Have a language expert improve your writing
Run a free plagiarism check in 10 minutes, generate accurate citations for free.
- Knowledge Base
- Null and Alternative Hypotheses | Definitions & Examples
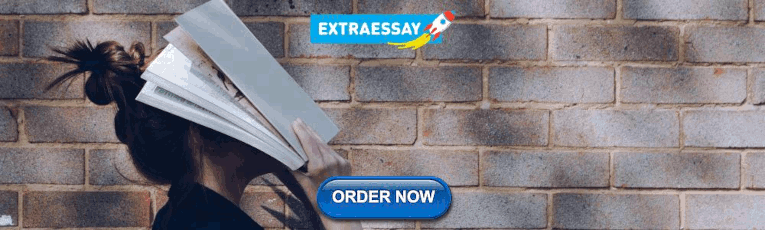
Null & Alternative Hypotheses | Definitions, Templates & Examples
Published on May 6, 2022 by Shaun Turney . Revised on June 22, 2023.
The null and alternative hypotheses are two competing claims that researchers weigh evidence for and against using a statistical test :
- Null hypothesis ( H 0 ): There’s no effect in the population .
- Alternative hypothesis ( H a or H 1 ) : There’s an effect in the population.
Table of contents
Answering your research question with hypotheses, what is a null hypothesis, what is an alternative hypothesis, similarities and differences between null and alternative hypotheses, how to write null and alternative hypotheses, other interesting articles, frequently asked questions.
The null and alternative hypotheses offer competing answers to your research question . When the research question asks “Does the independent variable affect the dependent variable?”:
- The null hypothesis ( H 0 ) answers “No, there’s no effect in the population.”
- The alternative hypothesis ( H a ) answers “Yes, there is an effect in the population.”
The null and alternative are always claims about the population. That’s because the goal of hypothesis testing is to make inferences about a population based on a sample . Often, we infer whether there’s an effect in the population by looking at differences between groups or relationships between variables in the sample. It’s critical for your research to write strong hypotheses .
You can use a statistical test to decide whether the evidence favors the null or alternative hypothesis. Each type of statistical test comes with a specific way of phrasing the null and alternative hypothesis. However, the hypotheses can also be phrased in a general way that applies to any test.
Receive feedback on language, structure, and formatting
Professional editors proofread and edit your paper by focusing on:
- Academic style
- Vague sentences
- Style consistency
See an example
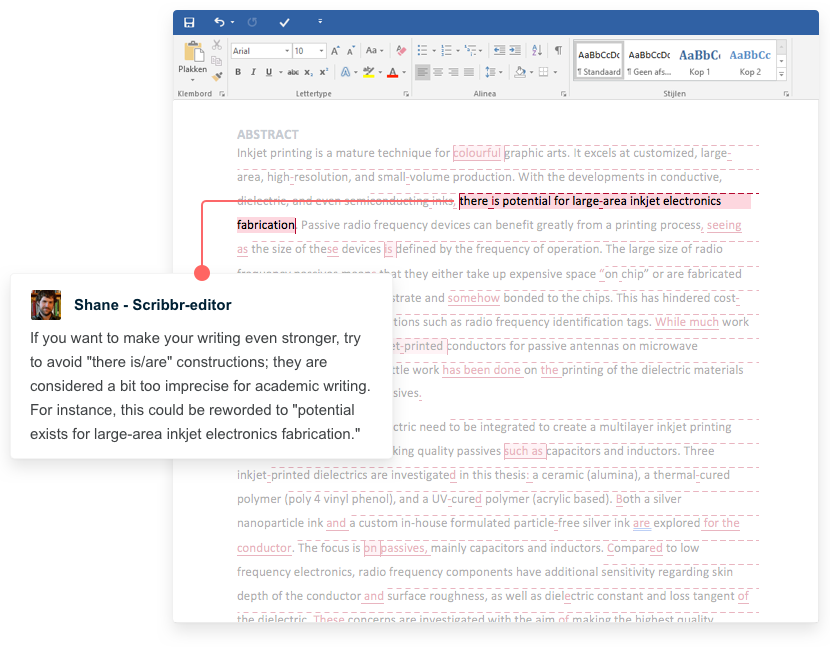
The null hypothesis is the claim that there’s no effect in the population.
If the sample provides enough evidence against the claim that there’s no effect in the population ( p ≤ α), then we can reject the null hypothesis . Otherwise, we fail to reject the null hypothesis.
Although “fail to reject” may sound awkward, it’s the only wording that statisticians accept . Be careful not to say you “prove” or “accept” the null hypothesis.
Null hypotheses often include phrases such as “no effect,” “no difference,” or “no relationship.” When written in mathematical terms, they always include an equality (usually =, but sometimes ≥ or ≤).
You can never know with complete certainty whether there is an effect in the population. Some percentage of the time, your inference about the population will be incorrect. When you incorrectly reject the null hypothesis, it’s called a type I error . When you incorrectly fail to reject it, it’s a type II error.
Examples of null hypotheses
The table below gives examples of research questions and null hypotheses. There’s always more than one way to answer a research question, but these null hypotheses can help you get started.
*Note that some researchers prefer to always write the null hypothesis in terms of “no effect” and “=”. It would be fine to say that daily meditation has no effect on the incidence of depression and p 1 = p 2 .
The alternative hypothesis ( H a ) is the other answer to your research question . It claims that there’s an effect in the population.
Often, your alternative hypothesis is the same as your research hypothesis. In other words, it’s the claim that you expect or hope will be true.
The alternative hypothesis is the complement to the null hypothesis. Null and alternative hypotheses are exhaustive, meaning that together they cover every possible outcome. They are also mutually exclusive, meaning that only one can be true at a time.
Alternative hypotheses often include phrases such as “an effect,” “a difference,” or “a relationship.” When alternative hypotheses are written in mathematical terms, they always include an inequality (usually ≠, but sometimes < or >). As with null hypotheses, there are many acceptable ways to phrase an alternative hypothesis.
Examples of alternative hypotheses
The table below gives examples of research questions and alternative hypotheses to help you get started with formulating your own.
Null and alternative hypotheses are similar in some ways:
- They’re both answers to the research question.
- They both make claims about the population.
- They’re both evaluated by statistical tests.
However, there are important differences between the two types of hypotheses, summarized in the following table.
To help you write your hypotheses, you can use the template sentences below. If you know which statistical test you’re going to use, you can use the test-specific template sentences. Otherwise, you can use the general template sentences.
General template sentences
The only thing you need to know to use these general template sentences are your dependent and independent variables. To write your research question, null hypothesis, and alternative hypothesis, fill in the following sentences with your variables:
Does independent variable affect dependent variable ?
- Null hypothesis ( H 0 ): Independent variable does not affect dependent variable.
- Alternative hypothesis ( H a ): Independent variable affects dependent variable.
Test-specific template sentences
Once you know the statistical test you’ll be using, you can write your hypotheses in a more precise and mathematical way specific to the test you chose. The table below provides template sentences for common statistical tests.
Note: The template sentences above assume that you’re performing one-tailed tests . One-tailed tests are appropriate for most studies.
If you want to know more about statistics , methodology , or research bias , make sure to check out some of our other articles with explanations and examples.
- Normal distribution
- Descriptive statistics
- Measures of central tendency
- Correlation coefficient
Methodology
- Cluster sampling
- Stratified sampling
- Types of interviews
- Cohort study
- Thematic analysis
Research bias
- Implicit bias
- Cognitive bias
- Survivorship bias
- Availability heuristic
- Nonresponse bias
- Regression to the mean
Hypothesis testing is a formal procedure for investigating our ideas about the world using statistics. It is used by scientists to test specific predictions, called hypotheses , by calculating how likely it is that a pattern or relationship between variables could have arisen by chance.
Null and alternative hypotheses are used in statistical hypothesis testing . The null hypothesis of a test always predicts no effect or no relationship between variables, while the alternative hypothesis states your research prediction of an effect or relationship.
The null hypothesis is often abbreviated as H 0 . When the null hypothesis is written using mathematical symbols, it always includes an equality symbol (usually =, but sometimes ≥ or ≤).
The alternative hypothesis is often abbreviated as H a or H 1 . When the alternative hypothesis is written using mathematical symbols, it always includes an inequality symbol (usually ≠, but sometimes < or >).
A research hypothesis is your proposed answer to your research question. The research hypothesis usually includes an explanation (“ x affects y because …”).
A statistical hypothesis, on the other hand, is a mathematical statement about a population parameter. Statistical hypotheses always come in pairs: the null and alternative hypotheses . In a well-designed study , the statistical hypotheses correspond logically to the research hypothesis.
Cite this Scribbr article
If you want to cite this source, you can copy and paste the citation or click the “Cite this Scribbr article” button to automatically add the citation to our free Citation Generator.
Turney, S. (2023, June 22). Null & Alternative Hypotheses | Definitions, Templates & Examples. Scribbr. Retrieved April 15, 2024, from https://www.scribbr.com/statistics/null-and-alternative-hypotheses/
Is this article helpful?
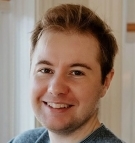
Shaun Turney
Other students also liked, inferential statistics | an easy introduction & examples, hypothesis testing | a step-by-step guide with easy examples, type i & type ii errors | differences, examples, visualizations, what is your plagiarism score.

What Is A Research (Scientific) Hypothesis? A plain-language explainer + examples
By: Derek Jansen (MBA) | Reviewed By: Dr Eunice Rautenbach | June 2020
If you’re new to the world of research, or it’s your first time writing a dissertation or thesis, you’re probably noticing that the words “research hypothesis” and “scientific hypothesis” are used quite a bit, and you’re wondering what they mean in a research context .
“Hypothesis” is one of those words that people use loosely, thinking they understand what it means. However, it has a very specific meaning within academic research. So, it’s important to understand the exact meaning before you start hypothesizing.
Research Hypothesis 101
- What is a hypothesis ?
- What is a research hypothesis (scientific hypothesis)?
- Requirements for a research hypothesis
- Definition of a research hypothesis
- The null hypothesis
What is a hypothesis?
Let’s start with the general definition of a hypothesis (not a research hypothesis or scientific hypothesis), according to the Cambridge Dictionary:
Hypothesis: an idea or explanation for something that is based on known facts but has not yet been proved.
In other words, it’s a statement that provides an explanation for why or how something works, based on facts (or some reasonable assumptions), but that has not yet been specifically tested . For example, a hypothesis might look something like this:
Hypothesis: sleep impacts academic performance.
This statement predicts that academic performance will be influenced by the amount and/or quality of sleep a student engages in – sounds reasonable, right? It’s based on reasonable assumptions , underpinned by what we currently know about sleep and health (from the existing literature). So, loosely speaking, we could call it a hypothesis, at least by the dictionary definition.
But that’s not good enough…
Unfortunately, that’s not quite sophisticated enough to describe a research hypothesis (also sometimes called a scientific hypothesis), and it wouldn’t be acceptable in a dissertation, thesis or research paper . In the world of academic research, a statement needs a few more criteria to constitute a true research hypothesis .
What is a research hypothesis?
A research hypothesis (also called a scientific hypothesis) is a statement about the expected outcome of a study (for example, a dissertation or thesis). To constitute a quality hypothesis, the statement needs to have three attributes – specificity , clarity and testability .
Let’s take a look at these more closely.
Need a helping hand?
Hypothesis Essential #1: Specificity & Clarity
A good research hypothesis needs to be extremely clear and articulate about both what’ s being assessed (who or what variables are involved ) and the expected outcome (for example, a difference between groups, a relationship between variables, etc.).
Let’s stick with our sleepy students example and look at how this statement could be more specific and clear.
Hypothesis: Students who sleep at least 8 hours per night will, on average, achieve higher grades in standardised tests than students who sleep less than 8 hours a night.
As you can see, the statement is very specific as it identifies the variables involved (sleep hours and test grades), the parties involved (two groups of students), as well as the predicted relationship type (a positive relationship). There’s no ambiguity or uncertainty about who or what is involved in the statement, and the expected outcome is clear.
Contrast that to the original hypothesis we looked at – “Sleep impacts academic performance” – and you can see the difference. “Sleep” and “academic performance” are both comparatively vague , and there’s no indication of what the expected relationship direction is (more sleep or less sleep). As you can see, specificity and clarity are key.
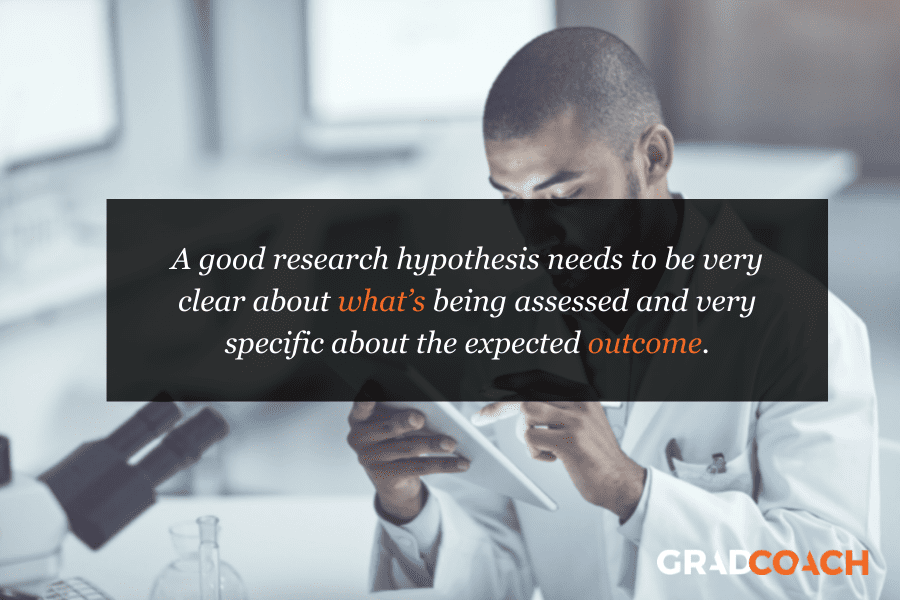
Hypothesis Essential #2: Testability (Provability)
A statement must be testable to qualify as a research hypothesis. In other words, there needs to be a way to prove (or disprove) the statement. If it’s not testable, it’s not a hypothesis – simple as that.
For example, consider the hypothesis we mentioned earlier:
Hypothesis: Students who sleep at least 8 hours per night will, on average, achieve higher grades in standardised tests than students who sleep less than 8 hours a night.
We could test this statement by undertaking a quantitative study involving two groups of students, one that gets 8 or more hours of sleep per night for a fixed period, and one that gets less. We could then compare the standardised test results for both groups to see if there’s a statistically significant difference.
Again, if you compare this to the original hypothesis we looked at – “Sleep impacts academic performance” – you can see that it would be quite difficult to test that statement, primarily because it isn’t specific enough. How much sleep? By who? What type of academic performance?
So, remember the mantra – if you can’t test it, it’s not a hypothesis 🙂
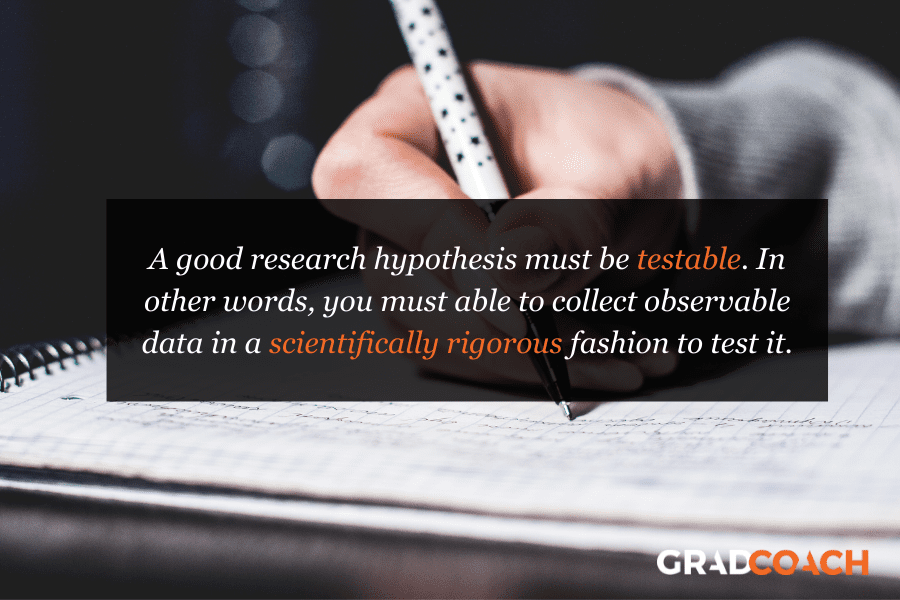
Defining A Research Hypothesis
You’re still with us? Great! Let’s recap and pin down a clear definition of a hypothesis.
A research hypothesis (or scientific hypothesis) is a statement about an expected relationship between variables, or explanation of an occurrence, that is clear, specific and testable.
So, when you write up hypotheses for your dissertation or thesis, make sure that they meet all these criteria. If you do, you’ll not only have rock-solid hypotheses but you’ll also ensure a clear focus for your entire research project.
What about the null hypothesis?
You may have also heard the terms null hypothesis , alternative hypothesis, or H-zero thrown around. At a simple level, the null hypothesis is the counter-proposal to the original hypothesis.
For example, if the hypothesis predicts that there is a relationship between two variables (for example, sleep and academic performance), the null hypothesis would predict that there is no relationship between those variables.
At a more technical level, the null hypothesis proposes that no statistical significance exists in a set of given observations and that any differences are due to chance alone.
And there you have it – hypotheses in a nutshell.
If you have any questions, be sure to leave a comment below and we’ll do our best to help you. If you need hands-on help developing and testing your hypotheses, consider our private coaching service , where we hold your hand through the research journey.
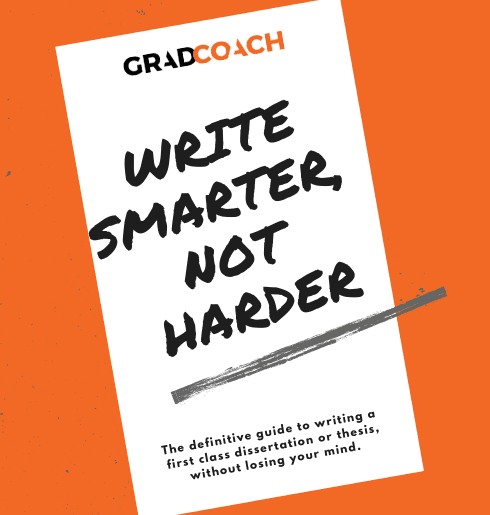
Psst… there’s more (for free)
This post is part of our dissertation mini-course, which covers everything you need to get started with your dissertation, thesis or research project.
You Might Also Like:
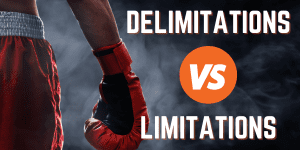
16 Comments
Very useful information. I benefit more from getting more information in this regard.
Very great insight,educative and informative. Please give meet deep critics on many research data of public international Law like human rights, environment, natural resources, law of the sea etc
In a book I read a distinction is made between null, research, and alternative hypothesis. As far as I understand, alternative and research hypotheses are the same. Can you please elaborate? Best Afshin
This is a self explanatory, easy going site. I will recommend this to my friends and colleagues.
Very good definition. How can I cite your definition in my thesis? Thank you. Is nul hypothesis compulsory in a research?
It’s a counter-proposal to be proven as a rejection
Please what is the difference between alternate hypothesis and research hypothesis?
It is a very good explanation. However, it limits hypotheses to statistically tasteable ideas. What about for qualitative researches or other researches that involve quantitative data that don’t need statistical tests?
In qualitative research, one typically uses propositions, not hypotheses.
could you please elaborate it more
I’ve benefited greatly from these notes, thank you.
This is very helpful
well articulated ideas are presented here, thank you for being reliable sources of information
Excellent. Thanks for being clear and sound about the research methodology and hypothesis (quantitative research)
I have only a simple question regarding the null hypothesis. – Is the null hypothesis (Ho) known as the reversible hypothesis of the alternative hypothesis (H1? – How to test it in academic research?
this is very important note help me much more
Trackbacks/Pingbacks
- What Is Research Methodology? Simple Definition (With Examples) - Grad Coach - […] Contrasted to this, a quantitative methodology is typically used when the research aims and objectives are confirmatory in nature. For example,…
Submit a Comment Cancel reply
Your email address will not be published. Required fields are marked *
Save my name, email, and website in this browser for the next time I comment.
- Print Friendly
9.1 Null and Alternative Hypotheses
The actual test begins by considering two hypotheses . They are called the null hypothesis and the alternative hypothesis . These hypotheses contain opposing viewpoints.
H 0 , the — null hypothesis: a statement of no difference between sample means or proportions or no difference between a sample mean or proportion and a population mean or proportion. In other words, the difference equals 0.
H a —, the alternative hypothesis: a claim about the population that is contradictory to H 0 and what we conclude when we reject H 0 .
Since the null and alternative hypotheses are contradictory, you must examine evidence to decide if you have enough evidence to reject the null hypothesis or not. The evidence is in the form of sample data.
After you have determined which hypothesis the sample supports, you make a decision. There are two options for a decision. They are reject H 0 if the sample information favors the alternative hypothesis or do not reject H 0 or decline to reject H 0 if the sample information is insufficient to reject the null hypothesis.
Mathematical Symbols Used in H 0 and H a :
H 0 always has a symbol with an equal in it. H a never has a symbol with an equal in it. The choice of symbol depends on the wording of the hypothesis test. However, be aware that many researchers use = in the null hypothesis, even with > or < as the symbol in the alternative hypothesis. This practice is acceptable because we only make the decision to reject or not reject the null hypothesis.
Example 9.1
H 0 : No more than 30 percent of the registered voters in Santa Clara County voted in the primary election. p ≤ 30 H a : More than 30 percent of the registered voters in Santa Clara County voted in the primary election. p > 30
A medical trial is conducted to test whether or not a new medicine reduces cholesterol by 25 percent. State the null and alternative hypotheses.
Example 9.2
We want to test whether the mean GPA of students in American colleges is different from 2.0 (out of 4.0). The null and alternative hypotheses are the following: H 0 : μ = 2.0 H a : μ ≠ 2.0
We want to test whether the mean height of eighth graders is 66 inches. State the null and alternative hypotheses. Fill in the correct symbol (=, ≠, ≥, <, ≤, >) for the null and alternative hypotheses.
- H 0 : μ __ 66
- H a : μ __ 66
Example 9.3
We want to test if college students take fewer than five years to graduate from college, on the average. The null and alternative hypotheses are the following: H 0 : μ ≥ 5 H a : μ < 5
We want to test if it takes fewer than 45 minutes to teach a lesson plan. State the null and alternative hypotheses. Fill in the correct symbol ( =, ≠, ≥, <, ≤, >) for the null and alternative hypotheses.
- H 0 : μ __ 45
- H a : μ __ 45
Example 9.4
An article on school standards stated that about half of all students in France, Germany, and Israel take advanced placement exams and a third of the students pass. The same article stated that 6.6 percent of U.S. students take advanced placement exams and 4.4 percent pass. Test if the percentage of U.S. students who take advanced placement exams is more than 6.6 percent. State the null and alternative hypotheses. H 0 : p ≤ 0.066 H a : p > 0.066
On a state driver’s test, about 40 percent pass the test on the first try. We want to test if more than 40 percent pass on the first try. Fill in the correct symbol (=, ≠, ≥, <, ≤, >) for the null and alternative hypotheses.
- H 0 : p __ 0.40
- H a : p __ 0.40
Collaborative Exercise
Bring to class a newspaper, some news magazines, and some internet articles. In groups, find articles from which your group can write null and alternative hypotheses. Discuss your hypotheses with the rest of the class.
As an Amazon Associate we earn from qualifying purchases.
This book may not be used in the training of large language models or otherwise be ingested into large language models or generative AI offerings without OpenStax's permission.
Want to cite, share, or modify this book? This book uses the Creative Commons Attribution License and you must attribute Texas Education Agency (TEA). The original material is available at: https://www.texasgateway.org/book/tea-statistics . Changes were made to the original material, including updates to art, structure, and other content updates.
Access for free at https://openstax.org/books/statistics/pages/1-introduction
- Authors: Barbara Illowsky, Susan Dean
- Publisher/website: OpenStax
- Book title: Statistics
- Publication date: Mar 27, 2020
- Location: Houston, Texas
- Book URL: https://openstax.org/books/statistics/pages/1-introduction
- Section URL: https://openstax.org/books/statistics/pages/9-1-null-and-alternative-hypotheses
© Jan 23, 2024 Texas Education Agency (TEA). The OpenStax name, OpenStax logo, OpenStax book covers, OpenStax CNX name, and OpenStax CNX logo are not subject to the Creative Commons license and may not be reproduced without the prior and express written consent of Rice University.
What Is a Hypothesis? (Science)
If...,Then...
Angela Lumsden/Getty Images
- Scientific Method
- Chemical Laws
- Periodic Table
- Projects & Experiments
- Biochemistry
- Physical Chemistry
- Medical Chemistry
- Chemistry In Everyday Life
- Famous Chemists
- Activities for Kids
- Abbreviations & Acronyms
- Weather & Climate
- Ph.D., Biomedical Sciences, University of Tennessee at Knoxville
- B.A., Physics and Mathematics, Hastings College
A hypothesis (plural hypotheses) is a proposed explanation for an observation. The definition depends on the subject.
In science, a hypothesis is part of the scientific method. It is a prediction or explanation that is tested by an experiment. Observations and experiments may disprove a scientific hypothesis, but can never entirely prove one.
In the study of logic, a hypothesis is an if-then proposition, typically written in the form, "If X , then Y ."
In common usage, a hypothesis is simply a proposed explanation or prediction, which may or may not be tested.
Writing a Hypothesis
Most scientific hypotheses are proposed in the if-then format because it's easy to design an experiment to see whether or not a cause and effect relationship exists between the independent variable and the dependent variable . The hypothesis is written as a prediction of the outcome of the experiment.
- Null Hypothesis and Alternative Hypothesis
Statistically, it's easier to show there is no relationship between two variables than to support their connection. So, scientists often propose the null hypothesis . The null hypothesis assumes changing the independent variable will have no effect on the dependent variable.
In contrast, the alternative hypothesis suggests changing the independent variable will have an effect on the dependent variable. Designing an experiment to test this hypothesis can be trickier because there are many ways to state an alternative hypothesis.
For example, consider a possible relationship between getting a good night's sleep and getting good grades. The null hypothesis might be stated: "The number of hours of sleep students get is unrelated to their grades" or "There is no correlation between hours of sleep and grades."
An experiment to test this hypothesis might involve collecting data, recording average hours of sleep for each student and grades. If a student who gets eight hours of sleep generally does better than students who get four hours of sleep or 10 hours of sleep, the hypothesis might be rejected.
But the alternative hypothesis is harder to propose and test. The most general statement would be: "The amount of sleep students get affects their grades." The hypothesis might also be stated as "If you get more sleep, your grades will improve" or "Students who get nine hours of sleep have better grades than those who get more or less sleep."
In an experiment, you can collect the same data, but the statistical analysis is less likely to give you a high confidence limit.
Usually, a scientist starts out with the null hypothesis. From there, it may be possible to propose and test an alternative hypothesis, to narrow down the relationship between the variables.
Example of a Hypothesis
Examples of a hypothesis include:
- If you drop a rock and a feather, (then) they will fall at the same rate.
- Plants need sunlight in order to live. (if sunlight, then life)
- Eating sugar gives you energy. (if sugar, then energy)
- White, Jay D. Research in Public Administration . Conn., 1998.
- Schick, Theodore, and Lewis Vaughn. How to Think about Weird Things: Critical Thinking for a New Age . McGraw-Hill Higher Education, 2002.
- Null Hypothesis Definition and Examples
- Definition of a Hypothesis
- What Are the Elements of a Good Hypothesis?
- Six Steps of the Scientific Method
- What Are Examples of a Hypothesis?
- Understanding Simple vs Controlled Experiments
- Scientific Method Flow Chart
- Scientific Method Vocabulary Terms
- What Is a Testable Hypothesis?
- Null Hypothesis Examples
- What 'Fail to Reject' Means in a Hypothesis Test
- How To Design a Science Fair Experiment
- What Is an Experiment? Definition and Design
- Hypothesis Test for the Difference of Two Population Proportions
- How to Conduct a Hypothesis Test
What is a scientific hypothesis?
It's the initial building block in the scientific method.
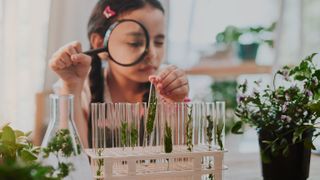
Hypothesis basics
What makes a hypothesis testable.
- Types of hypotheses
- Hypothesis versus theory
Additional resources
Bibliography.
A scientific hypothesis is a tentative, testable explanation for a phenomenon in the natural world. It's the initial building block in the scientific method . Many describe it as an "educated guess" based on prior knowledge and observation. While this is true, a hypothesis is more informed than a guess. While an "educated guess" suggests a random prediction based on a person's expertise, developing a hypothesis requires active observation and background research.
The basic idea of a hypothesis is that there is no predetermined outcome. For a solution to be termed a scientific hypothesis, it has to be an idea that can be supported or refuted through carefully crafted experimentation or observation. This concept, called falsifiability and testability, was advanced in the mid-20th century by Austrian-British philosopher Karl Popper in his famous book "The Logic of Scientific Discovery" (Routledge, 1959).
A key function of a hypothesis is to derive predictions about the results of future experiments and then perform those experiments to see whether they support the predictions.
A hypothesis is usually written in the form of an if-then statement, which gives a possibility (if) and explains what may happen because of the possibility (then). The statement could also include "may," according to California State University, Bakersfield .
Here are some examples of hypothesis statements:
- If garlic repels fleas, then a dog that is given garlic every day will not get fleas.
- If sugar causes cavities, then people who eat a lot of candy may be more prone to cavities.
- If ultraviolet light can damage the eyes, then maybe this light can cause blindness.
A useful hypothesis should be testable and falsifiable. That means that it should be possible to prove it wrong. A theory that can't be proved wrong is nonscientific, according to Karl Popper's 1963 book " Conjectures and Refutations ."
An example of an untestable statement is, "Dogs are better than cats." That's because the definition of "better" is vague and subjective. However, an untestable statement can be reworded to make it testable. For example, the previous statement could be changed to this: "Owning a dog is associated with higher levels of physical fitness than owning a cat." With this statement, the researcher can take measures of physical fitness from dog and cat owners and compare the two.
Types of scientific hypotheses
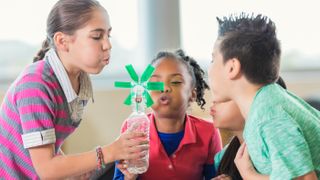
In an experiment, researchers generally state their hypotheses in two ways. The null hypothesis predicts that there will be no relationship between the variables tested, or no difference between the experimental groups. The alternative hypothesis predicts the opposite: that there will be a difference between the experimental groups. This is usually the hypothesis scientists are most interested in, according to the University of Miami .
For example, a null hypothesis might state, "There will be no difference in the rate of muscle growth between people who take a protein supplement and people who don't." The alternative hypothesis would state, "There will be a difference in the rate of muscle growth between people who take a protein supplement and people who don't."
If the results of the experiment show a relationship between the variables, then the null hypothesis has been rejected in favor of the alternative hypothesis, according to the book " Research Methods in Psychology " (BCcampus, 2015).
There are other ways to describe an alternative hypothesis. The alternative hypothesis above does not specify a direction of the effect, only that there will be a difference between the two groups. That type of prediction is called a two-tailed hypothesis. If a hypothesis specifies a certain direction — for example, that people who take a protein supplement will gain more muscle than people who don't — it is called a one-tailed hypothesis, according to William M. K. Trochim , a professor of Policy Analysis and Management at Cornell University.
Sometimes, errors take place during an experiment. These errors can happen in one of two ways. A type I error is when the null hypothesis is rejected when it is true. This is also known as a false positive. A type II error occurs when the null hypothesis is not rejected when it is false. This is also known as a false negative, according to the University of California, Berkeley .
A hypothesis can be rejected or modified, but it can never be proved correct 100% of the time. For example, a scientist can form a hypothesis stating that if a certain type of tomato has a gene for red pigment, that type of tomato will be red. During research, the scientist then finds that each tomato of this type is red. Though the findings confirm the hypothesis, there may be a tomato of that type somewhere in the world that isn't red. Thus, the hypothesis is true, but it may not be true 100% of the time.
Scientific theory vs. scientific hypothesis
The best hypotheses are simple. They deal with a relatively narrow set of phenomena. But theories are broader; they generally combine multiple hypotheses into a general explanation for a wide range of phenomena, according to the University of California, Berkeley . For example, a hypothesis might state, "If animals adapt to suit their environments, then birds that live on islands with lots of seeds to eat will have differently shaped beaks than birds that live on islands with lots of insects to eat." After testing many hypotheses like these, Charles Darwin formulated an overarching theory: the theory of evolution by natural selection.
"Theories are the ways that we make sense of what we observe in the natural world," Tanner said. "Theories are structures of ideas that explain and interpret facts."
- Read more about writing a hypothesis, from the American Medical Writers Association.
- Find out why a hypothesis isn't always necessary in science, from The American Biology Teacher.
- Learn about null and alternative hypotheses, from Prof. Essa on YouTube .
Encyclopedia Britannica. Scientific Hypothesis. Jan. 13, 2022. https://www.britannica.com/science/scientific-hypothesis
Karl Popper, "The Logic of Scientific Discovery," Routledge, 1959.
California State University, Bakersfield, "Formatting a testable hypothesis." https://www.csub.edu/~ddodenhoff/Bio100/Bio100sp04/formattingahypothesis.htm
Karl Popper, "Conjectures and Refutations," Routledge, 1963.
Price, P., Jhangiani, R., & Chiang, I., "Research Methods of Psychology — 2nd Canadian Edition," BCcampus, 2015.
University of Miami, "The Scientific Method" http://www.bio.miami.edu/dana/161/evolution/161app1_scimethod.pdf
William M.K. Trochim, "Research Methods Knowledge Base," https://conjointly.com/kb/hypotheses-explained/
University of California, Berkeley, "Multiple Hypothesis Testing and False Discovery Rate" https://www.stat.berkeley.edu/~hhuang/STAT141/Lecture-FDR.pdf
University of California, Berkeley, "Science at multiple levels" https://undsci.berkeley.edu/article/0_0_0/howscienceworks_19
Sign up for the Live Science daily newsletter now
Get the world’s most fascinating discoveries delivered straight to your inbox.
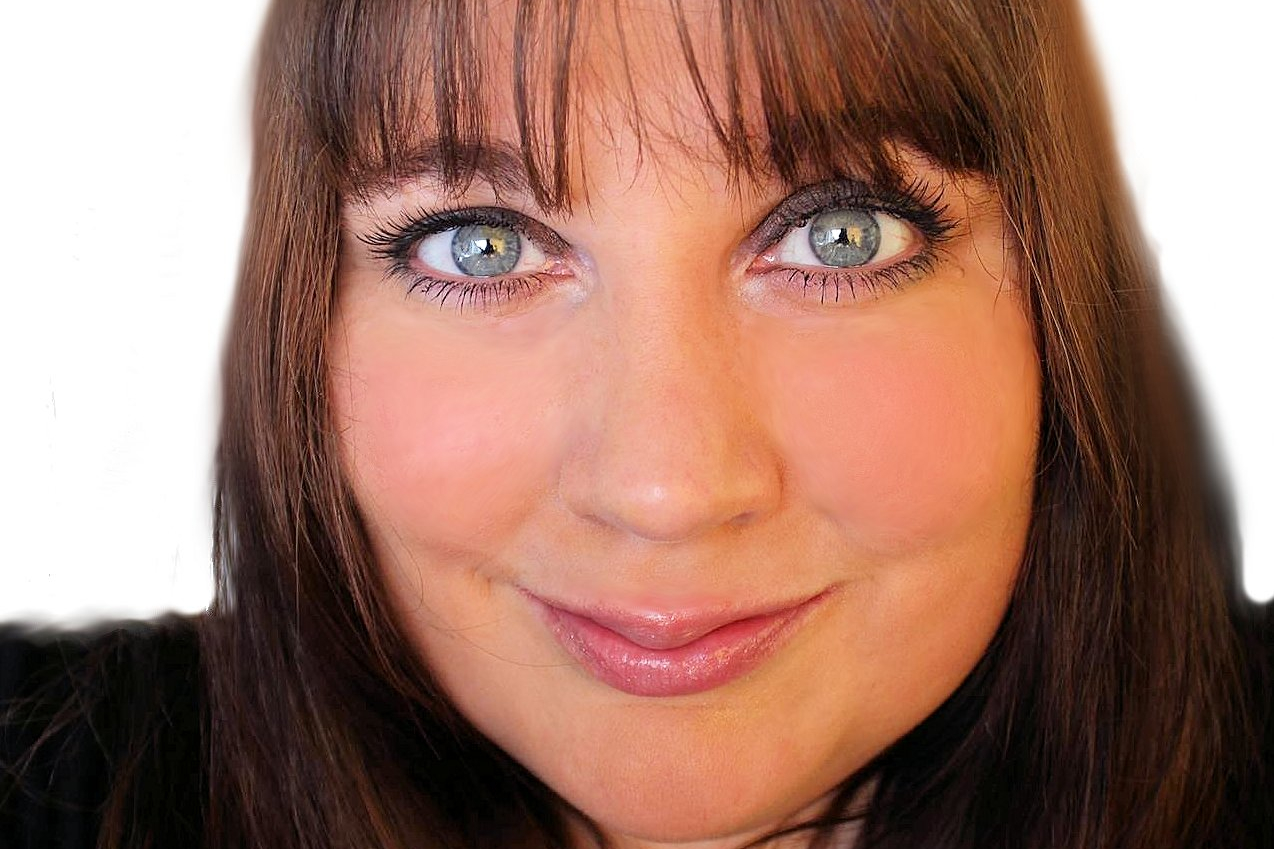
Part of the San Andreas fault may be gearing up for an earthquake
Antarctica is covered in volcanoes, could they erupt?
Tube-tying surgeries and vasectomies skyrocketed post-Roe
Most Popular
- 2 'Exceptional' prosthesis of gold, silver and wool helped 18th-century man live with cleft palate
- 3 Mass die-off half a billion years ago caused by shifting tectonic plates, ancient rocks reveal
- 4 Prehistoric henge accidentally discovered in England in search for Anglo-Saxon hermit
- 5 Car-size asteroid discovered 2 days ago flies by Earth at 1/30th the distance of the moon
- 2 Cancer patients can now be 'matched' to best treatment with DNA and lab-dish experiments
- 3 Space photo of the week: NASA spots enormous pink 'flames' during total solar eclipse. What are they?
- 4 Ancient Indigenous lineage of Blackfoot Confederacy goes back 18,000 years to last ice age, DNA reveals
- Resources Home 🏠
- Try SciSpace Copilot
- Search research papers
- Add Copilot Extension
- Try AI Detector
- Try Paraphraser
- Try Citation Generator
- April Papers
- June Papers
- July Papers

The Craft of Writing a Strong Hypothesis

Table of Contents
Writing a hypothesis is one of the essential elements of a scientific research paper. It needs to be to the point, clearly communicating what your research is trying to accomplish. A blurry, drawn-out, or complexly-structured hypothesis can confuse your readers. Or worse, the editor and peer reviewers.
A captivating hypothesis is not too intricate. This blog will take you through the process so that, by the end of it, you have a better idea of how to convey your research paper's intent in just one sentence.
What is a Hypothesis?
The first step in your scientific endeavor, a hypothesis, is a strong, concise statement that forms the basis of your research. It is not the same as a thesis statement , which is a brief summary of your research paper .
The sole purpose of a hypothesis is to predict your paper's findings, data, and conclusion. It comes from a place of curiosity and intuition . When you write a hypothesis, you're essentially making an educated guess based on scientific prejudices and evidence, which is further proven or disproven through the scientific method.
The reason for undertaking research is to observe a specific phenomenon. A hypothesis, therefore, lays out what the said phenomenon is. And it does so through two variables, an independent and dependent variable.
The independent variable is the cause behind the observation, while the dependent variable is the effect of the cause. A good example of this is “mixing red and blue forms purple.” In this hypothesis, mixing red and blue is the independent variable as you're combining the two colors at your own will. The formation of purple is the dependent variable as, in this case, it is conditional to the independent variable.
Different Types of Hypotheses

Types of hypotheses
Some would stand by the notion that there are only two types of hypotheses: a Null hypothesis and an Alternative hypothesis. While that may have some truth to it, it would be better to fully distinguish the most common forms as these terms come up so often, which might leave you out of context.
Apart from Null and Alternative, there are Complex, Simple, Directional, Non-Directional, Statistical, and Associative and casual hypotheses. They don't necessarily have to be exclusive, as one hypothesis can tick many boxes, but knowing the distinctions between them will make it easier for you to construct your own.
1. Null hypothesis
A null hypothesis proposes no relationship between two variables. Denoted by H 0 , it is a negative statement like “Attending physiotherapy sessions does not affect athletes' on-field performance.” Here, the author claims physiotherapy sessions have no effect on on-field performances. Even if there is, it's only a coincidence.
2. Alternative hypothesis
Considered to be the opposite of a null hypothesis, an alternative hypothesis is donated as H1 or Ha. It explicitly states that the dependent variable affects the independent variable. A good alternative hypothesis example is “Attending physiotherapy sessions improves athletes' on-field performance.” or “Water evaporates at 100 °C. ” The alternative hypothesis further branches into directional and non-directional.
- Directional hypothesis: A hypothesis that states the result would be either positive or negative is called directional hypothesis. It accompanies H1 with either the ‘<' or ‘>' sign.
- Non-directional hypothesis: A non-directional hypothesis only claims an effect on the dependent variable. It does not clarify whether the result would be positive or negative. The sign for a non-directional hypothesis is ‘≠.'
3. Simple hypothesis
A simple hypothesis is a statement made to reflect the relation between exactly two variables. One independent and one dependent. Consider the example, “Smoking is a prominent cause of lung cancer." The dependent variable, lung cancer, is dependent on the independent variable, smoking.
4. Complex hypothesis
In contrast to a simple hypothesis, a complex hypothesis implies the relationship between multiple independent and dependent variables. For instance, “Individuals who eat more fruits tend to have higher immunity, lesser cholesterol, and high metabolism.” The independent variable is eating more fruits, while the dependent variables are higher immunity, lesser cholesterol, and high metabolism.
5. Associative and casual hypothesis
Associative and casual hypotheses don't exhibit how many variables there will be. They define the relationship between the variables. In an associative hypothesis, changing any one variable, dependent or independent, affects others. In a casual hypothesis, the independent variable directly affects the dependent.
6. Empirical hypothesis
Also referred to as the working hypothesis, an empirical hypothesis claims a theory's validation via experiments and observation. This way, the statement appears justifiable and different from a wild guess.
Say, the hypothesis is “Women who take iron tablets face a lesser risk of anemia than those who take vitamin B12.” This is an example of an empirical hypothesis where the researcher the statement after assessing a group of women who take iron tablets and charting the findings.
7. Statistical hypothesis
The point of a statistical hypothesis is to test an already existing hypothesis by studying a population sample. Hypothesis like “44% of the Indian population belong in the age group of 22-27.” leverage evidence to prove or disprove a particular statement.
Characteristics of a Good Hypothesis
Writing a hypothesis is essential as it can make or break your research for you. That includes your chances of getting published in a journal. So when you're designing one, keep an eye out for these pointers:
- A research hypothesis has to be simple yet clear to look justifiable enough.
- It has to be testable — your research would be rendered pointless if too far-fetched into reality or limited by technology.
- It has to be precise about the results —what you are trying to do and achieve through it should come out in your hypothesis.
- A research hypothesis should be self-explanatory, leaving no doubt in the reader's mind.
- If you are developing a relational hypothesis, you need to include the variables and establish an appropriate relationship among them.
- A hypothesis must keep and reflect the scope for further investigations and experiments.
Separating a Hypothesis from a Prediction
Outside of academia, hypothesis and prediction are often used interchangeably. In research writing, this is not only confusing but also incorrect. And although a hypothesis and prediction are guesses at their core, there are many differences between them.
A hypothesis is an educated guess or even a testable prediction validated through research. It aims to analyze the gathered evidence and facts to define a relationship between variables and put forth a logical explanation behind the nature of events.
Predictions are assumptions or expected outcomes made without any backing evidence. They are more fictionally inclined regardless of where they originate from.
For this reason, a hypothesis holds much more weight than a prediction. It sticks to the scientific method rather than pure guesswork. "Planets revolve around the Sun." is an example of a hypothesis as it is previous knowledge and observed trends. Additionally, we can test it through the scientific method.
Whereas "COVID-19 will be eradicated by 2030." is a prediction. Even though it results from past trends, we can't prove or disprove it. So, the only way this gets validated is to wait and watch if COVID-19 cases end by 2030.
Finally, How to Write a Hypothesis
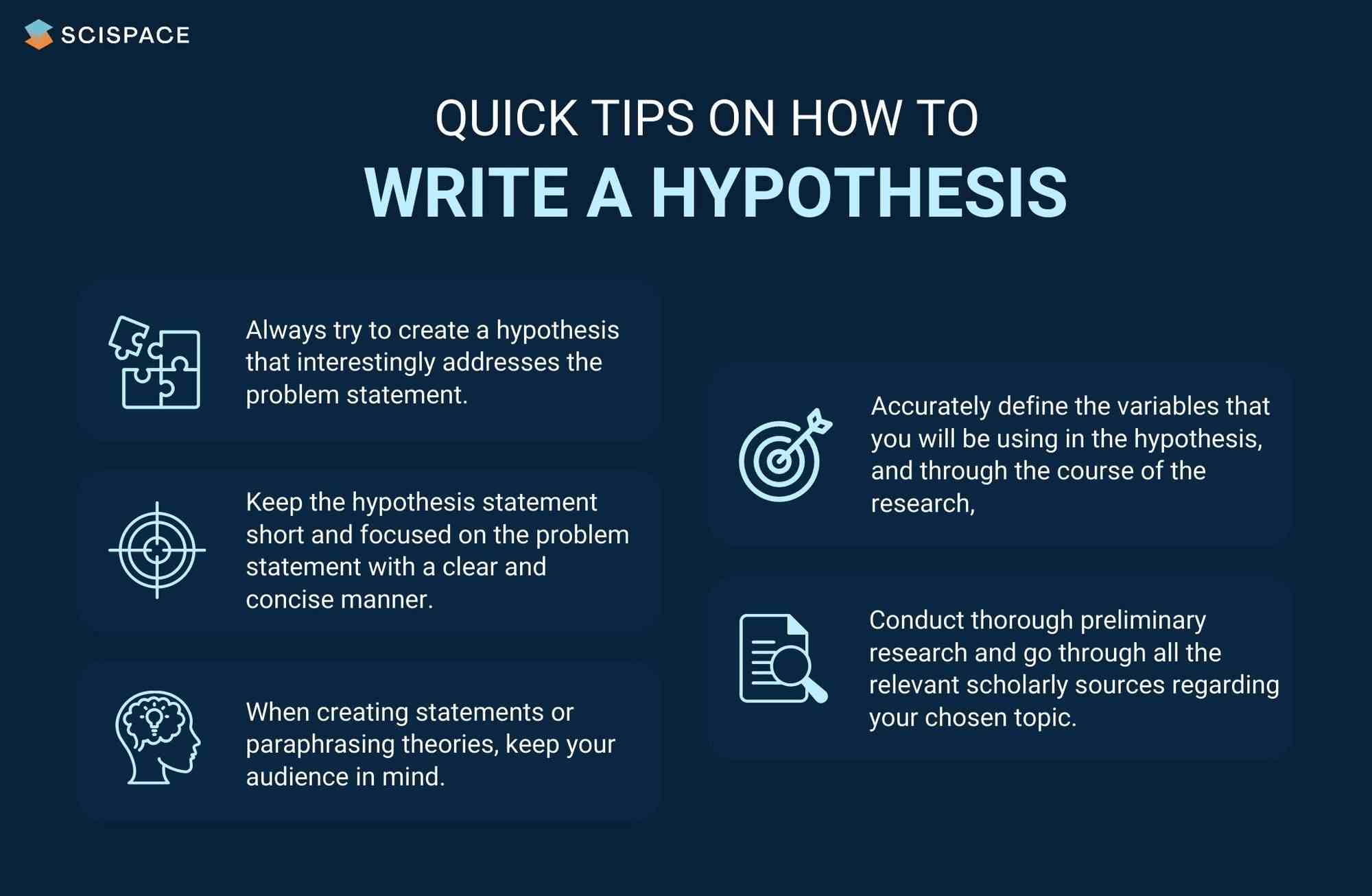
Quick tips on writing a hypothesis
1. Be clear about your research question
A hypothesis should instantly address the research question or the problem statement. To do so, you need to ask a question. Understand the constraints of your undertaken research topic and then formulate a simple and topic-centric problem. Only after that can you develop a hypothesis and further test for evidence.
2. Carry out a recce
Once you have your research's foundation laid out, it would be best to conduct preliminary research. Go through previous theories, academic papers, data, and experiments before you start curating your research hypothesis. It will give you an idea of your hypothesis's viability or originality.
Making use of references from relevant research papers helps draft a good research hypothesis. SciSpace Discover offers a repository of over 270 million research papers to browse through and gain a deeper understanding of related studies on a particular topic. Additionally, you can use SciSpace Copilot , your AI research assistant, for reading any lengthy research paper and getting a more summarized context of it. A hypothesis can be formed after evaluating many such summarized research papers. Copilot also offers explanations for theories and equations, explains paper in simplified version, allows you to highlight any text in the paper or clip math equations and tables and provides a deeper, clear understanding of what is being said. This can improve the hypothesis by helping you identify potential research gaps.
3. Create a 3-dimensional hypothesis
Variables are an essential part of any reasonable hypothesis. So, identify your independent and dependent variable(s) and form a correlation between them. The ideal way to do this is to write the hypothetical assumption in the ‘if-then' form. If you use this form, make sure that you state the predefined relationship between the variables.
In another way, you can choose to present your hypothesis as a comparison between two variables. Here, you must specify the difference you expect to observe in the results.
4. Write the first draft
Now that everything is in place, it's time to write your hypothesis. For starters, create the first draft. In this version, write what you expect to find from your research.
Clearly separate your independent and dependent variables and the link between them. Don't fixate on syntax at this stage. The goal is to ensure your hypothesis addresses the issue.
5. Proof your hypothesis
After preparing the first draft of your hypothesis, you need to inspect it thoroughly. It should tick all the boxes, like being concise, straightforward, relevant, and accurate. Your final hypothesis has to be well-structured as well.
Research projects are an exciting and crucial part of being a scholar. And once you have your research question, you need a great hypothesis to begin conducting research. Thus, knowing how to write a hypothesis is very important.
Now that you have a firmer grasp on what a good hypothesis constitutes, the different kinds there are, and what process to follow, you will find it much easier to write your hypothesis, which ultimately helps your research.
Now it's easier than ever to streamline your research workflow with SciSpace Discover . Its integrated, comprehensive end-to-end platform for research allows scholars to easily discover, write and publish their research and fosters collaboration.
It includes everything you need, including a repository of over 270 million research papers across disciplines, SEO-optimized summaries and public profiles to show your expertise and experience.
If you found these tips on writing a research hypothesis useful, head over to our blog on Statistical Hypothesis Testing to learn about the top researchers, papers, and institutions in this domain.
Frequently Asked Questions (FAQs)
1. what is the definition of hypothesis.
According to the Oxford dictionary, a hypothesis is defined as “An idea or explanation of something that is based on a few known facts, but that has not yet been proved to be true or correct”.
2. What is an example of hypothesis?
The hypothesis is a statement that proposes a relationship between two or more variables. An example: "If we increase the number of new users who join our platform by 25%, then we will see an increase in revenue."
3. What is an example of null hypothesis?
A null hypothesis is a statement that there is no relationship between two variables. The null hypothesis is written as H0. The null hypothesis states that there is no effect. For example, if you're studying whether or not a particular type of exercise increases strength, your null hypothesis will be "there is no difference in strength between people who exercise and people who don't."
4. What are the types of research?
• Fundamental research
• Applied research
• Qualitative research
• Quantitative research
• Mixed research
• Exploratory research
• Longitudinal research
• Cross-sectional research
• Field research
• Laboratory research
• Fixed research
• Flexible research
• Action research
• Policy research
• Classification research
• Comparative research
• Causal research
• Inductive research
• Deductive research
5. How to write a hypothesis?
• Your hypothesis should be able to predict the relationship and outcome.
• Avoid wordiness by keeping it simple and brief.
• Your hypothesis should contain observable and testable outcomes.
• Your hypothesis should be relevant to the research question.
6. What are the 2 types of hypothesis?
• Null hypotheses are used to test the claim that "there is no difference between two groups of data".
• Alternative hypotheses test the claim that "there is a difference between two data groups".
7. Difference between research question and research hypothesis?
A research question is a broad, open-ended question you will try to answer through your research. A hypothesis is a statement based on prior research or theory that you expect to be true due to your study. Example - Research question: What are the factors that influence the adoption of the new technology? Research hypothesis: There is a positive relationship between age, education and income level with the adoption of the new technology.
8. What is plural for hypothesis?
The plural of hypothesis is hypotheses. Here's an example of how it would be used in a statement, "Numerous well-considered hypotheses are presented in this part, and they are supported by tables and figures that are well-illustrated."
9. What is the red queen hypothesis?
The red queen hypothesis in evolutionary biology states that species must constantly evolve to avoid extinction because if they don't, they will be outcompeted by other species that are evolving. Leigh Van Valen first proposed it in 1973; since then, it has been tested and substantiated many times.
10. Who is known as the father of null hypothesis?
The father of the null hypothesis is Sir Ronald Fisher. He published a paper in 1925 that introduced the concept of null hypothesis testing, and he was also the first to use the term itself.
11. When to reject null hypothesis?
You need to find a significant difference between your two populations to reject the null hypothesis. You can determine that by running statistical tests such as an independent sample t-test or a dependent sample t-test. You should reject the null hypothesis if the p-value is less than 0.05.

You might also like

Consensus GPT vs. SciSpace GPT: Choose the Best GPT for Research
Literature Review and Theoretical Framework: Understanding the Differences

Types of Essays in Academic Writing - Quick Guide (2024)
- More from M-W
- To save this word, you'll need to log in. Log In
Definition of hypothesis
Did you know.
The Difference Between Hypothesis and Theory
A hypothesis is an assumption, an idea that is proposed for the sake of argument so that it can be tested to see if it might be true.
In the scientific method, the hypothesis is constructed before any applicable research has been done, apart from a basic background review. You ask a question, read up on what has been studied before, and then form a hypothesis.
A hypothesis is usually tentative; it's an assumption or suggestion made strictly for the objective of being tested.
A theory , in contrast, is a principle that has been formed as an attempt to explain things that have already been substantiated by data. It is used in the names of a number of principles accepted in the scientific community, such as the Big Bang Theory . Because of the rigors of experimentation and control, it is understood to be more likely to be true than a hypothesis is.
In non-scientific use, however, hypothesis and theory are often used interchangeably to mean simply an idea, speculation, or hunch, with theory being the more common choice.
Since this casual use does away with the distinctions upheld by the scientific community, hypothesis and theory are prone to being wrongly interpreted even when they are encountered in scientific contexts—or at least, contexts that allude to scientific study without making the critical distinction that scientists employ when weighing hypotheses and theories.
The most common occurrence is when theory is interpreted—and sometimes even gleefully seized upon—to mean something having less truth value than other scientific principles. (The word law applies to principles so firmly established that they are almost never questioned, such as the law of gravity.)
This mistake is one of projection: since we use theory in general to mean something lightly speculated, then it's implied that scientists must be talking about the same level of uncertainty when they use theory to refer to their well-tested and reasoned principles.
The distinction has come to the forefront particularly on occasions when the content of science curricula in schools has been challenged—notably, when a school board in Georgia put stickers on textbooks stating that evolution was "a theory, not a fact, regarding the origin of living things." As Kenneth R. Miller, a cell biologist at Brown University, has said , a theory "doesn’t mean a hunch or a guess. A theory is a system of explanations that ties together a whole bunch of facts. It not only explains those facts, but predicts what you ought to find from other observations and experiments.”
While theories are never completely infallible, they form the basis of scientific reasoning because, as Miller said "to the best of our ability, we’ve tested them, and they’ve held up."
- proposition
- supposition
hypothesis , theory , law mean a formula derived by inference from scientific data that explains a principle operating in nature.
hypothesis implies insufficient evidence to provide more than a tentative explanation.
theory implies a greater range of evidence and greater likelihood of truth.
law implies a statement of order and relation in nature that has been found to be invariable under the same conditions.
Examples of hypothesis in a Sentence
These examples are programmatically compiled from various online sources to illustrate current usage of the word 'hypothesis.' Any opinions expressed in the examples do not represent those of Merriam-Webster or its editors. Send us feedback about these examples.
Word History
Greek, from hypotithenai to put under, suppose, from hypo- + tithenai to put — more at do
1641, in the meaning defined at sense 1a
Phrases Containing hypothesis
- Whorfian hypothesis
- null hypothesis
- nebular hypothesis
- counter - hypothesis
- planetesimal hypothesis
Articles Related to hypothesis
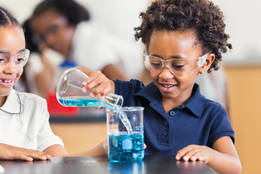
This is the Difference Between a...
This is the Difference Between a Hypothesis and a Theory
In scientific reasoning, they're two completely different things
Dictionary Entries Near hypothesis
hypothermia
hypothesize
Cite this Entry
“Hypothesis.” Merriam-Webster.com Dictionary , Merriam-Webster, https://www.merriam-webster.com/dictionary/hypothesis. Accessed 15 Apr. 2024.
Kids Definition
Kids definition of hypothesis, medical definition, medical definition of hypothesis, more from merriam-webster on hypothesis.
Nglish: Translation of hypothesis for Spanish Speakers
Britannica English: Translation of hypothesis for Arabic Speakers
Britannica.com: Encyclopedia article about hypothesis
Subscribe to America's largest dictionary and get thousands more definitions and advanced search—ad free!
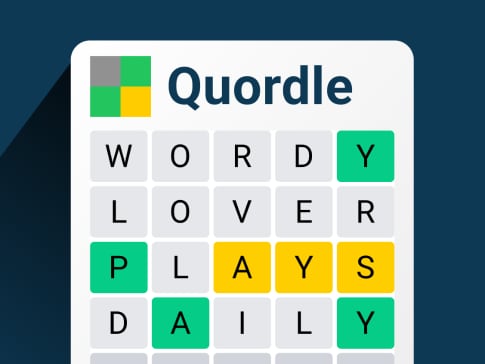
Can you solve 4 words at once?
Word of the day.
See Definitions and Examples »
Get Word of the Day daily email!
Popular in Grammar & Usage
Your vs. you're: how to use them correctly, every letter is silent, sometimes: a-z list of examples, more commonly mispronounced words, how to use em dashes (—), en dashes (–) , and hyphens (-), absent letters that are heard anyway, popular in wordplay, the words of the week - apr. 12, 10 scrabble words without any vowels, 12 more bird names that sound like insults (and sometimes are), 9 superb owl words, 15 words that used to mean something different, games & quizzes.
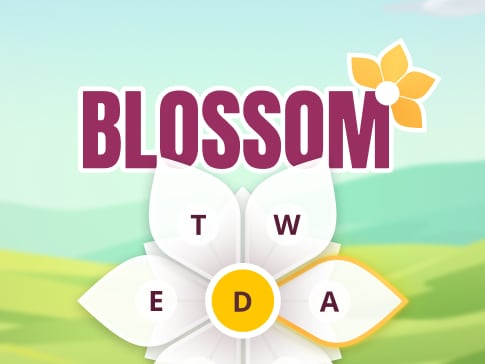

What is Hypothesis? Definition, Meaning, Characteristics, Sources
- Post last modified: 10 January 2022
- Reading time: 18 mins read
- Post category: Research Methodology

- What is Hypothesis?
Hypothesis is a prediction of the outcome of a study. Hypotheses are drawn from theories and research questions or from direct observations. In fact, a research problem can be formulated as a hypothesis. To test the hypothesis we need to formulate it in terms that can actually be analysed with statistical tools.
As an example, if we want to explore whether using a specific teaching method at school will result in better school marks (research question), the hypothesis could be that the mean school marks of students being taught with that specific teaching method will be higher than of those being taught using other methods.
In this example, we stated a hypothesis about the expected differences between groups. Other hypotheses may refer to correlations between variables.
Table of Content
- 1 What is Hypothesis?
- 2 Hypothesis Definition
- 3 Meaning of Hypothesis
- 4.1 Conceptual Clarity
- 4.2 Need of empirical referents
- 4.3 Hypothesis should be specific
- 4.4 Hypothesis should be within the ambit of the available research techniques
- 4.5 Hypothesis should be consistent with the theory
- 4.6 Hypothesis should be concerned with observable facts and empirical events
- 4.7 Hypothesis should be simple
- 5.1 Observation
- 5.2 Analogies
- 5.4 State of Knowledge
- 5.5 Culture
- 5.6 Continuity of Research
- 6.1 Null Hypothesis
- 6.2 Alternative Hypothesis
Thus, to formulate a hypothesis, we need to refer to the descriptive statistics (such as the mean final marks), and specify a set of conditions about these statistics (such as a difference between the means, or in a different example, a positive or negative correlation). The hypothesis we formulate applies to the population of interest.
The null hypothesis makes a statement that no difference exists (see Pyrczak, 1995, pp. 75-84).
Hypothesis Definition
A hypothesis is ‘a guess or supposition as to the existence of some fact or law which will serve to explain a connection of facts already known to exist.’ – J. E. Creighton & H. R. Smart
Hypothesis is ‘a proposition not known to be definitely true or false, examined for the sake of determining the consequences which would follow from its truth.’ – Max Black
Hypothesis is ‘a proposition which can be put to a test to determine validity and is useful for further research.’ – W. J. Goode and P. K. Hatt
A hypothesis is a proposition, condition or principle which is assumed, perhaps without belief, in order to draw out its logical consequences and by this method to test its accord with facts which are known or may be determined. – Webster’s New International Dictionary of the English Language (1956)
Meaning of Hypothesis
From the above mentioned definitions of hypothesis, its meaning can be explained in the following ways.
- At the primary level, a hypothesis is the possible and probable explanation of the sequence of happenings or data.
- Sometimes, hypothesis may emerge from an imagination, common sense or a sudden event.
- Hypothesis can be a probable answer to the research problem undertaken for study. 4. Hypothesis may not always be true. It can get disproven. In other words, hypothesis need not always be a true proposition.
- Hypothesis, in a sense, is an attempt to present the interrelations that exist in the available data or information.
- Hypothesis is not an individual opinion or community thought. Instead, it is a philosophical means which is to be used for research purpose. Hypothesis is not to be considered as the ultimate objective; rather it is to be taken as the means of explaining scientifically the prevailing situation.
The concept of hypothesis can further be explained with the help of some examples. Lord Keynes, in his theory of national income determination, made a hypothesis about the consumption function. He stated that the consumption expenditure of an individual or an economy as a whole is dependent on the level of income and changes in a certain proportion.
Later, this proposition was proved in the statistical research carried out by Prof. Simon Kuznets. Matthus, while studying the population, formulated a hypothesis that population increases faster than the supply of food grains. Population studies of several countries revealed that this hypothesis is true.
Validation of the Malthus’ hypothesis turned it into a theory and when it was tested in many other countries it became the famous Malthus’ Law of Population. It thus emerges that when a hypothesis is tested and proven, it becomes a theory. The theory, when found true in different times and at different places, becomes the law. Having understood the concept of hypothesis, few hypotheses can be formulated in the areas of commerce and economics.
- Population growth moderates with the rise in per capita income.
- Sales growth is positively linked with the availability of credit.
- Commerce education increases the employability of the graduate students.
- High rates of direct taxes prompt people to evade taxes.
- Good working conditions improve the productivity of employees.
- Advertising is the most effecting way of promoting sales than any other scheme.
- Higher Debt-Equity Ratio increases the probability of insolvency.
- Economic reforms in India have made the public sector banks more efficient and competent.
- Foreign direct investment in India has moved in those sectors which offer higher rate of profit.
- There is no significant association between credit rating and investment of fund.
Characteristics of Hypothesis
Not all the hypotheses are good and useful from the point of view of research. It is only a few hypotheses satisfying certain criteria that are good, useful and directive in the research work undertaken. The characteristics of such a useful hypothesis can be listed as below:
Conceptual Clarity
Need of empirical referents, hypothesis should be specific, hypothesis should be within the ambit of the available research techniques, hypothesis should be consistent with the theory, hypothesis should be concerned with observable facts and empirical events, hypothesis should be simple.
The concepts used while framing hypothesis should be crystal clear and unambiguous. Such concepts must be clearly defined so that they become lucid and acceptable to everyone. How are the newly developed concepts interrelated and how are they linked with the old one is to be very clear so that the hypothesis framed on their basis also carries the same clarity.
A hypothesis embodying unclear and ambiguous concepts can to a great extent undermine the successful completion of the research work.
A hypothesis can be useful in the research work undertaken only when it has links with some empirical referents. Hypothesis based on moral values and ideals are useless as they cannot be tested. Similarly, hypothesis containing opinions as good and bad or expectation with respect to something are not testable and therefore useless.
For example, ‘current account deficit can be lowered if people change their attitude towards gold’ is a hypothesis encompassing expectation. In case of such a hypothesis, the attitude towards gold is something which cannot clearly be described and therefore a hypothesis which embodies such an unclean thing cannot be tested and proved or disproved. In short, the hypothesis should be linked with some testable referents.
For the successful conduction of research, it is necessary that the hypothesis is specific and presented in a precise manner. Hypothesis which is general, too ambitious and grandiose in scope is not to be made as such hypothesis cannot be easily put to test. A hypothesis is to be based on such concepts which are precise and empirical in nature. A hypothesis should give a clear idea about the indicators which are to be used.
For example, a hypothesis that economic power is increasingly getting concentrated in a few hands in India should enable us to define the concept of economic power. It should be explicated in terms of measurable indicator like income, wealth, etc. Such specificity in the formulation of a hypothesis ensures that the research is practicable and significant.
While framing the hypothesis, the researcher should be aware of the available research techniques and should see that the hypothesis framed is testable on the basis of them. In other words, a hypothesis should be researchable and for this it is important that a due thought has been given to the methods and techniques which can be used to measure the concepts and variables embodied in the hypothesis.
It does not however mean that hypotheses which are not testable with the available techniques of research are not to be made. If the problem is too significant and therefore the hypothesis framed becomes too ambitious and complex, it’s testing becomes possible with the development of new research techniques or the hypothesis itself leads to the development of new research techniques.
A hypothesis must be related to the existing theory or should have a theoretical orientation. The growth of knowledge takes place in the sequence of facts, hypothesis, theory and law or principles. It means the hypothesis should have a correspondence with the existing facts and theory.
If the hypothesis is related to some theory, the research work will enable us to support, modify or refute the existing theory. Theoretical orientation of the hypothesis ensures that it becomes scientifically useful. According to Prof. Goode and Prof. Hatt, research work can contribute to the existing knowledge only when the hypothesis is related with some theory.
This enables us to explain the observed facts and situations and also verify the framed hypothesis. In the words of Prof. Cohen and Prof. Nagel, “hypothesis must be formulated in such a manner that deduction can be made from it and that consequently a decision can be reached as to whether it does or does not explain the facts considered.”
If the research work based on a hypothesis is to be successful, it is necessary that the later is as simple and easy as possible. An ambition of finding out something new may lead the researcher to frame an unrealistic and unclear hypothesis. Such a temptation is to be avoided. Framing a simple, easy and testable hypothesis requires that the researcher is well acquainted with the related concepts.
Sources of Hypothesis
Hypotheses can be derived from various sources. Some of the sources is given below:
Observation
State of knowledge, continuity of research.
Hypotheses can be derived from observation from the observation of price behavior in a market. For example the relationship between the price and demand for an article is hypothesized.
Analogies are another source of useful hypotheses. Julian Huxley has pointed out that casual observations in nature or in the framework of another science may be a fertile source of hypotheses. For example, the hypotheses that similar human types or activities may be found in similar geophysical regions come from plant ecology.
This is one of the main sources of hypotheses. It gives direction to research by stating what is known logical deduction from theory lead to new hypotheses. For example, profit / wealth maximization is considered as the goal of private enterprises. From this assumption various hypotheses are derived’.
An important source of hypotheses is the state of knowledge in any particular science where formal theories exist hypotheses can be deduced. If the hypotheses are rejected theories are scarce hypotheses are generated from conception frameworks.
Another source of hypotheses is the culture on which the researcher was nurtured. Western culture has induced the emergence of sociology as an academic discipline over the past decade, a large part of the hypotheses on American society examined by researchers were connected with violence. This interest is related to the considerable increase in the level of violence in America.
The continuity of research in a field itself constitutes an important source of hypotheses. The rejection of some hypotheses leads to the formulation of new ones capable of explaining dependent variables in subsequent research on the same subject.
Null and Alternative Hypothesis
Null hypothesis.
The hypothesis that are proposed with the intent of receiving a rejection for them are called Null Hypothesis . This requires that we hypothesize the opposite of what is desired to be proved. For example, if we want to show that sales and advertisement expenditure are related, we formulate the null hypothesis that they are not related.
Similarly, if we want to conclude that the new sales training programme is effective, we formulate the null hypothesis that the new training programme is not effective, and if we want to prove that the average wages of skilled workers in town 1 is greater than that of town 2, we formulate the null hypotheses that there is no difference in the average wages of the skilled workers in both the towns.
Since we hypothesize that sales and advertisement are not related, new training programme is not effective and the average wages of skilled workers in both the towns are equal, we call such hypotheses null hypotheses and denote them as H 0 .
Alternative Hypothesis
Rejection of null hypotheses leads to the acceptance of alternative hypothesis . The rejection of null hypothesis indicates that the relationship between variables (e.g., sales and advertisement expenditure) or the difference between means (e.g., wages of skilled workers in town 1 and town 2) or the difference between proportions have statistical significance and the acceptance of the null hypotheses indicates that these differences are due to chance.
As already mentioned, the alternative hypotheses specify that values/relation which the researcher believes hold true. The alternative hypotheses can cover a whole range of values rather than a single point. The alternative hypotheses are denoted by H 1 .
Business Ethics
( Click on Topic to Read )
- What is Ethics?
- What is Business Ethics?
- Values, Norms, Beliefs and Standards in Business Ethics
- Indian Ethos in Management
- Ethical Issues in Marketing
- Ethical Issues in HRM
- Ethical Issues in IT
- Ethical Issues in Production and Operations Management
- Ethical Issues in Finance and Accounting
- What is Corporate Governance?
- What is Ownership Concentration?
- What is Ownership Composition?
- Types of Companies in India
- Internal Corporate Governance
- External Corporate Governance
- Corporate Governance in India
- What is Enterprise Risk Management (ERM)?
- What is Assessment of Risk?
- What is Risk Register?
- Risk Management Committee
Corporate social responsibility (CSR)
- Theories of CSR
- Arguments Against CSR
- Business Case for CSR
- Importance of CSR in India
- Drivers of Corporate Social Responsibility
- Developing a CSR Strategy
- Implement CSR Commitments
- CSR Marketplace
- CSR at Workplace
- Environmental CSR
- CSR with Communities and in Supply Chain
- Community Interventions
- CSR Monitoring
- CSR Reporting
- Voluntary Codes in CSR
- What is Corporate Ethics?
Lean Six Sigma
- What is Six Sigma?
- What is Lean Six Sigma?
- Value and Waste in Lean Six Sigma
- Six Sigma Team
- MAIC Six Sigma
- Six Sigma in Supply Chains
- What is Binomial, Poisson, Normal Distribution?
- What is Sigma Level?
- What is DMAIC in Six Sigma?
- What is DMADV in Six Sigma?
- Six Sigma Project Charter
- Project Decomposition in Six Sigma
- Critical to Quality (CTQ) Six Sigma
- Process Mapping Six Sigma
- Flowchart and SIPOC
- Gage Repeatability and Reproducibility
- Statistical Diagram
- Lean Techniques for Optimisation Flow
- Failure Modes and Effects Analysis (FMEA)
- What is Process Audits?
- Six Sigma Implementation at Ford
- IBM Uses Six Sigma to Drive Behaviour Change
- Research Methodology
- What is Research?
Sampling Method
- Research Methods
- Data Collection in Research
Methods of Collecting Data
- Application of Business Research
- Levels of Measurement
- What is Sampling?
- Hypothesis Testing
- Research Report
- What is Management?
- Planning in Management
- Decision Making in Management
- What is Controlling?
- What is Coordination?
- What is Staffing?
- Organization Structure
- What is Departmentation?
- Span of Control
- What is Authority?
- Centralization vs Decentralization
- Organizing in Management
- Schools of Management Thought
- Classical Management Approach
- Is Management an Art or Science?
- Who is a Manager?
Operations Research
- What is Operations Research?
- Operation Research Models
- Linear Programming
- Linear Programming Graphic Solution
- Linear Programming Simplex Method
- Linear Programming Artificial Variable Technique
- Duality in Linear Programming
- Transportation Problem Initial Basic Feasible Solution
- Transportation Problem Finding Optimal Solution
- Project Network Analysis with Critical Path Method
- Project Network Analysis Methods
- Project Evaluation and Review Technique (PERT)
- Simulation in Operation Research
- Replacement Models in Operation Research
Operation Management
- What is Strategy?
- What is Operations Strategy?
- Operations Competitive Dimensions
- Operations Strategy Formulation Process
- What is Strategic Fit?
- Strategic Design Process
- Focused Operations Strategy
- Corporate Level Strategy
- Expansion Strategies
- Stability Strategies
- Retrenchment Strategies
- Competitive Advantage
- Strategic Choice and Strategic Alternatives
- What is Production Process?
- What is Process Technology?
- What is Process Improvement?
- Strategic Capacity Management
- Production and Logistics Strategy
- Taxonomy of Supply Chain Strategies
- Factors Considered in Supply Chain Planning
- Operational and Strategic Issues in Global Logistics
- Logistics Outsourcing Strategy
- What is Supply Chain Mapping?
- Supply Chain Process Restructuring
- Points of Differentiation
- Re-engineering Improvement in SCM
- What is Supply Chain Drivers?
- Supply Chain Operations Reference (SCOR) Model
- Customer Service and Cost Trade Off
- Internal and External Performance Measures
- Linking Supply Chain and Business Performance
- Netflix’s Niche Focused Strategy
- Disney and Pixar Merger
- Process Planning at Mcdonald’s
Service Operations Management
- What is Service?
- What is Service Operations Management?
- What is Service Design?
- Service Design Process
- Service Delivery
- What is Service Quality?
- Gap Model of Service Quality
- Juran Trilogy
- Service Performance Measurement
- Service Decoupling
- IT Service Operation
- Service Operations Management in Different Sector
Procurement Management
- What is Procurement Management?
- Procurement Negotiation
- Types of Requisition
- RFX in Procurement
- What is Purchasing Cycle?
- Vendor Managed Inventory
- Internal Conflict During Purchasing Operation
- Spend Analysis in Procurement
- Sourcing in Procurement
- Supplier Evaluation and Selection in Procurement
- Blacklisting of Suppliers in Procurement
- Total Cost of Ownership in Procurement
- Incoterms in Procurement
- Documents Used in International Procurement
- Transportation and Logistics Strategy
- What is Capital Equipment?
- Procurement Process of Capital Equipment
- Acquisition of Technology in Procurement
- What is E-Procurement?
- E-marketplace and Online Catalogues
- Fixed Price and Cost Reimbursement Contracts
- Contract Cancellation in Procurement
- Ethics in Procurement
- Legal Aspects of Procurement
- Global Sourcing in Procurement
- Intermediaries and Countertrade in Procurement
Strategic Management
- What is Strategic Management?
- What is Value Chain Analysis?
- Mission Statement
- Business Level Strategy
- What is SWOT Analysis?
- What is Competitive Advantage?
- What is Vision?
- What is Ansoff Matrix?
- Prahalad and Gary Hammel
- Strategic Management In Global Environment
- Competitor Analysis Framework
- Competitive Rivalry Analysis
- Competitive Dynamics
- What is Competitive Rivalry?
- Five Competitive Forces That Shape Strategy
- What is PESTLE Analysis?
- Fragmentation and Consolidation Of Industries
- What is Technology Life Cycle?
- What is Diversification Strategy?
- What is Corporate Restructuring Strategy?
- Resources and Capabilities of Organization
- Role of Leaders In Functional-Level Strategic Management
- Functional Structure In Functional Level Strategy Formulation
- Information And Control System
- What is Strategy Gap Analysis?
- Issues In Strategy Implementation
- Matrix Organizational Structure
- What is Strategic Management Process?
Supply Chain
- What is Supply Chain Management?
- Supply Chain Planning and Measuring Strategy Performance
- What is Warehousing?
- What is Packaging?
- What is Inventory Management?
- What is Material Handling?
- What is Order Picking?
- Receiving and Dispatch, Processes
- What is Warehouse Design?
- What is Warehousing Costs?
You Might Also Like
What is literature review importance, functions, process,, what is research design features, components, what is research types, purpose, characteristics, process, what is research design types, what is descriptive research types, features, what is experiments variables, types, lab, field, types of charts used in data analysis, what is causal research advantages, disadvantages, how to perform, research process | types, what is measure of dispersion, leave a reply cancel reply.
You must be logged in to post a comment.
World's Best Online Courses at One Place
We’ve spent the time in finding, so you can spend your time in learning
Digital Marketing
Personal growth.
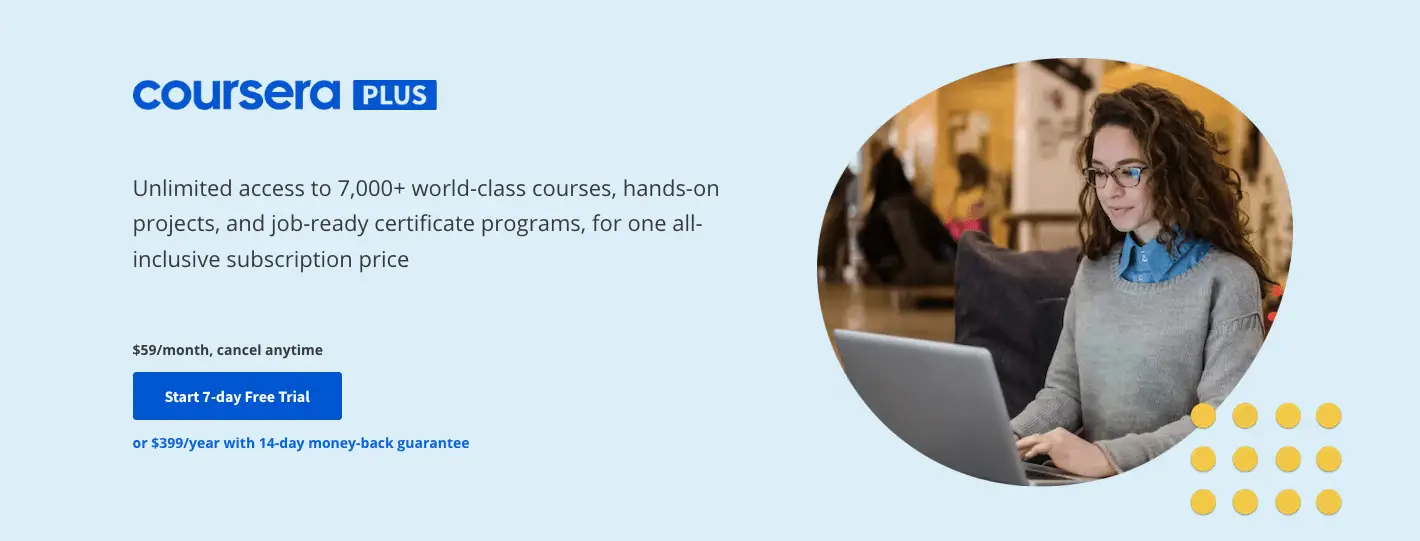
Development

13 Different Types of Hypothesis
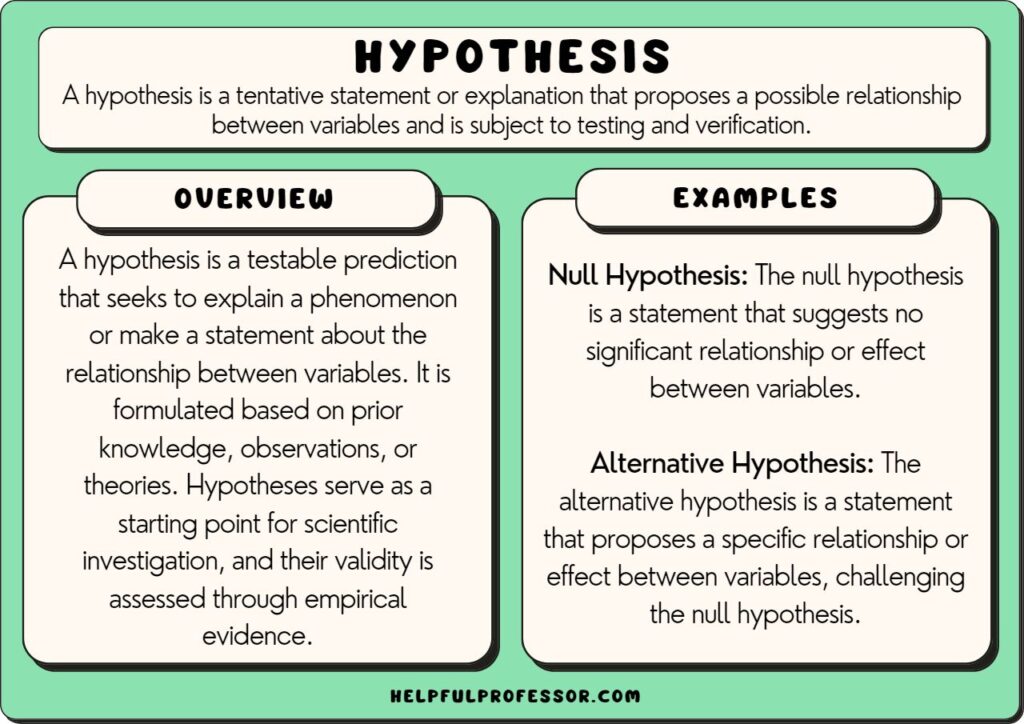
There are 13 different types of hypothesis. These include simple, complex, null, alternative, composite, directional, non-directional, logical, empirical, statistical, associative, exact, and inexact.
A hypothesis can be categorized into one or more of these types. However, some are mutually exclusive and opposites. Simple and complex hypotheses are mutually exclusive, as are direction and non-direction, and null and alternative hypotheses.
Below I explain each hypothesis in simple terms for absolute beginners. These definitions may be too simple for some, but they’re designed to be clear introductions to the terms to help people wrap their heads around the concepts early on in their education about research methods .
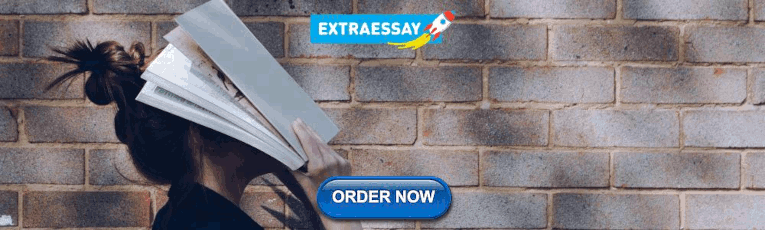
Types of Hypothesis
Before you Proceed: Dependent vs Independent Variables
A research study and its hypotheses generally examine the relationships between independent and dependent variables – so you need to know these two concepts:
- The independent variable is the variable that is causing a change.
- The dependent variable is the variable the is affected by the change. This is the variable being tested.
Read my full article on dependent vs independent variables for more examples.
Example: Eating carrots (independent variable) improves eyesight (dependent variable).
1. Simple Hypothesis
A simple hypothesis is a hypothesis that predicts a correlation between two test variables: an independent and a dependent variable.
This is the easiest and most straightforward type of hypothesis. You simply need to state an expected correlation between the dependant variable and the independent variable.
You do not need to predict causation (see: directional hypothesis). All you would need to do is prove that the two variables are linked.
Simple Hypothesis Examples
2. complex hypothesis.
A complex hypothesis is a hypothesis that contains multiple variables, making the hypothesis more specific but also harder to prove.
You can have multiple independent and dependant variables in this hypothesis.
Complex Hypothesis Example
In the above example, we have multiple independent and dependent variables:
- Independent variables: Age and weight.
- Dependent variables: diabetes and heart disease.
Because there are multiple variables, this study is a lot more complex than a simple hypothesis. It quickly gets much more difficult to prove these hypotheses. This is why undergraduate and first-time researchers are usually encouraged to use simple hypotheses.
3. Null Hypothesis
A null hypothesis will predict that there will be no significant relationship between the two test variables.
For example, you can say that “The study will show that there is no correlation between marriage and happiness.”
A good way to think about a null hypothesis is to think of it in the same way as “innocent until proven guilty”[1]. Unless you can come up with evidence otherwise, your null hypothesis will stand.
A null hypothesis may also highlight that a correlation will be inconclusive . This means that you can predict that the study will not be able to confirm your results one way or the other. For example, you can say “It is predicted that the study will be unable to confirm a correlation between the two variables due to foreseeable interference by a third variable .”
Beware that an inconclusive null hypothesis may be questioned by your teacher. Why would you conduct a test that you predict will not provide a clear result? Perhaps you should take a closer look at your methodology and re-examine it. Nevertheless, inconclusive null hypotheses can sometimes have merit.
Null Hypothesis Examples
4. alternative hypothesis.
An alternative hypothesis is a hypothesis that is anything other than the null hypothesis. It will disprove the null hypothesis.
We use the symbol H A or H 1 to denote an alternative hypothesis.
The null and alternative hypotheses are usually used together. We will say the null hypothesis is the case where a relationship between two variables is non-existent. The alternative hypothesis is the case where there is a relationship between those two variables.
The following statement is always true: H 0 ≠ H A .
Let’s take the example of the hypothesis: “Does eating oatmeal before an exam impact test scores?”
We can have two hypotheses here:
- Null hypothesis (H 0 ): “Eating oatmeal before an exam does not impact test scores.”
- Alternative hypothesis (H A ): “Eating oatmeal before an exam does impact test scores.”
For the alternative hypothesis to be true, all we have to do is disprove the null hypothesis for the alternative hypothesis to be true. We do not need an exact prediction of how much oatmeal will impact the test scores or even if the impact is positive or negative. So long as the null hypothesis is proven to be false, then the alternative hypothesis is proven to be true.
5. Composite Hypothesis
A composite hypothesis is a hypothesis that does not predict the exact parameters, distribution, or range of the dependent variable.
Often, we would predict an exact outcome. For example: “23 year old men are on average 189cm tall.” Here, we are giving an exact parameter. So, the hypothesis is not composite.
But, often, we cannot exactly hypothesize something. We assume that something will happen, but we’re not exactly sure what. In these cases, we might say: “23 year old men are not on average 189cm tall.”
We haven’t set a distribution range or exact parameters of the average height of 23 year old men. So, we’ve introduced a composite hypothesis as opposed to an exact hypothesis.
Generally, an alternative hypothesis (discussed above) is composite because it is defined as anything except the null hypothesis. This ‘anything except’ does not define parameters or distribution, and therefore it’s an example of a composite hypothesis.
6. Directional Hypothesis
A directional hypothesis makes a prediction about the positivity or negativity of the effect of an intervention prior to the test being conducted.
Instead of being agnostic about whether the effect will be positive or negative, it nominates the effect’s directionality.
We often call this a one-tailed hypothesis (in contrast to a two-tailed or non-directional hypothesis) because, looking at a distribution graph, we’re hypothesizing that the results will lean toward one particular tail on the graph – either the positive or negative.
Directional Hypothesis Examples
7. non-directional hypothesis.
A non-directional hypothesis does not specify the predicted direction (e.g. positivity or negativity) of the effect of the independent variable on the dependent variable.
These hypotheses predict an effect, but stop short of saying what that effect will be.
A non-directional hypothesis is similar to composite and alternative hypotheses. All three types of hypothesis tend to make predictions without defining a direction. In a composite hypothesis, a specific prediction is not made (although a general direction may be indicated, so the overlap is not complete). For an alternative hypothesis, you often predict that the even will be anything but the null hypothesis, which means it could be more or less than H 0 (or in other words, non-directional).
Let’s turn the above directional hypotheses into non-directional hypotheses.
Non-Directional Hypothesis Examples
8. logical hypothesis.
A logical hypothesis is a hypothesis that cannot be tested, but has some logical basis underpinning our assumptions.
These are most commonly used in philosophy because philosophical questions are often untestable and therefore we must rely on our logic to formulate logical theories.
Usually, we would want to turn a logical hypothesis into an empirical one through testing if we got the chance. Unfortunately, we don’t always have this opportunity because the test is too complex, expensive, or simply unrealistic.
Here are some examples:
- Before the 1980s, it was hypothesized that the Titanic came to its resting place at 41° N and 49° W, based on the time the ship sank and the ship’s presumed path across the Atlantic Ocean. However, due to the depth of the ocean, it was impossible to test. Thus, the hypothesis was simply a logical hypothesis.
- Dinosaurs closely related to Aligators probably had green scales because Aligators have green scales. However, as they are all extinct, we can only rely on logic and not empirical data.
9. Empirical Hypothesis
An empirical hypothesis is the opposite of a logical hypothesis. It is a hypothesis that is currently being tested using scientific analysis. We can also call this a ‘working hypothesis’.
We can to separate research into two types: theoretical and empirical. Theoretical research relies on logic and thought experiments. Empirical research relies on tests that can be verified by observation and measurement.
So, an empirical hypothesis is a hypothesis that can and will be tested.
- Raising the wage of restaurant servers increases staff retention.
- Adding 1 lb of corn per day to cows’ diets decreases their lifespan.
- Mushrooms grow faster at 22 degrees Celsius than 27 degrees Celsius.
Each of the above hypotheses can be tested, making them empirical rather than just logical (aka theoretical).
10. Statistical Hypothesis
A statistical hypothesis utilizes representative statistical models to draw conclusions about broader populations.
It requires the use of datasets or carefully selected representative samples so that statistical inference can be drawn across a larger dataset.
This type of research is necessary when it is impossible to assess every single possible case. Imagine, for example, if you wanted to determine if men are taller than women. You would be unable to measure the height of every man and woman on the planet. But, by conducting sufficient random samples, you would be able to predict with high probability that the results of your study would remain stable across the whole population.
You would be right in guessing that almost all quantitative research studies conducted in academic settings today involve statistical hypotheses.
Statistical Hypothesis Examples
- Human Sex Ratio. The most famous statistical hypothesis example is that of John Arbuthnot’s sex at birth case study in 1710. Arbuthnot used birth data to determine with high statistical probability that there are more male births than female births. He called this divine providence, and to this day, his findings remain true: more men are born than women.
- Lady Testing Tea. A 1935 study by Ronald Fisher involved testing a woman who believed she could tell whether milk was added before or after water to a cup of tea. Fisher gave her 4 cups in which one randomly had milk placed before the tea. He repeated the test 8 times. The lady was correct each time. Fisher found that she had a 1 in 70 chance of getting all 8 test correct, which is a statistically significant result.
11. Associative Hypothesis
An associative hypothesis predicts that two variables are linked but does not explore whether one variable directly impacts upon the other variable.
We commonly refer to this as “ correlation does not mean causation ”. Just because there are a lot of sick people in a hospital, it doesn’t mean that the hospital made the people sick. There is something going on there that’s causing the issue (sick people are flocking to the hospital).
So, in an associative hypothesis, you note correlation between an independent and dependent variable but do not make a prediction about how the two interact. You stop short of saying one thing causes another thing.
Associative Hypothesis Examples
- Sick people in hospital. You could conduct a study hypothesizing that hospitals have more sick people in them than other institutions in society. However, you don’t hypothesize that the hospitals caused the sickness.
- Lice make you healthy. In the Middle Ages, it was observed that sick people didn’t tend to have lice in their hair. The inaccurate conclusion was that lice was not only a sign of health, but that they made people healthy. In reality, there was an association here, but not causation. The fact was that lice were sensitive to body temperature and fled bodies that had fevers.
12. Causal Hypothesis
A causal hypothesis predicts that two variables are not only associated, but that changes in one variable will cause changes in another.
A causal hypothesis is harder to prove than an associative hypothesis because the cause needs to be definitively proven. This will often require repeating tests in controlled environments with the researchers making manipulations to the independent variable, or the use of control groups and placebo effects .
If we were to take the above example of lice in the hair of sick people, researchers would have to put lice in sick people’s hair and see if it made those people healthier. Researchers would likely observe that the lice would flee the hair, but the sickness would remain, leading to a finding of association but not causation.
Causal Hypothesis Examples
13. exact vs. inexact hypothesis.
For brevity’s sake, I have paired these two hypotheses into the one point. The reality is that we’ve already seen both of these types of hypotheses at play already.
An exact hypothesis (also known as a point hypothesis) specifies a specific prediction whereas an inexact hypothesis assumes a range of possible values without giving an exact outcome. As Helwig [2] argues:
“An “exact” hypothesis specifies the exact value(s) of the parameter(s) of interest, whereas an “inexact” hypothesis specifies a range of possible values for the parameter(s) of interest.”
Generally, a null hypothesis is an exact hypothesis whereas alternative, composite, directional, and non-directional hypotheses are all inexact.
See Next: 15 Hypothesis Examples
This is introductory information that is basic and indeed quite simplified for absolute beginners. It’s worth doing further independent research to get deeper knowledge of research methods and how to conduct an effective research study. And if you’re in education studies, don’t miss out on my list of the best education studies dissertation ideas .
[1] https://jnnp.bmj.com/content/91/6/571.abstract
[2] http://users.stat.umn.edu/~helwig/notes/SignificanceTesting.pdf
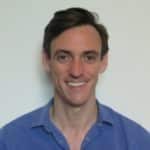
Chris Drew (PhD)
Dr. Chris Drew is the founder of the Helpful Professor. He holds a PhD in education and has published over 20 articles in scholarly journals. He is the former editor of the Journal of Learning Development in Higher Education. [Image Descriptor: Photo of Chris]
- Chris Drew (PhD) https://helpfulprofessor.com/author/chris-drew-phd/ 5 Top Tips for Succeeding at University
- Chris Drew (PhD) https://helpfulprofessor.com/author/chris-drew-phd/ 50 Durable Goods Examples
- Chris Drew (PhD) https://helpfulprofessor.com/author/chris-drew-phd/ 100 Consumer Goods Examples
- Chris Drew (PhD) https://helpfulprofessor.com/author/chris-drew-phd/ 30 Globalization Pros and Cons
2 thoughts on “13 Different Types of Hypothesis”
Wow! This introductionary materials are very helpful. I teach the begginers in research for the first time in my career. The given tips and materials are very helpful. Chris, thank you so much! Excellent materials!
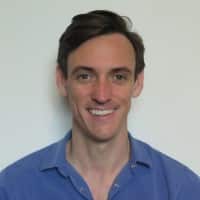
You’re more than welcome! If you want a pdf version of this article to provide for your students to use as a weekly reading on in-class discussion prompt for seminars, just drop me an email in the Contact form and I’ll get one sent out to you.
When I’ve taught this seminar, I’ve put my students into groups, cut these definitions into strips, and handed them out to the groups. Then I get them to try to come up with hypotheses that fit into each ‘type’. You can either just rotate hypothesis types so they get a chance at creating a hypothesis of each type, or get them to “teach” their hypothesis type and examples to the class at the end of the seminar.
Cheers, Chris
Leave a Comment Cancel Reply
Your email address will not be published. Required fields are marked *
Definition : Hypothesis/Also known as
Hypothesis : also known as.
For hypothesis , the word supposition is also found.
The word conjecture is frequently encountered, usually in the context of a statement whose truth value has remained unresolved for a considerable time after the passing of the one who first raised the question.
However, this usage is inconsistent.
The term open question is also encountered, usually in the context in which there is no evidence in either direction as to whether the statement is true or false .
Linguistic Note
The word hypothesis is pronounced hy- po -the-sis , the stress going on the second syllable.
Its plural is hypotheses , which is pronounced hy- po -the-seez .
The word hypothesis comes from the Greek for supposition , literally to put under , that is sub-position .
The idea is that one puts an idea under scrutiny .
The verb hypothesize (British English: hypothesise ) means to make a hypothesis , that is, to suppose .
The adjective hypothetical means having the nature of a hypothesis .
A hypothetical question is a question which relates to a situation that is supposed (or pretended) to be imaginary. One would, for example, announce that a question about to be posed is hypothetical if the questioner wishes to be believed to be at some distance from the possibility of actually being the subject of the question.
- 1989: Ephraim J. Borowski and Jonathan M. Borwein : Dictionary of Mathematics ... (previous) ... (next) : hypothesis : 2.
- 1998: David Nelson : The Penguin Dictionary of Mathematics (2nd ed.) ... (previous) ... (next) : conjecture (hypothesis)
- 2008: David Nelson : The Penguin Dictionary of Mathematics (4th ed.) ... (previous) ... (next) : conjecture (hypothesis)
- Weisstein, Eric W. "Hypothesis." From MathWorld --A Wolfram Web Resource. https://mathworld.wolfram.com/Hypothesis.html
- MathWorld Articles
- Definitions/Hypotheses
Navigation menu
- Scientific Methods
What is Hypothesis?
We have heard of many hypotheses which have led to great inventions in science. Assumptions that are made on the basis of some evidence are known as hypotheses. In this article, let us learn in detail about the hypothesis and the type of hypothesis with examples.
A hypothesis is an assumption that is made based on some evidence. This is the initial point of any investigation that translates the research questions into predictions. It includes components like variables, population and the relation between the variables. A research hypothesis is a hypothesis that is used to test the relationship between two or more variables.
Characteristics of Hypothesis
Following are the characteristics of the hypothesis:
- The hypothesis should be clear and precise to consider it to be reliable.
- If the hypothesis is a relational hypothesis, then it should be stating the relationship between variables.
- The hypothesis must be specific and should have scope for conducting more tests.
- The way of explanation of the hypothesis must be very simple and it should also be understood that the simplicity of the hypothesis is not related to its significance.
Sources of Hypothesis
Following are the sources of hypothesis:
- The resemblance between the phenomenon.
- Observations from past studies, present-day experiences and from the competitors.
- Scientific theories.
- General patterns that influence the thinking process of people.
Types of Hypothesis
There are six forms of hypothesis and they are:
- Simple hypothesis
- Complex hypothesis
- Directional hypothesis
- Non-directional hypothesis
- Null hypothesis
- Associative and casual hypothesis
Simple Hypothesis
It shows a relationship between one dependent variable and a single independent variable. For example – If you eat more vegetables, you will lose weight faster. Here, eating more vegetables is an independent variable, while losing weight is the dependent variable.
Complex Hypothesis
It shows the relationship between two or more dependent variables and two or more independent variables. Eating more vegetables and fruits leads to weight loss, glowing skin, and reduces the risk of many diseases such as heart disease.
Directional Hypothesis
It shows how a researcher is intellectual and committed to a particular outcome. The relationship between the variables can also predict its nature. For example- children aged four years eating proper food over a five-year period are having higher IQ levels than children not having a proper meal. This shows the effect and direction of the effect.
Non-directional Hypothesis
It is used when there is no theory involved. It is a statement that a relationship exists between two variables, without predicting the exact nature (direction) of the relationship.
Null Hypothesis
It provides a statement which is contrary to the hypothesis. It’s a negative statement, and there is no relationship between independent and dependent variables. The symbol is denoted by “H O ”.
Associative and Causal Hypothesis
Associative hypothesis occurs when there is a change in one variable resulting in a change in the other variable. Whereas, the causal hypothesis proposes a cause and effect interaction between two or more variables.
Examples of Hypothesis
Following are the examples of hypotheses based on their types:
- Consumption of sugary drinks every day leads to obesity is an example of a simple hypothesis.
- All lilies have the same number of petals is an example of a null hypothesis.
- If a person gets 7 hours of sleep, then he will feel less fatigue than if he sleeps less. It is an example of a directional hypothesis.
Functions of Hypothesis
Following are the functions performed by the hypothesis:
- Hypothesis helps in making an observation and experiments possible.
- It becomes the start point for the investigation.
- Hypothesis helps in verifying the observations.
- It helps in directing the inquiries in the right direction.
How will Hypothesis help in the Scientific Method?
Researchers use hypotheses to put down their thoughts directing how the experiment would take place. Following are the steps that are involved in the scientific method:
- Formation of question
- Doing background research
- Creation of hypothesis
- Designing an experiment
- Collection of data
- Result analysis
- Summarizing the experiment
- Communicating the results
Frequently Asked Questions – FAQs
What is hypothesis.
A hypothesis is an assumption made based on some evidence.
Give an example of simple hypothesis?
What are the types of hypothesis.
Types of hypothesis are:
- Associative and Casual hypothesis
State true or false: Hypothesis is the initial point of any investigation that translates the research questions into a prediction.
Define complex hypothesis..
A complex hypothesis shows the relationship between two or more dependent variables and two or more independent variables.
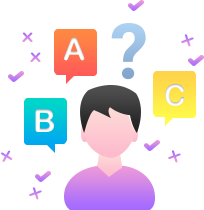
Put your understanding of this concept to test by answering a few MCQs. Click ‘Start Quiz’ to begin!
Select the correct answer and click on the “Finish” button Check your score and answers at the end of the quiz
Visit BYJU’S for all Physics related queries and study materials
Your result is as below
Request OTP on Voice Call
Leave a Comment Cancel reply
Your Mobile number and Email id will not be published. Required fields are marked *
Post My Comment

- Share Share
Register with BYJU'S & Download Free PDFs
Register with byju's & watch live videos.

Sapir–Whorf hypothesis (Linguistic Relativity Hypothesis)
Mia Belle Frothingham
Author, Researcher, Science Communicator
BA with minors in Psychology and Biology, MRes University of Edinburgh
Mia Belle Frothingham is a Harvard University graduate with a Bachelor of Arts in Sciences with minors in biology and psychology
Learn about our Editorial Process
Saul Mcleod, PhD
Editor-in-Chief for Simply Psychology
BSc (Hons) Psychology, MRes, PhD, University of Manchester
Saul Mcleod, PhD., is a qualified psychology teacher with over 18 years of experience in further and higher education. He has been published in peer-reviewed journals, including the Journal of Clinical Psychology.
Olivia Guy-Evans, MSc
Associate Editor for Simply Psychology
BSc (Hons) Psychology, MSc Psychology of Education
Olivia Guy-Evans is a writer and associate editor for Simply Psychology. She has previously worked in healthcare and educational sectors.
On This Page:
There are about seven thousand languages heard around the world – they all have different sounds, vocabularies, and structures. As you know, language plays a significant role in our lives.
But one intriguing question is – can it actually affect how we think?
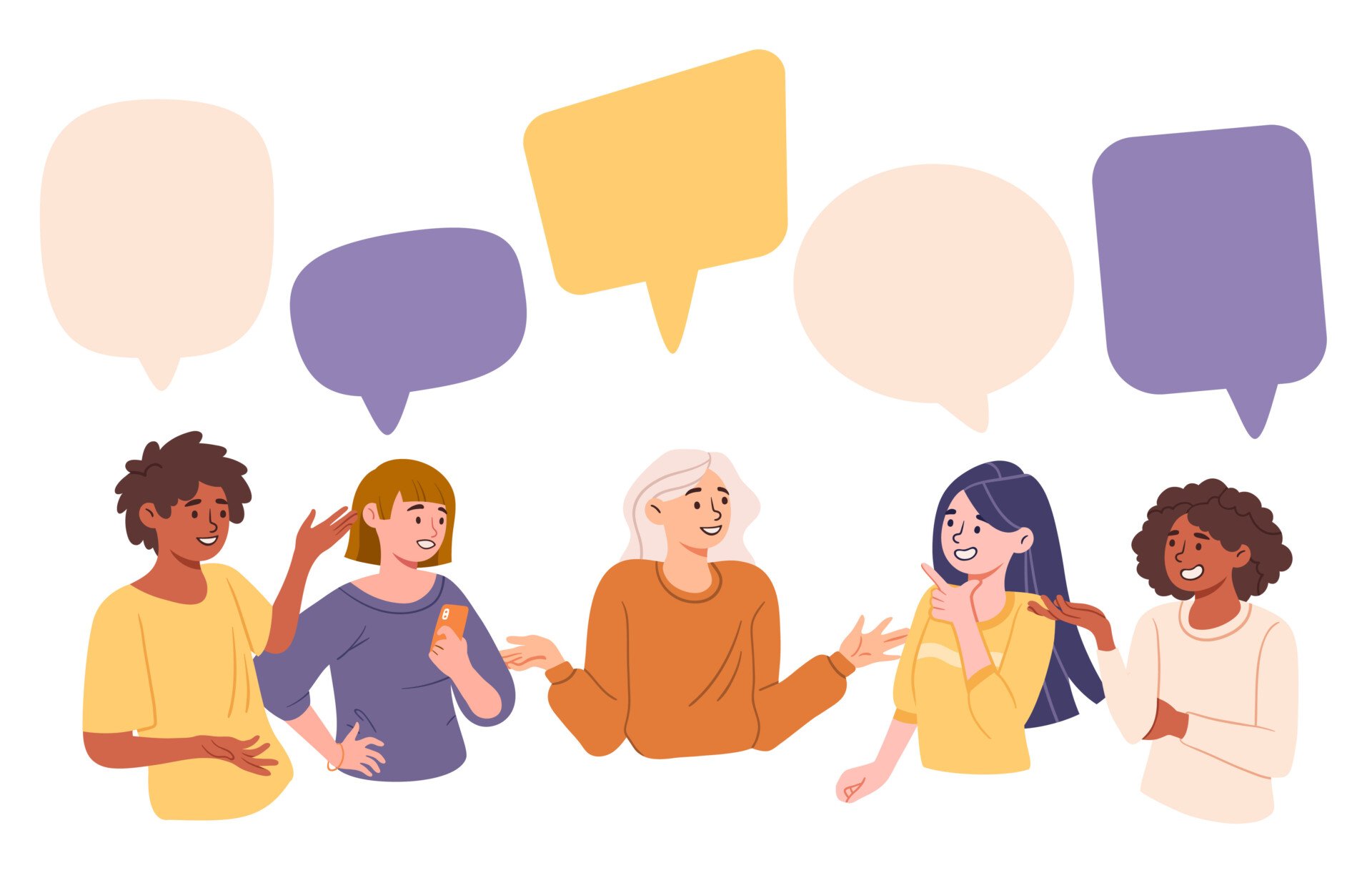
It is widely thought that reality and how one perceives the world is expressed in spoken words and are precisely the same as reality.
That is, perception and expression are understood to be synonymous, and it is assumed that speech is based on thoughts. This idea believes that what one says depends on how the world is encoded and decoded in the mind.
However, many believe the opposite.
In that, what one perceives is dependent on the spoken word. Basically, that thought depends on language, not the other way around.
What Is The Sapir-Whorf Hypothesis?
Twentieth-century linguists Edward Sapir and Benjamin Lee Whorf are known for this very principle and its popularization. Their joint theory, known as the Sapir-Whorf Hypothesis or, more commonly, the Theory of Linguistic Relativity, holds great significance in all scopes of communication theories.
The Sapir-Whorf hypothesis states that the grammatical and verbal structure of a person’s language influences how they perceive the world. It emphasizes that language either determines or influences one’s thoughts.
The Sapir-Whorf hypothesis states that people experience the world based on the structure of their language, and that linguistic categories shape and limit cognitive processes. It proposes that differences in language affect thought, perception, and behavior, so speakers of different languages think and act differently.
For example, different words mean various things in other languages. Not every word in all languages has an exact one-to-one translation in a foreign language.
Because of these small but crucial differences, using the wrong word within a particular language can have significant consequences.
The Sapir-Whorf hypothesis is sometimes called “linguistic relativity” or the “principle of linguistic relativity.” So while they have slightly different names, they refer to the same basic proposal about the relationship between language and thought.
How Language Influences Culture
Culture is defined by the values, norms, and beliefs of a society. Our culture can be considered a lens through which we undergo the world and develop a shared meaning of what occurs around us.
The language that we create and use is in response to the cultural and societal needs that arose. In other words, there is an apparent relationship between how we talk and how we perceive the world.
One crucial question that many intellectuals have asked is how our society’s language influences its culture.
Linguist and anthropologist Edward Sapir and his then-student Benjamin Whorf were interested in answering this question.
Together, they created the Sapir-Whorf hypothesis, which states that our thought processes predominantly determine how we look at the world.
Our language restricts our thought processes – our language shapes our reality. Simply, the language that we use shapes the way we think and how we see the world.
Since the Sapir-Whorf hypothesis theorizes that our language use shapes our perspective of the world, people who speak different languages have different views of the world.
In the 1920s, Benjamin Whorf was a Yale University graduate student studying with linguist Edward Sapir, who was considered the father of American linguistic anthropology.
Sapir was responsible for documenting and recording the cultures and languages of many Native American tribes disappearing at an alarming rate. He and his predecessors were well aware of the close relationship between language and culture.
Anthropologists like Sapir need to learn the language of the culture they are studying to understand the worldview of its speakers truly. Whorf believed that the opposite is also true, that language affects culture by influencing how its speakers think.
His hypothesis proposed that the words and structures of a language influence how its speaker behaves and feels about the world and, ultimately, the culture itself.
Simply put, Whorf believed that you see the world differently from another person who speaks another language due to the specific language you speak.
Human beings do not live in the matter-of-fact world alone, nor solitary in the world of social action as traditionally understood, but are very much at the pardon of the certain language which has become the medium of communication and expression for their society.
To a large extent, the real world is unconsciously built on habits in regard to the language of the group. We hear and see and otherwise experience broadly as we do because the language habits of our community predispose choices of interpretation.
Studies & Examples
The lexicon, or vocabulary, is the inventory of the articles a culture speaks about and has classified to understand the world around them and deal with it effectively.
For example, our modern life is dictated for many by the need to travel by some vehicle – cars, buses, trucks, SUVs, trains, etc. We, therefore, have thousands of words to talk about and mention, including types of models, vehicles, parts, or brands.
The most influential aspects of each culture are similarly reflected in the dictionary of its language. Among the societies living on the islands in the Pacific, fish have significant economic and cultural importance.
Therefore, this is reflected in the rich vocabulary that describes all aspects of the fish and the environments that islanders depend on for survival.
For example, there are over 1,000 fish species in Palau, and Palauan fishers knew, even long before biologists existed, details about the anatomy, behavior, growth patterns, and habitat of most of them – far more than modern biologists know today.
Whorf’s studies at Yale involved working with many Native American languages, including Hopi. He discovered that the Hopi language is quite different from English in many ways, especially regarding time.
Western cultures and languages view times as a flowing river that carries us continuously through the present, away from the past, and to the future.
Our grammar and system of verbs reflect this concept with particular tenses for past, present, and future.
We perceive this concept of time as universal in that all humans see it in the same way.
Although a speaker of Hopi has very different ideas, their language’s structure both reflects and shapes the way they think about time. Seemingly, the Hopi language has no present, past, or future tense; instead, they divide the world into manifested and unmanifest domains.
The manifested domain consists of the physical universe, including the present, the immediate past, and the future; the unmanifest domain consists of the remote past and the future and the world of dreams, thoughts, desires, and life forces.
Also, there are no words for minutes, minutes, or days of the week. Native Hopi speakers often had great difficulty adapting to life in the English-speaking world when it came to being on time for their job or other affairs.
It is due to the simple fact that this was not how they had been conditioned to behave concerning time in their Hopi world, which followed the phases of the moon and the movements of the sun.
Today, it is widely believed that some aspects of perception are affected by language.
One big problem with the original Sapir-Whorf hypothesis derives from the idea that if a person’s language has no word for a specific concept, then that person would not understand that concept.
Honestly, the idea that a mother tongue can restrict one’s understanding has been largely unaccepted. For example, in German, there is a term that means to take pleasure in another person’s unhappiness.
While there is no translatable equivalent in English, it just would not be accurate to say that English speakers have never experienced or would not be able to comprehend this emotion.
Just because there is no word for this in the English language does not mean English speakers are less equipped to feel or experience the meaning of the word.
Not to mention a “chicken and egg” problem with the theory.
Of course, languages are human creations, very much tools we invented and honed to suit our needs. Merely showing that speakers of diverse languages think differently does not tell us whether it is the language that shapes belief or the other way around.
Supporting Evidence
On the other hand, there is hard evidence that the language-associated habits we acquire play a role in how we view the world. And indeed, this is especially true for languages that attach genders to inanimate objects.
There was a study done that looked at how German and Spanish speakers view different things based on their given gender association in each respective language.
The results demonstrated that in describing things that are referred to as masculine in Spanish, speakers of the language marked them as having more male characteristics like “strong” and “long.” Similarly, these same items, which use feminine phrasings in German, were noted by German speakers as effeminate, like “beautiful” and “elegant.”
The findings imply that speakers of each language have developed preconceived notions of something being feminine or masculine, not due to the objects” characteristics or appearances but because of how they are categorized in their native language.
It is important to remember that the Theory of Linguistic Relativity (Sapir-Whorf Hypothesis) also successfully achieves openness. The theory is shown as a window where we view the cognitive process, not as an absolute.
It is set forth to look at a phenomenon differently than one usually would. Furthermore, the Sapir-Whorf Hypothesis is very simple and logically sound. Understandably, one’s atmosphere and culture will affect decoding.
Likewise, in studies done by the authors of the theory, many Native American tribes do not have a word for particular things because they do not exist in their lives. The logical simplism of this idea of relativism provides parsimony.
Truly, the Sapir-Whorf Hypothesis makes sense. It can be utilized in describing great numerous misunderstandings in everyday life. When a Pennsylvanian says “yuns,” it does not make any sense to a Californian, but when examined, it is just another word for “you all.”
The Linguistic Relativity Theory addresses this and suggests that it is all relative. This concept of relativity passes outside dialect boundaries and delves into the world of language – from different countries and, consequently, from mind to mind.
Is language reality honestly because of thought, or is it thought which occurs because of language? The Sapir-Whorf Hypothesis very transparently presents a view of reality being expressed in language and thus forming in thought.
The principles rehashed in it show a reasonable and even simple idea of how one perceives the world, but the question is still arguable: thought then language or language then thought?
Modern Relevance
Regardless of its age, the Sapir-Whorf hypothesis, or the Linguistic Relativity Theory, has continued to force itself into linguistic conversations, even including pop culture.
The idea was just recently revisited in the movie “Arrival,” – a science fiction film that engagingly explores the ways in which an alien language can affect and alter human thinking.
And even if some of the most drastic claims of the theory have been debunked or argued against, the idea has continued its relevance, and that does say something about its importance.
Hypotheses, thoughts, and intellectual musings do not need to be totally accurate to remain in the public eye as long as they make us think and question the world – and the Sapir-Whorf Hypothesis does precisely that.
The theory does not only make us question linguistic theory and our own language but also our very existence and how our perceptions might shape what exists in this world.
There are generalities that we can expect every person to encounter in their day-to-day life – in relationships, love, work, sadness, and so on. But thinking about the more granular disparities experienced by those in diverse circumstances, linguistic or otherwise, helps us realize that there is more to the story than ours.
And beautifully, at the same time, the Sapir-Whorf Hypothesis reiterates the fact that we are more alike than we are different, regardless of the language we speak.
Isn’t it just amazing that linguistic diversity just reveals to us how ingenious and flexible the human mind is – human minds have invented not one cognitive universe but, indeed, seven thousand!
Kay, P., & Kempton, W. (1984). What is the Sapir‐Whorf hypothesis?. American anthropologist, 86(1), 65-79.
Whorf, B. L. (1952). Language, mind, and reality. ETC: A review of general semantics, 167-188.
Whorf, B. L. (1997). The relation of habitual thought and behavior to language. In Sociolinguistics (pp. 443-463). Palgrave, London.
Whorf, B. L. (2012). Language, thought, and reality: Selected writings of Benjamin Lee Whorf. MIT press.


Search site
What is sapir-whorf hypothesis in behavioral science.
The Sapir-Whorf Hypothesis, also known as the linguistic relativity hypothesis, is a theory in linguistics and cognitive science that posits that the structure of a language influences the way its speakers perceive and think about the world. This hypothesis is named after its proponents, American linguists Edward Sapir and Benjamin Lee Whorf, who independently formulated and expanded upon the idea in the early 20th century.
The Sapir-Whorf Hypothesis is commonly divided into two versions:
- Strong version (linguistic determinism): This version asserts that language determines thought, meaning that the way people think is entirely shaped by their language. According to this perspective, speakers of different languages perceive and conceptualize the world in fundamentally different ways due to the unique structures and vocabulary of their languages.
- Weak version (linguistic relativity): This version proposes that language influences, but does not determine, thought. It suggests that while the structure of a language can affect the way its speakers perceive and think about the world, other cognitive factors and experiences also play a significant role in shaping their thoughts and perceptions.
The Sapir-Whorf Hypothesis has generated extensive debate and research, with empirical evidence supporting both its strong and weak versions to varying degrees. Some studies have demonstrated that language can indeed influence cognitive processes such as color perception, spatial reasoning, and time perception. However, other research has challenged the hypothesis, arguing that universal cognitive processes exist independently of language.
Despite the ongoing debate, the Sapir-Whorf Hypothesis has contributed to our understanding of the relationship between language, culture, and cognition. Its implications extend across various disciplines, including anthropology, psychology, sociology, and education, by informing the development of:
- Cross-cultural communication: Recognizing the influence of language on thought can help improve communication and understanding between speakers of different languages and cultural backgrounds.
- Language teaching and learning: The Sapir-Whorf Hypothesis highlights the importance of considering cultural and cognitive factors in language education, as language learning involves not only acquiring new vocabulary and grammar but also adapting to new ways of thinking and perceiving the world.
- Cognitive development research: Investigating the relationship between language and thought can provide insights into cognitive development and the role of linguistic factors in shaping cognitive abilities.
While the Sapir-Whorf Hypothesis remains a subject of debate and investigation, it has significantly impacted our understanding of the complex interplay between language, thought, and culture.
Related Behavioral Science Terms
Belief perseverance, crystallized intelligence, extraneous variable, representative sample, factor analysis, egocentrism, stimulus generalization, reciprocal determinism, divergent thinking, convergent thinking, social environment, decision making, related articles.
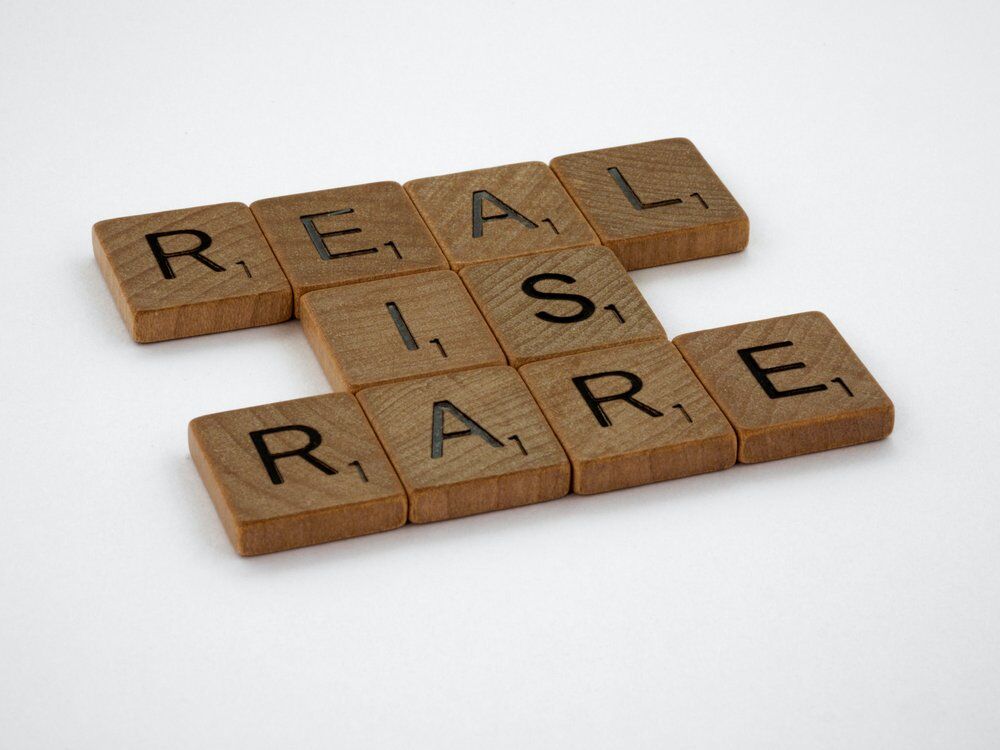
Default Nudges: Fake Behavior Change
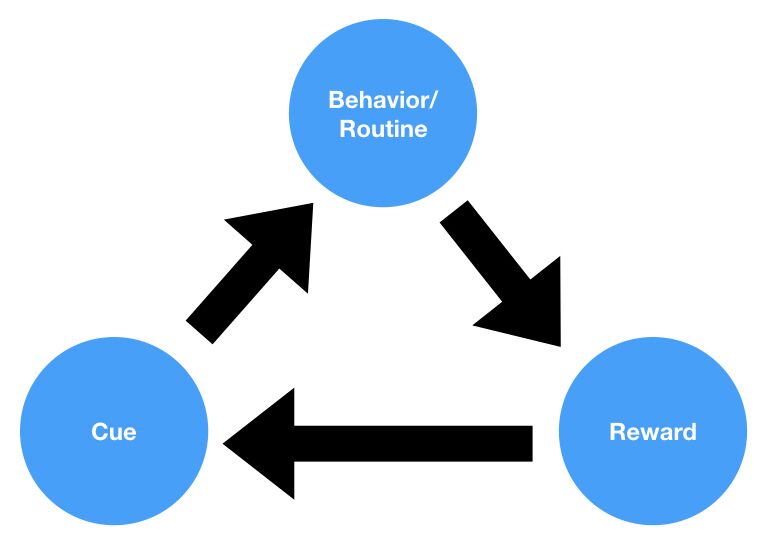
Here’s Why the Loop is Stupid
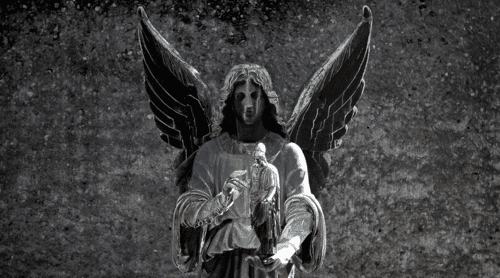
How behavioral science can be used to build the perfect brand
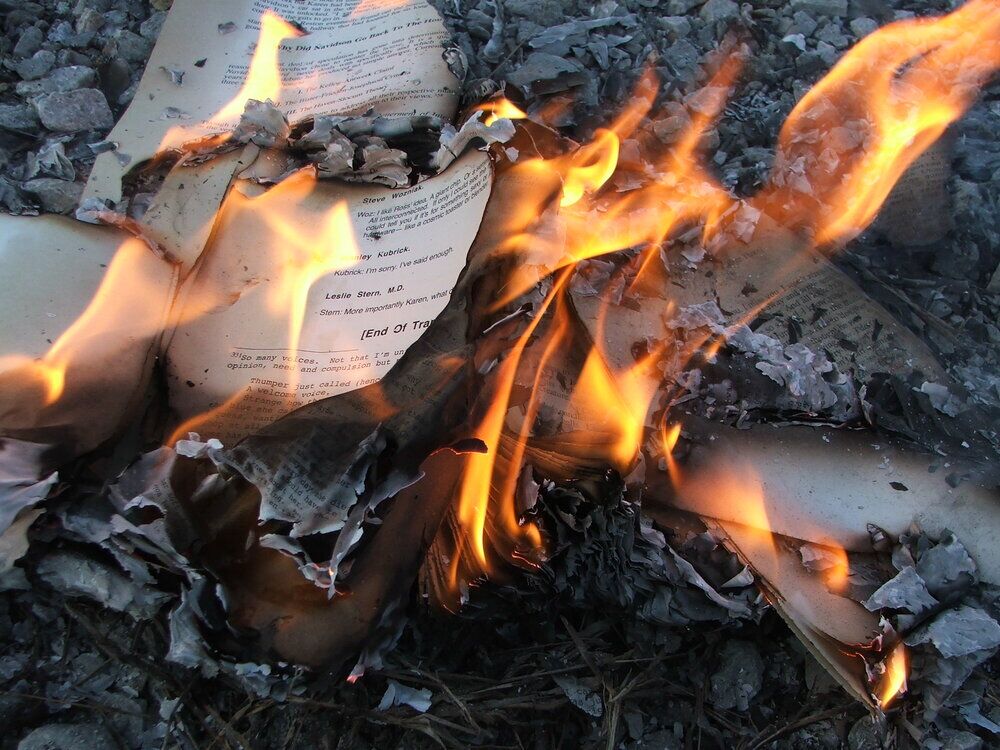
The Death Of Behavioral Economics
- Bipolar Disorder
- Therapy Center
- When To See a Therapist
- Types of Therapy
- Best Online Therapy
- Best Couples Therapy
- Best Family Therapy
- Managing Stress
- Sleep and Dreaming
- Understanding Emotions
- Self-Improvement
- Healthy Relationships
- Student Resources
- Personality Types
- Guided Meditations
- Verywell Mind Insights
- 2023 Verywell Mind 25
- Mental Health in the Classroom
- Editorial Process
- Meet Our Review Board
- Crisis Support
The Sapir-Whorf Hypothesis: How Language Influences How We Express Ourselves
Rachael is a New York-based writer and freelance writer for Verywell Mind, where she leverages her decades of personal experience with and research on mental illness—particularly ADHD and depression—to help readers better understand how their mind works and how to manage their mental health.
:max_bytes(150000):strip_icc():format(webp)/RachaelGreenProfilePicture1-a3b8368ef3bb47ccbac92c5cc088e24d.jpg)
Thomas Barwick / Getty Images
What to Know About the Sapir-Whorf Hypothesis
Real-world examples of linguistic relativity, linguistic relativity in psychology.
The Sapir-Whorf Hypothesis, also known as linguistic relativity, refers to the idea that the language a person speaks can influence their worldview, thought, and even how they experience and understand the world.
While more extreme versions of the hypothesis have largely been discredited, a growing body of research has demonstrated that language can meaningfully shape how we understand the world around us and even ourselves.
Keep reading to learn more about linguistic relativity, including some real-world examples of how it shapes thoughts, emotions, and behavior.
The hypothesis is named after anthropologist and linguist Edward Sapir and his student, Benjamin Lee Whorf. While the hypothesis is named after them both, the two never actually formally co-authored a coherent hypothesis together.
This Hypothesis Aims to Figure Out How Language and Culture Are Connected
Sapir was interested in charting the difference in language and cultural worldviews, including how language and culture influence each other. Whorf took this work on how language and culture shape each other a step further to explore how different languages might shape thought and behavior.
Since then, the concept has evolved into multiple variations, some more credible than others.
Linguistic Determinism Is an Extreme Version of the Hypothesis
Linguistic determinism, for example, is a more extreme version suggesting that a person’s perception and thought are limited to the language they speak. An early example of linguistic determinism comes from Whorf himself who argued that the Hopi people in Arizona don’t conjugate verbs into past, present, and future tenses as English speakers do and that their words for units of time (like “day” or “hour”) were verbs rather than nouns.
From this, he concluded that the Hopi don’t view time as a physical object that can be counted out in minutes and hours the way English speakers do. Instead, Whorf argued, the Hopi view time as a formless process.
This was then taken by others to mean that the Hopi don’t have any concept of time—an extreme view that has since been repeatedly disproven.
There is some evidence for a more nuanced version of linguistic relativity, which suggests that the structure and vocabulary of the language you speak can influence how you understand the world around you. To understand this better, it helps to look at real-world examples of the effects language can have on thought and behavior.
Different Languages Express Colors Differently
Color is one of the most common examples of linguistic relativity. Most known languages have somewhere between two and twelve color terms, and the way colors are categorized varies widely. In English, for example, there are distinct categories for blue and green .
Blue and Green
But in Korean, there is one word that encompasses both. This doesn’t mean Korean speakers can’t see blue, it just means blue is understood as a variant of green rather than a distinct color category all its own.
In Russian, meanwhile, the colors that English speakers would lump under the umbrella term of “blue” are further subdivided into two distinct color categories, “siniy” and “goluboy.” They roughly correspond to light blue and dark blue in English. But to Russian speakers, they are as distinct as orange and brown .
In one study comparing English and Russian speakers, participants were shown a color square and then asked to choose which of the two color squares below it was the closest in shade to the first square.
The test specifically focused on varying shades of blue ranging from “siniy” to “goluboy.” Russian speakers were not only faster at selecting the matching color square but were more accurate in their selections.
The Way Location Is Expressed Varies Across Languages
This same variation occurs in other areas of language. For example, in Guugu Ymithirr, a language spoken by Aboriginal Australians, spatial orientation is always described in absolute terms of cardinal directions. While an English speaker would say the laptop is “in front of” you, a Guugu Ymithirr speaker would say it was north, south, west, or east of you.
As a result, Aboriginal Australians have to be constantly attuned to cardinal directions because their language requires it (just as Russian speakers develop a more instinctive ability to discern between shades of what English speakers call blue because their language requires it).
So when you ask a Guugu Ymithirr speaker to tell you which way south is, they can point in the right direction without a moment’s hesitation. Meanwhile, most English speakers would struggle to accurately identify South without the help of a compass or taking a moment to recall grade school lessons about how to find it.
The concept of these cardinal directions exists in English, but English speakers aren’t required to think about or use them on a daily basis so it’s not as intuitive or ingrained in how they orient themselves in space.
Just as with other aspects of thought and perception, the vocabulary and grammatical structure we have for thinking about or talking about what we feel doesn’t create our feelings, but it does shape how we understand them and, to an extent, how we experience them.
Words Help Us Put a Name to Our Emotions
For example, the ability to detect displeasure from a person’s face is universal. But in a language that has the words “angry” and “sad,” you can further distinguish what kind of displeasure you observe in their facial expression. This doesn’t mean humans never experienced anger or sadness before words for them emerged. But they may have struggled to understand or explain the subtle differences between different dimensions of displeasure.
In one study of English speakers, toddlers were shown a picture of a person with an angry facial expression. Then, they were given a set of pictures of people displaying different expressions including happy, sad, surprised, scared, disgusted, or angry. Researchers asked them to put all the pictures that matched the first angry face picture into a box.
The two-year-olds in the experiment tended to place all faces except happy faces into the box. But four-year-olds were more selective, often leaving out sad or fearful faces as well as happy faces. This suggests that as our vocabulary for talking about emotions expands, so does our ability to understand and distinguish those emotions.
But some research suggests the influence is not limited to just developing a wider vocabulary for categorizing emotions. Language may “also help constitute emotion by cohering sensations into specific perceptions of ‘anger,’ ‘disgust,’ ‘fear,’ etc.,” said Dr. Harold Hong, a board-certified psychiatrist at New Waters Recovery in North Carolina.
As our vocabulary for talking about emotions expands, so does our ability to understand and distinguish those emotions.
Words for emotions, like words for colors, are an attempt to categorize a spectrum of sensations into a handful of distinct categories. And, like color, there’s no objective or hard rule on where the boundaries between emotions should be which can lead to variation across languages in how emotions are categorized.
Emotions Are Categorized Differently in Different Languages
Just as different languages categorize color a little differently, researchers have also found differences in how emotions are categorized. In German, for example, there’s an emotion called “gemütlichkeit.”
While it’s usually translated as “cozy” or “ friendly ” in English, there really isn’t a direct translation. It refers to a particular kind of peace and sense of belonging that a person feels when surrounded by the people they love or feel connected to in a place they feel comfortable and free to be who they are.
Harold Hong, MD, Psychiatrist
The lack of a word for an emotion in a language does not mean that its speakers don't experience that emotion.
You may have felt gemütlichkeit when staying up with your friends to joke and play games at a sleepover. You may feel it when you visit home for the holidays and spend your time eating, laughing, and reminiscing with your family in the house you grew up in.
In Japanese, the word “amae” is just as difficult to translate into English. Usually, it’s translated as "spoiled child" or "presumed indulgence," as in making a request and assuming it will be indulged. But both of those have strong negative connotations in English and amae is a positive emotion .
Instead of being spoiled or coddled, it’s referring to that particular kind of trust and assurance that comes with being nurtured by someone and knowing that you can ask for what you want without worrying whether the other person might feel resentful or burdened by your request.
You might have felt amae when your car broke down and you immediately called your mom to pick you up, without having to worry for even a second whether or not she would drop everything to help you.
Regardless of which languages you speak, though, you’re capable of feeling both of these emotions. “The lack of a word for an emotion in a language does not mean that its speakers don't experience that emotion,” Dr. Hong explained.
What This Means For You
“While having the words to describe emotions can help us better understand and regulate them, it is possible to experience and express those emotions without specific labels for them.” Without the words for these feelings, you can still feel them but you just might not be able to identify them as readily or clearly as someone who does have those words.
Rhee S. Lexicalization patterns in color naming in Korean . In: Raffaelli I, Katunar D, Kerovec B, eds. Studies in Functional and Structural Linguistics. Vol 78. John Benjamins Publishing Company; 2019:109-128. Doi:10.1075/sfsl.78.06rhe
Winawer J, Witthoft N, Frank MC, Wu L, Wade AR, Boroditsky L. Russian blues reveal effects of language on color discrimination . Proc Natl Acad Sci USA. 2007;104(19):7780-7785. 10.1073/pnas.0701644104
Lindquist KA, MacCormack JK, Shablack H. The role of language in emotion: predictions from psychological constructionism . Front Psychol. 2015;6. Doi:10.3389/fpsyg.2015.00444
By Rachael Green Rachael is a New York-based writer and freelance writer for Verywell Mind, where she leverages her decades of personal experience with and research on mental illness—particularly ADHD and depression—to help readers better understand how their mind works and how to manage their mental health.
Riemann zeros as quantized energies of scattering with impurities
- Regular Article - Theoretical Physics
- Open access
- Published: 11 April 2024
- Volume 2024 , article number 62 , ( 2024 )
Cite this article
You have full access to this open access article
- André LeClair ORCID: orcid.org/0000-0002-9242-7778 1 &
- Giuseppe Mussardo ORCID: orcid.org/0000-0001-5730-9963 2
37 Accesses
Explore all metrics
A preprint version of the article is available at arXiv.
We construct an integrable physical model of a single particle scattering with impurities spread on a circle. The S -matrices of the scattering with the impurities are such that the quantized energies of this system, coming from the Bethe Ansatz equations, correspond to the imaginary parts of the non-trivial zeros of the the Riemann ζ ( s ) function along the axis \(\mathfrak{R}\left(s\right)=\frac{1}{2}\) of the complex s -plane. A simple and natural generalization of the original scattering problem leads instead to Bethe Ansatz equations whose solutions are the non-trivial zeros of the Dirichlet L -functions again along the axis \(\mathfrak{R}\left(s\right)=\frac{1}{2}\) . The conjecture that all the non-trivial zeros of these functions are aligned along this axis of the complex s -plane is known as the Generalised Riemann Hypothesis (GRH). In the language of the scattering problem analysed in this paper the validity of the GRH is equivalent to the completeness of the Bethe Ansatz equations. Moreover the idea that the validity of the GRH requires both the duality equation (i.e. the mapping s → 1 – s ) and the Euler product representation of the Dirichlet L -functions finds additional and novel support from the physical scattering model analysed in this paper. This is further illustrated by an explicit counterexample provided by the solutions of the Bethe Ansatz equations which employ the Davenport-Heilbronn function \(\mathcal{D}\left(s\right)\) , i.e. a function whose completion satisfies the duality equation χ ( s ) = χ (1 – s ) but that does not have an Euler product representation. In this case, even though there are infinitely many solutions of the Bethe Ansatz equations along the axis \(\mathfrak{R}\left(s\right)=\frac{1}{2}\) , there are also infinitely many pairs of solutions away from this axis and symmetrically placed with respect to it.
Article PDF
Download to read the full article text
Avoid common mistakes on your manuscript.
H.M. Edwards, Riemann’s Zeta Function , Academic Press, New York (1974).
Google Scholar
E.C. Titchmarsh, The theory of the Riemann zeta function , second edition, Oxford University Press (1988).
P. Borwein, S. Choi, B. Rooney and A. Weirathmueller, The Riemann Hypothesis. A Resource for the Afficionado and Virtuoso Alike , Canadian Mathematical Society, Springer (2008).
Book Google Scholar
B. Conrey, The Riemann Hypothesis , Notices of AMS, March (2003) 341.
T. Apostol, Introduction to analytic number theory , Springer (1976).
D. Schumayer and D.A.W. Hutchinson, Physics of the Riemann Hypothesis , Rev. Mod. Phys. 83 (2011) 307 [ arXiv:1101.3116 ] [ INSPIRE ].
Article ADS Google Scholar
M.V. Berry and J.P. Keating, The Riemann Zeros and Eigenvalue Asymptotics , SIAM Review 41 (1999) 236.
Article ADS MathSciNet Google Scholar
M.V. Berry and J.P. Keating, A compact hamiltonian with the same asymptotic mean spectral density as the Riemann zeros , J. Phys. A 44 (2011) 285203.
Article MathSciNet Google Scholar
G. Sierra, The Riemann zeros as spectrum and the Riemann hypothesis , Symmetry 11 (2019) 494 [ arXiv:1601.01797 ] [ INSPIRE ].
M. Srednicki, Nonclassical Degrees of Freedom in the Riemann Hamiltonian , Phys. Rev. Lett. 107 (2011) 100201 [ arXiv:1105.2342 ] [ INSPIRE ].
C.M. Bender, D.C. Brody and M.P. Müller, Hamiltonian for the zeros of the Riemann zeta function , Phys. Rev. Lett. 118 (2017) 130201 [ arXiv:1608.03679 ] [ INSPIRE ].
G. Mussardo and A. LeClair, Generalized Riemann Hypothesis and Stochastic Time Series , J. Stat. Mech. 06 (2018) 3205 [ arXiv:1803.10223 ] [ INSPIRE ].
A. LeClair and G. Mussardo, Generalized Riemann hypothesis, time series and normal distributions , J. Stat. Mech. 1902 (2019) 023203 [ arXiv:1809.06158 ] [ INSPIRE ].
G. Mussardo and A. LeClair, Randomness of Möbius coefficients and Brownian motion: growth of the Mertens function and the Riemann hypothesis , J. Stat. Mech. 2111 (2021) 113106 [ arXiv:2101.10336 ] [ INSPIRE ].
Article Google Scholar
M.C. Gutzwiller, Stochastic behavior in quantum scattering , Physica D 7 (1983) 341.
G.N. Remmen, Amplitudes and the Riemann Zeta Function , Phys. Rev. Lett. 127 (2021) 241602 [ arXiv:2108.07820 ] [ INSPIRE ].
B. Sutherland, Beautiful Models: 70 years of exactly solved quantum many-body problems , World Scientific, Singapore (2004).
M. Gaudin, The Bethe wave function , Cambridge University Press (2014).
N. Beisert et al., Review of AdS/CFT Integrability: An Overview , Lett. Math. Phys. 99 (2012) 3 [ arXiv:1012.3982 ] [ INSPIRE ].
G. Delfino, G. Mussardo and P. Simonetti, Statistical models with a line of defect , Phys. Lett. B 328 (1994) 123 [ hep-th/9403049 ] [ INSPIRE ].
R. Konik and A. LeClair, Purely transmitting defect field theories , Nucl. Phys. B 538 (1999) 587 [ hep-th/9703085 ] [ INSPIRE ].
P. Bowcock, E. Corrigan and C. Zambon, Classically integrable field theories with defects , Int. J. Mod. Phys. A 19S2 (2004) 82 [ hep-th/0305022 ] [ INSPIRE ].
R.E. Crandall and B.R. Litt, Reassembly and time advance in reflectionless scattering , Annals Phys. 146 (1983) 458.
F. Cooper, A. Khare and U. Sukhatme, Supersymmetry and quantum mechanics , Phys. Rept. 251 (1995) 267 [ hep-th/9405029 ] [ INSPIRE ].
E. Grosswald and F.J. Schnitzer, A class of modified ζ and L-functions , Pacific J. Math. 74 (1978) 375.
G. Mussardo, Statistical Field Theory: An Introduction to Exactly Solved Models in Statistical Physics , Oxford University Press (2010) [ https://doi.org/10.1093/oso/9780198788102.001.0001 ].
C.-N. Yang and C.P. Yang, Thermodynamics of one-dimensional system of bosons with repulsive delta function interaction , J. Math. Phys. 10 (1969) 1115 [ INSPIRE ].
A.B. Zamolodchikov, Thermodynamic Bethe Ansatz in Relativistic Models. Scaling Three State Potts and Lee-yang Models , Nucl. Phys. B 342 (1990) 695 [ INSPIRE ].
A. LeClair, An electrostatic depiction of the validity of the Riemann Hypothesis and a formula for the N-th zero at large N , Int. J. Mod. Phys. A 28 (2013) 1350151 [ arXiv:1305.2613 ] [ INSPIRE ].
G. Franca and A. Leclair, Transcendental equations satisfied by the individual zeros of Riemann ζ, Dirichlet and modular L-functions , Commun. Num. Theor. Phys. 09 (2015) 1 [ arXiv:1502.06003 ] [ INSPIRE ].
H. Bohr and E. Landau, Beiträge zur Theorie der Riemannschen Zetafunktion , Math. Ann. 74 (1913) 3.
T.S. Trudgian, An improved upper bound for the argument of the Riemann zeta-function on the critical line , Math. Comput. 81 (2012) 1053.
D.A. Goldston and S.M. Gonek, A note on S(T) and the zeros of the Riemann zeta-function , Bull. Lond. Math. Soc. 39 (2007) 482 [ math/0511092 ].
A.A. Karatsuba and M.A. Korolev, The argument of the Riemann zeta function , Russ. Math. Surv. 60 (2005) 433.
J.W. Bober and G.A. Hiary, New computations of the Riemann zeta function on the critical line , Exp. Math. 27 (2018) 125 [ arXiv:1607.00709 ].
G.H. Hardy, Sur les Zeros de la Fonction ζ(s) de Riemann , C.R. Acad. Sci. Paris 158 (1914) 1012.
A. Selberg, Contributions to the theory of the Riemann zeta-function , Arch. Math. Naturvid. 48 (1946) 89.
MathSciNet Google Scholar
G. França and A. LeClair, Some Riemann Hypotheses from Random Walks over Primes , arXiv:1509.03643 [ https://doi.org/10.1142/S0219199717500857 ].
A. LeClair, Riemann Hypothesis and Random Walks: The Zeta Case , Symmetry 2021 (2014) 13 [ arXiv:1601.00914 ] [ INSPIRE ].
S.M. Gonek, Finite Euler products and the Riemann Hypothesis , Trans. Am. Math. Soc. 364 (2012) 2157 [ arXiv:0704.3448 ].
H.L. Montgomery, The pair correlation of zeros of the zeta function , in proceedings of Symposium on analytic number theory , St. Louis, Missouri, U.S.A., 27–30 March 1972, Proc. Sympos. Pure Math. 24 (1973) 181.
A.M. Odlyzko, On the distribution of spacings between zeros of the zeta function , Math. Comput. 48 (1987) 273.
H. Davenport and H. Heilbronn, On the Zeros of Certain Dirichlet Series , J. London Math. Soc. 1–11 (1936) 307.
Download references
Acknowledgments
We thank German Sierra and Ghaith Hiary for discussions. AL would like to thank SISSA where this work was started in June 2023. GM acknowledges the grants PNRR MUR Project PE0000023- NQSTI and PRO3 Quantum Pathfinder.
Author information
Authors and affiliations.
Cornell University, Physics Department, Ithaca, NY, 14850, USA
André LeClair
SISSA and INFN, Sezione di Trieste, via Bonomea 265, I-34136, Trieste, Italy
Giuseppe Mussardo
You can also search for this author in PubMed Google Scholar
Corresponding author
Correspondence to Giuseppe Mussardo .
Additional information
Publisher’s note.
Springer Nature remains neutral with regard to jurisdictional claims in published maps and institutional affiliations.
A r X iv e P rint : 2307.01254
Rights and permissions
Open Access . This article is distributed under the terms of the Creative Commons Attribution License ( CC-BY 4.0 ), which permits any use, distribution and reproduction in any medium, provided the original author(s) and source are credited.
Reprints and permissions
About this article
LeClair, A., Mussardo, G. Riemann zeros as quantized energies of scattering with impurities. J. High Energ. Phys. 2024 , 62 (2024). https://doi.org/10.1007/JHEP04(2024)062
Download citation
Received : 12 July 2023
Revised : 15 February 2024
Accepted : 16 March 2024
Published : 11 April 2024
DOI : https://doi.org/10.1007/JHEP04(2024)062
Share this article
Anyone you share the following link with will be able to read this content:
Sorry, a shareable link is not currently available for this article.
Provided by the Springer Nature SharedIt content-sharing initiative
- Bethe Ansatz
- Integrable Field Theories
- Find a journal
- Publish with us
- Track your research
- Open access
- Published: 12 April 2024
Different profiles of soil phosphorous compounds depending on tree species and availability of soil phosphorus in a tropical rainforest in French Guiana
- Albert Gargallo-Garriga 1 , 2 ,
- Jordi Sardans 3 , 4 ,
- Joan Llusià 3 , 4 ,
- Guille Peguero 3 , 4 ,
- Marta Ayala-Roque 2 ,
- Elodie A. Courtois 5 , 6 ,
- Clément Stahl 7 ,
- Otmar Urban 3 ,
- Karel Klem 3 ,
- Pau Nolis 8 ,
- Miriam Pérez-Trujillo 8 ,
- Teodor Parella 8 ,
- Andreas Richter 9 ,
- Ivan A. Janssens 5 &
- Josep Peñuelas 3 , 4
BMC Plant Biology volume 24 , Article number: 278 ( 2024 ) Cite this article
56 Accesses
6 Altmetric
Metrics details
The availability of soil phosphorus (P) often limits the productivities of wet tropical lowland forests. Little is known, however, about the metabolomic profile of different chemical P compounds with potentially different uses and about the cycling of P and their variability across space under different tree species in highly diverse tropical rainforests.
We hypothesised that the different strategies of the competing tree species to retranslocate, mineralise, mobilise, and take up P from the soil would promote distinct soil 31 P profiles. We tested this hypothesis by performing a metabolomic analysis of the soils in two rainforests in French Guiana using 31 P nuclear magnetic resonance (NMR). We analysed 31 P NMR chemical shifts in soil solutions of model P compounds, including inorganic phosphates, orthophosphate mono- and diesters, phosphonates, and organic polyphosphates. The identity of the tree species (growing above the soil samples) explained > 53% of the total variance of the 31 P NMR metabolomic profiles of the soils, suggesting species-specific ecological niches and/or species-specific interactions with the soil microbiome and soil trophic web structure and functionality determining the use and production of P compounds. Differences at regional and topographic levels also explained some part of the the total variance of the 31 P NMR profiles, although less than the influence of the tree species. Multivariate analyses of soil 31 P NMR metabolomics data indicated higher soil concentrations of P biomolecules involved in the active use of P (nucleic acids and molecules involved with energy and anabolism) in soils with lower concentrations of total soil P and higher concentrations of P-storing biomolecules in soils with higher concentrations of total P.
Conclusions
The results strongly suggest “niches” of soil P profiles associated with physical gradients, mostly topographic position, and with the specific distribution of species along this gradient, which is associated with species-specific strategies of soil P mineralisation, mobilisation, use, and uptake.
Peer Review reports
Tropical forests are characterised by high biodiversity and biomass despite growing in strongly weathered soils. All tropical rainforests tend to have high productivity, rapid nutrient turnover, highly weathered soil, and low soil pH [ 1 ]. Tropical regions, such as those in Africa, Asia, and South America, have distinct geological histories that underlie the high biodiversity [ 2 ]. The distribution of plant species and soils are highly variable at local scales within tropical regions [ 3 ]. Several mechanisms have been proposed to explain the coexistence of many plant species in small areas in tropical forest i.e. the high biodiversity observed in tropical forests [ 4 , 5 ]. Many of these mechanisms include heterogeneous disturbances and systems of regeneration [ 6 ]. A diverse topography [ 7 ], species-specific defenses against herbivores [ 8 ], soil traits heterogeneity [ 9 , 10 , 11 ], and differences in nutrient availability [ 10 , 12 , 13 , 14 ] are the other most frequently discussed mechanisms.
The amount of P and its availability limits the productivity of many terrestrial and aquatic ecosystems [ 15 ]. Stocks of total soil P, the chemical forms of P, and P availability in the soil change as ecosystems age and develop, and these transformations can strongly influence ecosystem properties [ 16 , 17 , 18 ]. In particular, biological productivity in wet tropical forests is frequently limited by the availability of soil P [ 19 , 20 ]. Soil taxonomic studies (Soil Survey Staff 2006) classify most tropical forest soils as Oxisols, Ultisols, Alfisols, Inceptisols, or Entisols [ 21 ]. P in these soils usually occurs as organic P and as occluded inorganic forms as part of pedogenic minerals. Occluded forms of P are especially common in older soils (Oxisols, Ultisols, and Alfisols), which frequently have very low or negligible amounts of primary minerals in their profiles and low concentrations of orthophosphate, which is the chemical form of P directly available to plants [ 17 , 22 ]. In contrast, organic P can account for a considerable proportion of total P in tropical mineral soils, accounting for an average of 29 ± 3% of the total P in equatorial forests and in Panama (soil organic P in lowland tropical forests) [ 23 , 24 ].
The turnover of organic P is the primary source of P for microbes and plants (soluble inorganic phosphate ions) in tropical soils [ 25 , 26 ], even though several plant-soil processes can make organic and inorganic/occluded P available to plants, such as mobilisation by roots [ 27 ] or the chemical reduction of iron-phosphate complexes under anaerobic conditions [ 28 ]. Organic P in forest soils is derived from fresh organic matter (leaf litter), microbial biomass, and non-microbial biomass. In general there is evidence suggesting rapid decomposition of leaf litter in the humid tropics (< 1 year; [ 29 ], despite other studies have observed slower rates of leaf litter decomposition (> 1 year) [ 30 ]. Litter nonetheless provides most of the P that supports growth in strongly weathered tropical soils. This phenomenon of “ direct nutrient cycling ” in tropical forests is characterised by the fast release of biologically available phosphate to roots and mycorrhizae by the decomposition of leaf litter and the consequent fast absorption of P by microbes and plants, which is so rapid that the plant-soil P cycle closes, nearly preventing P from being lost via leaching or sorption to iron and aluminium oxides [ 31 ].
Knowledge of the various chemical types of soil organic P and of how plants and microbes use these forms of P is essential to better understand the global cycling and use of P in the plant-soil systems of tropical rainforests. 31 P nuclear magnetic resonance (NMR) is an excellent tool for studying soil organic P because it provides quantitative data and allows the comparison of the various chemical forms of P [ 32 , 33 , 34 , 35 ]. In fact, 31 P NMR allows to discern different molecules where P is present, molecules with different metabolic function such as phosphate mono- and di-esters such as nucleic acids, ATP or acilglyrates involved directly in metabolism pathways, metabolism control and energy transfer from molecules such as orthophosphate, pyrophosphates and polyphosphates, which are involved in P storage. For example, 31 P NMR in soil studies provides data on the proportion of diester P associated with changes in microbial P compounds [ 36 , 37 ] and the ratio of monoester P to diester P, information needed to characterise the lability and rate of turnover of soil organic P.
In these conditions of high species diversity under strong P limitation, we hypothesised, as suggested in previous reports [ 38 ], a long-term adaptation of sympatric species to maximise the capacity of taking up P while avoiding competition. We tested this hypothesis using 31 P NMR spectroscopy in a P metabolomics study to determine the allocation of P to different biological functions in the soil. Our objective was to investigate the variation in soil P profiles along topographic gradients in two tropical forests with highly diverse compositions of tree species for determining whether changes in the profiles of soil organic P were correlated with changes in the composition of tree species and/or with distinct topographic environments and sites. We hypothesised that tree species would use P in specific ways, because the species occupy different functional [ 39 ] and biogeochemical [ 40 ] niches. Biogeochemical niche hypothesis captures niche parameters through species-specific elemental composition and stoichiometry [ 40 , 41 , 42 , 43 ]. The assumptions underlying it are based on the idea that each species is a unique genetic pool of individuals, a product of long-term evolutionary processes, so each species should have a specific structure and functionality (from gene expression to physiological processes). Since the fundamental biological processes (e.g. growth, secondary metabolism, reproduction and storage) have distinct rates in different species, depending on what selection has shaped, the different species have to allocate elements to various traits of tissues and organs differentially. The different use of bio-elements has proved to be even more different in sympatric species as a trait that could avoid direct competition [ 40 ]. Thus, as phosphorus is frequently limiting in tropical rainforests, we should expect that each species tends to have its own P-use strategy (P-metabolome niche) and that this should be underlying the higher species diversity of this high diverse ecosystem. We hypothesized that the tree species would use P in specific ways because each species occupies different functional [ 39 ] and biogeochemical [ 40 ] niches, thus providing species-specific litter that would accordingly modify the 31 P-NMR profile of the underlying soil. Our specific expectations were: (1) species composition would influence the proportions of different P compounds in the underlying soil, and (2) the 31 P NMR profiles would differ amongst and within sites (due to factors such as topography), indicative of differences in P use.
1D 31 P NMR
The spectra of acid-insoluble compounds indicated three main P resonances: monoesters, phospholipids (orthophosphate diester), and DNA. With NMR we were able to determine the family of P-compounds (Fig. 1 ), but we could not determine the exact compounds. Signals from nucleic acids (DNA: −0.37 ppm) and phospholipids were differentiated in the orthophosphate diester region and were readily identified in the soil samples. Inorganic and organic polyphosphates were differentiated by the presence of a signal at − 9 ppm from the α phosphate of organic polyphosphates. Some orthophosphate monoesters, such as mononucleotides derived from RNA and phosphatidyl choline, degraded rapidly to orthophosphate diesters in NaOH-EDTA, although DNA and phospholipids were more stable. The 31 P NMR spectra of NaOH-EDTA extracts from all soil samples generally presented monoesters ( ∼ 25%) at 3.4 to 5.4 ppm, unhydrolysed diesters ( ∼ 20%) at − 1 to 2.3 ppm, and DNA ( ∼ 20%) at − 0.3 ppm. The less abundant forms were polyphosphate ( ∼ 10%) at − 5.6 to − 3.8 ppm, inorganic orthophosphate (hereafter ‘phosphate’; ∼ 10%) at 5.7 to 6.5 ppm, and the pyrophosphate inorganic form of P ( ∼ 5%) at 0.5 to 0.6 ppm. Glucose-6 phosphates had low resonance intensities, at 5.3 to 5.4 ppm.
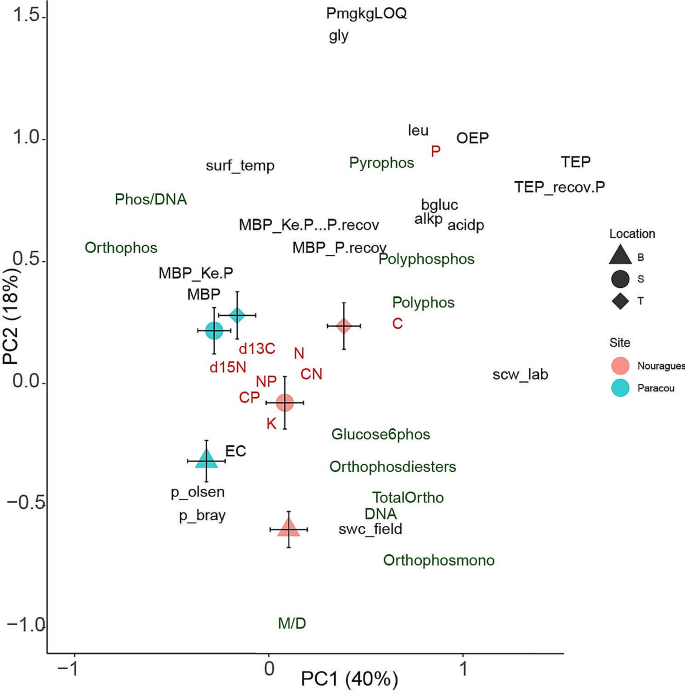
31 P NMR chromatogram with the different molecules shown in the chromatogram
Soil P compounds and nutrients
In the PCA of organic P compounds; nutrients N, P, and K; enzymatic activity, and ecophysiological variables PC1 axis correlated best with site whereas PC2 correlates with samples of different topographic positions within each site (PC2) (Fig. 2 ). We examined the PCA results to identify the components that correlated best with site for better understanding the relationships of site with organic P compounds and the concentrations of C, N, P, and K. The PC2 axes correlated with topographic position and separated samples from the top, slope, and bottom positions, in Nourague and those of top and slope with respect to those of bottom in Paracou site.
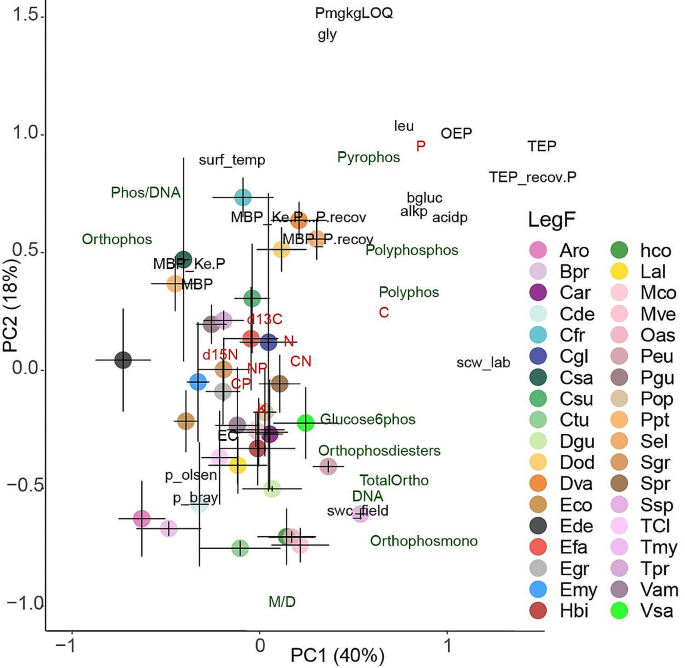
Principal component analyses of soil organic P compounds (green), nutrients (red), d 13 C, d 15 N, enzymatic activities, and ecophysiological variables (black) indicating significant effects of topographic position and site, with corresponding standard deviations Polyphos (polyphosphate); pyrophos (pyrophosphate) polyphosphos (polyphosphonate); orthophos (orthophosphate), orthophosmono (orthophosphate monosester), orthophosphate-diester (orthophosdiester); glucose6phos (glucose-6phosphates); SPAD—chlorophyll content; C—carbon; N—nitrogen; K—potassium; P—phosphorus. The abbreviations in the legend refer to the site (Paracou and Nouragues) and topographic position: T, top; S, slope; and B, bottom
The PCA results also indicated that soil with higher total P concentrations had higher concentrations of organic P compounds involved in storage (polyphosphates and polyphophos) and of C-free forms of P (orthophosphates and pyrophosphates). In contrast, soils with the lowest total P concentrations had higher concentrations of P compounds involved with genetic information, energy transfer, and protein anabolism such as orthophosphate monosester and orthophosphate-diester. Soils at the upper topographic positions had more total P (Table 1 ), which strongly correlated with the activities of acid and alkaline phosphatase, suggesting a greater “investment” of microbes and plants to allocation in P acquisition from soil when the level of total P is high. Tree characteristics also differed amongst the plots at the three topographic positions with wood density tending to be higher in the top plots whereas growth rate, and tree height tending to be higher in the bottom plots (Margalef et al. 2018). Each species occupied a different position in the 2D-plot of the PCA analysis as reinforced by the results of the PERMANOVAS (Table S3 ). The profiles of organic P compounds in the 31 P NMR did not significantly vary between sites (Paracou vs. Nouragues; pseudo-F = 1.93; R 2 = 0.015, P = 0.08), nor in function of topographic positions (top, slope, and bottom; pseudo-F = 1.73; R 2 = 0.027, P = 0.568)) (Table S2 ). The interaction between topographic position and site was neither significant (pseudo-F = 5.68; R 2 = 0.089, P = 0.195) (Table S2 ). Species had a significant effect on 31 P NMR profile (pseudo-F = 3.09; R 2 = 0.53, P < 0.001) (Table S3 ).
The concentrations of the most abundant fractions of P (residual P, total P extractable by NaOH-EDTA, organic P, and inorganic P) varied amongst the soil samples, without any clear trends (Fig. 2 ). The total P concentration was 58–299 mg kg − 1 ; 56–88% was extracted by NaOH-EDTA, and the nonextracted fraction represented ‘residual P’. For the P extracted with NaOH-EDTA, 59–74% was organic and 26–41% was inorganic. Thus, most P was present in organic compounds than in inorganic forms suggesting a high biological use of P. Moreover, among these organic forms the highest proportion of P was found in molecules involved in active functions, such as growth, cellular control, or energy transfer. This is the case of the di-ester forms present in nucleic acid chains or storing energy molecules such as ATP.
The ratio of monoester to diester P was near 1, and diester-2 P was more abundant than diester-1 P, except for soils at the upper topographic position (in which the ratios of monoesters to diesters and diester-1 [phospholipids] to diester-2 [DNA and acid-unstable compounds] in the NaOH-EDTA extracts were similar).
Species-specific utilization of phosphorus
Our findings support the hypothesis that soil phosphorus (P) profiles differ among samples collected beneath different tree species. The soil samples associated with each tree species occupy distinct positions in a 2D plot representing the main functional groups of metabolic P molecules and nutrient concentrations in the soils (Fig. 3 ; Table 2 , and table S4 ). Species accounted for over half of the total variance in 31P NMR data. These consistent results indicate a species-specific pattern of P uptake and utilization, as well as species-specific interactions between trees and microbes (including mycorrhiza) and P transformations specific to the microbes associated with each tree species. Additionally, we observed a clear gradient in P compounds from high anabolic energy to storage, with the highest concentrations of P compounds associated with anabolic energy in soils with the lowest total P concentrations.
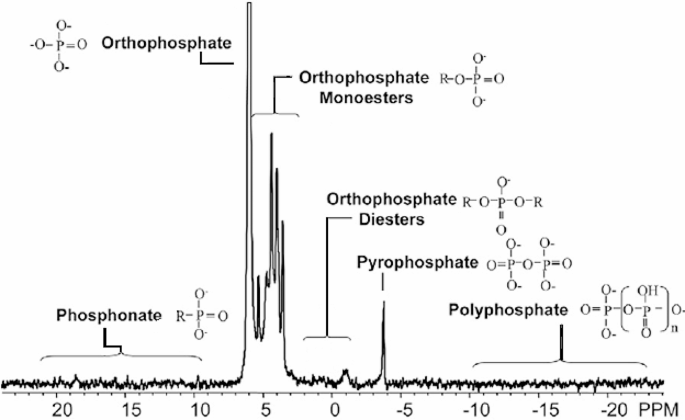
Principal component analyses of soil organic P compounds (green), nutrients (red), d 13 C, d 15 N, enzymatic activities, and ecophysiological variables (black) with species scores mean position, with corresponding confidence intervals (95%). Polyphos (polyphosphate); pyrophos (pyrophosphate) polyphosphos (polyphosphonate); orthophos (orthophosphate), orthophosmono (orthophosphate monosester), orthophosphate-diester (orthophosdiester); glucose6phos (glucose-6phosphates); SPAD—chlorophyll content; C—carbon; N—nitrogen; K—potassium; P—phosphorus. The abbreviations in the legend refer to the tree species: Aro, Aniba rosaeodora ; Bpr, Bocoa prouacensis ; Car, Chrysophyllum argenteum ; Cde, Capiro decorticans ; Cfr, Catostemma fragrans ; Cgl, Caryocar glabrum ; Csa, Chrysophyllum sanguinolentum ; Csu, Carapa surimensis ; Ctu, Chimarrhis turbita ; Dgu, Dicorynia guianensis ; Dod, Dipteryx odorata ; Dva, Drypetes variabilis ; Eco, Eschweilera coriacea ; Ede, Eschweilera decolorans ; Efa, Eperua falcata ; Egr, Eperua grandiflora ; Emy, Eugenia ; Hbi, Hirtella bicornis ; hco, Hymanea courbaril ; Lal, Licania alba ; Mco, Moronobea coccinea ; Mve, Micropholis venulosa ; Oas, Oxandra asbeckii ; Peu, Pouteria eugeniifolia ; Pgu, Paloue guianensis ; Pop, Protium opacum ; Ppt, Pradosia ptychandra ; Sel, Sloanea ; Sgr, Sapotaceae grandiflora ; Spr, Sterculia pruriens ; Ssp, Sterculia speciose ; TCl, Tovomita clusiaceae ; Tmy, Tetragastris ; Tpr, Talisia praealta ; Vam Vouacapoua america ; and Vsa, Vochysia sabatieri
Polyphosphates and polyphosphonates are chains of orthophosphates that serve as stored phosphate compounds. Our results revealed higher concentrations of orthophosphate, pyrophosphates, polyphosphonates, and polyphosphates in soils with higher total P concentrations and lower P availability at our study sites. Proportionally, more P was allocated to active metabolic molecules (e.g., DNA) and energy transfer (poly P, pyro P) in soils with lower total P concentrations and higher P availability. Higher concentrations of P storage compounds in the soil can be associated with low P availability, ensuring a controlled source of P for microbes when accessing soil P is challenging [ 44 ]. Moreover, the investment of microbes in building phosphatases was significantly lower in conditions of low carbon (C) and high poly P concentrations. This suggests that high polyphosphate concentrations may be associated with C conservation, particularly in sites where the soil has a high capacity to retain P. In these cases, higher polyphosphate reserves can serve as a P conservation trait, preserving C and providing a biological mechanism for accessing P from P reserves in microbial cells when necessary. Similarly, under conditions of very low C availability, higher PolyP levels may act as a compound for storing energy to conserve C [ 42 ].
Another study in the same ecosystem reported clear species-specific signatures in general foliar metabolomics profiles, indicating functional niches for dominant tree species in the region [ 43 , 44 ]. Our results align with our initial hypothesis that the quantities of different P compounds would differ among soil samples due to interspecific differences in P utilization strategies and cycling. This finding is consistent with previous observations [ 45 , 46 ]. For example, in a Panama rainforest, Condit et al. (2013) found that dry-season intensity and soil phosphorus were the strongest predictors, affecting the distribution of over half of a set of 550 tree species.
Our findings are also in line with previous studies that have identified associations between sets of species and specific habitats in tropical rainforests. The distribution of tree species is influenced by spatial variability in soil properties, such as nutrient availability and topography [ 47 , 48 , 49 , 50 ]. An extension of classical ecological niche theory, known as the “biogeochemical niche hypothesis“ [ 45 ], suggests that each species tends to reach an optimal chemical composition linked to a specific function that allows it to survive in its niche [ 39 , 51 , 52 ]. Our study provides evidence that organic P measurements can be used to characterize soil niches. The measurement and identification of different organic P molecules can serve as a powerful tool for characterizing most P-limited rainforests [ 23 , 53 ]. In these cases, “P-metabolome niches” arise due to soil niche differentiation along natural spatial gradients of biotic and abiotic factors that influence P availability, total concentrations, and chemical forms of P, which, in turn, are associated with P utilization and cycling. Tropical trees may exhibit finely subdivided niches [ 54 ], although direct evidence for measurable variables of overall functional differences among sympatric species and geological processes is currently limited. However, our results clearly indicate that soil beneath each tree species possesses a specific soil P-metabolome. The distinct strategies of individual tree species for P uptake, litter quality, retranslocation, allocation, soil exudates, and biotic interactions with soil microorganisms and other tree species contribute to a micro-scale soil space with a unique P-metabolome profile.
Topography and regional factors
In addition to the differences in P-metabolome profiles in soils associated with different tree species, we also observed relationships between P-metabolome profiles and other factors such as site and topographic position (Fig. 2 ). These relationships suggest that microsite variations in P-metabolome profiles are influenced by biotic conditions and associated with distinct soil traits, including texture, that vary across different topographic situations. Topography creates diverse soil P conditions, with higher total P concentrations and lower P availability at higher elevations, and vice versa at lower elevations. This finding is consistent with more conservative resource-use traits observed at higher elevations, such as the higher wood density of trees, and the opposite pattern at lower elevations. Furthermore, the spread of species along the 2D space generated by the two principal component axes was also associated with these findings. Thus, each species has developed a specific niche for highly efficient P utilization along the gradient, resulting in a distinctive distribution profile of P compounds that maximizes their use efficiency. These results also suggest that a “niche” of P profiles is associated with a physical gradient, primarily determined by topographic positions, and reflects species-specific strategies for mineralizing, mobilizing, utilizing, and acquiring soil P.
The abundance of organic P compounds, such as diesters and phosphonates, varied according to site and topographic position. Specifically, DNA and polyphosphate concentrations increased from the bottom to the top. DNA, polyphosphate, and phosphonates are abundant in soils with high microbial activity [ 31 ]. Site and topographic position explained some of the variance in the P profiles, likely due to clay content, which can form organomineral complexes and better retain organic material, including organic P. Variations in P profiles may also be influenced by metal oxides, as they can strongly occlude P, including organic P molecules. The occasional flooding of the bottom plots may have influenced the redox states of metal oxides (specifically iron and manganese oxides; aluminum oxides are less affected), which, in turn, could have affected the adsorption/desorption dynamics of P fractions in the soil.
Furthermore, the higher presence of mono- and diesters observed in bottom soils with higher sand content aligns with previous findings. A greater presence of monoester phosphorous compounds has been linked to the decomposition of organic matter rich in P metabolites, such as phosphatidylcholine and phospholipids [ 31 , 55 ]. Similarly, higher soil concentrations of diester phosphates have been positively associated with higher concentrations of sand-sized fractions compared to silt and clay fractions [ 56 , 57 ].
Significant variations were observed in the ratios of monoesters to diesters and the ratios of different diesters (diester-1 and diester-2; Table 1 ) across different parts of the 31P NMR spectra. Gressel et al. (1996) suggested that the correlation between monoester P and alkyl C in the organic horizons of a tropical forest soil indicates that the mineralization of monoester P fractions is linked to the decomposition of plant structural components, and that a significant portion of the monoester P in NaOH extracts may originate from hydrolyzed phospholipids derived from plants. The alkaline hydrolysis of phosphatidylcholine to monoesters is well-documented [ 31 , 55 , 58 ]. Soils in semi-arid northern Tanzania [ 59 ], enriched with diesters in sand-sized fractions relative to silt and clay fractions, likely derive these diesters from plants. Labile organic P compounds (e.g., phosphonates and diesters) and phosphate diesters (e.g., DNA and other diesters) often exhibit inverse relationships. For instance, diester P may increase while phosphonates decrease when comparing soils from a native savanna to Oxisol soil of an improved pasture in Colombia [ 36 , 53 ]. Similarly, in a Spodosol soil of a spruce-fir forest, diester P (0 ppm) increased while unidentified diester compounds (1.5 to 2.5 ppm) decreased with increasing decomposition levels [ 46 ].
The top plots had higher concentrations of total P but lower levels of available P due to adsorption onto fine-grained particles, particularly highly reactive P oxides. This occlusion mechanism of P requires greater investments in microbial and root activities to acquire P, which is consistent with the higher activities of acid and alkaline phosphatases observed. However, the higher presence of molecules associated with P storage (orthophosphate, pyrophosphates, and polyphosphate inorganic chains) in these top soils is consistent with a more conservative strategy [ 47 , 48 , 49 , 50 , 51 ], where more P is stored within cells to support biological functions that require P, independent of P uptake from the soil. Furthermore, in top soils, the limited availability of soil P was associated with tree species characterized by higher wood density, typically exhibiting more conservative traits. These findings provide further evidence of the links between soil P status, species distribution, and their life strategies in this tropical rainforest [ 52 , 53 , 54 ].
In conclusion, our study reveals that variations in P-metabolome profiles in soils are not only influenced by different tree species but also by other factors such as site and topographic position. Topography creates distinct soil P conditions, which, combined with the spread of species along the gradient, leads to species-specific strategies for P utilization and uptake. Additionally, the abundance of organic P compounds varies with site and topographic position, influenced by factors such as clay content and metal oxides. The presence of mono- and diesters in soils correlates with organic matter decomposition and sand content. The ratios of different P compounds further highlight the complex dynamics of P cycling in the soil. Our findings contribute to a better understanding of the interactions between vegetation, soil, and P dynamics in tropical rainforest ecosystems.
The P metabolomic profiles of soil samples collected at different sites under trees in French Guianese rainforests differed greatly, with very significant differences amongst soils collected beneath different tree species. This result is consistent with the ecological niche theory and the biogeochemical niche hypothesis (a correspondence of species with specific environmental conditions) in this highly diverse tropical ecosystem. Using 31 P-metabolomic profiles to analyse the functions of different plant species in a community thus allowed us to identify different soil 31 P NMR profiles associated with each tree species.
Our multivariate analyses of the soil 31 P NMR metabolomics data notably indicated a trend to find higher soil concentrations of P biomolecules associated with low P use and high P storage under higher total P concentrations at the top sites, coinciding with tree species with more conservative strategies associated with denser wood and with a soil texture and mineral composition providing high P immobilization capacity. The bottom sites tended, instead, to have higher soil concentrations of P biomolecules associated with biological activity under lower total P concentrations, but higher P-availability, coinciding with tree species with less dense wood and a soil coarser texture.
Our combined analysis of soil elemental composition and P metabolomics provides an improved understanding of environmentally linked shifts in soil P concentrations and availability with different allocations of P for growth and other functions, such as storage, defense, reproduction, or resistance that stress the key role of this element in tropical rainforest functioning. As more P is available, more P is invested in active metabolism and functional activity and less in storage.
French Guiana is on the northeastern coast of South America between 2°10′ and 5°45′N and 51°40′ and 54°30′W. 97% of the region is covered by lowland wet tropical forest [ 55 ]. A pronounced dry season, characterised by < 100 mm precipitation per month, extends from September to November and is associated with the displacement of the intertropical convergence zone. Mean daily temperature is 25.8 °C and varies by only 2 °C throughout the year; daily temperatures vary by 7 °C during the rainy season and by 10 °C in the dry season [ 56 , 57 ].
Field work was conducted at two sites of mature lowland tropical rainforest: the Paracou Research Station (5°18′N, 52°53′W) and the Nouragues Research Station (4°05′N, 52°40′W). Mean annual rainfall is 2990 mm at Nouragues and 3160 mm at Paracou [ 58 ], although the dry season is more severe at Paracou [ 58 ]. Three topographical locations were selected at each site: top of hills (top), middle of slopes at an intermediate elevation (slope), and bottom of slopes at a low elevation, immediately above a creek [ 59 ].
Study plots
We established 12 plots of 0.25 ha at each site stratified by three topographic positions to account for the heterogeneous soil texture: top of the hills, slope and bottom of the valleys, thus with 4 plots in each topographic position. In each plot, we delimited a central 20 × 20 m quadrat where we marked five evenly spaced sampling points around which we focused all our measurements. This design thus contained a total of 120 sampling points (2 sites × 3 topographic positions × 4 replicate plots per topographic position × 5 sampling points in each plot). In each sampling point we annotated the nearest tree in function of the linear distance to the trunk base. The bottom plots at both sites had higher sand contents and lower clay contents than the top and slope plots [ 57 ]. All trees (diameter at breast height ≥ 10 cm) within the 0.25-ha plots were mapped, tagged, and identified to species or genus using herbarium vouchers for determining the richness of the tree species for each plot.
Sample collection
Thus, we collected five soil samples per plot to a depth of 15 cm (Topsoil) in June (wet season) 2015. Each sample was collected using an auger/corer and was a composite of three borings near (< 2 m apart) each other. All voucher specimens were deposited in the Herbarium of International Center for Tropical Botany in Miami, FL 33,199 USA. A total of 120 samples were collected around the trees. We aliquoted 5 g of each sample for 31 P NMR analyses that were immediately placed into a paper bag and frozen in liquid nitrogen before transporting the samples to the laboratory. The rest of soil sample was transported to the lab and stored in plastic zip bags at 4ºC until all the other analyses (within 4 weeks). Soil storage at 4ºC has been shown to keep enzymatic activity of tropical soils better than frozen samples. Fresh soil was sieved to 2 mm; for each sample one part was used for enzymatic activity analyses and the other part was dried 24 h at 105ºC for gravimetric water content. The other soil variables were analyzed from the same soil samples as those used in the 31 P NMR analyses to determine the effect of the tree species.
Environmental biotic and abiotic data
We compiled data for 28 variables describing the pools of soil nutrients, activities of extracellular enzymes, and aboveground tree-community data for each site to characterise the potential micro-environmental and biotic drivers. Nutrient concentrations and ratios, d 13 C, d 15 N, enzymatic activities, and ecophysiological variables are abbreviated as: C, N, P, C:N, C:P, N:P, K, d 13 C, d 15 N, leu (enzymes leucine), gly (enzymes glycine aminopeptidases), alkp (enzymes alkaline phosphatases), acidp (enzymes acid phosphatases), bgluc (enzymes β- glucosidase), p_olsen (amount of soil phosphorus available by Olsen test), p_bray (amount of soil phosphorus available by Bray test), scw_lab (laboratory surface water content), swc_field (field surface water content), surf_temp (soil surface temperature), EC (soil electric conductivity, mS/cm), MBP (microbial biomass P, µg P / g soil DW), MBP_Ke-P (microbial biomass P, µg P / g soil DW with factor KeP = 0.40), MBP_P recov (microbial biomass P, µg P / g soil DW with P-recovery factor), MBP_Ke-P + P recov (microbial biomass P, ug P / g soil DW with P-recovery factor and KeP), TEP (Total extractable P, µg P / g soil DW), TEP_recov P (Total extractable P, µg P / g soil DW with recovery factor P applied ), OEP (Organic extractable P, µg P / g soil DW), and PmgkgLOQ (P concentration in soil, mg/kg Limit of Quantification). All the acronyms used throughout the text are described in Table S1 .
Nutrient pools
We collected soil cores from the topsoil of each sampling point using a soil auger (4 cm in diameter and 15 cm in length; Van Walt, Haslemere, UK) to analyse the nutrient status. The samples were sieved to 2 mm and then freeze-dried (Alpha 1–2 LDplus, Martin Chirst Freeze Dryers, Osterode, Germany). Subsamples were pulverised in a ball mill (MM400, Retsch, Haan, Germany) for the analysis of elemental composition. We weighed 0.15–0.2 g of soil using an MX5 microbalance (Mettler Toledo, Columbus, USA) for determining the concentrations of total carbon (C) and nitrogen (N) by combustion coupled to an isotopic ratio mass spectrometer at the Stable Isotopes Facility (UC Davis, USA). Concentrations of total P and potassium (K) were determined by diluting 0.25 g of soil with an acid mixture of HNO 3 (60%) and H 2 O 2 (30% w/v) and digested in a MARS Xpress microwave oven (CEM Corporation, Matthews, USA). The digested solutions were then diluted to final volumes of 50 mL with ultrapure water and 1% HNO 3 . Blank solutions (5 mL of HNO 3 with 2 mL of H 2 O 2 but no sample biomass) were regularly analysed. The content of each element was determined using inductively coupled plasma/optical emission spectrometry (ICP-OES Optima 4300DV, PerkinElmer, Wellesley, USA). We used the standard certified biomass NIST 1573a to assess the accuracy of the biomass digestion and analytical procedures.
Determination of activities of extracellular enzymes
We determined the activities of the extracellular enzymes β-glucosidase, leucine and glycine aminopeptidases, and acid and alkaline phosphatases (βgluc, leu, gly, acidP, and alkP, respectively) in all the 120 topsoil samples. The activities of these enzymes can serve as proximal variables of microbial nutritional metabolism and depend mostly on the interaction of the relationship between supply and demand with environmental kinetics [ 60 ]. Fresh subsamples of 2-mm sieved soil were stored in ziplock plastic bags and stored at 4 °C until analysis. We quantified the maximum potential activities of each enzyme by colorimetric assays using p -nitrophenylphosphate and p -nitroaniline derivative chromogenic substances. These enzymes are involved in the mineralization of C, N and P (Sinsabaugh and Shah, 2012). β -glucosidase participates in the decomposition of plant tissues, catalyzing the hydrolysis of 1–4 glucosidic bonds of labile cellulose (cellobiose and cellodextrins) to yield glucose. Leucine and glycine amino-peptidases cleave N-terminal residues from proteins and peptides. Acid and alkaline phosphatases release orthophosphate from organic P compounds like labile nucleic acids, phospholipids and inositol phosphates by the hydrolyzation of oxygen-P bonds.
Soil microbial biomass P and soil extractable P
Phosphorus in the microbial biomass (MBP) was measured using the chloroform fumigation extraction method according to Brookes et al. (1982) and Brookes et al. (1985). Two subsamples (10 g fresh weight) of sieved soil were taken for each topsoil sample. One subsample was fumigated for 24 h with chloroform and extracted with 0.5 M of NaHCO 3 (10:1 v:w) after 30 min shaking. The other subsample was directly extracted following the same protocol. The extracts were then filtered with Whatman 42 equivalent paper. Total P content in the extracts was determined after digestion of 2.5 g of aliquot with HNO 3 in a microwave oven (MARS Xpress, CEM Corporation, Matthews, USA). The digested solutions were then diluted to a final volume of 50 mL with ultrapure water and 1% HNO 3 . Blank solutions were regularly analysed in parallel. P concentration in the digested samples and blanks was determined using inductively coupled plasma/optical emission spectrometry (ICP-OES Optima 4300DV, Perkin-Elmer, Wellesley, USA). The microbial biomass P content was calculated from the difference between fumigated and non-fumigated samples and expressed per unit of soil dry mass. Given that inorganic P molecules can absorb onto soil surfaces (organic matter and minerals) it is necessary to account for this effect during the extraction of P from soil, this is done through the application of an empirically determined “P-recovery factor”. Thus, a known amount of inorganic P (12.5 µg of inorganic P added as KH 2 PO 4 ) was added to some of the controls to calculate the P-recovery factor in our soils. Microbial biomass was then calculated using the fumigated and non-fumigated samples corrected with the P-recovery coefficient (MBP_P.recov). Microbial biomass P was also corrected to take into account the efficiency of the fumigation (MBP_Ke.P), using the conversion factor KeP = 0.40 (Jenkinson et al. 2004). The factor KeP is an empirical coefficient used to relate the quantity of material solubilized by chloroform to the size of the original biomass, in this case it indicates that with fumigation 40% of the biomass-P is extracted as inorganic P. We have used all the microbial biomass P variables with and without corrections (MBP, MBP_P.recov, MBP_Ke.P and MBP_Ke.P…P.recov) in the analyses to give an overview of the methodological issues related to the quantification of extractable P in soils and microbes and to provide additional information for those readers with a background in soil biogeochemistry.
The total P concentration measured in non-fumigated bicarbonate-extracts was referred as the total extractable P (TEP) fraction. One aliquot of the non-fumigated extracts was used to determine the inorganic extractable P (P_Olsen) by Olsen’s method (Watanabe and Olsen, 1965). The organic extractable P (OEP) in the non-fumigated extracts was calculated as the difference between TEP and P_Olsen. All bicarbonate-extractable P fractions were expressed as µg of P per gram dry soil. Soil extractable P was also determined with Bray-P (P_Bray) acid fluoride extraction (Bray and Kurtz 1945) in oven-dried soil subsamples.
Sample processing for 31 P NMR analysis
The soils were frozen in liquid nitrogen, lyophilised, and stored in paper bags at -80 °C. The samples were ground with a ball mill at 1500 rpm for 3 min, and the fine powder was stored at -80 °C until extraction of the metabolites.
One-dimensional 31 P NMR
Conventional one-dimensional (1D) 31 P NMR (Box 1) was used to quantify the main organic and inorganic forms of extractable P using NaOH-EDTA, including DNA, total diesters and monoesters, phosphonates, pyrophosphate, and polyphosphate. All the identification of the target P classes was based on chemical shifts and previous reported data (Turner et al. 2003; Vestergren et al. 2012). P was extracted by shaking 1.5 g of dry ground soil from each composite sample for 4 h in 30 mL of a solution containing 250 mM NaOH and 50 mM Na 2 EDTA (Cade-Menun and Preston 1996). The extracts were centrifuged at 14 000 g for 30 min, and the supernatant (23 mL) was frozen at − 80 °C overnight and then lyophilised. Lyophilisation yielded 750 ± 50 mg of material, 80 mg of which was redissolved in 640 µL (1:8 w/v) of a solution containing 530 µL of D 2 O, 10 µL of 14.2 M NaOD, and 50 µL of 16 mM methylene diphosphonic acid trisodium salt (MDPA, Sigma-Aldrich product number M1886). The MDPA served as a P reference for quantifying individual compounds, and each 50-µL spike contained 50 µg of P. The redissolved solution was vortexed for 2 min and centrifuged at 10 000 g for 5 min, and 560 µL was then transferred to a 5-mm NMR tube for 1D 31 P NMR.
NMR spectra for 31 P were obtained using an Avance III 600 MHz spectrometer (Bruker, Ettlingen, Germany) operating at 161.76 MHz. NaOH-EDTA extracts were analysed using a 3.9-µs pulse (90°), a relaxation delay time of 2.0 s, an acquisition time of 0.9 s, and broadband proton decoupling. We recorded 15 000 scans per sample, and the experimental time was 12.5 h. Spectra were processed with a line broadening of 2 Hz, and chemical shifts of signals were determined in parts per million (ppm) relative to an external standard (85% orthophosphoric acid, H 3 PO 4 ). The main chemical types of P compounds were identified based on previously reported chemical shifts [ 32 , 61 ]. Peaks were first identified using an automatic procedure for fitting peaks; peaks that were clearly visible were manually selected using TopSpin 2.0 NMR software (Bruker, Germany). Signal areas were calculated by the deconvolution and integration of individual peaks. Concentrations of P compounds (mg P kg –1 soil) were calculated using the known P concentration of MDPA spiked in the sample, and concentrations per weight of air-dried soil were given. All NMR spectra were processed using TopSpin 2.0.
Signal intensity in the 31 P spectra was assigned to the different types of P by first integrating across the following regions of broad chemical shifts: -21.5 to -18.5 ppm for nonterminal polyphosphate (poly P), -5.3 to -4.8 ppm for pyrophosphate (pyro P), -4.8 to -4.0 for terminal poly P, -1.5 to 2.5 for diester-P, and 2.5 to 7 ppm for orthophosphate (ortho-P and monoester-P). Deconvolution was then used to determine the intensity of up to 16 resonances in the ortho-P and monoester-P regions. Deconvolution analysis began by manually identifying chemical shifts of peaks and shoulders. Peak chemical shifts varied only slightly amongst the samples. The orthophosphate signal shifted the most (range: 5.56–5.74 ppm), which shifted amongst soil samples, sites, species, and topographic positions. This variation was most likely due to slight differences in pH amongst the samples, because the orthophosphate peak is highly sensitive to variation in pH [ 62 ]. Thus, 31 P NMR allows the determination of the family of P-compounds (Fig. 3 ), but not of the exact compounds.
31 P nuclear magnetic resonance ( 31 P-NMR) is an analytical NMR technique that allows the detection of both the concentration and the chemical form of the most abundant phosphorus isotope, 31 P, in the analytical sample. In the concrete case of 31 P this analytical tool allows to separately detect the intensity of the signal (proportional to concentration in the analyzed sample) of each molecular structure where the 31 P is located. In this way, we can detect the most abundant types of compounds containing 31 P in biological samples. We explored different aqueous/organic solvent methods to extract as many groups of compounds as possible and the preliminary results showed that using an aqueous solution allowed extracting most of groups compounds. Standard 1D 31 P NMR was used to quantify concentrations of the main organic and inorganic P classes, including DNA, total orthophosphate diesters and monoesters, phosphonates, pyrophosphate and polyphosphate. Phosphorus was extracted by shaking 1.5 g of dry and ground soil from each composite soil sample in 30 mL of a solution containing 250 mM NaOH and 50 mM Na2EDTA (ethylenediaminetetraacetate) for 4 h (Cade-Menun and Preston 1996). Identification of the target P classes was based on chemical shifts and previous reported data [ 32 , 63 ].
Statistical analyses
The relationships of the P compounds with species, site and topographic position were identified by a PERMANOVA [ 64 ] of the NMR data for each soil sample. Euclidean distance, species identity, site, and topographic position were the fixed factors, and plot was a random effect, with 2000 permutations. Differences amongst species, site, and topographic position and amongst the P compounds most responsible for these differences were determined by comparing the areas of the different metabolite peaks normalized relatively to internal standards. Principal component analyses (PCAs) were used for processing the “omic” data sets together with the other potential influencing soil parameters, to detect the part of the variance explained by species [ 65 ]. Pearson’s correlation was used to identify the relationships of the 1D NMR measurements and the relationships between the concentrations of nutrients and organic P compounds. Error estimates are standard errors of the mean unless otherwise stated.
All statistical procedures were performed using R v 3.5 ( www.r-project.org ) with the SEQKNN, VEGAN, FACTOEXTRA, FACTOMINER, DPLYR, RANDOMFOREST, and MIXOMICS packages.
Data availability
All data generated or analysed during this study are included in this published article [and its supplementary information files].
Baraloto C, Rabaud S, Molto Q, Blanc L, Fortunel C, Harault B, et al. Disentangling stand and environmental correlates of aboveground biomass in amazonian forests. Glob Chang Biol. 2011;17:2677–88.
Article Google Scholar
Corlett RT, Primack RB. Tropical rainforests and the need for cross-continental comparisons. Trends Ecol Evol. 2006;21:105–7.
Vitousek PM, Sanford RL Jr. Nutrient cycling in moist tropical forest. Ann Rev Ecol Syst. 1986;17:137–67.
Rothstein DE, Vitousek PM, Simmons BL. An exotic Tree alters Decomposition and Nutrient Cycling in a hawaiian montane forest. Ecosystems. 2004;7:805–14.
Article CAS Google Scholar
Allison SD, Vitousek PM. Rapid nutrient cycling in leaf litter from invasive plants in Hawai. Oecologia. 2004;141:612–9.
Article PubMed Google Scholar
Connell JH. Diversity in tropical rain forests and coral reefs. Science (1979). 1978;199:1302–10.
Mandl NA, Kessler M, Robbert Gradstein S. Effects of environmental heterogeneity on species diversity and composition of terrestrial bryophyte assemblages in tropical montane forests of southern Ecuador. Plant Ecol Divers. 2009;2:313–21.
Becerra JX. On the factors that promote the diversity of herbivorous insects and plants in tropical forests. Proc Natl Acad Sci. 2015;112:6098–103.
Article CAS PubMed PubMed Central Google Scholar
Aiba SI, Sawada Y, Takyu M, Eino T, Kitayama K, Repin R. Structure, floristics and diversity of tropical montane rain forests over ultramafic soils on Mount Kinabalu (Borneo) compared with those on non-ultramafic soils. Aust J Bot. 2015;63:191–203.
John R, Dalling JW, Harms KE, Yavitt JB, Stallard RF, Mirabello M, et al. Soil nutrients influence spatial distributions of tropical tree species. Proc Natl Acad Sci U S A. 2007;104:864–9.
Martins KG, Marques MCM, dos Santos E, Marques R. Effects of soil conditions on the diversity of tropical forests across a successional gradient. Ecol Manage. 2015;349:4–11.
LeBauer DS, Treseder KK. Nitrogen limitation of net primary productivity in terrestrial ecosystems is globally distributed. Ecology. 2008;89:371–9.
Fujii K, Shibata M, Kitajima K, Ichie T, Kitayama K, Turner BL. Plant–soil interactions maintain biodiversity and functions of tropical forest ecosystems. Ecol Res. 2017;33:1–12.
Google Scholar
Xu W, Ci X, Song C, He T, Zhang W, Li Q, et al. Soil phosphorus heterogeneity promotes tree species diversity and phylogenetic clustering in a tropical seasonal rainforest. Ecol Evol. 2016;6:8719–26.
Article PubMed PubMed Central Google Scholar
Elser JJ, Bracken MES, Cleland EE, Gruner DS, Harpole WS, Hillebrand H, et al. Global analysis of nitrogen and phosphorus limitation of primary producers in freshwater, marine and terrestrial ecosystems. Ecol Lett. 2007;10:1135–42.
Wardle DA, Walker LR, Bardgett RD. Ecosystem Properties and Forest decline in contrasting long-term. Sci (1979). 2004;305:509–14.
Walker TW, Syers JK. The fate of phosphorus during pedogenesis. Geoderma. 1976;15:1–19.
Kichenin E, Wardle DA, Peltzer DA, Morse CW, Freschet GT. Contrasting effects of plant inter- and intraspecific variation on community-level trait measures along an environmental gradient. Funct Ecol. 2013;27:1254–61.
Tanner EVJ, Vitousek PM, Cuevas E. Experimental investigation of nutrient limitation of Forest Growth on Wet Tropical mountains. Ecology. 1998;79:10–22.
Vitousek PM. Nutrient cycling and limitation: Hawai ‘i as a model system. Princeton. Princeton, New Jersey, USA.; 2004.
Sanchez PA, Logan TJ. Myths and science about the Chemistry and Fertility of soils in the tropics. SSSA Special Publication. 1992;29:35–35.
CAS Google Scholar
Tiessen H, Stewart JWB, Cole CV. Pathways of Phosphorus transformations in Soils of Differing Pedogenesis 1. Soil Sci Soc Am J. 1984;48:853–8.
Harrison AF. Soil organic phosphorus: a review of world literature. Commonweal. 1987.
Turner BL, Engelbrecht BMJ. Soil organic phosphorus in lowland tropical rain forests. Biogeochemistry. 2011;103:297–315.
Tiessen H, Salcedo IH, Sampaio EVSB. Nutrient and soil organic matter dynamics under shifting cultivation in semi-arid northeastern. Agric Ecosyst Environ. 1992;38:139–51.
Johnson AH, Frizano J, Vann DR. Biogeochemical implications of labile phosphorus in forest soils determined by the Hedley fractionation procedure. Oecologia. 2003;135:487–99.
Cade-Menun BJ. Characterizing phosphorus in environmental and agricultural samples by 31P nuclear magnetic resonance spectroscopy. Talanta. 2005;66:359–71.
Article CAS PubMed Google Scholar
Chacon N, Silver WL, Dubinsky EA, Cusack DF. Iron reduction and soil phosphorus solubilization in humid tropical forests soils: the roles of labile carbon pools and an electron shuttle compound. Biogeochemistry. 2006;78:67–84.
Sampaio EVDSB, Dall’Olio A, Nunes KS, de Lemos EEP. A model of litterfall, litter layer losses and mass transfer in a humid tropical forest at Pernambuco, Brazil. J Trop Ecol. 1993;9:291–301.
HaTtenschwiler S, Coq S, Barantal S, Handa IT. Leaf traits and decomposition in tropical rainforests: revisiting some commonly held views and towards a new hypothesis. New Phytol. 2011;189:950–65.
Stark NM, Jordan CF. Nutrient Retention by the Root Mat of an amazonian rain forest author (s): Nellie M. Stark and Carl F. Jordan published by : Ecological Society of America NUTRIENT RETENTION BY THE ROOT MAT OF AN. Ecology. 1978;59:434–7.
Turner BL, Mahieu N, Condron LM. Phosphorus-31 nuclear magnetic resonance spectral assignments of Phosphorus compounds in Soil NaOH–EDTA extracts. Soil Sci Soc Am J. 2003;67:497–510.
Cade-Menun BJ, Liu CW, Nunlist R, McColl JG. Soil and litter Phosphorus-31 nuclear magnetic resonance spectroscopy. J Environ Qual. 2002;31:457–65.
CAS PubMed Google Scholar
Vincent AG, Turner BL, Tanner EVJ. Soil organic phosphorus dynamics following perturbation of litter cycling in a tropical moist forest. Eur J Soil Sci. 2010;61:48–57.
Vestergren J, Vincent AG, Jansson M, Persson P, Ilstedt U, Giesler R. High-resolution characterization of Organic Phosphorus in Soil extracts using 2D 1 H – 31 P NMR correlation spectroscopy. Environ Sci Technol. 2012;46:3950–6.
Sumann M, Amelung W, Haumaier L, Zech W. Climatic effects on Soil Organic Phosphorus in the North American Great Plains identified by Phosphorus-31 nuclear magnetic resonance. Soil Sci Soc Am J. 1998;62:1580–6.
Guggenberger G, Christensen BT, Rubaek G, Zech W. Land-use and fertilization effects on P forms in two EuroPean soils: resin extraction and 31P-NMR analysis. Eur J Soil Sci. 1996;47:605–14.
Turner BL. Soil organic phosphorus in tropical forests: an assessment of the NaOH-EDTA extraction procedure for quantitative analysis by solution 31P NMR spectroscopy. Eur J Soil Sci. 2008;59:453–66.
Díaz S, Kattge J, Cornelissen JHC, Wright IJ, Lavorel S, Dray S, et al. The global spectrum of plant form and function. Nature. 2016;529:167–71.
Peñuelas J, Fernández-Martinez M, Ciais P, Jou D, Piao S, Obersteiner M, et al. The bioelements, the elementome, and the biogeochemical niche. Ecology. 2019;100:e02652.
Peñuelas J, Sardans J, Ogaya R, Estiarte M. Nutrient stoichiometric relations and biogeochemical niche in coexisting plant species: Effect of simulated climate change. Pol J Ecol. 2008;56:613–22.
Penuelas J, Sardans J, Llusià J, Owen SM, Carnicer J, Giambelluca TW, et al. Faster returns on ‘leaf economics’ and different biogeochemical niche in invasive compared with native plant species. Glob Chang Biol. 2010;16:2171–85.
Sardans J, Peñuelas J. Climate and taxonomy underlie different elemental concentrations and stoichiometries of forest species: the optimum biogeochemical niche. Plant Ecol. 2014;215:441–55.
Turner BL, Haygarth PM. Phosphatase activity in temperate pasture soils: potential regulation of labile organic phosphorus turnover by phosphodiesterase activity. Sci Total Environ. 2005;344:27–36.
Ecological Niches: Linking Classical and Contemporary Approaches - Jonathan M. Chase,Mathew A. Leibold - Google Libros. University of Chica….
Turner BL, Brenes-Arguedas T, Condit R. Pervasive phosphorus limitation of tree species but not communities in tropical forests. Nature. 2018;555:367–70.
Sabatier D, Grimaldi M, Prévost MF, Guillaume J, Godron M, Dosso M, et al. The influence of soil cover organization on the floristic and structural heterogeneity of a guianan rain forest. Plant Ecol. 1997;131:81–108.
Pélissier R, Dray S, Sabatier D. Within-plot relationships between tree species occurrences and hydrological soil constraints: an example in French Guiana investigated through canonical correlation analysis. Plant Ecol. 2002;162:143–56.
Moulatlet GM, Rennó CD, Figueiredo FOG, Ruokolainen K, Banon L, Emilio T, et al. The role of topographic-derived hydrological variables in explaining plant species distributions in Amazonia. Acta Amazon. 2022;52:218–28.
Lopez OR, Kursar TA. Does flood tolerance explain tree species distribution in tropical seasonally flooded habitats? Oecologia. 2003;136:193–204.
Lescure J-P, Boulet R. Relationships between Soil and Vegetation in a tropical rain forest in French Guiana. Biotropica. 1985;17:155.
Fritsch E, Herbillon AJ, Do Nascimento NR, Grimaldi M, Melfi AJ. From Plinthic Acrisols to Plinthosols and Gleysols: iron and groundwater dynamics in the tertiary sediments of the upper Amazon basin. Eur J Soil Sci. 2007;58:989–1006.
Chacon N, Flores S, Gonzalez A. Implications of iron solubilization on soil phosphorus release in seasonally flooded forests of the lower Orinoco River, Venezuela. Soil Biol Biochem. 2006;38:1494–9.
Allié E, Pélissier R, Engel J, Petronelli P, Freycon V, Deblauwe V, et al. Pervasive Local-Scale Tree-Soil Habitat Association in a Tropical Forest Community. PLoS ONE. 2015;10:e0141488.
Me Chave JR, Ra BR, Dubois M-A. Estimation of biomass in a neotropical forest of French Guiana: spatial and temporal variability. J Trop Ecol. 2001;17:79–96.
Gourlet-Fleury S, Guehl J-M, Laroussinie O. Ecology and management of a neotropical rainforest: lessons drawn from Paracou, a long-term experimental research site in French Guiana. Elseiver. 2004.
Courtois EA, Stahl C, Van den Berge J, Bréchet L, Van Langenhove L, Richter A, et al. Spatial variation of Soil CO2, CH4 and N2O Fluxes across Topographical Positions in Tropical Forests of the Guiana Shield. Ecosystems. 2018. https://doi.org/10.1007/s10021-018-0232-6 .
Aguilos M, Hérault B, Burban B, Wagner F, Bonal D. Agricultural and Forest Meteorology what drives long-term variations in carbon fl ux and balance in a tropical rainforest in French Guiana ? Agric Meteorol. 2018;253–4 February:114–23.
Soong JL, Janssens IA, Grau O, Margalef O, Stahl C, Van Langenhove L, et al. Soil properties explain tree growth and mortality, but not biomass, across phosphorus-depleted tropical forests. Sci Rep 2020. 2020;10:1.
Sinsabaugh RL, Follstad Shah JJ. Ecoenzymatic Stoichiometry and Ecological Theory. Annu Rev Ecol Evol Syst. 2012;43:313–43.
Vestergren J, Vincent AG, Jansson M, Persson P, Ilstedt U, Gröbner G, et al. High-resolution characterization of Organic Phosphorus in Soil extracts using 2D 1 H– 31 P NMR correlation spectroscopy. Environ Sci Technol. 2012;46:3950–6.
Smernik RJ, Doolette AL, Marschner P, Bu EK, Stonor R, Wakelin SA et al. Soil Biology & Biochemistry Forms of phosphorus in bacteria and fungi isolated from two Australian soils. 2008;40:1908–15.
Vestergren J, Vincent AG, Jansson M, Persson P, Ilstedt U, Gröbner G, et al. High-resolution characterization of organic phosphorus in soil extracts using 2D 1H-31P NMR correlation spectroscopy. Environ Sci Technol. 2012;46:3950–6.
Anderson MJ, Gorley RN, Clarke KR, PERMANOVA + for. PRI-MER: Guide to Software and Statistical Methods. Primer-E. Plymouth,UK.; 2008.
Smith CA, Want EJ, ’maille O, Abagyan G, Siuzdak R. XCMS: Processing Mass Spectrometry Data for Metabolite Profiling using nonlinear peak alignment, matching, and identification. Anal Chem. 2006;78:779–87.
Download references
Acknowledgements
We appreciate the cooperation of all the people that help in anything related with this research.
This research was supported by the European Research Council Synergy grant ERC-2013-SyG-610028 IMBALANCE-P, the European FP7 S-Clima project PIEF-GA-2013-626234, the Spanish Government grant PID2019-110521GB-I00, the Fundacion Ramon Areces grant ELEMENTAL-CLIMATE, and the Catalan Government grant SGR 2017 − 1005. We thank the staff of the Nouragues station managed by USR mixte LEEISA (CNRS; Cayenne) and the Paracou station managed by UMR Ecofog (CIRAD, INRA; Kourou). Both research stations benefit from “Investissement d’Avenir” grants managed by Agence Nationale de la Recherche (CEBA: ANR-10-LABX-25-01; ANAEE-France: ANR-11-INBS-0001). A.G.G. was supported by the Ministry of Education, Youth and Sports of CR within the project Mobility CzechGlobe, CZ.02.2.69/0.0/0.0/16_027/0008137. A.G.G. and O.U. were also supported by the project SustES (CZ.02.1.01/0.0/0.0/16_019/0000797).
Author information
Authors and affiliations.
Global Change Research Institute, The Czech Academy of Sciences, Belidla 986/4a, Brno, CZ-60300, Czech Republic
Albert Gargallo-Garriga
Universitat Autònoma de Barcelona, Bellaterra, 08193, Spain
Albert Gargallo-Garriga & Marta Ayala-Roque
Global Ecology Unit, CSIC, CREAF-CSIC-UAB, Bellaterra, 08193, Catalonia, Spain
Jordi Sardans, Joan Llusià, Guille Peguero, Otmar Urban, Karel Klem & Josep Peñuelas
CREAF, Cerdanyola del vallès, Barcelona, Catalonia, 08193, Spain
Jordi Sardans, Joan Llusià, Guille Peguero & Josep Peñuelas
Centre of Excellence PLECO (Plants and Ecosystems), Department of Biology, University of Antwerp, Wilrijk, Belgium
Elodie A. Courtois & Ivan A. Janssens
Laboratoire écologie, évolution, Interactions des Systèmes Amazoniens (LEEISA), Université de Guyane, CNRS, IFREMER, Cayenne, France
Elodie A. Courtois
UMR ECOFOG - Ecologie des forêts de Guyane, Kourou cedex, 97379, France
Clément Stahl
Servei de Ressonància Magnètica Nuclear, Universitat Autònoma de Barcelona, Bellaterra, 08193, Spain
Pau Nolis, Miriam Pérez-Trujillo & Teodor Parella
Centre for Microbiology and Environmental Systems Science, University of Vienna, Althanstr. 14, Vienna, 1090, Austria
Andreas Richter
You can also search for this author in PubMed Google Scholar
Contributions
A.G.G., J.S. and J.P. designed the study with the help of all co-authors. A.G.G., J.L., G.P., M.A.R, E.C., C.S., O.U., K.K., P.N., M.P., T.P., A.R., I.J., J.S. and J.P. authors participated in the field measurements or chemical and statistical analyses. All authors contributed to the writing of the manuscript and the drafting of the figures. All authors read and approved the final version of the manuscript.
Corresponding author
Correspondence to Albert Gargallo-Garriga .
Ethics declarations
Ethical approval and consent to participate.
The owner gave permission for sampling.
Consent for publication
Not applicable.
Competing interests
The authors declare no competing interests.
Additional information
Publisher’s note.
Springer Nature remains neutral with regard to jurisdictional claims in published maps and institutional affiliations.
Electronic supplementary material
Below is the link to the electronic supplementary material.
Supplementary Material 1
Rights and permissions.
Open Access This article is licensed under a Creative Commons Attribution 4.0 International License, which permits use, sharing, adaptation, distribution and reproduction in any medium or format, as long as you give appropriate credit to the original author(s) and the source, provide a link to the Creative Commons licence, and indicate if changes were made. The images or other third party material in this article are included in the article’s Creative Commons licence, unless indicated otherwise in a credit line to the material. If material is not included in the article’s Creative Commons licence and your intended use is not permitted by statutory regulation or exceeds the permitted use, you will need to obtain permission directly from the copyright holder. To view a copy of this licence, visit http://creativecommons.org/licenses/by/4.0/ . The Creative Commons Public Domain Dedication waiver ( http://creativecommons.org/publicdomain/zero/1.0/ ) applies to the data made available in this article, unless otherwise stated in a credit line to the data.
Reprints and permissions
About this article
Cite this article.
Gargallo-Garriga, A., Sardans, J., Llusià, J. et al. Different profiles of soil phosphorous compounds depending on tree species and availability of soil phosphorus in a tropical rainforest in French Guiana. BMC Plant Biol 24 , 278 (2024). https://doi.org/10.1186/s12870-024-04907-x
Download citation
Received : 20 January 2023
Accepted : 13 March 2024
Published : 12 April 2024
DOI : https://doi.org/10.1186/s12870-024-04907-x
Share this article
Anyone you share the following link with will be able to read this content:
Sorry, a shareable link is not currently available for this article.
Provided by the Springer Nature SharedIt content-sharing initiative
- P metabolomic niche
- Metabolomics
BMC Plant Biology
ISSN: 1471-2229
- General enquiries: [email protected]
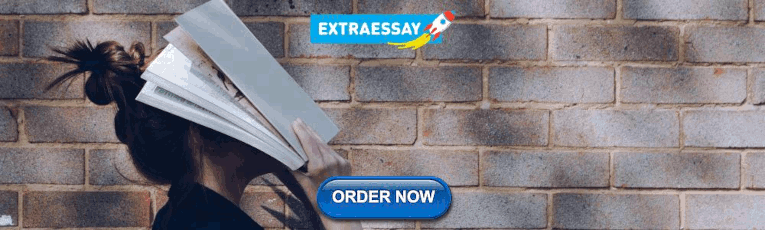
IMAGES
VIDEO
COMMENTS
5. Phrase your hypothesis in three ways. To identify the variables, you can write a simple prediction in if…then form. The first part of the sentence states the independent variable and the second part states the dependent variable. If a first-year student starts attending more lectures, then their exam scores will improve.
The alternative hypothesis (H a) is the other answer to your research question. It claims that there's an effect in the population. Often, your alternative hypothesis is the same as your research hypothesis. In other words, it's the claim that you expect or hope will be true. The alternative hypothesis is the complement to the null hypothesis.
A research hypothesis, in its plural form "hypotheses," is a specific, testable prediction about the anticipated results of a study, established at its outset. The research hypothesis is often referred to as the alternative hypothesis. ... A non-directional hypothesis, also known as a two-tailed hypothesis, predicts that there is a difference ...
A research hypothesis (also called a scientific hypothesis) is a statement about the expected outcome of a study (for example, a dissertation or thesis). To constitute a quality hypothesis, the statement needs to have three attributes - specificity, clarity and testability. Let's take a look at these more closely.
hypothesis. science. scientific hypothesis, an idea that proposes a tentative explanation about a phenomenon or a narrow set of phenomena observed in the natural world. The two primary features of a scientific hypothesis are falsifiability and testability, which are reflected in an "If…then" statement summarizing the idea and in the ...
The hypothesis of Andreas Cellarius, showing the planetary motions in eccentric and epicyclical orbits.. A hypothesis (pl.: hypotheses) is a proposed explanation for a phenomenon.For a hypothesis to be a scientific hypothesis, the scientific method requires that one can test it. Scientists generally base scientific hypotheses on previous observations that cannot satisfactorily be explained ...
The actual test begins by considering two hypotheses.They are called the null hypothesis and the alternative hypothesis.These hypotheses contain opposing viewpoints. H 0, the —null hypothesis: a statement of no difference between sample means or proportions or no difference between a sample mean or proportion and a population mean or proportion. In other words, the difference equals 0.
A hypothesis (plural hypotheses) is a proposed explanation for an observation. The definition depends on the subject. In science, a hypothesis is part of the scientific method. It is a prediction or explanation that is tested by an experiment. Observations and experiments may disprove a scientific hypothesis, but can never entirely prove one.
This is also known as a false negative, according to the University of California, Berkeley. A hypothesis can be rejected or modified, but it can never be proved correct 100% of the time.
Empirical hypothesis. Also referred to as the working hypothesis, an empirical hypothesis claims a theory's validation via experiments and observation. This way, the statement appears justifiable and different from a wild guess. ... a hypothesis is defined as "An idea or explanation of something that is based on a few known facts, but that ...
A hypothesis is a tentative statement about the relationship between two or more variables. Find hypothesis examples and how to format your research hypothesis. ... the researcher must also define how the variable will be manipulated and measured in the study. ... (known as the independent variable) and measures the effect on another variable ...
Hypothesis testing involves two statistical hypotheses. The first is the null hypothesis (H 0) as described above.For each H 0, there is an alternative hypothesis (H a) that will be favored if the null hypothesis is found to be statistically not viable.The H a can be either nondirectional or directional, as dictated by the research hypothesis. For example, if a researcher only believes the new ...
hypothesis: [noun] an assumption or concession made for the sake of argument. an interpretation of a practical situation or condition taken as the ground for action.
A hypothesis is 'a guess or supposition as to the existence of some fact or law which will serve to explain a connection of facts already known to exist.' - J. E. Creighton & H. R. Smart Hypothesis is 'a proposition not known to be definitely true or false, examined for the sake of determining the consequences which would follow from ...
An empirical hypothesis is the opposite of a logical hypothesis. It is a hypothesis that is currently being tested using scientific analysis. We can also call this a 'working hypothesis'. We can to separate research into two types: theoretical and empirical. Theoretical research relies on logic and thought experiments.
Hypothesis: Also known as. For hypothesis, the word supposition is also found.. The word conjecture is frequently encountered, usually in the context of a statement whose truth value has remained unresolved for a considerable time after the passing of the one who first raised the question.. However, this usage is inconsistent. The term open question is also encountered, usually in the context ...
If the hypothesis is a relational hypothesis, then it should be stating the relationship between variables. The hypothesis must be specific and should have scope for conducting more tests. The way of explanation of the hypothesis must be very simple and it should also be understood that the simplicity of the hypothesis is not related to its ...
A hypothesis is also known as an _____. ... True or false: All business managers need a basic understanding of hypothesis testing. TRUE. Even though repeated hypothesis tests could result in no strong conflicts between the observed data and the null hypothesis, one would still not state the null has been proved, one would state that they would ...
Study with Quizlet and memorize flashcards containing terms like A directional hypothesis is also known as a _____ hypothesis and a non-directional hypothesis is also known as a _____ hypothesis., In a study of the effects of exercise on stress, researchers predict simply that there will be a difference in stress level between the exercise and no exercise groups. The researchers are using a ...
Sapir also did pioneering work in language and gender, historical linguistics, psycholinguistics and in the study of a number of native American languages. However, he is best known for his contributions to what later became known as the Whorfian Hypothesis, also known as the Sapir-Whorf Hypothesis. Sapir maintained that language was "the ...
Their joint theory, known as the Sapir-Whorf Hypothesis or, more commonly, the Theory of Linguistic Relativity, holds great significance in all scopes of communication theories. ... Also, there are no words for minutes, minutes, or days of the week. Native Hopi speakers often had great difficulty adapting to life in the English-speaking world ...
The Sapir-Whorf Hypothesis, also known as the linguistic relativity hypothesis, is a theory in linguistics and cognitive science that posits that the structure of a language influences the way its speakers perceive and think about the world. This hypothesis is named after its proponents, American linguists Edward Sapir and Benjamin Lee Whorf ...
The Sapir-Whorf Hypothesis, also known as linguistic relativity, refers to the idea that the language a person speaks can influence their worldview, thought, and even how they experience and understand the world. While more extreme versions of the hypothesis have largely been discredited, a growing body of research has demonstrated that ...
The conjecture that all the non-trivial zeros of these functions are aligned along this axis of the complex s-plane is known as the Generalised Riemann Hypothesis (GRH). ... (s\right)=\frac{1}{2}\), there are also infinitely many pairs of solutions away from this axis and symmetrically placed with respect to it. Article PDF. Download to read ...
Background The availability of soil phosphorus (P) often limits the productivities of wet tropical lowland forests. Little is known, however, about the metabolomic profile of different chemical P compounds with potentially different uses and about the cycling of P and their variability across space under different tree species in highly diverse tropical rainforests. Results We hypothesised ...