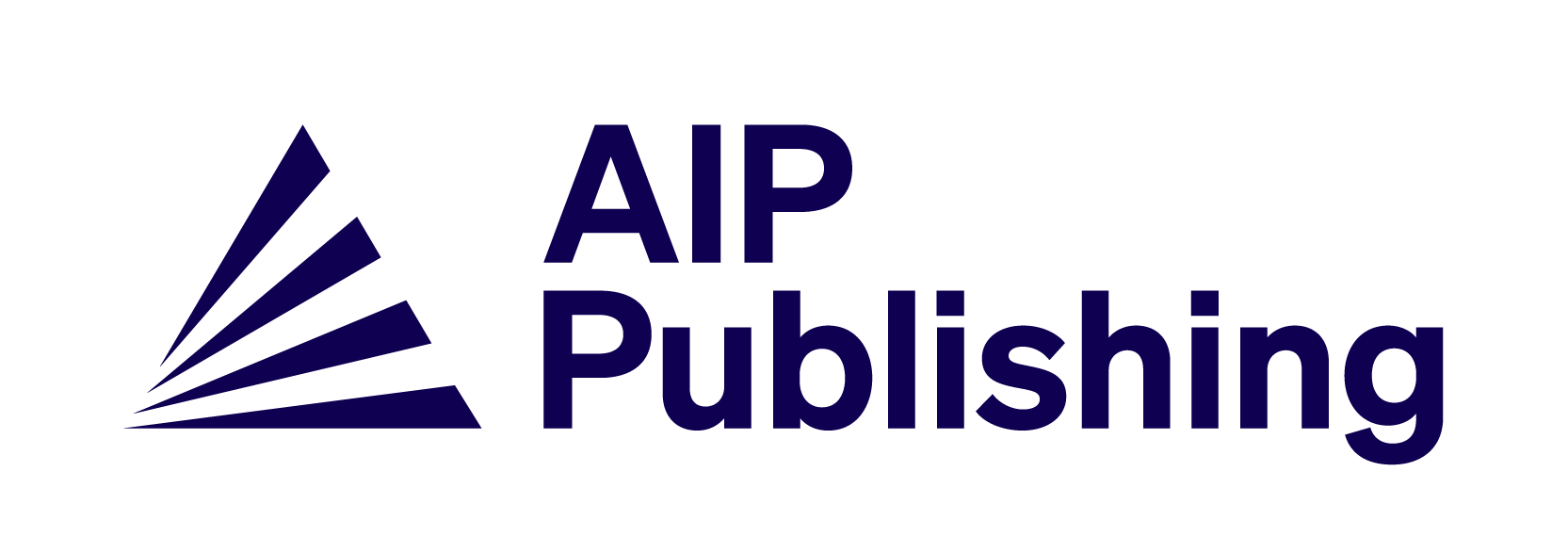
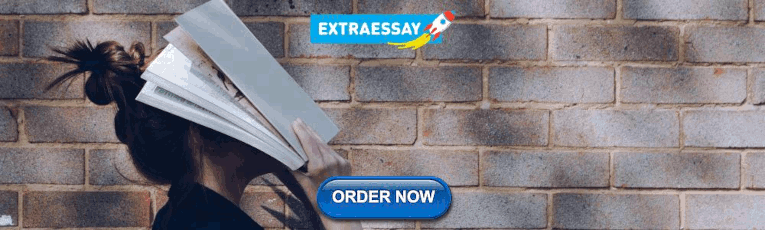
Literature review: Water quality and public health problems in developing countries
- Article contents
- Figures & tables
- Supplementary Data
- Peer Review
- Reprints and Permissions
- Cite Icon Cite
- Search Site
Eni Muryani; Literature review: Water quality and public health problems in developing countries. AIP Conf. Proc. 23 November 2021; 2363 (1): 050020. https://doi.org/10.1063/5.0061561
Download citation file:
- Ris (Zotero)
- Reference Manager
Water’s essential function as drinking water is a significant daily intake. Contamination by microorganisms (bacteria or viruses) on water sources and drinking water supplies is a common cause in developing countries like Indonesia. This paper will discuss the sources of clean water and drinking water and their problems in developing countries; water quality and its relation to public health problems in these countries; and what efforts that can be make to improve water quality. The method used is a literature review from the latest journals. Water quality is influenced by natural processes and human activities around the water source Among developed countries, public health problems caused by low water quality, such as diarrhea, dysentery, cholera, typhus, skin itching, kidney disease, hypertension, heart disease, cancer, and other diseases the nervous system. Good water quality has a role to play in decreasing the number of disease sufferers or health issues due to drinking and the mortality rate. The efforts made to improve water quality and public health are by improving WASH (water, sanitation, and hygiene) facilities and infrastructure and also WASH education.
Sign in via your Institution
Citing articles via, publish with us - request a quote.
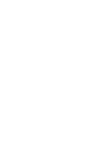
Sign up for alerts
- Online ISSN 1551-7616
- Print ISSN 0094-243X
- For Researchers
- For Librarians
- For Advertisers
- Our Publishing Partners
- Physics Today
- Conference Proceedings
- Special Topics
pubs.aip.org
- Privacy Policy
- Terms of Use
Connect with AIP Publishing
This feature is available to subscribers only.
Sign In or Create an Account
Thank you for visiting nature.com. You are using a browser version with limited support for CSS. To obtain the best experience, we recommend you use a more up to date browser (or turn off compatibility mode in Internet Explorer). In the meantime, to ensure continued support, we are displaying the site without styles and JavaScript.
- View all journals
- My Account Login
- Explore content
- About the journal
- Publish with us
- Sign up for alerts
- Review Article
- Open access
- Published: 20 February 2023
The determinants of household water consumption: A review and assessment framework for research and practice
- A. Cominola ORCID: orcid.org/0000-0002-4031-4704 1 , 2 ,
- L. Preiss ORCID: orcid.org/0000-0002-0033-0080 3 ,
- M. Thyer 3 ,
- H. R. Maier ORCID: orcid.org/0000-0002-0277-6887 3 ,
- P. Prevos ORCID: orcid.org/0000-0003-2768-031X 4 ,
- R. A. Stewart 5 , 6 &
- A. Castelletti ORCID: orcid.org/0000-0002-7923-1498 7
npj Clean Water volume 6 , Article number: 11 ( 2023 ) Cite this article
5933 Accesses
12 Citations
7 Altmetric
Metrics details
- Engineering
- Water resources
Achieving a thorough understanding of the determinants of household water consumption is crucial to support demand management strategies. Yet, existing research on household water consumption determinants is often limited to specific case studies, with findings that are difficult to generalize and not conclusive. Here, we first contribute an updated framework for review, classification, and analysis of the literature on the determinants of household water consumption. Our framework allows trade-off analysis of different criteria that account for the representation of a potential water consumption determinant in the literature, its impact across heterogeneous case studies, and the effort required to collect information on it. We then review a comprehensive set of 48 publications with our proposed framework. The results of our trade-off analysis show that distinct groups of determinants exist, allowing for the formulation of recommendations for practitioners and researchers on which determinants to consider in practice and prioritize in future research.
Similar content being viewed by others
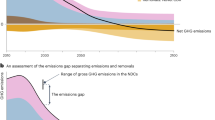
The carbon dioxide removal gap
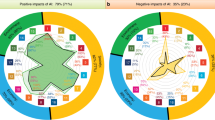
The role of artificial intelligence in achieving the Sustainable Development Goals
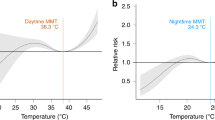
Heat health risk assessment in Philippine cities using remotely sensed data and social-ecological indicators
Introduction.
As urbanization is increasing globally, with trends that are unlikely to stabilize in the next decades 1 , 2 , water demand-side management strategies are emerging as key interventions to manage the current and future urban metabolism, and realize the potential of water conservation in cities 3 , 4 , 5 .
The domestic sector uses one of the largest portions of water in cities 6 , 7 . Therefore, achieving a thorough understanding of how, when, and how much water is used in households is of the upmost importance for water authorities and policy makers alike to design effective demand-side management strategies and inform future urban planning 8 . Knowledge of the behaviour surrounding water demand is vital to positively influence water conservation 9 and to implement effective and enforceable water demand management strategies. Additionally, better knowledge and improved predictions of water demands also allow water authorities to better size new water storage, distribution, and treatment infrastructure, as well as plan future upgrades of current systems 10 .
Recent literature has shown that there is a large number of climate and socio-demographic variables, attitudes, beliefs, and other factors that can vary between different households and can potentially influence water consumption. These factors, called determinants , can all influence household water use in different ways. In addition, the collection of data on each determinant is just as varied as the determinant itself. Some, such as average temperature, are readily accessible on a regional scale and can be obtained and analysed with ease 11 , 12 , 13 . Others, such as people’s perception of behavioural control, are difficult to capture. They represent subjective and stated information, rather than measurable observations, and often require detailed surveys with multiple questions, followed by lengthy and time-consuming analysis, to determine whether any information can be inferred from the survey data 14 , 15 .
The advent of advanced metering infrastructure (or smart meters) provides unprecedented access to high spatial and temporal resolution information on water consumption 16 , 17 , 18 . As smart metering is becoming more and more common and accessible on a global scale 19 , 20 , it provides the opportunity to greatly increase knowledge on the different potential factors driving household water consumption 21 , 22 .
Despite the advances described above, most of the state-of-the-art determinant analyses to date are often limited to a few case studies, and the existing findings are difficult to generalize and not conclusive. More research is needed to explore the trade-offs among different criteria that account for the relevance of potential determinants for water consumption modelling and management, their proven impact on heterogeneous case studies, and the cost of labour and/or of equipment required to collect information about a determinant. While previous studies have already performed literature reviews or meta analysis to identify key determinants of household water consumption and household water demand modelling, they usually only provide a descriptive analysis of the literature, without proposing analytic tools, quantitative trade-off analysis, and recommendations for both practitioners and researchers 8 , 9 , 20 , 23 , 24 , 25 , 26 , 27 . Furthermore, following the need for more data with a higher granularity also highlighted by some of these previous studies, the literature has seen numerous recent developments enabled by smart metering information. Here, we contribute an updated framework for review, classification, and analysis of the literature on household water consumption determinants. We also comprehensively analyze 48 peer-reviewed scientific publications focused on the identification and analysis of the determinants of household water consumption, selected after application of exclusion criteria from a larger data base of 231 papers analyzed for contextual information. Water consumption data recorded at the individual household level became only recently available, with the development of smart metering studies. Yet, previous residential water consumption studies included domestic water consumption data aggregated at coarser spatial scales. The set of reviewed papers includes studies using water consumption data gathered at different scales, from individual households to census tract/municipality level, depending on data availability.
The ultimate goal of this review is to identify which determinants have proven impact via extensive research and, thus, are recommended for consideration in practical applications related to household water consumption characterization, modelling, and prediction. After identifying these proven-impact determinants, the other determinants identified are those that require more research to fill existing gaps related to validation over multiple case studies, impact evaluation, and assessment of the costs and benefits of gathering information on a particular determinant. Specifically, the contribution of this review is three-fold. First, we develop a multi-criteria assessment framework for analyzing the key determinants that influence household water demand. Assessment criteria include their popularity in the literature, impact on household water consumption, and cost for determinant quantification. Second, we apply the proposed assessment framework to a comprehensive set of state-of-the-art studies to derive insights about the predominant determinants of household water consumption. Finally, we provide a classification system of household water use determinants and recommendations for researchers and practitioners that can be used to inform future research and applications.
Paper search and exclusion criteria
To gain an understanding of the current state of research on the determinants of household water consumption, we systematically searched for peer-reviewed journal papers and technical reports and comprehensively reviewed the state-of-the-art literature following a three-step procedure.
We first searched for the combinations of keywords reported in Fig. 1 in the subject/title/abstract of papers published in the last 40 years and stored in the Elsevier “Engineering Village” databases 28 . These keywords returned a search of over 8200 papers, requiring the “limit to” feature to be used to narrow the search. This allows most irrelevant topics to be filtered and removed from the search, reducing the list of papers to 4326 (See Supplementary Notes 1 for the full list of removed keywords).
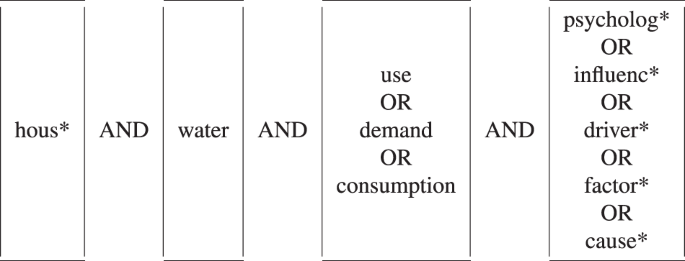
The represented query was used to search for papers on the determinants of household water consumption published in the last 40 years and stored in the Elsevier “Engineering Village” databases 28 . Subject, title, and abstract fields were considered for the initial search.
Second, we manually screened the title and abstract of each paper from the reduced sample of 4326 paper, checking for relevance within the scope of our study. This expert based screening for consistency returned a total of 231 papers referred to as general water consumption-related set of papers (see Supplementary References for the complete list). Some of these general water consumption-related papers are commonly cited within the literature found in the search. We reference them in the motivation of this study or discussion surrounding the results, but they were not necessarily analysed as part of the water consumption determinant assessment framework.
Finally, we reviewed all the general water consumption-related papers to determine if they actually analysed determinants against water use results, which is the main requirement for a paper to be included in our systematic review. Additionally, we formulated the following exclusion criteria:
We excluded papers that focus on water consumption in non-residential settings, including public buildings or touristic facilities 29 , 30 . However, we kept studies considering residential water consumption data or determinant data at the aggregate level for residential groups (e.g., census tracts).
We excluded papers that focus on theoretical models or self reported data, without quantitative comparison to actual (metered) water consumption data 31 , 32 .
We excluded papers that examine only a single type of indoor end use (e.g., only shower usage 33 ).
We excluded papers that do not focus on analysing determinants to water use, but were otherwise water related. These included papers with a primary focus on water use behaviour change, water restriction compliance, water efficient appliance uptake, water use estimation accuracy, or water price elasticity, without quantitative analysis of the determinants-to-water consumption relationship 34 , 35 , 36 , 37 , 38 , 39 .
We included papers that examined water consumption in both households and apartments. However, studies centred on just apartments (which often use water only indoor) were deemed to be beyond the scope of the review and were not included 40 .
As a result of the application of the above selection procedure, a database of 48 papers was compiled as the final set for systematic review and formulation of our assessment framework. This set of papers is hereafter referred to as framework analysis papers (see Supplementary Table 1 ).
Water consumption determinant assessment framework
In this study, we developed a two-phase comprehensive assessment framework to analyze the water consumption determinants reported in the framework analysis set of papers. At the conceptual level, the first phase of our framework, i.e., the determinant classification , is aimed at characterizing the nature of the identified water consumption determinants (e.g., physical, psychological) and categorizing them in groups based on their similarities, independently from their influence on water consumption. The second phase, named determinant analysis , is aimed at assessing the influence of different determinants in relation to water consumption, along with their relevance in the literature, and the effort required to retrieve them. The categories and attributes we defined for determinant classification and analysis are described in the next sections.
Determinant classification
We defined three main categories to classify the water consumption determinants: observable, latent, and external.
Observable determinants are defined as those determinants that can be physically seen or measured. They can be easily and/or directly measured and include objective features related to the occupants of the household and their house (e.g., occupant age, household size, household income, number of toilets).
Latent determinants relate to the way the occupants of the household think, feel, or act. Typically, they cannot be directly measured and need to be inferred from surveys/direct questions, and they can be subjective. Examples include attitude to water saving, individual habits, and beliefs.
External determinants are external to the house and might influence a suburb or groups of houses at a regional level. Examples might include weather variables, such as rainfall and/or temperature.
These three categories were used to facilitate the characterization and analysis of the determinants found in the literature, both in terms of ease of information gathering and impact on water consumption. For instance, observable determinants are generally easier to collect information on and, therefore, are expected to be more common in literature than latent determinants. External determinants often influence houses on a suburb or higher level, whereas observable determinants may have a different impact on every house on a street 41 . An overview of the determinant classification system is provided in Fig. 2 . As shown in the figure, we further separated each of the three categories above into sub-categories to group the individual determinants that were closely related into the same categories. A detailed summary of the sub-categories, the determinants included, and the question defining each determinant are reported in Table 1 (observable determinants), Table 2 (latent determinants), and Table 3 (external determinants). The category of observable determinants is further broken down into three sub-categories, i.e., socio-demographic (relating to the people inside the household), house (relating to the structure of the house itself), and yard characteristics (relating to the yard and its irrigation). Latent determinants are further separated into the following categories: gardening , awareness , perception , habits, and other . External determinants are sub-classified depending on the variable of interest, i.e., average temperature , average rainfall , water price , and other . This sub-categorization is primarily useful for discovering latent determinants, because it enables specific questions related to subjective behavioral attitudes and/or habits to be grouped and analysed together. However, due to the low number of papers for each individual question, the analyses for the latent determinants is mainly undertaken at the category level.
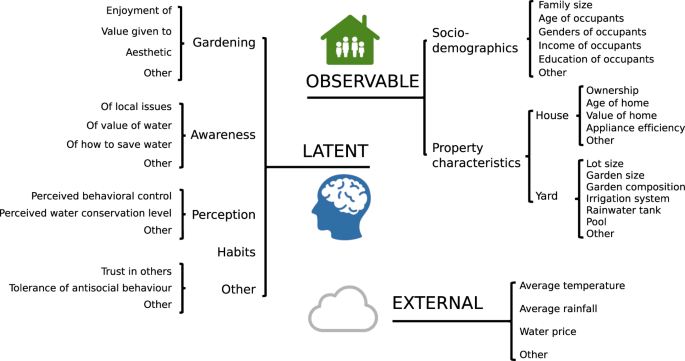
Potential water use determinants are classified in three categories, namely observable, latent, and external. Observable determinants are defined as those determinants that can be physically seen or measured. Latent determinants relate to the way the occupants of the household think, feel, or act. External determinants are external to the house and might influence a suburb or groups of houses at a regional level.
Determinant analysis
After extracting and categorising the determinants of household water consumption from each paper in the framework analysis set, we assessed the influence of different determinants in relation to water consumption, along with their relevance in the literature, and effort required to retrieve them. The goal of this determinant analysis phase is to find the trade-off among how much a determinant has been studied in the literature, how much it impacts water demand, and the cost required to obtain information about this determinant, relating to both labour and equipment. We defined three criteria to perform the determinant analysis: representation, impact, and effort.
Representation refers to how popular a determinant is in the reviewed literature on household water consumption. The representation R of a determinant i is thus defined as its relative frequency in the set of framework analysis papers:
where N i is the number of times a determinant i appeared in the studies considered and T is the total number of framework analysis papers (i.e., 48).
Impact refers to whether or not a particular determinant actually influences the water use in a household. If the measurement and inclusion of a determinant was found to change the accuracy of a prediction or have some other effect on the household water demand, then the determinant was said to have impact. Given a potential determinant of water consumption and the study where it was mentioned, we defined three possible categories of impact:
Yes (Y) . Impact found and analyzed: determinant information was collected in the study, numerical analysis was undertaken (e.g., statistical analysis, regression) and the determinant was found to have an impact on demand/predictability by the authors of the paper.
No/Low (NL) . Impact found and analyzed: determinant information was collected in the study, numerical analysis was undertaken (e.g., statistical analysis, regression), but the determinant was found to have no or low impact on demand/predictability.
Collected, but not analyzed (CNA) . Determinant information was collected in the study, but no analysis was undertaken for one or a number of reasons, such as lack of sufficient data or not selecting the determinant as a focus. Studies containing determinants that fall in this category also analyzed other determinants falling in the previous two categories. For this reason, they were not excluded from the framework analysis set of papers. Despite collecting data on several determinants, these studies only performed quantitative analysis for a subset of determinants. This category does not include determinants that were only superficially mentioned (e.g., in the paper introduction) and, thus, did not refer to the specific case study analyzed in the paper.
A blanket “Yes” rating was given to all determinants where impact was found. This was done rather than assigning a low, medium, high impact rating because each paper used a different technique for assessing impact. This means that different metrics are used in different papers, hampering a direct comparison and grouping determinants into individual impact categories. Some papers, such as 11 and 7 , compare determinants to other determinants in the paper, whereas other papers use statistics to determine which determinants have a larger impact. Some build mathematical models for impact assessment, such as structural equation models 42 , 43 , 44 , or multiple linear regression 13 , 45 , 46 . We defined the impact I of a determinant i as follows:
where \({N}_{i}^{\,{{\mbox{Yes}}}\,}\) is the number of papers where the determinant i is given a “Yes” rating and \({T}_{i}^{\,{{\mbox{(Yes + No)}}}\,}\) is the total of papers with “Yes” and “No” impact rating for determinant i . This excluded papers where the determinant had a CNA impact rating, because there was no definitive impact from this determinant from the papers that mentioned it.
Effort refers to the cost of labour and/or of equipment required to collect information about a determinant. For the purposes of this paper, effort is divided into three categories, i.e., Low , Medium , and High (Table 4 ). A low rating means that the information on the determinant is easily available for each house and can be obtained via a desktop study, with no interaction with the households needed. In contrast, the high effort rating corresponds to actually visiting the house and taking measurements and/or surveys.
In cases where the paper did not explicitly state the effort required to collect the information on the determinant, we assigned a low effort rating, assuming that no special effort was required (i.e., no ad hoc procedure for data gathering needed to be set up and described). Each of the categories is quantified by a corresponding effort rating factor, based on an estimate of the number of hours of labour required to collect information on a given determinant. This rating factor was then scaled such that the low rating is coupled with an effort rating factor equal to 1 (see more details in the Supplementary Notes 2) . For a given determinant i , the overall effort rating E i was determined by calculating the geometric mean of the effort rating across the analyzed papers:
where N i ,L , N i ,M , and N i ,H are the number of studies reporting determinant i with an associated low (L), medium (M), and high (H) effort, respectively, and T i is the total number of papers reporting determinant i . We used the geometric mean, rather than the arithmetic mean, because the effort rating factor varies across two orders of magnitude.
Overview of paper search outcome
A general overview of the 231 general water consumption-related and 48 framework analysis scientific publications reviewed in this study (Fig. 3 ) shows that the number of papers published per year from the general water consumption-related set has been increasing, particularly since the early 2000s. Peaks of more than 10 papers per year in this category emerge since 2011, with a maximum peak of 34 papers recorded in 2018. This increasing trend in time can be attributed to the increasing development of smart metering studies, which have been increasingly allowing detailed household water demand/consumption and behavioral analysis 20 , 47 . As a selected subset of the general water consumption-related papers set, the number of framework analysis papers has also increased in the last decade, compared to the ’80s and ’90s, constituting up to 5 papers per year. The final set of papers includes small-case studies comprising only a few units (11 individual households are considered as a minimum in 48 ), as well as large-scale studies comprising several thousands of households (e.g., more than 8000 individual households are considered in 49 ), or entire communities/towns 50 .
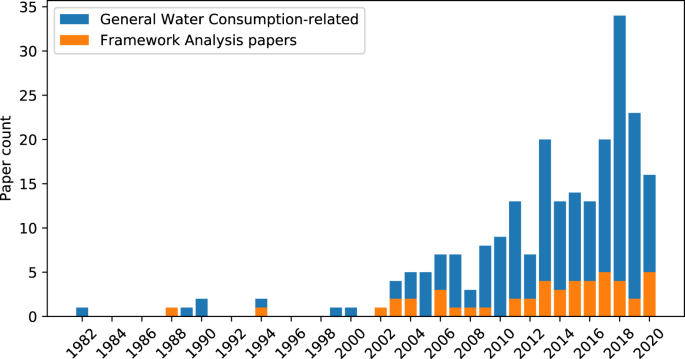
The yearly count of the 231 general water consumption-related (blue) and 48 framework analysis (orange) scientific publications reviewed in this study is represented for the last forty years.
Figure 4 shows the locations of the studies from the framework analysis set, with larger blue dots indicating more studies. The geographical distribution of the reviewed studies indicates that the interest in the determinants of water use is worldwide. Prominent interest emerges in particular areas, such as the US west coast, the east coast of Australia, and the Mediterranean area in Europe, perhaps reflecting the combination of areas more prone to drought and/or having the higher economic capacity to undertake water use related research.
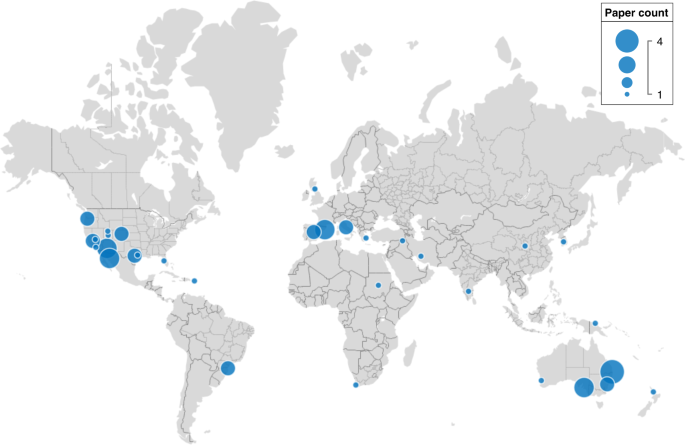
The location of the 48 framework analysis papers reviewed in this study is represented with blue markers. Marker size is proportional to the amount of studies in a specific location.
Determinant representation by class
From the analysis of the 48 framework analysis papers, we identified a range of heterogeneous determinants and quantified different combinations of determinant classes, namely observable, latent, and external (see Determinant classification). Figure 5 shows an overview of the representation for the different classes of determinants over the 48 analyzed papers. Observable determinants were the most popular (47 total studies, i.e., 98% representation), with latent and external having lower representation of 52% and 56%, respectively. The values represented in the figure confirm our hypotheses that observable determinants are more common in literature than latent determinants, due to their availability in public databases, either at the household level or at coarser spatial sub-urban scales (e.g., census data collected at the block group-level, such as those used in 49 ). The slightly higher representation of external determinants, compared to latent determinants, is also as expected due to the widespread availability of weather records (e.g., temperature, rainfall) from national or international environmental agencies. While there is no full consensus in the literature on the effect of weather or price variables on water consumption 51 , 52 , 53 , the high degree of representation of external determinants demonstrates that they are considered in more than half of the studies.
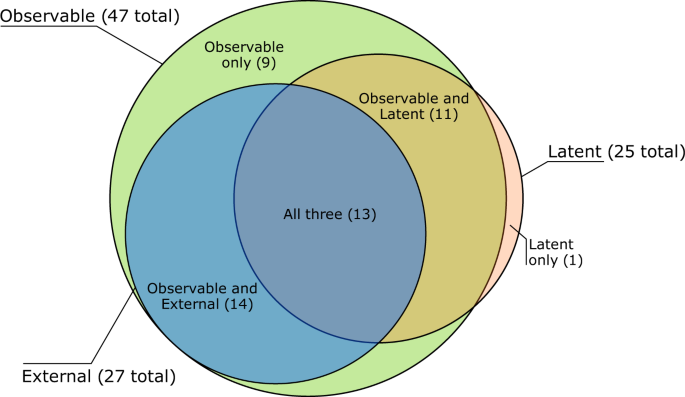
The representation of different classes of determinants (observable, latent, and external) in the 48 reviewed framework analysis papers is represented with coloured circles. Intersections are also visualized. The size of each circle and the numerical labels indicate the number of studies in which each combination of determinant classes appeared.
It is worth observing that multiple classes of determinants are simultaneously analyzed in most of the reviewed studies, with fewer than 20% analyzing observable variables alone. Further, almost every time external or latent variables are considered, they appear in combination with observable variables. Only one study specifically focused on analyzing the motivations for using and conserving water based on only latent determinants 42 , while no studies exclusively considered external variables. In contrast, nearly 30% of the studies included both observable and external variables, approximately 23% of the studies considered latent and external variables simultaneously, and 27% of the studies included all three types of determinant classes.
The high representation of observable determinants (Fig. 5) suggests that observable variables are widespread in the literature on modelling and forecasting of household water consumption. The prevalence of this class of determinants seems also to confirm the findings from previous studies, which demonstrated that meteorological variables have a greater influence on medium-term prediction and urban/suburban scales, but socio-demographics become more relevant when household-scale and short-term water demand models are developed 54 , 55 .
Individual determinant representation, impact, and effort
To facilitate interpretation of the numerical values we obtained for the three determinant assessment criteria (i.e., representation, impact, and effort) we defined some regions of interest for each criterion based on thresholds (see the regions labelled as low/high/very high in Fig. 6 ). We selected the threshold values used to delimit the above regions of interest based on visual inspection of the empirical distribution of the representation, impact, and effort values. This simplification is carried out to facilitate the inference of general qualitative conclusions, while accounting for the low number of papers and, at the same time, high number of determinants. As a result, representation values above/below 30% are considered high/low. Impact values below 75% are considered low, values between 75% and 90% are considered high, and values above 90% are considered very high. Effort rate values above/below 8 are considered high/low.
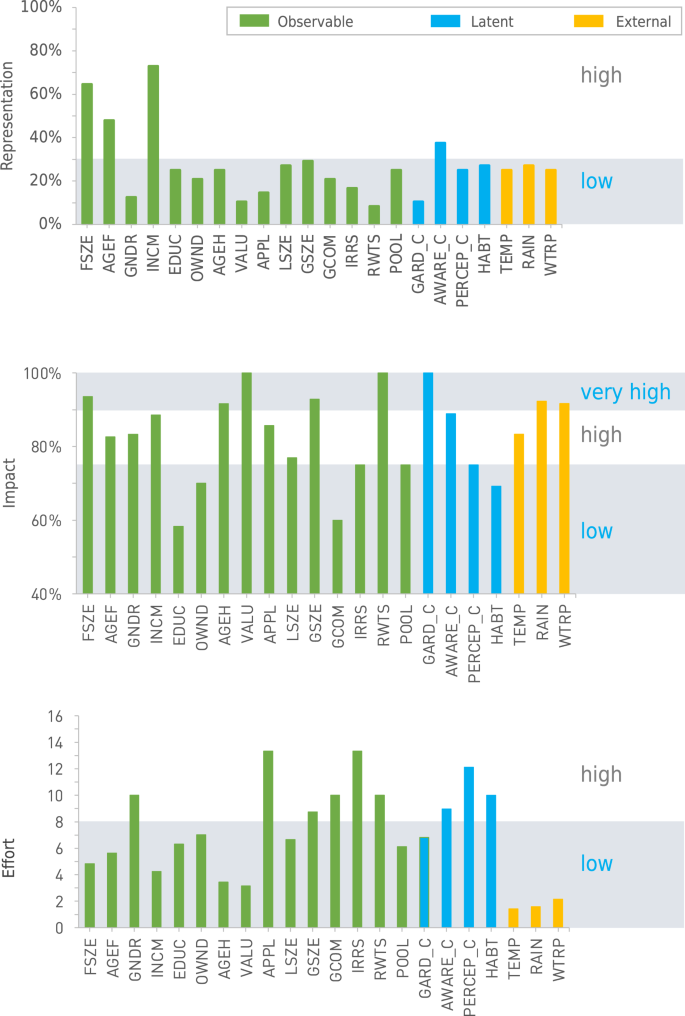
The three criteria to perform determinant analysis, i.e., representation (top), impact (middle), and effort rate (bottom) are associated with individual determinants. Observable determinant class is shown in green, latent class in blue, and external in orange. Shaded background indicates different levels of intensity for each analysis criterion. See Tables 1 – 3 for determinant acronyms definition (the determinants included in the categories marked as “Other” in the tables are not represented for better clarity).
From the resulting data visualized in Fig. 6 , we can infer the following insights about determinant representation, impact, and effort. First, the determinants with the highest representation (top plot in Fig. 6 ) were household income (> 70%), family size (> 60%), and age (> 45%). As already suggested by the outcomes of class representation (Fig. 5 ), all the above determinants with high representation are observable. One exception is the awareness determinant, which is the only non-observable determinant we found with high representation. The majority of the other determinants had a representation rate of 10% to 30%.
Second, the number of determinants with a high or very high impact (middle plot in Fig. 6) is much larger than the number of determinants with high representation. It must be noted that a high impact does not necessarily mean that a determinant was found to have a high influence on water consumption, but rather that it was found to have some influence on water consumption in many publications. Interestingly, some determinants from all classes achieve high or very high levels of impact. Observable determinants with very high impact include socio-demographic information (number of occupants), house characteristics (house age, value), and outdoor characteristics (garden size, and presence of rainwater tanks). While these latter attributes related to gardens ranked among those with the highest impact, garden composition was found to have one of the lowest impact rates across the analyzed studies. Also, the observable determinants related to the education level of occupants was found to have low impact. A latent variable that emerges as very important (GARD_C) is also related to garden characteristics, but, rather than representing any physical variable, it accounts for the psychological value given by occupants’ attitudes and habits towards gardening. Finally, all external variables were found to have high or very high impact, with rainfall and water price emerging as the two with impact above 90%.
The bottom plot of Fig. 6 shows that there was a wide variability in the effort rate for each individual determinant. Data on most of the observable determinants can be generally gathered with low effort, but some (e.g., appliance inventory and irrigation system) require house visits, and thus require high effort. In turn, all latent variables display an effort rate higher than 6, and three out of four are classified as high-effort. Conversely, data on all external determinants can be retrieved with low effort, as they are usually available from national agencies (weather data) and water utilities (water price). Obtaining information on higher effort determinants likely requires getting in contact with individual householders, via phone/online surveys, or house visits.
Overall, the results reported in Fig. 6 suggest that there are trade-offs between representation, impact, and effort. In the next section, we perform a joint analysis of the three criteria and their trade-off to infer the implications of the outcomes of this study for researchers and practitioners.
Trade-off analysis and implications for researchers and practitioners
Figure 7 shows the interaction between the representation, impact, and effort criteria. The distribution of blue and orange points in the figure demonstrates that there are different trade-offs among the three criteria. Each trade-off can have a different set of implications to derive recommendations for researchers and also practitioners. We identified the three groups of determinants marked with (A), (B), and (C) to illustrate the different needs of research and practice. Group A is characterized by high impact, high representation, and low effort. Determinants in this group include household family size, occupants’ age, and occupants’ income. This group of well-studied determinants with proven impact might be particularly interesting for practitioners aiming to gather knowledge on household water consumption with budget constraints. Group (B), which includes, among others, information on the household irrigation system, appliance efficiency, and occupant gender, is characterized by medium-to-high impact, but low representation, and a range of low to high effort. While this group might not be very appealing for practitioners due to low representation, researchers might be interested in focusing on these determinants to increase their representation and, thus, validate or contrast the limited findings on these determinants that appear in the literature. Finally, Group C refers to determinants with low representation and, compared to those in groups A and B, lower impact. As they also might require high gathering efforts, these determinants should be treated with caution until more research is performed to prove their potential impact on a larger sample of studies.
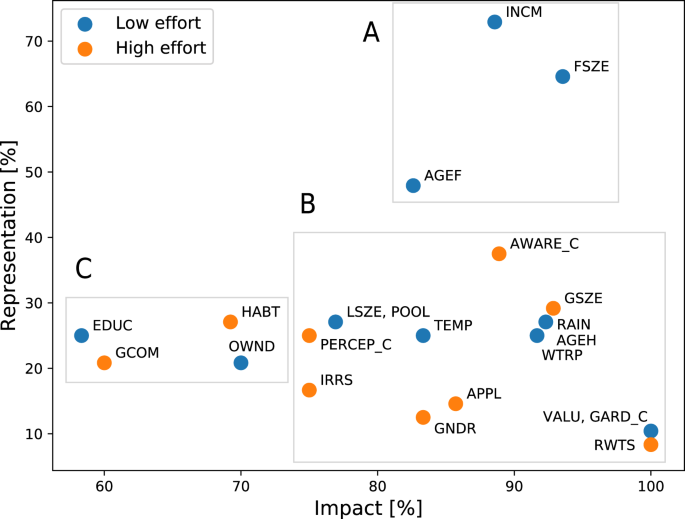
Impact (x-axis) vs Representation (y-axis) vs Effort (color) of each determinant. Each point refers to a specific determinant. See Tables 1 – 3 for determinant acronyms definition. The determinants classified as “High effort" are those with an effort value larger than 8.0, vice-versa for the “Low effort" determinants. Determinants are organized in three groups: Group A - high impact, high representation, and low effort; Group B - medium-to-high impact, low representation, and mixed low and high effort; Group C - low representation, low impact, mixed effort.
Accounting for similar trade-offs across the entire sample of determinants that we have identified from the review of the literature enables determinant-specific recommendations to be derived for practitioners and researchers. In the last step of this review and determinant classification effort we thus develop a trade-off analysis framework that considers different combinations of representation, effort, and impact to formulate such recommendations. In keeping with the goal of this study, our trade-off analysis aims at identifying groups of determinants that have proven cost-effective impact via extensive research and, thus, can be recommended for use in practice, compared with groups of determinants that require more research to address open questions related to representation, impact, and effort. The proposed trade-off analysis framework includes four main recommendation categories:
In this category, we include determinants characterized by high representation, high/very high impact, and low effort. We consider these determinants as determinants that practitioners can “definitely use" (U), as they have been extensively researched and have been shown to have an impact in most cases, while being affordable. For the same reasons, higher levels of research priority should be devoted to less explored determinants, while these can serve as references. The determinants included in box (A) in Fig. 7 belong to this group.
In this category, we classify those determinants characterized by low representation, high/very high impact, and low effort. Given their promising, but not extensively proven, impact, and overall affordability, further research on these determinants should be prioritized to increase their representation (IR). We consider these determinants as determinants that practitioners can “use with caution" (UC), as they have not been extensively researched, but at the same time might have high impact at low-cost. The determinants included in box (B) in Fig. 7 and classified as low effort (blue color) belong to this group.
In this category, we include determinants characterized by generally low representation, high/very high impact, and high effort. Similarly to the previous category, we believe that practitioners can use these determinants “with caution" (UC), as they have not been extensively researched and require high effort for data collection, but at the same time might have high/very high impact. Given their promising, but not extensively proven, impact, and high cost, further research on these determinants should be prioritized, primarily to lower the effort (LE) needed to collect them and, thus, facilitate their consideration in more studies (increased representation - IR). The determinants included in box (B) in Fig. 7 and classified as high effort (orange color) belong to this group.
IR/LE/AI-NP
In this category, we include determinants characterized by low representation, low impact, and mainly high effort. Given the limited knowledge on these determinants, we suggest that these determinants are “not prioritized" (NP) for use by practitioners unless further research demonstrates that the effort required to collect these determinants is worth the benefit of considering them. Further research should then aim at increasing their representation (IR), lowering the effort needed to obtain data on these determinants (LE), and further assessing their impact (AI) to acquire better knowledge on their actual value. The determinants included in box (C) in Fig. 7 belong to this group.
Summary information on the above categories is reported in Table 5 . Based on the proposed trade-off analysis framework and the threshold values defined in Fig. 6 , we associated each of the different determinants identified in the framework analysis papers with a level of recommendation (see Fig. 8 ). Some relevant insights for researchers and practitioners emerge. First, only observable determinants are classified as “U". At present, there are some socio-demographic determinants (i.e., number of occupants, income level, and occupant age) that can be reliably used by practitioners in most cases to model household water consumption and can be easily and affordably retrieved.
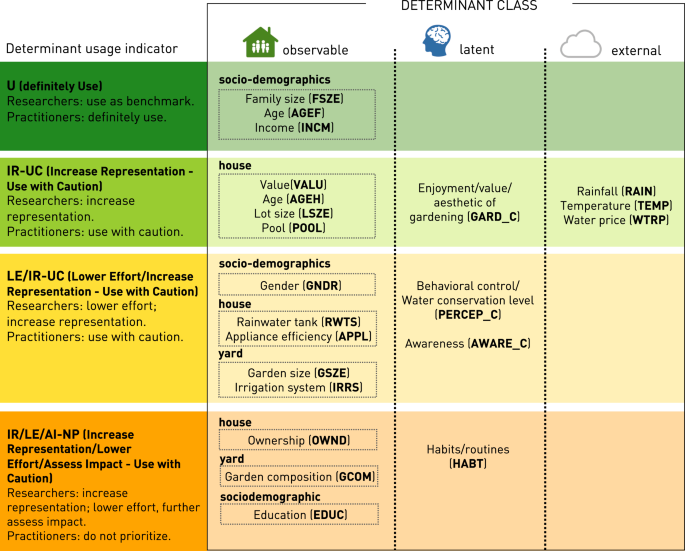
Each household water consumption determinant identified in the framework analysis papers is associated with a level of recommendation. Determinants are classified according to the three defined classes (columns), i.e., observable, latent, and external. Four levels of recommendation (rows) are formulated for practitioners and researchers. They are sorted in decreasing order of representation and proven impact in research, as well as confidence for use in practical applications. Confidence for use in practical applications decreases going from green ("U" level of recommendation) to orange ("IR/LE/AI-NP" level of recommendation).
Second, all external variables (i.e., average rainfall, temperature, and water price) are classified as IR-UC. Consequently, they have a proven impact, but have been used sporadically in connection with household water consumption (while they have been used more often at larger, urban scales), thus results might be case-specific and further research is needed to assess their impact on a larger number of studies.
Third, a mix of observable and latent external variables deserves further research to lower effort (e.g., by improving technology/data gathering practices or identifying lower-effort proxies for the same type of information) and increase representation. These variables are either observable determinants, the collection of which requires significant effort and house visits/calls to occupants (e.g., to build an inventory of appliance efficiency or storing information on irrigation systems), or latent variables the impact of which is still not proven due to low representation. The increasing availability of high-resolution metering and behavioral studies fostered by smart metering development is likely to contribute more knowledge on these determinants and more complete guidelines for use by practitioners in the coming years 7 , 17 , 20 .
Fourth, we would like to stress that the recommendation “Do not favor adoption until further research" for the determinants classified as IR/LE/AI-NP does not mean that they should not be considered in future applications or no research should be done on them. Conversely, we recognize that many existing studies are based on limited data or data with coarser spatio-temporal resolutions, thus conclusive statements on the impact of such determinants would require further validation. Since large uncertainty about their impact remains, more studies are actually needed to increase the representation of these determinants and increase the statistical significance and generality of their impact assessment. Joint research that also includes other determinants with higher levels of representation could be beneficial to discover more information on the determinants in this group and better understand whether practitioners should eventually include one/more of these determinants in their analysis. Further research could be also developed to assess the degree to which these determinants are correlated with others, and hence redundant, and to which extent these and other determinants can relate to particular characteristics of water consumption (e.g., demand peaks, end use components).
Finally, some of the determinants that we recommend to use with caution (UC) in practice, and that should be prioritized for research, might become determinants to definitely use (U) in the future. Two limitations currently prevent us to recommend “definitely use" for these determinants, i.e., generally low representation and high effort for data collection. Low representation indicates that the determinant has not been well-studied in the literature. Hence it might not be generalizable to a wide range of locations. To address the disadvantages of low representation, the following is recommended for practitioners:
Check the literature and if there are studies with similar context (location/climate/application) to the practitioners’ required application, and the impact of the determinant is high, then the determinant could be considered for use.
Continue to monitor the literature, to see if new studies appear using that determinant.
The other limitation, high effort, means that in the reviewed past studies it has been costly for practitioners to collect some of the required determinants. With the advent and widespread use of new technologies, the effort required to collect some of the required high-effort determinants may be substantially reduced. Lowering the effort related to some high-impact, yet also high-effort, determinants (see, e.g., those indicated with orange color in Group B in Fig. 7) would have a two-fold benefit. The direct reduction of the costs required to collect information on those determinants will also enable wider consideration of these determinants in a larger number of studies, thus increasing their representation. As technology enhances the capture of such determinants, there is an opportunity to revisit past studies/datasets and increase the representation of these determinants, which might then transition to determinant group A in in Fig. 7 . To address the current limitations and disadvantages of high effort, the following is recommended for practitioners:
Monitor the use of emerging technologies that provide an opportunity to lower the cost required to collect the determinant. For example, there is an opportunity for analysis of high resolution satellite maps/photos to provide automated estimates of observable determinants such as garden size (GSZE) over large number of households, which would lower the cost substantially 56 . Similarly, latent determinants such as water consumption awareness (AWARE_C) could be based on the uptake of user-friendly smart metering and phone apps on water consumption if they were widely available 17 , 57 .
Evaluate overall costs vs benefits based on preliminary experiments on small sample data (to evaluate benefits while avoiding high costs), and consider the use of lower cost proxy data for the “high effort” determinant.
These recommendations provide some guidance for practitioners to handle determinants classified as “use with caution”.
Limitations and future research
This work provides evidence and a quantitative framework for the analysis of household water consumption determinants, yet several limitations and questions remain for further research. First, alternative formulations of determinant representations, impact, and effort could lead to different results. This also stands for the subjective thresholds we adopted to distinguish between high and low representation, impact, and effort. Such thresholds and criteria formulation could be changed based on needs and subjective judgement.
Second, in this review we focused on the analysis of individual determinants of household water consumption. However, some determinants could be correlated, present redundant information, or be accounted for in alternative ways to build models for forecasting water demand (e.g., rainfall amount versus rainfall occurrence 53 ). Input feature engineering, variable redundancy, and data accuracy can substantially affect the performance of water demand models. Future studies focused on comparative analysis of alternative determinant formulations and inter-links/dependencies among different determinants can help define non-redundant determinant sets to train models of water demand and recommendations for variable pre-processing.
Third, the findings of this study are consistent with previous review papers that identified both observable and latent variables as the most important with respect to domestic water consumption 58 . Yet, other meta-analyses and review studies found partly contradictory results. Differently from our study 26 , found that the most important determinants of water use behaviour are related to individual opportunities and motivations, gender, income, and education level. In turn 59 , formulated a model that accounted for a wide range of variables including demographics, dwelling characteristics, household composition, conservation intention, trust, perceptions, habits, and perceived behavioral control. It must be noted that the above studies do not consider household water consumption per se as we do here, but relate potential determinants of water consumption also to individual consumption or behavior changes (i.e., changes in water consumption over time). Future, potentially contrasting, studies could then expand the scope of this work and relax the exclusion criteria we adopted here to achieve more inclusive comparative analyses that investigate the effect of different determinants in relation to quantified intervals of total household water consumption, and other heterogeneous aspects of domestic water demand, including statistics on end use components (e.g., flow rate, duration, or frequency of individual appliances) 60 and temporal changes of water consumption levels due to external stressors such as droughts, or demand management interventions 39 , 49 , for example.
Fourth, the set of framework analysis papers includes case studies primarily located in the United States, Australia, and Europe (see Fig. 4) . Geographical coverage is thus skewed. There is a need for more studies from other geographical regions (including countries with low-income economies) in order to obtain a more balanced picture and consolidate/expand the results obtained so far.
Finally, recent works have highlighted that urban and household water demands have been modelled at different spatial and temporal resolutions 47 . The choice of the temporal and spatial resolution of interest is determined both by data availability and the specific modelling and management purpose. Multi-scale studies combining different levels of spatial and temporal aggregation of water demands and potential determinants would further advance our analysis and contextualize specific recommendations for data collection and processing at the different spatial and temporal scales of interest.
Outlook and summary
In this paper, we contributed a comprehensive literature review and assessment framework to evaluate state-of-the-art research on the determinants of household water consumption. Starting from a search that returned over 8200 papers, we identified 48 papers that clearly identify whether a particular determinant can have an impact on household water demand (see Supplementary Table 1 for the list of selected papers). We then developed a classification system and assessment framework to analyze these 48 papers with the following two-fold goal. First, we classified the potential determinants of household water consumption into three main categories, i.e., observable , latent , and external based on their nature and ease of information retrieval. Second, we defined three quantitative criteria to analyze the influence of different determinants in relation to water consumption and quantified them for the determinants identified in the reviewed papers. These three indicators look at (i) how frequently a determinant appears in the literature ( representation ), (ii) whether or not a particular determinant has been observed to influence household water consumption ( impact ), and (iii) what the cost for labour and/or equipment required to collect information on a particular determinant is ( effort ).
Our trade-off analysis of representation, impact, and effort shows that there are some distinct groups of water consumption determinants. Each group has different implications for practitioners and researchers and our analysis provides valuable guidance for practitioners and researchers on which determinants to consider in a range of situations. We identified a group of high impact, high representation, and low effort determinants which include household family size, occupants’ age, and income. These observable determinants have been widely studied in the literature and their impact on household water consumption has been demonstrated in several cases. Moreover, as information on these determinants can be obtained with low effort, this group may be of interest for practitioners that need to estimate or model household water consumption with budget constraints and little room for exploratory analysis. A wide range of other determinants may be more interesting for research purposes. This range include information on the external determinants, including climate variables and water price, which is usually easily accessible and does not require ad hoc data gathering campaigns, yet only a limited number of studies has correlated these determinants with water consumption at the household scale, demonstrating potentially high impact. In turn, some other observable determinants and most latent determinants that relate to subjective perceptions, awareness, habits, or opinions, require a higher data-gathering effort and have a more uncertain impact on household water consumption, often only demonstrated in specific case studies. Given the higher cost and more uncertain return, further analysis on these determinants can be prioritized by researchers before direct use in practice. Practitioners and researchers should also monitor emerging technologies that could potentially lower the cost of data gathering on wide-scale and provide an opportunity to analyse past data sets and increase the representation of these determinants.
This study also highlights several limitations that required further research to achieve general and conclusive interpretations on the link between the multi-faceted characteristics of household water consumption (including end-use components 61 , consumption change, and demand patterns) and its determinants. Overall, our literature review contributes a further step to systematically analyze the determinants of household water demand, develop a general understanding, and derive several recommendations to guide future research and practice. Moreover, the assessment framework we proposed here is ready to be used by water authorities and other parties that are interested in identifying informative sets of variables to predict household water consumption with a high degree of confidence, while taking into account budget and data availability.
Data availability
The authors declare that all data supporting the findings of this review are available within the paper, in the Supplementary Information, and in the reference list.
Gerland, P. et al. World population stabilization unlikely this century. Science 346 , 234–237 (2014).
Article CAS Google Scholar
Kundu, D. & Pandey, A. K. World urbanisation: Trends and patterns. In Developing National Urban Policies , 13–49 (Springer, 2020).
Gleick, P. H. et al. Waste not, want not: The potential for urban water conservation in California (Pacific Institute for Studies in Development, Environment, and Security Oakland, CA, 2003).
Turner, A. et al. Guide to demand management and integrated resource planning (update on original 2008 guide). (2010).
Chini, C. M. & Stillwell, A. S. The metabolism of U.S. cities 2.0. J. Industrial Ecol. 23 , 1353–1362 (2019).
Collins, R., Kristensen, P. & Thyssen, N. Water resources across Europe-confronting water scarcity and drought . Ph.D. thesis, Univerza v Mariboru, Fakulteta za kmetijstvo in biosistemske vede (2009).
Arbon, N., Thyer, M., Hatton MacDonald, D., Beverley, K. & Lambert, M. Understanding and predicting household water use for Adelaide. Goyder Inst. Water Res. Technical Rep. Ser. 14 , 15 (2014).
Jorgensen, B., Graymore, M. & O’Toole, K. Household water use behavior: An integrated model. J. Environ. Management 91 , 227–236 (2009).
Article Google Scholar
Russell, S. & Fielding, K. Water demand management research: A psychological perspective. Water Resour. Res. 46 , https://doi.org/10.1029/2009WR008408 (2010).
Donkor, E. A., Mazzuchi, T. A., Soyer, R. & Alan Roberson, J. Urban water demand forecasting: review of methods and models. J. Water Resour. Plan. Management 140 , 146–159 (2014).
Beal, C., Stewart, R., Huang, T. & Rey, E. South East Queensland residential end use study (Urban Water Security Research Alliance Brisbane, Australia, 2011).
Bernardo, V., Fageda, X. & Termes, M. Do droughts have long-term effects on water consumption? Evidence from the urban area of Barcelona. Appl. Econ. 47 , 5131–5146 (2015).
Haque, M. M., Egodawatta, P., Rahman, A. & Goonetilleke, A. Assessing the significance of climate and community factors on urban water demand. Int. J. Sustainable Built Environ. 4 , 222–230 (2015).
Gregory, G. D. & Leo, M. D. Repeated behavior and environmental psychology: the role of personal involvement and habit formation in explaining water consumption. J. Appl. Soc. Psychol. 33 , 1261–1296 (2003).
Fielding, K. S., Russell, S., Spinks, A. & Mankad, A. Determinants of household water conservation: The role of demographic, infrastructure, behavior, and psychosocial variables. Water Resour. Res. 48 (2012).
Cominola, A., Giuliani, M., Castelletti, A., Rosenberg, D. E. & Abdallah, A. M. Implications of data sampling resolution on water use simulation, end-use disaggregation, and demand management. Environ. Modelling Softw. 102 , 199–212 (2018).
Stewart, R. A. et al. Integrated intelligent water-energy metering systems and informatics: Visioning a digital multi-utility service provider. Environ. Modelling Softw. 105 , 94–117 (2018).
Monks, I., Stewart, R. A., Sahin, O. & Keller, R. Revealing unreported benefits of digital water metering: Literature review and expert opinions. Water 11 , 838 (2019).
Beal, C. & Flynn, J. The 2014 review of smart metering and intelligent water networks in Australia and New Zealand. Report prepared for WSAA by the Smart Water Research Centre, Griffith University (2014).
Cominola, A., Giuliani, M., Piga, D., Castelletti, A. & Rizzoli, A. E. Benefits and challenges of using smart meters for advancing residential water demand modeling and management: A review. Environ. Modelling Softw. 72 , 198–214 (2015).
Cominola, A. et al. Segmentation analysis of residential water-electricity demand for customized demand-side management programs. J. Clean. Prod. 172 , 1607–1619 (2018).
Cominola, A. et al. Data mining to uncover heterogeneous water use behaviors from smart meter data. Water Resour. Res. 55 , 9315–9333 (2019).
Arbués, F., Garcıa-Valiñas, M. Á. & Martınez-Espiñeira, R. Estimation of residential water demand: a state-of-the-art review. J. Socio-Econ. 32 , 81–102 (2003).
Inman, D. & Jeffrey, P. A review of residential water conservation tool performance and influences on implementation effectiveness. Urban Water J. 3 , 127–143 (2006).
House-Peters, L. A. & Chang, H. Urban water demand modeling: Review of concepts, methods, and organizing principles. Water Resour. Res. 47 (2011).
Addo, I. B., Thoms, M. C. & Parsons, M. Household water use and conservation behavior: A meta-analysis. Water Resour. Res. 54 , 8381–8400 (2018).
Koop, S., Van Dorssen, A. & Brouwer, S. Enhancing domestic water conservation behaviour: A review of empirical studies on influencing tactics. J. Environ. Management 247 , 867–876 (2019).
Elsevier. Engineering Village. https://www.elsevier.com/solutions/engineering-village (Dec. 2020) (2020).
Bertone, E. et al. Role of financial mechanisms for accelerating the rate of water and energy efficiency retrofits in Australian public buildings: Hybrid bayesian network and system dynamics modelling approach. Appl. Energy 210 , 409–419 (2018).
Toth, E., Bragalli, C. & Neri, M. Assessing the significance of tourism and climate on residential water demand: Panel-data analysis and non-linear modelling of monthly water consumptions. Environ. Modelling Softw. 103 , 52–61 (2018).
Trumbo, C. W. & O’Keefe, G. J. Intention to conserve water: Environmental values, reasoned action, and information effects across time. Soc. Nat. Resour. 18 , 573–585 (2005).
Russell, S. V. & Knoeri, C. Exploring the psychosocial and behavioural determinants of household water conservation and intention. Int. J. Water Resour. Development 36 , 940–955 (2020).
Makki, A. A., Stewart, R. A., Panuwatwanich, K. & Beal, C. Revealing the determinants of shower water end use consumption: Enabling better targeted urban water conservation strategies. J. Cleaner Prod. 60 , 129–146 (2013).
Millock, K. & Nauges, C. Household adoption of water-efficient equipment: The role of socio-economic factors, environmental attitudes and policy. Environ. Resour. Econ. 46 , 539–565 (2010).
Cooper, B., Burton, M. & Crase, L. Urban water restrictions: Attitudes and avoidance. Water Resour. Res. 47 (2011).
Willis, R. M., Stewart, R. A., Panuwatwanich, K., Williams, P. R. & Hollingsworth, A. L. Quantifying the influence of environmental and water conservation attitudes on household end use water consumption. J. Environ. Manag. 92 , 1996–2009 (2011).
Polebitski, A. S. & Palmer, R. N. Analysis and predictive models of single-family customer response to water curtailments during drought 1. JAWRA J. American Water Resour. Assoc. 49 , 40–51 (2013).
Cooper, B. What drives compliance? An application of the theory of planned behaviour to urban water restrictions using structural equation modelling. Appl. Econ. 49 , 1426–1439 (2017).
Cominola, A. et al. Long-term water conservation is fostered by smart meter-based feedback and digital user engagement. npj Clean Water 4 , 1–10 (2021).
Jordán-Cuebas, F. et al. Understanding apartment end-use water consumption in two green residential multistory buildings. J. Water Resour. Plan. Manag. 144 , 04018009 (2018).
Xenochristou, M., Hutton, C., Hofman, J. & Kapelan, Z. Water demand forecasting accuracy and influencing factors at different spatial scales using a gradient boosting machine. Water Resour. Res. 56 , e2019WR026304 (2020).
Corral-Verdugo, V., Frias-Armenta, M., Pérez-Urias, F., Orduña-Cabrera, V. & Espinoza-Gallego, N. Residential water consumption, motivation for conserving water and the continuing tragedy of the commons. Environ. Manag. 30 , 527–535 (2002).
Syme, G. J., Shao, Q., Po, M. & Campbell, E. Predicting and understanding home garden water use. Landsc. Urb. Plan. 68 , 121–128 (2004).
Landon, A. C., Kyle, G. T. & Kaiser, R. A. An augmented norm activation model: The case of residential outdoor water use. Soc. Nat. Resour. 30 , 903–918 (2017).
Domene, E. & Saurí, D. Urbanisation and water consumption: Influencing factors in the metropolitan region of Barcelona. Urb. Stud. 43 , 1605–1623 (2006).
Ashoori, N., Dzombak, D. A. & Small, M. J. Modeling the effects of conservation, demographics, price, and climate on urban water demand in Los Angeles, California. Water Resour. Manag. 30 , 5247–5262 (2016).
Di Mauro, A., Cominola, A., Castelletti, A. & Di Nardo, A. Urban water consumption at multiple spatial and temporal scales. a review of existing datasets. Water 13 , 36 (2021).
Kossieris, P. & Makropoulos, C. Exploring the statistical and distributional properties of residential water demand at fine time scales. Water 10 , 1481 (2018).
Bolorinos, J., Ajami, N. K. & Rajagopal, R. Consumption change detection for urban planning: Monitoring and segmenting water customers during drought. Water Resour. Res. 56 , e2019WR025812 (2020).
Romano, G., Salvati, N. & Guerrini, A. Estimating the determinants of residential water demand in Italy. Water 6 , 2929–2945 (2014).
Adamowski, J. F. Peak daily water demand forecast modeling using artificial neural networks. J. Water Resour. Plan. Manag. 134 , 119–128 (2008).
Marzano, R. et al. Determinants of the price response to residential water tariffs: Meta-analysis and beyond. Environmental Modelling Softw. 101 , 236–248 (2018).
Zounemat-Kermani, M. et al. Neurocomputing in surface water hydrology and hydraulics: A review of two decades retrospective, current status, and future prospects. J. Hydrol. 125085 (2020).
Liu, J., Savenije, H. H. & Xu, J. Forecast of water demand in Weinan city in China using WDF-ANN model. Phys. Chemis. Earth, Parts A/B/C 28 , 219–224 (2003).
Babel, M. S. & Shinde, V. R. Identifying prominent explanatory variables for water demand prediction using artificial neural networks: a case study of Bangkok. Water Resour. Manag. 25 , 1653–1676 (2011).
Quesnel, K. J., Ajami, N. & Marx, A. Shifting landscapes: decoupled urban irrigation and greenness patterns during severe drought. Environmental Res. Lett. 14 , 064012 (2019).
Sarni, W., White, C., Webb, R., Cross, K. & Glotzbach, R. Digital water: Industry leaders chart the transformation journey. Int. Water Assoc. Xylem Inc. https://iwa-network.org/publications/digital-water/ (2019).
Llausàs, A. & Saurí, D. A research synthesis and theoretical model of relationships between factors influencing outdoor domestic water consumption. Soc. Nat. Resour. 30 , 377–392 (2017).
Jorgensen, B. S., Martin, J. F., Pearce, M. W. & Willis, E. M. Predicting household water consumption with individual-level variables. Environ. Behav. 46 , 872–897 (2014).
Makki, A. A., Stewart, R. A., Beal, C. D. & Panuwatwanich, K. Novel bottom-up urban water demand forecasting model: revealing the determinants, drivers and predictors of residential indoor end-use consumption. Resour., Conserv. Recycling 95 , 15–37 (2015).
Mazzoni, F. et al. Investigating the characteristics of residential end uses of water: A worldwide review. Water Res. 230 , 119500 (2023).
Download references
Acknowledgements
The authors acknowledge support by the German Research Foundation and the Open Access Publication Funds of the Technical University of Berlin for covering publication costs.
Open Access funding enabled and organized by Projekt DEAL.
Author information
Authors and affiliations.
Chair of Smart Water Networks, Technische Universität Berlin, Berlin, Germany
A. Cominola
Einstein Center Digital Future, Berlin, Germany
School of Architecture and Civil Engineering, University of Adelaide, Adelaide, SA, Australia
L. Preiss, M. Thyer & H. R. Maier
Victoria University, Melbourne, Australia
School of Engineering and Built Environment, Griffith University, Gold Coast, Australia
R. A. Stewart
Cities Research Institute, Griffith University, Gold Coast, Australia
Department of Electronics, Information, and Bioengineering, Politecnico di Milano, Milan, Italy
A. Castelletti
You can also search for this author in PubMed Google Scholar
Contributions
M.T. and H.M. designed the research. L.P. collected and analyzed most of the papers, and drafted an early-stage outline of this paper. All authors helped collect and review the papers in the final selection. A. C. (Andrea Cominola), L.P., M.T., H.M., P.P., R.S., and A.C. (Andrea Castelletti) developed the review framework. A.C. (Andrea Cominola), L.P., M.T., A.C. (Andrea Castelletti), and H.M. developed the final version of the paper. All authors reviewed the manuscript.
Corresponding authors
Correspondence to A. Cominola or M. Thyer .
Ethics declarations
Competing interests.
The authors declare no competing interests.
Additional information
Publisher’s note Springer Nature remains neutral with regard to jurisdictional claims in published maps and institutional affiliations.
Supplementary information
Supplementary information, rights and permissions.
Open Access This article is licensed under a Creative Commons Attribution 4.0 International License, which permits use, sharing, adaptation, distribution and reproduction in any medium or format, as long as you give appropriate credit to the original author(s) and the source, provide a link to the Creative Commons license, and indicate if changes were made. The images or other third party material in this article are included in the article’s Creative Commons license, unless indicated otherwise in a credit line to the material. If material is not included in the article’s Creative Commons license and your intended use is not permitted by statutory regulation or exceeds the permitted use, you will need to obtain permission directly from the copyright holder. To view a copy of this license, visit http://creativecommons.org/licenses/by/4.0/ .
Reprints and permissions
About this article
Cite this article.
Cominola, A., Preiss, L., Thyer, M. et al. The determinants of household water consumption: A review and assessment framework for research and practice. npj Clean Water 6 , 11 (2023). https://doi.org/10.1038/s41545-022-00208-8
Download citation
Received : 15 September 2021
Accepted : 15 December 2022
Published : 20 February 2023
DOI : https://doi.org/10.1038/s41545-022-00208-8
Share this article
Anyone you share the following link with will be able to read this content:
Sorry, a shareable link is not currently available for this article.
Provided by the Springer Nature SharedIt content-sharing initiative
This article is cited by
Tracking and tracing water consumption for informed water sensitive intervention through machine learning approach.
- Abraha Tesfay Abraha
- Tibebu Assefa Woldeamanuel
- Ephrem Gebremariam Beyene
npj Clean Water (2024)
Enhancing the explanation of household water consumption through the water-energy nexus concept
- Chunyan Wang
- Jiangshan Wang
An integrated approach to water conservation: fuzzy logic assessment of water tariffs in Abu Dhabi Emirate’s residential sector
- Issah M. AlHamad
- Osamah Al Hemyari
- Saeed Al Nuaimi
Bulletin of the National Research Centre (2023)
Quick links
- Explore articles by subject
- Guide to authors
- Editorial policies
Sign up for the Nature Briefing: Anthropocene newsletter — what matters in anthropocene research, free to your inbox weekly.

- Previous Article
- Next Article
INTRODUCTION
Results and discussion, conclusions, acknowledgements, data availability statement, conflict of interest, water affordability analysis: a critical literature review.

- Article contents
- Figures & tables
- Supplementary Data
- Open the PDF for in another window
- Guest Access
- Cite Icon Cite
- Permissions
- Search Site
Thalita Salgado Fagundes , Rui Cunha Marques , Tadeu Malheiros; Water affordability analysis: a critical literature review. AQUA - Water Infrastructure, Ecosystems and Society 1 August 2023; 72 (8): 1431–1445. doi: https://doi.org/10.2166/aqua.2023.035
Download citation file:
- Ris (Zotero)
- Reference Manager
Water and sanitation affordability has drawn researchers’ attention over the past years due to the recent economic crisis and the growing role of tariffs on funding services. This paper conducted a systematic review of previous research on water and/or sanitation affordability and a critical qualitative discussion on the methodologies adopted. The research framework relied on a hybrid approach that combines systematic quantitative and narrative analyses. The literature review protocol applied returned 79 relevant studies published by journals and recognized international organizations and four key topics, namely affordability indicators, threshold, data source, and practical use of the analysis were identified and critically discussed. This paper contributes to the literature by presenting and discussing the most important water affordability issues and enlightening the potential role of the analysis in public financial policies.
No consensus on a methodology or threshold for affordability analysis has been reached.
Water bill and income are the most common data used in affordability analysis.
Scarce and unreliable data are recurrent issues in low- and middle-income countries, misleading affordability analysis.
Affordability evaluation plays a crucial, but missing role in financial public policies.
Water supply and sanitation (WSS) services involve multidisciplinary complex factors, such as social, cultural, technical, economic, and political ones. Millions of dollars are spent on health care due to low quality or lack of those services, since many countries are unable to provide water supply and sanitation due to technical challenges, cost of required infrastructure expansion and upgrades, and of operation and maintenance. An estimated 2.2 billion people worldwide do not have access to safe drinking water and 4.2 billion people do not have access to safe sanitation ( WHO & UNICEF 2021a ). Besides the lack of physical access, society has become aware of the growing affordability issue, since the pressure of tariffs for funding the infrastructure sector has increased ( Reynaud 2016 ; Mack & Wrase 2017 ; Goddard et al. 2021 ). Costs for WSS connection, update of housing equipment, and payment of bills have become then a significant barrier for vulnerable families to benefit from water supply and sanitation.
Providing WSS services at an affordable price is included in Sustainable Development Goal (SDG) target 6.1, as several other human rights. As pointed out by WHO & UNICEF (2021b) , SDG targets 6.1 and 6.2 will not be reached unless affordability can be measured and monitored towards recognizing the population with payment difficulties and acting more efficiently. However, the lack of a consensus definition or established methodology of affordability measurements has hampered progress evaluation locally and globally.
Although studies have focused on water affordability in low- and middle-income countries, international concerns over poor families based in richer nations have highlighted the burgeoning crisis worldwide ( Sawkins & Dickie 2005 ; Martins et al. 2016 ; Mack & Wrase 2017 ; Goddard et al. 2021 ; Onda & Tewari 2021 ; Yoon et al. 2021 ; Meehan et al. 2021 ). Studies of water and sanitation affordability have employed a great variety of approaches, according to available and scarce data and local socioeconomic contexts, in order to express the struggles faced by the population.
This paper aims to provide a comprehensive, holistic, and critical review of the methodologies of water and sanitation affordability measurements and the current affordability understanding by updating previous findings and crossing studies with multiple goals. It also identifies the main insights offered by the relevant literature, through a hybrid methodology including systematic quantitative review and narrative analyses ( Jin & Wang 2016 ).
The literature review conducted provides readers with a better understanding of the limitations and advantages of the most used affordability indicators worldwide and discusses the different thresholds applied and the recurrent data availability problems. We observed that socioeconomic context plays a major role in the choice of the most appropriate indicator and threshold, and that application of affordability analysis in public financial policies deserves more attention and further research. Since this study is limited to the papers returned by the search methodology applied, local successful examples from regulators or utilities around the globe might have been missed.
The paper is organized as follows. Section 2 describes the research methodology applied. Section 3 presents and discusses the main topics addressed by the studies. The final section provides the concluding remarks and suggestions for further research.

Research framework based on titles, abstracts, and keywords.
The first phase searched papers based on title, abstract, and keywords. Scopus was selected as the primary search engine, as in previous studies ( Pinto & Marques 2015 ; Fuente 2019 ; Lima et al. 2021 ; Machete & Marques 2021 ). No time restrictions were applied, and further discussion is based on all studies found until June 2022.
Scopus search engine results
In the second phase, duplicated papers were eliminated. Based on the title and abstract, the third phase removed papers not related to the main topic – water and/or sanitation affordability. The full reading of all studies, during the fourth phase, selected the papers that empirically or theoretically analysed affordability measurements. Additional relevant published works were identified through citations in the core literature (e.g., scientific papers not found in the previous steps, and technical reports from the World Bank, Organisation for Economic Cooperation and Development (OECD), American Water Works Association, European Bank for Reconstruction and Development, World Health Organization (WHO), and the United Nations). Finally, the sixth and last phase was devoted to a systematic quantitative review and qualitative critical analysis of the publications. The former showed the studies’ geographical distribution, main journals, research groups’ and case studies’ countries. Power Business Intelligence software ( Power BI, 2022 ) was used for the geographical distribution analysis. As displayed in Figure 1 , from 1,276 papers initially found, the main findings from 79 papers and reports from international organizations are critically discussed. Papers that neither used types of affordability measurement, nor analysed the current methodologies were excluded – three empirical studies that did not mention the data source for affordability ratio calculation were also excluded. Reports from International Organizations were included due to their quantitative affordability analysis or critical qualitative discussion.
Systematic quantitative review
Leading journals and organizations

Frequency and geographical distribution of research centres.

Frequency and geographical distribution of case studies.
Critical qualitative discussion
The literature about water supply and sanitation affordability has become more frequent over the past years due to an economic crisis faced mostly by vulnerable families, and due to a (slow) path to full cost recovery tariff. Most studies returned by this systematic review are empirical, working with the scarce available data worldwide. A considerable variation in ratios, data sources, and thresholds used was observed and all those methodological dissimilarities hamper comparisons of countries, although affordability measurements should not be compared worldwide.
Nevertheless, governments must be aware of water affordability for targeting those that require public resources spending. As highlighted by Kessides et al. (2009) , the methodology for such a calculation must take into account the local socioeconomic context, including the macroeconomic situation and available data. This section critically discusses measurement approaches separated by main topics.
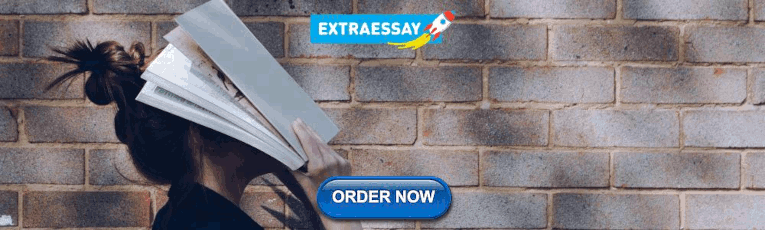
Affordability ratios

Possibilities of affordability ratio.

Ratio approaches.
As shown in Figure 5 , 40.6% of the studies adopted water bills as a numerator for calculating the amount spent by families, and 35.9% used water expenditure – the actual value households are spending on WSS (or only on water services, as observed in 20 studies). Those 75.6% considered average consumption, unlike the 18.8% that used the volume of water for basic needs and the water tariff structure to calculate the numerator, or the 4.7% that considered families’ self-reported spending for calculating a price for basic needs. Figure 5 also reveals that the denominator is slightly more standardized, since 65.5% of the studies used income, 15.5% adopted total expenditure, and 17.2% adopted disposable income. The different approaches applied by researchers were influenced by the available data and the context in which the study was conducted (such as low-income countries, rural, or urban areas), as discussed below.
Part of the studies claimed that the main advantage of self-reported water expenditure is to bring in all the costs inferred to a household, including those from multiple paid – and commonly informal – resources. Self-reported expenditure can also include a fraction of the coping costs ( Pattanayak et al. 2005 ; Nastiti et al. 2017 ; Komarulzaman et al. 2019 ), which are costs not paid directly to the provider, but expended by users, not necessarily in cash, such as travel time, storage tanks, and extra treatment equipment ( Cook et al. 2015 ). The value of time spent for obtaining WSS lacks consensus; however, researchers have advocated the inclusion of non-financial costs when analysing affordability to avoid technological options with low financial costs, but high coping costs (e.g., long journeys for obtaining the service) ( WHO & UNICEF 2021b ). As pointed out by Pattanayak et al. (2005) , coping costs affect both connected and non-connected households, according to the local service quality. The authors empirically studied all coping expenditures related to irregular water supply in Nepal, in 2001, and concluded approximately 45% of the coping costs were due to the value of time spent on water collection, 10% resulted from pumping, and 2% referred to water purchase. Moreover, such costs could represent up to 1% of monthly income and were lower than estimates of willingness to pay (WTP) for improved services. Based on data from a 2012 household survey conducted in Indonesia, Nastiti et al. (2017) estimated the hidden mitigation costs of groundwater extraction and water boiling in three scenarios, of which the worst level included the groundwater extraction, storage, and boiling costs, and the best one the cost for water supply 24 h. The burden of proper water supply service in the lowest income group was the same as that of the current situation with all hiding mitigation costs (5.2%).
In addition, self-reported water expenditure depends on surveys (official or not) and, most importantly, their methodologies. Besides the cost of implementing regular expenditure surveys, they are always a sample from a whole population and depend on users’ memories and perceptions. On top of that are the definitions of acceptable WSS that do not always match the acceptable levels of the United Nations ( WHO & UNICEF 2021a ). In addition, a one-time interview does not consider seasonal variations in services and goods consumption and in expenditure.
Self-reported water expenditure does not separate overconsumption from the essential or reasonable use of water, which can overestimate affordability ratios, misleading public action. The opposite also applies, i.e., using microeconomic data from the 2015/2016 Portuguese Household Budget Survey, Martins et al. (2019) observed vulnerable families in Portugal were consuming less than a reasonable volume of water due to budget constraints, while their affordability ratio was below the established threshold. Vanhille et al. (2018) and Gawel et al. (2013) also highlighted the importance of identifying whether households prefer such a consumption bundle and face non-income constraints. Overconsumption cases can be either real overconsumption, due to a family choice, or overconsumption, due to inefficient water equipment ( Sebri 2015 ; Vanhille et al. 2018 ), or large families – the latter two usually in poorer households.
Accurate measurement of affordability is particularly challenging in contexts where households have access to multiple sources of water, resulting in wide variations in service levels in terms of quality, distance, or reliability. Households lacking piped water connections on premises often rely on free sources, thus, falsely indicating that the water is affordable ( Israel 2007 ; Komarulzaman et al. 2019 ). Describing this as ‘hidden affordability’, which affects 17.8% of Indonesian households, Komarulzaman et al. (2019) calculated a proxy value for water expenditures by multiplying the average local water price by the minimum water needs. The results showed that unserved families would spend 6.3% of their total expenditure on water if they were connected to the public piped network. Similarly, in areas where water utilities are highly subsidized, households do not bear the true cost of water and hence, their water expenditure may be below the affordability threshold ( Andres et al. 2020 ).
The use of water bills or water tariff structures to calculate the service expenditure is easier for utilities and policymakers since data are available. However, the main disadvantage of the approach is the exclusion of non-connected households, which obtain their water from either free and questionable resources, or private vendors, who usually charge higher prices per cubic meter. Komarulzaman et al. (2019) reported 75% of Indonesian households do not have affordability problems, since the families do not pay for water, as they get it from free resources and are not connected to the water supply network. Moreover, the non-financial costs are not translated to the analysis when the affordability ratio relies on utilities’ bills or tariff structure.
For both calculation options of the numerator, policymakers struggle with a common problem, i.e., the unknown quality of service. Despite some useful international average prices, the health and environmental water and sanitation standards can vary among countries, and the affordability analysis does not capture quality – whether households are connected or not. When available, complementary information on providers’ performance might be necessary to understand the context of affordability analysis, given that an affordable but inadequate service is not reaching SDG 6.
Concerning the water volume used in the affordability ratio calculation, researchers have proposed a minimum amount of water for basic needs ( Miniaci et al. 2008 ; García-Valiñas et al. 2010a , 2010b ; Gawel et al. 2013 ; Martins et al. 2013 , 2019 ; Barde & Lehmann 2014 ; Sebri 2015 ; Teodoro 2018 ; Vanhille et al. 2018 ; Borja-Vega et al. 2019 ; Komarulzaman et al. 2019 ; Mastracchio et al. 2020 ; Balazs et al. 2021 ; Goddard et al. 2021 ). Instead of water expenditure or average water bill, those studies analysed the burden on families from a minimum volume perspective, avoiding over- or under-consumption. Although no consensus has been reached on the value, discussions surround the amount of water necessary for surviving and that required for regular life, including, for example, laundry. Such volume varied among the studies. American researchers used values from California and Texas authorities’ recommendations – around 189 litres per capita per day (lpcd) for indoor use ( Teodoro 2018 ) and Balazs et al. (2021) and Goddard et al. (2021) appropriated the available and regular information for 600 cubic feet in California, i.e., around 140 lpcd. European case studies used 100 lpcd ( García-Valiñas et al. 2010a , 2010b ; Vanhille et al. 2018 ). Martins et al. (2013) , for instance, calculated affordability ratios with 40 lpcd, and for the first tariff block in Portugal. In Peru's case, the volume was 70 lpcd ( Barde & Lehmann 2014 ). Some studies preferred mathematical water demand functions to find out the basic water volume for a normal life ( García-Valiñas et al . 2010a ; Sebri 2015 ; Reynaud 2016 ). Based on a Stone-Geary utility function, García-Valiñas et al. (2010a) estimated a minimum of 128 m 3 per year as appropriate for Southern Spain municipalities. Among the studies, the demand varied from as low as 7.5 lpcd in Cambodia ( Chamberlain & Sabatini 2014 ), up to 378 lpcd in the United States ( Mack & Wrase 2017 ), and 320 lpcd in Canada ( Janzen et al. 2016 ).
The topic is far from being a consensus among public authorities, utilities, or academics. As highlighted by several authors, the acceptable minimum consumption relies on local climate, culture, socioeconomic conditions, and water availability, thus hampering the establishment of a worldwide value. WHO recommends 20 lpcd as a minimum amount in case of scarcity, 50 lpcd as an intermedium amount with low health risk, and 100 lpcd as an optimum value, with very low health risk ( Howard & Bartram 2003 ). Although the importance of affordability for water basic needs has been highlighted, only 12% of the studies compared the current affordability situation with the one using basic needs volume ( García-Valiñas et al. 2010b ; Gawel et al. 2013 ; Sebri 2015 ; Vanhille et al. 2018 ; Borja-Vega et al. 2019 ; Komarulzaman et al. 2019 ; Martins et al. 2019 ).
Denominator
The denominator of an affordability indicator expresses the household's budget and can be either total expenditure or income. Some studies stated income data should be as reliable as possible, embracing seasonal, variable, and informal income, which is a common situation in low-income countries ( Fankhauser & Tepic 2007 ; Andres et al. 2020 ).
Some researchers choose total expenditure over income ( Fankhauser & Tepic 2007 ), since the former includes all families’ spending, and it might be a better way to express the local living expenses faced by families. However, total expenditure may be inaccurate due to its dependence on household members’ memories and on the period during which the survey was carried out. As an example, the total expenditure during the pandemic was probably very distinct from that of a normal lifetime, and the expenditure also can vary throughout the whole year. Another issue is related to savings and families’ choices. Many surveys do not take into account the family's savings in the total expenditure information, or the services chosen by users as their payment priority. In other words, the survey methodology largely affects the results, when it comes to total expenditure, as mentioned earlier. Andres et al. (2020) and Hutton (2012) recommend the use of income over total expenditure if the available data are sufficiently reliable.
As for income, some researchers have started to discuss the use of disposable income as a realistic indicator of user's ability to pay ( Reynaud 2010 ; Gawel et al. 2013 ; World Bank 2014 ; Teodoro 2018 ; WHO & UNICEF 2021b ). Teodoro (2018) considered disposable income the total income minus costs of taxes, housing, food, medicine, health care, and home energy, since, according to them, those services are as important as WSS for a regular life ( Gawel et al. 2013) . OECD (2003) suggests a procedure to express household incomes on a per equivalent adult basis, assigning a higher weight to the first adult in a household, lower values to subsequent adults or non-dependent children, and still lower values to each dependent child. The recommendation is based on the fact that some housing costs do not increase proportionately in larger households and children's needs are generally lower than adults’ ones.
Discussions about gross or disposable income rely on the fact that affordability is the ability to pay for a service without jeopardizing access to other essential goods. Therefore, from a public policy point of view, the whole situation faced by the population must be considered. Unfortunately, no consensus on what a minimum basket of services should be has been reached, which defines the heart of the affordability issue, since households must have access to all essential services (e.g., health, education, housing, energy, and heating), and goods at an affordable price. Researchers, governments, and other stakeholders have struggled to propose basic guidelines on the topic; however, it is still an area for further research. Since the purchasing power varies greatly even within a country, the definition of affordable service would involve multiple sectors and specific surveys, with close attention to poorer households ( WHO & UNICEF 2021b ).
More important than discussions on gross or disposable income is the fact that results are very different when the analysis is specific for the poorest households. Studies have introduced the use of quintiles or deciles for deeper analysis of poorer families’ situations. According to the literature review conducted, 53% of the studies included vulnerable families in the affordability analysis ( Carlos et al. 2002 ; Al-Ghuraiz & Enshassi 2005 ; Sawkins & Dickie 2005 ; Fankhauser & Tepic 2007 ; Israel 2007 ; Mahmood & Sharma 2009 ; OECD 2010 ; Wang et al. 2010 ; Gawel et al. 2013 ; Hoque & Wichelns 2013 ; Martins et al. 2013 ; Barde & Lehmann 2014 ; Chan 2015 ; Mack & Wrase 2017 ; Nastiti et al. 2017 ; Walter et al. 2017 ; Burt et al. 2018 ; Teodoro 2018 ; Vanhille et al. 2018 ; Borja-Vega et al. 2019 ; Cardenas & Whittington 2019 ; Yoon et al. 2019 ; Andres et al. 2020 ; Leflaive & Hjort 2020 ; López-Ruiz et al. 2020 ; Mastracchio et al. 2020 ; Goddard et al. 2021 ; Onda & Tewari 2021 ; Patterson & Doyle 2021 ; Zhang et al. 2022 ).
Although some governments use average income, the information provided can mask the real affordability problem at a vulnerable population level. Especially if the country shows high inequality, the average income as a denominator does not alert policymakers on the affordability issues faced by poorer families. As an example, 62% of the studies in this literature review reported affordability issues, and in 100% of those cases, poorer families were struggling to pay their water bills.
According to OECD (2003) , macro affordability indicators are developed by relating national average household water charges to either average household income or expenditure. Alternatively, micro affordability indicators disaggregate the former by income groups, family types, or regions. The study also states that in half the OECD countries, water affordability for low-income families either is a current issue or may become one.
Martins et al. (2016) used disaggregated household level data from a questionnaire-based survey performed in 2012 in Portugal to assess micro water affordability and concluded macro measures masked serious affordability issues for vulnerable households. The self-reported households’ income was matched with water bill information provided by utilities. While the macro affordability ratio for the whole sample (1.9%) suggested the absence of affordability issues, it was above the 3% threshold for the lowest income group. In addition, for the second lowest income group, the 2.3% affordability ratio hid the fact that 21.6% of households faced an affordability ratio above the 3% threshold.
Data source
WSS traditionally carry data problems, especially considering all their dimensions, namely social, technical, political, and economic. Information is either missing or controversial among the stakeholders involved ( López-Ruiz et al. 2020 ). The literature review showed 68% of the studies used National Official Surveys for income or total expenditure information for the affordability ratio denominator. Almost 20% carried out their own interviews, and around 12% adopted international data, as in this paper, reports from the World Bank, OECD, and the United Nations were included. Regarding the numerator, almost 75% of the studies used data from national or state level surveys, utility databases, or regulatory authorities’ reports, and around 19% employed water expenditure from their own questionnaires, due to lack of data. Studies often mixed different data sources to reach their goal, due to a lack of national or local official robust information.
Scarce or non-existent data are recurrent in low- and middle-income countries. All the studies, except one ( Martins et al. 2016 ), that applied interviews were conducted in those countries ( Al-Ghuraiz & Enshassi 2005 ; Wang et al. 2010 ; Gawel et al. 2013 ; Mason 2014 ; Nastiti et al. 2017 ; Walter et al. 2017 ; Burt et al. 2018 ; Acey et al. 2019 ; Cardenas & Whittington 2019 ; Hoque & Hope 2020 ). Although international organizations, such as the World Bank and the United Nations, have attempted – with some success – to influence the standardization of national surveys, they still face difficulties in benchmarking results worldwide ( WHO & UNICEF 2021b ). Studies also faced problems with the frequency of official data, given that some countries do not produce regular census surveys, leading to outdated information ( Lee 2011 ; Hutton 2012 ). Even though non-official surveys may be useful as a complementary source to affordability analysis, they are infrequently conducted and might not be publicly available ( WHO & UNICEF 2021b ). Carlos et al. (2002) evaluated inequities in water access in 11 countries of Latin America and the Caribbean, using different official available data and recommended countries should improve their surveys to facilitate national establishment of more equitable subsidy programs. Narzetti & Marques (2020) also faced data availability problems benchmarking models of subsidies for WSS in South American countries, especially in Brazil, Paraguay, and Argentina. The authors found that price discrimination was assigned according to the socioeconomic characteristics of customers or consumption levels, which make data accuracy even more crucial.
Policymakers rely on benchmarks to compare and judge results from affordability analysis and evaluate whether the tariff is affordable. Many organizations and governments have suggested different thresholds to classify WSS services as affordable, such as 3–5% by the World Bank, 5% by Asian Development Bank, 2.5% by the United States Environmental Protection Agency, and 3% by OECD.
According to the literature review, 90% of the studies that used some threshold to classify tariff affordability varied the percentage between 2 and 5%. Some used higher thresholds, considering the country's average income, such as in the USA ( Teodoro 2018 ; Mastracchio et al. 2020 ) or that the suggested poorer population is likely to spend higher proportions of their income on infrastructure services, with a case study of Cambodia ( Chamberlain & Sabatini 2014 ; Teodoro 2018 ; Mastracchio et al. 2020 ). Martins et al. (2019) , for instance, adopted 10, 15, and 20% thresholds, since their evaluation included joint affordability for electricity, water, and communication services in Portugal.
However, establishing a limit for expenditure on WSS may be too inflexible for a situation with such context – scarce data, growing need for investments, and interdependency with other sectors. If the affordability ratio is 3.2%, for example, it may be affordable, especially if other local expenses have a reasonable impact on families’ budgets. As discussed elsewhere, since affordability aims to maintain the ability to pay for all essential needs, the analysis is highly dependent on local reality ( Banerjee & Morella 2011 ; Andres et al. 2020 ; Hoque & Hope 2020 ; Pierce et al. 2021 ). High-income localities with affordable living costs may not face water affordability problems if they spend 6% of their budget on WSS bills. Contrastingly, some vulnerable families may struggle to spend as little as 2% of their income if the other essential services and goods are high in a broader context.
Although with no consensus, the use of a threshold (or a range) might be useful for the capture of local or regional affordability situations, such as the proportion of families above a specific threshold (or range). If the affordability problem is faced by a few households, policymakers and regulators may use cross-subsidy, social tariffs, or even direct subsidies to overcome the situation. On the contrary, localities with high percentages of poor families pose different challenges to governments, such as rethinking the public infrastructure financial policy or even improving social programs.
Additional indicators
Aware of the threshold matter, researchers and practitioners have started to introduce new indicators towards a holistic view of the community's socioeconomic status, gathering multiple information. This literature review found 10% of the studies used additional indicators to analyse affordability issues ( Gawel et al. 2013 ; Teodoro 2018 ; Mastracchio et al. 2020 ; Balazs et al. 2021 ; Goddard et al. 2021 ; Patterson & Doyle 2021 ).
Teodoro (2018) adopted hours of labour at minimum American wage, which measures the hours the user has to work to pay his/her WSS bill. Mastracchio et al. (2020) combined the affordability ratio for the lowest quintile and the Poverty Prevalence Indicator (PPI), which measures the number of poor households in a community – in this case, households earning below 200% of the U.S. Federal Poverty Level. PPI provides an idea of the economic situation of the whole community, indicating, for example, whether a cross-subsidy would be enough to solve the issue. Water shutoff rates have also become a concern among practitioners and researchers, and permanent or temporary water disconnection has been suggested as a water poverty indicator as well ( Meehan et al. 2021 ).
Analysing the water supply in informal settlements in Kampala (Uganda), a study revealed vulnerable population struggles to pay the connection fee, which could represent up to 74% of their average monthly income ( World Bank 2014 ). The results highlight connection fees and housing adaptation are a great and underestimated burden for vulnerable families and might be an extra indicator for the evaluation ( Kayaga & Franceys 2007 ; World Bank 2014 ).
The aforementioned indicators have been developed towards better-guiding policymakers on WSS policies, and their use (or not) will rely on the following main purposes: do decision-makers want to know the impact of basic water needs price on vulnerable families, or on the whole community? Could they solve the affordability issue by optimizing the cross-subsidies (if existing), or should a new social program be designed?
Potential affordability
Self-reported water expenditure or expenditure calculated from the water tariff structure may hide potential affordability issues ( Gawel et al. 2013 ; Martins et al. 2019 ). This might be an even more important analysis in contexts where tariffs are becoming the predominant revenue source, and full cost recovery is encouraged. As WSS coverage increases, it is essential to understand the impact on families’ budgets of the full cost recovery tariff of a regular improved service – which can be either national or SDG 6 ‘safely managed’ service standards ( WHO & UNICEF 2021b ). Martins et al. (2019) studied the potential affordability considering the expenses of families for covering basic water needs in Portugal. Using the ratio of individual services and total expenditure, they demonstrated from 9% of families facing affordability issues, 16% would struggle to pay their utilities bill for the national average consumption, considering 20% as the threshold for water, energy, and communication services combined.
Researchers have also calculated the burden on families’ budgets if a full cost recovery tariff were applied to the water sector ( Fankhauser & Tepic 2007 ; Banerjee & Morella 2011 ; Nauges et al. 2015 ; Janzen et al. 2016 ; Reynaud 2016 ; Burt et al. 2018 ). Janzen et al. (2016) investigated the cost of water supply to 25 small communities in Canada and concluded 48% of the systems would have to charge more than the 2% median household income, to achieve full cost recovery, thus raising affordability concerns. Reynaud (2016) assessed the impact of implementing the full cost recovery for water services on European households’ income. In general, and considering 3% of the threshold, no affordability problem was detected, except for the first income decile in Bulgaria and, to a lesser extent, in Estonia and France. Fankhauser & Tepic (2007) estimated future income growth and demand for utility services and calculated the affordability ratio for a hypothetical full cost recovery tariff scenario in 27 transition countries. The authors concluded that households in the three lowest income deciles would pay more than 10% of their household expenditure for power and heating and 5% for water. Besides those findings, 20 studies calculated the affordability ratios only for water services due to a lack of sewage or data, increasing the potential affordability if both services were in place.
WTP and application of affordability analysis
Some studies have combined affordability measurements with the WTP indicator and users’ perception of water prices to understand population acceptability. Walter et al. (2017) investigated the impact of packaged drinking water on affordability and equity of access by the urban poor of Jakarta, Indonesia, and reported that lower-income households consider packaged water the most affordable safe drinking water source available, despite representing the second highest per unit cost source. Concomitantly, the population considered piped water more expensive despite its low per unit volume cost, because of the total costs associated. Al-Ghuraiz & Enshassi (2005) revealed that people from the Gaza Strip were willing to pay the cost recovery of water improvement to meet the WHO standards, though it represented 4% of their income. Moreover, Wang et al. (2010) interviewed 1,500 households in China and concluded people would reject price rises, although the average economic burden was only 1.5–2.1%. Marques et al. (2016) investigated the WTP for the water supply service in Cape Verde and reported households would in general not be WTP more for significant improvements in their current water supply services. Non-connected areas demonstrated higher WTP, unlike places where water resources were abundant. Curiously, the overall results showed levels of WTP fell short of the reported current expenditure levels for water services.
According to the results, policymakers and researchers should analyse both indicators carefully. A community with very low-quality services probably would not be willing to pay the full cost recovery tariff due to a lack of trust in the provider. This does not mean the price is unaffordable. On the contrary, a community can be willing to pay a great amount of money to access services, but sooner or later would struggle to pay WSS bills due to its financial situation.
Achieving SDG 6 demands affordability measurement and monitoring, which in turn demands methodologies capable of translating the real and potentially hidden problem of accessing WSS services, especially considering that the principle of equity mentioned in several human rights demands that poorer households should not be disproportionately burdened with the costs comparing to richer families ( WHO & UNICEF 2021b ). The literature review showed no consensus on the best methodology and a threshold has been reached. Instead, researchers have adopted different approaches based on the local context in which the study was conducted and on the available data. Wherever surveys are updated and reliable, and coping costs are absent or insignificant, income data as denominator and water bills as numerator have been revealed to be the most preferred. Studies have also revealed affordability analysis must be performed for different income groups for elucidating the WSS economic access of vulnerable families, even in richer countries. The choice of the most appropriate indicator relies on local conditions, such as WSS coverage, socioeconomic reality, and, again, available data. Affordability analysis of connected households in high-income countries are much simpler when compared to non-connected rural or isolated areas in low-income nations, where poor quality water sources may be available at no cost and long travel times might be intrinsic to families’ lives. Thus, the WTP for service improvement is very low, or, in the worst scenarios, there is no ability to pay whatsoever. Towards not excluding those in real need, whoever desires to develop an affordability study should include more than one indicator, especially if the analysis area is heterogeneous. Since data strongly influence the robustness of the affordability analysis, national surveys can also be gradually improved, incorporating simple WSS affordability questions and covering the population in extreme poverty and/or living in very low-income neighbourhoods such as informal settlements ( WHO & UNICEF 2021b ). Meanwhile, complementary information can be useful, especially from regulatory agencies, which can gather accurate data. The review also highlighted the core problem of the WSS affordability concept, namely the threshold definition. Since every human being should have access to all essential services and goods at an affordable price, which greatly varies among localities, the establishment of an isolated WSS affordability threshold would be incomplete, and perhaps mistaken. Although still very difficult to implement, affordability standards should be defined by a participatory process at the national or local level, along with affordability analysis of other sectors, and the involvement of poorer families ( Heller 2015 ).
Identifying the population with payment difficulties has an important but missing role in financial public policies. Towards overcoming the challenge of universal access, the work of utilities, regulators, and governments must be synchronized to optimize subsidies and public resources. Utilities play a crucial role in universalizing WSS to all, keeping the services as efficient as possible, since efficient public services are reflected in lower tariffs. Nauges et al. (2015) simulated tariff reforms for the Egyptian Water Regulatory Agency, taking into account a 3-year planning period, operation and maintenance cost recovery, and affordability. According to the authors, despite the increase in prices, water services would remain affordable for the low-income group, considering planned water bills’ impact on total expenditure. Concomitantly, utilities can facilitate payment for poorer houses through additional time or punctual social aid ( Marques et al. 2023 ).
Regulators are also important key players in assuring physical and economic WSS access to all. Inducing high coverage and performance, along with a well-designed tariff structure, they can optimize subsidies and provide more affordable bills. Borja-Vega et al. (2019) showed that although Mexican households do not face affordability problems (water expenses lower than 2% of total income), consumption subsidies through increasing block tariffs do not perform well and are significantly regressive. The wealthy populations benefit more from water subsidies than the poor, i.e., proportionally, poor deciles spend a larger portion of their income when compared to rich ones. Nauges & Whittington (2017) developed a modelling framework to analyse alternative municipal water tariff designs and the results also showed poor performance of increasing block tariffs in terms of targeting subsidies to low-income households. According to the authors, if the correlation between household income and water use is low, subsidies are always poorly targeted, despite the level of cost recovery, or the size of the fixed charge. As observed, the WSS tariff and its design strongly influence the effectiveness of subsidies. Since the revenue for WSS derives mainly from the three T's (taxes, tariffs and transfers), as stated by OECD (2010) , a robust assessment of the cost of regular service and its appropriate financing mix has the potential to reach SDG 6. Affordability analysis could be the first step for the understanding where the tariff can play the main role and where public fund (or other source) complementation would be necessary. Therefore, through financial public policies, governments can target subsidies where they are actually required ( Kessides et al. 2009) .
The measurement of the affordability of public services is not an easy task. The first problem is to shape the most appropriate definition of affordability, which depends on available data and socioeconomic context, including other essential services’ prices. This literature review, based on 79 papers and international reports, revealed researchers use different ratios for best evaluating the population's ability to pay for WSS. According to the studies, affordability analysis should also take into account vulnerable groups, such as low income and large families, since poorer households tend to spend a higher proportion of their income on WSS in comparison to rich ones.
There are several limitations for affordability measurements, such as multiple data sources (when available), unconnected households, coping costs, and unknown service quality, which hamper benchmarking, leading to the conclusion that comparisons of affordability results between localities may not be the best approach. A set of indicators (e.g., affordability ratio for quintiles and deciles, average and basic water needs volume, water and sanitation coverage, and proportion of households at poverty and at poverty risk) can be adopted to enrich the analysis.
Affordability analysis also has an important but missing role in financial public policies. Since resources have become more and more scarce over time, governments, utility managers, and regulators must work together to optimize the allocation of public resources for WSS universal access, considering users’ ability to pay. OECD (2010) has already addressed the topic throughout the 3T's policy – taxes, tariffs, and transfers. It is evident the importance of strategic financial planning for shaping the right balance of the 3Ts and providing WSS for all, ensuring that even the most vulnerable households have physical and economic access to the services, without jeopardizing their access to other essential goods.
The present study faces some limitations. Although the results covered all studies published until the first semester of 2022, the sample was restricted to those listed by the Scopus search engine and technical reports from worldwide recognized international organizations. The documents were also restricted to the 24 keyword combinations used. Few studies that neither mentioned data sources nor made a deep critical analysis of the affordability status quo were discarded. Although the subject has seen increased interest in the past decade, further research on the use of affordability analysis for financial public policy and affordability of public services and essential goods combined are necessary for the development of methodologies that set local affordability thresholds (or range of).
The authors are grateful to the anonymous reviewers, and the Editors-in-chief for the improvements achieved from their insightful suggestions. This work is part of the research activity carried out at Civil Engineering Research and Innovation for Sustainability (CERIS) and has been funded by the Portuguese Foundation for Science and Technology, under the project 2022.13852.BD.
All relevant data are included in the paper or its Supplementary Information.
The authors declare there is no conflict.
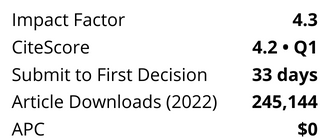
Affiliations
- ISSN 2709-8028 EISSN 2709-8036
- Open Access
- Collections
- Subscriptions
- Subscribe to Open
- Editorial Services
- Rights and Permissions
- Sign Up for Our Mailing List
- IWA Publishing
- Republic – Export Building, Units 1.04 & 1.05
- 1 Clove Crescent
- London, E14 2BA, UK
- Telephone: +44 208 054 8208
- Fax: +44 207 654 5555
- IWAPublishing.com
- IWA-network.org
- IWA-connect.org
- Cookie Policy
- Terms & Conditions
- Get Adobe Acrobat Reader
- ©Copyright 2024 IWA Publishing
This Feature Is Available To Subscribers Only
Sign In or Create an Account
- Open access
- Published: 18 April 2023
Asset management analytics for urban water mains: a literature review
- Atefeh Delnaz 1 ,
- Fuzhan Nasiri 1 &
- S. Samuel Li 1
Environmental Systems Research volume 12 , Article number: 12 ( 2023 ) Cite this article
2383 Accesses
2 Citations
1 Altmetric
Metrics details
This study presents a review of the state-of-the-art literature on water pipe failure predictions, assessment of water losses risk, optimal pipe maintenance plans, and maintenance coordination strategies. In addition, it provides a categorization of water main (WM) failures as well as a taxonomy of WM maintenance strategies. In particular, predictive and prescriptive analytics are highlighted with the investigation of their contributions and drawbacks from methodological and application perspectives. This review aims at providing a review of failure analytics developed recently in water mains domain either for prediction of failure or identification of optimal maintenance strategies conjointly. Future research directions and challenges are elaborated in advancing the understanding about the mechanisms leading to failures. The existing gaps between theory and practice in managing assets across water distribution networks ensuring cost-effectiveness and reliability are discussed. As knowledge about the state of the water mains and related areas is crucial, thus, this review provides an state-of-the-art update from recent studies, and accordingly, presents and discusses avenues for future research.
Introduction
A water distribution network (WDN) carries freshwater from one or more sources to municipalities for essential human consumption, economic development, and social activities. The WDN is a complex system. It consists of main and booster pumps, water mains typically buried underground, branching pipes, elevated water towers, and interconnected sub-networks for individual neighborhoods. The system or part of it will fail when one or more of its key components, in particular the water mains (WMs), break. There have been numerous cases of water main failures globally, with severe consequences. Examples of consequences include high replacement costs, revenue losses, water damages and contamination, traffic disruptions, and consumer service interruptions (Fares and Zayed 2010 ; Besner et al. 2011 ; Malm et al. 2015 ; Kakoudakis et al. 2017 ; Liang et al. 2018 ; Vishwakarma and Sinha 2020; Dawood et al. 2020a ).
Proactive interventions for reducing failure risks are necessary and cost-effective, particularly true in the context of aging WMs. Take major urban centers in Canada as example. Statistics Canada (Trudeau 2020 ) reported fair to very poor conditions for a significant portion of the WDNs. One simple reason is that these WMs have reached or are reaching the end of expected service life. Another reason is that the nature of water mains buried underground makes it complicated and costly to maintain and replace. As a result, there has been an increasing rate of failures over time (Asnaashari et al. 2013 ; Sattar et al. 2016 ; Folkman 2018 ; Snider and McBean 2018 , 2021 ). Over the past decade, studies of the WM problems have made a significant progress, taking advantages of constantly advancing data-driven techniques. The studies aimed to detect water main breaks, analyze failure risks, and optimize maintenance.
This study aims to scrutinize the recent literature of water main failure prediction models, failure consequences, failure risk, optimal WM maintenance strategies, and optimal coordinated maintenance strategies. An awareness of the state of the water mains and related areas is crucial. The existing review articles have mostly focused on water main prediction models (St. Clair and Sinha 2012 ; Dawood et al. 2020b ), the effect of availability and quantity of the database on WM failure models (Snider and McBean 2020a ; Chen et al. 2022 ), the effect of the limited, uncertain dataset on WM failure models (Jenkins et al. 2014 ), and the effect of combined datasets from different utilities on the performance of machine learning models for predicting future breaks (Chen et al. 2022 ). Other reviews have discussed different approaches to optimizing rehabilitation and maintenance strategies for WMs and integrated infrastructures (Abusamra 2018 ; Ghobadi et al. 2021 ; Ramos-Salgado et al. 2022 ; Shahata et al. 2022 ; Barton et al. 2022 ). This review aims to address issues of the failure prediction models developed recently and optimal maintenance strategies conjointly. This review also addresses future research directions in predictive and prescriptive analytics considering cold-region climatic variables.
It is important to consider water main maintenance in coordination with other infrastructures. It is also important to pay close attention to indirect costs and consequences, but the challenge lies in quantifying the indirect consequences in a monetary value (Muhlbauer 2004 ); to the best of the authors’ knowledge, the literature in this domain is scarce (Atef 2010 ; Yerri et al. 2017 ). Water main failure consequences are categorized into direct and indirect costs. Loss of production, repair and return to service and pipeline replacement are direct costs. Travel delay, supply outage and substitution, health risk, property damage, customer dissatisfaction and environmental damages are examples of indirect costs (Fares and Zayed 2010 ; Besner et al. 2011 ; Malm et al. 2015 ; Kakoudakis et al. 2017 ; Vishwakarma and Sinha 2020 ; Dawood et al. 2020a ). Considering indirect costs would make a major difference to WM maintenance plans (Yerri et al. 2017 ).
Issues exist in either the models or water main datasets themselves or both. They need to be discussed in detail. Thus, the purpose of this review is to provide an update of the knowledge from the recent studies and, more importantly, to explore ways to address the issues in future studies. This would help generate new ideas to improve failure prediction and risk analysis, and thus to reduce the costs in WM asset management planning, rehabilitation and renewal.
In the forthcoming review, the selection of literature is guided by the quest for answers to key questions pertinent to WMs. Some examples of such questions are given below:
Different methods and techniques have been proposed for locating and managing leaks in WMs (Misiunas 2005 ; Hamilton and Charalambous 2013 ; Zyoud and Fuchs-Hanusch 2019 , 2020 ; Karimian et al. 2021 ). What are the requirements of these approaches? What are the pros and cons?
Failure models of various types have been used to analyze water main datasets (Economou et al. 2012 ; American Water Works Association 2019 ; Snider and McBean 2020a ; Snider 2021 ; Barton et al. 2022 ). To what extent have the models met the expectation to predict the probability of future failures, time to next failure, and failure rate of pipe, or to predict whether or not a break will happen?
Failures reportedly could result from a large variety of factors: physical factors (e.g., pipe age, diameter, material, length, and wall thickness), environmental factors (e.g., soil type, climate, freeze/thaw properties, pipe bedding, trench backfill, traffic, and groundwater), and operational factors (e.g., number of pervious failures, water quality, internal water pressure, transient pressure, and leakage) (Stamou et al. 2000 ; Wang et al. 2009 ; Arsénio et al. 2015 ; Lin and Yuan 2019 ; Karimian et al. 2021 ). What are the main issues in data acquisition and quality? Are there factors with dominant influence on failures? Will these dominant factors change over time?
Availability of sensory and clouding systems has led to production of vast digital data from WMs. It is very crucial to take advantage of the available data to support short-term and long-term plans of asset management. The use of analytics has shown a rising trend. This review provides a timely update of the existing models for predicting failures and for management planning. Critical pipes are to be identified using the predictive models and then are further included in maintenance plans. The maintenance plans are efficiently optimized to save time, costs and resources.
Predictive analytics
Predictive models of water main failures and pipeline deteriorations (Kleiner and Rajani 2001 ; Rajani and Kleiner 2001 ; El-Abbasy et al. 2019 ; Robles-Velasco et al. 2020 ; Dawood et al. 2020a ) may be classified into two main types: a physical law-based model and a data-driven model (Rajani and Kleiner 2001 ; Snider and McBean 2020a ). The first type of model requires significant amounts of input data to analyze physical behaviors leading to a failure. The analysis involves comparing the resistance capacity of a pipeline to expected loads. The data includes an extensive list of parameters and needs to be collected from the field. Therefore, it is costly and time consuming to use physical law-based models (Rajani and Kleiner 2001 ). The implementation of the models should be limited to critical pipelines (Wilson et al. 2017 ). The second type of model uses historical data to discern patterns between historical values of some relevant parameters and breakage rates of pipelines. This type of model is much less expensive to use, compared to a physical law-based model. Thus, it is suitable to implement a data-driven model to all pipelines, as long as historical data exists (El-Abbasy et al. 2019 ; Snider and McBean 2020a ).
The data-driven models may be subdivided into a deterministic model, a probabilistic model, and an artificial intelligence model:
The deterministic model relies on regression techniques to predict time to next break of pipe or break rate and often assumes uniform breaks in water main groups. This assumption rules out uncertainties within a dataset.
The probabilistic model (e.g., a survival analysis model) uses historical data to predict the probability of water main failure. The model deals with inherent randomness that is expected to be within a dataset of pipe breaks.
The artificial intelligence model adopts a learning approach to recognizing complicated relationships between input and output data, without calculating the covariate relationships like the deterministic and probabilistic models. Using the artificial intelligence model has the potential to significantly reduce the number of field inspections needed, provide timely warning of break risks and thus avoid a large number of breaks as well as their consequences (Fu et al. 2013 ; Marzouk and Osama 2017 ; Kakoudakis et al. 2017 ; Snider and McBean 2018 ; Ghobadi et al. 2021 ).
The classification of data-driven models and their sub-categories are shown in Figure 1 .
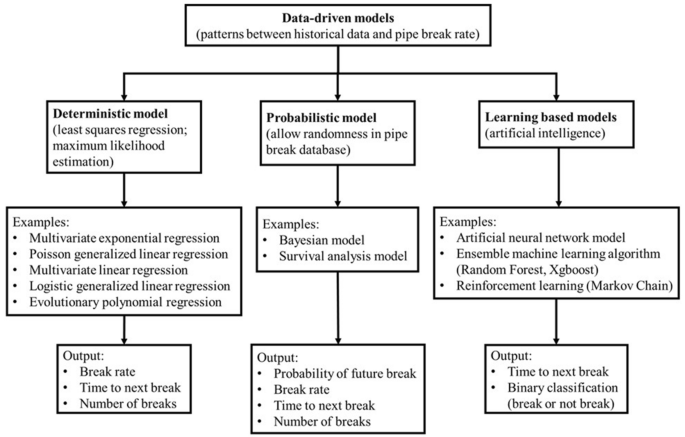
Classification of water main predictive models
WDNs are a complicated system consisting of interconnected pipes and hydraulic control elements in order to transport potable water to urban populations (Ostfeld 2015 ). The water infrastructures are aging and deteriorating drastically throughout the major urban centers, which leads to WM failures. They are major problems for municipalities due to high costs for replacement/repair and consequences such as the disruption of services, health issues resulting from contaminated water, and revenue losses (Snider and McBean 2020a ). Models should be used to predict breaks ahead of their occurrences and to plan rehabilitation and replacement. This would promote sustainable infrastructures and save costs.
This review focuses on the data-driven models, considering practicality, data requirement, and liableness. It provides a comprehensive overview of the past 15 years of literature to investigate the relationship and synergy between predictive analytics and prescriptive analytics for water mains. A structured survey of the literature was performed using such keywords as “water main deterioration”, “deterioration models”, “prediction models”, “probabilistic prediction models”, “asset management”, “water infrastructure”, “failure consequence”, “water main risk analysis”, “integrated municipal infrastructure” and “infrastructure optimization”. In total, over 60 articles were reviewed in their entirety.
The strengths and limitations of identified publications were analyzed. Several researchers have used regression, probabilistic and machine learning models for water main failure prediction, as explained above in detail. The application of these models highly depends on the availability of the dataset and desired output. The output of water main failure predictive models can be break rate, number of breaks, probability of future breaks, and time to next break. One of the great advantages of putting these studies together is that it will help municipalities to select and use the most appropriate model, depending on the availability of dataset. Therefore, by using these models, the key question of when and where the break will happen would be answered.
Wang et al. ( 2009 ) developed deterioration models, using data of water main breaks to predict annual break rates. These were multiple regression models involving pipe diameter, length, age and material, and identifying the length as having the greatest impact. This is possibly because their model output is breaks per kilometer length per year. They claimed that the models helped analyze break trends. Bruaset and Sægrov ( 2018 ) developed a linear regression model that correlated the failure rate of water main to frost heave of the ground due to the air temperature in a cold region. They found the failure rate increasing during the winter months and gray cast iron pipes (usually laid in trenches) being more vulnerable to fail. This implies that the failure rate would decrease under climate warming.
Xu and Sinha ( 2020 ) discussed some challenges and gaps in the use of survival analysis models. The use may give failure rate, number of failures, and time to next break (which can be interpreted as either the useful life span of a pipe or estimated remaining useful life). One challenge is the treatment of left truncation. In the literature, left truncation has not been addressed in most survival analyses of water pipeline failure. This would cause a bias in the results. The issue of left truncation needs attention and solutions.
An analysis of pipeline networks is costly and time consuming due to the complexity and large scale of the networks. Therefore, a failure analysis of pipelines is crucial for the efficient management of networks. There is a trend of increasing use of machine learning algorithms to predict the failure rate. Zakikhani et al. ( 2021 ) provided a review of failure prediction models, including machine learning models for oil and gas pipelines. Malek Mohammadi et al. ( 2021 ) used K-Nearest Neighbor (KNN) to predict the condition of sewer pipes. Also, machine learning algorithms have been recently used in prediction models of infrastructure failure. For example, Marcelino et al. ( 2021 ) used general machine learning to predict pavement performance.
Karimian et al. ( 2021 ) used an Evolutionary Polynomial Regression model to predict pipeline breaks. They clustered pipelines based on pipe age, diameter, length and material, and showed that pipelines of smaller diameter were more prone to failure. The occurrence of breaks was most sensitive to pipe diameter. For predicting the time to next break of ductile iron pipes, Snider and McBean ( 2018 ) made a comparison among a gradient-boosting algorithm model, an Artificial Neural Network (ANN) model and a Random Forest algorithm, suggesting the first one outperformed the other two. This is because gradient-boosting is an ensemble algorithm or a combination of multiple learning algorithms (usually decision trees) that form a stronger predictive model with better performance.
Al-Ali et al. ( 2019 ) reported a Logistic Regression (LR) model, aiming to find the most proper parameters for predicting the probability of water main failure, and leading to prioritizing pipes and planning an annual renewal. Dawood et al. ( 2020a ) suggested considering soil type, traffic loads, trenchless method of construction, contractor experience and other influential factors, for improved results of pipe deterioration model. They recommended fuzzy-based assessments to reduce the risks of failure incidents.
In the study of pipeline failures in Colombia’s WDN, Giraldo-González and Rodríguez ( 2020 ) assessed three regression models and four machine learning models. The regression models were Linear Regression, Poisson Regression (PR) and Evolutionary Polynomial Regression, and the machine learning models were ANN, Bayes, Support Vector Machine and Gradient-Boosted Tree (GBT). The machine learning models used physical factors (age, diameter and length), environmental factors (moisture content, soil contraction, expansion potential, precipitation and land use), and operational factors (valve, hydrant, and previous failure) as predictors. The study used confusion matrices, accuracy and Receiver Operating Characteristic (ROC) curves as an evaluation criterion. The study concluded that PR outperformed the other regression models and GBT outperformed the other machine learning models.
Rahbaralam et al. ( 2020 ) employed two machine learning algorithms (LR and extreme gradient boosting) and one survival analysis model (Cox proportional hazard model) to predict Barcelona’s water main failures. The algorithms were fed with data after being resampled for feature selection, feature engineering and balancing. The algorithms were evaluated using accuracy, F1 score, recall, precision, Area Under the ROC Curve (AUC) and Matthew’s Correlation Coefficient (MCC). The extreme gradient boosting technique was the best.
Water main breaks interrupt services and cause revenue losses (Snider and McBean 2020a ). Predictive models of break expected in the future help sustain WDNs and reduce costs. In Snider and McBean ( 2020b ), the gradient boosting decision tree machine learning (xgboost) was compared with Weibull proportional hazard survival analysis, in terms of the effect of censored events on time to next break of cast iron pipes. The xgboost model combines multiple decision trees, which strengthens the performance.
Snider and McBean ( 2020b ) reported that the xgboost model underpredicted time to next break because of the inability to include censored events. Removing censored events from a training dataset is not desirable for long-term planning of asset management. For this reason, they concluded that the model was adequate only for short-term planning of asset management. The Weibull proportional hazard survival analysis could learn from longer censored events in a training dataset; it frequently over-predicts break times (i.e., longer time to break) and therefore is appropriate for use for long-term planning. The analysis can give insights about pipe conditions by using historical data of pipe breaks. Note that unlike inspection data, historical data can easily be found in many water utilities (Xu and Sinha 2020 , 2021 ).
Aslani et al. ( 2021 ) used machine learning models to predict water pipeline breaks, with input of spatiotemporal data. Vulnerable locations were identified by conducting a spatial clustering. They converted the results of the clustering analysis to an independent feature called hotspot level for subsequent use in the modeling process. They suggested that the results were useful for municipalities to locate hotspots and mitigate the vulnerability by pipe component renovations.
Robles-Velasco et al. ( 2020 ) used LR and Support Vector Classification (SVC) to predict whether a pipe will break or not. LR performed slightly better than SVC. The model output was between 0 and 1. This can be interpreted as the probability of failure, which is highly desirable nowadays. The probability of failure could be used by municipalities to optimally manage their annual rehabilitation plans. Many studies apply machine learning models to pipes that have had breaks (Harvey et al. 2013 ; Shirzad et al. 2014 ; Sattar et al. 2016 ; Kutyłowska 2017 ; Kerwin and Adey 2018 ). Robles-Velasco et al. ( 2020 ) considered all pipes rather than just those which had experienced breaks. They used three homogenized models with respect to the types of material and then a global model. A correlation analysis identified the covariance between standardized variables. They reported that replacing only 3% of pipelines could prevent around 30% of failures.
Chen et al. ( 2022 ) investigated the effect of combined datasets from different utilities on the performance of machine learning models for predicting future breaks. They combined datasets belonging to six utilities in three ways: using the dataset of only one utility, using a stratified sampling of all utilities and using a combined data of all utilities. The results showed that having a large quantity of data does not result in a better prediction model, but instead a sufficient amount of high-quality data such as historical breaks gives a better prediction model.
The examination of the above studies shows that in the case where only a limited amount of input dataset is available and where the purpose is to interpret break trends, regression models could be the best choice. Although survival analysis models are more suitable for long-term management plans, they over-predict break time and cannot handle the complexity that exists in water main dataset. On the other hand, machine learning models are more appropriate for water mains with good amounts of dataset as the models can treat complex relationships between input and output variables. However, these models are suitable only for short-term management planning. Also, it seems that physical parameters which are more accessible in water main dataset and widely used throughout the literature, have more impact on the output of the models. However, the effect of other parameters has yet to be discovered. In the following subsections, the problems existing in either the models or water main datasets itself explained in detail.
Data preparation for modelling
Most machine learning algorithms require data preparations: standardization, encoding, and feature transformation. Standardization rescales all factors. Some machine learning algorithms do not need standardization, however it improves model convergence. Standardization also improves model accuracy (Buntine et al. 2009 ; Shen et al. 2016 ). Consider a support vector classifier (SVC). This algorithm works based on maximizing the distance between the separating plane (hyperplane) and the support vectors (data points closer to the hyperplane). When the algorithm calculates the distances, without standardization, features with larger values will dominate features with smaller values. Therefore, standardization is required to reduce the dominancy effect between features and improve the model convergence (Lokman et al. 2019 ). Consequently, depending on the type of machine learning model selected, standardization might help improve accuracy.
Encoding categorical attributes yields numerical values for use in SVC and LR. The two widely used coding systems: one-hot-encoding, and dummy coding, convert categorical data into binary values (Cohen et al. 2014 ; Rahbaralam et al. 2020 ; Aslani et al. 2021 ). The integer encoding assigns an integer to categorical attributes based on failure rate per unit length (Robles-Velasco et al. 2020 ). The first two coding systems have the limitation that there is a significant increase in predictors when there are a large number of categories in the categorical attributes. Therefore, depending on the amount of dataset, a suitable coding system should be selected.
In many WMs prediction models, some attributes are difficult to model because of their disparity. Consider pipe length for instance. Disparate lengths of pipes exist in a dataset. Therefore, despite the fact that length is an important predictor, it is problematic. Some authors re-cut the length by street (Winkler et al. 2018 ), some used feature transformation and logarithms of length rather than the actual length and improved the accuracy noticeably (Robles-Velasco et al. 2020 ), and others used mean values for all variables related to length (Berardi et al. 2008 ). Therefore, the length of water mains needs attention and preparation before being fed into the model for better accuracy.
In some machine learning algorithms, tuning hyperparameters is an important issue which is difficult to properly address (Liu and Zio 2019 ; Fujiwara et al. 2020 ). This is because only a few hyperparameters need to be calibrated, which is not enough to capture all the variations in the model. When there are extensive variations in a model but insufficient parameters to capture the variations, an overfitting may occur (Ahmadi et al. 2015 ). Thus, overfitting should constantly be checked and avoided.
Missing data
In WM dataset, the issue of missing data is common (Osman et al. 2018 ). Handling missing data in the preprocessing is crucial. Missing data leads to losing some valuable information and causing data insufficiency (Wu and Liu 2017 ; Winkler et al. 2018 ). Consequently, removing missing values from a dataset can result in negative effects on data-driven models, unreliable parameter predictions, loss of valuable information, bias, and poor models (Tang et al. 2019 ). Therefore, it is necessary to keep as much information as possible (Barton et al. 2022 ).
Alternatively, there are several imputation techniques to handle this issue, e.g., traditional methods such as simple ways of substituting missing data with mean, median and constant values, or more advanced methods such as imputation using machine learning algorithms (for example, substituting missing data with the mean values from KNN in the training dataset) (Levinas et al. 2021 ; Xu and Sinha 2021 ). Advanced imputation methods are often better than simple imputation methods (Osman and Bainbridge 2011 ; Kabir et al. 2019 ). It is concluded that prior to developing a prediction model, one must have clean data and ensure minimal missing data.
Imbalanced dataset
Imbalanced data, censoring, and left truncation are three important issues associated with predictions of water main failures (Scheidegger et al. 2015 ; Xu and Sinha 2020 ). In water supply networks majority of pipelines never suffered from a failure. If the majority of pipelines in a dataset have not experience a break (one class) and a minority of them have experienced at least one break (another class), the dataset is considered as imbalanced, also known as unbalanced (Robles-Velasco et al. 2020 ) and as censored (Li et al. 2016 ; Snider and McBean 2020a )). Figure 2 depicts imbalanced data belonging to the City of Kitchener water main break dataset.
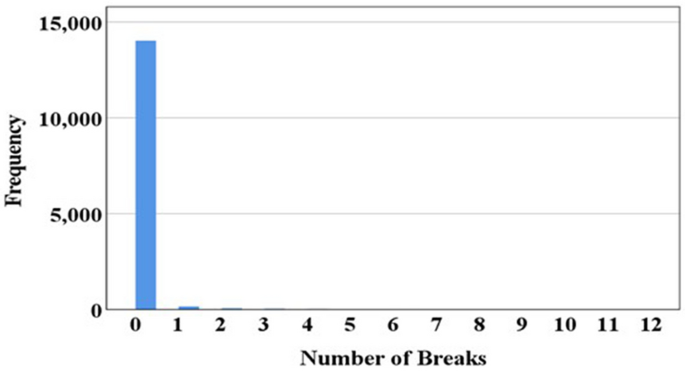
Frequency of number of breaks in a WDN (imbalanced dataset) (Data source: https://open-kitchenergis.opendata.arcgis.com/datasets/water-main-breaks/explore?location=43.459288%2C-80.434081%2C12.12 and https://open-kitchenergis.opendata.arcgis.com/datasets/water-mains/explore?location=43.434199%2C-80.474206%2C12.58 )
Dealing with imbalanced datasets is a challenging topic in data mining, receiving extensive research attention (Zhang and Wang 2013 ; Ribeiro and Reynoso-Meza 2020 ). Resampling may be implemented to an imbalanced dataset through random under-sampling, random over-sampling, and Synthetic Minority Over-sampling Technique (SMOTE) in classification models (He and Garcia 2009 ). Random under-sampling is a well-known method that removes examples of the majority class. Although this method decreases the computational time, it is at the expense of losing some valuable information (Japkowicz 2000 ; Seiffert et al. 2009 ).
Random over-sampling, on the other hand, randomly replicates the existing minority examples to make the dataset balanced. This technique also has its own limitations such as increasing the size of the dataset and causing the model to be overfitted. Thus, it is not applicable in the case of having an extensive dataset (García-Pedrajas et al. 2012 ). SMOTE randomly generates synthetic minority examples based on nearest neighbors and therefore it is a better way for balancing the dataset; it improves model performance (Fujiwara et al. 2020 ; Rahbaralam et al. 2020 ). Nevertheless, depending on the nature of dataset, one of the techniques might work better than the others.
An imbalanced dataset is also an issue in other fields, e.g., medical diagnostic and credit card fraud detection problems (Verhein and Chawla 2007 ). In such cases, the classification problem becomes very difficult since the main goal in imbalanced datasets is to predict the minority class (Huang et al. 2006 ). The models in question cannot be properly trained in the training phase and thus cannot correctly predict the minority class (Liu and Zio 2019 ). A naïve model could predict all data as the majority class and will likely achieve an accuracy of 99%. However, such models are useless in many cases. To evaluate the goodness of a model, accuracy serves a common metric measurement. However, accuracy alone is not considered as a suitable evaluation measurement in the case of an imbalanced data and might cause misinterpretation. Therefore, other metric measurements (e.g., F-measure) are very much demanded (Huang et al. 2006 ; Harvey and McBean 2014 ).
The confusion matrix is a good way of evaluation in the case of an imbalanced dataset. Accuracy and Recall are two metrics derived from the matrix. Accuracy gives the percentage of correctly predicted pipes while Recall measures the accuracy of true failures. However, higher Recall is at the expense of misclassification. AUC is another metric measurement that shows the capability of the model to avoid misclassification and can be computed from the ROC curve.
Censored events
Censoring happens when no pipe breaks are observed within a limited period of time, and this is the case in most water utilities datasets. Figure 3 illustrates an example of data censorship in water main break dataset. There are a large number of pipes in service, which have never experienced a break. Censored events can be handled by a traditional survival analysis (e.g., Cox proportional hazard models). On the contrary, many machine learning models are not capable of handling censored events. Although machine learning models are more capable of interpreting complex relationships that exist in a water main dataset, when using machine learning models, censoring is a concern.
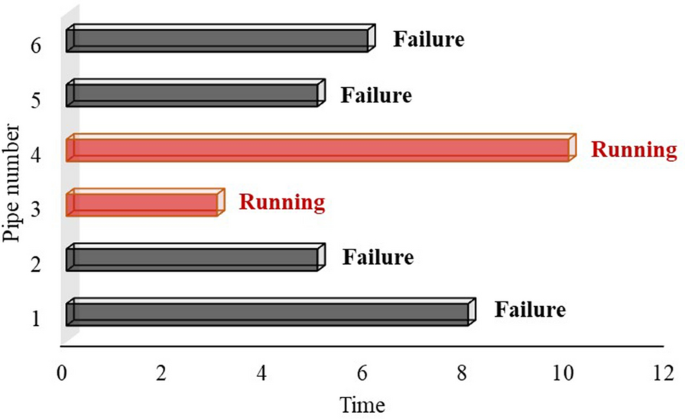
(Modified from Snider and McBean ( 2020a ))
Censored data
Censoring is almost the case for all WM datasets. Although survival models (e.g., Weibull proportional hazard survival analysis) can cope with censored data (Wang et al. 2019 ; Almheiri et al. 2021 ) and are good for long-term management planning (Snider and McBean 2020b ), they are not suitable for modeling complex relationships between variables. Machine learning algorithms, on the other hand, are very efficient to model complex relationships between variables, but they are good only for short-term management planning (Snider and McBean 2020b ). For example, xgboost has been found to surpass other single machine learning models such as Random Forest and ANN (Zhang et al. 2017 ; Snider and McBean 2018 ). The problem with xgboost is that it is not programmatically structured to deal with censored data. In fact, it removes censored data at the training stage so it cannot learn from the censored data, therefore it is constantly underpredict time to failure (Snider and McBean 2020b ).
Machine learning models are more desirable for use to predict WM failures. To cope with the problem of censoring, a survival machine learning model (a combination of machine learning with a survival statistics) can be used, one exampling being Random Survival Forest, which is relatively new. These models not only incorporate censored data but also utilize data-driven approaches to model complex relationship between input and output variables (Snider and McBean 2021 ).
Left truncation
Left truncation occurs when the records of pipe failures before collecting data are missing. Like censoring, this is also always the case in a water main dataset and it is acknowledged widely (Barton et al. 2022 ). The effects of left truncation have been overlooked in many studies (Snider and McBean 2020b ) even though this issue causes a systematic bias, especially for survival analysis models (Scheidegger et al. 2015 ; Xu and Sinha 2019 ). Instead, they assume the first recorded failure is the first real failure. This assumption will lead to bias and inaccurate predictions (Xu and Sinha 2020 ; Hawari et al. 2020 ). The scale and shape of the survival curve can be severely biased due to left truncation, which results in a change in estimates of the mean time to failure (MTTF) (Xu and Sinha 2021 ).
There are several ways to tackle the left truncation issue. One way is to revise the probability function (Mailhot et al. 2000 ; Scheidegger et al. 2013 ). Xu and Sinha ( 2021 ) proposed an integration of ANN imputation method with Weibull proportional hazard survival analysis to calibrate the survival curve and reduce MTTF estimation bias caused by left truncation. They showed a drop of bias from 14.3% to 2.1% by applying the method.
Correlation analysis
A correlation between predictors reduces the accuracy and increases computing time for most machine learning algorithms (Hall 1999 ; Kumar and Chong 2018 ), except tree-based algorithms which can handle correlations (Eisler and Holmes 2021 ). The issue of correlations between independent attributes has serious impacts, but it was not addressed (Snider and McBean 2018 ; Roccetti et al. 2019 ; Giraldo-González and Rodríguez 2020 ; Weeraddana et al. 2020 ; Rahbaralam et al. 2020 ; Dawood et al. 2020a ; Amini and Dziedzic 2021 ). There are different methods to investigate the correlation, e.g., the t-test, ANOVA, MANOVA, Chi Squared and Pearson’s correlation analysis. Depending on the nature of the dataset (i.e., being numerical or categorical), the above-mentioned methods are useful. Pearson’s correlation analysis is one of the most widely used methods, but it is useful only for identifying the correlation between numerical variables (Zhang et al. 2014 ).
Prescriptive analytics
The literature in the domain of water distribution networks can be divided into different categories in many ways. In this review, literature is divided into two main categories: predictive analytics and prescriptive analytics. In the following subsections, literature of prescriptive analytics is explained in more details.
Failure consequences assessment and risk analysis
This paper reviewed the existing literature related to identifying risk, criticality index and failure consequences for WMs. Fares and Zayed ( 2010 ) utilized a hierarchy fuzzy expert system to evaluate the risk of WM failure. Their considered 16 risk factors. According to their study, risk factors can be divided to factors that lead to failure (deterioration factors) and factors which result from failure (consequence factors). They demonstrated that the most significant influences on failure risk are pipe age, pipe material, and pipe breakage rate, respectively. Kabir et al. ( 2015 ) proposed a Bayesian Belief Network model to prioritize metallic WMs and evaluate the risk of WMs failure. They used structural integrity, hydraulic capacity, water quality and consequence factors in their model, and they claimed that any other factors could also be included in their model. They showed that the model can visualize the most vulnerable, sensitive and the highest risk pipes within a WDN.
Mugume et al. ( 2015 ) simulated a simplified synthetic water distribution system in EPANET and a synthetic urban drainage system in the Storm Water Management Model. They investigated the system performance under the condition of pipe failure. They focused on minimizing failure consequences to improve resilience in urban water systems. They also investigated the effect of rehabilitation strategies including pipe replacement on resilience. They showed that if failure scenarios are considered during urban water systems design, the loss of system functionality could be minimized.
Al-Zahrani et al. ( 2016 ) identified the vulnerable locations in a WDN using a fuzzy-based decision support system. These vulnerable locations experience more structural failures as well as failures in supplying water at the target quality. Their model was applied to a case where a risk index was developed to show both the probability of failures and their impacts. They showed that the model helped utilities to prioritize pipes within the system based on the overall failure risk.
Vishwakarma and Sinha ( 2020 ) used the fuzzy inference method for developing the consequence of failure. They proposed a quantitative risk matrix for risk visualization, that compared to semi-quantitative and qualitative risk matrix, reduce subjectivity in the design process. Their model framework covers different types of the consequence of failure assessment such as economic, environmental and social impacts, as well as operational intelligence and complexity of renewal activities. They improve previously developed techniques of assessing failure consequences by using a quantitative risk matrix. Utilizing risk assessment has multiple advantages for management programs, such as supporting pipes renewal prioritization decisions and moving from reactive maintenance plans to proactive plans.
Phan et al. ( 2019 ) used a risk assessment framework in a case study of WM in a WDN. The calculation of the probability of failure used Weibull distribution. They used a fuzzy inference system to aggregate failure consequences because unifying different types of consequences into one outcome is difficult. Consequences consist of impacts on the redundancy/vulnerability of the network, water loss and rehabilitation costs and of impacts on public health. They used the diameter to quantify the volume of water loss and algebraic connectivity to consider the topological consequence. The topological consequence is useful for redundancy reduction. In order to prioritize water mains, a risk map is developed for use by decision-makers.
Balekelayi and Tesfamariam ( 2021 ) performed a hydrodynamic assessment for the wastewater system of Calgary using ordered weighted averaging technique to identify the criticality index of the wastewater pipes. A dynamic deterioration model was combined with the proposed criticality index to determine the operational risk of the wastewater pipes. This technique helps municipalities to prioritize the inspection and replacement of sewer pipes. They showed that the technique can successfully identify the criticality index of wastewater pipes when hydrodynamic data are not available. Using the information, hydraulic models can be regularly updated and thus wastewater pipe inspection plans can be prioritized. The results of the study can also be used for water mains.
Risk is a multiplication of the probability of failure (POF) and consequences of failure (COF). The probability of WM failures can be derived from the prediction models explained earlier in predictive analytics section. However, in order to achieve a good maintenance plan, POF is not the only factor that matters, and COF is another important factor. This is because some pipes might have the least POF but the highest COF in the network, which might be overlooked in the prioritization plan. Therefore, assessing COF is also of relevance. The failure consequences can be economic, environmental and social impacts. The indirect costs of failure should also be taken into consideration. Thus, the determination of failure consequences and hence the risk are difficult, because of uncertainties and many factors involved. Often, fuzzy techniques are used to deal with uncertainties and to quantify failure consequences and risk factors.
Maintenance planning, scheduling and prioritization
In this section, papers in regard to water loss minimization and asset management plans have been collected. The deterioration of assets is inevitable due to aging. Thus, an efficient asset management becomes crucial for assets to continue delivering an adequate level of service. There are efficient asset management plans in various infrastructures sectors such as road networks, urban railways and metro systems, buildings, wastewater and drainage systems (Mohammadi et al. 2018 , 2019 , 2020 ; Dziedzic et al. 2021 ). However, there is less progress in case of water systems asset management.
Kleiner et al. ( 2010 ) developed a non-homogeneous Poisson model for the analysis and forecast of breakage patterns in individual water mains, considering both static and dynamic factors. Their case study was for a water utility in Eastern Ontario. Different costs associated with each pipe were considered, including the costs of pipe replacement and repair, the costs of water loss due to failure, and cost-saving due to roadwork coordination. They used the results of pipe break predictions for the water main renewal schedule plan, utilizing a multi-objective genetic algorithm.
Malm et al. ( 2015 ) developed a Cost-Benefit Analysis for leakage reduction. They compared the costs and benefits for each alternative over time. They also considered uncertainty analysis. The results show that considering uncertainty analysis improved the results of the Cost-Benefit Analysis. They considered four different alternatives to reduce leakage in their case study of Gothenburg. It was found that reactively repairing, despite a high leakage rate, is more cost effective, compared to proactively pipe replacement.
Zyoud and Fuchs-Hanusch ( 2019 , 2020 ) applied different techniques to a real water supply system in Palestine. They compared the traditional Multi Criteria Decision Making approach and the Analytic Hierarchy Process (AHP) method for a water loss management problem. Although AHP is easy to implement and has strong potential in structuring and decomposing complex decision problems, it cannot handle uncertainties. Therefore, Fuzzy AHP has been used to deal with uncertainty and incomplete information.
Barton et al. ( 2022 ) revealed that the quantity and quality of data have an important impact on the accuracy of WM failure models, and poor data results in low accuracy of models. They suggested that there should be increased focus on data collection since poor quality data makes it hard for utilities to manage WMs rehabilitation plan. They show that long term management plans for water mains remain a challenging issue and require further attention.
Ghobadi et al. ( 2021 ) proposed a pipe replacement scheduling method based on a life cycle cost assessment. In order to obtain an optimal replacement plan, a multi-objective nondominated sorting genetic algorithm (NSGA-II) is used. The proposed replacement plan avoids investment peaks and smooth the investment time series based on life cycle cost. Unlike many other studies, they considered that limitations exist in the annual budget in their model. They show that by using online monitoring and recording failure data, the accuracy of the pipe failure rate is improved, and the annual replacement plans can be updated. The scheduling plan becomes near optimal.
Decision making software tools and methodologies would help municipalities to perform their water infrastructure maintenance plans more efficiently. These plans usually consider a set of predefined alternatives. However, more practical replacement plans which affect several pipes simultaneously rather than just replacing individual pipes haven’t been considered in these methodologies. Ramos-Salgado et al. ( 2022 ) scheduled a sustainable water supply replacement plan with a five-step infrastructure asset management framework. (1) As the first step, a replacement priority index for every network asset has been obtained. (2) Despite the previous maintenance strategies (considering individual pipelines), they used street sections as the operational replacement unit to reduce the social consequences related to each intervention. (3) They considered the replacement plan of two adjacent pipes at the same time even with having different priority of replacement for the sake of operational and convenience criteria, since it is more acceptable by utilities and more aligned with their policies. Also, a fair budget allocation performed in their study based on social and geographic criteria to ensure a decent investment distribution between districts and towns. (4) After specifying the replacement priority of the network assets, a short-term, mid-term and long-term replacement plan is required. In this regard, a set of indicators for performance evaluation of the network is needed which specify the investment level and certain courses of action. A combination of four indicators is used name infrastructure value index (ratio between the value of the infrastructure at the current state and its replacement cost), average network age, average risk index, and the average probability of failure. These indicators are easy to calculate and interpret. They also present various information on the performance of the network. (5) Lastly a mathematical technique is used to calculate the required budget more efficiently.
Maintenance coordination and prioritization
There have been tremendous efforts on maintenance plans as an individual asset management plan. A coordinated asset management plan is much needed to better manage existing infrastructure assets, but the coordination has been neglected by many municipalities. This section gives particular attention to the coordination of interrelated infrastructures, optimum replacement time of them (e.g., roads, water and sewers) and prioritization of their budget allocation. Integrated rehabilitation actions among the co-located infrastructure assets are necessary when developing a renewal plan. This could decrease or avoid unnecessary rework, rehabilitation costs, service disruptions and risks (Halfawy 2008 ; Abusamra 2018 ).
Marzouk and Osama ( 2015 ) proposed a decision support tool to determine the optimal time of maintenance and replacement of mixed infrastructures simultaneously (i.e., pavement, water pipes, sewer pipes, gas pipes, and electrical cables). This approach could prevent costs associated with the surface layer of pavements to be destroyed multiple times (for example once for sewer pipes replacement and once for water pipes replacement). The useful life of different infrastructures was first identified by simulation, and then depending on the replacement time and costs, a decision was made on the optimal maintenance and replacement time. With regard to uncertainties of models, a fuzzy approach was applied. The key goal of their study was the minimization of the total costs of infrastructure replacement.
Marzouk and Osama ( 2017 ) presented a method for the coordinated maintenance of road, water distribution and wastewater distribution networks. First, a deterioration model is developed using a hierarchical fuzzy expert system technique to assess the condition of each infrastructure asset. Then, a risk model is developed using a fuzzy Monte Carlo simulation to calculate POF and AHP to calculate COF. Lastly, a multi-objective optimization using genetic algorithm (GA) is developed, with four objective functions: (1) minimizing the overall risk, (2) maximizing level of service (LOS), (3) maximizing the overall conditions of the assets, and (4) minimizing life cycle cost (LCC). The optimization model considers seven scenarios of actions for: (1) road segment only; (2) water only; (3) sewer only; (4) road and water; (5) road and sewer; (6) sewer and water; (7) road, sewer and water. The optimization constraints were set to meet the minimum requirements of the condition, performance and risk for all infrastructures within the annual budget. The results showed an average integrated risk index of 5.45 over a planning horizon of 20 years. Over 86% of the projects were recommended under integrated scenarios as follows: road, water and sewer at 38%; road and sewer at 24%; road and water at 24%. These maximize cost saving.
Abusamra ( 2018 ) pointed out numerous attempts to improve infrastructure maintenance and intervention plans within a limited budget. However, most of them were successful only in developing a plan for short-term planning and a single asset. The author proposed optimization models to help decision makers to identify a coordinated maintenance plan for the co-located infrastructure assets (i.e., roads, water, and sewer). Two multi-objective models were discussed: (1) evolutionary GAs optimization, which used a set of meta-heuristic rules to find a near-optimum solution; (2) linear programming optimization to find an exact solution. The objective function was to maximize an overall improvement and to maximize the network health index. The results showed an overall enhancement (time, cost, efficiency, risk, etc.) of 29% over a planning horizon of 25 years, achieved from coordinating the interventions. Compared to the conventional approach, coordination reduced disruptions and interventions by 67%.
Amador-Jimenez and Mohammadi ( 2020 ) considered different budgeting scenarios such as worst-first, silos, and trade-off optimization, to assess the pros and cons of proposed scenarios. They aimed to investigate the prioritization of budget allocation and management plans for different infrastructure assets (i.e., pavements, sanitary sewers, storm sewers and water mains), based on the proposed scenarios, and to select the superior management plan among all. They show that a trade-off optimization analysis improves results, giving the highest priority to water mains and lower priority to pavements and storm pipes in terms of investment management planning.
Very recently, Shahata et al. ( 2022 ) proposed a multi-stage integer programming that is capable of optimizing the most suitable, cost-effective renewal action (if any) for road, sewer and water infrastructure assets. The objective function was to maximize risk reduction in a cost-effective manner. Their decision-making approach used risk assessment and a performance rating model. The model also used rehabilitation alternatives, giving priority to integrated renewal actions. They showed that the approach is capable of reducing risk costs by using integrated actions (e.g., road, water and sewer by 36%; road and sewer by 23%; road and water by 25%). They also showed that their integrated model can enhance budget-saving, compared to the conventional silos approach (renewal plan of only each infrastructure). In order to improve the model's practicality, the consequence of each intervention alternative such as the impact on travel delay, noise pollution costs, lost business revenue, etc. should also be considered.
Discussions
This review of water asset management analytics has revealed: a) a need to explore the influence of environmental factors on WM failures; b) a need to consider both direct and indirect costs in optimal mitigation analysis and replacement prioritization. The environmental factors indirectly contribute to failures. The contribution is particularly significant for WMs in cold regions. Failure models should be coupled with costs (direct and indirect) as a constraint in optimal scheduling plans. The coupling renders failure predictions meaningful as the ultimate goals are to update asset management plans and prioritize rehabilitation or replacement.
Further research efforts are needed to reveal new insights about contributing mechanisms of WM failures, to create novel ideas for reliable predictions of failures, and to invent ways for putting theoretical predictions into practical use in managing and maintaining WMs in a cost-effective manner. The mechanisms are more complex in cold regions. More details about potential avenues for future research are discussed below under each category of analytics:
Directions of future research in predictive analytics
In spite of extensive studies of WM failures over the past decades, significant knowledge gaps exist in predictive analytics. Environmental factors (e.g., weather conditions, climate factors and so on) are reportedly less influential than physical factors (e.g., pipe diameter, pipe length and so on). However, the influence of the environmental factors such as climatic variations and freezing in cold regions has received little attention (Kleiner and Rajani 2002 ; Farmani et al. 2017 ; Demissie et al. 2017 ; Almheiri et al. 2020 ). In the cold regions, pipes are more susceptible to break due to temperature fluctuations. Frozen water inside a pipe expands. Even if the pipe does not break, it can significantly degrade. Freezing temperature fluctuations result in extra stresses on pipes. Moisture on the ground can cause frosts at freezing temperatures and lead to ground movement and hence stresses on the pipes. Cast-iron pipes are more prone to failures at freezing temperatures because of the erosion of soils around them. If they are not lined with protection materials, they begin to corrode from inside and ultimately break. In future research, it would be meaningful to create homogenous groups of pipes based on the environmental factors such as soil type, freezing index, temperature, precipitation and frost depth in order to investigate their influence on WM failures.
The past studies have overlooked issues related to the apparent age of pipes based on their conditions. An application of rehabilitation techniques such as lining and cathodic retrofit to existing pipes causes a change in the conditions of the pipes and thus redefines their ages. Therefore, the influence of applied rehabilitation techniques and the resulting change need to be investigated.
One important step before developing any prediction model is data preprocessing and preparation. The missing gap of data needs to be handled properly. If the available amount of the missing data is not meaningful, they can simply be excluded. Otherwise, an existing missing gap should be filled by predictions using advanced imputation methods. Correlated attributes must be removed as they will decrease modelling efficiency significantly. One needs to pay adequate attention to imbalanced dataset and general data preparation before applying any prediction method because these steps impact modelling reliability significantly. Resampling dataset is a good way to cope with imbalanced dataset.
Unresolved issues of censoring, and left truncation are common with WM datasets. One way to deal with the issues is to use survival machine learning models (a combination of machine learning with a survival statistics). The models can handle both censoring and a complex relationship between input and output variables. Table 1 presents a summary of predictive analytics and the applied techniques published in the past 13 years.
Directions of future research in prescriptive analytics
Previous studies using prescriptive analytics have been limited to consideration of economic costs as the maintenance objective to optimize. The social and environmental costs (indirect costs) associated with a failure are commonly ignored in WM maintenance planning and rehabilitation scheduling. Future research should aim to maximize the system reliability and at the same time minimize the risk index and failure consequences (costs). Beside economic costs, the social and environmental costs can have significant influence on maintenance planning and scheduling, and they should be considered.
The multiplication of probability and COF determines the risk factor; through this link, a risk map can be developed and utilized to develop a maintenance prioritization plan. The coupling of a WM prediction model, probability of WM failure and COF allows us to develop a precise, practical maintenance prioritization plan. This goal can be achieved using an optimization model, together with decision-making methods. The goal should be set in a way to reduce leakage, which in turn decreases expenses (direct and indirect) and increases the expectancy life of assets. However, long-term management plans remain challenging and further attention is needed.
The literature in related to optimization of maintenance/replacement time for infrastructures networks with coordination and prioritization of maintenance activities are rare. In reality, a WM infrastructure is often maintained in association with other infrastructure such as pavement, and thus the asset management impact of one infrastructure on the other is inevitable. The mutual impact remains essentially an under explored area. A prescriptive analysis of interdependent infrastructures would be helpful to prioritize budget allocations and to identify the optimal replacement/maintenance time in a realist setting.
In conclusion, the need to adopt a coordinated maintenance plan for integrated infrastructure assets is extensively acknowledged in industry and academia. When the assets reach an unacceptable LOS, which need some actions and interventions, the optimum decision on how to repair all overlapping assets using the pre-existing and limited budget and without overspending, remains challenging. Therefore, priorities should be set in a way to answer these questions: Which asset is more critical and needs immediate action? What are the actions/interventions (repair, rehabilitation, replace or do nothing)? When is the best time the work should be done? One important requirement for all coordinated maintenance plans is the ability to support long-term planning. In this regard, the life cycles of different infrastructure assets should be considered in these models. Table 2 presents a summary of prescriptive analytics and the applied techniques published in the past 12 years.
Proposition
In the light of the above literature review and after considering the knowledge gaps related to the existing analytics methods and issues associate with water main datasets, establishing an integrated approach for smart water mains asset management is advocated (Figure 4 ) incorporating the synergy between failure models (predictive analytics) and maintenance strategies (prescriptive analytics). Most WM datasets mainly consist of physical factors of pipes such as age, diameter, length and material. Usually, they do not include operational factors such as annual average daily traffic (AADT), number of breaks, water pressure, and environmental factors such as freezing and thawing index, temperature, precipitation, frost depth, and rain deficit. Therefore, in order to investigate the effects of the environmental factors, this study suggests merging them with WM datasets. After cleansing and careful data pre-processing, dimensionality reduction is useful to reduce dataset dimensions and computing time. To aggregate the efforts for similar regions with similar characteristics, one may perform clustering which is relatively new in this domain. The next step is to select features that contribute the most in failure prediction models. Concretely, with sufficient data, a prediction model can be developed as the ultimate step in predictive analytics.
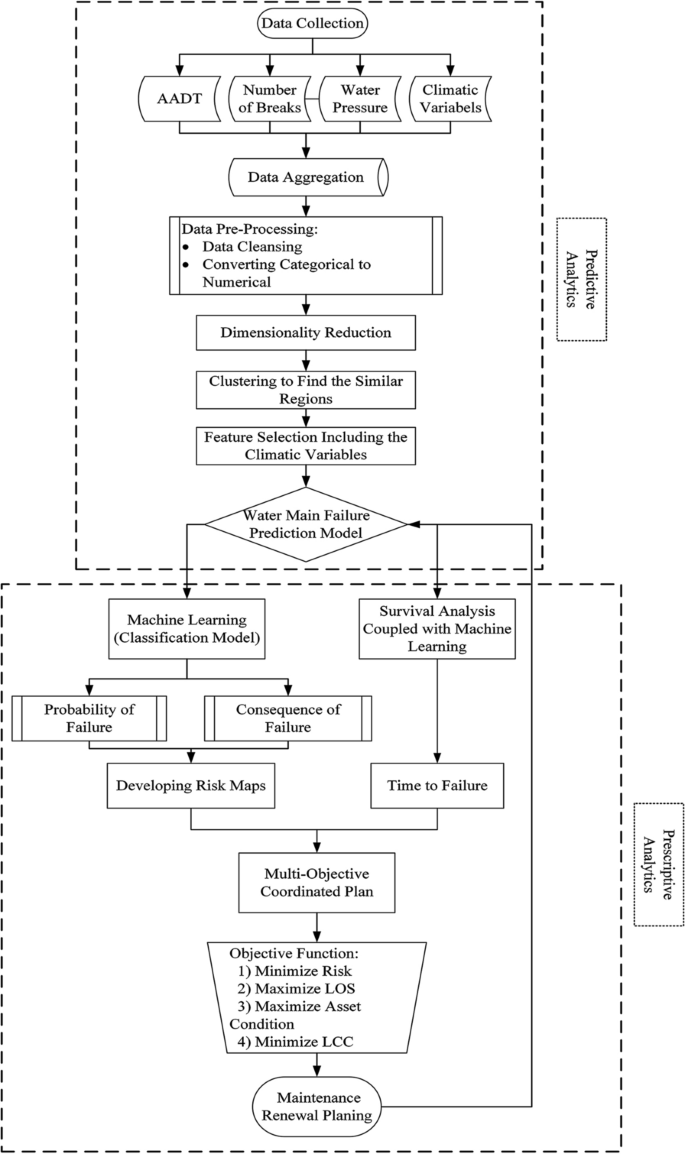
Proposed integrated predictive and prescriptive analytics for smart water main asset management
In regard to prescriptive analytics, depending on the types of prediction model in previous stage, either the time to failure or POF/COF could be mapped across the water mains network. To obtain COF, the indirect costs of failure, such as proximity to environmental/external factors (e.g., rail tracks and transmission gas mains) and the impact on the LOS and the costumer class (e.g., hospital, emergency services, residential) could be considered. By minimizing the risk of failure, the infrastructure maintenance plan can be prioritized accordingly. Using the prediction models resulted from the predictive analytics step, a multi-objective maintenance plan could be developed in coordination with other infrastructures such as roads and sewer pipes. The other optimization objectives could be maximizing LOS, maximizing asset condition, and minimizing LCC. Lastly, the rehabilitation/replacement plan will be scheduled. It is expected that after implementing the rehabilitation/replacement plan in WMs, the condition of the networks will change. So, the predictive models should be updated based on the new information after each prediction-prescription-implementation cycle.
Conclusions
Water pipe failures have increased drastically due to a slow rate of replacements and thus aging of WMs. This issue is difficult to resolve because such networks are complex and are typically buried underground. In many municipalities, most parts of the networks have reached the end of their service life, expediting even more failures in near future. Given that failures incur revenue losses and cause interruptions to service and economic activities, it becomes increasingly urgent to find better solutions. Various financial, societal, and technical constraints make it infeasible to think of replacing aging WMs, which typically serves many residential, commercial, industrial and institutional consumers, and which consists of a vast network of interconnected pipelines, pumps, valves, regulators and tanks. Thus, predicting near-future failures is of economic, social and environmental relevance.
This review provided a comprehensive overview of the methods proposed for predicting and minimizing the failures and their consequences. It has provided new insights into the knowledge gaps identified in the existing studies related to the applications of predictive and prescriptive analytics in water systems asset management. In spite of extensive research efforts over the past decades, the treatment of imbalanced data, censoring and left truncation remains as key research gaps. The other gaps correspond to how to increase sustainability, reliability and resilience of WM systems through the use of predictive models and efficient rehabilitation planning.
Considering the literature and the identified gaps, this study proposed a failure analytics framework for WMs and discussed a number of avenues for future research. It is worthy to highlight that the quality of dataset could have a significant impact on the performance of prediction models. To achieve this goal, this review recommends that municipalities use advanced inspection technologies which result in establishing more accurate prediction models, leading in turn to more precise data-drive prescription analytics that improve the reliability of WMs and create cost efficiency gains.
Abbreviations
Pipe age (year)
Area Under the ROC Curve
Artificial Neural Network
Break density (Breaks/Km 2 )
Analytic Hierarchy Process
Break year (year)
Consequences Of Failure
Pipe diameter (mm)
Freezing Index (degree days)
Genetic Algorithm
Gradient-Boosted Tree
K-Nearest Neighbor
Pipe length (m)
Level Of Service
Life Cycle Cost
Pipe location
Logistic Regression
Matthew’s Correlation Coefficient
Moisture content (%)
Number of previous breaks
Number of connections
Network type
Poisson Regression
Precipitation (mm)
Probability Of Failure
Receiver Operating Characteristic
Soil resistivity
Support Vector Classification
Synthetic Minority Over-sampling Technique
Pipe thickness
Water Distribution Network
Water pressure (KPa)
Installation year (year)
Abusamra S (2018) Coordination and multi-objective optimization framework for managing municipal infrastructure under performance-based contracts, PhD Thesis, Concordia University, Montreal, QC, Canada
Ahmadi M, Cherqui F, Aubin J-B, Le GP (2015) Sewer asset management: impact of sample size and its characteristics on the calibration outcomes of a decision-making multivariate model. Urban Water J 13:41–56. https://doi.org/10.1080/1573062X.2015.1011668
Article Google Scholar
Al-Ali AM, Laurent J, Dulot JP (2019) Developing deterioration prediction model for the potable water pipes renewal plan–case of Jubail Industrial City, KSA. Desalin Water Treat 176:324–332. https://doi.org/10.5004/dwt.2020.25539
Al-Zahrani M, Abo-Monasar A, Sadiq R (2016) Risk-based prioritization of water main failure using fuzzy synthetic evaluation technique. J Water Supply Res Technol 65:145–161. https://doi.org/10.2166/AQUA.2015.051
Almheiri Z, Meguid M, Zayed T (2021) Failure modeling of water distribution pipelines using meta-learning algorithms. Water Res 205:117680. https://doi.org/10.1016/J.WATRES.2021.117680
Article CAS Google Scholar
Almheiri Z, Meguid M, Zayed T (2020) An approach to predict the failure of water mains under climatic variations. Int J Geosynth Gr Eng 64(6):1–16. https://doi.org/10.1007/S40891-020-00237-8
Amador-Jimenez L, Mohammadi A (2020) Decision making methods to prioritise asset-management plans for municipal infrastructure. Infrastruct Asset Manag 8:11–24. https://doi.org/10.1680/JINAM.19.00064
American Water Works Association (2019) Condition assessment of water mains. American Water Works Association, Denver
Google Scholar
Amini M, Dziedzic R (2021) Comparison of machine learning classifiers for predicting water main failure. Proc Can Sociery Civ Eng Annu Conf. https://www.mdpi.com/2073-4441/12/4/1153/pdf
Arsénio AM, Dheenathayalan P, Hanssen R et al (2015) Pipe failure predictions in drinking water systems using satellite observations. Struct Infrastruct Eng 11:1102–1111. https://doi.org/10.1080/15732479.2014.938660
Aslani B, Mohebbi S, Axthelm H (2021) Predictive analytics for water main breaks using spatiotemporal data. Urban Water J. https://doi.org/10.1080/1573062X.2021.1893363
Asnaashari A, McBean EA, Gharabaghi B, Tutt D (2013) Forecasting watermain failure using artificial neural network modelling. Can Water Resour J 38:24–33. https://doi.org/10.1080/07011784.2013.774153
Atef A (2010) Optimal condition assessment policies for water and sewer infrastructure (Doctoral dissertation, Nile University)
Balekelayi N, Tesfamariam S (2021) Operational risk-based decision making for wastewater pipe management. J Infrastruct Syst 27:04020042. https://doi.org/10.1061/(asce)is.1943-555x.0000586
Barton NA, Hallett SH, Jude SR (2022) The challenges of predicting pipe failures in clean water networks: a view from current practice. Water Supply 22:527–541. https://doi.org/10.2166/WS.2021.255
Berardi L, Giustolisi O, Kapelan Z, Savic DA (2008) Development of pipe deterioration models for water distribution systems using EPR. J Hydroinformatics 10:113–126. https://doi.org/10.2166/HYDRO.2008.012
Besner MC, Prévost M, Regli S (2011) Assessing the public health risk of microbial intrusion events in distribution systems: Conceptual model, available data, and challenges. Water Res 45:961–979. https://doi.org/10.1016/J.WATRES.2010.10.035
Bruaset S, Sægrov S (2018) An analysis of the potential impact of climate change on the structural reliability of drinking water pipes in cold climate regions. Water 10:411. https://doi.org/10.3390/W10040411
Buntine W, Grobelnik M, Mladenić D, Shawe-Taylor J (2009) Machine learning and knowledge discovery in databases. Lect Notes Comput Sci. https://doi.org/10.1007/978-3-642-04174-7
Chen TY-J, Vladeanu G, Yazdekhasti S, Daly CM (2022) Performance evaluation of pipe break machine learning models using datasets from multiple utilities. J Infrastruct Syst 28:05022002. https://doi.org/10.1061/(ASCE)IS.1943-555X.0000683
Cohen P, West SG, Aiken LS (2014) Applied multiple regression/correlation analysis for the behavioral sciences. Psychol Press. https://doi.org/10.4324/9781410606266
Dawood T, Elwakil E, Novoa HM, Delgado JFG (2020) Artificial intelligence for the modeling of water pipes deterioration mechanisms. Autom Constr 120:103398. https://doi.org/10.1016/j.autcon.2020.103398
Dawood T, Elwakil E, Novoa HM, Delgado JFG (2020b) Water pipe failure prediction and risk models: state-of-the-art review. Can J Civ Eng 47:1117–1127. https://doi.org/10.1139/CJCE-2019-0481
Demissie G, Asce SM, Tesfamariam S et al (2017) Prediction of Pipe failure by considering time-dependent factors: dynamic Bayesian Belief Network Model. ASCE-ASME J Risk Uncertain Eng Syst Part A Civ Eng 3:04017017. https://doi.org/10.1061/AJRUA6.0000920
Dziedzic R, Amador L, An C et al (2021) A framework for asset management planning in sustainable and resilient cities. IEEE Int Symp Technol Soc. https://doi.org/10.1109/ISTAS52410.2021.9629158
Economou T, Kapelan Z, Bailey TC (2012) On the prediction of underground water pipe failures: zero inflation and pipe-specific effects. J Hydroinformatics 14:872–883. https://doi.org/10.2166/HYDRO.2012.144
Eisler C, Holmes M (2021) Applying automated machine learning to improve budget estimates for a naval fleet maintenance facility. In ICPRAM. https://doi.org/10.5220/0010302205860593
El-Abbasy MS, Zayed T, El CH et al (2019) Simulation-based deterioration patterns of water pipelines. Struct Infrastruct Eng 15:965–982. https://doi.org/10.1080/15732479.2019.1599965
Fares H, Zayed T (2010) Hierarchical fuzzy expert system for risk of failure of water mains. J Pipeline Syst Eng Pract 1:53–62. https://doi.org/10.1061/(ASCE)PS.1949-1204.0000037
Farmani R, Kakoudakis K, Behzadian K, Butler D (2017) Pipe failure prediction in water distribution systems considering static and dynamic factors. Procedia Eng 186:117–126. https://doi.org/10.1016/J.PROENG.2017.03.217
Folkman S (2018) Water main break rates in the USA and Canada: a comprehensive study. Mech Aerosp Eng Fac Publ
Fu G, Kapelan Z, Kasprzyk JR, Reed P (2013) Optimal design of water distribution systems using many-objective visual analytics. J Water Resour Plan Manag 139:624–633. https://doi.org/10.1061/(ASCE)WR.1943-5452.0000311
Fujiwara K, Huang Y, Hori K et al (2020) Over- and under-sampling approach for extremely imbalanced and small minority data problem in health record analysis. Front Public Heal 8:178. https://doi.org/10.3389/FPUBH.2020.00178
García-Pedrajas N, Peŕez-Rodríguez J, De Haro-Garciá A (2012) OligoIS: Scalable instance selection for class-imbalanced data sets. IEEE Trans Cybern 43:332–346. https://doi.org/10.1109/TSMCB.2012.2206381
Ghobadi F, Jeong G, Kang D (2021) Water pipe replacement scheduling based on life cycle cost assessment and optimization algorithm. Water (switzerland) 13:605. https://doi.org/10.3390/w13050605
Giraldo-González MM, Rodríguez JP (2020) Comparison of statistical and machine learning models for pipe failure modeling in water distribution networks. Water (Switzerland). https://doi.org/10.3390/W12041153
Halfawy MR (2008) Integration of Municipal Infrastructure Asset Management Processes: Challenges and Solutions. J Comput Civ Eng 22:216–229. https://doi.org/10.1061/(ASCE)0887-3801(2008)22:3(216)
Hall M (1999) Correlation-based feature selection for machine learning. Doctoral dissertation, University of Waikato, Dept. of Computer Science
Hamilton S, Charalambous B (2013) Leak detection: technology and implementation. IWA Publishing, London, UK
Book Google Scholar
Harvey R, McBean EA (2014) Comparing the utility of decision trees and support vector machines when planning inspections of linear sewer infrastructure. J Hydroinformatics 16:1265–1279. https://doi.org/10.2166/HYDRO.2014.007
Harvey R, McBean EA, Gharabaghi B (2013) Predicting the timing of water main failure using artificial neural networks. J Water Resour Plan Manag 140:425–434. https://doi.org/10.1061/(ASCE)WR.1943-5452.0000354
Hawari A, Alkadour F, Elmasry M, Zayed T (2020) A state of the art review on condition assessment models developed for sewer pipelines. Eng Appl Artif Intell 93:103721. https://doi.org/10.1016/J.ENGAPPAI.2020.103721
He H, Garcia EA (2009) IEEE Transactions on knowledge and data engineering. IEEE Trans Knowl Data Eng 21:1263–1284. https://doi.org/10.1109/TKDE.2008.239
Huang D-S, Li K, Irwin GW et al (2006) Under-sampling approaches for improving prediction of the minority class in an imbalanced dataset. Intell Control Autom. https://doi.org/10.1007/978-3-540-37256-1_89
Japkowicz N (2000) The class imbalance problem: Significance and strategies. In: In Proc. of the Int’l Conf. on Artificial Intelligence. pp 111–117
Jenkins L, Gokhale S, Asce F, Mcdonald M (2014) Comparison of pipeline failure prediction models for water distribution networks with uncertain and limited data. J Pipeline Syst Eng Pract 6:04014012. https://doi.org/10.1061/(ASCE)PS.1949-1204.0000181
Kabir G, Tesfamariam S, Francisque A, Sadiq R (2015) Evaluating risk of water mains failure using a Bayesian belief network model. Eur J Oper Res 240:220–234. https://doi.org/10.1016/J.EJOR.2014.06.033
Kabir G, Tesfamariam S, Hemsing J, Sadiq R (2019) Handling incomplete and missing data in water network database using imputation methods. Sustain Resilient Infrastruct 5:365–377. https://doi.org/10.1080/23789689.2019.1600960
Kakoudakis K, Behzadian K, Farmani R, Butler D (2017) Pipeline failure prediction in water distribution networks using evolutionary polynomial regression combined with K-means clustering. Urban Water J 14:737–742. https://doi.org/10.1080/1573062X.2016.1253755
Karimian F, Kaddoura K, Zayed T et al (2021) Prediction of breaks in municipal drinking water linear assets. J Pipeline Syst Eng Pract 12:04020060. https://doi.org/10.1061/(ASCE)PS.1949-1204.0000511
Kerwin S, Adey BT (2018) Performance comparison for pipe failure prediction using artificial neural networks. In: In Proc., 6th Int. Symp. on Life-Cycle Civil Engineering. pp 1337–1342
Kleiner Y, Nafi A, Rajani B (2010) Planning renewal of water mains while considering deterioration, economies of scale and adjacent infrastructure. Water Sci Technol Water Supply 10:897–906. https://doi.org/10.2166/ws.2010.571
Kleiner Y, Rajani B (2001) Comprehensive review of structural deterioration of water mains: statistical models. Urban Water 3:131–150. https://doi.org/10.1016/S1462-0758(01)00033-4
Kleiner Y, Rajani B (2002) Forecasting variations and trends in water-main breaks. J Infrastruct Syst 8:122–131. https://doi.org/10.1061/(ASCE)1076-0342(2002)8:4(122)
Kumar S, Chong I (2018) Correlation analysis to identify the effective data in machine learning: Prediction of depressive disorder and emotion states. Int J Environ Res Public Health 15:2907. https://doi.org/10.3390/ijerph15122907
Kutyłowska M (2017) Prediction of failure frequency of water-pipe network in the selected city. Period Polytech Civ Eng 61:548–553. https://doi.org/10.3311/PPCI.9997
Levinas D, Perelman G (2021) Ostfeld A (2021) Water leak localization using high-resolution pressure sensors. Water 13:591. https://doi.org/10.3390/W13050591
Li J, Zhou S, Han Y (2016) Advances in battery manufacturing, service, and management systems. Wiley, Hoboken
Liang B, Li Z, Wang Y, Chen F (2018) Long-term RNN: predicting hazard function for proactive maintenance of water mains. Int Conf Inf Knowl Manag Proc 1687–1690. https://doi.org/10.1145/3269206.3269321
Lin P, Yuan XX (2019) A two-time-scale point process model of water main breaks for infrastructure asset management. Water Res 150:296–309. https://doi.org/10.1016/J.WATRES.2018.11.066
Liu J, Zio E (2019) Integration of feature vector selection and support vector machine for classification of imbalanced data. Appl Soft Comput 75:702–711. https://doi.org/10.1016/J.ASOC.2018.11.045
Lokman S-F, Othman AT, Bakar MHA, Musa S (2019) The impact of different feature scaling methods on intrusion detection for in-vehicle controller area network (CAN). Int Conf Adv Cyber Secur. https://doi.org/10.1007/978-981-15-2693-0_14
Mailhot A, Pelletier G, Noël J-F, Villeneuve J-P (2000) Modeling the evolution of the structural state of water pipe networks with brief recorded pipe break histories: Methodology and application. Water Resour Res 36:3053–3062. https://doi.org/10.1029/2000WR900185
Malek Mohammadi M, Najafi M, Serajiantehrani R et al (2021) Using machine learning to predict condition of sewer pipes. Pipelines 2021:185–195. https://doi.org/10.1061/9780784483602.022
Malm A, Moberg F, Rosén L (2015) Pettersson TJR (2015) Cost-benefit analysis and uncertainty analysis of water loss reduction measures: case study of the gothenburg drinking water distribution system. Water Resour Manag 2915(29):5451–5468. https://doi.org/10.1007/s11269-015-1128-2
Marcelino P, de Lurdes AM, Fortunato E, Gomes MC (2021) Machine learning approach for pavement performance prediction. Int J Pavement Eng 22:341–354. https://doi.org/10.1080/10298436.2019.1609673
Marzouk M, Osama A (2017) Fuzzy-based methodology for integrated infrastructure asset management. Int J Comput Intell Syst 10:745–759. https://doi.org/10.2991/ijcis.2017.10.1.50
Marzouk M, Osama A (2015) Fuzzy approach for optimum replacement time of mixed infrastructures. Civ Eng Environ Syst 32:269–280. https://doi.org/10.1080/10286608.2014.1002715
Misiunas D (2005) Failure monitoring and asset condition assessment in water supply systems. PhD Thesis. Department of Electrical Engineering and Automation, Lund University, Sweden
Mohammadi A, Amador-Jimenez L, Nasiri F (2020) Reliable, effective, and sustainable Urban railways: a model for optimal planning and asset management. J Constr Eng Manag 146:04020057. https://doi.org/10.1061/(ASCE)CO.1943-7862.0001839
Mohammadi A, Amador-Jimenez L, Nasiri F (2019) Review of asset management for metro systems: challenges and opportunities. Transp Rev 39:309–326. https://doi.org/10.1080/01441647.2018.1470119
Mohammadi A, Igwe C, Amador L, Nasiri F (2018) Novel asset management framework for road maintenance. Canadian Society of Civil Engineers (CSCE) Annual Conference, June 13-18, Fredericton, NB, Canada
Mugume SN, Diao K, Astaraie-Imani M et al (2015) Enhancing resilience in urban water systems for future cities. Water Sci Technol Water Supply 15:1343–1352. https://doi.org/10.2166/ws.2015.098
Muhlbauer WK (2004) Pipeline risk management manual : ideas, techniques, and resources. Elsevier, Amsterdam
Osman H, Bainbridge K (2011) Comparison of statistical deterioration models for water distribution networks. J Perform Constr Facil 25:259–266. https://doi.org/10.1061/(ASCE)CF.1943-5509.0000157
Osman MS, Abu-Mahfouz AM, Page PR (2018) A Survey on data imputation techniques: water distribution system as a use case. IEEE Access 6:63279–63291. https://doi.org/10.1109/ACCESS.2018.2877269
Ostfeld A (2015) Water distribution networks. Stud. Comput Intell 565:101–124. https://doi.org/10.1007/978-3-662-44160-2_4
Phan HC, Dhar AS, Hu G, Sadiq R (2019) Managing water main breaks in distribution networks––A risk-based decision making. Reliab Eng Syst Saf 191:106581. https://doi.org/10.1016/J.RESS.2019.106581
Rahbaralam M, Modesto D, Cardús J, Abdollahi A, Cucchietti FM (2020) Predictive analytics for water asset management: machine learning and survival analysis. arXiv preprint arXiv:2007.03744
Rajani B, Kleiner Y (2001) Comprehensive review of structural deterioration of water mains: physically based models. Urban Water 3:151–164. https://doi.org/10.1016/S1462-0758(01)00032-2
Ramos-Salgado C, Muñuzuri J, Aparicio-Ruiz P, Onieva L (2022) A comprehensive framework to efficiently plan short and long-term investments in water supply and sewer networks. Reliab Eng Syst Saf. 219:108248. https://doi.org/10.1016/J.RESS.2021.108248
Ribeiro VHA, Reynoso-Meza G (2020) Ensemble learning by means of a multi-objective optimization design approach for dealing with imbalanced data sets. Expert Syst Appl 147:113232. https://doi.org/10.1016/J.ESWA.2020.113232
Robles-Velasco A, Cortés P, Muñuzuri J, Onieva L (2020) Prediction of pipe failures in water supply networks using logistic regression and support vector classification. Reliab Eng Syst Saf 196:106754. https://doi.org/10.1016/J.RESS.2019.106754
Roccetti M, Delnevo G, Casini L, Cappiello G (2019) Is bigger always better? a controversial journey to the center of machine learning design, with uses and misuses of big data for predicting water meter failures. J Big Data 6:1–23. https://doi.org/10.1186/S40537-019-0235-Y
Sattar AM, Gharabaghi B, McBean EA (2016) Prediction of timing of watermain failure using gene expression models. Water Resour Manag 30:1635–1651. https://doi.org/10.1007/S11269-016-1241-X
Scheidegger A, Leitão JP, Scholten L (2015) Statistical failure models for water distribution pipes – a review from a unified perspective. Water Res 83:237–247. https://doi.org/10.1016/J.WATRES.2015.06.027
Scheidegger A, Scholten L, Maurer M, Reichert P (2013) Extension of pipe failure models to consider the absence of data from replaced pipes. Water Res 47:3696–3705. https://doi.org/10.1016/J.WATRES.2013.04.017
Seiffert C, Khoshgoftaar TM, Van Hulse J, Napolitano A (2009) RUSBoost: A hybrid approach to alleviating class imbalance. IEEE Trans Syst Man, Cybern A Syst Humans 40:185–197. https://doi.org/10.1109/TSMCA.2009.2029559
Shahata K, El-Zahab S, Zayed T, Alfalah G (2022) Rehabilitation of municipal infrastructure using risk-based performance. Autom Constr 140:104335. https://doi.org/10.1016/J.AUTCON.2022.104335
Shen X, Gong X, Cai Y et al (2016) Normalization and integration of large-scale metabolomics data using support vector regression. Metabolomics 12:1–12. https://doi.org/10.1007/S11306-016-1026-5
Shirzad A, Tabesh M, Farmani R (2014) A comparison between performance of support vector regression and artificial neural network in prediction of pipe burst rate in water distribution networks. KSCE J Civ Eng 18:941–948. https://doi.org/10.1007/S12205-014-0537-8
Snider B (2021) Preparing for the replacement era: understanding north america’s aging water distribution systems
Snider B, McBean EA (2018) Improving time to failure predictions for water distribution systems using extreme gradient boosting algorithm. In: proceedings of the 1st international water system distribution analysis (WDSA)/ computing and control for the water industry conference, July 23-25, Kingston, ON, Canada
Snider B, McBean EA (2021) Combining machine learning and survival statistics to predict remaining service life of watermains. J Infrastruct Syst 27:04021019. https://doi.org/10.1061/(ASCE)IS.1943-555X.0000629
Snider B, McBean EA (2020a) Watermain breaks and data: the intricate relationship between data availability and accuracy of predictions. Urban Water J 17:163–176. https://doi.org/10.1080/1573062X.2020.1748664
Snider B, McBean EA (2020b) Improving urban water security through pipe-break prediction models: machine learning or survival analysis. J Environ Eng 146:04019129. https://doi.org/10.1061/(asce)ee.1943-7870.0001657
St.Clair AM, Sinha S (2012) State-of-the-technology review on water pipe condition, deterioration and failure rate prediction models. Urban Water J 9:85–112. https://doi.org/10.1080/1573062X.2011.644566
Stamou AI, Latsa M, Assimacopoulos D (2000) Design of two-storey final settling tanks using mathematical models. J Hydroinformatics 2:235–245. https://doi.org/10.2166/HYDRO.2000.0021
Tang K, Parsons DJ, Jude S (2019) Comparison of automatic and guided learning for Bayesian networks to analyse pipe failures in the water distribution system. Reliab Eng Syst Saf 186:24–36. https://doi.org/10.1016/J.RESS.2019.02.001
Trudeau MP (2020) SWM and urban water: Smart management for an absurd system? Water Int 45:678–692. https://doi.org/10.1080/02508060.2020.1783063
Verhein F, Chawla S (2007) Using significant, positively associated and relatively class correlated rules for associative classification of imbalanced datasets. Seventh IEEE Int Conf Data Min. https://doi.org/10.1109/ICDM.2007.63
Vishwakarma A, Sinha SK (2020) Development of a consequence of failure model and risk matrix for water pipelines infrastructure systems. Pipelines 2020. VA Am Soc Civ Eng. https://doi.org/10.1061/9780784483213019
Wang P, Li Y, Reddy CK (2019) Machine learning for survival analysis: a survey. ACM Comput Surv 51:1–36. https://doi.org/10.1145/3214306
Wang Y, Zayed T, Moselhi O (2009) Prediction models for annual break rates of water mains. J Perform Constr Facil 23:47–54. https://doi.org/10.1061/(asce)0887-3828(2009)23:1(47)
Weeraddana D, Liang B, Li Z, et al (2020) Utilizing machine learning to prevent water main breaks by understanding pipeline failure drivers. arXiv Prepr arXiv200603385. https://doi.org/10.48550/arXiv.2006.03385
Wilson D, Filion Y, Moore I (2017) State-of-the-art review of water pipe failure prediction models and applicability to large-diameter mains. Taylor Fr 14:173–184. https://doi.org/10.1080/1573062X.2015.1080848
Winkler D, Haltmeier M, Kleidorfer M et al (2018) Pipe failure modelling for water distribution networks using boosted decision trees. Struct Infrastruct Eng 14:1402–1411. https://doi.org/10.1080/15732479.2018.1443145
Wu Y, Liu S (2017) A review of data-driven approaches for burst detection in water distribution systems. Urban Water J 14:972–983. https://doi.org/10.1080/1573062X.2017.1279191
Xu H, Sinha SK (2020) Applying survival analysis to pipeline data: gaps and challenges Pipelines 2020. VA Am Soc Civ Eng. https://doi.org/10.1061/9780784483213017
Xu H, Sinha SK (2021) Modeling pipe break data using survival analysis with machine learning imputation methods. J Perform Constr Facil 35:04021071. https://doi.org/10.1061/(ASCE)CF.1943-5509.0001649
Xu H, Sinha SK (2019) A framework for statistical analysis of water pipeline field performance data. Pipelines 2019 Multidiscip Top Util Eng Surv. VA Am Soc Civ Eng DOI. https://doi.org/10.1061/9780784482506019
Yerri SR, Piratla KR, Matthews JC et al (2017) Empirical analysis of large diameter water main break consequences. Resour Conserv Recycl 123:242–248. https://doi.org/10.1016/J.RESCONREC.2016.03.015
Zakikhani K, Nasiri F, Zayed T (2021) A failure prediction model for corrosion in gas transmission pipelines: Proc Inst Mech Eng. Part O J Risk Reliab 235:374–390. https://doi.org/10.1177/1748006X20976802
Zhang C, Liu C, Zhang X, Almpanidis G (2017) An up-to-date comparison of state-of-the-art classification algorithms. Expert Syst Appl 82:128–150. https://doi.org/10.1016/J.ESWA.2017.04.003
Zhang Y, Wang D (2013) A cost-sensitive ensemble method for class-imbalanced datasets. Abstr Appl Anal. https://doi.org/10.1155/2013/196256
Zhang Z, McDonnell KT, Zadok E, Mueller K (2014) Visual correlation analysis of numerical and categorical data on the correlation map. IEEE Trans vis Comput Graph 21:289–303. https://doi.org/10.1109/TVCG.2014.2350494
Zyoud SH, Fuchs-Hanusch D (2019) Comparison of several decision-making techniques: a case of water losses management in developing countries. Int J Inf Technol Decis Mak 18:1551–1578. https://doi.org/10.1142/S0219622019500275
Zyoud SH, Fuchs-Hanusch D (2020) An integrated decision-making framework to appraise water losses in municipal water systems. Int J Inf Technol Decis Mak 19:1293–1326. https://doi.org/10.1142/S0219622020500297
Download references
Acknowledgements
The authors are very much thankful to four anonymous reviewers whose comments and suggestions were very helpful in improving the quality of this manuscript.
Not applicable.
Author information
Authors and affiliations.
Department of Building, Civil and Environmental Engineering, Concordia University, Montreal, QC, H3G 1M8, Canada
Atefeh Delnaz, Fuzhan Nasiri & S. Samuel Li
You can also search for this author in PubMed Google Scholar
Contributions
The work has been done under the supervision of FN and SI. The first draft of the manuscript was written by AD. Reviews, edits, and further analysis were performed by FN and SI. All authors commented on previous versions of the manuscript. All authors read and approved the final manuscript.
Corresponding author
Correspondence to Fuzhan Nasiri .
Ethics declarations
Ethics approval and consent to participate, consent for publication, competing interests.
The corresponding author, Fuzhan Nasiri, is an unpaid member of the editorial board of Environmental Systems Research. The authors declare that they have no other competing interests.
Additional information
Publisher's note.
Springer Nature remains neutral with regard to jurisdictional claims in published maps and institutional affiliations.
Rights and permissions
Open Access This article is licensed under a Creative Commons Attribution 4.0 International License, which permits use, sharing, adaptation, distribution and reproduction in any medium or format, as long as you give appropriate credit to the original author(s) and the source, provide a link to the Creative Commons licence, and indicate if changes were made. The images or other third party material in this article are included in the article's Creative Commons licence, unless indicated otherwise in a credit line to the material. If material is not included in the article's Creative Commons licence and your intended use is not permitted by statutory regulation or exceeds the permitted use, you will need to obtain permission directly from the copyright holder. To view a copy of this licence, visit http://creativecommons.org/licenses/by/4.0/ .
Reprints and permissions
About this article
Cite this article.
Delnaz, A., Nasiri, F. & Li, S.S. Asset management analytics for urban water mains: a literature review. Environ Syst Res 12 , 12 (2023). https://doi.org/10.1186/s40068-023-00287-7
Download citation
Received : 12 January 2023
Accepted : 23 February 2023
Published : 18 April 2023
DOI : https://doi.org/10.1186/s40068-023-00287-7
Share this article
Anyone you share the following link with will be able to read this content:
Sorry, a shareable link is not currently available for this article.
Provided by the Springer Nature SharedIt content-sharing initiative
- Urban water distribution networks
- Water mains
- Maintenance
- Asset management
- Data Analytics
- Failure analysis
- Reliability
Academia.edu no longer supports Internet Explorer.
To browse Academia.edu and the wider internet faster and more securely, please take a few seconds to upgrade your browser .
Enter the email address you signed up with and we'll email you a reset link.
- We're Hiring!
- Help Center
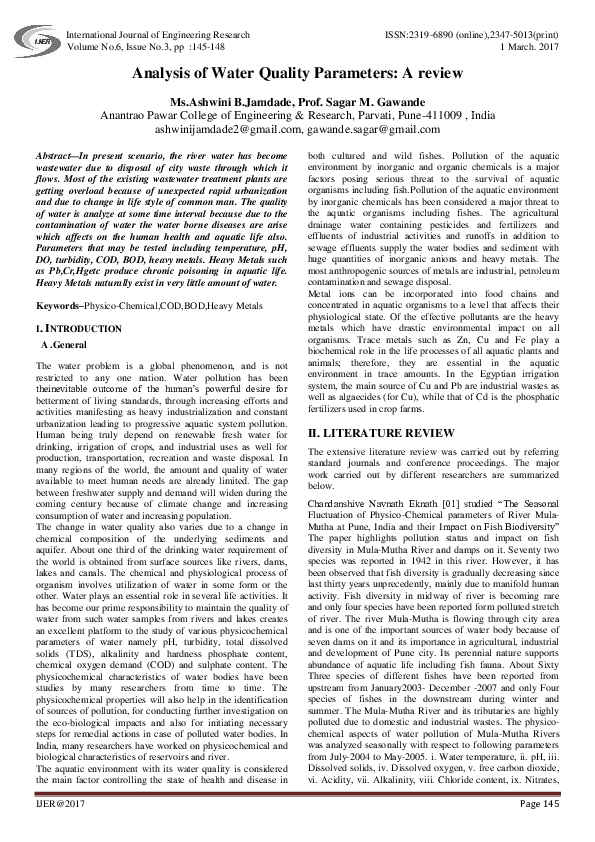
Analysis of Water Quality Parameters: A review

In present scenario, the river water has become wastewater due to disposal of city waste through which it flows. Most of the existing wastewater treatment plants are getting overload because of unexpected rapid urbanization and due to change in life style of common man. The quality of water is analyze at some time interval because due to the contamination of water the water borne diseases are arise which affects on the human health and aquatic life also. Parameters that may be tested including temperature, pH, DO, turbidity, COD, BOD, heavy metals. Heavy Metals such as Pb,Cr,Hgetc produce chronic poisoning in aquatic life. Heavy Metals naturally exist in very little amount of water.
RELATED PAPERS
Syuhada Dwi Setiaji
dialnet.unirioja.es
Henry Izquierdo
Http Www Theses Fr
Martin Kogelschatz
Sébastien Lemière
Canadian journal of surgery. Journal canadien de chirurgie
Ibrahima Sall
Azmi Novendri
ACM Transactions on Information Systems
Hongzhi Yin
Majallah-i ̒Ulum-i Bāghbānī
Vida Chalavi
Diagnostics
lucia lospalluti
American Journal of Respiratory and Critical Care Medicine
Shoji Kudoh
Revue des Maladies Respiratoires Actualités
Sarra Zairi
Multiciencias
Hector ramiro Viera moran
Clinical Neurophysiology
Social Inclusion
Hwok-Aun Lee
BMC Complementary Medicine and Therapies
Ossy Kasilo
Health & Social Care in the Community
Angela Dickinson
BMC Genomics
Johanna Björkroth
Archives of Transport
Andrzej Chudzikiewicz
Self Publication
Rasesh Singh Dikhit
Rutgers毕业证书 罗格斯大学学位证
Ana isabel Gomez Cabezuelo
Leandro Roberto Neves
실시간카지노 토토사이트
SHU文凭证书 SHU毕业证成绩单
RELATED TOPICS
- We're Hiring!
- Help Center
- Find new research papers in:
- Health Sciences
- Earth Sciences
- Cognitive Science
- Mathematics
- Computer Science
- Academia ©2024
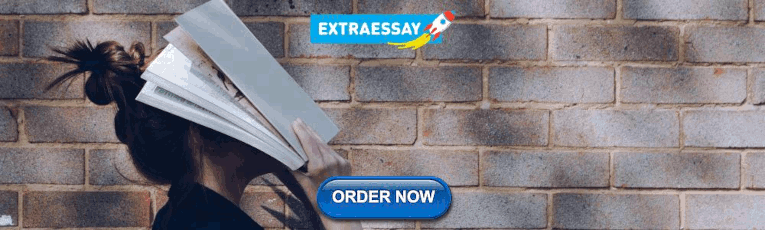
IMAGES
VIDEO
COMMENTS
Water quality analysis is required mainly for monitoring. purpose. Some importance of such assessment includes: (i) To check whether the water quality is in compliance. with the standards, and ...
Introduction" also describes the literature review of the related problems on water quality, in related works subsection, with an exhaustive survey of the various applications and case studies ...
This paper will discuss the sources of clean water and drinking water and their problems in developing countries; water quality and its relation to public health problems in these countries; and what efforts that can be make to improve water quality. The method used is a literature review from the latest journals.
Water quality index (WQI) is one of the most used tools to describe water quality. It is based on physical, chemical, and biological factors that are combined into a single value that ranges from 0 to 100 and involves 4 processes: (1) parameter selection, (2) transformation of the raw data into common scale, (3) providing weights and (4) aggregation of sub-index values. The background of WQI ...
The primary aim of this paper was to critically review the most commonly used WQI models and determine which were the most accurate. This involved a review of 110 published manuscripts from which we identified 21 WQI models used globally (see Fig. 1), which were then individually and comparatively assessed.The review identified seven basic WQI models from which most other WQI models have been ...
Water quality analysis was used to present the household perception of water quality from rural and urban areas. A questionnaire was prepared to focus on consumers' water quality perceptions on color, taste, and odor. ... Lumb A., Sharma T. C., Bibeault J. F. (2011). A review of genesis and evolution of water quality index (WQI) and some ...
Reviewing the literature revealed that most of the studies are based on four sections: underwater CDOM measurements, in situ hyperspectral measurements, water-surface reflected radiance by means of remote sensor on a satellite or an airborne platform, and functional data analysis [49,153]. The literature showed that CDOM could be quantified ...
Evaluation of water quality is a priority work nowadays. In order to monitor and map, the water quality for a wide range on different scales (spatial, temporal), the geospatial technique has the potential to minimize the field and laboratory work. The review has emphasized the advance of remote sensing for the effectiveness of spectral analysis, bio-optical estimation, empirical method, and ...
In this review, the importance of a robust frame work for drinking water quality monitoring, assessment and management has been discussed. This review presents the global overview of the drinking water quality, illuminating the global challenges of water supply system from catchment to consumers and briefly discussing appropriate regulatory frameworks and risk analysis tools.
While previous studies have already performed literature reviews or meta analysis to identify key determinants of household water consumption and household water demand modelling, they usually ...
The bibliographic coupling analysis identifies a cluster of 16 papers related to integration and connected to planning, decision, and management. There is a lack of contribution with a systemic approach to water resources by way of integrating actors and values, such as including local contexts. 1. Introduction.
Abstract. With the advent of this new era of water crisis, save water is the cry all over. Water sources are encroached from every existence on Earth. Saving water needs a systematic monitoring approach to determine its quality. Availability of Internet of Things (IoT) and remote sensing techniques mark the ease of congregating, analyzing and ...
The current review includes 1) a systematic literature search combined with a double expert quality assessment scoring (QAS) for each study that met inclusion criteria, 2) systematic data retrieval and analysis, 3) a comprehensive environmental hotspot analysis, 4) an assessment of the water and energy nexus at different aquaculture production ...
Research published from 2011 to 2021 includes 57 (72%) of the articles analysed, followed by 1999-2010 with 28% of relevant literature. This literature review revealed 37 journals published studies related to the topic - the leading journals in the publication of water and sanitation affordability are the Utilities Policy, Journal of ...
2.1 Purpose. This chapter aims to determine the current state of knowledge on river pollution management by discussing relevant water quality parameters and their significance in relation to river pollution assessment. It also reviews available policy instruments for river pollution management to better understand their implications.
This study presents a review of the state-of-the-art literature on water pipe failure predictions, assessment of water losses risk, optimal pipe maintenance plans, and maintenance coordination strategies. In addition, it provides a categorization of water main (WM) failures as well as a taxonomy of WM maintenance strategies. In particular, predictive and prescriptive analytics are highlighted ...
Water is a liquid at ambient conditions and it often co-exists on Earth with its solid state ice & gaseous state water vapors or steam. Water covers 70.9% of the earth's surface and is vital for all forms of life. Water on earth moves continually through a cycle of evaporation or transpiration, precipitation and runoff, ultimately reaching ...
Measurement of pH: The pH is important parameter of water, which determines the suitability of water for various purposes such as drinking, bathing, cooking, washing and agriculture etc. The pH level of water having desirable limit is 6.5 to 8.5 as specified by the BIS. Pure water is said to be neutral, with a pH of 7.
The purpose of this report is to present a review of literature on the water quality and environmental toxicology effects of TDA and RMA. The first part of this review focuses on field studies of TDA used both above and below the water table. This will be followed by a discussion of the water quality considerations of four specific applications: