Brought to you by:

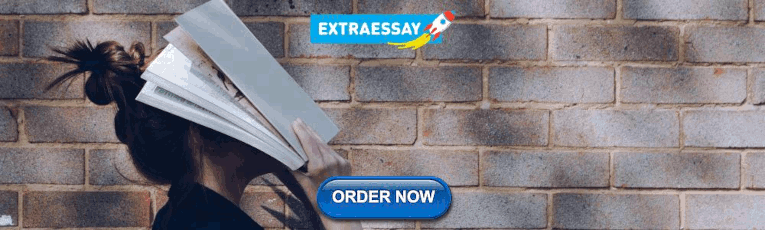
Financial Ratio Analysis
By: Richard H. Mimick
Financial ratio analysis is used to evaluate the financial performance and condition of a business enterprise by measuring its progress towards financial goals. Its purpose is to provide information…
- Length: 23 page(s)
- Publication Date: Jan 1, 1985
- Discipline: Finance
- Product #: 85K031-PDF-ENG
What's included:
- Educator Copy
$4.95 per student
degree granting course
$8.95 per student
non-degree granting course
Get access to this material, plus much more with a free Educator Account:
- Access to world-famous HBS cases
- Up to 60% off materials for your students
- Resources for teaching online
- Tips and reviews from other Educators
Already registered? Sign in
- Student Registration
- Non-Academic Registration
- Included Materials
Financial ratio analysis is used to evaluate the financial performance and condition of a business enterprise by measuring its progress towards financial goals. Its purpose is to provide information about the business entity for decision-making by both external and internal users. Terminology, definitions, and formulae (for vertical analyses, return on investment, investment utilization ratios, liquidity ratios, stability ratios, and growth ratios) are given in this technical note, and applied in a short exercise. This note would be suitable for a class in introductory finance.
Jan 1, 1985 (Revised: Apr 20, 2016)
Discipline:
Ivey Publishing
85K031-PDF-ENG
We use cookies to understand how you use our site and to improve your experience, including personalizing content. Learn More . By continuing to use our site, you accept our use of cookies and revised Privacy Policy .
Academia.edu no longer supports Internet Explorer.
To browse Academia.edu and the wider internet faster and more securely, please take a few seconds to upgrade your browser .
Enter the email address you signed up with and we'll email you a reset link.
- We're Hiring!
- Help Center
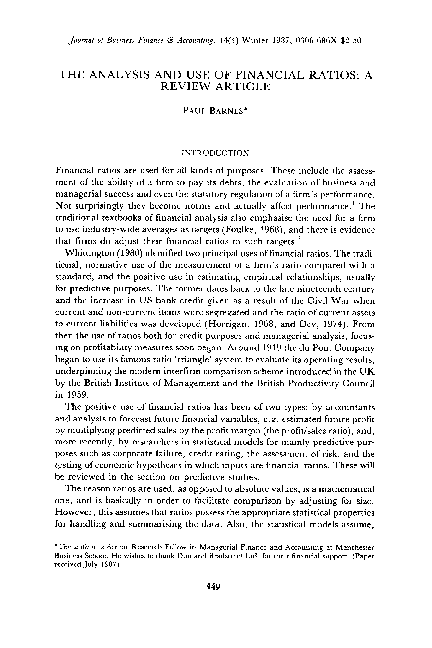
The Analysis and Use of Financial Ratios: A Review Article

1987, Journal of Business Finance & Accounting
Related Papers
Journal of Business Finance & Accounting
Ronald Bird
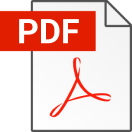
Usman kabir
The certainty of the reliance on the use of financial ratio analysis in making investment decisions by potential investors still remained a mystery. This has to do with the choice of ratios to select when making investment decisions. Many shareholders in Nigeria are uneducated or illiterate, and due to their ignorance, they cannot use ratio analysis in evaluating firms for investment decisions. Thus, this paper explores the concept of financial ratio analysis in terms of the decision usefulness of financial ratios. The paper suggests that the relevant financial information needed for the purposes of making investment decision can be sourced through the use of financial ratio analysis. Therefore, management must ensure that disclosure of comprehensive financial ratios form part of financial statement prepared for the overall appraisal of firms.
David Woodliff
Thankgod Agwor
Financial ratios are assumed to be very useful predictors of business performance, growth and financial status. This study evaluates the collinearity and correlation of financial ratios to establish a pattern of relationship that could serve s n empirically supported guide for financial ratios reliance in business evaluation decisions. The research data were gathered from the annual account report of vita foam Plc and analyzed using collinearity and correlation to test the research propositions. The paper found the existence of correlation and collinearity in the financial ratios, and concludes that financial ratios are reliable business status indicators. We therefore recommend the application of financial ratios in the evaluation of business performance, growth and financial strength.
navneet joshi
The current business environment has forced business units to devise innovative means and mechanisms so as to stay competitive in the market. This necessitates the need for constantly evaluating their performance measures. Business units have identified several measures which depict the performance yet ambiguities remain with respect to what is appropriate measure for performance measurement. Ratio analysis is one such measure which business units have been using for sufficient period of time. Yet there exists certain points of consideration which form the basis for this paper and their convergence into research question namely (a) What constitutes ratio analysis and (b) Which of the component of ratio analysis, has the greatest contribution? While addressing these questions, HUDCO’s secondary data is used as a base. Ratio analysis is used to depict the measure of performance while descriptive statistics is used for data interpretation. Findings indicate that there is no single comp...
Accounting, Management and Information Technologies
Duarte Trigueiros
Ratios are routinely used for extracting information from accounting reports. However, ratios present only part of the information available and can be easily complemented provided that information-technology facilities are accessible. This study infers the functional form of the information discarded by ratios. Then it develops extensions of ratios incorporating the discarded information, showing examples of their use and discussing the benefits obtained. Extensions of ratios seem promising as a facility attached to computerized databases of accounting reports. The concepts developed here are a step toward a more technology-supported analysis of financial statements.
Paul Barnes
Empirical studies have shown distributions of financial ratios are skewed. An explanation for this is given and it is argued that in such circumstances comparison of a fmancial ratio with some norm (e.g. industry average) is likely to misinform. It is also shown that where financial ratios are used as inputs to statistical models normality is irrelevant but a method of transformation into a normal distribution is provided whereby original interrelationships are preserved. Finally, because of the inadequacies of financial ratios, it is shown how regression analysis may be used in financial statement analysis.
MRS. OKWO IFEOMA MARY
The British Accounting Review
This study identifies general postulates underlying the validity of the financial ratio measurement. Then, new relationships are suggested obeying the same postulates, which may replace the ratio form in the case of non-proportionality. Where proportionality holds, these relationships revert to the traditional ratio. The paper also reviews the reasons for expecting non-proportional components in ratios and presents application examples of the new relationships.
Vichy Pattiasina , Victor Pattiasina
A Company will be interested in investors if its financial report is relevant and reliable. The relevance and reliable financial report can be reflected in the Asset Number and Liability Report on a Financial Position Statement (Balance Sheet). The success of a company can be measured by company ability, which is reflected in management performance. A company's performance parameter that commonly used is profit change. Profit itself is important to the company since this will be decision basis for investors to invest in the company. Profit Change reflected in financial statements using fair value will bring benefits to market participants, as the financial statements itself reflects the real market value. This research aims to examine the influence of Current Ratio, Total Asset Turnover, Price Earnings Ratio, and Return on Asset, towards profit changes that based on fair value. Research problem discussed in this study was how CR, TATO, PER, and ROA influence profit changes of a property company, real estate, and building construction, in period 2013-2016. The study was based on fair value. The population was company property, real estate, and building construction, that have been listed in Indonesia Stock Exchange (ISE). It consisted of 63 companies. Through Purposive Sampling technique, 12 companies were selected to be examined. Data analysis was conducted through a multiple linear regression analysis. Results of multiple linear regression analysis showed that the CR, TATO, and PER had an influence towards Profit Changes, while ROA had no influence toward it.
RELATED PAPERS
E. Gigli, Ortucchio nelle foto d'epoca
Paola Nardecchia
The Journal of biological chemistry
Violaine Harris
Physical Review E
Kenichi Yoshikawa
Antimicrobial Agents and Chemotherapy
Karine Steenkeste
European Respiratory Journal
Nico Westerhof
Franz-Joseph Meissner
Majalah Ilmiah Ekonomi Komputer
Irma Apriyanti sari
Cinzia Galasso
EDUTEC : Journal of Education And Technology
Sartika Hutasuhut
Journal of Fatima Jinnah Medical University
quratulain mehdi
Atenea (Concepción)
Pablo Galindo Fuentes
Gendered Labor in specialized Economies: Archaeological Perspectives on Female and Male Work
James Heidke
Electronic Imaging
Srijith Rajeev
Stephen Myers
Carol Eduarda Ricobom
in: Neue Zürcher Zeitung, 17. September 1998, Nr. 215, S. 33.
Jürgen Müller
Natacha Duroisin
English Language Teaching
Gulsah Tikiz Erturk
anindya sen
kjgg fdfdgg
Youssry Botros
Nigerian Journal of Animal Production
Ewetola, I. A.
Julian Camilo
Escola Anna Nery
Maria Manuela Martins
RELATED TOPICS
- We're Hiring!
- Help Center
- Find new research papers in:
- Health Sciences
- Earth Sciences
- Cognitive Science
- Mathematics
- Computer Science
- Academia ©2024
Financial statement analysis: Principal component analysis (PCA) approach case study on China telecoms industry
Asian Journal of Accounting Research
ISSN : 2459-9700
Article publication date: 3 September 2019
Issue publication date: 11 December 2019
The Chinese Telecoms Industry has been rapidly growing over the years since 2001. An analysis of financial performance of the three giants in this industry is very important. However, it is difficult to know how many ratios can be used best with little information loss. The paper aims to discuss this issue.
Design/methodology/approach
A total of 18 financial ratios were calculated based on the financial statements for three companies, namely, China Mobile, China Unicom and China Telecom for a period of 17 years. A principal component analysis was run to come up with variables with significance value above 0.5 from each component.
At the end, the authors conclude how financial performance can be analysed using 12 ratios instead of the costly analysis of too many ratios that may be complex to interpret. The results also showed that ratios are all related as they come from the same statements, hence, the authors can use a few to represent the rest with limited loss of information.
Originality/value
This study will help different stakeholders who are interested in the financial performance of each company by giving them a shorter way to analyse performance. It will also assist those who do financial reporting on picking the ratios which matter in reflecting the performance of their companies. The use of PCA gives unbiased ratios that are most significant in assessing performance.
- Performance analysis
- Financial ratios
- Principal component analysis
- China telecoms industry
Mbona, R.M. and Yusheng, K. (2019), "Financial statement analysis: Principal component analysis (PCA) approach case study on China telecoms industry", Asian Journal of Accounting Research , Vol. 4 No. 2, pp. 233-245. https://doi.org/10.1108/AJAR-05-2019-0037
Emerald Publishing Limited
Copyright © 2019, Reginald Masimba Mbona and Kong Yusheng
Published in Asian Journal of Accounting Research . Published by Emerald Publishing Limited. This article is published under the Creative Commons Attribution (CC BY 4.0) licence. Anyone may reproduce, distribute, translate and create derivative works of this article (for both commercial and non-commercial purposes), subject to full attribution to the original publication and authors. The full terms of this licence may be seen at http://creativecommons.org/licences/by/4.0/legalcode
Introduction
The financial performance of a company is a primary concern for every stakeholder especially for investors, both aspiring and current ones. The measurement of the financial health of a company through the reported financial statements gives a qualitative analysis of the company’s position as well as an account of how the company has utilised its capital in production. According to Bhunia et al. (2011) , financial performance analysis involves using reported results in a company’s financial statements to obtain the quantitative performance characteristics of a company with the aim of determining how efficient the company has been in terms of the use of their resources according to the decisions made by the management.
Financial statement analysis using ratios has been one of the most commonly used primary models of assessing business performance. It is one of the primary models of assessment of a firm’s performance over years and as well as comparing it to the rest of the players in the industry. Due to limited time for those who do the analysis of financial statements and also given the fact that these ratios are mostly correlated, the number of ratios that are being evaluated has to be reduced so that focus is given to a few with minimum loss of data ( Taylor, 1986 ). Using principal component analysis (PCA), the study reduced the number of variables for any further regression analysis from 17 variables to 3 variables. Likewise, the number of ratios that are important have also been reduced with only significant ratios for each principal component now being used to analyse the performance of these companies as well as their industry. This study proves that the performance of a company can be analysed using just a few factors or by focusing on fewer ratios, which is cost effective with lesser time as well as obtaining more precise results that have least duplication of calculations.
Since 2002, China has been the largest telecom market by subscriber base and the industry has been attracting a lot of investment within and outside China ( Uria-Recio and O’Connor, 2004 ). The telecom industry in China has been the backbone of the economy that is highly dependent on the internet and online services ( Grubman, 2010 ). Their research, innovation and building of different technologies including the current 5G that they are jointly working on have given China high growth to make it compete with countries like the USA that are considered as earlier entrants into this market. Their internet and data services have facilitated access to online shopping, IPTV, online messaging and calling platforms, data cloud and many other services that are available at very fast speed and cheap rates. The rapid build-up of the industry’s infrastructure has been the main sign of the aggressive growth and development over the past two decades ( Lu, 2000 ).
The Chinese Telecom industry was heavily controlled by the government through the ownership and formation of policies on investment, areas of operations and tariffs charged. In late 2001, China successfully joined the World Trade Organization and this meant that it had to adjust some of its policies including regulations on players in its telecoms industry. Even though they opened the doors for foreigners, this industry remained monopolised by the three state-owned companies who have been competing for the highest market share, best financial performance and top innovation into new technologies including 5G network. Over the past years, they have cemented their dominance by taking over the other small players in the industry that were state owned as well. This study, thus, seeks to assess the financial performance of this industry since the doors for open competition were opened in this sector.
China Telecom Limited
China Telecom is an incorporated company in the People’s Republic of China as China Telecom Corporation Limited with the aim of providing information services. These are, but not limited to wireline and telecommunication services, broadband and wireless internet access services, information services and other services that relate to information and telecommunications. According to the company’s report, there were at least 250m mobile subscribers, 134m broadband subscribers and 122m active access lines. The company is currently listed on the Hong Kong Stock Exchange and the New York Stock Exchange where they trade American Depositary Shares ( Telecom, 2019 ).
China Mobile Limited
This is a company which is incorporated on Hong Kong and New York Stock Exchanges since 1997 with a constituent stock of Hang Seng Index in Hong Kong. Since its formation, China Mobile has grown to have the highest market share in the telecommunications globally with the highest number of subscribers, of which 887m are mobile subscribers while 113m of them are broadband subscribers. Mobile services in the form of mobile voice and data services are the main businesses of the company and other services include wireline broadband and other services in the telecommunications industry ( Mobile, 2019 ).
China Unicom (Hong Kong) Limited
China Unicom, which was formed in 1994, is one of the oldest Telecoms Company in China and in the 2000s was listed on the Hong Kong and New York Stock Exchange. The company is the second largest mobile services provider in China with a subscriber base of 248m mobile subscribers, and 60m fixed line subscribers. Their service coverage includes all the telecommunications services and it has been doing exceptionally well in the mobile and fixed networks provision ( Unicom, 2019 ).
The rest of this paper is structured as follows: literature review, research objectives, methodology, discussion and analysis of results and finally the conclusion.
Literature review
Financial analysis involves the use of quantitative information from financial statements, that is, income statement, balance sheet and statement of cash flows in order to come up with relationships of the items that are reported by the company according to the accounting standards for reporting. In doing this, the company is able to evaluate its decisions during a financial year or a given period and see its strengths, weaknesses and areas that need attention in the organisation ( Abraham, 2004 ; Bhargava, 2017 ; Schönbohm, 2013 ). Additionally, “they also provide clues on where the management might find more resources to boost its revenue” ( Mahajan and Yaday, 2016 ). In a case study on India’s telecommunications industry, Bhargava (2017) concludes that due to the increased contribution of the telecoms industry to different economies the financial health of the industry is important to the whole economy. Therefore, there is need for measurement of this constantly to monitor the economic performance of the whole industry. The telecoms industry is highly capital intensive and investors will be interested in knowing the “the financial condition and worthiness” of the industry which is achieved through financial analysis. However, even though it is beneficial it has to be noted that the ratios isolate the assessed factors from the rest of the report; hence, precaution has to be taken when interpreting them ( Abraham, 2004 ).
Even though ratios were seen as less significant due to the introduction of more sophisticated statistical analysis tools, authors still believe that they are still a useful tool in measuring performance. For example, a study which was done by Altman (1968) proved how ratios are still useful in prediction of bankruptcy using the case of manufacturing firms. Other studies on proving the importance and usefulness of ratios by Lewellen (2004) and Floros et al. (2009) found that investment ratios are useful in predicting market values of shares.
With this in mind, this study looks at internal determinants of performance as in the study by Allen et al. (2011) and another by Burja (2011) . These are factors within the control of management and can be able to influence them through their decisions. Through this, “the management can anticipate changes in the external environment and try to position the company to take advantage of anticipated developments” ( Burja, 2011 ). The external environment includes factors like demographic changes, GDP, inflation and other external environmental factors. However, besides the quantitative factors, management also have to analyse qualitative factors internally and externally even though these have no standard set to assess them as their measurement can be highly subjective.
A lot of other case studies have been done on financial performance analysis using ratios ( Eversull and Rotan, 1997 ; Collier et al. , 2010 ; Hossan and Habib, 2010 ; Grubman, 2010 ; Bhargava, 2017 ). For example Al-Jafari and Al Samman (2015) investigated the determinants of profitability for industrial firms in Oman. By utilising ordinary least squares (OLS) model on seven ratios, they drew up conclusions on the relationship between profitability ratios and other calculated non-profitability ratios. They found that there is a positive significant relationship between profitability, firm size, growth, fixed assets and working capital. Additionally, they also conclude that management efficiency on these large firms gives them better profit returns.
While Burja (2011) only focussed on the micro or internal environment in his regression analysis of financial performance, Allen et al. (2011) carried out an investigation on both internal and external environment to see how it impacts the profitability of the firm. This was a distinguished study as it included both internal and external factors in the regression analysis.
In a case study on the furniture industry, Traian-Ovidiu and Daniel-Teodor (2013 ) and Tsuji (2014) made a detailed analysis of a company’s statements to aid those who use them for investment decisions. Their studies focussed on bringing together financial ratios from financial statements and market data from stock markets to see how the indices on the market are influenced by the performance of different rations on the reported statements.
A study on the Indian public sector looked at how strategic industries with the government as major player performs financially ( Bhunia et al. , 2011 ). The case study looked at the ratios for India’s pharmaceutical industry financial reports. Using a number of statistical methods including standard deviation, mean and also regression analysis, they established the relationship between profit measure and other performance measures.
According to Buse et al. (2010 ) economic rate of return (ERR) is an important ratio in financial statement analysis because they considered it as an indicator of the economic performance of a company. In their study, they took ERR as a comprehensive ratio that looks at the organisation return and contribution with consideration of both internal and external factors affecting the business.
Kofi-Akrofi (2013 ) carried out a similar study but he, however, used multiple regression to look at the profitability of Telecommunications in Ghana for a period of four years. In his research, the main objective was to establish the relationship between the two main statements, hence, he treated them as independent from each other. A study by Oloko et al. (2014) looked at the telecoms industry but they focussed on how management style, cost of labour and competition impacts performance and profitability of Kenya’s Telkom.
While the literature reviewed covers a number of case studies on financial statements using ratios, some gaps exist. First, we have found that none has so far focussed on the Chinese telecoms especially the period after the Chinese Government opened its doors to the world to invest in their industries. Second, few research studies have used PCA to find out which ratios give best performance analysis with least loss of data from amongst the pool of all ratios. From the review of the past studies, we realized that different ratios were used and some are correlated because they are all from the same statements. A similar study was done by Taylor (1986) which focussed on the Australian firms. The study did not point to a specific industry; hence, with differences in industries the model might not be a one-size-fits-all especially given also the differences in the operational environment between China and Australia. Third, using PCA allows the use of at least 18 ratios reducing subjectivity effects on which ratios should be used for further analysis which include regression analysis on performance. Finally, as shown in our correlation matric we can see that all ratios are related which means that there is no independency to carry out the regression. This relationship comes from the fact that these ratios use data from the same statement. By applying PCA we create new independent variables that allow for effective further analysis with even lesser variables.
To carry out a PCA on 18 financial ratios for the Chinese Telecoms Industry to reduce the number of variables.
To analyse how the components are related to each other.
To recommend a combination or mix of ratios that best assess and analyse performance in the industry.
To examine the ratios with highest variation and assess their impact on the industry.
Methodology
In previous studies on relationship analysis for financial statements and financial ratios, two models have mainly been used. The first one is the panel OLS regression model which has been applied by a number of accounting articles on financial performance and in most literature less than ten variables are used ( Al-Jafari and Al Samman, 2015 ; Jakob, 2017 ; Burja, 2011 ). The second model has been the multiple regression which was adopted by other researchers ( Bhunia et al. , 2011 ; Kofi-Akrofi, 2013 ; Buse et al. , 2010 ).
In this research, we use PCA which is a statistical tool that is used to reduce the variables that are used in data analysis with minimum loss of the original data ( Karamizadeh et al. , 2013 ). It has been used in a number of industries with one of the most common being in biometrics or “bioimaging” where physical features are used to identify a person with application on mobile phones, security systems. PCA has also been used for dimension reduction of large volumes of data and also in image compressing ( Arab et al. , 2018 ; Karamizadeh et al. , 2013 ; Polyak and Mikhail, 2017 ). The application of PCA in reducing variables as already noted in the literature review makes it a useful tool in modern days where large volumes of data are compiled and compared for its usefulness. In accounting field, PCA was used in a study by Taylor (1986) to reduce the number of ratios used in analysis of Australian companies since a lot of ratios are available, this makes the model very useful in helping investors and those who study ratios on knowing the most important ratios as it offers a way to reduce the numbers of ratios by statistically taking those that are most important with limited bias. The fact that PCA creates a new set of artificial variables which are independent makes it less complex to do regressions and come up with conclusions on related variables. In itself the PCA only reduces the variables that can be further used for regression analysis.
The first principal component combines the X -variables that have the maximum variance amongst all the combinations. Much of the variation in the data is taken by this first component. The second one likewise also takes the maximum remaining variation in the data with the condition that the correlation between the first and the second component is 0. This continues until the “ i th” component, which will account to the last variation that has not been accounted for by the other components with the condition still remaining that its correlation with the other components is 0. This condition is what creates the independence of the variable being used.
The principal component estimation uses eigenvectors as the coefficient to come up with the following basic equations: (1) Y 1 = e ˆ 11 Z X 1 + e ˆ 12 Z X 2 + e ˆ 13 Z X 3 + ⋯ + e ˆ 1 i Z X i , (2) Y 2 = e ˆ 21 Z X 1 + e ˆ 22 Z X 2 + e ˆ 23 Z X 3 + ⋯ + e ˆ 2 i Z X i , (3) Y i = e ˆ i 1 Z X 1 + e ˆ i 2 Z X 2 + e ˆ i 3 Z X 3 + ⋯ + e ˆ i i Z X i , where Y is the principal component; e ˆ the eigenvector; ZX the standardized value of the ratios used.
A total of 18 accounting ratios that are used in most literature in accounting and are deemed to be the most important measures of profitability, liquidity, management efficiency, leverage, valuation and growth, cash flow indicator and effective tax rate. This list, however, is not exhaustive; it has the ability to cover more ratios. Some of the ratios differ from the ones by Taylor (1986) because they are more relevant to the telecoms industry as used in previous studies. Second, in his study, Taylor used “debt coverage” due to missing data for interest cover but for this study we used interest cover as all relevant data were available ( Table I ).
The data used are from 2001 to 2017. This is the period that has financial statements available on the websites of the companies. Statistical Package for Social Sciences 20 was then used for the analysis of the financial statement with Microsoft Excel 13 used in the calculation of the final coefficients for each principal component. Standardized data were used instead of the original data as the ratios have different units of measurement. If the raw data are used, then a PCA will tend to give more emphasis to those variables that have higher variances than those variables that have lower variances meaning results will depend on the unit of measurement for each variable and since these data do not have that we used the standardized values.
All the financial data are secondary data taken from the company’s annual financial reports as presented on their websites and also from trusted journals and websites ( Table II ).
The matrix in Table III shows the relations within the ratios, which means we cannot have any ratio that is independent from the other, hence, we cannot use these ratios in regression as independent variables. This means we need to create new variables using PCA that will be independent from each other.
Results and discussion
The financial statements of the three telecoms companies in China were used to assess the firms’ performance. Thus, PCA was adopted to suggest the most suitable ratios that can best explain the firms’ performance while ensuring minimum data loss among the pool of ratios. Using the data from the industry, which is described in Table II , we use standardized values of the ratios in the extraction of the principal component.
In this section, we first examined the components that have been extracted and the new independent variables according to our first objectives. Then second, we looked at the ratio mix that can be used for analysis of the telecoms industry performance.
Based on the extraction in Table IV , we only need four components to use as variables in the telecoms industry instead of the initial 18. These new variables are not only independent but are more easily comparable than the initial variables we had. The first principal component covers the highest variation in the data with an initial eigenvalue of 7.982, which represent 44.344 per cent of the variance. Three other components needed to, at least, get to 85 per cent of the variance, which is significant with least data being lost in the analysis. This contradicts with the initial conclusion that was made on Australian firms, who used similar ratios but got four components at 64.8 per cent Taylor (1986) . This variation is huge and is likely to be because of these data being focussed on one industry, hence, reducing the differences in valuation and reports of numerous firms. The increased percentage means there is lesser data loss from the four components and this allows for better analysis of the financial statements. From the PCA for the telecoms industry, we remain with four variables, Y1–Y4 whose calculation based on Equations (1) –( 3) ( Table V ).
Table VI shows us the mix of ratios that PCA extract to give significant analysis on the performance of the telecoms industry. The 12 ratios represent the mix that has important ratios from the classes that we have in general. The performance of the telecoms industry is measured mainly with four classes of ratios.
First, profitability of the company is very important as also concluded in research studies by Altman (1968) , Collier et al. (2010) and Mahajan and Yaday (2016) . In component 1, we see profitability measures having a very significant value of above 0.8. This is supported through most literatures that use the profitability of a firm as one of the primary indicators of its performance or as the dependent variable in most of the regression analysis, for example, return on assets ROA which was used by Kofi-Akrofi (2013 ) and Al-Jafari and Al Samman (2015) . Profit maximisation should be the main goal of management so as to give the high return expected by their shareholders and measured by ROSE. This is achieved by maintaining a high and constant profit margin throughout operations of the industry with a balance being kept between the profits and the expenditure of the firm especially in the salaries.
Second, liquidity in the industry is very important as proved by component 1. From the analysis, we established the importance of working capital assets as well as the importance of the operating cash to the firms which are also included in literature as significant measures as in the studies published by Bhunia et al. (2011) , Neves (2011) , Baños-Caballero et al. (2012) , Knauer and Wöhrmann (2013) . Operating cash has the highest value in component 1 which may signify its importance in the industry. The combination of the cash and the other current assets shows how performance in this industry is dependent on the liquid assets at the disposal of the firms. These resources are important for use in growth and expansion of operations. In analysing the individual companies it is interesting to see how failure to maintain high liquidity has led to the struggle of China Telecom and China Unicom to perform well and this supports what Singhania et al. (2014) noted the necessity of maintaining a balance between liquidity and profitability. In an analysis done on individual companies as the study built on the industry average, it was observed that China Telecom and China Unicom have been struggling to maintain favourable liquidity balances which has always left them as market followers behind China Mobile in terms of ability to generate profits, launch new services and upgrad to newer technologies including 4G network coverage.
Third, the management efficiency in the industry has also been argued by Al-Jafari and Al Samman (2015) in their study on firms in Omani. The decisions of management in allocation of their short-term resources are of importance and the relationship of this with the liquidity is that short-term decision of management in allocation of their non-fixed assets has impact on their performance. The use of the current ratios to measure their performance gets rid of the historical cost effect from the valuation of fixed assets, which makes it difficult to measure current performance. This factor supports the fact that we can measure the quality of management decisions through analysis of the financial results by relating their outcome to turnover. Even though in 2015 the Chinese Government introduced the tower company to take over network towers of the industry, DBS Vickers Securities (2015) noted that the effect of this on the performance of the company cannot be reflected. This is even though we cannot ignore it as this analysis put more emphasis on liquid resources of the company. Management also has to effectively allocate its expenses to the salaries and wages of its workers as this ratio is important in this service industry. There is need to always keep a favourable rate of return per worker for the industry which means that worker reward should always be related to what is being gained from the output of that worker. Whilst within the service industry there is no specific and accurate measure of the output, we can use the revenue paid to workers to see their relationship with the turnover. The higher this is, the better.
Of particular interest in our findings is the importance of customer growth and revenue per customer. As companies in the industry compete for customers, they still need to find the balance between customer growth and what they gain from it (revenue from the customer). With the government regulating the tariffs Zhiling (2002) and also as companies running different promotions to lure customers to their network, there is a need for management to keep that growth balancing with the revenue growth. In analysing the industrial data, we have noted that the revenue growth is not matching the customer growth and as a result this is very risky for the industry players that may be aiming for customers at the expense of high revenues as it will not be beneficial to its investors. This eventually can lead to a conflict between the government and other shareholders as the company tries to offer services to all at affordable rates with the public shareholder that will mainly be looking forward to high returns from the customer base.
In the financing section, only equity ratio is included in the components. The absence of the interest cover in measuring of performance is likely to prove that the model of financing is not of great importance when measuring the performance in the industry, which has very high capital requirements through investment in fixed assets. However, the allocation of capital is very important as also supported by the return of stockholder equity ratio. This means that management is expected to generate high returns for its shareholders in all their operations. The interests of the shareholders are further cemented by the EPS that needs to remain high and be maintained as the firms grow. The other stakeholders with fixed returns like the fixed tax, fixed interest always have their returns regardless of the performance yet the shareholders’ returns are based on how the firm has performed. Management has to keep a low volatility in their EPS and ROCE to maintain high investment and also attract more investors and partners.
Based on the results from the PCA analysis carried on 18 ratios over 17 years, profitability, liquidity, customer growth and management efficiency are the main performance highlighters for the industry. Maintaining all these will result in favourable returns to the shareholder of this industry. We cannot look at these factors in isolation as they are correlated and they are all combined when assessing the overall performance of the industry or individual firms. A combination of 12 ratios is able to give meaningful conclusion about the industry and can effectively analyse operations of the firms.
Further studies
According to Jakob (2017) , state-owned enterprises are often claimed to be less profitable and less efficient compared to private corporations. A comparison can be carried out within an environment that has both private and public firms. In 2015, the Chinese Government introduced the tower company to take over network towers of the industry DBS Vickers Securities (2015 ). It will be important to see how this change is important and can benefit other governments and industry as well if they implement it.
List of accounting ratios used as variable
Descriptive statistics for telecoms industry
Inter-item correlation matrix for China telecoms Industry
Total variance explained
Abraham , A. ( 2004 ), “ A model of financial performance analysis adapted for nonprofit organisations ”, AFAANZ 2004 Conference Proceedings. University of Wollongong: Research Online , Alice Springs, NT , 4-6 July .
Al-Jafari , M.K. and Al Samman , H. ( 2015 ), “ Determinants of profitability: evidence from industrial companies listed on muscat securities market ”, Review of European Studies , Vol. 7 No. 11 , pp. 303 - 311 .
Allen , A.J. , Shaik , S. , Myles , A.E. and Yeboah , O.-A. ( 2011 ), “ Determinants of profitability performance: an analysis of class I railroads in the United States ”, Southern Agricultural Economics Association Annual Meeting. Corpus Christi, TX .
Altman , E.I. ( 1968 ), “ Financial ratios, discriminant analysis and the prediction of corporate bankruptcy ”, The Journal of Finance , Vol. 23 No. 4 , pp. 589 - 609 .
Arab , A. , Harbi , J. and Abbas , A.H. ( 2018 ), “ Image compression using principal component analysis ”, Al-Mustansiriyah Journal of Science , Vol. 29 No. 4 , pp. 141 - 146 .
Baños-Caballero , S. , García-Teruel , P.J. and Martínez-Solano , P. ( 2012 ), “ How does working capital management affect the profitability of Spanish SMEs? ”, Small Bus Econ , Vol. 39 , pp. 517 - 529 .
Bhargava , P.D. ( 2017 ), “ Financial analysis of information and technology industry of India (a case study of Wipro Ltd and Infosys Ltd) ”, Journal of Accounting, Finance and Auditing Studies , Vol. 3 No. 3 , pp. 1 - 13 .
Bhunia , A. , Mukhuti , S.S. and Roy , S.G. ( 2011 ), “ Financial performance analysis-a case study ”, Current Research Journal of Social Sciences , Vol. 3 No. 3 , pp. 269 - 275 .
Burja , C. ( 2011 ), “ Factors influencing the companies’ profitability ”, Annales Universitatis Apulensis Series Oeconomica , Vol. 3 No. 2 , pp. 215 - 224 .
Buse , L. , Ganea , M. and Circiumaru , D. ( 2010 ), Using Linear Regression in the Analysis of Financial-Economic Performances. C01-G30 , University of Craiova , Craiova , Vol. 2 No. 38 , pp. 1 - 12 .
Collier , H.W. , Grai , T. , Haslitt , S. and Mcgowan , C.B. Jr ( 2010 ), “ Using actual financial accounting information to conduct financial ratio analysis: the case of Motorola ”, Journal of Business Case Studies , Vol. 6 No. 4 , pp. 24 - 32 .
DBS Vickers Securities ( 2015 ), China Telecom Sector. China/Hong Kong Industry Focus , DBS Vickers (Hong Kong) Limited , Hong Kong .
Eversull , E.E. and Rotan , B.L. ( 1997 ), Analysis of Financial Statements: Local Farm Supply, Marketing Cooperatives , Rural Business-Cooperative Service US Department of Agriculture , Washington, DC , Vol. 154 , p. 37 .
Floros , C. , Jaffry , S. and Ghulam , Y. ( 2009 ), “ Predicting returns with financial ratios: evidence from Greece ”, International Journal of Financial Economics and Econometrics , Vol. 14 No. 1 , pp. 31 - 44 .
Grubman , J.B. ( 2010 ), “ Challenges for China’s telecom industry in an increasingly all broadband/mobile world ”, Deutsche Bank 2010 China Access Conference, Magee Group, LLC , Beijing , January 14 .
Hossan , F. and Habib , M.A. ( 2010 ), “ Performance evaluation and ratio analysis of pharmaceutical company in Bangladesh ”, master’s thesis, International Business, University West, Trollhättan , pp. 1 - 64 .
Jakob , B. ( 2017 ), “ Performance in strategic sectors: a comparison of profitability and efficiency of state-owned enterprises and private corporations ”, The Park Place Economist , Vol. 25 No. 1 , pp. 9 - 20 .
Karamizadeh , S. , Abdullah , S.M. , Manaf , A.A. , Zamani , M. and Hooman , A. ( 2013 ), “ An overview of principal component analysis ”, Journal of Signal and Information Processing , Vol. 4 , pp. 173 - 175 .
Knauer , T. and Wöhrmann , A. ( 2013 ), “ Working capital management and firm profitability ”, Journal of Management Control , Vol. 24 , pp. 77 - 87 .
Kofi-Akrofi , G.Y. ( 2013 ), “ Profitability analysis of the telecommunications industry in Ghana from 2002 to 2006 ”, Asian Journal of Business Management , Vol. 5 No. 1 , pp. 60 - 76 .
Lewellen , J. ( 2004 ), “ Predicting returns with financial ratios ”, Journal of Financial Economics , Vol. 74 No. 2 , pp. 209 - 235 .
Lu , D. ( 2000 ), “ China’s telecommunications infrastructure buildup on its own way ”, in Ito , T. and Krueger , A.O. (Eds), Deregulation and Interdependence in the Asia-Pacific Region, NBER-EASE , University of Chicago Press , pp. 371 - 413 .
Mahajan , N. and Yaday , A. ( 2016 ), “ A financial statement analysis- case study for an industry ”, International Journal of Engineering and Technical Research , Vol. 5 No. 3 , pp. 6 - 8 .
Mobile , C. ( 2019 ), “ Overview ”, available at: www.chinamobileltd.com/en/about/overview.php (accessed March 3, 2019 ).
Neves , L.F.G. ( 2011 ), “ Working capital management and profitability: the telecom case ”, Master in Finance, Instituto Universitario de Lisboa, Lisbon , pp. 1 - 63 .
Oloko , M. , Anene , E.B. and Kathambi , I. ( 2014 ), “ Empirical analysis on determinants of non-profitability margins: a case of Telkom Kenya in telecommunications industry ”, International Journal of Scientific and Research Publications , Vol. 4 No. 3 , pp. 1 - 4 .
Polyak , B. and Mikhail , K. ( 2017 ), “ Principle component analysis: robust versions ”, Automation and Remote Control , Vol. 78 No. 3 , pp. 490 - 506 .
Schönbohm , A. ( 2013 ), “ Performance measurement and management with financial ratios – the BASF SE Case ”, in Bruche , G. , Dörrenbächer , C. , Nagel , F. and Ripsas , S. (Eds), Berlin School of Economics and Law , Berlin , pp. 1 - 27 .
Singhania , M. , Rohit , Y.J. and Sharma , N. ( 2014 ), “ Working capital management and profitability: evidence from Indian manufacturing companies ”, Decision , Vol. 41 No. 3 , pp. 313 - 326 .
Taylor , S.L. ( 1986 ), “ Analysing financial statements: how many variables should we look at? ”, The Securities Institute Journal , Vol. 1 , April , pp. 19 - 21 .
Telecom , C. ( 2019 ), “ Overview ”, available at: www.chinatelecom-h.com/en/company/company_overview.php (accessed March 3, 2019 ).
Traian-Ovidiu , C. and Daniel-Teodor , V. ( 2013 ), “ Case study on analysis of financial statements at a furniture manufacturer ”, Journal of Knowledge Management, Economics and Information Technology , Vol. III No. 5 , pp. 1 - 24 .
Tsuji , C. ( 2014 ), “ Exploring the financial ratios: the case study of the famed chemical industry firms in the US ”, Case Studies in Business and Management , Vol. 1 No. 2 , pp. 11 - 21 .
Unicom , C. ( 2019 ), “ Company profile ”, available at: www.chinaunicom.com.hk/en/about/profile.php (accessed March 3, 2019 ).
Uria-Recio , P. and O’Connor , D.S.W.H. ( 2004 ), “ China telecommunications panorama ”, Telephony world, pp. 1-4, available at: www.casaasia.es/pdf/310550620PM1109693180696.pdf (accessed March 15, 2019 ).
Zhiling , S. ( 2002 ), China Telecommunications Industry – The Forbidden City in the Information Age? Political and Economic Structural Changes in Asia , Institute for Research in Economics and Business Administration Bergen , Bergen .
Corresponding author
Related articles, we’re listening — tell us what you think, something didn’t work….
Report bugs here
All feedback is valuable
Please share your general feedback
Join us on our journey
Platform update page.
Visit emeraldpublishing.com/platformupdate to discover the latest news and updates
Questions & More Information
Answers to the most commonly asked questions here
- Open access
- Published: 01 May 2024
The use of high-frequency data in cryptocurrency research: a meta-review of literature with bibliometric analysis
- Muhammad Anas 1 , 2 ,
- Syed Jawad Hussain Shahzad 3 &
- Larisa Yarovaya 4
Financial Innovation volume 10 , Article number: 90 ( 2024 ) Cite this article
Metrics details
As the crypto-asset ecosystem matures, the use of high-frequency data has become increasingly common in decentralized finance literature. Using bibliometric analysis, we characterize the existing cryptocurrency literature that employs high-frequency data. We highlighted the most influential authors, articles, and journals based on 189 articles from the Scopus database from 2015 to 2022. This approach enables us to identify emerging trends and research hotspots with the aid of co-citation and cartographic analyses. It shows knowledge expansion through authors’ collaboration in cryptocurrency research with co-authorship analysis. We identify four major streams of research: (i) return prediction and measurement of cryptocurrency volatility, (ii) (in)efficiency of cryptocurrencies, (iii) price dynamics and bubbles in cryptocurrencies, and (iv) the diversification, safe haven, and hedging properties of Bitcoin. We conclude that highly traded cryptocurrencies’ investment features and economic outcomes are analyzed predominantly on a tick-by-tick basis. This study also provides recommendations for future studies.
Introduction
With the advent of advanced high-frequency data analysis techniques, finance scholars have increasingly delved into the intricate dynamics of financial markets, thereby uncovering novel insights into their behavior and interconnectivity. However, accessing high-frequency data poses a significant obstacle for relatively new and immature financial assets, such as cryptocurrencies. As a result, most early studies in this field resorted to employing daily data, inadvertently missing out on the advantages of utilizing data with higher frequencies (Corbet et al. 2018a ). Nevertheless, as the infrastructure supporting cryptocurrency trading matured, collecting data at higher frequencies became possible, ushering in a new wave of cryptocurrency research that leveraged these more granular data (e.g., Katsiampa et al. 2022 ).
Contrary to low-frequency data, high-frequency data helps identify jumps in financial assets (Christensen et al. 2014 ), facilitates the detection of behavioral biases, accurate information about market microstructures, intraday changes, and patterns that cannot be easily captured but are concealed at daily frequency (see Hansen and Huang 2016 ). Using high-frequency (hourly) data helps search for arbitrage trades in both short- and long-term horizons (Sifat et al. 2019 ). Inefficiency in cryptocurrencies can also be better detected in high-frequency (intraday) data than in low-frequency (daily) data (Zargar and Kumar 2019 ). Moreover, low-frequency data, even daily data, cannot reflect the swiftly changing environments in crypto markets during trading. By contrast, high-frequency data that cover shorter time horizons can improve the precision of models and the understanding of market dynamics, i.e., monitoring price dynamics and formation, trading activity, microstructures, and investor behaviors in the crypto market. From COVID-19 onwards, the literature has evaluated cryptocurrencies at high frequencies from various perspectives (Katsiampat et al. 2022 ).
The results reported in cryptocurrency research papers, often developed in parallel, create discrepancies stemming from differences in observation periods and variations in the data frequencies used by scholars. Alexander and Dakos ( 2020 ) found that 80 of 124 papers published between January 2017 and March 2019 used questionable and/or non-traded data in their empirical analysis. To our knowledge, no previous studies have attempted to comprehensively explore cryptocurrency literature using high-frequency data, synthesize the available findings, and provide meaningful directions for future research in this domain. To fill this gap and enhance our understanding of the role of high-frequency cryptocurrency data in shaping the cryptocurrency literature, this study employs a bibliometric analysis.
The bibliometric technique is the most popular approach for analyzing growing literature, assessing the scholarly contributions of diverse stakeholders, and helping create thematic maps for scholarly applications. In addition, we performed content analysis. We attempt to answer the following questions: What are the prevailing publication trends in using high-frequency data in cryptography-related research? Which highly influential articles leverage high-frequency data in cryptographic studies? Does a surge in the number of publications by authors or journals lead to a proportional increase in the total number of citations and getting more attention? Does publishing in highly influential journals in this field contribute significantly to an increase in the number of citations of the authors’ articles? What are the primary research streams encompassing past and current themes in the cryptocurrency literature using high-frequency data? What are the prospective avenues of research within this field?
This literature review aimed to comprehensively explore diverse academic articles that have utilized high-frequency data to unravel the complexities of cryptocurrency trends, market volatility, and the interrelationships among different digital currencies. By synthesizing and critically analyzing the findings of these pivotal studies, we aimed to provide a nuanced understanding of the implications of high-frequency data analysis in shaping our comprehension of the ever-evolving cryptocurrency landscape.
This study contributes to the literature in several ways. First, we conducted a performance or citation analysis based on article production growth, contributing authors, institutions, countries, and key journals. We also examine the impact of increasing publication authors, and journals on their citations and attention. Further, we assess and identify the fields relevant to crypto market research and their associated links with other assets that dominate the use of high-frequency datasets. This topic is underexplored in the literature. Thus, this study aimed to fill this gap by classifying the body of knowledge, contributing to the rapidly growing cryptocurrency literature, and offering specific directions for further research on cryptocurrency scholars. Our study highlights the benefits of using high-frequency data in cryptocurrency research, and we believe that it will facilitate further research in these innovative areas.
Second, we conduct a meta-analysis of the literature through bibliometric and content analyses, employing qualitative and quantitative methods to synthesize research on the cryptocurrency market, particularly emphasizing the growing utilization of high-frequency datasets. Although several alternative approaches are available for categorizing existing papers based on their primary themes and methodologies, such as a systematic literature review (Corbet et al. 2019 ), bibliometric analysis is rapidly gaining prominence in finance. Therefore, our contribution builds upon previous studies that utilized alternative methodologies, including a systematic literature review (Ballis & Verousis 2022 ; Corbet et al. 2019 ) or survey method (Fang et al. 2022 ; Holub & Johnson 2018 ), by integrating both meta-analysis and bibliometric review approaches.
Finally, the novelty of our study lies in its distinction from recently published bibliometric analyses of cryptocurrencies. For example, several studies have exclusively conducted bibliometric analyses focusing on Bitcoin (Merediz-Solà & Bariviera 2019 ; Ramona et al. 2019 ; Shen et al. 2020 ), while others have explored cryptocurrencies from various perspectives, including their growth (García-Corral et al. 2022 ), economic implications (Yue et al. 2021 ), financial asset role (Jalal et al. 2021 ), adoption trends (Sousa et al. 2022 ), and the evolution from a technological to an economic standpoint (Jiang et al. 2021 ). However, our current systematic literature review and bibliometric analysis emphasize the use of high-frequency data in cryptocurrency market research. To our knowledge, no bibliometric reviews have focused on this subject.
We find that the highest number of publications is by Ahmet Sensoy (author) in Finance Research Letters (journals). Frequently publishing authors and journals are also influential, but the level of attention is not attached to the frequency of publications. Articles published in influential journals (with many citations per paper) attract more citations. The use of high-frequency data in cryptocurrency research can be divided into four major streams. The first group focuses on return prediction and the measurement of cryptocurrency volatility, which is expected given the lack of established pricing models and the high volatility in cryptocurrency markets. The second focuses on the (in)efficiency of cryptocurrencies. Market efficiency is an inherent feature of the market’s microstructure. The third examines price dynamics and bubbles in cryptocurrencies, and the fourth examines the hedging, diversification, and safe haven properties of Bitcoin.
The remainder of this paper is organized as follows: Sect. " Literature background " discusses the background literature, Sect. " Data and methodology " explains the data and methodology, Sect. " Bibliometric results " describes the bibliometric results, Sect. " Avenues of future research " identifies research hotspots in the field, Sect. " Conclusions " offers future research directions, and Sect. " Conclusions " concludes the paper.
Literature background
Cryptocurrency, initially a niche product in small online communities, has evolved into a digital medium of exchange prized for its economic efficiency and rapid transaction speeds. Its journey can be traced back to the 1980s when David Chaum ( 1983 ) introduced a groundbreaking encryption method still used for web-based security. It is the foundation for modern electronic fund transfers (Bunjaku et al. 2017 ). In the 1990s, several digital currencies were developed; however, many of them, such as Wei Dye’s money, failed to gain traction. A notable exception is PayPal, which has gradually expanded in influence. Notwithstanding past attempts to create virtual currencies, such as E-gold and Liberty Reserve in 1996 and 2006, the title of the first enduring and most famous blockchain-based cryptocurrency is Bitcoin, introduced by Nakamoto ( 2008 ). Before the inception of Bitcoin, the concept of cryptocurrency was virtually nonexistent, primarily because of concerns surrounding centralization and the legitimacy of digital currencies (Bakar et al. 2017 ).
The introduction of Bitcoin has ignited substantial excitement within financial markets, and this phenomenon poses an existential risk to certain conventional financial functions (Härdle et al. 2020 ). Subsequently, new cryptocurrencies such as Ethereum, Ripple, and Litecoin and stable coins such as Tether have also been introduced, potentially serving as competitors or alternatives to Bitcoin. According to Statista, the cryptocurrency landscape has experienced exponential growth, expanding from 63 in 2013 to the staggering 4,501 options available today. Among these, only the top 20 account for 90% of the worldwide market share, thus achieving global dominance (Ji et al. 2019 ).
From a notable paper by Nakomoto ( 2008 ), who introduced Bitcoin, many papers have been published in economics, finance, computer science, management, and other fields. Cryptocurrency has become a revolutionary payment tool that serves as an alternative to traditional financial markets due to its sage of innovative technology (blockchain) and cryptography, Footnote 1 thereby ensuring a move towards the evolution of digital finance.
These newly developed digital currencies have advantages over conventional currencies, such as decentralized transactions, auditability, and anonymity, to name just a few. Still, they also contain unique risks, such as uncontrolled network operations (Johnson et al. 2014 ). The recent COVID-19 outbreak has spiked the interest of retail and institutional investors in the crypto market, which can be accessed from home through fintech trading sites, such as Binance and Robinhood (Long et al. 2022 ). This increased accessibility to digital assets has led to enhanced intraday trading in the cryptocurrency market. Moreover, improved ecosystems such as Cosmos and Polkadot facilitate crypto investors to make cross-blockchain trades that were not previously viable.
The discussion of cryptocurrencies has become a hot topic, attracting readers from industrial and academic communities (Tschorsch and Scheuermann 2016 ). Initially, researchers focused on understanding blockchain technology (Zheng et al. 2018 ; Zyskind and Nathan 2015 ) and the potential of Bitcoin as a substitute for traditional currencies in economics (Böhme et al. 2015 ; Yermack 2015 ). The current literature analyzing the financial features of the crypto market shows enormous growth from the perspective of economics and finance (see Chu et al. 2021 ; Kayal and Rohilla 2021 ; Shi and Shi 2021 ) because this market, with billions of dollars of capitalization, serves as a complementary currency with diversification features resembling an investible asset class (Bouri et al. 2017a ; Carrick 2016 ; Gandal et al. 2018 ). Moreover, with the maturity of the blockchain space, thousands of cryptocurrencies are traded worldwide (Corbet et al. 2019 ). However, these digital assets demonstrate some standard features, such as heavy tails, long memory, volatility clustering, and adverse leverage effects (Fung et al. 2022 ), and are speculative investment tools, which means they are prone to explosiveness or speculative bubbles (Bouri et al. 2019a ; Shahzad et al. 2022 ; Cheah and Fry 2015 ) because of the potential to generate higher returns for investors, thereby attracting a new group of speculators.
Despite the increased accessibility of high-frequency crypto data, most studies employ low-frequency data in the crypto market and aligned research areas. This is highlighted by a survey conducted by Bariviera and Merediz-Solà ( 2021 ), which affirms that most studies consider the daily frequency as a natural data frequency, ignoring other options. Among the most relevant studies, only a few have employed high-frequency data and considered them more efficient. Besides the findings of this survey, numerous studies have explored different aspects of cryptocurrencies, predominantly Bitcoin, by using data of daily frequency such as bubbles (Cheah & Fry 2015 ), hedging capabilities (Dyhrberg 2016b ), liquidity, and efficiency (Wei 2018 ), long memory interdependency (Cheah et al. 2018 ), structural breaks (Thies and Molnár 2018 ), transaction activity (Koutmos 2018 ), tail risk (Borri 2019 ) and its dependence (Nguyen et al. 2020 ), long memory effects (Phillip et al. 2019 ), and price clustering (Urquhart 2017 ), volatility spillover (Yi et al. 2018 ), and cyber-attacks, contagion and spillover within crypto market (Caporale et al. 2021 ).
The crypto market offers 24 h trading throughout the year. In the existing traditional financial market, investors may hold assets for a short period of a few minutes (Glantz and Kissell 2013 ), and the crypto market is no different. Many online exchanges offer tools and services that enable cryptocurrency traders to trade for minutes or seconds, resulting in high profits (Brauneis et al. 2022 ; Chu et al. 2019 ). Moreover, compared to other financial instruments, cryptocurrencies demonstrate higher intraday volatility (movements of 3–5% are common), and a large portion of high-frequency trades are pursued through algorithmic trading bots, which signifies the use of high-frequency data in analyzing cryptocurrencies (Chan et al. 2022 ; Zhang et al. 2019b ).
Several studies have supported the significance of using high-frequency data. For instance, Mensi et al. ( 2020 ) suggested that high-frequency data can locate or trace fitful market activities over time. In contrast, daily prices also differ in their calculations, which may create issues. Vidal-Tomás ( 2021 ) affirms that daily prices are either closing prices at the last closing hour of a day or the weighted averages of the hourly closing prices of a day (24 h), which are statistically different. The last hour’s daily closing prices may fail to explain the daily behavior of cryptocurrencies owing to characteristic volatility, and these prices may change in the next minute. Meanwhile, weighted average daily prices may distort trader behavior, as they cause a reduction in the volatility of cryptocurrencies by averaging high-frequency (hourly) prices, leading to a persistent time series. Recently, Yarovaya and Zięba ( 2022 ) confirmed that the return and volume of cryptocurrencies are not very sensitive to variations in the sampling intervals of high-frequency data (i.e., 5, 10, 20 min, 40 min, and hourly) but to variations between weekly, daily, and intraday data.
In line with the highlighted importance of high-frequency data, the recent research is more inclined to using of high-frequency data in exploring different aspects of cryptocurrencies, such as extreme connectedness between return and volume of cryptocurrencies at higher tail risk (Chan et al. 2022 ), long memory in return and volatility (Arouxet et al. 2022 ), rough volatility (Takaishi 2020 ) market complexity (Drożdż et al. 2020 ), price clustering (Li et al. 2020 ; Quiroga-Garcia et al. 2022 ) potential profitability by using a momentum strategy (Chu et al. 2020 ), volatility spillover among cryptocurrencies (Sensoy et al. 2021 ) and exchanges such as Binance, Coinbase, etc., (Alexander et al. 2022 ; Ji et al. 2021 ).
Previous attempts have been made to aggregate and review cryptocurrency literature. Ballis and Verousis ( 2022 ) study the impact of behavioral factors on cryptocurrencies, while Corbet et al. ( 2019 ) examine the breadth of cryptocurrency-related research. Fang et al. ( 2022 ) summarize existing research articles on cryptocurrency trading, covering topics such as trading platforms, signals, strategies, and risk management and highlighting related outcomes. Holub and Johnson ( 2018 ) map research on Bitcoin and other cryptocurrencies across various disciplines, including technical fields, economics, law, public policy, finance, and accounting. However, to our knowledge, no existing studies have provided a comprehensive literature survey of recent cryptocurrency research employing high-frequency data. Therefore, in this study, we aimed to fill this gap using a bibliometric analysis and meta-review approaches.
Data and methodology
This study employed the Scopus database to search for keywords and extracted relevant articles using a literature retrieval procedure. For data extraction, we can use all platforms such as Scopus, Web of Science (Clarivate Analytics), EconLit, IDEAS/REPEC, and Google Scholar; however, it would necessitate a huge data-cleaning process to combine all datasets (Corbet et al. 2019 ). Compared with WoS and EconLit, Scopus has had a broader coverage of journals since 1996. Although it has the same features as REPEC, it does not include working papers. Information on citations is available in Scopus, WoS, and REPEC but not in EconLit (Ruane and Tol 2007 ). However, relying on Scopus compared to other databases is based on its distinct features, that is broader coverage with citation information and more than 15,000 peer-reviewed subjects (Levine-Clark and Gil 2008 ), which is notably 30% larger than the WoS database (Comerio and Strozzi 2019 ), and allows for a more subtle analysis compared to Google Scholar, which provides limited bibliometric information for conducting bibliometric analysis.
The keywords are “crypto-market” or “cryptocurrenc*” or “crypto-currenc*” or “digital currenc*” or “Bitcoin” or “BTC” or “Ethereum” or “ETH” and “high-frequency” or “high frequency” or “intra-day” or “intraday” or “hour*” or “tick data” or “5?min” or “30?min.” This search generated 344 documents written in English and published between January 2015 and July 2022. However, we limited our search to articles published or in the press by excluding book chapters, conference papers, editorials, errata, data papers, and review papers, and we got 240 articles. Finally, by further scrutinizing the articles for irrelevant terms such as high-frequency band, closing hours, peak hours, work hours, intraday snapshots, etc., we obtained a final list of 189 articles as a literature sample from 2015 to 2022. This bibliometric dataset was analyzed using a bibliometric technique. The year-wise publication trends are shown in Fig. 1 . A significant increase in publications using high-frequency data was observed after 2018.
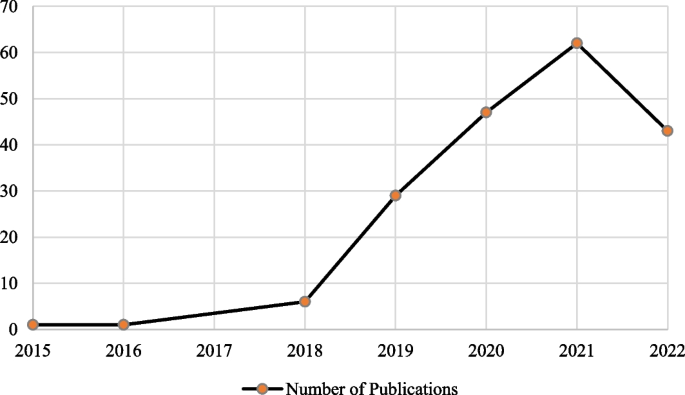
Publications trend over the years
Bibliometric analysis has gained popularity in several fields and has become a widely used approach in systematic reviews (Ruggeri et al. 2019 ; Patel et al. 2022 ). This approach can reveal unknown trends by collecting relevant information on a specific field or aspect of the subject. This can reduce subjective bias and steer authors to highly related fields to improve the quality of the literature review (Zupic and Čater 2015 ). Moreover, it helps researchers to comprehend a wider picture and creates links between publications from various sub-areas. Therefore, it is suitable for identifying the existing research state and future avenues (Paul and Bhukya 2021 ).
Bibliometric research methods use quantitative statistical analysis of a set of publications and then map in clusters of core authors, citations, and research institutions (De Bakker et al. 2005 ; Wang et al. 2021 ). It commonly focuses on citations, co-authors, co-citations, cartographic analyses, and bibliographic coupling (Zupic and Čater 2015 ). This study used bibliometric and content analyses to comprehensively examine the use of high-frequency data in cryptocurrency research to offer insight into existing research interests and discover streams of research on emerging topics in the field. Initially, the study employed a citation analysis (no networks) to highlight the volume of publications and their influential role in a specific topic. This step includes a citation analysis of authors, documents, and journals. Furthermore, regression analysis was performed to determine the authors’ and journals’ impact and the attention that they receive by publishing most frequently in this particular field. In addition, the authors’ impact those are publishing in journals that are found influencial this field. In the second stage, a co-authorship analysis was conducted to illustrate the network of collaborating authors in this field. In the third stage, cartographic or keyword co-occurrence analyses are performed to identify the evolution of research streams on a particular topic. Furthermore, co-citation (network) analysis was conducted to draw a scientific map showing the general topics or research streams through each cluster’s content analysis.
Several software tools are frequently used to conduct bibliometric studies easier such as HistCite, CiteSpace, CiteSpaceV, Publish or Perish, SciMAT, BibExcel, VOSviewer, Biblioshiny, Pajek, and Gephi. Considering the diversity of the features of different bibliometric tools, we decided to use two software tools: VOSviewer (van Eck and Waltman 2014 ) and Biblioshiny (Aria and Cuccurullo 2017 ). These fit our aim of examining citation counts and classifying the literature on crypto market research utilizing high-frequency data and offering the required analysis output. These software tools were chosen because of their reliability in dealing with large datasets, comprehensive ability to sort and deal with manifold information types, flexible visual impairments, expedient settings, built-in algorithms, and provision of an eclectic range of other available network and visualization analytic tools, such as Pajek and Gephi. Moreover, these tools are superior to other potential software tools for various reasons. For example, CiteSpaceV can be an alternative. Still, it is not that flexible and requires a lot of time to master its pretty complex user interface. At the same time, Histcite is confined to WoS and unable to interpret the Scopus dataset (Fahimnia et al. 2015 ), Bib Excel requires extra skill set and experience to conduct a modest analysis (Ampah et al. 2021 ), Pajek requires “. Net” dataset.
Therefore, the software tools opted for this study are easy to use without requiring profound familiarity with clustering techniques and advanced computer skills and are freely accessible to anyone (particularly aspiring scholars) to identify existing trends and gaps in scholarly research articles and publication databases. Biblioshiny has a well-ordered and instinctive interface (Moral-Muñoz et al. 2020 ). We adopt it for descriptive and citation analysis, including tables and graphs, while we use VOSviewer for science mapping for multiple reasons, such as data and network visualization; readers from different disciplines can easily understand the clustering and map created by it. For identifying clusters in mapping and deriving themes from them, VOSviewer is a compelling graphic interface for users who quickly observe maps (Cobo et al. 2011 ). We also use Microsoft Excel for frequency analysis and analyze the citation, co-citation, and keyword co-occurrence, thereby constructing a research atlas of the propensity to use high-frequency data in cryptocurrency and exploring the research flash points and frontlines in this field.
To this end, we perform three key bibliometric techniques: co-author, co-citation, and keyword co-occurrence analyses, thereby constructing a research atlas of the propensity to use high-frequency data in cryptocurrency and exploring the research flash points and frontlines in this field. A roadmap for this study is shown in Fig. 2 .
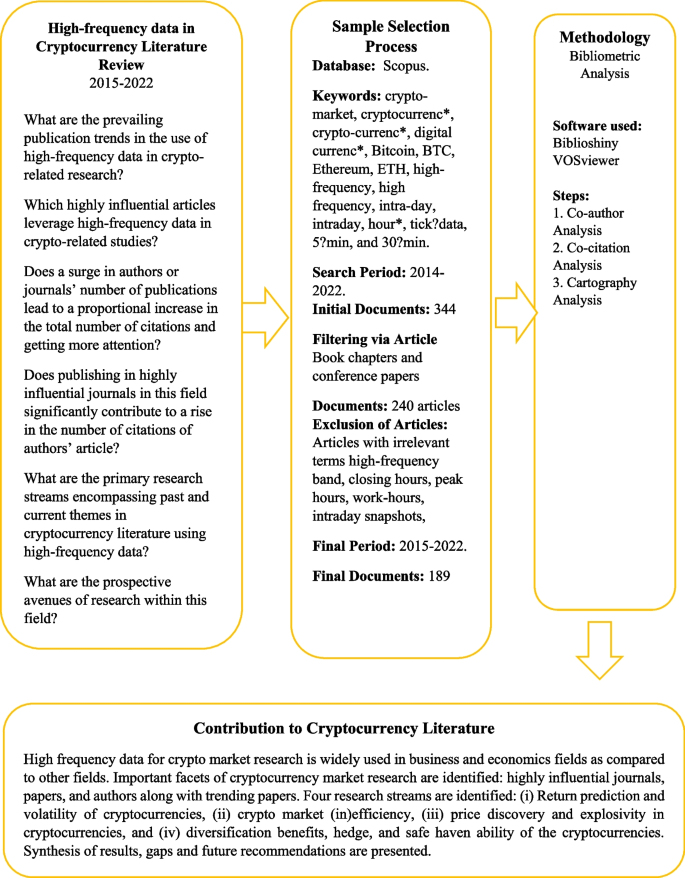
Road map from data extraction to analysis
Bibliometric results
This section reports the bibliometric results: the performance analysis of authors, their affiliated institutions, nations, and publishing journals in the form of citation tables using Biblioshiny and network analysis, including co-citation, co-authorship, and keyword co-occurrence networks generated through VOSviewer.
We explain the function of VOSviewer in network analyses. It is a compelling network visualization tool for structures comprising several elements based on a distance-based vision approach. This creates networks or maps based on the data. Generated networks contain items or nodes representing objects of interest, such as authors, sources, countries, keywords, and lines that demonstrate the connection or relationship between them (every pair of items) in the form of co-citation and co-occurrence (van Eck and Waltman 2019 ). The strength of the individual links was designated as a positive numerical value. This relationship becomes stronger, corresponding to higher values. The sum of the times two items (e.g., authors) are quoted together indicates a link’s strength. The items were grouped into clusters where every node was ascribed to one cluster. The number of resulting clusters depends on clustering parameters, such as the smallest cluster size. This clustering analysis allowed the samples to be engraved into smaller, more homogenous groups that could be recognized and explored. However, attaining the highest homogeneity among separate clusters in our analysis was impossible. The clustering technique requires a relevant and specific algorithm to solve an optimization query or problem (for details see, Waltman et al. 2010 ). In doing so, VOSviewer utilizes a smart local moving algorithm (Waltman and Van Eck 2013 ). Therefore, network analyses were performed using VOSviewer software.
The figures or network diagrams generated through VOSviewer use clustering procedures to allot articles with higher strengths to create results (networks) displayed in different colors. As described earlier, nodes or items represent authors, documents, keywords, etc., and their size signifies highly cited or co-cited literature. Edges represent relations in which the closeness of two nodes indicates a higher strength of the items (e.g., authors) and the co-citations or relations between them. However, they are distinguished from each other based on the variation in their total strength links, weighed-degree (computation is a built-in feature of VOSviewer), or other centrality measures such as degree, betweenness, and closeness centralities (can be computed through Gephi and Pajek). Therefore, the network relationship between the item (authors, documents, etc.) clusters helps determine the strength of the connection among the clusters that create a whole cluster analysis diagram of a discipline, field, or specific topic (Li et al. 2017 ).
Performance analysis
This section presents a performance analysis of the authors, their affiliated institutions, countries, and journals. We also categorize the top-trending and most influential articles published in this field. Furthermore, we examined the impact of the number of authors’ and journals’ publications on their citations, attention, and publishing in influential journals.
Citation analysis
Citation analysis is a widely used method for assessing the impact of a publication (Ding and Cronin 2011 ) through the citation numbers that an article receives from other authors (Zupic and Čater 2015 ). From the 189 sample documents, 86 sources, and 395 authors, we report the top 11 authors, journals, and countries, their respective numbers of articles produced (Table 1 ), and their influence or higher number of citations (Table 2 ) on the subject. We also categorized the highly cited papers (Table 3 ) and top-trending papers (Table 4 ).
Table 1 shows the classification of authors, journals, and affiliated countries at the forefront of publishing high-frequency cryptocurrency market research. For instance, Ahmet Sensoy was the most prolific author in this area, with the most publications. Finance Research Letters is the most frequent publisher of high-frequency cryptocurrency market research, with the United Kingdom being the top-affiliated country.
Table 2 presents the categorization of authors, journals, and countries of affiliation associated with highly cited articles on high-frequency cryptocurrency market research. For instance, Ahmet Sensoy is this field’s most frequently cited author. Finance Research Letters is the most influential journal on this topic, with the United Kingdom being the primary country of affiliation. From this list of papers, we can infer considerable research on cryptocurrency pricing and trading, focusing on Bitcoin. Researchers are exploring topics such as price discovery, volatility forecasting, price dynamics, correlations, inefficiencies, liquidity, transaction costs, and technical trading. This shows that the cryptocurrency market is gaining significant attention from researchers and investors and that high-frequency data and trading techniques are used to gain insight.
Table 3 presents the classification of highly cited or impactful papers that use high-frequency data and cover various topics. For instance, Brandvold et al. ( 2015 ) explored price discovery on Bitcoin exchanges, which has had a major influence on cryptocurrency research using high-frequency data. Urquhart and Zhang ( 2019 ) examined the hedge and safe haven abilities of Bitcoin for currencies using intraday data, which is the second most influential paper on cryptocurrency research that relies on high-frequency data.
This list covers the top ten papers that are cited the most on the subject.
Table 4 classifies the top articles that employ high-frequency data and encompass a variety of topics based on their higher citations per year. Urquhart and Zhang ( 2019 ) are the most trending papers in cryptocurrency research that explore the investment characteristics of Bitcoin as a hedge and safe haven. Chen et al. ( 2020 ) study examining the impact of fear and uncertainty on Bitcoin price dynamics during COVID-19 is the second most widely referenced study. In explaining the impact of articles, it is interesting to note that some articles reported in Table 3 , for example, Dyhrberg et al. ( 2018 ) and Nakano et al. ( 2018 ), are not reported in Table 4 because they have fewer citations per year. Whereas two articles reported in Table 4 , Baur and Hoang ( 2021 ) and Naeem et al. ( 2021 ), are not reported in Table 3 because they are not highly cited, but their per-year citations are higher.
Regression analysis
We use the dataset consisting of authors and journals’ number of publications, the total citations they received for articles, and citations per article to run OLS regressions in which we examine the link between authors and journal publication count to their corresponding citations and attention, as well as influential journals’ role in getting more citations (see Table 5 ).
Table 5 presents the regression results for the three panels. In panel A, we show that an author’s increase in the number of articles significantly increases the number of citations while reducing the citations per article. This implies that publishing may improve the author’s total citation count, but at the same time, it can adversely affect the average number of citations per article. The reduction in average citations per article may result from multiple factors, such as publishing in diverse research areas and variation in the importance of topics. For example, groundbreaking articles may get higher citations and reworking, or articles not aligned with the prevailing research trends cannot receive the same level of attention. This result was generated using the total number of authors’ citations (right panel A of Table 5 ) and citations per article (left panel A of Table 5 ) as dependent variables and the number of publications as a regressor. Similarly, in panel B, we repeat the same regressions with the journals’ number of articles as the independent variable and the journals’ total citations (right panel B of Table 5 ) and average citations per journal (left panel B of Table 5 ) as the dependent variables. We find that an increase in the journals’ number of publications enhances their citations; however, the attention and average citations per journal results are insignificant. Finally, in Panel C, we investigate the impact of publishing in highly influential journals (here, we consider highly cited journals publishing in this field) by running a regression for authors’ total citations (independent variable) and journals’ total citations. The results demonstrate that publishing in journals whose articles are most frequently cited because of publishing on similar topics, fields, or impactful content may increase authors’ citations.
- Network analysis
Co-authorship analysis
Co-authorship analysis enables us to create links between authors, institutions, and countries researching a subject or topic (Van Eck and Waltman 2014 ). In our analysis of co-author networks using high-frequency data in crypto markets, we maintain our criteria at a minimum of five documents per author, which generates a network of 14 authors distributed into four clusters (see Fig. 3 ). The colors of the clusters varied, with Cluster 1 in red, Cluster 2 in green, Cluster 3 in blue, and Cluster 4 in yellow.
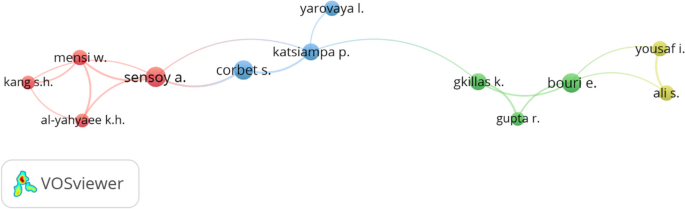
Co-author network of authors researching cryptocurrencies
Keywords co-occurrence analysis
Keywords: words used in a paper to reflect content. The authors mostly index keywords based on the influence of their predecessors and the connections between keywords in the article. When sufficient authors use similar keywords and accept those connections in their articles, they can be used to explore topics or hotspots in a field (Whittaker 1989 ). Co-word analysis reveals patterns or trends in any discipline by measuring the strength of linkages between keywords. In contrast, the co-word network, based on the frequency of their co-occurrences, reflects content similarities or words with similar meanings (Ding et al. 2001 ). We created a keyword co-occurrence network by selecting the authors’ keywords with a minimum of five occurrences to identify the evolution of research flashpoints and embedded relevant topics.
The keyword co-occurrence network generated five clusters (Fig. 4 ). The first cluster in violet focuses on assessing the volatility of cryptocurrencies, specifically Bitcoin, while the second cluster in yellow focuses on the price discovery and market efficiency of Ethereum and the stablecoin, that is, Tether. The third cluster, in red, highlights the role of the volatility and liquidity of cryptocurrencies using high-frequency data. The fourth green cluster focuses on asymmetry in cryptocurrency futures. The fifth cluster in blue shows the safe haven ability of Bitcoin and gold during COVID-19.
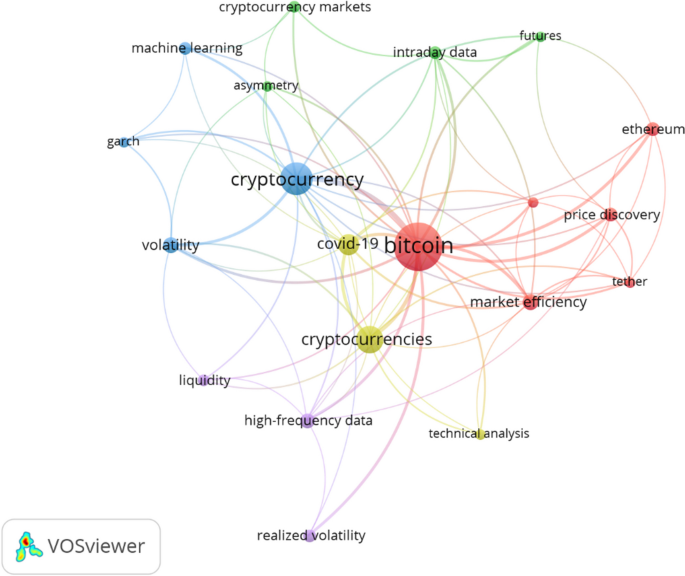
Keywords co-occurrence network of cryptocurrencies
Co-citation analysis
Co-citation analysis reveals the relationship between two papers if they are concurrently cited by a third (Small 1973 ). This shows the relationship by distributing documents with higher strength in the form of a network or clusters using clustering methods. This relationship can be used to examine the commonality, centrality, and progression of various research fields. We performed a co-citation analysis using VOSviewer and selected 20 as the minimum number of citations for an author.
This generated four clusters illustrated in different colors (see Fig. 5 ). Cluster 1 highlights the focus of studies on return predictions and the measurement of volatility in cryptocurrencies. Cluster 2 (green) includes studies focusing on the informational (in)efficiency of cryptocurrencies. Cluster 3 (red) represents the studies on price discovery and speculative bubbles in cryptocurrencies. Cluster 4, in blue, represents research contributing to portfolio diversification, examining the co-movement, spillover, hedge, and safe haven abilities of cryptocurrencies against other assets.
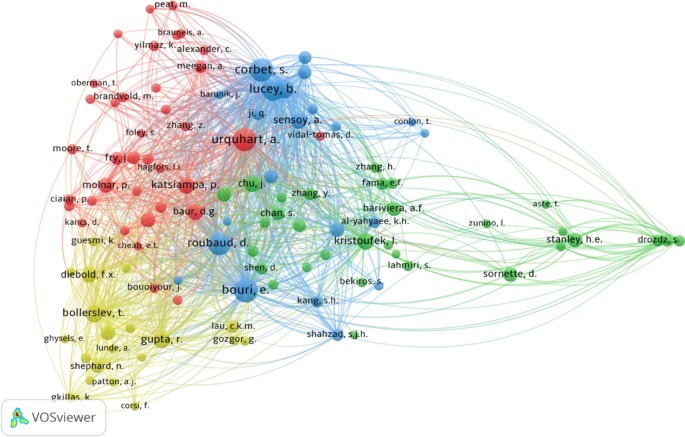
Co-citation network of authors researching cryptocurrencies
Identification of major streams of cryptocurrencies research
We performed content analysis (Potter and Levine-Donnerstein 1999 ) to reveal, arrange, and validate the research streams identified by the bibliometric analysis. This analysis reveals the concepts, classifications, evolution, and current research streams in cryptocurrency literature. Based on this, we identify four major cryptocurrency research streams through a co-citation network of cited authors (Fig. 5 ). Persson ( 1994 ) noted that citing publications constitute a research front, while cited publications form an intellectual base.
Return prediction and volatility in cryptocurrencies
This stream of research focuses on the peculiarities of return prediction and volatility forecasting in cryptocurrencies, emphasizing high-frequency data and various volatility models.
Return prediction and volatility in cryptocurrencies have been studied since the introduction of Bitcoin by Nakamoto ( 2008 ). The volatile nature of cryptocurrencies is attributed to their newness compared with equity markets (Katsiampa 2017 ). Various factors have been found to drive Bitcoin prices, such as searching sites (Kristoufek 2013 ), market forces (Ciaian et al. 2016a ), economic fundamentals (Li and Wang 2017 ), transaction speed (Corbet et al. 2018a ), market attention (Urquhart 2018 ), trading volume (Balcilar et al. 2017 ; Bouri et al. 2019b ), economic policy uncertainty (Demir et al. 2018 ; Fang et al. 2019 ), cryptocurrency liquidity (Wei 2018 ), Google search index (Bleher and Dimpfl 2019 ; Nasir et al. 2019 ), and Twitter tweets (Karalevicius et al. 2018 ; Shen et al. 2019 ). In addition to price, these factors have been found to predict cryptocurrency returns, volume, and volatility. Various models and machine learning techniques, such as long-short-term memory neural networks (Alonso-Monsalve et al. 2020 ; Lahmiri and Bekiros 2019 ; McNally et al. 2018 ) and support vector machines (Akyildirim et al. 2021 ), have been employed to predict cryptocurrency returns. Studies show that cryptocurrency liquidity relies on specific variables and is irrelevant to global financial market variables (Brauneis et al. 2021 ). Studies have also analyzed crypto market liquidity using high-frequency data (e.g., Dyhrberg et al. 2018 ; among others).
After introducing realized volatility based on high-frequency data by Anderson et al. ( 2003 ), and the provision of a framework for its integration into lower frequency return distributions and volatilities (Andersen et al. 2003 ), it is used to study the stochastic properties of returns (Barndorff‐Nielsen and Shephard 2002 ). In doing so, several volatility-based models, i.e., ARCH (Engle 1982 ) and GARCH (Bollerslev 1986 ), jump detection methods within realized volatility by separating bi-power variation and realized variance (Andersen et al. 2007 ; Barndorff-Nielsen and Shephard 2003 , 2004 ; Huang and Tauchen 2005 ), and up-and downside realized measures (semi-variances) entirely based on upward and downward intraday price fluctuations (Barndorff-Nielsen et al. 2006 ). High-frequency data can accurately assess volatility asymmetries over longer daily return horizons (Bollerslev et al. 2006 ). Subsequently, methods for filtering out rare and large jumps were introduced (Tauchen and Zhou 2011 ). However, volatility or volatility jumps can be predicted by several factors, such as geopolitical risk (Gkillas et al. 2018 ). Moreover, very good and bad news have diverse impacts on volatility. For example, relatively good (intra-daily) news reduces future or next-day volatility (Chen and Ghysels 2011 ). The prediction of Bitcoin’s realized volatility depends on historical realized volatilities and jumps (Qiu et al. 2021 ). For instance, Patton and Sheppard ( 2015 ) argue that future volatility highly depends on the volatility of past negative returns and that the sign of the jump matters for the impact of price jumps on volatility, such as positive (negative) jumps lower (raise) future volatility. Moreover, co-jumping behavior in the volatility of cryptocurrencies may spark future jumps (Gkillas et al. 2022 ).
Corsi ( 2009 ) recommends the HAR-RV model as a better method to reproduce the key empirical properties of financial returns (self-similarity, long memory, and fat tails). However, several studies (e.g., Dyhrberg 2016a ; Guesmi et al. 2019 ; Katsiampa 2017 ; Yu 2019 ; among others) examine the return prediction and volatility of cryptocurrencies using other volatility models (e.g., Bergsli et al. 2022 ; Chu et al. 2017 ; Diebold and Yılmaz 2012 , 2014 ; Hansen et al. 2012 ; among others). Studies also examine volatility cascades in cryptocurrencies against the US dollar (Gradojevic and Tsiakas 2021 ) and volatility spillover among cryptocurrencies (e.g., Yousaf and Ali 2020 ).
High-frequency data are an efficient way to predict cryptocurrency returns (Vidal-Tomás 2022 ). Volume and frequency are inversely proportional (Yarovaya and Zięba 2022 ). Thus, future research topics include detecting price manipulation in cryptocurrencies at the intraday level, using feature selection techniques to improve accuracy, analyzing the impact of hard and soft forks on intraday reversal and momentum, assessing time-based momentum strategies during price crashes and sharp recovery using high-frequency data (Wen et al. 2022 ), and examining causal patterns between Bitcoin and other cryptocurrencies. Additionally, the volume-return relationship of cryptocurrencies (Balcilar et al. 2017 ; Yarovaya and Zięba 2022 ) can be examined with the inclusion of seasonality and volatility effects.
Similarly, using machine learning approaches such as recurrent neural networks and long short-term memory, new research can predict Bitcoin’s intraday price dynamics and identify its drivers (e.g., online sentiment and media hype). Furthermore, we provide prospective avenues for researchers in this field. For instance, Gkillas et al. ( 2020 ) suggest examining the macrofinancial determinants of crypto market jumps, and Grobys and Huynh ( 2022 ) propose assessing the impact of jumps in stablecoins. Gkillas et al. ( 2022 ) suggest assessing the impact of cryptocurrencies and the jumping behavior of other financial assets (upside, downside, and large and small jumps). Finally, volatility forecasting requires models with different data frequencies. The in-sample and out-of-sample forecasts can be reconciled at the short-, medium-, and long-term horizons.
(In)efficiency in the cryptocurrency market
Studies have identified various factors and developed different models or approaches that can predict cryptocurrency prices, challenging the efficient market hypothesis (all information is already reflected in prices). Research on cryptocurrency price prediction has led to an investigation of the efficiency of the cryptocurrency market based on the efficient market hypothesis. Increased liquidity makes cryptocurrencies inefficient and less predictable (Brauneis and Mestel 2018 ).
Similar to other assets, stylized facts such as fat-tailed distributions, volatility clustering, long memory features (Bariviera 2017 ; Bariviera et al. 2017 ; Chu et al. 2015 ; Cont 2001 ; Takaishi 2018 ; Urquhart 2017 ), adaptive patterns of long memory (Khuntia and Pattanayak 2020 ), interactions among returns, volatility, volume, and bid-ask spread (Eross et al. 2019 ), and asymmetric relations with price trends are observed in cryptocurrencies (Nadarajah and Chu 2017 ). There is also evidence of strong asymmetric reverting behavior in Bitcoin for negative returns, whereas excessive levels of persistence are revealed for positive returns (Corbet and Katsiampa 2020 ). In a similar vein, studies examine and analyze the microstructure properties or their impact on cryptocurrencies (e.g., Scaillet et al. 2020 ), stylized facts including multifractal properties (Stavroyiannis et al. 2019 ) by utilizing high-frequency data and different methods such as volatility autocorrelation, multi scaling effects, etc., (Drożdż et al. 2018 ), including Detrended Fluctuation Analysis, and Hurst exponents (Zhang et al. 2019a ), and herding behavior during the COVID-19 pandemic and other different situations (e.g., Choi et al. 2022 ; Jalal et al. 2020 ; Mandaci and Cagli 2022 ; Yarovaya et al. 2021 ). Moreover, Bitcoin may respond more to negative than positive news (Fang et al. 2019 ). Negative market sentiment predicts bitcoin returns, volatility, trading volumes, and jumps. For instance, bitcoin returns and volatility fall in response to news relevant to crypto fraud and cyberattacks (Rognone et al. 2020 ). Moreover, evidence indicates that behavioral patterns in Bitcoin-related Google searches and tweets amplify herding, whereas EPU patterns and dependence between foreign exchange and equity markets dampen herding (Philippas et al. 2020 ). Thus, events like the COVID-19 pandemic may steer cryptocurrency markets away from weak-form efficiency, making it possible to predict future cryptocurrency prices based on past information. Furthermore, long-range dependence on cryptocurrency price returns and their amplification during the COVID-19 period points to inefficiency (Naeem et al. 2021 ).
In efficient markets (Fama 1970 ), past information should be reflected in prices; however, Bitcoin’s anti-persistence behavior (Takaishi 2021 ) indicates a frequent reversal in its moving direction, and asymmetric volatility induces inefficiency (Kristoufek 2018 ; Phillip et al. 2018 ), thereby increasing the possibility of achieving abnormal profits (Caporale et al. 2018 ). However, Bitcoin has predictive information for the price trends of other cryptocurrencies (Wang and Ngene 2020 ). To study the efficiency of crypto markets, several approaches, such as the Hurst exponent (Carbone et al. 2004 ), multifractal analysis (Drożdż et al. 2010 ; Jiang et al. 2018 ), wavelet and detrended fluctuation analysis (Oświȩcimka et al. 2006 ), detrending analysis (Oświȩcimka et al. 2014 ), and multifractal detrended analysis (Kantelhardt et al. 2002 ) have been employed for various assets (Jiang et al. 2019 ; Wang and Wang 2021 ) and or cryptocurrencies (Alvarez-Ramirez et al. 2018 ; Aslan and Sensoy 2020 ; Bariviera 2017 ; da Silva Filho et al. 2018 ; Dimitrova et al. 2019 ; Kristoufek 2018 ; Takaishi and Adachi 2020 ; Takaishi 2018 ; Urquhart 2016 ; Zhang et al. 2018 ).
Along with the approaches used to study the multifractal properties of Bitcoin, efficiency in the cryptocurrency market is examined through benchmark currency, varying sampling frequency, etc. (Bariviera 2017 ; Chu et al. 2019 ; Khuntia and Pattanayak 2018 ; Jiang et al. 2018 ; Sensoy 2019 ; Tiwari et al. 2018 ; Vidal-Tomás and Ibañez 2018 ). Despite the various perspectives, the primary conclusion of this study is that Bitcoin is inefficient (Kristoufek 2018 ; Mensi et al. 2019 ; Nadarajah and Chu 2017 ; Urquhart 2016 ) but may achieve weak-form efficiency over time (Chu et al. 2019 ; Gradojevic et al. 2022 ). Based on evidence of increased economic value and trading anomalies during the COVID-19 pandemic, Wen et al. ( 2022 ) suggest that traders can form a profitable strategy based on historical intra-day returns, leading to an inefficient cryptocurrency market.
However, as previous studies have examined herding behavior in bitcoin futures markets (e.g., Blasco et al. 2022 ), investigating stylized facts and empirical results of cryptocurrency futures and spot markets is important. Similarly, herding effects and their intensity should be studied across countries and markets while considering the impact of distinct factors. Examining the macro-financial, sentiment, psychological, and uncertainty determinants of herding behavior in the cryptocurrency market can provide detailed insights. The efficiency of cryptocurrencies in different regimes (upward and downward trends and asymmetric behavior) should be assessed, and the relative efficiency of other assets with cryptocurrencies should be compared. Variations in persistence and market efficiency should be evaluated during the outbreak of COVID-19 and the Ukraine-Russia War, and the anomalies and efficiency of more and less liquid cryptocurrencies should be assessed. Furthermore, as Dong et al. ( 2022 ) suggest, anomalous returns (displaying long or short legs) differ from those identified in equity markets, and the fundamental economic mechanisms of market anomalies can be explored in future research.
Price discovery and speculative bubbles in cryptocurrencies
In the preceding section, we discussed how the EMH and asset pricing theory follow the assumption that asset prices move around or mean revert to their fundamental value. The presence of explosiveness or bubbles in asset prices indicates a significant departure from their fundamental values. Similarly, studies have attempted to predict bitcoin prices through different approaches, such as the network-based properties of blockchain or bitcoin transaction graphs (e.g., Greaves and Au 2015 ). However, according to Tiwari et al. ( 2018 ) and Urquhart ( 2016 ), Bitcoin’s fundamental value is zero, and individual investors drive the market such that noise traders can distort the price discovery mechanism, leading to inefficiencies. Therefore, in addition to return and volatility forecasting, price explosiveness or bubbles in the cryptocurrency market indicate inefficiency. Moreover, high-frequency data has proven to be a valuable tool for examining price formation, discovery, and speculative bubbles.
This research stream indicates that the literature on cryptocurrencies is vast, with a range of studies exploring their higher volatility than conventional currencies (e.g., Katsiampa 2017 ; Dwyer 2015 ) and internally-driven nature (e.g., Baek and Elbeck 2015 ), making it highly speculative (Yermack 2015 ). However, some argue that Bitcoin is a currency or synthetic commodity money with monetary value, not a speculative investment (Blau 2017 , 2018 ; Selgin 2015 ), although it possesses both speculative and financial asset properties (Kristoufek 2015 ) and competes with other cryptocurrencies (Gandal and Halaburda 2016 ). By studying Bitcoin’s price formation mechanism, Ciaian et al. ( 2016b ) report that speculators influence Bitcoin prices but that macroeconomic factors only affect them in the short term. Subsequently, Ciaian et al. ( 2018 ) argue that Bitcoin’s transactions and speculative demand significantly impact its price formation. Meanwhile, Hu et al. ( 2019 ) suggest that prices usually form through negotiations and strategic trading.
However, price deviation across exchanges varies (Brandvold et al. 2015 ), with price discovery driven by shocks (Makarov and Schoar 2020 ). Using data from several exchanges, Brandvold et al. ( 2015 ) were the first to examine Bitcoin’s price discovery mechanism by applying the econometric approaches of Hasbrouck ( 1995 ) and Gonzalo and Granger ( 1995 ). They argue that the exchanges with the highest trading volumes are pronounced price leaders concerning information sharing and find the United States BTC-e and Japan’s Mtgox to be price setters or leaders. Pagnottoni and Dimpfl ( 2019 ) find that price discovery largely takes place on China-based OKcoin, Baur and Dimpfl ( 2019 ), Corbet et al. ( 2018b ) find that spot prices lead the process, and Fassas et al. ( 2020 ) and Kapar and Olmo ( 2019 ) find that Bitcoin futures are dominant. Giudici and Polinesi ( 2021 ) and Giudici and Pagnottoni ( 2020 ) find Bitstamp, Coinbase, and Bitfinex to lead and Kraken as a follower exchange. Chang and Shi ( 2020 ) found Bitcoin to be a highly dominant cryptocurrency driving price discovery at the market level.
In addition to examining the short-term effects of speculators and macroeconomic factors, researchers have examined the endogeneity of price dynamics and the potential for bubbles. For example, the endogeneity of price dynamics is an important concept for understanding the dynamics of price bubbles because it explains how investors’ expectations can drive prices. The endogeneity of price dynamics is the idea that investors’ expectations of future prices are based on current prices. This can lead to a feedback loop of rising prices resulting from increased expectations of future prices. Price behavior in price discovery and bubbles in the Bitcoin market are two sides of the debate. Thus, explosiveness distorts the price discovery process. Turanova ( 2017 ) holds that Bitcoin’s price discovery mechanism is unlikely to operate without an extreme price rise, suggesting its true value is higher than its current level. By contrast, Ché and Fry ( 2015 ) argue that the intrinsic value of a cryptocurrency is zero and that its exponential growth is a bubble. As discussed earlier, Bouoiyour and Selmi ( 2015 ), Bouoiyour et al. ( 2016 ), Corbet et al. ( 2018a ) and Kristoufek ( 2013 ) consider Bitcoin a speculative bubble rather than a currency.
Fry and Cheah ( 2016 ) find a negative bubble in Bitcoin and Ripple, with Ripple’s spillover intensifying price drops in Bitcoin. Fry ( 2018 ) identifies bubbles in Bitcoin and Ethereum and argues that boom-bust cycles could occur without bubbles. Cheung et al. ( 2015 ) identified multiple transitory and three major bubbles in Bitcoin, with bursts corresponding to major events in the market. Bariviera et al. ( 2018 ) applied permutation information theory quantifiers to study the high-frequency price dynamics of 12 cryptocurrencies during a bubble and bust in the starting month of 2018. They found that cryptocurrencies demonstrate distinct dynamics, indicating they are not homogenous assets. Corbet et al. ( 2018a ) reported Bitcoin to be in the bubble phase after crossing a $1000 value. Bouri et al. ( 2019a ) examine the link between explosive periods in the cryptocurrency market and find multidirectional co-explosivity, not necessarily from older to newer or larger to smaller markets.
There is a space for future research to study the relative price discovery of stablecoins, gold-backed cryptocurrencies, and tokens. These assets can contribute to price discovery and the crypto market efficiency. Moreover, the degree of price deviation and integration between these digital currencies during the onset of specific events, such as COVID-19 and the Ukraine-Russia war, may help broaden the understanding of overall cryptocurrency market behavior. As proposed by Su et al. ( 2022 ), it is important to examine the dramatic variations in the price discovery of Bitcoin at the intraday level in the post-COVID period. The explosivity or bubbles in cryptocurrency markets (Cheung et al. 2015 ), co-explosivity (Bouri et al. 2019a ), price explosiveness, and the role of tweets (Shahzad et al. 2022 ) have been examined. However, the bubble contagion or spillover between cryptocurrencies and other financial assets can also be examined. The impact of supply and demand on the boom and bust of bubbles can be analyzed, along with using supply and demand graphs to simulate boom and bust periods. The connection and coherency of pre- and post-price explosiveness in the crypto market would be another avenue for future research, as information dynamics tools involving the microstructure in the cryptocurrency market may provide early warning signals of crashes. As Vasiliauskaite et al. ( 2022 ) suggest, the price and liquidity information dynamics concerning the microstructure in times of price explosiveness can be examined in the future.
Diversification, hedge, and safe-haven assets
In times of crisis, economic and financial shocks distort return predictability and price discovery processes. Smaller cryptocurrencies may start following larger ones (Vidal-Tomás et al. 2019 ), herding behavior tends to increase with uncertainty (Bouri et al. 2019c ), and increasing prices can create a potential market bubble that can burst anytime. Additionally, in the cryptocurrency market, cryptocurrencies portray different patterns of volatility spillover owing to the difference in the structure of return and volatility clusters among them (Sensoy et al. 2021 ). The highly market cap cryptocurrencies, such as Bitcoin, Ethereum, and Litecoin, act as both transmitters and receivers of volatility in the crypto market (Polat and Kabakçı Günay 2021 ), while low cap ones, such as Maidsafe Coin may also act as its transmitters (Yi et al. 2018 ). This shock transmission results in lower diversification benefits. In such differing situations, investors start adjusting their investments and opt for portfolio optimization or hedging strategies. In particular, during periods of downturn, rising demand for hedging and safe haven assets may drive up their prices, leading to inefficiency in the market. Moreover, for the cryptocurrency market, several factors, such as the price formation process and limited liquidity, may lead to unreliable safe haven assets (Smales 2019 ).
This research stream discusses the cryptocurrency literature, which includes cryptocurrencies in financial asset portfolios as diversifiers, hedges, and safe havens. In addition to the daily interdependencies within cryptocurrency markets (e.g., Ciaian and Rajcaniova 2018 ), studies have examined diversification opportunities using high-frequency data (e.g., Katsiampa et al. 2022 ; Sifat et al. 2019 ; Yarovaya and Zięba 2022 ). Bitcoin investment possesses the most distinctive features: remarkably high average returns and volatility and a lower correlation with other financial assets. Its inclusion, even in a small fraction, dramatically improves the portfolio’s risk-return trade-off (Brière et al. 2015 ) and diversifies the portfolios (Guesmi et al. 2019 ; Platanakis and Urquhart 2020 ). As a financial asset, it is a useful instrument for portfolio, risk management, and market sentiment analysis based on its value-storing and exchanging benefits (Dyhrberg 2016a ). Cryptocurrencies offer diversification benefits over other financial assets in short-term investment horizons (Corbet et al. 2018c ). Similarly, Guesmi et al. ( 2019 ) report that including Bitcoin, gold, emerging equity markets, and oil in a portfolio can significantly decrease portfolio risk. Bitcoin offers income hedging as well.
Bitcoin displays hedging abilities like gold against market risk (Dyhrberg 2016b ). It can act as a diversifier and poor hedge for the US dollar, stock, bond, gold, oil, and commodity markets. In contrast, its safe haven properties are especially visible in extreme downward movements in Asian stock markets. Data frequency matters to Bitcoin investors as its properties vary across time horizons (Bouri et al. 2017a ). It acts as a strong hedge and safe haven against fluctuations in commodity market indices (Bouri et al. 2017b ). However, Klein et al. ( 2018 ) find no hedging ability against a decline in equity prices, and it can hedge against uncertainties (Bouri et al. 2017c ). In intraday trading, Urquhart and Zhang ( 2019 ) find that it can diversify the risk of the Japanese yen and Australian and Canadian dollars; hedge against the euro, Swiss franc, and pound; and act as a safe haven for the Swiss franc, Canadian dollar, and pound. Shahzad et al. ( 2019 ) find a weak safe haven ability of Bitcoin, commodities, and gold against global stock markets, with varying properties across equity market indices. Shahzad et al. ( 2020 ) find distinctive safe haven and hedge properties of Bitcoin and gold in G7 stock markets. Bouri et al. ( 2020a ) find evidence that Bitcoin, Ripple, and Stellar are safe havens for US equity indices, whereas Monero and Litecoin do so for the US equity market index. Bitcoin has the least dependence on other assets and a superior safe-haven ability compared to gold and commodities (Bouri et al. 2020b ).
Recently, investor concerns over the COVID-19 outbreak have led to research on the role of Bitcoin as a safe haven asset. Corbet et al. ( 2020a ) find that investors are more inclined to view digital currency as a safe haven. However, a few studies (e.g., Conlon and McGee 2020 ; Corbet et al. 2020b ) suggest cryptocurrencies may even increase contagion. Unlike Tether, Conlon et al. ( 2020 ) indicate that Bitcoin and Ethereum do not act as safe havens for most equity markets. Yousaf and Ali ( 2020 ) reveal that the portfolios of Bitcoin, Litecoin, and Ethereum are costlier and more effective in hedging during the pandemic. Finally, Das et al. ( 2020 ) conclude that Bitcoin is better at hedging crude oil uncertainty than commodities and gold but not the US dollar.
It is possible to examine liquidity spillovers in cryptocurrencies to identify diversification benefits. This behavior can also be examined across assets. Diversification opportunities can be identified by examining the linkages among cryptocurrencies, meme stocks, and rare-earth stocks, considering various investment horizons. Since COVID-19 and its induced financial market turbulence have been the initial tests of the safe haven ability of Bitcoin (Kristoufek 2020 ), it is important to assess the safe haven property of Bitcoin by considering the differential impacts of the intensity and type of crisis. Another possible line of future research could be the assessment of the hedging effectiveness and safe haven ability of Bitcoin and other cryptocurrencies in the recovery phase of the COVID-19 pandemic and during the Russia-Ukraine War. The impact of health- and war-induced crises may provide new insights, depending on the nature of the events. Gkillas et al. ( 2020 ) suggest the inclusion of Bitcoin, gold, and oil in a single portfolio to analyze diversification benefits while studying the spillover effects of skewness, kurtosis, and jumps. Baur and Hoang ( 2021 ) find that the six largest stablecoins act as safe havens against Bitcoin, and Baumöhl and Vyrost ( 2020 ) find a few stable coins that act as safe havens against other top cryptocurrencies. There are many prospective avenues for examining the safe haven ability of stable coins and tokens (compared with unstable cryptocurrencies) against other assets. The symmetric or asymmetric multifractality and detrended correlation analysis of safe haven assets, including cryptocurrencies, stablecoins, and other financial assets, is a potential topic to explore. Since cryptocurrencies are becoming less influential, except Bitcoin, dApps, and Protocols, which are receiving much attention from investors (Katsiampa et al. 2022 ), upcoming research can focus on these distinctive cryptocurrencies in their market analysis.
Avenues of future research
Research opportunities opened by cryptocurrencies and Bitcoin in the scientific domain have prompted an in-depth examination of their future paths and influences. Various digital currencies, such as stablecoins, altcoins, and gold-backed cryptocurrencies, combined with the gradual progress of legalization in some countries, have led researchers to explore the financial properties of these currencies.
In this section, we offer a few future research questions that do not unconditionally fit into one of the four previously mentioned research streams: What are the causes of the cryptocurrency market crash in 2022? How do investors perceive the cryptocurrency market’s future? Is there a need to revisit pre-crash research findings empirically? How do Bitcoin’s hard and soft forks affect intraday reversal and momentum? What are the causal patterns of Bitcoin and other cryptocurrencies? What is the impact of changing regulations on the cryptocurrency market? What is the impact of an increase in decentralized finance (DeFi) on the cryptocurrency market? What is the impact of institutional investors on the cryptocurrency market? What effects do cryptocurrency derivatives have on the cryptocurrency market? What is the impact of news events on the cryptocurrency market? What are the effects of cryptocurrency mining on the cryptocurrency markets? What are the environmental impacts of cryptocurrency mining? What pricing models are used in cryptocurrency exchanges? What is the role of social media in influencing the cryptocurrency market? What are the effects of algorithmic trading on the cryptocurrency markets? What are the effects of “whales” on cryptocurrency markets? What challenges do stakeholders in the crypto market face, and how can they overcome them?
Conclusions
In recent years, the intensified use of high-frequency data has revolutionized the analysis of cryptocurrencies, which are now actively traded 24/7 across global markets. We adopted a comprehensive bibliometric technique, leveraging co-citation and keyword co-occurrence analyses of 189 pertinent papers extracted from multidisciplinary domains in the Scopus database from 2015 to 2022. Our findings illuminate the expanding literature within the cryptocurrency market and shed light on leading articles, eminent authors, prominent institutions, reputable journals, and influential countries actively contributing to this domain.
Through a meticulous analysis of a bibliometric network and a thorough content assessment of scholarly articles, we identify and delineate four primary research streams that shape the discourse within the cryptocurrency market. These include studies focused on (1) examining the intricate dynamics of return and volatility behavior, encompassing predictive models and the phenomenon of abrupt fluctuations within cryptocurrencies; (2) analyzing the inherent inefficiencies within the cryptocurrency landscape; (3) investigating the mechanisms of price discovery, including its formation, potential manipulation, and the emergence of speculative bubbles within cryptocurrency markets; and (4) evaluating the diversification benefits, hedging strategies, and safe haven properties, particularly on the flagship cryptocurrency Bitcoin.
In our analysis, we meticulously pinpointed the critical gaps and unexplored avenues within each research stream, thereby providing scholars with comprehensive insights into potential future directions for research utilizing high-frequency data in the cryptocurrency domain. It is worth noting that, as Zha et al. ( 2020 ) highlighted, the study of investor behavior in the context of cryptocurrencies necessitates a multidimensional and integrated interdisciplinary approach. Therefore, the trajectory of cryptocurrency research transcends the confines of any single discipline, necessitating collaboration across diverse academic fields and methodologies.
It is imperative to acknowledge that while this study offers significant insights into research conducted using high-frequency data on the cryptocurrency market, it is constrained by its reliance on the Scopus database and its focus on the 24/7 trading nature of cryptocurrencies. Moving forward, the scope of this study can be expanded to encompass a broader spectrum of financial assets and alternate databases, such as the Web of Science and SpringerLink, to capture a holistic view of the evolving landscape within the realm of digital financial assets. As bibliometric studies continue to serve as influential tools for highlighting the growing impact of scholarly works and journals within specific domains, our research lays a strong foundation for further exploration and advancement in the realm of cryptocurrency research and analysis.
The insights gained from our comprehensive analysis of high-frequency data in the cryptocurrency market have significant practical and policy implications for stakeholders. As the cryptocurrency landscape continues to expand and evolve, investors, financial regulators, and market participants can leverage identified research streams and trends to make informed decisions, manage risks, and develop robust strategies that capitalize on the unique properties of digital assets. Policymakers can use their findings to establish a more comprehensive regulatory framework that addresses the intricacies of the cryptocurrency market, thereby fostering transparency, stability, and investor protection. By embracing emerging research trends and addressing the highlighted gaps, stakeholders can foster a more resilient and secure cryptocurrency ecosystem aligned with the evolving demands of global financial markets. In addition, our study underscores the significance of interdisciplinary collaboration and the integration of diverse analytical tools in the study of cryptocurrency dynamics, underscoring the need for a cohesive and interdisciplinary approach that incorporates insights from fields such as economics, finance, computer science, and data analytics to ensure a holistic understanding of the multifaceted nature of the cryptocurrency market.
Availability of data and materials
The data is available from the authors on request.
Cryptography word originated from military and different intelligence agencies those employ various codes to protect the leakage of information, for further details along with advantages and disadvantages of cryptocurrencies see, Bunjaku et al. ( 2017 ).
Abbreviations
Total publications of an author are a measure of the academic output of a researcher.
Total number of publications of a source or journal
Affiliated countries or a country from which the publishing author is affiliated
Total number of publications of authors affiliated with a country that is used as a metric to gauge the research and academic output of that country
Total citations of an author are a measure of the impact of a researcher.
Total number of citations of a source or journal
Total number of citations of authors affiliated with a country that is used as a metric to gauge the research impact of that country.
Total citations of journals
Citations per journal
Aalborg HA, Molnár P, de Vries JE (2019) What can explain the price, volatility and trading volume of Bitcoin? Finance Res Lett 29:255–265
Akyildirim E, Goncu A, Sensoy A (2021) Prediction of cryptocurrency returns using machine learning. Ann Oper Res 297(1):3–36
Article MathSciNet Google Scholar
Alexander C, Dakos M (2020) A critical investigation of cryptocurrency data and analysis. Quant Finance 20(2):173–188
Alexander C, Heck DF, Kaeck A (2022) The role of binance in bitcoin volatility transmission. Appl Math Finance 29(1):1–32
Alonso-Monsalve S, Suárez-Cetrulo AL, Cervantes A, Quintana D (2020) Convolution on neural networks for high-frequency trend prediction of cryptocurrency exchange rates using technical indicators. Expert Syst Appl 149:113250
Article Google Scholar
Alvarez-Ramirez J, Rodriguez E, Ibarra-Valdez C (2018) Long-range correlations and asymmetry in the Bitcoin market. Physica A 492:948–955
Article ADS Google Scholar
Ampah JD, Yusuf AA, Afrane S, Jin C, Liu H (2021) Reviewing two decades of cleaner alternative marine fuels: towards IMO’s decarbonization of the maritime transport sector. J Clean Prod 320:128871
Article CAS Google Scholar
Andersen TG, Bollerslev T, Diebold FX (2007) Roughing it up: Including jump components in the measurement, modeling, and forecasting of return volatility. Rev Econ Stat 89(4):701–720
Andersen TG, Bollerslev T, Diebold FX, Labys P (2003) Modeling and forecasting realized volatility. Econometrica 71(2):579–625
Anderson TG, Bollerslev T, Diebold FX, Labys P (1999) The distribution of exchange rate volatility, vol. 6961. National Bureau of Economic Research
Aria M, Cuccurullo C (2017) bibliometrix: an R-tool for comprehensive science mapping analysis. J Informet 11(4):959–975
Arouxet MB, Bariviera AF, Pastor VE, Vampa V (2022) Covid-19 impact on cryptocurrencies: evidence from a wavelet-based Hurst exponent. Physica A 596:127170
Aslan A, Sensoy A (2020) Intraday efficiency-frequency nexus in the cryptocurrency markets. Financ Res Lett 35:101298
Baek C, Elbeck M (2015) Bitcoins as an investment or speculative vehicle? A first look. Appl Econ Lett 22(1):30–34
Bakar NA, Rosbi S, Uzaki K (2017) Cryptocurrency framework diagnostics from Islamic finance perspective: a new insight of Bitcoin system transaction. Int J Manag Sci Bus Adm 4(1):19–28
Google Scholar
De Bakker FG, Groenewegen P, Den Hond F (2005) A bibliometric analysis of 30 years of research and theory on corporate social responsibility and corporate social performance. Bus Soc 44(3):283–317
Balcilar M, Bouri E, Gupta R, Roubaud D (2017) Can volume predict Bitcoin returns and volatility? A quantiles-based approach. Econ Model 64:74–81
Ballis A, Verousis T (2022) Behavioural finance and cryptocurrencies. Review of Behavioral Finance , (ahead-of-print)
Bariviera AF (2017) The inefficiency of Bitcoin revisited: a dynamic approach. Econ Lett 161:1–4
Bariviera AF, Basgall MJ, Hasperué W, Naiouf M (2017) Some stylized facts of the Bitcoin market. Physica A 484:82–90
Bariviera AF, Merediz-Solà I (2021) Where do we stand in cryptocurrencies economic research? A survey based on hybrid analysis. J Econ Surv 35(2):377–407
Bariviera AF, Zunino L, Rosso OA (2018) An analysis of high-frequency cryptocurrencies prices dynamics using permutation-information-theory quantifiers. Chaos Interdisc J Nonlinear Sci 28(7)
Barndorff-Nielsen OE, Shephard N (2002) Econometric analysis of realized volatility and its use in estimating stochastic volatility models. J R Stat Soc Ser B (statistical Methodology) 64(2):253–280
Barndorff-Nielsen OE, Shephard N (2003) Realized power variation and stochastic volatility models. Bernoulli 9(2):243–265
Barndorff-Nielsen OE, Shephard N (2004) Power and bipower variation with stochastic volatility and jumps. J Financ Economet 2(1):1–37
Barndorff-Nielsen OE, Shephard N (2006) Econometrics of testing for jumps in financial economics using bipower variation. J Financ Economet 4(1):1–30
Baumöhl E, Vyrost T (2020) Stablecoins as a crypto safe haven? Not all of them!. http://hdl.handle.net/10419/215484
Baur DG, Dimpfl T (2019) Price discovery in bitcoin spot or futures? J Futur Mark 39(7):803–817
Baur DG, Hoang LT (2021) A crypto safe haven against Bitcoin. Financ Res Lett 38:101431
Bergsli LØ, Lind AF, Molnár P, Polasik M (2022) Forecasting volatility of Bitcoin. Res Int Bus Financ 59:101540
Blasco N, Corredor P, Satrústegui N (2022) The witching week of herding on bitcoin exchanges. Financial Innov 8(1):1–18
Blau BM (2017) Price dynamics and speculative trading in bitcoin. Res Int Bus Financ 41:493–499
Blau BM (2018) Price dynamics and speculative trading in Bitcoin. Res Int Bus Financ 43:15–21
Bleher J, Dimpfl T (2019) Today I got a million, tomorrow, I don’t know: on the predictability of cryptocurrencies by means of Google search volume. Int Rev Financ Anal 63:147–159
Bollerslev T (1986) Generalized autoregressive conditional heteroskedasticity. J Econom 31(3):307–327
Bollerslev T, Litvinova J, Tauchen G (2006) Leverage and volatility feedback effects in high-frequency data. J Financ Economet 4(3):353–384
Borri N (2019) Conditional tail-risk in cryptocurrency markets. J Empir Financ 50:1–19
Bouoiyour J, Selmi R, Tiwari AK, Olayeni OR (2016) What drives Bitcoin price. Econ Bull 36(2):843–850
Bouoiyour J, Selmi R (2015) What does Bitcoin look like?. Ann Econ Finance 16(2)
Bouri E, Gupta R, Roubaud D (2019c) Herding behaviour in cryptocurrencies. Financ Res Lett 29:216–221
Bouri E, Gupta R, Tiwari AK, Roubaud D (2017c) Does Bitcoin hedge global uncertainty? Evidence from wavelet-based quantile-in-quantile regressions. Financ Res Lett 23:87–95
Bouri E, Jalkh N, Molnár P, Roubaud D (2017b) Bitcoin for energy commodities before and after the December 2013 crash: diversifier, hedge or safe haven? Appl Econ 49(50):5063–5073
Bouri E, Lau CKM, Lucey B, Roubaud D (2019b) Trading volume and the predictability of return and volatility in the cryptocurrency market. Financ Res Lett 29:340–346
Bouri E, Molnár P, Azzi G, Roubaud D, Hagfors LI (2017a) On the hedge and safe haven properties of Bitcoin: Is it really more than a diversifier? Financ Res Lett 20:192–198
Bouri E, Shahzad SJH, Roubaud D (2019a) Co-explosivity in the cryptocurrency market. Financ Res Lett 29:178–183
Bouri E, Shahzad SJH, Roubaud D (2020a) Cryptocurrencies as hedges and safe-havens for US equity sectors. Q Rev Econ Finance 75:294–307
Bouri E, Shahzad SJH, Roubaud D, Kristoufek L, Lucey B (2020b) Bitcoin, gold, and commodities as safe havens for stocks: New insight through wavelet analysis. Q Rev Econ Finance 77:156–164
Brandvold M, Molnár P, Vagstad K, Valstad OCA (2015) Price discovery on Bitcoin exchanges. J Int Finan Markets Inst Money 36:18–35
Brauneis A, Mestel R (2018) Price discovery of cryptocurrencies: Bitcoin and beyond. Econ Lett 165:58–61
Brauneis A, Mestel R, Riordan R, Theissen E (2022) Bitcoin unchained: determinants of cryptocurrency exchange liquidity. J Empir Financ 69:106–122
Brauneis A, Mestel R, Theissen E (2021) What drives the liquidity of cryptocurrencies? A long-term analysis. Finance Res Lett 39:101537
Brière M, Oosterlinck K, Szafarz A (2015) Virtual currency, tangible return: portfolio diversification with bitcoin. J Asset Manag 16(6):365–373
Bunjaku F, Gjorgieva-Trajkovska O, Miteva-Kacarski E (2017) Cryptocurrencies–advantages and disadvantages. J Econ 2(1):31–39
Böhme R, Christin N, Edelman B, Moore T (2015) Bitcoin: Economics, technology, and governance. J Econ Perspect 29(2):213–238
Caporale GM, Gil-Alana L, Plastun A (2018) Persistence in the cryptocurrency market. Res Int Bus Financ 46:141–148
Caporale GM, Kang WY, Spagnolo F, Spagnolo N (2021) Cyber-attacks, spillovers and contagion in the cryptocurrency markets. J Int Finan Markets Inst Money 74:101298
Carbone A, Castelli G, Stanley HE (2004) Time-dependent Hurst exponent in financial time series. Physica A 344(1–2):267–271
Article MathSciNet ADS Google Scholar
Carrick J (2016) Bitcoin as a complement to emerging market currencies. Emerg Mark Financ Trade 52(10):2321–2334
Chan S, Chu J, Zhang Y, Nadarajah S (2022) An extreme value analysis of the tail relationships between returns and volumes for high frequency cryptocurrencies. Res Int Bus Financ 59:101541
Chang L, Shi Y (2020) Does Bitcoin dominate the price discovery of the Cryptocurrencies market? A time-varying information share analysis. Oper Res Lett 48(5):641–645
Chaum D (1983) Blind signatures for untraceable payments. In: Advances in Cryptology: Proceedings of Crypto 82, Springer US, Boston, MA. pp 199–203
Cheah ET, Fry J (2015) Speculative bubbles in Bitcoin markets? An empirical investigation into the fundamental value of Bitcoin. Econ Lett 130:32–36
Cheah ET, Mishra T, Parhi M, Zhang Z (2018) Long memory interdependency and inefficiency in Bitcoin markets. Econ Lett 167:18–25
Chen X, Ghysels E (2011) News—good or bad—and its impact on volatility predictions over multiple horizons. Rev Financial Stud 24(1):46–81
Chen C, Liu L, Zhao N (2020) Fear sentiment, uncertainty, and bitcoin price dynamics: the case of COVID-19. Emerg Mark Finance Trade 56(10):2298–2309
Cheung A, Roca E, Su JJ (2015) Crypto-currency bubbles: an application of the Phillips–Shi–Yu (2013) methodology on Mt Gox Bitcoin prices. Appl Econ 47(23):2348–2358
Choi KH, Kang SH, Yoon SM (2022) Herding behaviour in Korea’s cryptocurrency market. Appl Econ 54(24):2795–2809
Christensen K, Oomen RC, Podolskij M (2014) Fact or friction: jumps at ultra high frequency. J Financ Econ 114(3):576–599
Chu J, Chan S, Nadarajah S, Osterrieder J (2017) GARCH modelling of cryptocurrencies. J Risk Financ Manag 10(4):17
Chu J, Chan S, Zhang Y (2020) High frequency momentum trading with cryptocurrencies. Res Int Bus Financ 52:101176
Chu J, Chan S, Zhang Y (2021) Bitcoin versus high-performance technology stocks in diversifying against global stock market indices. Physica A 580:126161
Chu J, Nadarajah S, Chan S (2015) Statistical analysis of the exchange rate of bitcoin. PLoS ONE 10(7):e0133678
Article PubMed PubMed Central Google Scholar
Chu J, Zhang Y, Chan S (2019) The adaptive market hypothesis in the high frequency cryptocurrency market. Int Rev Financ Anal 64:221–231
Ciaian P, Rajcaniova M (2018) Virtual relationships: short-and long-run evidence from BitCoin and altcoin markets. J Int Finan Markets Inst Money 52:173–195
Ciaian P, Rajcaniova M, Kancs DA (2016a) The economics of BitCoin price formation. Appl Econ 48(19):1799–1815
Ciaian P, Rajcaniova M, Kancs DA (2016b) The digital agenda of virtual currencies: Can BitCoin become a global currency? IseB 14(4):883–919
Ciaian P, Kancs DA, Rajcaniova M (2018) The price of Bitcoin: GARCH evidence from high frequency data. arXiv preprint https://arxiv.org/abs/1812.09452
Cobo MJ, López-Herrera AG, Herrera-Viedma E, Herrera F (2011) Science mapping software tools: review, analysis, and cooperative study among tools. J Am Soc Inform Sci Technol 62(7):1382–1402
Comerio N, Strozzi F (2019) Tourism and its economic impact: a literature review using bibliometric tools. Tour Econ 25(1):109–131
Conlon T, Corbet S, McGee RJ (2020) Are cryptocurrencies a safe haven for equity markets? An international perspective from the COVID-19 pandemic. Res Int Bus Financ 54:101248
Conlon T, McGee R (2020) Safe haven or risky hazard? Bitcoin during the COVID-19 bear market. Financ Res Lett 35:101607
Cont R (2001) Empirical properties of asset returns: stylized facts and statistical issues. Quant Finance 1(2):223
Corbet S, Hou YG, Hu Y, Larkin C, Oxley L (2020a) Any port in a storm: cryptocurrency safe-havens during the COVID-19 pandemic. Econ Lett 194:109377
Corbet S, Katsiampa P (2020) Asymmetric mean reversion of Bitcoin price returns. Int Rev Financ Anal 71:101267
Corbet S, Larkin C, Lucey B (2020b) The contagion effects of the COVID-19 pandemic: Evidence from gold and cryptocurrencies. Financ Res Lett 35:101554
Article PubMed Central Google Scholar
Corbet S, Lucey B, Peat M, Vigne S (2018b) Bitcoin Futures—What use are they? Econ Lett 172:23–27
Corbet S, Lucey B, Urquhart A, Yarovaya L (2019) Cryptocurrencies as a financial asset: a systematic analysis. Int Rev Financ Anal 62:182–199
Corbet S, Lucey B, Yarovaya L (2018a) Datestamping the Bitcoin and Ethereum bubbles. Financ Res Lett 26:81–88
Corbet S, Meegan A, Larkin C, Lucey B, Yarovaya L (2018c) Exploring the dynamic relationships between cryptocurrencies and other financial assets. Econ Lett 165:28–34
Corsi F (2009) A simple approximate long-memory model of realized volatility. J Financ Economet 7(2):174–196
Das D, Le Roux CL, Jana RK, Dutta A (2020) Does Bitcoin hedge crude oil implied volatility and structural shocks? A comparison with gold, commodity and the US Dollar. Financ Res Lett 36:101335
Demir E, Gozgor G, Lau CKM, Vigne SA (2018) Does economic policy uncertainty predict the Bitcoin returns? An empirical investigation. Financ Res Lett 26:145–149
Diebold FX, Yilmaz K (2012) Better to give than to receive: predictive directional measurement of volatility spillovers. Int J Forecast 28(1):57–66
Diebold FX, Yılmaz K (2014) On the network topology of variance decompositions: measuring the connectedness of financial firms. J Econom 182(1):119–134
Dimitrova V, Fernández-Martínez M, Sánchez-Granero MA, Trinidad Segovia JE (2019) Some comments on Bitcoin market (in) efficiency. PLoS ONE 14(7):e0219243
Article CAS PubMed PubMed Central Google Scholar
Ding Y, Chowdhury GG, Foo S (2001) Bibliometric cartography of information retrieval research by using co-word analysis. Inf Process Manage 37(6):817–842
Ding Y, Cronin B (2011) Popular and/or prestigious? Measures of scholarly esteem. Inf Process Manage 47(1):80–96
Dong B, Jiang L, Liu J, Zhu Y (2022) Liquidity in the cryptocurrency market and commonalities across anomalies. Int Rev Financ Anal 81:102097
Drożdż S, Gȩbarowski R, Minati L, Oświȩcimka P, Watorek M (2018) Bitcoin market route to maturity? Evidence from return fluctuations, temporal correlations and multiscaling effects. Chaos Interdiscip J Nonlinear Sci 28(7):071101
Drożdż S, Kwapień J, Oświecimka P, Rak R (2010) Quantitative features of multifractal subtleties in time series. EPL (europhysics Letters) 88(6):60003
Drożdż S, Kwapień J, Oświęcimka P, Stanisz T, Wątorek M (2020) Complexity in economic and social systems: cryptocurrency market at around COVID-19. Entropy 22(9):1043
Article ADS PubMed PubMed Central Google Scholar
Dwyer GP (2015) The economics of Bitcoin and similar private digital currencies. J Financ Stab 17:81–91
Dyhrberg AH (2016a) Bitcoin, gold and the dollar–a GARCH volatility analysis. Financ Res Lett 16:85–92
Dyhrberg AH (2016b) Hedging capabilities of bitcoin. Is it the virtual gold? Financ Res Lett 16:139–144
Dyhrberg AH, Foley S, Svec J (2018) How investible is Bitcoin? Analyzing the liquidity and transaction costs of Bitcoin markets. Econ Lett 171:140–143
Van Eck NJ, Waltman L (2014) Visualizing bibliometric networks. In: Ding Y, Rousseau R, Wolfram D (eds) Measuring Scholarly Impact. Springer, Berlin, pp 285–320
Engle RF (1982) Autoregressive conditional heteroscedasticity with estimates of the variance of United Kingdom inflation. Econom J Econom Soc 50(4):987–1007
MathSciNet Google Scholar
Eross A, McGroarty F, Urquhart A, Wolfe S (2019) The intraday dynamics of bitcoin. Res Int Bus Financ 49:71–81
Fahimnia B, Sarkis J, Davarzani H (2015) Green supply chain management: a review and bibliometric analysis. Int J Prod Econ 162:101–114
Fama EF (1970) Efficient capital markets: a review of theory and empirical work. J Financ 25(2):383–417
Fang L, Bouri E, Gupta R, Roubaud D (2019) Does global economic uncertainty matter for the volatility and hedging effectiveness of Bitcoin? Int Rev Financ Anal 61:29–36
Fang F, Ventre C, Basios M, Kanthan L, Martinez-Rego D, Wu F, Li L (2022) Cryptocurrency trading: a comprehensive survey. Financial Innov 8(1):1–59
Fassas AP, Papadamou S, Koulis A (2020) Price discovery in bitcoin futures. Res Int Bus Financ 52:101116
Fry J (2018) Booms, busts and heavy-tails: The story of Bitcoin and cryptocurrency markets? Econ Lett 171:225–229
Fry J, Cheah ET (2016) Negative bubbles and shocks in cryptocurrency markets. Int Rev Financ Anal 47:343–352
Fung K, Jeong J, Pereira J (2022) More to cryptos than bitcoin: a GARCH modelling of heterogeneous cryptocurrencies. Financ Res Lett 47:102544
Gandal N, Halaburda H (2016) Can we predict the winner in a market with network effects? Competition in cryptocurrency market. Games 7(3):16
Gandal N, Hamrick JT, Moore T, Oberman T (2018) Price manipulation in the Bitcoin ecosystem. J Monet Econ 95:86–96
García-Corral FJ, Cordero-García JA, de Pablo-Valenciano J, Uribe-Toril J (2022) A bibliometric review of cryptocurrencies: how have they grown? Financial Innov 8(1):1–31
Giudici P, Pagnottoni P (2020) Vector error correction models to measure connectedness of Bitcoin exchange markets. Appl Stoch Model Bus Ind 36(1):95–109
Giudici P, Polinesi G (2021) Crypto price discovery through correlation networks. Ann Oper Res 299(1):443–457
Gkillas K, Bouri E, Gupta R, Roubaud D (2020) Spillovers in higher-order moments of crude oil, gold, and Bitcoin. Q Rev Econ Finance 84:398–406
Gkillas K, Gupta R, Wohar ME (2018) Volatility jumps: the role of geopolitical risks. Financ Res Lett 27:247–258
Gkillas K, Katsiampa P, Konstantatos C, Tsagkanos A (2022) Discontinuous movements and asymmetries in cryptocurrency markets. Eur J Finance. https://doi.org/10.1080/1351847X.2021.2015416
Glantz M, Kissell RL (2013) Multi-asset risk modeling: techniques for a global economy in an electronic and algorithmic trading era. Academic Press, London
Gonzalo J, Granger C (1995) Estimation of common long-memory components in cointegrated systems. J Bus Econ Stat 13(1):27–35
Gradojevic N, Kukolj D, Adcock R, Djakovic V (2022) Forecasting Bitcoin with technical analysis: A not-so-random forest? Int J Forecast 39(1):1–17
Gradojevic N, Tsiakas I (2021) Volatility cascades in cryptocurrency trading. J Empir Financ 62:252–265
Greaves A, Au B (2015) Using the bitcoin transaction graph to predict the price of bitcoin. No Data 8:416–443
Grobys K, Huynh TLD (2022) When Tether says “JUMP!” Bitcoin asks “How low?” Financ Res Lett 47:102644
Guesmi K, Saadi S, Abid I, Ftiti Z (2019) Portfolio diversification with virtual currency: evidence from bitcoin. Int Rev Financ Anal 63:431–437
Hansen PR, Huang Z (2016) Exponential GARCH modeling with realized measures of volatility. J Bus Econ Stat 34(2):269–287
Hansen PR, Huang Z, Shek HH (2012) Realized GARCH: a joint model for returns and realized measures of volatility. J Appl Economet 27(6):877–906
Hasbrouck J (1995) One security, many markets: determining the contributions to price discovery. J Financ 50(4):1175–1199
Holub M, Johnson J (2018) Bitcoin research across disciplines. Inf Soc 34(2):114–126
Hu B, McInish T, Miller J, Zeng L (2019) Intraday price behavior of cryptocurrencies. Financ Res Lett 28:337–342
Huang X, Tauchen G (2005) The relative contribution of jumps to total price variance. J Financ Economet 3(4):456–499
Härdle WK, Harvey CR, Reule RC (2020) Understanding cryptocurrencies. J Financ Economet 18(2):181–208
Jalal RNUD, Alon I, Paltrinieri A (2021) A bibliometric review of cryptocurrencies as a financial asset. Technol Anal Strateg Manag. https://doi.org/10.1080/09537325.2021.1939001
Jalal RUD, Sargiacomo M, Sahar NU, Fayyaz UER (2020) Herding behavior and cryptocurrency: market asymmetries, inter-dependency and intra-dependency. J Asian Finance Econ Bus 7(7):27–34
Ji Q, Bouri E, Kristoufek L, Lucey B (2021) Realised volatility connectedness among Bitcoin exchange markets. Financ Res Lett 38:101391
Ji Q, Bouri E, Lau CKM, Roubaud D (2019) Dynamic connectedness and integration in cryptocurrency markets. Int Rev Financ Anal 63:257–272
Jiang S, Li X, Wang S (2021) Exploring evolution trends in cryptocurrency study: from underlying technology to economic applications. Financ Res Lett 38:101532
Jiang Y, Nie H, Ruan W (2018) Time-varying long-term memory in Bitcoin market. Financ Res Lett 25:280–284
Jiang ZQ, Xie WJ, Zhou WX, Sornette D (2019) Multifractal analysis of financial markets: a review. Rep Prog Phys 82(12):125901
Article MathSciNet ADS PubMed Google Scholar
Johnson B, Laszka A, Grossklags J, Vasek M, Moore T (2014) Game-theoretic analysis of DDoS attacks against Bitcoin mining pools. In: International Conference on Financial Cryptography and Data Security, Springer, Berlin, Heidelberg. pp 72–86
Kantelhardt JW, Zschiegner SA, Koscielny-Bunde E, Havlin S, Bunde A, Stanley HE (2002) Multifractal detrended fluctuation analysis of nonstationary time series. Physica A 316(1–4):87–114
Kapar B, Olmo J (2019) An analysis of price discovery between Bitcoin futures and spot markets. Econ Lett 174:62–64
Karalevicius V, Degrande N, De Weerdt J (2018) Using sentiment analysis to predict interday Bitcoin price movements. J Risk Finance 19(1):56–75
Katsiampa P (2017) Volatility estimation for Bitcoin: a comparison of GARCH models. Econ Lett 158:3–6
Katsiampa P, Corbet S, Lucey B (2019) High frequency volatility co-movements in cryptocurrency markets. J Int Finan Markets Inst Money 62:35–52
Katsiampa P, Yarovaya L, Zięba D (2022) High-Frequency connectedness between bitcoin and other top-traded crypto assets during the COVID-19 crisis. J Int Financ Mark Inst Money 79:101578
Kayal P, Rohilla P (2021) Bitcoin in the economics and finance literature: a survey. SN Bus Econ 1(7):1–21
Khuntia S, Pattanayak JK (2018) Adaptive market hypothesis and evolving predictability of bitcoin. Econ Lett 167:26–28
Khuntia S, Pattanayak JK (2020) Adaptive long memory in volatility of intra-day bitcoin returns and the impact of trading volume. Finance Res Lett 32:101077
Klein T, Thu HP, Walther T (2018) Bitcoin is not the New Gold–A comparison of volatility, correlation, and portfolio performance. Int Rev Financ Anal 59:105–116
Koutmos D (2018) Bitcoin returns and transaction activity. Econ Lett 167:81–85
Kristoufek L (2013) BitCoin meets Google Trends and Wikipedia: quantifying the relationship between phenomena of the Internet era. Sci Rep 3(1):1–7
Kristoufek L (2015) What are the main drivers of the Bitcoin price? Evidence from wavelet coherence analysis. PLoS ONE 10(4):e0123923
Kristoufek L (2018) On Bitcoin markets (in) efficiency and its evolution. Physica A 503:257–262
Kristoufek L (2020) Grandpa, grandpa, tell me the one about Bitcoin being a safe haven: new evidence from the COVID-19 pandemic. Front Phys 8:296
Lahmiri S, Bekiros S (2019) Cryptocurrency forecasting with deep learning chaotic neural networks. Chaos Solitons Fractals 118:35–40
Levine-Clark M, Gil EL (2008) A comparative citation analysis of Web of science, scopus, and google scholar. J Bus Financ Librariansh 14(1):32–46
Li X, Li S, Xu C (2020) Price clustering in Bitcoin market—an extension. Financ Res Lett 32:101072
Li X, Qiao H, Wang S (2017) Exploring evolution and emerging trends in business model study: a co-citation analysis. Scientometrics 111(2):869–887
Li X, Wang CA (2017) The technology and economic determinants of cryptocurrency exchange rates: the case of Bitcoin. Decis Support Syst 95:49–60
Long S, Lucey B, Xie Y, Yarovaya L (2022) “I Just Like the Stock”: the role of reddit sentiment in the gamestop share rally. Financ Rev 58(1):19–37
Makarov I, Schoar A (2020) Trading and arbitrage in cryptocurrency markets. J Financ Econ 135(2):293–319
Mandaci PE, Cagli EC (2022) Herding intensity and volatility in cryptocurrency markets during the COVID-19. Financ Res Lett 46:102382
McNally S, Roche J, Caton S (2018) Predicting the price of bitcoin using machine learning. In: 2018 26th euromicro international conference on parallel, distributed and network-based processing (PDP), pp 339–343. IEEE
Mensi W, Al-Yahyaee KH, Al-Jarrah IMW, Vo XV, Kang SH (2020) Dynamic volatility transmission and portfolio management across major cryptocurrencies: evidence from hourly data. N Am J Econ Finance 54:101285
Mensi W, Lee YJ, Al-Yahyaee KH, Sensoy A, Yoon SM (2019) Intraday downward/upward multifractality and long memory in Bitcoin and Ethereum markets: an asymmetric multifractal detrended fluctuation analysis. Financ Res Lett 31:19–25
Merediz-Solà I, Bariviera AF (2019) A bibliometric analysis of bitcoin scientific production. Res Int Bus Financ 50:294–305
Moral-Muñoz JA, Herrera-Viedma E, Santisteban-Espejo A, Cobo MJ (2020) Software tools for conducting bibliometric analysis in science: an up-to-date review. Prof Inf. https://doi.org/10.3145/epi.2020.ene.03
Nadarajah S, Chu J (2017) On the inefficiency of Bitcoin. Econ Lett 150:6–9
Naeem MA, Bouri E, Peng Z, Shahzad SJH, Vo XV (2021) Asymmetric efficiency of cryptocurrencies during COVID19. Physica A 565:125562
Article CAS PubMed Google Scholar
Nakamoto S (2008) Bitcoin: a peer-to-peer electronic cash system. Decentralized Business Review, 21260
Nakano M, Takahashi A, Takahashi S (2018) Bitcoin technical trading with artificial neural network. Physica A 510:587–609
Nasir MA, Huynh TLD, Nguyen SP, Duong D (2019) Forecasting cryptocurrency returns and volume using search engines. Financ Innov 5(1):1–13
Nguyen LH, Chevapatrakul T, Yao K (2020) Investigating tail-risk dependence in the cryptocurrency markets: a LASSO quantile regression approach. J Empir Financ 58:333–355
Oświȩcimka P, Drożdż S, Forczek M, Jadach S, Kwapień J (2014) Detrended cross-correlation analysis consistently extended to multifractality. Phys Rev E 89(2):023305
Oświȩcimka P, Kwapień J, Drożdż S (2006) Wavelet versus detrended fluctuation analysis of multifractal structures. Phys Rev E 74(1):016103
Pagnottoni P, Dimpfl T (2019) Price discovery on Bitcoin markets. Digital Finance 1(1):139–161
Patel R, Goodell JW, Oriani ME, Paltrinieri A, Yarovaya, (2022) A bibliometric review of financial market integration literature. Int Rev Financ Anal 80:102035
Patton AJ, Sheppard K (2015) Good volatility, bad volatility: Signed jumps and the persistence of volatility. Rev Econ Stat 97(3):683–697
Paul J, Bhukya R (2021) Forty-five years of International Journal of Consumer Studies: a bibliometric review and directions for future research. Int J Consum Stud 45(5):937–963
Peng Y, Albuquerque PHM, de Sá JMC, Padula AJA, Montenegro MR (2018) The best of two worlds: forecasting high frequency volatility for cryptocurrencies and traditional currencies with Support Vector Regression. Expert Syst Appl 97:177–192
Persson O (1994) The intellectual base and research fronts of JASIS 1986–1990. J Am Soc Inf Sci 45(1):31–38
Philippas D, Philippas N, Tziogkidis P, Rjiba H (2020) Signal-herding in cryptocurrencies. J Int Finan Markets Inst Money 65:101191
Phillip A, Chan JS, Peiris S (2018) A new look at cryptocurrencies. Econ Lett 163:6–9
Phillip A, Chan J, Peiris S (2019) On long memory effects in the volatility measure of cryptocurrencies. Financ Res Lett 28:95–100
Platanakis E, Urquhart A (2020) Should investors include bitcoin in their portfolios? A portfolio theory approach. Br Account Rev 52(4):100837
Polat O, Kabakçı Günay E (2021) Cryptocurrency connectedness nexus the COVID-19 pandemic: evidence from time-frequency domains. Stud Econ Financ 38(5):946–963
Potter WJ, Levine-Donnerstein D (1999) Rethinking validity and reliability in content analysis. J Appl Commun Res 27(3):258–284
Qiu Y, Wang Y, Xie T (2021) Forecasting Bitcoin realized volatility by measuring the spillover effect among cryptocurrencies. Econ Lett 208:110092
Quiroga-Garcia R, Pariente-Martinez N, Arenas-Parra M (2022) Evidence for round number effects in cryptocurrencies prices. Financ Res Lett 47:102811
Ramona O, Cristina MS, Raluca S (2019) Bitcoin in the scientific literature–a bibliometric study. Stud Bus Econ 14(3):160–174
Rognone L, Hyde S, Zhang SS (2020) News sentiment in the cryptocurrency market: an empirical comparison with Forex. Int Rev Financ Anal 69:101462
Ruane F, Tol RS (2007) Economics in Ireland (No. 180). ESRI Working Paper
Ruggeri G, Orsi L, Corsi S (2019) A bibliometric analysis of the scientific literature on Fairtrade labelling. Int J Consum Stud 43(2):134–152
Scaillet O, Treccani A, Trevisan C (2020) High-frequency jump analysis of the bitcoin market. J Financ Economet 18(2):209–232
Selgin G (2015) Synthetic commodity money. J Financ Stab 17:92–99
Sensoy A (2019) The inefficiency of Bitcoin revisited: a high-frequency analysis with alternative currencies. Financ Res Lett 28:68–73
Sensoy A, Silva TC, Corbet S, Tabak BM (2021) High-frequency return and volatility spillovers among cryptocurrencies. Appl Econ 53(37):4310–4328
Shahzad SJH, Anas M, Bouri E (2022) Price explosiveness in cryptocurrencies and Elon Musk’s tweets. Finance Res Lett 47:102695
Shahzad SJH, Bouri E, Roubaud D, Kristoufek L (2020) Safe haven, hedge and diversification for G7 stock markets: gold versus bitcoin. Econ Model 87:212–224
Shahzad SJH, Bouri E, Roubaud D, Kristoufek L, Lucey B (2019) Is Bitcoin a better safe-haven investment than gold and commodities? Int Rev Financ Anal 63:322–330
Shen D, Urquhart A, Wang P (2019) Does twitter predict Bitcoin? Econ Lett 174:118–122
Shen CW, Chang LC, Su TC (2020) Research development of Bitcoin: a network and concept linking analysis. Library Hi Tech.
Shi S, Shi Y (2021) Bitcoin futures: trade it or ban it? Eur J Finance 27(4–5):381–396
Sifat IM, Mohamad A, Shariff MSBM (2019) Lead-lag relationship between bitcoin and ethereum: evidence from hourly and daily data. Res Int Bus Financ 50:306–321
da Silva Filho AC, Maganini ND, de Almeida EF (2018) Multifractal analysis of Bitcoin market. Physica A 512:954–967
Smales LA (2019) Bitcoin as a safe haven: Is it even worth considering? Financ Res Lett 30:385–393
Small H (1973) Co-citation in the scientific literature: a new measure of the relationship between two documents. J Am Soc Inf Sci 24(4):265–269
Sousa A, Calçada E, Rodrigues P, Borges AP (2022) Cryptocurrency adoption: a systematic literature review and bibliometric analysis. EuroMed J Bus 17(3):374–390
Stavroyiannis S, Babalos V, Bekiros S, Lahmiri S, Uddin GS (2019) The high frequency multifractal properties of Bitcoin. Physica A 520:62–71
Su F, Wang X, Yuan Y (2022) The intraday dynamics and intraday price discovery of bitcoin. Res Int Bus Financ 60:101625
Takaishi T (2018) Statistical properties and multifractality of Bitcoin. Physica A 506:507–519
Takaishi T (2020) Rough volatility of Bitcoin. Financ Res Lett 32:101379
Takaishi T (2021) Time-varying properties of asymmetric volatility and multifractality in Bitcoin. PLoS ONE 16(2):e0246209
Takaishi T, Adachi T (2020) Market efficiency, liquidity, and multifractality of Bitcoin: a dynamic study. Asia-Pacific Finan Markets 27(1):145–154
Tauchen G, Zhou H (2011) Realized jumps on financial markets and predicting credit spreads. J Econom 160(1):102–118
Thies S, Molnár P (2018) Bayesian change point analysis of Bitcoin returns. Financ Res Lett 27:223–227
Tiwari AK, Jana RK, Das D, Roubaud D (2018) Informational efficiency of Bitcoin—An extension. Econ Lett 163:106–109
Tschorsch F, Scheuermann B (2016) Bitcoin and beyond: a technical survey on decentralized digital currencies. IEEE Commun Surv Tutor 18(3):2084–2123
Turanova G (2017) Is bitcoin a bubble? 29 December. http://www.nasdaq.com/article/is-bitcoin-a-bubble-cm898150 . Accessed 1 July 2022
Urquhart A (2016) The inefficiency of Bitcoin. Econ Lett 148:80–82
Urquhart A (2017) Price clustering in Bitcoin. Econ Lett 159:145–148
Urquhart A (2018) What causes the attention of Bitcoin? Econ Lett 166:40–44
Urquhart A, Zhang H (2019) Is Bitcoin a hedge or safe haven for currencies? An intraday analysis. Int Rev Financ Anal 63:49–57
Van Eck NJ, Waltman L (2019) Accuracy of citation data in Web of Science and Scopus. arXiv preprint https://arxiv.org/abs/1906.07011
Vasiliauskaite V, Lillo F, Antulov-Fantulin N (2022) Information dynamics of price and liquidity around the 2017 Bitcoin markets crash. Chaos Interdiscip J Nonlinear Sci 32(4):043123
Vidal-Tomás D (2021) An investigation of cryptocurrency data: the market that never sleeps. Quantit Finance 21(12):2007–2024
Vidal-Tomás D (2022) All the frequencies matter in the Bitcoin market: an efficiency analysis. Appl Econ Lett 29(3):212–218
Vidal-Tomás D, Ibañez A (2018) Semi-strong efficiency of Bitcoin. Financ Res Lett 27:259–265
Vidal-Tomás D, Ibáñez AM, Farinós JE (2019) Herding in the cryptocurrency market: CSSD and CSAD approaches. Financ Res Lett 30:181–186
Waltman L, Van Eck NJ (2013) A smart local moving algorithm for large-scale modularity-based community detection. Eur Phys J B 86:1–14
Waltman L, Van Eck NJ, Noyons EC (2010) A unified approach to mapping and clustering of bibliometric networks. J Informet 4(4):629–635
Wang M, Li X, Wang S (2021) Discovering research trends and opportunities of green finance and energy policy: a data-driven scientometric analysis. Energy Policy 154:112295
Wang J, Ngene GM (2020) Does Bitcoin still own the dominant power? An intraday analysis. Int Rev Financ Anal 71:101551
Wang J, Wang X (2021) COVID-19 and financial market efficiency: evidence from an entropy-based analysis. Financ Res Lett 42:101888
Wei WC (2018) Liquidity and market efficiency in cryptocurrencies. Econ Lett 168:21–24
Wen Z, Bouri E, Xu Y, Zhao Y (2022) Intraday return predictability in the cryptocurrency markets: momentum, reversal, or both. N Am J Econ Finance 62:101733
Whittaker J (1989) Creativity and conformity in science: titles, keywords and co-word analysis. Soc Stud Sci 19(3):473–496
Yarovaya L, Matkovskyy R, Jalan A (2021) The effects of a “black swan” event (COVID-19) on herding behavior in cryptocurrency markets. J Int Finan Markets Inst Money 75:101321
Yarovaya L, Zięba D (2022) Intraday volume-return nexus in cryptocurrency markets: a novel evidence from cryptocurrency classification. Res Int Bus Finance 60:101592
Yermack D (2015) Is Bitcoin a real currency? An economic appraisal. Handbook of digital currency. Academic Press, London, pp 31–43
Chapter Google Scholar
Yi S, Xu Z, Wang GJ (2018) Volatility connectedness in the cryptocurrency market: Is Bitcoin a dominant cryptocurrency? Int Rev Financ Anal 60:98–114
Yousaf I, Ali S (2020) Discovering interlinkages between major cryptocurrencies using high-frequency data: new evidence from COVID-19 pandemic. Financ Innov 6(1):1–18
Yu M (2019) Forecasting Bitcoin volatility: the role of leverage effect and uncertainty. Physica A 533:120707
Yue Y, Li X, Zhang D, Wang S (2021) How cryptocurrency affects economy? A network analysis using bibliometric methods. Int Rev Financ Anal 77:101869
Zargar FN, Kumar D (2019) Informational inefficiency of Bitcoin: a study based on high-frequency data. Res Int Bus Financ 47:344–353
Zha Q, Kou G, Zhang H, Liang H, Chen X, Li CC, Dong Y (2020) Opinion dynamics in finance and business: a literature review and research opportunities. Financ Innov 6:1–22
Zhang Y, Chan S, Chu J, Nadarajah S (2019a) Stylised facts for high frequency cryptocurrency data. Physica A 513:598–612
Zhang Y, Chan S, Nadarajah S (2019b) Extreme value analysis of high-frequency cryptocurrencies. High Freq 2(1):61–69
Zhang W, Wang P, Li X, Shen D (2018) The inefficiency of cryptocurrency and its cross-correlation with Dow Jones Industrial Average. Physica A 510:658–670
Zheng Z, Xie S, Dai HN, Chen X, Wang H (2018) Blockchain challenges and opportunities: a survey. Int J Web Grid Serv 14(4):352–375
Zupic I, Čater T (2015) Bibliometric methods in management and organization. Organ Res Methods 18(3):429–472
Zyskind G, Nathan O (2015) Decentralizing privacy: using blockchain to protect personal data. In: 2015 IEEE Security and Privacy Workshops, pp 180–184. IEEE
Download references
Acknowledgements
Not applicable.
Author information
Authors and affiliations.
Department of Management Sciences, College of Flying Training (CFT), Air University, Risalpur, Pakistan
Muhammad Anas
Campus Saint-Jean, University of Alberta, Edmonton, AB, Canada
Montpellier Business School, Montpellier, France
Syed Jawad Hussain Shahzad
Southampton Business School, Southampton, UK
Larisa Yarovaya
You can also search for this author in PubMed Google Scholar
Contributions
MA carried out conceptualization, methodology, software, formal analysis and writing. SJHS carried out conceptualization, supervision and writing—review and editing. LY carried out supervision, review and editing. All authors read and approved the final manuscript.
Corresponding author
Correspondence to Larisa Yarovaya .
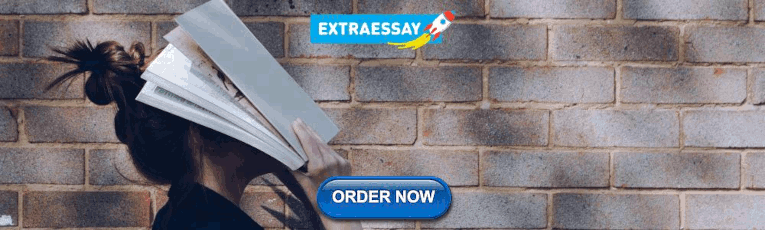
Ethics declarations
Competing interests.
We have no competing interests to disclose.
Additional information
Publisher's note.
Springer Nature remains neutral with regard to jurisdictional claims in published maps and institutional affiliations.
Rights and permissions
Open Access This article is licensed under a Creative Commons Attribution 4.0 International License, which permits use, sharing, adaptation, distribution and reproduction in any medium or format, as long as you give appropriate credit to the original author(s) and the source, provide a link to the Creative Commons licence, and indicate if changes were made. The images or other third party material in this article are included in the article's Creative Commons licence, unless indicated otherwise in a credit line to the material. If material is not included in the article's Creative Commons licence and your intended use is not permitted by statutory regulation or exceeds the permitted use, you will need to obtain permission directly from the copyright holder. To view a copy of this licence, visit http://creativecommons.org/licenses/by/4.0/ .
Reprints and permissions
About this article
Cite this article.
Anas, M., Shahzad, S.J.H. & Yarovaya, L. The use of high-frequency data in cryptocurrency research: a meta-review of literature with bibliometric analysis. Financ Innov 10 , 90 (2024). https://doi.org/10.1186/s40854-023-00595-y
Download citation
Received : 14 October 2022
Accepted : 26 December 2023
Published : 01 May 2024
DOI : https://doi.org/10.1186/s40854-023-00595-y
Share this article
Anyone you share the following link with will be able to read this content:
Sorry, a shareable link is not currently available for this article.
Provided by the Springer Nature SharedIt content-sharing initiative
- Cryptocurrencies
- High-frequency data
- Intra-day data
- Bibliometric analysis
- Meta-literature review
Burden of Antimicrobial Resistance in Japan: A Systematic Literature Review and Meta-Analysis
- Original Research
- Open access
- Published: 25 April 2024
Cite this article
You have full access to this open access article
- Tetsuya Matsumoto 1 ,
- Akira Yuasa ORCID: orcid.org/0000-0002-3699-7231 2 ,
- Hiroyuki Matsuda 3 ,
- Dilinuer Ainiwaer 3 &
- Naohiro Yonemoto 2
205 Accesses
1 Altmetric
Explore all metrics
Introduction
Antimicrobial resistance (AMR) is one of the most serious public health challenges worldwide, including in Japan. However, there is limited evidence assessing the AMR burden in Japan. Thus, this systematic literature review (SLR) and meta-analysis (MA) were conducted to assess the clinical and economic burden of AMR in Japan.
Comprehensive literature searches were performed on EMBASE, MEDLINE, the Cochrane Library, and ICHUSHI between 2012 and 2022 following the Preferred Reporting Items for Systematic Reviews and Meta-Analysis guidelines. MA estimated a pooled effect between the two comparative arms (AMR vs. non-AMR). The results were reported in measures of odds ratios (ORs) for in-hospital mortality and in standardized mean differences (SMDs) for length of stay (LOS) and direct medical costs.
Literature searches identified 1256 de-duplicated records, of which 56 observational studies (English, n = 35; Japanese, n = 21) were included. Of note, twenty-two studies (39.3%) compared the AMR group with non-AMR group. In the SLR, in-hospital mortality, LOS, and direct medical costs were higher in the AMR group compared to the non-AMR group. Eight studies were selected for the MA. In the AMR group, the pooled estimate showed a statistically higher in-hospital mortality [random effect (RE)—OR 2.25, 95% CI 1.34–3.79; I 2 = 89%; τ 2 = 0.2257, p < 0.01], LOS (RE—SMD 0.37, 95% CI − 0.09–0.84; I 2 = 99%; τ 2 = 0.3600, p < 0.01), and direct medical cost (RE—SMD 0.53, 95% CI 0.43–0.62; I 2 = 0.0%; τ 2 = 0.0, p = 0.88) versus the non-AMR group.
Our study presents an overview of the clinical and economic burden of AMR in Japan. Patients with AMR infections experience significantly higher in-hospital mortality, LOS, and direct medical costs compared with patients without AMR infections.
Similar content being viewed by others
Estimating the burden of antimicrobial resistance: a systematic literature review.
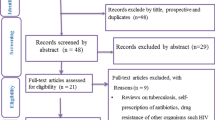
Guideline Recommendations for Empirical Antimicrobial Therapy: An Appraisal of Research Evidence for Clinical Decision-Making in Ethiopia
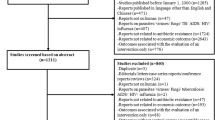
Economic burden of antibiotic resistance in ESKAPE organisms: a systematic review
Avoid common mistakes on your manuscript.
Antimicrobials are medications used to prevent and treat infections. These include antibiotics, antivirals, antifungals, and antiparasitic drugs. Antimicrobial resistance (AMR) occurs when pathogens develop mechanisms to thrive or survive in the presence of the drug. As a result, antimicrobials show reduced clinical efficacy, and infections become increasingly difficult or impossible to treat. These result in an increased risk of disease spread, severe illness, and death [ 1 ]. The irrational use of antibiotics is directly correlated with the increase in AMR and varies from country to country, depending on the respective antibiotic usage patterns [ 2 , 3 ].
The global review on AMR estimated 700,000 deaths in 2014 attributable to drug-resistant strains of common bacterial infections, human immunodeficiency virus (HIV), tuberculosis, and malaria [ 4 ]. However, bacterial AMR emerged as one of the leading public health threats of the twenty-first century, with 1.27 million deaths directly caused by bacterial AMR globally in 2019 [ 5 ]. The total annual number of deaths caused by AMR in Japan is unknown; however, a 2020 study by Tsuzuki et al. estimated that, in 2017, the number of deaths attributed to bloodstream infections (BSIs) caused by methicillin-resistant Staphylococcus aureus (MRSA) and fluoroquinolone-resistant Escherichia coli (FQREC) was approximately 8100 [ 6 ]. According to the Japan Nosocomial Infections Surveillance (JANIS) Annual Open Report 2022, the resistance rates of third-generation cephalosporin antibiotics, including cefotaxime and ceftazidime, were 26.8% and 12.8% for E. coli and 12.6% and 10.3% for Klebsiella pneumoniae , respectively, whereas for levofloxacin, the resistance rate to E. coli was 39.6% [ 7 ]. An analysis of 2289 hospitals across Japan, conducted as part of the JANIS program 2022, reported that the percentage of hospitals reporting at least one corresponding specific AMR bacteria in 2022 was the highest for MRSA (99.7%), followed by penicillin-resistant Streptococcus pneumoniae (PRSP; 53.9%) and carbapenem-resistant Enterobacteriaceae (CRE; 51.2%) [ 7 ].
Rising AMR has severe health and economic consequences [ 8 ]. A 2018 report by the Organization for Economic Co-operation and Development (OECD) that analyzed 33 OECD, European Union (EU), and European Economic Area countries estimated that AMR caused a total of $3.5 billion annually in health care costs. In Japan, Matsumoto et al. [ 9 ] estimated the clinical and economic outcomes of drug-resistant gram-negative pathogens in Japan and demonstrated that economic and clinical values could be considerably increased by reducing AMR levels in the country. It was reported that savings of ¥2.5 billion ($23 million) to ¥6.4 billion ($60 million) in hospitalization costs could be achieved in a year by reducing AMR by 50% in Japan [ 9 ].
In March 2021, the Japan Agency for Medical Research and Development (AMED) Public and Private Partnerships for Infectious Diseases R&D created the first edition of the Japanese version of the Priority Pathogen List, which was approved by seven academic societies and the Drug Discovery Promotion Review Committee [ 10 ].
In Japan, there is limited evidence assessing AMR burden. Since AMR strains and resistance rates that require attention differ from country to country, there is a need to appropriately assess the country-wise burden of AMR by referring to previous data and reports. This study aims to conduct a systematic literature review (SLR) and meta-analysis (MA) to determine the clinical and economic burden of AMR in Japan.
The searches were designed by considering the combination of sensitivity and specificity. The SLR was conducted in accordance with the general recommendations of the Cochrane Handbook for Systematic Reviews of Interventions [ 11 ], the general principles of the Centre for Reviews and Dissemination (University of York) guidance [ 12 ] for undertaking reviews in health care, and the Preferred Reporting Items for Systematic Reviews and Meta-Analyses (PRISMA) guidelines [ 13 ].
Eligibility Criteria
Table 1 contains the eligibility criteria defined under the Population, Intervention, Comparison, Outcomes, and Study design (PICOS) framework [ 14 ]. The systematic literature search was conducted using a predefined search strategy to identify eligible studies; the search strategies are presented in Supplementary Table 1. The target population for this SLR was adult Japanese patients (≥ 18 years of age) with at least one AMR infectious disease for any treatment. The focus was on interventional and observational studies assessing the clinical burden [in-hospital mortality, and length of stay (LOS)—including intensive care unit (ICU) and isolation bed stays] and economic burden (direct medical costs, duration of antibiotics, and amount of antibiotic usage) of AMR in Japan.
The definition of AMR in this study refers to the first edition of the Japanese version of the Priority Pathogen List that was created by the AMED Public and Private Partnerships for Infectious Diseases R&D and which was approved by seven academic societies and the Drug Discovery Promotion Review Committee [ 10 ].
Data Sources
Evidence published between 2012 and 2022 was sourced from various databases, including EMBASE, MEDLINE, the Cochrane Library (via Ovid SP ® ), and ICHUSHI (a Japanese database). Gray literature and conference abstracts for the last 3 years were also hand-searched to identify records on the treatments used for AMR infection in Japan in the following databases: Infectious Diseases Society of America, European Congress of Clinical Microbiology & Infectious Diseases/European Society of Clinical Microbiology and Infectious Diseases, International Society for Pharmacoeconomics and Outcomes Research, The Japanese Association for Infectious Diseases, Japanese Society of Chemotherapy, and Japan Society of Environmental Infectious.
Search Strategy
The search strategy was developed by combining free-text words, indexing terms, and Boolean terms with terms pertinent to disease areas, interventions, and study designs. The search strings were modified to conform to the syntax of each database. According to the eligibility criteria, all retrieved studies were then evaluated. Two reviewers independently assessed the eligibility (inclusion/exclusion) of titles and abstracts identified during the search using predetermined criteria using the PICOS framework. Every instance of uncertainty or ambiguity was resolved by consulting a third independent reviewer. Publications selected as potentially relevant from the abstract screening were retained for the full-text review. Two independent reviewers evaluated full-text publications, and discrepancies were resolved by consulting a third independent reviewer.
Data Extraction
A standardized MS Excel ® data extraction template was used to conduct data extraction from the full-text studies identified by the searches. The key methodology, patient characteristics, and results were extracted and tabulated for each study. One researcher performed data extraction, which was checked by another independent researcher. The results of the data extraction were used for the feasibility assessment and the MA. Means, medians, standard deviations (SDs), 95% confidence intervals (95% CIs), and interquartile ranges (reporting 25th and 75th values) were used to report continuous outcomes, while counts and percentages were used to report categorical outcomes. For the comparison of direct medical costs in the MA, the selected cost data of the studies were adjusted to represent the values for 2022 in local currency [ 15 ]. The annual exchange rate for 2022 by the OECD was used to convert Japanese yen to United States (US) dollars ($1 = ¥131.498) [ 16 ]. Other cost data were presented as reported in the studies.
Quality Assessment
The quality of randomized controlled trial (RCT) studies included in the SLR was evaluated to ensure that this review's conclusions and findings are based on the best available evidence and to identify any potential sources of bias in the data. The quality of RCT studies retained for data extraction was assessed using a Cochrane risk of bias tool for randomized trials. This checklist (also called “RoB”) is the most recommended tool for RCTs. The RoB 2.0 tool is suitable for individually randomized, parallel-group, and cluster-randomized trials [ 17 ]. The Newcastle-Ottawa scales for cohort case-control studies were used to evaluate the risk of bias in each individual article included in this study [ 18 ] (Supplementary Table 2). Gray literature that did not encompass full-text articles was evaluated using the Authority, Accuracy, Coverage, Objectivity, Date, Significance checklist [ 19 ].
Data Analysis
Statistical analysis.
The MA estimated the clinical and economic burden between the two comparative arms: the AMR (resistant) and non-AMR (susceptible) arms. A high degree of heterogeneity was estimated because of differences in underlying diseases, causative organisms, patient backgrounds, and clinical characteristics among individual studies. The MA was performed only on studies when they used design and/or analysis for adjustment of confounders, such as propensity score matching performed on the two comparative arms. All analyses were performed using the R software environment for statistical computing and graphics (version 4.1 or above). The ‘meta,’ ‘metafor,’ package in R was used to conduct the analyses.
The MA was used to estimate the pooled effect between the two arms (i.e., summary of proportions, time-to-event outcomes, and mean of outcomes) across all eligible studies. The weighted average of each outcome measure of the studies was calculated using inverse variance weighting to estimate the pooled effect [ 20 ]. Both random effect (RE) and fixed effect (FE) models were applied; however, the RE model was selected as the primary model [ 20 ]. The results were reported with the measure of effect as odds ratios (ORs) and standardized mean differences (SMDs) with 95% CIs. In-hospital mortality was reported with dichotomous outcomes. Also, LOS and direct medical costs were reported as continuous outcomes. The Box-Cox method [ 21 ] was applied to studies not reporting mean and SD directly to estimate the mean and SD according to the reported sample size, median, minimum value, maximum value, first quartile, or third quartile.
Assessment of Statistical Heterogeneity and Publication Bias
Heterogeneity among the selected studies was evaluated using both the Q test and I 2 index [ 22 ]. In the Q test, a p value cutoff < 0.1 for the test for heterogeneity indicated presence of heterogeneity. The I 2 index (0–40% no heterogeneity, 30–60% moderate heterogeneity, 50–90% substantial heterogeneity, and 75–100% considerable heterogeneity) was used to describe the percentage of total variation across analyses due to heterogeneity [ 11 , 23 ]. In case of existing substantial heterogeneity in MA and sufficient studies, subgroup analysis was conducted according to the characteristic of the study or patient, including studies in MA. Moreover, as another analysis, the study with extreme value, which might lead to substantial heterogeneity, was excluded in MA.
Publication bias was assessed through funnel plot for base case analysis in this study, Egger’s test was not applicable because of the small number of studies for each outcome ( n < 10 studies) [ 24 , 25 ].
Ethical Approval
Ethics approval was not required for this study. This article is based on previously conducted studies and does not contain any new studies with human participants or animals performed by any of the authors.
Study Overview
A search of the databases returned a total of 1262 results. Following the removal of duplicates and a thorough review, 57 records (consisting of 56 studies) that met the inclusion criteria were included in the SLR (Supplementary Table 3). Figure 1 presents the PRISMA flow diagram for the SLR, and Supplementary Table 4 presents the PRISMA check list.
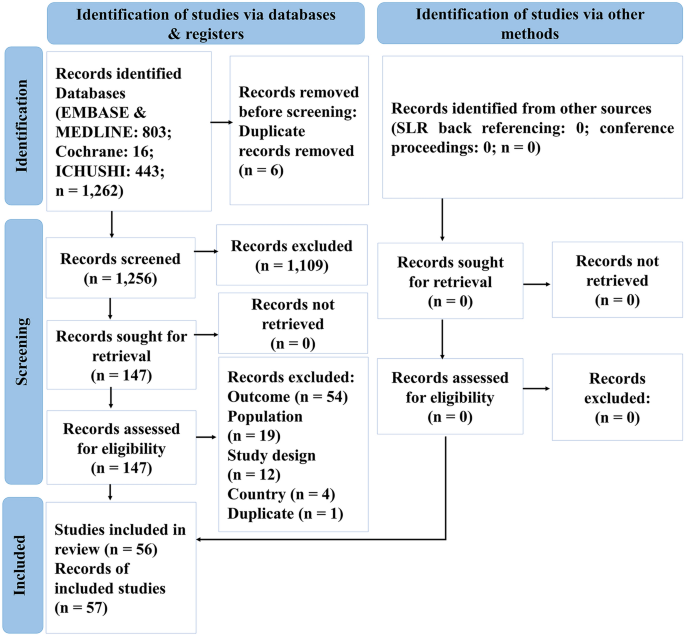
PRISMA flow diagram for SLR. PRISMA Preferred Reporting Items for Systematic Reviews and Meta-Analyses, SLR systematic literature review
Study Characteristics
For the SLR, a total of 56 studies (35 in English and 21 in Japanese) were included. The details of these studies are presented in Supplementary Table 5. All studies were conducted in Japan, with the majority being conducted in single-center settings (62.5%), followed by multicenter settings (33.9%) and unclear settings (3.6%). Fifty-three of the 56 studies (94.6%) were cohort studies, two (3.6%) were case-control studies, and one (1.8%) was a cross-sectional study. Twenty-two studies (39.3%) compared the AMR group with the non-AMR group. The overall sample size ranged from 33 to 7,772,050 participants, while the sample size for resistant populations ranged from 12 to 93,838 participants. Fourteen of the 56 studies (25.0%) included patients with BSI, nine (16.1%) included patients with pneumonia, four (7.1%) included patients with surgical site infection, and two studies each (3.6%) included patients with urinary tract infection and sepsis. One study each (1.8%) enrolled patients infected with vertebral osteomyelitis and invasive pneumococcal disease, and the remaining twenty-three studies (41.1%) included patients with multiple infections. Twenty-nine (51.8%) of the 56 studies were on MRSA, and S. aureus was the most common causative pathogen (53.6%). Table 2 presents details of the study characteristics.
Baseline Patient Characteristics
Supplementary Table 6 presents the baseline characteristics of the patients in the 56 included studies. The median age distribution of the population ranged from 60 to 89 years. The average body mass index (BMI) varied between 20.8 and 22.9 kg/m 2 . There were marginally more male than female participants. The majority of the population in this study included patients with MRSA infections. Average Sequential Organ Failure Assessment (SOFA) scores ranged between 4.7 and 8.5. A total of 51.8% of the patients had a SOFA score ≥ 2, indicating that the infection was associated with an increased risk of death or prolonged hospital stay. The median Charlson Comorbidity Index (CCI) score ranged from 0.99 to 3.9. The proportion of patients with CCI scores ≥ 3 and ≥ 2 was greater in the resistant group (69.0% and 45.4%, respectively) than in the susceptible group (35.0% and 35.9%, respectively). The most prevalent comorbidity was diabetes.
Clinical Outcomes
In-hospital mortality.
Forty studies reported in-hospital mortality (which includes 7-, 28-, 30-, and 90-day mortality) [ 26 , 27 , 28 , 29 , 30 , 31 , 32 , 33 , 34 , 35 , 36 , 37 , 38 , 39 , 40 , 41 , 42 , 43 , 44 , 45 , 46 , 47 , 48 , 49 , 50 , 51 , 52 , 53 , 54 , 55 , 56 , 57 , 58 , 59 , 60 , 61 , 62 , 63 , 64 , 65 ]. See Supplementary Table 7 for more details and other results of the SLR.
Twenty-four studies evaluated MRSA [ 42 , 43 , 44 , 45 , 46 , 47 , 48 , 49 , 50 , 51 , 52 , 53 , 54 , 55 , 56 , 57 , 58 , 59 , 60 , 61 , 62 , 63 , 64 , 65 ], and of those, seventeen studies reported in-hospital mortality, which ranged from 0% [ 54 , 59 ] to 73.0% [ 44 ]. The MRSA group had significantly higher in-hospital mortality compared with the non-MRSA group (31.2% vs. 11.6%, p < 0.001) [ 48 ] and the methicillin-susceptible S. aureus (MSSA) group (36.7% vs. 15.0%, p = 0.012) [ 62 ]. In 2016, Uematsu et al. compared in-hospital mortality between an anti-MRSA group and a control group and found that the anti-MRSA group had significantly higher in-hospital mortality (22.6% vs. 6.2%, p < 0.001, respectively) than the control group [ 56 ].
Three studies [ 39 , 40 , 41 ] evaluated carbapenem-resistant patients, and the in-hospital mortality rate ranged from 8.1 to 36.4% [ 40 ]. In a 2022 study by Imai et al., the carbapenem-resistant group had higher in-hospital mortality than the susceptible group; however, the difference was not statistically significant (25.6% vs. 21.9%, p = 0.407, respectively) [ 41 ].
A significant association among lower BMI, lower Barthel Index, higher Hugh-Jones grade, higher A-DROP score, C-reactive protein ≥ 20 mg/dl or infiltration of at least two-thirds of one lung, mechanical ventilation at admission, interstitial lung disease, aspiration pneumonia, a high CCI score, a high SOFA score, and having a vancomycin minimum inhibitory concentration ≥ 1.5 µg/ml was observed with increased in-hospital mortality in the MRSA group. Meanwhile, in carbapenem-resistant patients, older age, male gender, immunosuppressive drug use, pneumonia, sepsis, and a CCI score ≥ 1 were significantly associated with increased in-hospital mortality.
Length of Stay
A total of thirty studies reported LOS [ 26 , 27 , 31 , 40 , 41 , 42 , 43 , 47 , 49 , 52 , 54 , 55 , 56 , 58 , 62 , 63 , 65 , 66 , 67 , 68 , 69 , 70 , 71 , 72 , 73 , 74 , 75 , 76 , 77 , 78 ], the details and results of which are presented in Supplementary Table 8. Sixteen studies investigated MRSA infections [ 42 , 43 , 47 , 49 , 52 , 54 , 55 , 56 , 58 , 62 , 63 , 65 , 66 , 68 , 69 , 76 ], for which the mean LOS ranged from 8.6 [ 54 ] to 123.6 days [ 68 ]. The MRSA population had a significantly longer LOS than the non-MRSA group (35.0 days vs. 14.0 days, p < 0.001, respectively) [ 63 ].
Carbapenem-resistant groups [ 40 , 41 , 67 , 72 , 74 , 77 ] were reported in six studies, and the median LOS ranged from 14.0 to 83.0 days [ 40 ]. Imai et al. demonstrated in 2022 that carbapenem-resistant infections had a significantly longer LOS than carbapenem-susceptible infections (64.0 days vs. 46.0 days, p < 0.001, respectively), and even after adjusting with the inverse probability of treatment weight method, a similar trend was observed (median: 63.0 days vs. 51.0 days, p = 0.004, respectively) [ 41 ].
Economic Outcomes
Direct medical costs.
In total, fifteen studies reported direct medical costs [ 41 , 56 , 58 , 59 , 62 , 63 , 65 , 67 , 68 , 69 , 70 , 71 , 73 , 79 , 80 ]. Supplementary Table 9 presents the details and results of the studies. Nine studies evaluated direct medical costs in the MRSA population [ 56 , 58 , 59 , 62 , 63 , 65 , 68 , 69 , 80 ] with a median hospitalization cost ranging from $496 [ 62 ] to $29,596 [ 65 ]. The median hospitalization cost in the MRSA group was significantly higher than that in the non-MRSA group ($12,156 vs. $4665, p < 0.001, respectively) [ 63 ], as well as in the MSSA group ($26,035 vs. $19,823, p = 0.036, and mean: $15,762 vs. $14,152, p < 0.001, both respectively) [ 58 ]. Tsuzuki et al., in 2021, also evaluated the 5-year total intervention cost (including drug, transplantation, radiation, surgery, and blood transfusion costs), which was $475,059 in the MRSA group and $344,418 in the MSSA group [ 62 ].
Two studies evaluated the costs in the carbapenem-resistant population [ 41 , 67 ]. The average hospitalization cost per patient in the carbapenem-resistant group was higher than that in the carbapenem-sensitive group ($1648 vs. $532, p < 0.001, respectively) [ 67 ]. In the carbapenem-resistant group, the median costs for consultation ($163 vs. $143, p = 0.238, respectively), laboratory tests ($2498 vs. $1845, p = 0.002, respectively), and hospital stay ($14,307 vs. $10,560, p < 0.001, respectively) were higher than those in the carbapenem-sensitive group [ 41 ]. In addition, the cost of the intervention, which includes medications and surgeries, was higher in the carbapenem-resistant group than in the carbapenem-sensitive group [ 41 , 67 ].
Amount of Antibiotic Usage
Only four studies [ 50 , 55 , 77 , 80 ] reported the amount of antibiotic usage; hence, very few data were available. Supplementary Table 10 presents the details and results of the studies. According to a study published in 2015 by Suzuki et al., between 2006 and 2008, the mean amount of vancomycin in MRSA patients was high (2,591.6 g and 2,563.5 g per year, respectively). In contrast, the amount decreased between 2007 and 2009 (2348.8 g and 2384.5 g, respectively). In a study by Ogasawara et al. in 2012, 20 of the 33 patients treated with meropenem were resistant to Pseudomonas aeruginosa . The daily dose of meropenem for the resistant group was 1, and for the non-resistant group, it was 1.5 (the unit of measurement was not specified) [ 77 ].
Duration of Antibiotics
In total, twelve studies reported the duration of antibiotics [ 37 , 38 , 49 , 55 , 59 , 63 , 73 , 74 , 75 , 77 , 79 , 81 ]. Supplementary Table 11 presents the details and results of the studies. The median duration of antibiotic use in MRSA patients ranged from 10.0 to 21.5 days [ 49 , 55 ], whereas for ESBL bacteremia, it ranged from 9.0 to 15.9 days [ 38 , 75 ]. In those studies, the duration of antibiotic use was reported for the MRSA (median: 24.0 days, p < 0.001) [ 63 ], carbapenem-resistant (mean: 14.9 days) [ 74 ], and meropenem-resistant (median: 9.0 days, p > 0.05) [ 77 ] populations, which required longer antibiotic treatment than the non-MRSA (median: 9.0 days), non-carbapenem-resistant (mean: 11.0 days), and non-meropenem-resistant (median: 7.0 days) populations.
Pooled Clinical Outcomes
A total of eight studies from the SLR were included for the MA, of which four [ 41 , 56 , 62 , 63 ], seven [ 41 , 56 , 62 , 63 , 72 , 75 , 77 ], and three [ 41 , 56 , 62 ] studies evaluated in-hospital mortality, LOS, and direct medical costs, respectively. The outcomes of the amount and the duration of antibiotics were not evaluated because of a very limited number of eligible studies.
Among the 20,387 patients from the four studies [ 41 , 56 , 62 , 63 ], the pooled estimate showed a statistically significantly higher mortality in the AMR group compared with the non-AMR group for both the FE (OR 3.19, 95% CI 2.92–3.48) and RE models (OR 2.25, 95% CI 1.34–3.79, I 2 = 89%, τ 2 = 0.2257, p < 0.01) (Fig. 2 ). The funnel plot of in-hospital mortality is shown in Supplementary Fig. 1. The plot of in-hospital mortality represents bias as the dots outside of the triangle.
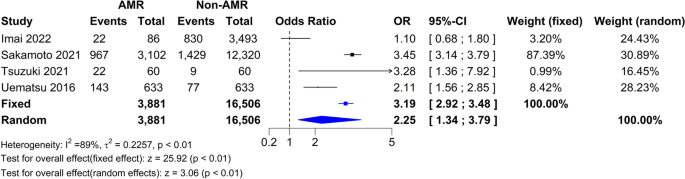
Forest plot of in-hospital mortality. AMR antimicrobial resistance, CI confidence interval, OR odds ratio
In-Hospital Mortality Sensitivity Analyses
A series of sensitivity analyses were conducted after excluding the carbapenem-resistant group. Analysis conducted after excluding the carbapenem-resistant population retained only the MRSA population [ 56 , 62 , 63 ], and the results suggested higher mortality in the AMR group versus the non-AMR group, with statistically significant ORs for the FE (OR 3.31, 95% CI 3.02–3.61) and RE (OR 2.84, 95% CI 1.95–4.14; I 2 = 79%, τ 2 = 0.0735, p < 0.01) models (Supplementary Fig. 2).
Additionally, a sensitivity analysis conducted after excluding the study that might include other antimicrobial resistance mechanisms [ 63 ], and it showed similar results (Supplementary Figs. 3 and 4). This study was defined as an outlier study based on the influence analysis.
Among the 20,662 patients from the seven studies [ 41 , 56 , 62 , 63 , 72 , 75 , 77 ], the pooled estimate showed a statistically significantly higher LOS in the AMR group versus the non-AMR group for FE (SMD 1.27, 95% CI 1.23–1.31) and a higher LOS in the AMR group versus the non-AMR group for RE (SMD 0.37, 95% CI − 0.09–0.84, I 2 = 99%, τ 2 = 0.3600, p < 0.01) models (Fig. 3 ). The funnel plot of length of stay was shown in Supplementary Fig. 5. The plot of length of stay represents bias as the dots outside of the triangle.
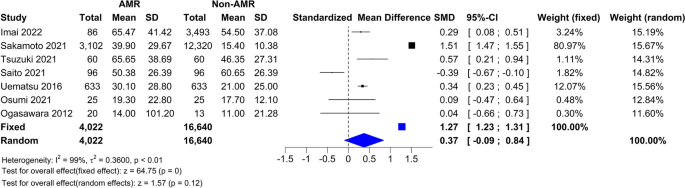
Forest plot of LOS. AMR antimicrobial resistance, CI confidence interval, LOS length of stay, SD standard deviation, SMD standardized mean difference
Length of Stay Sensitivity Analyses
A series of sensitivity analyses was conducted after excluding (1) MRSA, ESBL, and meropenem populations and (2) carbapenem-resistant, ESBL, and meropenem populations. The results of the sensitivity analysis conducted after excluding the carbapenem-resistant, ESBL, and meropenem populations retained only the MRSA population [ 56 , 62 , 63 ] (Supplementary Fig. 6). The results showed that the AMR group had a significantly longer LOS compared with the non-AMR group (for FE and RE models, respectively, SMD 1.35, 95% CI 1.31–1.39 and SMD 0.81, 95% CI 0.10–1.53, I 2 = 99%, τ 2 = 0.3905, p < 0.01). The results of the sensitivity analysis conducted after excluding the MRSA, ESBL, and meropenem populations retained only the carbapenem-resistant population [ 41 , 72 ] (Supplementary Fig. 7). The results showed that the AMR group had a longer LOS compared with the non-AMR group; however, the results were not statistically significant (for FE and RE models, respectively, SMD 0.05, 95% CI − 0.12 to 0.22 and SMD − 0.04, 95% CI − 0.71 to 0.63, I 2 = 93%, τ 2 = 0.2162, p < 0.01).
Additionally, a sensitivity analysis conducted after excluding the study [ 63 ] which might include other antimicrobial resistance mechanisms showed similar results (Supplemental Figs. 8–10). This study was defined as an outlier study based on the influence analysis.
Direct Medical Cost
Among the 4965 patients from the three studies [ 41 , 56 , 62 ], the pooled estimate showed a statistically significant increase in direct medical costs among the AMR groups compared with the non-AMR groups (for both FE and RE models, SMD 0.53, 95% CI 0.43–0.62, I 2 = 0.0%, τ 2 = 0.0, p = 0.88) (Fig. 4 ). The funnel plot of direct medical cost is shown in Supplementary Fig. 11. The plot did not show bias with the limitation of small sample size (i.e., study number) included in MA.

Forest plot of direct medical cost in US dollar. AMR antimicrobial resistance, CI confidence interval, SD standard deviation, SMD standardized mean difference, US United States
Direct Medical Cost Sensitivity Analyses
The sensitivity analysis was conducted after excluding the carbapenem-resistant population retained only the MRSA population [ 56 , 62 ] (Supplementary Fig. 12). The analysis showed significantly higher direct medical costs for the AMR group (for both FE and RE models, SMD 0.54, 95% CI 0.43–0.65, I 2 = 0.0%, τ 2 = 0.0, p = 0.71) compared to the non-AMR group.
This SLR and MA were conducted to determine the clinical and economic burden of AMR in Japan. In-hospital mortality and LOS were the clinical outcomes analyzed, while direct medical costs and the duration and amount of antibiotics were the economic outcomes.
Most of the studies (53.6%) included in the SLR evaluated patients with S. aureus infections. In a previous global SLR study, S. aureus was the most identified causative bacterium (23.4%) [ 82 ]. In Japan, nearly half of the cases were confirmed to be S. aureus [ 82 ]. The newly published Japanese National Action Plan on Antimicrobial Resistance (AMR) 2023–2027 reported that, as of 2020, the MRSA rate in Japan (48.1%) was higher compared to the EU (16.7%) and other developed countries [ 83 ]. In addition, this study found only two studies targeting vancomycin resistance. The reason for this is thought to be that the prevalence of vancomycin-resistant enterococci (VRE) is extremely low in Japan. In the 2022 report, the proportion of VRE in Enterococcus faecium was 2.6% [ 7 ]. On the other hand, the percentage of VRE in Europe and the US was reported to be 16.8% and 82.1%, respectively [ 84 , 85 ]. Additionally, AMR-related mortality rates have been reported to vary by region, with rates tending to be higher in developing countries [ 5 ]. This is thought to be influenced not only by the prevalence of AMR but also by access to medical care and the state of public health. In this way, to understand the burden of disease caused by AMR in a specific country or region and to compare existing research results, it is necessary to consider differences in the prevalence of AMR and other influences.
In Japan, a 2019 domestic survey reported that approximately 8000 people died in 2017 because of MRSA and FQREC, which are two of the most common drug-resistant bacteria [ 6 ]. Japan's AMR Countermeasure Action Plan 2016–2020 set a goal of “reducing the methicillin resistance rate of S. aureus to 20% or less in 2020,” but the actual annual rate of decrease was only 2% [ 86 ]. On the other hand, the UK has achieved an annual decrease rate of 5%. By thoroughly implementing infection prevention and control, and promoting the appropriate use of antimicrobials, it has been confirmed that Japan aims to reduce the drug resistance rate to < 20%, which is the same level as in other developed countries. Under these circumstances, countermeasures against MRSA are urgently needed in Japan, and this study was considered to be extremely useful for understanding the clinical and economic burden of AMR and MRSA in Japan.
According to our results, the MRSA group had significantly higher in-hospital mortality, LOS, and median or average hospitalization cost relative to the non-MRSA, MSSA, and control groups [ 51 , 56 , 58 , 62 , 63 , 65 ]. Even after adjusting for multiple variables such as age, sex, CCI, Barthel Index, nosocomial infection, BSI, ICU admission, surgery, and submission dates of positive S. aureus cultures, the correlation remained statistically significant [ 58 ]. Published results from developed countries were also in agreement with our results, in that the median LOS was longer for the MRSA group than for the MSSA group (9 days vs. 7 days, p = 0.045 from the US [ 87 ], respectively, and 22.5 days vs. 14 days from Canada [ 88 ], respectively).
The carbapenem-resistant population displayed a higher in-hospital mortality rate than the non-resistant population; however, the results were not statistically significant because the resistant group had a limited sample size [ 41 ], but LOS in the carbapenem-resistant population was significantly longer than that in the non-resistant population [ 58 ]. The multi-drug resistant (MDR) population also had a significantly longer LOS compared with the non-MDR population [ 78 ]. Hospitalization costs were also greater in the carbapenem-, penicillin-, oxacillin-, cephalosporin-, fluroquinolone-, and gentamicin-resistant groups than in the respective non-resistant groups [ 41 , 67 , 70 , 73 ]. Similar reviews conducted by Dadgostar et al. in 2019 [ 89 ] and Pulingam et al. in 2022 [ 3 ] also reported that AMR imposes a significant economic burden.
The results of our MA revealed that in-hospital mortality, hospital stays, and direct medical costs were significantly greater in the AMR group relative to the non-AMR group. Our results were consistent with another MA conducted by Poudel et al. in 2023, which reported that the resistant group had significantly higher mortality (OR 1.844 [95% CI 1.187–2.865]), LOS (mean: 7.4 days [95% CI 3.4–11.4]), and readmission (OR 1.492 [95% CI 1.231–1.807]). The same study also reported that costs attributable to the resistant group were higher in the resistant group compared with the non-resistant group [ 90 ]. Similarly, the MAs conducted by Cosgrove et al. in 2003 [ 91 ] (OR = 1.93, 95% CI 1.54–2.42, p < 0.001) and Rödenbeck et al. in 2023 [ 92 ] (OR 2.29, 95% CI 1.91–2.75) also suggested that the resistant group had significantly higher mortality than the susceptible group. Furthermore, the SLR conducted by Naylor et al. in 2018 [ 82 ] demonstrated that the resistant population had higher mortality and greater costs compared with the non-resistant population.
Several SLRs and MAs on the disease burden of AMR have been conducted globally [ 82 , 90 , 93 , 94 ]. These studies highlight that the global burden of AMR is substantial from both economic and clinical perspectives [ 90 ]. Resistant bacterial infections are associated with significant mortality [ 93 ]. There is considerable variability in burden estimates, which can lead to inaccurate intervention evaluations and poor policy or investment decisions [ 82 ]. AMR decision-making and policy should be driven by reliable, unbiased estimates of the effect size, which can be achieved through MAs [ 94 ].
Substantial heterogeneity between studies was observed in our MA because of the considerable diversity in antibiotic resistance. Pooled estimates were calculated to integrate studies regardless of the type of antibiotic resistance for the AMR and non-AMR comparisons, and the sensitivity analysis was conducted by sub-grouping antibiotic resistance types. While the MA results of in-hospital mortality and LOS in the pooled estimates showed high heterogeneity, a few results from the sensitivity analysis showed low heterogeneity. On the other hand, the heterogeneity in the pooled estimates and the sensitivity analysis of direct medical costs was consistent.
Although there is limited evidence in this literature review, most of the eligible studies used for the MA were published after 2016; therefore, there is a possibility of data overlapping among the multicenter studies involved in the MAs. For studies that adjusted patient background for both resistance and non-resistance groups, there is a possibility that the patients showed resistance to other antibiotics than those targeted in the study. In Japan, during the study period, the treatment environment has not significantly changed because of almost no new antibiotics being available; however, the prevalence of resistant bacteria may have been changing.
Conclusions
In Japan, AMR is associated with a significant clinical and economic burden that impacts both patients and society. There is a pressing need for payers, providers, and policymakers to make informed decisions regarding interventions to mitigate AMR-associated burdens. However, since AMR strains and resistance rates show country-wise diversity, appropriately assessing the burden of AMR by referring to existing data and reports is of utmost importance.
Data Availability
All data generated or analyzed during this study are included in this published article as supplementary information files. Except for confidential data associated with data analysis, all data are available from the corresponding author upon reasonable request.
World Health Organization. Antimicrobial resistance. 2021. Available from: https://www.who.int/news-room/fact-sheets/detail/antimicrobial-resistance .
Altarac D, Gutch M, Mueller J, Ronsheim M, Tommasi R, Perros M. Challenges and opportunities in the discovery, development, and commercialization of pathogen-targeted antibiotics. Drug Discov Today. 2021;26(9):2084–9.
Article CAS PubMed Google Scholar
Pulingam T, Parumasivam T, Gazzali AM, Sulaiman AM, Chee JY, Lakshmanan M, et al. Antimicrobial resistance: prevalence, economic burden, mechanisms of resistance and strategies to overcome. Eur J Pharm Sci. 2022;170: 106103.
O’Neill J. Tackling drug-resistant infections globally: final report and recommendations: The Review on Antimicrobial Resistance. 2016. Available from: https://amr-review.org/sites/default/files/160518_Final%20paper_with%20cover.pdf .
Antimicrobial RC. Global burden of bacterial antimicrobial resistance in 2019: a systematic analysis. Lancet. 2022;399(10325):629–55.
Article Google Scholar
Tsuzuki S, Matsunaga N, Yahara K, Gu Y, Hayakawa K, Hirabayashi A, et al. National trend of blood-stream infection attributable deaths caused by Staphylococcus aureus and Escherichia coli in Japan. J Infect Chemother. 2020;26(4):367–71.
Japan Nosocomial Infections Surveillance (JANIS). Annual Open Report 2022. 2023. Available from: https://janis.mhlw.go.jp/english/report/open_report/2022/3/1/ken_Open_Report_Eng_202200_clsi2012.pdf .
Van Katwyk SR, Grimshaw JM, Hoffman SJ. Ten Years of inaction on antimicrobial resistance: an environmental scan of policies in Canada from 2008 to 2018. Healthc Policy. 2020;15(4):48–62.
PubMed PubMed Central Google Scholar
Matsumoto T, Darlington O, Miller R, Gordon J, McEwan P, Ohashi T, et al. Estimating the economic and clinical value of reducing antimicrobial resistance to three gram-negative pathogens in Japan. J Health Econ Outcomes Res. 2021;8(2):64–75.
Article PubMed PubMed Central Google Scholar
AMED Public and Private Partnerships for Infectious Diseases R&D. Priority Pathogens Lists for R&D of New Antibiotics (2021 version). 2021. Available from: https://id3catalyst.jp/apid/en/list.html .
The Cochrane Collaboration. Cochrane handbook for systematic reviews of interventions. 2023. Available from: https://training.cochrane.org/handbook/current .
Centre for Reviews and Dissemination, University of York. Systematic Reviews: CRD's guidance for undertaking reviews in health care. 2009. Available from: https://www.york.ac.uk/media/crd/Systematic_Reviews.pdf .
Page MJ, McKenzie JE, Bossuyt PM, Boutron I, Hoffmann TC, Mulrow CD, et al. The PRISMA 2020 statement: an updated guideline for reporting systematic reviews. BMJ. 2021;372: n71.
Amir-Behghadami M, Janati A. Population, Intervention, Comparison, Outcomes and Study (PICOS) design as a framework to formulate eligibility criteria in systematic reviews. Emerg Med J. 2020;37(6):387.
Article PubMed Google Scholar
Medical Data Vision. Part #1: 2022 Reform of Medical Fee Structure; what you see, what you don’t. 2022. Available from: https://en.mdv.co.jp/ebm/column/article/13.html .
Organisation for Economic Co-operation and Development. OECD Exchange rates. 2022. Available from: https://data.oecd.org/conversion/exchange-rates.htm .
Ma LL, Wang YY, Yang ZH, Huang D, Weng H, Zeng XT. Methodological quality (risk of bias) assessment tools for primary and secondary medical studies: what are they and which is better? Mil Med Res. 2020;7(1):7.
Wells GA, Wells G, Shea B, Shea B, O'Connell D, Peterson J, et al., editors. The Newcastle-Ottawa Scale (NOS) for assessing the quality of nonrandomised studies in meta-analyses. 2014.
Tyndall J. The AACODS checklist. 2010. Available from: https://www.library.sydney.edu.au/research/systematic-review/downloads/AACODS_Checklist.pdf .
Cochran WG. The combination of estimates from different experiments. Biometrics. 1954;10(1):101–29.
McGrath S, Sohn H, Steele R, Benedetti A. Meta-analysis of the difference of medians. Biom J. 2020;62(1):69–98.
Harrer M, Cuijpers P, Furukawa TA, Ebert DD. Doing meta-analysis with R: a hands-on guide. 1st ed. Boca Raton: Chapman & Hall/CRC Press; 2021. p. 2021.
Book Google Scholar
Higgins JP, Thompson SG. Quantifying heterogeneity in a meta-analysis. Stat Med. 2002;21(11):1539–58.
Sterne JA, Sutton AJ, Ioannidis JP, Terrin N, Jones DR, Lau J, et al. Recommendations for examining and interpreting funnel plot asymmetry in meta-analyses of randomised controlled trials. BMJ. 2011;343: d4002.
Egger M, Davey Smith G, Schneider M, Minder C. Bias in meta-analysis detected by a simple, graphical test. BMJ. 1997;315(7109):629–34.
Article CAS PubMed PubMed Central Google Scholar
Suehiro T, Takahashi Y, Okushita Y, Shiraishi A, Ogawa M, Imozuka K, et al. Detection of MRSA and ESBL-producing bacteria in emergency hospitalized patients [Japanese] [臨牀指針 緊急入院患者における MRSA および ESBL 産生菌の検出状況について]. Jpn J Clin Exp Med. 2012;89(6):813–5.
Google Scholar
Kohno J, Kawamura T, Kikuchi A, Akaishi T, Takayama S, Ishii T. A Japanese traditional medicine Hochuekkito promotes negative conversion of vancomycin-resistant Enterococci. Sci Rep. 2021;11(1):11300.
Hanada S, Iwata S, Kishi K, Morozumi M, Chiba N, Wajima T, et al. Host factors and biomarkers associated with poor outcomes in adults with invasive pneumococcal disease. PLoS One. 2016;11(1): e0147877.
Yamagata A, Ito A, Nakanishi Y, Ishida T. Prognostic factors in nursing and healthcare-associated pneumonia. J Infect Chemother. 2020;26(6):563–9.
Shindo Y, Ito R, Kobayashi D, Ando M, Ichikawa M, Shiraki A, et al. Risk factors for drug-resistant pathogens in community-acquired and healthcare-associated pneumonia. Am J Respir Crit Care Med. 2013;188(8):985–95.
Hamada Y, Magarifuchi H, Oho M, Kusaba K, Nagasawa Z, Fukuoka M, et al. Clinical features of enterococcal bacteremia due to ampicillin-susceptible and ampicillin-resistant enterococci: an eight-year retrospective comparison study. J Infect Chemother. 2015;21(7):527–30.
Hattori H, Maeda M, Nagatomo Y, Takuma T, Niki Y, Naito Y, et al. Epidemiology and risk factors for mortality in bloodstream infections: a single-center retrospective study in Japan. Am J Infect Control. 2018;46(12):e75–9.
Kainuma A, Momiyama K, Kimura T, Akiyama K, Inoue K, Naito Y, et al. An outbreak of fluoroquinolone-resistant Pseudomonas aeruginosa ST357 harboring the exoU gene. J Infect Chemother. 2018;24(8):615–22.
Yamada K, Imoto W, Yamairi K, Shibata W, Namikawa H, Yoshii N, et al. The intervention by an antimicrobial stewardship team can improve clinical and microbiological outcomes of resistant gram-negative bacteria. J Infect Chemother. 2019;25(12):1001–6.
Hanaoka N, Etoh S, Fujiyoshi N, Hirata T, Omura K. Antimicrobial therapy for multi-drug resistant Pseudomonas aeruginosa (MDRP) infection in patients with severe burns, admitted to Chiba emergency medical center [Japanese] [当医療センターでの重症熱傷患者における多剤耐性緑膿菌感染症に対しての抗菌薬療法]. Jpn J Intensive Care Med. 2013;37(4):311–8.
Nagao M. A multicentre analysis of epidemiology of the nosocomial bloodstream infections in Japanese university hospitals. Clin Microbiol Infect. 2013;19(9):852–8.
Ueda S, Okada M, Ito T, Kobayashi K, Takahashi T, Marumo S, et al. Comparative study of the effects of different dosing frequencies of cefmetazole on urinary tract infection caused by extended-spectrum β-lactamase-producing Escherichia coli [Japanese] [基質特異性拡張型 β-ラクタマーゼ産生大腸菌に起因する尿路感染症に対するセフメタゾールの投与回数の違いによる有効性の比較検討]. J Jpn Soc Hosp Pharm. 2022;58(2):167–72.
Mitsuboshi S, Tsuruma N, Watanabe K, Takahashi S, Ito A, Nakashita M, et al. Advanced age is not a risk factor for mortality in patients with Bacteremia caused by extended-spectrum β-lactamase-producing organisms: a multicenter cohort Study. Jpn J Infect Dis. 2020;73(4):288–92.
Tetsuka N, Hirabayashi A, Matsumoto A, Oka K, Hara Y, Morioka H, et al. Molecular epidemiological analysis and risk factors for acquisition of carbapenemase-producing Enterobacter cloacae complex in a Japanese university hospital. Antimicrob Resist Infect Control. 2019;8:126.
Hayakawa K, Nakano R, Hase R, Shimatani M, Kato H, Hasumi J, et al. Comparison between IMP carbapenemase-producing Enterobacteriaceae and non-carbapenemase-producing Enterobacteriaceae: a multicentre prospective study of the clinical and molecular epidemiology of carbapenem-resistant Enterobacteriaceae. J Antimicrob Chemother. 2020;75(3):697–708.
Imai S, Inoue N, Nagai H. Economic and clinical burden from carbapenem-resistant bacterial infections and factors contributing: a retrospective study using electronic medical records in Japan. BMC Infect Dis. 2022;22(1):581.
Furukawa D, Asai S, Nakgohri T. Trend of methicillin - resistant Staphylococcus aureus isolation in gastroenterological surgery] [Japanese] [消化器外科におけるメチシリン耐性黄色ブドウ球菌検出の動向]. J Jpn Soc Surg Infect. 2012;9(2):143–50.
Sano T. MRSA infection of department of respiratory medicine [Japanese] [本学における MRSA 感染症の現状 呼吸器内科における MRSA 感染症の現状]. J Med Soc Toho Univ. 2012;59(6):320–2.
Iwabuchi H, Oguchi K. Actual status of sepsis and countermeasures [Japanese] [4. 敗血症の実態と対策]. J Jpn Soc Dial Ther. 2013;46(2):176–8.
The Japanese Society of Intensive Care Medicine CoSR. 2007 JSICM Sepsis 1st Registry: management of severe sepsis and septic shock in Japan. J Jpn Soc Intensive Care Med. 2013;20(2):329–34.
Nakatsuka Y, Morimoto C, Yasuda I, Tsuji T, Kaji Y, Yasuda T, et al. An analysis of the correlation between guidelines-concordant treatment and the treatment outcome on nursing and healthcare-associated pneumonia patients. Kansenshogaku Zasshi. 2013;87(6):739–45.
Sasaki S, Kuwana Y, Chimori A, Yoshida K. Epidemiological characterization of clinically isolated MRSA in Kinki Central Hospital, mainly for vancomycin MIC [Japanese] [近畿中央病院における MRSA 臨床分離株の疫学調査]. Med J Kinki Central Hosp. 2015;35:25–32.
Kawamura I, Sekiya N, Araoka H, Nei T, Harada S, Kurai H, et al. Surveillance of Methicillin-resistant Staphylococcus aureus in 7 Japanese Hospitals, 2015. Jpn J Infect Prevent Control. 2017;32(3):135–40.
Fukushima M, Maeda A, Soga H, Tomono Y, Machida S, Oi Z, et al. Effects of AST activity on MRSA bacteremia using the automatic simultaneous multi-item genetic testing system (Verigene system) [Japanese] [自動多項目同時遺伝子関連検査システム (Verigeneシステム) を利用した MRSA 菌血症に対する AST 活動の効果]. J Jpn Soc Hosp Pharm. 2022;58(5):545–50.
Mizokami F, Shibasaki M, Yoshizue Y, Noro T, Mizuno T, Furuta K. Pharmacodynamics of vancomycin in elderly patients aged 75 years or older with methicillin-resistant Staphylococcus aureus hospital-acquired pneumonia. Clin Interv Aging. 2013;8:1015–21.
Shoji H, Urakawa T, Watanabe K, Hirano T, Katsumi K, Ohashi M, et al. Clinical features, outcomes, and survival factor in patients with vertebral osteomyelitis infected by methicillin-resistant staphylococci. J Orthop Sci. 2016;21(3):282–6.
Isobe M, Uejima E, Seki M, Yamagishi Y, Miyawaki K, Yabuno K, et al. Methicillin-resistant Staphylococcus aureus bacteremia at a university hospital in Japan. J Infect Chemother. 2012;18(6):841–7.
Kaku N, Yanagihara K, Morinaga Y, Yamada K, Harada Y, Migiyama Y, et al. Influence of antimicrobial regimen on decreased in-hospital mortality of patients with MRSA bacteremia. J Infect Chemother. 2014;20(6):350–5.
Seki M, Takahashi H, Yamamoto N, Hamaguchi S, Ojima M, Hirose T, et al. Polymerase chain reaction-based active surveillance of MRSA in emergency department patients. Infect Drug Resist. 2015;8:113–8.
Shoji H, Maeda M, Shirakura T, Takuma T, Ugajin K, Fukuchi K, et al. More accurate measurement of vancomycin minimum inhibitory concentration indicates poor outcomes in meticillin-resistant Staphylococcus aureus bacteraemia. Int J Antimicrob Agents. 2015;46(5):532–7.
Uematsu H, Yamashita K, Kunisawa S, Fushimi K, Imanaka Y. The economic burden of methicillin-resistant Staphylococcus aureus in community-onset pneumonia inpatients. Am J Infect Control. 2016;44(12):1628–33.
Shime N, Saito N, Bokui M, Sakane N, Kamimura M, Shinohara T, et al. Clinical outcomes after initial treatment of methicillin-resistant Staphylococcus aureus infections. Infect Drug Resist. 2018;11:1073–81.
Uematsu H, Yamashita K, Mizuno S, Kunisawa S, Shibayama K, Imanaka Y. Effect of methicillin-resistant Staphylococcus aureus in Japan. Am J Infect Control. 2018;46(10):1142–7.
Yuasa A, Murata T, Imai K, Yamamoto Y, Fujimoto Y. Treatment procedures and associated medical costs of methicillin-resistant Staphylococcus aureus infection in Japan: a retrospective analysis using a database of Japanese employment-based health insurance. SAGE Open Med. 2019;7:2050312119871181.
Miyazaki T, Yanagihara K, Kakeya H, Izumikawa K, Mukae H, Shindo Y, et al. Daily practice and prognostic factors for pneumonia caused by methicillin-resistant Staphylococcus aureus in Japan: a multicenter prospective observational cohort study. J Infect Chemother. 2020;26(2):242–51.
Umemura Y, Ogura H, Takuma K, Fujishima S, Abe T, Kushimoto S, et al. Current spectrum of causative pathogens in sepsis: a prospective nationwide cohort study in Japan. Int J Infect Dis. 2021;103:343–51.
Tsuzuki S, Yu J, Matsunaga N, Ohmagari N. Length of stay, hospitalisation costs and in-hospital mortality of methicillin-susceptible and methicillin-resistant Staphylococcus aureus bacteremia in Japan. Public Health. 2021;198:292–6.
Sakamoto Y, Yamauchi Y, Jo T, Michihata N, Hasegawa W, Takeshima H, et al. In-hospital mortality associated with community-acquired pneumonia due to methicillin-resistant Staphylococcus aureus : a matched-pair cohort study. BMC Pulm Med. 2021;21(1):345.
Shimizu M, Mihara T, Ohara J, Inoue K, Kinoshita M, Sawa T. Relationship between mortality and molecular epidemiology of methicillin-resistant Staphylococcus aureus bacteremia. PLoS One. 2022;17(7): e0271115.
Uematsu H, Yamashita K, Kunisawa S, Fushimi K, Imanaka Y. Estimating the disease burden of methicillin-resistant Staphylococcus aureus in Japan: retrospective database study of Japanese hospitals. PLoS One. 2017;12(6): e0179767.
Aoyagi T, Kaito C, Sekimizu K, Omae Y, Saito Y, Mao H, et al. Impact of psm-mec in the mobile genetic element on the clinical characteristics and outcome of SCCmec-II methicillin-resistant Staphylococcus aureus bacteraemia in Japan. Clin Microbiol Infect. 2014;20(9):912–9.
Asai N, Sakanashi D, Suematsu H, Kato H, Hagihara M, Nishiyama N, et al. The epidemiology and risk factor of carbapenem-resistant enterobacteriaceae colonization and infections: case control study in a single institute in Japan. J Infect Chemother. 2018;24(7):505–9.
Fukuda H, Sato D, Iwamoto T, Yamada K, Matsushita K. Healthcare resources attributable to methicillin-resistant Staphylococcus aureus orthopedic surgical site infections. Sci Rep. 2020;10(1):17059.
Kashimura N, Kusachi S, Konishi T, Shimizu J, Kusunoki M, Oka M, et al. Impact of surgical site infection after colorectal surgery on hospital stay and medical expenditure in Japan. Surg Today. 2012;42(7):639–45.
Naylor NR, Yamashita K, Iwami M, Kunisawa S, Mizuno S, Castro-Sanchez E, et al. Code-sharing in cost-of-illness calculations: an application to antibiotic-resistant bloodstream infections. Front Public Health. 2020;8: 562427.
Obara H, Saitou J, Fukuda H. Increased burden on medical resources of penicillin-resistant streptococcus pneumoniae infections: estimates using JANIS data. Jpn J Infect Prevent Control. 2015;30(3):165–73.
Saito S, Hayakawa K, Tsuzuki S, Ishikane M, Nagashima M, Mezaki K, et al. A matched case-case-control study of the impact of clinical outcomes and risk factors of patients with IMP-type carbapenemase-producing carbapenem-resistant enterobacteriaceae in Japan. Antimicrob Agents Chemother. 2021;65(3). https://doi.org/10.1128/aac.01483-20 .
Uryu K, Nishiura S, Yamamoto T, Umakosi T, Nisida M, Suzuki M, et al. Changes in medical treatment fees for pneumococcal pneumonia in our hospital before and after the introduction of DPC. J Jpn Soc Clin Pathway. 2012;14(2):113–21.
Maeda M, Oto Y, Murayama J, Minemura A, Baba T, Yoshida H, et al. A study of risk factors for antibiotic selective pressure in Carbapenem-treated patients [Japanese] [カルバペネム系薬投与患者における耐性菌選択リスク因子の検討]. J Showa Univ Soc. 2014;74(1):67–72.
Osumi T, Tanaka D, Eguchi T, Imai T, Shimizu H, Sakai M, et al. retrospective study of initiation of empirical therapies out of the antibacterial spectrum for urinary tract infections presumed to be caused by extended spectrum β-lactamases producing bacteria [Japanese] [ESBL 産生菌が原因と推定される尿路感染症にスペクトラムが外れた経験的治療を開始したことが及ぼす影響の後方視的検討]. J Jpn Soc Hosp Pharm. 2021;57(11):1215–20.
Kobayashi H, Moriyama Y, Kurosu H. Report methicillin-resistant Staphylococcus aureus hospital infection surveillance for the fiscal year 2011 [Japanese] [報告 2011年度の Methicillin-resistant Staphylococcus aureus 病院感染症サーベイランス]. Jpn J Infect Prevent Control. 2013;28(3):178–9.
Ogasahara Y, Nagasaki N, Ohno K, Harino T, Yoshida T, Maruko M. Factors causing P. aeruginosa resistance in patients treated with meropenem [Japanese] [Meropenem 投与患者における緑膿菌耐性化因子の検討]. Jpn J Infect Prevent Control. 2012;27(6):419–24.
Kosuge Y, Nagashima G, Enomoto K, Kato A, Noda M, Morishima H, et al. Clinical characteristics of multidrug-resistant bacteria infection with stroke [Japanese] [脳卒中患者における多剤耐性菌感染症の臨床検討]. Neurosurg Emerg. 2013;18(2):173–6.
Fukatsu M, Umemura T, Mizuno T, Ohguchi H, Iwatsu S, Matsumoto S, et al. Positive impacts of antimicrobial stewardship on intial eradication success and drug costs of treatment for clarithromycin-reisistant helicobacter pyloriInfections [Japanese] [薬剤師によるクラリスロマイシン耐性 Helicobacter pylori 感染に対する抗菌薬適正使用支援が初回除菌率と薬剤費に与える影響]. Jpn J Pharm Health Care Sci. 2021;47(11):609–15.
Article CAS Google Scholar
Suzuki T, Tsuchiya M, Niwa T, Watanabe T, Ohta H, Fukako A, et al. Cost effectiveness of controlling healthcare-associated spread of methicillin-resistant Staphylococcus aureus [Japanese] [当院における MRSA 感染制御活動の経済的評価に関する検討]. Jpn J Infect Prevent Control. 2015;30(2):91–6.
Goto Y, Hayashi M, Akasaki J, Ito M, Yamamoto K, Sawa A, et al. Study of risk factors for carriage of vancomycin-resistant enterococcus [Japanese] [バンコマイシン耐性腸球菌の保菌におけるリスク因子の検討]. J Jpn Soc Hospital Pharm. 2014;4:499–502.
Naylor NR, Atun R, Zhu N, Kulasabanathan K, Silva S, Chatterjee A, et al. Estimating the burden of antimicrobial resistance: a systematic literature review. Antimicrob Resist Infect Control. 2018;7:58.
The Government of Japan. National Action Plan on Antimicrobial Resistance (AMR) 2023–2027. 2023. Available from: https://www.mhlw.go.jp/content/10900000/001096228.pdf .
Surveillance of antimicrobial resistance in Europe, 2020 data. 2020. Available from: https://www.ecdc.europa.eu/sites/default/files/documents/Surveillance-antimicrobial-resistance-in-Europe-2020.pdf .
Weiner-Lastinger LM, Abner S, Edwards JR, Kallen AJ, Karlsson M, Magill SS, et al. Antimicrobial-resistant pathogens associated with adult healthcare-associated infections: Summary of data reported to the National Healthcare Safety Network, 2015–2017. Infect Control Hosp Epidemiol. 2020;41(1):1–18.
The Government of Japan. National Action Plan on Antimicrobial Resistance (AMR) 2016–2020. 2016. Available from: https://www.mhlw.go.jp/content/10900000/0000138942.pdf .
Cosgrove SE, Qi Y, Kaye KS, Harbarth S, Karchmer AW, Carmeli Y. The impact of methicillin resistance in Staphylococcus aureus bacteremia on patient outcomes: mortality, length of stay, and hospital charges. Infect Control Hosp Epidemiol. 2005;26(2):166–74.
Thampi N, Showler A, Burry L, Bai AD, Steinberg M, Ricciuto DR, et al. Multicenter study of health care cost of patients admitted to hospital with Staphylococcus aureus bacteremia: impact of length of stay and intensity of care. Am J Infect Control. 2015;43(7):739–44.
Dadgostar P. Antimicrobial resistance: implications and costs. Infect Drug Resist. 2019;12:3903–10.
Poudel AN, Zhu S, Cooper N, Little P, Tarrant C, Hickman M, et al. The economic burden of antibiotic resistance: a systematic review and meta-analysis. PLoS One. 2023;18(5): e0285170.
Cosgrove SE, Sakoulas G, Perencevich EN, Schwaber MJ, Karchmer AW, Carmeli Y. Comparison of mortality associated with methicillin-resistant and methicillin-susceptible Staphylococcus aureus bacteremia: a meta-analysis. Clin Infect Dis. 2003;36(1):53–9.
Rodenbeck M, Ayobami O, Eckmanns T, Pletz MW, Bleidorn J, Markwart R. Clinical epidemiology and case fatality due to antimicrobial resistance in Germany: a systematic review and meta-analysis, 1 January 2010 to 31 December 2021. Euro Surveill. 2023. https://doi.org/10.2807/1560-7917.ES.2023.28.20.2200672 .
MacKinnon MC, Sargeant JM, Pearl DL, Reid-Smith RJ, Carson CA, Parmley EJ, et al. Evaluation of the health and healthcare system burden due to antimicrobial-resistant Escherichia coli infections in humans: a systematic review and meta-analysis. Antimicrob Resist Infect Control. 2020;9(1):200.
Wozniak TM, Barnsbee L, Lee XJ, Pacella RE. Using the best available data to estimate the cost of antimicrobial resistance: a systematic review. Antimicrob Resist Infect Control. 2019;8:26.
Download references
Medical Writing, Editorial, and Other Assistance.
The authors’ heartfelt appreciation goes to Aditya K. Kumar and Anil Dasari from IQVIA India, and Karin Matsumoto and Yawen Dai from IQVIA Solutions Japan for their systematic literature review support; Annoda Kumar and Rosario Vivek from IQVIA India for providing medical writing support; and Todd D. Taylor from IQVIA Solutions Japan for providing editorial assistance; all of which was funded by Pfizer Japan Inc. in accordance with the Good Publication Practice (GPP3) guidelines. ( http://www.ismpp.org/gpp3 ).
Authorship.
All authors met all four requirements for authorship as outlined by the International Committee of Medical Journal Editors (ICMJE). All authors read and approved the final version of the manuscript.
This work, including the journal’s Rapid Service Fee, was supported by Pfizer Japan Inc.
Author information
Authors and affiliations.
Department of Infectious Diseases, School of Medicine, International University of Health and Welfare, Narita, Japan
Tetsuya Matsumoto
Japan Access & Value, Pfizer Japan Inc., Shinjuku Bunka Quint Building, 3-22-7, Yoyogi, Shibuya-ku, Tokyo, 151-8589, Japan
Akira Yuasa & Naohiro Yonemoto
Real World Evidence Solutions & HEOR, IQVIA Solutions Japan G.K., Tokyo, Japan
Hiroyuki Matsuda & Dilinuer Ainiwaer
You can also search for this author in PubMed Google Scholar
Contributions
Tetsuya Matsumoto, Akira Yuasa, and Naohiro Yonemoto conceptualized and designed the study. Hiroyuki Matsuda and Dilinuer Ainiwaer were responsible for data analysis. All authors contributed to interpretation of the results, preparation and review of the manuscript, and approval of the final manuscript for publication.
Corresponding author
Correspondence to Akira Yuasa .
Ethics declarations
Conflict of interest.
The authors declare the following potential conflicts of interest with respect to the research, authorship and/or publication of this article: Tetsuya Matsumoto has been on the speakers’ bureau for Pfizer Japan Inc., KYORIN Pharmaceutical Co., Ltd., and MSD K.K. Akira Yuasa and Naohiro Yonemoto are full-time employees of Pfizer Japan Inc., and hold stocks and stock options from Pfizer Inc. Hiroyuki Matsuda and Dilinuer Ainiwaer are employees of IQVIA Solutions Japan G.K., which received funding from Pfizer Japan Inc. to undertake the research outlined in this study.
Additional information
Publisher's note.
Springer Nature remains neutral with regard to jurisdictional claims in published maps and institutional affiliations.
Prior Presentation: Akira Yuasa, Tetsuya Matsumoto. Systematic Literature Review on the Clinical and Economic Burdens of Antimicrobial Resistance in the Japanese Population. The 70th East Japan Chapter General Meeting of the Japanese Society of Chemotherapy, October 2023, Tokyo, Japan. Akira Yuasa, Naohiro Yonemoto, Hiroyuki Matsuda, et al. EPH230 Systematic Literature Review on the Clinical and Economic Burdens of Antimicrobial Resistance in the Japanese Population. ISPOR Europe 2023, November 2023, Copenhagen, Denmark.
Supplementary Information
Below is the link to the electronic supplementary material.
Supplementary file1 (PDF 3071 KB)
Rights and permissions.
Open Access This article is licensed under a Creative Commons Attribution-NonCommercial 4.0 International License, which permits any non-commercial use, sharing, adaptation, distribution and reproduction in any medium or format, as long as you give appropriate credit to the original author(s) and the source, provide a link to the Creative Commons licence, and indicate if changes were made. The images or other third party material in this article are included in the article's Creative Commons licence, unless indicated otherwise in a credit line to the material. If material is not included in the article's Creative Commons licence and your intended use is not permitted by statutory regulation or exceeds the permitted use, you will need to obtain permission directly from the copyright holder. To view a copy of this licence, visit http://creativecommons.org/licenses/by-nc/4.0/ .
Reprints and permissions
About this article
Matsumoto, T., Yuasa, A., Matsuda, H. et al. Burden of Antimicrobial Resistance in Japan: A Systematic Literature Review and Meta-Analysis. Infect Dis Ther (2024). https://doi.org/10.1007/s40121-024-00960-z
Download citation
Received : 21 December 2023
Accepted : 11 March 2024
Published : 25 April 2024
DOI : https://doi.org/10.1007/s40121-024-00960-z
Share this article
Anyone you share the following link with will be able to read this content:
Sorry, a shareable link is not currently available for this article.
Provided by the Springer Nature SharedIt content-sharing initiative
- Antimicrobial resistance
- Meta-Analysis
- Find a journal
- Publish with us
- Track your research
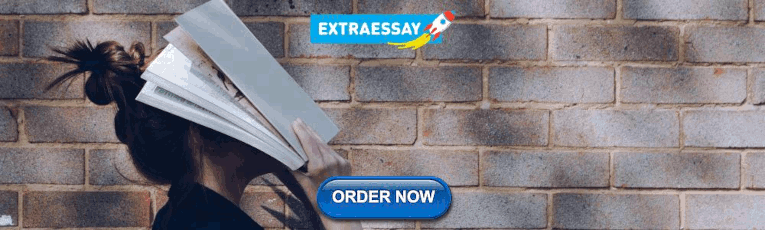
IMAGES
VIDEO
COMMENTS
The purpose of this study is, first, to descriptively investigate various financial risk measures used in the lodging financial literature by performing factor analysis and identifying four distinct risk groups. Second, this study examines the predictive ability of the four risk groups for lodging firm performance.
2. REVIEW OF LITERATURE According to the study of Myšková and Hájek (2017)[11], financial ratios are the most popular method of financial analysis due to their use as an input for complex mathematical models. Predictive models such as Altman model and Ohlson model are based on financial ratios. In
Abstract. Financial ratio is most important tool for accounting analysis. In this paper, researcher will study on ratio analysis, its usefulness, its effectiveness with using various past ...
Financial ratio as a variable and. The Efficiency of Financial Ratios Analysis to Evaluate Company's Profitability. Volume 2, Number 4, 2021, ISSN: Print 2735 -9344, Online 2735-9352 Page | 126 ...
2 Literature Review on Performance, Financial Performance and Its Determinants. ... Two methods of financial performance analysis have been often used: analysis based on financial ratios (making it possible to diagnose financial health of companies) and cash flow analysis (helping managers manage—for operational, financial and investment ...
Financial ratio is most important tool for accounting analysis. In this paper, researcher will study on ratio analysis, its usefulness, its effectiveness with using various past published papers and articles. This paper is based on review of literature. There are so many ratios are used by different user as research tool.
However, through financial ratio analysis, one may be able to work with these numbers in an organized fashion. Ratio analysis is a diagnostic tool that helps to identify problem areas and opportunities within a company. ... The Analysis and Use of Financial Ratios: A Review Article, Journal of Business Finance & Accounting, Vol. 14, No, 4 ...
The literature on financial statement analysis attempts to improve fundamental analysis and to identify market inefficiencies with respect to financial statement information. In this paper, I review the extant research on financial statement analysis. ... Jackson, Andrew B., Financial Statement Analysis: A Review and Current Issues (October 28 ...
Financial ratios are used for all kinds of purposes. These include the assessment of the ability of a firm to pay its debts, the evaluation of business and managerial success and even the statutory regulation ofa firm's performance. Not surprisingly they become norms and actually affect performance.' The traditional textbooks of financial analysis also emphasise the need for a firm to use ...
Review of Accounting Studies, 6, 109-154, 2001 C 2001 Kluwer Academic Publishers. Manufactured in The Netherlands. Ratio Analysis and Equity Valuation: From Research to Practice DORON NISSIM [email protected] Columbia University, Graduate School of Business, 3022 Broadway, Uris Hall 604, New York, NY 10027 ... Financial statement analysis ...
The Literature review of this study will emphasis on the related ... White ratios and other relationships based on past performance may be helpful in predicting the future ... Financial analysis ...
Findings - The study was able to identify and categorise past studies into areas of Financial evaluation, Insolvency Prediction, Valuation, Inter-linkage studies, Benchmarking & Decision making, Technical Analysis. Limitations of ratios identified from the literature are Proliferation of ratios, Lack of Normality and Accounting framework impact.
Financial ratio analysis is used to evaluate the financial performance and condition of a business enterprise by measuring its progress towards financial goals. Its purpose is to provide information about the business entity for decision-making by both external and internal users. Terminology, definitions, and formulae (for vertical analyses, return on investment, investment utilization ratios ...
The Analysis and Use of Financial Ratios: A Review Article. ... He wishes to thank Dun and Bradstreet Ltd. for their financial support. Search for more papers by this author. Paul Barnes, ... Citing Literature. Volume 14, Issue 4. December 1987. Pages 449-461. References; Related; Information;
Literature review. 2.1. Concepts and definitions of IFRS and GAAP ... and cash flow statements are used to perform quantitative analysis. The financial ratios used in this study are discussed below. 3.2.1.1. Return on assets (ROA) It is a financial ratio that measures a bank's profitability in relation to its total assets. ROA is a metric ...
REVIEW OF LITERATURE Prasanta Paul (2011) stated on the Financial Performance Evaluation - Some of the selected NBFCs are taken for the comparative study. In the study, five of the listed NBFCs are considered for the analyzation of comparative financial ... M Kumbirai, R Webb (2010) A financial ratio analysis of commercial bank performance in ...
There are steps to consider in the breaking-down analysis of the ROI and the ROA: Step1: Consider the firm ROI and ROA financial ratios. The financial ratio of the ROI formula is: ROI = Net income / Total assets. As studied above, this ratio tells how efficient a firm has used its assets to generate sales.
The traditional textbooks of financial analysis also emphasise the need for a firm to use industry-wide averages as targets (Foulke, 1968), and there is evidence that firms do adjust their financial ratios to such targets.*. Whittington (1980) identified two principal uses of financial ratios. The traditional, normative use of the measurement ...
Methodology. In previous studies on relationship analysis for financial statements and financial ratios, two models have mainly been used. The first one is the panel OLS regression model which has been applied by a number of accounting articles on financial performance and in most literature less than ten variables are used (Al-Jafari and Al Samman, 2015; Jakob, 2017; Burja, 2011).
The study is based on the theory background and relevant researches in the areas of performance measures disclosed in financial statements. The sample of the case studies and sorts of literature are specifically collected from the well-known and respected accounting journals investigating in performance measures areas from 2010 to 2016, which are available on open access.
Author contributions. Amar Johri: Conceptualization and designing, Methodology, Formal analysis and interpretation of data, Writing - original draft, Writing - review & editing. Raj Kumar Singh: Conceptualization and designing, Methodology, Formal analysis and interpretation of data, Writing - original draft, Writing - review & editing. All authors agree to be accountable for all ...
4 .1 Ratio analysis. Ratio analysis is a devise of financial statement analysis conducive to business dec ision-making in multiple crux. ar eas. This analysis supports perception of sea changes in ...
A systematic literature review design was used in this study following the guidelines of Paul and Criado (Citation 2020). There are various types of systematic literature reviews, including structured reviews, framework-based reviews, bibliometric reviews, and meta-analysis reviews.
During our study, critical analysis of the literature, mathematical modeling and inference were used. A detailed analysis of transport orders executed by SMEs (small and medium-sized enterprises), which are characterized by a fleet of light commercial vehicles with a capacity of up to 3.5 t, was carried out in the FMCG (Fast-Moving Consumer ...
As the crypto-asset ecosystem matures, the use of high-frequency data has become increasingly common in decentralized finance literature. Using bibliometric analysis, we characterize the existing cryptocurrency literature that employs high-frequency data. We highlighted the most influential authors, articles, and journals based on 189 articles from the Scopus database from 2015 to 2022.
Public debt-to-GDP ratios have undergone substantial fluctuations over both the short and long term. Most recently, global debt-to-GDP ratios peaked at 100% on average in 2020 due to COVID-19, retracting substantially by 2022. To understand what drives these movements, we propose a structural approach to debt decompositions based on a SVAR identified with narrative sign restrictions. We find ...
Financial statement analysis has traditionally been seen as part of thefundamental analysis required for equity valuation. But the analysis has typicallybeen ad hoc. Drawing on recent research on accounting-based valuation, this paperoutlines a financial statement analysis for use in equity valuation. Standardprofitability analysis is incorporated, and extended, and is complemented with ...
In his paper reviewing the use of^ and analysis of^ Financial Ratios, Barnes (1987) expanded Whittington's (1980) description of these predictive uses. Barnes (1987) defined two main users of ...
Methods?We performed a systematic search across PubMed, the Cochrane Library, and Embase databases, encompassing the literature up to February 2024, to inform our systematic review and meta-analysis. When assessing outcome indicators, the risk ratio (RR) and its corresponding 95% confidence interval (CI) were calculated for dichotomous variables.
Introduction Antimicrobial resistance (AMR) is one of the most serious public health challenges worldwide, including in Japan. However, there is limited evidence assessing the AMR burden in Japan. Thus, this systematic literature review (SLR) and meta-analysis (MA) were conducted to assess the clinical and economic burden of AMR in Japan. Methods Comprehensive literature searches were ...