How To Make Conceptual Framework (With Examples and Templates)
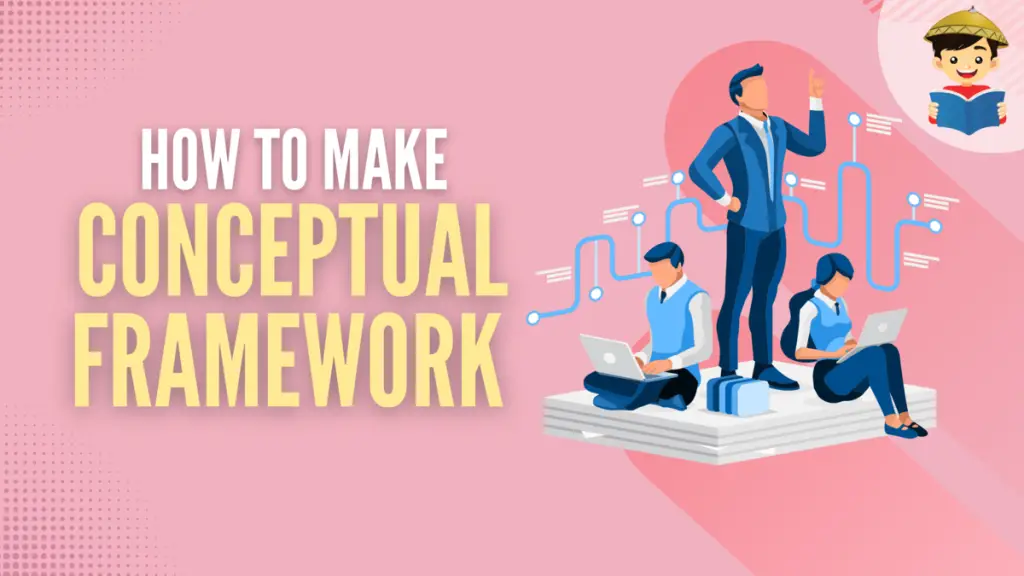
We all know that a research paper has plenty of concepts involved. However, a great deal of concepts makes your study confusing.
A conceptual framework ensures that the concepts of your study are organized and presented comprehensively. Let this article guide you on how to make the conceptual framework of your study.
Related: How to Write a Concept Paper for Academic Research
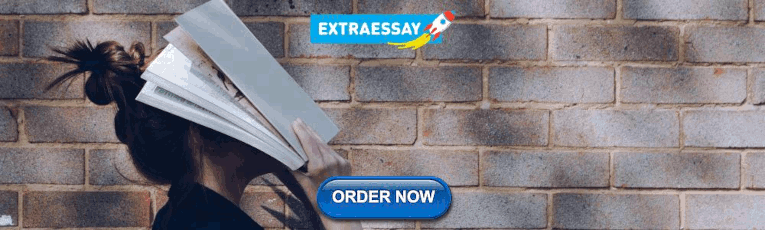
Table of Contents
At a glance: free conceptual framework templates.
Too busy to create a conceptual framework from scratch? No problem. We’ve created templates for each conceptual framework so you can start on the right foot. All you need to do is enter the details of the variables. Feel free to modify the design according to your needs. Please read the main article below to learn more about the conceptual framework.
Conceptual Framework Template #1: Independent-Dependent Variable Model
Conceptual framework template #2: input-process-output (ipo) model, conceptual framework template #3: concept map, what is a conceptual framework.
A conceptual framework shows the relationship between the variables of your study. It includes a visual diagram or a model that summarizes the concepts of your study and a narrative explanation of the model presented.
Why Should Research Be Given a Conceptual Framework?
Imagine your study as a long journey with the research result as the destination. You don’t want to get lost in your journey because of the complicated concepts. This is why you need to have a guide. The conceptual framework keeps you on track by presenting and simplifying the relationship between the variables. This is usually done through the use of illustrations that are supported by a written interpretation.
Also, people who will read your research must have a clear guide to the variables in your study and where the research is heading. By looking at the conceptual framework, the readers can get the gist of the research concepts without reading the entire study.
Related: How to Write Significance of the Study (with Examples)
What Is the Difference Between Conceptual Framework and Theoretical Framework?
Both of them show concepts and ideas of your study. The theoretical framework presents the theories, rules, and principles that serve as the basis of the research. Thus, the theoretical framework presents broad concepts related to your study. On the other hand, the conceptual framework shows a specific approach derived from the theoretical framework. It provides particular variables and shows how these variables are related.
Let’s say your research is about the Effects of Social Media on the Political Literacy of College Students. You may include some theories related to political literacy, such as this paper, in your theoretical framework. Based on this paper, political participation and awareness determine political literacy.
For the conceptual framework, you may state that the specific form of political participation and awareness you will use for the study is the engagement of college students on political issues on social media. Then, through a diagram and narrative explanation, you can show that using social media affects the political literacy of college students.
What Are the Different Types of Conceptual Frameworks?
The conceptual framework has different types based on how the research concepts are organized 1 .
1. Taxonomy
In this type of conceptual framework, the phenomena of your study are grouped into categories without presenting the relationship among them. The point of this conceptual framework is to distinguish the categories from one another.
2. Visual Presentation
In this conceptual framework, the relationship between the phenomena and variables of your study is presented. Using this conceptual framework implies that your research provides empirical evidence to prove the relationship between variables. This is the type of conceptual framework that is usually used in research studies.
3. Mathematical Description
In this conceptual framework, the relationship between phenomena and variables of your study is described using mathematical formulas. Also, the extent of the relationship between these variables is presented with specific quantities.
How To Make Conceptual Framework: 4 Steps
1. identify the important variables of your study.
There are two essential variables that you must identify in your study: the independent and the dependent variables.
An independent variable is a variable that you can manipulate. It can affect the dependent variable. Meanwhile, the dependent variable is the resulting variable that you are measuring.
You may refer to your research question to determine your research’s independent and dependent variables.
Suppose your research question is: “Is There a Significant Relationship Between the Quantity of Organic Fertilizer Used and the Plant’s Growth Rate?” The independent variable of this study is the quantity of organic fertilizer used, while the dependent variable is the plant’s growth rate.
2. Think About How the Variables Are Related
Usually, the variables of a study have a direct relationship. If a change in one of your variables leads to a corresponding change in another, they might have this kind of relationship.
However, note that having a direct relationship between variables does not mean they already have a cause-and-effect relationship 2 . It takes statistical analysis to prove causation between variables.
Using our example earlier, the quantity of organic fertilizer may directly relate to the plant’s growth rate. However, we are not sure that the quantity of organic fertilizer is the sole reason for the plant’s growth rate changes.
3. Analyze and Determine Other Influencing Variables
Consider analyzing if other variables can affect the relationship between your independent and dependent variables 3 .
4. Create a Visual Diagram or a Model
Now that you’ve identified the variables and their relationship, you may create a visual diagram summarizing them.
Usually, shapes such as rectangles, circles, and arrows are used for the model. You may create a visual diagram or model for your conceptual framework in different ways. The three most common models are the independent-dependent variable model, the input-process-output (IPO) model, and concept maps.
a. Using the Independent-Dependent Variable Model
You may create this model by writing the independent and dependent variables inside rectangles. Then, insert a line segment between them, connecting the rectangles. This line segment indicates the direct relationship between these variables.
Below is a visual diagram based on our example about the relationship between organic fertilizer and a plant’s growth rate.
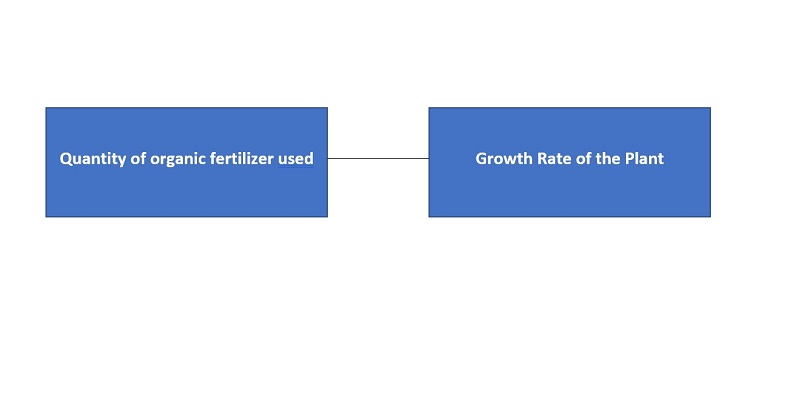
b. Using the Input-Process-Output (IPO) Model
If you want to emphasize your research process, the input-process-output model is the appropriate visual diagram for your conceptual framework.
To create your visual diagram using the IPO model, follow these steps:
- Determine the inputs of your study . Inputs are the variables you will use to arrive at your research result. Usually, your independent variables are also the inputs of your research. Let’s say your research is about the Level of Satisfaction of College Students Using Google Classroom as an Online Learning Platform. You may include in your inputs the profile of your respondents and the curriculum used in the online learning platform.
- Outline your research process. Using our example above, the research process should be like this: Data collection of student profiles → Administering questionnaires → Tabulation of students’ responses → Statistical data analysis.
- State the research output . Indicate what you are expecting after you conduct the research. In our example above, the research output is the assessed level of satisfaction of college students with the use of Google Classroom as an online learning platform.
- Create the model using the research’s determined input, process, and output.
Presented below is the IPO model for our example above.
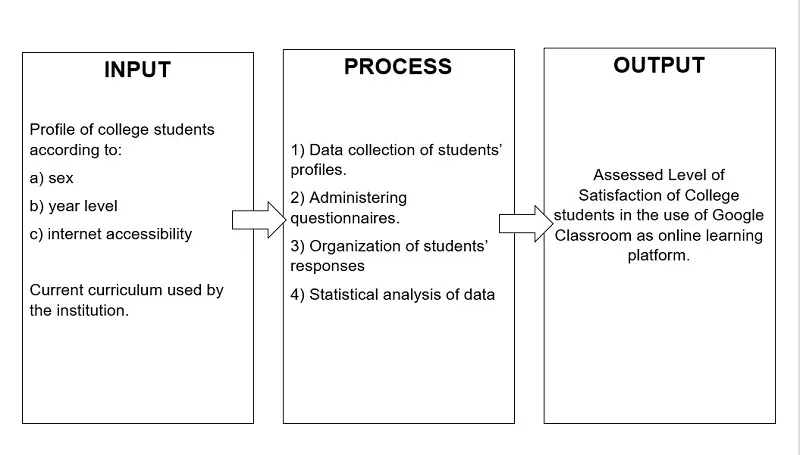
c. Using Concept Maps
If you think the two models presented previously are insufficient to summarize your study’s concepts, you may use a concept map for your visual diagram.
A concept map is a helpful visual diagram if multiple variables affect one another. Let’s say your research is about Coping with the Remote Learning System: Anxiety Levels of College Students. Presented below is the concept map for the research’s conceptual framework:
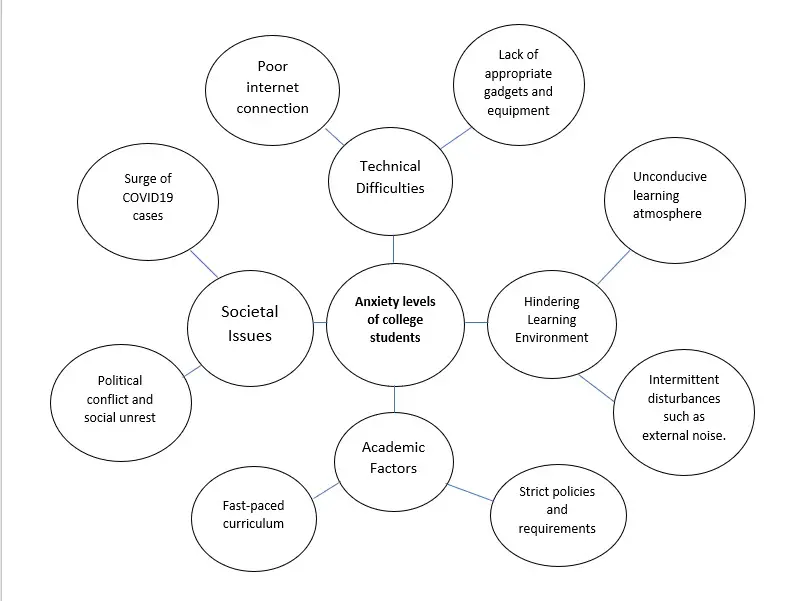
5. Explain Your Conceptual Framework in Narrative Form
Provide a brief explanation of your conceptual framework. State the essential variables, their relationship, and the research outcome.
Using the same example about the relationship between organic fertilizer and the growth rate of the plant, we can come up with the following explanation to accompany the conceptual framework:
Figure 1 shows the Conceptual Framework of the study. The quantity of the organic fertilizer used is the independent variable, while the plant’s growth is the research’s dependent variable. These two variables are directly related based on the research’s empirical evidence.
Conceptual Framework in Quantitative Research
You can create your conceptual framework by following the steps discussed in the previous section. Note, however, that quantitative research has statistical analysis. Thus, you may use arrows to indicate a cause-and-effect relationship in your model. An arrow implies that your independent variable caused the changes in your dependent variable.
Usually, for quantitative research, the Input-Process-Output model is used as a visual diagram. Here is an example of a conceptual framework in quantitative research:
Research Topic : Level of Effectiveness of Corn (Zea mays) Silk Ethanol Extract as an Antioxidant
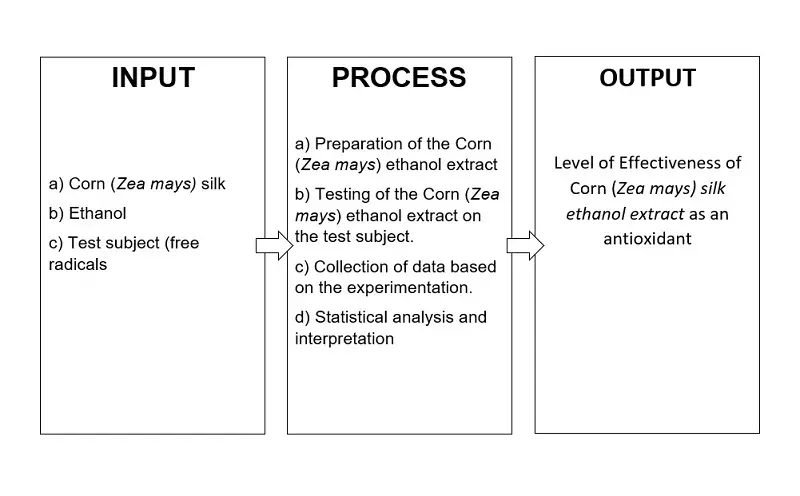
Conceptual Framework in Qualitative Research
Again, you can follow the same step-by-step guide discussed previously to create a conceptual framework for qualitative research. However, note that you should avoid using one-way arrows as they may indicate causation . Qualitative research cannot prove causation since it uses only descriptive and narrative analysis to relate variables.
Here is an example of a conceptual framework in qualitative research:
Research Topic : Lived Experiences of Medical Health Workers During Community Quarantine
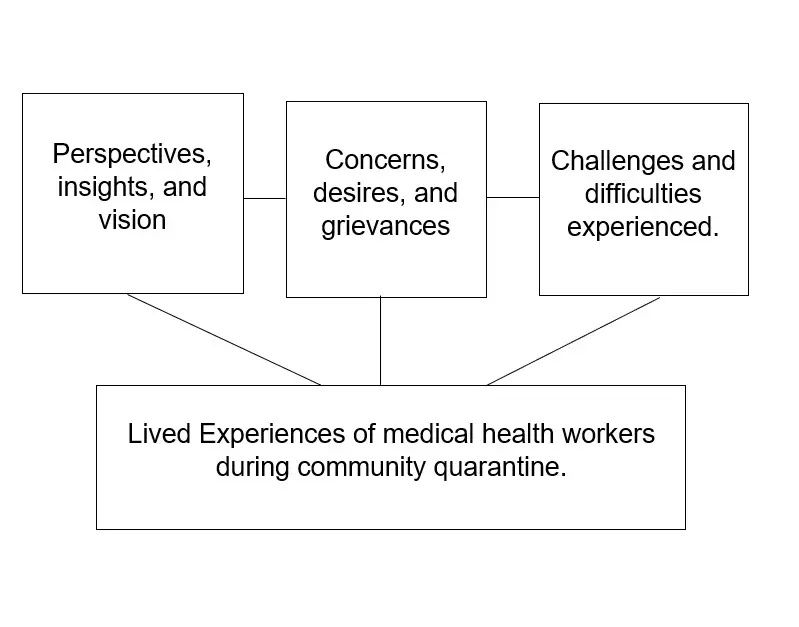
Conceptual Framework Examples
Presented below are some examples of conceptual frameworks.
Research Topic : Hypoglycemic Ability of Gabi (Colocasia esculenta) Leaf Extract in the Blood Glucose Level of Swiss Mice (Mus musculus)
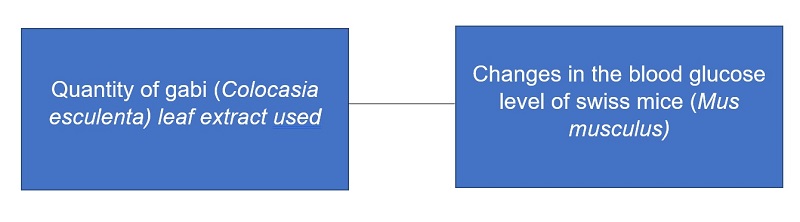
Figure 1 presents the Conceptual Framework of the study. The quantity of gabi leaf extract is the independent variable, while the Swiss mice’s blood glucose level is the study’s dependent variable. This study establishes a direct relationship between these variables through empirical evidence and statistical analysis .
Research Topic : Level of Effectiveness of Using Social Media in the Political Literacy of College Students
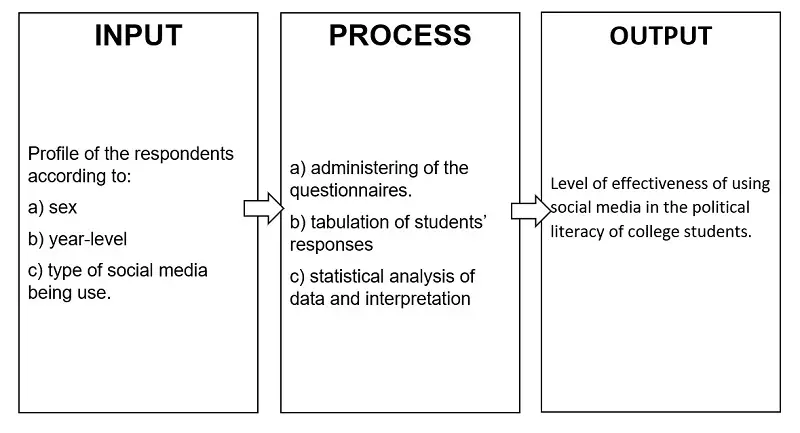
Figure 1 shows the Conceptual Framework of the study. The input is the profile of the college students according to sex, year level, and the social media platform being used. The research process includes administering the questionnaires, tabulating students’ responses, and statistical data analysis and interpretation. The output is the effectiveness of using social media in the political literacy of college students.
Research Topic: Factors Affecting the Satisfaction Level of Community Inhabitants
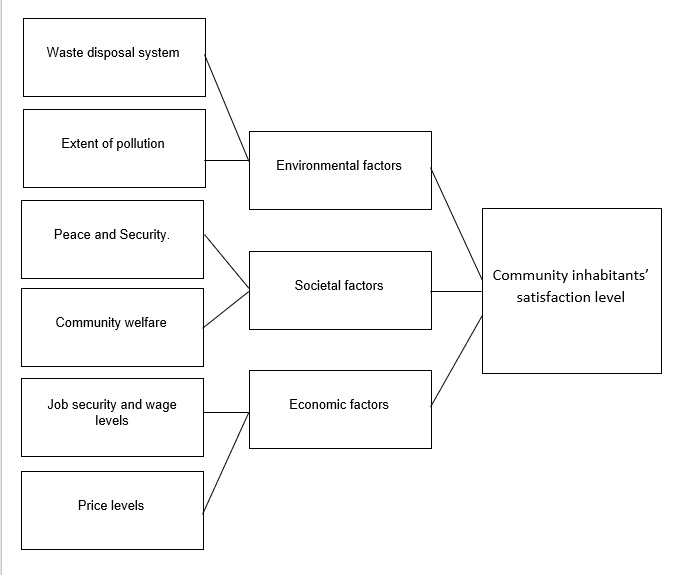
Figure 1 presents a visual illustration of the factors that affect the satisfaction level of community inhabitants. As presented, environmental, societal, and economic factors influence the satisfaction level of community inhabitants. Each factor has its indicators which are considered in this study.
Tips and Warnings
- Please keep it simple. Avoid using fancy illustrations or designs when creating your conceptual framework.
- Allot a lot of space for feedback. This is to show that your research variables or methodology might be revised based on the input from the research panel. Below is an example of a conceptual framework with a spot allotted for feedback.
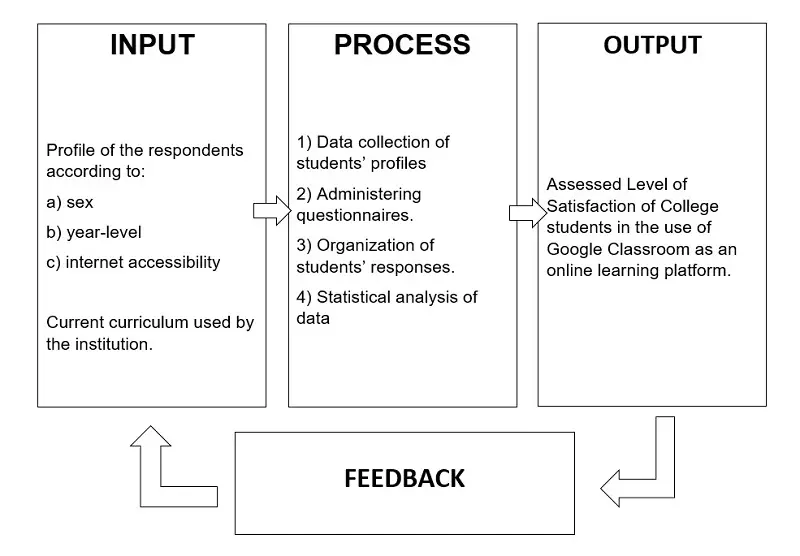
Frequently Asked Questions
1. how can i create a conceptual framework in microsoft word.
First, click the Insert tab and select Shapes . You’ll see a wide range of shapes to choose from. Usually, rectangles, circles, and arrows are the shapes used for the conceptual framework.
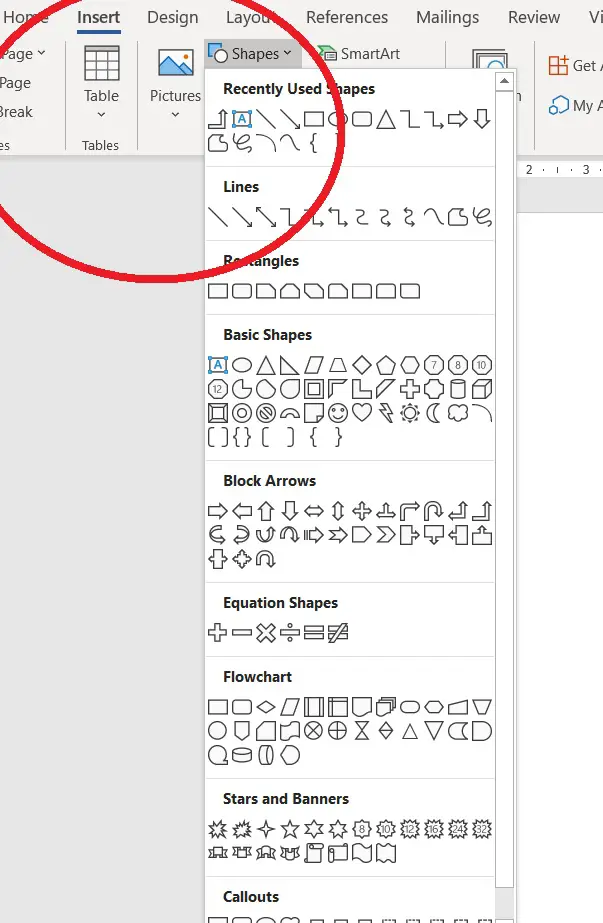
Next, draw your selected shape in the document.
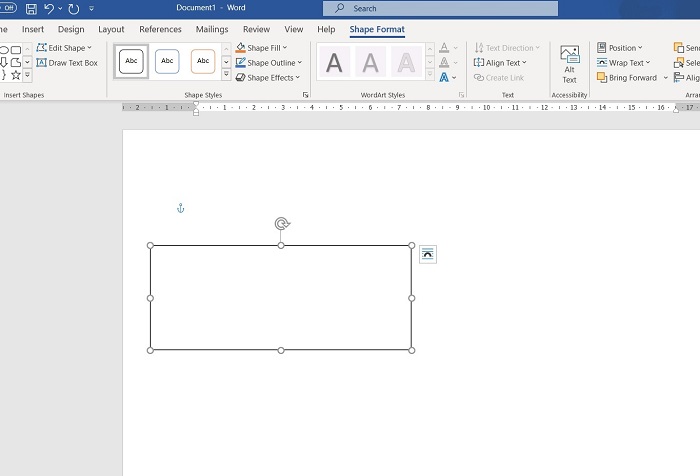
Insert the name of the variable inside the shape. You can do this by pointing your cursor to the shape, right-clicking your mouse, selecting Add Text , and typing in the text.
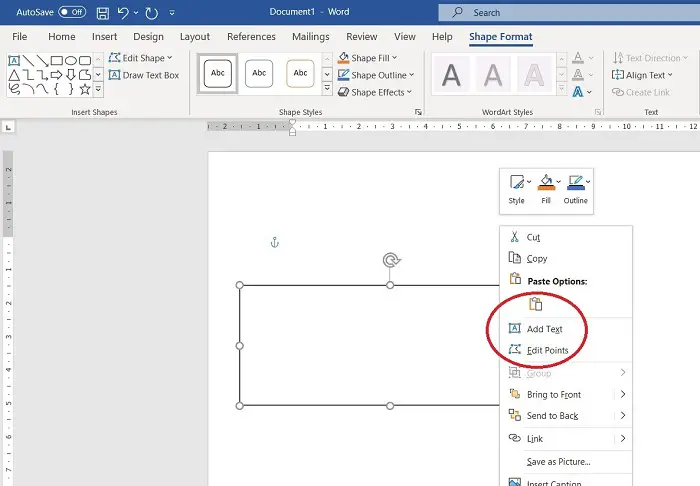
Repeat the same process for the remaining variables of your study. If you need arrows to connect the different variables, you can insert one by going to the Insert tab, then Shape, and finally, Lines or Block Arrows, depending on your preferred arrow style.
2. How to explain my conceptual framework in defense?
If you have used the Independent-Dependent Variable Model in creating your conceptual framework, start by telling your research’s variables. Afterward, explain the relationship between these variables. Example: “Using statistical/descriptive analysis of the data we have collected, we are going to show how the <state your independent variable> exhibits a significant relationship to <state your dependent variable>.”
On the other hand, if you have used an Input-Process-Output Model, start by explaining the inputs of your research. Then, tell them about your research process. You may refer to the Research Methodology in Chapter 3 to accurately present your research process. Lastly, explain what your research outcome is.
Meanwhile, if you have used a concept map, ensure you understand the idea behind the illustration. Discuss how the concepts are related and highlight the research outcome.
3. In what stage of research is the conceptual framework written?
The research study’s conceptual framework is in Chapter 2, following the Review of Related Literature.
4. What is the difference between a Conceptual Framework and Literature Review?
The Conceptual Framework is a summary of the concepts of your study where the relationship of the variables is presented. On the other hand, Literature Review is a collection of published studies and literature related to your study.
Suppose your research concerns the Hypoglycemic Ability of Gabi (Colocasia esculenta) Leaf Extract on Swiss Mice (Mus musculus). In your conceptual framework, you will create a visual diagram and a narrative explanation presenting the quantity of gabi leaf extract and the mice’s blood glucose level as your research variables. On the other hand, for the literature review, you may include this study and explain how this is related to your research topic.
5. When do I use a two-way arrow for my conceptual framework?
You will use a two-way arrow in your conceptual framework if the variables of your study are interdependent. If variable A affects variable B and variable B also affects variable A, you may use a two-way arrow to show that A and B affect each other.
Suppose your research concerns the Relationship Between Students’ Satisfaction Levels and Online Learning Platforms. Since students’ satisfaction level determines the online learning platform the school uses and vice versa, these variables have a direct relationship. Thus, you may use two-way arrows to indicate that the variables directly affect each other.
- Conceptual Framework – Meaning, Importance and How to Write it. (2020). Retrieved 27 April 2021, from https://afribary.com/knowledge/conceptual-framework/
- Correlation vs Causation. Retrieved 27 April 2021, from https://www.jmp.com/en_ph/statistics-knowledge-portal/what-is-correlation/correlation-vs-causation.html
- Swaen, B., & George, T. (2022, August 22). What is a conceptual framework? Tips & Examples. Retrieved December 5, 2022, from https://www.scribbr.com/methodology/conceptual-framework/
Written by Jewel Kyle Fabula
in Career and Education , Juander How
Last Updated May 6, 2023 10:37 AM
Jewel Kyle Fabula
Jewel Kyle Fabula is a Bachelor of Science in Economics student at the University of the Philippines Diliman. His passion for learning mathematics developed as he competed in some mathematics competitions during his Junior High School years. He loves cats, playing video games, and listening to music.
Browse all articles written by Jewel Kyle Fabula
Copyright Notice
All materials contained on this site are protected by the Republic of the Philippines copyright law and may not be reproduced, distributed, transmitted, displayed, published, or broadcast without the prior written permission of filipiknow.net or in the case of third party materials, the owner of that content. You may not alter or remove any trademark, copyright, or other notice from copies of the content. Be warned that we have already reported and helped terminate several websites and YouTube channels for blatantly stealing our content. If you wish to use filipiknow.net content for commercial purposes, such as for content syndication, etc., please contact us at legal(at)filipiknow(dot)net
- Skip to main content
- Skip to primary sidebar
IResearchNet
Input-Process-Output Model
Much of the work in organizations is accomplished through teams. It is therefore crucial to determine the factors that lead to effective as well as ineffective team processes and to better specify how, why, and when they contribute. Substantial research has been conducted on the variables that influence team effectiveness, yielding several models of team functioning. Although these models differ in a number of aspects, they share the commonality of being grounded in an input-process-output (IPO) framework. Inputs are the conditions that exist prior to group activity, whereas processes are the interactions among group members. Outputs are the results of group activity that are valued by the team or the organization.
The input-process-output model has historically been the dominant approach to understanding and explaining team performance and continues to exert a strong influence on group research today. The framework is based on classic systems theory, which states that the general structure of a system is as important in determining how effectively it will function as its individual components. Similarly, the IPO model has a causal structure, in that outputs are a function of various group processes, which are in turn influenced by numerous input variables. In its simplest form, the model is depicted as the following:
Input —> Process —> Output
Inputs reflect the resources that groups have at their disposal and are generally divided into three categories: individual-level factors, group-level factors, and environmental factors. Individual-level factors are what group members bring to the group, such as motivation, personality, abilities, experiences, and demographic attributes. Examples of group-level factors are work structure, team norms, and group size. Environmental factors capture the broader context in which groups operate, such as reward structure, stress level, task characteristics, and organizational culture.
Processes are the mediating mechanisms that convert inputs to outputs. A key aspect of the definition is that processes represent interactions that take place among team members. Many different taxonomies of teamwork behaviors have been proposed, but common examples include coordination, communication, conflict management, and motivation.
In comparison with inputs and outputs, group processes are often more difficult to measure, because a thorough understanding of what groups are doing and how they complete their work may require observing members while they actually perform a task. This may lead to a more accurate reflection of the true group processes, as opposed to relying on members to self-report their processes retrospectively. In addition, group processes evolve over time, which means that they cannot be adequately represented through a single observation. These difficult methodological issues have caused many studies to ignore processes and focus only on inputs and outputs. Empirical group research has therefore been criticized as treating processes as a “black box” (loosely specified and unmeasured), despite how prominently featured they are in the IPO model. Recently, however, a number of researchers have given renewed emphasis to the importance of capturing team member interactions, emphasizing the need to measure processes longitudinally and with more sophisticated measures.
Indicators of team effectiveness have generally been clustered into two general categories: group performance and member reactions. Group performance refers to the degree to which the group achieves the standard set by the users of its output. Examples include quality, quantity, timeliness, efficiency, and costs. In contrast, member reactions involve perceptions of satisfaction with group functioning, team viability, and personal development. For example, although the group may have been able to produce a high-quality product, mutual antagonism may be so high that members would prefer not to work with one another on future projects. In addition, some groups contribute to member well-being and growth, whereas others block individual development and hinder personal needs from being met.
Both categories of outcomes are clearly important, but performance outcomes are especially valued in the teams literature. This is because they can be measured more objectively (because they do not rely on team member self-reports) and make a strong case that inputs and processes affect the bottom line of group effectiveness.
Steiner’s Formula
Consistent with the IPO framework, Ivan Steiner derived the following formula to explain why teams starting off with a great deal of promise often end up being less than successful:
Actual productivity = potential productivity – process loss
Although potential productivity is the highest level of performance attainable, a group’s actual productivity often falls short of its potential because of the existence of process loss. Process loss refers to the suboptimal ways that groups operate, resulting in time and energy spent away from task performance. Examples of process losses include group conflict, communication breakdown, coordination difficulty, and social loafing (group members shirking responsibility and failing to exert adequate individual effort). Consistent with the assumptions of the IPO model, Steiner’s formula highlights the importance of group processes and reflects the notion that it is the processes and not the inputs (analogous to group potential) that create the group’s outputs. In other words, teams are a function of the interaction of team members and not simply the sum of individuals who perform tasks independently.
Limitations of the IPO Model
The major criticism that has been levied against the IPO model is the assumption that group functioning is static and follows a linear progression from inputs through outputs. To incorporate the reality of dynamic change, feedback loops were added to the original IPO model, emanating primarily from outputs and feeding back to inputs or processes. However, the single-cycle, linear IPO path has been emphasized in most of the empirical research. Nevertheless, in both theory and measurement, current team researchers are increasingly invoking the notion of cyclical causal feedback, as well as nonlinear or conditional relationships.
Although the IPO framework is the dominant way of thinking about group performance in the teams literature, relatively few empirical studies have been devoted to the validity of the model itself. In addition, research directly testing the input-process-output links has frequently been conducted in laboratory settings, an approach that restricts the number of relevant variables that would realistically occur in an organization. However, although the IPO model assumes that process fully mediates the association between inputs and outputs, some research has suggested that a purely mediated model may be too limited. Therefore, alternative models have suggested that inputs may directly affect both processes and outputs.
Without question, the IPO model reflects the dominant way of thinking about group performance in the groups literature. As such, it has played an important role in guiding research design and encouraging researchers to sample from the input, process, and output categories in variable selection. Recent research is increasingly moving beyond a strictly linear progression and incorporating the reality of dynamic change. In addition, alternatives to the traditional IPO model have been suggested in which processes are not purely mediated.
References:
- Hackman, J. R. (1987). The design of work teams. In J. Lorsch (Ed.), Handbook of organizational behavior (pp. 315-342). New York: Prentice Hall.
- Ilgen, D. R., Hollenbeck, J. R., Johnson, M., & Jundt, D. (2005). Teams in organizations: From input-process-output models to IMOI models. Annual Review of Psychology, 56, 517-543.
- Steiner, I. D. (1972). Group process and productivity. New York: Academic Press.
- Group Dynamics
- Industrial-Organizational Psychology
Learn how to use the input-process-output (IPO) model

You might have read that the input-process-output (IPO) model can be helpful in business and other organizations, but you’re not sure precisely what it means and how to apply it. That’s understandable — the phrase might sound like a complex computer system or an intimidating theory.
The reality is that the IPO model is very easy to understand. While it has roots in computer programming, it appears in many different industries and settings because it’s an effective way to plan, analyze, and improve how work gets done.
After reading this post, you’ll understand what the input-process-output model is all about and be ready to apply it to your business processes. You’ll learn:
- What the input-process-output (IPO) model is
How to use the IPO model
Benefits of the ipo model, examples of input-process-output in different industries, best practices when thinking about ipo, what is input-process-output (ipo).
Input-process-output (IPO) — also called an IPO model or IPO diagram — is a visual tool used to describe a workflow, the flow of information, or activities within a system. An IPO diagram helps you identify all the factors that influence a process and all the process’s outcomes, and it gives you a structured approach to analyzing and improving the system.
The IPO diagram consists of three columns listing inputs on the left, describing the process in the middle, and then tracking the outputs on the right. By diagramming a process in this way, almost any business — including computer science, systems analysis, and business analysis — can better identify the cause of a problem and improve a system’s performance.

As part of Six Sigma methodology , the IPO model fits within a more complex technique called DMAIC — which stands for Define, Measure, Analyze, Improve, and Control. The input-process-output model is an important part of the Define stage of DMAIC because it helps clarify and define a project’s goals, scope, and boundaries. This clarity helps to establish a solid foundation for the subsequent stages.
IPO assumes that if we control causal factors, we can also control their effects. Drawing the workflow using an IPO diagram can help visualize any system where it’s difficult to see how all the pieces connect. Although it’s primarily descriptive, teams can use IPO for analytical, quality control, or planning purposes. It’s useful in production, manufacturing, teamwork, and many other areas.
Creating your own initial IPO model is relatively easy. The IPO diagram gives you a simple framework for listing the inputs and outputs of any system. The details will depend on your industry and the specific process you want to explore.
Getting the details right — and knowing the difference between inputs, process steps, and outputs — can require careful thinking. Let’s examine each category:
- Input can include data, information, or resources that enter the system.
- Process can include activities, transformations, or operations performed on the inputs.
- Output can include results, products, or outcomes produced by the processes.
In manufacturing, a general, high-level IPO diagram might look something like this:

Although this diagram establishes a cause-and-effect pattern from left to right, you don’t necessarily need to start with inputs. If you’re planning a new process, try starting with the desired output and working back. The output should be thorough, specific, and measurable. Output is usually focused on four categories of standards that organizations are trying to achieve — quality, cost, time, and safety.
One drawback of the IPO method is it’s hard to account for random variables. To identify all possible inputs, consider people, environmental factors, methods, measurements, materials, and machines. As you define the process, break it down into clearly defined steps. Once the model is complete, you can analyze the process to determine relationships and identify areas for improvement.
There are many benefits to using the IPO model, such as:

- It’s easy to use . You don’t need to know how to use software or have specific expertise and training. Anyone can create an IPO diagram and spend time thinking about factors in a process.

- It’s versatile . It’s used in different industries and can be applied to almost any business process. You don’t have to follow a set of prescribed rules. You can apply this framework creatively, depending on your goals.

- It helps define key process input variables. Use the model to gain insights into how different factors contribute to the overall output and identify how to improve your input.

- It helps streamline operations . Identify bottlenecks, redundancies, or inefficiencies in operations. This understanding allows teams to reconfigure processes, allocate resources effectively, and streamline operations for better performance and productivity.

- It helps with problem-solving. Businesses can analyze the IPO diagram to identify potential causes and effects when an issue or challenge arises. They can pinpoint areas where disruptions may occur, allowing them to troubleshoot and rectify the problem more effectively.

- It can enable training and documentation. The model provides a visual representation of a business process, making it easier to convey information and train new employees. IPO can be a reference tool for documenting and preserving knowledge about processes within an organization.

- It helps clarify process steps and why. It can help eliminate what does not produce a good outcome.

- It’s a good communication tool. The model allows teams or stakeholders to visualize and discuss the flow of information within a system. It facilitates effective communication and collaboration by providing a common understanding of how inputs become outputs. This shared understanding enables teams to work together more efficiently and make informed decisions.

- It’s a good introduction to more complicated Six Sigma process mapping. The SIPOC (Supplier, Input, Process, Output, Customer) diagram adds the supplier to the start of the process chain and the customer to the end of it. This method can extend the IPO diagram beyond internal processes to map out a larger chain of cause and effect. IPO is also a helpful concept to master before exploring value stream mapping .
The input-process-output model emerged in the twentieth century as a fundamental model for describing complex computer systems. However, IPO quickly found applications outside of computer programming as a practical methodology in general systems theory and design. Many businesses found that by diagramming processes, they could improve quality and efficiency. IPO diagrams are now used in various industries. Some of the most common are:
- Manufacturing. The IPO model can show the most important factors influencing production outcomes. Companies can then identify bottlenecks, optimize resource allocation, improve quality, and enhance efficiency.
- Commerce. In online order processing, the input happens when a customer places an online order. The process happens when the order is received, the cost is calculated, and payment is processed. The output includes an order confirmation, an inventory check, shipping, and a confirmation email.
- Software development. A software development process could include gathering requirements, designing, coding, testing, and deployment. In software, it’s a good idea to start with output, break down the input variables, and determine the process of code needed to generate a fully functioning application as the output.
You might also encounter the use of IPO diagrams in the social sciences, food and hospitality, economics, finance planning, and other areas. The principles of the model are foundational enough that they can be applied almost anywhere.
While practices differ depending on the industry, if you are looking to improve the performance of a team or system, there are a few basic principles to follow when applying this model.
To avoid common pitfalls, plan ahead and check that your diagram is:

- Achievable. Define an attainable scope for your processes and make sure your processes are within that scope.
- Comprehensive. Consider all the possible outputs before capturing or changing your inputs. Likewise, check that you have anticipated all possible inputs, both those you add intentionally and others that might be harder to control.
- Inclusive. Make sure to involve the whole team to avoid bias on what should go into the inputs and outputs.
Get started with the input-process-output model
The IPO model is a simple but effective way to think carefully about cause and effect in small and large systems. Whether you want to improve teamwork, discover the cause of a problem, increase revenue, or design a more efficient system, this framework can help you achieve your business goals.
When you’re ready to get started, decide what business process you want to analyze and sketch it out with paper and pen using this method. Then explore Adobe Workfront , which can help you design a workflow using the input-process-output model and identify all the factors in your work processes that lead to success.
Adobe Workfront
Workfront is enterprise work management software that connects work to strategy and drives better collaboration to deliver measurable business outcomes. It integrates people, data, processes, and technology across an organization so you can manage the entire lifecycle of projects from start to finish. By optimizing and centralizing digital projects, cross-functional teams can connect, collaborate, and execute from anywhere to help them do their best work.
Take a product tour or watch the overview video to learn more.
https://business.adobe.com/blog/basics/dpu-dpmo-ppm-rty
https://business.adobe.com/blog/basics/what-is-workflow-automation
https://business.adobe.com/blog/basics/workplace-collaboration-tips

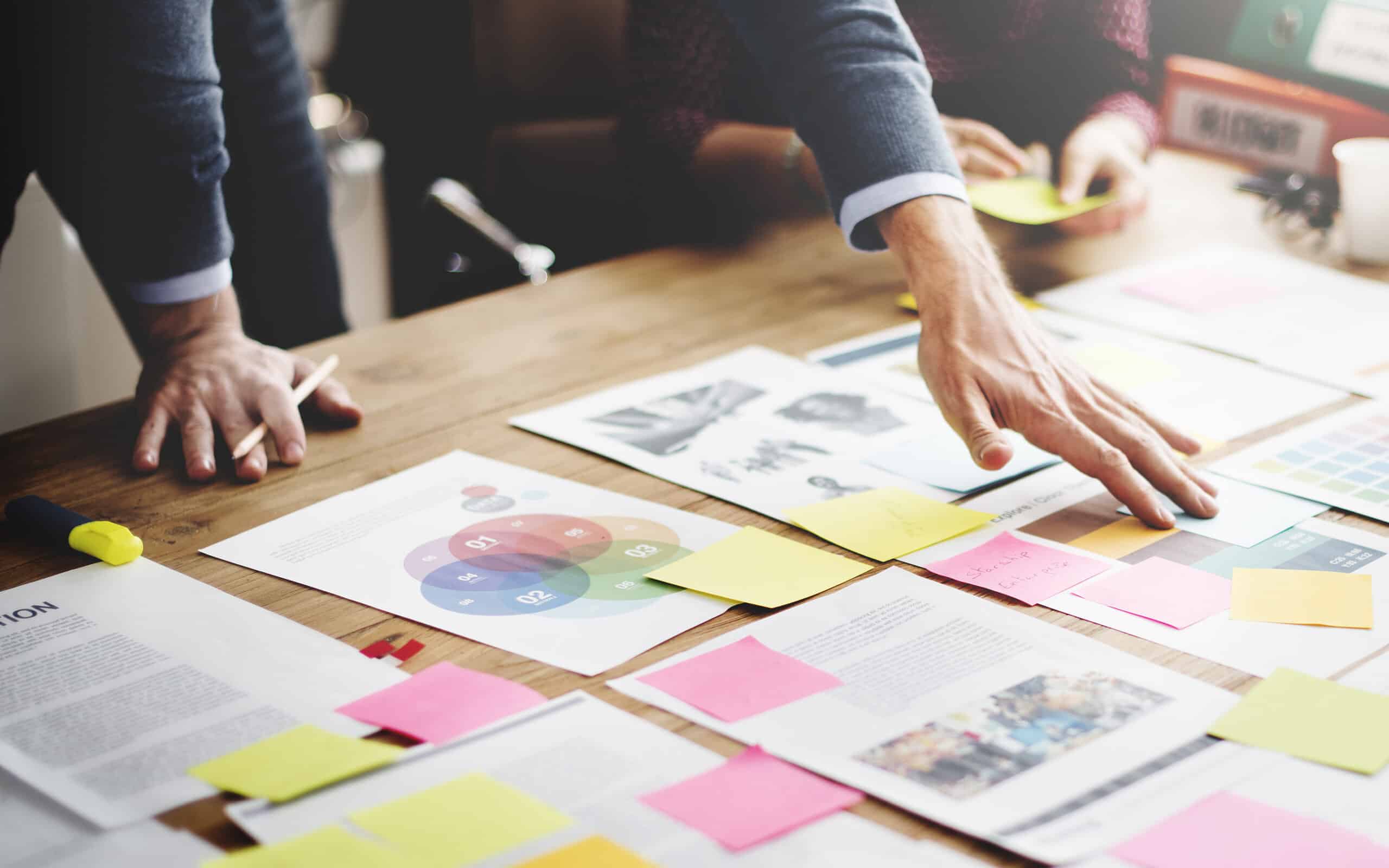
A Comprehensive Guide to Input-Process-Output Models
Updated: January 31, 2024 by Ken Feldman
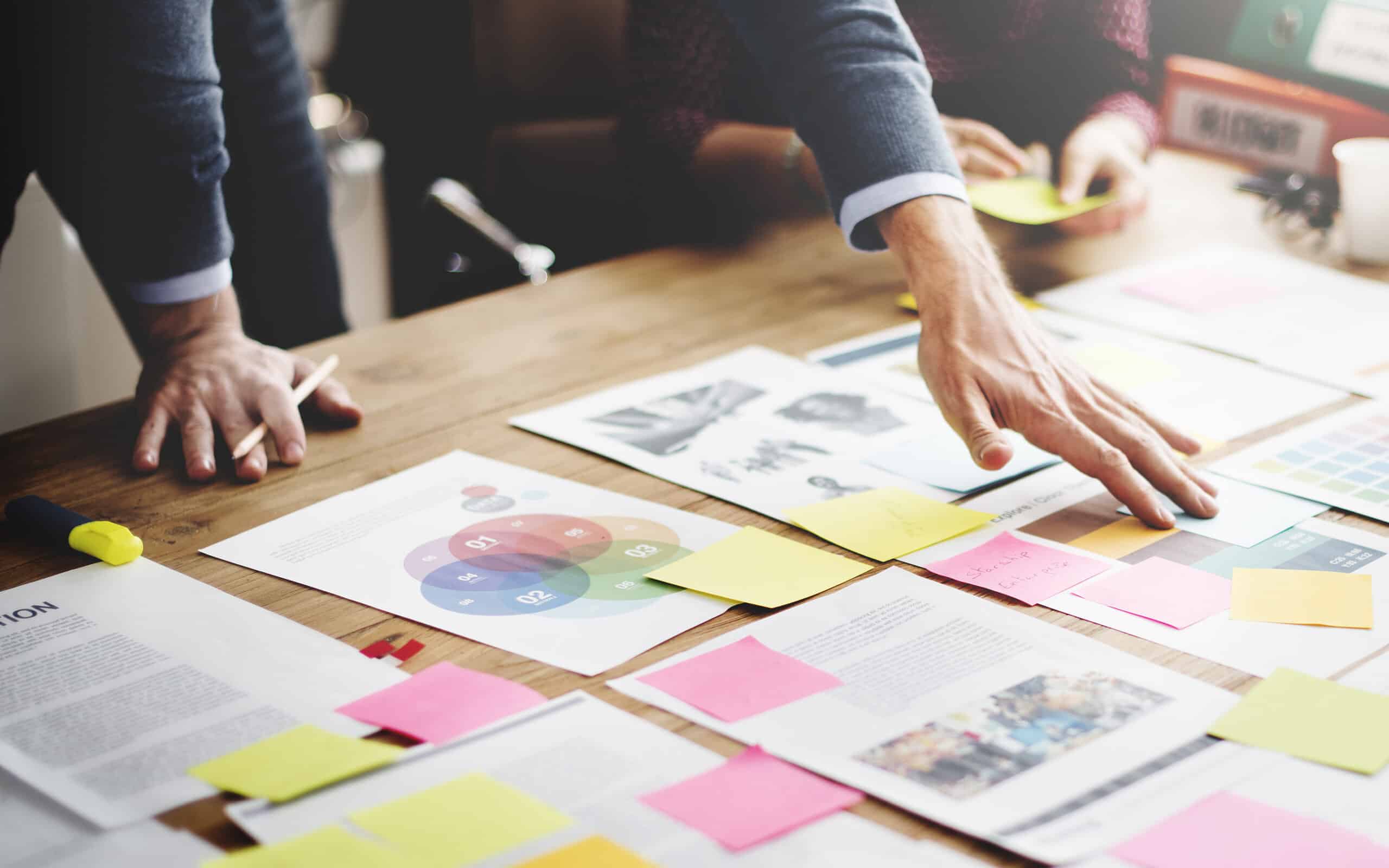
Are you looking for a business improvement tool that is intuitive, simple to use, and visual in nature? Do you want to explore your internal business process and make sure you understand all of the inputs, outputs, and potential error states?
If you are answering yes to these questions, then using input-process-output could be the perfect methodology for you. Let’s find out more.
Overview: What is input-process-output (I-P-O)?
Input-process-output (I-P-O) is a structured methodology for capturing and visualizing all of the inputs, outputs, and process steps that are required to transform inputs into outputs. It is often referred to, interchangeably, as an I-P-O model or an I-P-O diagram, both of which make reference to the intended visual nature of the method.
A simple example is shown below from research in healthcare.
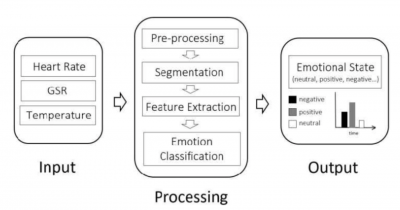
https://www.researchgate.net/figure/The-Input-Process-Output-diagram-of-the-proposed-system_fig2_323935725
As the methodology is incredibly versatile, it is used across many industries and sectors with (inevitably) some modifications and adaptations. These can include, for example, the addition of feedback loops from output to input, in doing so creating models analogous to closed-loop control theory.
Typically, we would use I-P-O in the “define” stage of a Six Sigma DMAIC project and follow a specific method for generating the model. The steps are:
- Decide upon the process steps that will be in scope of the I-P-O model. Try to ensure the the scope is manageable with, ideally, less than 10 process steps defined.
- List all of the possible outputs, including potential error states.
- List all of the inputs to your process steps, using clear descriptive language.
- Create a visual I-P-O model.
- Check that the inputs are transformed to the outputs via the process steps as shown in the model.
Often, it can be helpful to have the team that’s generating the I-P-O model complete a Gemba walk. Visiting the actual place of work and viewing the process in action can tease out some of the less obvious inputs and outputs and contributes to continuous improvement of the existing process steps.
2 benefits and 1 drawback of I-P-O
Used correctly, the I-P-O model offers a simple, practical, and efficient way to analyse and document a transformation process. Let’s explore some benefits and drawbacks of I-P-O.
1. It’s visual and easy to explain
It’s often said that the best business improvement tools are simple to use, intuitive, and visual, and I-P-O ticks all three of these boxes. A sheet of paper, marker pen, and an enthusiastic team willing to contribute will get you a long way. It’s also versatile, suitable for use with the executive management group as well as the wider business improvement team.
2. It’s easy to execute
There is a clear and simple methodology to generate I-P-O models, and this helps you recognise and document all of the possible inputs, outputs, and error states. As it’s visual, it’s easy to update and change as the team explores many potential inputs and outputs.
3. It’s internally focused without regard for external customers or suppliers
Developing I-P-O models is usually all about internal business processes, and we often hear this called micro-process-mapping. This typically means we do not consider our external suppliers and customers in the analysis. However, don’t worry, we have complimentary models such as SIPOC and COPIS that help us make sense of the bigger (macro) picture.
Why is I-P-O important to understand?
For such a relatively simple mapping tool, it provides a really powerful insight into our internal business processes. Let’s dig a little deeper.
It helps with defining your key process input variables
Once we’ve documented and visualised our inputs and outputs, we can turn our attention to determining and controlling which inputs provide a significant impact on the output variation — these are known as our key process input variables .
It’s aligned with Six Sigma and Lean principles
In a classic Six Sigma and Lean project approach, we strive to reduce process variation and remove defects and waste. With I-P-O, we identify inputs, outputs, and error states from our processes so we can begin to explore and understand the Y(output) = f ((X) input) equation.
It’s the perfect springboard to create full process maps
Once we have created I-P-O models, we have the perfect starting place for generating complete process maps . This could be moving on to value stream mapping , spaghetti maps, or one of many other types of process maps that are available.
An industry example of I-P-O
A government agency with multiple departments was embarking upon a business transformation project to improve customer service times and efficiency. As part of the transformation project, a Six Sigma Black Belt who was assigned to the activity was requested to explore and document existing processes and prepare the teams for process improvement.
The Black Belt chose to create I-P-O models due to the ease of use and versatility of the approach. Each of the business departments designated a team to work on the I-P-O models and, alongside the Black Belt, defined the process scope, ensuring this was of manageable size.
With the teams in place and scope defined the process outputs were brainstormed and captured visually using whiteboards. The corresponding inputs were added, and the I-P-O models checked for completeness.
Generating the I-P-O models highlighted a number of potential output error states that were subsequently investigated as part of the business transformation project and contributed to improved customer service times. As the models were captured visually on whiteboards, they were easily updated during the project and used to inform staff of their contribution towards continuous improvement.
3 best practices when thinking about I-P-O
Like many process-driven mapping activities, there are some key things for us to consider when creating I-P-O models. Let’s look at three of these.
1. Remember: It’s a team sport; don’t go it alone
Even relatively simple processes have multiple inputs and outputs. Often we find that different team members have detailed knowledge of specific process inputs and outputs, and we should make good use of this collective knowledge.
2. Make sure the scope is achievable
Don’t be overly ambitious with the scope and try to include too many process steps for your I-P-O model. If you find yourself listing 10 or more process steps, it’s probably time to stop and re-evaluate.
3. Consider all of the inputs and outputs
Be diligent, get all the team involved, and make sure there is no bias — we don’t want to just list the things we think should be inputs and outputs in an ideal world. In addition, we should consider and document all of the possible output error states.
Frequently Asked Questions (FAQ) about I-P-O
Is i-p-o related to sipoc .
It can be a logical next step to create a SIPOC model from an I-P-O model. With SIPOC, we consider both suppliers (S) and customers (C) in the analysis, the so-called wider or bigger picture. With I-P-O, we concentrate more on the internal business process.
Where do I start with an I-P-O model?
Start by defining the processes that are in scope, making sure the scope is manageable. Then consider and document all of the possible outputs from the process steps before moving on to capture the inputs.
Do I need a software program to generate I-P-O models?
Definitely not. You can start with paper, pen, and a pack of sticky notes. However, there are a number of free templates available for download that can help you and your team as you start to populate the I-P-O model.
A final thought on I-P-O
Ease of use and versatility are just two of the major plus points of developing I-P-O models for your internal business processes. Add in their highly visual nature, and this means you can easily engage your team on a journey to continuous improvement.
About the Author
Ken Feldman

Data Science Journal
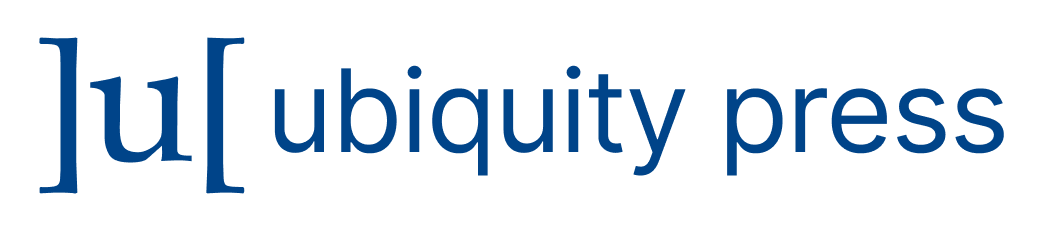
- Download PDF (English) XML (English)
- Alt. Display
Research Papers
Kadistudio: fair modelling of scientific research processes.
- Philipp Zschumme
- Matthieu Laqua
- Nico Brandt
- Ephraim Schoof
- Patrick Altschuh
- Michael Selzer
FAIR handling of scientific data plays a significant role in current efforts towards a more sustainable research culture and serves as a prerequisite for the fourth scientific paradigm, that is, data-driven research. To enforce the FAIR principles by ensuring the reproducibility of scientific data and tracking their provenance comprehensibly, the FAIR modelling of research processes in form of automatable workflows is necessary. By providing reusable procedures containing expert knowledge, such workflows contribute decisively to the quality and the acceleration of scientific research. In this work, the requirements for a system to be capable of modelling FAIR workflows are defined and a generic concept for modelling research processes as workflows is developed. For this, research processes are iteratively divided into impartible subprocesses at different detail levels using the input-process-output model. The concrete software implementation of the identified, universally applicable concept is finally presented in form of the workflow editor KadiStudio of the Karlsruhe Data Infrastructure for Materials Science (Kadi4Mat).
- FAIR principles
- research data management
- electronic lab notebook
- inputprocess-output model
1 Introduction
Through technological advances in instrumentation and computational performance, the amount of data produced in engineering sciences, and especially materials science, has increased significantly over the past decades. This development paves the way for a new scientific paradigm, commonly known as data science ( Hey et al. 2009 ), that focuses on the systematic analysis of data to generate new knowledge or insight. It allows to accelerate the innovation of new materials and can thus be seen as a driving force for future developments. Prerequisite for this paradigm is the availability, completeness, and reproducibility of the research data to be examined.
Establishing the paradigm thus requires an extensive data sharing concept that enables structured storage and management of research data according to the FAIR – Findable, Accessible, Interoperable, and Reusable – principles ( Draxl & Scheffler 2020 ; Wilkinson et al. 2016 ). A sophisticated infrastructure in form of a repository in which data can be recorded and administered as well as analysed, transformed, and visualised is therefore beneficial. Moreover, a system capable of modelling scientific processes and data flows as automatable and configurable workflows is necessary. It not only ensures the datas’ reproducibility and tracks their provenance comprehensibly but also allows to generate new knowledge and insight by processing the stored data. In this way, workflows contribute decisively to the quality assurance and acceleration of scientific research. As for scientific data, workflows need to be formulated in a FAIR manner in order to be accessible and usable for a broad scientific audience as well as for data science approaches. Implementing a system capable of FAIR modelling of research processes as such workflows requires two contradictory conditions to be met. Firstly, as scientific research exhibits heterogeneous tools and procedures, the proposed workflow system must be kept generic and easily extensible. Secondly, it must be simple and intuitive in use to minimise the effort required to formulate workflows and thus increase acceptance among researchers ( Pizzi et al. 2016 ).
Infrastructures which integrate the creation, exchange and execution of workflows are, to date, already realised in various implementations, such as Jupyter Notebooks ( Kluyver et al. 2016 ), Galaxy ( Afgan et al. 2018 ), Fireworks ( Jain et al. 2015 ) and AiiDA ( Pizzi et al. 2016 ). The aforementioned infrastructures as well as all other implementations known to us, however, do not satisfy the named conditions of simple usability, generic extensibility and FAIR process modelling. Jupyter Notebooks for example, enables the modelling of scientific processes, but its focus lies on computer-aided scientists. Hence, programming experience is required on part of the user to formulate workflows, which in our experience, is a hindrance for many scientists. Galaxy, on the other hand, allows researchers without programming knowledge to formulate workflows. However, these are limited to the field of life sciences and thus do not represent a generic solution for FAIR process modelling. Fireworks is also limited to a specific domain that is simulations and the management of computer resources. A generic, domain-independent use that also includes manual work steps is therefore not possible. AiiDA presents a generic solution for formulating workflows in form of scripts. Nevertheless, as the focus is on computational science, it is not possible to implement manual steps into workflows, thus excluding scientists working in analogue from the target group. Additionally, programming experience is again required. Consequently, to our knowledge, there is no system that offers a generic, domain independent approach to formulate workflows in a FAIR manner, which targets not only computer-based working scientists with an affinity for programming but also analogue working researchers with little programming expertise. In this paper we therefore introduce a possible solution for FAIR modelling of scientific processes that takes these requirements into account and present its concrete implementation in form of the workflow editor KadiStudio. For this, a concept is first developed that allows to abstract scientific processes according to a uniform schema that serves as the basis for FAIR modelling. Subsequently the technical implementation of the openly accessible software KadiStudio ( Kadi4Mat Team & Contributors 2022e ) (available under https://doi.org/10.5281/zenodo.6810891 ) is presented in detail, with reference to this concept.
The development of a generic system for modelling research processes requires the identification of a common structure that can be imposed on any process. For this purpose, we iteratively disassemble scientific processes into atomistic descriptions at different levels of detail. The term atomistic description here refers to the subdivision of a process into impartible parts. This description allows to identify generic elements within processes that can be reused in other use-cases. Basis for the disassembly is the Input Process Output (IPO) model presented in Figure 1 , which is known from systems analysis ( Goel 2010 ; Zelle 2004 ).
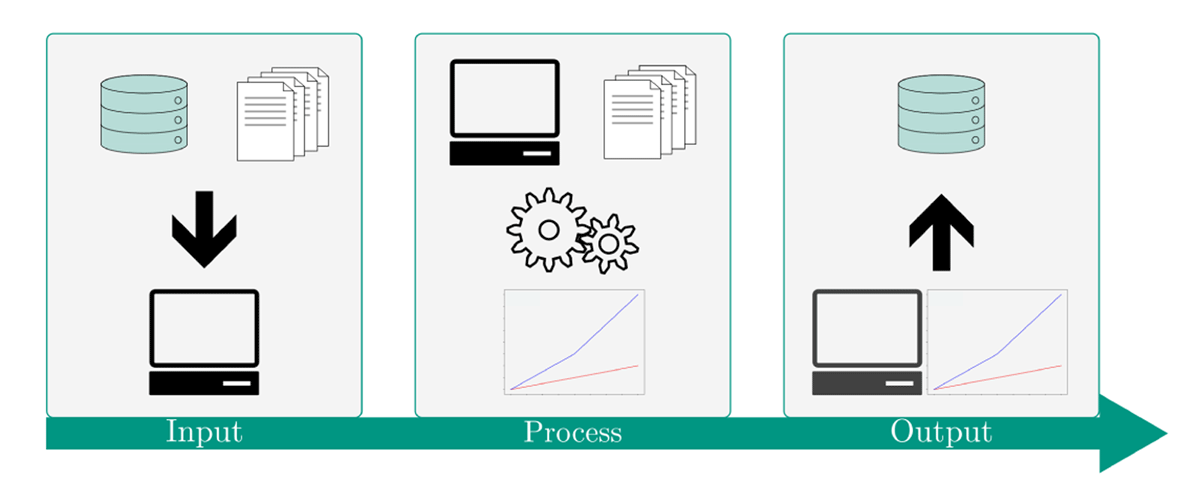
Schematic description of the IPO concept. Through defined inputs a process is parameterised and subsequently executed. The generated results are available via defined outputs.
This model describes processes as the combination of input, process and output. Accordingly, a process commences with the collection and preparation of the data to be investigated through specified inputs. The subsequent processing of the collected data is then performed according to a defined process. The results obtained in this process are finally available for further use via defined outputs.
The most extensive atomistic process description is at project level and includes the complete process as shown in Figure 2 . This is to be understood in the sense that a research project can only be described by the entire process. Describing the experimental investigation of a sample, for instance, requires the entire experiment to be modelled; no further subdivision is possible.
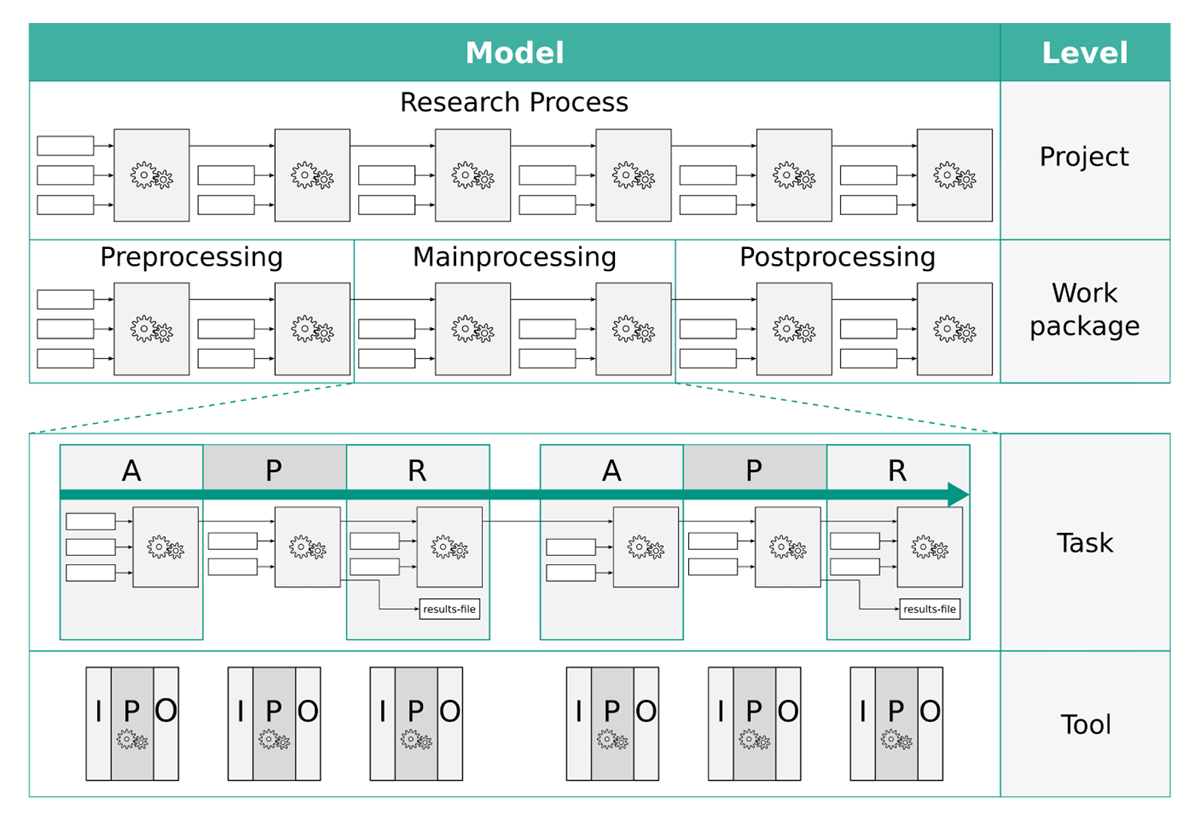
Abstraction of a research process at different levels of detail. Each grey box with gears models a work step while the white boxes represent their parameterisation. APR refers to the description of tasks as data acquisition, data processing, and data routing. Iteratively structuring a research process according to the IPO model ultimately defines it via multiple generic tools.
To describe the process at the less complex work package level, the IPO model is imposed onto it. This model divides the research process into three sequential work packages that correspond to the different elements of the IPO-model. These packages are pre-processing, main-processing, and finally post-processing. Applied to the aforementioned experimental investigation of a sample, the pre-processing corresponds to the preparation of the experiment, the main-processing to the actual experiment and the post-processing to the final analysis of the obtained data or the interpretation of the results. Each of these work packages can consist of an arbitrary number of work steps. The pre-processing of an experiment, for instance, could include the grinding and polishing of a material sample as well as the calibration of the microscope. Abstracting the process into the work packages pre-, main- and post-processing already enables the identification of elements that might be reusable in other use-cases. The pre-processing of the experiment in form of the microscope calibration and the sample preparation can for example be reused for similar investigations. This level of detail however is not sufficient to be used as a basis for a generic workflow modelling system. Instead, a more in-depth description of the individual work packages is required. For this purpose, the IPO model is applied to each coherent task within the identified work packages. A coherent task refers to the logically separable work steps within a work package. The pre-processing of the aforementioned experimental investigation of a sample could for instance contain the tasks sample grinding and microscope calibration . Structuring these tasks according to the IPO model results in a process description at task level, which we refer to as APR structure, that is schematically illustrated in Figure 2 . It describes the data flow within and between the individual steps of a task through data A cquisition, data P rocessing and data R outing. In this abstraction, the data acquisition contains all work steps that collect and prepare the necessary data for the corresponding task and then forwards them to data processing. Within data processing, these inputs are processed to generate new data. The data thus obtained in form of results or intermediate results are finally forwarded in the data routing. The routing of results can be realised to any desired destination such as a file or the data acquisition of a subsequent task. The work packages Pre-, Main- and Post-processing can consist of any number of such APR processes as indicated in Figure 2 , and can hence be understood as the concatenation of these elements.
Accordingly, the APR model mainly serves to describe the data flow within each task. This allows for the comprehensible traceability of the data flow within them, promoting a better understanding of the process. Moreover, the identified APR structures can be reused and applied to different use-cases. However, as the APR processes consist of a defined combination of multiple individual work steps, they are too use case specific to be used as a generic workflow modelling structure. Consequently, the IPO model is again applied to each work step within the APR processes. This results in an atomistic description of the research process at tool level. The individual work steps of each APR processes are now defined as generic and reusable tools with specific inputs and outputs as well as a process. In the considered sample preparation example, these could be the work steps grinding, polishing, and etching. Since this structuring corresponds to the original definition of the IPO model, it is here also referred to as IPO. The actual data generation of the research project takes place within this abstraction step. The data is generated in the process step and then forwarded through the output. This allows the origin of the generated data to be precisely determined and to be provided with the corresponding metadata and dependencies, thus enabling its FAIR storage. The generic descriptions of the work steps can now be used and rearranged to form any desired workflow making a further abstraction of the process unreasonable at this point.
Summarising, the applied method illustrated schematically in Figure 3 can be applied to reduce the complexity of arbitrary research processes up to the atomistic descriptions of the individual work steps, that is tools. Consequently, the inverse use of this concept enables the implementation of a generic system for modelling research processes. This modelling is thus based on the general description of the used tools according to the IPO model. Subsequently adding information through connections describing the data flow and the parameterisation of the used tools as described by the APR model, enables the further specification of the process. The concatenation of multiple APR processes finally allows to model the pre-, main- and post-processing, which in sum ultimately represent the complete research process. Similar concepts that use atomistic tool descriptions according to the IPO model which are linked together to form a workflow are used in many well-known workflow management systems. CWL ( Crusoe et al. 2022 ), snakemake ( Mölder et al. 2021 ) and nextflow ( Di Tommaso et al. 2017 ), for example, implement it in a script-based system, while programmes such as KNIME ( Berthold et al. 2009 ) or Orange ( Demšar et al. 2013 ) realise it within in a graphical interface. This widespread use of similar concepts in established systems illustrates the suitability of the found concept for the formulation of workflows.
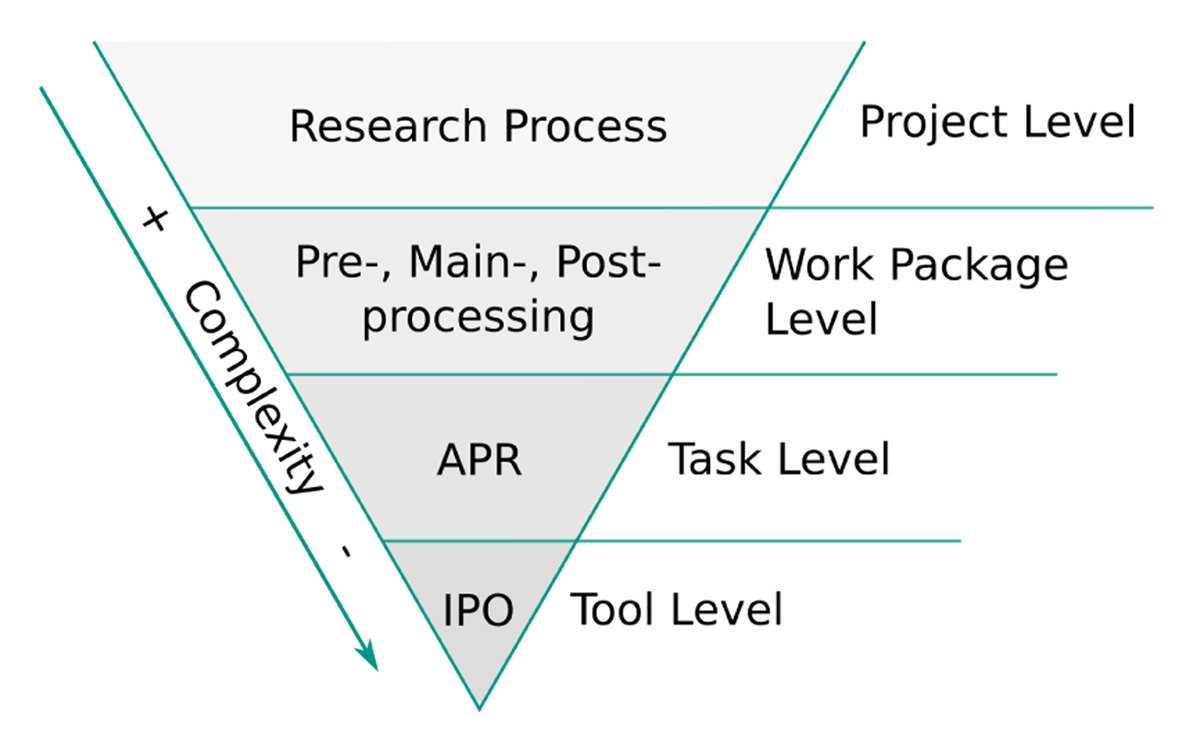
Reducing the process complexity by iteratively applying the IPO model to the identified processes. On each abstraction level, the process can be atomistically described. The tool level description presents a generically usable approach for modelling arbitrary research processes.
The concrete implementation of the described concept into a generic workflow system for the FAIR formulation of research processes, incorporated into the research data infrastructure Kadi4Mat, will be presented in the following.
3 Implementation
The Karlsruhe Data Infrastructure for Materials Science – Kadi4Mat – ( Kadi4Mat Team & Contributors 2022b ) offers its users multiple functionalities, illustrated in Figure 4 . They can be summarised as a Community Repository and as an Electronic Lab Notebook ( ELN ). While the community repository provides an extensive data sharing and managing infrastructure, the ELN allows for the logging of conducted research, the visualisation, transformation and analysis of stored data, and the generation of reproducible workflows. These workflows serve to model recurring processes in the work of researchers in form of digital twins that allow to process data stored within the repository and to guarantee the reproducibility of said data. The digital twins thus not only facilitate the day to day work of researchers by automation, but also allow to make process knowledge accessible and repurposable for a wider scientific community indicating the importance of their FAIR formulation.
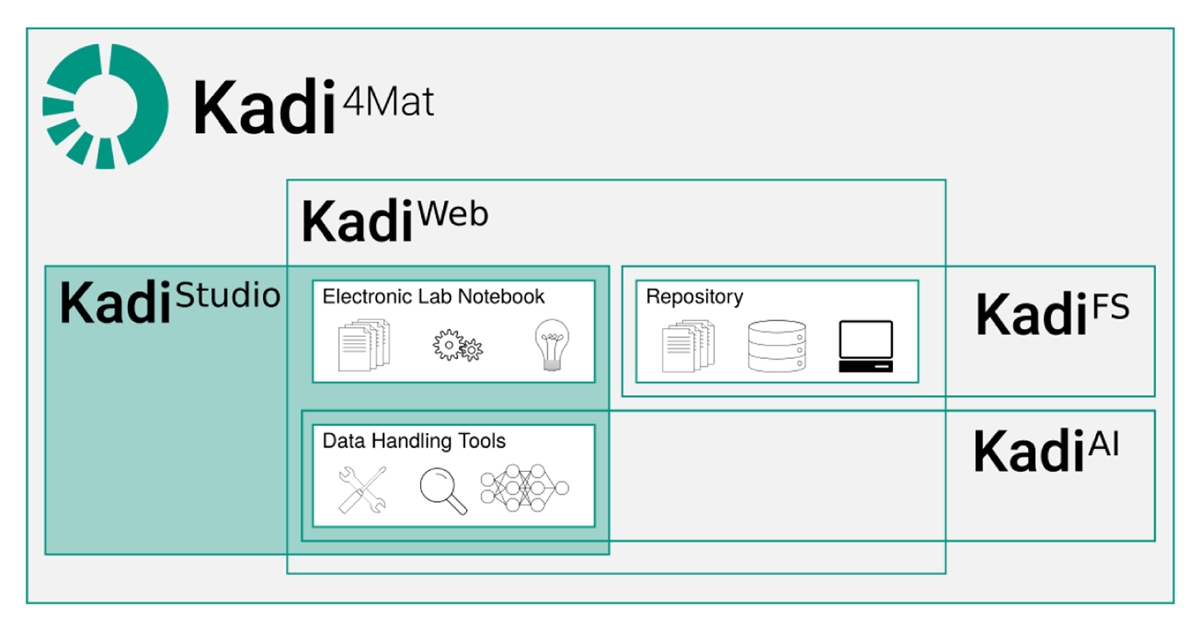
Conceptual overview of Kadi4Mat. Currently two software modules are available: (1) KadiWeb, a web-based virtual research environment incorporating ELN functionalities and repositories and (2) KadiStudio, a desktop-based software version which allows for the formulation and execution of workflows. Further modules such as a machine learning implementation referred to as KadiAI and a desktop-based repository called KadiFS are subject of current developments.
Aiming to provide a user friendly software solution that incorporates the FAIR modelling of research processes, a workflow editor, which is based on an open source node editor library for the Qt GUI framework ( Dmitry 2017 ), has been created and integrated within the ELN functionality of Kadi4Mat. Basis for this workflow system was the process structuring concept presented in the previous section. Within the framework of Kadi4Mat two versions of this editor exist, which both use the same JSON-based data format to describe workflows, ensuring their interoperability. On the one hand a desktop-based, standalone software version called KadiStudio exists, which can be used without an internet connection or running web server, and on the other hand, a web-based version that is integrated into KadiWeb . KadiWeb (available under https://kadi.iam-cms.kit.edu/ ) refers to the generally accessible web version of Kadi4Mat, which incorporates both the community repository and the ELN functionalities including its built-in data handling tools. In Figure 4 the structure of Kadi4Mat is illustrated schematically. Apart from the described components – KadiWeb and KadiStudio – additional modules are currently being developed, including a machine learning tool set called KadiAI and a filesystem integration for the repository referred to as KadiFS . Generally, Kadi4Mat can be understood as an overarching concept, that encompasses various modules, which in sum, create a generic research data infrastructure, extensible to all kinds of research disciplines in the future.
To illustrate how the workflow system implemented into the infrastructure of Kadi4Mat enables FAIR modelling of research processes, the term FAIR will first be defined in more detail. FAIR was introduced by Wilkinson et al. ( 2016 ) and is the acronym for Findable, Accessible, Interoperable, and Reusable. These principles impose the following requirements on the storage of scientific data:
- F indable: Data must be provided with descriptive metadata that can be searched specifically by humans and machines alike.
- A ccessible: Stored data must be accessible, possibly with appropriate authentication or authorisation.
- I nteroperable: Data must be formulated in a broadly applicable language and thus be interoperable with applications and workflows.
- R eusable: Data should be reusable. For this, metadata and data need to be rich in information and associated with a detailed provenance.
When applying these principles to scientific processes, however, their definitions have to be partially adjusted. While the wording of Findable and Accessible can be adopted, the elements Interoperable and Reusable need to be adapted. The principle Interoperable must additionally imply that various generally accepted data formats can be used in a modelled workflow. When necessary, an existing workflow must be adaptable accordingly. Moreover, according to the interpretation of the Reusable principle described in ( Draxl &Scheffler 2020 ), a workflow must, on the one hand enable the reliable reproduction of results and, on the other hand, be fully or partially repurposable for different use cases. The conditions Findable and Accessible are already fulfilled in the case of the workflow system presented here through the direct integration in Kadi4Mat. This allows workflows to be stored in the community repository and thus to be shared with the scientific community. As for ordinary research data, the workflows stored in Kadi4Mat can be equipped with descriptive metadata, that can be selectively searched. Consequently, these principles need not to be specifically considered in the implementation of the actual workflow system. The technical implementation of the workflow editor presented hereafter, therefore, concentrates on the interoperability and reusability of the modelled workflows. Additionally, the requirements of the editor to be generic and simple in use ( Pizzi et al. 2016 ) are taken into consideration.
The scheme described in Chapter 2 for the abstraction of scientific processes, defines individual functions or tools described according to the IPO model as the basic building blocks of a process. These basic building blocks are implemented in KadiStudio in the form of various nodes.
In both workflow editors — standalone and web-based version —, these nodes can be added and connected to model a process within a graphical user interface (GUI) using an intuitive point-and-click mechanism as shown in Figure 5 .
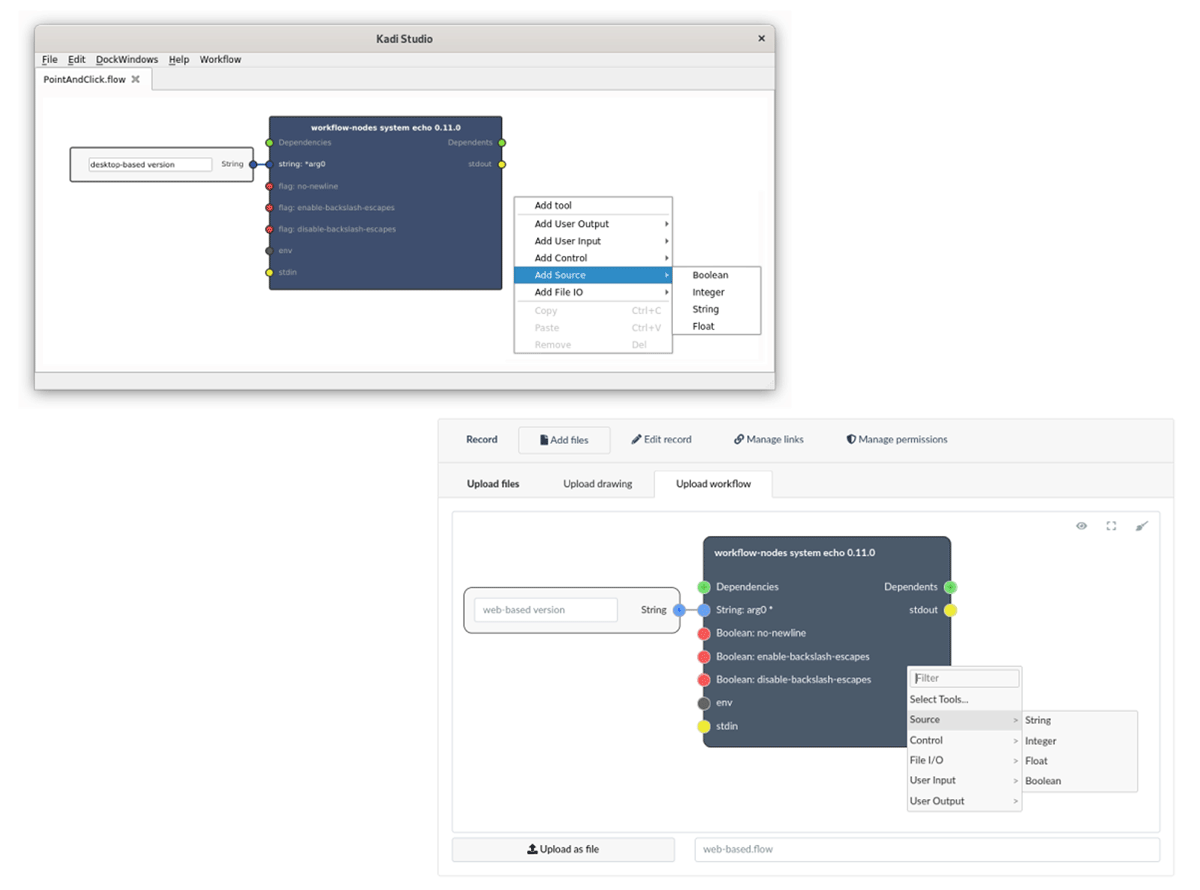
Overview of the available workflow editors, showing the GUI of the desktop (top) and the web-based version (bottom). Workflows can be modelled by adding and connecting nodes using a point-and-click interface.
Each of the insertable nodes represents a certain process, modelled according to the IPO model and can be differentiated between (1) tool nodes, which serve to integrate various programs or functions, (2) environment nodes, which are used in combination with tool nodes, and finally (3) built-in nodes, which allow to influence the execution of the workflow and add interactive options as well as variables to the editor. These node types are presented in Figure 6 .
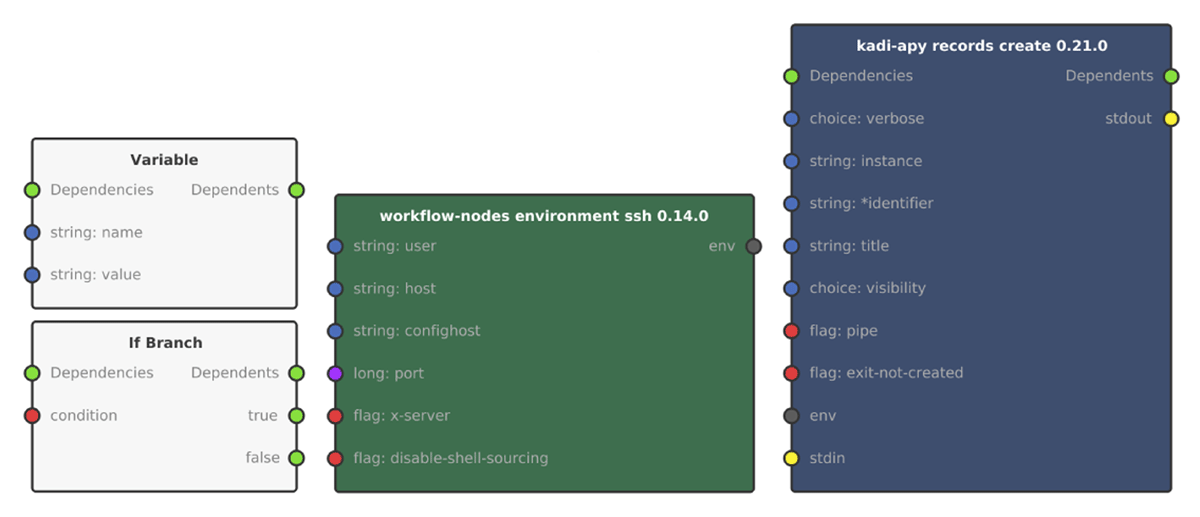
Available node types. Built-in nodes are grey, environment nodes green and tool nodes blue.
In accordance with the IPO model, each node describes a specific process that contains both inputs and outputs represented by the input and output ports respectively. The input ports are located on the left-hand side of the node and the output ports on the right-hand side. Depending on their task, the ports can be divided into parameterisation, dependency, environment, and stdin/stdout ports. Stdin and stdout ports refer to the standard input and standard output streams of the underlying program, respectively. Parameterisation ports are used to pass arguments and options necessary to execute the node, such as string or boolean values. The execution order of the nodes, including control mechanisms such as if-conditions and for-loops, can be defined using the dependency ports. Environment ports are used to set a prefix to a tool node to execute it in a specific environment, such as a secure shell (SSH), that enables the remote execution of tools. Piping the output of a node into another node can further be realised using stdin and stdout ports. The provision of nodes that have these defined inputs and outputs is the basis of workflow modelling in KadiStudio. This is in accordance to the identified structure illustrated in Figure 3 . Connecting and parameterising the nodes using the named ports allows to add the data flow according to the APR model to the workflow as described in Chapter 2. This puts the added nodes into defined relations and allows the user to see at first glance which inputs a process uses and to which process its output is forwarded. This structures the data flow in a comprehensible manner.
When executing a workflow, the added nodes and their connections are processed and translated into command line interface (CLI) commands. Hence, the workflow editor can be understood as a graphical programming language with simple usability due to its intuitive character, also allowing inexperienced users to model their workflows. The use of this modelling mechanism will be presented in the following examples.
4.1 Parameterisation and use of nodes
As mentioned in the previous section, tool nodes represent CLI commands, structured according to the IPO model, thus possessing defined inputs and outputs. To parameterise the underlying CLI command, the node’s input ports are used. A simple example of such a parameterisation is presented in Figure 7 .
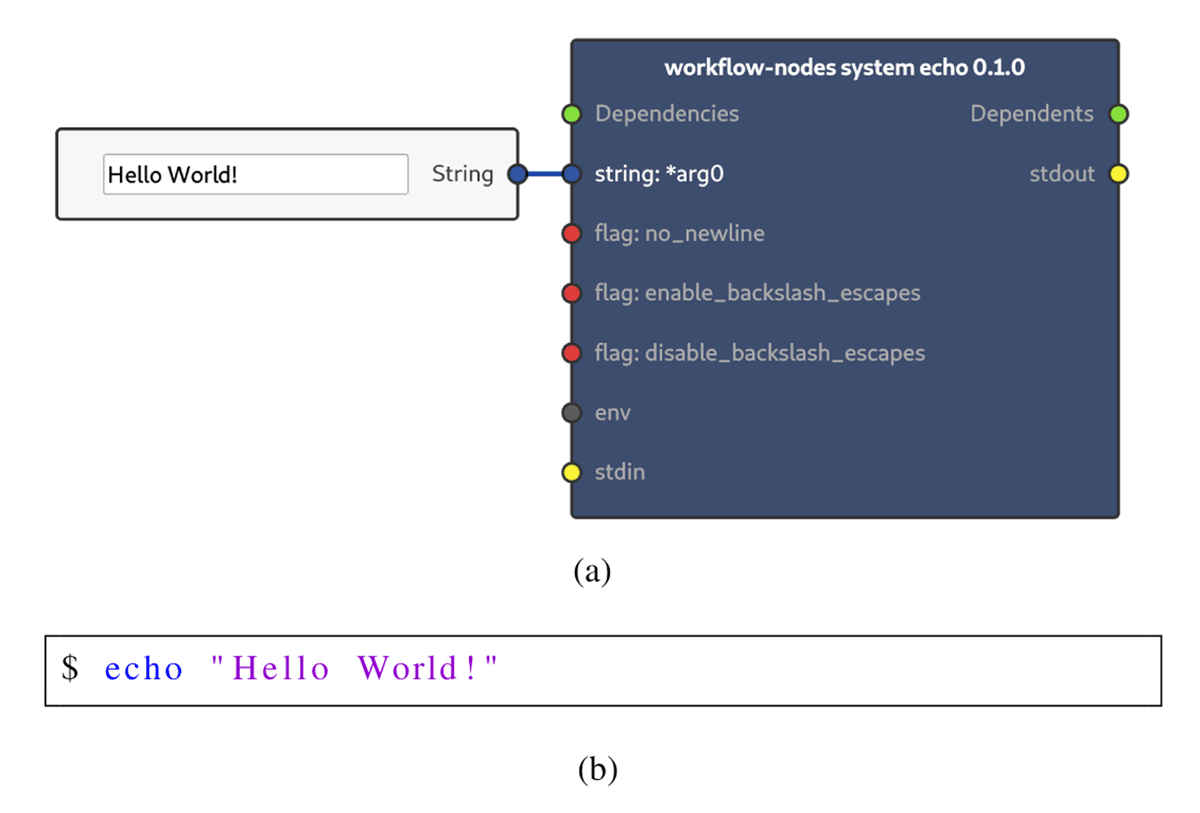
Overview of the node parameterisation. The parameterisation of an echo node using a string source node is shown in (a) . this is equivalent to the command shown in (b) .
The added tool node represents the ‘echo’ CLI command. Adding a source node of type string and connecting it to the tool node allows for the parameterisation of the echo command. In the presented example, ‘Hello World!’ is passed to the tool node, resulting in the command shown in Figure 7(b) . To pipe a node’s output into another node, the stdout and stdin ports are used. Adding a File Output node to the workflow example of Figure 7 and connecting it to the tool node as shown in Figure 8(a) activates this piping functionality. As can be seen in the resulting command shown in Figure 8(b) , the standard output is forwarded to the second node.
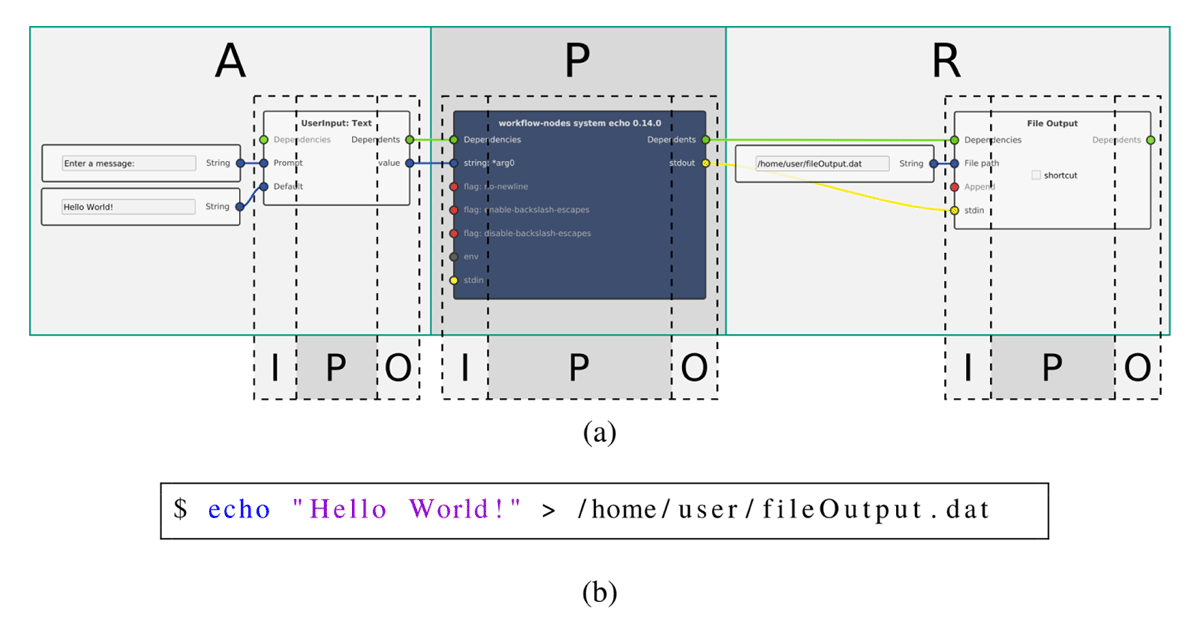
Visualisation of the workflow modelling concept implemented in KadiStudio. Multiple tools are connected according to the APR model forming a simple workflow. Connecting the stdout port to the stdin port as shown in (a) pipes the standard output stream of the tool node into the standard input stream of the File Output node that finally routes them into a file. This demonstrates structuring the tools of a task depending on their purpose in the data flow. This has the same effect as the command shown in (b) .
Moreover, the parameterisation of the tool node in Figure 8 is realised using a UserInput: Text node that prompts the user for an input when executed. In addition, the dependency ports have also been connected in this example. Defining the dependencies of the workflow nodes supports the process engine in determining the execution order of the added nodes. In the shown example, this implies that the File Output node is not executed until the echo node has been called and executed successfully. In general, when modelling a workflow, it is strongly recommended that the dependency ports are connected, as undefined dependencies may result in a wrong execution order, possibly rendering the workflow inoperable.
Figure 8 also vividly illustrates the workflow modelling concept implemented in KadiStudio. The used tools are defined by certain inputs, outputs and a process thus complying with the IPO model. Depending on their purpose they can be assigned to the elements of the APR model. Specifically, the data acquisition consists of the UserInput: Text node that prompts the user for an input, which is forwarded to the data processing in form of the echo node by connecting the ports. After processing this input in the echo node, the result is forwarded to the next node in the subsequent data routing. In this case, the received input is routed into a file using the File Output node. This demonstrates the idea of structuring the work steps of each task in the APR model according to their purpose in the present data flow. In the previous examples, the nodes were parameterised using different built-in nodes. On the one hand with source nodes, that provide the computational values string, boolean, integer , and float , which are set when the workflow is created and remain constant for each execution. On the other hand, by using user input nodes, that allow to formulate more generic workflows by granting the possibility to interactively redefine certain parameters during the workflow execution. These nodes pause the execution of the workflow and prompt the user for an input via a dialogue box before continuing the execution, as shown in Figure 9 .
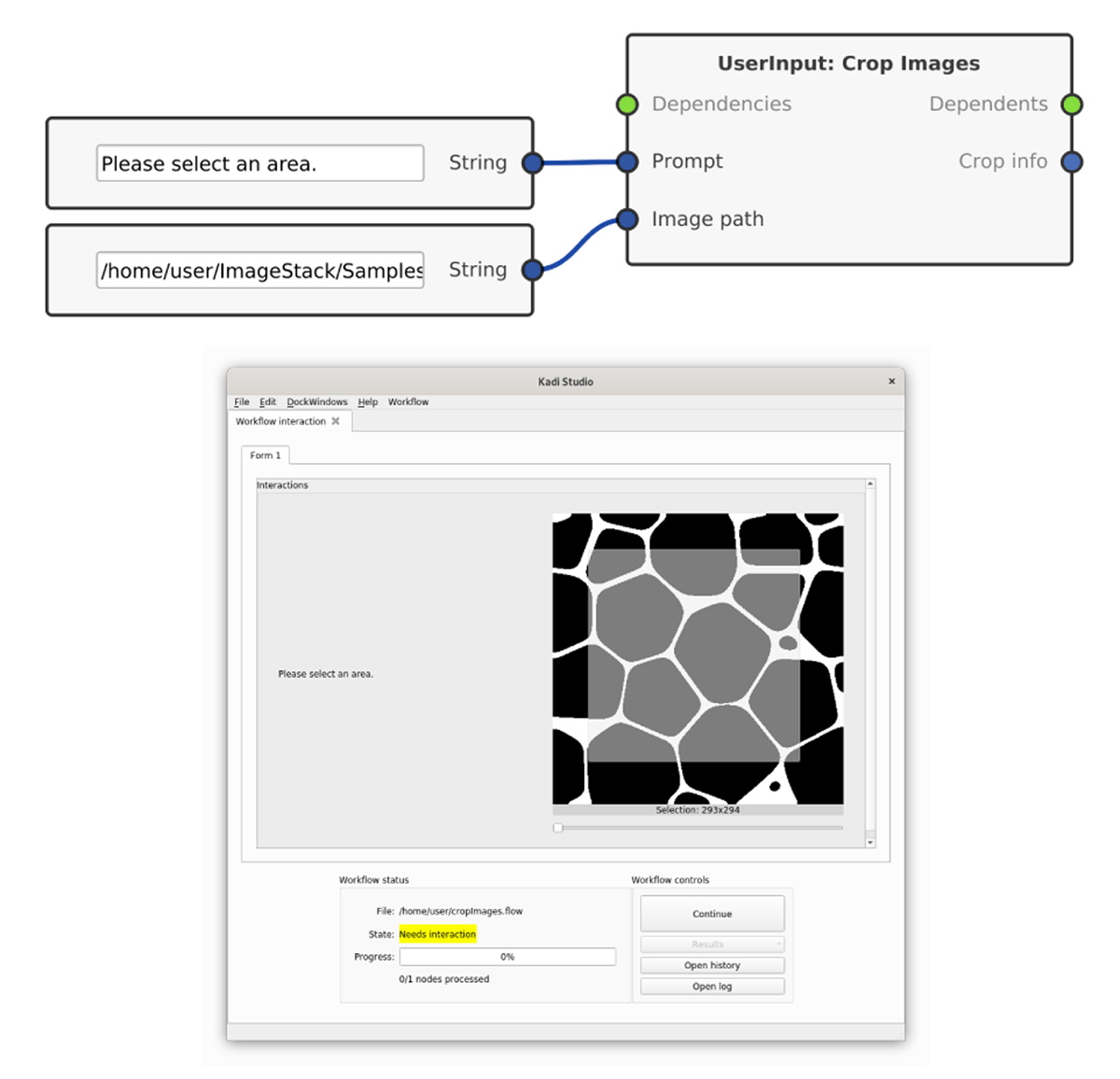
Usage of UserInput nodes. During the execution, the user is prompted for an input, such as to select an image area, as shown in this example. The selected area can then be used in further investigations.
Through the use of such user interactions the user is given control over the workflow execution allowing to adapt to different use cases. Workflows are therefore not just predefined scripts that can be applied under certain circumstances, but can be seen as generic tools adaptable to the current use case during execution. The interactively definable parameters are manifold and include not only the query of basic computational values but also the selection of files and, for example, the cropping of images to a section to be examined, as depicted in Figure 9 . Using this prompting mechanism also allows to model manual worksteps in KadiStudio. This is realised by requesting the user to conduct a workstep with certain inputs and querying the results obtained as shown in Figure 10 . To ensure the reproducibility of the results obtained in workflows that use such user interactions, the interactively defined user inputs are saved within a log file. When uploading the results to a repository such as Kadi4Mat, the logged user inputs can be used as metadata for the generated data.
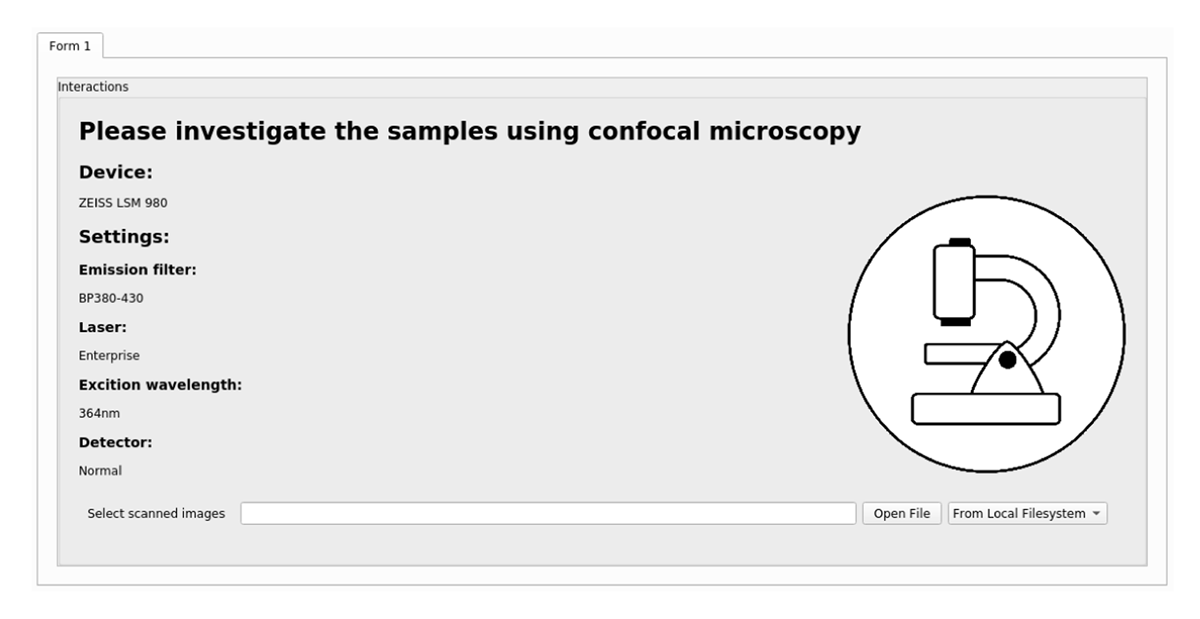
Integrating manual work steps by giving the user all necessary parameters and asking them to select the generated results.
Apart from the interactive nodes, KadiStudio offers various other built-in nodes such as Variable, Loop or ifBranch that allow to manipulate the workflow execution and facilitate the formulation of generic research workflows. The provision of these built-in nodes in the workflow system KadiStudio thus not only guarantees the reproducibility of any manual or digital research process, but also allows for the repurposability of the workflows.
4.2 Adding new nodes
To guarantee the interoperability of modelled workflows and to model the heterogeneous tool landscape present in scientific research, the repertoire of nodes available in KadiStudio must be easily extendable. In this way, custom functionalities and data conversion nodes can be incorporated into the workflow editor, enabling formulation of arbitrary workflows and their application to different file formats for instance. The interface for integrating new tools was therefore kept as simple as possible. Prerequisites for a new node to be added to the editor are only that the underlying CLI-command is (1) executable and (2) provides the --xmlhelp option. The --xmlhelp option returns a machine-readable description of the command, that is needed by the workflow editor to create the visual representation of the command within the editor. In case the desired tool does not provide this option, it can be added retroactively, for example, with a wrapper script using the xmlhelpy Python library ( Kadi4Mat Team & Contributors 2022d ). Listing 1 of appendix A shows an abbreviated, exemplary implementation of such a Python wrapper for the echo command. The XML output generated by this wrapper is shown in Figure 11 .

XML output of the wrapper script shown in Listing 1 of appendix A, that is printed when using the --xmlhelp option. The root element program specifies the command as a regular tool. Each of the param elements represents a configurable option of the wrapped command.
The structure of an xmlhelp output always follows the same pattern. After the declaration as an XML document, a root element of type program or env follows, which indicates a tool or an environment node respectively and is provided with the name, description and version attributes. Within this element, a param element is specified for each possible parameter of the command, which must contain the name, description and type attributes in order to be rendered within the editor. Additionally, further attributes such as a default value can be defined. The definition of these params specifies the inputs of the final node and thus serves to represent the underlying process with respect to the IPO model. The tool node derived from the xmlhelp above is shown in Figure 12 .

Created echo node. Echo node derived from the XML output shown in Figure 11 . Each param is represented by an input port. Tool node specific ports such as env are added automatically.
The wrapper script shown in Listing 1 of appendix A is part of the workflow-nodes library ( Kadi4Mat Team & Contributors 2022c ), that already contains various Python-based nodes covering basic as well as some more specialized functions. The tool thus fulfills both prerequisites: (1) executable , (2) --xmlhelp option and can be added to the editor’s tool list using its GUI as shown in Figure 13 . In the dialogue every executable within the PATH environment variable is listed. Selecting a tool will permanently add it to the usable tools of the editor.
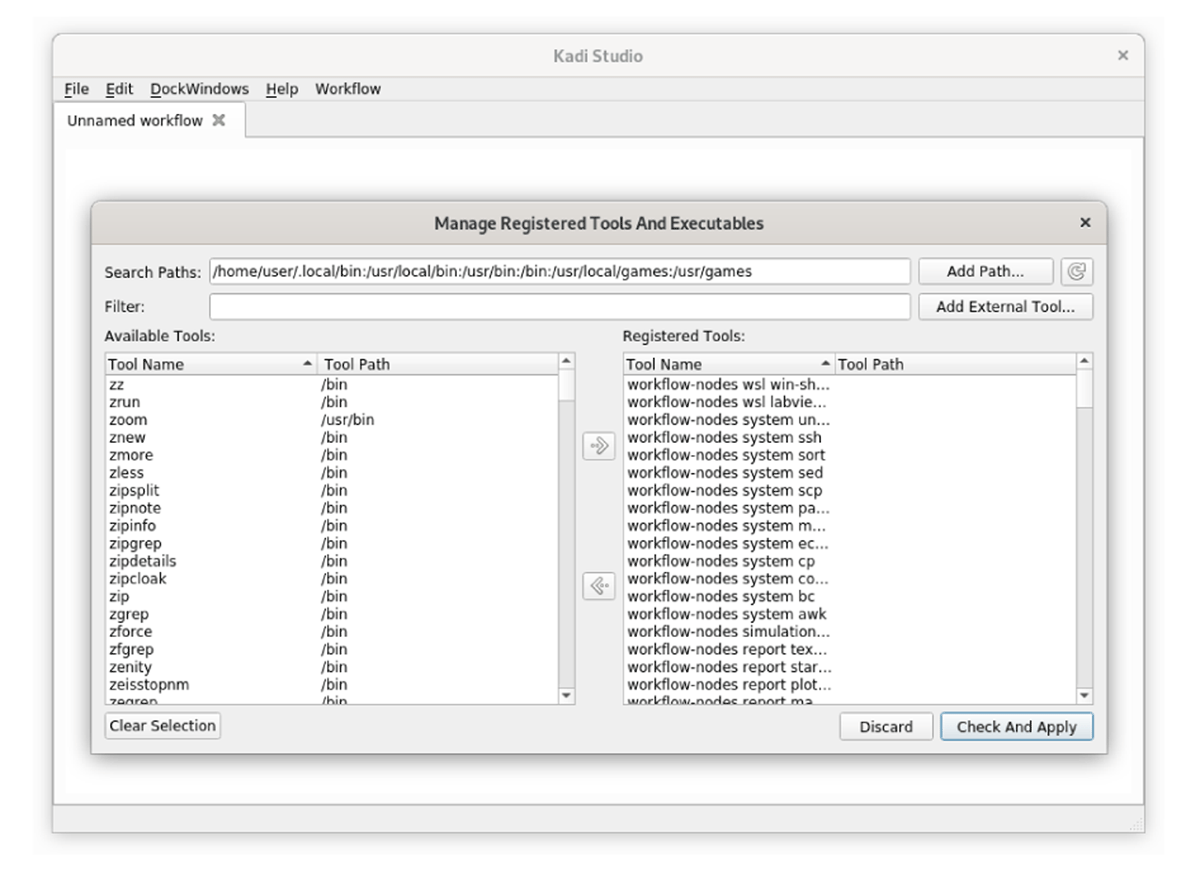
Dialogue for registering tools in the editor of KadiStudio. All executables in the PATH are listed. Commands can be queried and the default search path can be extended by the user.
The provision of the described interface for adding new nodes to KadiStudio enables not only the formulation of arbitrary workflows but also allows for the simple adaptation of existing workflows to different file formats. This contributes to the generic character of KadiStudio and ensures the interoperability of the workflows modelled in it.
4.3 Link between Kadistudio and a repository
As already mentioned, workflows created in KadiStudio can be stored directly in Kadi4Mat and provided with metadata. This permits the findable and accessible storage of workflows. Since the FAIR idea however does not only apply to workflows but to all scientific data, KadiStudio aims to provide FAIR documentation of the data created during a workflow. For this purpose, a link between the workflow editor and an arbitrary repository can be established. As a reference, this link has already been implemented for the repository of Kadi4Mat in form of tool nodes collected in the kadi-apy library ( Kadi4Mat Team & Contributors 2022a ). These nodes access the repository and its functionalities via the application programming interface (API) provided by Kadi4Mat, which offers a set of defined functions and interfaces to interact with KadiWeb . Registering a repository to KadiStudio is realised using a graphical user interface as shown in Figure 14 . In case of Kadi4Mat this requires its host address as well as a personal access token (PAT).
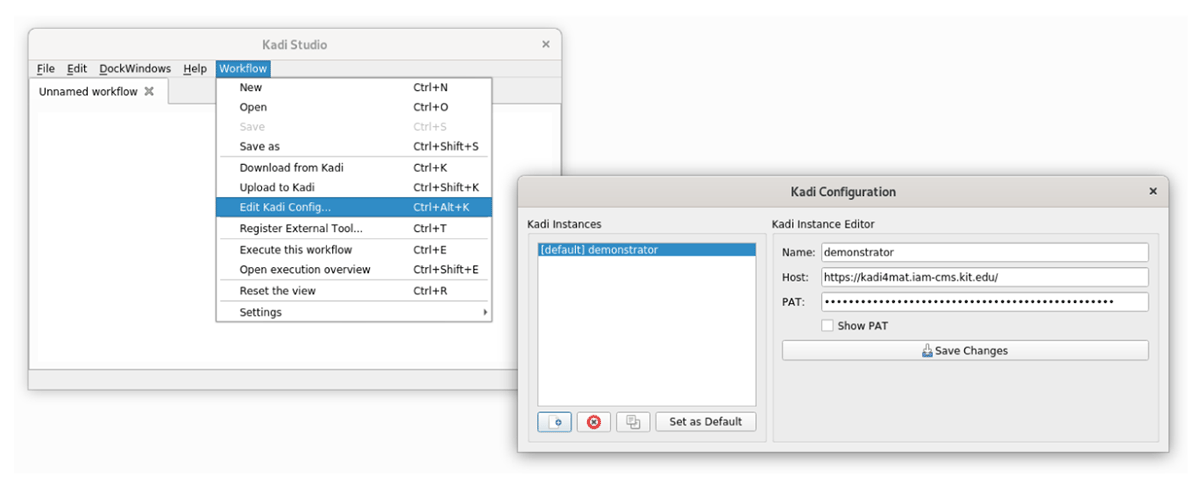
Dialogue for registering Kadi4Mat instances. Registered instances can be accessed during a workflow to use the functionalities of the repository and to exchange data.
Linking the local editor with the Kadi4Mat repository opens up various possibilities. Workflows saved in the repository can be loaded directly into the local editor to be edited. In addition, data stored in the repository can be loaded and processed within workflows and the corresponding results can be uploaded automatically to Kadi4Mat and provided with descriptive metadata. Establishing a link to a repository such as Kadi4Mat thus allows to holistically model research processes and the data used therein in a comprehensible manner. In summary, KadiStudio’s access to the data allows to map the entire scientific work from the raw or source data, through its analysis and use up to the structured storage within the repository. Structuring the generated data in Kadi4Mat and defining their interrelationships further enables their provenance tracking. In this way KadiStudio provides the possibility for FAIR handling of data generated during a workflow.
5 Technical Aspects
The technical details of the workflow editor, its architecture and related software components are presented in the following. The focus of these developments was again on the objective of creating a generic and intuitive software system, that allows for the FAIR formulation of research processes.
5.1 xmlhelpy
The generic character of the workflow editor is partly implemented by supporting the simple extension of the available tools. To incorporate a new tool, it must provide the --xmlhelp option, that returns a machine-readable description of the tool. Adding this option to existing tools can be realised using the xmlhelpy library. Xmlhelpy is a Python library available on PyPI . It is based on the open source framework Click ( The Pallets Projects 2014 ), and extends it with custom classes and functions. As other related libraries for argument parsing, xmlhelpy allows to conveniently specify a program’s command line arguments and takes care of parsing and validating them. It then automatically adds the --xmlhelp option to the program allowing to obtain its specification in a machine-readable format.
5.2 Architecture for workflow execution
Figure 15 gives an overview of the workflow system’s architecture. As mentioned in the previous sections, two versions of the workflow editor exist, which both use the same JSON-based file format to load and save workflows. For execution, the workflow files are managed by the process manager, a software component dedicated to providing a unified interface for workflow execution. The process manager does not read or analyse the workflow files by itself but only acts as a thin layer of abstraction for the GUI components using its interface. Instead, it passes the workflow files on to a suitable process engine and instructs it with the execution. This way, the process engine implementations can be exchanged easily, enabling the flexible adaptation of the workflow system to changing requirements, thus strengthening the generic character of the Kadi4Mat workflow system. For this, a variety of process engines with specific characteristics suitable for a certain use case—remote execution, parallel execution, distributed execution, or running on large computer clusters/high performance computers—or workflow file format can be registered in the process manager. It is therefore conceivable to integrate other programs for workflow execution, such as Fireworks ( Jain et al. 2015 ), in form of a specialised process engine. As a reference implementation, a fully functional process engine is provided ( Zschumme, Schoof, et al. 2022 ), which follows a sequential execution approach. A detailed description of this process-engine implementation and of the process-manager is given in the following sections.

Architecture of the workflow system. Based on Figure 3 ( Brandt et al. 2021 ) (CC BY 4.0). The process manager orchestrates and monitors the execution of workflows by delegating it to a suitable process engine and tracking its status.
5.3 Process engine
The term process engine refers to a software component dedicated to running workflows and performing all tasks formulated in the workflow. As shown in Figure 15 , the general concept takes into account that several different implementations might be available to flexibly adapt the execution to different technical emphases or capabilities.
Since each process engine implementation is free in how it executes the workflow, there is a wide field of possibilities, ranging from executing the workflow locally to delegating the execution to another application or computer to just printing a workflow description in a format like common workflow language (CWL) ( Crusoe et al. 2021 ) or workflow description language (WDL) ( Frazer et al. 2012 ). Prototype implementations of process engines running Kadi4Mat workflows with Fireworks ( Jain et al. 2015 ) and CWL ( Crusoe et al. 2021 ) have already been implemented. These promising approaches are to be continued in the future and could expand the current possibilities.
At the moment there is one fully functional process engine implementation ( Zschumme, Schoof, et al. 2022 ), which is a CLI-based application written in C++, published under the Apache-2.0 license. It serves as a reference implementation and allows local execution of workflows created with any of the workflow editors presented in Chapter 3. Its schematic functioning is outlined in the following.
When instructed to execute a workflow, the process engine first reads the workflow file and parses its JSON-structured content. During this step the process engine creates an internal in-memory representation of the workflow based on the unsorted lists of node descriptions and connections contained in the workflow file. An important aspect of this analysis is the determination of the execution order. For this, the data connections in the workflow as well as the explicit dependency connections are considered. The dependency connections also help with identifying conditional execution paths implied by If Branch or For Loop nodes for example.
After determining the correct order of execution, the implemented process engine executes each node of the workflow sequentially. This sequential and separate execution requires the nodes to provide its own execution function, which can be called as soon as the process engine decides to run the corresponding node. In order to manage the workflow execution, the implemented process engine uses a number of control files that are stored in an execution folder unique to every execution. Within these files, information about the progress of the execution, log files, and other necessary information is stored. This central storage of all relevant information eases debugging and ensures the reproducibility of the obtained results. When all nodes have been processed successfully, the execution is completed.
5.4 Process manager
The process manager is a CLI-based application written in C++ published under the Apache-2.0 license ( Zschumme, Steinhülb & Brandt 2022 ). As shown in Figure 15 , it provides an interface for the GUI components to run workflows and to monitor and interact with already existing execution instances. It further administers the different process engines, which can be configured in a JSON-based configuration file that contains information on how to use the interface of each engine. When executing a workflow, the user can select an engine suitable for the current use-case from this list of available engines. In case the user omits this choice, the process manager instructs the default process engine with the workflow execution.
Once a workflow is started, the process manager will interact with the responsible process engine to obtain the status and log of the execution or to provide user input. For this, the process manager assigns a unique identifier to each execution instance and creates an execution folder which is used exclusively during this particular execution. The process engine is then free to use this provided, empty folder for storage. This eliminates the risk of overwriting important data from previous executions, which is why the execution folder is used as the base path for all executed programs by the current process engine implementation ( Zschumme, Schoof, et al. 2022 ). Depending on the internals of the workflow, however, different save paths can be used as well.
6 Conclusion
In this paper, a concept for the FAIR formulation of research processes was presented and its concrete implementation in form of the workflow system KadiStudio introduced. The implemented software allows for the FAIR modelling of scientific research processes in form of automatable and reproducible workflows. Basis for this was the identification of a generic structure in research processes that can be used for their modelling. For this purpose, the input-process-output model was iteractively applied to research processes until a structure emerged, that enables the bottom-up modelling of any process. The found structure abstracts processes as the concatenation of various functions or programs that are defined by certain inputs, a process, and outputs. The interconnection and parameterisation of these programs subsequently allows to model the data flow within the research process comprehensibly. Stringing all work steps of a workflow together through the said connections finally models the complete workflow. Using this method, arbitrary workflows can be created. The atomistic process description on different levels further permits the identification of reusable elements in workflows. Thus, using workflows research results can not only be made reproducible, but the used methods can also be applied for other purposes. This reuse of certain procedures additionally minimises the susceptibility to errors in scientific workflows and thereby guarantees the quality assurance of scientific data.
In KadiStudio the identified process structure is implemented by providing different nodes, each of which represents a certain function or program. By adding and connecting them in a graphical user interface using an intuitive point-and-click mechanism, research processes can be modelled. To guarantee the FAIR formulation of workflows in KadiStudio, certain design choices have been made and specific functionalities have been included. The aspects findable and accessible are ensured by directly integrating KadiStudio into Kadi4Mat, thus allowing the structured storage and management of workflows within its repository. This also applies to the scientific data generated in workflows, which can be automatically provided with descriptive metadata and stored in Kadi4Mat in a FAIR manner. The interoperability of workflows is further guaranteed by giving the user the possibility to add and adapt nodes in KadiStudio. In this way adaptions necessary to process different file formats can be easily implemented into existing workflows. Through the simple extension of the available tools the heterogeneous tool landscape present in science can additionally be represented in KadiStudio. Hence, the workflow editor can be used in any scientific domain to model the occurring processes. This also incorporates manually performed worksteps, which can be integrated through the provided user interaction nodes and prompts. These interactive elements additionally enable the formulation of generic workflows that can be applied to different use cases therefore ensuring the repurposablility of modelled workflows. Logging all inputs provided during the execution of a workflow finally realises the reproducibility of a workflow execution as well as of all data generated within it.
Summarising, KadiStudio offers an easily adaptable and intuitively usable solution to holistically model arbitrary research processes and the data generated therein in a FAIR manner. The implemented system is simple in use and can be flexibly adapted to any scientific domain, therefore also satisfying the requirements to a workflow system formulated by ( Pizzi et al. 2016 ). KadiStudio thus makes a decisive contribution to promoting FAIR handling of data and scientific processes and therefore to the realisation of the fourth scientific paradigm.
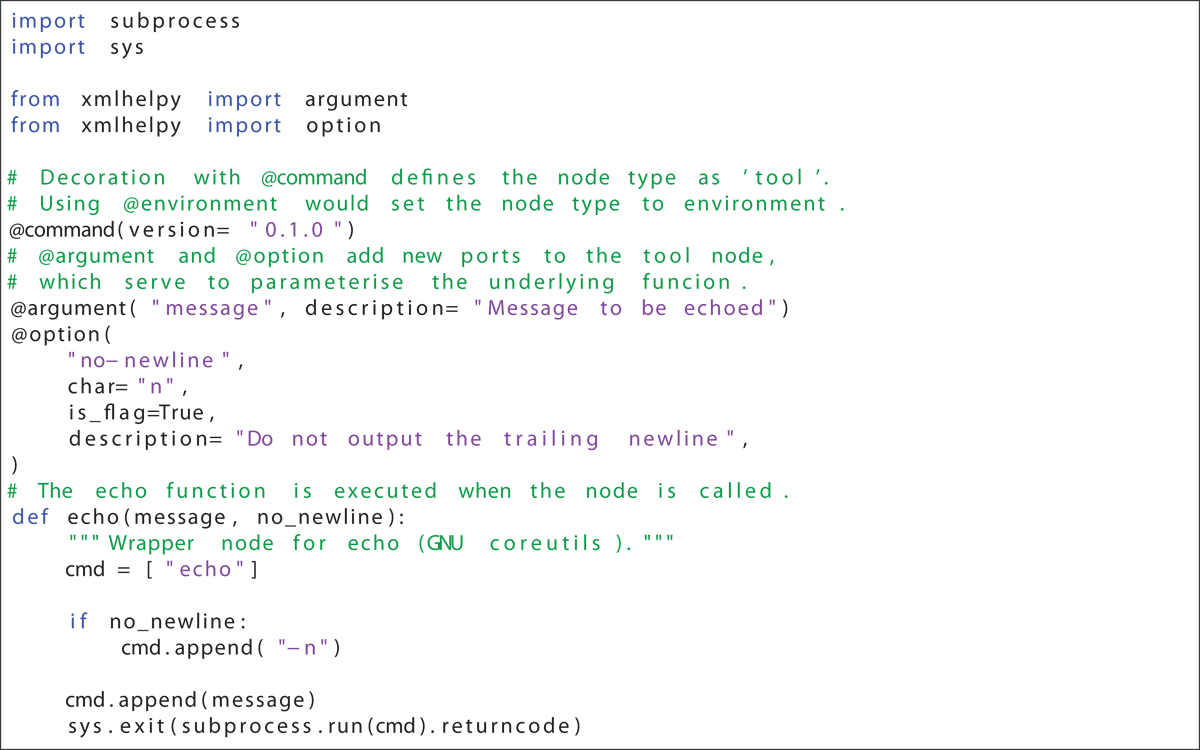
Echo node example.
Acknowledgements
This work is partly funded by the German Research Foundation (DFG) under Project ID 390874152 (POLiS Cluster of Excellence), by the German Federal Ministry of Education and Research (BMBF) in the project FB2 TheoDat (project number 03XP0435D), by the Ministry of Science, Research and Art Baden-Württemberg (MWK-BW) in the project MoMaF–Science Data Center, with funds from the state digitization strategy digital@bw (project number 57), by the Helmholtz association in the project INNOPOOL MDMC (program No. 43.35.01) and also funded by the BMBF and MWK-BW as part of the Excellence Strategy of the German Federal and State Governments in the project Kadi4X. We would also like to acknowledge the German Federal Ministry of Education and Research (BMBF) for its financial support within the project AQuaBP, under the grant number 03XP0315B. Some ideas presented in this paper are enhanced by the fruitful discussions in different working groups of the project NFDI4Ing.
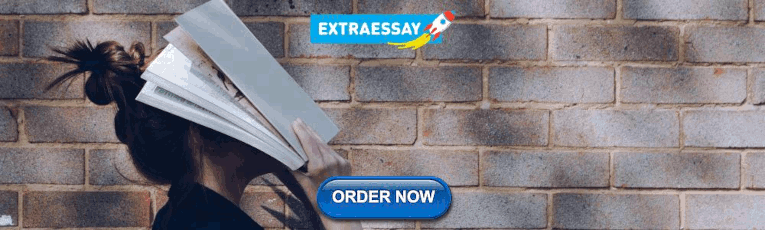
Competing Interests
The authors have no competing interests to declare.
Afgan, E, et al. 2018. The Galaxy platform for accessible, reproducible and collaborative biomedical analyses: 2018 update. Nucleic acids research , 46(W1): W537–W544. DOI: https://doi.org/10.1093/nar/gky379
Berthold, MR, et al. 2009. KNIME-the Konstanz information miner: version 2.0 and beyond. AcM SIGKDD explorations Newsletter , 11(1): 26–31. DOI: https://doi.org/10.1145/1656274.1656280
Brandt, N, et al. 2021. Kadi4Mat: A Research Data Infrastructure for Materials Science. Data Science Journal 20.1. DOI: https://doi.org/10.5334/dsj-2021-008
Crusoe, MR, et al. 2021. Methods included: standardizing computational reuse and portability with the common workflow language. arXiv preprint arXiv, 2105.07028 . DOI: https://doi.org/10.1145/3486897
Crusoe, MR, et al. May 2022. Methods Included: Standardizing Computational Reuse and Portability with the Common Workflow Language. Commun. ACM , 65(6): 54–63. DOI: https://doi.org/10.1145/3486897
Demšar, J, et al. 2013. Orange: Data Mining Toolbox in Python. Journal of Machine Learning Research , 14: 2349–2353.
Di Tommaso, P, et al. 2017. Nextflow enables reproducible computational workflows. Nature biotechnology , 35(4): 316–319. DOI: https://doi.org/10.1038/nbt.3820
Dmitry, PEA. 2017. Qt5 Node Editor . https://github.com/paceholder/nodeeditor .
Draxl, C and Scheffler, M. 2020. Big data-driven materials science and its FAIR data infrastructure. Handbook of Materials Modeling: Methods: Theory and Modeling , 49–73. DOI: https://doi.org/10.1007/978-3-319-44677-6_104
Frazer, S, et al. 2012. Workflow Description Language – Specification and Implementations . https://libraries.io/github/openwdl/wdl .
Goel, A. 2010. Computer fundamentals . Pearson Education India.
Hey, AJ, Tansley, S, Tolle, KM, et al. 2009. The fourth paradigm: data-intensive scientific discovery . Vol. 1. WA: Microsoft research Redmond.
Jain, A, et al. 2015. FireWorks: A dynamic workflow system designed for high-throughput applications. Concurrency and Computation: Practice and Experience , 27(17): 5037–5059. DOI: https://doi.org/10.1002/cpe.3505
Kadi4Mat Team and Contributors. June 2022a. IAM-CMS/kadi-apy: Kadi4Mat API Library . Version 0.23.0. DOI: https://doi.org/10.5281/zenodo.6623518
Kadi4Mat Team and Contributors. June 2022b. IAM-CMS/kadi: Kadi4Mat . Version 0.25.1. DOI: https://doi.org/10.5281/zenodo.6623521
Kadi4Mat Team and Contributors. July 2022c. IAM-CMS/workflow-nodes . Version 0.15.0. DOI: https://doi.org/10.5281/zenodo.6806747
Kadi4Mat Team and Contributors. February 2022d. IAM-CMS/xmlhelpy . Version 0.9.2. DOI: https://doi.org/10.5281/zenodo.5971732
Kadi4Mat Team and Contributors. July 2022e. kadistudio: 0.1.0.alpha1 . Version 0.1.0.alpha1. DOI: https://doi.org/10.5281/zenodo.6810891
Kluyver, T, et al. 2016. Jupyter Notebooks? A publishing format for reproducible computational workflows. In: Loizides, F and Scmidt, B (eds.), Positioning and Power in Academic Publishing: Players, Agents and Agendas . IOS Press. pp. 87–90. DOI: https://doi.org/10.3233/978-1-61499-649-1-87
Mölder, F, et al. 2021. Sustainable data analysis with Snakemake. F1000 Research , 10. DOI: https://doi.org/10.12688/f1000research.29032.1
Pizzi, G, et al. 2016. AiiDA: automated interactive infrastructure and database for computational science. Computational Materials Science , 111: 218–230. DOI: https://doi.org/10.1016/j.commatsci.2015.09.013
The Pallets Projects. 2014. Click – The Pallets Projects . https://palletsprojects.com/p/click/ .
Wilkinson, MD, et al. 2016. The FAIR Guiding Principles for scientific data management and stewardship. Scientific data , 3(1): 1–9. DOI: https://doi.org/10.1038/sdata.2016.18
Zelle, JM. 2004. Python programming: an introduction to computer science . Franklin: Beedle & Associates, Inc.
Zschumme, P, Schoof, E, et al. July 2022. IAM-CMS/process-engine . Version 0.5.0. DOI: https://doi.org/10.5281/zenodo.6806707
Zschumme, P, Steinhülb, J and Brandt, N. February 2022. IAM-CMS/process-manager . Version 0.2.0. DOI: https://doi.org/10.5281/zenodo.5972885
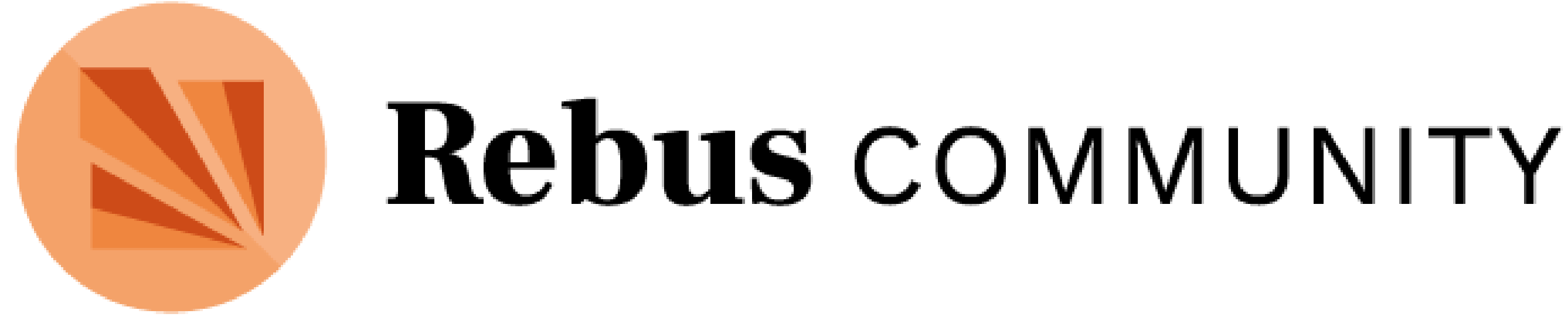
Want to create or adapt books like this? Learn more about how Pressbooks supports open publishing practices.
Input-Process-Output Model
Dave Braunschweig
The input–process–output (IPO) model is a widely used approach in systems analysis and software engineering for describing the structure of an information processing program or another process. Many introductory programming and systems analysis texts introduce this as the most basic structure for describing a process. [1]
A computer program or any other sort of process using the input-process-output model receives inputs from a user or other source, does some computations on the inputs, and returns the results of the computations. The system divides the work into three categories: [2]
- A requirement from the environment (input)
- A computation based on the requirement (process)
- A provision for the environment (output)
For example, a program might be written to convert Fahrenheit temperatures into Celsius temperatures. Following the IPO model, the program must:
- Ask the user for the Fahrenheit temperature (input)
- Perform a calculation to convert the Fahrenheit temperature into the corresponding Celsius temperature (process)
- Display the Celsius temperature (output)
- Wikiversity: Computer Programming
- Flowgorithm – Flowchart Programming Language
- Wikipedia: IPO model ↵
Programming Fundamentals Copyright © 2018 by Dave Braunschweig is licensed under a Creative Commons Attribution-ShareAlike 4.0 International License , except where otherwise noted.
Share This Book
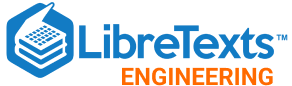
- school Campus Bookshelves
- menu_book Bookshelves
- perm_media Learning Objects
- login Login
- how_to_reg Request Instructor Account
- hub Instructor Commons
- Download Page (PDF)
- Download Full Book (PDF)
- Periodic Table
- Physics Constants
- Scientific Calculator
- Reference & Cite
- Tools expand_more
- Readability
selected template will load here
This action is not available.
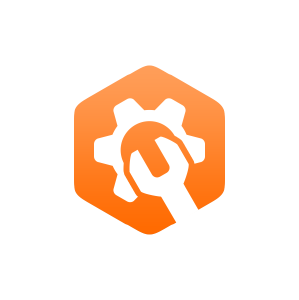
2.20: Input-Process-Output Model
- Last updated
- Save as PDF
- Page ID 10612
The input–process–output (IPO) model is a widely used approach in systems analysis and software engineering for describing the structure of an information processing program or another process. Many introductory programming and systems analysis texts introduce this as the most basic structure for describing a process. [1]
A computer program or any other sort of process using the input-process-output model receives inputs from a user or other source, does some computations on the inputs, and returns the results of the computations. The system divides the work into three categories: [2]
- A requirement from the environment (input)
- A computation based on the requirement (process)
- A provision for the environment (output)
For example, a program might be written to convert Fahrenheit temperatures into Celsius temperatures. Following the IPO model, the program must:
- Ask the user for the Fahrenheit temperature (input)
- Perform a calculation to convert the Fahrenheit temperature into the corresponding Celsius temperature (process)
- Display the Celsius temperature (output)
- Wikiversity: Computer Programming
- Flowgorithm – Flowchart Programming Language
- Wikipedia: IPO model ↵

An official website of the United States government
The .gov means it’s official. Federal government websites often end in .gov or .mil. Before sharing sensitive information, make sure you’re on a federal government site.
The site is secure. The https:// ensures that you are connecting to the official website and that any information you provide is encrypted and transmitted securely.
- Publications
- Account settings
Preview improvements coming to the PMC website in October 2024. Learn More or Try it out now .
- Advanced Search
- Journal List
- J Educ Health Promot
- v.10(1); 2021
Context, Input, Process, and Product Evaluation Model in medical education: A systematic review
Monireh toosi.
PhD Candidate, Department of Reproductive Health and Midwifery, School of Nursing and Midwifery, Tehran University of Medical Sciences, Tehran, Iran
Maryam Modarres
1 Department of Reproductive Health and Midwifery, School of Nursing and Midwifery, Member of Nursing and Midwifery Care Research Center, Tehran University of Medical Sciences, Tehran, Iran
Mitra Amini
2 Clinical Education Research Center, Shiraz University of Medical Sciences, Shiraz, Iran
Mehrnaz Geranmayeh
3 Department of Reproductive Health and Midwifery, Faculty of Nursing and Midwifery, Tehran University of Medical Sciences, Tehran, Iran
BACKGROUND:
Evaluation is one of the most important tools for determining the quality of any educational program, which can lead to reformation, revision, or termination of programs. Quality in higher education requires assessment and judgment of goals and strategies, executive policies, operational processes, products, and outcomes. The Context, Input, Process, and Product (CIPP) model is a comprehensive perspective that attempts to provide information in order to make the best decisions related to CIPP. Due to the importance of this topic, the present study examined the application of the CIPP model in the evaluation of medical education programs through a systematic review.
MATERIALS AND METHODS:
In this systematic review, Persian databases including ISC, SID, Mag Iran, CivilicaL, and Noormags and English databases including PubMed, Web of Science, Scopus, ProQuest Dissertations, Embase, CINAHL, ERIC, and Google Scholar were searched using relevant keywords, such as evaluation, program evaluations, outcome and process assessment, educational assessment, and educational measurements. The search was done with no time limits and 41 papers were obtained until May 22, 2020. This systematic review was performed by following the data extraction steps and assessing the quality of the studies and findings. Critical Appraisal Skills Programs and Mixed-Methods Appraisal Tool checklists were used to check the quality of the papers.
This systematic review was conducted on 41 studies, 40 of which were research papers and one was a review paper. From the perspective of the CIPP model of evaluation, most papers showed quite a good level of evaluation of educational programs although some studies reported poor levels of evaluation. Moreover, factors such as modern teaching methods, faculty members, financial credits, educational content, facilities and equipment, managerial and supervisory process, graduates’ skills, produced knowledge, and teaching and learning activities were reported as the factors that could influence the evaluation of educational programs.
CONCLUSION:
Due to the important role of evaluation in improvement of the quality of educational programs, policymakers in education should pay special attention to the evaluation of educational programs and removal of their barriers and problems. To promote the quality of educational programs, policymakers and officials are recommended to make use of the CIPP model of evaluation as a systemic approach that can be used to evaluate all stages of an educational program from development to implementation.
Introduction
Today, improving the quality of higher education is the most important and fundamental tool for the sustainable and comprehensive growth and development of a country.[ 1 ] The system of higher education is effective and useful when its activities are implemented based on appropriate and acceptable standards, and achieving such a quality in the higher education entails using appropriate research and evaluation.[ 1 ] Because the quality of an educational program is a multidimensional and complex concept, it is very difficult to judge a program. Hence, evaluation as a means of judging and documenting quality is of paramount importance.[ 2 ] Evaluation also makes it possible to assess the development and implementation of programs as well as the achievement of educational goals and aspirations. By evaluating an educational program, it is possible to understand the degree of compatibility and harmony of that program with the needs of individuals and the target community and to determine the effective factors in the development of the program.[ 3 ] Principled evaluation, while ameliorating the strengths and minimizing the weaknesses, can be the foundation for many educational decisions and plans and can provide the required tools for improving universities’ academic levels.[ 4 ] Evaluation makes education transform from a static state to a dynamic one. One of the most important factors influencing effective evaluation is certainly the existence of an effective tool and model that can properly evaluate educational programs.[ 5 ] There are several ways to evaluate educational programs. One of these models is the CIPP evaluation model, which is the acronym of Context, Input, Process, and Product and evaluates educational programs in these four areas.[ 6 ] Evaluation of the context aims to provide a logical ground for setting educational goals. It also attempts to identify problems, needs, and opportunities in a context or educational situation. The purpose of input evaluation is to facilitate the implementation of the program designed in the context stage. In addition, it focuses on human and financial resources, policies, educational strategies, barriers, and limitations of the education system. Process evaluation refers to identification or prediction of performance problems during educational activities and determining the desirability of the implementation process. In the process stage, the implementation of the program and the effect of the educational program on learners are discussed. Output evaluation is done in order to judge the appropriateness and efficiency of educational activities. In fact, the results of the program are compared to the goals of the program, and the match between the expectations and the actual results is determined.[ 7 ] The most important goal of evaluation based on the CIPP model is to improve the performance of the program. Stufflebeam and Zhang referred to the CIPP evaluation model as a cyclical process that focuses more on the process than on the product, and the most important goal of the evaluation, he maintained, is to improve the curriculum or the educational program.[ 8 ] In addition, studies have indicated that the CIPP evaluation model covers all stages of revising an educational program, which is consistent with the complex nature of medical education programs. This model provides constructive information required to improve educational programs and to make informed decisions.[ 8 ] The CIPP model does not only emphasize answering clear questions, but it also focuses on the general and systematic determination of the competencies of an educational program.
To the best knowledge of the researchers, most studies in medical sciences have been done to prove the achievement of predetermined goals in an educational program, while the CIPP model aims to help improve the quality of an educational program rather than documenting the achievement of goals.[ 9 ] This research policy of the CIPP model and the necessity to examine the researchers’ approach toward using it in the evaluation of educational programs prompted the researchers to use a systematic review to study the scope and manner of research on the application of the CIPP evaluation model in medical sciences.
Materials and Methods
In this systematic review, 14 international and national databases were systematically searched from April 22, 2020, to May 22, 2020. The research population included all domestic and foreign papers that used the CIPP evaluation model to evaluate educational programs in medical sciences. Because the number of papers in this domain was limited, the search was not limited temporally. All steps of evaluating the papers for inclusion in the study were done separately by two independent researchers. In case of discrepancy between the two researchers, a third expert was asked to evaluate the papers and the final decision was made based on the agreement among the three evaluators.
Search strategy
Searching for the papers was done with a specific strategy with no time limit from April 22, 2020, to May 22, 2020. The search was carried out in Persian databases including SID, Mag Iran, CivilicaL, Iran Medical Articles Bank, Noormags, and ISC and English databases including Scopus, PubMed, Web of Science, ProQuest Dissertations, Embase, CINAHL, and ERIC. Google Scholar search engine was used in both English and Persian. The search was separately performed in each database based on the relevant keywords. An example of the search method in the PubMed database is given in Table 1 .
PubMed search query
A multistage approach was adopted in the selection of studies. To achieve the relevant studies, initially, a wide range of keywords listed in the MeSH, such as evaluation, program evaluations, outcome and process assessment, educational assessment, and educational measurements, were searched. In order to increase the likelihood of finding relevant studies, the terms “medical” and “education” were searched both as separate words and as a combination. It should be noted that there was no other equivalent for the CIPP model in the list of MeSH terms. The studies were reviewed and selected in three stages. In the first step, citation information and abstracts of the papers extracted from the databases were transferred to Endnote. Then, the titles of the selected papers were reviewed and the papers that were repetitive or irrelevant to the main topic of the research were deleted. In the second step, reading the abstracts of the remaining papers, those related to the main purpose of the research were selected. In the third step, the full texts of the papers were analyzed based on the inclusion and exclusion criteria [ Table 2 ].
Inclusion and exclusion criteria for the studies
CIPP=Context, Input, Process, and Product
Finally, 41 studies that were in line with the purpose of the study, were written in English or Persian, and had full texts available to the researchers were selected and qualitatively analyzed [ Figure 1 ].

The process of selection of final articles
Data extraction and synthesis
For the selected papers, two researchers extracted the relevant information independently using a standard data-mining form.
They discussed any mismatches in data mining, which was followed by a complementary analysis done by a third researcher to ensure the precision of the extracted information. This form included the following specifications: first author's name, year, geographical area, research design, and objectives. After completing this form, the results obtained from the analysis of the papers were summarized and reported.
Quality assessment
Critical Appraisal Skills Programs (CASP) checklist, which is a standard tool for evaluating the quality of papers, was used to check the quality of the papers.[ 10 ] The checklist used in the present study included 18 items and each item was given a score of 1 (indicating that the item was noticed in the paper) or 0 (indicating that the item was ignored in the paper). These items were divided into four areas: participant characteristics (five items), attitude assessment tools (three items), study design (five items), and results (five items). The total score of this checklist could range from 0 to 18.[ 11 ] After a thorough study of the full text of each article, the checklist of paper quality was completed by the first researcher and the items were scored. The second researcher followed the same procedure in the re-evaluation process of each paper. In case of disagreement in scoring the items, a final score was obtained in a joint session. Next, based on the scores obtained from this checklist, the reviewed papers were divided into three categories of good, moderate, and poor quality. The cutoff point was determined based on that reported in similar papers and experts’ judgments. Accordingly, the total scores of 75% and above were classified as good quality (scores 13 and above), total scores between 25% and 75% were classified as moderate quality (scores 6–12), and total scores lower than 25% (scores 5 and below) were classified as poor quality.[ 11 ] In order to assess the quality of mixed-methods papers, Mixed-Methods Appraisal Tool (MMAT) was used in this study.[ 12 , 13 ] Four areas of the qualitative criteria used in the MMAT are as follows: (1) eligibility of participants and appropriateness of sampling procedure; (2) data analysis process including data collection procedure, data format, and data analysis; (3) attention to the effect of setting on data collection; and (4) attention to the impact of the researchers’ ontological and epistemological beliefs. The critical appraisal of mixed-methods also included three areas, namely relevance of mixed-methods design, synthesis of data, and attention to methodology limitations. Each study was given an overall quality score (unclassified, 25%, 50%, 75%, or 100%) based on the MMAT scoring system.[ 12 , 13 ]
In the first step, the titles of the 1275 papers obtained in the initial search of the studies were examined, and duplicate titles were deleted either using Endnote or manually. At this stage, 836 papers with duplicate titles were deleted and 439 papers remained. In the second step, the abstracts were studied by the researcher and an expert colleague. As a result, 395 papers unrelated to the main research topic were removed and 44 papers related to the main objective of the project were selected. In the third step, after reading the full texts of the 44 papers, three studies were deleted and 41 using the CIPP model in medical sciences were selected [ Figure 1 ].
The results showed that the quantitative methodology was used slightly more by researchers compared to other methods [ Table 3 ].
Types of studies
All studies aimed at examining the attitudes of students, instructors, and those involved in the quality of educational programs based on the CIPP evaluation model. In addition, most studies examined students’ perspectives on educational programs. A large number of papers ( n = 29) were descriptive, cross-sectional studies and evaluated educational programs using researcher-made questionnaires. In addition, nine studies used a mixed-methods design where the authors used questionnaires and individual interviews to examine the participants’ attitudes. In two studies, qualitative methodology and individual interviews were used to evaluate educational programs. Finally, one study included a review of other papers that had used the CIPP model [ Table 3 ]. Most studies ( n = 29) on the evaluation of curricula based on the CIPP model were conducted in Iran [ Table 4 ].
The summary of the studies’ results
HSM=Health Services Management, CIPP=Context, Input, Process, and Product, FPDP= Faculty Professional Development Program, BL= Blended Learning program, INNCT= Indonesian National Nursing Competency Test, MSc= Master of Science
Examining the quality of studies based on the indicators of CASP showed that 23 studies had good quality, 13 ones had moderate quality, and only five studies had poor quality. The results of the quality assessment of the studies are displayed in Table 4 . Moreover, most studies were performed on the assessment of the nursing curriculum based on the CIPP model, while the lowest number of studies was conducted on medical records [ Table 5 ].
Frequency distribution of context, input, process, and product-based evaluation of educational programs in medical sciences
This systematic review examined the scope of research conducted in medical sciences based on the CIPP model. The CIPP model evaluates the context, input, process, and output of educational programs and curricula using a systematic approach and by identifying their weaknesses and strengths, it can help policymakers at the macro level to plan expert actions and decide whether to continue, stop, or revise the educational program, ultimately promoting the satisfaction with the implementation of the program. Various factors can influence the satisfaction with educational programs.[ 55 ] Factors, such as experienced professors, suitable facilities and equipment, educational and research budgets, appropriate educational content, and proper educational environment, which are measured in the CIPP model, can affect the satisfaction with educational programs. Although most studies have evaluated the satisfaction with educational programs as relatively high,[ 18 , 19 , 22 , 26 , 36 , 38 , 47 , 51 ] some other studies have reported moderate or low satisfaction levels.[ 33 , 45 , 46 , 48 , 54 ]
Due to the nature of the CIPP model, educational programs are evaluated in four areas (context, input, process, and output). Context evaluation involves identifying the relevant elements in the educational environment as well as identifying problems, needs, and opportunities in a context or educational situation. Through this evaluation, it is possible to judge the appropriateness of predetermined goals. In context evaluation, factors such as needs, facilities, and problems are examined in a specific and defined environment. At this stage, the education system is evaluated in terms of goals and the target population.[ 7 ] Context has been evaluated in different studies. For instance, Okhovati et al . evaluated the curriculum of health services management in Kerman University of Medical Sciences. Evaluation of context showed that the mean score obtained in the domain of goals had a poor situation, whereas the mean score obtained in providing scientific and specialized services indicated that the situation was relatively satisfactory. The overall mean score of context evaluation of the curriculum was reported as relatively high.[ 37 ] Consistently, Akhlaghi et al . evaluated the Master's curriculum in medical records at Iran University of Medical Sciences and revealed that the context was relatively desirable.[ 36 ] Yazdani and Moradi also reported a desirable evaluation of the context of the undergraduate nursing curriculum at Ahvaz University.[ 38 ] In the same line, Mohebbi and Yarmohammadian studied the undergraduate curriculum of medical records and found that the context was satisfactory.[ 51 ] In another study by Kool et al ., the context of the gynecology curriculum was desirable in achieving the goals.[ 18 ] The results of the study by AbdiShahshahani et al . also showed that the context of the Iranian doctoral curriculum in reproductive health was desirable.[ 17 ] However, the results of a study conducted by Lee on a Humanities Course in College of Medicine showed that there were problems with the context of the curriculum. Although the educational goals were clearly stated in the curriculum, the results of content analysis indicated that the goals of the curriculum were not clear and that the students demanded the goals of the curriculum to be clearly stated.[ 21 ] Moreover, the results of another study performed by Niazi on the selected faculties of Tehran University of Medical Sciences demonstrated that the context was not desirable and that the students believed that they were not adequately informed about the goals and policies of the department[ 33 ] In general, problems related to the contexts of curricula can be due to the lack of periodic review of program goals, incompatibility of goals with the job needs of the target population, incomprehensive goals, vague goals, expectations, capabilities that students must learn, and different structures of educational environments.
In the input dimension, the use of the resources and strategies to achieve the goals of an educational program or system is evaluated. Input includes all individuals and human resources, including students, professors, principals, financial resources, and scientific resources that are connected to an educational program. At this stage of evaluation, the required information is collected on how the resources are used to achieve the goals of the educational program.[ 7 ] The main purpose of input evaluation is to help develop a program that can bring about educational changes to achieve the goals set in the context evaluation stage so that the consequences and outputs of the educational system have high utility and value.[ 7 ] The study by Okhovati et al . showed that there were major weaknesses in the input dimension of the curriculum. It seemed that the management curriculum was not up to date and needed to be reviewed and revised. The facilities and equipment were not satisfactory, as well.[ 37 ] In Yazdani and Moradi's study, the evaluation of input showed that educational resources were available, but theoretical and practical courses were not proportionate, nor were educational facilities and equipment appropriate.[ 38 ]
In Mohebbi and Yarmohammadian's study, input evaluation showed that the educational budget and financial resources were not satisfactory.[ 51 ] Similarly, Alimohammadi et al . evaluated the School of Medicine at Rafsanjan University of Medical Sciences and reported that input, students’ abilities, educational content, facilities, and equipment were not desirable.[ 44 ] Input evaluation of Master's program of the neonatal intensive care was also reported to be unsatisfactory by Hemati et al .[ 45 ] Furthermore, Phattharayuttawat aimed at evaluating the curriculum of the master of clinical psychology and indicated that educational resources were available for learning and teaching and were quite appropriate. Although the input was appropriate in terms of students, professors, and educational content, some educational resources, such as clinical wards and availability of patients, were not adequate.[ 22 ]
Nagata et al . studied the nursing doctoral curriculum in Japan and found that in terms of input, the number of professors, facilities, and equipment such as the library and computer systems was not appropriate.[ 34 ] So young Lee stated that in order to improve the input of the curricula, their educational contents had to be improved.[ 35 ]
Process focuses on the way the program is implemented and determines the effect of the educational program on learners. Process evaluation involves evaluation of teaching–learning activities as well as instructors’ behaviors, knowledge, and experiences and examines the management and supervision procedures. In other words, process refers to all activities that take place during the implementation of the program. It also provides an opportunity to simultaneously apply the results of the two previous stages of evaluation to improve the implementation of the educational program.[ 7 ]
Output evaluates and determines the effects of the educational program on graduates, compares the results of the educational program to the goals of the program, and determines the relationship between expectations and actual results. Output refers to all graduates, newly produced knowledge, and achievements of the program. This type of evaluation is performed to judge the desirability of the effectiveness of educational activities.[ 7 ] In a study carried out by Tazakkori based on the CIPP model, it was found that the Iranian nursing doctoral program was devoid of basic defects and flaws in terms of history, philosophy, mission, vision, and aims. In addition, course specifications and contents were in accordance with the philosophy and goals of the program. However, the evaluation results showed that there were major problems in the process and implementation of the program, and that the output was affected by the poor implementation of the process.[ 46 ]
Ehsanpour conducted a research in the School of Nursing and Midwifery of Isfahan University of Medical Sciences in order to evaluate undergraduate midwifery students’ achievement of the minimum requirements of midwifery learning. Based on the results, the students did not have enough experience in rare cases in clinical education.[ 15 ] Pakdaman et al . also examined the achievement of educational goals of periodontics and oral health programs at the University of Tehran based on the CIPP model. They concluded that students were more satisfied with the content, but believed that instructors were not sufficiently motivated and skilled. Overall, the students were not very satisfied with the process and assessed the output of some courses as poor.[ 40 ] Okhovati's et al . study showed that the process was relatively satisfactory in terms of students’ activities, teaching–learning activities, and research activities. However, evaluation of the input of the curriculum showed that the graduates’ specialized skills were not satisfactory.[ 37 ] On the contrary to the results of the abovementioned studies, the findings of the study by Phattharayuttawat et al . showed that in terms of context, the goals of the curriculum were clearly stated and matched social needs. The structure of the curriculum was also well designed. In addition, input evaluation showed that educational resources were available for learning and teaching, but they were not quite adequate. The results also showed that the process and educational performance were very good and the evaluation of the output showed that the graduates had achieved the general and specialized competencies stated in the goals of the program.[ 22 ]
Based on the comprehensive and systematic CIPP model, it is expected that all elements of the education system be consistently interconnected, as it is assumed that education is an ongoing process and the educational system is designed based on these processes. However, the findings of the present study showed that such an interconnection has not been fully established between the components of the educational system in different studies, and there have been discrepancies in some cases. The results of some studies also showed that students did not achieve the intended educational goals. Therefore, revision of educational programs and systems and provision of guidelines were found to be necessary.[ 15 , 22 , 35 , 37 , 40 , 46 ]
What was very noteworthy in the present study was that many studies tended to adopt a quantitative approach to the evaluation of educational programs. However, in order to conduct a comprehensive evaluation, both quantitative and qualitative data must be analyzed. A careful and comprehensive examination of the methods and results of numerous domestic and international evaluation studies, especially those conducted in medical sciences education, demonstrated that most of these studies focused on answering explicit and clear questions rather than on viewing and measuring the overall value and competence of an educational program. While such studies have often been conducted to find the success or failure of educational programs in achieving predetermined goals, the most important goal of CIPP evaluation is to improve the quality of the program and not to prove its quality.[ 9 ] Although the underlying assumption of the CIPP model is that evaluation is a prognostic phenomenon and is done gradually along with the development of a program,[ 56 ] most published papers have sufficed to conduct a cross-sectional study using a questionnaire including the four components of the CIPP model. Therefore, using questionnaires with items on the context, input, process, and output does not necessarily mean using the CIPP evaluation model.[ 9 ] Studies by Makarem et al ., Pakdaman et al ., Hemati et al ., and others have all examined some aspects or views of some program beneficiaries based on a quantitative approach through using questionnaires and are consequently subject to the same criticism because they have adopted a goal-oriented approach and have evaluated the achievement of the final results,[ 40 , 43 , 45 ] while the systematic evaluation process should formatively evaluate all aspects of the program according to the views of all stakeholders and parties involved in the educational program and the results of each stage should be used simultaneously to enhance the program.[ 9 ] In terms of study participants, most studies have evaluated educational programs from the viewpoint of a particular group and have failed to take qualitative approaches and viewpoints of different parties into account. Evaluating educational programs from the perspective of different people involved in the program can help discover different aspects of the program or the weaknesses that have been less addressed. Paying attention to the views of other people involved in the educational program in different societies according to the cultural conditions prevailing in that society can help reform and revise the educational programs, as well. In this way, using a holistic approach to the educational program makes it possible to provide a framework for interventions that can be implemented in educational programs.
Limitations
One of the limitations of this systematic review was the potential for incomplete retrieval of studies due to the restriction of the search to the articles published in English.
This was the first systematic review examining the CIPP model of evaluation in medical education.
The results of this review study emphasized the need for formative evaluation through a systematic CIPP model with a holistic approach during the implementation of educational programs. Using the quantitative and qualitative results of such studies, various aspects of educational programs should be revised to improve their competencies. Until now, various previous studies have been investigated with a focus on the CIPP evaluation model from a practical perspective. These results showed that evaluations using the CIPP model, which could be considered rather difficult, could provide the basis for education improvement. Specifically, omission of evaluation of the unset parts becomes more vulnerable for quantitative evaluations. These materials can contribute to obtaining a diverse range of opinions that cannot be explained by quantitative materials. Furthermore, rather than utilizing a single group such as students as the evaluation material collection source, having a balanced perspective of various interested parties regarding education can improve the reliability and validity of an evaluation, which can then be utilized as a convincing database.
Financial support and sponsorship
This study was financially supported by Tehran University of Medical Sciences.
Conflicts of interest
There are no conflicts of interest.
Acknowledgment
This paper was extracted from a PhD dissertation in Reproductive Health (IR.TUMS.FNM.REC.1398.057) approved by Tehran University of Medical Sciences. The authors would like to thank Ms. A. Keivanshekouh at the Research Improvement Center of Shiraz University of Medical Sciences for improving the use of English in the manuscript.
Lean Six Sigma Training Certification

- Facebook Instagram Twitter LinkedIn YouTube
- (877) 497-4462
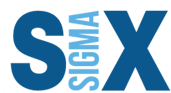
How to Use Input Process Output Model For Business Success
March 18th, 2024
Teams are the backbone of modern organizations, driving innovation, problem-solving, and organizational success.
However, building and sustaining high-performing teams is a complex endeavor that requires a deep understanding of the underlying dynamics and processes.
This is where the Input-Process-Output (IPO) model comes into play, providing a framework for analyzing and optimizing team effectiveness .
Key Highlights
- The Input Process Output Model (IPO model) is a fundamental framework for understanding and analyzing group dynamics and team effectiveness.
- It provides a systematic approach to examining the factors that influence group performance, including inputs, processes, and outputs.
- Input factors include team composition, resources, and environmental conditions that shape group interactions.
- Group processes refer to the interactions, communication patterns, and decision-making approaches within the team.
- Outputs are the tangible and intangible results of group efforts, such as productivity, quality, and satisfaction.
- Understanding the IPO model can help organizations optimize team performance, foster collaboration, and achieve desired outcomes.
More About Input Process Output Model
The Input-Process-Output (IPO) model provides a comprehensive framework for analyzing and optimizing team effectiveness.
Developed by researchers in the field of organizational behavior, this model offers a systematic approach to examining the factors that influence group performance.
From the initial inputs that shape team interactions to the processes that unfold within the group, ultimately leading to specific outputs or outcomes.
By breaking down the complexities of group dynamics into these three distinct components, the IPO model empowers organizations to identify areas for improvement , implement targeted interventions, and foster an environment conducive to high-performing teams.
Whether you’re a team leader, a project manager, or a member of a collaborative group, understanding the IPO model can unlock valuable insights and strategies for maximizing team potential and achieving desired results.
What is the Input Process Output Model (IPO Model)?
The Input Process Output Model (IPO model) is a widely used framework for understanding and analyzing team effectiveness and group performance.
Developed by organizational psychologists, the IPO model provides a structured approach to examining the various factors that influence how teams function and achieve their goals.
Definition and Overview of the Input Process Output Model
The IPO model proposes that team effectiveness is a result of the interplay between three key components:
Inputs refer to the individual characteristics, group-level factors, and environmental conditions that exist before the team begins its work,
Processes encompass the interactions, behaviors, and dynamics that occur within the team as it undertakes its tasks.
Outputs represent the results or outcomes achieved by the team, such as productivity, quality, and member satisfaction.
Importance of the IPO Model in Understanding Team Effectiveness
The IPO model is valuable for several reasons. First, it offers a comprehensive framework for analyzing team performance by considering a wide range of factors that can impact team functioning.
By examining inputs, processes, and outputs, researchers and practitioners can identify strengths, weaknesses, and areas for improvement within teams.
Second, the IPO model highlights the interdependence between inputs, processes, and outputs.
It recognizes that team effectiveness is not solely determined by any single factor but rather by the complex interplay among various elements.
For example, a team with highly skilled members (input) may still struggle if they lack effective communication processes or face environmental constraints.
Furthermore, the IPO model emphasizes the importance of team processes, which are often overlooked or underestimated.
It underscores that team interactions, such as communication, coordination, and conflict management , are crucial in shaping team outcomes.
By focusing on processes, the model provides insights into how teams can optimize their functioning and improve their performance.
Overall, the Input Process Output model offers a systematic and holistic approach to understanding team effectiveness, making it a valuable tool for researchers, managers, and team leaders.
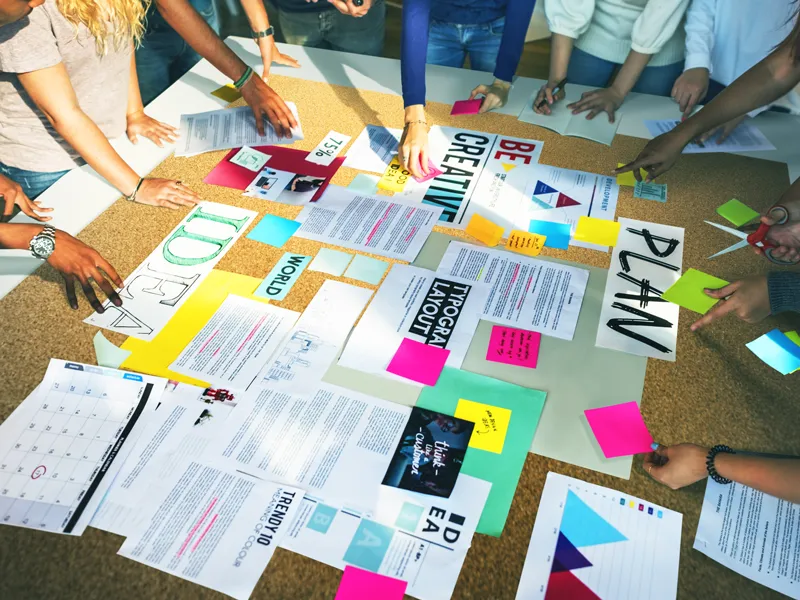
Inputs in the Input Process Output Model
The input stage of the Input Process Output model refers to the various factors that influence a team’s functioning and performance.
These inputs can be categorized into three levels: individual, group, and environmental.
Individual-level input factors encompass the unique characteristics, skills, and experiences that each team member brings to the table.
These include:
- Skills: The knowledge, abilities, and competencies that individuals possess, such as technical expertise, problem-solving skills, communication skills, and creativity.
- Personalities: Individual traits, values, and behavioral tendencies that shape how team members interact and contribute to the group dynamic.
- Experiences: Prior experiences, backgrounds, and perspectives that shape an individual’s approach to teamwork and problem-solving.
Group-level input factors refer to the characteristics and dynamics of the team itself.
- Group size: The number of members in a team can significantly impact group processes, communication, and coordination.
- Team norms: The shared expectations, values, and standards that govern a team’s behavior, decision-making processes, and interactions.
- Work structure: The way tasks are divided, roles are assigned, and responsibilities are distributed among team members.
Environmental input factors are external factors that can influence a team’s functioning and performance.
- Organizational culture: The values, beliefs, and practices that shape the overall work environment and expectations within an organization .
- Reward systems: The incentives, recognition, and compensation structures in place that can motivate or demotivate team members.
- Physical environment: The physical workspace, tools, and resources available to the team, can impact productivity and collaboration.
By understanding and optimizing these input factors, teams can increase their chances of success by ensuring they have the right mix of skills, personalities, and resources to tackle the tasks at hand effectively.
Effective team composition, clear norms, and a supportive organizational environment can lay the foundation for productive team processes and desirable outputs.
Processes in the Input Process Output Model
The processes in the IPO model refer to the interactions, mechanisms, and dynamics that occur within a team as they work towards their goals.
These group processes act as the critical link between the inputs (individual characteristics, team composition, resources, etc.) and the eventual outputs or outcomes.
Team Interactions and Group Processes
At the heart of team processes are the interactions and exchanges that take place among team members.
This includes critical processes like communication, coordination, conflict management, and decision-making.
Effective communication ensures that information, ideas, and feedback flow smoothly within the team.
Coordination involves orchestrating the sequence and integration of team activities. Conflict management refers to strategies for resolving disagreements and tensions productively.
Group decision-making encompasses the methods and procedures teams use to analyze problems, evaluate alternatives, and reach consensus.
Other key group processes include:
- Team motivation and effort norms
- Emotional support and interpersonal cohesion
- Performance monitoring and feedback loops
- Problem-solving approaches and creativity
- Task assignment and role negotiation
The quality and patterns of these group processes can significantly impact team effectiveness and productivity.
Challenges in Measuring Group Processes
While the importance of group processes is widely acknowledged, measuring and quantifying them presents challenges.
Many group processes are inherently dynamic, fluid, and context-dependent, making them difficult to capture with static measures.
Processes like communication and conflict resolution often involve subtle cues, tones, and unspoken behaviors that are hard to assess objectively.
Researchers have employed techniques like direct observation, video coding, self-report surveys , and social network analysis to study group processes.
However, these methods have their limitations and can be time-consuming, subjective, or disruptive to the team’s natural functioning.
Dynamic Nature of Team Processes Over Time
Team processes are not static; they evolve and change as the team progresses through different phases of development and task cycles.
The patterns of interaction, the intensity of certain processes, and the team’s foci can shift substantially from the initial formation stage to periods of high task execution and ultimately to project completion.
For instance, in the early stages, teams may emphasize processes like getting to know each other, establishing norms, and clarifying roles.
During midpoint task work, the emphasis may shift to coordination, motivation, and monitoring. As deadlines approach, decision-making, and conflict-resolution processes may become more pronounced.
The dynamic nature of team processes poses challenges for managers and researchers alike.
It requires ongoing assessment, adjustment of interventions, and a recognition that different processes may need to be prioritized at various points in the team’s journey.
Capturing these temporal dynamics is crucial for a comprehensive understanding of how inputs get transformed into outputs through team processes.
Outputs in the Input Process Output Model
The outputs in the IPO model refer to the results or outcomes that emerge from the team processes.
These outputs can be evaluated at the team and individual member levels.
Team Performance Outcomes
One of the primary outputs of interest is the team’s performance, which can be measured in terms of productivity, quality, and efficiency.
Productivity refers to the quantity of work accomplished or outputs generated by the team within a given timeframe.
Quality, on the other hand, focuses on the excellence and accuracy of the team’s deliverables, ensuring they meet or exceed established standards.
Efficiency considers the ratio of inputs (resources, time, effort) to outputs, aiming to maximize productivity while minimizing waste .
Individual Member Reactions
In addition to team-level outcomes, the IPO model also considers individual member reactions as important outputs.
These include job satisfaction, which reflects how content and fulfilled team members feel about their roles and experiences within the team.
Team viability refers to the likelihood that the team will continue to work together effectively in the future, based on factors such as cohesion, commitment, and perceived success.
Personal growth represents the extent to which individual team members have developed new skills, knowledge, or abilities through their participation in the team.
Steiner’s Formula: Actual Productivity vs. Potential Productivity
One way to evaluate team performance is to compare the team’s actual productivity to its potential productivity, as described by Steiner’s formula.
Steiner’s formula suggests that a team’s actual productivity is equal to its potential productivity minus the losses due to faulty processes.
These process losses can stem from various factors, such as poor coordination, communication breakdowns, motivation issues, or interpersonal conflicts within the team.
By understanding the gap between actual and potential productivity, teams can identify areas for improvement in their processes and inputs to minimize process losses and enhance overall team effectiveness.
The IPO model provides a framework for analyzing and addressing these discrepancies, ultimately leading to better team performance and individual member outcomes.
Limitations and Extensions of the IPO Model
While the Input Process Output Model provides a useful framework for understanding group dynamics and team effectiveness, it is important to recognize its limitations.
One key assumption of the IPO model is its linearity and static nature. The model presents a simplified, linear sequence where inputs lead to processes, which then lead to outputs.
However, in reality, group interactions are often more complex, dynamic, and cyclical.
The IPO model does not fully account for the feedback loops and reciprocal relationships that exist between inputs, processes, and outputs.
For example, the outputs achieved by a team can influence the motivation levels (an input factor) and group processes in subsequent tasks or projects.
Additionally, the model assumes a relatively static set of inputs and processes, whereas, in practice, these elements can change and evolve as the team progresses through different stages of development.
To address these limitations, researchers have proposed extensions and alternative models that incorporate feedback loops and dynamic change.
One such extension is the Input-Mediator-Output-Input (IMOI) model , which recognizes the cyclical nature of team interactions by including an additional feedback loop from outputs back to inputs.
This model acknowledges that the outcomes achieved by a team can shape future inputs, such as team composition, resources, or environmental factors.
Another alternative is the direct input-output links model, which suggests that certain input factors can directly influence outputs without necessarily going through group processes.
For example, the expertise or experience levels of team members (input factors) may have a direct impact on the quality of the team’s output, regardless of the specific group processes involved.
These extensions and alternative models highlight the importance of considering the dynamic and non-linear nature of team interactions.
While the IPO model provides a useful starting point, it is essential to recognize its simplifications and limitations.
By incorporating feedback loops, dynamic change , and direct input-output links, researchers and practitioners can develop a more comprehensive understanding of group dynamics and team effectiveness.
Applications and Best Practices
The input-process-output (IPO) model provides a valuable framework for understanding and optimizing team effectiveness.
By breaking down team dynamics into distinct components, the model offers practical applications for team building and performance improvement efforts.
Using the IPO Model for Team Building and Performance Improvement
One of the primary applications of the IPO model is in the realm of team building and development initiatives.
The model can serve as a diagnostic tool to identify potential areas of improvement within a team.
For instance, if a team is consistently underperforming (output), the IPO model can help pinpoint whether the root cause lies in the input factors (e.g., lack of necessary skills or resources) or the team processes (e.g., poor communication or coordination).
Once the areas for improvement have been identified, targeted interventions can be designed and implemented.
These interventions may include training programs to enhance individual skills (input optimization), facilitated workshops to improve group processes ( process optimization ), or structural changes to the team’s composition or environment (input reconfiguration).
Strategies for Optimizing Inputs, Processes, and Outputs
The IPO model provides a structured approach to optimizing team effectiveness by addressing each component systematically:
Input Optimization
- Conduct thorough job analyses and person-job fit assessments during team member selection.
- Provide training and development opportunities to enhance individual skills and competencies.
- Ensure that team members have access to necessary resources and information.
- Align team composition with task requirements (e.g., diversity, size, expertise).
Process Optimization
- Implement team-building activities to foster cohesion, trust, and shared norms.
- Establish clear communication channels and protocols for effective information sharing.
- Encourage constructive conflict resolution and decision-making processes.
- Promote accountability and feedback mechanisms for continuous improvement .
Output Monitoring and Adjustment
- Establish clear performance metrics and regularly measure team outputs.
- Identify and address process losses that hinder team productivity.
- Celebrate and reinforce positive team behaviors and outcomes.
- Adapt and reconfigure inputs or processes as needed based on feedback and changing circumstances.
Case Studies
The IPO model has been successfully applied across various organizational settings.
Software Development Teams
Agile methodologies like Scrum heavily rely on the IPO model principles.
Input factors like cross-functional team composition and co-location are emphasized, while iterative processes like daily stand-ups and retrospectives facilitate continuous improvement.
Healthcare Teams
Interprofessional healthcare teams often face challenges in coordinating their diverse expertise and backgrounds (inputs).
Interventions focused on improving team processes, such as structured communication protocols (e.g., SBAR) and shared decision-making, have been shown to enhance patient outcomes (outputs).
Virtual Teams
With the rise of remote work and global teams, optimizing virtual team inputs (e.g., communication tools, cultural awareness) and processes (e.g., establishing team norms, and managing time zone differences) becomes crucial for achieving desired outputs.
Cross-functional Project Teams
Organizations frequently assemble cross-functional teams to tackle complex projects.
Applying the Input Process Output model principles can help manage the diverse inputs (functional expertise, goals) and optimize processes like conflict resolution and decision-making to deliver successful project outcomes.
By leveraging the Input Process Output model’s principles and strategies, organizations can proactively address team dynamics, foster collaboration, and drive sustained high performance.
SixSigma.us offers both Live Virtual classes as well as Online Self-Paced training. Most option includes access to the same great Master Black Belt instructors that teach our World Class in-person sessions. Sign-up today!
Virtual Classroom Training Programs Self-Paced Online Training Programs
SixSigma.us Accreditation & Affiliations
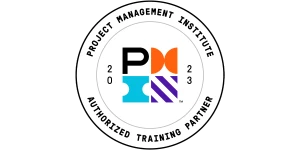
Monthly Management Tips
- Be the first one to receive the latest updates and information from 6Sigma
- Get curated resources from industry-experts
- Gain an edge with complete guides and other exclusive materials
- Become a part of one of the largest Six Sigma community
- Unlock your path to become a Six Sigma professional
" * " indicates required fields
Marketing Research Through the Input-Output Approach in Developing Countries
- Conference paper
- Cite this conference paper
- Dr. W. Brauers 4
Part of the book series: Developments in Marketing Science: Proceedings of the Academy of Marketing Science ((DMSPAMS))
1691 Accesses
Information furnished by input-output may appear to have limited use for the market researcher. Economic information on developing countries however is already limited. The input-output approach may be helpful for that purpose.
Download to read the full chapter text
Chapter PDF
Similar content being viewed by others.
Input-Output Tables and Marketing Purposes: The Belgian Case
The role of marketing in economic development.
Theory and Methodologies: Input–Output, SCPM and CGE
- Quadratic Programming
- Market Research
- National Economy
- Foreign Firm
- Economic Information
These keywords were added by machine and not by the authors. This process is experimental and the keywords may be updated as the learning algorithm improves.
Author information
Authors and affiliations.
University of Antwerp (RUCA), Logan, USA
Dr. W. Brauers
You can also search for this author in PubMed Google Scholar
Editor information
Editors and affiliations.
Utah State University, Logan, Utah, USA
John C. Rogers III
Texas Christian University, Fort Worth, Texas, USA
Charles W. Lamb, Jr.
Rights and permissions
Reprints and permissions
Copyright information
© 2015 Academy of Marketing Science
About this paper
Cite this paper.
Brauers, D.W. (2015). Marketing Research Through the Input-Output Approach in Developing Countries. In: Rogers III, J., Lamb, Jr., C. (eds) Proceedings of the 1983 Academy of Marketing Science (AMS) Annual Conference. Developments in Marketing Science: Proceedings of the Academy of Marketing Science. Springer, Cham. https://doi.org/10.1007/978-3-319-16937-8_139
Download citation
DOI : https://doi.org/10.1007/978-3-319-16937-8_139
Publisher Name : Springer, Cham
Print ISBN : 978-3-319-16936-1
Online ISBN : 978-3-319-16937-8
eBook Packages : Business and Economics Business and Management (R0)
Share this paper
Anyone you share the following link with will be able to read this content:
Sorry, a shareable link is not currently available for this article.
Provided by the Springer Nature SharedIt content-sharing initiative
- Publish with us
Policies and ethics
- Find a journal
- Track your research
Research to Action
The Global Guide to Research Impact
Social Media
Impact Practitioners
- How to develop input, activity, output, outcome and impact indicators
By Karolina Bohacova 23/03/2023
This 29-page guidance note put together by the Vera Institute of Justice describes when and how to use indicators, what to measure and how to choose data sources to monitor activities, results and progress of your research programme. It was originally intended for the DFID Crime, Conflict & Violence Programming , but it will be useful for any development research programmes. It is a very accessible and useful resource, especially for those starting to learn about indicators, programme staff and those responsible for populating log frames.
In general, indicators help you determine if your projects are meeting their goals, whether there are any areas for improvement and if the programmes are implemented as planned. They are an essential management tool to see if your projects are efficient and provide value for money. The guidance note looks at input, activity, output, outcome and impact indicators:
Input Indicators – What resources are required
Firstly, you will need to develop a set of input indicators that will allow you to monitor the availability of essential resources. Ideally, your input indicators will draw upon existing project management tools such as budget reports, reference letters, CVs and letters of support. Your indicators should alert you early to logistical challenges that might limit your project’s effectiveness.
Activity Indicators – What your project does
Activity indicators will help you see if your project is being delivered as planned and potentially highlight any challenges. They should answer three main questions: who conducted the activity, what they did and where they were working. Ideally, they will also include cost measures so that you can determine the project’s efficiency and economy. When developing the activity indicators, consult key stakeholders who can help you identify which elements are crucial for the project’s success. Try to track activities on an ongoing basis.
Output Indicators – What your project produces
Output indicators describe the delivery of products such as training and new equipment. It is necessary to track output indicators at regular intervals to assess progress, detect delays and understand if they provide value for money. Try to include both measures of the number of outputs (for instance number of tasks achieved or products produced) as well as their quality (for example asking participants if the training was clear and relevant).

Outcome Indicators – What your project achieves
Outcome indicators describe the real-world changes after the production of outputs. They ensure transparency and accountability, demonstrate the return on investment and highlight the benefits that your project delivers. In other words, outcome indicators define the criteria for assessing whether the project is successful. Therefore, they need to be realistic, measurable and achievable given your capacity and resources.
Typically, they are a combination of quantitative and qualitative measures, meaning they tell you the number of people benefitting from your project and the nature of those benefits.
Make sure the indicators are gender-sensitive and pro-poor .
Impact Indicators – How your project contributes to higher-level strategic goals
Impact indicators describe progress made towards higher-level goals, which are shared with other development partners and national agencies (for instance reducing poverty, increasing access to justice or improving the accountability of national institutions). Impact indicators can illustrate the connection between your project and the priorities of governments or development organisations.
Apart from the chapters dedicated to indicators , the guidance note also provides tips for developing a theory of change and choosing the right data sources. It is written in a very accessible way, providing examples from practice, checklists, recommended tools and explanation boxes helping you understand the different aspects of developing and monitoring indicators.
This article is part of our initiative, R2A Impact Practitioners. To find out more, please click here .
Contribute Write a blog post, post a job or event, recommend a resource
Partner with Us Are you an institution looking to increase your impact?
Most Recent Posts
- How can we achieve locally led practice in international development?
- Post-Doctoral Researcher, IDS – Deadline 30 April
- Ten guidelines for an effective research impact assessment
- How is the communication landscape changing?
- How to Drive Impact: Insights from the RDI Network
This Week's Most Read
- What do we mean by ‘impact’?
- How to write actionable policy recommendations
- 12ft Ladder: Making research accessible
- Gap analysis for literature reviews and advancing useful knowledge
- Outcome Mapping: A Basic Introduction
- Stakeholder Engagement a Tool to Measure Public Policy
- Policymaker, policy maker, or policy-maker?
- AEN Evidence 23 – Online Access Registration now open!
- Post-Doctoral Researcher, IDS – Deadline 30 April
Research To Action (R2A) is a learning platform for anyone interested in maximising the impact of research and capturing evidence of impact.
The site publishes practical resources on a range of topics including research uptake, communications, policy influence and monitoring and evaluation. It captures the experiences of practitioners and researchers working on these topics and facilitates conversations between this global community through a range of social media platforms.
R2A is produced by a small editorial team, led by CommsConsult . We welcome suggestions for and contributions to the site.
Subscribe to our newsletter!
Our contributors

Browse all authors
Friends and partners
- Global Development Network (GDN)
- Institute of Development Studies (IDS)
- International Initiative for Impact Evaluation (3ie)
- On Think Tanks
- Politics & Ideas
- Research for Development (R4D)
- Research Impact
Research Korner
Latest research articles on Business,Marketing & Management
A Sample Theoretical Framework : Input-Process-Output Model
Methodology
Methodologies are outlooks on research; they set out an image for what research is and how it should be carried out. Basically, axioms and methods are connected to each other. Methods are tools or techniques of gathering of data, techniques of analysis, and techniques of writing. Since it is a tool, then a scrupulous method can often be used by many different methodologies (both qualitative and quantitative). Therefore, methodologies are at a more abstract (or general) level than are methods. Apparently, www.encyclopedia.com defined ‘methodology’ as a strategy or plan for achieving some goal. In contrast to this, methods are the tactics that can be used to service the goals of the methodology. In essence, methodologies provide the blueprints that prescribe how the tools should be used. Those prescriptions can be traced to the axioms -- beliefs about how research should be conducted.
According to Saunders, Mark; Lewis, Philip & Thornhill, Adrian (2004), all research will possibly involve categorical or numerical data or data that can be use for analysis to help the researcher answer the research questions. In connection to this, Saunders, Mark; Lewis, Philip & Thornhill, Adrian (2004; p.327) defined quantitative as a type of empirical knowledge. Actually, qualitative data are described in expressions of quality. Qualitative is the converse of quantitative, which more precisely describes data in terms of quantity (that is, using 'formal' numerical measurement).
In connection to this, t his chapter will discuss the research design, significance of the study, conceptual framework, participants & the methods course, data sources, historical thinking skills, data collection, data analysis, validation of the data, ethical consideration and summary of the chapter.
The Research Design
In order to come up with the most suitable research approaches and strategies for this study, the research process “onion” is undertaken. This is because conducting a research is like peeling the back layers of an onion—in order to come to the central issue of how to collect the necessary data needed to answer the research questions and objectives, important layers should be first peeled away. With the said process, the researcher was able to create an outline on what measures are most appropriate to be applied in the study.
Saunders et al (2004) said that while it is not unusual for a researcher to first think of his research undertaking by considering whether one should, for instance, administer a questionnaire or conduct interviews, thoughts on this question should belong to the centre of the research ‘onion’. That is, in order to come to the central issue of how to collect the data needed to answer one’s research questions, there are important layers of the onion that need to be peeled away: the first layer raises the question of the research philosophy to adopt, the second considers the subject of research approach that flows from the research philosophy, the third examines the research strategy most applicable, the fourth layer refers to the time horizon a researcher applies to his research, and the fifth layer is the data collection methods to be used.
Figure 1 shows how the researcher conceptualised the research approach to be applied in this study by Saunder, Lewis, and Thornhill (2003), in order to come up with the pertinent data needed to answer the research questions stated in the first chapter, as well as to arrive to the fulfilment of this research undertaking’s objectives.
As shown in Figure 1, the research philosophy that is reflected in this study is positivism. With this research philosophy, a researcher prefers to work with an observable social reality in order to come up with law-like generalisations similar to those produced by the physical and natural scientists (Remenyi et al, 1998), and in this tradition, the researcher becomes an objective analyst, coolly making detached interpretations about those data that have been collected in an apparently value-free manner (Saunders et al, 2004).
Meanwhile, the second layer shows that this study has undertaken a deductive approach. Accordingly, this approach has five sequential stages: deducing a hypothesis; expressing the hypothesis in operational terms; testing this operational hypothesis; examining the specific outcome of the inquiry to either confirm the theory or indicate the need for its modification; and finally, modifying the theory in the light of the findings (if necessary) (Robson, 1993, p. 19).
Significance of the study
In recent years, historians and history educators have concluded that historical literacy requires children in grades four through twelve learn and refine historical thinking skills (National Standards for History in School, 1996; National Council for the Social Studies Standards, 1994). Therefore, young people in high school history classes should become increasingly adept at analyzing and interpreting primary sources such as diaries, old photographs, government documents, and other artifacts from the past. Furthermore, students should demonstrate increased historical literacy. They should be able to compose more refined and coherent narratives of what happened and why. To demonstrate historical literacy, students must also be able to properly cite evidence on which they base their historical narratives and interpretations. The requirement of documenting claims about the past, in part, distinguishes historical literacy from other kinds of literacy skills.
History teachers could help students improve the above skills by helping students comprehend broad historical themes. They could help them to weave historical materials from various historical sources into coherent historical narratives (Fehn & Koeppen, 1998). This could be done because effective history teachers have a deep understanding of how historians investigate and reconstruct the past and understand that historical knowledge is always open to revision and interpretation (Yeager & Davis, 1994; Wineburg, 1991, 2001). These teachers are comfortable with the ambiguity of conflicting evidence and are aware of the sources of bias in historical documents. History teachers who possessed a deep understanding of history and the historiograghic principles are the ones who will become successful teachers of history (National Standards for History in the Schools 1996).
This study joined the lines of research that have investigated the nature of historical thinking skills among professional historians, secondary social studies teachers, elementary school teachers, high school students and elementary school students (Yeager & Wilson, 1997; Fehn & Koeppen 1998; Wunder, 2002; Wineburg, 1991 & 2001.) It explores the historical thinking skills of pre service teachers so far neglected by previous researchers (Wunder, 2002; Seixas, 1998). In order to do so, this study investigated the historical thinking skills exhibited by five would-be history teachers in a, mid western public university in the United States and how they planned their teaching strategies to teach the thinking skills. This study shed some understanding on the similarities and differences between these individuals. This study managed to identify, describe and compare the historical thinking skills of the participants and described and analyzed the instructional strategies that they utilized to teach historical thinking skills.
Theoretical/Conceptual Framework
The theoretical framework that will be used in the study is the Input-Process-Output Model. In the IPO model, a process is viewed as a series of boxes (processing elements) connected by inputs and outputs. Information or material objects flow through a series of tasks or activities based on a set of rules or decision points. (Harris & Taylor, 1997) Flow charts and process diagrams are often used to represent the process. (Harris & Taylor, 1997) What goes in is the input; what causes the change is the process; what comes out is the output. (Armstrong, 2001) Figure 1.1 illustrates the basic IPO model:
The IPO model will provide the general structure and guide for the direction of the study. Substituting the variables of this study on the IPO model, the researcher came up with the following:
Participants and The Methods Course
The researcher enlisted five would be secondary education social studies teachers in a mid western public university in the United States. The participants were going through the social studies methods course when they were enlisted, and they volunteered to take part in this study.
Data Sources
The data sources consisted of five historical documents and the lesson plans that were developed by the participants.
Historical Thinking Skills
To elicit and detect historical thinking skills, the researcher presented the participants with five different categories of historical documents. They depicted an overarching theme of slavery. Those documents were:
a. an etching from the 1700s illustrating a coffle of slaves in Africa marching under guard towards the sea,
b. a painting of the “Amistad” depicting a slave revolt at sea (based upon an actual event),
c. an excerpt from the 1852 Alabama Slave Code,
d. a letter from James Henry Hammond, and
e. a photograph of armed African American in the Union army uniform in front of a barrack.
The documents were selected to simulate a professional historian’s task of weaving together a coherent historical narrative from a mix of historical sources: a painting, an etching, a photograph, a letter, and printed documents. This mix of historical materials offered the participants opportunities to interpret non-written materials as well as written materials. Both type of materials are essential in elucidating historical thinking abilities (Wineburg, 2001 and Levstik & Barton, 2001).
The task given to the participants potentially enabled inter-textual comparisons between historical sources. On the back of each source was printed the author of the source and the date it was produced. If the respondents checked the date and source of the document, they would exhibit their historical heuristic skills which is to evaluate the attribution of the source, assess the author’s stance and its truthfulness by examining the date and source of the documents, relate it to the historical background that represents that period, and assess the truth of the author’s biases and intentions (Wineburg, 1991).
The five historical sources provided the participants the chance to reconstruct a rich portrait of slavery in the United States. Each of the five selected historical sources for this study was chosen for specific reasons:
- Slave coffle (1700). This etching is an indication that slavery was a terrible system of exploitation. Together with the other sources, the etching could suggest an overarching theme of exploitation and oppression that the respondent could narrate in their story. This generalization would cohere with the respondent’s previous understanding about slavery (Fehn, et.al.1997). The etching, through the subtext, also could direct the participant’s attention to the fact that Africans were implicated in the enslavement of other Africans, portraying a complex view of slavery. It shows that slavery happened everywhere and the researcher intends to discern whether the participants will include these notions in their narratives. Furthermore, the researcher wanted to detect if the participants evaluate etching as more or less reliable historical evidence than other types of historical sources, thus displaying their sourcing heuristic skills described above.
- Amistad (1841) . The painting was selected to see whether the participants are aware that slaves rebelled violently against their captors. The painting should motivate the participants to explore the theme of slave resistance and detect whether they can weave the theme of resistance into a more complex history of slavery. The Armistad tested the sourcing heuristic skills of the participants by inviting them to ponder its validity since it was painted more than a hundred years after the actual rebellion (Fehn, et. Al. 1997).
- Alabama Slave Code (1852). The excerpts from the code gave the opportunity to the participants to form diverse possibilities of interpretations and to an overarching theme of a legally sanctioned and rigid system of slave control. The code provided specific evidence portraying restricted lives experienced by the slaves. This document allowed the participants to identify a “subtext” an inference that slaves tended to rebel or flee for their freedom.
- James Hammond’s Letter . James Hammond’s Letter provided the participants the opportunity to analyze the thoughts of a slave owner justifying slavery to an English abolitionist staying in England. The researcher could detect whether the participants provided valid interpretations about the thoughts of the slave owner in the context of the period the letter was written, particularly with regards to the other sources that were presented to them. With hope, the participants will evaluated the letter in terms of its reliability as a historical source (Fehn, et.al. 1997).
- African-American Soldiers (1864) . A photograph of Civil War soldiers will be included to elucidate the theme of resistance in the narrative of the respondents. The researcher wanted to know if the respondents could weave a narration from the photo to include discussions about the slaves’ participation in their own liberation through armed struggle. The researcher also wanted to see if the participants regarded photograph as a reliable historical event.
Lesson Plan . On the other hand, the lesson plan of the participants was chosen to identify the ability of the participants to develop effective lessons for teaching historical thinking skills. Each participant was required to develop a lesson plan using primary sources as part of the course requirement. The teachers’ lesson plans were collected and photocopied as a data source for this study since the purpose of this study is also to describe the instructional strategies that the participants developed. The content of the lesson plans were viewed, analyzed, and coded for characteristics of an effective history lesson.
Furthermore, lesson plans were chosen to analyze effective history teaching strategies because lesson plans revealed information such as teacher activities and how they communicated with the students. Generally an effective lesson plan has an introduction, body, opportunity for questions, and summary. These components are bound together with time cues, media cues and practice (Toney, 1991).
According to Cramer, R. & Schwartz, R., (1989), lesson planning can be divided into three types; content, process, and context. Each type helps to organize classroom activities, and together they establish the ambiance of the learners’ learning process. Content plans focus on information students should know. Instructional strategies designed to introduce or elaborate the content should be included in the plan.
Apparently, process plans help student learn how to perform cognitive skills or procedures. Process skills include procedural knowledge that supports independent learning. Context plans set the larger framework in which content and process lessons occur. Context plan can include decisions about grouping, discipline, and grading. The lesson planner can develop process lesson plans in six steps: a) decide what process would improve student performance, b) help student understand the purpose of the lesson, c) help students connect prior knowledge to process new information, d) break instruction into incremental steps to help students develop their performance theory, e) provide meaningful practice in the process, f) extend the lesson by making applications to other areas (Cramer &Schwartz, 1989). Well structured content plans and process plans will help insure that all students make progress in acquiring the knowledge and skills set by the lesson objectives (Cramer, & Schwartz, 1989).
Data Collection
The Interview
For the face-to-face interview part, open-ended questions will be used to obtain as much information as possible about how the interviewees feel about the research topic. The researcher will interview 5 purposively selected individuals .
The researcher will design a semi-structured interview. Using this type of interview enables the researcher/interviewer probe deeper on the issues of biracial identity development. Unlike structured interviews which are standardised and do not allow the interviewer to deviate from the questions (Saunders, Lewis, and Thornhill, 2004), this type of interview does not limit response of the interviewees.
Open questioning, in addition, will help me explore the topic and produce a fuller account. In this case, interviewees are encouraged to clarify vague statements and to further elaborate on brief comments. The researcher will not also share her own beliefs and opinions so as not to influence the answer of the interviewee. Importantly, the researcher will avoid leading questions and showing personal bias as these may result to interviewee or response bias (Saunders, Lewis, and Thornhill, 2004).
In the face-to-face interview, the distribution and collation methods that will be used to manage the process will ensure anonymity. A cover letter will explain to them what the research is all about and how the researcher intends to regard the survey with high confidentiality. The results from the interview will be given in question and answer format. Moreover, content analysis will be drawn from the interviews to identify the development of biracial identity.
Actually, the best way to understand a respondent’s past experience, thoughts, and attitudes are through interviews and document analysis (Guba & Lincoln, (1981). Data was gathered and recorded through one 30 minute semi-structured interview session with each participant. Semi-structured interviews allow for flexibility, therefore obtaining optimum information regarding a topic from the participant, and they are more likely than other forms of inquiry to provide a complete picture (Guba and Lincoln, 1981). The recorded interviews were transcribed by the researcher and the content was analyzed for the presence of historical thinking skills. The member check procedure was used to validate the accuracy. The participants were given the transcript to confirm the authenticity of its content. The interview began with a warm up phase to familiarize the participants with the exercise. The participants were shown the photograph of a slave with horrendous whipping scars on his back and were asked questions about this photograph stands out to you? What grabs your attention?” These questions elicited from the respondents what they considered the central elements that provide an overarching theme for the documents or sources. Then they were asked, “What does the photograph convey to you about slavery? What was slavery like?” These questions guided the respondents to initiate reconstruction of historical narratives with regard to slavery. Then the participants’ attention was directed towards the reverse side of the photograph that was printed with the photograph’s title, the person who took it and when it was taken. This procedure encouraged them to exhibit their sourcing heuristic capability. The warm up phase usually lasted about five minutes.
After the warm-up session, the participants were presented with the five historical sources mentioned before. The participants were instructed to examine the five sources and were asked the same questions as during the warm-up session. Following that, the participants were asked to tell a narrative that tied all the sources together while emphasizing that there is no right or wrong answers. The participants were encouraged to take notes and make an outline of the narrative. The participants were allowed to refer to their notes while voicing their narratives to the researcher but none of them did. After examining the sources, the participants shared their narratives with the researcher without any interruption.
Then, the participants were asked to identify the most important source for their narrative and the reasons they considered the source importance. Subsequently, the participant’s historical heuristic ability was assessed by asking the following questions: “Which documents are the best ones for writing an accurate history of slavery? Which document is the best source of information? Which is the least reliable source of information?” This phase lasted for about 15 minutes.
Throughout each session, the researcher acted as a naïve listener and accepting anything the participants said and declining to interpret any responses. The researcher from time to time however offered clarification or prodded the participants to elaborate on a comment or observation they had made.
Lesson Plan
The lesson plans that the participants developed were collected over duration of the course and analyzed to detect characteristics of effective history teaching.
Data Analysis
The qualitative method of data analysis utilized in this study consist of making sense of raw data by identifying sign posts that determine the presence of characteristics of historical thinking skills and effective teaching strategies to teach those skills. The content was analyzed in order to make sense out of a phenomenon. In this study the phenomenon was historical thinking skills and effective teaching strategies to teach these skills. The data allowed the researcher to describe historical thinking skills and effective teaching strategies after analyzing interview transcripts and the lesson plans. These characteristics were explicitly analyzed to detect sign posts of historical thinking skills and of effective teaching strategies.
Basically, Mays & Pope, (2000) believes that qualitative research uses explanatory methods in describing the variables wherein the data, situations, or other facts collected will be explained or correlated with other data. According to them qualitative research methods are useful when conducting a study wherein the data are immeasurable, such as feelings, beliefs, thoughts, and others.
Moreover, Yin (1984) stated that qualitative methods is important to management analysis, organisation studies and even to business development since it assist the researchers who desire to understand complex social phenomena. They are appropriate when seeking knowledge about the fundamental characteristics of a phenomenon being studied before theorising about it. This knowledge often surfaces through close contact with subjects of a study, allowing the researcher to understand their points of view about and experiences with the phenomenon.
Researchers even disagree on the definition of "qualitative." For example, some researchers use terms such as naturalistic and descriptive, as well as field, product, and case study. Perhaps the best way to clear up some of the confusion about qualitative research is to examine some its most accepted methodologies and characteristics.
On the other hand, Wolcott (1992) proposes that there are but three general types of data-gathering techniques in qualitative studies: experiencing, enquiring, and examining. These three techniques are used, Wolcott argues, in such diverse qualitative approaches as case studies, non-participant observed studies, interviews, participant observation, phenomenology, ethnomethodology, enography, and ethnology. As Wolcott (1992) notes, most qualitative research is based on a case study that uses one or several of these qualitative techniques, enabling researchers to immerse themselves within a culture or a context and producing questions to pursue for further research and understanding of phenomena.
As an extension of the qualitative technique of interviewing, Byers and Wolcott, H. F. (1992) proposed that focus groups offer researchers a rich source in which to gather genuine information about participants' perceptions, experiences, and attitudes which provide a basis from which to build theory. Another variation of interviewing techniques proposed by Martin and Chaney (1992) is the Delphi technique, which can be valuable in gathering data on a subject from a panel of experts.
In connection to this, the collected and analyzed data were checked for accuracy by a social studies professor who teaches social studies methods course. He was selected because of his knowledge and familiarity with conducting similar research on a similar topic and similarity in reporting such research. This expertise is necessary so that he is able conduct an informal examination of the data. The expert examined the raw data, transcripts, data that have been coded and analyzed, and reconstructed and synthesized items.
For this study, a participant was considered to exhibit interpretive skills if he or she focused on aspects of a historical source in deriving meanings about slavery or slave owners. As part of the analysis, the participants were assessed for whether or not they provided elaborate or circumscribed interpretations of the sources. Numerous possible interpretations and detailed analysis of the sources indicated a higher level of interpretation skill (Wineburg, 1991, 2001; Fehn, 1997).
The researcher also checked the ability of the participants to recognize overarching themes or generalizations to provide a coherent relationship among the sources. The participants’ ability to construct a sophisticated narrative, such as the ability to weave the themes of oppression and resistance in their narrative, was also assessed. The researcher assessed if and how the participant used all or a few of the sources as authentic evidence in support of his or her generalization or theme. Finally the researcher analyzed interview transcripts to determine the usage of historical heuristic as the participants narrated their stories. Historical heuristic is the ability of the participants to critically evaluate and distinguish between a more reliable source and less reliable source with substantive arguments or reasons (Wineburg, 1991, 2001 ; Fehn, 1997). The researcher compared the similarities and differences between the participants because the individual differences affect an individual teacher’s perception of historical thinking and learning (Larsson, 1998).
In identifying effective teaching strategies for teaching historical thinking skills the researcher analyzed and evaluated the lesson plan based on three principles derived from the literature review on chapter three with regard to teaching and learning history. The principles are; (a) history is interpretive and is explained through narratives; (b) learning history is through in depth understanding; (c) history is learned through disciplined inquiry.
History is interpretive and is explained through narratives suggested that a single historical account is not entirely objective. The historical events were over and cannot be directly observed. The only way to find out what happened is through using primary sources and artifacts which students can interpret and develop into narratives of what might have happened. In other words, an effective history lesson should utilize historical documents and artifacts that are relevant to the topic and objectives of the lesson.
Learning history through in depth understanding means that students should know how to interpret history and write narratives about history. Just knowing historical facts such as dates, events, and people does not mean greater historical understanding. To attain in depth understanding, teachers should use teaching strategies that help students to organize ideas and engage them in sustained activities so they have enough time to understand and reflect on the meaning and significance of what they are studying. This could be achieved by encouraging students to interrogate the primary sources, collecting data, questioning, interpreting, explaining, developing historical narratives and organizing a community of critical learners
History learned through discipline inquiry means that students should learn history through a process of systematic inquiry that is specifically historical. They should be taught the historian’s craft of interrogating primary sources. They have to learn how to evaluate sources, how to reconcile and explain conflicting accounts, and how to properly narrate their own account of historical events.
In conclusion, this chapter provides the explanation on the methodical procedures that the researcher followed to collect, analyze and validate the data for this study. The findings, conclusions, and recommendations of this study were derived from the guidelines presented in this chapter for the purpose of producing a responsible and thrust worthy research findings.
Validation of the Data
According to Stewart and Kamins (1993), the use of secondary data is advantageous for a researcher since one can already evaluate the suitability of a data as it is already in existence, thus, much time can be saved. Needless to say, an evaluation of potential secondary data is very important before one incorporates it in his/her study.
In this study, the researcher adopted the three-stage process devised by Saunders et al (2004, p. 205):
The first stage is assessing the overall suitability of data to research questions and objectives. During this stage, the researcher paid particular attention to measurement validity (measuring / estimating whether the secondary data will result to a valid answer to the research questions and objectives) and coverage (this includes ensuring whether or not the data is wanted and can be included, as well as making sure that sufficient data remain for analyses to be undertaken once unwanted data have been excluded).
The second stage is evaluating precisely the suitability of data for analyses needed to answer and meet the research questions and objectives. In this stage, the researcher made sure of the validity and reliability of the secondary data by assessing how it was previously gathered, who are its sources, and the likes. Also, the researcher was cautious not to commit measurement bias (which can occur due to deliberate distortion of data or changes in the way data are collected) had been paid close attention.
Finally, the researcher judged whether to use data based on an assessment of costs and benefits in comparison with alternative sources.
Ethical Consideration
The data generated will be used solely to understand the development of teaching and learning theories. The researcher is solely responsible for conducting the whole research process and shall abide all the policies regarding the organization as well as the university. The data will not be transferable for any means in person or organization. The research is being done according to the guidelines and rules and regulations of the university. The researcher does not belong to any professional bodies to share the outcome of the research results. The four stages of ethics in doing research are followed by the way of a good design, modes of data collection, analysis of data and for proper dissemination. Both confidentiality and anonymity will be maintained of the informants who have participated or shared information in the research. There will be no Coercion or force to take advantage from the informants. Full voluntary guarantee will be taken from the informants. Due consideration and approval will be taken from the organization which is being studied. Prior objectives and motive of the research will be intimated. There shall be no misrepresentation or misuse of the data collected from the organization. Strict confidentiality shall be maintained. Finally, the university for dissemination of academic purposes might take the data collected.
As stated in this chapter, the researcher will undergo stages. In the research design, the researcher will collect secondary data and will formulate and develop an interview. In this stage, this instrument will be subjected to approval and validation. During the data collection, the researcher will collate and summarize the data obtained from the literatures and survey. The researcher will then analyze these data and from these, findings and recommendations will be presented.
In summary, the researcher will have taken four major phases to complete the study.
Phase 1: Problem Identification for Research
In the first phase, the researcher identifies the specific focus of the problem to be researched. This involves reviewing existing theory, research, and practices from professional literature. This process helps me integrate theoretical perspectives and empirical findings with my own understanding of the problem, and discern the aspect of the problem the researcher want to research and learn more about.
Phase 2: Administration of the Instrument
After reviewing literature, the researcher formulates questions for the survey and makes a set of guide questionnaires for the interview. These are then presented to the advisor for validation purposes.
Phase 3: Data Collection and Analysis
In the third phase, the researcher will collect and analyse data for the purposes of identifying critical variables specific to their setting. These data will enable me to achieve a specific understanding of the problem.
Phase 4: Data Synthesis and Generation of Recommendations
In the fourth phase, the researcher will synthesise findings from the previous phases and relevant previous research. The focus of this stage is to synthesise these data to modify existing hypotheses and account for different factors, as well as generating recommendations based on new understandings. During this phase, research-based, culture-specific recommendations for action will be generated.
0 comments:
Post a comment.
404 Not found
Our approach
- Responsibility
- Infrastructure
- Try Meta AI
RECOMMENDED READS
- 5 Steps to Getting Started with Llama 2
- The Llama Ecosystem: Past, Present, and Future
- Introducing Code Llama, a state-of-the-art large language model for coding
- Meta and Microsoft Introduce the Next Generation of Llama
- Today, we’re introducing Meta Llama 3, the next generation of our state-of-the-art open source large language model.
- Llama 3 models will soon be available on AWS, Databricks, Google Cloud, Hugging Face, Kaggle, IBM WatsonX, Microsoft Azure, NVIDIA NIM, and Snowflake, and with support from hardware platforms offered by AMD, AWS, Dell, Intel, NVIDIA, and Qualcomm.
- We’re dedicated to developing Llama 3 in a responsible way, and we’re offering various resources to help others use it responsibly as well. This includes introducing new trust and safety tools with Llama Guard 2, Code Shield, and CyberSec Eval 2.
- In the coming months, we expect to introduce new capabilities, longer context windows, additional model sizes, and enhanced performance, and we’ll share the Llama 3 research paper.
- Meta AI, built with Llama 3 technology, is now one of the world’s leading AI assistants that can boost your intelligence and lighten your load—helping you learn, get things done, create content, and connect to make the most out of every moment. You can try Meta AI here .
Today, we’re excited to share the first two models of the next generation of Llama, Meta Llama 3, available for broad use. This release features pretrained and instruction-fine-tuned language models with 8B and 70B parameters that can support a broad range of use cases. This next generation of Llama demonstrates state-of-the-art performance on a wide range of industry benchmarks and offers new capabilities, including improved reasoning. We believe these are the best open source models of their class, period. In support of our longstanding open approach, we’re putting Llama 3 in the hands of the community. We want to kickstart the next wave of innovation in AI across the stack—from applications to developer tools to evals to inference optimizations and more. We can’t wait to see what you build and look forward to your feedback.
Our goals for Llama 3
With Llama 3, we set out to build the best open models that are on par with the best proprietary models available today. We wanted to address developer feedback to increase the overall helpfulness of Llama 3 and are doing so while continuing to play a leading role on responsible use and deployment of LLMs. We are embracing the open source ethos of releasing early and often to enable the community to get access to these models while they are still in development. The text-based models we are releasing today are the first in the Llama 3 collection of models. Our goal in the near future is to make Llama 3 multilingual and multimodal, have longer context, and continue to improve overall performance across core LLM capabilities such as reasoning and coding.
State-of-the-art performance
Our new 8B and 70B parameter Llama 3 models are a major leap over Llama 2 and establish a new state-of-the-art for LLM models at those scales. Thanks to improvements in pretraining and post-training, our pretrained and instruction-fine-tuned models are the best models existing today at the 8B and 70B parameter scale. Improvements in our post-training procedures substantially reduced false refusal rates, improved alignment, and increased diversity in model responses. We also saw greatly improved capabilities like reasoning, code generation, and instruction following making Llama 3 more steerable.

*Please see evaluation details for setting and parameters with which these evaluations are calculated.
In the development of Llama 3, we looked at model performance on standard benchmarks and also sought to optimize for performance for real-world scenarios. To this end, we developed a new high-quality human evaluation set. This evaluation set contains 1,800 prompts that cover 12 key use cases: asking for advice, brainstorming, classification, closed question answering, coding, creative writing, extraction, inhabiting a character/persona, open question answering, reasoning, rewriting, and summarization. To prevent accidental overfitting of our models on this evaluation set, even our own modeling teams do not have access to it. The chart below shows aggregated results of our human evaluations across of these categories and prompts against Claude Sonnet, Mistral Medium, and GPT-3.5.

Preference rankings by human annotators based on this evaluation set highlight the strong performance of our 70B instruction-following model compared to competing models of comparable size in real-world scenarios.
Our pretrained model also establishes a new state-of-the-art for LLM models at those scales.

To develop a great language model, we believe it’s important to innovate, scale, and optimize for simplicity. We adopted this design philosophy throughout the Llama 3 project with a focus on four key ingredients: the model architecture, the pretraining data, scaling up pretraining, and instruction fine-tuning.
Model architecture
In line with our design philosophy, we opted for a relatively standard decoder-only transformer architecture in Llama 3. Compared to Llama 2, we made several key improvements. Llama 3 uses a tokenizer with a vocabulary of 128K tokens that encodes language much more efficiently, which leads to substantially improved model performance. To improve the inference efficiency of Llama 3 models, we’ve adopted grouped query attention (GQA) across both the 8B and 70B sizes. We trained the models on sequences of 8,192 tokens, using a mask to ensure self-attention does not cross document boundaries.
Training data
To train the best language model, the curation of a large, high-quality training dataset is paramount. In line with our design principles, we invested heavily in pretraining data. Llama 3 is pretrained on over 15T tokens that were all collected from publicly available sources. Our training dataset is seven times larger than that used for Llama 2, and it includes four times more code. To prepare for upcoming multilingual use cases, over 5% of the Llama 3 pretraining dataset consists of high-quality non-English data that covers over 30 languages. However, we do not expect the same level of performance in these languages as in English.
To ensure Llama 3 is trained on data of the highest quality, we developed a series of data-filtering pipelines. These pipelines include using heuristic filters, NSFW filters, semantic deduplication approaches, and text classifiers to predict data quality. We found that previous generations of Llama are surprisingly good at identifying high-quality data, hence we used Llama 2 to generate the training data for the text-quality classifiers that are powering Llama 3.
We also performed extensive experiments to evaluate the best ways of mixing data from different sources in our final pretraining dataset. These experiments enabled us to select a data mix that ensures that Llama 3 performs well across use cases including trivia questions, STEM, coding, historical knowledge, etc.
Scaling up pretraining
To effectively leverage our pretraining data in Llama 3 models, we put substantial effort into scaling up pretraining. Specifically, we have developed a series of detailed scaling laws for downstream benchmark evaluations. These scaling laws enable us to select an optimal data mix and to make informed decisions on how to best use our training compute. Importantly, scaling laws allow us to predict the performance of our largest models on key tasks (for example, code generation as evaluated on the HumanEval benchmark—see above) before we actually train the models. This helps us ensure strong performance of our final models across a variety of use cases and capabilities.
We made several new observations on scaling behavior during the development of Llama 3. For example, while the Chinchilla-optimal amount of training compute for an 8B parameter model corresponds to ~200B tokens, we found that model performance continues to improve even after the model is trained on two orders of magnitude more data. Both our 8B and 70B parameter models continued to improve log-linearly after we trained them on up to 15T tokens. Larger models can match the performance of these smaller models with less training compute, but smaller models are generally preferred because they are much more efficient during inference.
To train our largest Llama 3 models, we combined three types of parallelization: data parallelization, model parallelization, and pipeline parallelization. Our most efficient implementation achieves a compute utilization of over 400 TFLOPS per GPU when trained on 16K GPUs simultaneously. We performed training runs on two custom-built 24K GPU clusters . To maximize GPU uptime, we developed an advanced new training stack that automates error detection, handling, and maintenance. We also greatly improved our hardware reliability and detection mechanisms for silent data corruption, and we developed new scalable storage systems that reduce overheads of checkpointing and rollback. Those improvements resulted in an overall effective training time of more than 95%. Combined, these improvements increased the efficiency of Llama 3 training by ~three times compared to Llama 2.
Instruction fine-tuning
To fully unlock the potential of our pretrained models in chat use cases, we innovated on our approach to instruction-tuning as well. Our approach to post-training is a combination of supervised fine-tuning (SFT), rejection sampling, proximal policy optimization (PPO), and direct preference optimization (DPO). The quality of the prompts that are used in SFT and the preference rankings that are used in PPO and DPO has an outsized influence on the performance of aligned models. Some of our biggest improvements in model quality came from carefully curating this data and performing multiple rounds of quality assurance on annotations provided by human annotators.
Learning from preference rankings via PPO and DPO also greatly improved the performance of Llama 3 on reasoning and coding tasks. We found that if you ask a model a reasoning question that it struggles to answer, the model will sometimes produce the right reasoning trace: The model knows how to produce the right answer, but it does not know how to select it. Training on preference rankings enables the model to learn how to select it.
Building with Llama 3
Our vision is to enable developers to customize Llama 3 to support relevant use cases and to make it easier to adopt best practices and improve the open ecosystem. With this release, we’re providing new trust and safety tools including updated components with both Llama Guard 2 and Cybersec Eval 2, and the introduction of Code Shield—an inference time guardrail for filtering insecure code produced by LLMs.
We’ve also co-developed Llama 3 with torchtune , the new PyTorch-native library for easily authoring, fine-tuning, and experimenting with LLMs. torchtune provides memory efficient and hackable training recipes written entirely in PyTorch. The library is integrated with popular platforms such as Hugging Face, Weights & Biases, and EleutherAI and even supports Executorch for enabling efficient inference to be run on a wide variety of mobile and edge devices. For everything from prompt engineering to using Llama 3 with LangChain we have a comprehensive getting started guide and takes you from downloading Llama 3 all the way to deployment at scale within your generative AI application.
A system-level approach to responsibility
We have designed Llama 3 models to be maximally helpful while ensuring an industry leading approach to responsibly deploying them. To achieve this, we have adopted a new, system-level approach to the responsible development and deployment of Llama. We envision Llama models as part of a broader system that puts the developer in the driver’s seat. Llama models will serve as a foundational piece of a system that developers design with their unique end goals in mind.

Instruction fine-tuning also plays a major role in ensuring the safety of our models. Our instruction-fine-tuned models have been red-teamed (tested) for safety through internal and external efforts. Our red teaming approach leverages human experts and automation methods to generate adversarial prompts that try to elicit problematic responses. For instance, we apply comprehensive testing to assess risks of misuse related to Chemical, Biological, Cyber Security, and other risk areas. All of these efforts are iterative and used to inform safety fine-tuning of the models being released. You can read more about our efforts in the model card .
Llama Guard models are meant to be a foundation for prompt and response safety and can easily be fine-tuned to create a new taxonomy depending on application needs. As a starting point, the new Llama Guard 2 uses the recently announced MLCommons taxonomy, in an effort to support the emergence of industry standards in this important area. Additionally, CyberSecEval 2 expands on its predecessor by adding measures of an LLM’s propensity to allow for abuse of its code interpreter, offensive cybersecurity capabilities, and susceptibility to prompt injection attacks (learn more in our technical paper ). Finally, we’re introducing Code Shield which adds support for inference-time filtering of insecure code produced by LLMs. This offers mitigation of risks around insecure code suggestions, code interpreter abuse prevention, and secure command execution.
With the speed at which the generative AI space is moving, we believe an open approach is an important way to bring the ecosystem together and mitigate these potential harms. As part of that, we’re updating our Responsible Use Guide (RUG) that provides a comprehensive guide to responsible development with LLMs. As we outlined in the RUG, we recommend that all inputs and outputs be checked and filtered in accordance with content guidelines appropriate to the application. Additionally, many cloud service providers offer content moderation APIs and other tools for responsible deployment, and we encourage developers to also consider using these options.
Deploying Llama 3 at scale
Llama 3 will soon be available on all major platforms including cloud providers, model API providers, and much more. Llama 3 will be everywhere .
Our benchmarks show the tokenizer offers improved token efficiency, yielding up to 15% fewer tokens compared to Llama 2. Also, Group Query Attention (GQA) now has been added to Llama 3 8B as well. As a result, we observed that despite the model having 1B more parameters compared to Llama 2 7B, the improved tokenizer efficiency and GQA contribute to maintaining the inference efficiency on par with Llama 2 7B.
For examples of how to leverage all of these capabilities, check out Llama Recipes which contains all of our open source code that can be leveraged for everything from fine-tuning to deployment to model evaluation.
What’s next for Llama 3?
The Llama 3 8B and 70B models mark the beginning of what we plan to release for Llama 3. And there’s a lot more to come.
Our largest models are over 400B parameters and, while these models are still training, our team is excited about how they’re trending. Over the coming months, we’ll release multiple models with new capabilities including multimodality, the ability to converse in multiple languages, a much longer context window, and stronger overall capabilities. We will also publish a detailed research paper once we are done training Llama 3.
To give you a sneak preview for where these models are today as they continue training, we thought we could share some snapshots of how our largest LLM model is trending. Please note that this data is based on an early checkpoint of Llama 3 that is still training and these capabilities are not supported as part of the models released today.

We’re committed to the continued growth and development of an open AI ecosystem for releasing our models responsibly. We have long believed that openness leads to better, safer products, faster innovation, and a healthier overall market. This is good for Meta, and it is good for society. We’re taking a community-first approach with Llama 3, and starting today, these models are available on the leading cloud, hosting, and hardware platforms with many more to come.
Try Meta Llama 3 today
We’ve integrated our latest models into Meta AI, which we believe is the world’s leading AI assistant. It’s now built with Llama 3 technology and it’s available in more countries across our apps.
You can use Meta AI on Facebook, Instagram, WhatsApp, Messenger, and the web to get things done, learn, create, and connect with the things that matter to you. You can read more about the Meta AI experience here .
Visit the Llama 3 website to download the models and reference the Getting Started Guide for the latest list of all available platforms.
You’ll also soon be able to test multimodal Meta AI on our Ray-Ban Meta smart glasses.
As always, we look forward to seeing all the amazing products and experiences you will build with Meta Llama 3.
Our latest updates delivered to your inbox
Subscribe to our newsletter to keep up with Meta AI news, events, research breakthroughs, and more.
Join us in the pursuit of what’s possible with AI.

Product experiences
Foundational models
Latest news
Meta © 2024
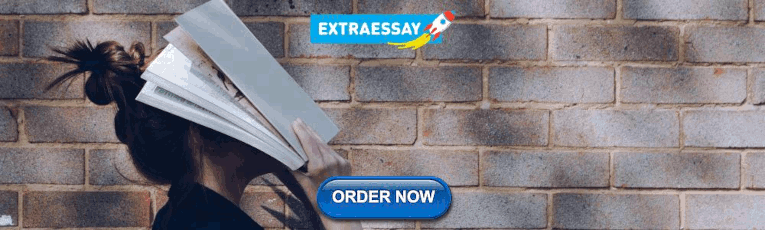
IMAGES
VIDEO
COMMENTS
State the research output. Indicate what you are expecting after you conduct the research. In our example above, the research output is the assessed level of satisfaction of college students with the use of Google Classroom as an online learning platform. Create the model using the research's determined input, process, and output.
The input-process-output model has historically been the dominant approach to understanding and explaining team performance and continues to exert a strong influence on group research today. The framework is based on classic systems theory, which states that the general structure of a system is as important in determining how effectively it ...
Examples of input-process-output in different industries. The input-process-output model emerged in the twentieth century as a fundamental model for describing complex computer systems. However, IPO quickly found applications outside of computer programming as a practical methodology in general systems theory and design. Many businesses found ...
Input-process-output (I-P-O) is a structured methodology for capturing and visualising all of the inputs, outputs, and process steps that are required to transform inputs into outputs. It is often referred to, interchangeably, as an I-P-O model or an I-P-O diagram, both of which make reference to the intended visual nature of the method.
Although previous research has not considered the importance of the process by studying only the relationship between the input and outcomes or output (Salas et al., 2008, 2014), IPO research should try to put in light the role of team learning processes (Edmondson et al., 2007).
The input-process-output model. The input-process-output (IPO) model, or input-process-output pattern, is a widely used approach in systems analysis and software engineering for describing the structure of an information processing program or other process. Many introductory programming and systems analysis texts introduce this as the most basic structure for describing a process.
For this, research processes are iteratively divided into impartible subprocesses at different detail levels using the input-process-output model. The concrete software implementation of the identified, universally applicable concept is finally presented in form of the workflow editor KadiStudio of the Karlsruhe Data Infrastructure for ...
Thus, the I-P-O framework is deficient for summarizing the recent research and constrains thinking about teams. As an alternative model, we use the term IMOI (input-mediator-output-input). Substituting "M" for "P" reflects the broader range of variables that are important mediational influences with explanatory power for
The input-process-output (IPO) model is a widely used approach in systems analysis and software engineering for describing the structure of an information processing program or another process. Many introductory programming and systems analysis texts introduce this as the most basic structure for describing a process. [1]
Viewed as a predictive device, input-output analysis specifies a method of predicting the total output of a series of industries from the so-called "final-demand schedule," or "bill of goods." Its actual. use to forecast total output for a future year involves, first, forecasting.
Overview. The input-process-output (IPO) model is a widely used approach in systems analysis and software engineering for describing the structure of an information processing program or another process. Many introductory programming and systems analysis texts introduce this as the most basic structure for describing a process.
The input, process, and output details at this level are also not indicated in Figure 1, as neither of this level is a focus for quality improvement in this article. Like the tertiary level, the pre-school level also receives inputs from the context and from the national education level in the form of human and other resources (see Figure 1 ).
Abstract. This chapter provides a general introduction to input-output analysis and input-output models. A brief description of the historical development of the framework, leading to its widespread use, is given. A qualitative discussion of the general framework is presented, followed by a discussion of the key assumptions that underlie ...
The CIPP model evaluates the context, input, process, and output of educational programs and curricula using a systematic approach and by identifying their weaknesses and strengths, it can help policymakers at the macro level to plan expert actions and decide whether to continue, stop, or revise the educational program, ultimately promoting the ...
Input-process-output (I-P-O) is a structured procedure for capturing and visualizations all of the intakes, outputs, and process steps that what requires to transform input into outputs. Computers is often referred to, interchangeably, as an I-P-O model or an I-P-O plot, both of which construct reference to the intended ocular nature of the method.
The third goal of this article is to present the Simplified Model of Group Social Influence Processes, an interactive input-process-output model relevant to decision-making groups. The article ends with a discussion of the implications of this model for future research and further model development.
4.1 The Input-Process-Output-Outcome Conceptual Framework Quality assurance can be depicted on the basis of a conceptual framework that considers a university as a productive system, in which inputs are transformed, through processes, into outputs. The outputs have impacts or outcomes which are the long-term effects produced by the transformation
The input stage of the Input Process Output model refers to the various factors that influence a team's functioning and performance. These inputs can be categorized into three levels: individual, group, and environmental. Individual-level input factors encompass the unique characteristics, skills, and experiences that each team member brings ...
As shown in Fig. 1, the effects are influenced by input factors, which describe the structure of the project, and process factors, which include the specificities of the research process.Institutions that summarize external factors to the project influence not only the effects, but also the input and process factors.
Abstract. Information furnished by input-output may appear to have limited use for the market researcher. Economic information on developing countries however is already limited. The input-output approach may be helpful for that purpose. Download to read the full chapter text.
Firstly, you will need to develop a set of input indicators that will allow you to monitor the availability of essential resources. Ideally, your input indicators will draw upon existing project management tools such as budget reports, reference letters, CVs and letters of support. Your indicators should alert you early to logistical challenges ...
In the IPO model, a process is viewed as a series of boxes (processing elements) connected by inputs and outputs. Information or material objects flow through a series of tasks or activities based on a set of rules or decision points. (Harris & Taylor, 1997) Flow charts and process diagrams are often used to represent the process.
State the research output. Indicate what you what expecting after you conduct and research. In to example above, the choose output is the assessed level of satisfied of college students in the use of Google Classroom while an online learning plateau. Create the model employing the research's determined input, procedure, and output.
Today, we're introducing Meta Llama 3, the next generation of our state-of-the-art open source large language model. Llama 3 models will soon be available on AWS, Databricks, Google Cloud, Hugging Face, Kaggle, IBM WatsonX, Microsoft Azure, NVIDIA NIM, and Snowflake, and with support from hardware platforms offered by AMD, AWS, Dell, Intel, NVIDIA, and Qualcomm.