- Privacy Policy

Home » Case Study – Methods, Examples and Guide
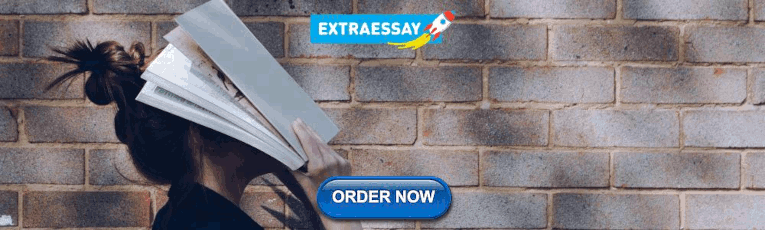
Case Study – Methods, Examples and Guide
Table of Contents

A case study is a research method that involves an in-depth examination and analysis of a particular phenomenon or case, such as an individual, organization, community, event, or situation.
It is a qualitative research approach that aims to provide a detailed and comprehensive understanding of the case being studied. Case studies typically involve multiple sources of data, including interviews, observations, documents, and artifacts, which are analyzed using various techniques, such as content analysis, thematic analysis, and grounded theory. The findings of a case study are often used to develop theories, inform policy or practice, or generate new research questions.
Types of Case Study
Types and Methods of Case Study are as follows:
Single-Case Study
A single-case study is an in-depth analysis of a single case. This type of case study is useful when the researcher wants to understand a specific phenomenon in detail.
For Example , A researcher might conduct a single-case study on a particular individual to understand their experiences with a particular health condition or a specific organization to explore their management practices. The researcher collects data from multiple sources, such as interviews, observations, and documents, and uses various techniques to analyze the data, such as content analysis or thematic analysis. The findings of a single-case study are often used to generate new research questions, develop theories, or inform policy or practice.
Multiple-Case Study
A multiple-case study involves the analysis of several cases that are similar in nature. This type of case study is useful when the researcher wants to identify similarities and differences between the cases.
For Example, a researcher might conduct a multiple-case study on several companies to explore the factors that contribute to their success or failure. The researcher collects data from each case, compares and contrasts the findings, and uses various techniques to analyze the data, such as comparative analysis or pattern-matching. The findings of a multiple-case study can be used to develop theories, inform policy or practice, or generate new research questions.
Exploratory Case Study
An exploratory case study is used to explore a new or understudied phenomenon. This type of case study is useful when the researcher wants to generate hypotheses or theories about the phenomenon.
For Example, a researcher might conduct an exploratory case study on a new technology to understand its potential impact on society. The researcher collects data from multiple sources, such as interviews, observations, and documents, and uses various techniques to analyze the data, such as grounded theory or content analysis. The findings of an exploratory case study can be used to generate new research questions, develop theories, or inform policy or practice.
Descriptive Case Study
A descriptive case study is used to describe a particular phenomenon in detail. This type of case study is useful when the researcher wants to provide a comprehensive account of the phenomenon.
For Example, a researcher might conduct a descriptive case study on a particular community to understand its social and economic characteristics. The researcher collects data from multiple sources, such as interviews, observations, and documents, and uses various techniques to analyze the data, such as content analysis or thematic analysis. The findings of a descriptive case study can be used to inform policy or practice or generate new research questions.
Instrumental Case Study
An instrumental case study is used to understand a particular phenomenon that is instrumental in achieving a particular goal. This type of case study is useful when the researcher wants to understand the role of the phenomenon in achieving the goal.
For Example, a researcher might conduct an instrumental case study on a particular policy to understand its impact on achieving a particular goal, such as reducing poverty. The researcher collects data from multiple sources, such as interviews, observations, and documents, and uses various techniques to analyze the data, such as content analysis or thematic analysis. The findings of an instrumental case study can be used to inform policy or practice or generate new research questions.
Case Study Data Collection Methods
Here are some common data collection methods for case studies:
Interviews involve asking questions to individuals who have knowledge or experience relevant to the case study. Interviews can be structured (where the same questions are asked to all participants) or unstructured (where the interviewer follows up on the responses with further questions). Interviews can be conducted in person, over the phone, or through video conferencing.
Observations
Observations involve watching and recording the behavior and activities of individuals or groups relevant to the case study. Observations can be participant (where the researcher actively participates in the activities) or non-participant (where the researcher observes from a distance). Observations can be recorded using notes, audio or video recordings, or photographs.
Documents can be used as a source of information for case studies. Documents can include reports, memos, emails, letters, and other written materials related to the case study. Documents can be collected from the case study participants or from public sources.
Surveys involve asking a set of questions to a sample of individuals relevant to the case study. Surveys can be administered in person, over the phone, through mail or email, or online. Surveys can be used to gather information on attitudes, opinions, or behaviors related to the case study.
Artifacts are physical objects relevant to the case study. Artifacts can include tools, equipment, products, or other objects that provide insights into the case study phenomenon.
How to conduct Case Study Research
Conducting a case study research involves several steps that need to be followed to ensure the quality and rigor of the study. Here are the steps to conduct case study research:
- Define the research questions: The first step in conducting a case study research is to define the research questions. The research questions should be specific, measurable, and relevant to the case study phenomenon under investigation.
- Select the case: The next step is to select the case or cases to be studied. The case should be relevant to the research questions and should provide rich and diverse data that can be used to answer the research questions.
- Collect data: Data can be collected using various methods, such as interviews, observations, documents, surveys, and artifacts. The data collection method should be selected based on the research questions and the nature of the case study phenomenon.
- Analyze the data: The data collected from the case study should be analyzed using various techniques, such as content analysis, thematic analysis, or grounded theory. The analysis should be guided by the research questions and should aim to provide insights and conclusions relevant to the research questions.
- Draw conclusions: The conclusions drawn from the case study should be based on the data analysis and should be relevant to the research questions. The conclusions should be supported by evidence and should be clearly stated.
- Validate the findings: The findings of the case study should be validated by reviewing the data and the analysis with participants or other experts in the field. This helps to ensure the validity and reliability of the findings.
- Write the report: The final step is to write the report of the case study research. The report should provide a clear description of the case study phenomenon, the research questions, the data collection methods, the data analysis, the findings, and the conclusions. The report should be written in a clear and concise manner and should follow the guidelines for academic writing.
Examples of Case Study
Here are some examples of case study research:
- The Hawthorne Studies : Conducted between 1924 and 1932, the Hawthorne Studies were a series of case studies conducted by Elton Mayo and his colleagues to examine the impact of work environment on employee productivity. The studies were conducted at the Hawthorne Works plant of the Western Electric Company in Chicago and included interviews, observations, and experiments.
- The Stanford Prison Experiment: Conducted in 1971, the Stanford Prison Experiment was a case study conducted by Philip Zimbardo to examine the psychological effects of power and authority. The study involved simulating a prison environment and assigning participants to the role of guards or prisoners. The study was controversial due to the ethical issues it raised.
- The Challenger Disaster: The Challenger Disaster was a case study conducted to examine the causes of the Space Shuttle Challenger explosion in 1986. The study included interviews, observations, and analysis of data to identify the technical, organizational, and cultural factors that contributed to the disaster.
- The Enron Scandal: The Enron Scandal was a case study conducted to examine the causes of the Enron Corporation’s bankruptcy in 2001. The study included interviews, analysis of financial data, and review of documents to identify the accounting practices, corporate culture, and ethical issues that led to the company’s downfall.
- The Fukushima Nuclear Disaster : The Fukushima Nuclear Disaster was a case study conducted to examine the causes of the nuclear accident that occurred at the Fukushima Daiichi Nuclear Power Plant in Japan in 2011. The study included interviews, analysis of data, and review of documents to identify the technical, organizational, and cultural factors that contributed to the disaster.
Application of Case Study
Case studies have a wide range of applications across various fields and industries. Here are some examples:
Business and Management
Case studies are widely used in business and management to examine real-life situations and develop problem-solving skills. Case studies can help students and professionals to develop a deep understanding of business concepts, theories, and best practices.
Case studies are used in healthcare to examine patient care, treatment options, and outcomes. Case studies can help healthcare professionals to develop critical thinking skills, diagnose complex medical conditions, and develop effective treatment plans.
Case studies are used in education to examine teaching and learning practices. Case studies can help educators to develop effective teaching strategies, evaluate student progress, and identify areas for improvement.
Social Sciences
Case studies are widely used in social sciences to examine human behavior, social phenomena, and cultural practices. Case studies can help researchers to develop theories, test hypotheses, and gain insights into complex social issues.
Law and Ethics
Case studies are used in law and ethics to examine legal and ethical dilemmas. Case studies can help lawyers, policymakers, and ethical professionals to develop critical thinking skills, analyze complex cases, and make informed decisions.
Purpose of Case Study
The purpose of a case study is to provide a detailed analysis of a specific phenomenon, issue, or problem in its real-life context. A case study is a qualitative research method that involves the in-depth exploration and analysis of a particular case, which can be an individual, group, organization, event, or community.
The primary purpose of a case study is to generate a comprehensive and nuanced understanding of the case, including its history, context, and dynamics. Case studies can help researchers to identify and examine the underlying factors, processes, and mechanisms that contribute to the case and its outcomes. This can help to develop a more accurate and detailed understanding of the case, which can inform future research, practice, or policy.
Case studies can also serve other purposes, including:
- Illustrating a theory or concept: Case studies can be used to illustrate and explain theoretical concepts and frameworks, providing concrete examples of how they can be applied in real-life situations.
- Developing hypotheses: Case studies can help to generate hypotheses about the causal relationships between different factors and outcomes, which can be tested through further research.
- Providing insight into complex issues: Case studies can provide insights into complex and multifaceted issues, which may be difficult to understand through other research methods.
- Informing practice or policy: Case studies can be used to inform practice or policy by identifying best practices, lessons learned, or areas for improvement.
Advantages of Case Study Research
There are several advantages of case study research, including:
- In-depth exploration: Case study research allows for a detailed exploration and analysis of a specific phenomenon, issue, or problem in its real-life context. This can provide a comprehensive understanding of the case and its dynamics, which may not be possible through other research methods.
- Rich data: Case study research can generate rich and detailed data, including qualitative data such as interviews, observations, and documents. This can provide a nuanced understanding of the case and its complexity.
- Holistic perspective: Case study research allows for a holistic perspective of the case, taking into account the various factors, processes, and mechanisms that contribute to the case and its outcomes. This can help to develop a more accurate and comprehensive understanding of the case.
- Theory development: Case study research can help to develop and refine theories and concepts by providing empirical evidence and concrete examples of how they can be applied in real-life situations.
- Practical application: Case study research can inform practice or policy by identifying best practices, lessons learned, or areas for improvement.
- Contextualization: Case study research takes into account the specific context in which the case is situated, which can help to understand how the case is influenced by the social, cultural, and historical factors of its environment.
Limitations of Case Study Research
There are several limitations of case study research, including:
- Limited generalizability : Case studies are typically focused on a single case or a small number of cases, which limits the generalizability of the findings. The unique characteristics of the case may not be applicable to other contexts or populations, which may limit the external validity of the research.
- Biased sampling: Case studies may rely on purposive or convenience sampling, which can introduce bias into the sample selection process. This may limit the representativeness of the sample and the generalizability of the findings.
- Subjectivity: Case studies rely on the interpretation of the researcher, which can introduce subjectivity into the analysis. The researcher’s own biases, assumptions, and perspectives may influence the findings, which may limit the objectivity of the research.
- Limited control: Case studies are typically conducted in naturalistic settings, which limits the control that the researcher has over the environment and the variables being studied. This may limit the ability to establish causal relationships between variables.
- Time-consuming: Case studies can be time-consuming to conduct, as they typically involve a detailed exploration and analysis of a specific case. This may limit the feasibility of conducting multiple case studies or conducting case studies in a timely manner.
- Resource-intensive: Case studies may require significant resources, including time, funding, and expertise. This may limit the ability of researchers to conduct case studies in resource-constrained settings.
About the author
Muhammad Hassan
Researcher, Academic Writer, Web developer
You may also like

Correlational Research – Methods, Types and...

Basic Research – Types, Methods and Examples

One-to-One Interview – Methods and Guide

Mixed Methods Research – Types & Analysis

Transformative Design – Methods, Types, Guide

Quasi-Experimental Research Design – Types...
- AI & Tech
- Case Studies
- Churn & Loyalty
- Customer Experience
- Customer Journeys
- Data analytics
- Feedback Analysis
- Product Experience
- Product Updates
- Sentiment Analysis
- Surveys & Feedback Collection
- Text Analytics
- Thematic vs Others
- Using Thematic
- Voice of Customer
- Try Thematic
- Data Analytics
- Thematic vs others
- AI and tech
Welcome to the community
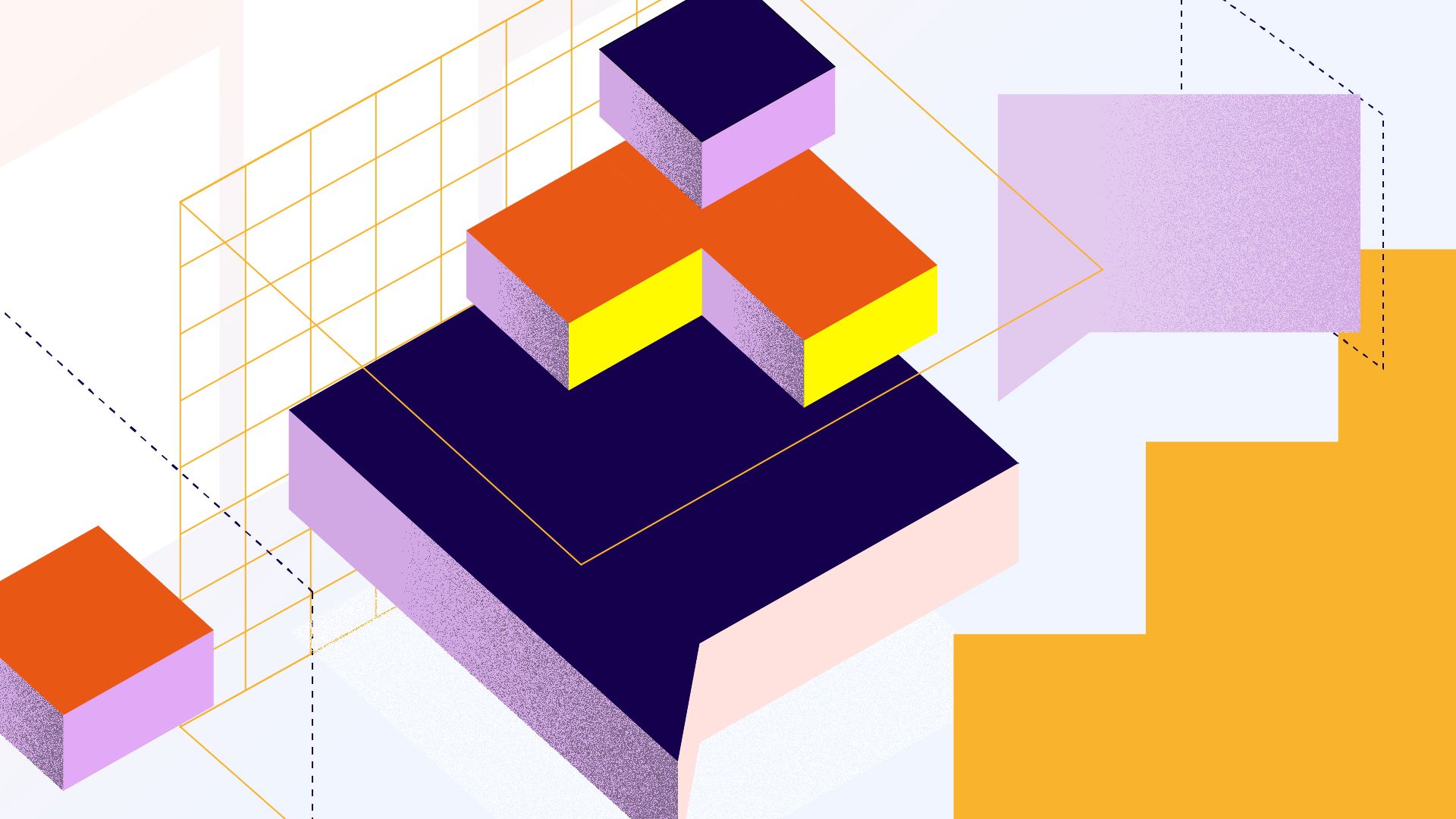
Qualitative Data Analysis: Step-by-Step Guide (Manual vs. Automatic)
When we need to understand and report on people's opinions, for example customer feedback, we always turn to qualitative data. Qualitative data is typically generated through:
- Interview transcripts
- Surveys with open-ended questions
- Contact center transcripts
- Reviews, emails or complaints
- Audio and video recordings
- Employee notes
Compared to quantitative data, which captures structured information, qualitative data is unstructured and has more depth. It can answer our questions, can help formulate hypotheses and build understanding.
It's important to understand the differences between quantitative data & qualitative data . But unfortunately, analyzing qualitative data is difficult. While tools like Excel, Tableau and PowerBI crunch and visualize quantitative data with ease, there are a limited number of mainstream tools for analyzing qualitative data . Despite the rise of Generative AI , the majority of qualitative data analysis still happens manually.
The good news is that we are moving from purely manual analysis, to assisted qualitative research. Depending on data privacy rules in relation to Gen AI in your business, you might be using Microsoft Co-Pilot or ChatGPT directly. And when you hit the wall with these simple tool, there are plenty of user-friendly software that harness AI for qualitative research. Both help automate the qualitative data analysis process.
In this post we want to teach you how to conduct a successful qualitative data analysis. First, we'll review the basics of conducting the analysis manually. Then, we'll look at how a DIY solution utilizing ChatGPT or CoPilot might look like. Finally, we'll review software solutions powered by AI that can make it even easier.
More businesses are switching to fully-automated analysis of qualitative customer data because it is cheaper, faster, and just as accurate. Primarily, businesses purchase subscriptions to feedback analytics platforms so that they can understand customer pain points and sentiment.
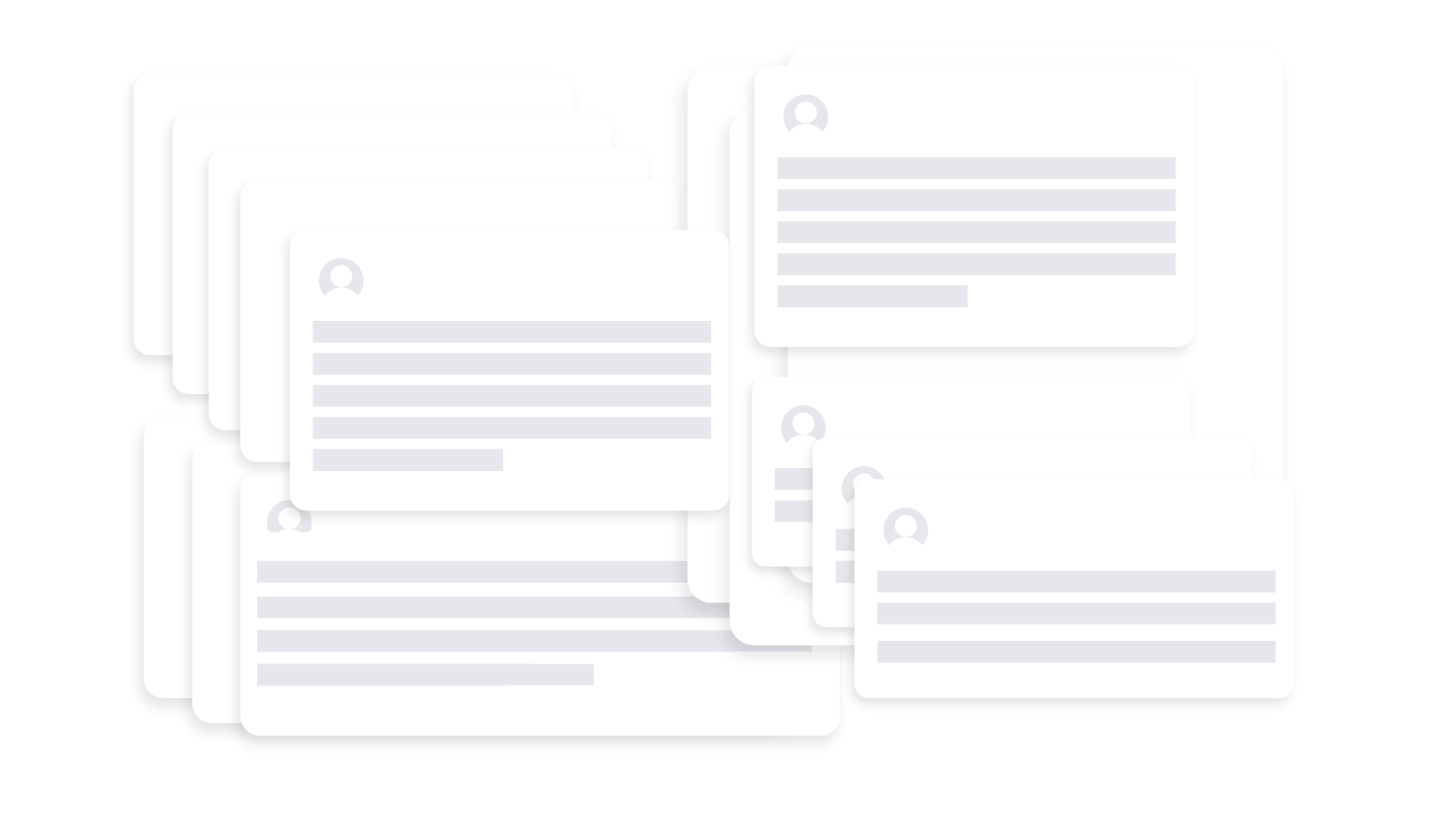
We’ll take you through 5 steps to conduct a successful qualitative data analysis. Within each step we will highlight the key difference between the manual, and automated approach of qualitative researchers. Here's an overview of the steps:
The 5 steps to doing qualitative data analysis
- Gathering and collecting your qualitative data
- Organizing and connecting into your qualitative data
- Coding your qualitative data
- Analyzing the qualitative data for insights
- Reporting on the insights derived from your analysis
What is Qualitative Data Analysis?
Qualitative data analysis is a process of gathering, structuring and interpreting qualitative data to understand what it represents.
Qualitative data is non-numerical and unstructured. Qualitative data generally refers to text, such as open-ended responses to survey questions or user interviews, but also includes audio, photos and video.
Businesses often perform qualitative data analysis on customer feedback. And within this context, qualitative data generally refers to verbatim text data collected from sources such as reviews, complaints, chat messages, support centre interactions, customer interviews, case notes or social media comments.
How is qualitative data analysis different from quantitative data analysis?
Understanding the differences between quantitative & qualitative data is important. When it comes to analyzing data, Qualitative Data Analysis serves a very different role to Quantitative Data Analysis. But what sets them apart?
Qualitative Data Analysis dives into the stories hidden in non-numerical data such as interviews, open-ended survey answers, or notes from observations. It uncovers the ‘whys’ and ‘hows’ giving a deep understanding of people’s experiences and emotions.
Quantitative Data Analysis on the other hand deals with numerical data, using statistics to measure differences, identify preferred options, and pinpoint root causes of issues. It steps back to address questions like "how many" or "what percentage" to offer broad insights we can apply to larger groups.
In short, Qualitative Data Analysis is like a microscope, helping us understand specific detail. Quantitative Data Analysis is like the telescope, giving us a broader perspective. Both are important, working together to decode data for different objectives.
Qualitative Data Analysis methods
Once all the data has been captured, there are a variety of analysis techniques available and the choice is determined by your specific research objectives and the kind of data you’ve gathered. Common qualitative data analysis methods include:
Content Analysis
This is a popular approach to qualitative data analysis. Other qualitative analysis techniques may fit within the broad scope of content analysis. Thematic analysis is a part of the content analysis. Content analysis is used to identify the patterns that emerge from text, by grouping content into words, concepts, and themes. Content analysis is useful to quantify the relationship between all of the grouped content. The Columbia School of Public Health has a detailed breakdown of content analysis .
Narrative Analysis
Narrative analysis focuses on the stories people tell and the language they use to make sense of them. It is particularly useful in qualitative research methods where customer stories are used to get a deep understanding of customers’ perspectives on a specific issue. A narrative analysis might enable us to summarize the outcomes of a focused case study.
Discourse Analysis
Discourse analysis is used to get a thorough understanding of the political, cultural and power dynamics that exist in specific situations. The focus of discourse analysis here is on the way people express themselves in different social contexts. Discourse analysis is commonly used by brand strategists who hope to understand why a group of people feel the way they do about a brand or product.
Thematic Analysis
Thematic analysis is used to deduce the meaning behind the words people use. This is accomplished by discovering repeating themes in text. These meaningful themes reveal key insights into data and can be quantified, particularly when paired with sentiment analysis . Often, the outcome of thematic analysis is a code frame that captures themes in terms of codes, also called categories. So the process of thematic analysis is also referred to as “coding”. A common use-case for thematic analysis in companies is analysis of customer feedback.
AI-powered software to transform qualitative data into powerful insights that drive decision making.
Grounded Theory
Grounded theory is a useful approach when little is known about a subject. Grounded theory starts by formulating a theory around a single data case. This means that the theory is “grounded”. Grounded theory analysis is based on actual data, and not entirely speculative. Then additional cases can be examined to see if they are relevant and can add to the original grounded theory.
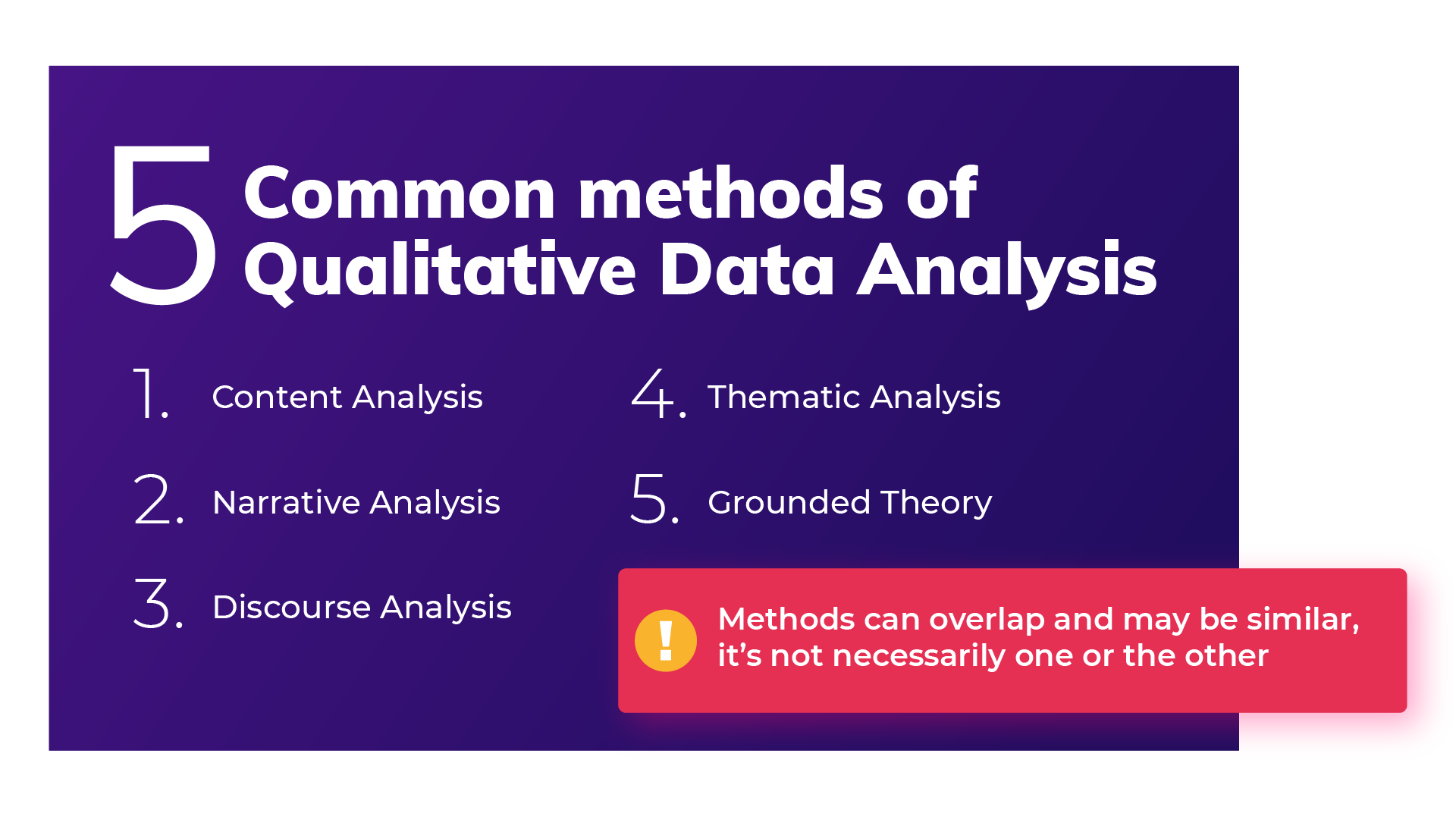
Challenges of Qualitative Data Analysis
While Qualitative Data Analysis offers rich insights, it comes with its challenges. Each unique QDA method has its unique hurdles. Let’s take a look at the challenges researchers and analysts might face, depending on the chosen method.
- Time and Effort (Narrative Analysis): Narrative analysis, which focuses on personal stories, demands patience. Sifting through lengthy narratives to find meaningful insights can be time-consuming, requires dedicated effort.
- Being Objective (Grounded Theory): Grounded theory, building theories from data, faces the challenges of personal biases. Staying objective while interpreting data is crucial, ensuring conclusions are rooted in the data itself.
- Complexity (Thematic Analysis): Thematic analysis involves identifying themes within data, a process that can be intricate. Categorizing and understanding themes can be complex, especially when each piece of data varies in context and structure. Thematic Analysis software can simplify this process.
- Generalizing Findings (Narrative Analysis): Narrative analysis, dealing with individual stories, makes drawing broad challenging. Extending findings from a single narrative to a broader context requires careful consideration.
- Managing Data (Thematic Analysis): Thematic analysis involves organizing and managing vast amounts of unstructured data, like interview transcripts. Managing this can be a hefty task, requiring effective data management strategies.
- Skill Level (Grounded Theory): Grounded theory demands specific skills to build theories from the ground up. Finding or training analysts with these skills poses a challenge, requiring investment in building expertise
See Thematic in Action
Experience the power of AI
Benefits of qualitative data analysis
Qualitative Data Analysis (QDA) is like a versatile toolkit, offering a tailored approach to understanding your data. The benefits it offers are as diverse as the methods. Let’s explore why choosing the right method matters.
- Tailored Methods for Specific Needs: QDA isn't one-size-fits-all. Depending on your research objectives and the type of data at hand, different methods offer unique benefits. If you want emotive customer stories, narrative analysis paints a strong picture. When you want to explain a score, thematic analysis reveals insightful patterns
- Flexibility with Thematic Analysis: thematic analysis is like a chameleon in the toolkit of QDA. It adapts well to different types of data and research objectives, making it a top choice for any qualitative analysis.
- Deeper Understanding, Better Products: QDA helps you dive into people's thoughts and feelings. This deep understanding helps you build products and services that truly matches what people want, ensuring satisfied customers
- Finding the Unexpected: Qualitative data often reveals surprises that we miss in quantitative data. QDA offers us new ideas and perspectives, for insights we might otherwise miss.
- Building Effective Strategies: Insights from QDA are like strategic guides. They help businesses in crafting plans that match people’s desires.
- Creating Genuine Connections: Understanding people’s experiences lets businesses connect on a real level. This genuine connection helps build trust and loyalty, priceless for any business.
How to do Qualitative Data Analysis: 5 steps
Now we are going to show how you can do your own qualitative data analysis. We will guide you through this process step by step. As mentioned earlier, you will learn how to do qualitative data analysis manually , and also automatically using modern qualitative data and thematic analysis software .
To get best value from the analysis process and research process, it’s important to be super clear about the nature and scope of the question that’s being researched. This will help you select the research collection channels that are most likely to help you answer your question.
Depending on if you are a business looking to understand customer sentiment, or an academic surveying a school, your approach to qualitative data analysis will be unique.
Once you’re clear, there’s a sequence to follow. And, though there are differences in the manual and automatic approaches, the process steps are mostly the same.
The use case for our step-by-step guide is a company looking to collect data (customer feedback data), and analyze the customer feedback - in order to improve customer experience. By analyzing the customer feedback the company derives insights about their business and their customers. You can follow these same steps regardless of the nature of your research. Let’s get started.
Step 1: Gather your qualitative data
The first step of qualitative research is to do data collection. Put simply, data collection is gathering all of your data for analysis. A common situation is when qualitative data is spread across various sources.
Classic methods of gathering qualitative data
Most companies use traditional methods for gathering qualitative data: conducting interviews with research participants, running surveys, and running focus groups. This data is typically stored in documents, CRMs, databases and knowledge bases. It’s important to examine which data is available and needs to be included in your research project, based on its scope.
Using your existing qualitative feedback
As it becomes easier for customers to engage across a range of channels, companies are gathering even more solicited and unsolicited qualitative feedback.
Most organizations have now invested in Voice of Customer programs , support ticketing systems, chatbot and support conversations, emails and even customer Slack chats.
These new channels provide companies with new ways of getting feedback, and also allow the collection of unstructured feedback data at scale.
The great thing about this data is that it contains a wealth of valubale insights and that it’s already there! When you have a new question about user behavior or your customers, you don’t need to create a new research study or set up a focus group. You can find most answers in the data you already have.
Most commonly, qualitative data stored in third-party solutions. Some businesses pull all data into a central database, such as Snowflake, Amazon Redshift, BigQuery or Databricks. You can export this data manually for a one-off project, but if you need to conduct the analysis more regularly, try to find an automated solution. For example, Voice of Customer or feedback analysis solutions often provide integrations into third-party tools and databases. Alternatively, APIs can be used to gather feedback.
Utilize untapped qualitative data channels
There are many online qualitative data sources you may not have considered. For example, you can find useful qualitative data in social media channels like Twitter or Facebook. Online forums, review sites, and online communities such as Discourse or Reddit also contain valuable data about your customers, or research questions.
If you are considering performing a qualitative benchmark analysis against competitors - the internet is your best friend, and review analysis is a great place to start. Gathering feedback in competitor reviews on sites like Trustpilot, G2, Capterra, Better Business Bureau or on app stores is a great way to perform a competitor benchmark analysis.
Customer feedback analysis software often has integrations into social media and review sites, or you could scrape the reviews with a third-party tool.
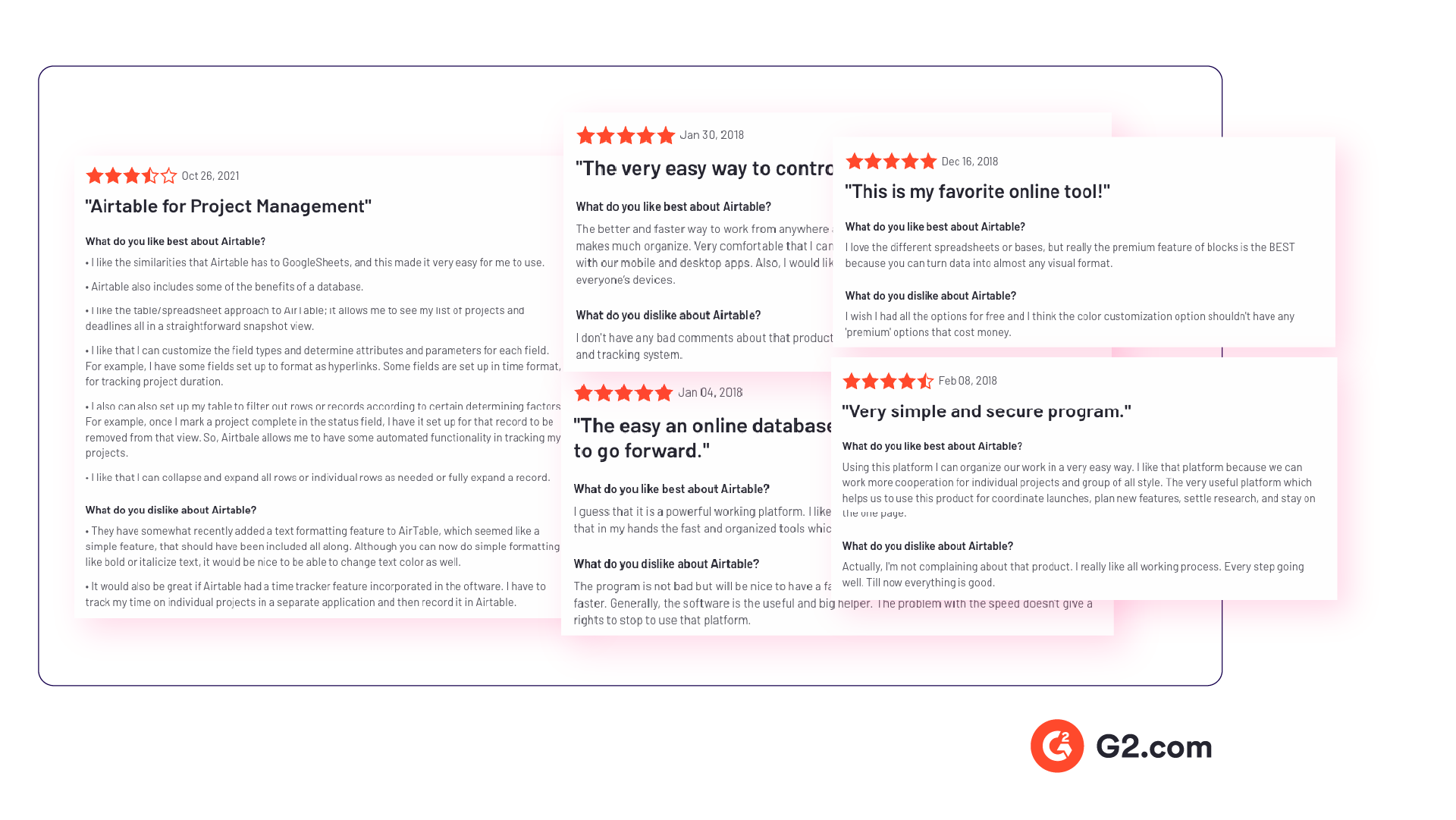
Step 2: Connect & organize all your qualitative data
Now you all have this qualitative data but there’s a problem, the data is unstructured. Before feedback can be analyzed and assigned any value, it needs to be organized in a single place. Why is this important? Consistency!
If all data is easily accessible in one place and analyzed in a consistent manner, you will have an easier time summarizing and making decisions based on this data.
The manual approach to organizing your data
The classic method of structuring qualitative data is to plot all the raw data you’ve gathered into a spreadsheet.
Typically, research and support teams would share large Excel sheets and different business units would make sense of the qualitative feedback data on their own. Each team collects and organizes the data in a way that best suits them, which means the feedback tends to be kept in separate silos.
An alternative and a more robust solution is to store feedback in a central database, like Snowflake or Amazon Redshift .
Keep in mind that when you organize your data in this way, you are often preparing it to be imported into another software. If you go the route of a database, you would need to use an API to push the feedback into a third-party software.
Computer-assisted qualitative data analysis software (CAQDAS)
Traditionally within the manual analysis approach (but not always), qualitative data is imported into CAQDAS software for coding.
In the early 2000s, researchers have been using CAQDAS software such as ATLAS.ti , NVivo and MAXQDA . Another popular option was IBM SPSS , which handled both quant and qual data.
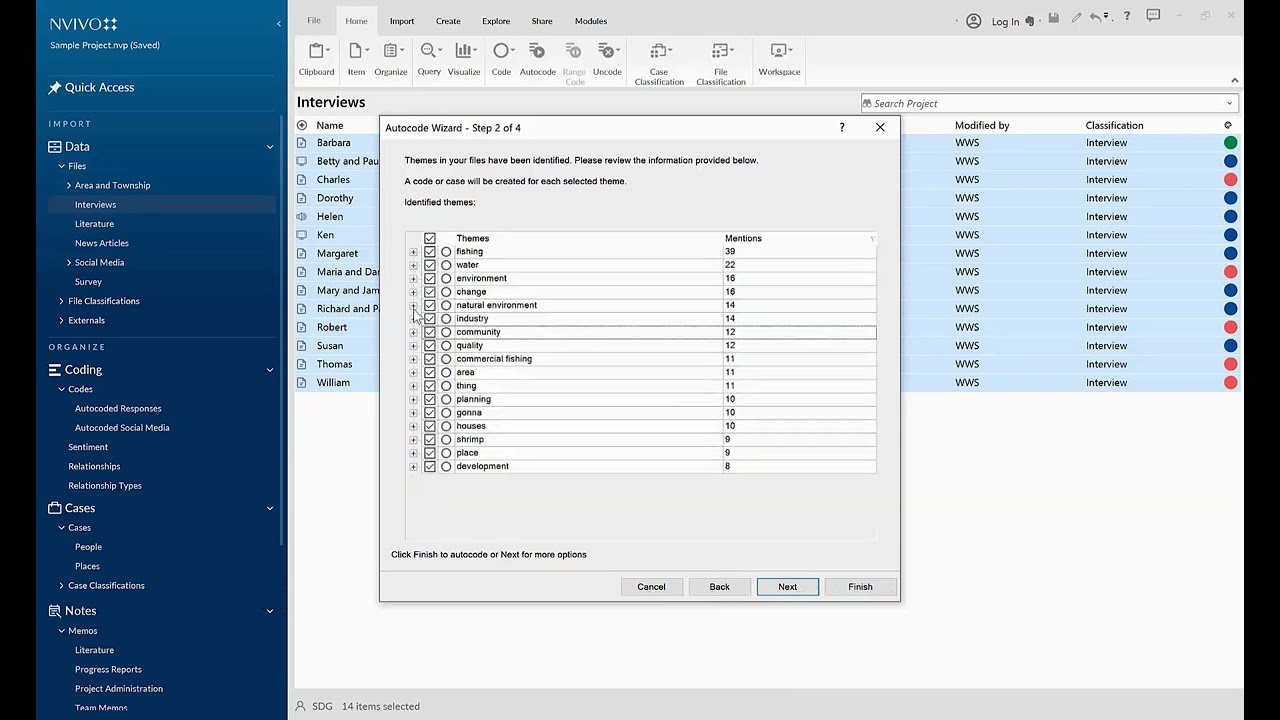
The benefits of using computer-assisted qualitative data analysis software:
- Assists in the organizing of your data
- Help view different interpretations of the data
- Allows you to share your data with others for collaboration
Most of these solutions now offer some degree of AI assistance. The main thing to look out for is the ease of use and the ability to bring in your input into AI analysis.
Organizing your qualitative data in a feedback repository
Another solution to organizing your qualitative data is to upload it into a feedback repository where it can be unified with your other data , and easily searchable and taggable. There are a number of software solutions that act as a central repository for your qualitative research data. Here are a couple solutions that you could investigate:
- Dovetail: Dovetail is a research repository with a focus on video and audio transcriptions. You can tag your transcriptions within the platform for theme analysis. You can also upload your other qualitative data such as research reports, survey responses, support conversations ( conversational analytics ), and customer interviews. Dovetail acts as a single, searchable repository. And makes it easier to collaborate with other people around your qualitative research.
- EnjoyHQ: EnjoyHQ is another research repository with similar functionality to Dovetail. It boasts a more sophisticated search engine, but it has a higher starting subscription cost.
Organizing your qualitative data in a feedback analytics platform
If you have a lot of qualitative customer or employee feedback, you will benefit from a feedback analytics platform. A feedback analytics platform is a software that automates the process of sentiment and thematic analysis , as well as the reporting of the results to the business. Typically, it's managed by a central Voice of Customer or research team to ensure consistent analysis methodology. But others in the company can login to get quick answers or reviews. These platforms can directly tap into qualitative data sources (review sites, social media, survey responses, etc) through one-click integrations or custom connectors. The data collected is then organized and analyzed consistently within the platform.
If you have data prepared in a spreadsheet, it can also be imported into feedback analytics platforms.
Once all this rich data has been organized within the feedback analytics platform, it is ready to be coded and themed, within the same platform. Thematic is a feedback analytics platform that offers one of the largest libraries of integrations with qualitative data sources.
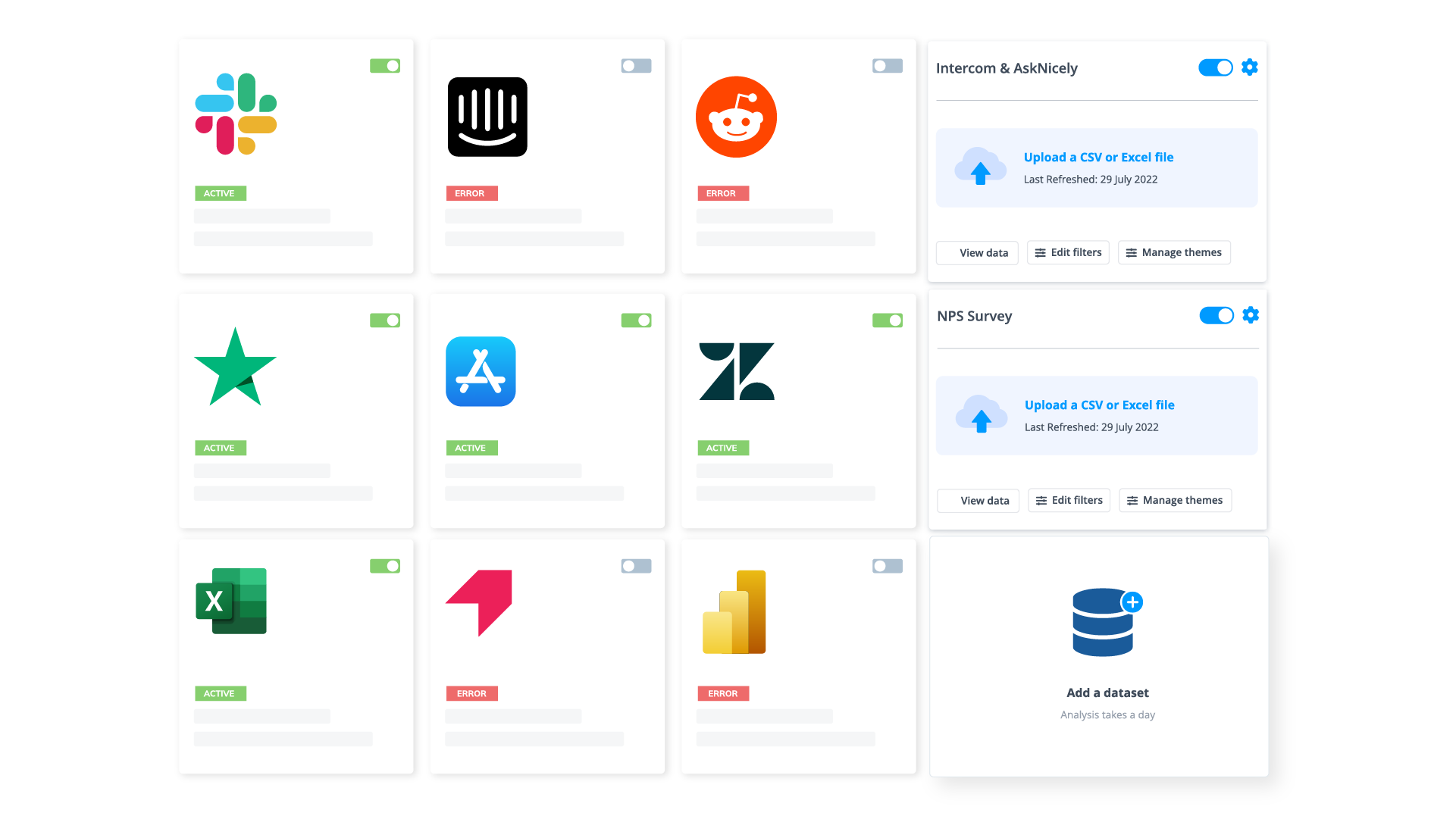
Step 3: Coding your qualitative data
Your feedback data is now organized in one place. Either within your spreadsheet, CAQDAS, feedback repository or within your feedback analytics platform. The next step is to code this data to extract meaningful insights.
Coding is the process of labelling and organizing your data by theme, i.e. to perform thematic analysis on this data. The main goal of coding is to find trends in the data and relationships between the themes.
When coding manually, start by taking small samples of your customer feedback data, come up with a set of codes, or categories capturing themes, and label each piece of feedback, systematically, for patterns and meaning. Then you will take a larger sample of data, revising and refining the codes for greater accuracy and consistency as you go.
If you use a tool like ChatGPT, you can automate the process of coming up with codes. But if your entire dataset does not fit into a context window, you'll need to manually batch analyze the remainder of the data, adjusting the prompts as you go. Make sure to read our guide on how to analyze feedback using ChatGPT .
If you choose to use a feedback analytics platform, much of this process will be automated for you.
The terms to describe different categories of meaning (‘theme’, ‘code’, ‘tag’, ‘category’ etc) can be confusing as they are often used interchangeably. For clarity, this article will use the term ‘code’.
To code means to identify key words or phrases and assign them to a category of meaning. In a sentence such as “I really hate the customer service of this computer software company”, the phrase "hate the customer service" would be coded as “poor customer service”.
How to manually code your qualitative data
- Decide whether you will use deductive or inductive coding. Deductive coding is when you create a list of predefined codes, and then assign them to the qualitative data. Inductive coding is the opposite of this, you create codes based on the data itself. Codes arise directly from the data and you label them as you go. You need to weigh up the pros and cons of each coding method and select the most appropriate.
- Read through the feedback data to get a broad sense of what it reveals. Now it’s time to start assigning your first set of codes to statements and sections of text.
- Keep repeating step 2, adding new codes and revising the code description as often as necessary. Once it has all been coded, go through everything again, to be sure there are no inconsistencies and that nothing has been overlooked.
- Create a code frame to group your codes. The coding frame is the organizational structure of all your codes. And there are two commonly used types of coding frames, flat, or hierarchical. A hierarchical code frame will make it easier for you to derive insights from your analysis.
- Based on the number of times a particular code occurs, you can now see the common themes in your feedback data. This is insightful! If ‘bad customer service’ is a common code, it’s time to take action.
We have a detailed guide dedicated to manually coding your qualitative data .
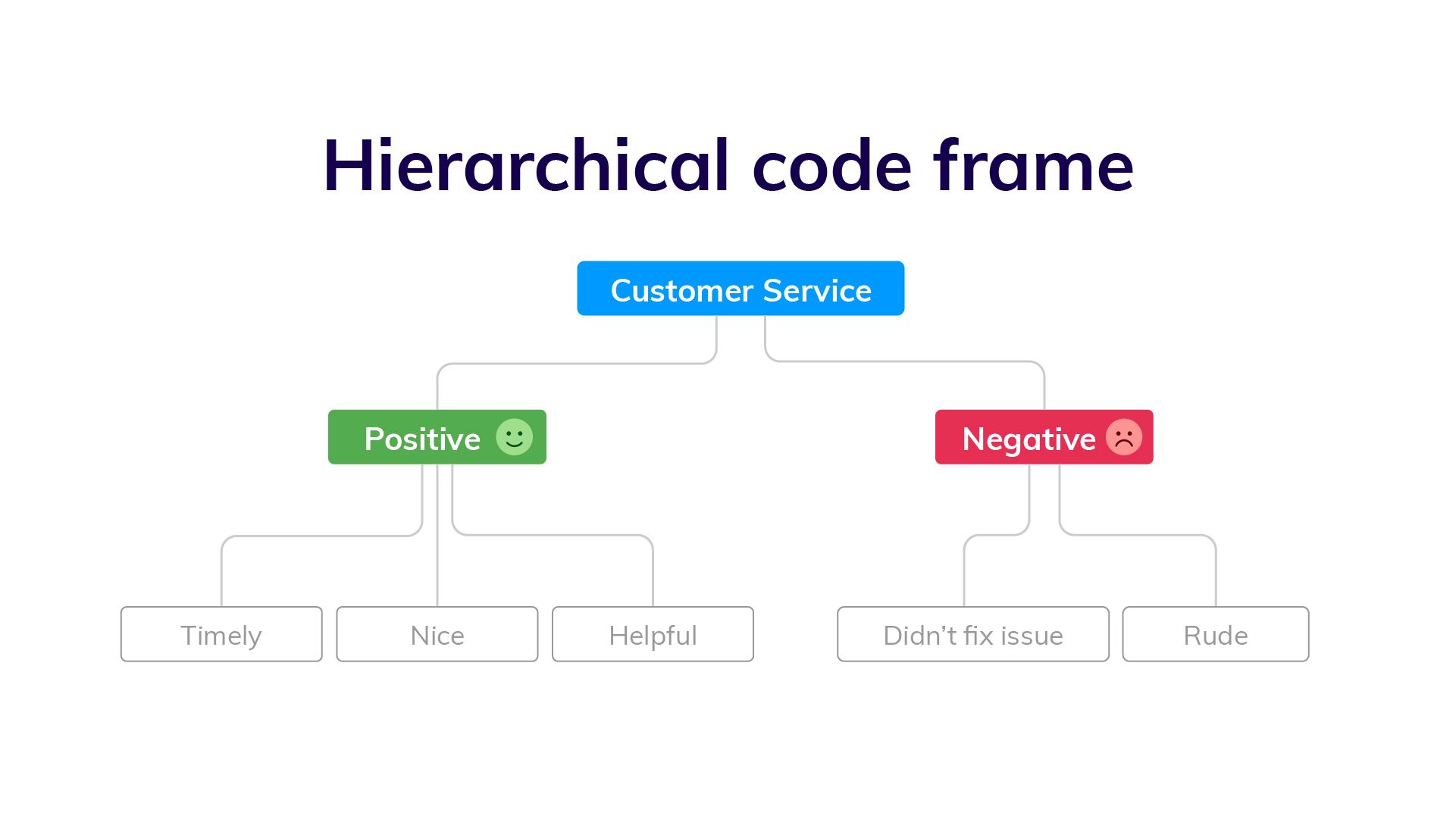
Using software to speed up manual coding of qualitative data
An Excel spreadsheet is still a popular method for coding. But various software solutions can help speed up this process. Here are some examples.
- CAQDAS / NVivo - CAQDAS software has built-in functionality that allows you to code text within their software. You may find the interface the software offers easier for managing codes than a spreadsheet.
- Dovetail/EnjoyHQ - You can tag transcripts and other textual data within these solutions. As they are also repositories you may find it simpler to keep the coding in one platform.
- IBM SPSS - SPSS is a statistical analysis software that may make coding easier than in a spreadsheet.
- Ascribe - Ascribe’s ‘Coder’ is a coding management system. Its user interface will make it easier for you to manage your codes .
Most of these solutions have now introduced AI-assistance. But they weren't build with the idea of automated coding from the ground up, like thematic analysis software described in next section.
Automating the qualitative coding process using thematic analysis software
Advances in AI have now made it possible to read, code and structure qualitative data automatically. This type of automated coding is offered by thematic analysis software , designed specifically for this task. Learn more: How to use Thematic Analysis AI to theme qualitative data.
Automation makes it far simpler and faster to code the feedback and group it into themes. The AI can be used in various ways:
- looks across sentences and phrases to identify meaningful statements worth coding
- analyze a sample of the data and decide on top-level categories or themes based on the implied context of the research
- be guided by the user about what they'd like to discover in the data
- create on the fly a taxonomy of themes
- identify sentiment and synthesize other scores from the feedback
- let you ask any question about feedback, e.g. what did customers say about our new trolleys?
And much more! Some automated solutions detect repeating patterns and assign codes to them, others make you train the AI by providing examples. You could say that the AI learns the meaning of the feedback on its own.
Thematic automates the coding of qualitative feedback with no training or pre-configuring required. There’s no need to set up themes or categories in advance. Simply upload your data and wait a few minutes. You can also manually edit the codes to further refine their accuracy. Experiments conducted indicate that Thematic’s automated coding is just as accurate as manual coding .
Paired with sentiment analysis and advanced text analytics - these automated solutions become powerful for deriving quality business or research insights.
The key benefits of using an automated coding solution
Automated analysis can often be set up fast and there’s the potential to uncover things that would never have been revealed if you had given the software a prescribed list of themes to look for.
Because the model applies a consistent rule to the data, it captures phrases or statements that a human eye might have missed.
Complete and consistent analysis of customer feedback enables more meaningful findings. Leading us into step 4.
Step 4: Analyze your data: Find meaningful insights
Now we are going to analyze our data to find insights. This is where we start to answer our research questions. Keep in mind that step 4 and step 5 (tell the story) have some overlap . This is because creating visualizations is both part of analysis process and reporting.
The task of uncovering insights is to scour through the codes that emerge from the data and draw meaningful correlations from them. It is also about making sure each insight is distinct and has enough data to support it.
Part of the analysis is to establish how much each code relates to different demographics and customer profiles, and identify whether there’s any relationship between these data points.
Manually create sub-codes to improve the quality of insights
If your code frame only has one level, you may find that your codes are too broad to be able to extract meaningful insights. This is where it is valuable to create sub-codes to your primary codes. This process is sometimes referred to as meta coding.
Note: If you take an inductive coding approach, you can create sub-codes as you are reading through your feedback data and coding it.
While time-consuming, this exercise will improve the quality of your analysis. Here is an example of what sub-codes could look like.
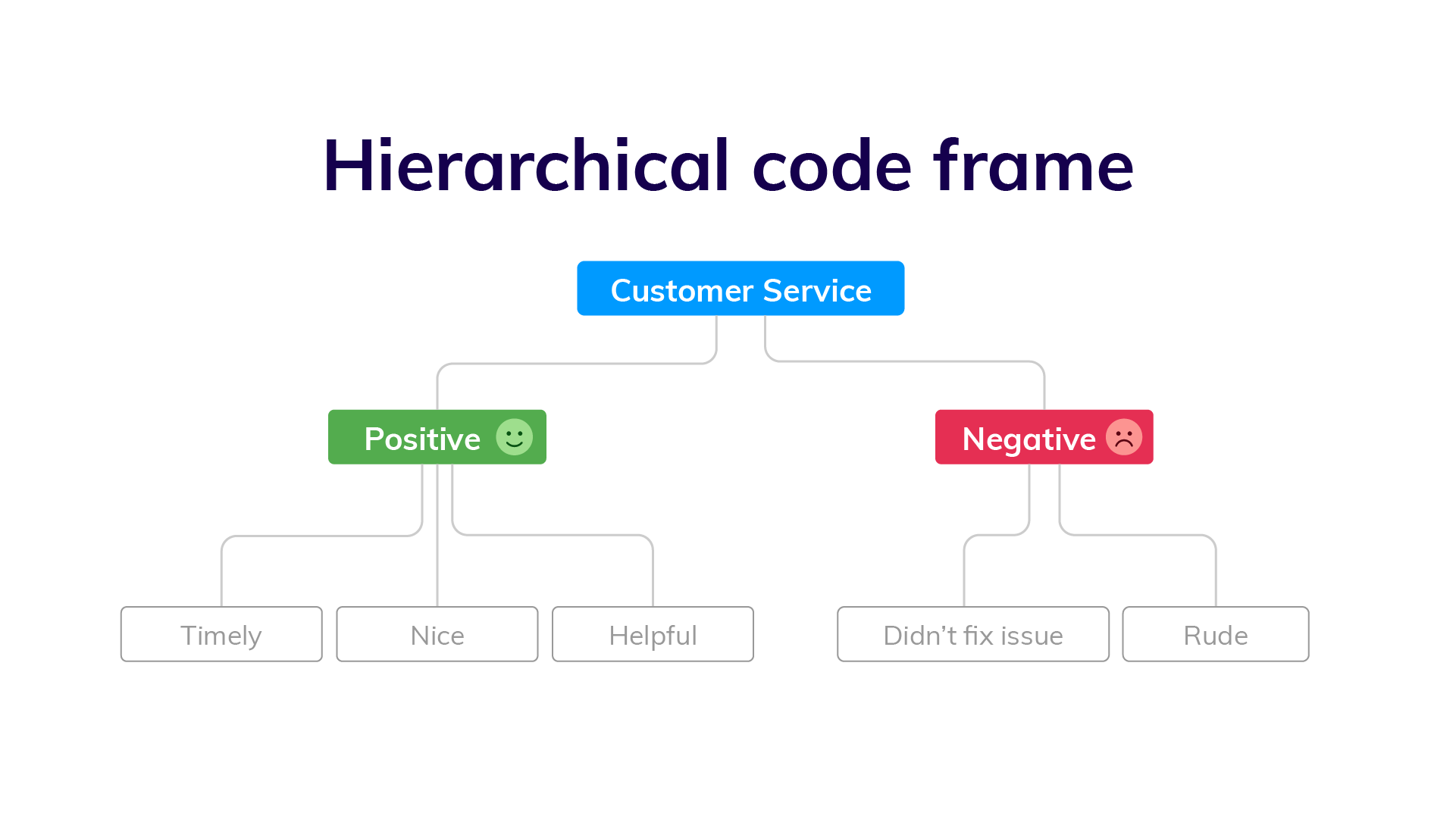
You need to carefully read your qualitative data to create quality sub-codes. But as you can see, the depth of analysis is greatly improved. By calculating the frequency of these sub-codes you can get insight into which customer service problems you can immediately address.
Correlate the frequency of codes to customer segments
Many businesses use customer segmentation . And you may have your own respondent segments that you can apply to your qualitative analysis. Segmentation is the practise of dividing customers or research respondents into subgroups.
Segments can be based on:
- Demographic
- And any other data type that you care to segment by
It is particularly useful to see the occurrence of codes within your segments. If one of your customer segments is considered unimportant to your business, but they are the cause of nearly all customer service complaints, it may be in your best interest to focus attention elsewhere. This is a useful insight!
Visualizing coded qualitative data
The most common way of visualizing coded data is by frequency. Here's an example of how we do it in Thematic, which can be replicated in PowerBI, Tableau or Looker.
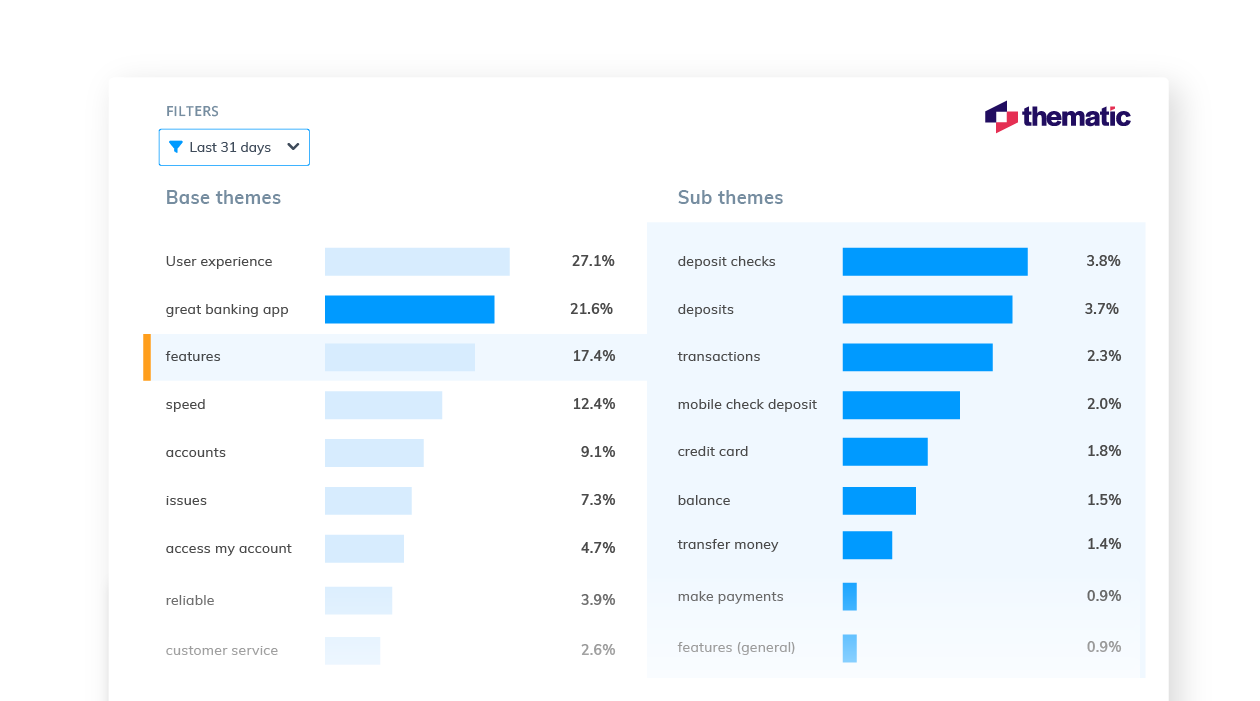
But frequency is not always a good gauge of importance. For example, if some people are happy with "deposit checks" feature and others unhappy, what's the overall importance of this theme in feedback? Should we prioritize working on it? This is where a driver analysis, aka impact, becomes important.
If you are collecting a metric alongside your qualitative data this is a key visualization. Impact answers the question: “What’s the impact of a code on my overall score?”. Using Net Promoter Score (NPS) as an example, first you need to:
- Calculate overall NPS
- Calculate NPS in the subset of responses that do not contain that theme
- Subtract B from A
Then you can use this simple formula to calculate code impact on NPS .
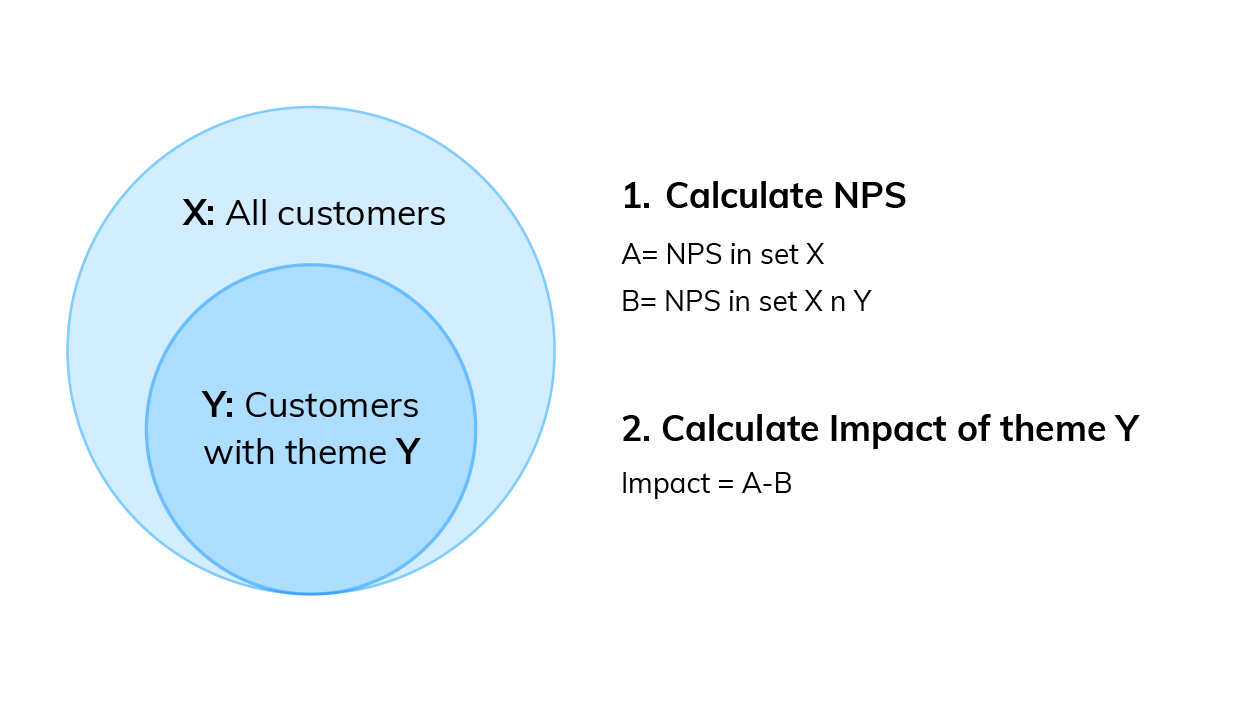
You can then visualize this data using a bar chart. It will tell you which themes are dragging the score up or down, and you can even view this over time. If this sounds interesting, check out the demo videos showing how we do it in Thematic .
You can also download our CX toolkit - it includes a template to recreate this.
Trends over time
This analysis can help you answer questions like: “Which codes are linked to decreases or increases in my score over time?”
We need to compare two sequences of numbers: NPS over time and code frequency over time . Using Excel, calculate the correlation between the two sequences, which can be either positive (the more codes the higher the NPS, see picture below), or negative (the more codes the lower the NPS).
Now you need to plot code frequency against the absolute value of code correlation with NPS. Here is the formula:
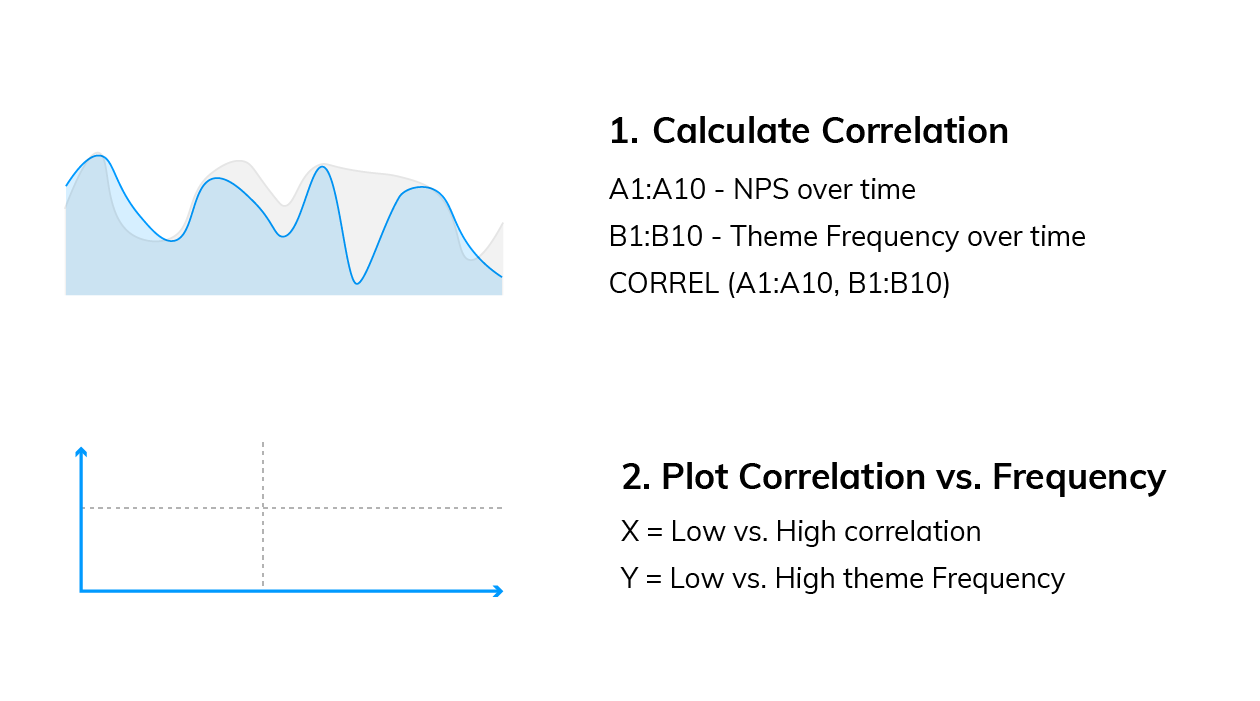
The visualization could look like this:
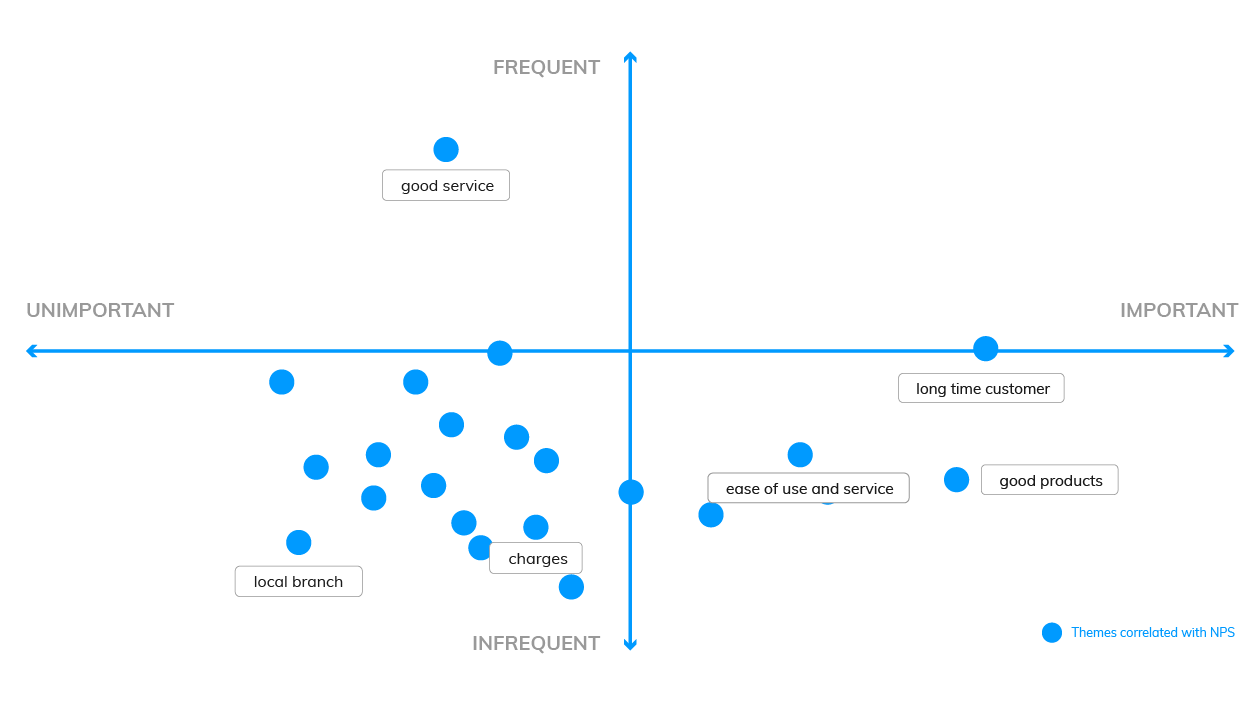
These are two examples, but there are more. For a third manual formula, and to learn why word clouds are not an insightful form of analysis, read our visualizations article .
Using a text analytics solution to automate analysis
Automated text analytics solutions enable codes and sub-codes to be pulled out of the data automatically. This makes it far faster and easier to identify what’s driving negative or positive results. And to pick up emerging trends and find all manner of rich insights in the data.
Another benefit of AI-driven text analytics software is its built-in capability for sentiment analysis, which provides the emotive context behind your feedback and other qualitative textual data therein.
Thematic provides text analytics that goes further by allowing users to apply their expertise on business context to edit or augment the AI-generated outputs.
Since the move away from manual research is generally about reducing the human element, adding human input to the technology might sound counter-intuitive. However, there are 3 main reasons why it's important:
1) To bring in the business nuance that AI cannot learn from the data itself. For example, are there specific teams responsible for acting on feedback? It's worth organizing themes so that each team can easily see what they can impact.
2) To iron out any erros in the analysis. Even the best AI will still be wrong occasionally.
3) To build trust in the analysis. In Thematic, we show why AI has chosen each theme, so that you can verify its approach.
The result is a higher accuracy of analysis. This is sometimes referred to as augmented intelligence or human in the loop.
Step 5: Report on your data: Tell the story
The last step of analyzing your qualitative data is to report on it, to tell the story. At this point, the codes are fully developed and the focus is on communicating the narrative to the audience.
A coherent outline of the qualitative research, the findings and the insights is vital for stakeholders to discuss and debate before they can devise a meaningful course of action.
Creating graphs and reporting in Powerpoint
Typically, qualitative researchers take the tried and tested approach of distilling their report into a series of charts, tables and other visuals which are woven into a narrative for presentation in Powerpoint.
Using visualization software for reporting
With data transformation and APIs, the analyzed data can be shared with data visualisation software, such as Power BI or Tableau , Google Studio or Looker. Power BI and Tableau are among the most preferred options.
Visualizing your insights in a feedback analytics platform
Feedback analytics platforms, like Thematic, incorporate visualisation tools that intuitively turn key data and insights into graphs. This removes the time consuming work of constructing charts to visually identify patterns and creates more time to focus on building a compelling narrative that highlights the insights, in bite-size chunks, for executive teams to review.
Using a feedback analytics platform with visualization tools means you don’t have to use a separate product for visualizations. You can export graphs into Powerpoints straight from the platforms.
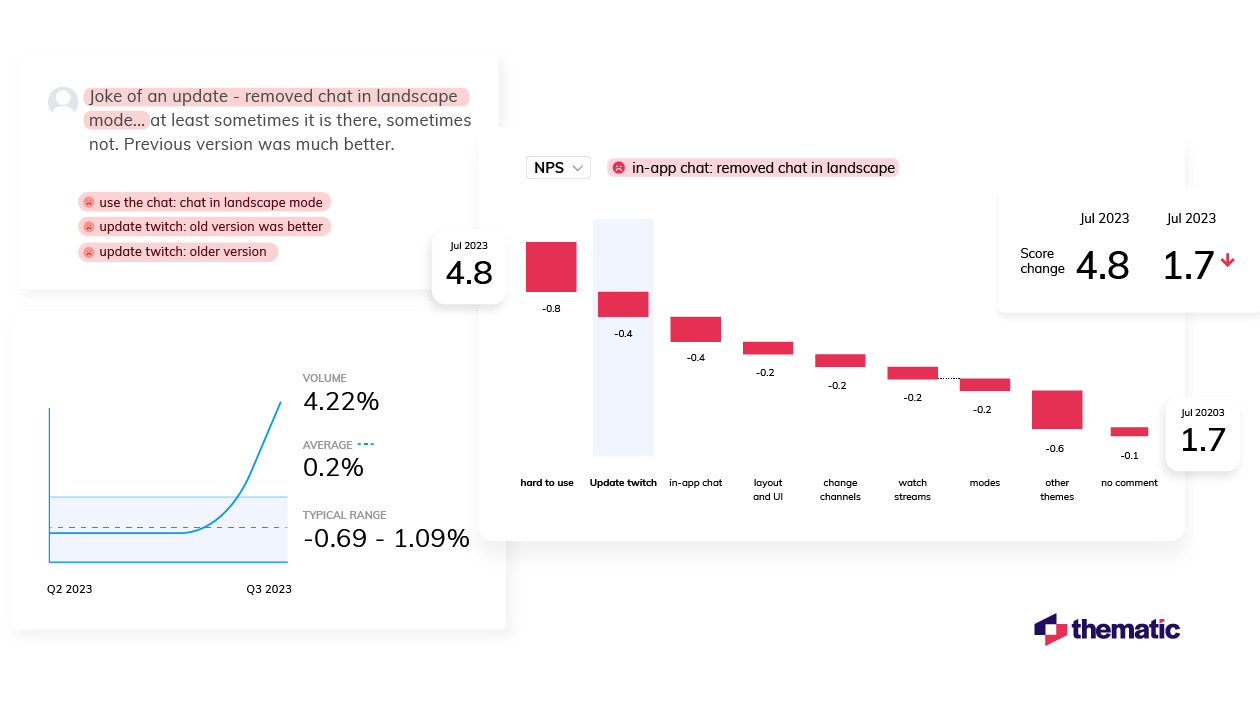
Conclusion: Seek automation with human oversight
AI technology is here to stay, and it's powerful enough to automate most of qualitative data analysis. So, as a researcher, you need to learn not just the basics of how to do this task manually, but also how to harness AI to complete this task quicker.
For projects that involve small datasets or one-offs, use ChatGPT or a similar solution. For example, if the objective is simply to quantify a simple question like “Do customers prefer X concepts to Y?”. And if the findings are being extracted from a small set of focus groups and interviews, sometimes it’s easier to just read them.
However, as new generations come into the workplace, it’s technology-driven solutions that feel more comfortable and practical. Especially, once you have huge volumes of data and you need a deeper understanding of the data. For example, the ‘why’ behind customers’ preference for X or Y. Being able to do this fast to help your business move quickly is critical.
The ability to collect a free flow of qualitative feedback data and customer metrics means AI can cost-effectively scan, crunch, score and analyze a ton of feedback from one system in one go. And time-intensive processes like focus groups, or coding, that used to take weeks, can now be completed in a matter of hours or days.
But aside from the ever-present business case to speed things up and keep costs down, there are also powerful research imperatives for automated analysis of qualitative data: namely, accuracy and consistency.
Finding insights hidden in feedback requires consistency, especially in coding. Not to mention catching all the ‘unknown unknowns’ that can skew research findings and steering clear of cognitive bias.
Some say without manual data analysis researchers won’t get an accurate “feel” for the insights. However, the larger data sets are, the harder it is to sort through the feedback and organize feedback that has been pulled from different places. And, the more difficult it is to stay on course, the greater the risk of drawing incorrect, or incomplete, conclusions grows.
Though the process steps for qualitative data analysis have remained pretty much unchanged since psychologist Paul Felix Lazarsfeld paved the path a hundred years ago, the impact digital technology has had on types of qualitative feedback data and the approach to the analysis are profound.
If you want to try an automated feedback analysis solution on your own qualitative data, you can get started with Thematic .
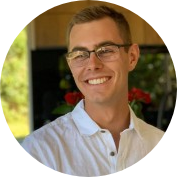
Community & Marketing
Tyler manages our community of CX, insights & analytics professionals. Tyler's goal is to help unite insights professionals around common challenges.
We make it easy to discover the customer and product issues that matter.
Unlock the value of feedback at scale, in one platform. Try it for free now!
- Questions to ask your Feedback Analytics vendor
- How to end customer churn for good
- Scalable analysis of NPS verbatims
- 5 Text analytics approaches
- How to calculate the ROI of CX
Our experts will show you how Thematic works, how to discover pain points and track the ROI of decisions. To access your free trial, book a personal demo today.
Recent posts
With AI-driven thematic analysis software, you can generate actionable insights effortlessly. Here’s what you need to know about this method of data analysis tool.
Become a qualitative theming pro! Creating a perfect code frame is hard, but thematic analysis software makes the process much easier.
Discover the power of thematic analysis to unlock insights from qualitative data. Learn about manual vs. AI-powered approaches, best practices, and how Thematic software can revolutionize your analysis workflow.
- PRO Courses Guides New Tech Help Pro Expert Videos About wikiHow Pro Upgrade Sign In
- EDIT Edit this Article
- EXPLORE Tech Help Pro About Us Random Article Quizzes Request a New Article Community Dashboard This Or That Game Forums Popular Categories Arts and Entertainment Artwork Books Movies Computers and Electronics Computers Phone Skills Technology Hacks Health Men's Health Mental Health Women's Health Relationships Dating Love Relationship Issues Hobbies and Crafts Crafts Drawing Games Education & Communication Communication Skills Personal Development Studying Personal Care and Style Fashion Hair Care Personal Hygiene Youth Personal Care School Stuff Dating All Categories Arts and Entertainment Finance and Business Home and Garden Relationship Quizzes Cars & Other Vehicles Food and Entertaining Personal Care and Style Sports and Fitness Computers and Electronics Health Pets and Animals Travel Education & Communication Hobbies and Crafts Philosophy and Religion Work World Family Life Holidays and Traditions Relationships Youth
- Browse Articles
- Learn Something New
- Quizzes Hot
- Happiness Hub
- This Or That Game
- Train Your Brain
- Explore More
- Support wikiHow
- About wikiHow
- Log in / Sign up
- Education and Communications
How to Analyse a Case Study
Last Updated: October 8, 2024 Approved
This article was co-authored by Sarah Evans . Sarah Evans is a Public Relations & Social Media Expert based in Las Vegas, Nevada. With over 14 years of industry experience, Sarah is the Founder & CEO of Sevans PR. Her team offers strategic communications services to help clients across industries including tech, finance, medical, real estate, law, and startups. The agency is renowned for its development of the "reputation+" methodology, a data-driven and AI-powered approach designed to elevate brand credibility, trust, awareness, and authority in a competitive marketplace. Sarah’s thought leadership has led to regular appearances on The Doctors TV show, CBS Las Vegas Now, and as an Adobe influencer. She is a respected contributor at Entrepreneur magazine, Hackernoon, Grit Daily, and KLAS Las Vegas. Sarah has been featured in PR Daily and PR Newswire and is a member of the Forbes Agency Council. She received her B.A. in Communications and Public Relations from Millikin University. wikiHow marks an article as reader-approved once it receives enough positive feedback. This article received 18 testimonials and 88% of readers who voted found it helpful, earning it our reader-approved status. This article has been viewed 415,511 times.
Case studies are used in many professional education programs, primarily in business school, to present real-world situations to students and to assess their ability to parse out the important aspects of a given dilemma. In general, a case study should include, in order: background on the business environment, description of the given business, identification of a key problem or issue, steps taken to address the issue, your assessment of that response, and suggestions for better business strategy. The steps below will guide you through the process of analyzing a business case study in this way.
Examine and describe the business environment relevant to the case study.

Describe the structure and size of the main business under consideration.

Identify the key issue or problem in the case study.

Describe how the business responds to these issues or problems.

===Identify the successful aspects of this response as well as its failures.=== #Indicate whether or not each aspect of the response met its goal. Talk about whether the response overall was well-crafted. Use numerical benchmarks, like a desired customer share, to show whether goals were met; analyze broader issues, like employee management policies, to talk about the response as a whole. [3] X Research source

Point to successes, failures, unforeseen results, and inadequate measures.
- Suggest alternative or improved measures that could have been taken. Use specific examples and backing up your suggestions with data and calculations.

Describe what changes you would make in the business.

Conclude your analysis by reviewing your findings.

Community Q&A

- Always read a case study several times. At first, you should read just for the basic details. On each subsequent reading, look for details about a specific topic: competitors, business strategy, management structure, financial loss. Highlight phrases and sections relating to these topics and take notes. Thanks Helpful 0 Not Helpful 0
- In the preliminary stages of analyzing a case study, no detail is insignificant. The biggest numbers can often be misleading, and the point of an analysis is often to dig deeper and find otherwise unnoticed variables that drive a situation. Thanks Helpful 0 Not Helpful 0
- If you are analyzing a case study for a consulting company interview, be sure to direct your comments towards the matters handled by the company. For example, if the company deals with marketing strategy, focus on the business's successes and failures in marketing; if you are interviewing for a financial consulting job, analyze how well the business keeps their books and their investment strategy. Thanks Helpful 0 Not Helpful 0

- Do not use impassioned or emphatic language in your analysis. Business case studies are a tool for gauging your business acumen, not your personal beliefs. When assigning blame or identifying flaws in strategy, use a detached, disinterested tone. Thanks Helpful 16 Not Helpful 4
Things You'll Need
You might also like.

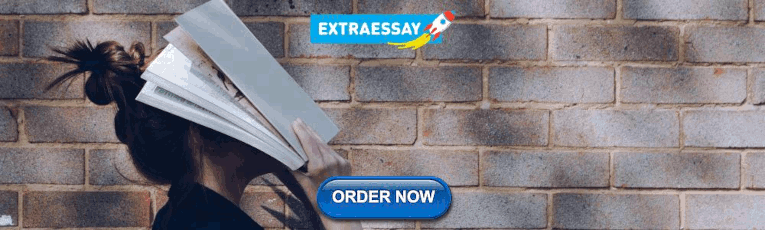
Expert Interview

Thanks for reading our article! If you’d like to learn more about business writing, check out our in-depth interview with Sarah Evans .
- ↑ https://www.gvsu.edu/cms4/asset/CC3BFEEB-C364-E1A1-A5390F221AC0FD2D/business_case_analysis_gg_final.pdf
- ↑ https://bizfluent.com/12741914/how-to-analyze-a-business-case-study
- ↑ http://www.business-fundas.com/2009/how-to-analyze-business-case-studies/
- ↑ https://writingcenter.uagc.edu/writing-case-study-analysis
- http://college.cengage.com/business/resources/casestudies/students/analyzing.htm
About This Article

- Send fan mail to authors
Reader Success Stories

Lisa Upshur
Jun 15, 2019
Did this article help you?

Tejiri Aren
Jul 21, 2016

Jul 15, 2017

Jenn M.T. Tseka
Jul 3, 2016

Devanand Sbuurayan
Dec 6, 2020

Featured Articles

Trending Articles

Watch Articles

- Terms of Use
- Privacy Policy
- Do Not Sell or Share My Info
- Not Selling Info
wikiHow Tech Help Pro:
Develop the tech skills you need for work and life
Qualitative case study data analysis: an example from practice
Affiliation.
- 1 School of Nursing and Midwifery, National University of Ireland, Galway, Republic of Ireland.
- PMID: 25976531
- DOI: 10.7748/nr.22.5.8.e1307
Aim: To illustrate an approach to data analysis in qualitative case study methodology.
Background: There is often little detail in case study research about how data were analysed. However, it is important that comprehensive analysis procedures are used because there are often large sets of data from multiple sources of evidence. Furthermore, the ability to describe in detail how the analysis was conducted ensures rigour in reporting qualitative research.
Data sources: The research example used is a multiple case study that explored the role of the clinical skills laboratory in preparing students for the real world of practice. Data analysis was conducted using a framework guided by the four stages of analysis outlined by Morse ( 1994 ): comprehending, synthesising, theorising and recontextualising. The specific strategies for analysis in these stages centred on the work of Miles and Huberman ( 1994 ), which has been successfully used in case study research. The data were managed using NVivo software.
Review methods: Literature examining qualitative data analysis was reviewed and strategies illustrated by the case study example provided. Discussion Each stage of the analysis framework is described with illustration from the research example for the purpose of highlighting the benefits of a systematic approach to handling large data sets from multiple sources.
Conclusion: By providing an example of how each stage of the analysis was conducted, it is hoped that researchers will be able to consider the benefits of such an approach to their own case study analysis.
Implications for research/practice: This paper illustrates specific strategies that can be employed when conducting data analysis in case study research and other qualitative research designs.
Keywords: Case study data analysis; case study research methodology; clinical skills research; qualitative case study methodology; qualitative data analysis; qualitative research.
- Case-Control Studies*
- Data Interpretation, Statistical*
- Nursing Research / methods*
- Qualitative Research*
- Research Design

Qualitative Data Analysis Methods
The “Big 6” Qualitative Methods + Examples
By: Kerryn Warren (PhD) | Reviewed By: Eunice Rautenbach (D.Tech) | May 2020 (Updated April 2023)
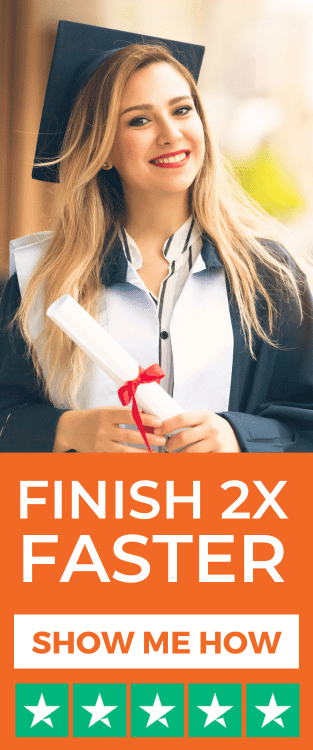
If you’re new to the world of research, qualitative data analysis can look rather intimidating. So much bulky terminology and so many abstract, fluffy concepts. It certainly can be a minefield!
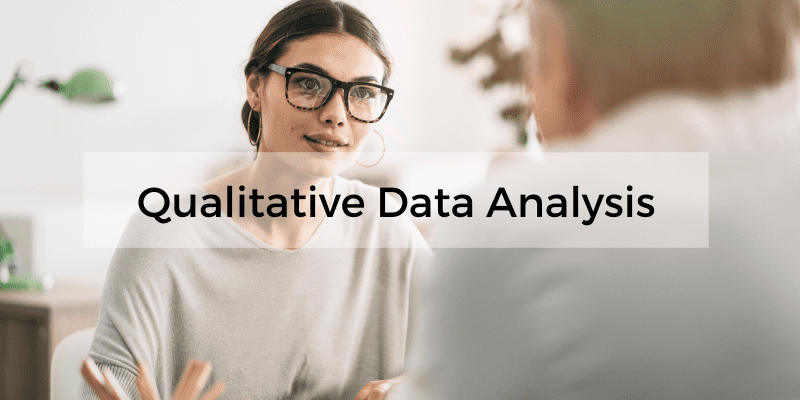
What (exactly) is qualitative data analysis?
To understand qualitative data analysis, we need to first understand qualitative data – so let’s step back and ask the question, “what exactly is qualitative data?”.
Qualitative data refers to pretty much any data that’s “not numbers” . In other words, it’s not the stuff you measure using a fixed scale or complex equipment, nor do you analyse it using complex statistics or mathematics.
So, if it’s not numbers, what is it?
Words, you guessed? Well… sometimes , yes. Qualitative data can, and often does, take the form of interview transcripts, documents and open-ended survey responses – but it can also involve the interpretation of images and videos. In other words, qualitative isn’t just limited to text-based data.
So, how’s that different from quantitative data, you ask?
Simply put, qualitative research focuses on words, descriptions, concepts or ideas – while quantitative research focuses on numbers and statistics . Qualitative research investigates the “softer side” of things to explore and describe , while quantitative research focuses on the “hard numbers”, to measure differences between variables and the relationships between them. If you’re keen to learn more about the differences between qual and quant, we’ve got a detailed post over here .
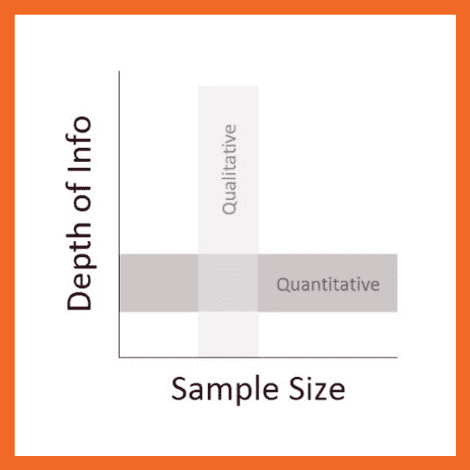
So, qualitative analysis is easier than quantitative, right?
Not quite. In many ways, qualitative data can be challenging and time-consuming to analyse and interpret. At the end of your data collection phase (which itself takes a lot of time), you’ll likely have many pages of text-based data or hours upon hours of audio to work through. You might also have subtle nuances of interactions or discussions that have danced around in your mind, or that you scribbled down in messy field notes. All of this needs to work its way into your analysis.
Making sense of all of this is no small task and you shouldn’t underestimate it. Long story short – qualitative analysis can be a lot of work! Of course, quantitative analysis is no piece of cake either, but it’s important to recognise that qualitative analysis still requires a significant investment in terms of time and effort.
Need a helping hand?
The “Big 6” Qualitative Analysis Methods
There are many different types of qualitative data analysis, all of which serve different purposes and have unique strengths and weaknesses . We’ll start by outlining the analysis methods and then we’ll dive into the details for each.
The 6 most popular methods (or at least the ones we see at Grad Coach) are:
- Content analysis
- Narrative analysis
- Discourse analysis
- Thematic analysis
- Grounded theory (GT)
- Interpretive phenomenological analysis (IPA)
QDA Method #1: Qualitative Content Analysis
Content analysis is possibly the most common and straightforward QDA method. At the simplest level, content analysis is used to evaluate patterns within a piece of content (for example, words, phrases or images) or across multiple pieces of content or sources of communication. For example, a collection of newspaper articles or political speeches.
With content analysis, you could, for instance, identify the frequency with which an idea is shared or spoken about – like the number of times a Kardashian is mentioned on Twitter. Or you could identify patterns of deeper underlying interpretations – for instance, by identifying phrases or words in tourist pamphlets that highlight India as an ancient country.
Because content analysis can be used in such a wide variety of ways, it’s important to go into your analysis with a very specific question and goal, or you’ll get lost in the fog. With content analysis, you’ll group large amounts of text into codes , summarise these into categories, and possibly even tabulate the data to calculate the frequency of certain concepts or variables. Because of this, content analysis provides a small splash of quantitative thinking within a qualitative method.
Naturally, while content analysis is widely useful, it’s not without its drawbacks . One of the main issues with content analysis is that it can be very time-consuming , as it requires lots of reading and re-reading of the texts. Also, because of its multidimensional focus on both qualitative and quantitative aspects, it is sometimes accused of losing important nuances in communication.
Content analysis also tends to concentrate on a very specific timeline and doesn’t take into account what happened before or after that timeline. This isn’t necessarily a bad thing though – just something to be aware of. So, keep these factors in mind if you’re considering content analysis. Every analysis method has its limitations , so don’t be put off by these – just be aware of them ! If you’re interested in learning more about content analysis, the video below provides a good starting point.
QDA Method #2: Narrative Analysis
As the name suggests, narrative analysis is all about listening to people telling stories and analysing what that means . Since stories serve a functional purpose of helping us make sense of the world, we can gain insights into the ways that people deal with and make sense of reality by analysing their stories and the ways they’re told.
You could, for example, use narrative analysis to explore whether how something is being said is important. For instance, the narrative of a prisoner trying to justify their crime could provide insight into their view of the world and the justice system. Similarly, analysing the ways entrepreneurs talk about the struggles in their careers or cancer patients telling stories of hope could provide powerful insights into their mindsets and perspectives . Simply put, narrative analysis is about paying attention to the stories that people tell – and more importantly, the way they tell them.
Of course, the narrative approach has its weaknesses , too. Sample sizes are generally quite small due to the time-consuming process of capturing narratives. Because of this, along with the multitude of social and lifestyle factors which can influence a subject, narrative analysis can be quite difficult to reproduce in subsequent research. This means that it’s difficult to test the findings of some of this research.
Similarly, researcher bias can have a strong influence on the results here, so you need to be particularly careful about the potential biases you can bring into your analysis when using this method. Nevertheless, narrative analysis is still a very useful qualitative analysis method – just keep these limitations in mind and be careful not to draw broad conclusions . If you’re keen to learn more about narrative analysis, the video below provides a great introduction to this qualitative analysis method.
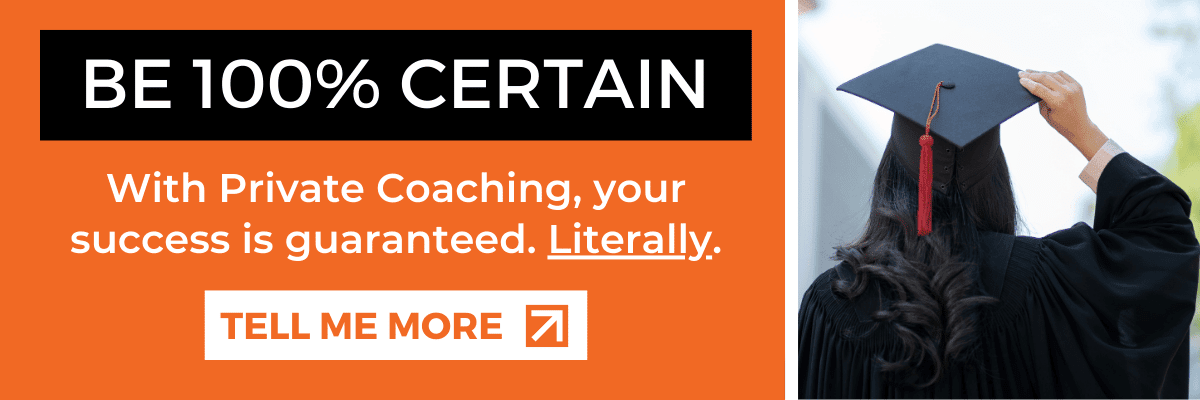
QDA Method #3: Discourse Analysis
Discourse is simply a fancy word for written or spoken language or debate . So, discourse analysis is all about analysing language within its social context. In other words, analysing language – such as a conversation, a speech, etc – within the culture and society it takes place. For example, you could analyse how a janitor speaks to a CEO, or how politicians speak about terrorism.
To truly understand these conversations or speeches, the culture and history of those involved in the communication are important factors to consider. For example, a janitor might speak more casually with a CEO in a company that emphasises equality among workers. Similarly, a politician might speak more about terrorism if there was a recent terrorist incident in the country.
So, as you can see, by using discourse analysis, you can identify how culture , history or power dynamics (to name a few) have an effect on the way concepts are spoken about. So, if your research aims and objectives involve understanding culture or power dynamics, discourse analysis can be a powerful method.
Because there are many social influences in terms of how we speak to each other, the potential use of discourse analysis is vast . Of course, this also means it’s important to have a very specific research question (or questions) in mind when analysing your data and looking for patterns and themes, or you might land up going down a winding rabbit hole.
Discourse analysis can also be very time-consuming as you need to sample the data to the point of saturation – in other words, until no new information and insights emerge. But this is, of course, part of what makes discourse analysis such a powerful technique. So, keep these factors in mind when considering this QDA method. Again, if you’re keen to learn more, the video below presents a good starting point.
QDA Method #4: Thematic Analysis
Thematic analysis looks at patterns of meaning in a data set – for example, a set of interviews or focus group transcripts. But what exactly does that… mean? Well, a thematic analysis takes bodies of data (which are often quite large) and groups them according to similarities – in other words, themes . These themes help us make sense of the content and derive meaning from it.
Let’s take a look at an example.
With thematic analysis, you could analyse 100 online reviews of a popular sushi restaurant to find out what patrons think about the place. By reviewing the data, you would then identify the themes that crop up repeatedly within the data – for example, “fresh ingredients” or “friendly wait staff”.
So, as you can see, thematic analysis can be pretty useful for finding out about people’s experiences , views, and opinions . Therefore, if your research aims and objectives involve understanding people’s experience or view of something, thematic analysis can be a great choice.
Since thematic analysis is a bit of an exploratory process, it’s not unusual for your research questions to develop , or even change as you progress through the analysis. While this is somewhat natural in exploratory research, it can also be seen as a disadvantage as it means that data needs to be re-reviewed each time a research question is adjusted. In other words, thematic analysis can be quite time-consuming – but for a good reason. So, keep this in mind if you choose to use thematic analysis for your project and budget extra time for unexpected adjustments.
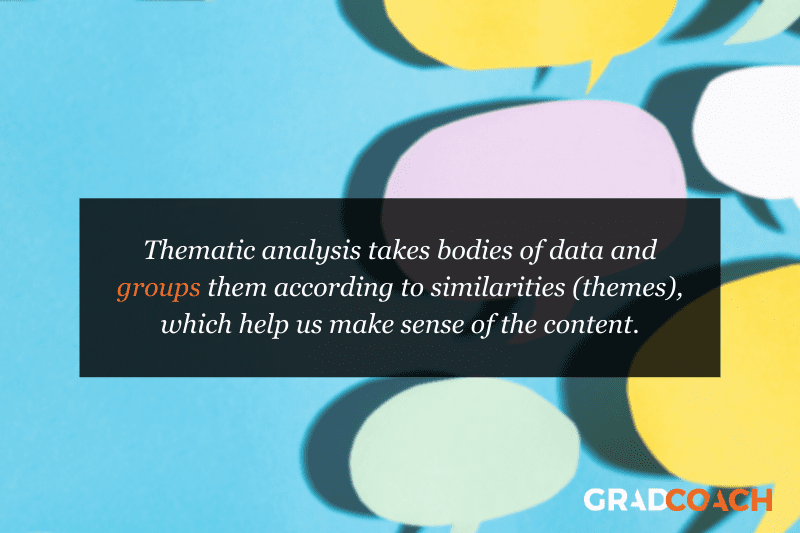
QDA Method #5: Grounded theory (GT)
Grounded theory is a powerful qualitative analysis method where the intention is to create a new theory (or theories) using the data at hand, through a series of “ tests ” and “ revisions ”. Strictly speaking, GT is more a research design type than an analysis method, but we’ve included it here as it’s often referred to as a method.
What’s most important with grounded theory is that you go into the analysis with an open mind and let the data speak for itself – rather than dragging existing hypotheses or theories into your analysis. In other words, your analysis must develop from the ground up (hence the name).
Let’s look at an example of GT in action.
Assume you’re interested in developing a theory about what factors influence students to watch a YouTube video about qualitative analysis. Using Grounded theory , you’d start with this general overarching question about the given population (i.e., graduate students). First, you’d approach a small sample – for example, five graduate students in a department at a university. Ideally, this sample would be reasonably representative of the broader population. You’d interview these students to identify what factors lead them to watch the video.
After analysing the interview data, a general pattern could emerge. For example, you might notice that graduate students are more likely to read a post about qualitative methods if they are just starting on their dissertation journey, or if they have an upcoming test about research methods.
From here, you’ll look for another small sample – for example, five more graduate students in a different department – and see whether this pattern holds true for them. If not, you’ll look for commonalities and adapt your theory accordingly. As this process continues, the theory would develop . As we mentioned earlier, what’s important with grounded theory is that the theory develops from the data – not from some preconceived idea.
So, what are the drawbacks of grounded theory? Well, some argue that there’s a tricky circularity to grounded theory. For it to work, in principle, you should know as little as possible regarding the research question and population, so that you reduce the bias in your interpretation. However, in many circumstances, it’s also thought to be unwise to approach a research question without knowledge of the current literature . In other words, it’s a bit of a “chicken or the egg” situation.
Regardless, grounded theory remains a popular (and powerful) option. Naturally, it’s a very useful method when you’re researching a topic that is completely new or has very little existing research about it, as it allows you to start from scratch and work your way from the ground up .
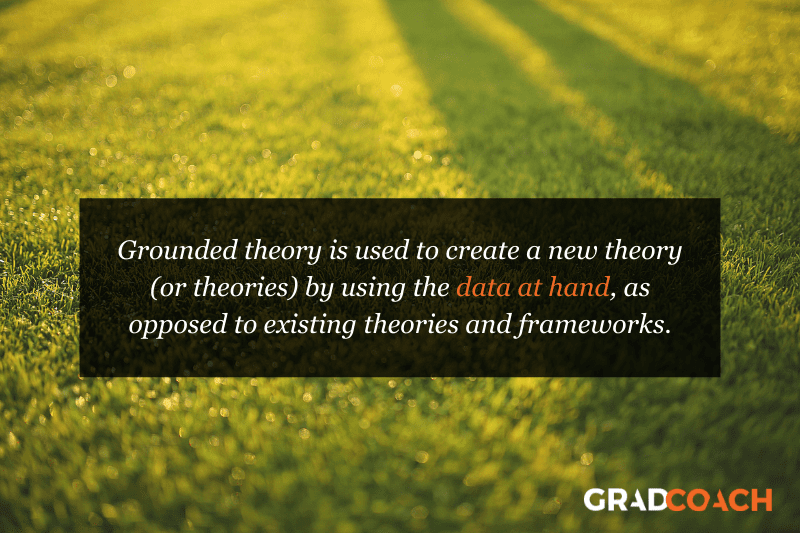
QDA Method #6: Interpretive Phenomenological Analysis (IPA)
Interpretive. Phenomenological. Analysis. IPA . Try saying that three times fast…
Let’s just stick with IPA, okay?
IPA is designed to help you understand the personal experiences of a subject (for example, a person or group of people) concerning a major life event, an experience or a situation . This event or experience is the “phenomenon” that makes up the “P” in IPA. Such phenomena may range from relatively common events – such as motherhood, or being involved in a car accident – to those which are extremely rare – for example, someone’s personal experience in a refugee camp. So, IPA is a great choice if your research involves analysing people’s personal experiences of something that happened to them.
It’s important to remember that IPA is subject – centred . In other words, it’s focused on the experiencer . This means that, while you’ll likely use a coding system to identify commonalities, it’s important not to lose the depth of experience or meaning by trying to reduce everything to codes. Also, keep in mind that since your sample size will generally be very small with IPA, you often won’t be able to draw broad conclusions about the generalisability of your findings. But that’s okay as long as it aligns with your research aims and objectives.
Another thing to be aware of with IPA is personal bias . While researcher bias can creep into all forms of research, self-awareness is critically important with IPA, as it can have a major impact on the results. For example, a researcher who was a victim of a crime himself could insert his own feelings of frustration and anger into the way he interprets the experience of someone who was kidnapped. So, if you’re going to undertake IPA, you need to be very self-aware or you could muddy the analysis.
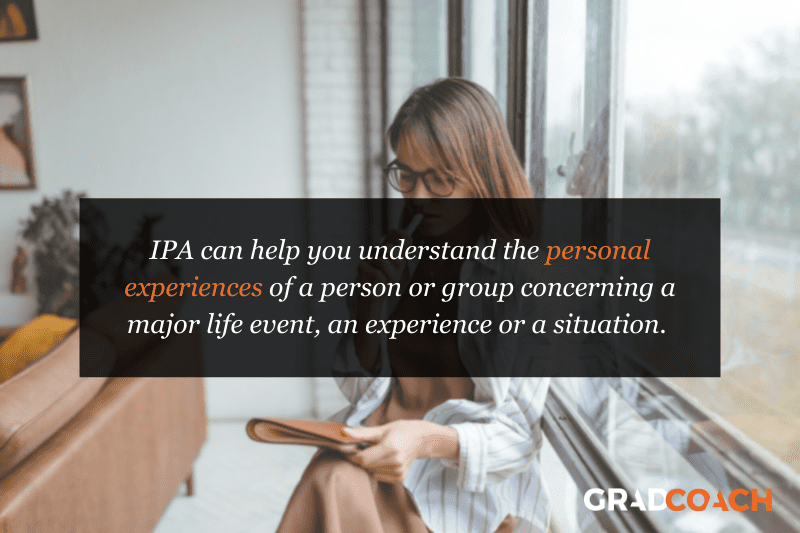
How to choose the right analysis method
In light of all of the qualitative analysis methods we’ve covered so far, you’re probably asking yourself the question, “ How do I choose the right one? ”
Much like all the other methodological decisions you’ll need to make, selecting the right qualitative analysis method largely depends on your research aims, objectives and questions . In other words, the best tool for the job depends on what you’re trying to build. For example:
- Perhaps your research aims to analyse the use of words and what they reveal about the intention of the storyteller and the cultural context of the time.
- Perhaps your research aims to develop an understanding of the unique personal experiences of people that have experienced a certain event, or
- Perhaps your research aims to develop insight regarding the influence of a certain culture on its members.
As you can probably see, each of these research aims are distinctly different , and therefore different analysis methods would be suitable for each one. For example, narrative analysis would likely be a good option for the first aim, while grounded theory wouldn’t be as relevant.
It’s also important to remember that each method has its own set of strengths, weaknesses and general limitations. No single analysis method is perfect . So, depending on the nature of your research, it may make sense to adopt more than one method (this is called triangulation ). Keep in mind though that this will of course be quite time-consuming.
As we’ve seen, all of the qualitative analysis methods we’ve discussed make use of coding and theme-generating techniques, but the intent and approach of each analysis method differ quite substantially. So, it’s very important to come into your research with a clear intention before you decide which analysis method (or methods) to use.
Start by reviewing your research aims , objectives and research questions to assess what exactly you’re trying to find out – then select a qualitative analysis method that fits. Never pick a method just because you like it or have experience using it – your analysis method (or methods) must align with your broader research aims and objectives.
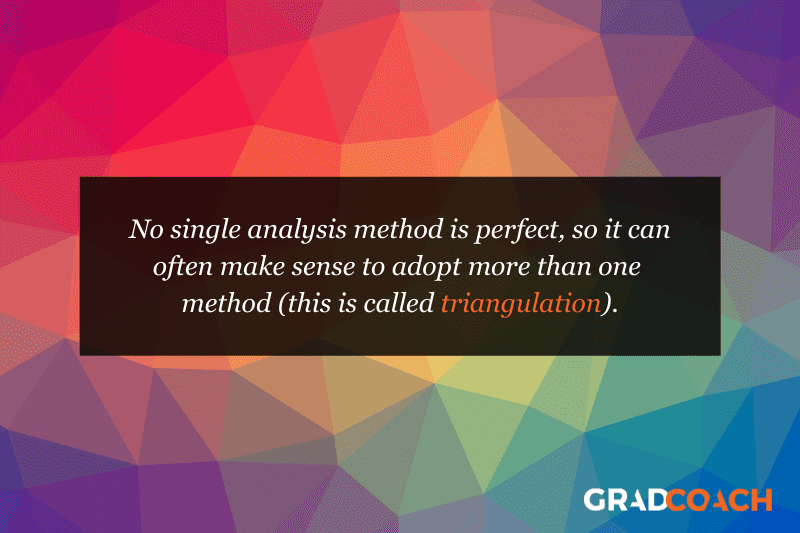
Let’s recap on QDA methods…
In this post, we looked at six popular qualitative data analysis methods:
- First, we looked at content analysis , a straightforward method that blends a little bit of quant into a primarily qualitative analysis.
- Then we looked at narrative analysis , which is about analysing how stories are told.
- Next up was discourse analysis – which is about analysing conversations and interactions.
- Then we moved on to thematic analysis – which is about identifying themes and patterns.
- From there, we went south with grounded theory – which is about starting from scratch with a specific question and using the data alone to build a theory in response to that question.
- And finally, we looked at IPA – which is about understanding people’s unique experiences of a phenomenon.
Of course, these aren’t the only options when it comes to qualitative data analysis, but they’re a great starting point if you’re dipping your toes into qualitative research for the first time.
If you’re still feeling a bit confused, consider our private coaching service , where we hold your hand through the research process to help you develop your best work.
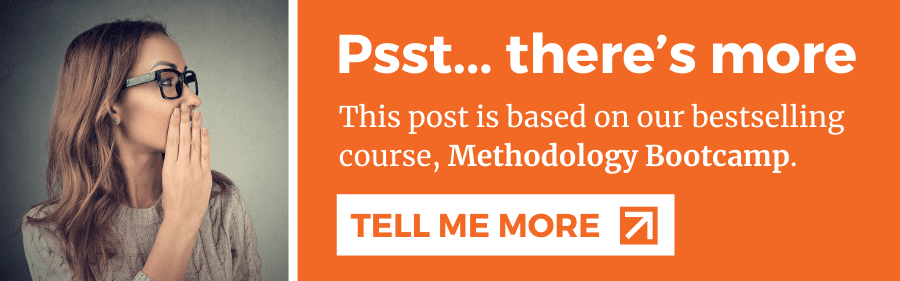
Learn More About Qualitative:
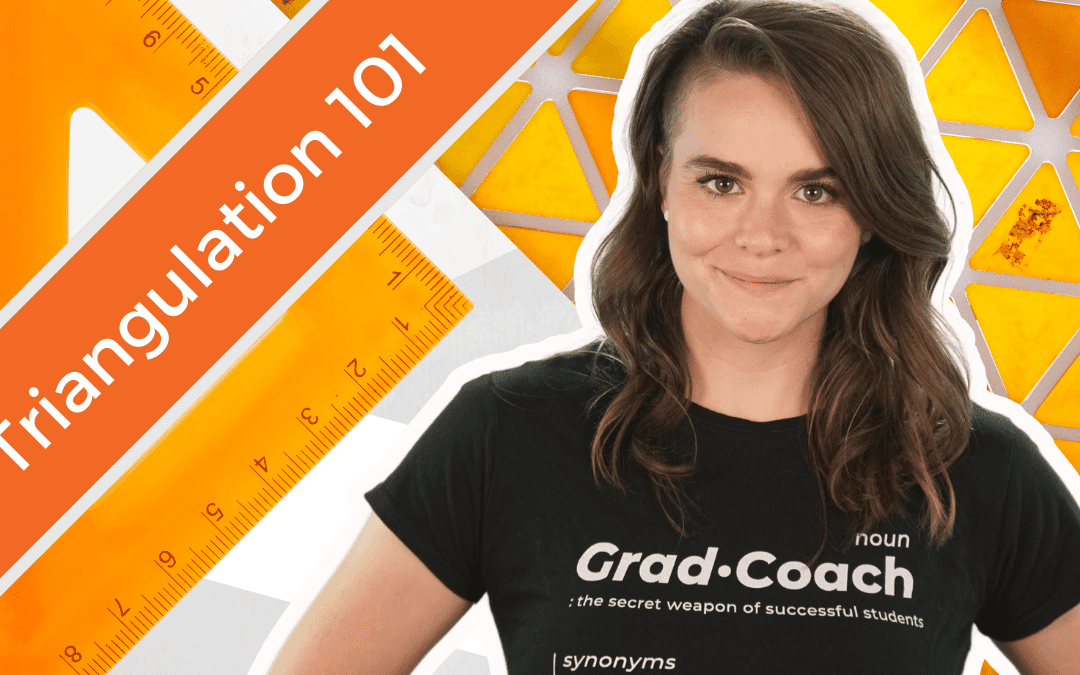
Triangulation: The Ultimate Credibility Enhancer
Triangulation is one of the best ways to enhance the credibility of your research. Learn about the different options here.
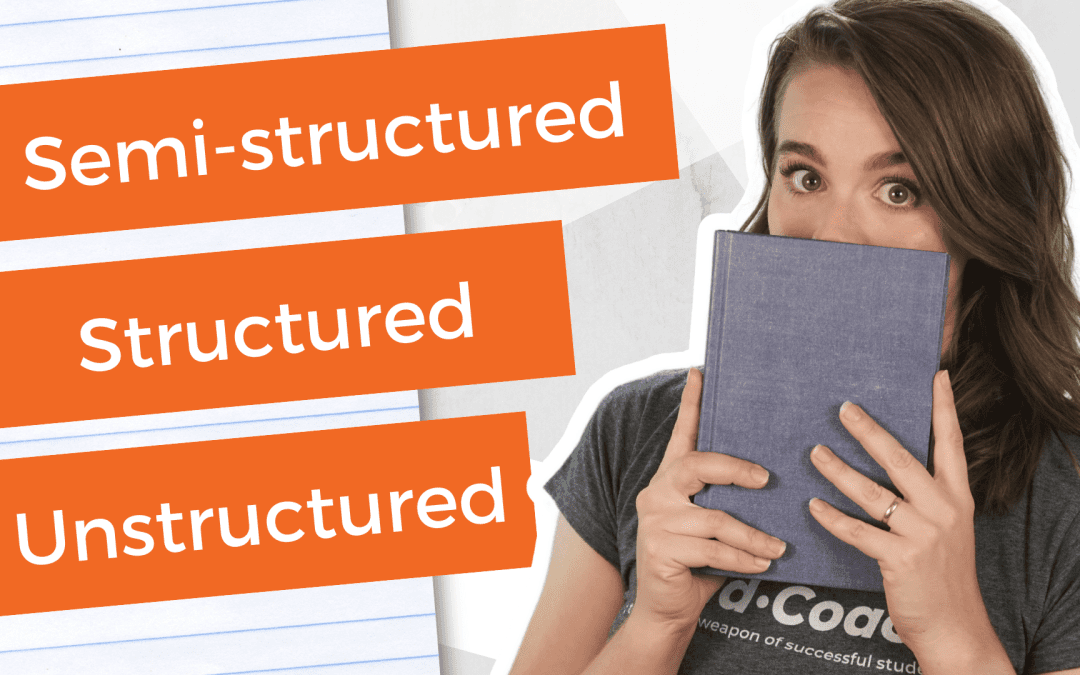
Structured, Semi-Structured & Unstructured Interviews
Learn about the differences (and similarities) between the three interview approaches: structured, semi-structured and unstructured.
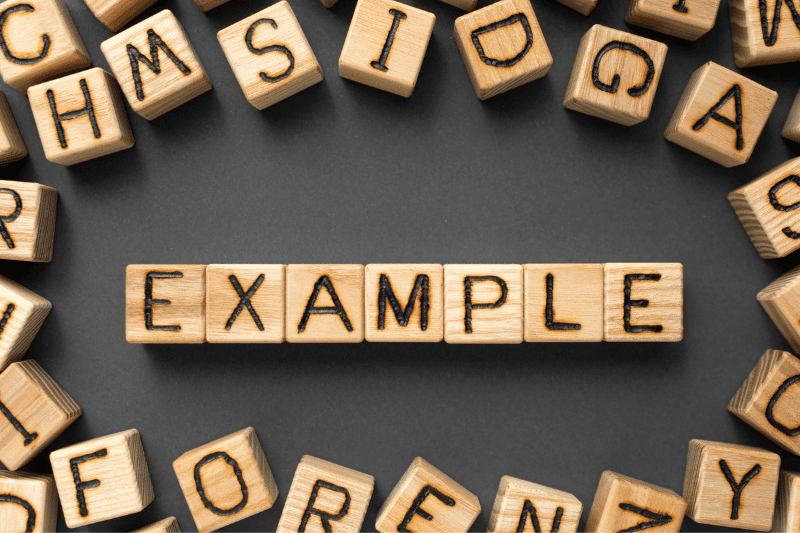
Qualitative Coding Examples: Process, Values & In Vivo Coding
See real-world examples of qualitative data that has been coded using process coding, values coding and in vivo coding.
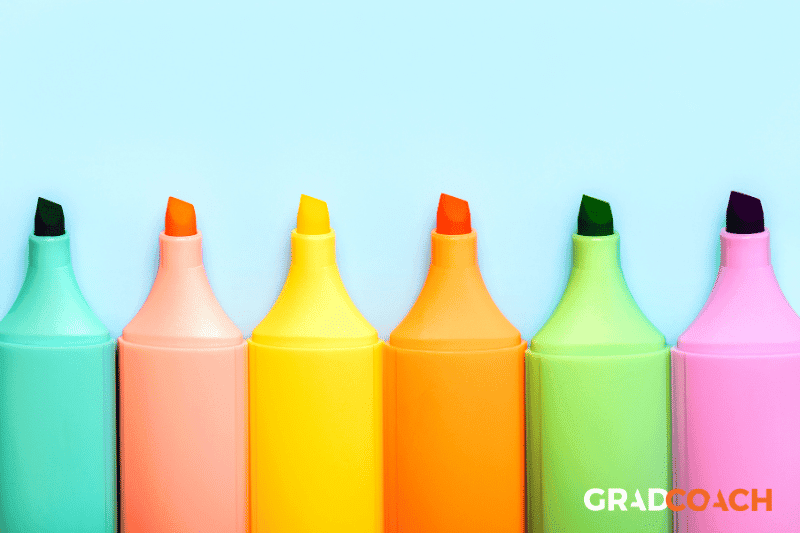
In Vivo Coding 101: Full Explainer With Examples
Learn about in vivo coding, a popular qualitative coding technique ideal for studies where the nuances of language are central to the aims.
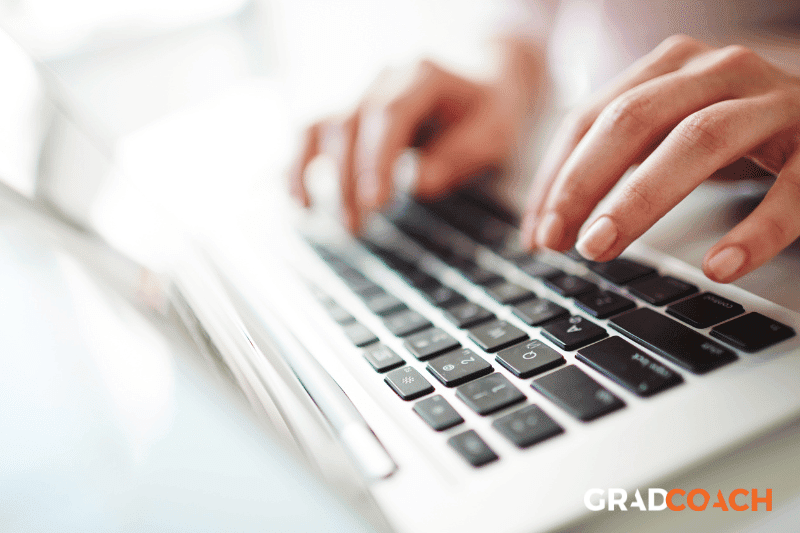
Process Coding 101: Full Explainer With Examples
Learn about process coding, a popular qualitative coding technique ideal for studies exploring processes, actions and changes over time.
📄 FREE TEMPLATES
Research Topic Ideation
Proposal Writing
Literature Review
Methodology & Analysis
Academic Writing
Referencing & Citing
Apps, Tools & Tricks
The Grad Coach Podcast
88 Comments
This has been very helpful. Thank you.
Thank you madam,
Thank you so much for this information
I wonder it so clear for understand and good for me. can I ask additional query?
Very insightful and useful
Good work done with clear explanations. Thank you.
Thanks so much for the write-up, it’s really good.
Thanks madam . It is very important .
thank you very good
Great presentation
very informative. Thank you!!
This has been very well explained in simple language . It is useful even for a new researcher.
Great to hear that. Good luck with your qualitative data analysis, Pramod!
This is very useful information. And it was very a clear language structured presentation. Thanks a lot.
Thank you so much.
very informative sequential presentation
Precise explanation of method.
Hi, may we use 2 data analysis methods in our qualitative research?
Thanks for your comment. Most commonly, one would use one type of analysis method, but it depends on your research aims and objectives.
You explained it in very simple language, everyone can understand it. Thanks so much.
Thank you very much, this is very helpful. It has been explained in a very simple manner that even a layman understands
Thank nicely explained can I ask is Qualitative content analysis the same as thematic analysis?
Thanks for your comment. No, QCA and thematic are two different types of analysis. This article might help clarify – https://onlinelibrary.wiley.com/doi/10.1111/nhs.12048
This is my first time to come across a well explained data analysis. so helpful.
I have thoroughly enjoyed your explanation of the six qualitative analysis methods. This is very helpful. Thank you!
Thank you very much, this is well explained and useful
i need a citation of your book.
Thanks a lot , remarkable indeed, enlighting to the best
Hi Derek, What other theories/methods would you recommend when the data is a whole speech?
Keep writing useful artikel.
It is important concept about QDA and also the way to express is easily understandable, so thanks for all.
Thank you, this is well explained and very useful.
Very helpful .Thanks.
Hi there! Very well explained. Simple but very useful style of writing. Please provide the citation of the text. warm regards
The session was very helpful and insightful. Thank you
This was very helpful and insightful. Easy to read and understand
As a professional academic writer, this has been so informative and educative. Keep up the good work Grad Coach you are unmatched with quality content for sure.
Keep up the good work Grad Coach you are unmatched with quality content for sure.
Its Great and help me the most. A Million Thanks you Dr.
It is a very nice work
Very insightful. Please, which of this approach could be used for a research that one is trying to elicit students’ misconceptions in a particular concept ?
This is Amazing and well explained, thanks
great overview
What do we call a research data analysis method that one use to advise or determining the best accounting tool or techniques that should be adopted in a company.
Informative video, explained in a clear and simple way. Kudos
Waoo! I have chosen method wrong for my data analysis. But I can revise my work according to this guide. Thank you so much for this helpful lecture.
This has been very helpful. It gave me a good view of my research objectives and how to choose the best method. Thematic analysis it is.
Very helpful indeed. Thanku so much for the insight.
This was incredibly helpful.
Very helpful.
very educative
Nicely written especially for novice academic researchers like me! Thank you.
choosing a right method for a paper is always a hard job for a student, this is a useful information, but it would be more useful personally for me, if the author provide me with a little bit more information about the data analysis techniques in type of explanatory research. Can we use qualitative content analysis technique for explanatory research ? or what is the suitable data analysis method for explanatory research in social studies?
that was very helpful for me. because these details are so important to my research. thank you very much
I learnt a lot. Thank you
Relevant and Informative, thanks !
Well-planned and organized, thanks much! 🙂
I have reviewed qualitative data analysis in a simplest way possible. The content will highly be useful for developing my book on qualitative data analysis methods. Cheers!
Clear explanation on qualitative and how about Case study
This was helpful. Thank you
This was really of great assistance, it was just the right information needed. Explanation very clear and follow.
Wow, Thanks for making my life easy
This was helpful thanks .
Very helpful…. clear and written in an easily understandable manner. Thank you.
This was so helpful as it was easy to understand. I’m a new to research thank you so much.
so educative…. but Ijust want to know which method is coding of the qualitative or tallying done?
Thank you for the great content, I have learnt a lot. So helpful
precise and clear presentation with simple language and thank you for that.
very informative content, thank you.
You guys are amazing on YouTube on this platform. Your teachings are great, educative, and informative. kudos!
Brilliant Delivery. You made a complex subject seem so easy. Well done.
Beautifully explained.
Thanks a lot
Is there a video the captures the practical process of coding using automated applications?
Thanks for the comment. We don’t recommend using automated applications for coding, as they are not sufficiently accurate in our experience.
content analysis can be qualitative research?
THANK YOU VERY MUCH.
Thank you very much for such a wonderful content
do you have any material on Data collection
What a powerful explanation of the QDA methods. Thank you.
Great explanation both written and Video. i have been using of it on a day to day working of my thesis project in accounting and finance. Thank you very much for your support.
very helpful, thank you so much
The tutorial is useful. I benefited a lot.
This is an eye opener for me and very informative, I have used some of your guidance notes on my Thesis, I wonder if you can assist with your 1. name of your book, year of publication, topic etc., this is for citing in my Bibliography,
I certainly hope to hear from you
Submit a Comment Cancel reply
Your email address will not be published. Required fields are marked *
Save my name, email, and website in this browser for the next time I comment.
Submit Comment
- Print Friendly
How to Write a Case Study Analysis
Before writing a case study analysis, it is important to identify a relevant subject and research problem. While preparing to write, the author should critically assess the potential problem and the need for in-depth analysis. For example, hidden problems, outdated information, or a feasible recommendation are all indications that a problem lends itself to a case study. The introduction should not only include a problem statement but should also note its importance, provide background information, supply a rationale for applying a case study method, and suggest potential advancement of knowledge in the given field.
Literature Review
The key purpose of the literature review in a case study analysis is to present the historical context of a problem and its background based on academic evidence. Synthesis of existing studies contributes to the subsequent substantial aspects that should be taken into account:
- Identify the context of the problem under consideration and locate related studies, showing the link between them. Each of the academic pieces used to write a case study should make a particular contribution to understanding or resolving the target problem.
- Connect all the studies together and provide a critical appraisal. One of the most common mistakes is to merely present existing evidence without any analysis or clarification of how one or another research investigation relates to the problem.
- Discover and discuss any gaps in the literature, demonstrating how the case study adds more value and credibility to the available evidence. For example, if the case study focuses on strategies used to promote feminism, it is possible to explore the gap of how this movement is developing in the Middle East, where it is not as evident as in Western countries.
- Identify conflicts and contradictory issues that can be found in the literature. This helps in coming closer to the resolution of the problem. If, for example, two different approaches are regarded as mutually exclusive in one study, and the same approaches are considered compatible in another, it is an evident conflict. A discussion of such contradictory issues should be placed in the case study to suggest feasible solutions.
- Locate the case study in the context of the literature and within the larger scale. More to the point, by synthesizing the pertinent literature and indicating the role of the case study, the researcher shows that he or she has made essential efforts toward addressing the problem.
In this section, it is important to clarify why a specific subject and problem were chosen for study. A range of subjects is possible, and the type chosen will impact how the method will be described. The key types of subjects include phenomena, places, persons, and events.
- The investigation of a phenomenon as a target of a case study implies that certain facts or circumstances exist and require change and improvement. The behavioral and social sciences often require the study of challenging issues such as the causes of high employee turnover or a low level of motivation on the part of managers. In such a case, cause-and-effect relationships should be established to evaluate the environment.
- If an accident or event composes the basis of the case study, its time and place will set the specifics of the research. A rare or critical event as well as any one of a number of common situations may be selected; in any case, a rationale should be provided to support the choice. As a rule, rare events require forging a new way of thinking regarding the occurrence, while dealing with a commonly encountered situation is associated with challenging existing hypotheses. In both types of cases, the following elements should be included in the case analysis: timeframe, circumstances, and consequences.
- A focus on a person as the subject of a study refers to scrutinizing the individual’s experience, relationships, and behaviors. For example, a discussion of Mark Zuckerberg as the creator of Facebook requires identifying the background, conflicts, and positive and negative consequences of his contributions to society.
- A place as the subject of a case study should be special, and it should be clear to readers why a particular area or neighborhood was chosen. What is, for example, the aim of focusing on China and Japan instead of the United States when exploring homelessness issues?
An important part of case study research or development in the field of the social and behavioral sciences involves discussing and interpreting findings. Therefore, after a thorough literature review, it is necessary to compare, reiterate, and revise the findings based on the experiences reported in previous studies along with the writer’s personal understanding of the subject studied.
- The discussion section may start with a brief summary of the findings that are of the greatest importance and particularly thought-provoking.
- The results should be assessed in terms of the hypothesis and objectives of the research. Briefly reiterating these, indicate whether the data obtained have confirmed the hypothesis, the goal has been achieved, and the research tasks have been addressed.
- The researcher should indicate how the findings are consistent with the conclusions of other researchers and theories described in the literature review. Comparing and contrasting the findings with the articles mentioned in the literature review will be beneficial as this will support the importance of the new study.
- To identify the possibilities and ways of applying the results to solve practical problems, recommendations for practical implementation should be as specific as possible and describe actions that organizations or individuals can take to put the findings into practice.
- The advantages of the research methodology used and the limitations of the generalization of the results obtained should be clarified. Such a discussion is especially necessary in a case study since it demonstrates an understanding of methodological nuances.
- It is advantageous to outline the prospects and suggestions for further research on the given problem to expand the scope of application of the results. It is also crucial to point out possible ways to improve the methodology and organization of a case study analysis.
In the conclusion, restating the key findings and noting their importance is essential to allow readers to understand the key points of the case study report. The conclusion should be written concisely, including a description of the problem and elaborating on solutions while presenting the expected future effect of the actions proposed for practical implementation. While the body of the case study paper focuses on details, the conclusion contains a generalized scope of the research problem. However, overgeneralization as well as failure to specify limitations should be avoided. Only the documentary evidence serves as a basis for assumptions, and the statement of limitations will help preclude unjustified conclusions.
- How to Write a Discussion Board Post
- How to Write a Case Study Like a Pro
- How to Write a Capstone Project Like an Expert
- Emails (Email Etiquette)
- Grant Proposal
- How to Write a Successful Motivation Letter
Main Tips On How To Write Case Study Analysis

Table of contents
- 1 What is a Case Study Analysis?
- 2 Difference Between Research Paper and Case Study
- 3 Types of Case Studies
- 4 Writing a Case Study Draft
- 5 How to Write a Case Study Outline
- 6 How to Write a Case Study
- 7 How to Analyze a Case Study
- 8.1 Tips for a Successful Case Analysis
- 9 How to Format a Case Study
- 10 How to Create a Title Page and Cite a Case Study
Many students struggle with how to do a case study analysis. Writing such an assignment is always daunting, as it requires you to analyze something and form conclusions based on your research.
It usually focuses on phenomena you can’t study in a typical way. Therefore, when writing such a text, you have to prepare thoughtfully. In the PapersOwl article, you will find out what this academic writing is and how to write a case analysis.
What is a Case Study Analysis?
A case study analysis is a form of writing that analyzes a specific situation, event, object, person, or even place. The said analysis should be written and structured to lead to a conclusion. Typically, you cannot analyze the subject of this essay via quantitative methods.
Note that such studies can be used in various fields and require the use of many theories that can give you a unique approach to the matter. For example, you can write a paper like this about social sciences, business, medicine, and many other fields. Each of these will require a particular approach.
Difference Between Research Paper and Case Study
Both research papers and case studies share common features, yet they also differ in several key aspects. Hence, knowing these parallels and distinctions, you will be able to learn how to write a case study assignment correctly.
A case study introduction can present the topic but does not require a citation of other similar works or the writer’s opinion. In contrast, a research paper requires citations right from the introduction, as it builds on the research of others.
Furthermore, authors of case studies should share their insights and perspectives on the case they study. A major difference is that research papers concentrate on a specific issue and use solid evidence. In contrast, case studies examine a subject in depth, offer detailed information, and help develop critical thinking skills.
Types of Case Studies
When it comes to writing case study analysis, there are five types you must learn to differentiate. That is important because whether you get such an assignment, you will have to understand the task first and then start with the writing.
Here are the types of case studies which you will encounter most often:
- Problem-oriented – this type focuses on real-life situations or theoretical issues and aims to solve them. For example, “World Hunger.”
- The second type is critical , also known as innate . The goal is to investigate a specific case, particularly its effects and what causes them – “Why Toys Remain Gender Stereotyped.”
Historical case studies focus on events from our past. The text should contain information about a specific historical period of this type. Your goal will be to provide different perspectives of an event and parallel them to current-day issues. An example of such a topic is “Racism During Ancient Times – Roman Empire.”
- The illustrative or Instrumental type focuses on describing a particular event. Here you have to explain the event’s outcome and what you have learned from it. A sample of such a topic is “The Effects of Dance Therapy in Depressed Adolescents.”
- Collective case studies are the fifth type. They include a collection of data about a specific case you will use to compare. E.g., “The Management Leadership at Work.”
- Exploratory Case Studies . This type often applies in new fields of study or in cases where little data exists. An example of this type is “Initial Insights into Behavioral Trends in Cryptocurrency Trading.”
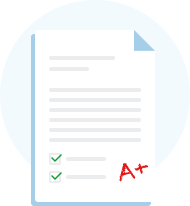
Writing a Case Study Draft
Creating a rough draft is the foremost step to take while writing such a paper. It is an essential step you must take, no matter how experienced you are. By doing it, you will be able to get more creative. In addition, you can explore options and decide on what to focus on more precisely, which will eventually result in a higher grade for your work.
So, sit down in a quiet place, bring an old-fashioned pen and paper, and start drafting ideas. Read them briefly while sipping on your tea and edit. After you have decided where your focus will lay, you have to develop these ideas and thoughts a bit more, then pick the best one.
How to Write a Case Study Outline
Creating a case study outline is an essential step in the writing process using the case study research method. Here’s how you can structure this preparation effectively:
- Conduct Research: Begin by using academic search engines like Google Scholar, or refer to books and published materials to gather relevant data. This research will help clarify the structure of your case study and determine the main points that need addressing.
- Formulate Your Thesis Statement: Develop a clear thesis statement that will guide the narrative of your case study. This statement should be based on the specific topic you have chosen.
- Outline Preparation
- Review Case Details: Thoroughly review the case you are analyzing to ensure a deep understanding of the subject.
- Note-taking and Question Formulation: Write down important notes and questions that arise during your review. Highlight relevant facts and critical data points that will support your analysis.
- Identify Problems and Causes: Identify the main problems and consider what their causes might be. This includes figuring out who is responsible and how these problems impact the company.
- Preliminary Research: Perform initial research to discover if similar problems have occurred previously and how they were resolved. This can offer insights into possible solutions and strategies for your case study.
The outline for your case study paper is essential to your writing process. It lets your professor assess your understanding of the topic, the correctness of your format, and the structure of your paper. They can spot any potential problems with your work. Having an outline serves as a guide for both you and your professor, making it easier to plan and write your paper . With the help of a well-crafted outline, your professor can navigate your paper more easily and spot any issues before they arise. Writing a case study can be challenging, but having a strong outline makes the process simpler.
A case study outline will most likely consist of the following sections and information:
- Case study title;
- Student’s name;
- Educational instructor’s name;
- Course name.
Introduction/Summary
- It briefly overviews your case study, thesis statement, and essential findings.
Main Body Paragraphs – usually three to five
- Literature Review/Background Information;
- Method/Findings;
- Discussion/Solutions/Recommendations.
- Repeat a paraphrased version of your thesis;
- Summarize your case study key points;
- Finish with a statement that can recommend the audience to read further by giving them thoughts to contemplate and develop new ideas.
Reference List or Bibliography
- List all the sources of evidence used to create your case study in your educational organization’s required citation style (APA, MLA, Chicago, Harvard, Turabian, etc.).
How to Write a Case Study
The way to write a case study is by strictly following the main idea of your thesis. You already know that a study’s main body consists of an introduction, literature review, method, discussion, and conclusion sections. Thus, all that is left is to focus on these parts and understand how to make them perfect.
- The Introduction/Summary: The introduction of a case study should start with a solid first sentence that will hook the reader. Afterward, you must explain the question you will be answering and why you are doing it. You should include some of the topic’s relevant history and details here. Also, you should explain how your case study will enrich the available information. Also, briefly summarize your literature review, which your findings will use as a base. Try to finish positively and make the reader see the benefits of reading your work.
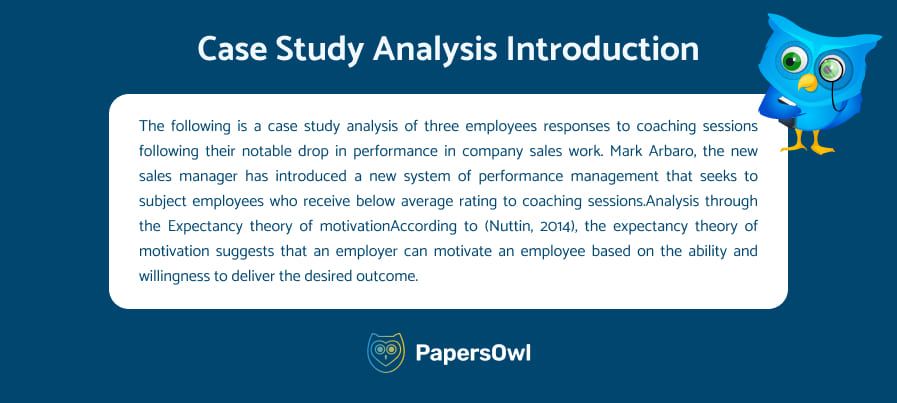
- Background Information/Literature Review: Structure and present the data from your academic sources . This section will show the reader how vital your work is and the basis for it.
- Method/Findings: This part aims to explain the case you selected, how it connects to the issue, and why you chose them. You can also add what methods you use. Here you must note that the data collection methods are qualitative, not quantitative, for case studies. That means the data is not random but well-structured and chronically taken from interviews, focus groups, and other sources.
- Discussion/Solutions: Restate your thesis but rephrase it, then draw your conclusions from what you have discovered via your research and link to your statement. Inform the audience of your main findings and define why the results are relevant to the field. Think about the following questions:
Were the results unexpected? Why/Why not?
How do your findings compare to previous similar case studies in your literature review?
Do your findings correlate to previous results, or do they contradict them?
Are your findings helpful in deepening the current understanding of the topic?
Next, explore possible alternative explanations or interpretations of your findings. Be subjective and explain your paper’s limitations. End with some suggestions for further exploration based on the limits of your work.
- Conclusion: Inform the reader precisely why your case study and findings are relevant, and restate your thesis and main results. Give a summary of previous studies you reviewed and how you contributed to expanding current knowledge. The final should explain how your work can be helpful and implemented in future research.
Your instructor should have an excellent example they can show you, so feel free to ask. They will surely want to help you learn how to write a case study!
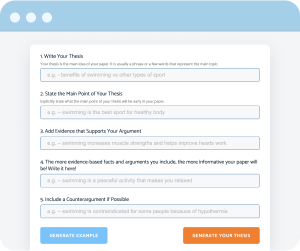
How to Analyze a Case Study
Analyzing a case study involves a structured approach that simulates real-life scenarios and is key to developing actionable insights. Here is a step-by-step guide adapted from Ellet, W. (2007) to help you effectively analyze a case study:
- Identify the Type of Case Study
Begin by determining the type of case study you are examining. This could be:
- Problems: Where something significant has occurred, and the cause is unknown.
- Decisions : Where a clear decision needs making, requiring options, criteria, and relevant evidence.
- Evaluations : Where you assess the effectiveness of a performance or outcome.
- Rules : These involve using quantitative methods to analyze a business area.
- Develop a Hypothesis
From the perspective of the protagonist, formulate a hypothesis to address the dilemma. Consider what you need to know about the situation:
- For problems: Understand the aspects, significance, and responsible parties.
- For decisions: Identify the options available, the stakes involved, and the decision-making criteria.
- For evaluations: Determine who or what is evaluated, the stakes, and the key evaluation criteria.
- Evidence and Alternatives
Evaluate your hypothesis by considering:
- The evidence supporting your hypothesis and any additional evidence needed.
- The weaknesses of your hypothesis and possible alternatives.
Writing a Case Analysis
Writing a case analysis involves a structured approach that enables you to communicate your understanding and analytical skills effectively. Here’s how you can craft your analysis to be insightful and comprehensive:
- Start with a Clear Definition or Position Statement
Begin your case analysis by stating your main conclusion, which serves as the answer to “What?” This initial statement should clearly outline what you have concluded from your analysis of the case.
- Build a Strong Argument with Evidence
Support your position statement with solid evidence, which answers “Why?” This evidence can be quantitative (numerical data) or qualitative (observations and interviews). The purpose here is to provide a foundation for your conclusions, showing how you arrived at them through logical reasoning.
- Outline a Chronological Action Plan
Detail the steps necessary to solve the problem, implement a decision, or enhance performance. This section answers “How?” and should include specific, realistic steps that address any major risks associated with your plan.
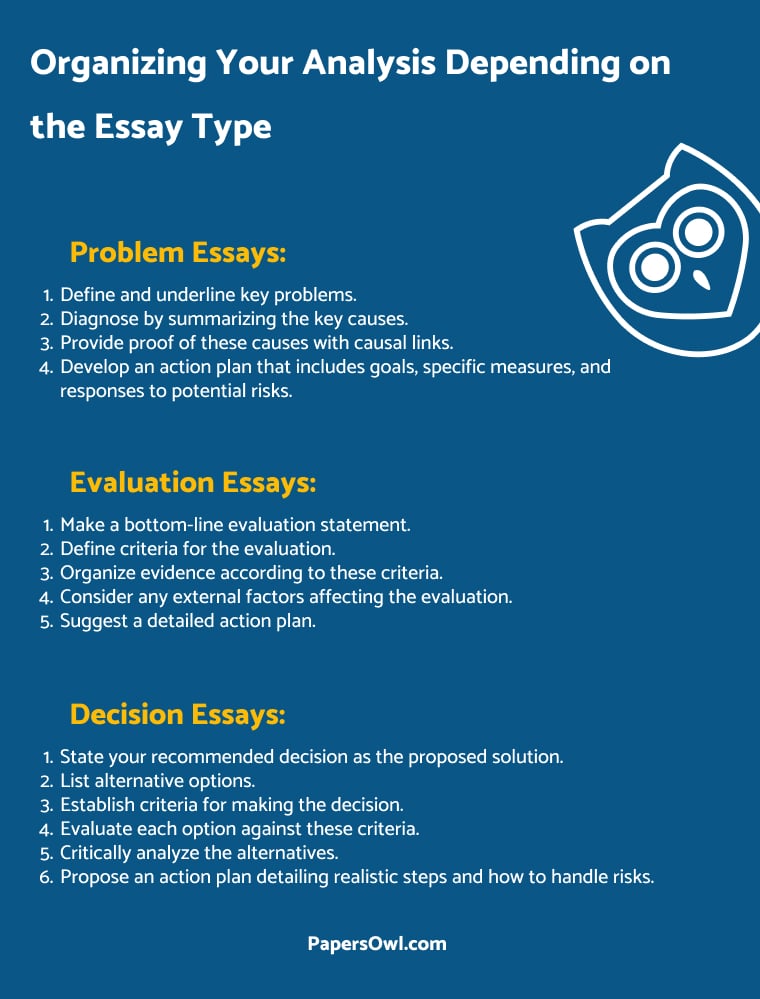
Tips for a Successful Case Analysis
- Aim to deliver concise and clear results of your analytical process rather than a simple summary or a complete transcript of your thoughts.
- Ensure your reader understands and is persuaded by your analysis by linking your conclusions directly to credible evidence.
- Discuss the advantages and also acknowledge any disadvantages of your conclusions to provide a balanced view.
How to Format a Case Study
Knowing how a case study analysis format should look is crucial. Therefore, you must know what the text structure should look like. The standard one contains about eight sections:
- Introduction/The Executive Summary: As the first part here, you have to hook the reader’s attention, so the introduction of the case study is the most important part of the writing. Then present them with a brief overview of your case study analyses and their findings. Make sure to form a good thesis statement , as this is the pivotal point of your work.
- Literary Review/Background information: Similarly to other papers, in this part, you have to write your most important facts or findings while identifying the case issue.
- Method/Findings/Discussion: This section can be written separately based on how your text flows. Here you will have to explore more about the case and its findings. Allow yourself to go into more detail instead of just briefly covering them.
- Solutions/Recommendations/Implementation Part: You have to discuss the answers you came up with. Basically, you say why they are fit to solve the case and how you think they can be used in practice. Note that you must write only realistic and practical solutions for the problem. It’s possible to write testable evidence that can support your recommendations.
- Conclusion: Here, you are supposed to cover your whole paper briefly and even repeat the thesis (rephrased). Make sure to highlight the critical points of your case study.
- References or Bibliography: This section must include the sources from which you collected data or whom you consulted. Usually, this part is on a separate page, and the listing should be according to your academic institution’s requirements.
- Appendices (include only if applicable): It is usual for some parts of your materials to be too lengthy or unfit for the other sections of the case study. Therefore, you have to include them here. That can be pictures, raw data of statistics, graphs, notes, etc. The appendix section is strictly for subsidiary materials, do not put the most relevant ones here.
- Author Note: Remember that all educational institutions have their requirement for a case study format. The abovementioned is an example; thus, you may see a section or another is missing, or there are additional ones.
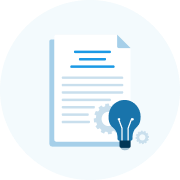
How to Create a Title Page and Cite a Case Study
A case study in APA format for students can differ from one institution to another. So, knowing your college or school requirements is crucial before you start writing. Nonetheless, the general one should look like this:
- Title – A header no longer than nine words has “Case Study” and reflects the content and the idea behind it yet is engaging to read;
- Write your full name;
- The name of your course/class;
- Next is your professor or instructor name;
- The university/school name;
- The date of submission.
When citing in your paper, you must ensure it is done accurately and in your academic style. If you are unsure how to do it, research the requirements and google “How to do a case study analysis in Harvard”, for example. Note that short citations can be in your text, but longer ones should be in the bibliography section.
Hruby, A. (2018). Hruby, A., & Hu, F. B. (2015). The epidemiology of obesity: a big picture. Pharmacoeconomics, 33(7), 673-689. www.sciepub.com. http://www.sciepub.com/reference/254744
Case studies strive to analyze an event, location, case, or person. They can be similar to research papers, so you must pay close attention to the structure and what your professor has requested from you.
Finally, the process of writing can be overwhelming due to the many sections. However, if you take the process step by step and do your preparations properly, you will have an easy time writing the paper. You can also look for assistance online – many services offer to order case study online help . With the right kind of assistance, you can be sure that your paper is of high quality and is due on time!
Readers also enjoyed
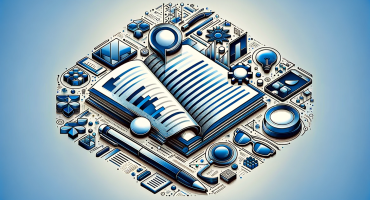
WHY WAIT? PLACE AN ORDER RIGHT NOW!
Just fill out the form, press the button, and have no worries!
We use cookies to give you the best experience possible. By continuing we’ll assume you board with our cookie policy.
What is case study research?
Last updated
8 February 2023
Reviewed by
Cathy Heath
Short on time? Get an AI generated summary of this article instead
Suppose a company receives a spike in the number of customer complaints, or medical experts discover an outbreak of illness affecting children but are not quite sure of the reason. In both cases, carrying out a case study could be the best way to get answers.
Organization
Case studies can be carried out across different disciplines, including education, medicine, sociology, and business.
Most case studies employ qualitative methods, but quantitative methods can also be used. Researchers can then describe, compare, evaluate, and identify patterns or cause-and-effect relationships between the various variables under study. They can then use this knowledge to decide what action to take.
Another thing to note is that case studies are generally singular in their focus. This means they narrow focus to a particular area, making them highly subjective. You cannot always generalize the results of a case study and apply them to a larger population. However, they are valuable tools to illustrate a principle or develop a thesis.
Analyze case study research
Dovetail streamlines case study research to help you uncover and share actionable insights
- What are the different types of case study designs?
Researchers can choose from a variety of case study designs. The design they choose is dependent on what questions they need to answer, the context of the research environment, how much data they already have, and what resources are available.
Here are the common types of case study design:
Explanatory
An explanatory case study is an initial explanation of the how or why that is behind something. This design is commonly used when studying a real-life phenomenon or event. Once the organization understands the reasons behind a phenomenon, it can then make changes to enhance or eliminate the variables causing it.
Here is an example: How is co-teaching implemented in elementary schools? The title for a case study of this subject could be “Case Study of the Implementation of Co-Teaching in Elementary Schools.”
Descriptive
An illustrative or descriptive case study helps researchers shed light on an unfamiliar object or subject after a period of time. The case study provides an in-depth review of the issue at hand and adds real-world examples in the area the researcher wants the audience to understand.
The researcher makes no inferences or causal statements about the object or subject under review. This type of design is often used to understand cultural shifts.
Here is an example: How did people cope with the 2004 Indian Ocean Tsunami? This case study could be titled "A Case Study of the 2004 Indian Ocean Tsunami and its Effect on the Indonesian Population."
Exploratory
Exploratory research is also called a pilot case study. It is usually the first step within a larger research project, often relying on questionnaires and surveys . Researchers use exploratory research to help narrow down their focus, define parameters, draft a specific research question , and/or identify variables in a larger study. This research design usually covers a wider area than others, and focuses on the ‘what’ and ‘who’ of a topic.
Here is an example: How do nutrition and socialization in early childhood affect learning in children? The title of the exploratory study may be “Case Study of the Effects of Nutrition and Socialization on Learning in Early Childhood.”
An intrinsic case study is specifically designed to look at a unique and special phenomenon. At the start of the study, the researcher defines the phenomenon and the uniqueness that differentiates it from others.
In this case, researchers do not attempt to generalize, compare, or challenge the existing assumptions. Instead, they explore the unique variables to enhance understanding. Here is an example: “Case Study of Volcanic Lightning.”
This design can also be identified as a cumulative case study. It uses information from past studies or observations of groups of people in certain settings as the foundation of the new study. Given that it takes multiple areas into account, it allows for greater generalization than a single case study.
The researchers also get an in-depth look at a particular subject from different viewpoints. Here is an example: “Case Study of how PTSD affected Vietnam and Gulf War Veterans Differently Due to Advances in Military Technology.”
Critical instance
A critical case study incorporates both explanatory and intrinsic study designs. It does not have predetermined purposes beyond an investigation of the said subject. It can be used for a deeper explanation of the cause-and-effect relationship. It can also be used to question a common assumption or myth.
The findings can then be used further to generalize whether they would also apply in a different environment. Here is an example: “What Effect Does Prolonged Use of Social Media Have on the Mind of American Youth?”
Instrumental
Instrumental research attempts to achieve goals beyond understanding the object at hand. Researchers explore a larger subject through different, separate studies and use the findings to understand its relationship to another subject. This type of design also provides insight into an issue or helps refine a theory.
For example, you may want to determine if violent behavior in children predisposes them to crime later in life. The focus is on the relationship between children and violent behavior, and why certain children do become violent. Here is an example: “Violence Breeds Violence: Childhood Exposure and Participation in Adult Crime.”
Evaluation case study design is employed to research the effects of a program, policy, or intervention, and assess its effectiveness and impact on future decision-making.
For example, you might want to see whether children learn times tables quicker through an educational game on their iPad versus a more teacher-led intervention. Here is an example: “An Investigation of the Impact of an iPad Multiplication Game for Primary School Children.”
- When do you use case studies?
Case studies are ideal when you want to gain a contextual, concrete, or in-depth understanding of a particular subject. It helps you understand the characteristics, implications, and meanings of the subject.
They are also an excellent choice for those writing a thesis or dissertation, as they help keep the project focused on a particular area when resources or time may be too limited to cover a wider one. You may have to conduct several case studies to explore different aspects of the subject in question and understand the problem.
- What are the steps to follow when conducting a case study?
1. Select a case
Once you identify the problem at hand and come up with questions, identify the case you will focus on. The study can provide insights into the subject at hand, challenge existing assumptions, propose a course of action, and/or open up new areas for further research.
2. Create a theoretical framework
While you will be focusing on a specific detail, the case study design you choose should be linked to existing knowledge on the topic. This prevents it from becoming an isolated description and allows for enhancing the existing information.
It may expand the current theory by bringing up new ideas or concepts, challenge established assumptions, or exemplify a theory by exploring how it answers the problem at hand. A theoretical framework starts with a literature review of the sources relevant to the topic in focus. This helps in identifying key concepts to guide analysis and interpretation.
3. Collect the data
Case studies are frequently supplemented with qualitative data such as observations, interviews, and a review of both primary and secondary sources such as official records, news articles, and photographs. There may also be quantitative data —this data assists in understanding the case thoroughly.
4. Analyze your case
The results of the research depend on the research design. Most case studies are structured with chapters or topic headings for easy explanation and presentation. Others may be written as narratives to allow researchers to explore various angles of the topic and analyze its meanings and implications.
In all areas, always give a detailed contextual understanding of the case and connect it to the existing theory and literature before discussing how it fits into your problem area.
- What are some case study examples?
What are the best approaches for introducing our product into the Kenyan market?
How does the change in marketing strategy aid in increasing the sales volumes of product Y?
How can teachers enhance student participation in classrooms?
How does poverty affect literacy levels in children?
Case study topics
Case study of product marketing strategies in the Kenyan market
Case study of the effects of a marketing strategy change on product Y sales volumes
Case study of X school teachers that encourage active student participation in the classroom
Case study of the effects of poverty on literacy levels in children
Should you be using a customer insights hub?
Do you want to discover previous research faster?
Do you share your research findings with others?
Do you analyze research data?
Start for free today, add your research, and get to key insights faster
Editor’s picks
Last updated: 30 January 2024
Last updated: 11 January 2024
Last updated: 17 January 2024
Last updated: 12 December 2023
Last updated: 30 April 2024
Last updated: 4 July 2024
Last updated: 12 October 2023
Last updated: 5 March 2024
Last updated: 6 March 2024
Last updated: 31 January 2024
Last updated: 23 January 2024
Last updated: 13 May 2024
Last updated: 20 December 2023
Latest articles
Related topics, decide what to build next, log in or sign up.
Get started for free

Data Analytics Case Study: Complete Guide in 2024
What are data analytics case study interviews.
When you’re trying to land a data analyst job, the last thing to stand in your way is the data analytics case study interview.
One reason they’re so challenging is that case studies don’t typically have a right or wrong answer.
Instead, case study interviews require you to come up with a hypothesis for an analytics question and then produce data to support or validate your hypothesis. In other words, it’s not just about your technical skills; you’re also being tested on creative problem-solving and your ability to communicate with stakeholders.
This article provides an overview of how to answer data analytics case study interview questions. You can find an in-depth course in the data analytics learning path .
How to Solve Data Analytics Case Questions
Check out our video below on How to solve a Data Analytics case study problem:
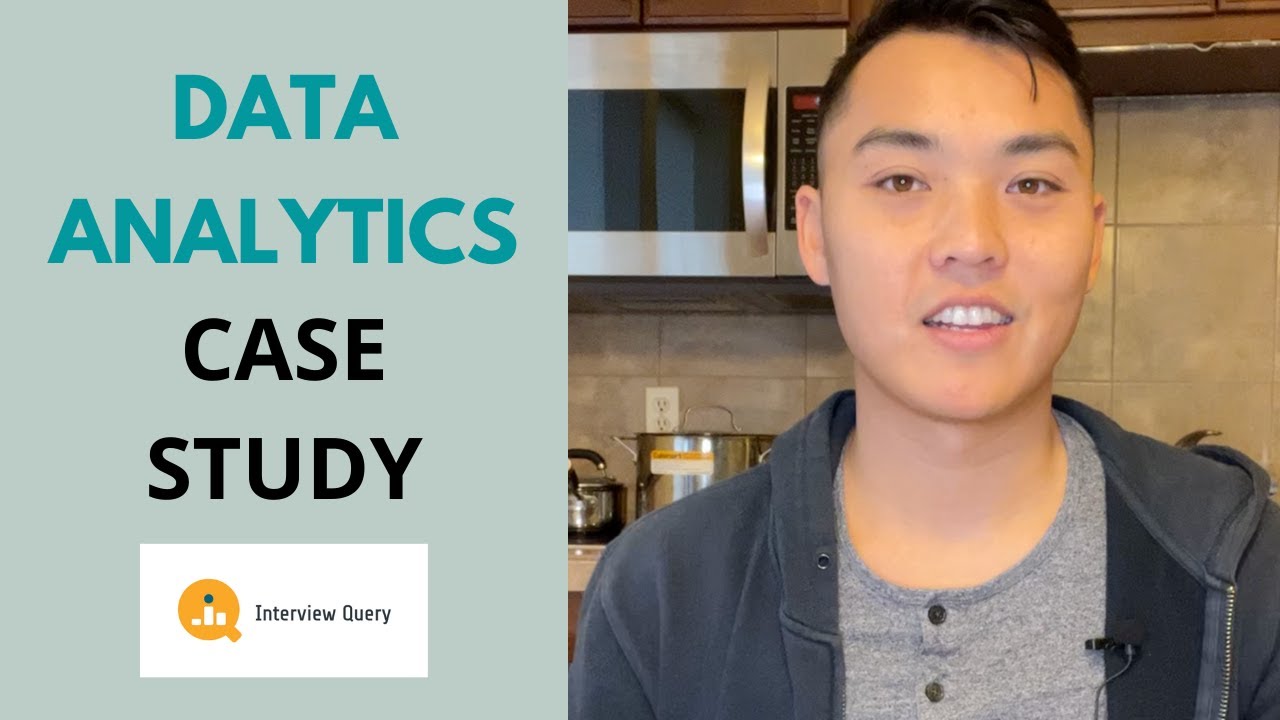
With data analyst case questions, you will need to answer two key questions:
- What metrics should I propose?
- How do I write a SQL query to get the metrics I need?
In short, to ace a data analytics case interview, you not only need to brush up on case questions, but you also should be adept at writing all types of SQL queries and have strong data sense.
These questions are especially challenging to answer if you don’t have a framework or know how to answer them. To help you prepare , we created this step-by-step guide to answering data analytics case questions.
We show you how to use a framework to answer case questions, provide example analytics questions, and help you understand the difference between analytics case studies and product metrics case studies .
Data Analytics Cases vs Product Metrics Questions
Product case questions sometimes get lumped in with data analytics cases.
Ultimately, the type of case question you are asked will depend on the role. For example, product analysts will likely face more product-oriented questions.
Product metrics cases tend to focus on a hypothetical situation. You might be asked to:
Investigate Metrics - One of the most common types will ask you to investigate a metric, usually one that’s going up or down. For example, “Why are Facebook friend requests falling by 10 percent?”
Measure Product/Feature Success - A lot of analytics cases revolve around the measurement of product success and feature changes. For example, “We want to add X feature to product Y. What metrics would you track to make sure that’s a good idea?”
With product data cases, the key difference is that you may or may not be required to write the SQL query to find the metric.
Instead, these interviews are more theoretical and are designed to assess your product sense and ability to think about analytics problems from a product perspective. Product metrics questions may also show up in the data analyst interview , but likely only for product data analyst roles.
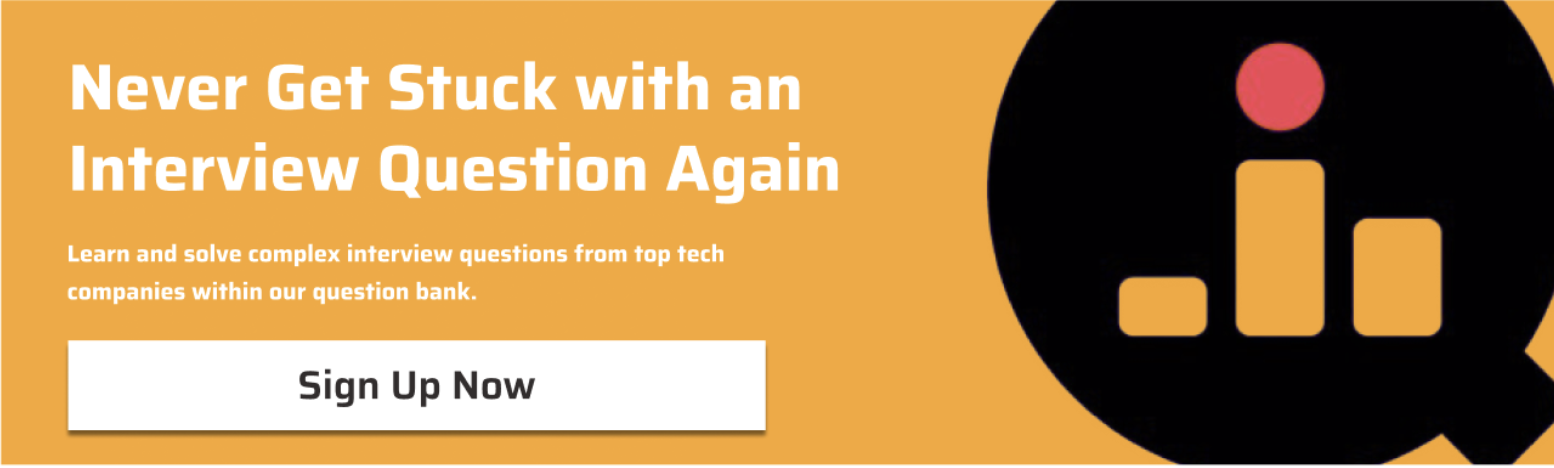
TRY CHECKING: Marketing Analytics Case Study Guide
Data Analytics Case Study Question: Sample Solution
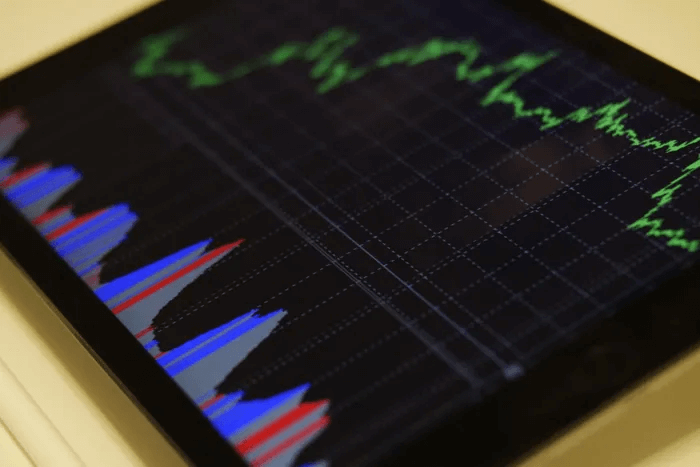
Let’s start with an example data analytics case question :
You’re given a table that represents search results from searches on Facebook. The query column is the search term, the position column represents each position the search result came in, and the rating column represents the human rating from 1 to 5, where 5 is high relevance, and 1 is low relevance.
Each row in the search_events table represents a single search, with the has_clicked column representing if a user clicked on a result or not. We have a hypothesis that the CTR is dependent on the search result rating.
Write a query to return data to support or disprove this hypothesis.
search_results table:
search_events table
Step 1: With Data Analytics Case Studies, Start by Making Assumptions
Hint: Start by making assumptions and thinking out loud. With this question, focus on coming up with a metric to support the hypothesis. If the question is unclear or if you think you need more information, be sure to ask.
Answer. The hypothesis is that CTR is dependent on search result rating. Therefore, we want to focus on the CTR metric, and we can assume:
- If CTR is high when search result ratings are high, and CTR is low when the search result ratings are low, then the hypothesis is correct.
- If CTR is low when the search ratings are high, or there is no proven correlation between the two, then our hypothesis is not proven.
Step 2: Provide a Solution for the Case Question
Hint: Walk the interviewer through your reasoning. Talking about the decisions you make and why you’re making them shows off your problem-solving approach.
Answer. One way we can investigate the hypothesis is to look at the results split into different search rating buckets. For example, if we measure the CTR for results rated at 1, then those rated at 2, and so on, we can identify if an increase in rating is correlated with an increase in CTR.
First, I’d write a query to get the number of results for each query in each bucket. We want to look at the distribution of results that are less than a rating threshold, which will help us see the relationship between search rating and CTR.
This CTE aggregates the number of results that are less than a certain rating threshold. Later, we can use this to see the percentage that are in each bucket. If we re-join to the search_events table, we can calculate the CTR by then grouping by each bucket.
Step 3: Use Analysis to Backup Your Solution
Hint: Be prepared to justify your solution. Interviewers will follow up with questions about your reasoning, and ask why you make certain assumptions.
Answer. By using the CASE WHEN statement, I calculated each ratings bucket by checking to see if all the search results were less than 1, 2, or 3 by subtracting the total from the number within the bucket and seeing if it equates to 0.
I did that to get away from averages in our bucketing system. Outliers would make it more difficult to measure the effect of bad ratings. For example, if a query had a 1 rating and another had a 5 rating, that would equate to an average of 3. Whereas in my solution, a query with all of the results under 1, 2, or 3 lets us know that it actually has bad ratings.
Product Data Case Question: Sample Solution
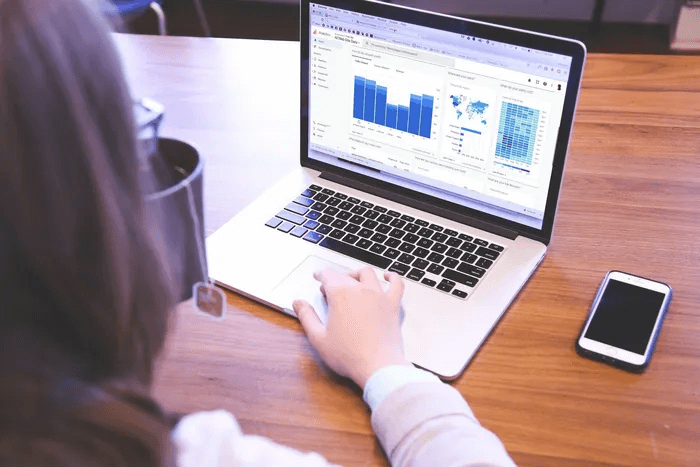
In product metrics interviews, you’ll likely be asked about analytics, but the discussion will be more theoretical. You’ll propose a solution to a problem, and supply the metrics you’ll use to investigate or solve it. You may or may not be required to write a SQL query to get those metrics.
We’ll start with an example product metrics case study question :
Let’s say you work for a social media company that has just done a launch in a new city. Looking at weekly metrics, you see a slow decrease in the average number of comments per user from January to March in this city.
The company has been consistently growing new users in the city from January to March.
What are some reasons why the average number of comments per user would be decreasing and what metrics would you look into?
Step 1: Ask Clarifying Questions Specific to the Case
Hint: This question is very vague. It’s all hypothetical, so we don’t know very much about users, what the product is, and how people might be interacting. Be sure you ask questions upfront about the product.
Answer: Before I jump into an answer, I’d like to ask a few questions:
- Who uses this social network? How do they interact with each other?
- Has there been any performance issues that might be causing the problem?
- What are the goals of this particular launch?
- Has there been any changes to the comment features in recent weeks?
For the sake of this example, let’s say we learn that it’s a social network similar to Facebook with a young audience, and the goals of the launch are to grow the user base. Also, there have been no performance issues and the commenting feature hasn’t been changed since launch.
Step 2: Use the Case Question to Make Assumptions
Hint: Look for clues in the question. For example, this case gives you a metric, “average number of comments per user.” Consider if the clue might be helpful in your solution. But be careful, sometimes questions are designed to throw you off track.
Answer: From the question, we can hypothesize a little bit. For example, we know that user count is increasing linearly. That means two things:
- The decreasing comments issue isn’t a result of a declining user base.
- The cause isn’t loss of platform.
We can also model out the data to help us get a better picture of the average number of comments per user metric:
- January: 10000 users, 30000 comments, 3 comments/user
- February: 20000 users, 50000 comments, 2.5 comments/user
- March: 30000 users, 60000 comments, 2 comments/user
One thing to note: Although this is an interesting metric, I’m not sure if it will help us solve this question. For one, average comments per user doesn’t account for churn. We might assume that during the three-month period users are churning off the platform. Let’s say the churn rate is 25% in January, 20% in February and 15% in March.
Step 3: Make a Hypothesis About the Data
Hint: Don’t worry too much about making a correct hypothesis. Instead, interviewers want to get a sense of your product initiation and that you’re on the right track. Also, be prepared to measure your hypothesis.
Answer. I would say that average comments per user isn’t a great metric to use, because it doesn’t reveal insights into what’s really causing this issue.
That’s because it doesn’t account for active users, which are the users who are actually commenting. A better metric to investigate would be retained users and monthly active users.
What I suspect is causing the issue is that active users are commenting frequently and are responsible for the increase in comments month-to-month. New users, on the other hand, aren’t as engaged and aren’t commenting as often.
Step 4: Provide Metrics and Data Analysis
Hint: Within your solution, include key metrics that you’d like to investigate that will help you measure success.
Answer: I’d say there are a few ways we could investigate the cause of this problem, but the one I’d be most interested in would be the engagement of monthly active users.
If the growth in comments is coming from active users, that would help us understand how we’re doing at retaining users. Plus, it will also show if new users are less engaged and commenting less frequently.
One way that we could dig into this would be to segment users by their onboarding date, which would help us to visualize engagement and see how engaged some of our longest-retained users are.
If engagement of new users is the issue, that will give us some options in terms of strategies for addressing the problem. For example, we could test new onboarding or commenting features designed to generate engagement.
Step 5: Propose a Solution for the Case Question
Hint: In the majority of cases, your initial assumptions might be incorrect, or the interviewer might throw you a curveball. Be prepared to make new hypotheses or discuss the pitfalls of your analysis.
Answer. If the cause wasn’t due to a lack of engagement among new users, then I’d want to investigate active users. One potential cause would be active users commenting less. In that case, we’d know that our earliest users were churning out, and that engagement among new users was potentially growing.
Again, I think we’d want to focus on user engagement since the onboarding date. That would help us understand if we were seeing higher levels of churn among active users, and we could start to identify some solutions there.
Tip: Use a Framework to Solve Data Analytics Case Questions
Analytics case questions can be challenging, but they’re much more challenging if you don’t use a framework. Without a framework, it’s easier to get lost in your answer, to get stuck, and really lose the confidence of your interviewer. Find helpful frameworks for data analytics questions in our data analytics learning path and our product metrics learning path .
Once you have the framework down, what’s the best way to practice? Mock interviews with our coaches are very effective, as you’ll get feedback and helpful tips as you answer. You can also learn a lot by practicing P2P mock interviews with other Interview Query students. No data analytics background? Check out how to become a data analyst without a degree .
Finally, if you’re looking for sample data analytics case questions and other types of interview questions, see our guide on the top data analyst interview questions .

- eLibrary Home
- eLibrary Login
- < Previous
Home > CHAPTERS > ACIS > ACIS 2002 > 38
ACIS 2002 Proceedings
Four steps to analyse data from a case study method.
John Atkinson , Charles Sturt University
Four steps are proposed to assist the novice researcher analyse their data that has been collected using a case study method. The first step proposes the creation of a data repository using basic relational database theory. The second step involves creating codes to identify the respective ‘chunks’ of data. These resulting codes are then analysed and rationalised. The third step involves analysing the case study data by generating a variety of reports. The fourth step generates the final propositions by linking the rationalised codes back to the initial propositions and where appropriate new propositions are generated. The outcome of these steps is a series of propositions that reflect the nature of the data associated with the case studies data.
Recommended Citation
Atkinson, John, "Four Steps to Analyse Data from a Case Study Method" (2002). ACIS 2002 Proceedings . 38. https://aisel.aisnet.org/acis2002/38
Since August 21, 2008
Advanced Search
- Notify me via email or RSS
- ACIS 2002 Website
- All Content
Author Corner
- eLibrary FAQ
Home | About | FAQ | My Account | Accessibility Statement
Privacy Copyright
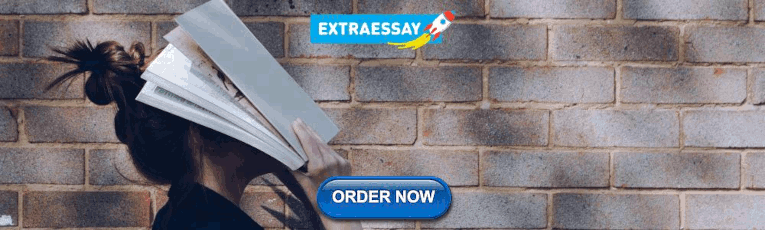
IMAGES
VIDEO
COMMENTS
The data collection method should be selected based on the research questions and the nature of the case study phenomenon. Analyze the data: The data collected from the case study should be analyzed using various techniques, such as content analysis, thematic analysis, or grounded theory. The analysis should be guided by the research questions ...
Step 1: Gather your qualitative data. The first step of qualitative research is to do data collection. Put simply, data collection is gathering all of your data for analysis. A common situation is when qualitative data is spread across various sources.
How to Analyze a Case Study Adapted from Ellet, W. (2007). The case study handbook. Boston, MA: Harvard Business School. A business case simulates a real situation and has three characteristics: 1. a significant issue, 2. enough information to reach a reasonable conclusion, 3. no stated conclusion. A case may include 1. irrelevant information 2.
Describe annual revenues and profit. Provide figures on employment. Include details about private ownership, public ownership, and investment holdings. Provide a brief overview of the business's leaders and command chain. [1] 3. Identify the key issue or problem in the case study. Download Article.
Furthermore, the ability to describe in detail how the analysis was conducted ensures rigour in reporting qualitative research. Data sources: The research example used is a multiple case study that explored the role of the clinical skills laboratory in preparing students for the real world of practice. Data analysis was conducted using a ...
Case study protocol is a formal document capturing the entire set of procedures involved in the collection of empirical material . It extends direction to researchers for gathering evidences, empirical material analysis, and case study reporting . This section includes a step-by-step guide that is used for the execution of the actual study.
For case study analysis, one of the most desirable techniques is to use a pattern-matching logic. Such a logic (Trochim, 1989) compares an empiri-cally based pattern with a predicted one (or with several alternative predic-tions). If the patterns coincide, the results can help a case study to strengthen its internal validity. If the case study ...
Following this, we describe thematic analysis in more detail, including why we believe it is a particularly useful analytic approach to consider when first learning about qualitative analysis. We share seven common practices or important considerations for carrying out a thematic analysis and conclude by highlighting key considerations for ...
Case studies tend to focus on qualitative data using methods such as interviews, observations, and analysis of primary and secondary sources (e.g., newspaper articles, photographs, official records). Sometimes a case study will also collect quantitative data.
QDA Method #3: Discourse Analysis. Discourse is simply a fancy word for written or spoken language or debate. So, discourse analysis is all about analysing language within its social context. In other words, analysing language - such as a conversation, a speech, etc - within the culture and society it takes place.
Case study is also described as a method, given particular approaches used to collect and analyze data. Case study research is conducted by almost every social science discipline: business, education, sociology, psychology. Case study research, with its reliance on multiple sources, is also a natural choice for researchers interested in trans ...
Before writing a case study analysis, it is important to identify a relevant subject and research problem. While preparing to write, the author should critically assess the potential problem and the need for in-depth analysis. ... Briefly reiterating these, indicate whether the data obtained have confirmed the hypothesis, the goal has been ...
ve as a brief refresher to the case study method. As a refresher, the chapter does not fully cover all the options or nuances that you might encounter when customizing your own case study (refer to Yin, 2009a, to obtain a full rendition of the entire method).Besides discussing case study design, data collection, and analysis, the refresher addr.
How to Analyze a Case Study. Analyzing a case study involves a structured approach that simulates real-life scenarios and is key to developing actionable insights. Here is a step-by-step guide adapted from Ellet, W. (2007) to help you effectively analyze a case study: Identify the Type of Case Study
A case study is one of the most commonly used methodologies of social research. This article attempts to look into the various dimensions of a case study research strategy, the different epistemological strands which determine the particular case study type and approach adopted in the field, discusses the factors which can enhance the effectiveness of a case study research, and the debate ...
1. Select a case. Once you identify the problem at hand and come up with questions, identify the case you will focus on. The study can provide insights into the subject at hand, challenge existing assumptions, propose a course of action, and/or open up new areas for further research. 2.
Identify the key problems and issues in the case study. Formulate and include a thesis statement, summarizing the outcome of your analysis in 1-2 sentences. Background. Set the scene: background information, relevant facts, and the most important issues. Demonstrate that you have researched the problems in this case study. Evaluation of the Case
Step 1: With Data Analytics Case Studies, Start by Making Assumptions. Hint: Start by making assumptions and thinking out loud. With this question, focus on coming up with a metric to support the hypothesis. If the question is unclear or if you think you need more information, be sure to ask.
A case study analysis must not merely summarize the case. It should identify key issues and. problems, outline and assess alternative courses of action, and draw appropriate. conclusions. The case ...
Four steps are proposed to assist the novice researcher analyse their data that has been collected using a case study method. The first step proposes the creation of a data repository using basic relational database theory. The second step involves creating codes to identify the respective 'chunks' of data. These resulting codes are then analysed and rationalised. The third step involves ...
Residential location choices are traditionally modelled using factors related to accessibility and socioeconomic environments, neglecting the importance of local street-level conditions. Arguably, this neglect is due to data practices. Today, however, street-level images -- which are highly effective at encoding street-level conditions -- are widely available. Additionally, recent advances in ...