Purdue Online Writing Lab Purdue OWL® College of Liberal Arts
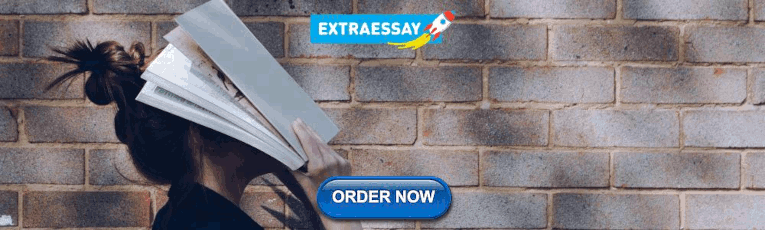
Thesis and Dissertation: Getting Started
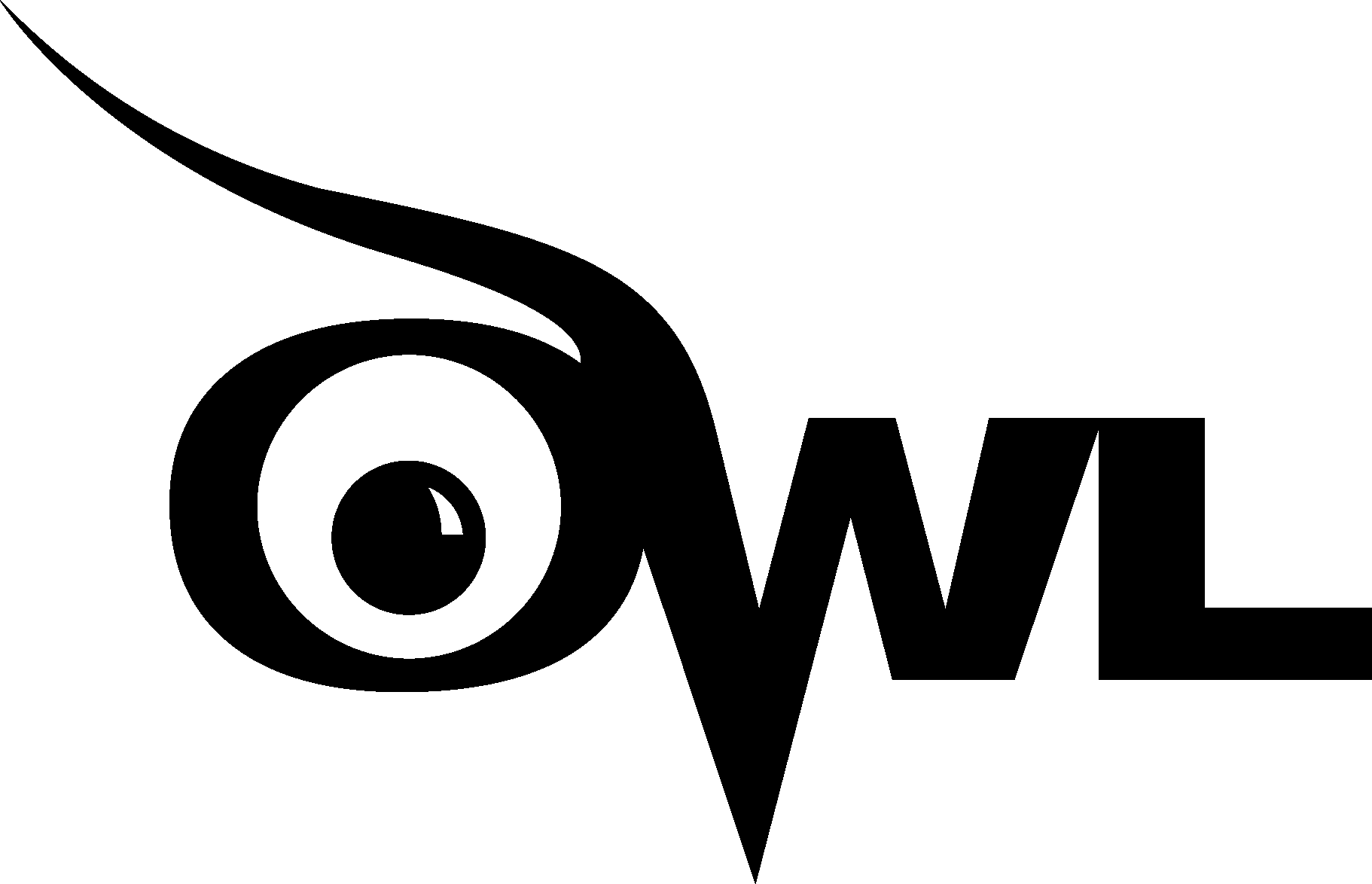
Welcome to the Purdue OWL
This page is brought to you by the OWL at Purdue University. When printing this page, you must include the entire legal notice.
Copyright ©1995-2018 by The Writing Lab & The OWL at Purdue and Purdue University. All rights reserved. This material may not be published, reproduced, broadcast, rewritten, or redistributed without permission. Use of this site constitutes acceptance of our terms and conditions of fair use.
The resources in this section are designed to provide guidance for the first steps of the thesis or dissertation writing process. They offer tools to support the planning and managing of your project, including writing out your weekly schedule, outlining your goals, and organzing the various working elements of your project.
Weekly Goals Sheet (a.k.a. Life Map) [Word Doc]
This editable handout provides a place for you to fill in available time blocks on a weekly chart that will help you visualize the amount of time you have available to write. By using this chart, you will be able to work your writing goals into your schedule and put these goals into perspective with your day-to-day plans and responsibilities each week. This handout also contains a formula to help you determine the minimum number of pages you would need to write per day in order to complete your writing on time.
Setting a Production Schedule (Word Doc)
This editable handout can help you make sense of the various steps involved in the production of your thesis or dissertation and determine how long each step might take. A large part of this process involves (1) seeking out the most accurate and up-to-date information regarding specific document formatting requirements, (2) understanding research protocol limitations, (3) making note of deadlines, and (4) understanding your personal writing habits.
Creating a Roadmap (PDF)
Part of organizing your writing involves having a clear sense of how the different working parts relate to one another. Creating a roadmap for your dissertation early on can help you determine what the final document will include and how all the pieces are connected. This resource offers guidance on several approaches to creating a roadmap, including creating lists, maps, nut-shells, visuals, and different methods for outlining. It is important to remember that you can create more than one roadmap (or more than one type of roadmap) depending on how the different approaches discussed here meet your needs.
The Thesis Process
The thesis is an opportunity to work independently on a research project of your own design and contribute to the scholarly literature in your field. You emerge from the thesis process with a solid understanding of how original research is executed and how to best communicate research results. Many students have gone on to publish their research in academic or professional journals.
To ensure affordability, the per-credit tuition rate for the 8-credit thesis is the same as our regular course tuition. There are no additional fees (regular per-credit graduate tuition x 8 credits).
Below are the steps that you need to follow to fulfill the thesis requirement. Please know that through each step, you will receive guidance and mentorship.
1. Determine Your Thesis Topic and Tentative Question
When you have completed between 24 and 32 credits, you work with your assigned research advisor to narrow down your academic interests to a relevant and manageable thesis topic. Log in to MyDCE , then ALB/ALM Community to schedule an appointment with your assigned research advisor via the Degree Candidate Portal.
Thesis Topic Selection
We’ve put together this guide to help frame your thinking about thesis topic selection.
Every effort is made to support your research interests that are grounded in your ALM course work, but faculty guidance is not available for all possible projects. Therefore, revision or a change of thesis topic may be necessary.
- The point about topic selection is particularly pertinent to scientific research that is dependent upon laboratory space, project funding, and access to private databases. It is also critical for our candidates in ALM, liberal arts fields (English, government, history, international relations, psychology, etc.) who are required to have Harvard faculty direct their thesis projects. Review Harvard’s course catalog online ( my.harvard.edu ) to be sure that there are faculty teaching courses related to your thesis topic. If not, you’ll need to choose an alternative topic.
- Your topic choice must be a new area of research for you. Thesis work represents thoughtful engagement in new academic scholarship. You cannot re-purpose prior research. If you want to draw or expand upon your own previous scholarship for a small portion of your thesis, you need to obtain the explicit permission of your research advisor and cite the work in both the proposal and thesis. Violations of this policy will be referred to the Administrative Board.
2. Prepare Prework for the Crafting the Thesis Proposal (CTP) Course or Tutorial
The next step in the process is to prepare and submit Prework in order to gain registration approval for the Crafting the Thesis Proposal (CTP) tutorial or course. The Prework process ensures that you have done enough prior reading and thinking about your thesis topic to benefit from the CTP.
The CTP provides an essential onramp to the thesis, mapping critical issues of research design, such as scope, relevance to the field, prior scholarly debate, methodology, and perhaps, metrics for evaluating impact as well as bench-marking. The CTP identifies and works through potential hurdles to successful thesis completion, allowing the thesis project to get off to a good start.
In addition to preparing, submitting, and having your Prework approved, to be eligible for the CTP, you need to be in good standing, have completed a minimum of 32 degree-applicable credits, including the statistics/research methods requirement (if pertinent to your field). You also need to have completed Engaging in Scholarly Conversation (if pertinent to your field). If you were admitted after 9/1/2023 Engaging in Scholarly Conversation (A and B) is required, if admitted before 9/1/2023 this series is encouraged.
Advising Note for Biology, Biotechnology, and Bioengineering and Nanotechnology Candidates : Thesis projects in these fields are designed to support ongoing scientific research happening in Harvard University, other academic institutions, or life science industry labs and usually these are done under the direction of a principal investigator (PI). Hence, you need to have a thesis director approved by your research advisor prior to submitting CTP prework. Your CTP prework is then framed by the lab’s research. Schedule an appointment with your research advisor a few months in advance of the CTP prework deadlines in order to discuss potential research projects and thesis director assignment.
CTP Prework is sent to our central email box: [email protected] between the following firm deadlines:
- April 1 and June 1 for fall CTP
- September 1 and November 1 for spring CTP.
- August 1 and October 1 for the three-week January session (ALM sustainability candidates only)
- International students who need a student visa to attend Harvard Summer School should submit their prework on January 1, so they can register for the CTP on March 1 and submit timely I-20 paperwork. See international students guidelines for more information.
Your research advisor will provide feedback on your prework submission to gain CTP registration approval. If your prework is not approved after 3 submissions, your research advisor cannot approve your CTP registration. If not approved, you’ll need to take additional time for further revisions, and submit new prework during the next CTP prework submission time period for the following term (if your five-year degree completion deadline allows).
3. Register and Successfully Complete the Crafting the Thesis Proposal Tutorial or Course
Once CTP prework is approved, you register for the Crafting the Thesis Proposal (CTP) course or tutorial as you would any other course. The goal of the CTP is to produce a complete, well-written draft of a proposal containing all of the sections required by your research advisor. Creating an academically strong thesis proposal sets the foundation for a high-quality thesis and helps garner the attention of a well-respected thesis director. The proposal is normally between 15 to 25 pages in length.
The CTP tutorial is not a course in the traditional sense. You work independently on your proposal with your research advisor by submitting multiple proposal drafts and scheduling individual appointments. You need to make self-directed progress on the proposal without special prompting from the research advisor. You receive a final grade of SAT or UNSAT (failing grade).
The CTP for sustainability is a three-week course in the traditional sense and you receive a letter grade, and it must be B- or higher to receive degree credit for the course.
You are expected to incorporate all of your research advisor’s feedback and be fully committed to producing an academically strong proposal leading to a thesis worthy of a Harvard degree. If you are unable to take advice from your research advisor, follow directions, or produce an acceptable proposal, you will not pass the CTP.
Successful CTP completion also includes a check on the proper use of sources according to our academic integrity guidelines. Violations of our academic integrity policy will be referred to the Administrative Board.
Maximum of two attempts . If you don’t pass that CTP, you’ll have — if your five-year, degree-completion date allows — just one more attempt to complete the CTP before being required to withdraw from the program. If you fail the CTP just once and have no more time to complete the degree, your candidacy will automatically expire. Please note that a WD grade counts as an attempt.
If by not passing the CTP you fall into poor academic standing, you will need to take additional degree-applicable courses to return to good standing before enrolling in the CTP for your second and final time, only if your five-year, degree-completion date allows. If you have no more time on your five-year clock, you will be required to withdraw.
Human Subjects
If your thesis, regardless of field, will involve the use of human subjects (e.g., interviews, surveys, observations), you will need to have your research vetted by the Committee on the Use of Human Subjects (CUHS) of Harvard University. Please review the IRB LIFECYCLE GUIDE located on the CUHS website. Your research advisor will help you prepare a draft copy of the project protocol form that you will need to send to CUHS. The vetting process needs to be started during the CTP tutorial, before a thesis director has been assigned.
4. Thesis Director Assignment and Thesis Registration
We expect you to be registered in thesis soon after CTP completion or within 3 months — no later. You cannot delay. It is critical that once a research project has been approved through the CTP process, the project must commence in a timely fashion to ensure the academic integrity of the thesis process.
Once you (1) successfully complete the CTP and (2) have your proposal officially approved by your research advisor (RA), you move to the thesis director assignment phase. Successful completion of the CTP is not the same as having an officially approved proposal. These are two distinct steps.
If you are a life science student (e.g., biology), your thesis director was identified prior to the CTP, and now you need the thesis director to approve the proposal.
The research advisor places you with a thesis director. Do not approach faculty to ask about directing your thesis. You may suggest names of any potential thesis directors to your research advisor, who will contact them, if they are eligible/available to direct your thesis, after you have an approved thesis proposal.
When a thesis director has been identified or the thesis proposal has been fully vetted by the preassigned life science thesis director, you will receive a letter of authorization from the Assistant Dean of Academic Programs officially approving your thesis work and providing you with instructions on how to register for the eight-credit Master’s Thesis. The letter will also have a tentative graduation date as well as four mandatory thesis submission dates (see Thesis Timetable below).
Continuous Registration Tip: If you want to maintain continued registration from CTP to thesis, you should meet with your RA prior to prework to settle on a workable topic, submit well-documented prework, work diligently throughout the CTP to produce a high-quality proposal that is ready to be matched with a thesis director as soon as the CTP is complete.
Good academic standing. You must be good academic standing to register for the thesis. If not, you’ll need to complete additional courses to bring your GPA up to the 3.0 minimum prior to registration.
Thesis Timetable
The thesis is a 9 to 12 month project that begins after the Crafting the Thesis Proposal (CTP); when your research advisor has approved your proposal and identified a Thesis Director.
The date for the appointment of your Thesis Director determines the graduation cycle that will be automatically assigned to you:
Once registered in the thesis, we will do a 3-month check-in with you and your thesis director to ensure progress is being made. If your thesis director reports little to no progress, the Dean of Academic Programs reserves the right to issue a thesis not complete (TNC) grade (see Thesis Grading below).
As you can see above, you do not submit your thesis all at once at the end, but in four phases: (1) complete draft to TA, (2) final draft to RA for format review and academic integrity check, (3) format approved draft submitted to TA for grading, and (4) upload your 100% complete graded thesis to ETDs.
Due dates for all phases for your assigned graduation cycle cannot be missed. You must submit materials by the date indicated by 5 PM EST (even if the date falls on a weekend). If you are late, you will not be able to graduate during your assigned cycle.
If you need additional time to complete your thesis after the date it is due to the Thesis Director (phase 1), you need to formally request an extension (which needs to be approved by your Director) by emailing that petition to: [email protected] . The maximum allotted time to write your thesis, including any granted extensions of time is 12 months.
Timing Tip: If you want to graduate in May, you should complete the CTP in the fall term two years prior or, if a sustainability student, in the January session one year prior. For example, to graduate in May 2025:
- Complete the CTP in fall 2023 (or in January 2024, if a sustainability student)
- Be assigned a thesis director (TD) in March/April 2024
- Begin the 9-12 month thesis project with TD
- Submit a complete draft of your thesis to your TD by February 1, 2025
- Follow through with all other submission deadlines (April 1, April 15 and May 1 — see table above)
- Graduate in May 2025
5. Conduct Thesis Research
When registered in the thesis, you work diligently and independently, following the advice of your thesis director, in a consistent, regular manner equivalent to full-time academic work to complete the research by your required timeline.
You are required to produce at least 50 pages of text (not including front matter and appendices). Chapter topics (e.g., introduction, background, methods, findings, conclusion) vary by field.
6. Format Review — Required of all Harvard Graduate Students and Part of Your Graduation Requirements
All ALM thesis projects must written in Microsoft Word and follow a specific Harvard University format. A properly formatted thesis is an explicit degree requirement; you cannot graduate without it.
Your research advisor will complete the format review prior to submitting your thesis to your director for final grading according to the Thesis Timetable (see above).
You must use our Microsoft Word ALM Thesis Template or Microsoft ALM Thesis Template Creative Writing (just for creative writing degree candidates). It has all the mandatory thesis formatting built in. Besides saving you a considerable amount of time as you write your thesis, the preprogrammed form ensures that your submitted thesis meets the mandatory style guidelines for margins, font, title page, table of contents, and chapter headings. If you use the template, format review should go smoothly, if not, a delayed graduation is highly likely.
Format review also includes a check on the proper use of sources according to our academic integrity guidelines. Violations of our academic integrity policy will be referred directly to the Administrative Board.
7. Mandatory Thesis Archiving — Required of all Harvard Graduate Students and Part of Your Graduation Requirements
Once your thesis is finalized, meaning that the required grade has been earned and all edits have been completed, you must upload your thesis to Harvard University’s electronic thesis and dissertation submission system (ETDs). Uploading your thesis ETDs is an explicit degree requirement; you cannot graduate without completing this step.
The thesis project will be sent to several downstream systems:
- Your work will be preserved using Harvard’s digital repository DASH (Digital Access to Scholarship at Harvard).
- Metadata about your work will be sent to HOLLIS (the Harvard Library catalog).
- Your work will be preserved in Harvard Library’s DRS2 (digital preservation repository).
By submitting work through ETDs @ Harvard you will be signing the Harvard Author Agreement. This license does not constrain your rights to publish your work subsequently. You retain all intellectual property rights.
For more information on Harvard’s open access initiatives, we recommend you view the Director of the Office of Scholarly Communication (OSC), Peter Suber’s brief introduction .
Thesis Grading
You need to earn a grade of B- or higher in the thesis. All standard course letter grades are available to your thesis director. If you fail to complete substantial work on the thesis, you will earn a grade of TNC (thesis not complete). If you have already earned two withdrawal grades, the TNC grade will count as a zero in your cumulative GPA.
If you earn a grade below B-, you will need to petition the Administrative Board for permission to attempt the thesis for a second and final time. The petition process is only available if you are in good academic standing and your five-year, degree-completion deadline allows for more time. Your candidacy will automatically expire if you do not successfully complete the thesis by your required deadline.
If approved for a second attempt, you may be required to develop a new proposal on a different topic by re-enrolling in the CTP and being assigned a different thesis director. Tuition for the second attempt is calculated at the current year’s rate.
If by not passing the thesis you fall into poor academic standing, you’ll need to take additional degree-applicable courses to return to good standing before re-engaging with the thesis process for the second and final time. This is only an option if your five-year, degree-completion deadline allows for more time.
The Board only reviews cases in which extenuating circumstances prevented the successful completion of the thesis.
Harvard Division of Continuing Education
The Division of Continuing Education (DCE) at Harvard University is dedicated to bringing rigorous academics and innovative teaching capabilities to those seeking to improve their lives through education. We make Harvard education accessible to lifelong learners from high school to retirement.
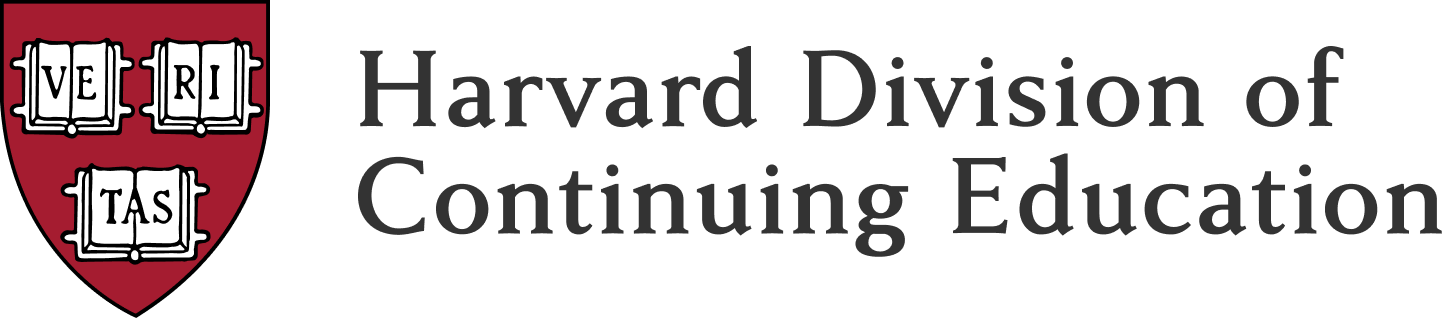
Advertisement
Designing and developing smart production planning and control systems in the industry 4.0 era: a methodology and case study
- Open access
- Published: 04 July 2021
- Volume 33 , pages 311–332, ( 2022 )
Cite this article
You have full access to this open access article
- Olumide Emmanuel Oluyisola ORCID: orcid.org/0000-0001-7944-3776 1 ,
- Swapnil Bhalla ORCID: orcid.org/0000-0003-4905-2488 1 ,
- Fabio Sgarbossa ORCID: orcid.org/0000-0002-9541-3515 1 &
- Jan Ola Strandhagen ORCID: orcid.org/0000-0003-3741-9000 1
23k Accesses
64 Citations
1 Altmetric
Explore all metrics
In furtherance of emerging research within smart production planning and control (PPC), this paper prescribes a methodology for the design and development of a smart PPC system. A smart PPC system uses emerging technologies such as the internet of things, big-data analytics tools and machine learning running on the cloud or on edge devices to enhance performance of PPC processes. It achieves this by using a wider range of data sources from the production system, capturing and utilizing the experience of production planners, using analytics and machine learning to harness insights from the data and allowing dynamic and near real-time action to the continuously changing production system. The proposed methodology is illustrated with a case study in a sweets and snacks manufacturing company, to highlight the key considerations and challenges production managers might face during its application. The case further demonstrates considerations for scalability and flexibility via a loosely coupled, service-oriented architecture and the selection of fitting algorithms respectively to address a business requirement for a short-term, multi-criteria and event-driven production planning and control solution. Finally, the paper further discusses the challenges of PPC in smart manufacturing and the importance of fitting smart technologies to planning environment characteristics.
Similar content being viewed by others
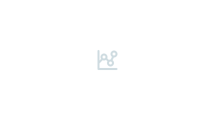
Smart manufacturing systems for Industry 4.0: Conceptual framework, scenarios, and future perspectives
Pai Zheng, Honghui wang, … Xun Xu
Smart Production Planning and Control Model
Manufacturing Operations Management for Smart Manufacturing – A Case Study
Avoid common mistakes on your manuscript.
Introduction
Production planning and control (PPC) refers to the activities of loading, scheduling, sequencing, monitoring, and controlling the use of resources and materials during production. Loading concerns how much to do; scheduling concerns when to do things; sequencing concerns in what order to do things; and monitoring and control is concerned with whether activities are going to plan, and corrective actions needed to bring activities within plan (Slack et al., 2013 ). Commonly, these activities of PPC are carried out and coordinated using enterprise resource planning (ERP) systems (Arnold et al., 2011 ) and spreadsheet solutions (de Man & Strandhagen, 2018 ). However, ERP systems are typically unwieldy and do not support real-time decision-making that today’s market environments demand. Furthermore, manufacturing execution systems (MES) and advance planning and scheduling (APS) systems have also been developed in the last two decades to address some of these weaknesses of ERP systems (Öztürk & Ornek, 2014 ). While APS systems have been associated with various potential benefits, including support for real-time decision-making, the challenges associated with their implementation and integration with ERP systems render these benefits far from achievable in practice (Lupeikiene et al., 2014 ).
Currently, the business environment is typified by increasing market and supply chain complexity, globalization and global competition, waves of protectionism, and customer expectations of more sophisticated products. From time immemorial, notable studies have highlighted the aforementioned challenges as the impetus for increased efficiency in production systems and have devised strategies that could be used to achieve this aim – including those that involve the use of information technology (IT) and lean manufacturing techniques (Chan, 2005 ; Hong et al., 2010 ; Skinner, 1974 ). Additionally, production systems are generating increasingly large volumes of data and the potential for enhancement of planning systems to use this data for performance improvements has been widely promoted in industry and academia, but with limited adoption (Chavez et al., 2017 ; Fatorachian & Kazemi, 2020 ; Nagy et al., 2018 ). This brings one to the concept of smart manufacturing or Industry 4.0, which presents a new frontier for the advancement of manufacturing planning and control for its potential realization, spurred by the concurrent maturation of emerging ‘smart’ technologies such as cloud computing, internet of things (IoT), big-data analytics (BDA) and machine learning (ML) for improving the PPC system and processes (Bueno et al., 2020 ; Cadavid et al., 2020 ; Oluyisola et al., 2020 ).
For smart manufacturing (and the associated terms of industry 4.0), while many authors have addressed the potential impact, the expectations, industry implementation experiences, strategies for adoption, there is currently no clear methodological guide towards the design and development of a smart PPC system – the valley that separates conceptual literature from implementation reality. There are gaps, of architectural designs and concepts, and more importantly about how to translate the system requirements and attributes to the lower level elements – of data structures, of class definitions, of entity-relationship diagrams, of matching appropriate algorithms, etc . – in a way that supports the development of smart PPC systems that fit the near- and long- term requirements of a production system. This is particularly important for smaller companies who have more restrictive research and development budgets, and now for big industry leading companies at times of global economic crises. In this regard, Kusiak ( 2017 ) notes that:
“Academics push technological frontiers, from artificial intelligence to deep learning, without considering how they will be applied. Manufacturers want to know what types of data to sample, which sensors to use and where along the production line to install them.”
Smart PPC focuses on the ‘brain’ of manufacturing operations and aims to intelligently plan and control current industrial assets and materials as well as future, more adaptive production systems. A Smart PPC system should employ emerging technologies to: enable the reduction of forecast uncertainty by using real-time demand and production system data; offer dynamic re-planning in the sense that it enables frequently updating and the ability to re-plan when there are new developments in the production system; capture the influence of an expanded set of factors including telemetry factors especially for the process and semi-process industries; to capture the experience of the operators or the production planners over time; and predict short-term system parameter values and enable increase agility (Bueno et al., 2020 ; Oluyisola et al., 2020 ; Strandhagen et al., 2017 ).
Currently, there is no systematic, holistic design and development guide for the design and development of a smart PPC system. This paper presents an attempt to address these gaps by discussing the design principles for smart PPC solutions and demonstrating (with a case illustration from a semi-process industry) the use of a 5-step methodology for designing and developing smart PPC solutions. Note that in this paper, design refers to the architectural design rather than a user-interface of graphical design. The presented method details how to capture a production system’s attributes into the design and development process. Consequently, the following research question motivates this study:
How should a smart PPC system be designed and developed so that it is fitting with the current characteristics and the future requirements of the production system?
A design science research approach was used to address this research question. Design science, as an active problem solving research method, is useful when researchers aim to develop an artefact (Holmström et al., 2009 ). The case study used for illustrating the artefact – the methodology – was selected because it offers a production environment amenable to a smart process strategy where it is easier to demonstrate the benefits of smart PPC (Oluyisola et al., 2020 ; Tenhiälä, 2011 ). The proposed smart PPC design and development methodology is developed from a combination of extant primary and secondary literature and thereafter illustrated by the case study. While the methodology is designed to be generic, the illustration highlights the importance of case context during application. Furthermore, while an attempt has been made at providing details for the implementation, the complete technical implementation tools are not within the scope of this paper. This is because the technology stack or platform each company chooses could differ based on company policy and currently held expertise within the company and thus excessive implementation details will be of little cross platform value. Therefore, this study emphasizes only those details that present the elements which any sufficiently trained IT person can understand and use in designing a smart PPC solution for their case.
The remaining sections of the paper are structured as follows. In Sect. 2 , a theoretical background is presented which covers PPC, emerging technologies applications for PPC processes, design considerations for complex information systems and data and systems’ architecture requirements. In Sect. 3 , a derived method is presented. In Sect. 4 , the method is illustrated in a case to show its strengths and weaknesses. In Sect. 5 , the implications of the proposed methodology for the future development of smart PPC within both research and practice are discussed. In the final section, conclusions, limitations, and further research ideas are presented.
Theoretical background
In this section, the practice and the fundamental challenges in current applications of PPC and the limitations of the enterprise planning systems to address most of the requirements of smart PPC are outlined. Thereafter, the uses of smart, emerging technologies within the PPC domain are evaluated, followed by design and development considerations.
Production planning and control theory and the limitations of classical PPC systems
Fundamentally, PPC is tasked with the problem of managing uncertainty in production systems, either through stabilizing the system (common with lean approaches) or through predicting and reacting effectively and speedily to events and changes in state of the production system. The latter requires few or frequent rescheduling depending on the kind of operation and the stability of the production environment (Vieira et al., 2003 ). In achieving these goals, various process logics and methods have been developed at different levels of detail and time (hierarchical systems) and at different domains, for example, algorithmic research, strategic selection of PPC systems and implementation challenges and limitations. This diversity of topics and issues have led to different streams of research.
One stream of research has focused on investigating the effectiveness of enterprise resource planning (ERP) systems for PPC in different industrial environments, e.g., in dynamic market environments (Tenhiälä & Helkiö, 2015 ), in make-to-order manufacturing environments (Aslan et al., 2012 , 2015 ), in small and medium enterprises (Ahmad & Cuenca, 2013 ), etc. The research within this stream has often been triggered by perceived limitations and inadequacies of ERP systems in supporting manufacturing planning and control activities. The most frequently mentioned limitation of ERP systems is generating unrealistic or infeasible production schedules due to infinite capacity scheduling (Arica & Powell, 2014 ; Steger-Jensen et al., 2011 ). Meanwhile, these limitations of ERP systems have paved way for the second research stream, which concerns auxiliary planning and control systems such as MES and APS. Consequently, the infeasibility of production schedules generated by ERP systems and the inability to tightly control operations have led to some large manufacturers using APS systems for planning and MES for production control respectively (Saenz de Ugarte et al., 2009 ; Steger-Jensen et al., 2011 ).
While MES and APS systems can address some limitations of ERP systems, these planning and control systems are known to have their own limitations. The processes within these systems have remained simplistic or too rigid, which limits the factors that can be considered within production planning and control decisions. Adjustment to schedules based on real-time or near-real-time data is infeasible and commonly avoided by production planners. It is also expensive to integrate additional software (called ‘add-ons’) with the large, monolithic systems, often making it difficult to adapt to changing business needs and leading many manufacturing managers and planners to build simpler, easier to manage, but disparate tools outside their PPC systems (Carvalho et al., 2014 ; Shaikh et al., 2011 ). Consequently, another stream of research has looked at the development of complementary decision support systems for addressing some of the challenges being faced by companies implementing ERP, APS and MES systems. Indeed, it is commonly reported that planners and supervisors, in many instances, tend to prefer simpler and more flexible tools and are more likely to avoid more complex, albeit theoretically performance-improved methods for addressing many of the production planning and control needs (de Man & Strandhagen, 2018 ; Tenhiälä, 2011 ). Therefore, while enterprise planning systems inhibited high efficiency for PPC processes by being unwieldy and not including additional real-time system data, flexible approaches have been limited in that they are often very manual, dependent on the availability of specific people and also not holistic (Oluyisola et al., 2020 ).
Emerging uses of smart technologies for smarter PPC
The adoption of smart technologies has seen tremendous increase in recent decades due to increased availability and affordability of computing power (Guha & Kumar, 2018 ). Generally, there are two ways in which companies adopt a technology: either a company (or its leadership) is pushed by its industry peers in the form of a market trend, or a business need leads to a search for a technology solution (Beckman & Rosenfield, 2008 ). In either case, the technology’s potential value is fully harnessed only when there is a fit between the requirements and application of the technology, and the firm’s strategy, processes – both manufacturing and support – and its planning environment (Bharadwaj, 2000 ; Buer et al., 2020 ). With the enormous hype that came with Industry 4.0, it could be said that technology push has been the driver for most of its recent research and applications thus far.
Within the last two decades, there have been huge interest in research exploring the use of smart technologies to improve the performance of production systems and these studies can be grouped into three categories. The first group consists of studies where smart technologies are used individually. For instance, there are studies on the use of radio frequency identification (RFID) or other IoT technologies for tracking of materials and goods within a manufacturing system to provide data for evaluation and optimization of material flows and layouts (Lee & Özer, 2007 ; Ngai et al., 2008 ). An example is Zhong et al. ( 2013 ) who used RFID in a mass-customisation production environment to track and trace items on the shop floor, collecting real-time production data to identify and control shop floor disturbances through an MES. In another example, Ngai et al. ( 2007 ) report on a case study on the development of an RFID-based traceability system for tracing repairable items in aircraft maintenance operations.
The second group are those that build upon the use IoT technology and other tracking and tracing technology, adding the power of cloud computing to these solutions. This addition typically enables the management of several thousands more IoT sensors thereby allowing for a more nuanced tracking of materials and resources on the shopfloor and in the wider supply-chain. The concept of digital twin falls within this category of research and application especially when applied to a factory or individual machines in the factory. For example, Qu et al. ( 2016 ) develop a concept and system for IoT-based dynamic logistics control with cloud manufacturing and demonstrate their approach within a paint-manufacturing company in China which uses the make-to-order strategy. The solution concept offers real-time tracking and dynamic re-planning based on changes to the state of the system. In another example, Tao et al. ( 2018 ), in their conceptual study on data-driven smart manufacturing, discuss the distribution and tracking of materials, and the integration of data from the production process into production plans using an example in wafer production. The paper raises several points that can be useful in the design of smart PPC systems (such as the integration of digital twins and IoT technologies like edge gateways and edge computing) but does not address this explicitly. In a related study, Sun et al. ( 2020 ) propose a visual analytics approach to production planning, to address the need for solutions that will enable a quick response to sudden changes in the operations and market environment, and with the ability to handle the deluge of data in emerging industry 4.0 manufacturing systems.
The third group is newly emerging, with the recent interest in advanced analytics tools and artificial intelligence and its derivatives/subsets – i.e., machine learning and deep learning (Bueno et al., 2020 ; Cadavid et al., 2020 ). The interest in using machine learning in PPC by itself is not entirely new. Garetti and Taisch ( 1999 ) long ago investigated the application of machine learning in production scheduling problems. However, as with several studies of its type, their approach to the use of machine learning to improve manufacturing through smart PPC suffers from the solution linearity problem (Cadavid et al., 2020 ). The solution linearity problem is the issue that most of these studies are linear from data cleaning, to data exploration, and so on until insights generation and retraining, typically carried out through desktop operations. However, for production scenarios where scalability and autonomous system operation is desirable, these linear solutions are inadequate and will require continuous, often expensive human expert management to use in production. Thus, there is a need for self-sustaining solutions.
These studies have raised, although indirectly, some pertinent issues as regards the design and development of smart PPC systems. These issues are perhaps best synthesized in Bueno et al. ( 2020 ), where the authors identified several gaps and suggestions for future research in the smart PPC research domain. First, (on p .15), they highlight a scarcity in extant literature regarding the question of fit of industry 4.0 solutions and the integration of PPC in different environments. This question determines whether a solution, even if well executed, will deliver any real and lasting value to a manufacturing operation. Secondly, they emphasize on the need for research within development of intelligent decision support systems, frameworks and architectures that can advance smart PPC. And thirdly, they highlight that there is the need to determine the types of data to collect and use, the types of sensors to use and where in the production system to deploy them. This paper aims to address these gaps.
Design and development considerations
Concerning the application of smart technologies for PPC processes, the common cases reported in the literature can be categorized according to whether they address the strategic or long-term, tactical or medium-term, and operational or short-term scope within the PPC domain. The strategic use cases remain scarce in the literature (Bueno et al., 2020 ). This could be due to the immaturity of the emerging technologies to handle such broad data types and sources that typically feed into the strategic process, currently typified by use of managerial judgement who are able to also include those data sources that are difficult – but not impossible – to codify or assign a numerical value to. Meanwhile the tactical and operational PPC domains have seen increasing use of data with big data and machine learning for decision-making especially because of greater automation in operations processes. Furthermore, the distinction in the application of emerging technologies at the tactical and operational levels is not always clear, and use cases often overlap. Examples of use cases include real-time visualization and scenarios’ simulations (Sun et al., 2020 ), product quality control, and integrated production-maintenance scheduling (Biondi et al., 2017 ).
When using machine learning, the choice of appropriate algorithms and the system features to be used in training models can both be critical factors on project outcomes because different algorithms fit or perform better depending on the use case, features’ data quality and data architecture, and system architecture (O’Mahony et al., 2008 ; Pineda-Jaramillo, 2019 ). As noted by James et al. ( 2013 ), “ on a particular data set, one method may work best, but some other method may work better on a similar but different data set ” [ p . 29]. Therefore, it is crucial to find a fitting method to fit the use-case when using ML. An overview of machine learning algorithms in PPC use-cases and some architecture considerations follows.
Choosing an appropriate machine learning algorithm
As there are several ML tools and algorithms in the public domain currently, it can be a daunting task in finding one appropriate for a PPC use case. Within each of the three general categories of machine learning–that is, supervised, unsupervised, and reinforcement–new and more efficient algorithms and hybrids are being created continually, encouraged by the deluge of data, geometric reduction in computing cost that cloud computing brought about in the last decade, and advances in algorithm development and transference across multiple domains (Cadavid et al., 2020 ; Risi & Togelius, 2020 ).
Supervised learning concerns the approximation of a function based on a given set of input–output pairs. In this learning paradigm, the learning algorithm is provided (training) data which provides both, input values and output values, and the algorithm approximates the function that relates the inputs to the outputs. The approximated function can then be used to predict the outputs, given a set of inputs from outside the training set. The second machine learning paradigm, i.e., unsupervised learning is more exploratory in nature. Unlike supervised learning, there is no requirement for predefined input–output relationships in the training data that is used in unsupervised learning. Instead, the learning algorithm explores the data to find patterns and structures in the dataset, revealing which data-elements can be used as predictors of other elements. The third paradigm, i.e., reinforcement learning involves the use of iterative trial-and-error logic to train an algorithm to generate responses to inputs, that are expected to yield the highest reward (Monostori et al., 1996 ). Some use cases for the different machine learning types are presented in the following paragraphs and a summary in Table 1 .
Examples of supervised in the literature include Gyulai et al. ( 2014 ) who report on a case where supervised learning is used in optimizing the allocation of different products to two types of assembly lines, namely, reconfigurable and dedicated assembly lines. They use a random forest algorithm for predicting production costs for given order volumes and resource pools. In subsequent work, the authors use multivariate linear regression for predicting capacity requirements for future production scenarios on a flexible assembly line based on data from the manufacturing execution system (Gyulai et al., 2015 ). Heger et al. ( 2016 ) use Gaussian process regression for estimating the effect of different parameter settings on dispatching rules for scheduling. Examples of the use of unsupervised learning includes Pillania and Khan ( 2008 ) who applied k-means cluster analysis for categorizing firms in a supply chain according to each firms agility. Huang et al. ( 2019 ) propose the use of deep neural network for predicting future bottlenecks in a flexible manufacturing system, which is a use case for unsupervised learning. In another example, Shiue et al. ( 2012 ) propose the use of self-organising maps for selection of scheduling rules in semiconductor wafer fabrication.
Reinforcement learning, despite its huge potential for systems such as manufacturing systems, has only seen timid interest for PPC applications. Of interest within PPC research is the type of reinforcement learning called inverse reinforcement learning (IRL). According to Ng and Russell ( 2000 ), IRL may be useful when an agent is learning a “skilled behaviour,” such as the planning optimal scheduling process, and for which the reward function being optimized is determined by “a natural system”, such as a production system. Li et al. ( 2012 ) propose the use of Q-learning algorithm-based reinforcement learning for joint pricing and lead time decisions in a make-to-order system, where the decision problem is modelled as a semi-Markov decision problem. Tuncel et al. ( 2014 ) propose a Monte Carlo reinforcement learning algorithm for line balancing in disassembly operations under uncertain demand. Aissani et al. ( 2012 ) use a multi-agent based SARSA (state-action-reward-state-action) algorithm for production and distribution scheduling in a multi-site production network of a clothing company. Palombarini and Martínez ( 2012 ) use relational reinforcement learning for real-time (re)scheduling of extrusion operations in a secondary case study, i.e., the problem formulation is taken from literature. Lin et al. ( 2019 ) demonstrated an adaptation of the deep-Q network using an edge computing framework with multiple dispatching rules to demonstrate improved simulation results for job shop scheduling problems compared to methods using singular dispatching rules.
A related topic which has also seen significant recent developments is the use of ML algorithms in conjunction with heuristics and metaheuristics to address planning and control problems, especially at the operational level. Due to the mathematical intractability of production scheduling problems, using exact algorithms is often infeasible in practice, and heuristic policies are sometimes more pragmatic alternatives (Ðurasević & Jakobović, 2018 ). Metaheuristics such as genetic algorithms, tabu search, particle swarm optimization, etc. provide better results than heuristics for some scheduling applications (Maoudj et al., 2019 ; Ouelhadj & Petrovic, 2009 ; Xiong & Fu, 2018 ). However, most metaheuristic algorithms are non-deterministic and require long solution times for large problem sizes (Maoudj et al., 2019 ). Recent studies explore the use of ML to address these limitations and to support more efficient use of metaheuristics, for example, by using ML for the reduction of the solution space for metaheuristics (Bouzary et al., 2021 ) or for identifying when it is beneficial to rerun the metaheuristic (Li et al., 2020 ). Bouzary et al. ( 2021 ) propose a combination of support vector machines and genetic algorithm for addressing the service composition problem in a cloud manufacturing context, where they use ML for identifying the solution space for the metaheuristic. Li et al. ( 2020 ) use tabu search and genetic algorithm for schedule optimization, and a random forest classifier for identifying instances when production should be rescheduled based on whether the metaheuristic is likely to yield a more efficient schedule than the one available.
In all these developments, one important area that has seen little overage in the smart PPC literature is about the management of data acquisition and integration, data exploration, and a process to continually update and retrain ML models during use (Cadavid et al., 2020 ). In practice, the absence of a complete (or “ circular ”) workflow leads to changes to the system going undetected over time, a phenomenon known as concept drift. This is a major shortcoming of extant data analytics and machine learning research in general, and especially with regards to application within the PPC domain (Cadavid et al., 2020 ; Hammami et al., 2017 ).
Data architecture considerations
The data architecture describes the design, structure and control of the data generating and collection elements. As data is the foundation for smart manufacturing and related concepts including smart PPC, the data architecture plays a vital role in the implementation and long-term viability and flexibility (to adjust to change) for any such system. For convenience and for hierarchical analysis, data from the manufacturing system should be amenable to grouping according the familial associations. This can be achieved using classes and objects belonging to those classes, in fitting with the object-oriented architecture. The objects that are members of the same class with similar attributes such as usage area, etc. For example, a ‘Sugarproducts’ class can have members such as ‘Orangemix’, ‘Gingercandy’ (all random names) which comprise that class. The machines can also be grouped into classes for instance the ‘Driers’ class could comprise all the driers in a factory’s production line.
Furthermore, data quality played a key role in the value companies were able to derive from enterprise planning systems like ERP and MES systems before the emergence of smart PPC systems (Gustavsson & Wänström, 2009 ). The importance of data quality is now more crucial because of the data intensiveness of smart PPC systems which use data from a wide range of sources including from within the plant, (potentially) from other partner systems, and from the production system’s environment (Oluyisola et al., 2020 ). And while current enterprise systems collect sales transaction data from external customers and transactions generated directly from operations such as materials consumption in warehouses and factory floor production data (Koh et al., 2011 ; Mantravadi et al., 2019 ), the capacity to derive value from the abundant data in real-life environments has been a challenge (Kusiak, 2017 ).
Furthermore, there are different types of data available to any PPC system. Based on the temporal proximity of the data generation and collection processes, they can be classified as being either batch data, where data is collected and updated periodically, or stream data, where data is being generated, collected, and potentially analyzed in real-time. In production environments, many data processing systems implement some kind of runner using the Apache Beam model (Li et al., 2018 ). Most of data from the factory’s environment and some of the machines in the production lines are time-series, stream data. An example of the time-series data snippet from an IoT device on a production machine in the JavaScript Object Notation (JSON) format is as shown in Fig. 1 below. But there are also batch data which are seldom revised, for example the setup cost, and are input to the PPC processes.
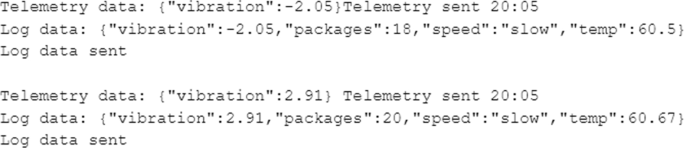
Example of the telemetry data generated by an IoT sensor on a production line
Systems architecture considerations
An information system’s architecture can be defined as a collection of artefacts, namely a definition of constituent components of the IS, a specification of the properties of those components, and a description of the relationship among those components and their interactions during operation (Bass et al., 2013 ; Goepp et al., 2006 ). The use of the term design in this paper generally refers to the creation of the architecture of the smart PPC system. Because smart PPC systems are information systems, its developers must follow similar principles. This design must be made early in the overall development process, and in a way that allows for enough detail so that the developers have enough guidance, while at the same time it must allow sufficient freedom for the developers to make decisions during the actual development stage (Bass et al., 2013 ).
Within the broader Industry 4.0 research domain, generic architectural models have been proposed for the industry 4.0 production system and these can provide inspiration for the smart PPC solutions designers and architects. Common examples include the Reference Architecture Model for Industrie 4.0 (RAMI 4.0), the Industrial Internet Reference Architecture (IIRA) and the internet-of-things reference architectures (IoT RA) standard in the ISO/IEC 30,141:2018 (Standardization, 2018 ). Nevertheless, these models should only serve as reference due to their generic nature and the fact that they do not cater for the context each production manager must address.
Typically, enterprise planning systems are designed as hierarchical control systems using a monolithic architecture (Themistocleous et al., 2005 ). This is the case in which the system is built on a single, large, highly powered computer hardware. Such an architecture has several benefits, not least its speed due to its low natural latency, its limited need to manage integrations with several units, and that there is only a single hardware device to be managed instead of potentially several. And this was important for many decades before the advent of cloud computing, since companies had to create a physical datacenter with server hardware and all the attendant management requirements. But this architecture also hard several shortcomings. It limited flexibility to, for example, add new tools and functionalities as this needed to be upgraded every time the main server itself received an upgrade from its suppliers who are often very big software vendors and who made upgrades based on general needs, and not on the specific needs of each customer. It is more costly to start-up, manage and run with a savings of up to 50% in terms of total cost of ownership (Mattison & Raj, 2012 ). This contrasts with the emerging smart technologies which are changing so fast, that there is an intrinsic need to design for flexibility and frequent changes.
These design considerations are addressed by the modular-by-design microservices architecture instead of a monolithic architecture. The microservices architecture presents a considerable benefit for several reasons: it can scale easily, and it is highly adaptable. It has been reported that self-adapting and self-optimizing multi-agent distributed production control systems have been demonstrated to perform better during transitions when used in job-shop environments where hierarchical systems are too rigid to adjust to the flexibility requirements of such environments (Ma et al., 2020 ). Thus, smart PPC systems initiatives have a better chance at success if they employ a microservices architecture.
Finally, most research on the use of ML in PPC suffer from workflow-design linearity in addition to being based on artificial or historic, sampled data (Cadavid et al., 2020 ). While these conditions make testing specific models for confined problems easy, they are not feasible in real-life industrial practice. The challenge with linear design is that to use it in practice, a human operator needs to administer the intelligence creation process of the system, as seem in reported case literature, for example, Garetti and Taisch ( 1999 ) and Brintrup et al. ( 2019 ). In real-life industrial scenarios however, the smart PPC system should be able to collect data, clean it, prepare it for analysis, retrain its models, and offer refreshed insights without human intervention potentially self-adjusting its control parameters (Oluyisola et al., 2020 ; Rojas & Garcia, 2020 ). It should address the risk of concept drift, for instance by using adaptive time windows (Bifet & Gavalda, 2007 ). This could be achieved using data processing pipelines and monitoring scripts connected to a version control system for managing model versioning, a concept referred to as MLOps – that is, machine learning operations, which is a derivation of DevOps for ML.
In summary, there are two main perspectives in the literature through which topics related to smart PPC have been viewed. First, in the puristic production and operations management perspective, information and communication technologies (ICTs) are viewed as add-ons or auxiliary that can enable or improve information flow but are usually considered exogenous (Slack et al., 2013 ). A contrasting view is that of information systems-centered research within the context of manufacturing, that considers ICTs as an integral variable and focuses on opportunities for performance improvements by employing ICTs – for example, Huang ( 2017 ). In the methodology proposed in this paper, an attempt is made at using a more balanced, multi-disciplinary view. In this context, material flow is controlled and monitored with ICT-enabled information flow, thus making ICTs integral components of the industrial system. Smart technologies or advanced ICTs are thus viewed as intrinsic elements of the smart production system as opposed to being add-ons.
A methodology for designing and developing a smart PPC Solution
Having already established the need for a systematic methodology and guide for manufacturing firms who may want to develop a smart PPC solution, the key steps that such an initiative could follow and the elements that should be given proper consideration are presented in this section. Here, the presented ‘steps’ suggests ‘sequence’ suggesting which steps should precede what. However, as it will be explained in the case study, the process does not have to be linear. In practice, it is often necessary to revisit preceding steps while at another as the requirements become clearer to the stakeholders of the project. The following steps can be followed in developing a smart PPC solution:
Stem 1. Preliminary study: determine objectives and priorities in fitting with the planning environment variables.
Stem 2. System requirements specification: validate the operations’ problems and identify performance indicators.
Stem 3. Identify data sources and select relevant analytics and machine learning algorithms that fits the problem.
Stem 4. Design system and data architecture with consideration for integration with extant systems and IoT telemetry.
Stem 5. Implement with considerations for development methodologies, continuous innovation and long-term adaptability.
Step 1: preliminary study: determine objectives and priorities in fitting with the planning environment variables
Most digitalization projects are driven by either an identified business problem or a perceived market opportunity. And since they are innovation projects, the immediate goals of the smart PPC solution must be determined ex ante to reduce the risk of scope creep and to increase the chance of success. The preliminary study takes a high-level view of the problem or opportunity, and with particular emphasis on how the market, product, and process attributes inhibits or promotes the problem. Also, the management sets the objective regarding how much of the opportunity the company is willing to pursue or to what extent an issue needs to be addressed. For example, if a production planning process is having a fulfillment rate of 75 percent on average and leading to unacceptable underutilization of booked operator hours and wastage of materials, management could set an objective to improve this key performance indicator (KPI) with the use of smart technologies to, say, 90 percent in a year’s time. These objectives and priorities must be weighed against the constraints imposed by the planning environment attributes of the operation.
It is also a common occurrence for there to be a need to make tradeoffs over which elements of the solution requirements to prioritize in the short and long term. For instance, a company in the process industry manufacturing, say, industrial paint, may see several opportunities and use cases for digitalizing its operations and PPC processes. Easily, managers could be interested in digitalizing the production line with IoT sensors that will collect various kinds of data about the production processes and send these data to the cloud for analytics and predictions, or on an edge device for real-time response. Another use case could involve attaching sensors to the packaging containers (which may be a bucket) or pallet, enabling a full tracking and tracing of the inventory coming out of the production line; yet another could involve the tracking of weather or climate factors and how this affect demand or sales at the stores; and so on.
Now, if this were a large multinational with millions of euros in research and development budget, then the company could start with and run multiple projects simultaneously, bearing in mind that results will be mixed. However, for a smaller company with a tighter budget, it will be critical to prioritize, focusing only on projects with a high expected return be it financial or digital competence gains for the company. In the example, following the argument that a process strategy is has great potential in this type of production environment, and the budget-constrained producer will prioritize those initiatives that lead to a smart process, for instance digitalizing the production line with IoT sensors capturing parameters that affect the yield of the operations. This could also be combined with other telemetry data from the production line’s immediate environment.
Step 2: system requirements specification: validate the operations’ problems and identify performance indicators
Step 2 takes the preliminary objectives and initial assessment from the top-management horizon in step 1 down to the detailed, solution-specific design requirements that could be used for the technical design and the actual development of the solution. As previously explained in step 1, the objectives are typically the prerogative of the company’s management team and often represent their interpretation of the problems that must be addressed from a top-down view of the operation. However, a lot of the data driven decisions and insights affect or are affected by junior managers and operators on the factory floor. Therefore, there is a need to validate the objectives of the solution from the perspectives of persons directly interacting with the production system before specifying the functional and non-functional requirements of the proposed solution. One way to achieve this is to formalize the requirements using user stories. User stories are written in the format “As an [role/persona], I want to [action] so that [why],” and each user story should be clear and descriptive. For example, a user story could go as follows: “As a production planner, I want to be able to upload productions orders for the next two weeks into the solution with approved production orders from the ERP system so that I do not have to copy this manually.” User stories should be independent, negotiable, value-focused, estimable, small, and testable. Later during implementation, production managers and the system developers will determine how to prioritize the user stories for development. In addition, there should be flexibility in terms of which elements of the system remain on the list of functionalities to be developed, while allowing for future adjustments (Pressman & Maxim, 2015 ).
In addition, performance indicators (PIs) are needed to monitor both the quality of analysis and predictions being generated by the smart PPC system and of the reliability of the system. The PIs relating to the quality of the results can include the standard deviation and errors for individual predictions determined through random spot measurements. Those relating to the performance of the smart PPC system can include prediction lag, simulation request processing time, and general indicators like availability/downtime hours and the like. While PIs relating to the quality of the analysis and predictions will be context specific, most of the system PIs are generic and common to service-oriented, cloud-based ICT systems.
Step 3: identify data sources and select relevant analytics and machine learning algorithms that fits the problem
The user stories give an indication of the services that primary users – production planners and operations managers – require the smart PPC system to fulfill. After identifying these services, the next step is to determine the relevant data sources from the production system and identify the appropriate analytics tools and machine learning algorithms that works best for the kind of insight or prediction required. This determination and identification can be done by a small technical team involving a machine learning engineer or data scientist with a good understanding of not just the technical problem but also the business problem.
In many manufacturing use-cases, pilot projects could start with simpler ML algorithms such as Gaussian linear regression and logistic regression (supervised), and with PCA and k-means clustering (unsupervised) with an acceptable level of success. However, after the pilot phase of such projects–that is, during the real-life implementation–there will be a need to improve the performance of the solution and which can be achieved using hybrid models which combine multiple features of the basic algorithms. For example, when the use case involves sparse data inputs and an extensive feature list, the hybrid algorithm called the DNNCombinedLinearRegression can be used in place of the common supervised learning to combine the strengths of neural networks (generalization) and linear regression models (memorization of feature interactions) (Cheng et al., 2016 ).
Step 4: design system and data architecture with consideration for integration with extant systems and IoT technology
Many large manufacturing organizations, in addition to having an ERP system also have full-fledged solutions for the control of manufacturing operations on the factory floor–the MES. Some MES systems have basic analytics capabilities built in such as statistical process control charts that allows process-tracking, and most collect time-series stream data from the discrete units of production lines to which they are connected. Alone, using the MES for manufacturing control misses the opportunity that a holistic, connected smart system affords. Therefore, the system architecture should cater for the introduction of IoT sensors to the factory even for factories are already automated. The MES and ERP systems provide a good starting point for developing smart PPC solutions. The data from these systems and other factory IT systems might however require extensive transformations before they can be used in combination with newly installed IoT technology in the smart factory.
In general, modular smart PPC solution design would perform better than a monolithic solution since it will allow for future improvements within each module independent of others and will also ensures that failure in one service does not break the entire system. Furthermore, when the solution is built on a service architecture from the onset, the it is easier to add more modules in the future and to update individual modules that are already in use. This is achieved by designing the modules as services and building application programming interfaces (APIs) to manage interaction among services. The data processing, model development, and prediction processes can be carried out without manual human interaction by automating the data preparation and prediction processes using ML pipelines.
Moreover, in cases where an active control (rather than just a monitoring) of the production process is required, it is advisable to have the trained machine learning model interacting with the production machines and processes on the “edge” without the need to send to the cloud and send instructions back to the plant. However, because the real-time data processing occurs at the edge, this creates a challenge due to the limited processing power at the edge and need for continuously monitoring the performance of the model to avert model drift. Furthermore, edge devices may lose their connection to the cloud and thus the solution must cater for offline operations. Otherwise, where there is no need for any serious computing at the edge, it suffices to send all data generated from the production system to the cloud.
Step 5: implement with considerations for development methodologies, continuous innovation and long-term adaptability
For the implementation of smart PPC solutions, there are at least four key considerations: whether to outsource or develop in-house, which software development methodology to adopt, whether to choose managed-cloud services or to use completely open-source technologies, and how to design the system so that it supports continuous innovation. It is possible to develop in-house or to establish joint development partnership arrangements with service providers for small-scale functionalities. But it is more likely to outsource major system development activities to established IT firms if the needed project execution competence is lacking in-house. In addition, the development of the solution will often require the choice of building almost from scratch with the use of open source technologies, or – if faster deployment is desired – the use of any one or a combination of the several managed-cloud services for a faster development process, while allowing for agile development.
Smart PPC systems need to support continuous innovation. Continuous innovation in this sense relates to how the established IT infrastructure and software development processes eliminates tedious manual processes for making changes and improvements to working system, and allow a seamless, continuous integration, testing, and deployment of those changes without any downtime. Therefore, non-agile methodologies will generally be insufficient for their development because of the relative rigidity of such methods. And because many of the technologies being used in smart PPC systems are experiencing constant, fast-paced advancements, the success of any smart PPC solution requires that there is a smooth and simple process in place for its continuous improvement. Moreover, the alignment or integration of the workflows and processes of the both the machine learning engineers and software developers will enable the streamlining of continuous innovation and the refinement of ML models as new data becomes available from the production system being monitored.
Finally, for information systems’ developers, the concept of DevOps has emerged as a preferred way to manage the continuous, version-controlled, code development cycle – that is, write, test, (revise,) build, (revise,) deploy (revise). While machine learning engineers and data scientists take the ML cycle – that is, experimentation, model-creation, testing, operations, and maintenance. By integrating these two workflows – to have what is now referred to as DevOps for machine learning (MLOps) – productivity can be improved significantly through software development process automation, allowing machine learning engineers and data scientists to focus on the model performance rather than being bogged down in tedious software development operations’ activities. One way this is achieved is by using infrastructure-as-code and process automation in managing the system’s improvements and the revisions’ process. Process automation could be achieved using Bash or Python scripts, or through robotic process automation software that allows automation using drag-and-drop tools. The latter, less programming-intensive option can be managed by a trained production planner, thereby lowering the cost of development.
In this section, the method and processes presented above are illustrated with an application within a case, which will be referred to as Sweets and Nuts ASA (not real name) or SNASA for short. The company manufactures sweets and nuts-based products in its factory based in Norway from where it supplies grocery chains, kiosks, and petrol stations’ mini-stores in the Scandinavian region. The nuts production section of the factory is isolated from the rest of the factory in line with regulations concerning the control of allergens. The rest of the factory produces chocolate-based and non-chocolate sweets such as pastilles. The unit of analysis in this case study is the non-chocolate-based (henceforth, NCB) section of the operation.
Determine objectives and priorities in fitting with the planning environment variables
The NCB production is serviced by two production lines. The production process for the NCB products is as shown in the Fig. 2 below.
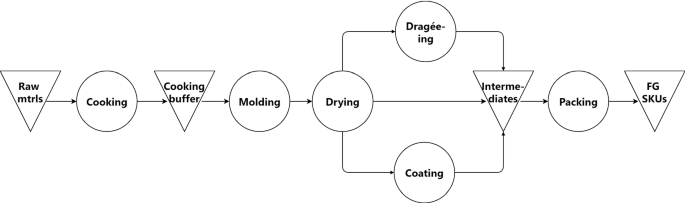
The NCB production process at SNASA
The operations at the NCB section falls into the semi-process class. Raw materials are fed into the cooking drums in amounts determined by the recipe for the batch to be produced. When the cooking process is completed, the output is temporarily stored in a cooking buffer before molding, using mold trays with the shapes of the sweets engraved. The trays are thereafter arranged in racks which are loaded into one of the seven drying chambers in the drying section of the factory. The production data currently used in the production planning process includes the estimated lead time for all processes, stock levels of the different stock keeping units (SKUs) in the finished goods warehouse, recipes (which also provide a bill of materials). The maximum batch size the line can produce for each product is pre-calculated based on the capacity of the production processes.
The challenges of this current PPC system can be described in three categories namely, market (demand and supply) related, product related, and process related. First, market related demand related challenges stem from the high competitiveness of the industry and the fickle nature of human taste preferences. A popular product can sometimes loose its spark with consumers or get overshadowed by new trending products. For this reason, the NCB industry witnesses a lot of promotions and discount sales to drive and sustain demand. Secondly, the product related challenges are minimal in this case because the products are neither complex nor have any deep bill-of-materials which could have required extensive materials requirements planning tools. Furthermore, the simplicity of products made by this case company (packed sweets) and the price per unit implies that the product itself will not benefit from a smart product strategy. Rather, a smart process strategy will be for fitting for this type of case (Oluyisola et al., 2020 ). Such process approach must be able to track the remaining life for any product or batch in the finished goods storage and in the various warehouse within the company’s value chain and must also be able to trace its journey through the value (Høyer et al., 2019 ).
Lastly, the process related challenges are generally due to the nature of the materials being processed and the level of maturity of the process technologies. Currently, there is a long set-up and changeover time due to the need to wash the machines and equipment producing every new batch. This is also required to meet regulatory requirements for cleanliness and food safety. There is also a yield uncertainty that planners currently must guess when issuing production orders and this causes additional variability in the production system. Also related to the process is the operator-planning related challenges relate to how labour is planned in the company. Over several years, the company has developed a practice of planning batch sizes that can be completed within a production shift. This is a suboptimal constraint on the planning process. Therefore, with the attributes of this production environment, this company’s approach to smart manufacturing should take a smart process strategy, rather than a smart product strategy since the product is simple and the unit price is very small.
System requirements specification: operations’ problems and performance indicators
Problem specification.
The company SNASA faces an immediate challenge: finding an optimal production schedule and managing the scheduling process to minimize variation. Thus, the production planning problem for this case comprises two main elements, namely: the determination of the optimal plan, which maximizes throughput through the bottleneck drying process and assumes no yield variation (that is, yield = 1.0 or 100%); and an estimation of the yield uncertainty factor, to improve the accuracy of production plans. Currently, planners must guess the what the yield will be and add some buffer to the amount that is produced so that at least the final production output for each batch exceeds the planned amount required to meet order forecasts. This leads to overproduction, and it particularly expensive for products which serve as inputs into ‘mix’-type products. The mix-type products are made by combining three to five different types of products into one assortment.
The planning problem can be summarized as follows:
Given a set of firmed customer orders, and master production scheduling orders (MPS orders are those generated by the ERP system based on demand and supply forecasts), with each order characterized by: its drying time (which is an indication of the throughput time), its due date, its volume or amount; and
Given a set of drying sections or rooms, each with a fixed drying capacity, and given a set of packaging lines, each characterized by a fixed capacity;
Find the schedule of orders that maximizes the number of completed orders at the two stages drying and packaging.
Furthermore, the planning problem can also be viewed as a multi-stage or multi-echelon scheduling problem for which although the drying stage, which is used for all products from the production line, is not always the bottleneck. This is because the average speed of the packaging machines is low enough that they can cause delays if poorly scheduled and depending on the product. This is partly because there are several packaging lines with varying speeds and no single product has a dedicated packaging machine. After production schedule is made, the plan must be adjusted for reality by estimating a yield uncertainty factor. This yield uncertainty is a factor of environmental parameters such as humidity and temperature.
Requirements specification
The requirements, shown in Table 2 , were gathered from the production managers and planners of SNASA during this research-based improvement project towards smart manufacturing. An overview of the solution concept is presented in Fig. 3 . KPI result data going into recommender system will include actual production performance (lateness, earliness, on-time, etc.), specific operator working the process (this shows how specific operators affect performance), etc. The newly added elements of this smart PPC system are described in Sect. 4.3 . A description of each step in Fig. 3 is provided in Table 3 .
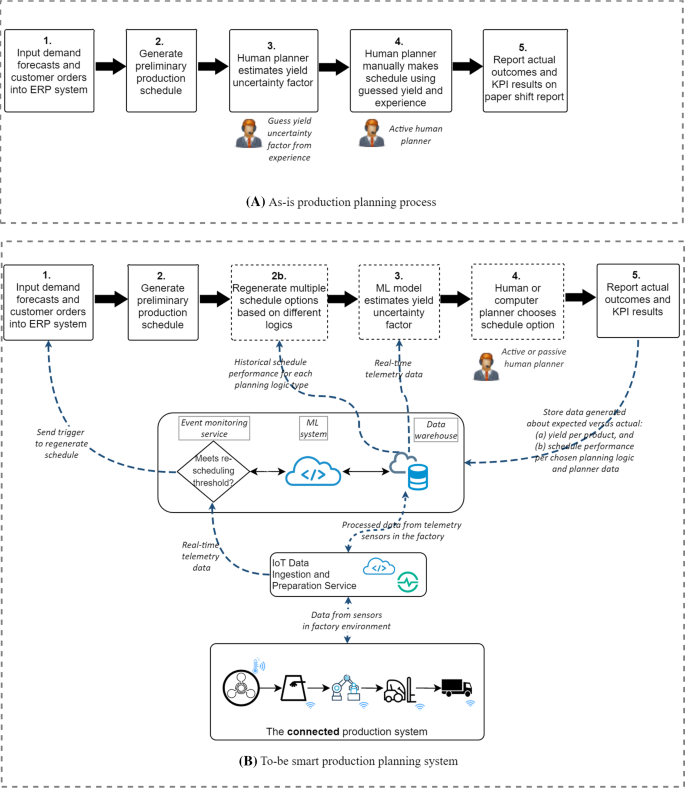
Conceptual overview of the as-is compared to the to-be smart PPC solution
Performance indicators
It is important to have predetermined how the performance of the system will be measured. In the selection of performance measures or indicators for this case, there are two categories namely, operations reliability and services quality. The operations reliability measure has to do with how the software system is designed, architected, and developed. It is measured by reliability measures such as up-time (a maximizing measure) or downtime (a minimizing measure), percent failed schedule requests from the user interface and waiting time between schedule launch and results presentation on the dashboard. The services quality refers to the quality of the results, estimates and recommendations offered by the smart PPC solution. Measures include the amount of deviation of the estimated yield from the actual yield, the average performance of the recommended schedule logic over period.
Step 3: identify relevant tools and algorithms
There are two choices to be made regarding the two applications of machine learning within this SNASA case: one for estimating the yield and the other for recommending which schedule logic alternative will perform best for each planning scenario. The yield estimation (or prediction) can be hypothesized to be the dependent variable of a linear or non-linear system. As such, a simple linear regression model is a good start for this use case. Once the system is built and in place, other variants of the linear regression can be tested in a development environment to see how much improvement in performance is possible. Examples of those are models combining basic models with more performant neural networks such as the wide and deep DNNCombinedLinearRegression algorithm or similar. This model will be fitting for this purpose due to the potential sparseness of the features. The data fields that will be used in the model are shown (without telemetry) in the class diagram in Fig. 5 and a detailed list (with telemetry) is provided in the table in Fig. 5 . Meanwhile, the subsystem for recommending which planning logic option to choose appears amenable to inverse reinforcement learning.
Step 4: solution architecture –data and systems architecture design
While academic projects on the use of ML in PPC tend to use linear development processes, live production projects require the use of recyclable, reproducible machine learning pipelines which can be automated. For the SNASA case, an illustrative system architecture for the yield estimator use-case is presented in Fig. 4 .
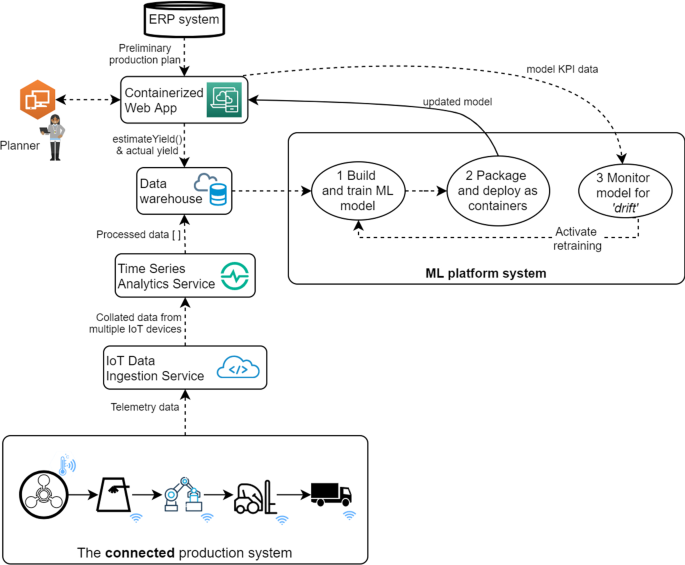
An example smart PPC solution architecture for the yield estimator use-case
In the illustrative smart PPC architecture in Fig. 4 , the ‘connected’ production system which is connected by IoT sensors sends data via secure connections to a cloud data-ingestion service. This service can use a distributed commit log technology such as the open-source Apache Kafka or one of the easier-to-use IT vendor solutions. This is to be configured so that it guarantees that every data sent by a sensor is delivered, and so that the data are in the right sequence when they arrive at the Analytics Service. The real-time analytics output from the analytics service could be made available on a dashboard on the factory floor or in the production supervisor’s office for real-time monitoring of the factory. This data then flows from the analytics service to the data warehouse where it is accessed by the ML solution. The ML solution, which also runs in the cloud, will continuously check for model drift and it will activate retraining when set KPI thresholds are met. The ML model works within a web app with a graphical user interface for the production planner to interact with. The planner will input the actual yield and production order data after every production order is fulfilled. The web app will also ingest production plan data from the ERP system for the computation of the yield, and it will continually send both the estimated and actual yields to the data warehouse for later use during model retraining. (Fig. 4 ).
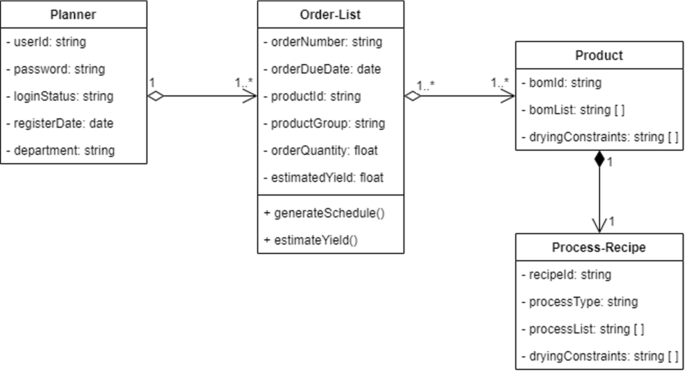
Class diagram for a demo in UML notation
In this case study, the assumption of a one week “fixed” planning window is made in line with current practices by the production planners, during which the list of orders to be processed is assumed to be deterministic – except if a major disruption or urgent firmed customer order is received. However, during this one-week period, the forecasts for some of model feature variables (for example, environmental data) are only precise for two days into the future at any given point. Therefore, there will be a need for re-scheduling at least once every two days to take advantage of the trained model. In the future, when a lengthy historical data has been gathered, it will be possible to train the model using only the historical data without the need to use the weather forecast data whose accuracy diminishes materially beyond a 48-h from the reference point.
Steps 5: implementation considerations and performance assessment
This use-case is illustrated using open source technologies for the sake of demonstration. However, for production, the company might be better served by using managed services on any of the major cloud platforms. One could start with a small pilot to test an idea, or go big, with a large-scale project and iterate on improvements. The latter approach can lead to faster business impact. There are also pilot versus real-life production implementation considerations. The nature of this production planning is such as that the properties of the system of interest changes frequently relative to the target precision of prediction results. Furthermore, as the data scientist and the developers working on this project will need close collaboration, and there is a requirement to be able to scale the solution to address other PPC use cases as the companies gains organizational competence with PPC. These factors strengthen the need for MLOps.
Insights from the case study and implications for research and practice
In the preceding sections, a methodology for designing and developing smart PPC systems was described and the application of this methodology was illustrated through a case study. In this section, the application of the methodology within the case study is reviewed, after which insights gained from the case study and the implications for research and practice are discussed.
The objectives and priorities identified in the first step of the methodology were used as basis for formalizing the problem and specifying the requirements and relevant performance indicators. This step helped refine the requirements that were put forth by production managers and planners, who are the intended beneficiaries of the smart PPC system. These requirements included having multiple schedule logic options, integration with existing ERP system, dynamic rescheduling or more frequent scheduling updates, yield estimation using telemetry factors and capturing the experience of managers. While these were the functional requirements for the PPC system, non-functional requirements such as ease of use and readability of the user-interface layout were also identified although the latter non-functional requirements were not the subject of this case study. Consequently, operations reliability and services quality were deemed as the relevant performance measures for the smart PPC solution design.
Of interest in this case study was the problem of yield of estimation at the drying station. This was important in this case because the yield, which affects the precision of the entire planning process, is highly influenced by exogenous factors, e.g., temperature, humidity, etc., factors which can be modelled and predicted using analytics and ML tools. This again reemphasizes the importance of fitting smart technologies to production systems according to fit as pointed out in Oluyisola et al. ( 2020 ). By the same principle, a smart product strategy would not be beneficial in this case company. In addition, the formalized problem and specified requirements were used to identify candidate tools and algorithms to address the problem and fulfill the requirements. For the purpose of this case study, this selection of tools and algorithms was based on extant literature on smart PPC (reviewed in Sect. 2.3.1 ). While this was lightly covered in this paper, this as an area that future research need to address for the ML value in PPC to be realizable. The final step in the methodology focuses on continuous innovation and/or development, i.e., the system should be adaptable when weaknesses are identified during use or as opportunities for utilization of better or more mature technologies become available. The performance of the current, as-is process is compared with the improvements that can be achieved by the proposed smart PPC (when fully operational) in Table 4 . These are measured against the general goals of the smart PPC system established in the literature. By reason of the capacity, consistency and flexibility that the smart PPC system affords, as the case illustration highlights, the improvements are such that the manufacturing organization will be able to anticipate and react more precisely to changes in the production environment.
The general implications of having a methodology such as the one presented in this paper are significant for research and practice. By having a methodology which starts with the determination of fit according to the planning and control environment variables, it will be possible to streamline smart PPC initiatives and increase their chances of success. Based on the PPC environment characteristics, it was possible to determine early in the process that the case company would benefit more from a smart process strategy rather than a smart product strategy. And while the issues of interest in the case study are primarily operational, the methodology itself is not constrained vis-à-vis the application context or decision levels and can be applied for initiatives pursuing strategic and tactical decision support.
Furthermore, due to the current rate of innovation within the disciplines of big-data analytics and machine learning, the availability of tools and algorithms for a given set of problems is constrained by the state of art at any point of time and may change as time progresses. Therefore, this step of the methodology could be reviewed after an interval, which should be decided during the initial or pilot implementation. The next step in the methodology concerns architectural considerations for the implementation of the solution. This step not only considers the architectural design for the proposed solution itself, but also considers the integration of the solution with the existing enterprise systems, thus re-emphasizing the focus of the methodology on ensuring fit of the smart PPC system with the planning environment. Furthermore, while designing the data architecture in this step, due consideration must be given to future scenarios, such that the developed system is scalable and amenable to future operational demands.
Additionally, as Cadavid et al. ( 2020 ) highlight in a recent review paper, there is a need to address the linearity limitations of extant research on ML-enhanced PPC and also a need to link tools, techniques and activities for industry get real benefits from research on the subject. The architectural considerations prescribed in this methodology addresses this key issue and should be a major consideration for future applied research on the subject. This cannot be overemphasized considering how small and medium sized manufacturing companies must grapple with the uncertainties of a pandemic-battered global economy and the post-pandemic global market.
Additionally, anecdotal evidence with manufacturing companies in the Scandinavia region shows that while increasing automation and digitalization has led to the creation of massive volumes of big data in manufacturing systems, a lot of the data is neither used nor is useful. The reasons vary for each case, but a recurring theme is that the data architectures are often designed primarily as a logging system for use in maintenance activities and many manufacturing companies still are yet to fully adoption an IoT strategy. All these factors then make it more challenging to derive value using analytics or machine learning to build intelligence into these production environments.
From the foregoing, the several considerations to be made when developing a smart PPC solution include the planning environment challenges which are often relatively consistent in the long-term, and the technology-related challenges which are related to the fast-paced evolution. And due to the significant uncertainty involved in the innovation process, and the high risk of project failure, the selection of use cases cannot be done randomly or based sole on what is trending with competitors. Indeed, while over 60 percent of IT projects fail outrightly or when defined by one of the performance metrics of timeliness, cost or quality (Mark, 2016 ), anecdotal evidence suggests that this may be even worse for projects involving emerging technologies. In one example, a major distribution and logistics center recently had an innovation project where it tried to deploy autonomous robots with machine learning capabilities in one its warehouses. The project failed both technologically and operationally, and the company did not share information about this failure publicly potentially because it does not help the company’s brand posture as a technology savvy organization.
It can therefore be assumed that there is a greater likelihood or perhaps a tendency for companies to want to report only successful digitalization projects. This may, over time, lead to a ‘survivorship bias’, as researchers would only have cases of successful projects to extract knowledge from, while losing access to the valuable knowledge that could be extracted from the failed implementations. Furthermore, this creates a lacuna because while there may be ‘local learning’ within each company, there is a global loss due to several companies repeating pilot projects that many others previously tried and failed at. Therefore, a systematic method of the type proposed in this study can help reduce the risk of smart PPC project failure and can reduce the variation amongst several subsequent smart PPC initiatives, thus enabling easier shared learning.
Conclusions, limitations, and further research
The question of how a smart PPC system should be designed and developed for an environment has been addressed in this paper through a five-step methodology. The steps of the methodology have been formulated and structured with the consideration that the resulting PPC system should fit the characteristics of the environment in question. Furthermore, the importance of contextual fit in algorithm selection, solution scalability and amenability of the smart PPC system to address future demands have been highlighted. In summary, the principles and considerations that guide the design in a smart PPC system are as follows:
The design of the smart PPC system should fit the characteristics of planning environment. This highlights an issue that has been observed in numerous ERP and APS implementation case studies – expensive monolithic systems forcing managers to modify the production system to fit an inflexible PPC system. The proposed methodology can guide the design and development of such a fitting smart PPC system.
The design and architecture of the PPC system should be scalable and amenable to variations in future demand volumes, demand patterns, product portfolios, number of users, etc. Since these parameters cannot be controlled or accurately predicted in advance, it is important to have provisions in the architecture to adapt as these parameters change during drift .
The implementation plan of a smart PPC system should also include a period of ‘incubation’ where data can be collected to train the ML models, if the data is not already available. Simultaneously, the models can be tested for accuracy, such that the estimation errors can be accounted for in the planning activities.
However, this study has the following limitations. First, implementing the methodology requires experience and judgement to ensure that the relevant contextual variables have been considered in assessing the fit of objectives and priorities with the planning environment variables. A framework of contextual variables could provide an exhaustive reference and reduce the requirements for experience in implementing the methodology effectively and will be addressed by future research. Finally, in future studies, this methodology will be tested in other types of production environments and industry sectors to assess its weaknesses and improve its robustness and generalizability.
Ahmad, M. M., & Cuenca, R. P. (2013). Critical success factors for Erp implementation in smes. Robotics and Computer-Integrated Manufacturing, 29 , 104–111.
Article Google Scholar
Aissani, N., Bekrar, A., Trentesaux, D., & Beldjilali, B. (2012). Dynamic scheduling for multi-site companies: A decisional approach based on reinforcement multi-agent learning. Journal of Intelligent Manufacturing, 23 , 2513–2529.
Arica, E., & Powell, D. J. (2014). A framework for Ict-enabled real-time production planning and control. Advances in Manufacturing, 2 , 158–164.
Arnold, J. T., Chapman, S. N. & Clive, L. M. 2011. Introduction To Materials Management , Pearson Higher Ed
Aslan, B., Stevenson, M., & Hendry, L. C. (2012). Enterprise resource planning systems: An assessment of applicability to make-to-order companies. Computers in Industry, 63 , 692–705.
Aslan, B., Stevenson, M., & Hendry, L. C. (2015). The applicability and impact of enterprise resource planning (Erp) systems: results from a mixed method study on make-to-order (mto) companies. Computers in Industry, 70 , 127–143.
Bass, L., Clements, P., & Kazman, R. (2013). Software architecture in practice . Addison-Wesley Professional.
Google Scholar
Beckman, S. L., & Rosenfield, D. B. (2008). Operations strategy: Competing in the 21st century . Boston: Mcgraw-Hill/Irwin.
Bharadwaj, A. S. 2000. A Resource-Based Perspective On Information Technology Capability And Firm Performance: An Empirical Investigation. Mis Quarterly , 169–196.
Bifet, A. & Gavalda, R. Learning From Time-Changing Data With Adaptive Windowing. Proceedings Of The 2007 Siam International Conference On Data Mining, 2007. Siam, 443–448.
Biondi, M., Sand, G., & Harjunkoski, I. (2017). Optimization of multipurpose process plant operations: A multi-time-scale maintenance and production scheduling approach. Computers and Chemical Engineering, 99 , 325–339.
Bouzary, H., Chen, F. F. & Shahin, M. 2021. Using Machine Learning For Service Candidate Sets Retrieval In Service Composition Of Cloud-Based Manufacturing. The International Journal Of Advanced Manufacturing Technology , 1–8.
Brintrup, A., Pak, J., Ratiney, D., Pearce, T., Wichmann, P., Woodall, P., & Mcfarlane, D. (2019). Supply chain data analytics for predicting supplier disruptions: A case study in complex asset manufacturing. International Journal Of Production Research, 58 , 1–12.
Bueno, A. F., Godinho Filho, M. & Frank, A. G. 2020. Smart Production Planning And Control In The Industry 4.0 Context: A Systematic Literature Review. Computers & Industrial Engineering , 106774.
Buer, S.-V., Semini, M., Strandhagen, J. O. & Sgarbossa, F. 2020. The Complementary Effect Of Lean Manufacturing And Digitalisation On Operational Performance. International Journal Of Production Research , 1–17.
Cadavid, J. P. U., Lamouri, S., Grabot, B., Pellerin, R. & Fortin, A. 2020. Machine Learning Applied In Production Planning And Control: A State-Of-The-Art In The Era Of Industry 4.0. Journal Of Intelligent Manufacturing , 1–28.
Carvalho, A. N., Scavarda, L. F., & Lustosa, L. J. (2014). Implementing finite capacity production scheduling: Lessons from a practical case. International Journal of Production Research, 52 , 1–16.
Chan, J. W. K. (2005). Competitive strategies and manufacturing logistics: An empirical study of Hong Kong manufacturers. International Journal of Physical Distribution and Logistics Management, 35 , 20–43.
Chavez, R., Yu, W., Jacobs, M. A., & Feng, M. (2017). Data-driven supply chains, manufacturing capability and customer satisfaction. Production Planning and Control, 28 , 906–918.
Cheng, H.-T., Koc, L., Harmsen, J., Shaked, T., Chandra, T., Aradhye, H., Anderson, G., Corrado, G., Chai, W. & Ispir, M. Wide & Deep Learning For Recommender Systems. Proceedings Of The 1st Workshop On Deep Learning For Recommender Systems, 2016. 7–10.
De Man, J. C., & Strandhagen, J. O. (2018). Spreadsheet application still dominates enterprise resource planning and advanced planning systems. Ifac-Papersonline, 51 , 1224–1229.
Ðurasević, M., & Jakobović, D. (2018). A survey of dispatching rules for the dynamic unrelated machines environment. Expert Systems with Applications, 113 , 555–569.
Fatorachian, H., & Kazemi, H. (2020). Impact of industry 4.0 on supply chain performance. Production Planning and Control, 32 , 1–19.
Garetti, M., & Taisch, M. (1999). Neural networks in production planning and control. Production Planning and Control, 10 , 324–339.
Goepp, V., Kiefer, F., & Geiskopf, F. (2006). Design of information system architectures using a key-problem framework. Computers in Industry, 57 , 189–200.
Guha, S., & Kumar, S. (2018). Emergence of big data research in operations management, information systems, and healthcare: Past contributions and future roadmap. Production and Operations Management, 27 , 1724–1735.
Gustavsson, M., & Wänström, C. (2009). Assessing information quality in manufacturing planning and control processes. International Journal Of Quality and Reliability Management, 26 , 325.
Gyulai, D., Kádár, B., & Monosotori, L. (2015). Robust production planning and capacity control for flexible assembly lines. Ifac-Papersonline, 48 , 2312–2317.
Gyulai, D., Kádár, B., & Monostori, L. (2014). Capacity planning and resource allocation in assembly systems consisting of dedicated and reconfigurable lines. Procedia Cirp, 25 , 185–191.
Hammami, Z., Mouelhi, W., & Said, L. B. (2017). On-line self-adaptive framework for tailoring a neural-agent learning model addressing dynamic real-time scheduling problems. Journal of Manufacturing Systems, 45 , 97–108.
Heger, J., Branke, J., Hildebrandt, T., & Scholz-Reiter, B. (2016). Dynamic adjustment of dispatching rule parameters in flow shops with sequence-dependent set-up times. International Journal of Production Research, 54 , 6812–6824.
Holmström, J., Ketokivi, M., & Hameri, A. P. (2009). Bridging practice and theory: A design science approach. Decision Sciences, 40 , 65–87.
Hong, P. C., Dobrzykowski, D. D., & Vonderembse, M. A. (2010). Integration of supply chain it and lean practices for mass customization: benchmarking of product and service focused manufacturers. Benchmarking, 17 , 561–592.
Huang, B., Wang, W., Ren, S., Zhong, R. Y., & Jiang, J. (2019). A proactive task dispatching method based on future bottleneck Prediction for the smart factory. International Journal of Computer Integrated Manufacturing, 32 , 278–293.
Huang, Y. (2017). Information architecture for effective workload control: An insight from a successful implementation. Production Planning and Control, 28 , 351–366.
Høyer, M. R., Oluyisola, O. E., Strandhagen, J. O., & Semini, M. G. (2019). Exploring the challenges with applying tracking and tracing technology in the dairy industry. Ifac-Papersonline, 52 , 1727–1732.
James, G., Witten, D., Hastie, T., & Tibshirani, R. (2013). An introduction to statistical learning . Springer.
Book Google Scholar
Koh, S. L., Gunasekaran, A., & Goodman, T. (2011). Drivers, barriers and critical success factors for Erpii implementation in supply chains: A critical analysis. The Journal of Strategic Information Systems, 20 , 385–402.
Kusiak, A. (2017). Smart manufacturing must embrace big data. Nature, 544 , 23–25.
Lee, H., & Özer, Ö. (2007). Unlocking the value of Rfid. Production and Operations Management, 16 , 40–64.
Li, S., Gerver, P., Macmillan, J., Debrunner, D., Marshall, W., & Wu, K.-L. (2018). Challenges and experiences in building an efficient apache beam runner for Ibm streams. Proceedings of the Vldb Endowment, 11 , 1742–1754.
Li, X., Wang, J., & Sawhney, R. (2012). Reinforcement learning for joint pricing, lead-time and scheduling decisions in make-to-order systems. European Journal of Operational Research, 221 , 99–109.
Li, Y., Carabelli, S., Fadda, E., Manerba, D., Tadei, R., & Terzo, O. (2020). Machine learning and optimization for production rescheduling in industry 4.0. The International Journal of Advanced Manufacturing Technology, 110 , 2445–2463.
Lin, C. C., Deng, D. J., Chih, Y. L., & Chiu, H. T. (2019). Smart manufacturing scheduling with edge computing using multiclass deep Q network. Ieee Transactions on Industrial Informatics, 15 , 4276–4284.
Lupeikiene, A., Dzemyda, G., Kiss, F., & Caplinskas, A. (2014). Advanced planning and scheduling systems: Modeling and implementation challenges. Informatica, 25 , 581–616.
Ma, A., Frantzén, M., Snider, C., & Nassehi, A. (2020). Anarchic manufacturing: Distributed control for product transition. Journal of Manufacturing Systems, 56 , 1–10.
Mantravadi, S., Li, C. & Møller, C. Multi-Agent Manufacturing Execution System (Mes): Concept, Architecture & Ml Algorithm For A Smart Factory Case. Iceis 2019 - Proceedings Of The 21st International Conference On Enterprise Information Systems, 2019. 465–470.
Maoudj, A., Bouzouia, B., Hentout, A., Kouider, A., & Toumi, R. (2019). Distributed multi-agent scheduling and control system for robotic flexible assembly cells. Journal of Intelligent Manufacturing, 30 , 1629–1644.
Mark, A. Pmi’s Pulse Of The Profession: The High Cost Of Low Performance. How Will You Improve Business Results. Project Management Institute, 2016.
Mattison, J. B. & Raj, S. 2012. Key Questions Every It And Business Executive Should Ask About Cloud Computing And Erp. Accenture White Paper .
Monostori, L., Márkus, A., Van Brussel, H., & Westkämpfer, E. (1996). Machine learning approaches to manufacturing. Cirp Annals, 45 , 675–712.
Nagy, J., Oláh, J., Erdei, E., Máté, D., & Popp, J. (2018). The role and impact of industry 4.0 and the internet of things on the business strategy of the value chain—the case of hungary. Sustainability, 10 , 3491.
Ng, A. Y. & Russell, S. J. Algorithms For Inverse Reinforcement Learning. Icml, 2000. 2.
Ngai, E., Cheng, T., Lai, K. H., Chai, P., Choi, Y., & Sin, R. (2007). Development of an Rfid-based traceability system: Experiences and lessons learned from an aircraft engineering company. Production and Operations Management, 16 , 554–568.
Ngai, E., Moon, K. K., Riggins, F. J., & Candace, Y. Y. (2008). Rfid research: An academic literature review (1995–2005) and future research directions. International Journal of Production Economics, 112 , 510–520.
O’mahony, E., Hebrard, E., Holland, A., Nugent, C. & O’sullivan, B. Using Case-Based Reasoning In An Algorithm Portfolio For Constraint Solving. Irish Conference On Artificial Intelligence And Cognitive Science, 2008. 210–216.
Oluyisola, O. E., Sgarbossa, F., & Strandhagen, J. O. (2020). Smart production planning and control: Concept use-cases and sustainability implications. Sustainability, 12 , 3791.
Ouelhadj, D., & Petrovic, S. (2009). A survey of dynamic scheduling in manufacturing systems. Journal of Scheduling, 12 , 417–431.
Öztürk, C., & Ornek, A. M. (2014). Operational extended model formulations for advanced planning and scheduling systems. Applied Mathematical Modelling, 38 , 181–195.
Palombarini, J., & Martínez, E. (2012). Smartgantt–an intelligent system for real time rescheduling based on relational reinforcement learning. Expert Systems with Applications, 39 , 10251–10268.
Petroni, A., & Braglia, M. (2000). Vendor selection using principal component analysis. Journal of Supply Chain Management, 36 , 63–69.
Pillania, R. K. & Khan, A. 2008. Strategic Sourcing For Supply Chain Agility And Firms' Performance. Management Decision .
Pineda-Jaramillo, J. D. (2019). A review of machine learning (Ml) algorithms used for modeling travel mode choice. Dyna, 86 , 32–41.
Pressman, R. S., & Maxim, B. (2015). Software engineering: A practitioner’s approach . Mc Graw-Hill Education.
Qu, T., Lei, S., Wang, Z., Nie, D., Chen, X., & Huang, G. Q. (2016). Iot-based real-time production logistics synchronization system under smart cloud manufacturing. The International Journal of Advanced Manufacturing Technology, 84 , 147–164.
Risi, S., & Togelius, J. (2020). Increasing generality in machine learning through procedural content generation. Nature Machine Intelligence, 2 , 1–9.
Rojas, R. A., & Garcia, M. A. R. (2020). Implementation of industrial internet of things and cyber-physical systems in smes for distributed and service-oriented control industry 4.0 for smes . Palgrave Macmillan.
Saenz De Ugarte, B., Hajji, A., Pellerin, R., & Artiba, A. (2009). Development and integration of a reactive real-time decision support system in the aluminum industry. Engineering Applications of Artificial Intelligence, 22 , 897–905.
Shaikh, N., Prabhu, V., Abril, D., Sánchez, D., Arias, J., Rodríguez, E., & Riaño, G. (2011). Kimberly-Clark Latin America builds an optimization-based system for machine scheduling. Interfaces, 41 , 455–465.
Shiue, Y.-R., Guh, R.-S., & Tseng, T.-Y. (2012). Study on shop floor control system in semiconductor fabrication by self-organizing Map-based intelligent multi-controller. Computers and Industrial Engineering, 62 , 1119–1129.
Skinner, W. (1974). The focused factory . Harvard Business Review Brighton.
Slack, N., Brandon-Jones, A., & Johnston, R. (2013). Operations management . Pearson.
Standardization, I. O. F. 2018. Internet Of Things (Lot) — Reference Architecture. Information Technology (It) In General.
Steger-Jensen, K., Hvolby, H. H., Nielsen, P., & Nielsen, I. (2011). Advanced planning and scheduling technology. Production Planning and Control, 22 , 800–808.
Strandhagen, J. O., Vallandingham, L. R., Fragapane, G., Strandhagen, J. W., Stangeland, A. B. H., & Sharma, N. (2017). Logistics 4.0 and emerging sustainable business models. Advances in Manufacturing, 5 , 359–369.
Sun, D., Huang, R., Chen, Y., Wang, Y., Zeng, J., Yuan, M., Pong, T. C., & Qu, H. (2020). Planningvis: A visual analytics approach to production planning in smart factories. Ieee Transactions on Visualization and Computer Graphics, 26 , 579–589.
Tao, F., Qi, Q., Liu, A., & Kusiak, A. (2018). Data-driven smart manufacturing. Journal of Manufacturing Systems, 48 , 157–169.
Tenhiälä, A. (2011). Contingency theory of capacity planning: The link between process types and planning methods. Journal of Operations Management, 29 , 65–77.
Tenhiälä, A., & Helkiö, P. (2015). Performance effects of using an Erp system for manufacturing planning and control under dynamic market requirements. Journal of Operations Management, 36 , 147–164.
Themistocleous, M., Roseman, M., Loos, P. & Møller, C. 2005. Erp Ii: A Conceptual Framework For Next‐Generation Enterprise Systems? Journal Of Enterprise Information Management .
Tuncel, E., Zeid, A., & Kamarthi, S. (2014). Solving large scale disassembly line balancing problem with uncertainty using reinforcement learning. Journal of Intelligent Manufacturing, 25 , 647–659.
Vieira, G. E., Herrmann, J. W., & Lin, E. (2003). Rescheduling manufacturing systems: A framework of strategies, policies, and methods. Journal of Scheduling, 6 , 39–62.
Xiong, W., & Fu, D. (2018). A new immune multi-agent system for the flexible job shop scheduling problem. Journal of Intelligent Manufacturing, 29 , 857–873.
Zhong, R. Y., Dai, Q., Qu, T., Hu, G., & Huang, G. Q. (2013). Rfid-enabled real-time manufacturing execution system for mass-customization production. Robotics and Computer-Integrated Manufacturing, 29 , 283–292.
Download references
Open access funding provided by NTNU Norwegian University of Science and Technology (incl St. Olavs Hospital - Trondheim University Hospital).
Author information
Authors and affiliations.
Department of Mechanical and Industrial Engineering, Norwegian University of Science and Technology, Trondheim, Norway
Olumide Emmanuel Oluyisola, Swapnil Bhalla, Fabio Sgarbossa & Jan Ola Strandhagen
You can also search for this author in PubMed Google Scholar
Corresponding author
Correspondence to Olumide Emmanuel Oluyisola .
Additional information
Publisher's note.
Springer Nature remains neutral with regard to jurisdictional claims in published maps and institutional affiliations.
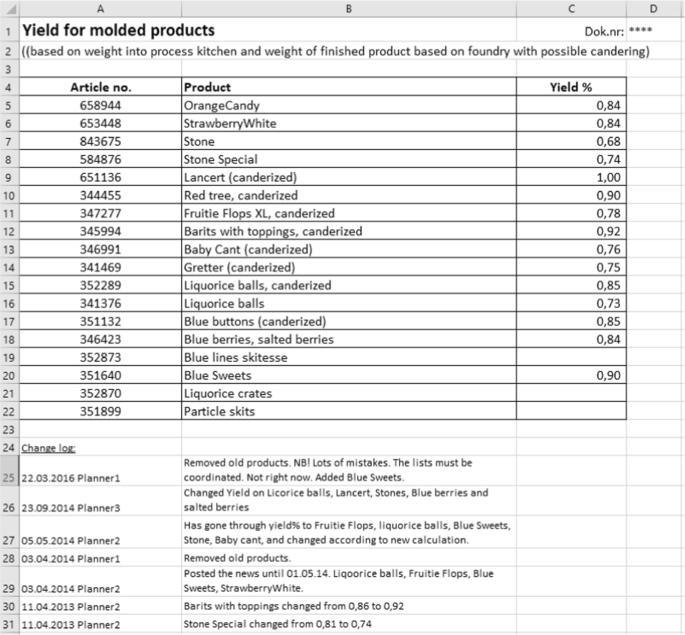
The current manually updated yield estimate spreadsheet
5 and 6 , Table 5
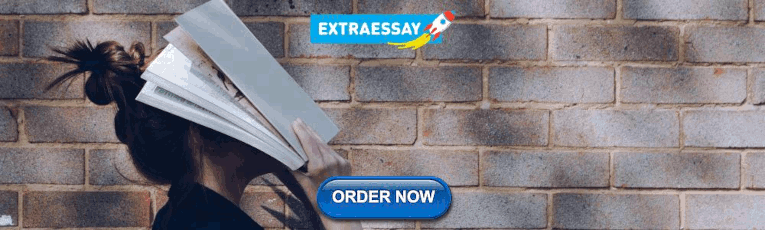
Rights and permissions
Open Access This article is licensed under a Creative Commons Attribution 4.0 International License, which permits use, sharing, adaptation, distribution and reproduction in any medium or format, as long as you give appropriate credit to the original author(s) and the source, provide a link to the Creative Commons licence, and indicate if changes were made. The images or other third party material in this article are included in the article's Creative Commons licence, unless indicated otherwise in a credit line to the material. If material is not included in the article's Creative Commons licence and your intended use is not permitted by statutory regulation or exceeds the permitted use, you will need to obtain permission directly from the copyright holder. To view a copy of this licence, visit http://creativecommons.org/licenses/by/4.0/ .
Reprints and permissions
About this article
Oluyisola, O.E., Bhalla, S., Sgarbossa, F. et al. Designing and developing smart production planning and control systems in the industry 4.0 era: a methodology and case study. J Intell Manuf 33 , 311–332 (2022). https://doi.org/10.1007/s10845-021-01808-w
Download citation
Received : 30 November 2020
Accepted : 21 June 2021
Published : 04 July 2021
Issue Date : January 2022
DOI : https://doi.org/10.1007/s10845-021-01808-w
Share this article
Anyone you share the following link with will be able to read this content:
Sorry, a shareable link is not currently available for this article.
Provided by the Springer Nature SharedIt content-sharing initiative
- Production planning and control
- Smart manufacturing
- Internet of things
- Machine learning
- Industry 4.0
- Decision support systems
- Find a journal
- Publish with us
- Track your research
- Resources Home 🏠
- Try SciSpace Copilot
- Search research papers
- Add Copilot Extension
- Try AI Detector
- Try Paraphraser
- Try Citation Generator
- April Papers
- June Papers
- July Papers

What is a thesis | A Complete Guide with Examples

Table of Contents
A thesis is a comprehensive academic paper based on your original research that presents new findings, arguments, and ideas of your study. It’s typically submitted at the end of your master’s degree or as a capstone of your bachelor’s degree.
However, writing a thesis can be laborious, especially for beginners. From the initial challenge of pinpointing a compelling research topic to organizing and presenting findings, the process is filled with potential pitfalls.
Therefore, to help you, this guide talks about what is a thesis. Additionally, it offers revelations and methodologies to transform it from an overwhelming task to a manageable and rewarding academic milestone.
What is a thesis?
A thesis is an in-depth research study that identifies a particular topic of inquiry and presents a clear argument or perspective about that topic using evidence and logic.
Writing a thesis showcases your ability of critical thinking, gathering evidence, and making a compelling argument. Integral to these competencies is thorough research, which not only fortifies your propositions but also confers credibility to your entire study.
Furthermore, there's another phenomenon you might often confuse with the thesis: the ' working thesis .' However, they aren't similar and shouldn't be used interchangeably.
A working thesis, often referred to as a preliminary or tentative thesis, is an initial version of your thesis statement. It serves as a draft or a starting point that guides your research in its early stages.
As you research more and gather more evidence, your initial thesis (aka working thesis) might change. It's like a starting point that can be adjusted as you learn more. It's normal for your main topic to change a few times before you finalize it.
While a thesis identifies and provides an overarching argument, the key to clearly communicating the central point of that argument lies in writing a strong thesis statement.
What is a thesis statement?
A strong thesis statement (aka thesis sentence) is a concise summary of the main argument or claim of the paper. It serves as a critical anchor in any academic work, succinctly encapsulating the primary argument or main idea of the entire paper.
Typically found within the introductory section, a strong thesis statement acts as a roadmap of your thesis, directing readers through your arguments and findings. By delineating the core focus of your investigation, it offers readers an immediate understanding of the context and the gravity of your study.
Furthermore, an effectively crafted thesis statement can set forth the boundaries of your research, helping readers anticipate the specific areas of inquiry you are addressing.
Different types of thesis statements
A good thesis statement is clear, specific, and arguable. Therefore, it is necessary for you to choose the right type of thesis statement for your academic papers.
Thesis statements can be classified based on their purpose and structure. Here are the primary types of thesis statements:
Argumentative (or Persuasive) thesis statement
Purpose : To convince the reader of a particular stance or point of view by presenting evidence and formulating a compelling argument.
Example : Reducing plastic use in daily life is essential for environmental health.
Analytical thesis statement
Purpose : To break down an idea or issue into its components and evaluate it.
Example : By examining the long-term effects, social implications, and economic impact of climate change, it becomes evident that immediate global action is necessary.
Expository (or Descriptive) thesis statement
Purpose : To explain a topic or subject to the reader.
Example : The Great Depression, spanning the 1930s, was a severe worldwide economic downturn triggered by a stock market crash, bank failures, and reduced consumer spending.
Cause and effect thesis statement
Purpose : To demonstrate a cause and its resulting effect.
Example : Overuse of smartphones can lead to impaired sleep patterns, reduced face-to-face social interactions, and increased levels of anxiety.
Compare and contrast thesis statement
Purpose : To highlight similarities and differences between two subjects.
Example : "While both novels '1984' and 'Brave New World' delve into dystopian futures, they differ in their portrayal of individual freedom, societal control, and the role of technology."
When you write a thesis statement , it's important to ensure clarity and precision, so the reader immediately understands the central focus of your work.
What is the difference between a thesis and a thesis statement?
While both terms are frequently used interchangeably, they have distinct meanings.
A thesis refers to the entire research document, encompassing all its chapters and sections. In contrast, a thesis statement is a brief assertion that encapsulates the central argument of the research.
Here’s an in-depth differentiation table of a thesis and a thesis statement.
Now, to craft a compelling thesis, it's crucial to adhere to a specific structure. Let’s break down these essential components that make up a thesis structure
15 components of a thesis structure
Navigating a thesis can be daunting. However, understanding its structure can make the process more manageable.
Here are the key components or different sections of a thesis structure:
Your thesis begins with the title page. It's not just a formality but the gateway to your research.

Here, you'll prominently display the necessary information about you (the author) and your institutional details.
- Title of your thesis
- Your full name
- Your department
- Your institution and degree program
- Your submission date
- Your Supervisor's name (in some cases)
- Your Department or faculty (in some cases)
- Your University's logo (in some cases)
- Your Student ID (in some cases)
In a concise manner, you'll have to summarize the critical aspects of your research in typically no more than 200-300 words.

This includes the problem statement, methodology, key findings, and conclusions. For many, the abstract will determine if they delve deeper into your work, so ensure it's clear and compelling.
Acknowledgments
Research is rarely a solitary endeavor. In the acknowledgments section, you have the chance to express gratitude to those who've supported your journey.

This might include advisors, peers, institutions, or even personal sources of inspiration and support. It's a personal touch, reflecting the humanity behind the academic rigor.
Table of contents
A roadmap for your readers, the table of contents lists the chapters, sections, and subsections of your thesis.
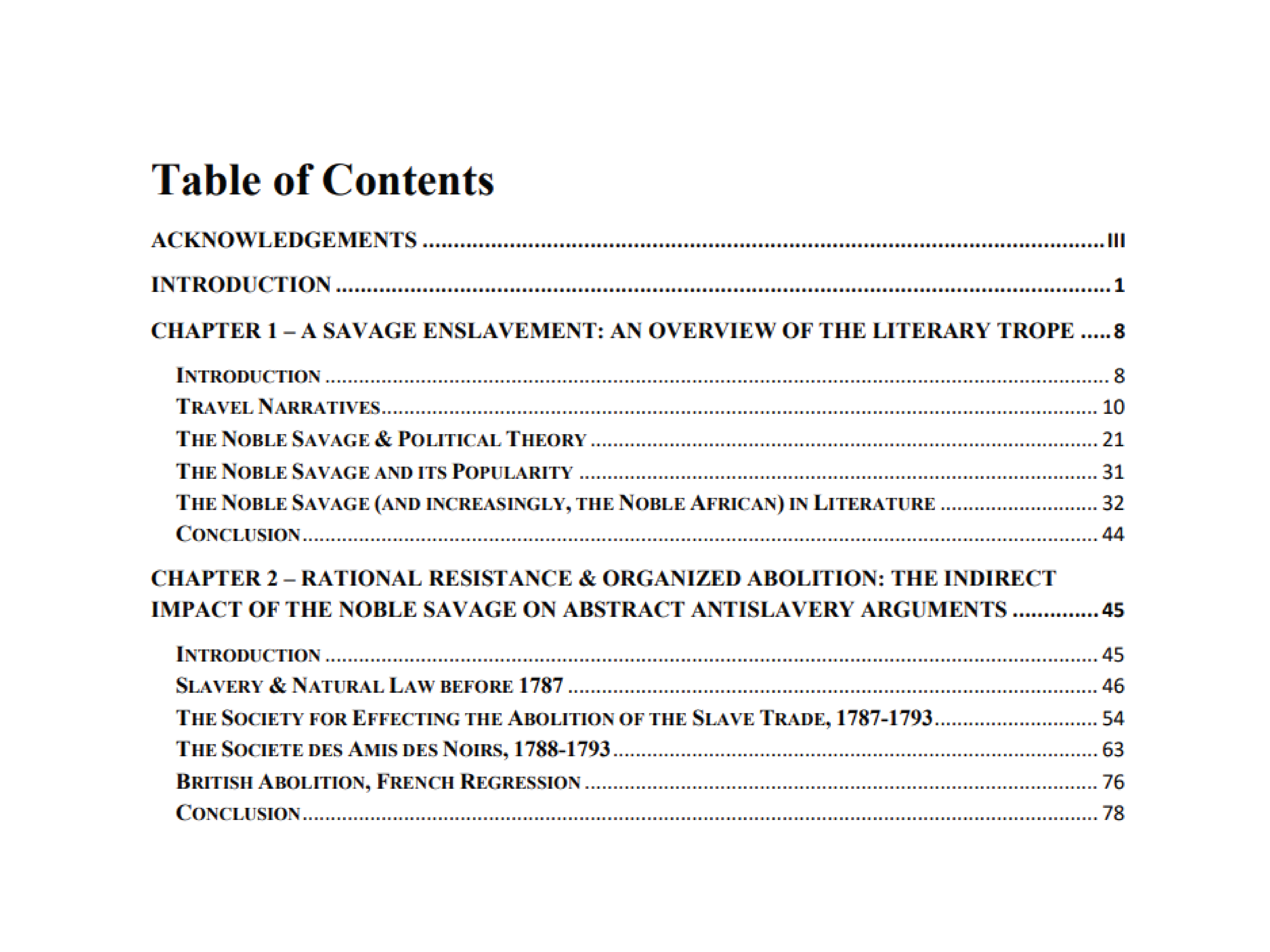
By providing page numbers, you allow readers to navigate your work easily, jumping to sections that pique their interest.
List of figures and tables
Research often involves data, and presenting this data visually can enhance understanding. This section provides an organized listing of all figures and tables in your thesis.

It's a visual index, ensuring that readers can quickly locate and reference your graphical data.
Introduction
Here's where you introduce your research topic, articulate the research question or objective, and outline the significance of your study.

- Present the research topic : Clearly articulate the central theme or subject of your research.
- Background information : Ground your research topic, providing any necessary context or background information your readers might need to understand the significance of your study.
- Define the scope : Clearly delineate the boundaries of your research, indicating what will and won't be covered.
- Literature review : Introduce any relevant existing research on your topic, situating your work within the broader academic conversation and highlighting where your research fits in.
- State the research Question(s) or objective(s) : Clearly articulate the primary questions or objectives your research aims to address.
- Outline the study's structure : Give a brief overview of how the subsequent sections of your work will unfold, guiding your readers through the journey ahead.
The introduction should captivate your readers, making them eager to delve deeper into your research journey.
Literature review section
Your study correlates with existing research. Therefore, in the literature review section, you'll engage in a dialogue with existing knowledge, highlighting relevant studies, theories, and findings.
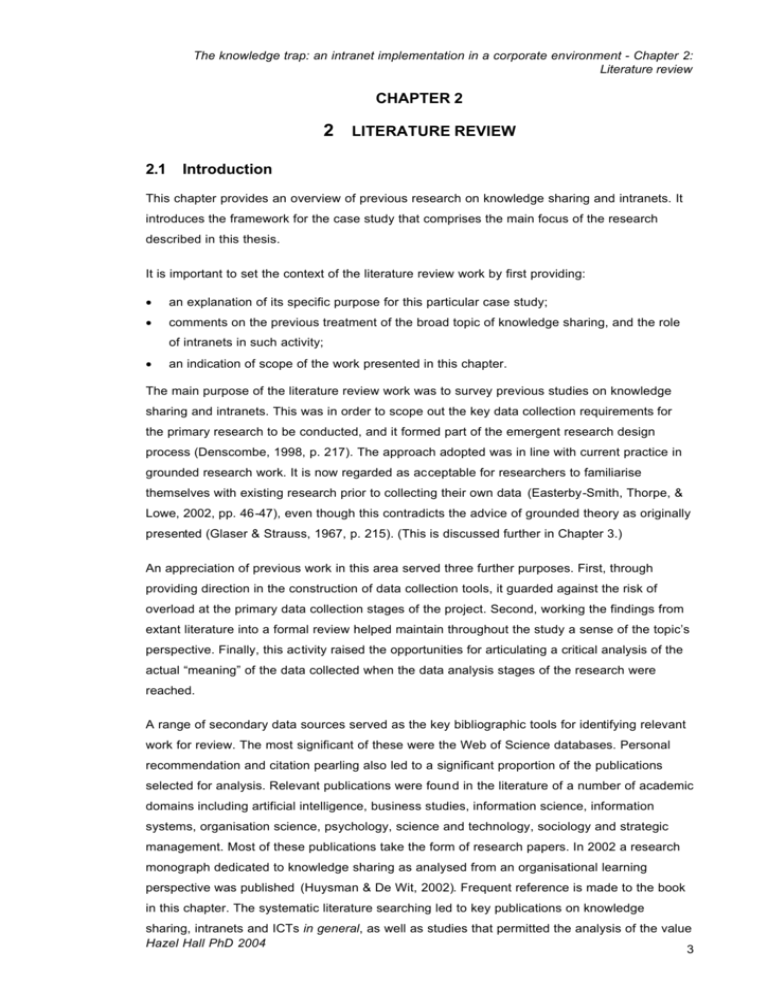
It's here that you identify gaps in the current knowledge, positioning your research as a bridge to new insights.
To streamline this process, consider leveraging AI tools. For example, the SciSpace literature review tool enables you to efficiently explore and delve into research papers, simplifying your literature review journey.
Methodology
In the research methodology section, you’ll detail the tools, techniques, and processes you employed to gather and analyze data. This section will inform the readers about how you approached your research questions and ensures the reproducibility of your study.
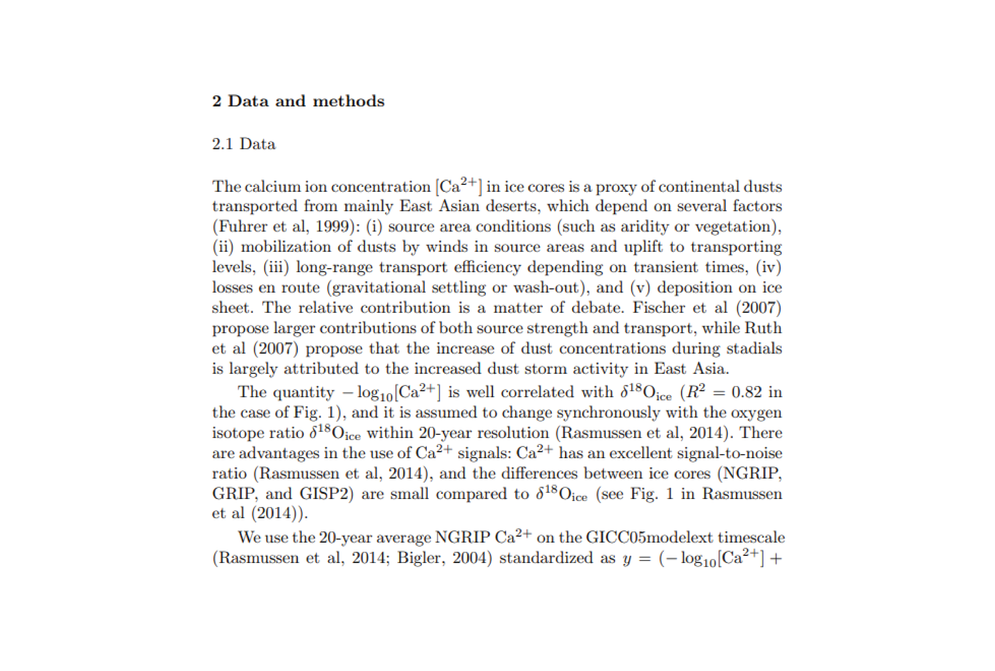
Here's a breakdown of what it should encompass:
- Research Design : Describe the overall structure and approach of your research. Are you conducting a qualitative study with in-depth interviews? Or is it a quantitative study using statistical analysis? Perhaps it's a mixed-methods approach?
- Data Collection : Detail the methods you used to gather data. This could include surveys, experiments, observations, interviews, archival research, etc. Mention where you sourced your data, the duration of data collection, and any tools or instruments used.
- Sampling : If applicable, explain how you selected participants or data sources for your study. Discuss the size of your sample and the rationale behind choosing it.
- Data Analysis : Describe the techniques and tools you used to process and analyze the data. This could range from statistical tests in quantitative research to thematic analysis in qualitative research.
- Validity and Reliability : Address the steps you took to ensure the validity and reliability of your findings to ensure that your results are both accurate and consistent.
- Ethical Considerations : Highlight any ethical issues related to your research and the measures you took to address them, including — informed consent, confidentiality, and data storage and protection measures.
Moreover, different research questions necessitate different types of methodologies. For instance:
- Experimental methodology : Often used in sciences, this involves a controlled experiment to discern causality.
- Qualitative methodology : Employed when exploring patterns or phenomena without numerical data. Methods can include interviews, focus groups, or content analysis.
- Quantitative methodology : Concerned with measurable data and often involves statistical analysis. Surveys and structured observations are common tools here.
- Mixed methods : As the name implies, this combines both qualitative and quantitative methodologies.
The Methodology section isn’t just about detailing the methods but also justifying why they were chosen. The appropriateness of the methods in addressing your research question can significantly impact the credibility of your findings.
Results (or Findings)
This section presents the outcomes of your research. It's crucial to note that the nature of your results may vary; they could be quantitative, qualitative, or a mix of both.

Quantitative results often present statistical data, showcasing measurable outcomes, and they benefit from tables, graphs, and figures to depict these data points.
Qualitative results , on the other hand, might delve into patterns, themes, or narratives derived from non-numerical data, such as interviews or observations.
Regardless of the nature of your results, clarity is essential. This section is purely about presenting the data without offering interpretations — that comes later in the discussion.
In the discussion section, the raw data transforms into valuable insights.
Start by revisiting your research question and contrast it with the findings. How do your results expand, constrict, or challenge current academic conversations?
Dive into the intricacies of the data, guiding the reader through its implications. Detail potential limitations transparently, signaling your awareness of the research's boundaries. This is where your academic voice should be resonant and confident.
Practical implications (Recommendation) section
Based on the insights derived from your research, this section provides actionable suggestions or proposed solutions.
Whether aimed at industry professionals or the general public, recommendations translate your academic findings into potential real-world actions. They help readers understand the practical implications of your work and how it can be applied to effect change or improvement in a given field.
When crafting recommendations, it's essential to ensure they're feasible and rooted in the evidence provided by your research. They shouldn't merely be aspirational but should offer a clear path forward, grounded in your findings.
The conclusion provides closure to your research narrative.
It's not merely a recap but a synthesis of your main findings and their broader implications. Reconnect with the research questions or hypotheses posited at the beginning, offering clear answers based on your findings.

Reflect on the broader contributions of your study, considering its impact on the academic community and potential real-world applications.
Lastly, the conclusion should leave your readers with a clear understanding of the value and impact of your study.
References (or Bibliography)
Every theory you've expounded upon, every data point you've cited, and every methodological precedent you've followed finds its acknowledgment here.

In references, it's crucial to ensure meticulous consistency in formatting, mirroring the specific guidelines of the chosen citation style .
Proper referencing helps to avoid plagiarism , gives credit to original ideas, and allows readers to explore topics of interest. Moreover, it situates your work within the continuum of academic knowledge.
To properly cite the sources used in the study, you can rely on online citation generator tools to generate accurate citations!
Here’s more on how you can cite your sources.
Often, the depth of research produces a wealth of material that, while crucial, can make the core content of the thesis cumbersome. The appendix is where you mention extra information that supports your research but isn't central to the main text.

Whether it's raw datasets, detailed procedural methodologies, extended case studies, or any other ancillary material, the appendices ensure that these elements are archived for reference without breaking the main narrative's flow.
For thorough researchers and readers keen on meticulous details, the appendices provide a treasure trove of insights.
Glossary (optional)
In academics, specialized terminologies, and jargon are inevitable. However, not every reader is versed in every term.
The glossary, while optional, is a critical tool for accessibility. It's a bridge ensuring that even readers from outside the discipline can access, understand, and appreciate your work.

By defining complex terms and providing context, you're inviting a wider audience to engage with your research, enhancing its reach and impact.
Remember, while these components provide a structured framework, the essence of your thesis lies in the originality of your ideas, the rigor of your research, and the clarity of your presentation.
As you craft each section, keep your readers in mind, ensuring that your passion and dedication shine through every page.
Thesis examples
To further elucidate the concept of a thesis, here are illustrative examples from various fields:
Example 1 (History): Abolition, Africans, and Abstraction: the Influence of the ‘Noble Savage’ on British and French Antislavery Thought, 1787-1807 by Suchait Kahlon.
Example 2 (Climate Dynamics): Influence of external forcings on abrupt millennial-scale climate changes: a statistical modelling study by Takahito Mitsui · Michel Crucifix
Checklist for your thesis evaluation
Evaluating your thesis ensures that your research meets the standards of academia. Here's an elaborate checklist to guide you through this critical process.
Content and structure
- Is the thesis statement clear, concise, and debatable?
- Does the introduction provide sufficient background and context?
- Is the literature review comprehensive, relevant, and well-organized?
- Does the methodology section clearly describe and justify the research methods?
- Are the results/findings presented clearly and logically?
- Does the discussion interpret the results in light of the research question and existing literature?
- Is the conclusion summarizing the research and suggesting future directions or implications?
Clarity and coherence
- Is the writing clear and free of jargon?
- Are ideas and sections logically connected and flowing?
- Is there a clear narrative or argument throughout the thesis?
Research quality
- Is the research question significant and relevant?
- Are the research methods appropriate for the question?
- Is the sample size (if applicable) adequate?
- Are the data analysis techniques appropriate and correctly applied?
- Are potential biases or limitations addressed?
Originality and significance
- Does the thesis contribute new knowledge or insights to the field?
- Is the research grounded in existing literature while offering fresh perspectives?
Formatting and presentation
- Is the thesis formatted according to institutional guidelines?
- Are figures, tables, and charts clear, labeled, and referenced in the text?
- Is the bibliography or reference list complete and consistently formatted?
- Are appendices relevant and appropriately referenced in the main text?
Grammar and language
- Is the thesis free of grammatical and spelling errors?
- Is the language professional, consistent, and appropriate for an academic audience?
- Are quotations and paraphrased material correctly cited?
Feedback and revision
- Have you sought feedback from peers, advisors, or experts in the field?
- Have you addressed the feedback and made the necessary revisions?
Overall assessment
- Does the thesis as a whole feel cohesive and comprehensive?
- Would the thesis be understandable and valuable to someone in your field?
Ensure to use this checklist to leave no ground for doubt or missed information in your thesis.
After writing your thesis, the next step is to discuss and defend your findings verbally in front of a knowledgeable panel. You’ve to be well prepared as your professors may grade your presentation abilities.
Preparing your thesis defense
A thesis defense, also known as "defending the thesis," is the culmination of a scholar's research journey. It's the final frontier, where you’ll present their findings and face scrutiny from a panel of experts.
Typically, the defense involves a public presentation where you’ll have to outline your study, followed by a question-and-answer session with a committee of experts. This committee assesses the validity, originality, and significance of the research.
The defense serves as a rite of passage for scholars. It's an opportunity to showcase expertise, address criticisms, and refine arguments. A successful defense not only validates the research but also establishes your authority as a researcher in your field.
Here’s how you can effectively prepare for your thesis defense .
Now, having touched upon the process of defending a thesis, it's worth noting that scholarly work can take various forms, depending on academic and regional practices.
One such form, often paralleled with the thesis, is the 'dissertation.' But what differentiates the two?
Dissertation vs. Thesis
Often used interchangeably in casual discourse, they refer to distinct research projects undertaken at different levels of higher education.
To the uninitiated, understanding their meaning might be elusive. So, let's demystify these terms and delve into their core differences.
Here's a table differentiating between the two.
Wrapping up
From understanding the foundational concept of a thesis to navigating its various components, differentiating it from a dissertation, and recognizing the importance of proper citation — this guide covers it all.
As scholars and readers, understanding these nuances not only aids in academic pursuits but also fosters a deeper appreciation for the relentless quest for knowledge that drives academia.
It’s important to remember that every thesis is a testament to curiosity, dedication, and the indomitable spirit of discovery.
Good luck with your thesis writing!
Frequently Asked Questions
A thesis typically ranges between 40-80 pages, but its length can vary based on the research topic, institution guidelines, and level of study.
A PhD thesis usually spans 200-300 pages, though this can vary based on the discipline, complexity of the research, and institutional requirements.
To identify a thesis topic, consider current trends in your field, gaps in existing literature, personal interests, and discussions with advisors or mentors. Additionally, reviewing related journals and conference proceedings can provide insights into potential areas of exploration.
The conceptual framework is often situated in the literature review or theoretical framework section of a thesis. It helps set the stage by providing the context, defining key concepts, and explaining the relationships between variables.
A thesis statement should be concise, clear, and specific. It should state the main argument or point of your research. Start by pinpointing the central question or issue your research addresses, then condense that into a single statement, ensuring it reflects the essence of your paper.
You might also like

AI for Meta-Analysis — A Comprehensive Guide
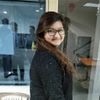
How To Write An Argumentative Essay

Beyond Google Scholar: Why SciSpace is the best alternative
Have a language expert improve your writing
Run a free plagiarism check in 10 minutes, generate accurate citations for free.
- Knowledge Base
- Dissertation
How to Write a Thesis or Dissertation Introduction
Published on September 7, 2022 by Tegan George and Shona McCombes. Revised on November 21, 2023.
The introduction is the first section of your thesis or dissertation , appearing right after the table of contents . Your introduction draws your reader in, setting the stage for your research with a clear focus, purpose, and direction on a relevant topic .
Your introduction should include:
- Your topic, in context: what does your reader need to know to understand your thesis dissertation?
- Your focus and scope: what specific aspect of the topic will you address?
- The relevance of your research: how does your work fit into existing studies on your topic?
- Your questions and objectives: what does your research aim to find out, and how?
- An overview of your structure: what does each section contribute to the overall aim?
Instantly correct all language mistakes in your text
Upload your document to correct all your mistakes in minutes
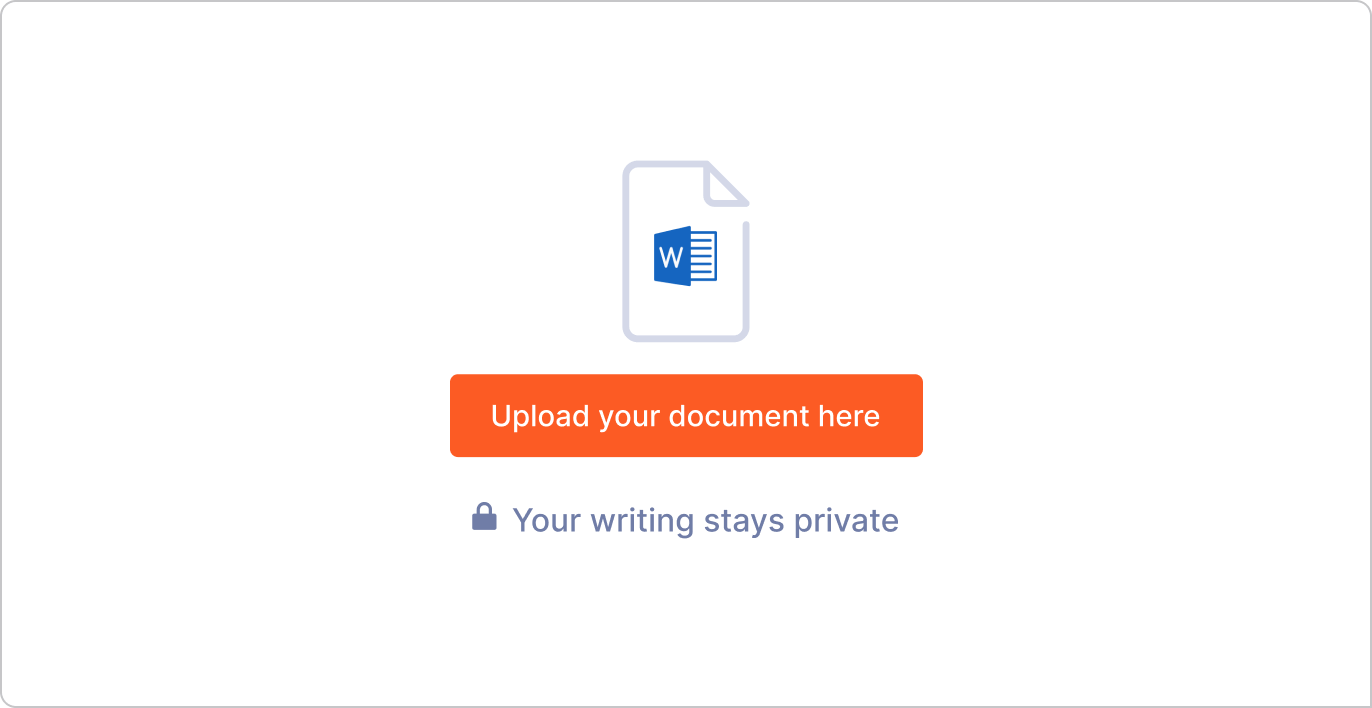
Table of contents
How to start your introduction, topic and context, focus and scope, relevance and importance, questions and objectives, overview of the structure, thesis introduction example, introduction checklist, other interesting articles, frequently asked questions about introductions.
Although your introduction kicks off your dissertation, it doesn’t have to be the first thing you write — in fact, it’s often one of the very last parts to be completed (just before your abstract ).
It’s a good idea to write a rough draft of your introduction as you begin your research, to help guide you. If you wrote a research proposal , consider using this as a template, as it contains many of the same elements. However, be sure to revise your introduction throughout the writing process, making sure it matches the content of your ensuing sections.
Receive feedback on language, structure, and formatting
Professional editors proofread and edit your paper by focusing on:
- Academic style
- Vague sentences
- Style consistency
See an example
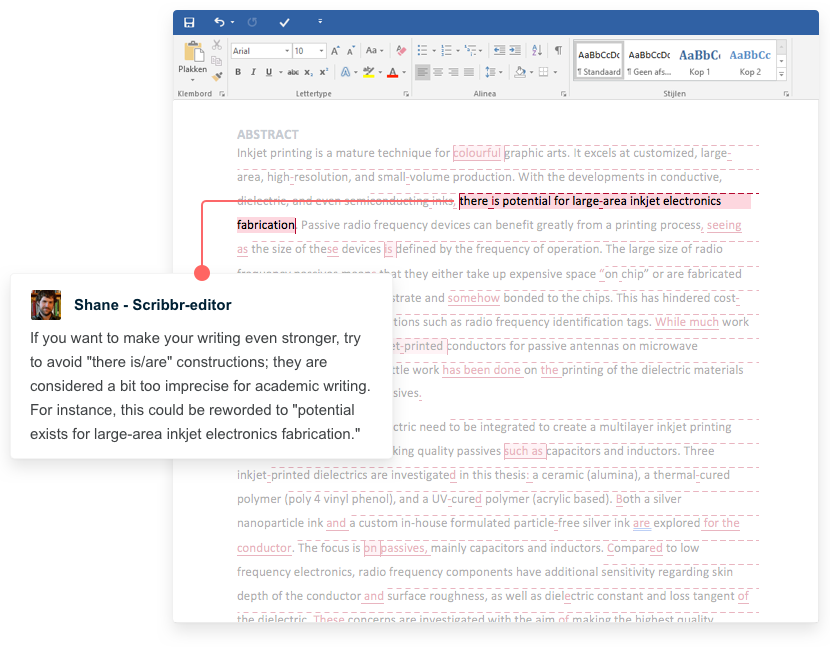
Begin by introducing your dissertation topic and giving any necessary background information. It’s important to contextualize your research and generate interest. Aim to show why your topic is timely or important. You may want to mention a relevant news item, academic debate, or practical problem.
After a brief introduction to your general area of interest, narrow your focus and define the scope of your research.
You can narrow this down in many ways, such as by:
- Geographical area
- Time period
- Demographics or communities
- Themes or aspects of the topic
It’s essential to share your motivation for doing this research, as well as how it relates to existing work on your topic. Further, you should also mention what new insights you expect it will contribute.
Start by giving a brief overview of the current state of research. You should definitely cite the most relevant literature, but remember that you will conduct a more in-depth survey of relevant sources in the literature review section, so there’s no need to go too in-depth in the introduction.
Depending on your field, the importance of your research might focus on its practical application (e.g., in policy or management) or on advancing scholarly understanding of the topic (e.g., by developing theories or adding new empirical data). In many cases, it will do both.
Ultimately, your introduction should explain how your thesis or dissertation:
- Helps solve a practical or theoretical problem
- Addresses a gap in the literature
- Builds on existing research
- Proposes a new understanding of your topic
Prevent plagiarism. Run a free check.
Perhaps the most important part of your introduction is your questions and objectives, as it sets up the expectations for the rest of your thesis or dissertation. How you formulate your research questions and research objectives will depend on your discipline, topic, and focus, but you should always clearly state the central aim of your research.
If your research aims to test hypotheses , you can formulate them here. Your introduction is also a good place for a conceptual framework that suggests relationships between variables .
- Conduct surveys to collect data on students’ levels of knowledge, understanding, and positive/negative perceptions of government policy.
- Determine whether attitudes to climate policy are associated with variables such as age, gender, region, and social class.
- Conduct interviews to gain qualitative insights into students’ perspectives and actions in relation to climate policy.
To help guide your reader, end your introduction with an outline of the structure of the thesis or dissertation to follow. Share a brief summary of each chapter, clearly showing how each contributes to your central aims. However, be careful to keep this overview concise: 1-2 sentences should be enough.
I. Introduction
Human language consists of a set of vowels and consonants which are combined to form words. During the speech production process, thoughts are converted into spoken utterances to convey a message. The appropriate words and their meanings are selected in the mental lexicon (Dell & Burger, 1997). This pre-verbal message is then grammatically coded, during which a syntactic representation of the utterance is built.
Speech, language, and voice disorders affect the vocal cords, nerves, muscles, and brain structures, which result in a distorted language reception or speech production (Sataloff & Hawkshaw, 2014). The symptoms vary from adding superfluous words and taking pauses to hoarseness of the voice, depending on the type of disorder (Dodd, 2005). However, distortions of the speech may also occur as a result of a disease that seems unrelated to speech, such as multiple sclerosis or chronic obstructive pulmonary disease.
This study aims to determine which acoustic parameters are suitable for the automatic detection of exacerbations in patients suffering from chronic obstructive pulmonary disease (COPD) by investigating which aspects of speech differ between COPD patients and healthy speakers and which aspects differ between COPD patients in exacerbation and stable COPD patients.
Checklist: Introduction
I have introduced my research topic in an engaging way.
I have provided necessary context to help the reader understand my topic.
I have clearly specified the focus of my research.
I have shown the relevance and importance of the dissertation topic .
I have clearly stated the problem or question that my research addresses.
I have outlined the specific objectives of the research .
I have provided an overview of the dissertation’s structure .
You've written a strong introduction for your thesis or dissertation. Use the other checklists to continue improving your dissertation.
If you want to know more about AI for academic writing, AI tools, or research bias, make sure to check out some of our other articles with explanations and examples or go directly to our tools!
Research bias
- Survivorship bias
- Self-serving bias
- Availability heuristic
- Halo effect
- Hindsight bias
- Deep learning
- Generative AI
- Machine learning
- Reinforcement learning
- Supervised vs. unsupervised learning
(AI) Tools
- Grammar Checker
- Paraphrasing Tool
- Text Summarizer
- AI Detector
- Plagiarism Checker
- Citation Generator
The introduction of a research paper includes several key elements:
- A hook to catch the reader’s interest
- Relevant background on the topic
- Details of your research problem
and your problem statement
- A thesis statement or research question
- Sometimes an overview of the paper
Don’t feel that you have to write the introduction first. The introduction is often one of the last parts of the research paper you’ll write, along with the conclusion.
This is because it can be easier to introduce your paper once you’ve already written the body ; you may not have the clearest idea of your arguments until you’ve written them, and things can change during the writing process .
Research objectives describe what you intend your research project to accomplish.
They summarize the approach and purpose of the project and help to focus your research.
Your objectives should appear in the introduction of your research paper , at the end of your problem statement .
Scope of research is determined at the beginning of your research process , prior to the data collection stage. Sometimes called “scope of study,” your scope delineates what will and will not be covered in your project. It helps you focus your work and your time, ensuring that you’ll be able to achieve your goals and outcomes.
Defining a scope can be very useful in any research project, from a research proposal to a thesis or dissertation . A scope is needed for all types of research: quantitative , qualitative , and mixed methods .
To define your scope of research, consider the following:
- Budget constraints or any specifics of grant funding
- Your proposed timeline and duration
- Specifics about your population of study, your proposed sample size , and the research methodology you’ll pursue
- Any inclusion and exclusion criteria
- Any anticipated control , extraneous , or confounding variables that could bias your research if not accounted for properly.
Cite this Scribbr article
If you want to cite this source, you can copy and paste the citation or click the “Cite this Scribbr article” button to automatically add the citation to our free Citation Generator.
George, T. & McCombes, S. (2023, November 21). How to Write a Thesis or Dissertation Introduction. Scribbr. Retrieved April 15, 2024, from https://www.scribbr.com/dissertation/introduction-structure/
Is this article helpful?
Tegan George
Other students also liked, how to choose a dissertation topic | 8 steps to follow, how to write an abstract | steps & examples, unlimited academic ai-proofreading.
✔ Document error-free in 5minutes ✔ Unlimited document corrections ✔ Specialized in correcting academic texts
Academia.edu no longer supports Internet Explorer.
To browse Academia.edu and the wider internet faster and more securely, please take a few seconds to upgrade your browser .
Enter the email address you signed up with and we'll email you a reset link.
- We're Hiring!
- Help Center
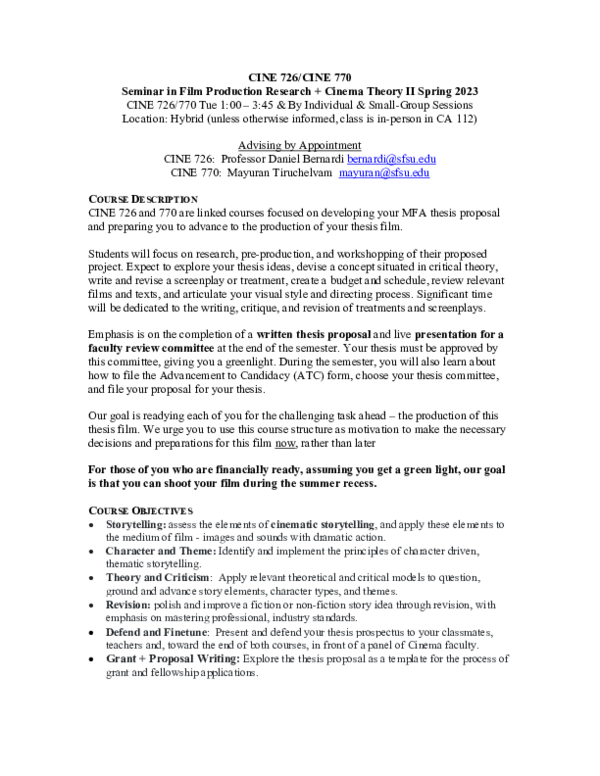
Syllabus: "Thesis Film Pre-production: Production and Theory"

CINE 726 and 770 are linked courses focused on developing your MFA thesis proposal and preparing you to advance to the production of your thesis film. Students will focus on research, pre-production, and workshopping of their proposed project. Expect to explore your thesis ideas, devise a concept situated in critical theory, write and revise a screenplay or treatment, create a budget and schedule, review relevant films and texts, and articulate your visual style and directing process. Significant time will be dedicated to the writing, critique, and revision of treatments and screenplays.
RELATED TOPICS
- We're Hiring!
- Help Center
- Find new research papers in:
- Health Sciences
- Earth Sciences
- Cognitive Science
- Mathematics
- Computer Science
- Academia ©2024
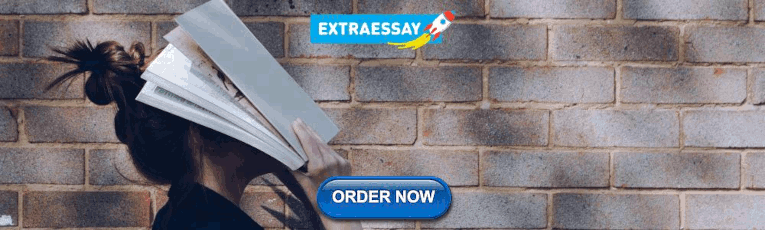
IMAGES
VIDEO
COMMENTS
The goal of this thesis is to design a future-state manufacturing platform capable of supporting JJV's shift toward a higher-mix, lower-volume product portfolio. Analysis focuses on rede ning the manufacturing process for one new product, ACUVUE® Theravision™with Ketotifen (Therav-
production. A methodology is proposed that helps to improve the productivity of any production process. The methodology consists of selecting a product or product family to be studied followed by current process study. Once the existing process is documented, all the assembly tasks involved must be timed using time study techniques.
This editable handout can help you make sense of the various steps involved in the production of your thesis or dissertation and determine how long each step might take. A large part of this process involves (1) seeking out the most accurate and up-to-date information regarding specific document formatting requirements, (2) understanding ...
The thesis proposed in the article is: the effective quality improvement based on implementation of the quality management method contributes to achieve the planned quality in the production ...
The industrial application of production planning and process scheduling optimization is addressed in this thesis. The rst part of the thesis addresses the research into process scheduling application. Several scheduling models are developed based on both discrete and continuous time modelling frameworks. Extensions to both frameworks are presented
This primary study on FrED production can be used to estimate the production required for a larger batch production, and further improve manufacturing processes to reduce the production time required thus resulting in a higher throughput rate of mass production in the future. Thesis Supervisor: Dr. Brian Anthony Title: Principal Research Scientist
1.1 Theory of Process Optimization. When starting process optimization, there first needs to be a base case process that. can be improved. In the case of the vapor-phase ethylbenzene production process, a base. case was given to work off of, but in most cases a base case will have to be created. Chapter.
processes in manufacturing need to be adjusted to successfully implement Industry 4.0. The purpose of this thesis is to contribute to successful Industry 4.0 adoption in the manufacturing process and therefore to contribute to technological advancement. Method This thesis conducts a multiple case study and gathers qualitative data by
In this thesis, we aim to fill in the gaps in studies related to production planning under uncertainty in a manufacturing environment. Through our study, we propose a two-stage stochastic programming framework for planning the production process at different stages in the supply chain.
The Thesis-Writing Process. Thesis research and writing need to satisfy several different institutional expectations, so planning is important. Here are the main phases of the process: ... followed by questions and answers. Thesis defense takes a chunk of time at the end of the term when time is short, so make plans for it. ## Thesis-Writing ...
The Bessemer process came to be the first efficient industrial process for steel mass production. There were significant innovations in manufacturing, and the machine tool industry was es- tablished. Rails and Maritime technologies, rubber and automobiles for transportation/logistics and electrification and engines/turbines for energy set forth ...
analyses the actual production system for one product. Production parameters such as throughput, cycle time, inventories in the production process, and idle time were investigated. This research paper is based on achieving two different goals. The first goal is to reduce production costs while maintaining the same production capacity.
This thesis seeks to find out the impact of Lean manufacturing (LM) on different sectors of process industries. The theory of this thesis was established mainly on published high-impact scholarly literature, such as books, journals, conferences and theses, as well as several online websites on the subject matters of LM.
The Thesis Process. The thesis is an opportunity to work independently on a research project of your own design and contribute to the scholarly literature in your field. You emerge from the thesis process with a solid understanding of how original research is executed and how to best communicate research results.
optimized production processes and optimally using resources present. Variability that exists in the manufacturing environment of such companies makes the planning and execution of the production process even more challenging. This thesis was conducted at Mars Inc., one of the biggest producers of candy bars and other chocolate products in the ...
In furtherance of emerging research within smart production planning and control (PPC), this paper prescribes a methodology for the design and development of a smart PPC system. A smart PPC system uses emerging technologies such as the internet of things, big-data analytics tools and machine learning running on the cloud or on edge devices to enhance performance of PPC processes. It achieves ...
a study of queuing theory in low to high rework environments with process availability, adam j. brown. pdf. economic modeling & optimization of a region specific multi-feedstock biorefinery supply chain, william h. faulkner. theses/dissertations from 2011 pdf
A thesis is an in-depth research study that identifies a particular topic of inquiry and presents a clear argument or perspective about that topic using evidence and logic. Writing a thesis showcases your ability of critical thinking, gathering evidence, and making a compelling argument. Integral to these competencies is thorough research ...
The conventional manufacturing process of making LIB electrodes consists of: (1) mixing active materials, conductive agent, and binder in a solvent, (2) casting the. slurry onto Al foil for making the positive electrode or Cu foil for making the negative.
1. Production and Rehearsal Processes For the Senior Thesis in Acting, the production process begins in the spring semester of the junior year, when the Acting thesis students audition for and are cast by the following year's directors. Thesis actors are expected to use the time between casting and the beginning of rehearsals to begin research and
Senior Thesis Design The Thesis in Performance: Design is comprised of the research, design, production, and rehearsal process, the final design, and a Casebook. 1. Production and Rehearsal Processes Designers are assigned in the junior year either to a faculty show, which can take place during either
The aim of this thesis is to understand the theory of making a prepreg, how manufactur-ers are doing it, and how to apply the same process to a smaller scale. Therefore, the objectives of the thesis are listed as follow: • Study the process line of making prepreg in the industry and the principles be-hind making a good prepreg.
The major five principles of Lean are as follows (Burton T. and Boeder, 2003, p. 122): Principle 1: Accurately specify value from customer perspective for both products and services. Principle 2: Identify the value stream for products and services and remove non-value-adding waste along the value stream.
Overview of the structure. To help guide your reader, end your introduction with an outline of the structure of the thesis or dissertation to follow. Share a brief summary of each chapter, clearly showing how each contributes to your central aims. However, be careful to keep this overview concise: 1-2 sentences should be enough.
CINE 726 and 770 are linked courses focused on developing your MFA thesis proposal and preparing you to advance to the production of your thesis film. Students will focus on research, pre-production, and workshopping of their proposed project. ... and articulate your visual style and directing process. Significant time will be dedicated to the ...
This Thesis is about the Process Engineering Design of manufacturing Bio-Ethanol by using Molasses which is a by-product of Sugar production. Discover the world's research 25+ million members