Artificial intelligence in strategy
Can machines automate strategy development? The short answer is no. However, there are numerous aspects of strategists’ work where AI and advanced analytics tools can already bring enormous value. Yuval Atsmon is a senior partner who leads the new McKinsey Center for Strategy Innovation, which studies ways new technologies can augment the timeless principles of strategy. In this episode of the Inside the Strategy Room podcast, he explains how artificial intelligence is already transforming strategy and what’s on the horizon. This is an edited transcript of the discussion. For more conversations on the strategy issues that matter, follow the series on your preferred podcast platform .
Joanna Pachner: What does artificial intelligence mean in the context of strategy?
Yuval Atsmon: When people talk about artificial intelligence, they include everything to do with analytics, automation, and data analysis. Marvin Minsky, the pioneer of artificial intelligence research in the 1960s, talked about AI as a “suitcase word”—a term into which you can stuff whatever you want—and that still seems to be the case. We are comfortable with that because we think companies should use all the capabilities of more traditional analysis while increasing automation in strategy that can free up management or analyst time and, gradually, introducing tools that can augment human thinking.
Joanna Pachner: AI has been embraced by many business functions, but strategy seems to be largely immune to its charms. Why do you think that is?
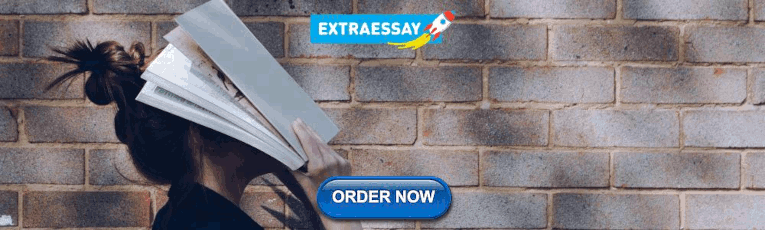
Subscribe to the Inside the Strategy Room podcast
Yuval Atsmon: You’re right about the limited adoption. Only 7 percent of respondents to our survey about the use of AI say they use it in strategy or even financial planning, whereas in areas like marketing, supply chain, and service operations, it’s 25 or 30 percent. One reason adoption is lagging is that strategy is one of the most integrative conceptual practices. When executives think about strategy automation, many are looking too far ahead—at AI capabilities that would decide, in place of the business leader, what the right strategy is. They are missing opportunities to use AI in the building blocks of strategy that could significantly improve outcomes.
I like to use the analogy to virtual assistants. Many of us use Alexa or Siri but very few people use these tools to do more than dictate a text message or shut off the lights. We don’t feel comfortable with the technology’s ability to understand the context in more sophisticated applications. AI in strategy is similar: it’s hard for AI to know everything an executive knows, but it can help executives with certain tasks.
When executives think about strategy automation, many are looking too far ahead—at AI deciding the right strategy. They are missing opportunities to use AI in the building blocks of strategy.
Joanna Pachner: What kind of tasks can AI help strategists execute today?
Yuval Atsmon: We talk about six stages of AI development. The earliest is simple analytics, which we refer to as descriptive intelligence. Companies use dashboards for competitive analysis or to study performance in different parts of the business that are automatically updated. Some have interactive capabilities for refinement and testing.
The second level is diagnostic intelligence, which is the ability to look backward at the business and understand root causes and drivers of performance. The level after that is predictive intelligence: being able to anticipate certain scenarios or options and the value of things in the future based on momentum from the past as well as signals picked in the market. Both diagnostics and prediction are areas that AI can greatly improve today. The tools can augment executives’ analysis and become areas where you develop capabilities. For example, on diagnostic intelligence, you can organize your portfolio into segments to understand granularly where performance is coming from and do it in a much more continuous way than analysts could. You can try 20 different ways in an hour versus deploying one hundred analysts to tackle the problem.
Predictive AI is both more difficult and more risky. Executives shouldn’t fully rely on predictive AI, but it provides another systematic viewpoint in the room. Because strategic decisions have significant consequences, a key consideration is to use AI transparently in the sense of understanding why it is making a certain prediction and what extrapolations it is making from which information. You can then assess if you trust the prediction or not. You can even use AI to track the evolution of the assumptions for that prediction.
Those are the levels available today. The next three levels will take time to develop. There are some early examples of AI advising actions for executives’ consideration that would be value-creating based on the analysis. From there, you go to delegating certain decision authority to AI, with constraints and supervision. Eventually, there is the point where fully autonomous AI analyzes and decides with no human interaction.
Because strategic decisions have significant consequences, you need to understand why AI is making a certain prediction and what extrapolations it’s making from which information.
Joanna Pachner: What kind of businesses or industries could gain the greatest benefits from embracing AI at its current level of sophistication?
Yuval Atsmon: Every business probably has some opportunity to use AI more than it does today. The first thing to look at is the availability of data. Do you have performance data that can be organized in a systematic way? Companies that have deep data on their portfolios down to business line, SKU, inventory, and raw ingredients have the biggest opportunities to use machines to gain granular insights that humans could not.
Companies whose strategies rely on a few big decisions with limited data would get less from AI. Likewise, those facing a lot of volatility and vulnerability to external events would benefit less than companies with controlled and systematic portfolios, although they could deploy AI to better predict those external events and identify what they can and cannot control.
Third, the velocity of decisions matters. Most companies develop strategies every three to five years, which then become annual budgets. If you think about strategy in that way, the role of AI is relatively limited other than potentially accelerating analyses that are inputs into the strategy. However, some companies regularly revisit big decisions they made based on assumptions about the world that may have since changed, affecting the projected ROI of initiatives. Such shifts would affect how you deploy talent and executive time, how you spend money and focus sales efforts, and AI can be valuable in guiding that. The value of AI is even bigger when you can make decisions close to the time of deploying resources, because AI can signal that your previous assumptions have changed from when you made your plan.
Joanna Pachner: Can you provide any examples of companies employing AI to address specific strategic challenges?
Yuval Atsmon: Some of the most innovative users of AI, not coincidentally, are AI- and digital-native companies. Some of these companies have seen massive benefits from AI and have increased its usage in other areas of the business. One mobility player adjusts its financial planning based on pricing patterns it observes in the market. Its business has relatively high flexibility to demand but less so to supply, so the company uses AI to continuously signal back when pricing dynamics are trending in a way that would affect profitability or where demand is rising. This allows the company to quickly react to create more capacity because its profitability is highly sensitive to keeping demand and supply in equilibrium.
Joanna Pachner: Given how quickly things change today, doesn’t AI seem to be more a tactical than a strategic tool, providing time-sensitive input on isolated elements of strategy?
Yuval Atsmon: It’s interesting that you make the distinction between strategic and tactical. Of course, every decision can be broken down into smaller ones, and where AI can be affordably used in strategy today is for building blocks of the strategy. It might feel tactical, but it can make a massive difference. One of the world’s leading investment firms, for example, has started to use AI to scan for certain patterns rather than scanning individual companies directly. AI looks for consumer mobile usage that suggests a company’s technology is catching on quickly, giving the firm an opportunity to invest in that company before others do. That created a significant strategic edge for them, even though the tool itself may be relatively tactical.
Joanna Pachner: McKinsey has written a lot about cognitive biases and social dynamics that can skew decision making. Can AI help with these challenges?
Yuval Atsmon: When we talk to executives about using AI in strategy development, the first reaction we get is, “Those are really big decisions; what if AI gets them wrong?” The first answer is that humans also get them wrong—a lot. [Amos] Tversky, [Daniel] Kahneman, and others have proven that some of those errors are systemic, observable, and predictable. The first thing AI can do is spot situations likely to give rise to biases. For example, imagine that AI is listening in on a strategy session where the CEO proposes something and everyone says “Aye” without debate and discussion. AI could inform the room, “We might have a sunflower bias here,” which could trigger more conversation and remind the CEO that it’s in their own interest to encourage some devil’s advocacy.
We also often see confirmation bias, where people focus their analysis on proving the wisdom of what they already want to do, as opposed to looking for a fact-based reality. Just having AI perform a default analysis that doesn’t aim to satisfy the boss is useful, and the team can then try to understand why that is different than the management hypothesis, triggering a much richer debate.
In terms of social dynamics, agency problems can create conflicts of interest. Every business unit [BU] leader thinks that their BU should get the most resources and will deliver the most value, or at least they feel they should advocate for their business. AI provides a neutral way based on systematic data to manage those debates. It’s also useful for executives with decision authority, since we all know that short-term pressures and the need to make the quarterly and annual numbers lead people to make different decisions on the 31st of December than they do on January 1st or October 1st. Like the story of Ulysses and the sirens, you can use AI to remind you that you wanted something different three months earlier. The CEO still decides; AI can just provide that extra nudge.
Joanna Pachner: It’s like you have Spock next to you, who is dispassionate and purely analytical.
Yuval Atsmon: That is not a bad analogy—for Star Trek fans anyway.
Joanna Pachner: Do you have a favorite application of AI in strategy?
Yuval Atsmon: I have worked a lot on resource allocation, and one of the challenges, which we call the hockey stick phenomenon, is that executives are always overly optimistic about what will happen. They know that resource allocation will inevitably be defined by what you believe about the future, not necessarily by past performance. AI can provide an objective prediction of performance starting from a default momentum case: based on everything that happened in the past and some indicators about the future, what is the forecast of performance if we do nothing? This is before we say, “But I will hire these people and develop this new product and improve my marketing”— things that every executive thinks will help them overdeliver relative to the past. The neutral momentum case, which AI can calculate in a cold, Spock-like manner, can change the dynamics of the resource allocation discussion. It’s a form of predictive intelligence accessible today and while it’s not meant to be definitive, it provides a basis for better decisions.
Joanna Pachner: Do you see access to technology talent as one of the obstacles to the adoption of AI in strategy, especially at large companies?
Yuval Atsmon: I would make a distinction. If you mean machine-learning and data science talent or software engineers who build the digital tools, they are definitely not easy to get. However, companies can increasingly use platforms that provide access to AI tools and require less from individual companies. Also, this domain of strategy is exciting—it’s cutting-edge, so it’s probably easier to get technology talent for that than it might be for manufacturing work.
The bigger challenge, ironically, is finding strategists or people with business expertise to contribute to the effort. You will not solve strategy problems with AI without the involvement of people who understand the customer experience and what you are trying to achieve. Those who know best, like senior executives, don’t have time to be product managers for the AI team. An even bigger constraint is that, in some cases, you are asking people to get involved in an initiative that may make their jobs less important. There could be plenty of opportunities for incorporating AI into existing jobs, but it’s something companies need to reflect on. The best approach may be to create a digital factory where a different team tests and builds AI applications, with oversight from senior stakeholders.
The big challenge is finding strategists to contribute to the AI effort. You are asking people to get involved in an initiative that may make their jobs less important.
Joanna Pachner: Do you think this worry about job security and the potential that AI will automate strategy is realistic?
Yuval Atsmon: The question of whether AI will replace human judgment and put humanity out of its job is a big one that I would leave for other experts.
The pertinent question is shorter-term automation. Because of its complexity, strategy would be one of the later domains to be affected by automation, but we are seeing it in many other domains. However, the trend for more than two hundred years has been that automation creates new jobs, although ones requiring different skills. That doesn’t take away the fear some people have of a machine exposing their mistakes or doing their job better than they do it.
Joanna Pachner: We recently published an article about strategic courage in an age of volatility that talked about three types of edge business leaders need to develop. One of them is an edge in insights. Do you think AI has a role to play in furnishing a proprietary insight edge?
Yuval Atsmon: One of the challenges most strategists face is the overwhelming complexity of the world we operate in—the number of unknowns, the information overload. At one level, it may seem that AI will provide another layer of complexity. In reality, it can be a sharp knife that cuts through some of the clutter. The question to ask is, Can AI simplify my life by giving me sharper, more timely insights more easily?
Joanna Pachner: You have been working in strategy for a long time. What sparked your interest in exploring this intersection of strategy and new technology?
Yuval Atsmon: I have always been intrigued by things at the boundaries of what seems possible. Science fiction writer Arthur C. Clarke’s second law is that to discover the limits of the possible, you have to venture a little past them into the impossible, and I find that particularly alluring in this arena.
AI in strategy is in very nascent stages but could be very consequential for companies and for the profession. For a top executive, strategic decisions are the biggest way to influence the business, other than maybe building the top team, and it is amazing how little technology is leveraged in that process today. It’s conceivable that competitive advantage will increasingly rest in having executives who know how to apply AI well. In some domains, like investment, that is already happening, and the difference in returns can be staggering. I find helping companies be part of that evolution very exciting.
Explore a career with us
Related articles.

Strategic courage in an age of volatility
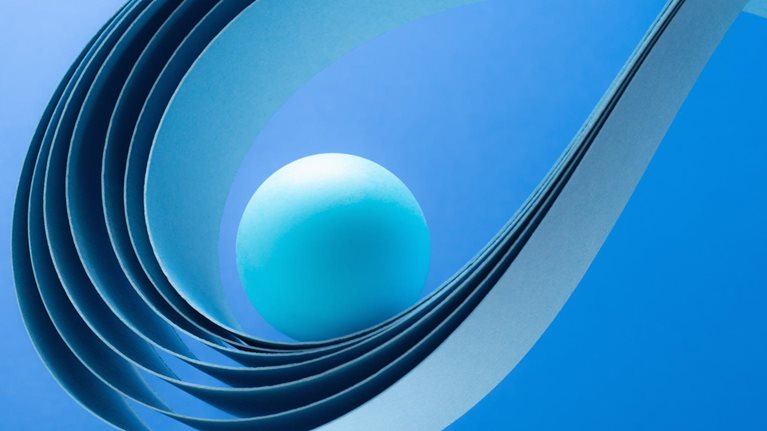
Bias Busters Collection
- Share full article
Advertisement
Supported by
Canadian Arrests Highlight Alleged Gang Role in India’s Intelligence Operations
India’s external spy agency, the Research and Analysis Wing, has long been accused of tapping into criminal networks to carry out operations in South Asia. Is the agency now doing similar operations in the West?

By Mujib Mashal and Suhasini Raj
Reporting from New Delhi
Months after Prime Minister Justin Trudeau of Canada accused India’s government of plotting a murder on Canadian soil — plunging diplomatic relations between the two countries to their lowest level ever — the first arrests in the killing, which came on Friday, did little to demystify the basis of his claim.
The police didn’t offer clues or present any evidence that India had orchestrated the killing of Hardeep Singh Nijjar, a Sikh nationalist leader who was gunned down at the temple he led in Surrey, British Columbia, in June. What they did say was that three Indian men had committed the killing and that an investigation into India’s role was ongoing.
Before the arrests, Indian officials had maintained that Canada was trying to drag New Delhi into what it described as essentially a rivalry between gangs whose members were long wanted for crimes back in India.
After the arrests, a report from the CBC, Canada’s public broadcasting corporation , based on anonymous sources, also said the suspects belonged to an Indian criminal gang.
But analysts and former officials said that the possible role of a gang in the killing does not necessarily mean the Indian government was not involved in the crime.
India’s external spy agency, the Research and Analysis Wing, or RAW, has long been suspected of tapping into criminal networks to carry out operations in its immediate neighborhood in South Asia while maintaining deniability.
Canada’s accusation, if proven, that India orchestrated the Nijjar killing — and a similar accusation made soon after by the United States in a different case — may suggest that RAW is now extending its playbook of working with criminals to carry out operations in Western countries, analysts said.
U.S. officials have produced strong evidence in their accusation that an agent of the Indian government participated in a foiled attempt to assassinate a dual American-Canadian citizen. And Canada and allied officials have maintained that Canada has evidence supporting Mr. Trudeau’s claim that Indian agents carried out Mr. Nijjar’s killing.
But the Canadian failure to reveal any evidence that India took part, nine months after Mr. Trudeau’s explosive allegation, leaves the killing of Mr. Nijjar in the realm of accusations and counter-accusation in what is a highly tense political environment in both countries, analysts said.
Prime Minister Narendra Modi has been flexing his muscles as a nationalist strongman, pitching himself during his ongoing campaign for a third-term in office as a protector of India who would go as far as it takes to target security threats.
During speeches, he has boasted about how his government eliminates enemies by “descending in their homes.” While he has made those references in relation to the country’s archenemy — Pakistan — right wing accounts on social media had celebrated the slaying of Mr. Nijjar in Canada as a similar reach of Mr. Modi’s long arm.
Mr. Trudeau, on the other hand, had been facing criticism of weakness in the face of Chinese election interference activities on Canadian soil, and his getting ahead of the Nijjar killing was seen as compensating for that.
Canadian police announced on Friday that they had arrested the three Indian men in Edmonton, Alberta, the same day and charged them with first-degree murder and conspiracy to commit murder in the killing of Mr. Nijjar. The suspects had been living in Canada for three to five years but were not permanent residents of Canada, the police said.
The gang that the CBC reported that the hit-men are connected to is led by Lawrence Bishnoi, 31, who is accused of several cases of murder, extortion and narcotics trafficking. He has orchestrated much of it from an Indian jail, where he has been held since 2014 . His members are seen as being behind the murder of a popular Punjabi rapper, and threats of attacks on Bollywood celebrities.
Indian security officials have frequently arrested criminals connected to Mr. Bishnoi, often with allegations that the gang’s network stretched as far as Canada and overlapped with those promoting from Canadian soil the cause of Khalistan, a once deeply violent separatist movement with the goal of carving out the Indian state of Punjab as an independent nation.
A large Sikh diaspora resides in Canada, many of them having migrated there after a violent and often indiscriminate crackdown by the Indian government in the 1980s against the movement for an independent Khalistan. While the cause has largely died down inside India, it continues to have supporters among some segments of the diaspora. The Indian government has accused Canada, and several other Western countries, of not doing enough to crack down on the separatists.
Analysts and former security officials said that in India’s immediate geographic neighborhood, RAW has often been willing to venture into murky spaces to recruit killers. Senior officials of Mr. Modi’s administration, including Ajit Doval, the storied former spymaster who now serves as his longtime national security adviser, have in the past been accused of reaching into the underworld to find hit men willing to go after targets both inside the country as well as abroad.
Mr. Bishnoi has demonstrated enormous power from behind bars, even giving a television interview from jail last year to pitch himself as a nationalist warrior rather than a criminal mastermind. That, one former security official said, was a signal of his trying to align himself with the spirit of nationalism for a potential deal.
“I am a nationalist,” Mr. Bishnoi said in that interview. “I am against Khalistan. I am against Pakistan.”
Ajai Sahni, a security analyst who runs the South Asia Terrorism Portal in New Delhi, said the exploitation of criminal gangs by spy agencies to carry out operations with deniability was something that “happens all over the world.”
“It is definitely possible for agencies like RAW to use gang rivalries instead of exposing their own covert operators,” Mr. Sahni added. “But just because that is generally how one would expect it to be done, it doesn’t necessarily mean we know this is exactly the case in Nijjar’s killing.”
The failed plot on American soil had some of the sloppy hallmarks of an agency trying to extend an old playbook into a different, unfamiliar space.
A U.S. indictment in November laid out evidence, including electronic communication and cash transactions between the hired hit man — who turned out to be an undercover cop — a boastful middleman, and an Indian intelligence handler whom The Washington Post recently identified as Vikram Yadav .
The Indian government’s response suggested worry: India’s top diplomat said the action was not government policy, while the government announced an investigation into the matter and promised cooperation with the United States.
Canada’s case has played out very differently. The country has not publicly disclosed any evidence backing up Mr. Trudeau’s claim, even as allied officials said in September that Canadian officials had found a “smoking gun”: intercepted communications of Indian diplomats in Canada indicating involvement in the plot.
Indian officials have pushed back against Mr. Trudeau’s claims with the kind of aggression that suggested it either wasn’t involved or that it was confident of its deniability.
The Indian government expelled Canadian diplomats , and doubled down by putting out a list of individuals on Canadian soil that it said were long wanted as part of what it described as a crime and terror nexus.
Last week, officials in Mr. Modi’s government jumped on scenes of an event that Mr. Trudeau had attended to say it showed his accusations were simply to appease what they say is a Sikh vote bank for him. They pointed to videos of an event where Mr. Trudeau was the chief guest and where chants of “long live Khalistan” were shouted. Mr. Trudeau, in his speech, said he will always be there “to protect your rights and your freedoms, and we will always defend your community against hatred.”
After the speech, the Indian foreign ministry summoned Canada’s second highest ranking diplomat in New Delhi to lodge a complaint.
“His remarks to us illustrates once again the kind of political space that has been given in Canada to separatism, extremism and people who practice violence,” Randhir Jaiswal, the foreign ministry spokesman, said at a news conference.
Mujib Mashal is the South Asia bureau chief for The Times, helping to lead coverage of India and the diverse region around it, including Bangladesh, Sri Lanka, Nepal and Bhutan. More about Mujib Mashal
Suhasini Raj is a reporter based in New Delhi who has covered India for The Times since 2014. More about Suhasini Raj
A data-driven robust decision-making model for configuring a resilient and responsive relief supply chain under mixed uncertainty
- Original Research
- Published: 15 May 2024
Cite this article
- Bahar Javan-Molaei 1 ,
- Reza Tavakkoli-Moghaddam ORCID: orcid.org/0000-0002-6757-926X 2 , 3 ,
- Mohssen Ghanavati-Nejad 1 &
- Amin Asghari-Asl 4
The crucial role of the Relief Supply Chains (RSCs) in the response phase of disaster management is undeniable. However, the literature shows that the simultaneous consideration of the resilience and responsiveness dimensions in designing the RSCs under mixed uncertainty has been ignored by researchers. In this regard, to cover the mentioned gap, the current study aims to configure an RSC by considering two critically important features namely resilience and responsiveness under mixed uncertainty. For this purpose, this work proposed a multi-stage Decision-Making Framework (DMF). In the first stage, a Multi-Objective Model (MOM) is proposed that minimizes the total cost, maximizes the responsiveness level, and maximizes the resilience of the RSC. In the second stage, to deal with mixed uncertainty, a data-driven robust approach based on the Fuzzy Robust Stochastic (FRS), Seasonal Auto-Regressive Integrated Moving Average Exogenous (SARIMAX), and Artificial Neural Networks (ANN) methods is developed. In the third stage, to solve the proposed model, a novel variant of the goal programming method is developed. In general, the main contribution of this study is to develop a novel data-driven DMF to design a resilient-responsive RSC. To show the applicability and efficiency of the developed decision-making method, a real-world case study, the flood that happened in 2019 in Golestan province, Iran, is considered. Eventually, sensitivity analysis, managerial insights, and theoretical implications are presented. According to the achieved results, primary suppliers 1, 3, 5, and 7 and also backup supplier 1 are selected. Also, the results demonstrate that distribution centers 1, 2, 3, and 5 are established. Moreover, the optimal utilization of different transportation modes is specified in the achieved results. The outputs demonstrate that the developed data-driven FRS approach has better performance in comparison with the deterministic and traditional FRS models. Besides, the outputs indicate that the developed solution method has better performance in comparison with the traditional approaches.
This is a preview of subscription content, log in via an institution to check access.
Access this article
Price includes VAT (Russian Federation)
Instant access to the full article PDF.
Rent this article via DeepDyve
Institutional subscriptions
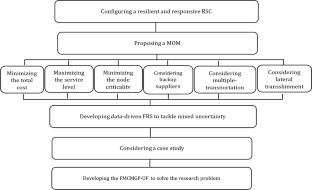
Data availability
All data generated or analyzed during this research are available from the corresponding author upon reasonable request.
Abiodun, O. I., Jantan, A., Omolara, A. E., Dada, K. V., Umar, A. M., Linus, O. U., Kazaure, A. A., Gana, U., & Kiru, M. U. (2019). Comprehensive review of artificial neural network applications to pattern recognition. IEEE Access, 7 , 158820–158846.
Article Google Scholar
Aghajani, M., Torabi, S. A., & Altay, N. (2023). Resilient relief supply planning using an integrated procurement-warehousing model under supply disruption. Omega, 118 , 102871.
Aliakbari, A., Rashidi Komijan, A., Tavakkoli-Moghaddam, R., & Najafi, E. (2022). A new robust optimization model for relief logistics planning under uncertainty: A real-case study. Soft Computing, 26 (8), 3883–3901.
Ampountolas, A. (2021). Modeling and forecasting daily hotel demand: A comparison based on sarimax, neural networks, and garch models. Forecasting, 3 (3), 580–595.
Arbabi, H., Vahedi-Nouri, B., Iranmanesh, S., & Tavakkoli-Moghaddam, R. (2022). A data-driven multi-criteria decision-making approach for assessing new product conceptual designs. Proceedings of the Institution of Mechanical Engineers, Part B: Journal of Engineering Manufacture, 236 (14), 1900–1911.
Bairamzadeh, S., Saidi-Mehrabad, M., & Pishvaee, M. S. (2018). Modelling different types of uncertainty in biofuel supply network design and planning: A robust optimization approach. Renewable Energy, 116 , 500–517.
Bankian-Tabrizi, B., Shahanaghi, K., & Jabalameli, M. S. (2012). Fuzzy multi-choice goal programming. Applied Mathematical Modelling, 36 (4), 1415–1420.
Boroun, M., Alizadeh, Z., & Jalilzadeh, A. (2023). Accelerated primal-dual scheme for a class of stochastic nonconvex-concave saddle point problems. In: 2023 American control conference (ACC) , 31 May 31-02 June 2023, San Diego, California, USA (pp. 204–209). IEEE.
Cheraghi, S., & Hosseini-Motlagh, S.-M. (2020). Responsive and reliable injured-oriented blood supply chain for disaster relief: A real case study. Annals of Operations Research, 291 (1), 129–167.
Davoodi, S. M. R., & Goli, A. (2019). An integrated disaster relief model based on covering tour using hybrid Benders decomposition and variable neighborhood search: Application in the Iranian context. Computers & Industrial Engineering, 130 , 370–380.
Dubey, R., Gunasekaran, A., & Papadopoulos, T. (2019). Disaster relief operations: Past, present and future. Annals of Operations Research, 283 , 1–8.
Dubey, R., Gunasekaran, A., Bryde, D. J., Dwivedi, Y. K., & Papadopoulos, T. (2020). Blockchain technology for enhancing swift-trust, collaboration and resilience within a humanitarian supply chain setting. International Journal of Production Research, 58 (11), 3381–3398.
Elluru, S., Gupta, H., Kaur, H., & Singh, S. P. (2019). Proactive and reactive models for disaster resilient supply chain. Annals of Operations Research, 283 (1), 199–224.
Farrokh, M., Azar, A., Jandaghi, G., & Ahmadi, E. (2018). A novel robust fuzzy stochastic programming for closed loop supply chain network design under hybrid uncertainty. Fuzzy Sets and Systems, 341 , 69–91.
Fazli-Khalaf, M., Mirzazadeh, A., & Pishvaee, M. S. (2017). A robust fuzzy stochastic programming model for the design of a reliable green closed-loop supply chain network. Human and Ecological Risk Assessment: An International Journal, 23 (8), 2119–2149.
Foroughi, A., Moghaddam, B. F., Behzadi, M. H., & Sobhani, F. M. (2022). Developing a bi-objective resilience relief logistic considering operational and disruption risks: A post-earthquake case study in Iran. Environmental Science and Pollution Research, 29 (37), 56323–56340.
Goli, A., & Malmir, B. (2020a). A covering tour approach for disaster relief locating and routing with fuzzy demand. International Journal of Intelligent Transportation Systems Research, 18 (1), 140–152.
Goli, A., Zare, H. K., Tavakkoli-Moghaddam, R., & Sadegheih, A. (2020b). Multiobjective fuzzy mathematical model for a financially constrained closed-loop supply chain with labor employment. Computational Intelligence, 36 (1), 4–34.
Goli, A., Zare, H. K., Tavakkoli-Moghaddam, R., & Sadeghieh, A. (2019). Hybrid artificial intelligence and robust optimization for a multi-objective product portfolio problem Case study: The dairy products industry. Computers & Industrial Engineering, 137 , 106090.
Homayouni, Z., Pishvaee, M. S., Jahani, H., & Ivanov, D. (2023). A robust-heuristic optimization approach to a green supply chain design with consideration of assorted vehicle types and carbon policies under uncertainty. Annals of Operations Research, 324 , 395–435.
Jamali, A., Ranjbar, A., Heydari, J., & Nayeri, S. (2022). A multi-objective stochastic programming model to configure a sustainable humanitarian logistics considering deprivation cost and patient severity. Annals of Operations Research, 319 , 1265–1300.
Kaur, H., & Singh, S. P. (2019). Sustainable procurement and logistics for disaster resilient supply chain. Annals of Operations Research, 283 (1–2), 309–354.
Kaur, H., & Singh, S. P. (2022). Disaster resilient proactive and reactive procurement models for humanitarian supply chain. Production Planning & Control, 33 (6–7), 576–589.
Kumar, P., Singh, R. K., & Shahgholian, A. (2022). Learnings from COVID-19 for managing humanitarian supply chains: Systematic literature review and future research directions. Annals of Operations Research , 1–37.
Lotfi, R., Mehrjerdi, Y. Z., Pishvaee, M. S., Sadeghieh, A., & Weber, G.-W. (2021a). A robust optimization model for sustainable and resilient closed-loop supply chain network design considering conditional value at risk. Numerical Algebra, Control & Optimization, 11 (2), 221.
Lotfi, R., Safavi, S., Gharehbaghi, A., Ghaboulian Zare, S., Hazrati, R., & Weber, G.-W. (2021b). Viable supply chain network design by considering blockchain technology and cryptocurrency. Mathematical Problems in Engineering, 2021 , 7347389.
Lotfi, R., Nazarpour, H., Gharehbaghi, A., Sarkhosh, S. M. H., & Khanbaba, A. (2022b). Viable closed-loop supply chain network by considering robustness and risk as a circular economy. Environmental Science and Pollution Research, 29 (46), 70285–70304.
Lotfi, R., Kargar, B., Gharehbaghi, A., Afshar, M., Rajabi, M. S., & Mardani, N. (2022d). A data-driven robust optimization for multi-objective renewable energy location by considering risk. Environment, Development and Sustainability , Article in Press, 1-22.
Lotfi, R., Kargar, B., Rajabzadeh, M., Hesabi, F., & Özceylan, E. (2022c). Hybrid fuzzy and data-driven robust optimization for resilience and sustainable health care supply chain with vendor-managed inventory approach. International Journal of Fuzzy Systems, 24 (2), 1216–1231.
Lotfi, R., Hazrati, R., Aghakhani, S., Afshar, M., Amra, M., & Ali, S. S. (2024). A data-driven robust optimization in viable supply chain network design by considering open innovation and blockchain technology. Journal of Cleaner Production, 436 , 140369.
Lotfi, R., Kargar, B., Gharehbaghi, A., & Weber, G.-W. (2022a). Viable medical waste chain network design by considering risk and robustness. Environmental Science and Pollution Research , 1–16.
Madani Saatchi, H., Arshadi Khamseh, A., & Tavakkoli-Moghaddam, R. (2021). Solving a new bi-objective model for relief logistics in a humanitarian supply chain by bi-objective meta-heuristic algorithms. Scientia Iranica, 28 (5), 2948–2971.
Google Scholar
Mamashli, Z., Bozorgi-Amiri, A., Dadashpour, I., Nayeri, S., & Heydari, J. (2021). A heuristic-based multi-choice goal programming for the stochastic sustainable-resilient routing-allocation problem in relief logistics. Neural Computing and Applications, 33 , 14283–14309.
Mohammadi, M., Torabi, S. A., & Tavakkoli-Moghaddam, R. (2014). Sustainable hub location under mixed uncertainty. Transportation Research Part E: Logistics and Transportation Review, 62 , 89–115.
Naor, M., & Bernardes, E. (2016). Self-sufficient healthcare logistics systems and responsiveness: Ten cases of foreign field hospitals deployed to disaster relief supply chains. Journal of Operations and Supply Chain Management, 9 (1), 1–22.
Nayeri, S., Paydar, M. M., Asadi-Gangraj, E., & Emami, S. (2020). Multi-objective fuzzy robust optimization approach to sustainable closed-loop supply chain network design. Computers & Industrial Engineering, 148 , 106716.
Nayeri, S., Torabi, S. A., Tavakoli, M., & Sazvar, Z. (2021). A multi-objective fuzzy robust stochastic model for designing a sustainable-resilient-responsive supply chain network. Journal of Cleaner Production, 311 , 127691.
Nayeri, S., Sazvar, Z., & Heydari, J. (2022). A global-responsive supply chain considering sustainability and resiliency: Application in the medical devices industry. Socio-Economic Planning Sciences, 82 , 101303.
Nayeri, S., Khoei, M. A., Rouhani-Tazangi, M. R., GhanavatiNejad, M., Rahmani, M., & Tirkolaee, E. B. (2023). A data-driven model for sustainable and resilient supplier selection and order allocation problem in a responsive supply chain: A case study of healthcare system. Engineering Applications of Artificial Intelligence, 124 , 106511.
Nezhadroshan, A. M., Fathollahi-Fard, A. M., & Hajiaghaei-Keshteli, M. (2021). Scena. International Journal of Systems Science: Operations & Logistics, 8 (4), 321–347.
Sabouhi, F., Bozorgi-Amiri, A., & Vaez, P. (2021). Stochastic optimization for transportation planning in disaster relief under disruption and uncertainty. Kybernetes, 50 (9), 2632–2650.
Sazvar, Z., Tavakoli, M., Ghanavati-Nejad, M., & Nayeri, S. (2022). Sustainable-resilient supplier evaluation for high-consumption drugs during COVID-19 pandemic using a data-driven decision-making approach . Article in Press.
Book Google Scholar
Seraji, H., Tavakkoli-Moghaddam, R., Asian, S., & Kaur, H. (2022). An integrative location-allocation model for humanitarian logistics with distributive injustice and dissatisfaction under uncertainty. Annals of Operations Research, 319 (1), 211–257.
Shokouhifar, M., & Goli, A. (2023). Designing a resilient–sustainable supply chain network of age-differentiated blood platelets using vertical–horizontal transshipment and grey wolf optimizer. International Journal of Environmental Research and Public Health, 20 (5), 4078.
Singh, R. K., Gupta, A., & Gunasekaran, A. (2018). Analysing the interaction of factors for resilient humanitarian supply chain. International Journal of Production Research, 56 (21), 6809–6827.
Subulan, K., Taşan, A. S., & Baykasoğlu, A. (2015). A fuzzy goal programming model to strategic planning problem of a lead/acid battery closed-loop supply chain. Journal of Manufacturing Systems, 37 , 243–264.
Tafakkori, K., Jolai, F., & Tavakkoli-Moghaddam, R. (2023). Disruption-resilient supply chain entities with decentralized robust-stochastic capacity planning. Reliability Engineering & System Safety, 238 , 109447.
Tavakoli, M., Tavakkoli-Moghaddam, R., Mesbahi, R., Ghanavati-Nejad, M., & Tajally, A. (2022). Simulation of the COVID-19 patient flow and investigation of the future patient arrival using a time-series prediction model: A real-case study. Medical & Biological Engineering & Computing, 60 (4), 969–990.
Tavakoli, M., Tajally, A., Ghanavati-Nejad, M., & Jolai, F. (2023). A Markovian-based fuzzy decision-making approach for the customer-based sustainable-resilient supplier selection problem. Soft Computing, 27 (20), 15153–15184.
Timperio, G., Panchal, G. B., De Souza, R., Goh, M., & Samvedi, A. (2016). Decision making framework for emergency response preparedness: A supply chain resilience approach. In: 2016 IEEE international conference on management of innovation and technology (ICMIT) , Bangkok, Thailand, 19–22 September 2016, (pp. 78–82). IEEE.
Tirkolaee, E. B., Torkayesh, A. E., Tavana, M., Goli, A., Simic, V., & Ding, W. (2023). An integrated decision support framework for resilient vaccine supply chain network design. Engineering Applications of Artificial Intelligence, 126 , 106945.
Yousefi-Babadi, A., Bozorgi-Amiri, A., Tavakkoli-Moghaddam, R., & Govindan, K. (2023). Redesign of the sustainable wheat-flour-bread supply chain network under uncertainty: An improved robust optimization. Transportation Research Part E: Logistics and Transportation Review, 176 , 103215.
Zahiri, B., Zhuang, J., & Mohammadi, M. (2017). Toward an integrated sustainable-resilient supply chain: A pharmaceutical case study. Transportation Research Part E: Logistics and Transportation Review, 103 , 109–142.
Zhalechian, M., Tavakkoli-Moghaddam, R., Zahiri, B., & Mohammadi, M. (2016). Sustainable design of a closed-loop location-routing-inventory supply chain network under mixed uncertainty. Transportation Research Part E: Logistics and Transportation Review, 89 , 182–214.
Download references
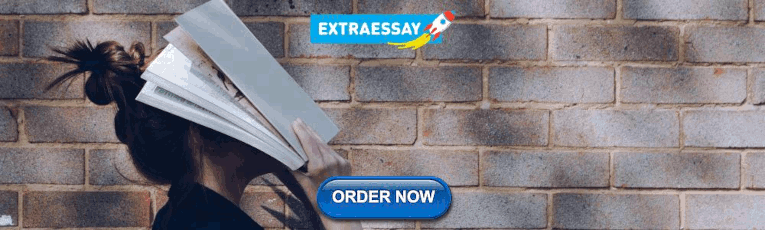
Author information
Authors and affiliations.
Department of Industrial Engineering, Kish International Campus, University of Tehran, Kish, Iran
Bahar Javan-Molaei & Mohssen Ghanavati-Nejad
School of Industrial Engineering, College of Engineering, University of Tehran, Tehran, Iran
Reza Tavakkoli-Moghaddam
Research Center of Performance and Productivity Analysis, Istinye University, Istanbul, Turkey
Department of Industrial Engineering, Sharif University of Technology, Tehran, Iran
Amin Asghari-Asl
You can also search for this author in PubMed Google Scholar
Contributions
All authors contributed to all parts of this research including Conceptualization; Data collection; Formal analysis; Investigation; Methodology; Resources; Software; Supervision; Validation; Visualization; and Writing–review & editing.
Corresponding author
Correspondence to Reza Tavakkoli-Moghaddam .
Ethics declarations
Conflict of interest.
The authors declare that there is no potential conflict of interest concerning the research, authorship, and/or publication of this paper.
Ethical approval
The authors certify that they have no affiliation with or involvement with human participants or animals performed by any of the authors in any organization or entity with any financial or non-financial interest in the subject matter or materials discussed in this paper.
Additional information
Publisher's note.
Springer Nature remains neutral with regard to jurisdictional claims in published maps and institutional affiliations.
Rights and permissions
Springer Nature or its licensor (e.g. a society or other partner) holds exclusive rights to this article under a publishing agreement with the author(s) or other rightsholder(s); author self-archiving of the accepted manuscript version of this article is solely governed by the terms of such publishing agreement and applicable law.
Reprints and permissions
About this article
Javan-Molaei, B., Tavakkoli-Moghaddam, R., Ghanavati-Nejad, M. et al. A data-driven robust decision-making model for configuring a resilient and responsive relief supply chain under mixed uncertainty. Ann Oper Res (2024). https://doi.org/10.1007/s10479-024-06038-w
Download citation
Received : 02 January 2024
Accepted : 24 April 2024
Published : 15 May 2024
DOI : https://doi.org/10.1007/s10479-024-06038-w
Share this article
Anyone you share the following link with will be able to read this content:
Sorry, a shareable link is not currently available for this article.
Provided by the Springer Nature SharedIt content-sharing initiative
- Relief supply chain
- Responsiveness
- Data-driven decision-making
- Mixed uncertainty
- Find a journal
- Publish with us
- Track your research
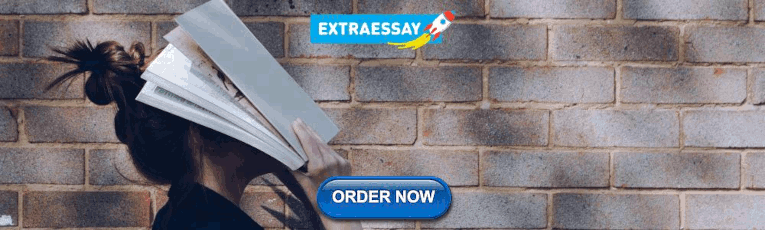
COMMENTS
problems involved in military operations. The objective was to find the most effective utilization of limited military resources by the use of quantitative techniques. Following the war, numerous peacetime applications emerged, leading to the use of OR and management science in many industries and occupations.
speaking, an O.R. project comprises three steps: (1) building a model, (2) solving it, and. (3) implementing the results. The emphasis of this chapter is on the first and third steps. The second step typically involves specific methodologies or techniques, which could be.
Operations Research provides the manager mathematical techniques, tools and various models to analyse the problem. It also helps us to evaluate the outcomes of various alternatives and make an ... Establishment of the objectives and the values related to the operation. Determination of suitable measures of effectiveness
Operations research refers to scientific methods (statistical and mathematical modeling, experiments, simulation, and optimization) applied to the solution of complex business problems. Operations ...
1.1 Objectives. The needs of using Operations Research (OR) The historical perspective of the approach of OR Several definitions of OR in different contexts and various phases of scienti fic study The classication and usage of various models for solving a problem under fi consideration Applications of OR in different areas.
31.1 Introduction. Operations research is a multidisciplinary field that is concerned with the application of mathematical and analytic techniques to assist in decision-making. It includes techniques such as mathematical modelling, statistical analysis, and mathematical optimization as part of its goal to achieve optimal (or near optimal ...
The subject matter, operations research or management science (even though there may be philosophical differences, we use the two terms interchangeably), has been defined by many researchers in the field. Definitions range from "a scientific approach to decision making" to "the use of quantitative tools for systems that originate from real life," "scientific decision making," and ...
Operations research is neither a method nor a technique; it is or is becoming a science and as such is defined by a combination of the phenomena it studies. Ackoff(1956) Abstract Throughout its history, Operational Research has evolved to include a variety of methods, models and al-gorithms that have been applied to a diverse and wide range of ...
1.6 Applications of Operations Research 1.7 Limitations of Operations Research 1.8 Summary 1.9 Key Terms 1.10 Self Assessment Questions 1.11 Further References Objectives After Studying this lesson, you should be able to: Understand the meaning, purpose, and tools of Operations Research Describe the history of Operations Research
IEOR 4004: Introduction to Operations Research - Deterministic Models. The notes were meant to provide a succint summary of the material, most of which was loosely based on the book Winston-Venkataramanan: Introduction to Mathematical Programming (4th ed.), Brooks/Cole 2003. Other material (such as the dictionary notation) was adapted
THE HISTORY, NATURE, AND USE OF OPERATIONS RESEARCH, Hugh J. Miser 3 1. The Beginnings 3 2. The Science of Operations Research 6 2.1 Science and Its Method 7 2.2 Operations Research as a Science 8 3. The Practice of Operations Research 10 4. The Growth of Operations Research, 1945-1975 11 ... Definition of Objectives 41 3. Development of the ...
Catalog Description: The development of mathematical concepts and models concerned with industrial engineering problems. Topics include queuing theory, linear and dynamic programming. Prerequisite: IEE 2610, IEE 2620. Credit/Contact Hrs: This is a 3 credit hour required course in the IEE Program and is scheduled for 3 contact hours/week.
UNIT - I - ELEMENTS OF OPERATION RESEARCH - SBAA1305
Operations Research has number of applications; similarly it also has certain limitations. These ... definition the objectives, uses and limitations of O.R. study of the problem also defined. The outputs of this step are clear grasp of need for a solution and its nature understanding.
1.6 Applications of Operations Research 1.7 Limitations of Operations Research 1.8 Summary 1.9 Key Terms 1.10 Self Assessment Questions 1.11 Further References Objectives After Studying this lesson, you should be able to: Understand the meaning, purpose, and tools of Operations Research Describe the history of Operations Research
„Operations Research‟. In India, Operations Research came into existence in 1949 with the opening of an Operations Research unit at the Regional Research Laboratory in Hyderabad and also in the Defence Science Laboratory. Operations Research gained momentum in the market place in the nineteen fifties and sixties.
The objectives of this chapter are to discuss: The needs of using Operations Research (OR) The historical perspective of the approach of OR. Several definitions of OR in different contexts and various phases of scientific study. The classification and usage of various models for solving a problem under consideration. Applications in different ...
below. (a) "Operations Research is the application of scientific methods, techniques and tools. to problems involving the operation of a system so as to provide those in controls of. the system ...
research includes both developments of theories (which is called model, in operation research) and application of this science for improvement of systems' operations (Warner, 1996). Objectives of Operation Research We can present objectives of operation research as the following: 1. Solving operational questions 2.
operations research, application of scientific methods to the management and administration of organized military, governmental, commercial, and industrial processes.. Basic aspects. Operations research attempts to provide those who manage organized systems with an objective and quantitative basis for decision; it is normally carried out by teams of scientists and engineers drawn from a ...
Course Objectives: This module aims to introduce students to use quantitive methods and techniques for effective decisions-making; model formulation and applications that are used in solving business decision problems. Course Components • Introduction to Operations Research (OR) • Introduction to Foundation mathematics and statistics
By. Sarah Lewis. Operations research (OR) is an analytical method of problem-solving and decision-making that is useful in the management of organizations. In operations research, problems are broken down into basic components and then solved in defined steps by mathematical analysis. The process of operations research can be broadly broken ...
markets operations research as the "science of better." What all of this essentially means is that the science uses indeed quantitative techniques to make and prepare ... diverging goals and objectives of multiple constituents or stakeholders when locating a new sewage treatment plants; the project manager, who has to
Joanna Pachner: Do you think this worry about job security and the potential that AI will automate strategy is realistic? Yuval Atsmon: The question of whether AI will replace human judgment and put humanity out of its job is a big one that I would leave for other experts. The pertinent question is shorter-term automation. Because of its complexity, strategy would be one of the later domains ...
Engineering, Operations & Technology Boeing Research & Technology | Applied Mathematics • Small COTS cells offer many advantages: • Inherent cell level safety devices (e.g. PTC, CID, etc.) • High reliability/high volume automated manufacturing • Improved volumetric efficiency (small cells package more efficiently)
India's external spy agency, the Research and Analysis Wing, has long been accused of tapping into criminal networks to carry out operations in South Asia. Is the agency now doing similar ...
The crucial role of the Relief Supply Chains (RSCs) in the response phase of disaster management is undeniable. However, the literature shows that the simultaneous consideration of the resilience and responsiveness dimensions in designing the RSCs under mixed uncertainty has been ignored by researchers. In this regard, to cover the mentioned gap, the current study aims to configure an RSC by ...