . 393 : 440 – 442 .
Christensen AP . 2018 . NetworkToolbox: methods and measures for brain, cognitive, and psychometric network analysis in R . R J . 10 : 422 .
Hagberg AA , Schult DA , Swart PJ . 2008 . Exploring network structure, dynamics, and function using Network . Varoquaux XG , Vaught T , Millman J . Proceedings of the 7th Python in Science Conference (SciPy2008) . Pasadena, CA USA . pp. 11 – 15 .
Cohen R , Havlin S . 2010 . Complex networks: structure, robustness and function . Cambridge : Cambridge University Press .
Lenci A , Sahlgren M , Jeuniaux P , Cuba Gyllensten A , Miliani M . 2022 . A comparative evaluation and analysis of three generations of distributional semantic models . Lang Resour Eval . 56 : 1269 – 1313 .. https://doi.org/10.1007/s10579-021-09575-z .
Bojanowski P , Grave E , Joulin A , Mikolov T . 2017 . Enriching word vectors with subword information . Trans Assoc Comput Linguist . 5 : 135 – 146 .
Grave E , Bojanowski P , Gupta P , Joulin A , Mikolov T . 2018 . Learning word vectors for 157 languages . Proceedings of the Eleventh International Conference on Language Resources and Evaluation (LREC) . 3483 – 3487 .. Miyazaki, Japan .
Yamada I , et al. 2018 . Wikipedia2Vec: an efficient toolkit for learning and visualizing the embeddings of words and entities from Wikipedia . Proceedings of the 2020 Conference on Empirical Methods in Natural Language Processing: System Demonstrations . 23 – 30 .. https://aclanthology.org/2020.emnlp-demos.4 .
Van Paridon J , Thompson B . 2021 . subs2vec: word embeddings from subtitles in 55 languages . Behav Res Methods . 53 : 629 – 655 .
Van der Maaten L , Hinton G . 2008 . Visualizing data using t-SNE . J Mach Learn Res . 9 : 2579 – 2605 .
Abadi M , et al. 2016 . Tensorflow: large-scale machine learning on heterogeneous distributed systems . arXiv, https://arxiv.org/abs/1603.04467 .
Supplementary data
Email alerts, citing articles via.
- Contact PNAS Nexus
- Advertising and Corporate Services
- Journals Career Network
Affiliations
- Online ISSN 2752-6542
- Copyright © 2024 National Academy of Sciences
- About Oxford Academic
- Publish journals with us
- University press partners
- What we publish
- New features
- Open access
- Institutional account management
- Rights and permissions
- Get help with access
- Accessibility
- Advertising
- Media enquiries
- Oxford University Press
- Oxford Languages
- University of Oxford
Oxford University Press is a department of the University of Oxford. It furthers the University's objective of excellence in research, scholarship, and education by publishing worldwide
- Copyright © 2024 Oxford University Press
- Cookie settings
- Cookie policy
- Privacy policy
- Legal notice
This Feature Is Available To Subscribers Only
Sign In or Create an Account
This PDF is available to Subscribers Only
For full access to this pdf, sign in to an existing account, or purchase an annual subscription.
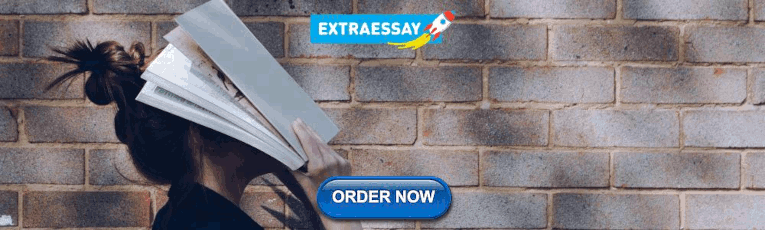
IMAGES
VIDEO
COMMENTS
What it Means to be Creative: The Ability to Solve Problems with Relevance and Novelty. When many people think of creativity, they think of artists, graphic designers, writers, painters, etc. But ...
Problem solving frequently occupies creativity research investigations. Problem solving that requires a creative solution is quite broad and can be classified as well-defined and as ill-defined (Wu et al., 2017). Well-defined problems have clearly stated goals, such as solving a complicated mathematical equation in a new way or building a ...
Its benefits include: Finding creative solutions to complex problems: User research can insufficiently illustrate a situation's complexity. While other innovation processes rely on this information, creative problem-solving can yield solutions without it. Adapting to change: Business is constantly changing, and business leaders need to adapt.
Yes, an artist could be creative, but so could a software engineer, a mathematician, a salesperson or a CEO. What does it actually mean to be creative? LinkedIn Learning Instructor Stefan Mumaw, who has authored six books on creativity, has this definition: "Creativity is problem-solving with relevance and novelty." Let's break that ...
The standard view in current philosophy of creativity says that being creative has two requirements: being novel and being valuable (to which a third intentionality requirement is often added; Sternberg and Lubart 1999; Boden 2004; Gaut 2010). The standard view on creativity has recently become an object of critical scrutiny. Hills and Bird (2018) have specifically proposed to remove the value ...
Though the precise terminology can vary (e.g., novelty may be referred to as originality or uniqueness, while usefulness may be referred to as appropriateness, relevance, or effectiveness), the twin criteria of novelty and usefulness have formed principal components of numerous definitions of creativity dating back at least 70 years (Amabile ...
Creativity has long been defined in terms of novelty and usefulness. Surprisingly, however, there is relatively little agreement about the precise meaning of either dimension, the relationship between them, or the process through which they are produced. In this paper, we explore how novelty and usefulness have been used explicitly and implicitly in the creativity literature to reveal three ...
Humans are innate creative problem-solvers. Since early humans developed the first stone tools to crack open fruit and nuts more than 2 million years ago, the application of creative thinking to solve problems has been a distinct competitive advantage for our species (Puccio 2017).Originally used to solve problems related to survival, the tendency toward the use of creative problem-solving to ...
A schematic overview of the neurobiology of creativity as outlined in this review. It symbolizes the brain systems and neuromodulatory pathways underlying and modulating creative cognition and creative drive in health and disease. The creative cognition is based on various cognitive functions such as cognitive flexibility, inhibitory control ...
A noted CPS educator and practitioner, Ruth Noller, describes Creative Problem Solving as the sum of its parts: Creative means having an element of newness and innovation, and relevance. Problem encompasses any situation that presents a challenge, offers an opportunity or is a concern.
Creativity is considered a crucial element in new product design and development. It is an integral part of design, contributing to problem-solving and innovative product development, occurring between the problem and solution space (Demirkan and Afacan 2012; Hsiao and MacDonald 2013).It is also claimed that creativity is a prerequisite for the generation of ideas for breakthrough products ...
The creative problem solving process also involves managing ... disruptive or breakthrough innovations with a higher degree of novelty (Steiner, 2009). ... followed by a more expansive problem frame that includes relevant values (financial, human and environmental sustainability) and barriers (cost), it may be possible to provoke more ...
You can get better creatively because creativity at its core is problem solving with two characteristics, relevance and novelty. Relevance is the degree by which a solution actually solves the ...
Abstract. Four processes are at the core of "creative" problem-solving: finding problems, generating novelty, defining solutions, and recognizing solutions. The statement of the problem itself ...
ELIZABETH C. HIRSCHMAN*. AA conceptual framework is presented that incorporates three constructs. highly relevant to consumer behavior-innovativeness, novelty seeking, and. consumer creativity. A fourth construct, role accumulation, is also discussed. AA model of the relational linkages among these constructs is developed, to- gether with their ...
Creative problem solving is a fundamental skill of human cognition and is conceived as a search process whereby a novel and appropriate solution is generated. However, it is unclear whether children are able to balance novelty and appropriateness to generate creative solutions and what are the underlying computational mechanisms.
conscious reasoning. Evidence for the importance of each of these activities to the creative process is drawn from (a) historical and introspective accounts of novel problem solving by noted scientists and mathematicians; (b) cognitive psychology and neuroscience; and (c) a recent empirical study of novel mathematics problem solving.
Learning to solve problems with high degrees of relevance ensures that we are setting ourselves up for creative success. In this video, dive deeper into the role of relevance in problem-solving.
The cognitive approach to creativity emphasizes the processes involved in producing effective novelty, as well as the control mechanisms that regulate novelty production, and the structures that result. Merely novel structures display surprisingness and incongruity, to be sure, but they must also be meaningful and practicable to be effective. There are no special processes or control ...
Creativity and problem-solving From the course: Creativity: Generate ... The role of relevance 3m 45s (Locked) The role of novelty, part 1 2m 34s ...
Join Julian Velard, Stefan Mumaw, Amy Wynne, Jim Krause, Ben Long, Tracey Clark, Denise Jacobs, and George Maestri for an in-depth discussion in this video, Problem-solve with relevance and ...