Empower students to do their best,
Original work.
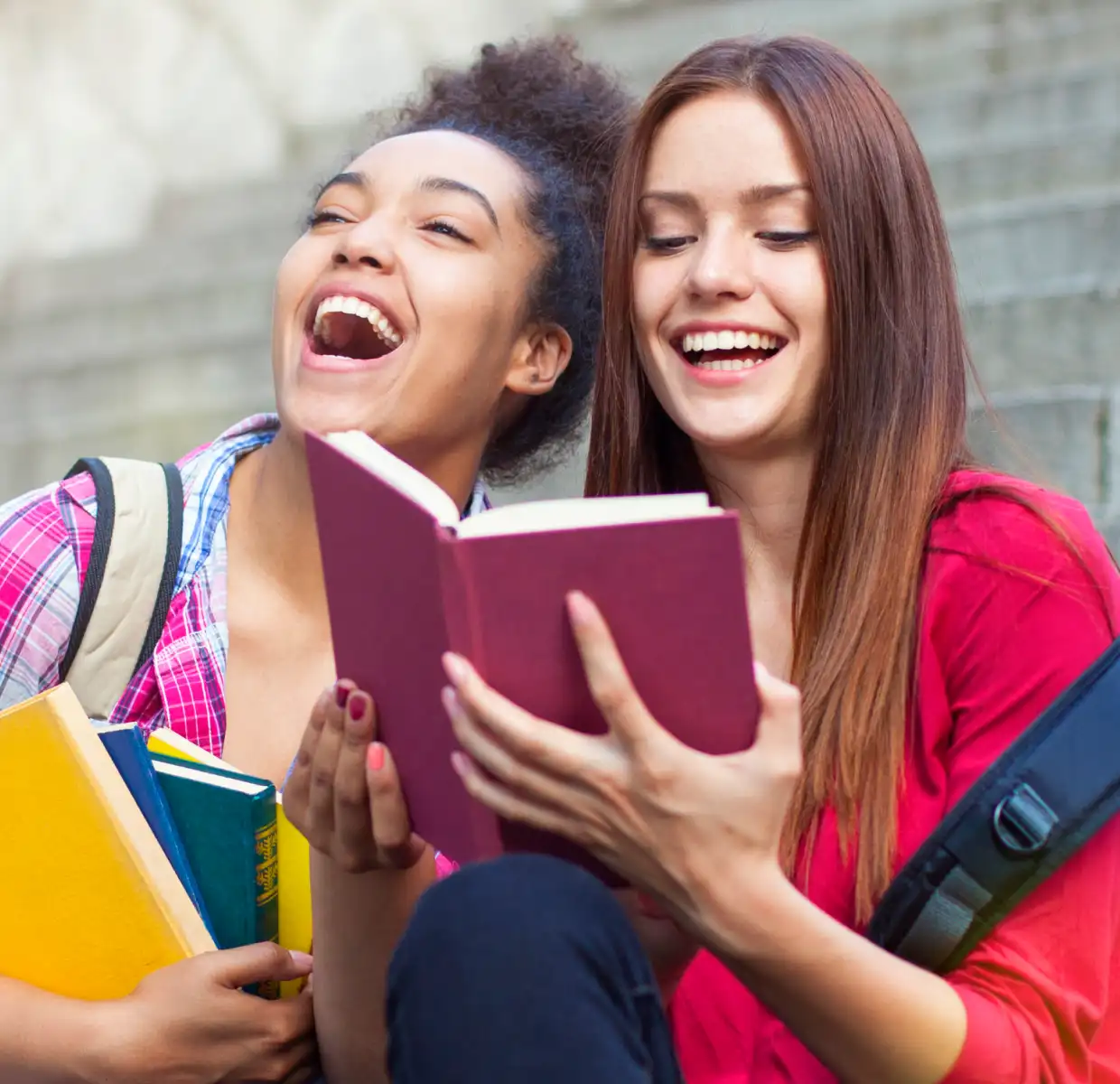
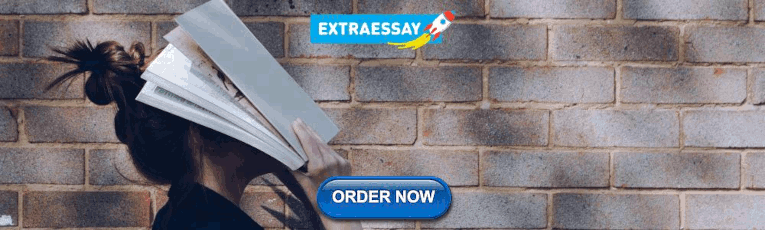
Advance learning with an AI writing detection solution built for educators
Our advanced AI writing detection technology is highly reliable and proficient in distinguishing between AI- and human-written text and is specialized for student writing. What’s more, it’s integrated into your workflow for a seamless experience.
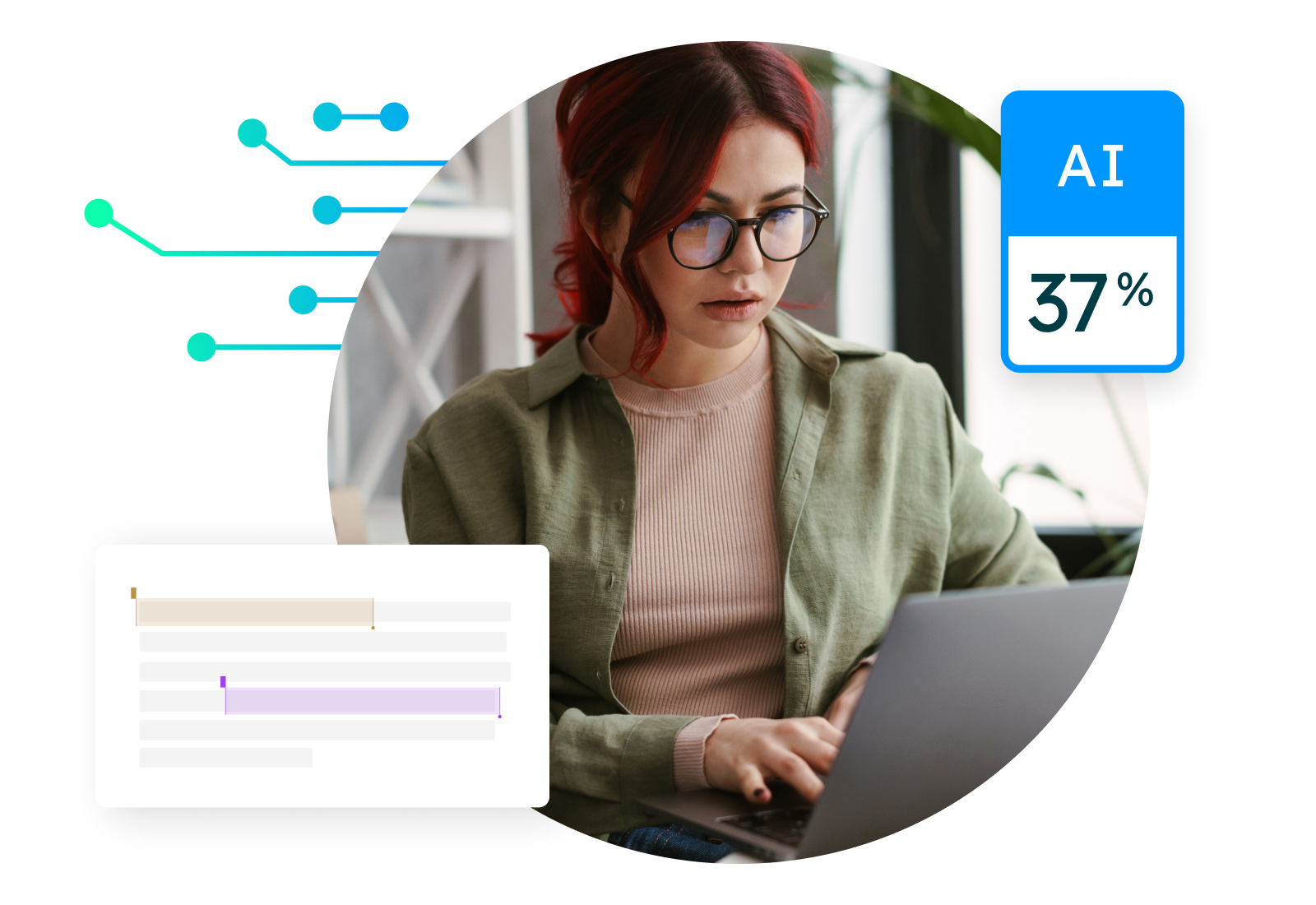
Student success starts here
Uphold academic integrity.
Ensure original work from students and safeguard the value of writing.
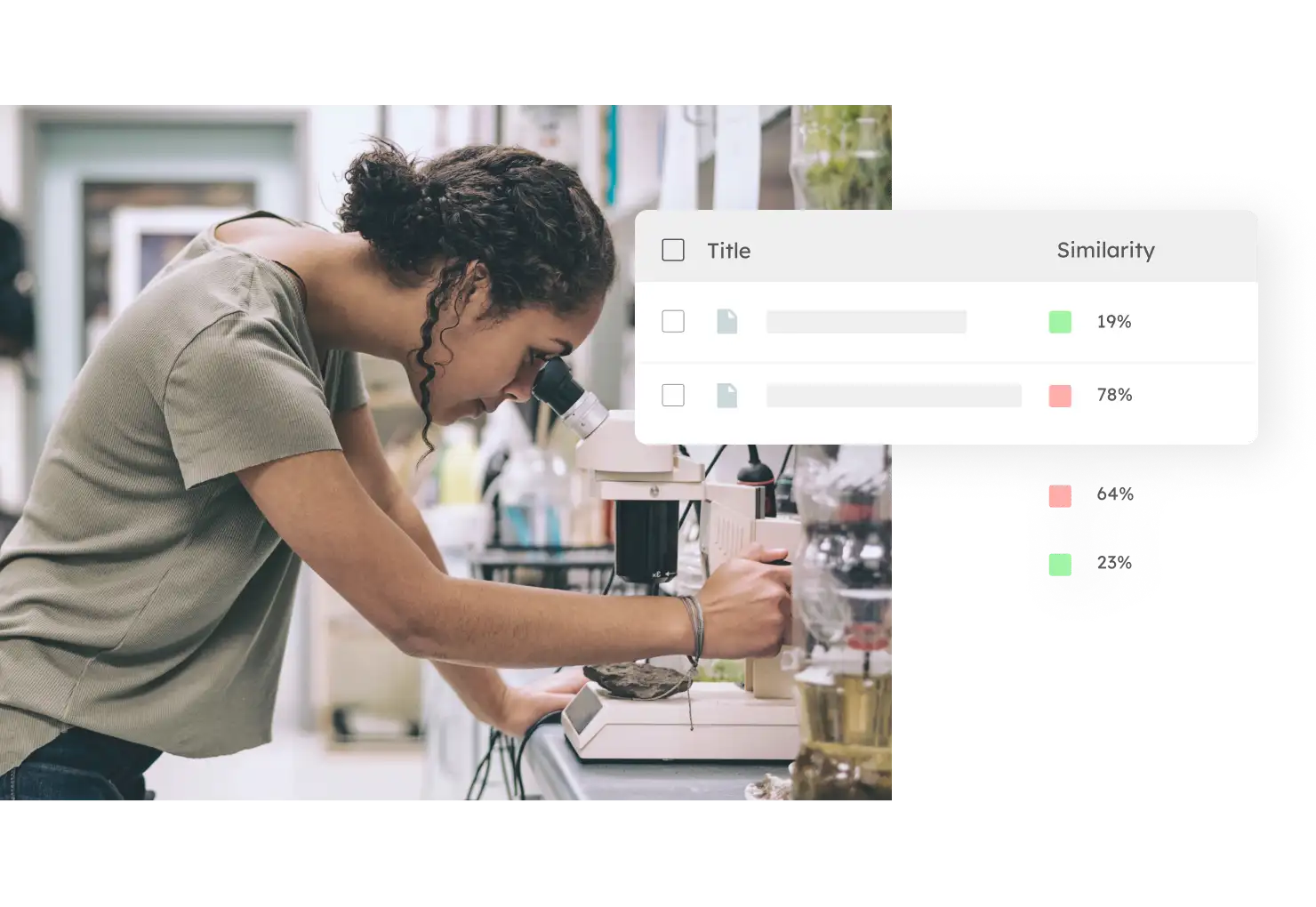
Assessments with transparency into AI usage
Flexible solutions enabling educators to design and deliver student assessments their way, while shaping AI-enhanced student writing with integrity and confidence.
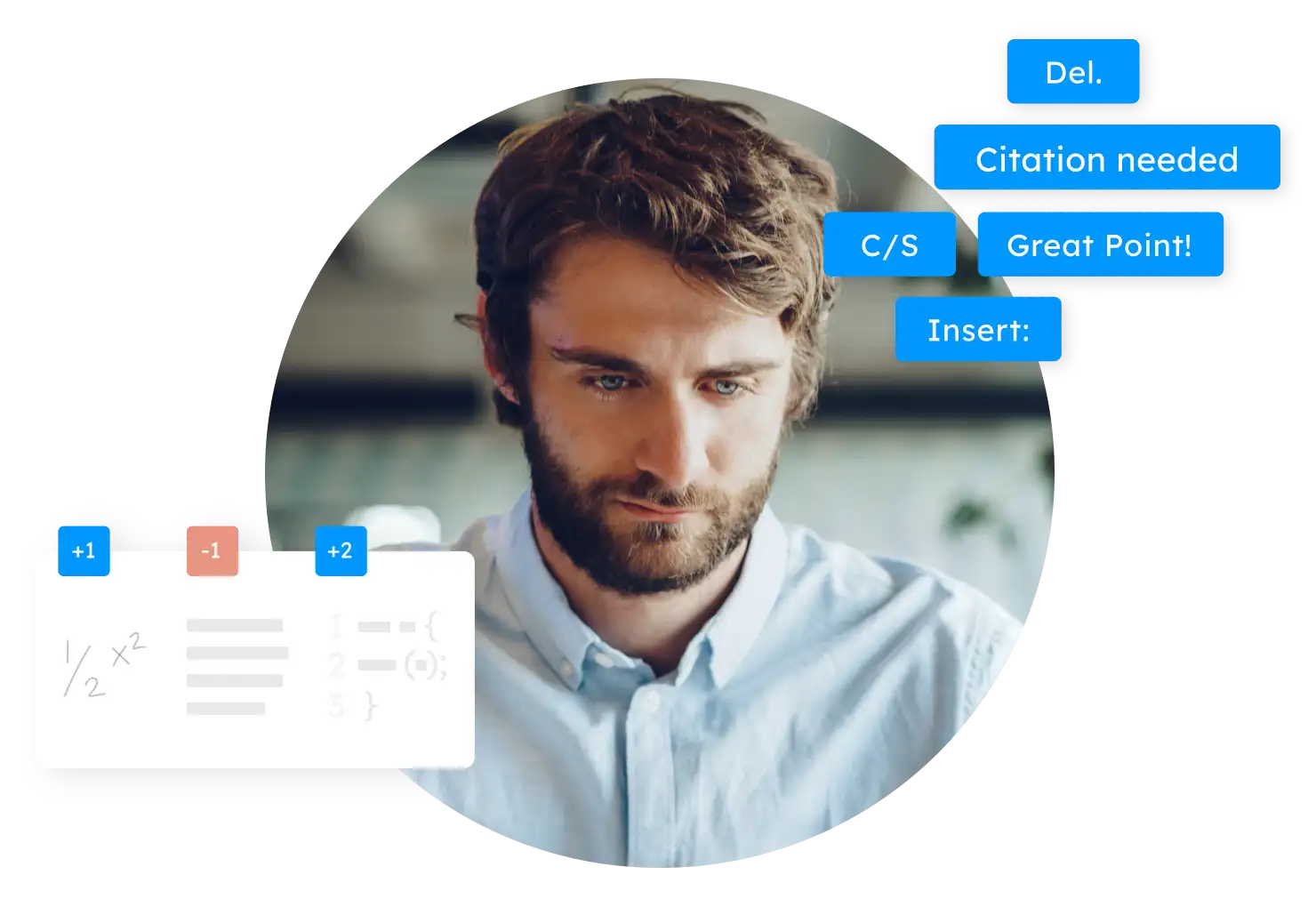
Foster original thinking
Help develop students’ original thinking skills with high-quality, actionable feedback that fits easily into teachers’ existing workflows.
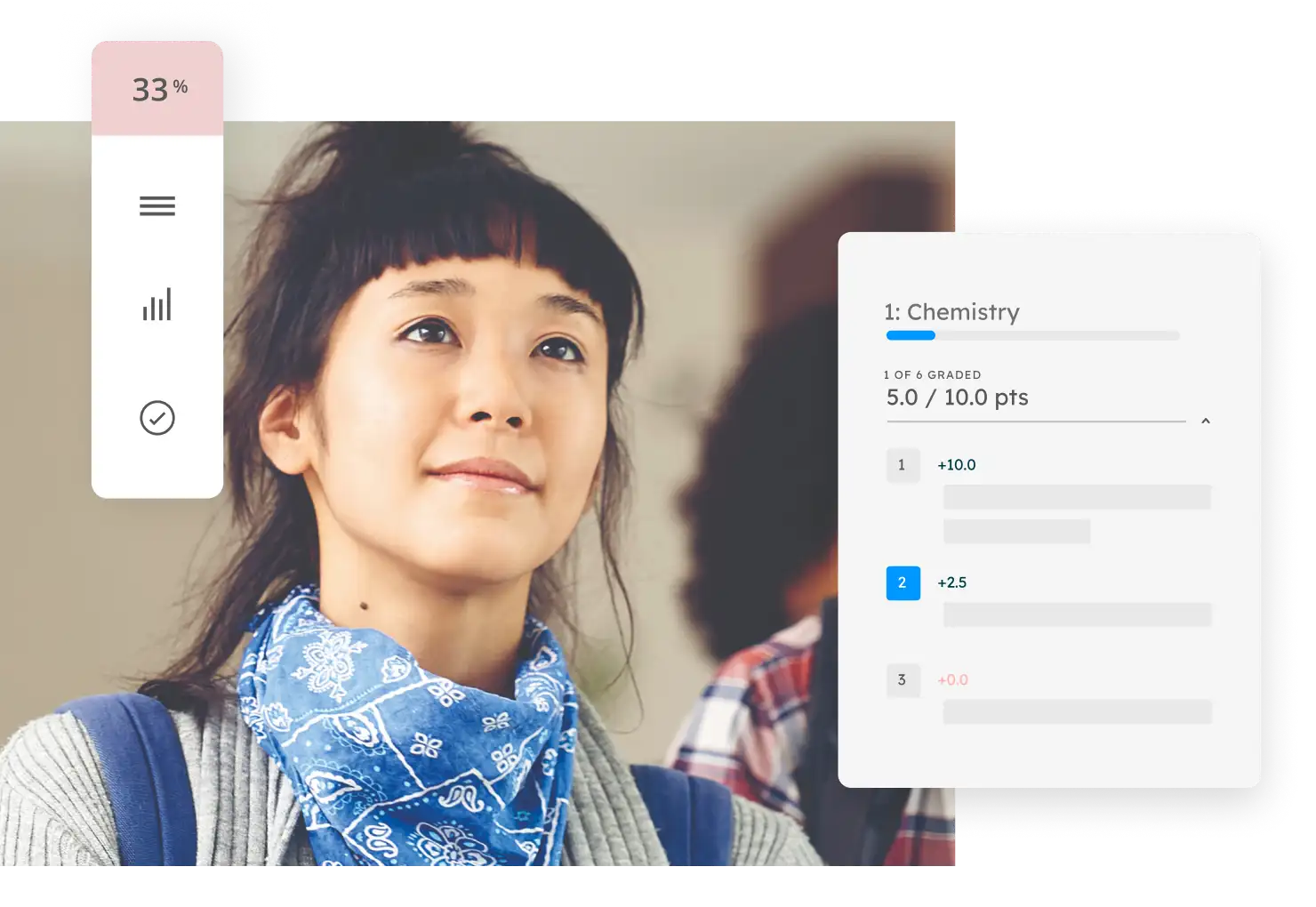
Customer stories
University of leeds widely adopts gradescope for online assessment.
With campuswide digital transformation in mind, rollout to thousands of faculty across disciplines took only two months, far quicker than anticipated.
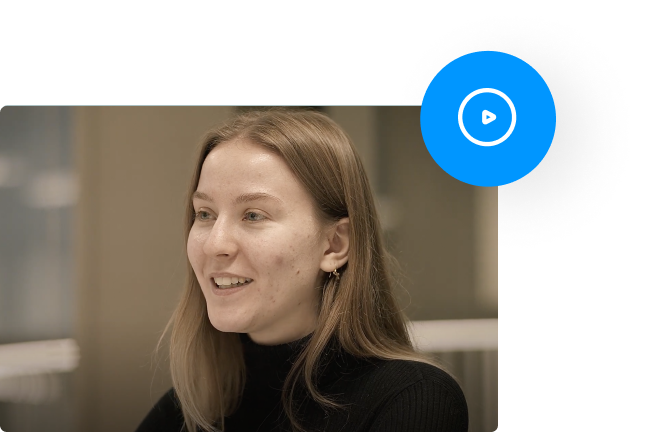
University of Maryland Global Campus builds a culture of academic integrity by pairing policy with technology
Keeping student and instructor needs at the core is key to upholding their rigorous standards of integrity while centering students in their learning.
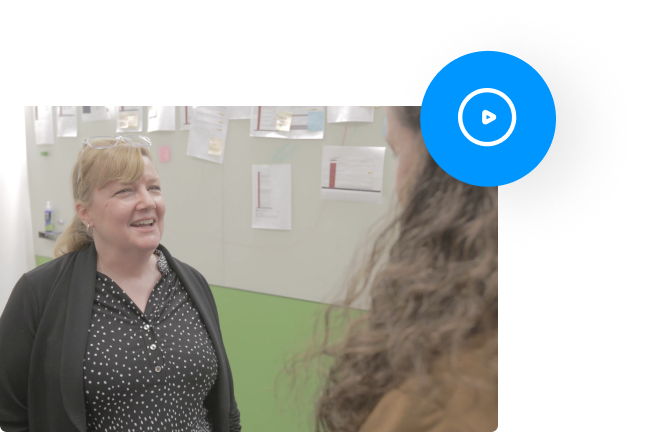
Solutions made just for you
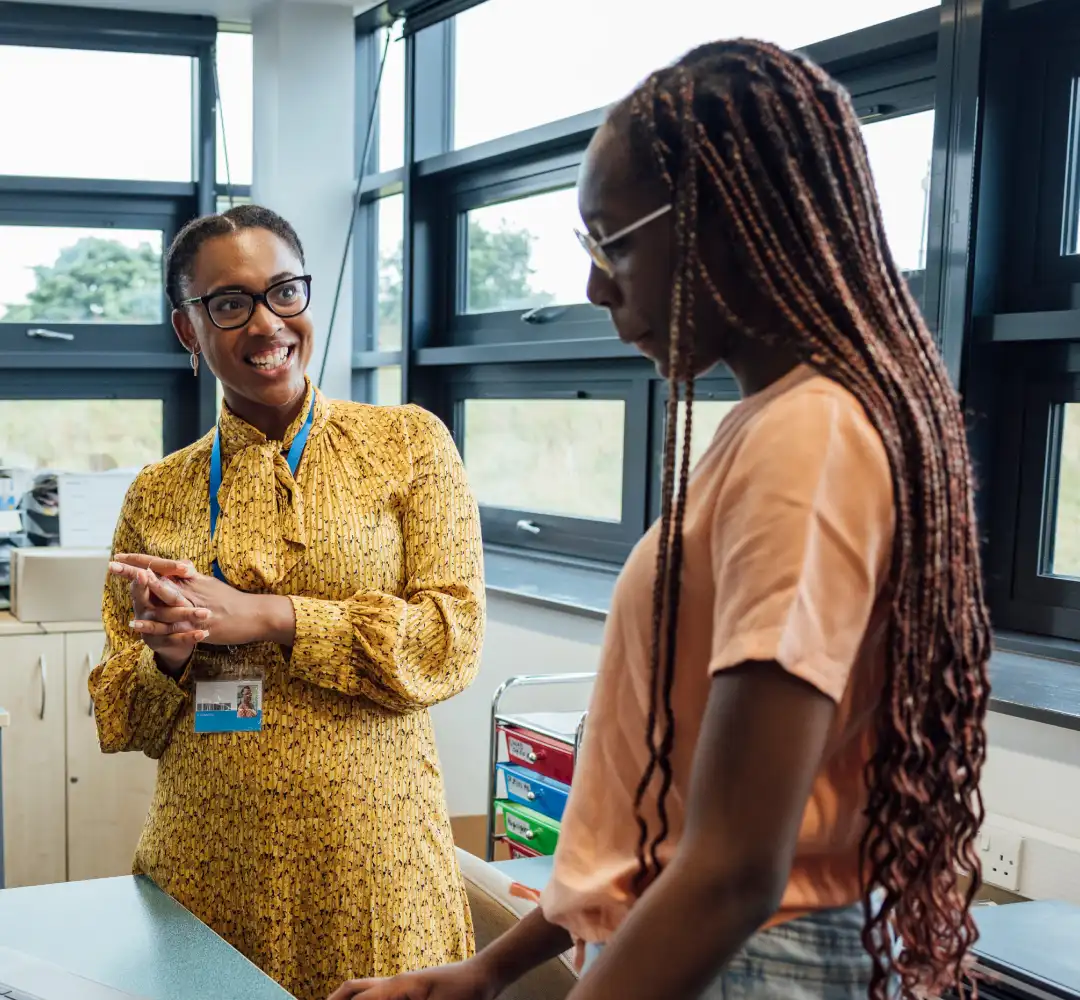
See solutions for Higher Education
Prepare students for success with tools designed to uphold academic integrity and advance assessment.

See solutions for Secondary Education
Help students develop original thinking skills with tools that improve their writing and check for similarities to existing works.

See solutions for Research and Publication
The rigorous academic research and publishing process gets a trusted tool to ensure the originality of scholarly work.
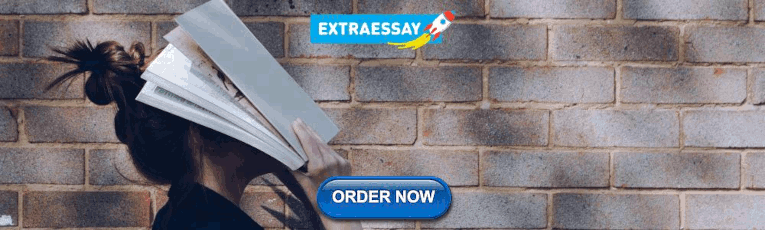
Keep integrity at the core of every assessment.
Everything you need no matter how or where you assess student work.
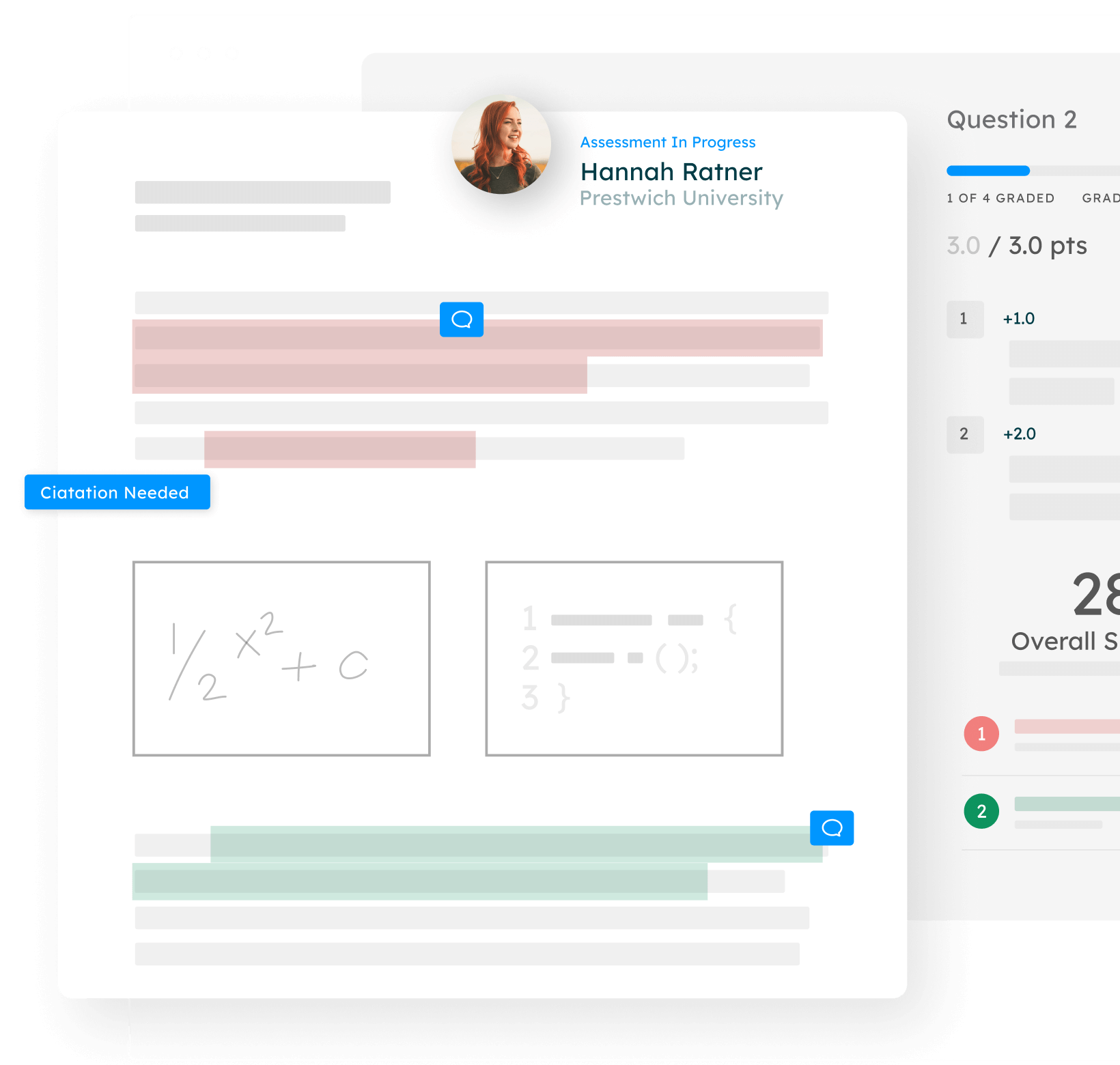
Feedback Studio
Give feedback and grade essays and long-form writing assignments with the tool that fosters writing excellence and academic integrity.
Originality
Check for existing as well as new forms of plagiarism - from text similarity and synonym swapping to contract cheating, AI writing, and AI paraphrasing.
Grade paper-based, bubble sheet, and code assignments faster than ever while giving more robust feedback and gaining valuable insights.
Address potential gaps in curriculum and assessment to prepare students for their careers and meet accreditation requirements.
This robust, comprehensive plagiarism checker fits seamlessly into existing workflows.
iThenticate
Screen personal statements for potential plagiarism and ensure the highest level of integrity before matriculation.
The Turnitin difference
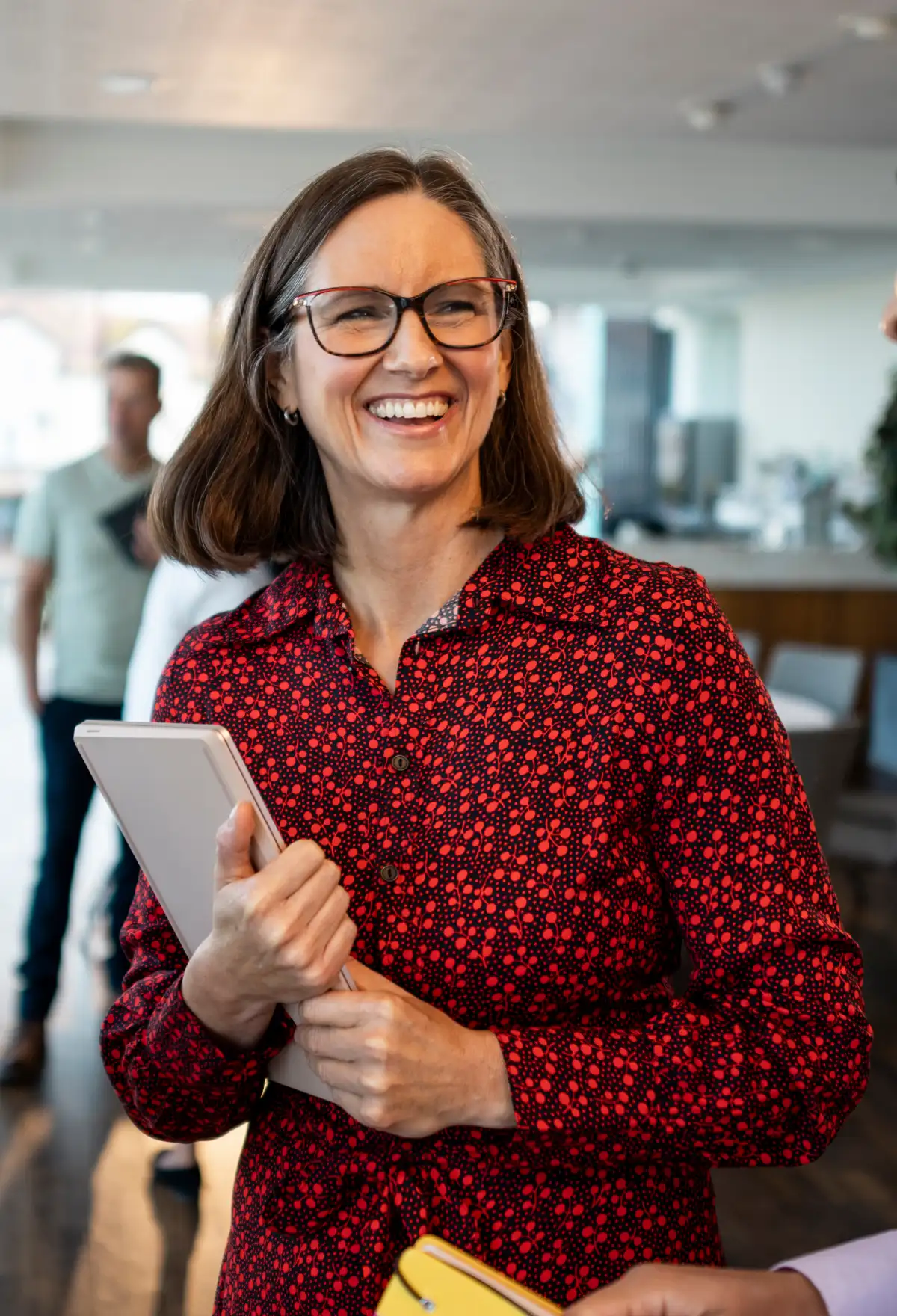
Complete Coverage
Your coverage is comprehensive, with the largest content database of scholarly content, student papers, and webpages.
Extensive Support
Have the help you need with 24/7 tech support, curricular resources created by real educators, and customized training and onboarding services.
Human-Centered AI
Our people-centered approach to artificial intelligence improves academic integrity and makes assessment better for all.
Unparalleled Access
Access Turnitin tools at scale through integrations with over 100 systems in the educational ecosystem.
Further Reading
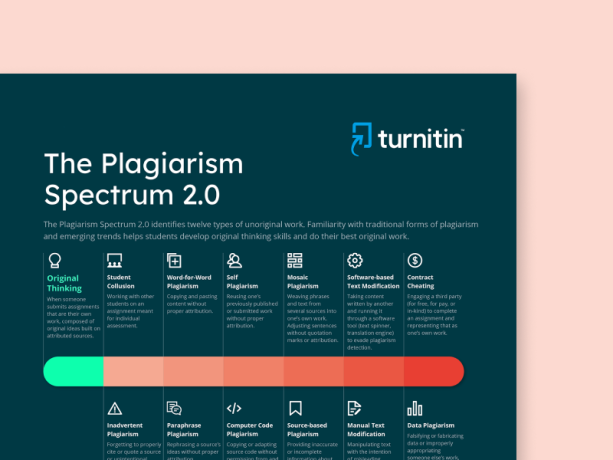
The Plagiarism Spectrum 2.0 identifies twelve types of unoriginal work — both traditional forms of plagiarism and emerging trends. Understanding these forms of plagiarism supports the development of original thinking skills and helps students do their best, original work.
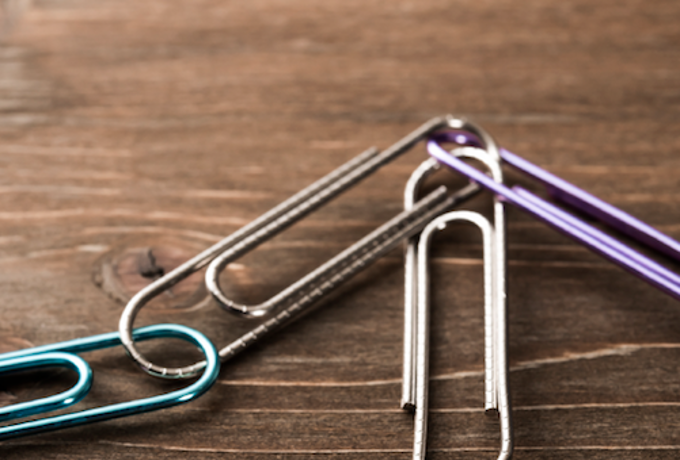
An educator guide providing suggestions for how to adapt instruction when faced with student use of AI.
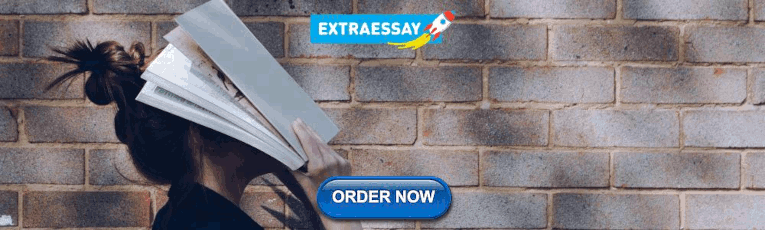
IMAGES
VIDEO
COMMENTS
Detect plagiarism using software similar to what most universities and publishers use. Spot missing citations and improperly quoted or paraphrased content. Avoid grade …
Protect your institution's academic standards with Turnitin's plagiarism detector. Identify copied content and ensure originality in every submission.
The EasyBib plagiarism checker is conveniently available 24 hours a day and seven days a week. You can cancel anytime. Check your paper for free today!. *See Terms and Conditions. Visit www.easybib.com for more information on …
Typos and grammar errors can slip into anyone’s work. ProWritingAid’s AI-powered thesis checker can catch and fix even the trickiest issues so your ideas can shine.
Avoid accidental or self-plagiarism with Paperpal’s free plagiarism checker, in partnership with Turnitin. Get a similarity score, side-by-side comparisons, and color-coded results with the full report. Instant comparison against billions of …
PaperRater proofreads and rates your essays & papers. It picks out grammar & spelling errors, detects plagiarism and grades your writing. It includes resources on grammar, writing, spelling & more. ... login, or download. You won't find …
Instant plagiarism check for essays and documents. Detect plagiarism, fix grammar errors, and improve your vocabulary in seconds.
Your plagiarism report within 10 minutes. How it works. 1. Upload. Upload a Microsoft Word, PDF or ODT file of your paper, enter your details and pay. 2. Wait a few minutes. Your document is compared to billions of web pages, books, …