Careers in STEM: Why Should I Study Data Science?
Careers in data science are in high demand and offer high salaries and advancement opportunities. Learn five reasons to consider a career in the field.
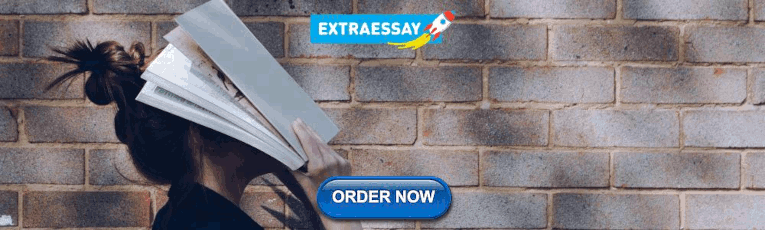
Valerie Kirk
Data is often referred to as the new gold because it has become an essential raw material.
From smartphones to traffic cameras to weather satellites, modern technology devices are collecting massive amounts of data that support everything from cancer research to city planning.
The importance of data in today’s world spans all industries including healthcare, education, travel, and government. Business decisions are made based on data and improving customer experiences relies on data. It is also critical for our national defense. Simply put, today’s world runs on data.
But unlike gold, data does not have value in its raw state. To tap into the power of data to make smart, data-driven decisions, it has to be collected, cleaned, organized, and analyzed.
This is why data is also called the new oil, which also needs to be extracted and refined in order to have value.
That’s where the field of data science comes in.
What is Data Science?
Data science is the study of data to extract meaningful insights for business and government.
People who pursue a degree in data science study math and computer science. Their career path includes jobs where they handle, organize, and interpret massive volumes of information with the goal of discerning patterns. They also construct complex algorithms to build predictive models. Data science tasks include data processing, data analytics, and data visualization.
Data scientists are on the leading edge of innovation and emerging technology, including machine learning and artificial intelligence, which relies on a significant amount of digital data to generate insights.
Careers in data science are growing fast. Data science jobs are in high demand and can be found in nearly every industry. A few of the most common data science jobs include:
- Chief Data Officer
- Artificial Intelligence Engineer
- Data Scientist
- Data Engineer
- Machine Learning Engineer
- Software Engineer
- Data Modeler
- Data Analyst
- Big Data Engineer
Why is Data Science Important?
Just as data is the new gold and the new oil, data is also the new currency. For businesses, the insights derived from data science are essential for data-driven decision-making. They guide everything from the product lifecycle to fulfillment to office or warehouse locations. Data scientists provide information that’s critical to a company’s growth.
The benefits of data science extend beyond business. Government agencies from the federal level down to state and local entities also rely on data insights for emergency planning and response, public safety, city planning, intelligence gathering, national defense, and many other services.
Another reason why data science is important? It taps into the potential of artificial intelligence, which can improve productivity and efficiencies, provide stronger cybersecurity, and personalize customer experiences. To be effective, artificial intelligence relies on a lot of data, which is often pulled from massive data repositories and organized and analyzed by data scientists.
Learn About Our Data Science Graduate Degree Program
5 Reasons to Study Data Science
The field of data science is a great career choice that offers high salaries, opportunities across several industries, and long-term job security. Here are five reasons to consider a career in data science.
1. Data Scientists Are in High Demand
According to the United States Bureau of Labor Statistics , data scientist jobs are projected to grow 36% by 2031, which is much faster than the average for all occupations. Data science careers also offer significant potential for advancement, with the relatively new role of chief data officer becoming a key C-suite position across all types of businesses.
Because the high-demand field requires a special skill set, professionals with data science degrees or certificates are more likely to land a desired position in a top company and enjoy more job security.
2. Careers in Data Science Have High Earning Potential
That high demand also leads to higher salaries relative to other careers. According to Glassdoor, the estimated total pay for a data scientist in the United States is $126,200 per year .
New data scientists can expect starting salaries of around $100,000 per year, with experienced data scientists earning more than $200,000 per year. The average annual salary for chief data officers is $636,000 , with top data executives clearing more than $1 million a year.
The salary potential is only expected to grow as data drives artificial intelligence innovations.
3. Data Science Skills are Going to Grow in Value
Think about this — smartphones, drones, satellites, sensors, security cameras, and other devices collect data 24 hours a day, seven days a week. Data is also being generated by organizations from every project, product launch, customer sale, employee action, and other business activities.
Then think about data that comes from every financial transaction, healthcare interaction, scholarly research project, and other initiatives outside of the business world. Data is continuously being generated from multiple sources for multiple uses — and that isn’t going to stop.
Turning all of that data into actionable insights is a unique, high-demand skill that will only grow in value as more data is generated. As technology advances, data scientists will be at the forefront of new breakthroughs and innovations. It’s an exciting and evolving career.
4. Data Science Provides a Wide Range of Job Opportunities
Every business, government agency, and educational institution generates data. They all need support in gaining insights from that data. Having a degree or certificate in data science gives people the flexibility to work in the industry that interests and inspires them.
5. Data Scientists Can Make the World a Better Place
While data scientists can offer insights to help businesses grow, they can also offer insights to help humanity. Data science careers include unique opportunities to make an impact on the world. Consider these initiatives where data science is playing a significant role:
- Climate change. To support climate control measures that could lower carbon dioxide emissions, the California Air Resources Board, Plant Labs, and the Environmental Defense Fund are working together on a Climate Data Partnership to track climate change from space.
- Medical research. The National Institutes of Health is working to improve biomedical research through its NIH Science and Technology Research Infrastructure for Discovery, Experimentation, and Sustainability Initiative , which enables access to rich datasets and breaking down data silos to support medical researchers.
- Rural planning. The U.S. Department of Agriculture launched a Rural Data Gateway to support farmers and ranchers in accessing the resources they need to support everything from sustainable farming practices to how to lower energy costs.
Other data-driven service-oriented initiatives include making cities safer for pedestrians and bikers, supporting affordable housing for underserved communities, and improving access to social services. Hear about other initiatives that are tapping into the power of AI for good in this fireside chat with Harvard Extension School’s director of IT programs, Bruce Huang.
Study Data Science at Harvard Extension School
If you are ready to start, advance, or pivot to a career in this exciting and growing field, Harvard Extension School offers a Data Science Master’s Degree Program .
The program focuses on mastering the technical, analytical, and practical skills needed to solve real-world, data-driven problems. The program covers predictive modeling, data mining, machine learning, artificial intelligence, data visualization, and big data. You will also learn how to apply data science and analytical methods to address data-rich problems and develop the skills for quantitative thought leadership, including the ethical and legal dimensions of data analytics.
The program includes 11 courses that can be taken online and one on-campus course, in which you develop a plan for a capstone project with peers and faculty. In the final capstone course, you will apply your new skills to a real-world challenge. Capstone project teams collaborate with industry, government, or academic institutions to explore the possibilities of using data science and analytics for good. Recent capstone projects include:
- Improving the climate change model used by NASA.
- Developing a tool that combines aerial imagery and advanced georeferencing techniques to assess damage in disaster-stricken areas.
- Using computer vision and video classification to develop a crime detection system for analyzing surveillance videos and identifying suspicious activities, contributing to enhanced public safety and crime prevention efforts.
- Predicting patient MRI scans in a hospital system to optimize resource allocation and ensure efficient patient care delivery.
- Streamlining the medical coding process to reduce errors and improve efficiencies.
You can also earn a Data Science Graduate Certificate through the Harvard Extension School . In this certificate program, you will:
- Master key facets of data investigation, including data wrangling, cleaning, sampling, management, exploratory analysis, regression and classification, prediction, and data communication
- Implement foundational concepts of data computation, such as data structure, algorithms, parallel computing, simulation, and analysis.
- Leverage your knowledge of key subject areas, such as game theory, statistical quality control, exponential smoothing, seasonally adjusted trend analysis, or data visualization.
Four courses are required for the program and vary based on the data science career path you are interested in pursuing.
If you are thinking about advancing your career or making a career change into the growing data science field, learn more about the Data Science Master’s Degree program or the Data Science Graduate Certificate program including class requirements, tuition, and how to apply.
About the Author
Valerie Kirk is a freelance writer and corporate storyteller specializing in customer and community outreach and topics and trends in education, technology, and healthcare. Based in Maryland near the Chesapeake Bay, she spends her free time exploring nature by bike, paddleboard, or on long hikes with her family.
Computer Science vs. Systems Engineering Programs — Which is Right for You?
This blog post explores the difference between the fields of computer science and systems engineering — and which might be right for you.
Harvard Division of Continuing Education
The Division of Continuing Education (DCE) at Harvard University is dedicated to bringing rigorous academics and innovative teaching capabilities to those seeking to improve their lives through education. We make Harvard education accessible to lifelong learners from high school to retirement.
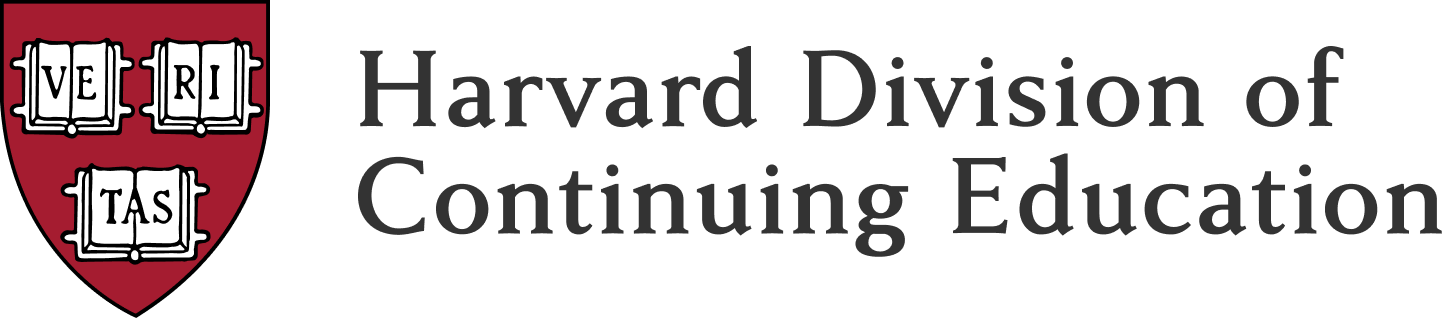
Free PDF: The 4 questions every SOP must answer → Master’s or PhD

The Data Science Statement of Purpose: A Guide with Examples
- By Jordan Dotson
- Updated: April 28, 2023

Ready to start writing your Data Science statement of purpose? Well, it’s your lucky day. This article isn’t just a “how to” guide — it’s an object lesson in so many of the common anxieties grad applicants face every year:
- How do I write about overcoming obstacles (health, low grades, family death) as an undergraduate?
- How do I describe my career path (I’ve been away from school for awhile)?
- Should I mention MOOCs in my SOP?
- Can I get admitted with no research experience?
- Can I get admitted if I wasn’t a Data Science or CS major in undergrad?
The sample essay you’re about to read and model is a perfect answer to these questions. Why? Because the applicant, despite his circuitous background and previous academic struggles, earned admission to 6 of the best MSDS programs in the US .
To protect the author’s privacy, we won’t name the schools. But rest assured, it’s a “Who’s Who” list of sterling, fancy-pants universities that you’re definitely also considering. Thus, this isn’t just a brilliant data science statement of purpose — it’s a brilliant SOP in general. The author employed this framework successfully for both DS and CS programs, and honestly, ANY applicant in ANY field can use this essay as inspiration…
…and hopefully achieve the same wild success as my fascinating friend, Bennett.
The Student
As an applicant, Bennett ticked a lot of boxes:
- First-gen college student and child of immigrants;
- Undergrad Cognitive Science major at elite state university;
- Modest, less-than-perfect GPA;
- Multiple DS certifications with supplemental CS coursework (essentially self-taught);
- Online Executive MBA graduate;
- 4 years of post-undergrad work experience;
- Extensive work experience during undergrad;
- ZERO research experience
Some aspects of Bennett’s profile were fascinating. (He was an NSA analyst in undergrad!) Other parts were fairly normal. (No research, average GPA.)
What then made Bennett and his SOP so special? What made top MSDS programs excited to admit him?
The Structure of a Successful Data Science SOP
It goes without saying that Bennett used the SOP Starter Kit to outline his essay. That means he structured the paragraphs as follows:
- Introduction Frame Narrative – 1 paragraph (12% of word count)
- Why This Program – 2 paragraphs (23% of word count)
- Why I’m Qualified – 4 paragraphs (58% of word count…extremely long, but more on this later)
- Concluding Frame Narrative – 1 paragraph (7% of word count)
Before we read the actual essay, let’s examine these sections and see how you can mirror Bennett’s example in your own SOP.
1. Introduction Frame Narrative
In the intro, Bennett describes his work as a software engineer. He gets specific. He tells us exactly what he does, and the company he does it for. Most importantly, he describes a moment when he discovered a new intellectual purpose at work:
“Thus, for the first time, I was able to personalize parameters in the pipeline for unaccounted customers. Learning the importance of context for efficient yet equitable automation, I found myself incredibly curious about data-modeling methodologies that can truly represent real-world situations .”
You should do the same as Bennett. Your intro should have some color, some life. It should allow us to see a real human being in there. But it MUST also introduce the sub-niche intellectual problems you hope to tackle in grad school. Chances are, these problems and this sub-niche will define your professional career afterward. They’re the hinge of your whole candidacy.
Common Question #1: “What if I don’t know which sub-niche I want to specialize in?”
Find one. (The SOP Starter Kit has an exercise that will help you figure this out.) Otherwise, you won’t be as competitive as you could be.
Common Question #2: “What if I don’t have an interesting moment (or moments) to write about?”
Stop lying to yourself. No matter where you are, no matter what you’ve done, there was a moment when you decided you needed a graduate degree. There is absolutely a subfield of data science that’s most interesting to you. There are undoubtedly specific applications, in specific industries, you want to work on in the future. How did you discover them?
Bennett wants to study representation of data minorities in ML models for the healthcare industry. That’s the work he wants to do in the future. What kind of work do you want to do in the future? When did you realize this?
That’s the story you tell in your Introduction.
2. Why This Program
Either at the end of your introduction, or in the beginning of this new section, you’re going to include a Sentence of Purpose . It’s a thesis statement for your essay. Bennett’s looks like this:
“Through Gotham University’s Master’s program in Data Science, I hope to further explore how to enhance representation of data minorities in ML models, and thus ensure inclusive healthcare access for the customers I serve.”
The “Why This Program” section of your SOP provides all the evidence for how you’ll pursue this goal in grad school. It should take about 2 paragraphs. Which classes will you take? Which professors do you hope to work with? What will you study in your capstone project?
Let’s make this easy. Just complete the exercise in this article: How to Dominate Your SOP’s Why This Program Section . Trust me, it’s that easy! Then, you’re halfway done with your essay.
3. Why I’m Qualified
This section of your SOP is the easiest to write. It’s your “greatest hits” list – all the proof that you’re a smart student. Everything you write here should support the argument that you’re going to succeed in grad school: your GPA, advanced classes you’ve taken, research experience, etc. It doesn’t have to be long, and shouldn’t include every menial detail of every project you’ve ever done. (That’s what the CV is for .)
Yet, if it shouldn’t be long…why did Bennett write 4 paragraphs?!
Typically, I’d yell and scream at an applicant who spends half the SOP talking about his past credentials. That’s what most applicants do, and why most get rejected .
But Bennett had a unique situation.
His career was wild and fascinating. He’d never formally studied Data Science. He’d even done an MBA. But he had taken lots of MOOCS and online certification courses (seriously, like 10+), he did have amazing experience as a software engineer, and he also had one bad undergrad semester he felt he needed to explain. Thus, he’s a very atypical applicant, and his background required a lot of explaining.
Unless you too have an MBA, 10+ MOOCs, and a completely unrelated major, then I suggest you keep your “Why I’m Qualified” section much shorter – 2 paragraphs is enough.
4. Concluding Frame Narrative
If you have the previous sections in order, this final paragraph should write itself. Make sure to reemphasize the topical problem (your hopeful subfield) from the Intro Frame Narrative. Consider including a career goals statement . But in the end, this section should be easy to write.
That’s it. Four sections, tightly interwoven, all supporting the argument that you are going to be an A+ data science grad student. Now, let’s see how Bennett brought it altogether, so you can attempt to do the same.
A Brilliant Data Science Statement of Purpose
As a software engineer with WayneHealth Group, I maintain data pipelines and batch processing in the modernization team. In 2020, following a health check on existing infrastructure, I discovered that pipelines were delivering data too slowly to clients. After comparing our runtimes to industry standards, I pitched a project using open-source Apache Airflow to help automate pipelines and centralize patient data into a single workflow. However, when considering how to automate 35% of the data, I learned from the billing team how frequently bills are refinanced in our long-term elderly care programs. Thus, for the first time, I was able to personalize parameters in the pipeline for unaccounted customers. Learning the importance of context for efficient yet equitable automation, I found myself incredibly curious about data-modeling methodologies that can truly represent real-world situations.
Through Gotham University’s Master’s program in Data Science, I hope to further explore how to enhance representation of data minorities in ML models, and thus ensure inclusive healthcare access for the customers I serve. Earning my MBA at Metropolis University taught me how to coordinate the need for quantitative reasoning and human intuition through A/B testing, and I believe the MSDS program will build on that foundation. Mathematical Foundations in Computer Science , for example, will help me build real-time analytics dashboards that account for insurance claim data-entry errors through discrete probabilistic models. In the same vein, elective offerings such as Big Data Analytics and Artificial Intelligence will enable me to choose predictive models and evaluate their accuracy when applied to large data sets — particularly useful when predicting whether an insurance claim will necessitate revisions.
Resources like the IGNITE competition will also offer opportunities to collaborate on flexible models that solve real-world situations. Having worked on Apache Airflow implementation in WayneHealth, I understand how collaboration can play a key role in implementing a new idea. Having my IGNITE team’s project evaluated by MSDS professors, with their expertise in modular design and user experience, will only help me evaluate my own performance as I translate my education into functional healthcare applications. Thus, I am certain that Gotham’s MSDS program will prepare me to succeed in a team setting that balances many developer roles, while equipping me to better deliver sales pitches to investors.
Upon graduating, I endeavor to apply my education toward applied healthcare projects that focus on providing easy access to preventative care. Transparency is an integral part of healthcare access because it reduces the expenses and time necessary to find patient care. To help facilitate this transparency, I plan to transition into a Senior Data Scientist role in the Emerging Technologies Collaborative (ETC) at WayneHealth, hopefully working on projects that implement data-driven recommendations for our automated batch processes and servers. When storing vast amounts of patient data across different platforms, vulnerability patches and triage alerts often lead to reactive outcomes that can create downtime for end users. As a result, I seek to implement agentless server monitoring to, first, predict unscheduled outages for our billing and medical coverage systems, and second, recognize patterns in server behavior. Helping recognize outage patterns will not only help me identify problems beforehand, but also decipher the causes of live servers crashing. However, projects outside of WayneHealth excite me as well, including Amgen’s Crystal Bone algorithm which uses AI and machine-learning models to detect bones at risk for osteoporotic fractures. This project was the first tool I have ever seen that uses diagnostic codes sourced from WayneHealth electronic health records, and it inspired me to create my own model using EHR data. In the future, I hope to use EHR diagnostic codes to predict the cost of treatment for those prone to risk, as indicated by the algorithm.
Not only has my position as a software engineer equipped me with strong technical skills, but it has also given me the discipline to continuously learn what I do not yet know. On a project named Karra, an optical-character recognition engine which scans personal information from faxed hospital claim forms, I learned how to develop my own algorithms to calculate the coordinates of form fields to parse data. The technical skills I have gained, in tandem with the unwavering tenacity I developed in this position, will allow me to face any challenge that arises during the MSDS program.
To further prepare for the rigors of the MSDS program, I completed University of Pennsylvania Engineering MOOCs on Coursera, including the Introduction to Python and Java specialization taught by Brandon Krakowsky and the course Computational Thinking for Problem Solving by Susan Davidson. These MOOCs helped me comprehend important programming paradigms such as unit testing and debugging, which will help me test edge cases in MSDS course projects. Also, MOOCs from UC San Diego, such as Python for Data Science and Probability and Statistics in Data Science Using Python , enabled me to optimize data-cleansing techniques for better runtimes. The MCDS program’s Big Data Analytics course will culminate this self-learning effort, providing a solid theoretical understanding of the tools and techniques used to extract insights from large datasets.
While I have taken on a breadth of challenging problems in computer science and implemented solutions at WayneHealth, my prior undergraduate performance did not always reflect my best ability. Between Spring 2016 and Spring 2017, I experienced a personal health challenge that required substantial time away from the UC Coast City campus. I was further distracted by the realities of personally financing my education – working full-time for the National Security Agency (NSA) – while also suffering the loss of a close family member. Even as I struggled I knew the importance of higher education, and, advocating for my own success, I persisted. To strengthen my educational background, I enrolled in online courses and built coping mechanisms, such as managing my time between online courses and on-campus courses efficiently. In the end, these efforts helped me graduate early in the fall of 2018, and I plan to apply the same level of resilience throughout the rest of my academic and professional career.
As I grow increasingly aware of the intersection between ML and social computing, I am determined to study learning techniques such as principal component analysis, and to perform research in data organization/completeness. With my strong self-guided background in applied computer science, and my professional experience with ML and software development in the healthcare insurance industry, the practical knowledge I build at Gotham will help me make voices heard in the data we interact with in our daily lives.
What Makes This SOP Truly Special?
Some might argue that Bennett’s essay doesn’t fit the template described in the SOP Starter Kit. I disagree. The virtuosity of Bennett’s writing shows that the model is adaptable to all kinds of intellectual demands.
(In fact, he’s pointed out himself that the framework helped beautifully with his Computer Science SOPs, which should give confidence to anyone who may be still deciding between DS and CS.)
Personally, I love how Bennett began his Why I’m Qualified section with an expanded Career Goals Statement. It shows us, in painstaking detail, exactly what he’s going to achieve if the school admits him:
“Upon graduating, I endeavor to apply my education toward applied healthcare projects that focus on providing easy access to preventative care.”
There are real data problems in the healthcare insurance industry. Bennett is all-too-familiar with them. Few if any other applicants will ever be able to solve these problems the way he will. We know this because he tells us exactly what he’s going to do in his career afterward :
- Pursue a Senior Data Scientist role in his company;
- Automate batch processes and servers to predict unscheduled outages medical coverage systems;
- And use EHR diagnostic codes to predict treatment costs for high-risk patients.
In this way, Bennett’s expansive, thoughtful SOP makes certain that he isn’t just a boring applicant looking to acquire base knowledge in data science. He already has it! He got it for free from Coursera!
Instead, it shows that he’s deadly focused on his unique sub-niche — solving real data problems in the healthcare insurance industry — and will do everything it takes to succeed. Thus, when Bennett discusses the many obstacles he overcame in the past, we don’t worry about them. We have tremendous confidence in Bennett because he’s already succeeded. He’s already acquired great expertise. And he knows exactly what he needs to do to make an impact in the future.
Though the middle paragraphs are somewhat long, they never feel boring or clunky. They feel intelligent and interesting. Finally, when we get to the last paragraph, we can’t help feeling certain of one thing: “Wow, this guy is unstoppable.”
As you start planning your own data science statement of purpose, there’s one aspect of Bennett’s essay you should mimic. It’s not the MOOCs , his atypical background, or the obstacles he overcame. It’s this:
Bennett mapped out the intellectual problems he wants to study in grad school, and how he will address them pragmatically in his career afterward. It’s not a complex argument:
- In the last few years, I’ve grown fascinated with Problem X in Industry Z;
- At Gotham University, I plan to study Problem X in these specific ways;
- After graduating, I will be able to solve Problem X for companies in Industry Z;
- I know I’m capable of this because of my skills and record of success;
- Admission to Gotham is my immediate and necessary next step, so I hope we can begin solving these problems together.
I offer endless gratitude to Bennett for allowing me to share his story, his brilliant essay, and his resounding success. Data Science, Analytics, and Applied Statistics have become insanely competitive. But if you take the time to follow his example, you too can become a champion in the field, and start your journey toward solving unique problems that the world desperately needs you to solve.
Still need help structuring your own data science statement of purpose? The SOP Starter Kit will help, or, I’d love to hear from you !
Which data science problems do you plan to solve in grad school and beyond?
Need advice on other application essays? Check out our free guides!
- Structure is Magic: A Guide to the Graduate SOP
- Statement of Purpose for PhD Admission: A Universal Formula
- Diversity Statements 101: A Guide to All Personal Essays
Was this post helpful? Spread the love:
The sop starter kits.
These FREE (and highly insightful) guides will tell you exactly what to write, step-by-step, and leave you feeling super-confident and ready to hit “submit.”

© 2022 WriteIvy
[ninja_form id=3]
The Data Scientist
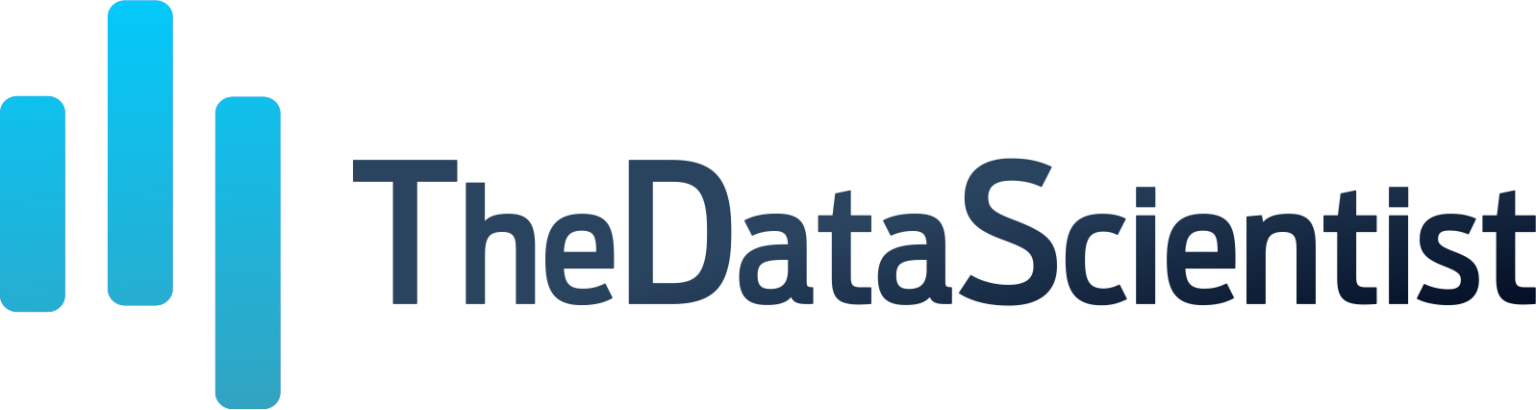
a well-structured essay can distinguish between a reader grasping the essence of data findings and being overwhelmed by the technical details. Here’s how to structure your data science essays to enhance clarity and impact.
- April 24, 2024 April 24, 2024
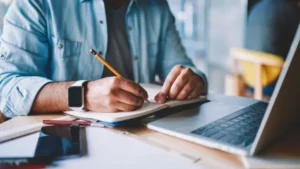
Leave a Reply Cancel reply
Your email address will not be published. Required fields are marked *
Save my name, email, and website in this browser for the next time I comment.
Advertisement
Data science: a game changer for science and innovation
- Regular Paper
- Open access
- Published: 19 April 2021
- Volume 11 , pages 263–278, ( 2021 )
Cite this article
You have full access to this open access article
- Valerio Grossi 1 ,
- Fosca Giannotti 1 ,
- Dino Pedreschi 2 ,
- Paolo Manghi 3 ,
- Pasquale Pagano 3 &
- Massimiliano Assante 3
13k Accesses
19 Citations
57 Altmetric
Explore all metrics
This paper shows data science’s potential for disruptive innovation in science, industry, policy, and people’s lives. We present how data science impacts science and society at large in the coming years, including ethical problems in managing human behavior data and considering the quantitative expectations of data science economic impact. We introduce concepts such as open science and e-infrastructure as useful tools for supporting ethical data science and training new generations of data scientists. Finally, this work outlines SoBigData Research Infrastructure as an easy-to-access platform for executing complex data science processes. The services proposed by SoBigData are aimed at using data science to understand the complexity of our contemporary, globally interconnected society.
Similar content being viewed by others
What Is Data Science?
Data science.
Responsible Data Science in a Dynamic World
Avoid common mistakes on your manuscript.
1 Introduction: from data to knowledge
Data science is an interdisciplinary and pervasive paradigm where different theories and models are combined to transform data into knowledge (and value). Experiments and analyses over massive datasets are functional not only to the validation of existing theories and models but also to the data-driven discovery of patterns emerging from data, which can help scientists in the design of better theories and models, yielding a deeper understanding of the complexity of the social, economic, biological, technological, cultural, and natural phenomenon. The products of data science are the result of re-interpreting available data for analysis goals that differ from the original reasons motivating data collection. All these aspects are producing a change in the scientific method, in research and in the way our society makes decisions [ 2 ].
Data science emerges to concurring facts: (i) the advent of big data that provides the critical mass of actual examples to learn from, (ii) the advances in data analysis and learning techniques that can produce predictive models and behavioral patterns from big data, and (iii) the advances in high-performance computing infrastructures that make it possible to ingest and manage big data and perform complex analysis [ 16 ].
Paper organization Section 2 discusses how data science impacts our science and society at large in the coming years. Section 3 outlines the main issues related to the ethical problems in studying human behaviors that data science introduces. In Sect. 4 , we show how concepts such as open science and e-infrastructure are effective tools for supporting, disseminating ethical uses of the data, and training new generations of data scientists. We will illustrate the importance of an open data science with examples provided later in the paper. Finally, we show some use cases of data science through thematic environments that bind the datasets with social mining methods.
2 Data science for society, science, industry and business
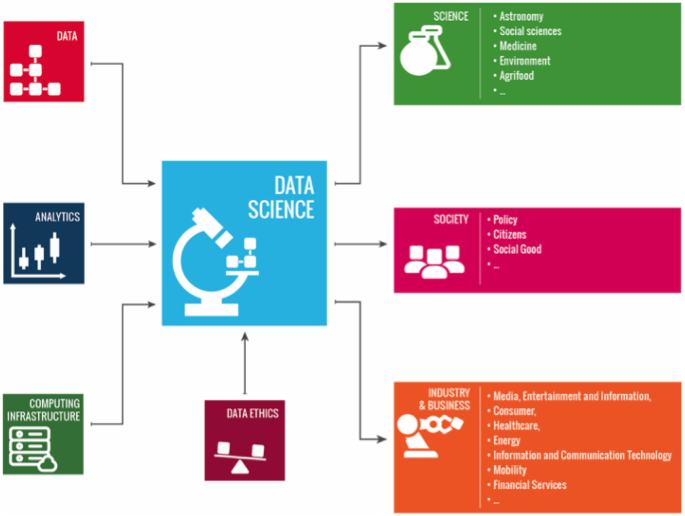
Data science as an ecosystem: on the left, the figure shows the main components enabling data science (data, analytical methods, and infrastructures). On the right, we can find the impact of data science into society, science, and business. All the activities related to data science should be done under rigid ethical principles
The quality of business decision making, government administration, and scientific research can potentially be improved by analyzing data. Data science offers important insights into many complicated issues, in many instances, with remarkable accuracy and timeliness.
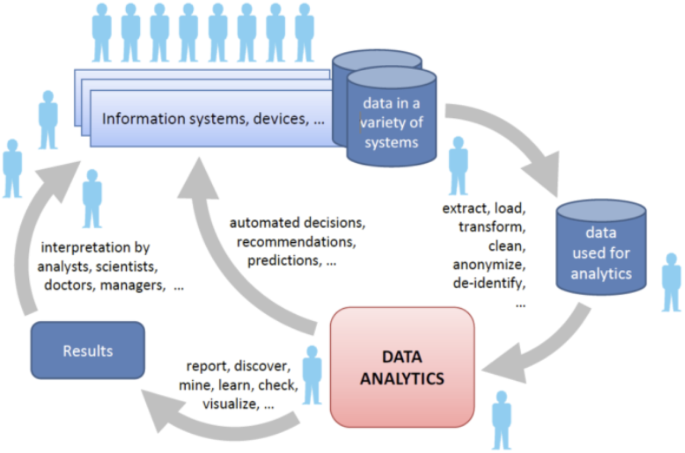
The data science pipeline starts with raw data and transforms them into data used for analytics. The next step is to transform these data into knowledge through analytical methods and then provide results and evaluation measures
As shown in Fig. 1 , data science is an ecosystem where the following scientific, technological, and socioeconomic factors interact:
Data Availability of data and access to data sources;
Analytics & computing infrastructures Availability of high performance analytical processing and open-source analytics;
Skills Availability of highly and rightly skilled data scientists and engineers;
Ethical & legal aspects Availability of regulatory environments for data ownership and usage, data protection and privacy, security, liability, cybercrime, and intellectual property rights;
Applications Business and market ready applications;
Social aspects Focus on major societal global challenges.
Data science envisioned as the intersection between data mining, big data analytics, artificial intelligence, statistical modeling, and complex systems is capable of monitoring data quality and analytical processes results transparently. If we want data science to face the global challenges and become a determinant factor of sustainable development, it is necessary to push towards an open global ecosystem for science, industrial, and societal innovation [ 48 ]. We need to build an ecosystem of socioeconomic activities, where each new idea, product, and service create opportunities for further purposes, and products. An open data strategy, innovation, interoperability, and suitable intellectual property rights can catalyze such an ecosystem and boost economic growth and sustainable development. This strategy also requires a “networked thinking” and a participatory, inclusive approach.
Data are relevant in almost all the scientific disciplines, and a data-dominated science could lead to the solution of problems currently considered hard or impossible to tackle. It is impossible to cover all the scientific sectors where a data-driven revolution is ongoing; here, we shall only provide just a few examples.
The Sloan Digital Sky Survey Footnote 1 has become a central resource for astronomers over the world. Astronomy is being transformed from the one where taking pictures of the sky was a large part of an astronomer’s job, to the one where the images are already in a database, and the astronomer’s task is to find interesting objects and phenomenon in the database. In biological sciences, data are stored in public repositories. There is an entire discipline of bioinformatics that is devoted to the analysis of such data. Footnote 2 Data-centric approaches based on personal behaviors can also support medical applications analyzing data at both human behavior levels and lower molecular ones. For example, integrating genome data of medical reactions with the habits of the users, enabling a computational drug science for high-precision personalized medicine. In humans, as in other organisms, most cellular components exert their functions through interactions with other cellular components. The totality of these interactions (representing the human “interactome”) is a network with hundreds of thousand nodes and a much larger number of links. A disease is rarely a consequence of an abnormality in a single gene. Instead, the disease phenotype is a reflection of various pathological processes that interact in a complex network. Network-based approaches can have multiple biological and clinical applications, especially in revealing the mechanisms behind complex diseases [ 6 ].
Now, we illustrate the typical data science pipeline [ 50 ]. People, machines, systems, factories, organizations, communities, and societies produce data. Data are collected in every aspect of our life, when: we submit a tax declaration; a customer orders an item online; a social media user posts a comment; a X-ray machine is used to take a picture; a traveler sends a review on a restaurant; a sensor in a supply chain sends an alert; or a scientist conducts an experiment. This huge and heterogeneous quantity of data needs to be extracted, loaded, understood, transformed, and in many cases, anonymized before they may be used for analysis. Analysis results include routines, automated decisions, predictions, and recommendations, and outcomes that need to be interpreted to produce actions and feedback. Furthermore, this scenario must also consider ethical problems in managing social data. Figure 2 depicts the data science pipeline. Footnote 3 Ethical aspects are important in the application of data science in several sectors, and they are addressed in Sect. 3 .
2.1 Impact on society
Data science is an opportunity for improving our society and boosting social progress. It can support policymaking; it offers novel ways to produce high-quality and high-precision statistical information and empower citizens with self-awareness tools. Furthermore, it can help to promote ethical uses of big data.
Modern cities are perfect environments densely traversed by large data flows. Using traffic monitoring systems, environmental sensors, GPS individual traces, and social information, we can organize cities as a collective sharing of resources that need to be optimized, continuously monitored, and promptly adjusted when needed. It is easy to understand the potentiality of data science by introducing terms such as urban planning , public transportation , reduction of energy consumption , ecological sustainability, safety , and management of mass events. These terms represent only the front line of topics that can benefit from the awareness that big data might provide to the city stakeholders [ 22 , 27 , 29 ]. Several methods allowing human mobility analysis and prediction are available in the literature: MyWay [ 47 ] exploits individual systematic behaviors to predict future human movements by combining individual and collective learned models. Carpooling [ 22 ] is based on mobility data from travelers in a given territory and constructs a network of potential carpooling users, by exploiting topological properties, highlighting sub-populations with higher chances to create a carpooling community and the propensity of users to be either drivers or passengers in a shared car. Event attendance prediction [ 13 ] analyzes users’ call habits and classifies people into behavioral categories, dividing them among residents, commuters, and visitors and allows to observe the variety of behaviors of city users and the attendance in big events in cities.
Electric mobility is expected to gain importance for the world. The impact of a complete switch to electric mobility is still under investigation, and what appears to be critical is the intensity of flows due to charge (and fast recharge) systems that may challenge the stability of the power network. To avoid instabilities regarding the charging infrastructure, an accurate prediction of power flows associated with mobility is needed. The use of personal mobility data can estimate the mobility flow and simulate the impact of different charging behavioral patterns to predict power flows and optimize the position of the charging infrastructures [ 25 , 49 ]. Lorini et al. [ 26 ] is an example of an urban flood prediction that integrates data provided by CEM system Footnote 4 and Twitter data. Twitter data are processed using massive multilingual approaches for classification. The model is a supervised model which requires a careful data collection and validation of ground truth about confirmed floods from multiple sources.
Another example of data science for society can be found in the development of applications with functions aimed directly at the individual. In this context, concepts such as personal data stores and personal data analytics are aimed at implementing a new deal on personal data, providing a user-centric view where data are collected, integrated and analyzed at the individual level, and providing the user with better awareness of own behavioral, health, and consumer profiles. Within this user-centric perspective, there is room for an even broader market of business applications, such as high-precision real-time targeted marketing, e.g., self-organizing decision making to preserve desired global properties, and sustainability of the transportation or the healthcare system. Such contexts emphasize two essential aspects of data science: the need for creativeness to exploit and combine the several data sources in novel ways and the need to give awareness and control of the personal data to the users that generate them, to sustain a transparent, trust-based, crowd-sourced data ecosystem [ 19 ].
The impact of online social networks in our society has changed the mechanisms behind information spreading and news production. The transformation of media ecosystems and news consumption are having consequences in several fields. A relevant example is the impact of misinformation on society, as for the Brexit referendum when the massive diffusion of fake news has been considered one of the most relevant factors of the outcome of this political event. Examples of achievements are provided by the results regarding the influence of external news media on polarization in online social networks. These achievements indicate that users are highly polarized towards news sources, i.e., they cite (and tend to cite) sources that they identify as ideologically similar to them. Other results regard echo chambers and the role of social media users: there is a strong correlation between the orientation of the content produced and consumed. In other words, an opinion “echoes” back to the user when others are sharing it in the “chamber” (i.e., the social network around the user) [ 36 ]. Other results worth mentioning regard efforts devoted to uncovering spam and bot activities in stock microblogs on Twitter: taking inspiration from biological DNA, the idea is to model the online users’ behavior through strings of characters representing sequences of online users’ actions. As a result of the following papers, [ 11 , 12 ] report that 71% of suspicious users were classified as bots; furthermore, 37% of them also got suspended by Twitter few months after our investigation. Several approaches can be found in the literature. However, they generally display some limitations. Some of them work only on some of the features of the diffusion of misinformation (bot detections, segregation of users due to their opinions or other social analysis), or there is a lack of comprehensive frameworks for interpreting results. While the former case is somehow due to the innovation of the research field and it is explainable, the latter showcases a more fundamental need, as, without strict statistical validation, it is hard to state which are the crucial elements that permit a well-grounded description of a system. For avoiding fake news diffusion, we can state that building a comprehensive fake news dataset providing all information about publishers, shared contents, and the engagements of users over space and time, together with their profile stories, can help the development of innovative and effective learning models. Both unsupervised and supervised methods will work together to identify misleading information. Multidisciplinary teams made up of journalists, linguists, and behavioral scientists and similar will be needed to identify what amounts to information warfare campaigns. Cyberwarfare and information warfare will be two of the biggest threats the world will face in the 21st Century.
Social sensing methods collect data produced by digital citizens, by either opportunistic or participatory crowd-sensing, depending on users’ awareness of their involvement. These approaches present a variety of technological and ethical challenges. An example is represented by Twitter Monitor [ 10 ], that is crowd-sensing tool designed to access Twitter streams through the Twitter Streaming API. It allows launching parallel listening for collecting different sets of data. Twitter Monitor represents a tool for creating services for listening campaigns regarding relevant events such as political elections, natural and human-made disasters, popular national events, etc. [ 11 ]. This campaign can be carried out, specifying keywords, accounts, and geographical areas of interest.
Nowcasting Footnote 5 financial and economic indicators focus on the potential of data science as a proxy for well-being and socioeconomic applications. The development of innovative research methods has demonstrated that poverty indicators can be approximated by social and behavioral mobility metrics extracted from mobile phone data and GPS data [ 34 ]; and the Gross Domestic Product can be accurately nowcasted by using retail supermarket market data [ 18 ]. Furthermore, nowcasting of demographic aspects of territory based on Twitter data [ 1 ] can support official statistics, through the estimation of location, occupation, and semantics. Networks are a convenient way to represent the complex interaction among the elements of a large system. In economics, networks are gaining increasing attention because the underlying topology of a networked system affects the aggregate output, the propagation of shocks, or financial distress; or the topology allows us to learn something about a node by looking at the properties of its neighbors. Among the most investigated financial and economic networks, we cite a work that analyzes the interbank systems, the payment networks between firms, the banks-firms bipartite networks, and the trading network between investors [ 37 ]. Another interesting phenomenon is the advent of blockchain technology that has led to the innovation of bitcoin crypto-currency [ 31 ].
Data science is an excellent opportunity for policy, data journalism, and marketing. The online media arena is now available as a real-time experimenting society for understanding social mechanisms, like harassment, discrimination, hate, and fake news. In our vision, the use of data science approaches is necessary for better governance. These new approaches integrate and change the Official Statistics representing a cheaper and more timely manner of computing them. The impact of data science-driven applications can be particularly significant when the applications help to build new infrastructures or new services for the population.
The availability of massive data portraying soccer performance has facilitated recent advances in soccer analytics. Rossi et al. [ 42 ] proposed an innovative machine learning approach to the forecasting of non-contact injuries for professional soccer players. In [ 3 ], we can find the definition of quantitative measures of pressing in defensive phases in soccer. Pappalardo et al. [ 33 ] outlined the automatic and data-driven evaluation of performance in soccer, a ranking system for soccer teams. Sports data science is attracting much interest and is now leading to the release of a large and public dataset of sports events.
Finally, data science has unveiled a shift from population statistics to interlinked entities statistics, connected by mutual interactions. This change of perspective reveals universal patterns underlying complex social, economic, technological, and biological systems. It is helpful to understand the dynamics of how opinions, epidemics, or innovations spread in our society, as well as the mechanisms behind complex systemic diseases, such as cancer and metabolic disorders revealing hidden relationships between them. Considering diffusive models and dynamic networks, NDlib [ 40 ] is a Python package for the description, simulation, and observation of diffusion processes in complex networks. It collects diffusive models from epidemics and opinion dynamics and allows a scientist to compare simulation over synthetic systems. For community discovery, two tools are available for studying the structure of a community and understand its habits: Demon [ 9 ] extracts ego networks (i.e., the set of nodes connected to an ego node) and identifies the real communities by adopting a democratic, bottom-up merging approach of such structures. Tiles [ 41 ] is dedicated to dynamic network data and extracts overlapping communities and tracks their evolution in time following an online iterative procedure.
2.2 Impact on industry and business
Data science can create an ecosystem of novel data-driven business opportunities. As a general trend across all sectors, massive quantities of data will be made accessible to everybody, allowing entrepreneurs to recognize and to rank shortcomings in business processes, to spot potential threads and win-win situations. Ideally, every citizen could establish from these patterns new business ideas. Co-creation enables data scientists to design innovative products and services. The value of joining different datasets is much larger than the sum of the value of the separated datasets by sharing data of various nature and provenance.
The gains from data science are expected across all sectors, from industry and production to services and retail. In this context, we cite several macro-areas where data science applications are especially promising. In energy and environment , the digitization of the energy systems (from production to distribution) enables the acquisition of real-time, high-resolution data. Coupled with other data sources, such as weather data, usage patterns, and market data (accompanied by advanced analytics), efficiency levels can be increased immensely. The positive impact to the environment is also enhanced by geospatial data that help to understand how our planet and its climate are changing and to confront major issues such as global warming, preservation of the species, the role and effects of human activities.
The manufacturing and production sector with the growing investments into Industry 4.0 and smart factories with sensor-equipped machinery that are both intelligent and networked (see internet of things . Cyber-physical systems ) will be one of the major producers of data in the world. The application of data science into this sector will bring efficiency gains and predictive maintenance. Entirely new business models are expected since the mass production of individualized products becomes possible where consumers may have direct access to influence and control.
As already stated in Sect. 2.1 , data science will contribute to increasing efficiency in public administrations processes and healthcare. In the physical and the cyber-domain, security will be enhanced. From financial fraud to public security, data science will contribute to establishing a framework that enables a safe and secure digital economy. Big data exploitation will open up opportunities for innovative, self-organizing ways of managing logistical business processes. Deliveries could be based on predictive monitoring, using data from stores, semantic product memories, internet forums, and weather forecasts, leading to both economic and environmental savings. Let us also consider the impact of personalized services for creating real experiences for tourists. The analysis of real-time and context-aware data (with the help of historical and cultural heritage data) will provide customized information to each tourist, and it will contribute to the better and more efficient management of the whole tourism value chain.
3 Data science ethics
Data science creates great opportunities but also new risks. The use of advanced tools for data analysis could expose sensitive knowledge of individual persons and could invade their privacy. Data science approaches require access to digital records of personal activities that contain potentially sensitive information. Personal information can be used to discriminate people based on their presumed characteristics. Data-driven algorithms yield classification and prediction models of behavioral traits of individuals, such as credit score, insurance risk, health status, personal preferences, and religious, ethnic, or political orientation, based on personal data disseminated in the digital environment by users (with or often without their awareness). The achievements of data science are the result of re-interpreting available data for analysis goals that differ from the original reasons motivating data collection. For example, mobile phone call records are initially collected by telecom operators for billing and operational aims, but they can be used for accurate and timely demography and human mobility analysis at a country or regional scale. This re-purposing of data clearly shows the importance of legal compliance and data ethics technologies and safeguards to protect privacy and anonymity; to secure data; to engage users; to avoid discrimination and misuse; to account for transparency; and to the purpose of seizing the opportunities of data science while controlling the associated risks.
Several aspects should be considered to avoid to harm individual privacy. Ethical elements should include the: (i) monitoring of the compliance of experiments, research protocols, and applications with ethical and juridical standards; (ii) developing of big data analytics and social mining tools with value-sensitive design and privacy-by-design methodologies; (iii) boosting of excellence and international competitiveness of Europe’s big data research in safe and fair use of big data for research. It is essential to highlight that data scientists using personal and social data also through infrastructures have the responsibility to get acquainted with the fundamental ethical aspects relating to becoming a “data controller.” This aspect has to be considered to define courses for informing and training data scientists about the responsibilities, the possibilities, and the boundaries they have in data manipulation.
Recalling Fig. 2 , it is crucial to inject into the data science pipeline the ethical values of fairness : how to avoid unfair and discriminatory decisions; accuracy : how to provide reliable information; confidentiality : how to protect the privacy of the involved people and transparency : how to make models and decisions comprehensible to all stakeholders. This value-sensitive design has to be aimed at boosting widespread social acceptance of data science, without inhibiting its power. Finally, it is essential to consider also the impact of the General Data Protection Regulation (GDPR) on (i) companies’ duties and how these European companies should comply with the limits in data manipulation the Regulation requires; and on (ii) researchers’ duties and to highlight articles and recitals which specifically mention and explain how research is intended in GDPR’s legal system.
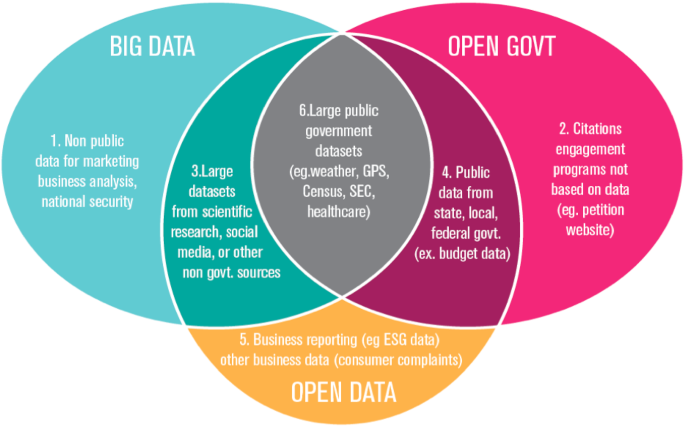
The relationship between big and open data and how they relate to the broad concept of open government
We complete this section with another important aspect related to open data, i.e., accessible public data that people, companies, and organizations can use to launch new ventures, analyze patterns and trends, make data-driven decisions, and solve complex problems. All the definitions of open data include two features: (i) the data must be publicly available for anyone to use, and (ii) data must be licensed in a way that allows for its reuse. All over the world, initiatives are launched to make data open by government agencies and public organizations; listing them is impossible, but an UN initiative has to be mentioned. Global Pulse Footnote 6 meant to implement the vision for a future in which big data is harnessed safely and responsibly as a public good.
Figure 3 shows the relationships between open data and big data. Currently, the problem is not only that government agencies (and some business companies) are collecting personal data about us, but also that we do not know what data are being collected and we do not have access to the information about ourselves. As reported by the World Economic forum in 2013, it is crucial to understand the value of personal data to let the users make informed decisions. A new branch of philosophy and ethics is emerging to handle personal data related issues. On the one hand, in all cases where the data might be used for the social good (i.e., medical research, improvement of public transports, contrasting epidemics), and understanding the personal data value means to correctly evaluate the balance between public benefits and personal loss of protection. On the other hand, when data are aimed to be used for commercial purposes, the value mentioned above might instead translate into simple pricing of personal information that the user might sell to a company for its business. In this context, discrimination discovery consists of searching for a-priori unknown contexts of suspect discrimination against protected-by-law social groups, by analyzing datasets of historical decision records. Machine learning and data mining approaches may be affected by discrimination rules, and these rules may be deeply hidden within obscure artificial intelligence models. Thus, discrimination discovery consists of understanding whether a predictive model makes direct or indirect discrimination. DCube [ 43 ] is a tool for data-driven discrimination discovery, a library of methods on fairness analysis.
It is important to evaluate how a mining model or algorithm takes its decision. The growing field of methods for explainable machine learning provides and continuously expands a set of comprehensive tool-kits [ 21 ]. For example, X-Lib is a library containing state-of-the-art explanation methods organized within a hierarchical structure and wrapped in a similar fashion way such that they can be easily accessed and used from different users. The library provides support for explaining classification on tabular data and images and for explaining the logic of complex decision systems. X-Lib collects, among the others, the following collection of explanation methods: LIME [ 38 ], Anchor [ 39 ], DeepExplain that includes Saliency maps [ 44 ], Gradient * Input, Integrated Gradients, and DeepLIFT [ 46 ]. Saliency method is a library containing code for SmoothGrad [ 45 ], as well as implementations of several other saliency techniques: Vanilla Gradients, Guided Backpropogation, and Grad-CAM. Another improvement in this context is the use of robotics and AI in data preparation, curation, and in detecting bias in data, information and knowledge as well as in the misuse and abuse of these assets when it comes to legal, privacy, and ethical issues and when it comes to transparency and trust. We cannot rely on human beings to do these tasks. We need to exploit the power of robotics and AI to help provide the protections required. Data and information lawyers will play a key role in legal and privacy issues, ethical use of these assets, and the problem of bias in both algorithms and the data, information, and knowledge used to develop analytics solutions. Finally, we can state that data science can help to fill the gap between legislators and technology.
4 Big data ecosystem: the role of research infrastructures
Research infrastructures (RIs) play a crucial role in the advent and development of data science. A social mining experiment exploits the main components of data science depicted in Fig. 1 (i.e., data, infrastructures, analytical methods) to enable multidisciplinary scientists and innovators to extract knowledge and to make the experiment reusable by the scientific community, innovators providing an impact on science and society.
Resources such as data and methods help domain and data scientists to transform research or an innovation question into a responsible data-driven analytical process. This process is executed onto the platform, thus supporting experiments that yield scientific output, policy recommendations, or innovative proofs-of-concept. Furthermore, an operational ethical board’s stewardship is a critical factor in the success of a RI.
An infrastructure typically offers easy-to-use means to define complex analytical processes and workflows , thus bridging the gap between domain experts and analytical technology. In many instances, domain experts may become a reference for their scientific communities, thus facilitating new users engagement within the RI activities. As a collateral feedback effect, experiments will generate new relevant data, methods, and workflows that can be integrated into the platform by data scientists, contributing to the resource expansion of the RI. An experiment designed in a node of the RI and executed on the platform returns its results to the entire RI community.
Well defined thematic environments amplify new experiments achievements towards the vertical scientific communities (and potential stakeholders) by activating appropriate dissemination channels.
4.1 The SoBigData Research Infrastructure
The SoBigData Research Infrastructure Footnote 7 is an ecosystem of human and digital resources, comprising data scientists, analytics, and processes. As shown in Fig. 4 , SoBigData is designed to enable multidisciplinary scientists and innovators to realize social mining experiments and to make them reusable by the scientific communities. All the components have been introduced for implementing data science from raw data management to knowledge extraction, with particular attention to legal and ethical aspects as reported in Fig. 1 . SoBigData supports data science serving a cross-disciplinary community of data scientists studying all the elements of societal complexity from a data- and model-driven perspective.
Currently, SoBigData includes scientific, industrial, and other stakeholders. In particular, our stakeholders are data analysts and researchers (35.6%), followed by companies (33.3%) and policy and lawmakers (20%). The following sections provide a short but comprehensive overview of the services provided by SoBigData RI with special attention on supporting ethical and open data science [ 15 , 16 ].
4.1.1 Resources, facilities, and access opportunities
Over the past decade, Europe has developed world-leading expertise in building and operating e-infrastructures. They are large-scale, federated and distributed online research environments through which researchers can share access to scientific resources (including data, instruments, computing, and communications), regardless of their location. They are meant to support unprecedented scales of international collaboration in science, both within and across disciplines, investing in economy-of-scale and common behavior, policies, best practices, and standards. They shape up a common environment where scientists can create , validate , assess , compare , and share their digital results of science, such as research data and research methods, by using a common “digital laboratory” consisting of agreed-on services and tools.
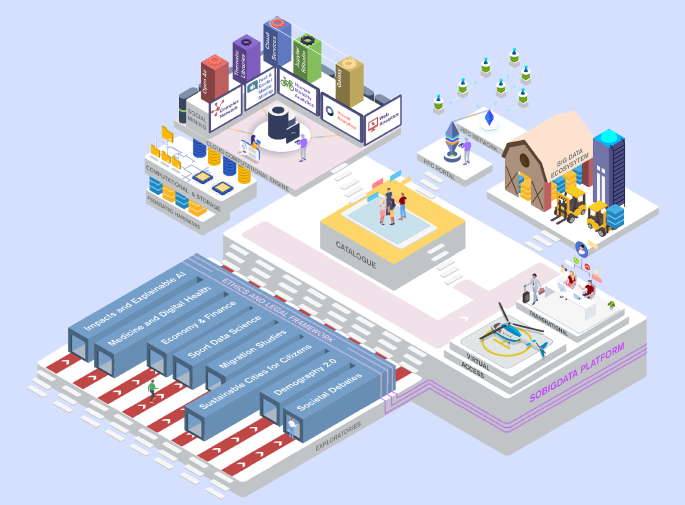
The SoBigData Research Infrastructure: an ecosystem of human and digital resources, comprising data scientists, analytical methods, and processes. SoBigData enables multidisciplinary scientists and innovators to carry out experiments and to make them reusable by the community
However, the implementation of workflows, possibly following Open Science principles of reproducibility and transparency, is hindered by a multitude of real-world problems. One of the most prominent is that e-infrastructures available to research communities today are far from being well-designed and consistent digital laboratories, neatly designed to share and reuse resources according to common policies, data models, standards, language platforms, and APIs. They are instead “patchworks of systems,” assembling online tools, services, and data sources and evolving to match the requirements of the scientific process, to include new solutions. The degree of heterogeneity excludes the adoption of uniform workflow management systems, standard service-oriented approaches, routine monitoring and accounting methods. The realization of scientific workflows is typically realized by writing ad hoc code, manipulating data on desktops, alternating the execution of online web services, sharing software libraries implementing research methods in different languages, desktop tools, web-accessible execution engines (e.g., Taverna, Knime, Galaxy).
The SoBigData e-infrastructure is based on D4Science services, which provides researchers and practitioners with a working environment where open science practices are transparently promoted, and data science practices can be implemented by minimizing the technological integration cost highlighted above.
D4Science is a deployed instance of the gCube Footnote 8 technology [ 4 ], a software conceived to facilitate the integration of web services, code, and applications as resources of different types in a common framework, which in turn enables the construction of Virtual Research Environments (VREs) [ 7 ] as combinations of such resources (Fig. 5 ). As there is no common framework that can be trusted enough, sustained enough, to convince resource providers that converging to it would be a worthwhile effort, D4Science implements a “system of systems.” In such a framework, resources are integrated with minimal cost, to gain in scalability, performance, accounting, provenance tracking, seamless integration with other resources, visibility to all scientists. The principle is that the cost of “participation” to the framework is on the infrastructure rather than on resource providers. The infrastructure provides the necessary bridges to include and combine resources that would otherwise be incompatible.
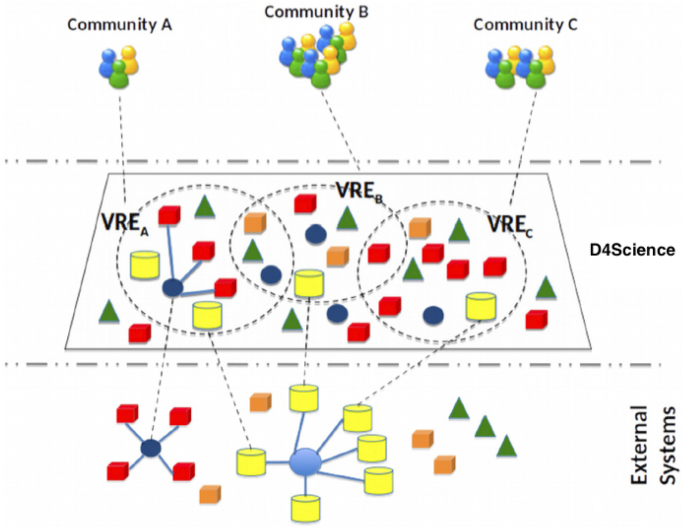
D4Science: resources from external systems, virtual research environments, and communities
More specifically, via D4Science, SoBigData scientists can integrate and share resources such as datasets, research methods, web services via APIs, and web applications via Portlets. Resources can then be integrated, combined, and accessed via VREs, intended as web-based working environments tailored to support the needs of their designated communities, each working on a research question. Research methods are integrated as executable code, implementing WPS APIs in different programming languages (e.g., Java, Python, R, Knime, Galaxy), which can be executed via the Data Miner analytics platform in parallel, transparently to the users, over powerful and extensible clusters, and via simple VRE user interfaces. Scientists using Data Miner in the context of a VRE can select and execute the available methods and share the results with other scientists, who can repeat or reproduce the experiment with a simple click.
D4Science VREs are equipped with core services supporting data analysis and collaboration among its users: ( i ) a shared workspace to store and organize any version of a research artifact; ( ii ) a social networking area to have discussions on any topic (including working version and released artifacts) and be informed on happenings; ( iii ) a Data Miner analytics platform to execute processing tasks (research methods) either natively provided by VRE users or borrowed from other VREs to be applied to VRE users’ cases and datasets; and iv ) a catalogue-based publishing platform to make the existence of a certain artifact public and disseminated. Scientists operating within VREs use such facilities continuously and transparently track the record of their research activities (actions, authorship, provenance), as well as products and links between them (lineage) resulting from every phase of the research life cycle, thus facilitating publishing of science according to Open Science principles of transparency and reproducibility [ 5 ].
Today, SoBigData integrates the resources in Table 1 . By means of such resources, SoBigData scientists have created VREs to deliver the so-called SoBigData exploratories : Explainable Machine Learning , Sports Data Science , Migration Studies , Societal Debates , Well-being & Economy , and City of Citizens . Each exploratory includes the resources required to perform Data science workflows in a controlled and shared environment. Resources range from data to methods, described more in detail in the following, together with their exploitation within the exploratories.
All the resources and instruments integrate into SoBigData RI are structured in such a way as to operate within the confines of the current data protection law with the focus on General Data Protection Regulation (GDPR) and ethical analysis of the fundamental values involved in social mining and AI. Each item into the catalogue has specific fields for managing ethical issues (e.g., if a dataset contains personal info) and fields for describing and managing intellectual properties.
4.1.2 Data resources: social mining and big data ecosystem
SoBigData RI defines policies supporting users in the collection, description, preservation, and sharing of their data sets. It implements data science making such data available for collaborative research by adopting various strategies, ranging from sharing the open data sets with the scientific community at large, to share the data with disclosure restriction allowing data access within secure environments.
Several big data sets are available through SoBigData RI including network graphs from mobile phone call data; networks crawled from many online social networks, including Facebook and Flickr, transaction micro-data from diverse retailers, query logs both from search engines and e-commerce, society-wide mobile phone call data records, GPS tracks from personal navigation devices, survey data about customer satisfaction or market research, extensive web archives, billions of tweets, and data from location-aware social networks.
4.1.3 Data science through SoBigData exploratories
Exploratories are thematic environments built on top of the SoBigData RI. An exploratory binds datasets with social mining methods providing the research context for supporting specific data science applications by: (i) providing the scientific context for performing the application. This context can be considered a container for binding specific methods, applications, services, and datasets; (ii) stimulating communities on the effectiveness of the analytical process related to the analysis, promoting scientific dissemination, result sharing, and reproducibility. The use of exploratories promotes the effectiveness of the data science trough research infrastructure services. The following sections report a short description of the six SoBigData exploratories. Figure 6 shows the main thematic areas covered by each exploratory. Due to its nature, Explainable Machine Learning exploratory can be applied to each sector where a black-box machine learning approach is used. The list of exploratories (and the data and methods inside them) are updated continuously and continue to grow over time. Footnote 9
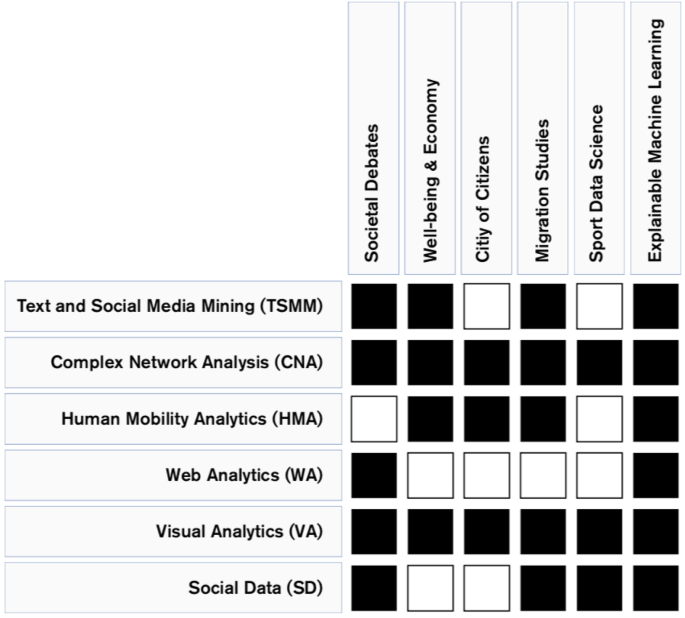
SoBigData covers six thematic areas listed horizontally. Each exploratory covers more than one thematic area
City of citizens. This exploratory aims to collect data science applications and methods related to geo-referenced data. The latter describes the movements of citizens in a city, a territory, or an entire region. There are several studies and different methods that employ a wide variety of data sources to build models about the mobility of people and city characteristics in the scientific literature [ 30 , 32 ]. Like ecosystems, cities are open systems that live and develop utilizing flows of energy, matter, and information. What distinguishes a city from a colony is the human component (i.e., the process of transformation by cultural and technological evolution). Through this combination, cities are evolutionary systems that develop and co-evolve continuously with their inhabitants [ 24 ]. Cities are kaleidoscopes of information generated by a myriad of digital devices weaved into the urban fabric. The inclusion of tracking technologies in personal devices enabled the analysis of large sets of mobility data like GPS traces and call detail records.
Data science applied to human mobility is one of the critical topics investigated in SoBigData thanks to the decennial experience of partners in European projects. The study of human mobility led to the integration into the SoBigData of unique Global Positioning System (GPS) and call detail record (CDR) datasets of people and vehicle movements, and geo-referenced social network data as well as several mobility services: O/D (origin-destination) matrix computation, Urban Mobility Atlas Footnote 10 (a visual interface to city mobility patterns), GeoTopics Footnote 11 (for exploring patterns of urban activity from Foursquare), and predictive models: MyWay Footnote 12 (trajectory prediction), TripBuilder Footnote 13 (tourists to build personalized tours of a city). In human mobility, research questions come from geographers, urbanists, complexity scientists, data scientists, policymakers, and Big Data providers, as well as innovators aiming to provide applications for any service for the smart city ecosystem. The idea is to investigate the impact of political events on the well-being of citizens. This exploratory supports the development of “happiness” and “peace” indicators through text mining/opinion mining pipeline on repositories of online news. These indicators reveal that the level of crime of a territory can be well approximated by analyzing the news related to that territory. Generally, we study the impact of the economy on well-being and vice versa, e.g., also considering the propagation of shocks of financial distress in an economic or financial system crucially depends on the topology of the network interconnecting the different elements.
Well-being and economy. This exploratory tests the hypothesis that well-being is correlated to the business performance of companies. The idea is to combine statistical methods and traditional economic data (typically at low-frequency) with high-frequency data from non-traditional sources, such as, i.e., web, supermarkets, for now-casting economic, socioeconomic and well-being indicators. These indicators allow us to study and measure real-life costs by studying price variation and socioeconomic status inference. Furthermore, this activity supports studies on the correlation between people’s well-being and their social and mobility data. In this context, some basic hypothesis can be summarized as: (i) there are curves of age- and gender-based segregation distribution in boards of companies, which are characteristic to mean credit risk of companies in a region; (ii) low mean credit risk of companies in a region has a positive correlation to well-being; (iii) systemic risk correlates highly with well-being indices at a national level. The final aim is to provide a set of guidelines to national governments, methods, and indices for decision making on regulations affecting companies to improve well-being in the country, also considering effective policies to reduce operational risks such as credit risk, and external threats of companies [ 17 ].
Big Data, analyzed through the lenses of data science, provides means to understand our complex socioeconomic and financial systems. On the one hand, this offers new opportunities to measure the patterns of well-being and poverty at a local and global scale, empowering governments and policymakers with the unprecedented opportunity to nowcast relevant economic quantities and compare different countries, regions, and cities. On the other hand, this allows us to investigate the network underlying the complex systems of economy and finance, and it affects the aggregate output, the propagation of shocks or financial distress and systemic risk.
Societal debates. This exploratory employs data science approaches to answer research questions such as who is participating in public debates? What is the “big picture” response from citizens to a policy, election, referendum, or other political events? This kind of analysis allows scientists, policymakers, and citizens to understand the online discussion surrounding polarized debates [ 14 ]. The personal perception of online discussions on social media is often biased by the so-called filter bubble, in which automatic curation of content and relationships between users negatively affects the diversity of opinions available to them. Making a complete analysis of online polarized debates enables the citizens to be better informed and prepared for political outcomes. By analyzing content and conversations on social media and newspaper articles, data scientists study public debates and also assess public sentiment around debated topics, opinion diffusion dynamics, echo chambers formation and polarized discussions, fake news analysis, and propaganda bots. Misinformation is often the result of a distorted perception of concepts that, although unrelated, suddenly appear together in the same narrative. Understanding the details of this process at an early stage may help to prevent the birth and the diffusion of fake news. The misinformation fight includes the development of dynamical models of misinformation diffusion (possibly in contrast to the spread of mainstream news) as well as models of how attention cycles are accelerated and amplified by the infrastructures of online media.
Another important topic covered by this exploratory concerns the analysis of how social bots activity affects fake news diffusion. Determining whether a human or a bot controls a user account is a complex task. To the best of our knowledge, the only openly accessible solution to detect social bots is Botometer, an API that allows us to interact with an underlying machine learning system. Although Botometer has been proven to be entirely accurate in detecting social bots, it has limitations due to the Twitter API features: hence, an algorithm overcoming the barriers of current recipes is needed.
The resources related to Societal Debates exploratory, especially in the domain of media ecology and the fight against misinformation online, provide easy-to-use services to public bodies, media outlets, and social/political scientists. Furthermore, SoBigData supports new simulation models and experimental processes to validate in vivo the algorithms for fighting misinformation, curbing the pathological acceleration and amplification of online attention cycles, breaking the bubbles, and explore alternative media and information ecosystems.
Migration studies. Data science is also useful to understand the migration phenomenon. Knowledge about the number of immigrants living in a particular region is crucial to devise policies that maximize the benefits for both locals and immigrants. These numbers can vary rapidly in space and time, especially in periods of crisis such as wars or natural disasters.
This exploratory provides a set of data and tools for trying to answer some questions about migration flows. Through this exploratory, a data scientist studies economic models of migration and can observe how migrants choose their destination countries. A scientist can discover what is the meaning of “opportunities” that a country provides to migrants, and whether there are correlations between the number of incoming migrants and opportunities in the host countries [ 8 ]. Furthermore, this exploratory tries to understand how public perception of migration is changing using an opinion mining analysis. For example, social network analysis enables us to analyze the migrant’s social network and discover the structure of the social network for people who decided to start a new life in a different country [ 28 ].
Finally, we can also evaluate current integration indices based on official statistics and survey data, which can be complemented by Big Data sources. This exploratory aims to build combined integration indexes that take into account multiple data sources to evaluate integration on various levels. Such integration includes mobile phone data to understand patterns of communication between immigrants and natives; social network data to assess sentiment towards immigrants and immigration; professional network data (such as LinkedIn) to understand labor market integration, and local data to understand to what extent moving across borders is associated with a change in the cultural norms of the migrants. These indexes are fundamental to evaluate the overall social and economic effects of immigration. The new integration indexes can be applied with various space and time resolutions (small area methods) to obtain a complete image of integration, and complement official index.
Sports data science. The proliferation of new sensing technologies that provide high-fidelity data streams extracted from every game, is changing the way scientists, fans and practitioners conceive sports performance. The combination of these (big) data with the tools of data science provides the possibility to unveil complex models underlying sports performance and enables to perform many challenging tasks: from automatic tactical analysis to data-driven performance ranking; game outcome prediction, and injury forecasting. The idea is to foster research on sports data science in several directions. The application of explainable AI and deep learning techniques can be hugely beneficial to sports data science. For example, by using adversarial learning, we can modify the training plans of players that are associated with high injury risk and develop training plans that maximize the fitness of players (minimizing their injury risk). The use of gaming, simulation, and modeling is another set of tools that can be used by coaching staff to test tactics that can be employed against a competitor. Furthermore, by using deep learning on time series, we can forecast the evolution of the performance of players and search for young talents.
This exploratory examines the factors influencing sports success and how to build simulation tools for boosting both individual and collective performance. Furthermore, this exploratory describes performances employing data, statistics, and models, allowing coaches, fans, and practitioners to understand (and boost) sports performance [ 42 ].
Explainable machine learning. Artificial Intelligence, increasingly based on Big Data analytics, is a disruptive technology of our times. This exploratory provides a forum for studying effects of AI on the future society. In this context, SoBigData studies the future of labor and the workforce, also through data- and model-driven analysis, simulations, and the development of methods that construct human understandable explanations of AI black-box models [ 20 ].
Black box systems for automated decision making map a user’s features into a class that predicts the behavioral traits of individuals, such as credit risk, health status, without exposing the reasons why. Most of the time, the internal reasoning of these algorithms is obscure even to their developers. For this reason, the last decade has witnessed the rise of a black box society. This exploratory is developing a set of techniques and tools which allow data analysts to understand why an algorithm produce a decision. These approaches are designed not for discovering a lack of transparency but also for discovering possible biases inherited by the algorithms from human prejudices and artefacts hidden in the training data (which may lead to unfair or wrong decisions) [ 35 ].
5 Conclusions: individual and collective intelligence
The world’s technological per-capita capacity to store information has roughly doubled every 40 months since the 1980s [ 23 ]. Since 2012, every day 2.5 exabytes (2.5 \(\times \) 10 \(^18\) bytes) of data were created; as of 2014, every day 2.3 zettabytes (2.3 \(\times \) 10 \(^21\) bytes) of data were generated by Super-power high-tech Corporation worldwide. Soon zettabytes of useful public and private data will be widely and openly available. In the next years, smart applications such as smart grids, smart logistics, smart factories, and smart cities will be widely deployed across the continent and beyond. Ubiquitous broadband access, mobile technology, social media, services, and internet of think on billions of devices will have contributed to the explosion of generated data to a total global estimate of 40 zettabytes.
In this work, we have introduced data science as a new challenge and opportunity for the next years. In this context, we have tried to summarize in a concise way several aspects related to data science applications and their impacts on society, considering both the new services available and the new job perspectives. We have also introduced issues in managing data representing human behavior and showed how difficult it is to preserve personal information and privacy. With the introduction of SoBigData RI and exploratories, we have provided virtual environments where it is possible to understand the potentiality of data science in different research contexts.
Concluding, we can state that social dilemmas occur when there is a conflict between the individual and public interest. Such problems also appear in the ecosystem of distributed AI systems (based on data science tools) and humans, with additional difficulties due: on the one hand, to the relative rigidity of the trained AI systems and the necessity of achieving social benefit, and, on the other hand, to the necessity of keeping individuals interested. What are the principles and solutions for individual versus social optimization using AI, and how can an optimum balance be achieved? The answer is still open, but these complex systems have to work on fulfilling collective goals, and requirements, with the challenge that human needs change over time and move from one context to another. Every AI system should operate within an ethical and social framework in understandable, verifiable, and justifiable way. Such systems must, in any case, work within the bounds of the rule of law, incorporating protection of fundamental rights into the AI infrastructure. In other words, the challenge is to develop mechanisms that will result in the system converging to an equilibrium that complies with European values and social objectives (e.g., social inclusion) but without unnecessary losses of efficiency.
Interestingly, data science can play a vital role in enhancing desirable behaviors in the system, e.g., by supporting coordination and cooperation that is, more often than not, crucial to achieving any meaningful improvements. Our ultimate goal is to build the blueprint of a sociotechnical system in which AI not only cooperates with humans but, if necessary, helps them to learn how to collaborate, as well as other desirable behaviors. In this context, it is also essential to understand how to achieve robustness of the human and AI ecosystems in respect of various types of malicious behaviors, such as abuse of power and exploitation of AI technical weaknesses.
We conclude by paraphrasing Stephen Hawking in his Brief Answers to the Big Questions: the availability of data on its own will not take humanity to the future, but its intelligent and creative use will.
http://www.sdss3.org/collaboration/ .
e.g., https://www.nature.com/sdata/policies/repositories .
Responsible Data Science program: https://redasci.org/ .
https://emergency.copernicus.eu/ .
Nowcasting in economics is the prediction of the present, the very near future, and the very recent past state of an economic indicator.
https://www.unglobalpulse.org/ .
http://sobigdata.eu .
https://www.gcube-system.org/ .
https://sobigdata.d4science.org/catalogue-sobigdata .
http://www.sobigdata.eu/content/urban-mobility-atlas .
http://data.d4science.org/ctlg/ResourceCatalogue/geotopics_-_a_method_and_system_to_explore_urban_activity .
http://data.d4science.org/ctlg/ResourceCatalogue/myway_-_trajectory_prediction .
http://data.d4science.org/ctlg/ResourceCatalogue/tripbuilder .
Abitbol, J.L., Fleury, E., Karsai, M.: Optimal proxy selection for socioeconomic status inference on twitter. Complexity 2019 , 60596731–605967315 (2019). https://doi.org/10.1155/2019/6059673
Article Google Scholar
Amato, G., Candela, L., Castelli, D., Esuli, A., Falchi, F., Gennaro, C., Giannotti, F., Monreale, A., Nanni, M., Pagano, P., Pappalardo, L., Pedreschi, D., Pratesi, F., Rabitti, F., Rinzivillo, S., Rossetti, G., Ruggieri, S., Sebastiani, F., Tesconi, M.: How data mining and machine learning evolved from relational data base to data science. In: Flesca, S., Greco, S., Masciari, E., Saccà, D. (eds.) A Comprehensive Guide Through the Italian Database Research Over the Last 25 Years, Studies in Big Data, vol. 31, pp. 287–306. Springer, Berlin (2018). https://doi.org/10.1007/978-3-319-61893-7_17
Chapter Google Scholar
Andrienko, G.L., Andrienko, N.V., Budziak, G., Dykes, J., Fuchs, G., von Landesberger, T., Weber, H.: Visual analysis of pressure in football. Data Min. Knowl. Discov. 31 (6), 1793–1839 (2017). https://doi.org/10.1007/s10618-017-0513-2
Article MathSciNet Google Scholar
Assante, M., Candela, L., Castelli, D., Cirillo, R., Coro, G., Frosini, L., Lelii, L., Mangiacrapa, F., Marioli, V., Pagano, P., Panichi, G., Perciante, C., Sinibaldi, F.: The gcube system: delivering virtual research environments as-a-service. Future Gener. Comput. Syst. 95 , 445–453 (2019). https://doi.org/10.1016/j.future.2018.10.035
Assante, M., Candela, L., Castelli, D., Cirillo, R., Coro, G., Frosini, L., Lelii, L., Mangiacrapa, F., Pagano, P., Panichi, G., Sinibaldi, F.: Enacting open science by d4science. Future Gener. Comput. Syst. (2019). https://doi.org/10.1016/j.future.2019.05.063
Barabasi, A.L., Gulbahce, N., Loscalzo, J.: Network medicine: a network-based approach to human disease. Nature reviews. Genetics 12 , 56–68 (2011). https://doi.org/10.1038/nrg2918
Candela, L., Castelli, D., Pagano, P.: Virtual research environments: an overview and a research agenda. Data Sci. J. 12 , GRDI75–GRDI81 (2013). https://doi.org/10.2481/dsj.GRDI-013
Coletto, M., Esuli, A., Lucchese, C., Muntean, C.I., Nardini, F.M., Perego, R., Renso, C.: Sentiment-enhanced multidimensional analysis of online social networks: perception of the mediterranean refugees crisis. In: Proceedings of the 2016 IEEE/ACM International Conference on Advances in Social Networks Analysis and Mining, ASONAM’16, pp. 1270–1277. IEEE Press, Piscataway, NJ, USA (2016). http://dl.acm.org/citation.cfm?id=3192424.3192657
Coscia, M., Rossetti, G., Giannotti, F., Pedreschi, D.: Uncovering hierarchical and overlapping communities with a local-first approach. TKDD 9 (1), 6:1–6:27 (2014). https://doi.org/10.1145/2629511
Cresci, S., Minutoli, S., Nizzoli, L., Tardelli, S., Tesconi, M.: Enriching digital libraries with crowdsensed data. In: P. Manghi, L. Candela, G. Silvello (eds.) Digital Libraries: Supporting Open Science—15th Italian Research Conference on Digital Libraries, IRCDL 2019, Pisa, Italy, 31 Jan–1 Feb 2019, Proceedings, Communications in Computer and Information Science, vol. 988, pp. 144–158. Springer (2019). https://doi.org/10.1007/978-3-030-11226-4_12
Cresci, S., Petrocchi, M., Spognardi, A., Tognazzi, S.: Better safe than sorry: an adversarial approach to improve social bot detection. In: P. Boldi, B.F. Welles, K. Kinder-Kurlanda, C. Wilson, I. Peters, W.M. Jr. (eds.) Proceedings of the 11th ACM Conference on Web Science, WebSci 2019, Boston, MA, USA, June 30–July 03, 2019, pp. 47–56. ACM (2019). https://doi.org/10.1145/3292522.3326030
Cresci, S., Pietro, R.D., Petrocchi, M., Spognardi, A., Tesconi, M.: Social fingerprinting: detection of spambot groups through dna-inspired behavioral modeling. IEEE Trans. Dependable Sec. Comput. 15 (4), 561–576 (2018). https://doi.org/10.1109/TDSC.2017.2681672
Furletti, B., Trasarti, R., Cintia, P., Gabrielli, L.: Discovering and understanding city events with big data: the case of rome. Information 8 (3), 74 (2017). https://doi.org/10.3390/info8030074
Garimella, K., De Francisci Morales, G., Gionis, A., Mathioudakis, M.: Reducing controversy by connecting opposing views. In: Proceedings of the 10th ACM International Conference on Web Search and Data Mining, WSDM’17, pp. 81–90. ACM, New York, NY, USA (2017). https://doi.org/10.1145/3018661.3018703
Giannotti, F., Trasarti, R., Bontcheva, K., Grossi, V.: Sobigdata: social mining & big data ecosystem. In: P. Champin, F.L. Gandon, M. Lalmas, P.G. Ipeirotis (eds.) Companion of the The Web Conference 2018 on The Web Conference 2018, WWW 2018, Lyon , France, April 23–27, 2018, pp. 437–438. ACM (2018). https://doi.org/10.1145/3184558.3186205
Grossi, V., Rapisarda, B., Giannotti, F., Pedreschi, D.: Data science at sobigdata: the european research infrastructure for social mining and big data analytics. I. J. Data Sci. Anal. 6 (3), 205–216 (2018). https://doi.org/10.1007/s41060-018-0126-x
Grossi, V., Romei, A., Ruggieri, S.: A case study in sequential pattern mining for it-operational risk. In: W. Daelemans, B. Goethals, K. Morik (eds.) Machine Learning and Knowledge Discovery in Databases, European Conference, ECML/PKDD 2008, Antwerp, Belgium, 15–19 Sept 2008, Proceedings, Part I, Lecture Notes in Computer Science, vol. 5211, pp. 424–439. Springer (2008). https://doi.org/10.1007/978-3-540-87479-9_46
Guidotti, R., Coscia, M., Pedreschi, D., Pennacchioli, D.: Going beyond GDP to nowcast well-being using retail market data. In: A. Wierzbicki, U. Brandes, F. Schweitzer, D. Pedreschi (eds.) Advances in Network Science—12th International Conference and School, NetSci-X 2016, Wroclaw, Poland, 11–13 Jan 2016, Proceedings, Lecture Notes in Computer Science, vol. 9564, pp. 29–42. Springer (2016). https://doi.org/10.1007/978-3-319-28361-6_3
Guidotti, R., Monreale, A., Nanni, M., Giannotti, F., Pedreschi, D.: Clustering individual transactional data for masses of users. In: Proceedings of the 23rd ACM SIGKDD International Conference on Knowledge Discovery and Data Mining, Halifax, NS, Canada, 13–17 Aug 2017, pp. 195–204. ACM (2017). https://doi.org/10.1145/3097983.3098034
Guidotti, R., Monreale, A., Ruggieri, S., Turini, F., Giannotti, F., Pedreschi, D.: A survey of methods for explaining black box models. ACM Comput. Surv. 51 (5), 93:1–93:42 (2019). https://doi.org/10.1145/3236009
Guidotti, R., Monreale, A., Turini, F., Pedreschi, D., Giannotti, F.: A survey of methods for explaining black box models. CoRR abs/1802.01933 (2018). arxiv: 1802.01933
Guidotti, R., Nanni, M., Rinzivillo, S., Pedreschi, D., Giannotti, F.: Never drive alone: boosting carpooling with network analysis. Inf. Syst. 64 , 237–257 (2017). https://doi.org/10.1016/j.is.2016.03.006
Hilbert, M., Lopez, P.: The world’s technological capacity to store, communicate, and compute information. Science 332 (6025), 60–65 (2011)
Kennedy, C.A., Stewart, I., Facchini, A., Cersosimo, I., Mele, R., Chen, B., Uda, M., Kansal, A., Chiu, A., Kim, K.g., Dubeux, C., Lebre La Rovere, E., Cunha, B., Pincetl, S., Keirstead, J., Barles, S., Pusaka, S., Gunawan, J., Adegbile, M., Nazariha, M., Hoque, S., Marcotullio, P.J., González Otharán, F., Genena, T., Ibrahim, N., Farooqui, R., Cervantes, G., Sahin, A.D., : Energy and material flows of megacities. Proc. Nat. Acad. Sci. 112 (19), 5985–5990 (2015). https://doi.org/10.1073/pnas.1504315112
Korjani, S., Damiano, A., Mureddu, M., Facchini, A., Caldarelli, G.: Optimal positioning of storage systems in microgrids based on complex networks centrality measures. Sci. Rep. (2018). https://doi.org/10.1038/s41598-018-35128-6
Lorini, V., Castillo, C., Dottori, F., Kalas, M., Nappo, D., Salamon, P.: Integrating social media into a pan-european flood awareness system: a multilingual approach. In: Z. Franco, J.J. González, J.H. Canós (eds.) Proceedings of the 16th International Conference on Information Systems for Crisis Response and Management, València, Spain, 19–22 May 2019. ISCRAM Association (2019). http://idl.iscram.org/files/valeriolorini/2019/1854-_ValerioLorini_etal2019.pdf
Lulli, A., Gabrielli, L., Dazzi, P., Dell’Amico, M., Michiardi, P., Nanni, M., Ricci, L.: Scalable and flexible clustering solutions for mobile phone-based population indicators. Int. J. Data Sci. Anal. 4 (4), 285–299 (2017). https://doi.org/10.1007/s41060-017-0065-y
Moise, I., Gaere, E., Merz, R., Koch, S., Pournaras, E.: Tracking language mobility in the twitter landscape. In: C. Domeniconi, F. Gullo, F. Bonchi, J. Domingo-Ferrer, R.A. Baeza-Yates, Z. Zhou, X. Wu (eds.) IEEE International Conference on Data Mining Workshops, ICDM Workshops 2016, 12–15 Dec 2016, Barcelona, Spain., pp. 663–670. IEEE Computer Society (2016). https://doi.org/10.1109/ICDMW.2016.0099
Nanni, M.: Advancements in mobility data analysis. In: F. Leuzzi, S. Ferilli (eds.) Traffic Mining Applied to Police Activities—Proceedings of the 1st Italian Conference for the Traffic Police (TRAP-2017), Rome, Italy, 25–26 Oct 2017, Advances in Intelligent Systems and Computing, vol. 728, pp. 11–16. Springer (2017). https://doi.org/10.1007/978-3-319-75608-0_2
Nanni, M., Trasarti, R., Monreale, A., Grossi, V., Pedreschi, D.: Driving profiles computation and monitoring for car insurance crm. ACM Trans. Intell. Syst. Technol. 8 (1), 14:1–14:26 (2016). https://doi.org/10.1145/2912148
Pappalardo, G., di Matteo, T., Caldarelli, G., Aste, T.: Blockchain inefficiency in the bitcoin peers network. EPJ Data Sci. 7 (1), 30 (2018). https://doi.org/10.1140/epjds/s13688-018-0159-3
Pappalardo, L., Barlacchi, G., Pellungrini, R., Simini, F.: Human mobility from theory to practice: Data, models and applications. In: S. Amer-Yahia, M. Mahdian, A. Goel, G. Houben, K. Lerman, J.J. McAuley, R.A. Baeza-Yates, L. Zia (eds.) Companion of The 2019 World Wide Web Conference, WWW 2019, San Francisco, CA, USA, 13–17 May 2019., pp. 1311–1312. ACM (2019). https://doi.org/10.1145/3308560.3320099
Pappalardo, L., Cintia, P., Ferragina, P., Massucco, E., Pedreschi, D., Giannotti, F.: Playerank: data-driven performance evaluation and player ranking in soccer via a machine learning approach. ACM TIST 10 (5), 59:1–59:27 (2019). https://doi.org/10.1145/3343172
Pappalardo, L., Vanhoof, M., Gabrielli, L., Smoreda, Z., Pedreschi, D., Giannotti, F.: An analytical framework to nowcast well-being using mobile phone data. CoRR abs/1606.06279 (2016). arxiv: 1606.06279
Pasquale, F.: The Black Box Society: The Secret Algorithms That Control Money and Information. Harvard University Press, Cambridge (2015)
Book Google Scholar
Piškorec, M., Antulov-Fantulin, N., Miholić, I., Šmuc, T., Šikić, M.: Modeling peer and external influence in online social networks: Case of 2013 referendum in croatia. In: Cherifi, C., Cherifi, H., Karsai, M., Musolesi, M. (eds.) Complex Networks & Their Applications VI. Springer, Cham (2018)
Google Scholar
Ranco, G., Aleksovski, D., Caldarelli, G., Mozetic, I.: Investigating the relations between twitter sentiment and stock prices. CoRR abs/1506.02431 (2015). arxiv: 1506.02431
Ribeiro, M.T., Singh, S., Guestrin, C.: “why should I trust you?”: Explaining the predictions of any classifier. In: B. Krishnapuram, M. Shah, A.J. Smola, C.C. Aggarwal, D. Shen, R. Rastogi (eds.) Proceedings of the 22nd ACM SIGKDD International Conference on Knowledge Discovery and Data Mining, San Francisco, CA, USA, 13–17 Aug 2016, pp. 1135–1144. ACM (2016). https://doi.org/10.1145/2939672.2939778
Ribeiro, M.T., Singh, S., Guestrin, C.: Anchors: High-precision model-agnostic explanations. In: S.A. McIlraith, K.Q. Weinberger (eds.) Proceedings of the Thirty-Second AAAI Conference on Artificial Intelligence, (AAAI-18), the 30th innovative Applications of Artificial Intelligence (IAAI-18), and the 8th AAAI Symposium on Educational Advances in Artificial Intelligence (EAAI-18), New Orleans, Louisiana, USA, 2–7 Feb 2018, pp. 1527–1535. AAAI Press (2018). https://www.aaai.org/ocs/index.php/AAAI/AAAI18/-paper/view/16982
Rossetti, G., Milli, L., Rinzivillo, S., Sîrbu, A., Pedreschi, D., Giannotti, F.: Ndlib: a python library to model and analyze diffusion processes over complex networks. Int. J. Data Sci. Anal. 5 (1), 61–79 (2018). https://doi.org/10.1007/s41060-017-0086-6
Rossetti, G., Pappalardo, L., Pedreschi, D., Giannotti, F.: Tiles: an online algorithm for community discovery in dynamic social networks. Mach. Learn. 106 (8), 1213–1241 (2017). https://doi.org/10.1007/s10994-016-5582-8
Rossi, A., Pappalardo, L., Cintia, P., Fernández, J., Iaia, M.F., Medina, D.: Who is going to get hurt? predicting injuries in professional soccer. In: J. Davis, M. Kaytoue, A. Zimmermann (eds.) Proceedings of the 4th Workshop on Machine Learning and Data Mining for Sports Analytics co-located with 2017 European Conference on Machine Learning and Principles and Practice of Knowledge Discovery in Databases (ECML PKDD 2017), Skopje, Macedonia, 18 Sept 2017., CEUR Workshop Proceedings, vol. 1971, pp. 21–30. CEUR-WS.org (2017). http://ceur-ws.org/Vol-1971/paper-04.pdf
Ruggieri, S., Pedreschi, D., Turini, F.: DCUBE: discrimination discovery in databases. In: A.K. Elmagarmid, D. Agrawal (eds.) Proceedings of the ACM SIGMOD International Conference on Management of Data, SIGMOD 2010, Indianapolis, Indiana, USA, 6–10 June 2010, pp. 1127–1130. ACM (2010). https://doi.org/10.1145/1807167.1807298
Simonyan, K., Vedaldi, A., Zisserman, A.: Deep inside convolutional networks: Visualising image classification models and saliency maps. CoRR abs/1312.6034 (2013). http://dblp.uni-trier.de/db/journals/corr/corr1312.html#SimonyanVZ13
Smilkov, D., Thorat, N., Kim, B., Viégas, F.B., Wattenberg, M.: Smoothgrad: removing noise by adding noise. CoRR abs/1706.03825 (2017). arxiv: 1706.03825
Sundararajan, M., Taly, A., Yan, Q.: Axiomatic attribution for deep networks. In: D. Precup, Y.W. Teh (eds.) Proceedings of the 34th International Conference on Machine Learning, Proceedings of Machine Learning Research, vol. 70, pp. 3319–3328. PMLR, International Convention Centre, Sydney, Australia (2017). http://proceedings.mlr.press/v70/sundararajan17a.html
Trasarti, R., Guidotti, R., Monreale, A., Giannotti, F.: Myway: location prediction via mobility profiling. Inf. Syst. 64 , 350–367 (2017). https://doi.org/10.1016/j.is.2015.11.002
Traub, J., Quiané-Ruiz, J., Kaoudi, Z., Markl, V.: Agora: Towards an open ecosystem for democratizing data science & artificial intelligence. CoRR abs/1909.03026 (2019). arxiv: 1909.03026
Vazifeh, M.M., Zhang, H., Santi, P., Ratti, C.: Optimizing the deployment of electric vehicle charging stations using pervasive mobility data. Transp Res A Policy Practice 121 (C), 75–91 (2019). https://doi.org/10.1016/j.tra.2019.01.002
Vermeulen, A.F.: Practical Data Science: A Guide to Building the Technology Stack for Turning Data Lakes into Business Assets, 1st edn. Apress, New York (2018)
Download references
Acknowledgements
This work is supported by the European Community’s H2020 Program under the scheme ‘INFRAIA-1-2014-2015: Research Infrastructures’, grant agreement #654024 ‘SoBigData: Social Mining and Big Data Ecosystem’ and the scheme ‘INFRAIA-01-2018-2019: Research and Innovation action’, grant agreement #871042 ’SoBigData \(_{++}\) : European Integrated Infrastructure for Social Mining and Big Data Analytics’
Open access funding provided by Università di Pisa within the CRUI-CARE Agreement.
Author information
Authors and affiliations.
CNR - Istituto Scienza e Tecnologia dell’Informazione A. Faedo, KDDLab, Pisa, Italy
Valerio Grossi & Fosca Giannotti
Department of Computer Science, University of Pisa, Pisa, Italy
Dino Pedreschi
CNR - Istituto Scienza e Tecnologia dell’Informazione A. Faedo, NeMIS, Pisa, Italy
Paolo Manghi, Pasquale Pagano & Massimiliano Assante
You can also search for this author in PubMed Google Scholar
Corresponding author
Correspondence to Dino Pedreschi .
Additional information
Publisher's note.
Springer Nature remains neutral with regard to jurisdictional claims in published maps and institutional affiliations.
Rights and permissions
Open Access This article is licensed under a Creative Commons Attribution 4.0 International License, which permits use, sharing, adaptation, distribution and reproduction in any medium or format, as long as you give appropriate credit to the original author(s) and the source, provide a link to the Creative Commons licence, and indicate if changes were made. The images or other third party material in this article are included in the article’s Creative Commons licence, unless indicated otherwise in a credit line to the material. If material is not included in the article’s Creative Commons licence and your intended use is not permitted by statutory regulation or exceeds the permitted use, you will need to obtain permission directly from the copyright holder. To view a copy of this licence, visit http://creativecommons.org/licenses/by/4.0/ .
Reprints and permissions
About this article
Grossi, V., Giannotti, F., Pedreschi, D. et al. Data science: a game changer for science and innovation. Int J Data Sci Anal 11 , 263–278 (2021). https://doi.org/10.1007/s41060-020-00240-2
Download citation
Received : 13 July 2019
Accepted : 15 December 2020
Published : 19 April 2021
Issue Date : May 2021
DOI : https://doi.org/10.1007/s41060-020-00240-2
Share this article
Anyone you share the following link with will be able to read this content:
Sorry, a shareable link is not currently available for this article.
Provided by the Springer Nature SharedIt content-sharing initiative
- Responsible data science
- Research infrastructure
- Social mining
- Find a journal
- Publish with us
- Track your research

Data Science for Undergraduates: Opportunities and Options (2018)
Chapter: 6 conclusions, 6 conclusions.
Data science education is well into its formative stages of development; it is evolving into a self-supporting discipline and producing professionals with distinct and complementary skills relative to professionals in the computer, information, and statistical sciences. However, regardless of its potential eventual disciplinary status, the evidence points to robust growth of data science education that will indelibly shape the undergraduate students of the future. In fact, fueled by growing student interest and industry demand, data science education will likely become a staple of the undergraduate experience. There will be an increase in the number of students majoring, minoring, earning certificates, or just taking courses in data science as the value of data skills becomes even more widely recognized. The adoption of a general education requirement in data science for all undergraduates will endow future generations of students with the basic understanding of data science that they need to become responsible citizens. Continuing education programs such as data science boot camps, career accelerators, summer schools, and incubators will provide another stream of talent. This constitutes the emerging watershed of data science education that feeds multiple streams of generalists and specialists in society; citizens are empowered by their basic skills to examine, interpret, and draw value from data.
Today, the nation is in the formative phase of data science education, where educational organizations are pioneering their own programs, each with different approaches to depth, breadth, and curricular emphasis (e.g., business, computer science, engineering, information science, math-
ematics, social science, or statistics). It is too early to expect consensus to emerge on certain best practices of data science education. However, it is not too early to envision the possible forms that such practices might take. Nor is it too early to make recommendations that can help the data science education community develop strategic vision and practices. The following is a summary of the findings and recommendations discussed in the preceding four chapters of this report.
Finding 2.1: Data scientists today draw largely from extensions of the “analyst” of years past trained in traditional disciplines. As data science becomes an integral part of many industries and enriches research and development, there will be an increased demand for more holistic and more nuanced data science roles.
Finding 2.2: Data science programs that strive to meet the needs of their students will likely evolve to emphasize certain skills and capabilities. This will result in programs that prepare different types of data scientists.
Recommendation 2.1: Academic institutions should embrace data science as a vital new field that requires specifically tailored instruction delivered through majors and minors in data science as well as the development of a cadre of faculty equipped to teach in this new field.
Recommendation 2.2: Academic institutions should provide and evolve a range of educational pathways to prepare students for an array of data science roles in the workplace.
Finding 2.3: A critical task in the education of future data scientists is to instill data acumen. This requires exposure to key concepts in data science, real-world data and problems that can reinforce the limitations of tools, and ethical considerations that permeate many applications. Key concepts involved in developing data acumen include the following:
- Mathematical foundations,
- Computational foundations,
- Statistical foundations,
- Data management and curation,
- Data description and visualization,
- Data modeling and assessment,
- Workflow and reproducibility,
- Communication and teamwork,
- Domain-specific considerations, and
- Ethical problem solving.
Recommendation 2.3: To prepare their graduates for this new data-driven era, academic institutions should encourage the development of a basic understanding of data science in all undergraduates.
Recommendation 2.4: Ethics is a topic that, given the nature of data science, students should learn and practice throughout their education. Academic institutions should ensure that ethics is woven into the data science curriculum from the beginning and throughout.
Recommendation 2.5: The data science community should adopt a code of ethics; such a code should be affirmed by members of professional societies, included in professional development programs and curricula, and conveyed through educational programs. The code should be reevaluated often in light of new developments.
Finding 3.1: Undergraduate education in data science can be experienced in many forms. These include the following:
- Integrated introductory courses that can satisfy a general education requirement;
- A major in data science, including advanced skills, as the primary field of study;
- A minor or track in data science, where intermediate skills are connected to the major field of study;
- Two-year degrees and certificates;
- Other certificates, often requiring fewer courses than a major but more than a minor;
- Massive open online courses, which can engage large numbers of students at a variety of levels; and
- Summer programs and boot camps, which can serve to supplement academic or on-the-job training.
Recommendation 3.1: Four-year and two-year institutions should establish a forum for dialogue across institutions on all aspects of data science education, training, and workforce development.
Finding 4.1: The nature of data science is such that it offers multiple pathways for students of different backgrounds to engage at levels ranging from basic to expert.
Finding 4.2: Data science would particularly benefit from broad participation by underrepresented minorities because of the many applications to problems of interest to diverse populations.
Recommendation 4.1: As data science programs develop, they should focus on attracting students with varied backgrounds and degrees of preparation and preparing them for success in a variety of careers.
Finding 4.3: Institutional flexibility will involve the development of curricula that take advantage of current course availability and will potentially be constrained by the availability of teaching expertise. Whatever organizational or infrastructure model is adopted, incentives are needed to encourage faculty participation and to overcome barriers.
Finding 4.4: The economics of developing programs has recently changed with the shift to cloud-based approaches and platforms.
Finding 5.1: The evolution of data science programs at a particular institution will depend on the particular institution’s pedagogical style and the students’ backgrounds and goals, as well as the requirements of the job market and graduate schools.
Recommendation 5.1: Because these are early days for undergraduate data science education, academic institutions should be prepared to evolve programs over time. They should create and maintain the flexibility and incentives to facilitate the sharing of courses, materials, and faculty among departments and programs.
Finding 5.2: There is a need for broadening the perspective of faculty who are trained in particular areas of data science to be knowledgeable of the breadth of approaches to data science so that they can more effectively educate students at all levels.
Recommendation 5.2: During the development of data science programs, institutions should provide support so that the faculty can become more cognizant of the varied aspects of data science through discussion, co-teaching, sharing of materials, short courses, and other forms of training.
Finding 5.3: The data science community would benefit from the creation of websites and journals that document and make available best
practices, curricula, education research findings, and other materials related to undergraduate data science education.
Finding 5.4: The evolution of undergraduate education in data science can be driven by data science. Exploiting administrative records, in conjunction with other data sources such as economic information and survey data, can enable effective transformation of programs to better serve their students.
Finding 5.5: Data science methods applied both to individual programs and comparatively across programs can be used for both evaluation and evolution of data science program components. It is essential that both processes are sustained as new pathways emerge at institutions.
Recommendation 5.3: Academic institutions should ensure that programs are continuously evaluated and should work together to develop professional approaches to evaluation. This should include developing and sharing measurement and evaluation frameworks, data sets, and a culture of evolution guided by high-quality evaluation. Efforts should be made to establish relationships with sector-specific professional societies to help align education evaluation with market impacts.
Finding 5.6: As professional societies adapt to data science, improved coordination could offer new opportunities for additional collaboration and cross-pollination. A group or conference with bridging capabilities would be helpful. Professional societies may find it useful to collaborate to offer such training and networking opportunities to their joint communities.
Recommendation 5.4: Existing professional societies should coordinate to enable regular convening sessions on data science among their members. Peer review and discussion are essential to share ideas, best practices, and data.
This page intentionally left blank.
Data science is emerging as a field that is revolutionizing science and industries alike. Work across nearly all domains is becoming more data driven, affecting both the jobs that are available and the skills that are required. As more data and ways of analyzing them become available, more aspects of the economy, society, and daily life will become dependent on data. It is imperative that educators, administrators, and students begin today to consider how to best prepare for and keep pace with this data-driven era of tomorrow. Undergraduate teaching, in particular, offers a critical link in offering more data science exposure to students and expanding the supply of data science talent.
Data Science for Undergraduates: Opportunities and Options offers a vision for the emerging discipline of data science at the undergraduate level. This report outlines some considerations and approaches for academic institutions and others in the broader data science communities to help guide the ongoing transformation of this field.
READ FREE ONLINE
Welcome to OpenBook!
You're looking at OpenBook, NAP.edu's online reading room since 1999. Based on feedback from you, our users, we've made some improvements that make it easier than ever to read thousands of publications on our website.
Do you want to take a quick tour of the OpenBook's features?
Show this book's table of contents , where you can jump to any chapter by name.
...or use these buttons to go back to the previous chapter or skip to the next one.
Jump up to the previous page or down to the next one. Also, you can type in a page number and press Enter to go directly to that page in the book.
Switch between the Original Pages , where you can read the report as it appeared in print, and Text Pages for the web version, where you can highlight and search the text.
To search the entire text of this book, type in your search term here and press Enter .
Share a link to this book page on your preferred social network or via email.
View our suggested citation for this chapter.
Ready to take your reading offline? Click here to buy this book in print or download it as a free PDF, if available.
Get Email Updates
Do you enjoy reading reports from the Academies online for free ? Sign up for email notifications and we'll let you know about new publications in your areas of interest when they're released.

An official website of the United States government
The .gov means it’s official. Federal government websites often end in .gov or .mil. Before sharing sensitive information, make sure you’re on a federal government site.
The site is secure. The https:// ensures that you are connecting to the official website and that any information you provide is encrypted and transmitted securely.
- Publications
- Account settings
Preview improvements coming to the PMC website in October 2024. Learn More or Try it out now .
- Advanced Search
- Journal List
- Springer Nature - PMC COVID-19 Collection

Data Science and Analytics: An Overview from Data-Driven Smart Computing, Decision-Making and Applications Perspective
Iqbal h. sarker.
1 Swinburne University of Technology, Melbourne, VIC 3122 Australia
2 Department of Computer Science and Engineering, Chittagong University of Engineering & Technology, Chittagong, 4349 Bangladesh
The digital world has a wealth of data, such as internet of things (IoT) data, business data, health data, mobile data, urban data, security data, and many more, in the current age of the Fourth Industrial Revolution (Industry 4.0 or 4IR). Extracting knowledge or useful insights from these data can be used for smart decision-making in various applications domains. In the area of data science, advanced analytics methods including machine learning modeling can provide actionable insights or deeper knowledge about data, which makes the computing process automatic and smart. In this paper, we present a comprehensive view on “Data Science” including various types of advanced analytics methods that can be applied to enhance the intelligence and capabilities of an application through smart decision-making in different scenarios. We also discuss and summarize ten potential real-world application domains including business, healthcare, cybersecurity, urban and rural data science, and so on by taking into account data-driven smart computing and decision making. Based on this, we finally highlight the challenges and potential research directions within the scope of our study. Overall, this paper aims to serve as a reference point on data science and advanced analytics to the researchers and decision-makers as well as application developers, particularly from the data-driven solution point of view for real-world problems.
Introduction
We are living in the age of “data science and advanced analytics”, where almost everything in our daily lives is digitally recorded as data [ 17 ]. Thus the current electronic world is a wealth of various kinds of data, such as business data, financial data, healthcare data, multimedia data, internet of things (IoT) data, cybersecurity data, social media data, etc [ 112 ]. The data can be structured, semi-structured, or unstructured, which increases day by day [ 105 ]. Data science is typically a “concept to unify statistics, data analysis, and their related methods” to understand and analyze the actual phenomena with data. According to Cao et al. [ 17 ] “data science is the science of data” or “data science is the study of data”, where a data product is a data deliverable, or data-enabled or guided, which can be a discovery, prediction, service, suggestion, insight into decision-making, thought, model, paradigm, tool, or system. The popularity of “Data science” is increasing day-by-day, which is shown in Fig. Fig.1 1 according to Google Trends data over the last 5 years [ 36 ]. In addition to data science, we have also shown the popularity trends of the relevant areas such as “Data analytics”, “Data mining”, “Big data”, “Machine learning” in the figure. According to Fig. Fig.1, 1 , the popularity indication values for these data-driven domains, particularly “Data science”, and “Machine learning” are increasing day-by-day. This statistical information and the applicability of the data-driven smart decision-making in various real-world application areas, motivate us to study briefly on “Data science” and machine-learning-based “Advanced analytics” in this paper.

The worldwide popularity score of data science comparing with relevant areas in a range of 0 (min) to 100 (max) over time where x -axis represents the timestamp information and y -axis represents the corresponding score
Usually, data science is the field of applying advanced analytics methods and scientific concepts to derive useful business information from data. The emphasis of advanced analytics is more on anticipating the use of data to detect patterns to determine what is likely to occur in the future. Basic analytics offer a description of data in general, while advanced analytics is a step forward in offering a deeper understanding of data and helping to analyze granular data, which we are interested in. In the field of data science, several types of analytics are popular, such as "Descriptive analytics" which answers the question of what happened; "Diagnostic analytics" which answers the question of why did it happen; "Predictive analytics" which predicts what will happen in the future; and "Prescriptive analytics" which prescribes what action should be taken, discussed briefly in “ Advanced analytics methods and smart computing ”. Such advanced analytics and decision-making based on machine learning techniques [ 105 ], a major part of artificial intelligence (AI) [ 102 ] can also play a significant role in the Fourth Industrial Revolution (Industry 4.0) due to its learning capability for smart computing as well as automation [ 121 ].
Although the area of “data science” is huge, we mainly focus on deriving useful insights through advanced analytics, where the results are used to make smart decisions in various real-world application areas. For this, various advanced analytics methods such as machine learning modeling, natural language processing, sentiment analysis, neural network, or deep learning analysis can provide deeper knowledge about data, and thus can be used to develop data-driven intelligent applications. More specifically, regression analysis, classification, clustering analysis, association rules, time-series analysis, sentiment analysis, behavioral patterns, anomaly detection, factor analysis, log analysis, and deep learning which is originated from the artificial neural network, are taken into account in our study. These machine learning-based advanced analytics methods are discussed briefly in “ Advanced analytics methods and smart computing ”. Thus, it’s important to understand the principles of various advanced analytics methods mentioned above and their applicability to apply in various real-world application areas. For instance, in our earlier paper Sarker et al. [ 114 ], we have discussed how data science and machine learning modeling can play a significant role in the domain of cybersecurity for making smart decisions and to provide data-driven intelligent security services. In this paper, we broadly take into account the data science application areas and real-world problems in ten potential domains including the area of business data science, health data science, IoT data science, behavioral data science, urban data science, and so on, discussed briefly in “ Real-world application domains ”.
Based on the importance of machine learning modeling to extract the useful insights from the data mentioned above and data-driven smart decision-making, in this paper, we present a comprehensive view on “Data Science” including various types of advanced analytics methods that can be applied to enhance the intelligence and the capabilities of an application. The key contribution of this study is thus understanding data science modeling, explaining different analytic methods for solution perspective and their applicability in various real-world data-driven applications areas mentioned earlier. Overall, the purpose of this paper is, therefore, to provide a basic guide or reference for those academia and industry people who want to study, research, and develop automated and intelligent applications or systems based on smart computing and decision making within the area of data science.
The main contributions of this paper are summarized as follows:
- To define the scope of our study towards data-driven smart computing and decision-making in our real-world life. We also make a brief discussion on the concept of data science modeling from business problems to data product and automation, to understand its applicability and provide intelligent services in real-world scenarios.
- To provide a comprehensive view on data science including advanced analytics methods that can be applied to enhance the intelligence and the capabilities of an application.
- To discuss the applicability and significance of machine learning-based analytics methods in various real-world application areas. We also summarize ten potential real-world application areas, from business to personalized applications in our daily life, where advanced analytics with machine learning modeling can be used to achieve the expected outcome.
- To highlight and summarize the challenges and potential research directions within the scope of our study.
The rest of the paper is organized as follows. The next section provides the background and related work and defines the scope of our study. The following section presents the concepts of data science modeling for building a data-driven application. After that, briefly discuss and explain different advanced analytics methods and smart computing. Various real-world application areas are discussed and summarized in the next section. We then highlight and summarize several research issues and potential future directions, and finally, the last section concludes this paper.
Background and Related Work
In this section, we first discuss various data terms and works related to data science and highlight the scope of our study.
Data Terms and Definitions
There is a range of key terms in the field, such as data analysis, data mining, data analytics, big data, data science, advanced analytics, machine learning, and deep learning, which are highly related and easily confusing. In the following, we define these terms and differentiate them with the term “Data Science” according to our goal.
The term “Data analysis” refers to the processing of data by conventional (e.g., classic statistical, empirical, or logical) theories, technologies, and tools for extracting useful information and for practical purposes [ 17 ]. The term “Data analytics”, on the other hand, refers to the theories, technologies, instruments, and processes that allow for an in-depth understanding and exploration of actionable data insight [ 17 ]. Statistical and mathematical analysis of the data is the major concern in this process. “Data mining” is another popular term over the last decade, which has a similar meaning with several other terms such as knowledge mining from data, knowledge extraction, knowledge discovery from data (KDD), data/pattern analysis, data archaeology, and data dredging. According to Han et al. [ 38 ], it should have been more appropriately named “knowledge mining from data”. Overall, data mining is defined as the process of discovering interesting patterns and knowledge from large amounts of data [ 38 ]. Data sources may include databases, data centers, the Internet or Web, other repositories of data, or data dynamically streamed through the system. “Big data” is another popular term nowadays, which may change the statistical and data analysis approaches as it has the unique features of “massive, high dimensional, heterogeneous, complex, unstructured, incomplete, noisy, and erroneous” [ 74 ]. Big data can be generated by mobile devices, social networks, the Internet of Things, multimedia, and many other new applications [ 129 ]. Several unique features including volume, velocity, variety, veracity, value (5Vs), and complexity are used to understand and describe big data [ 69 ].
In terms of analytics, basic analytics provides a summary of data whereas the term “Advanced Analytics” takes a step forward in offering a deeper understanding of data and helps to analyze granular data. Advanced analytics is characterized or defined as autonomous or semi-autonomous data or content analysis using advanced techniques and methods to discover deeper insights, predict or generate recommendations, typically beyond traditional business intelligence or analytics. “Machine learning”, a branch of artificial intelligence (AI), is one of the major techniques used in advanced analytics which can automate analytical model building [ 112 ]. This is focused on the premise that systems can learn from data, recognize trends, and make decisions, with minimal human involvement [ 38 , 115 ]. “Deep Learning” is a subfield of machine learning that discusses algorithms inspired by the human brain’s structure and the function called artificial neural networks [ 38 , 139 ].
Unlike the above data-related terms, “Data science” is an umbrella term that encompasses advanced data analytics, data mining, machine, and deep learning modeling, and several other related disciplines like statistics, to extract insights or useful knowledge from the datasets and transform them into actionable business strategies. In [ 17 ], Cao et al. defined data science from the disciplinary perspective as “data science is a new interdisciplinary field that synthesizes and builds on statistics, informatics, computing, communication, management, and sociology to study data and its environments (including domains and other contextual aspects, such as organizational and social aspects) to transform data to insights and decisions by following a data-to-knowledge-to-wisdom thinking and methodology”. In “ Understanding data science modeling ”, we briefly discuss the data science modeling from a practical perspective starting from business problems to data products that can assist the data scientists to think and work in a particular real-world problem domain within the area of data science and analytics.
Related Work
In the area, several papers have been reviewed by the researchers based on data science and its significance. For example, the authors in [ 19 ] identify the evolving field of data science and its importance in the broader knowledge environment and some issues that differentiate data science and informatics issues from conventional approaches in information sciences. Donoho et al. [ 27 ] present 50 years of data science including recent commentary on data science in mass media, and on how/whether data science varies from statistics. The authors formally conceptualize the theory-guided data science (TGDS) model in [ 53 ] and present a taxonomy of research themes in TGDS. Cao et al. include a detailed survey and tutorial on the fundamental aspects of data science in [ 17 ], which considers the transition from data analysis to data science, the principles of data science, as well as the discipline and competence of data education.
Besides, the authors include a data science analysis in [ 20 ], which aims to provide a realistic overview of the use of statistical features and related data science methods in bioimage informatics. The authors in [ 61 ] study the key streams of data science algorithm use at central banks and show how their popularity has risen over time. This research contributes to the creation of a research vector on the role of data science in central banking. In [ 62 ], the authors provide an overview and tutorial on the data-driven design of intelligent wireless networks. The authors in [ 87 ] provide a thorough understanding of computational optimal transport with application to data science. In [ 97 ], the authors present data science as theoretical contributions in information systems via text analytics.
Unlike the above recent studies, in this paper, we concentrate on the knowledge of data science including advanced analytics methods, machine learning modeling, real-world application domains, and potential research directions within the scope of our study. The advanced analytics methods based on machine learning techniques discussed in this paper can be applied to enhance the capabilities of an application in terms of data-driven intelligent decision making and automation in the final data product or systems.
Understanding Data Science Modeling
In this section, we briefly discuss how data science can play a significant role in the real-world business process. For this, we first categorize various types of data and then discuss the major steps of data science modeling starting from business problems to data product and automation.
Types of Real-World Data
Typically, to build a data-driven real-world system in a particular domain, the availability of data is the key [ 17 , 112 , 114 ]. The data can be in different types such as (i) Structured—that has a well-defined data structure and follows a standard order, examples are names, dates, addresses, credit card numbers, stock information, geolocation, etc.; (ii) Unstructured—has no pre-defined format or organization, examples are sensor data, emails, blog entries, wikis, and word processing documents, PDF files, audio files, videos, images, presentations, web pages, etc.; (iii) Semi-structured—has elements of both the structured and unstructured data containing certain organizational properties, examples are HTML, XML, JSON documents, NoSQL databases, etc.; and (iv) Metadata—that represents data about the data, examples are author, file type, file size, creation date and time, last modification date and time, etc. [ 38 , 105 ].
In the area of data science, researchers use various widely-used datasets for different purposes. These are, for example, cybersecurity datasets such as NSL-KDD [ 127 ], UNSW-NB15 [ 79 ], Bot-IoT [ 59 ], ISCX’12 [ 15 ], CIC-DDoS2019 [ 22 ], etc., smartphone datasets such as phone call logs [ 88 , 110 ], mobile application usages logs [ 124 , 149 ], SMS Log [ 28 ], mobile phone notification logs [ 77 ] etc., IoT data [ 56 , 11 , 64 ], health data such as heart disease [ 99 ], diabetes mellitus [ 86 , 147 ], COVID-19 [ 41 , 78 ], etc., agriculture and e-commerce data [ 128 , 150 ], and many more in various application domains. In “ Real-world application domains ”, we discuss ten potential real-world application domains of data science and analytics by taking into account data-driven smart computing and decision making, which can help the data scientists and application developers to explore more in various real-world issues.
Overall, the data used in data-driven applications can be any of the types mentioned above, and they can differ from one application to another in the real world. Data science modeling, which is briefly discussed below, can be used to analyze such data in a specific problem domain and derive insights or useful information from the data to build a data-driven model or data product.
Steps of Data Science Modeling
Data science is typically an umbrella term that encompasses advanced data analytics, data mining, machine, and deep learning modeling, and several other related disciplines like statistics, to extract insights or useful knowledge from the datasets and transform them into actionable business strategies, mentioned earlier in “ Background and related work ”. In this section, we briefly discuss how data science can play a significant role in the real-world business process. Figure Figure2 2 shows an example of data science modeling starting from real-world data to data-driven product and automation. In the following, we briefly discuss each module of the data science process.
- Understanding business problems: This involves getting a clear understanding of the problem that is needed to solve, how it impacts the relevant organization or individuals, the ultimate goals for addressing it, and the relevant project plan. Thus to understand and identify the business problems, the data scientists formulate relevant questions while working with the end-users and other stakeholders. For instance, how much/many, which category/group, is the behavior unrealistic/abnormal, which option should be taken, what action, etc. could be relevant questions depending on the nature of the problems. This helps to get a better idea of what business needs and what we should be extracted from data. Such business knowledge can enable organizations to enhance their decision-making process, is known as “Business Intelligence” [ 65 ]. Identifying the relevant data sources that can help to answer the formulated questions and what kinds of actions should be taken from the trends that the data shows, is another important task associated with this stage. Once the business problem has been clearly stated, the data scientist can define the analytic approach to solve the problem.
- Understanding data: As we know that data science is largely driven by the availability of data [ 114 ]. Thus a sound understanding of the data is needed towards a data-driven model or system. The reason is that real-world data sets are often noisy, missing values, have inconsistencies, or other data issues, which are needed to handle effectively [ 101 ]. To gain actionable insights, the appropriate data or the quality of the data must be sourced and cleansed, which is fundamental to any data science engagement. For this, data assessment that evaluates what data is available and how it aligns to the business problem could be the first step in data understanding. Several aspects such as data type/format, the quantity of data whether it is sufficient or not to extract the useful knowledge, data relevance, authorized access to data, feature or attribute importance, combining multiple data sources, important metrics to report the data, etc. are needed to take into account to clearly understand the data for a particular business problem. Overall, the data understanding module involves figuring out what data would be best needed and the best ways to acquire it.
- Data pre-processing and exploration: Exploratory data analysis is defined in data science as an approach to analyzing datasets to summarize their key characteristics, often with visual methods [ 135 ]. This examines a broad data collection to discover initial trends, attributes, points of interest, etc. in an unstructured manner to construct meaningful summaries of the data. Thus data exploration is typically used to figure out the gist of data and to develop a first step assessment of its quality, quantity, and characteristics. A statistical model can be used or not, but primarily it offers tools for creating hypotheses by generally visualizing and interpreting the data through graphical representation such as a chart, plot, histogram, etc [ 72 , 91 ]. Before the data is ready for modeling, it’s necessary to use data summarization and visualization to audit the quality of the data and provide the information needed to process it. To ensure the quality of the data, the data pre-processing technique, which is typically the process of cleaning and transforming raw data [ 107 ] before processing and analysis is important. It also involves reformatting information, making data corrections, and merging data sets to enrich data. Thus, several aspects such as expected data, data cleaning, formatting or transforming data, dealing with missing values, handling data imbalance and bias issues, data distribution, search for outliers or anomalies in data and dealing with them, ensuring data quality, etc. could be the key considerations in this step.
- Machine learning modeling and evaluation: Once the data is prepared for building the model, data scientists design a model, algorithm, or set of models, to address the business problem. Model building is dependent on what type of analytics, e.g., predictive analytics, is needed to solve the particular problem, which is discussed briefly in “ Advanced analytics methods and smart computing ”. To best fits the data according to the type of analytics, different types of data-driven or machine learning models that have been summarized in our earlier paper Sarker et al. [ 105 ], can be built to achieve the goal. Data scientists typically separate training and test subsets of the given dataset usually dividing in the ratio of 80:20 or data considering the most popular k -folds data splitting method [ 38 ]. This is to observe whether the model performs well or not on the data, to maximize the model performance. Various model validation and assessment metrics, such as error rate, accuracy, true positive, false positive, true negative, false negative, precision, recall, f-score, ROC (receiver operating characteristic curve) analysis, applicability analysis, etc. [ 38 , 115 ] are used to measure the model performance, which can guide the data scientists to choose or design the learning method or model. Besides, machine learning experts or data scientists can take into account several advanced analytics such as feature engineering, feature selection or extraction methods, algorithm tuning, ensemble methods, modifying existing algorithms, or designing new algorithms, etc. to improve the ultimate data-driven model to solve a particular business problem through smart decision making.
- Data product and automation: A data product is typically the output of any data science activity [ 17 ]. A data product, in general terms, is a data deliverable, or data-enabled or guide, which can be a discovery, prediction, service, suggestion, insight into decision-making, thought, model, paradigm, tool, application, or system that process data and generate results. Businesses can use the results of such data analysis to obtain useful information like churn (a measure of how many customers stop using a product) prediction and customer segmentation, and use these results to make smarter business decisions and automation. Thus to make better decisions in various business problems, various machine learning pipelines and data products can be developed. To highlight this, we summarize several potential real-world data science application areas in “ Real-world application domains ”, where various data products can play a significant role in relevant business problems to make them smart and automate.
Overall, we can conclude that data science modeling can be used to help drive changes and improvements in business practices. The interesting part of the data science process indicates having a deeper understanding of the business problem to solve. Without that, it would be much harder to gather the right data and extract the most useful information from the data for making decisions to solve the problem. In terms of role, “Data Scientists” typically interpret and manage data to uncover the answers to major questions that help organizations to make objective decisions and solve complex problems. In a summary, a data scientist proactively gathers and analyzes information from multiple sources to better understand how the business performs, and designs machine learning or data-driven tools/methods, or algorithms, focused on advanced analytics, which can make today’s computing process smarter and intelligent, discussed briefly in the following section.

An example of data science modeling from real-world data to data-driven system and decision making
Advanced Analytics Methods and Smart Computing
As mentioned earlier in “ Background and related work ”, basic analytics provides a summary of data whereas advanced analytics takes a step forward in offering a deeper understanding of data and helps in granular data analysis. For instance, the predictive capabilities of advanced analytics can be used to forecast trends, events, and behaviors. Thus, “advanced analytics” can be defined as the autonomous or semi-autonomous analysis of data or content using advanced techniques and methods to discover deeper insights, make predictions, or produce recommendations, where machine learning-based analytical modeling is considered as the key technologies in the area. In the following section, we first summarize various types of analytics and outcome that are needed to solve the associated business problems, and then we briefly discuss machine learning-based analytical modeling.
Types of Analytics and Outcome
In the real-world business process, several key questions such as “What happened?”, “Why did it happen?”, “What will happen in the future?”, “What action should be taken?” are common and important. Based on these questions, in this paper, we categorize and highlight the analytics into four types such as descriptive, diagnostic, predictive, and prescriptive, which are discussed below.
- Descriptive analytics: It is the interpretation of historical data to better understand the changes that have occurred in a business. Thus descriptive analytics answers the question, “what happened in the past?” by summarizing past data such as statistics on sales and operations or marketing strategies, use of social media, and engagement with Twitter, Linkedin or Facebook, etc. For instance, using descriptive analytics through analyzing trends, patterns, and anomalies, etc., customers’ historical shopping data can be used to predict the probability of a customer purchasing a product. Thus, descriptive analytics can play a significant role to provide an accurate picture of what has occurred in a business and how it relates to previous times utilizing a broad range of relevant business data. As a result, managers and decision-makers can pinpoint areas of strength and weakness in their business, and eventually can take more effective management strategies and business decisions.
- Diagnostic analytics: It is a form of advanced analytics that examines data or content to answer the question, “why did it happen?” The goal of diagnostic analytics is to help to find the root cause of the problem. For example, the human resource management department of a business organization may use these diagnostic analytics to find the best applicant for a position, select them, and compare them to other similar positions to see how well they perform. In a healthcare example, it might help to figure out whether the patients’ symptoms such as high fever, dry cough, headache, fatigue, etc. are all caused by the same infectious agent. Overall, diagnostic analytics enables one to extract value from the data by posing the right questions and conducting in-depth investigations into the answers. It is characterized by techniques such as drill-down, data discovery, data mining, and correlations.
- Predictive analytics: Predictive analytics is an important analytical technique used by many organizations for various purposes such as to assess business risks, anticipate potential market patterns, and decide when maintenance is needed, to enhance their business. It is a form of advanced analytics that examines data or content to answer the question, “what will happen in the future?” Thus, the primary goal of predictive analytics is to identify and typically answer this question with a high degree of probability. Data scientists can use historical data as a source to extract insights for building predictive models using various regression analyses and machine learning techniques, which can be used in various application domains for a better outcome. Companies, for example, can use predictive analytics to minimize costs by better anticipating future demand and changing output and inventory, banks and other financial institutions to reduce fraud and risks by predicting suspicious activity, medical specialists to make effective decisions through predicting patients who are at risk of diseases, retailers to increase sales and customer satisfaction through understanding and predicting customer preferences, manufacturers to optimize production capacity through predicting maintenance requirements, and many more. Thus predictive analytics can be considered as the core analytical method within the area of data science.
- Prescriptive analytics: Prescriptive analytics focuses on recommending the best way forward with actionable information to maximize overall returns and profitability, which typically answer the question, “what action should be taken?” In business analytics, prescriptive analytics is considered the final step. For its models, prescriptive analytics collects data from several descriptive and predictive sources and applies it to the decision-making process. Thus, we can say that it is related to both descriptive analytics and predictive analytics, but it emphasizes actionable insights instead of data monitoring. In other words, it can be considered as the opposite of descriptive analytics, which examines decisions and outcomes after the fact. By integrating big data, machine learning, and business rules, prescriptive analytics helps organizations to make more informed decisions to produce results that drive the most successful business decisions.
In summary, to clarify what happened and why it happened, both descriptive analytics and diagnostic analytics look at the past. Historical data is used by predictive analytics and prescriptive analytics to forecast what will happen in the future and what steps should be taken to impact those effects. In Table Table1, 1 , we have summarized these analytics methods with examples. Forward-thinking organizations in the real world can jointly use these analytical methods to make smart decisions that help drive changes in business processes and improvements. In the following, we discuss how machine learning techniques can play a big role in these analytical methods through their learning capabilities from the data.
Various types of analytical methods with examples
Machine Learning Based Analytical Modeling
In this section, we briefly discuss various advanced analytics methods based on machine learning modeling, which can make the computing process smart through intelligent decision-making in a business process. Figure Figure3 3 shows a general structure of a machine learning-based predictive modeling considering both the training and testing phase. In the following, we discuss a wide range of methods such as regression and classification analysis, association rule analysis, time-series analysis, behavioral analysis, log analysis, and so on within the scope of our study.

A general structure of a machine learning based predictive model considering both the training and testing phase
Regression Analysis
In data science, one of the most common statistical approaches used for predictive modeling and data mining tasks is regression techniques [ 38 ]. Regression analysis is a form of supervised machine learning that examines the relationship between a dependent variable (target) and independent variables (predictor) to predict continuous-valued output [ 105 , 117 ]. The following equations Eqs. 1 , 2 , and 3 [ 85 , 105 ] represent the simple, multiple or multivariate, and polynomial regressions respectively, where x represents independent variable and y is the predicted/target output mentioned above:
Regression analysis is typically conducted for one of two purposes: to predict the value of the dependent variable in the case of individuals for whom some knowledge relating to the explanatory variables is available, or to estimate the effect of some explanatory variable on the dependent variable, i.e., finding the relationship of causal influence between the variables. Linear regression cannot be used to fit non-linear data and may cause an underfitting problem. In that case, polynomial regression performs better, however, increases the model complexity. The regularization techniques such as Ridge, Lasso, Elastic-Net, etc. [ 85 , 105 ] can be used to optimize the linear regression model. Besides, support vector regression, decision tree regression, random forest regression techniques [ 85 , 105 ] can be used for building effective regression models depending on the problem type, e.g., non-linear tasks. Financial forecasting or prediction, cost estimation, trend analysis, marketing, time-series estimation, drug response modeling, etc. are some examples where the regression models can be used to solve real-world problems in the domain of data science and analytics.
Classification Analysis
Classification is one of the most widely used and best-known data science processes. This is a form of supervised machine learning approach that also refers to a predictive modeling problem in which a class label is predicted for a given example [ 38 ]. Spam identification, such as ‘spam’ and ‘not spam’ in email service providers, can be an example of a classification problem. There are several forms of classification analysis available in the area such as binary classification—which refers to the prediction of one of two classes; multi-class classification—which involves the prediction of one of more than two classes; multi-label classification—a generalization of multiclass classification in which the problem’s classes are organized hierarchically [ 105 ].
Several popular classification techniques, such as k-nearest neighbors [ 5 ], support vector machines [ 55 ], navies Bayes [ 49 ], adaptive boosting [ 32 ], extreme gradient boosting [ 85 ], logistic regression [ 66 ], decision trees ID3 [ 92 ], C4.5 [ 93 ], and random forests [ 13 ] exist to solve classification problems. The tree-based classification technique, e.g., random forest considering multiple decision trees, performs better than others to solve real-world problems in many cases as due to its capability of producing logic rules [ 103 , 115 ]. Figure Figure4 4 shows an example of a random forest structure considering multiple decision trees. In addition, BehavDT recently proposed by Sarker et al. [ 109 ], and IntrudTree [ 106 ] can be used for building effective classification or prediction models in the relevant tasks within the domain of data science and analytics.

An example of a random forest structure considering multiple decision trees
Cluster Analysis
Clustering is a form of unsupervised machine learning technique and is well-known in many data science application areas for statistical data analysis [ 38 ]. Usually, clustering techniques search for the structures inside a dataset and, if the classification is not previously identified, classify homogeneous groups of cases. This means that data points are identical to each other within a cluster, and different from data points in another cluster. Overall, the purpose of cluster analysis is to sort various data points into groups (or clusters) that are homogeneous internally and heterogeneous externally [ 105 ]. To gain insight into how data is distributed in a given dataset or as a preprocessing phase for other algorithms, clustering is often used. Data clustering, for example, assists with customer shopping behavior, sales campaigns, and retention of consumers for retail businesses, anomaly detection, etc.
Many clustering algorithms with the ability to group data have been proposed in machine learning and data science literature [ 98 , 138 , 141 ]. In our earlier paper Sarker et al. [ 105 ], we have summarized this based on several perspectives, such as partitioning methods, density-based methods, hierarchical-based methods, model-based methods, etc. In the literature, the popular K-means [ 75 ], K-Mediods [ 84 ], CLARA [ 54 ] etc. are known as partitioning methods; DBSCAN [ 30 ], OPTICS [ 8 ] etc. are known as density-based methods; single linkage [ 122 ], complete linkage [ 123 ], etc. are known as hierarchical methods. In addition, grid-based clustering methods, such as STING [ 134 ], CLIQUE [ 2 ], etc.; model-based clustering such as neural network learning [ 141 ], GMM [ 94 ], SOM [ 18 , 104 ], etc.; constrained-based methods such as COP K-means [ 131 ], CMWK-Means [ 25 ], etc. are used in the area. Recently, Sarker et al. [ 111 ] proposed a hierarchical clustering method, BOTS [ 111 ] based on bottom-up agglomerative technique for capturing user’s similar behavioral characteristics over time. The key benefit of agglomerative hierarchical clustering is that the tree-structure hierarchy created by agglomerative clustering is more informative than an unstructured set of flat clusters, which can assist in better decision-making in relevant application areas in data science.
Association Rule Analysis
Association rule learning is known as a rule-based machine learning system, an unsupervised learning method is typically used to establish a relationship among variables. This is a descriptive technique often used to analyze large datasets for discovering interesting relationships or patterns. The association learning technique’s main strength is its comprehensiveness, as it produces all associations that meet user-specified constraints including minimum support and confidence value [ 138 ].
Association rules allow a data scientist to identify trends, associations, and co-occurrences between data sets inside large data collections. In a supermarket, for example, associations infer knowledge about the buying behavior of consumers for different items, which helps to change the marketing and sales plan. In healthcare, to better diagnose patients, physicians may use association guidelines. Doctors can assess the conditional likelihood of a given illness by comparing symptom associations in the data from previous cases using association rules and machine learning-based data analysis. Similarly, association rules are useful for consumer behavior analysis and prediction, customer market analysis, bioinformatics, weblog mining, recommendation systems, etc.
Several types of association rules have been proposed in the area, such as frequent pattern based [ 4 , 47 , 73 ], logic-based [ 31 ], tree-based [ 39 ], fuzzy-rules [ 126 ], belief rule [ 148 ] etc. The rule learning techniques such as AIS [ 3 ], Apriori [ 4 ], Apriori-TID and Apriori-Hybrid [ 4 ], FP-Tree [ 39 ], Eclat [ 144 ], RARM [ 24 ] exist to solve the relevant business problems. Apriori [ 4 ] is the most commonly used algorithm for discovering association rules from a given dataset among the association rule learning techniques [ 145 ]. The recent association rule-learning technique ABC-RuleMiner proposed in our earlier paper by Sarker et al. [ 113 ] could give significant results in terms of generating non-redundant rules that can be used for smart decision making according to human preferences, within the area of data science applications.
Time-Series Analysis and Forecasting
A time series is typically a series of data points indexed in time order particularly, by date, or timestamp [ 111 ]. Depending on the frequency, the time-series can be different types such as annually, e.g., annual budget, quarterly, e.g., expenditure, monthly, e.g., air traffic, weekly, e.g., sales quantity, daily, e.g., weather, hourly, e.g., stock price, minute-wise, e.g., inbound calls in a call center, and even second-wise, e.g., web traffic, and so on in relevant domains.
A mathematical method dealing with such time-series data, or the procedure of fitting a time series to a proper model is termed time-series analysis. Many different time series forecasting algorithms and analysis methods can be applied to extract the relevant information. For instance, to do time-series forecasting for future patterns, the autoregressive (AR) model [ 130 ] learns the behavioral trends or patterns of past data. Moving average (MA) [ 40 ] is another simple and common form of smoothing used in time series analysis and forecasting that uses past forecasted errors in a regression-like model to elaborate an averaged trend across the data. The autoregressive moving average (ARMA) [ 12 , 120 ] combines these two approaches, where autoregressive extracts the momentum and pattern of the trend and moving average capture the noise effects. The most popular and frequently used time-series model is the autoregressive integrated moving average (ARIMA) model [ 12 , 120 ]. ARIMA model, a generalization of an ARMA model, is more flexible than other statistical models such as exponential smoothing or simple linear regression. In terms of data, the ARMA model can only be used for stationary time-series data, while the ARIMA model includes the case of non-stationarity as well. Similarly, seasonal autoregressive integrated moving average (SARIMA), autoregressive fractionally integrated moving average (ARFIMA), autoregressive moving average model with exogenous inputs model (ARMAX model) are also used in time-series models [ 120 ].
In addition to the stochastic methods for time-series modeling and forecasting, machine and deep learning-based approach can be used for effective time-series analysis and forecasting. For instance, in our earlier paper, Sarker et al. [ 111 ] present a bottom-up clustering-based time-series analysis to capture the mobile usage behavioral patterns of the users. Figure Figure5 5 shows an example of producing aggregate time segments Seg_i from initial time slices TS_i based on similar behavioral characteristics that are used in our bottom-up clustering approach, where D represents the dominant behavior BH_i of the users, mentioned above [ 111 ]. The authors in [ 118 ], used a long short-term memory (LSTM) model, a kind of recurrent neural network (RNN) deep learning model, in forecasting time-series that outperform traditional approaches such as the ARIMA model. Time-series analysis is commonly used these days in various fields such as financial, manufacturing, business, social media, event data (e.g., clickstreams and system events), IoT and smartphone data, and generally in any applied science and engineering temporal measurement domain. Thus, it covers a wide range of application areas in data science.

An example of producing aggregate time segments from initial time slices based on similar behavioral characteristics
Opinion Mining and Sentiment Analysis
Sentiment analysis or opinion mining is the computational study of the opinions, thoughts, emotions, assessments, and attitudes of people towards entities such as products, services, organizations, individuals, issues, events, topics, and their attributes [ 71 ]. There are three kinds of sentiments: positive, negative, and neutral, along with more extreme feelings such as angry, happy and sad, or interested or not interested, etc. More refined sentiments to evaluate the feelings of individuals in various situations can also be found according to the problem domain.
Although the task of opinion mining and sentiment analysis is very challenging from a technical point of view, it’s very useful in real-world practice. For instance, a business always aims to obtain an opinion from the public or customers about its products and services to refine the business policy as well as a better business decision. It can thus benefit a business to understand the social opinion of their brand, product, or service. Besides, potential customers want to know what consumers believe they have when they use a service or purchase a product. Document-level, sentence level, aspect level, and concept level, are the possible levels of opinion mining in the area [ 45 ].
Several popular techniques such as lexicon-based including dictionary-based and corpus-based methods, machine learning including supervised and unsupervised learning, deep learning, and hybrid methods are used in sentiment analysis-related tasks [ 70 ]. To systematically define, extract, measure, and analyze affective states and subjective knowledge, it incorporates the use of statistics, natural language processing (NLP), machine learning as well as deep learning methods. Sentiment analysis is widely used in many applications, such as reviews and survey data, web and social media, and healthcare content, ranging from marketing and customer support to clinical practice. Thus sentiment analysis has a big influence in many data science applications, where public sentiment is involved in various real-world issues.
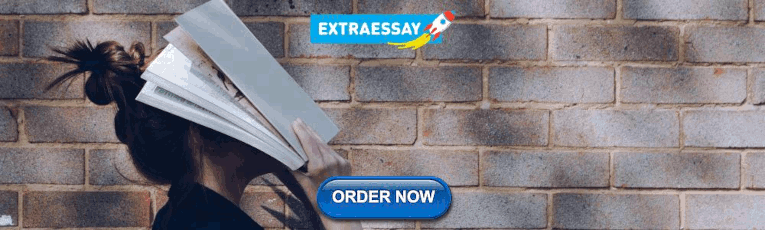
Behavioral Data and Cohort Analysis
Behavioral analytics is a recent trend that typically reveals new insights into e-commerce sites, online gaming, mobile and smartphone applications, IoT user behavior, and many more [ 112 ]. The behavioral analysis aims to understand how and why the consumers or users behave, allowing accurate predictions of how they are likely to behave in the future. For instance, it allows advertisers to make the best offers with the right client segments at the right time. Behavioral analytics, including traffic data such as navigation paths, clicks, social media interactions, purchase decisions, and marketing responsiveness, use the large quantities of raw user event information gathered during sessions in which people use apps, games, or websites. In our earlier papers Sarker et al. [ 101 , 111 , 113 ] we have discussed how to extract users phone usage behavioral patterns utilizing real-life phone log data for various purposes.
In the real-world scenario, behavioral analytics is often used in e-commerce, social media, call centers, billing systems, IoT systems, political campaigns, and other applications, to find opportunities for optimization to achieve particular outcomes. Cohort analysis is a branch of behavioral analytics that involves studying groups of people over time to see how their behavior changes. For instance, it takes data from a given data set (e.g., an e-commerce website, web application, or online game) and separates it into related groups for analysis. Various machine learning techniques such as behavioral data clustering [ 111 ], behavioral decision tree classification [ 109 ], behavioral association rules [ 113 ], etc. can be used in the area according to the goal. Besides, the concept of RecencyMiner, proposed in our earlier paper Sarker et al. [ 108 ] that takes into account recent behavioral patterns could be effective while analyzing behavioral data as it may not be static in the real-world changes over time.
Anomaly Detection or Outlier Analysis
Anomaly detection, also known as Outlier analysis is a data mining step that detects data points, events, and/or findings that deviate from the regularities or normal behavior of a dataset. Anomalies are usually referred to as outliers, abnormalities, novelties, noise, inconsistency, irregularities, and exceptions [ 63 , 114 ]. Techniques of anomaly detection may discover new situations or cases as deviant based on historical data through analyzing the data patterns. For instance, identifying fraud or irregular transactions in finance is an example of anomaly detection.
It is often used in preprocessing tasks for the deletion of anomalous or inconsistency in the real-world data collected from various data sources including user logs, devices, networks, and servers. For anomaly detection, several machine learning techniques can be used, such as k-nearest neighbors, isolation forests, cluster analysis, etc [ 105 ]. The exclusion of anomalous data from the dataset also results in a statistically significant improvement in accuracy during supervised learning [ 101 ]. However, extracting appropriate features, identifying normal behaviors, managing imbalanced data distribution, addressing variations in abnormal behavior or irregularities, the sparse occurrence of abnormal events, environmental variations, etc. could be challenging in the process of anomaly detection. Detection of anomalies can be applicable in a variety of domains such as cybersecurity analytics, intrusion detections, fraud detection, fault detection, health analytics, identifying irregularities, detecting ecosystem disturbances, and many more. This anomaly detection can be considered a significant task for building effective systems with higher accuracy within the area of data science.
Factor Analysis
Factor analysis is a collection of techniques for describing the relationships or correlations between variables in terms of more fundamental entities known as factors [ 23 ]. It’s usually used to organize variables into a small number of clusters based on their common variance, where mathematical or statistical procedures are used. The goals of factor analysis are to determine the number of fundamental influences underlying a set of variables, calculate the degree to which each variable is associated with the factors, and learn more about the existence of the factors by examining which factors contribute to output on which variables. The broad purpose of factor analysis is to summarize data so that relationships and patterns can be easily interpreted and understood [ 143 ].
Exploratory factor analysis (EFA) and confirmatory factor analysis (CFA) are the two most popular factor analysis techniques. EFA seeks to discover complex trends by analyzing the dataset and testing predictions, while CFA tries to validate hypotheses and uses path analysis diagrams to represent variables and factors [ 143 ]. Factor analysis is one of the algorithms for unsupervised machine learning that is used for minimizing dimensionality. The most common methods for factor analytics are principal components analysis (PCA), principal axis factoring (PAF), and maximum likelihood (ML) [ 48 ]. Methods of correlation analysis such as Pearson correlation, canonical correlation, etc. may also be useful in the field as they can quantify the statistical relationship between two continuous variables, or association. Factor analysis is commonly used in finance, marketing, advertising, product management, psychology, and operations research, and thus can be considered as another significant analytical method within the area of data science.
Log Analysis
Logs are commonly used in system management as logs are often the only data available that record detailed system runtime activities or behaviors in production [ 44 ]. Log analysis is thus can be considered as the method of analyzing, interpreting, and capable of understanding computer-generated records or messages, also known as logs. This can be device log, server log, system log, network log, event log, audit trail, audit record, etc. The process of creating such records is called data logging.
Logs are generated by a wide variety of programmable technologies, including networking devices, operating systems, software, and more. Phone call logs [ 88 , 110 ], SMS Logs [ 28 ], mobile apps usages logs [ 124 , 149 ], notification logs [ 77 ], game Logs [ 82 ], context logs [ 16 , 149 ], web logs [ 37 ], smartphone life logs [ 95 ], etc. are some examples of log data for smartphone devices. The main characteristics of these log data is that it contains users’ actual behavioral activities with their devices. Similar other log data can be search logs [ 50 , 133 ], application logs [ 26 ], server logs [ 33 ], network logs [ 57 ], event logs [ 83 ], network and security logs [ 142 ] etc.
Several techniques such as classification and tagging, correlation analysis, pattern recognition methods, anomaly detection methods, machine learning modeling, etc. [ 105 ] can be used for effective log analysis. Log analysis can assist in compliance with security policies and industry regulations, as well as provide a better user experience by encouraging the troubleshooting of technical problems and identifying areas where efficiency can be improved. For instance, web servers use log files to record data about website visitors. Windows event log analysis can help an investigator draw a timeline based on the logging information and the discovered artifacts. Overall, advanced analytics methods by taking into account machine learning modeling can play a significant role to extract insightful patterns from these log data, which can be used for building automated and smart applications, and thus can be considered as a key working area in data science.
Neural Networks and Deep Learning Analysis
Deep learning is a form of machine learning that uses artificial neural networks to create a computational architecture that learns from data by combining multiple processing layers, such as the input, hidden, and output layers [ 38 ]. The key benefit of deep learning over conventional machine learning methods is that it performs better in a variety of situations, particularly when learning from large datasets [ 114 , 140 ].
The most common deep learning algorithms are: multi-layer perceptron (MLP) [ 85 ], convolutional neural network (CNN or ConvNet) [ 67 ], long short term memory recurrent neural network (LSTM-RNN) [ 34 ]. Figure Figure6 6 shows a structure of an artificial neural network modeling with multiple processing layers. The Backpropagation technique [ 38 ] is used to adjust the weight values internally while building the model. Convolutional neural networks (CNNs) [ 67 ] improve on the design of traditional artificial neural networks (ANNs), which include convolutional layers, pooling layers, and fully connected layers. It is commonly used in a variety of fields, including natural language processing, speech recognition, image processing, and other autocorrelated data since it takes advantage of the two-dimensional (2D) structure of the input data. AlexNet [ 60 ], Xception [ 21 ], Inception [ 125 ], Visual Geometry Group (VGG) [ 42 ], ResNet [ 43 ], etc., and other advanced deep learning models based on CNN are also used in the field.

A structure of an artificial neural network modeling with multiple processing layers
In addition to CNN, recurrent neural network (RNN) architecture is another popular method used in deep learning. Long short-term memory (LSTM) is a popular type of recurrent neural network architecture used broadly in the area of deep learning. Unlike traditional feed-forward neural networks, LSTM has feedback connections. Thus, LSTM networks are well-suited for analyzing and learning sequential data, such as classifying, sorting, and predicting data based on time-series data. Therefore, when the data is in a sequential format, such as time, sentence, etc., LSTM can be used, and it is widely used in the areas of time-series analysis, natural language processing, speech recognition, and so on.
In addition to the most popular deep learning methods mentioned above, several other deep learning approaches [ 104 ] exist in the field for various purposes. The self-organizing map (SOM) [ 58 ], for example, uses unsupervised learning to represent high-dimensional data as a 2D grid map, reducing dimensionality. Another learning technique that is commonly used for dimensionality reduction and feature extraction in unsupervised learning tasks is the autoencoder (AE) [ 10 ]. Restricted Boltzmann machines (RBM) can be used for dimensionality reduction, classification, regression, collaborative filtering, feature learning, and topic modeling, according to [ 46 ]. A deep belief network (DBN) is usually made up of a backpropagation neural network and unsupervised networks like restricted Boltzmann machines (RBMs) or autoencoders (BPNN) [ 136 ]. A generative adversarial network (GAN) [ 35 ] is a deep learning network that can produce data with characteristics that are similar to the input data. Transfer learning is common worldwide presently because it can train deep neural networks with a small amount of data, which is usually the re-use of a pre-trained model on a new problem [ 137 ]. These deep learning methods can perform well, particularly, when learning from large-scale datasets [ 105 , 140 ]. In our previous article Sarker et al. [ 104 ], we have summarized a brief discussion of various artificial neural networks (ANN) and deep learning (DL) models mentioned above, which can be used in a variety of data science and analytics tasks.
Real-World Application Domains
Almost every industry or organization is impacted by data, and thus “Data Science” including advanced analytics with machine learning modeling can be used in business, marketing, finance, IoT systems, cybersecurity, urban management, health care, government policies, and every possible industries, where data gets generated. In the following, we discuss ten most popular application areas based on data science and analytics.
- Business or financial data science: In general, business data science can be considered as the study of business or e-commerce data to obtain insights about a business that can typically lead to smart decision-making as well as taking high-quality actions [ 90 ]. Data scientists can develop algorithms or data-driven models predicting customer behavior, identifying patterns and trends based on historical business data, which can help companies to reduce costs, improve service delivery, and generate recommendations for better decision-making. Eventually, business automation, intelligence, and efficiency can be achieved through the data science process discussed earlier, where various advanced analytics methods and machine learning modeling based on the collected data are the keys. Many online retailers, such as Amazon [ 76 ], can improve inventory management, avoid out-of-stock situations, and optimize logistics and warehousing using predictive modeling based on machine learning techniques [ 105 ]. In terms of finance, the historical data is related to financial institutions to make high-stakes business decisions, which is mostly used for risk management, fraud prevention, credit allocation, customer analytics, personalized services, algorithmic trading, etc. Overall, data science methodologies can play a key role in the future generation business or finance industry, particularly in terms of business automation, intelligence, and smart decision-making and systems.
- Manufacturing or industrial data science: To compete in global production capability, quality, and cost, manufacturing industries have gone through many industrial revolutions [ 14 ]. The latest fourth industrial revolution, also known as Industry 4.0, is the emerging trend of automation and data exchange in manufacturing technology. Thus industrial data science, which is the study of industrial data to obtain insights that can typically lead to optimizing industrial applications, can play a vital role in such revolution. Manufacturing industries generate a large amount of data from various sources such as sensors, devices, networks, systems, and applications [ 6 , 68 ]. The main categories of industrial data include large-scale data devices, life-cycle production data, enterprise operation data, manufacturing value chain sources, and collaboration data from external sources [ 132 ]. The data needs to be processed, analyzed, and secured to help improve the system’s efficiency, safety, and scalability. Data science modeling thus can be used to maximize production, reduce costs and raise profits in manufacturing industries.
- Medical or health data science: Healthcare is one of the most notable fields where data science is making major improvements. Health data science involves the extrapolation of actionable insights from sets of patient data, typically collected from electronic health records. To help organizations, improve the quality of treatment, lower the cost of care, and improve the patient experience, data can be obtained from several sources, e.g., the electronic health record, billing claims, cost estimates, and patient satisfaction surveys, etc., to analyze. In reality, healthcare analytics using machine learning modeling can minimize medical costs, predict infectious outbreaks, prevent preventable diseases, and generally improve the quality of life [ 81 , 119 ]. Across the global population, the average human lifespan is growing, presenting new challenges to today’s methods of delivery of care. Thus health data science modeling can play a role in analyzing current and historical data to predict trends, improve services, and even better monitor the spread of diseases. Eventually, it may lead to new approaches to improve patient care, clinical expertise, diagnosis, and management.
- IoT data science: Internet of things (IoT) [ 9 ] is a revolutionary technical field that turns every electronic system into a smarter one and is therefore considered to be the big frontier that can enhance almost all activities in our lives. Machine learning has become a key technology for IoT applications because it uses expertise to identify patterns and generate models that help predict future behavior and events [ 112 ]. One of the IoT’s main fields of application is a smart city, which uses technology to improve city services and citizens’ living experiences. For example, using the relevant data, data science methods can be used for traffic prediction in smart cities, to estimate the total usage of energy of the citizens for a particular period. Deep learning-based models in data science can be built based on a large scale of IoT datasets [ 7 , 104 ]. Overall, data science and analytics approaches can aid modeling in a variety of IoT and smart city services, including smart governance, smart homes, education, connectivity, transportation, business, agriculture, health care, and industry, and many others.
- Cybersecurity data science: Cybersecurity, or the practice of defending networks, systems, hardware, and data from digital attacks, is one of the most important fields of Industry 4.0 [ 114 , 121 ]. Data science techniques, particularly machine learning, have become a crucial cybersecurity technology that continually learns to identify trends by analyzing data, better detecting malware in encrypted traffic, finding insider threats, predicting where bad neighborhoods are online, keeping people safe while surfing, or protecting information in the cloud by uncovering suspicious user activity [ 114 ]. For instance, machine learning and deep learning-based security modeling can be used to effectively detect various types of cyberattacks or anomalies [ 103 , 106 ]. To generate security policy rules, association rule learning can play a significant role to build rule-based systems [ 102 ]. Deep learning-based security models can perform better when utilizing the large scale of security datasets [ 140 ]. Thus data science modeling can enable professionals in cybersecurity to be more proactive in preventing threats and reacting in real-time to active attacks, through extracting actionable insights from the security datasets.
- Behavioral data science: Behavioral data is information produced as a result of activities, most commonly commercial behavior, performed on a variety of Internet-connected devices, such as a PC, tablet, or smartphones [ 112 ]. Websites, mobile applications, marketing automation systems, call centers, help desks, and billing systems, etc. are all common sources of behavioral data. Behavioral data is much more than just data, which is not static data [ 108 ]. Advanced analytics of these data including machine learning modeling can facilitate in several areas such as predicting future sales trends and product recommendations in e-commerce and retail; predicting usage trends, load, and user preferences in future releases in online gaming; determining how users use an application to predict future usage and preferences in application development; breaking users down into similar groups to gain a more focused understanding of their behavior in cohort analysis; detecting compromised credentials and insider threats by locating anomalous behavior, or making suggestions, etc. Overall, behavioral data science modeling typically enables to make the right offers to the right consumers at the right time on various common platforms such as e-commerce platforms, online games, web and mobile applications, and IoT. In social context, analyzing the behavioral data of human being using advanced analytics methods and the extracted insights from social data can be used for data-driven intelligent social services, which can be considered as social data science.
- Mobile data science: Today’s smart mobile phones are considered as “next-generation, multi-functional cell phones that facilitate data processing, as well as enhanced wireless connectivity” [ 146 ]. In our earlier paper [ 112 ], we have shown that users’ interest in “Mobile Phones” is more and more than other platforms like “Desktop Computer”, “Laptop Computer” or “Tablet Computer” in recent years. People use smartphones for a variety of activities, including e-mailing, instant messaging, online shopping, Internet surfing, entertainment, social media such as Facebook, Linkedin, and Twitter, and various IoT services such as smart cities, health, and transportation services, and many others. Intelligent apps are based on the extracted insight from the relevant datasets depending on apps characteristics, such as action-oriented, adaptive in nature, suggestive and decision-oriented, data-driven, context-awareness, and cross-platform operation [ 112 ]. As a result, mobile data science, which involves gathering a large amount of mobile data from various sources and analyzing it using machine learning techniques to discover useful insights or data-driven trends, can play an important role in the development of intelligent smartphone applications.
- Multimedia data science: Over the last few years, a big data revolution in multimedia management systems has resulted from the rapid and widespread use of multimedia data, such as image, audio, video, and text, as well as the ease of access and availability of multimedia sources. Currently, multimedia sharing websites, such as Yahoo Flickr, iCloud, and YouTube, and social networks such as Facebook, Instagram, and Twitter, are considered as valuable sources of multimedia big data [ 89 ]. People, particularly younger generations, spend a lot of time on the Internet and social networks to connect with others, exchange information, and create multimedia data, thanks to the advent of new technology and the advanced capabilities of smartphones and tablets. Multimedia analytics deals with the problem of effectively and efficiently manipulating, handling, mining, interpreting, and visualizing various forms of data to solve real-world problems. Text analysis, image or video processing, computer vision, audio or speech processing, and database management are among the solutions available for a range of applications including healthcare, education, entertainment, and mobile devices.
- Smart cities or urban data science: Today, more than half of the world’s population live in urban areas or cities [ 80 ] and considered as drivers or hubs of economic growth, wealth creation, well-being, and social activity [ 96 , 116 ]. In addition to cities, “Urban area” can refer to the surrounding areas such as towns, conurbations, or suburbs. Thus, a large amount of data documenting daily events, perceptions, thoughts, and emotions of citizens or people are recorded, that are loosely categorized into personal data, e.g., household, education, employment, health, immigration, crime, etc., proprietary data, e.g., banking, retail, online platforms data, etc., government data, e.g., citywide crime statistics, or government institutions, etc., Open and public data, e.g., data.gov, ordnance survey, and organic and crowdsourced data, e.g., user-generated web data, social media, Wikipedia, etc. [ 29 ]. The field of urban data science typically focuses on providing more effective solutions from a data-driven perspective, through extracting knowledge and actionable insights from such urban data. Advanced analytics of these data using machine learning techniques [ 105 ] can facilitate the efficient management of urban areas including real-time management, e.g., traffic flow management, evidence-based planning decisions which pertain to the longer-term strategic role of forecasting for urban planning, e.g., crime prevention, public safety, and security, or framing the future, e.g., political decision-making [ 29 ]. Overall, it can contribute to government and public planning, as well as relevant sectors including retail, financial services, mobility, health, policing, and utilities within a data-rich urban environment through data-driven smart decision-making and policies, which lead to smart cities and improve the quality of human life.
- Smart villages or rural data science: Rural areas or countryside are the opposite of urban areas, that include villages, hamlets, or agricultural areas. The field of rural data science typically focuses on making better decisions and providing more effective solutions that include protecting public safety, providing critical health services, agriculture, and fostering economic development from a data-driven perspective, through extracting knowledge and actionable insights from the collected rural data. Advanced analytics of rural data including machine learning [ 105 ] modeling can facilitate providing new opportunities for them to build insights and capacity to meet current needs and prepare for their futures. For instance, machine learning modeling [ 105 ] can help farmers to enhance their decisions to adopt sustainable agriculture utilizing the increasing amount of data captured by emerging technologies, e.g., the internet of things (IoT), mobile technologies and devices, etc. [ 1 , 51 , 52 ]. Thus, rural data science can play a very important role in the economic and social development of rural areas, through agriculture, business, self-employment, construction, banking, healthcare, governance, or other services, etc. that lead to smarter villages.
Overall, we can conclude that data science modeling can be used to help drive changes and improvements in almost every sector in our real-world life, where the relevant data is available to analyze. To gather the right data and extract useful knowledge or actionable insights from the data for making smart decisions is the key to data science modeling in any application domain. Based on our discussion on the above ten potential real-world application domains by taking into account data-driven smart computing and decision making, we can say that the prospects of data science and the role of data scientists are huge for the future world. The “Data Scientists” typically analyze information from multiple sources to better understand the data and business problems, and develop machine learning-based analytical modeling or algorithms, or data-driven tools, or solutions, focused on advanced analytics, which can make today’s computing process smarter, automated, and intelligent.
Challenges and Research Directions
Our study on data science and analytics, particularly data science modeling in “ Understanding data science modeling ”, advanced analytics methods and smart computing in “ Advanced analytics methods and smart computing ”, and real-world application areas in “ Real-world application domains ” open several research issues in the area of data-driven business solutions and eventual data products. Thus, in this section, we summarize and discuss the challenges faced and the potential research opportunities and future directions to build data-driven products.
- Understanding the real-world business problems and associated data including nature, e.g., what forms, type, size, labels, etc., is the first challenge in the data science modeling, discussed briefly in “ Understanding data science modeling ”. This is actually to identify, specify, represent and quantify the domain-specific business problems and data according to the requirements. For a data-driven effective business solution, there must be a well-defined workflow before beginning the actual data analysis work. Furthermore, gathering business data is difficult because data sources can be numerous and dynamic. As a result, gathering different forms of real-world data, such as structured, or unstructured, related to a specific business issue with legal access, which varies from application to application, is challenging. Moreover, data annotation, which is typically the process of categorization, tagging, or labeling of raw data, for the purpose of building data-driven models, is another challenging issue. Thus, the primary task is to conduct a more in-depth analysis of data collection and dynamic annotation methods. Therefore, understanding the business problem, as well as integrating and managing the raw data gathered for efficient data analysis, may be one of the most challenging aspects of working in the field of data science and analytics.
- The next challenge is the extraction of the relevant and accurate information from the collected data mentioned above. The main focus of data scientists is typically to disclose, describe, represent, and capture data-driven intelligence for actionable insights from data. However, the real-world data may contain many ambiguous values, missing values, outliers, and meaningless data [ 101 ]. The advanced analytics methods including machine and deep learning modeling, discussed in “ Advanced analytics methods and smart computing ”, highly impact the quality, and availability of the data. Thus understanding real-world business scenario and associated data, to whether, how, and why they are insufficient, missing, or problematic, then extend or redevelop the existing methods, such as large-scale hypothesis testing, learning inconsistency, and uncertainty, etc. to address the complexities in data and business problems is important. Therefore, developing new techniques to effectively pre-process the diverse data collected from multiple sources, according to their nature and characteristics could be another challenging task.
- Understanding and selecting the appropriate analytical methods to extract the useful insights for smart decision-making for a particular business problem is the main issue in the area of data science. The emphasis of advanced analytics is more on anticipating the use of data to detect patterns to determine what is likely to occur in the future. Basic analytics offer a description of data in general, while advanced analytics is a step forward in offering a deeper understanding of data and helping to granular data analysis. Thus, understanding the advanced analytics methods, especially machine and deep learning-based modeling is the key. The traditional learning techniques mentioned in “ Advanced analytics methods and smart computing ” may not be directly applicable for the expected outcome in many cases. For instance, in a rule-based system, the traditional association rule learning technique [ 4 ] may produce redundant rules from the data that makes the decision-making process complex and ineffective [ 113 ]. Thus, a scientific understanding of the learning algorithms, mathematical properties, how the techniques are robust or fragile to input data, is needed to understand. Therefore, a deeper understanding of the strengths and drawbacks of the existing machine and deep learning methods [ 38 , 105 ] to solve a particular business problem is needed, consequently to improve or optimize the learning algorithms according to the data characteristics, or to propose the new algorithm/techniques with higher accuracy becomes a significant challenging issue for the future generation data scientists.
- The traditional data-driven models or systems typically use a large amount of business data to generate data-driven decisions. In several application fields, however, the new trends are more likely to be interesting and useful for modeling and predicting the future than older ones. For example, smartphone user behavior modeling, IoT services, stock market forecasting, health or transport service, job market analysis, and other related areas where time-series and actual human interests or preferences are involved over time. Thus, rather than considering the traditional data analysis, the concept of RecencyMiner, i.e., recent pattern-based extracted insight or knowledge proposed in our earlier paper Sarker et al. [ 108 ] might be effective. Therefore, to propose the new techniques by taking into account the recent data patterns, and consequently to build a recency-based data-driven model for solving real-world problems, is another significant challenging issue in the area.
- The most crucial task for a data-driven smart system is to create a framework that supports data science modeling discussed in “ Understanding data science modeling ”. As a result, advanced analytical methods based on machine learning or deep learning techniques can be considered in such a system to make the framework capable of resolving the issues. Besides, incorporating contextual information such as temporal context, spatial context, social context, environmental context, etc. [ 100 ] can be used for building an adaptive, context-aware, and dynamic model or framework, depending on the problem domain. As a result, a well-designed data-driven framework, as well as experimental evaluation, is a very important direction to effectively solve a business problem in a particular domain, as well as a big challenge for the data scientists.
- In several important application areas such as autonomous cars, criminal justice, health care, recruitment, housing, management of the human resource, public safety, where decisions made by models, or AI agents, have a direct effect on human lives. As a result, there is growing concerned about whether these decisions can be trusted, to be right, reasonable, ethical, personalized, accurate, robust, and secure, particularly in the context of adversarial attacks [ 104 ]. If we can explain the result in a meaningful way, then the model can be better trusted by the end-user. For machine-learned models, new trust properties yield new trade-offs, such as privacy versus accuracy; robustness versus efficiency; fairness versus robustness. Therefore, incorporating trustworthy AI particularly, data-driven or machine learning modeling could be another challenging issue in the area.
In the above, we have summarized and discussed several challenges and the potential research opportunities and directions, within the scope of our study in the area of data science and advanced analytics. The data scientists in academia/industry and the researchers in the relevant area have the opportunity to contribute to each issue identified above and build effective data-driven models or systems, to make smart decisions in the corresponding business domains.
In this paper, we have presented a comprehensive view on data science including various types of advanced analytical methods that can be applied to enhance the intelligence and the capabilities of an application. We have also visualized the current popularity of data science and machine learning-based advanced analytical modeling and also differentiate these from the relevant terms used in the area, to make the position of this paper. A thorough study on the data science modeling with its various processing modules that are needed to extract the actionable insights from the data for a particular business problem and the eventual data product. Thus, according to our goal, we have briefly discussed how different data modules can play a significant role in a data-driven business solution through the data science process. For this, we have also summarized various types of advanced analytical methods and outcomes as well as machine learning modeling that are needed to solve the associated business problems. Thus, this study’s key contribution has been identified as the explanation of different advanced analytical methods and their applicability in various real-world data-driven applications areas including business, healthcare, cybersecurity, urban and rural data science, and so on by taking into account data-driven smart computing and decision making.
Finally, within the scope of our study, we have outlined and discussed the challenges we faced, as well as possible research opportunities and future directions. As a result, the challenges identified provide promising research opportunities in the field that can be explored with effective solutions to improve the data-driven model and systems. Overall, we conclude that our study of advanced analytical solutions based on data science and machine learning methods, leads in a positive direction and can be used as a reference guide for future research and applications in the field of data science and its real-world applications by both academia and industry professionals.
Declarations
The author declares no conflict of interest.
This article is part of the topical collection “Advances in Computational Approaches for Artificial Intelligence, Image Processing, IoT and Cloud Applications” guest edited by Bhanu Prakash K N and M. Shivakumar.
Publisher's Note
Springer Nature remains neutral with regard to jurisdictional claims in published maps and institutional affiliations.
Data Scientist Career Path: How To Become a Data Scientist
Join over 2 million students who advanced their careers with 365 Data Science. Learn from instructors who have worked at Meta, Spotify, Google, IKEA, Netflix, and Coca-Cola and master Python, SQL, Excel, machine learning, data analysis, AI fundamentals, and more.
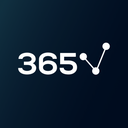
Author’s Note: This article was originally published in 2017 and offers valuable insights and perspectives based on the available knowledge at the time of its publication. You can find the most up-to-date information in our new research, The Data Scientist Job Outlook in 2024 .
The data scientist career path is probably the hottest career choice you can currently make. It is not only the $108,000 median base salary that makes the position appealing to job seekers, data science also hits high on satisfaction with a score of 4.2 out of 5, as findings from the latest Glassdoor report reveal.
Big data is the new oil
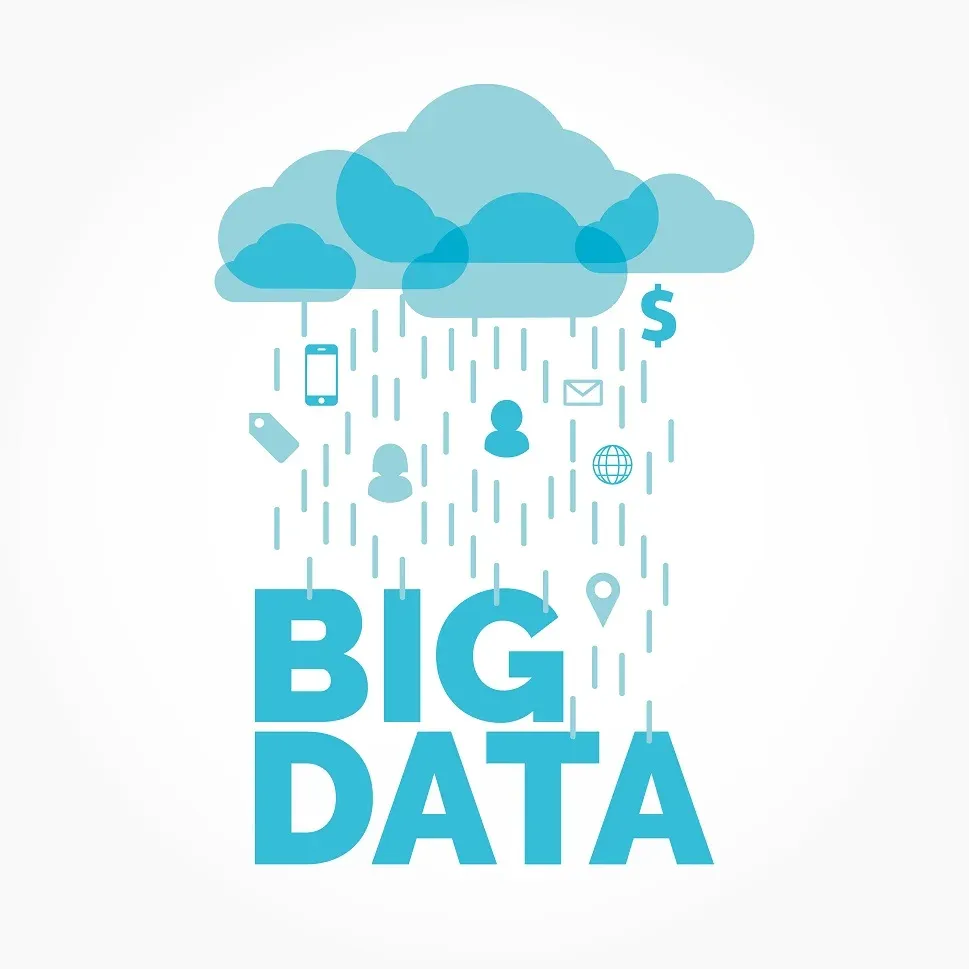
Data scientist career path: Dealing with massive volumes of data.
You are now probably asking yourself: “Okay, cool. But how do I get a job in data science?” . And you are certainly not the only one. There is so much hype about big data . Organizations and individuals are “always on”, leaving digital traces for everything, all the time, everywhere. And someone needs to handle that information. In simple terms, data scientists set up the systems required to generate insights that can be gained from humongous volumes of data. Therefore, it does not come as a surprise that this is the black gold which fuels productivity, better decision-making, and profit gains. The data scientist career path is on the rise.
What Does a Data Scientist Do?
Data scientist is the sexiest job of the 21 st century Sounds overstated? Think again. A few years ago, The Harvard Business Review (HBR) hailed ‘data scientist’ as the sexiest job position .People with the skills and curiosity to find meaning from swimming in data are an object of desire for many industries including finance, retail, and e-commerce.
The HBR’s juicy title is still spot-on. IBM predicts an explosion in the demand for data scientists, with more than 300,000 job openings on the US labor market by 2020. As HBR gurus put it, “if ‘sexy’ means having rare qualities that are in high demand, data scientists are already there.”
Sounds amazing! But what does a data scientist do?
"That word. 'Sexy.' What does it mean?
It means loving someone you don't know."
- Jhumpha Lahiri
As the novelist Jhumpha Lahiri puts it, there is a great deal of mystery in that word: “sexy”. Do we really know who data scientists are, where they come from, and what they do? ‘Data scientist’ is a loose term, and it is therefore not surprising that you are struggling to find the right career track . A data scientist job implies some statistics and modeling knowledge, combined with programming skills, that ultimately result in actionable insights for businesses.
Author's note: If you are interested in learning more about the data science field and the career opportunities it offers, you can do that by downloading our free data science career guide )
To reach those insights, data scientists need a unique blend of rare qualifications. But don’t be discouraged. We will show you how to choose the right data scientist career path , which will enable you to remain focused, to leverage your strengths, and to develop only the specific skills needed to match the roles you want .
Becoming a Data Scientists: A Survival Guide
We offer you a data science survival guide. In this article, we will navigate your way through the pool of data science job opportunities. We will show you the data science pipeline, or the sequence of events that gets a project up and running. A real-life case study on Heineken comes in handy when explaining the different job roles in a data science team. In the end, you will be equipped with actionable tips about what you can do right now to become a data scientist .
The Data Science Process
The Main Steps of the Data Science Process Any data science project is a process; understanding this fact is important if you want to find your way through the data science maze .
It is about going through a sequence of steps in a systematic manner.
First, come the project objectives. Have you identified a business issue or an attractive market opportunity? You need to be clear about what you are trying to accomplish in order to help your company gain a competitive edge.
Then , you need to figure out where to collect data from, to plan resources, and to coordinate people to get the job done.
Part three is data preparation . Data needs to be carefully cleaned and explored. Associations will start to emerge; the sample and the variables will be refined. Then, comes the creation of models, their validation, evaluation, and potential refinement.
Finally , you need to communicate the team’s experiences during the data science process. Data needs to take a compelling form and structure. At the final reporting stage, visualizations are necessary to tell the full story .
Job Roles in Data Science
So, what did you learn?
Roles in a data science team aren’t exclusively technical. While programming and statistics are needed for the core stages of the process, contextual skills are essential for the planning and reporting stages.
Indeed, the data scientist role is a crossover between many different disciplines. Data scientists are multi-talented professionals, who can see the big picture, while also being programmers, statisticians, and good data storytellers.
However, in a data science team, there are people with diverse roles, and they all contribute in different ways. If the data scientist career path is the ultimate goal, there are various ways you can get there.
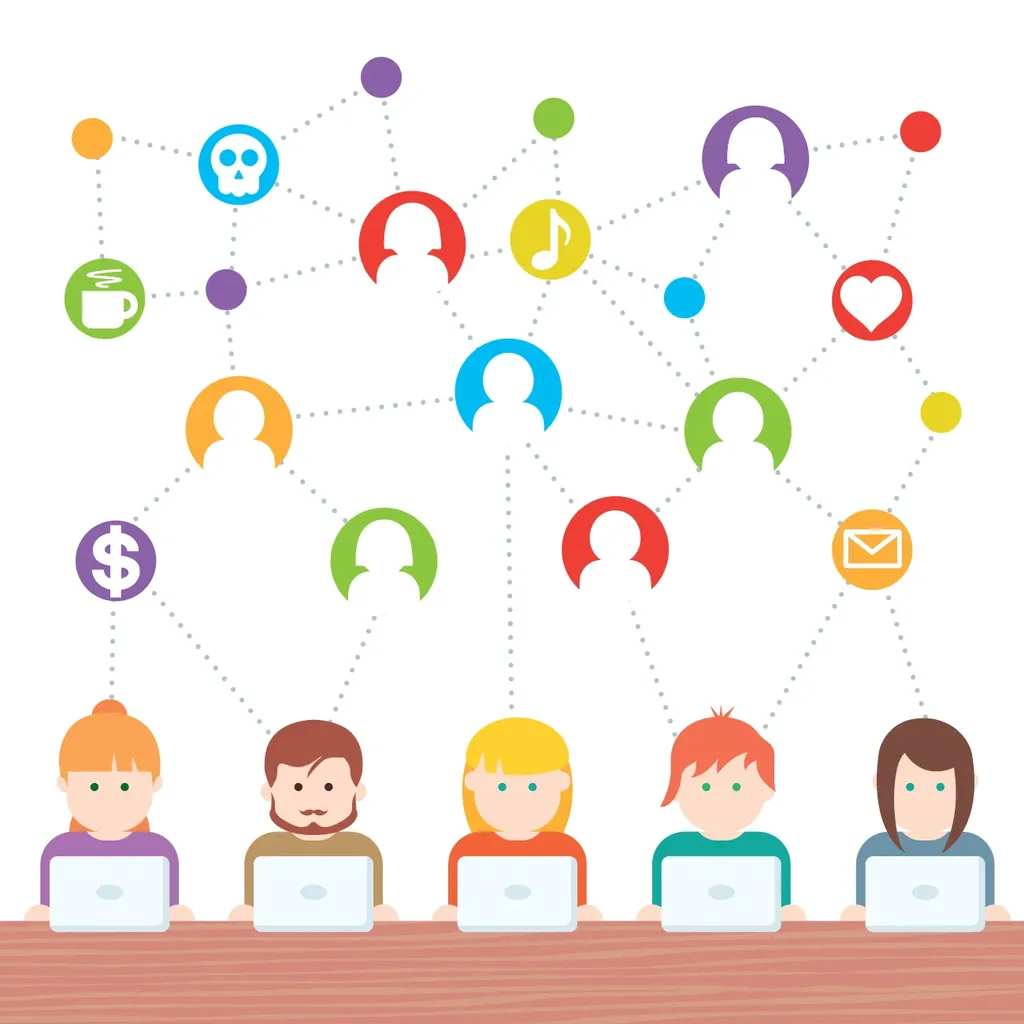
Taking the data scientist career path: Find out what role fits you best
Data analysts , for example, are more involved in day-to-day tasks, focused on gathering data, structuring databases, creating and running models, trend analysis, making recommendations, and storytelling.
Business intelligence (BI) analysts , on the other hand, should be able to see the big picture, situate the business unit in the market, having considered its trends. Normally, BI analysts have expertise in business, management, economics or a similar field. However, they must also “speak data”. BI analysts work with tons of information, and spend most of their time analyzing and visualizing the data they have gathered from multiple sources.
Are you fascinated by marketing problems? The marketing analyst is a special kind of data analyst. However, they are not that involved in programming and machine learning , as their core strength lies in analyzing consumer behavior data through specialized software.
Real Life Data Science Use Case: Heineken
Let’s look at an example to show the synergy in a real-life data science team.
The beer giant Heineken spotted an opportunity.
Traditionally, beer is not very well positioned in restaurants, because it is not the typical drink that goes well with food. Wine takes the lead there as it pairs with basically everything, from steak to pasta. Therefore, selling more beer by creating the perfect beer-food combinations is a genius solution!
Who do you think looked at wine data and spotted industry trends to suggest growth directions for Heineken ? Sounds like the job for a BI analyst !
After the attractive market opportunity was identified, Heineken were ready for action.
Data science in action
To find beer-food combos, Heineken leveraged data science. Teamwork is essential for a project as complex as this one. Data scientists were indeed on top of the process, sifting through huge quantities of data to find insights for Heineken.
And it was data scientists who suggested the smart solution to run machine learning checks on beer and food molecules. Creating a beer with the right molecules to perfectly fit the ingredients of a market’s most popular meal would be both mouth-watering and money-making. Imagine the perfect match between beer molecules and best-sellers such as a burger or chicken tikka masala!
Then, empirical data from beer lovers was collected. At this stage, marketing analysts were needed to create the research design and gather consumer data that could be later used for marketing planning.
919 citizens of Paris and New York sampled various beer-food pairs. As an interesting aside, for some participants, the gourmet experience took place in nice bistros, whereas others were offered virtual reality gear to mimic the context.
Data analysts offered a helping hand in the cleaning and preprocessing of data collected during the bistro sessions. Statistical analyses were performed, and findings were visualized.
Care to find out what the Heineken’s BFFs are?
Both machine learning checks and French respondents reported that beer with pate is a killer combination! Findings also confirmed the suitability of the beer/cheesecake combo, so consider trying that as well. Not as visual and compelling as a data scientist’s presentation, but still interesting enough.
What conclusions can we draw?
We used a real-life project to demonstrate that data science research is dynamic and multi-dimensional. This is why it requires the expertise of many, many people. They will have different job titles, knowledge, skills, and experience, which will ultimately result in truly valuable insights from a business perspective.
What Is the Right Data Scientist Career Path for You?
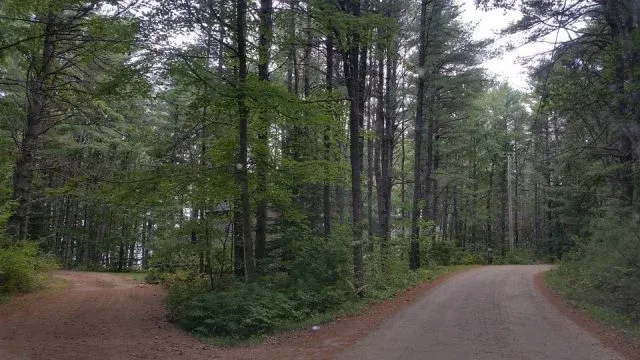
Are you now allured by any of these roles? If yes, good for you, as each of these career paths will help you to gain the valuable skills needed. Work hard and eventually, you will land a data scientist job. (We don't like repeating ourselves, but make sure you go through our career guide before making any career decisions and embarking on a career path)
In this section, we will take a closer look at the key roles in a data science team.
Data Analyst Career Path
There is a joke circulating on Twitter saying that “A data scientist is a data analyst who lives in California”. While there is certainly an overlap, there are crucial differences between the two roles. Data scientists essentially see the bigger picture. Their multifaceted skills see them through the whole data science process. On the other hand, the efforts of data analysts are far more concentrated, they have a specific goal in mind, normally assigned to them by the data scientists. Therefore, data analysts need a narrower skill set to perform their daily work.
Most notably though, it takes a long time to reach the top of the ladder, where data scientists are normally positioned. Working your way up isn’t easy, or quick, but becoming a data analyst is a great starting point.
A data analyst is in charge of scrutinizing information using analytical tools and programming languages. Ultimately, they should produce meaningful results extracted from raw data, but it is the management that makes the decisions about what to do with those results.
The key responsibilities of a data analyst are data cleaning and maintenance, programming and analysis, and presentation of findings. Therefore, knowledge of business and strategy is not in the forefront. The job is actually quite technical, but that’s where its strength lies. To develop the data scientist intuition, you must be very well prepared on the technical side.
Care to get more insights from a real-life data analyst? Check out our interview with Ina .
Skills needed
The top skills necessary for this position are Microsoft Excel , market research, advanced statistics, and SQL . In addition, you’ll also need visualization tools like Tableau , and at least one programming language, such as R and/or Python.
That said, if you want to learn everything there is to know about Python, check out our super comprehensive Python programming guide .
The business intelligence (BI) analyst path
In simple terms, business intelligence digests corporate data to provide actionable information. There are two main skill sets needed for a BI job: business, and data. A BI analyst understands the functions of a business well. However, they can also perform technical tasks, including data mining, modeling data, and data analysis.
A BI analyst also looks at competitors’ data and business trends to figure out how their own company can improve its competitive positioning. It is through their multilayered preparation that they manage to find and understand patterns and trends in large chunks of data.
You should be extremely familiar with Microsoft Excel, market research, basic statistics, and Tableau. Valuable additions are SQL and a programming language like R or Python.
Marketing Analyst Career Path
Marketing analysts spend most of their time trying to enhance the marketing mix while making comparisons between past and current market data.
If you pursue the marketing analyst track, you are required to have in-depth knowledge of fundamental marketing concepts, such as strategy and planning, marketing research, and campaign management.
But that’s not the whole story. In today’s world, tech skills are also essential in managing digital campaigns, including mastery of Google products, such as AdWords and Analytics.
And last but not least, the basics of statistics are a must for any analyst. A marketing analyst must be able to design and monitor metrics, then visualize them, and prepare reports.
The responsibilities of a marketing analyst may vary, but 95% of the time, you will be using one of these five areas of expertise: Microsoft Excel , market research, basic statistics, or digital marketing. While not essential, some knowledge of Tableau will considerably increase your chances of landing the job.
5 Steps to Embark on Your Data Science Career Path
Are you now drawn by the tremendous opportunities that each data scientist career path offers? It is not a straightforward task to figure out what you should focus on in order to land a data scientist position. Nevertheless, learning more about the various roles in data science is the very first step to career success.
If you are looking for smart and affordable ways to boost your employability in data science, here is a list of five actionable steps:
1) Check out the 365 Data Science Career Guide (third invitiation is the last one)
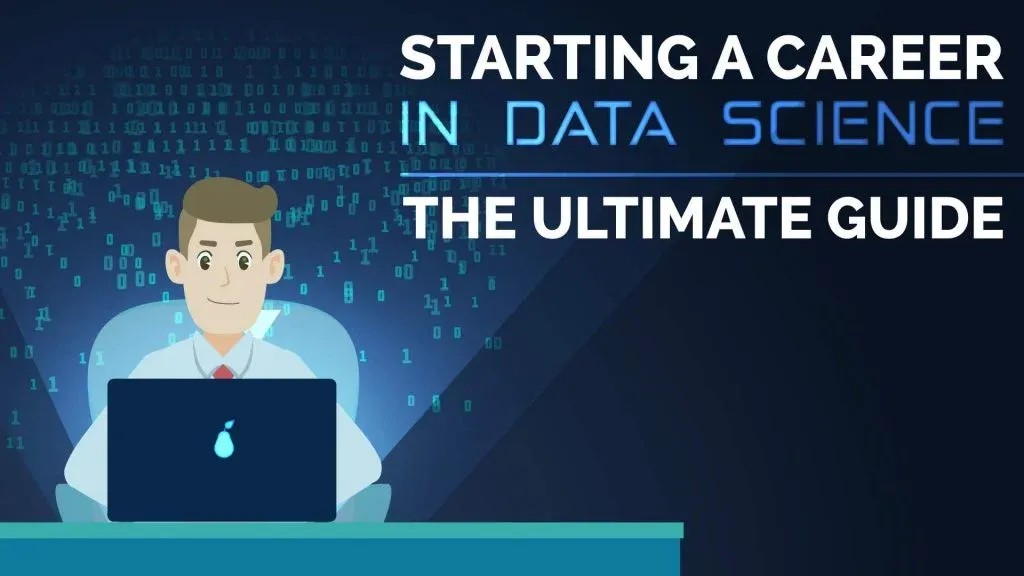
It offers a comprehensive overview of the most popular paths you can take to end up in a data science position. Just subscribe in the form here and the career guide pdf will be sent to you FREE .
2) Join data science groups and follow influencers
Joining groups on LinkedIn is a cheap and effective way to stay up-to-date and maintain relationships with data science fellows. Moreover, many data scientists have taken to Twitter to share know-how, so make sure you are following the influencers.
3) Build up a public portfolio of data science projects
Even if you are new to tech, you can start building a portfolio of simple, yet interesting works. It’s an opportunity to create a personal brand with great potential for future growth in the data scientist career path. A great platform on which to showcase your work is Github ; you can also create a website.
4) Take online courses
Did you know that your resume will be discarded if you don’t include at least 75% of the desired qualifications? Gee, thanks, electronic screening systems. If you want to make sure that your resume will beat the bot, you need to boost your skill set. Perhaps the most effective and least costly way is to enroll in courses that develop hot skills like Python, SQL, R, Tableau, and machine learning. Get started with our tutorials to give you a hint at our teaching style.
5) Define your target employers
Once you are clear about your desired data scientist career path, figure out who you are targeting.
Data science is not ‘one size fits all’
Data science is not just for big companies. Every business generates data, be it a multinational giant or the local street corner market. While not every company can afford a full-stack data science team, small firms also need capable analysts. Ask yourself this: ‘Do I see myself in a small firm or am I more attracted to big players?’ Then create a list of target organizations , and start following them on social media.
If you liked this article, then you will definitely love our article in which we compare terms like Data Science, Machine Learning, Data Analytics, and Business Analytics . Make sure you check it out!
Ready to take the first step towards a career in data science?
Check out the complete Data Science Program today. We also offer a free preview version of the Data Science Program. You’ll receive 12 hours of beginner to advanced content for free. It’s a great way to see if the program is right for you.
Try Intro to Data and Data Science course for free!
World-Class
Data Science
Learn with instructors from:
The 365 Team
The 365 Data Science team creates expert publications and learning resources on a wide range of topics, helping aspiring professionals improve their domain knowledge, acquire new skills, and make the first successful steps in their data science and analytics careers.
We Think you'll also like
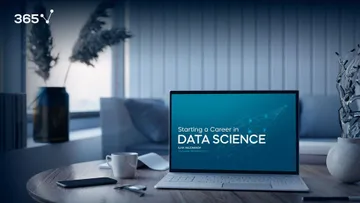
Career Guides
Starting a Career in Data Science: The Ultimate Guide (2024)
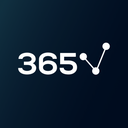
Can I Become a Data Scientist: Research into 1,001 Data Scientists
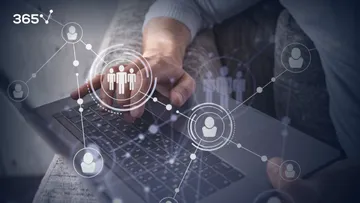
Career Advice
How to Become a Data Scientist in Telecom
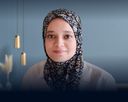
How to Become a Data Scientist in India: Salary, Job Outlook & Skills
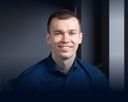
- Columbia Engineering Boot Camps
- Data Analytics
The Data Scientist Career Path: Everything You Need to Know
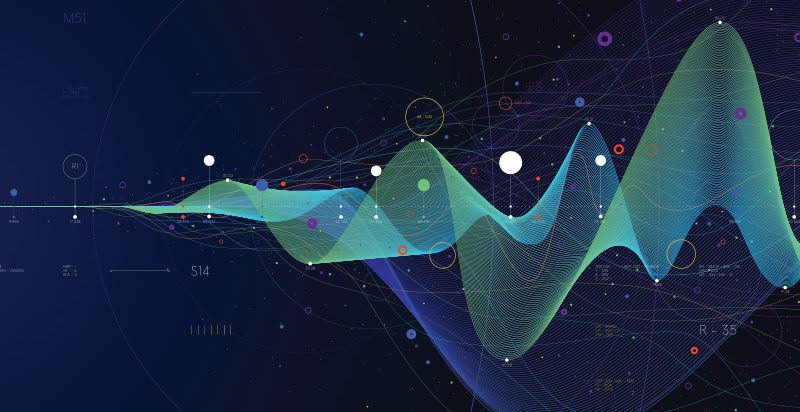
Nearly every type of organization — from government, to retail, to healthcare — needs data scientists. Data scientists organize and analyze raw data from various sources, enabling these enterprises to make informed decisions to ensure efficiency, boost profitability, and fuel growth.
Demand for data science professionals is expected to increase significantly in the next decade. The U.S. Bureau of Labor Statistics (BLS) estimates 22 percent growth through 2030 , which far exceeds the 7.7 percent projected increase for all occupations. That translates to a need for an average of 3,200 data scientists each year through 2030.
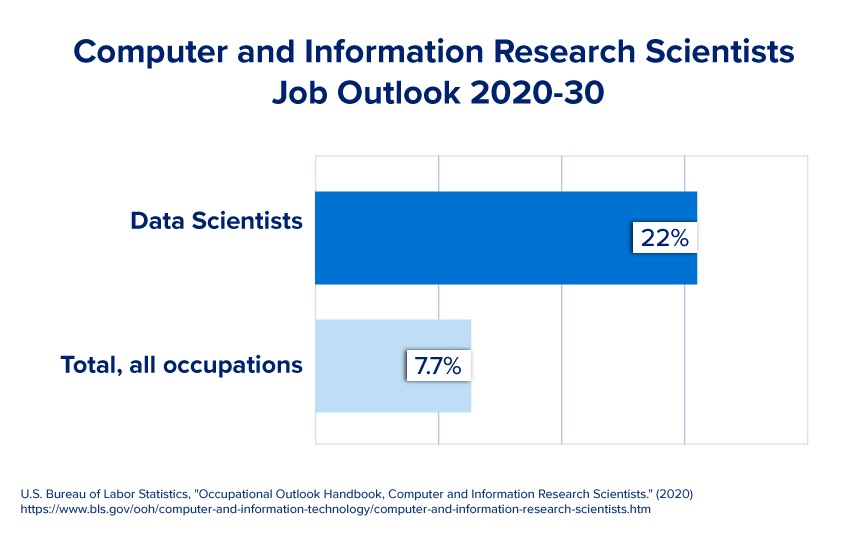
These positions (which according to the BLS earn a mean annual salary of $103,930 ) tend to be clustered in Maryland and Virginia, as data scientists are in high demand with the federal government. Data scientists are also in high demand in New York, California, Texas, and Washington state.
The demand for data scientists coincides with a marked increase in the sheer amount of available data. According to Statista, just two zettabytes of data (i.e., two trillion gigabytes) were created, copied, captured, or consumed in 2010 , a number that is expected to increase to 79 zettabytes by the end of 2021, and then mushroom to 181 zettabytes by 2025.
These data increases are prompting organizations to seek highly skilled data professionals as they pivot toward data-driven decision making. For example, 92 percent of the executives responding to the 2019 MIT Sloan Management Review said they had begun investing more heavily in data and AI . That same year, Entrepreneur reported that companies that leveraged big data were 8 percent more profitable than those that did not.
Clearly, data scientists have a vital role — one that will only continue to increase in importance and value over time.
Data Scientist Career Path: How to Get Into Data Science
CareerOneStop indicates that 37 percent of data scientists have obtained their bachelor’s degree , usually in a field such as statistics, computer science, information technologies, mathematics, or data science. In addition, 35 percent of data scientists hold a master’s degree, and 14 percent have attained a doctoral degree.
Yet, some believe that a degree is not as crucial to career success as gaining early proficiency in programming languages such as Python, Java, and R, which can provide significant benefit in the long run. Carlos Melendez, COO and Co-Founder of the artificial intelligence and software development company Wovenware, stressed in an October 2021 Forbes piece that education should begin as early as elementary school :
“Every student, regardless of their occupation, will need to be data-literate to succeed in a world where data will increasingly be king.”
A data analytics boot camp will teach you the skills to pursue an entry-level data science role and to enter this exciting career. Such boot camps are short-term, intensive courses lasting three to six months and offer flexible scheduling, online coursework, and practical training.
To learn all these skills and more, check out Columbia Engineering Data Analytics Boot Camp , as it can serve as your gateway to an exciting, fulfilling career.
Data Science Requirements
Again, a solid foundation is essential, and Columbia Engineering Data Analytics Boot Camp can help you learn the skills needed to become a data analyst . These skills include learning Python, Java, R, MATLAB, and NoSQL. Additional in-demand skills include
Data Cleaning
Machine learning.
Data visualization uses maps or graphs to give data visual context. LinkedIn Senior Content Marketing Manager Paul Petrone has likened it to “telling stories with insights gleaned from the data.”
Data cleaning is the process of removing data that is incorrect, redundant, corrupted, incomplete, or incorrectly formatted.
Machine learning uses algorithms to discern patterns in data sets and powers search engines, social media platforms, voice assistants, and the recommendation systems used by content providers.
Linear algebra/calculus are advanced math skills that are crucial for those in data science. Linear algebra has been called “the mathematics of data,” in that it has applications to machine and deep learning, and calculus is no less crucial in building algorithms.
Microsoft Excel, while not as sophisticated a skill as others listed here, remains important given its widespread popularity and usage within the field of data.
Soft skills like critical thinking and communication are also taught in data bootcamps. Melendez notes the importance of such skills in his most recent Forbes piece, as well as an earlier article published in July 2021. He lists empathy, teamwork, open-mindedness, and a business mindset as important soft skills, indicating that problem-solving has also become a vital skill as the pandemic has worn on and “the neat and orderly world of data scientists was turned upside down.”
Melendez’s point is that the data informing predictive algorithms may no longer be reliable at present. He offered an example illustrating the recent spike in visits to doctors’ offices, as COVID-19 began to wane in certain areas and patients could move about more freely. While such an uptick would normally suggest that customers are poised to change carriers, it is more realistically due to the fact so many people put off doctors’ appointments due to lockdowns or fear of exposure to the virus. As you can see, understanding the various causes behind consumer behaviors is crucial to being able to glean relevant insights from collected data.
In other words, a data scientist must consider data context and additional variables while also applying analytic best practices and common sense.
Data Scientist Career Path — Data Science Careers
While there are many different roles in the data science field (including software engineers, business analysts, etc.), the focus here will be on the data science career path.
Junior Data Scientists
Mid-level data scientist, senior data scientists, data science managers.
As you learn how to become a data analyst , sometimes referred to as a junior data scientist, you will need a strong skill foundation to be successful. Applicable skills may include a proper math background, aptitude in data visualization and data cleaning, and familiarity with different programming languages.
Junior data scientists work on the more basic aspects of data analysis, including extracting, cleaning, integrating, and loading data. Focused mainly on predictive analysis, they often use pre-existing statistical models or work with specifications laid out by a more senior data scientist.
Those entering the data science field usually remain junior data scientists for a year or two before becoming mid-level data scientists. Mid-level data scientists enjoy greater autonomy with less frequent check-ins, and are expected to know how to perform exploratory data analysis and build the necessary statistical models for problem-solving.
In addition, mid-level data scientists may have the opportunity to work with senior data scientists in more advanced areas of machine learning and AI.
Individuals three to seven years into their data careers may qualify for a promotion to senior data scientists. While mid-level data scientists construct the statistical models that will solve problems, senior data scientists put that model to use in conjunction with other advanced tools. Moreover, senior data scientists are responsible for monitoring and fine-tuning an organization’s methodologies, while collaborating with key stakeholders and communicating the organization’s data insights to customers and company leaders. Senior data scientists are also responsible for mentoring junior data scientists.
Data science managers are responsible for the big picture — hiring the right people, establishing high standards, setting worthwhile goals, and understanding which KPIs are appropriate for the team.
As with managers in other sectors, the idea is to create a productive work environment while maintaining flexibility as products and industries continually evolve. A data science manager should be cognizant of new developments and prepare their team accordingly, as that will ensure their organization remains competitive.
Data science managers typically have at least five years of previous experience as data scientists , and many disciplines require one to three years of prior supervisory experience as well.
Data Scientist Career Path — The Future of Data Science
Data science job growth is occurring across a variety of industries every year. In fact, CareerOneStop is bullish on the future of data science, predicting a 3 1 percent increase in data science roles annually through the next decade . And, according to the U.S. Bureau of Labor Statistics, the top three states employing the most data scientists are California, Texas, and New York (respectively) with New York City being the top metropolitan area for data scientist employment in the U.S. While demand for data scientists is extremely high in these areas, these professionals are in high demand across the country and the globe.
Through the explosive growth in the Internet of Things (IoT) — i.e., wearable tech, smart home devices, baby monitors, etc. — more granular data will be generated to inform decision-making and provide additional insights. Moreover, with the ongoing rollout of 5G and its impact on data flow, as well as the potential of 6G bringing the advent of the “ Internet of Everything ,” the need for data scientists will only continue to increase. Consider the potential impact in the following sectors:
Transportation: Data is critical to the development of autonomous vehicles (AVs) because transportation-related information may soon be processed by vehicles rather than humans. Because AVs are such advanced forms of artificial intelligence (AI), they will require an exponential amount of data to function. If the technology reaches its full potential, one of the biggest benefits will be safer roads.
Data scientist Stefano Cosentino was hired by the German engineering firm Bosch in 2017 as part of the team developing autonomous vehicles. While he was uncertain of his role at first, over the next two years it evolved to the point where he was leading a 10-person team that contributed to the development of such vehicles by providing on-demand data analyses. In addition, Cosentino wrote on the website Towards Data Science:
“We have developed rule-based and probabilistic root cause analysis solutions to support the forensic team. We have created a feature bank that is enabling various ML projects. One is scenario identification, which we use for KPI estimation, verification and validation, as well as issue tracking. Another use of the feature bank is for anomaly detection.”
Healthcare: Some 30 percent of the world’s data is created by the healthcare field, and by 2025 it is expected to increase to 36 percent. Too often, however, this information is siloed, making it inaccessible to all who need it during a patient’s care journey. This issue — interoperability, or the ability of systems or organizations to share data — is an ongoing challenge, and one data scientists can help solve. This can be done by culling data from various sources (electronic health records, genomics, imaging, etc.) and analyzing it, thereby providing clinicians with insights that will enable them to personalize care.
Finance: So much of the finance field involves interpreting real-time data and forecasting future trends or market events. Technologies like artificial intelligence (AI) and machine learning (ML) are becoming increasingly essential to those processes, and data scientists use those tools to analyze and manage risk, leading to better decision-making and greater profitability.
Supply chain management: The global supply chain was already undergoing a digital transformation before the pandemic hit, but the outbreak of COVID-19 accelerated that trend; making the need for advanced technologies like AI, blockchain, and robotics more pronounced.
Data scientists in this sector use predictive analytics to make the supply chain more agile and efficient. This includes anticipating demand, determining where inventory should be positioned proactively to avoid out-of-stock events, determining the optimal network of manufacturers and storage facilities, and developing optimized routes for transporting inventory.
Columbia Engineering Data Analytics Boot Camp , based in New York City, offers learners the opportunity to gain in-demand data science skills via practical, real-world scenarios and professional instruction with flexible scheduling.
Data Scientist Career Path — Data Science Salary
Another appealing aspect of a data science career is the compensation. The mean annual salary for a data scientist in the U.S. is $103,930 , according to the Bureau of Labor Statistics.
And, according to the BLS, the states with the highest mean annual salary were California ($129,060), New York ($124,240), and Washington ($118,320). The business sectors reporting the highest annual salary for data scientists include computer/peripheral equipment ($144,090), finance ($143,490), and merchant wholesalers ($142,300). As you can see, data science isn’t just an exciting and in-demand field, it’s also a lucrative career path!
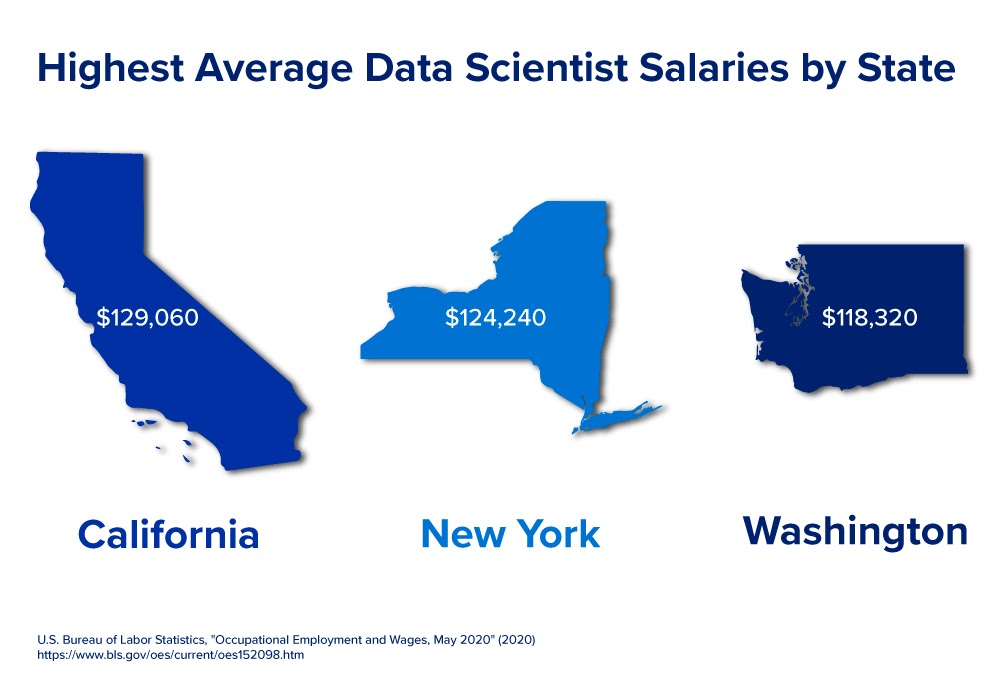
New York City’s Columbia Engineering Data Analytics Boot Camp can help you to prepare to become a data scientist and jumpstart your transition into this exciting field.
Begin Your Data Science Career Path
A career as a data scientist can offer considerable opportunities and rewards. The need for these professionals is only growing on a national and global scale, with unprecedented growth in both the quantity and granularity of data, as well as the growing usage of that data to drive decision making and fuel AI and ML.
Columbia Engineering Data Analytics Boot Camp is the place to prepare to join this exciting field. Become one of the data science professionals on the leading edge of data discovery and change your future today.
Get Boot Camp Info
Step 1 of 6
Essay Questions
Four short essays are required in your application. These short essays are an opportunity to articulate your candidacy for the Master of Science in Data Science program at the University of Washington. The best essays are clear, succinct, thoughtful, well-written, and engaging. Your essays play an important role in our holistic admissions process, and we expect that they are your own original work.
Essay 1: Why UW MSDS? (350 words maximum)
Please review the information about the MS in Data Science program provided on our website. In 350 words or fewer please describe what this degree will enable you to do that you would be unable to do otherwise. You may consider any of our program elements but please be specific. This should not be a general statement of purpose, but rather a specific discussion of this program and how it might change the trajectory of your life.
Essay 2: Data Visualization (500 words maximum)
The UW MS in Data Science program places a premium on strong communication skills. In addition to excellent quantitative abilities, good Data Scientists must be able to communicate their findings in a way that makes them meaningful to a variety of different audiences.
Select an example from the list of data communications below. For the example you have selected, please write an essay addressing the following three questions:
Who is the intended audience of your example? How does the communication method enhance or suppress accessibility in this example? How does the communication method address bias in the data?
Communication Examples:
https://www.smithsonianmag.com/history/first-time-together-and-color-book-displays-web-du-bois-visionary-infographics-180970826/
https://coronavirus.jhu.edu/map.html
https://ourworldindata.org/water-use-stress
https://www.datacamp.com/blog/florence-nightingale-pioneer-of-data-visualization
https://www.reuters.com/graphics/CLIMATE-CHANGE/WILDFIRE-EMISSIONS/zjvqkrwmnvx/index.html
Essay 3: Communication and Collaboration (350 words maximum)
Professional Data Scientists need strong technical skills, in addition to strong collaborative and communication skills to effectively work with others. Please describe a specific time you had a miscommunication with a peer. What steps did you take to build a collaborative relationship? What did you learn from this experience?
Essay 4: Diversity and Equity (500 words maximum)
The MS in Data Science program is committed to developing a community of Data Science leaders who will promote and advance diversity, equity, and inclusion in the profession. In what ways do you contribute to creating an inclusive environment? Please be specific.
Admissions Timelines
Applications for Autumn 2024 admissions are now closed.
Decisions Release Date: Mid-March 2024 (no early decisions granted)
Admissions Updates
Be boundless, connect with us:.
© 2024 University of Washington | Seattle, WA
Data Scientist Essays
Data scientist and cloud computing, popular essay topics.
- American Dream
- Artificial Intelligence
- Black Lives Matter
- Bullying Essay
- Career Goals Essay
- Causes of the Civil War
- Child Abusing
- Civil Rights Movement
- Community Service
- Cultural Identity
- Cyber Bullying
- Death Penalty
- Depression Essay
- Domestic Violence
- Freedom of Speech
- Global Warming
- Gun Control
- Human Trafficking
- I Believe Essay
- Immigration
- Importance of Education
- Israel and Palestine Conflict
- Leadership Essay
- Legalizing Marijuanas
- Mental Health
- National Honor Society
- Police Brutality
- Pollution Essay
- Racism Essay
- Romeo and Juliet
- Same Sex Marriages
- Social Media
- The Great Gatsby
- The Yellow Wallpaper
- Time Management
- To Kill a Mockingbird
- Violent Video Games
- What Makes You Unique
- Why I Want to Be a Nurse
- Send us an e-mail
Everything you need to know about Data Science

Checked : Soha K. , Eddy L.
Latest Update 18 Jan, 2024
Table of content
What is Data Science?
Are data science and business analytics the same, why use data science, the data science process, 1) predictive causal analysis, 2) prescription analysis, 3) machine learning to make predictions, the main phases of the data science process, 1) knowledge and analysis of the problem, 2) data preparation, 3) model planning, 4) the realization of the model, 5) communicating the results, conclusions.
We commonly talk about Data Science, because today data is a competitive advantage for companies, but what exactly does it mean? We will try to deepen this theme in this essential guide.
Data Science is the study that concerns the retrieval and analysis of data sets, intending to identify information and correspondences hidden in the unprocessed data, defined as raw. Data Science, in other words, is the science that combines programming skills and mathematical and statistical knowledge to extract meaningful information from data.
Data Science consists of the application of machine learning algorithms to numerical, textual data, images, video, and audio content. The algorithms, therefore, perform specific tasks that concern the extraction, cleaning, and processing of data, generating in turn, data that are transformed into real value for each organization.
Often the terms Data Science and Business Analytics are considered synonymous. After all, both the Business Analytics and Data Science activities deal with the data, their acquisition, and the development of models and information processing.
What then is the difference between Data Science and Business Analytics? As the name suggests, Business Analytics is focused on the processing of data, business or sectorial, to extract information useful to the company, focused on its market and on that of its competitors.
Data Science instead responds to questions about the influence of customer behavior on the company's business results. Data Science combines the potential of data with the creation of algorithms and the use of technology to answer a series of questions. Recently the functions of machine learning and artificial intelligence have evolved and will bring data science to levels that are still difficult to imagine. Business Analytics, on the other hand, continues to be a form of business data analysis with statistical concepts to obtain solutions and in-depth analysis by relating past data to those relating to the present.
The Data Science aims to identify the most significant datasets to answer the questions asked by the companies, elaborate them to extract new data related to behaviors, needs, and trends that are the basis of the data-driven decisions of their managers.
The data thus identified can help an organization contain costs, increase efficiency, recognize new market opportunities and increase competitive advantage.
Can the data produce other useful data? Of course yes! Data Science was created to understand the data and their relationships, analyze them, but above all to extract value and ensure that, properly interrogated and correlated, they generate information that is useful not only to understand the phenomena but above all to orient them.
Data Science is indispensable for companies dealing with digital transformation because it allows them to direct their products or services towards the customer, their purchasing behavior and respond to their needs. Leading companies in the global market, such as Netflix, Amazon, and Spotify use applications developed by Data Scientists. Thanks to artificial intelligence , allow creating recommendation engines that suggest what to buy, what to listen to and which films to see based on the tastes of the individual user. These algorithms are also able to evaluate what were the suggestions that did not affect the user's interest thanks to the machine learning process, which allows refining the proposals more and more and thus increase conversions and optimizing the ROI.
Data Science is mainly used to provide forecasts and trends. It also used to make decisions using tools for predictive analysis, prescriptive analysis, and machine learning.
If the data analysis has the purpose of obtaining a prediction that a certain event will occur in the future, it is necessary to apply the predictive causal analysis. Suppose that a bank that provides loans wants to predict the likelihood that customers will repay the loan in the future. In this case, Data Science uses a model that can perform predictive analysis on the customer's payment history to predict whether future payments will be properly received.
On the other hand, if you want to create a model or pattern that applies AI to make decisions autonomously and can constantly update with dynamic self-learning functions, it is certainly necessary to create a prescriptive analysis model. This relatively recent area of Data Science consists of providing advice or directly assuming consequent behavior.
In other words, this model is not only able to predict but suggests or applies a series of prescribed actions. The best example of this is the self-driving car: the data collected by the vehicles are used to optimize the software that drives the car without human intervention. The model will be able to make decisions independently, establishing when to turn, which path to take, when to slow down or break decisively.
If you have, for example, transactional data from a credit card company and you need to build a model to determine the future trend, you need to use machine learning algorithms through supervised learning. It is called supervised because the data based on which the algorithm can be trained is already available. An example could be the continuous optimization of the voice recognition of Alexa or Google voice assistants.
The concrete application of Data Science involves a series of sequential phases, now codified in a sort of process.
Before starting an analysis project, it is essential to understand the objectives, the context of reference, the priorities and the budget available. In this phase the Data Scientist must identify the needs of those who commission the analysis, the questions to which the project must respond, the data sets already available and those to be found to make the analysis work more effective. Finally, it is necessary to formulate the initial hypotheses, in a research framework open to the answers generated by relating the data, whose combinations can reserve surprises.
In this phase, the data coming from various sources, generally inhomogeneous, are extracted and cleaning is performed to transform them into elements that can be analyzed. In this phase, an analytical sandbox is needed in which it is possible to perform analyzes for the entire duration of the project. Often we use models in R language to clean, transform and display data . This will help identify outliers and establish a relationship between the variables. Once the data has been cleaned and prepared, it is now possible to perform the data analysis activity by entering them in a data warehouse.
We then proceed to determine the methods and techniques for identifying the relationships between the variables. These relationships will be the basis of the algorithms that will be implemented for that function. In this phase, we use R, which has a complete set of modeling features and provides a good environment for the construction of interpretative models. SQL analysis services that perform processing using data mining functions and basic predictive models are also useful. Although there are many tools on the market, R is the most used programming language for these activities.
After investigating the nature of the data available and designing the algorithms to be used, it is time to apply the model. This is tested with data sets specifically identified and made available for self-learning of the algorithm. We will evaluate if the existing tools will be sufficient for the execution of the models or we will need a more structured elaboration, then we move on to the optimization of the model and the elaboration is launched.

We Will Write an Essay for You Quickly
Here is the moment in which the Data Science activity is called to make the relationships identified between the data and the answers to the questions envisaged in the project understandable. In this phase, we reach the objective of the analysis. It is, therefore, necessary to elaborate one or more reports, destined to the managers of the various business functions, making the data emerged from the data science process easily understandable, adopting elements of graphic display, such as infographics and graphics. The text will be understandable even to those who do not have too much experience with data and will simplify their interpretation. It is also useful for those who are involved in product design, marketing management like top managers, who can make data-driven decisions based on data.
Data Science is revolutionizing in many sectors. It is just all about to know your client, analyzing his behavior by identifying relationships between data that can turn into predictive results regarding market trends and orientations. Today we are at an early stage, which already allows us to obtain results, but through the development of the IoT, sensors and other tools for data collection will be possible developments now only imaginable.
Looking for a Skilled Essay Writer?

- University of California, Los Angeles (UCLA) Bachelor of Arts
No reviews yet, be the first to write your comment
Write your review
Thanks for review.
It will be published after moderation
Latest News

What happens in the brain when learning?
10 min read
20 Jan, 2024

How Relativism Promotes Pluralism and Tolerance

Everything you need to know about short-term memory

ESSAY SAUCE
FOR STUDENTS : ALL THE INGREDIENTS OF A GOOD ESSAY
Essay: Data science and its applications
Essay details and download:.
- Subject area(s): Information technology essays
- Reading time: 8 minutes
- Price: Free download
- Published: 1 February 2016*
- File format: Text
- Words: 2,127 (approx)
- Number of pages: 9 (approx)
Text preview of this essay:
This page of the essay has 2,127 words. Download the full version above.
With vast amounts of data now available, organizations in almost every industry are focused on exploiting data for competitive advantage. On the other hand broad availability of data has led to increasing interest in new methods for extracting beneficial information and knowledge from data. The new discipline called data science has arose as a new paradigm to tackle these vast accumulation of data. Today it applies all most every fields in the world for different aspects. Mainly in security, health care, business, agriculture, transport, education, prediction, telecommunication, etc. Each area will also garner a different amount of return on their data science investment. This review aims to provide an overview of data science and mainly how some of these fields are currently using data science and how they could leverage it in their favor in the future. What is Data Science? According to Dhar, V. (2012), the fact that we now have vast amounts of data should not in and of itself justify the need for a new term âData scienceâ. It is a known fact that the extraction of information from data has been done by the use of statistics over decades. Nevertheless there are many reasons to consider Data science as a new field. First, the raw material, the âdataâ part of Data Science, is increasingly diverse and unstructured â” text, images, and video, frequently arising from networks with complex relationships among its entities. Further he reveals that the relative expected volumes of unstructured and structured data between 2008 and 2015, projecting a difference of almost 200 petabytes in 2015 compared to a difference of 50 petabytes in 2012. Secondly, the creation of markup languages, tags, etc. are mainly designed to let computers interpret data automatically, making them active agents in the manner of decision making (Dhar, V. (2012)). i.e. computers are increasingly doing the background work for each other. In the proceedings paper by Zhu, Y. & Xiong, Y. (2015), they have mentioned that the Data science research objects, goals, and techniques are essentially different from those of computer science, information science or knowledge science. Throughout the paper they always use a comparative method to discuss how data science differs from existing technologies and established sciences. According to them data science supports natural science and social science and dealing with data is one of the driving forces behind data science. Hence, they referred data science as a data-intensive science. It is evident that data science should be considered as a new science and new techniques and methods should be introduced in order to deal with its vast amount of data. Dhar, V. (2012) nicely explains how traditional data base methods are not suited for knowledge discovery. He explains that traditional data base methods are optimized for summarization of data given what the user wants to ask but not discovery of patterns in massive amount of data when the user does not have a well formulated query. i.e. Unlike database querying which asks âwhat data satisfy this patternâ discovery is about asking âwhat patterns satisfy the given data?â. Specifically ultimate goal of Data science is to finding interesting and robust patterns that satisfy the data. When the new technologies emerged it lead to research on the data themselves. Lot of fields such as health care, business got the advantage of that and they were able to discover growth patterns of data and predict the scale of data in cyberspace ten years into the future(Zhu, Y. & Xiong, Y. (2015)).This causes to discover lot of new theories, inventions, that havenât been uncovered for years. Many health related issues have been identified and solved using big data analytics. Applications of Data Science 1) Health care A key contemporary trend emerging in big data science is the quantified self (QS) -individuals engaged in the self-tracking of any kind of biological, physical, behavioral, or environmental information as n = 1 individuals or in groups(Swan, M. (2013)). In this article writer emphasis that Quantified self-projects are becoming an interesting data management and manipulation challenge for big data science in the areas of data collection, integration, and analysis. But at the same time he reveals, when as much larger QS data sets are being generated the quantified self, and health and biology more generally, are becoming full-fledged big data problems in many ways. Variety of self-tracking projects were conducted recently including food visualization, investigation of media consumption and reading habits, multilayer investigation in to diabetes and heart disease risk, idea tracking process, etc. These projects demonstrate the range of topics, depth of problem solving, and variety of methodologies of QS projects. Big health data streams are the main data stream in QS and most difficult task is to integrating big health data streams, especially blending genomic and environmental data. It was found that genetics has a one third of contribution of outcome to diseases like cancer and heart disease. Projects such as DIY genomics studies, 4P medicine personalized, Crohnâs disease tracking microbiomic sequencing, lactoferrin analysis project and Thyroid Hormone testing project are famous examples for applications of QS in genomics. These findings couldnât be found if data science doesnât exist or the newly tools which can handle large volume of data were not invented. Further Swan, M. (2013) suggests QS data streams need to be linked to healthy population longitudinal self-tracking more generally as they are the corresponding healthy cohorts to patient cohorts in clinical trials and he predicts eye tracking and emotion measurement could be coming in the future. X. Shi and S. Wang (2015) have written an article to provide an overview of theoretical background of applying the cyberGIS (Geographical information science) approach to spatial analysis for health studies. (cyberGIS is defined as geographic information systems and science based on advanced cyberinfrastructure.) As spatial analysis is a tool for analyze big data it has a high usage in medical fields. According to them many review of literature find that a majority of the methods use only geographically local information and generate non-parametric measurements. It can be found multiple related cases where computational and data sciences are central to solving challenging problems in the framework of health-GIS. Disease mapping is one of the major areas and it is basically used to measure the intensity of a disease in a particular area. Data aggregation is a method which was developed to deal with cancer registry data bases and birth defect data bases. These applications were not only limited for the disease base assessments but it also assess the environmental facts which associate with health such as disparities in geographic access to health care. X. Shi and S. Wang (2015) mentioned a study which estimated the distances or travel times from patientsâ locations represented by polygon level data. In conclusion, the above mentioned two articles review that health care is a field with lot of untapped potentials and the use of big data or data science is not only limited for find remedies for diseases but also it assess the other factors such as which causes the efficiency of health care. 2) Social media and networks Swan, M. (2013) points out having large data quantities continues to allow for new methods and discovery. As Google has proved, finally having large enough data sets was the key moment for progress in many venues, where simple machine-learning algorithms could then be run over large data amounts to produce significant results. She explains this through googlesâ spelling correction and language translation, image recognition and cultural anthropology via word searches on a database of 5 million digitally scanned books. Dhar, V. (2012) also address this manner in his article and further he explains that Googleâs language translator doesnât âunderstandâ language, nor do its algorithms know the contents on webpages. So such efficient and accurate systems were invented using machine learning algorithms not by tackle this problem through an extensive enumeration of possibilities but rather, âtrainâ a computer to interpret questions correctly based on large numbers of examples. In addition, he emphasis knowledge of text processing or âtext miningâ is becoming essential in light of the explosion of text and other unstructured data in healthcare systems, social networks, and other sectors. 3) Education Data science has been attracting a great deal of attention nowadays in academia and environments which dealt with theories and formulas. It improves the current research methods for scientific research in order to form new methods and improve specific theories, methods, and technologies in various fields (Zhu, Y. & Xiong, Y. (2015)). Vast accumulation of data provides the opportunity to filter considerably large portion which is useful to a particular object. It provides a great platform to research rare and important matters in any field. At the same time they argued data science itself requires more fundamental theories and new methods and techniques; for example, the existence of data, the measurement of data, time in cyberspace, data algebra, data similarities and the theory of clusters, data classification etc. New action plans, conferences, workshops, Data science journals, institutes specifically for data science, study materials in universities to study data science as a subject, etc. will increase the awareness and understanding on this new science. 4) Business Business field is one of the major sectors which gain benefits from data science principals and data mining techniques. Data mining techniques are widely used in marketing for tasks such as targeted marketing, online advertising, and recommendations for cross-selling (Provost, F. & Fawcett, T. (2013)). According to them data science is mainly used in business fields with the objective of improving decision making. They have mentioned two types of decisions; (1) decisions for which âdiscoveriesâ need to be made within data, and (2) decisions that repeat, especially at massive scale. In this article Provost, F. & Fawcett, T. (2013) nicely explain how the companies trying to increase their customers by using data science approach. They give an example using company called âTargetâ who sells baby related products. In order to increase the no of customers they were interested in whether they could predict that people are expecting a baby in advance. If so, they could make offers to them before their competitors. Usually most birth records are public, so retailers obtain those information and aware the new parents about their new offers. If the information could get before the baby was born then the ones who got that information first would gain an advantage on their marketing campaign. By using data science techniques, they analyzed historical data on customers and identified group of customers who later revealed to have been pregnant. This can be predicted by using change in motherâs diet, vitamin regimens, etc. According to Provost, F. & Fawcett, T. (2013), banking sector is also gain advantage of using Data science, and they were able to do more sophisticated predictive modeling on pricing, credit limits, low-initial-rate balance transfers, cash back, loyalty points, etc. Specially credit card system is also a outcome of big data analytics. Further they claim that the banks with bigger data assets may have an important strategic advantage over their smaller competitors.so the net result will be either increased acceptance of the bankâs products, decreased cost of customer acquirement, or both. 5) Telecommunication Customer churn is the most critical problem that service providers face. Customers switching from one company to another is called churn (Provost, F. & Fawcett, T. (2013)). They state that attracting new customers is much more expensive than retaining existing customers. So each and every service provider is trying to prevent customer chain by giving them a new retention offer. Data mining techniques are majorly used to identify customers who tend to churn. Challenges and barriers In this process lot of personal data were stored in each individual from any sector. With these vast amount of personal data there are certain boundaries and issues which the researchers or data scientists should be considered. Mainly in health data lot of patients are not comfortable with sharing their data in public (Swan, M. (2013)). In her opinion it is necessary to think about personal data privacy rights and neural data privacy rights proactively to facilitate humanityâs future directions in a mature, comfortable, and empowering way. Dhar, V. (2012) also address this matter. He explains with the vast technology development computer has become the decision maker, unaided by the humans and it raises multitude issues such as cost of incorrect decisions and ethical issues. Conclusion It is evident Data science is a newly emerged science that requires overall knowledge mainly in computational science, statistics and mathematics. New technologies are emerged to dealing with massive amount of data in any field and benefits are many and they are ranging from health care to telecommunication. At the same time they should be handled cautiously to ensure that the respondentsâ information are not exploited. In the near future data science will uncover many discoveries that support humans to improve their life style in every aspect.
...(download the rest of the essay above)
About this essay:
If you use part of this page in your own work, you need to provide a citation, as follows:
Essay Sauce, Data science and its applications . Available from:<https://www.essaysauce.com/information-technology-essays/data-science-and-its-applications/> [Accessed 27-04-24].
These Information technology essays have been submitted to us by students in order to help you with your studies.
* This essay may have been previously published on Essay.uk.com at an earlier date.
Essay Categories:
- Accounting essays
- Architecture essays
- Business essays
- Computer science essays
- Criminology essays
- Economics essays
- Education essays
- Engineering essays
- English language essays
- Environmental studies essays
- Essay examples
- Finance essays
- Geography essays
- Health essays
- History essays
- Hospitality and tourism essays
- Human rights essays
- Information technology essays
- International relations
- Leadership essays
- Linguistics essays
- Literature essays
- Management essays
- Marketing essays
- Mathematics essays
- Media essays
- Medicine essays
- Military essays
- Miscellaneous essays
- Music Essays
- Nursing essays
- Philosophy essays
- Photography and arts essays
- Politics essays
- Project management essays
- Psychology essays
- Religious studies and theology essays
- Sample essays
- Science essays
- Social work essays
- Sociology essays
- Sports essays
- Types of essay
- Zoology essays
Data Science and Crime Analysis for Crime Scientists
For previous occurrences, try the 2023 version of this year.
Prerequisites: SCRIM101 or CRSCI101
Data analysis is a core part of understanding and preventing security and crime problems. This paper introduces students to a variety of methods for analysing security and crime data.
Additional information
Subject regulations
- Paper details current as of 28 Jan 2024 00:04am
- Indicative fees current as of 9 Apr 2024 01:30am
You’re viewing this website as a domestic student
You’re currently viewing the website as a domestic student, you might want to change to international.
You're a domestic student if you are:
- A citizen of New Zealand or Australia
- A New Zealand permanent resident
You're an International student if you are:
- Intending to study on a student visa
- Not a citizen of New Zealand or Australia
Numbers, Facts and Trends Shaping Your World
Read our research on:
Full Topic List
Regions & Countries
- Publications
- Our Methods
- Short Reads
- Tools & Resources
Read Our Research On:
How Pew Research Center will report on generations moving forward
Journalists, researchers and the public often look at society through the lens of generation, using terms like Millennial or Gen Z to describe groups of similarly aged people. This approach can help readers see themselves in the data and assess where we are and where we’re headed as a country.
Pew Research Center has been at the forefront of generational research over the years, telling the story of Millennials as they came of age politically and as they moved more firmly into adult life . In recent years, we’ve also been eager to learn about Gen Z as the leading edge of this generation moves into adulthood.
But generational research has become a crowded arena. The field has been flooded with content that’s often sold as research but is more like clickbait or marketing mythology. There’s also been a growing chorus of criticism about generational research and generational labels in particular.
Recently, as we were preparing to embark on a major research project related to Gen Z, we decided to take a step back and consider how we can study generations in a way that aligns with our values of accuracy, rigor and providing a foundation of facts that enriches the public dialogue.
A typical generation spans 15 to 18 years. As many critics of generational research point out, there is great diversity of thought, experience and behavior within generations.
We set out on a yearlong process of assessing the landscape of generational research. We spoke with experts from outside Pew Research Center, including those who have been publicly critical of our generational analysis, to get their take on the pros and cons of this type of work. We invested in methodological testing to determine whether we could compare findings from our earlier telephone surveys to the online ones we’re conducting now. And we experimented with higher-level statistical analyses that would allow us to isolate the effect of generation.
What emerged from this process was a set of clear guidelines that will help frame our approach going forward. Many of these are principles we’ve always adhered to , but others will require us to change the way we’ve been doing things in recent years.
Here’s a short overview of how we’ll approach generational research in the future:
We’ll only do generational analysis when we have historical data that allows us to compare generations at similar stages of life. When comparing generations, it’s crucial to control for age. In other words, researchers need to look at each generation or age cohort at a similar point in the life cycle. (“Age cohort” is a fancy way of referring to a group of people who were born around the same time.)
When doing this kind of research, the question isn’t whether young adults today are different from middle-aged or older adults today. The question is whether young adults today are different from young adults at some specific point in the past.
To answer this question, it’s necessary to have data that’s been collected over a considerable amount of time – think decades. Standard surveys don’t allow for this type of analysis. We can look at differences across age groups, but we can’t compare age groups over time.
Another complication is that the surveys we conducted 20 or 30 years ago aren’t usually comparable enough to the surveys we’re doing today. Our earlier surveys were done over the phone, and we’ve since transitioned to our nationally representative online survey panel , the American Trends Panel . Our internal testing showed that on many topics, respondents answer questions differently depending on the way they’re being interviewed. So we can’t use most of our surveys from the late 1980s and early 2000s to compare Gen Z with Millennials and Gen Xers at a similar stage of life.
This means that most generational analysis we do will use datasets that have employed similar methodologies over a long period of time, such as surveys from the U.S. Census Bureau. A good example is our 2020 report on Millennial families , which used census data going back to the late 1960s. The report showed that Millennials are marrying and forming families at a much different pace than the generations that came before them.
Even when we have historical data, we will attempt to control for other factors beyond age in making generational comparisons. If we accept that there are real differences across generations, we’re basically saying that people who were born around the same time share certain attitudes or beliefs – and that their views have been influenced by external forces that uniquely shaped them during their formative years. Those forces may have been social changes, economic circumstances, technological advances or political movements.
When we see that younger adults have different views than their older counterparts, it may be driven by their demographic traits rather than the fact that they belong to a particular generation.
The tricky part is isolating those forces from events or circumstances that have affected all age groups, not just one generation. These are often called “period effects.” An example of a period effect is the Watergate scandal, which drove down trust in government among all age groups. Differences in trust across age groups in the wake of Watergate shouldn’t be attributed to the outsize impact that event had on one age group or another, because the change occurred across the board.
Changing demographics also may play a role in patterns that might at first seem like generational differences. We know that the United States has become more racially and ethnically diverse in recent decades, and that race and ethnicity are linked with certain key social and political views. When we see that younger adults have different views than their older counterparts, it may be driven by their demographic traits rather than the fact that they belong to a particular generation.
Controlling for these factors can involve complicated statistical analysis that helps determine whether the differences we see across age groups are indeed due to generation or not. This additional step adds rigor to the process. Unfortunately, it’s often absent from current discussions about Gen Z, Millennials and other generations.
When we can’t do generational analysis, we still see value in looking at differences by age and will do so where it makes sense. Age is one of the most common predictors of differences in attitudes and behaviors. And even if age gaps aren’t rooted in generational differences, they can still be illuminating. They help us understand how people across the age spectrum are responding to key trends, technological breakthroughs and historical events.
Each stage of life comes with a unique set of experiences. Young adults are often at the leading edge of changing attitudes on emerging social trends. Take views on same-sex marriage , for example, or attitudes about gender identity .
Many middle-aged adults, in turn, face the challenge of raising children while also providing care and support to their aging parents. And older adults have their own obstacles and opportunities. All of these stories – rooted in the life cycle, not in generations – are important and compelling, and we can tell them by analyzing our surveys at any given point in time.
When we do have the data to study groups of similarly aged people over time, we won’t always default to using the standard generational definitions and labels. While generational labels are simple and catchy, there are other ways to analyze age cohorts. For example, some observers have suggested grouping people by the decade in which they were born. This would create narrower cohorts in which the members may share more in common. People could also be grouped relative to their age during key historical events (such as the Great Recession or the COVID-19 pandemic) or technological innovations (like the invention of the iPhone).
By choosing not to use the standard generational labels when they’re not appropriate, we can avoid reinforcing harmful stereotypes or oversimplifying people’s complex lived experiences.
Existing generational definitions also may be too broad and arbitrary to capture differences that exist among narrower cohorts. A typical generation spans 15 to 18 years. As many critics of generational research point out, there is great diversity of thought, experience and behavior within generations. The key is to pick a lens that’s most appropriate for the research question that’s being studied. If we’re looking at political views and how they’ve shifted over time, for example, we might group people together according to the first presidential election in which they were eligible to vote.
With these considerations in mind, our audiences should not expect to see a lot of new research coming out of Pew Research Center that uses the generational lens. We’ll only talk about generations when it adds value, advances important national debates and highlights meaningful societal trends.
- Age & Generations
- Demographic Research
- Generation X
- Generation Z
- Generations
- Greatest Generation
- Methodological Research
- Millennials
- Silent Generation
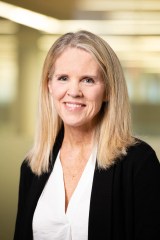
Kim Parker is director of social trends research at Pew Research Center
How Teens and Parents Approach Screen Time
Who are you the art and science of measuring identity, u.s. centenarian population is projected to quadruple over the next 30 years, older workers are growing in number and earning higher wages, teens, social media and technology 2023, most popular.
1615 L St. NW, Suite 800 Washington, DC 20036 USA (+1) 202-419-4300 | Main (+1) 202-857-8562 | Fax (+1) 202-419-4372 | Media Inquiries
Research Topics
- Coronavirus (COVID-19)
- Economy & Work
- Family & Relationships
- Gender & LGBTQ
- Immigration & Migration
- International Affairs
- Internet & Technology
- News Habits & Media
- Non-U.S. Governments
- Other Topics
- Politics & Policy
- Race & Ethnicity
- Email Newsletters
ABOUT PEW RESEARCH CENTER Pew Research Center is a nonpartisan fact tank that informs the public about the issues, attitudes and trends shaping the world. It conducts public opinion polling, demographic research, media content analysis and other empirical social science research. Pew Research Center does not take policy positions. It is a subsidiary of The Pew Charitable Trusts .
Copyright 2024 Pew Research Center
Terms & Conditions
Privacy Policy
Cookie Settings
Reprints, Permissions & Use Policy
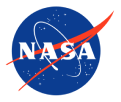
NASA’s Webb Reveals an Exoplanet Atmosphere as Never Seen Before
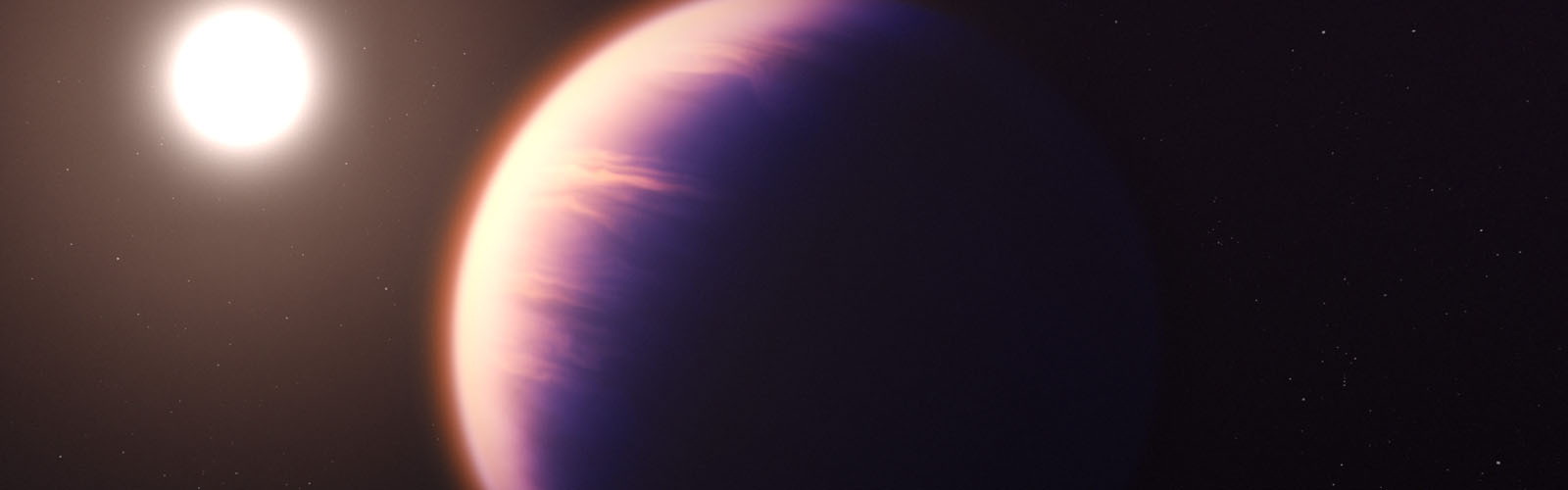
More Firsts from Webb
The exoplanet WASP-39 b is also known as Bocaprins, a name bestowed by the International Astronomical Union for a scenic beach in Aruba. NASA's James Webb Space Telescope provided the most detailed analysis of an exoplanet atmosphere ever with WASP-39 b analysis released in November 2022. Among the 'firsts': Identifying sulfur dioxide in an exoplanet atmosphere Observing photocemistry (reactions triggered by starlight) at work on an exoplanet
NASA’s James Webb Space Telescope just scored another first: a molecular and chemical profile of a distant world’s skies.
While Webb and other space telescopes, including NASA's Hubble and Spitzer, previously have revealed isolated ingredients of this broiling planet’s atmosphere, the new readings from Webb provide a full menu of atoms, molecules, and even signs of active chemistry and clouds.
The latest data also give a hint of how these clouds might look up close: broken up rather than a single, uniform blanket over the planet.
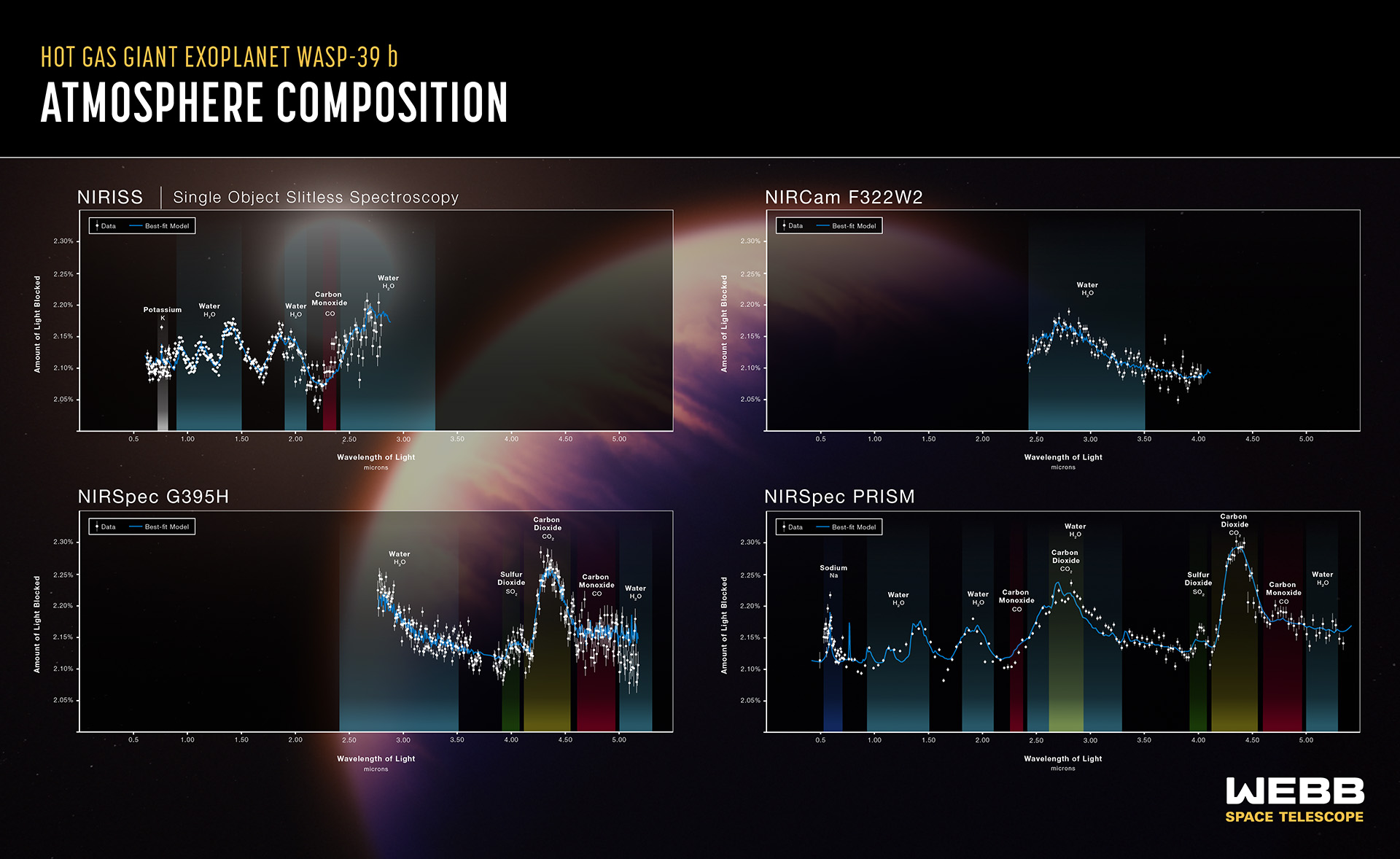
The telescope’s array of highly sensitive instruments was trained on the atmosphere of WASP-39 b, a “hot Saturn” (a planet about as massive as Saturn but in an orbit tighter than Mercury) orbiting a star some 700 light-years away.
The findings bode well for the capability of Webb’s instruments to conduct the broad range of investigations of all types of exoplanets – planets around other stars – hoped for by the science community. That includes probing the atmospheres of smaller, rocky planets like those in the TRAPPIST-1 system.
“We observed the exoplanet with multiple instruments that, together, provide a broad swath of the infrared spectrum and a panoply of chemical fingerprints inaccessible until [this mission],” said Natalie Batalha, an astronomer at the University of California, Santa Cruz, who contributed to and helped coordinate the new research. “Data like these are a game changer.”
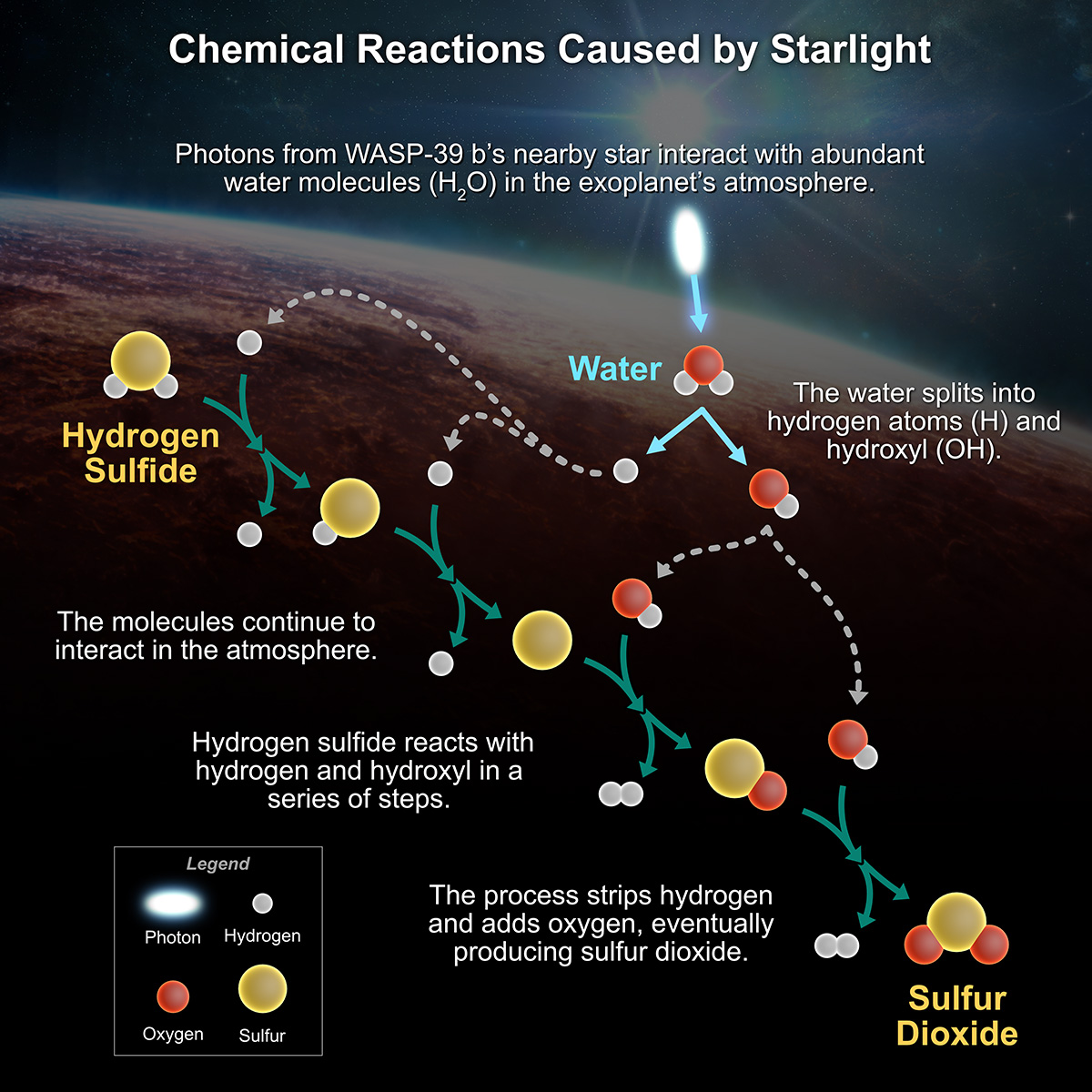
The suite of discoveries is detailed in a set of five new scientific papers, three of which are in press and two of which are under review.
Among the unprecedented revelations is the first detection in an exoplanet atmosphere of sulfur dioxide (SO 2 ), a molecule produced from chemical reactions triggered by high-energy light from the planet’s parent star. On Earth, the protective ozone layer in the upper atmosphere is created in a similar way.
“This is the first time we see concrete evidence of photochemistry – chemical reactions initiated by energetic stellar light – on exoplanets,” said Shang-Min Tsai, a researcher at the University of Oxford in the United Kingdom and lead author of the paper explaining the origin of sulfur dioxide in WASP-39 b’s atmosphere. “I see this as a really promising outlook for advancing our understanding of exoplanet atmospheres with [this mission].”
This led to another first: scientists applying computer models of photochemistry to data that requires such physics to be fully explained. The resulting improvements in modeling will help build the technological know-how to interpret potential signs of habitability in the future.
“Planets are sculpted and transformed by orbiting within the radiation bath of the host star,” Batalha said. “On Earth, those transformations allow life to thrive.”
The planet’s proximity to its host star – eight times closer than Mercury is to our Sun – also makes it a laboratory for studying the effects of radiation from host stars on exoplanets. Better knowledge of the star-planet connection should bring a deeper understanding of how these processes affect the diversity of planets observed in the galaxy.
To see light from WASP-39 b, Webb tracked the planet as it passed in front of its star, allowing some of the star’s light to filter through the planet’s atmosphere. Different types of chemicals in the atmosphere absorb different colors of the starlight spectrum, so the colors that are missing tell astronomers which molecules are present. By viewing the universe in infrared light, Webb can pick up chemical fingerprints that can’t be detected in visible light.
Other atmospheric constituents detected by the Webb telescope include sodium (Na), potassium (K), and water vapor (H 2 O), confirming previous space- and ground-based telescope observations as well as finding additional fingerprints of water, at these longer wavelengths, that haven’t been seen before.
Webb also saw carbon dioxide (CO 2 ) at higher resolution, providing twice as much data as reported from its previous observations . Meanwhile, carbon monoxide (CO) was detected, but obvious signatures of both methane (CH 4 ) and hydrogen sulfide (H 2 S) were absent from the Webb data. If present, these molecules occur at very low levels.
To capture this broad spectrum of WASP-39 b’s atmosphere, an international team numbering in the hundreds independently analyzed data from four of the Webb telescope’s finely calibrated instrument modes.
"We had predicted what [the telescope] would show us, but it was more precise, more diverse and more beautiful than I actually believed it would be,” said Hannah Wakeford, an astrophysicist at the University of Bristol in the United Kingdom who investigates exoplanet atmospheres.
Having such a complete roster of chemical ingredients in an exoplanet atmosphere also gives scientists a glimpse of the abundance of different elements in relation to each other, such as carbon-to-oxygen or potassium-to-oxygen ratios. That, in turn, provides insight into how this planet – and perhaps others – formed out of the disk of gas and dust surrounding the parent star in its younger years.
WASP-39 b’s chemical inventory suggests a history of smashups and mergers of smaller bodies called planetesimals to create an eventual goliath of a planet.
“The abundance of sulfur [relative to] hydrogen indicated that the planet presumably experienced significant accretion of planetesimals that can deliver [these ingredients] to the atmosphere,” said Kazumasa Ohno, a UC Santa Cruz exoplanet researcher who worked on Webb data. “The data also indicates that the oxygen is a lot more abundant than the carbon in the atmosphere. This potentially indicates that WASP-39 b originally formed far away from the central star.”
In so precisely parsing an exoplanet atmosphere, the Webb telescope’s instruments performed well beyond scientists’ expectations – and promise a new phase of exploration among the broad variety of exoplanets in the galaxy.
“We are going to be able to see the big picture of exoplanet atmospheres,” said Laura Flagg, a researcher at Cornell University and a member of the international team. “It is incredibly exciting to know that everything is going to be rewritten. That is one of the best parts of being a scientist.”
The James Webb Space Telescope is the world's premier space science observatory. Webb will solve mysteries in our solar system, look beyond to distant worlds around other stars, and probe the mysterious structures and origins of our universe and our place in it. Webb is an international program led by NASA with its partners, ESA (European Space Agency) and CSA (Canadian Space Agency).
Read the papers:
The science paper by L. Alderson et al. The science paper by Z. Rustamkulov et al. The science paper by E. Ahrer et al. The science paper by A. Feinstein et al. The science paper by S. Tsai et al.
Related Terms
- Gas Giant Exoplanets
- James Webb Space Telescope (JWST)
Explore More
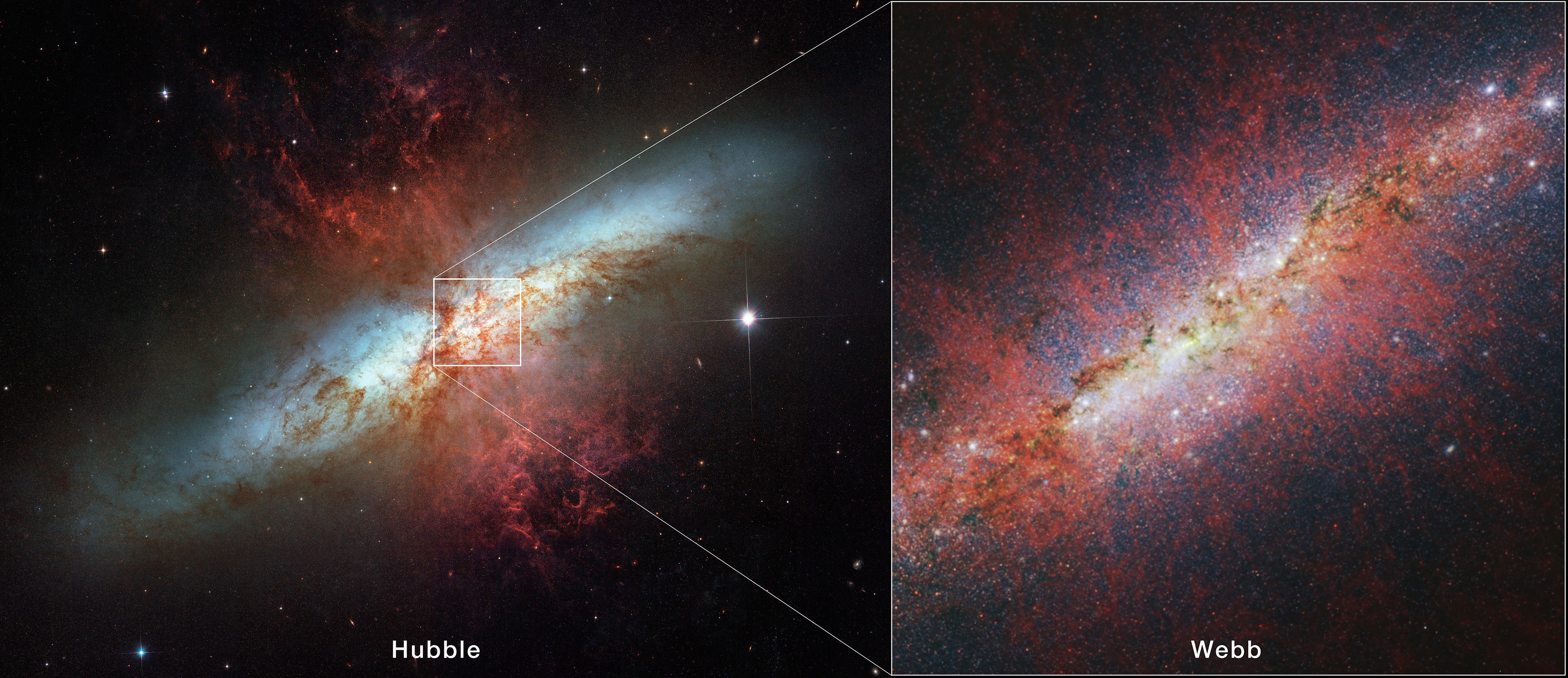
NASA’s Webb Probes an Extreme Starburst Galaxy
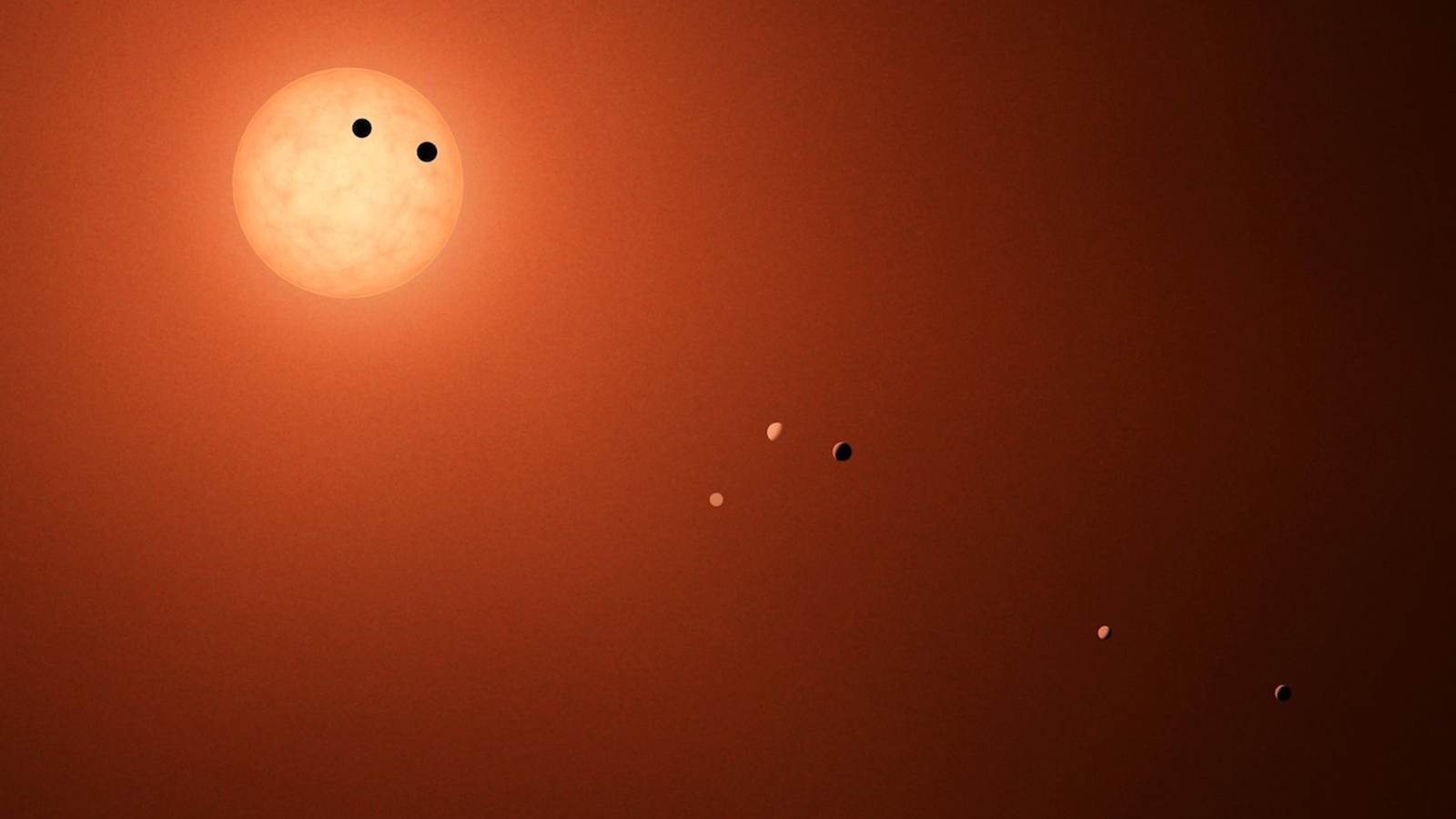
That Starry Night Sky? It’s Full of Eclipses
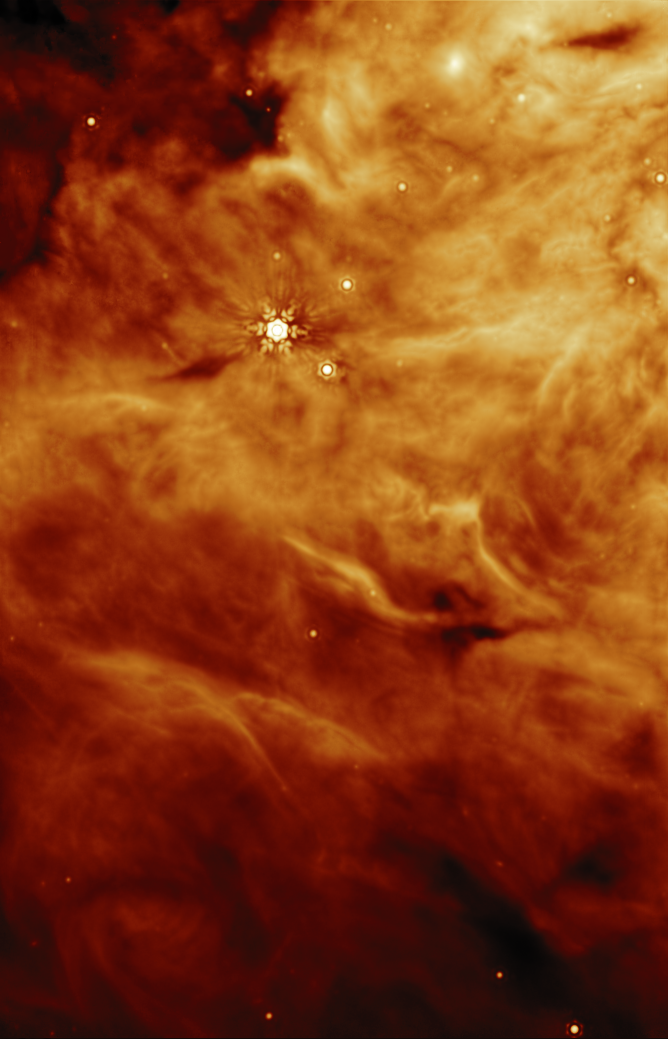
Cheers! NASA’s Webb Finds Ethanol, Other Icy Ingredients for Worlds
Discover more topics from nasa.
Search for Life
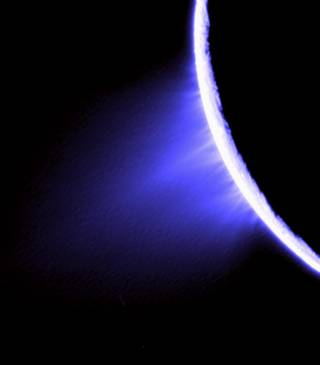
Black Holes
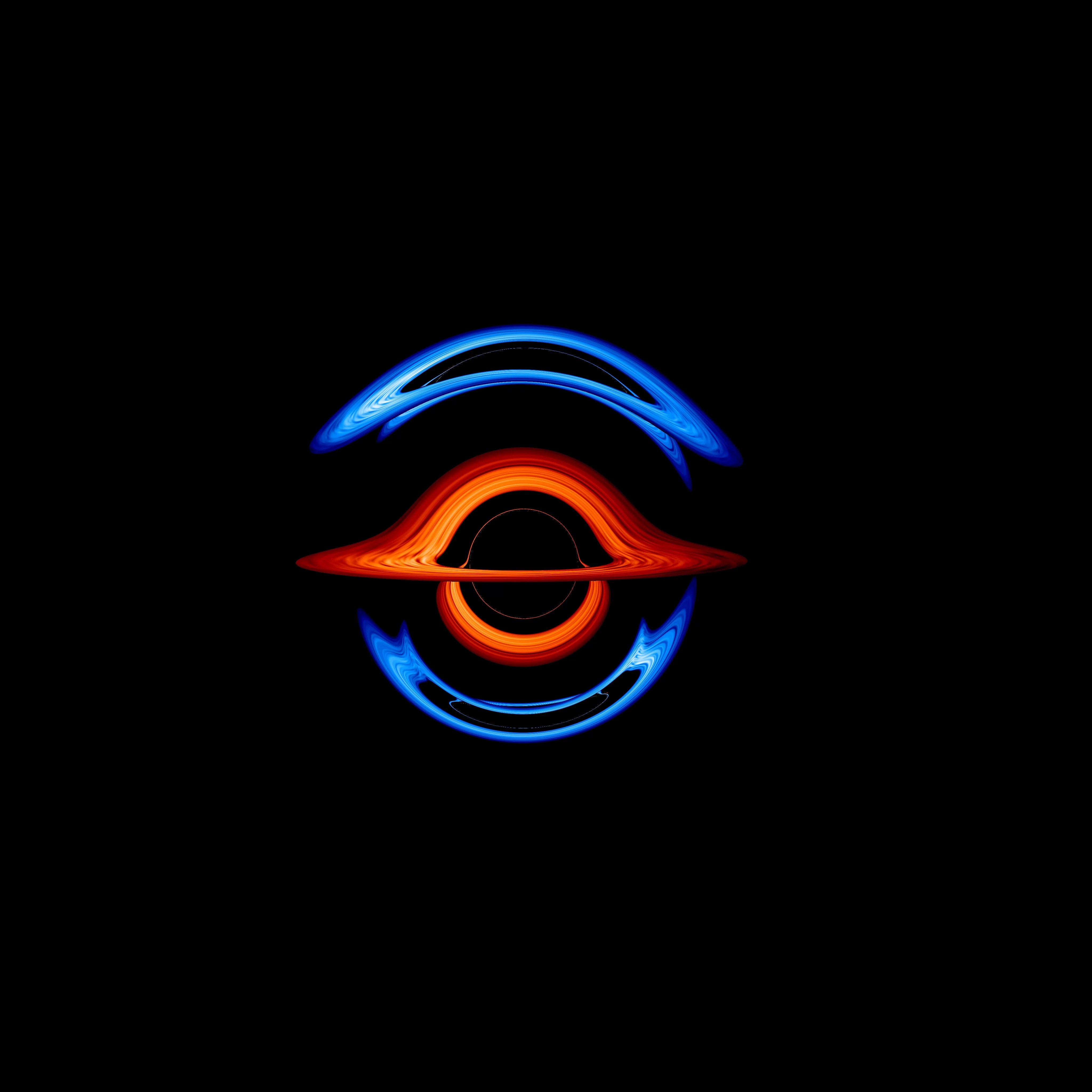
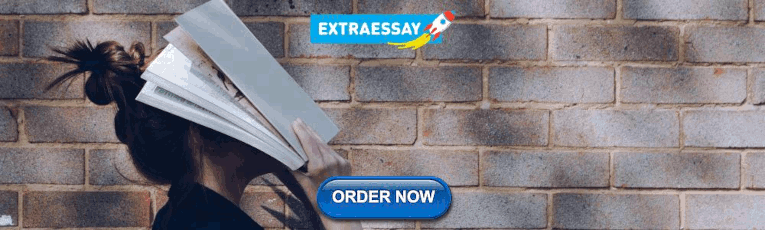
IMAGES
VIDEO
COMMENTS
As an open access platform of the Harvard Data Science Initiative, Harvard Data Science Review (HDSR) features foundational thinking, research milestones, educational innovations, and major applications, with a primary emphasis on reproducibility, replicability, and readability.We aim to publish content that helps define and shape data science as a scientifically rigorous and globally ...
5 Reasons to Study Data Science. The field of data science is a great career choice that offers high salaries, opportunities across several industries, and long-term job security. Here are five reasons to consider a career in data science. 1. Data Scientists Are in High Demand.
A data scientist earns an average salary of $108,659 in the United States, according to Lightcast™ [1]. Demand is high for data professionals—data scientists occupations are expected to grow by 36 percent in the next 10 years (much faster than average), according to the US Bureau of Labor Statistics (BLS) [ 2 ].
The Structure of a Successful Data Science SOP. It goes without saying that Bennett used the SOP Starter Kit to outline his essay. That means he structured the paragraphs as follows: Introduction Frame Narrative - 1 paragraph (12% of word count) Why This Program - 2 paragraphs (23% of word count)
Each sub-layer of the transformers in the Attention paper is wrapped by a skip-layer, or a residual-block (see image below). The idea behind this block is that given an input x, a network tries to learn some function H (x), which can be loosely written as H (x) = F (x) + x. Using the skip-layer mechanism, we force the middle layer to learn F (x ...
a well-structured essay can distinguish between a reader grasping the essence of data findings and being overwhelmed by the technical details. Here's how to structure your data science essays to enhance clarity and impact. by erika; April 24, 2024 April 24, 2024
Definition, Examples, Tools & More. Data science is an interdisciplinary field that uses scientific methods, processes, algorithms, and systems to extract knowledge and insights from structured and unstructured data. Data science has been hailed as the 'sexiest job of the 21st century', and this is not just a hyperbolic claim.
This paper shows data science's potential for disruptive innovation in science, industry, policy, and people's lives. We present how data science impacts science and society at large in the coming years, including ethical problems in managing human behavior data and considering the quantitative expectations of data science economic impact. We introduce concepts such as open science and e ...
Recommendation 2.3: To prepare their graduates for this new data-driven era, academic institutions should encourage the development of a basic understanding of data science in all undergraduates. Recommendation 2.4: Ethics is a topic that, given the nature of data science, students should learn and practice throughout their education.
The data science blogging ecosystem is rich and growing. TDS alone has an archive of more than 20,000 posts across numerous topics. Many experts have launched Substacks, newsletters, or personal blogs. ... PhD., an experienced researcher, wrote the article he wished he had read before writing research papers: How to Write and Publish a Research ...
Introduction. We are living in the age of "data science and advanced analytics", where almost everything in our daily lives is digitally recorded as data [].Thus the current electronic world is a wealth of various kinds of data, such as business data, financial data, healthcare data, multimedia data, internet of things (IoT) data, cybersecurity data, social media data, etc [].
The data scientist career path is probably the hottest career choice you can currently make. It is not only the $108,000 median base salary that makes the position appealing to job seekers, data science also hits high on satisfaction with a score of 4.2 out of 5, as findings from the latest Glassdoor report reveal.
This is an example personal statement for a Masters degree application in Data Science. See our guide for advice on writing your own postgraduate personal statement. The emergence of big data over the past decade as a power for good - and, dare I say it, evil - has convinced me of the importance of developing and honing my skills in this arena.
Data Scientist Career Path: How to Get Into Data Science. CareerOneStop indicates that 37 percent of data scientists have obtained their bachelor's degree, usually in a field such as statistics, computer science, information technologies, mathematics, or data science.In addition, 35 percent of data scientists hold a master's degree, and 14 percent have attained a doctoral degree.
As a Data Scientist: My first 6 months of being a Data Scientist were the most challenging period in my career. It was a small team, there were 5 of us, and thankfully I had one of the best leads that I could hope for. He taught the real life Data Science as opposed to what I learnt from Kaggle and from doing MOOCs. I struggled a bit in the ...
1. Introduction. Statistics and data science are more popular than ever in this era of data explosion and technological advances. Decades ago, John Tukey (Brillinger, 2014) said, "The best thing about being a statistician is that you get to play in everyone's backyard."More recently, Xiao-Li Meng (2009) said, "We no longer simply enjoy the privilege of playing in or cleaning up everyone ...
Introduction. Academic and applied research in data-intensive fields requires the development of a diverse skillset, of which clear writing and communication are among the most important. 1 However, in our experience, the art of scientific communication is often ignored in the formal training of data scientists in diverse fields spanning the life, social, physical, mathematical, and medical ...
Essay 3: Communication and Collaboration (350 words maximum) Professional Data Scientists need strong technical skills, in addition to strong collaborative and communication skills to effectively work with others. Please describe a specific time you had a miscommunication with a peer.
Career paths in data science. Data science professionals can work in technology companies, government agencies, non-profit organisations, etc. Once you learn the skills, they are transferable between industries. Here are some career paths to choose from: Data Scientist. Data scientists use analytical data skills to solve complex business problems.
Data Scientist and Cloud Computing. Introduction Data Scientist is a role in which data professionals analyze and process data to produce meaningful insights. This can range from statistical analysis of financial statements to developing computer algorithms that predict trends, detect defects, or recommend the best route to a particular ...
Data Science, in other words, is the science that combines programming skills and mathematical and statistical knowledge to extract meaningful information from data. Data Science consists of the application of machine learning algorithms to numerical, textual data, images, video, and audio content. The algorithms, therefore, perform specific ...
Interviewers might appreciate hearing a detailed response about technology, statistics and the data science life cycle, which would prove your expertise. They might also want to know that you understand the role data science plays in business. This question also provides insight into your career goals. The interviewer can learn what drew you to ...
An answer I get often is: "I love being able to tell people what to do based on data". I'm sure everyone can relate to that being a good feeling. Imagine going up to your boss and saying, "well, that campaign you did last month was a mistake, here is why and here is what you should do instead". Of course, not every data scientist does ...
Applications of Data Science. 1) Health care. A key contemporary trend emerging in big data science is the quantified self (QS) -individuals engaged in the self-tracking of any kind of biological, physical, behavioral, or environmental information as n = 1 individuals or in groups (Swan, M. (2013)). In this article writer emphasis that ...
Data Science and Crime Analysis for Crime Scientists. 2024. Change year. 2023; 2022; This paper is not offered for 2024. For previous occurrences, try the 2023 version of this year. 15. 200. Prerequisites: SCRIM101 or CRSCI101 Jump to. Data analysis is a core part of understanding and preventing security and crime problems. ...
Even when we have historical data, we will attempt to control for other factors beyond age in making generational comparisons. If we accept that there are real differences across generations, we're basically saying that people who were born around the same time share certain attitudes or beliefs - and that their views have been influenced ...
My pivot point for choosing to pursue data science came last April when my team competed at the University of Chicago Econometrics Games. Like a Hackathon for young econometricians, the games pitted students of economics from Cambridge to Santa Clara. The objective was to stage, and answer, a question of economic importance within fourteen hours.
This study was designed to recruit participants for and conduct cognitive testing of the Office of Management and Budget's (OMB's) initial set of recommended revisions to the statistical standards for collecting race and ethnicity data (88 FR 5373).
This illustration shows what exoplanet WASP-39 b could look like, based on current understanding of the planet. NASA's James Webb Space Telescope's exquisitely sensitive instruments have provided a profile of WASP-39 b's atmospheric constituents and identified a plethora of contents, including water, sulfur dioxide, carbon monoxide, sodium and potassium.