- Open access
- Published: 13 October 2022
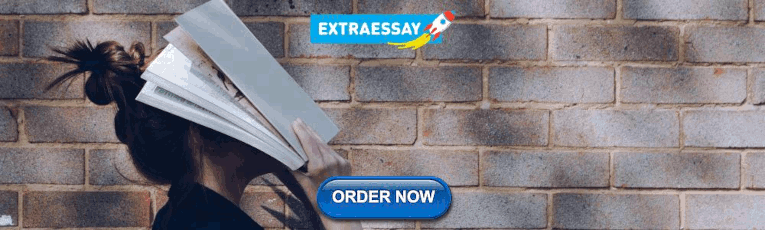
What challenges still exist in the critical care of children?
- Fola Odetola 1 &
- John Pappachan 2
BMC Pediatrics volume 22 , Article number: 592 ( 2022 ) Cite this article
1937 Accesses
1 Altmetric
Metrics details
Paediatric Intensive Care provides organ-specific support to critically ill children with a very broad range of diagnoses. A relatively new specialty, its birth followed the emergence of adult intensive care and was necessitated by developments in neonatal intensive care (that produced long term survivors with ongoing needs for critical care), paediatric cardiac surgery, and anaesthesia. The first paediatric intensive care unit (PICU) was established in Sweden in Europe in 1955, ten years before the unit at Children's Hospital of the District of Columbia was developed [ 1 , 2 ]. There are now an estimated over 300 PICUs in the United States alone [ 3 ].
Multiple quality of care factors in PICU including the existence of dedicated training programs have had a significant impact on the survival of children with critical illness [ 4 ]. Child mortality from critical illness or injury is lower in settings where a PICU is available [ 5 ] and has decreased from 1 in 5 children in the early 1990s [ 6 ], to less than 5% currently, according to recent estimates [ 7 ].
The exponential growth in pediatric intensive care research (1 citation under PICU in 1979 in PubMed compared with 904 in 2020), the development of training programmes, and advancements in technology, diagnostics and surgical/anaesthetic techniques have contributed to a remarkable reduction in unadjusted and case-mix adjusted PICU mortality, but challenges and unmet needs still exist in the critical care of children as highlighted below.
The development of the specialty has allowed for paediatric-specific definitions and a multi-disciplinary approach to the care of children with sepsis using care bundles that incorporate evidence-based approaches to ventilation, fluid resuscitation, inotropic support, and extracorporeal membrane oxygenation (ECMO) for refractory cases. This evidence-based care has been associated with improved mortality [ 8 ]. Recent international data suggest however, that although the prevalence of severe sepsis has fallen to 8.2%, the mortality in both industrialised and non-industrialised nations remains at 25% [ 9 ]. The COVID-19 pandemic has created a legacy of new research re-exploring the role of targeted immunomodulation in adults and in children with the Multi System Inflammatory Syndrome (MIS-C) [ 10 ] The future may include research exploring the use of immunomodulatory strategies in cytokine storm syndromes including severe sepsis in children.
Paediatric Acute Respiratory Distress Syndrome (PARDS)
Improvements in outcomes in adults with acute respiratory distress syndrome (ARDS) with the adoption of low tidal volume ventilation [ 11 ] and prone ventilation [ 12 ], has not been replicated in children, with mortality in severe PARDS remaining high at 33% [ 13 ]. Further, 27% of children with PARDS are managed with a level of PEEP lower than that recommended by adult guidelines, a gap in practice that is independently associated with mortality [ 14 ]. Importantly, non-adherence to lung-protective ventilation principles in PARDS has been associated with adverse outcomes [ 15 ]. These findings suggest an urgent need to close the gap between evidence and practice to enhance the outcomes for children with PARDS.
Traumatic Brain Injury (TBI)
TBI is one of the leading causes of death and long-term disability across all ages worldwide, but children and the elderly are the most at risk. Unlike the elderly, the burden of disability in children is life long and thus has far greater socioeconomic impact. The care delivered in PICU to children with TBI is limited by the poorly described but important anatomical and physiological differences between the adult and immature brain and despite this, the need to extrapolate adult data to the paediatric population because of the lack of paediatric-specific TBI research. A pragmatic update to the Brain Trauma Foundation Guidelines was published in 2019 and contained 22 recommendations, however, none were based on Level I data and only three incorporated Level II data [ 16 ]. Approaches and Decisions for Acute Pediatric TBI Trial (ADAPT) [ 17 ], a multi-national comparative effectiveness research study is under way but is likely to generate more questions than answers.
Neurodevelopmental comorbidity
Critically ill children requiring PICU admission increasingly survive but with multimorbidity. Post-intensive care syndrome-pediatrics (PICS-p) includes abnormalities in neurocognition, physical and social scores, and health-related quality of life. Two recent reports [ 18 , 19 ] have been published which raise awareness of this unresolved issue and establish a core set of outcome measures to enhance quantification of the effects of PICS-p across multiple domains. 50% of bed days in the UK are now occupied by only 10% of admissions comprised of children who have neurodevelopmental comorbidity and are often technology dependent. Moreover, many children hospitalized with critical illness have baseline developmental impairment which heightens the risk for critical illness, death and prolonged PICU stay, making them among the most vulnerable PICU patients.
Management of sedation, withdrawal and delirium
We still have a long way to go in terms of understanding the optimal management of sedation, iatrogenic medication withdrawal syndrome, and delirium in children who survive critical illness. These issues have been extensively studied in adults but less so in children in whom an additional burden of the longer-term effects of sedation on a developing brain may be much more important [ 20 ].
The survivors of critical illness often have long term neurodevelopmental morbidity and technology dependence. In addition, the availability of novel therapies for previously fatal diseases and the availability in many countries of long term ventilatory support has meant that many more technology dependent children are surviving and being admitted to PICU. There is, therefore, an urgent need to enhance our understanding of disease pathogenesis and of innovative approaches to improve diagnosis and treatment. This call for articles also seeks to discover new approaches to multidisciplinary clinical care of children in the hospital setting while appreciating the need for disease prevention and the value of post-hospital care of critically ill children. We would welcome articles dealing with basic and translational science, clinical care and health services and systems research.
The Journal is keenly interested in research articles that report findings from randomized controlled trials, trial protocols, and observational studies. Review articles on important issues in the care of critically ill children requiring PICU will also be considered.
Downes JJ. The historical evolution, current status, and prospective development of pediatric critical care. Crit Care Clin. 1992;8(1):1–22.
Article CAS Google Scholar
Berlin CM. The pediatric Intensive Care Unit. Med Ann Dist Columbia. 1970;39(9):483–6 (passim).
CAS PubMed Google Scholar
Horak RV, Griffin JF, Brown AM, Nett ST, Christie LM, Forbes ML, et al. Pediatric Acute Lung Injury and Sepsis Investigator’s (PALISI) Network. Growth and Changing Characteristics of Pediatric Intensive Care 2001–2016. Crit Care Med. 2019;47(8):1135–42.
Article Google Scholar
Pollack MM, Cuerdon TT, Patel KM, Ruttimann UE, Getson PR, Levetown M. Impact of quality-of-care factors on pediatric intensive care unit mortality. JAMA. 1994;272:941–6.
Odetola FO, Miller WC, Davis MM, Bratton SL. The relationship between the location of pediatric intensive care unit facilities and child death from trauma: a county-level ecologic study. J Pediatr. 2005;147(1):74–7. https://doi.org/10.1016/j.jpeds.2005.02.012 (PMID: 16027699).
Article PubMed Google Scholar
Butt W, Shann F, Tibballs J, et al. Long-term outcome of children after intensive care. Crit Care Med. 1990;18(9):961–5.
Pollack MM, Holubkov R, Funai T, et al. The Pediatric Risk of Mortality Score: Update 2015. Pediatr Crit Care Med. 2016;17(1):2–9.
Evans IVR, Phillips GS, Alpern ER, Angus DC, Friedrich ME, Kissoon N, et al. Association Between the New York Sepsis Care Mandate and In-Hospital Mortality for Pediatric Sepsis. JAMA. 2018;320(4):358–67.
Weiss SL, Fitzgerald JC, Pappachan J, Wheeler D, Jaramillo-Bustamante JC, Salloo A, et al. Sepsis Prevalence, Outcomes, and Therapies (SPROUT) Study Investigators and Pediatric Acute Lung Injury and Sepsis Investigators (PALISI) Network Global epidemiology of pediatric severe sepsis: the sepsis prevalence, outcomes, and therapies study. Am J Respir Crit Care Med. 2015;191(10):1147–57.
McArdle AJ, Vito O, Patel H, et al. Treatment of Multisystem Inflammatory Syndrome in Children. N Engl J Med. 2021;385(1):11–22.
Brower RG, Matthay MA, Morris A, Schoenfeld D, Thompson BT, Wheeler A. Ventilation with lower tidal volumes as compared with traditional tidal volumes for acute lung injury and the acute respiratory distress syndrome. N Engl J Med. 2000;342:1301–8.
Guérin C, Reignier J, Richard JC, Beuret P, Gacouin A, Boulain T, et al. PROSEVA Study Group. Prone positioning in severe acute respiratory distress syndrome. N Engl J Med. 2013;368(23):2159–68.
Khemani RG, Smith L, Lopez-Fernandez YM, Kwok J, Morzov R, Klein MJ, et al. Pediatric Acute Respiratory Distress Syndrome Incidence and Epidemiology (PARDIE) Investigators; Pediatric Acute Lung Injury and Sepsis Investigators (PALISI) Network. Paediatric acute respiratory distress syndrome incidence and epidemiology (PARDIE): an international, observational study. Lancet Respir Med. 2019;7(2):115–28.
Khemani RG, Parvathaneni K, Yehya N, Bhalla AK, Thomas NJ, Newth CJL. Positive End-Expiratory Pressure Lower Than the ARDS Network Protocol Is Associated with Higher Pediatric Acute Respiratory Distress Syndrome Mortality. Am J Respir Crit Care Med. 2018;198(1):77–89.
Bhalla AK, Klein MJ, Emeriaud G, Lopez-Fernandez YM, Napolitano N, Fernandez A, et al. Pediatric Acute Respiratory Distress Syndrome Incidence and Epidemiology (PARDIE) V.2. Investigators and Pediatric Acute Lung Injury and Sepsis Investigators (PALISI) Network. Adherence to Lung-Protective Ventilation Principles in Pediatric Acute Respiratory Distress Syndrome: A Pediatric Acute Respiratory Distress Syndrome Incidence and Epidemiology Study. Crit Care Med. 2021;49(10):1779–89.
Kochanek PM, Tasker RC, Carney N, et al. Guidelines for the management of pediatric severe traumatic brain injury, third edition: update of the Brain Trauma Foundation guidelines. Pediatr Crit Care Med. 2019;20(3S Suppl1):S1–82.
Kochanek PM, Adelson PD, Rosario BL, et al. Comparison of Intracranial Pressure Measurements Before and After Hypertonic Saline or Mannitol Treatment in Children With Severe Traumatic Brain Injury. JAMA Netw Open. 2022;5(3):e220891.
Heneghan JA, Sobotka SA, Hallman M, Pinto N, Killien EY, Palumbo K, et al. Outcome Measures Following Critical Illness in Children With Disabilities: A Scoping Review. Front Pediatr. 2021;2(9):689485.
Fink EL, Maddux AB, Pinto N, Sorenson S, Notterman D, Dean JM, et al. Pediatric Outcomes STudies after PICU (POST-PICU) Investigators of the Pediatric Acute Lung Injury and Sepsis Investigators (PALISI) Network and the Eunice Kennedy Shriver National Institute of Child Health and Human Development Collaborative Pediatric Critical Care Research Network (CPCCRN). A Core Outcome Set for Pediatric Critical Care. Crit Care Med. 2020;48(12):1819–28.
Agbeko RS, Argent A, MacLaren G. Mind over matter? Pain, withdrawal and sedation in paediatric critical care. Intensive Care Med. 2016;42(8):1261–3.
Download references
Author information
Authors and affiliations.
Department of Pediatrics, University of Michigan, Ann Arbor, USA
Fola Odetola
Southampton Childrens Hospital, Southampton, UK
John Pappachan
You can also search for this author in PubMed Google Scholar
Contributions
The authors jointly wrote the manuscript, read the final draft, and approved the final manuscript for publication.
Corresponding author
Correspondence to Fola Odetola .
Ethics declarations
Competing interests.
The authors declare that they have no competing interests to disclose.
Additional information
Publisher’s note.
Springer Nature remains neutral with regard to jurisdictional claims in published maps and institutional affiliations.
Rights and permissions
Open Access This article is licensed under a Creative Commons Attribution 4.0 International License, which permits use, sharing, adaptation, distribution and reproduction in any medium or format, as long as you give appropriate credit to the original author(s) and the source, provide a link to the Creative Commons licence, and indicate if changes were made. The images or other third party material in this article are included in the article's Creative Commons licence, unless indicated otherwise in a credit line to the material. If material is not included in the article's Creative Commons licence and your intended use is not permitted by statutory regulation or exceeds the permitted use, you will need to obtain permission directly from the copyright holder. To view a copy of this licence, visit http://creativecommons.org/licenses/by/4.0/ . The Creative Commons Public Domain Dedication waiver ( http://creativecommons.org/publicdomain/zero/1.0/ ) applies to the data made available in this article, unless otherwise stated in a credit line to the data.
Reprints and permissions
About this article
Cite this article.
Odetola, F., Pappachan, J. What challenges still exist in the critical care of children?. BMC Pediatr 22 , 592 (2022). https://doi.org/10.1186/s12887-022-03649-9
Download citation
Published : 13 October 2022
DOI : https://doi.org/10.1186/s12887-022-03649-9
Share this article
Anyone you share the following link with will be able to read this content:
Sorry, a shareable link is not currently available for this article.
Provided by the Springer Nature SharedIt content-sharing initiative
BMC Pediatrics
ISSN: 1471-2431
- Submission enquiries: [email protected]
- General enquiries: [email protected]
Log in using your username and password
- Search More Search for this keyword Advanced search
- Latest content
- Current issue
- BMJ Journals More You are viewing from: Google Indexer
You are here
- Volume 109, Issue 3
- Paediatric intensive care admissions of preterm children born
- Article Text
- Article info
- Citation Tools
- Rapid Responses
- Article metrics

- http://orcid.org/0000-0001-6157-1083 Tim J van Hasselt 1 ,
- http://orcid.org/0000-0003-0707-876X Chris Gale 2 ,
- http://orcid.org/0000-0002-2898-553X Cheryl Battersby 2 ,
- Peter J Davis 3 ,
- Elizabeth Draper 1 ,
- http://orcid.org/0000-0001-8711-4817 Sarah E Seaton 1
- On behalf of the United Kingdom Neonatal Collaborative and the Paediatric Critical Care Society Study Group (PCCS-SG)
- 1 Department of Population Health Sciences , University of Leicester , Leicester , UK
- 2 Neonatal Medicine, School of Public Health, Faculty of Medicine , Imperial College London , London , UK
- 3 Paediatric Intensive Care Unit, Bristol Royal Hospital for Children , University Hospitals Bristol NHS Foundation Trust , Bristol , UK
- Correspondence to Dr Tim J van Hasselt, Department of Population Health Sciences, University of Leicester, Leicester LE1 7RH, UK; t.vanhasselt{at}nhs.net
Objective Survival of babies born very preterm (<32 weeks gestational age) has increased, although preterm-born children may have ongoing morbidity. We aimed to investigate the risk of admission to paediatric intensive care units (PICUs) of children born very preterm following discharge home from neonatal care.
Design Retrospective cohort study, using data linkage of National Neonatal Research Database and the Paediatric Intensive Care Audit Network datasets.
Setting All neonatal units and PICUs in England and Wales.
Patients Children born very preterm between 1 January 2013 and 31 December 2018 and admitted to neonatal units.
Main outcome measures Admission to PICU after discharge home from neonatal care, before 2 years of age.
Results Of the 40 690 children discharged home from neonatal care, there were 2308 children (5.7%) with at least one admission to PICU after discharge. Of these children, there were 1901 whose first PICU admission after discharge was unplanned.
The percentage of children with unplanned PICU admission varied by gestation, from 10.2% of children born <24 weeks to 3.3% born at 31 weeks.
Following adjustment, unplanned PICU admission was associated with lower gestation, male sex (adjusted OR (aOR) 0.79), bronchopulmonary dysplasia (aOR 1.37), necrotising enterocolitis requiring surgery (aOR 1.39) and brain injury (aOR 1.42). For each week of increased gestation, the aOR was 0.90.
Conclusions Most babies born <32 weeks and discharged home from neonatal care do not require PICU admission in the first 2 years. The odds of unplanned admissions to PICU were greater in the most preterm and those with significant neonatal morbidity.
- intensive care units, neonatal
- intensive care units, paediatric
- paediatrics
- neonatology
Data availability statement
Data may be obtained from a third party and are not publicly available. Data may be obtained from a third party and are not publicly available. PICANet data may be requested from the data controller, the Healthcare Quality Improvement Partnership (HQIP). A Data Access Request Form can be obtained from https://www.hqip.org.uk/national-programmes/accessing-ncapop-data/%23.XQeml_lKhjU .
This is an open access article distributed in accordance with the Creative Commons Attribution 4.0 Unported (CC BY 4.0) license, which permits others to copy, redistribute, remix, transform and build upon this work for any purpose, provided the original work is properly cited, a link to the licence is given, and indication of whether changes were made. See: https://creativecommons.org/licenses/by/4.0/ .
https://doi.org/10.1136/archdischild-2023-325970
Statistics from Altmetric.com
Request permissions.
If you wish to reuse any or all of this article please use the link below which will take you to the Copyright Clearance Center’s RightsLink service. You will be able to get a quick price and instant permission to reuse the content in many different ways.
WHAT IS ALREADY KNOWN ON THIS TOPIC
Children born very preterm (<32 weeks gestational age) are at increased risk of morbidity in childhood.
For such children, survival has increased, particularly for babies born <28 weeks.
It is not known what proportion of very preterm babies discharged from neonatal units will need further intensive care.
WHAT THIS STUDY ADDS
2308 babies (5.7%) born very preterm and discharged home from neonatal care were subsequently admitted to paediatric intensive care units (PICUs) before the age of 2 years, and the majority of these admissions were unplanned.
The percentage of children with unplanned PICU admission following neonatal discharge varied by gestation, from 10.2% of babies born <24 weeks to 3.3% born at 31 weeks.
Most unplanned PICU admissions of children born very preterm and discharged home occur in the first few months after neonatal discharge.
Major neonatal morbidities (brain injury, severe necrotising enterocolitis and bronchopulmonary dysplasia) were associated with unplanned PICU admission after neonatal discharge.
HOW THIS STUDY MIGHT AFFECT RESEARCH, PRACTICE OR POLICY
Neonatal healthcare professionals may be able to identify babies at highest risk of being admitted to PICU when planning neonatal discharge.
Appropriate, sensitive and tailored discussion with families may improve understanding of this risk; we plan to work with families to develop infographics to assist these discussions.
In recent decades, survival following very preterm birth (<32 weeks gestation) has increased, both within the UK 1 and in other high-income countries. 2 Despite improvements in survival, very preterm-born children experience ongoing morbidity 3 and increased frequency of hospitalisation. 4 A proportion of children born preterm become critically ill after discharge from neonatal care, and require admission to paediatric intensive care units (PICUs), particularly due to respiratory viruses in the first years of life. 5 Previous estimates using Office for National Statistics (ONS) data suggest that up to 20%–25% of babies born <25 weeks in the UK may be admitted to PICU in the first 2 years of life, 6 however to our knowledge no study has been published within the UK or internationally using data linkage of national neonatal and PICU datasets to examine PICU admissions of preterm-born children.
The risk of requiring PICU admission after neonatal discharge for very preterm-born children is uncertain, as are factors that affect this risk. Understanding these may be helpful for counselling families of very preterm children, and for neonatal discharge planning. We aimed to use a novel linkage of the National Neonatal Research Database (NNRD) and Paediatric Intensive Care Audit Network (PICANet) datasets to investigate the risk of, and risk factors associated with, admission to PICU after neonatal discharge for babies born at 22–31 weeks gestational age.
We identified all babies born at <32 weeks gestational age from 1 January 2013 to 31 December 2018, and admitted for neonatal care in England and Wales. All babies born at this gestation should all receive neonatal care and therefore have associated NNRD data. We excluded babies born <22 weeks, and those whose neonatal admissions were recorded as occurring after day 1. Each child was followed up until 2 years of age (a period when children are particularly susceptible to respiratory infections) to investigate if they were admitted to PICUs in England and Wales.
We identified children discharged home from neonatal units, and classified subsequent PICU admission in two ways: (1) ‘PICU admission from home’ was identified as PICU admission at least 24 hours following neonatal discharge home; (2) ‘unplanned PICU admissions’, a subset of (1), excluding elective PICU admissions such as those for planned surgery.
Previous PICU admissions during the neonatal stay, with return to the neonatal unit, were not examined, although these children were included within the cohort if they were subsequently discharged home from the neonatal unit.
Information about the care the babies received in the neonatal unit was provided by the NNRD, which captures demographic and clinical data related to neonatal unit admissions, daily clinical care and discharges from all neonatal units in England since 2012 and Wales since 2013. 7 This was linked with PICU admissions provided by the PICANet, a national audit database of demographic and clinical data collected from every PICU admission across the UK and Ireland, with complete coverage for England and Wales from 2003. 8 Data are submitted to PICANet within three months of a child’s discharge, and subsequently data are subject to validation before any analysis.
Personal identifiers for babies and children (NHS number; date of birth; surname; postcode) were provided by NNRD and PICANet to NHS Digital (now NHS England) who identified children common to both cohorts and provided pseudonymised linked data. Over 99% of children had unique NHS numbers in both datasets, for whom probabilistic linkage was not required. Linked information on deaths in the first 2 years of life was provided by the ONS.
Statistical analysis
We performed descriptive statistics of the cohort, presenting frequencies with percentages for categorical variables, mean with SD for parametric variables and median with IQR for non-parametric variables. We calculated postmenstrual age (PMA) at neonatal discharge and at first unplanned PICU admission.
Our primary analysis was a logistic regression model predicting unplanned PICU admission using characteristics from the neonatal period. Variables were selected for inclusion by a multidisciplinary advisory panel ( online supplemental table 1 ). The selected variables were: gestation, sex, small for gestational age (SGA) and the major neonatal morbidities of bronchopulmonary dysplasia (BPD) requiring oxygen at 36 weeks, severe necrotising enterocolitis (NEC) requiring surgery 9 and brain injury. Brain injury included grade III/IV intraventricular haemorrhage, periventricular leukomalacia, hypoxic ischaemic encephalopathy, meningitis and seizures after exclusions for congenital or inherited causes. 10 The major neonatal morbidities also reflected the core outcome set for neonatal research. 11
Supplemental material
Implausible birth weights over 3 SD from the median for gestation and sex, 12 or <300 g/>2500 g, were excluded from multivariable models. SGA was defined as birth weight <10th centile for gestation and sex using centiles defined elsewhere. 13 14
Gestational age in completed weeks was modelled linearly, with sensitivity analysis re-fitting the model using categorisation of gestation at birth to investigate the robustness of this approach. The primary model and sensitivity analyses were compared using the Akaike Information Criterion (AIC) for model quality, 15 Hosmer-Lemeshow 16 and link tests 17 for model fit, Brier’s score 18 and c-statistic for predictive ability 19 and variance inflation factor for multicollinearity. 20
Repeat PICU admission in the first 2 years was explored graphically using a Sankey diagram, created using SankeyMATIC (sankeymatic.com).
There were 46 698 children born <32 weeks between 2013 and 2018 and admitted to neonatal units within England and Wales. After exclusions for neonatal admission after day 1 (n=13), and gestation <22 weeks (n=1), there were 46 684 children ( figure 1 ). In total, 3929 babies died in neonatal care and 2065 were discharged to receive ongoing care in other settings. Of the 40 690 children discharged home from neonatal care, 2308 children (5.7%) had at least one admission to PICU after discharge, comprising 3270 PICU admissions in total.
- Download figure
- Open in new tab
- Download powerpoint
Study flowchart. PICU, paediatric intensive care unit.
All PICU admissions
The subgroup of preterm-born children admitted to PICU had a greater proportion of males (60.0% vs 54.1%), lower birth weight (mean 1131 g vs 1246 g), lower gestational age at birth (median 28 weeks vs 29 weeks), and a greater proportion with neonatal morbidity such as brain injury (11.4% vs 6.0%) compared with the overall cohort of babies discharged home ( table 1 ).
- View inline
Birth and neonatal characteristics of cohort: children born <32 weeks between 2013 and 2018 in England and Wales and discharged home from neonatal care
The observed percentage of children who were admitted to PICU after discharge varied by gestational age at birth ( table 2 ), from 13.6% of children born <24 weeks to 3.7% of children born at 31 weeks.
Number and percentage of children with one or more PICU admissions after discharge home from the neonatal unit, and PMA at neonatal discharge and at first unplanned PICU admission, by gestational age at birth
Examining the first PICU admission after neonatal discharge home ( figure 2 , a Sankey diagram in which the width of the connections represents the number of children), the primary diagnosis on admission was most commonly respiratory disease (n=1436, 62.2%) followed by infection (n=223, 9.7%) and cardiovascular disease (n=196, 8.5%).
Sankey diagram showing diagnostic category of first and subsequent paediatric intensive care unit admissions after neonatal discharge home of children born <32 weeks.
Overall, 507 (22.0%) children admitted to PICU from home had at least one further PICU admission before the age of 2, this increased to 31.9% for children born <24 weeks. Respiratory conditions were consistently the most common cause of admission ( figure 2 ).
The observed mortality within PICU for children of all gestations was 2.4% (n=56).
Unplanned PICU admissions
The majority of first admissions to PICU following neonatal discharge were unplanned (n=1901, 82.4%). The percentage of children discharged home who had subsequent unplanned PICU admissions varied by gestation, from 10.2% of children discharged born <24 weeks to 3.3% of those born 31 weeks ( table 2 ). As gestational age at birth increased, neonatal discharge occurred at an earlier PMA, as did the first unplanned PICU admission ( table 2 ). Among unplanned admissions, 431 (22.7%) children had further PICU admissions (including planned or unplanned), again this was highest in those born at <24 weeks (33.3%).
A total of 40 290 children were included in logistic regression analysis after exclusions for missing variable data (0.98% missing) ( table 1 ). Following adjustment, unplanned PICU admission was associated with lower gestation at birth, male sex, BPD, severe NEC and brain injury ( table 3 ). Of the neonatal morbidities, brain injury had the greatest increase in adjusted OR (aOR 1.42) followed by severe NEC (aOR 1.39) then BPD (aOR 1.37).
Logistic regression analysis for unplanned PICU admission for children discharged home from neonatal care, using gestation as continuous variable (n=40 290)
We compared model predictions for unplanned PICU admission by gestation and morbidity with overall observed percentages ( online supplemental figure 1 ). Increases in predicted risk of unplanned PICU admission appeared to relate to the total number of neonatal morbidities, increasing to 16.8% (95% CI 12.9% to 20.8%) for children born <24 weeks with BPD, NEC and brain injury.
Results remained consistent in planned sensitivity analyses in which gestational age was modelled as a category; and after exclusion of the 760 (1.9%) children with any congenital anomaly ( online supplemental tables 2–4 ). Values for AIC showed little change, indicating similar model quality. Tests of the primary model showed moderate predictive ability (c-statistic 0.614, Brier score 0.042) and acceptable model fit and collinearity ( online supplemental table 5 ).
In this work, we explored the probability of PICU admission for children who were born very preterm and admitted for neonatal care in England and Wales between 2013 and 2018. The observed percentage of children admitted to PICU after neonatal discharge home was highly correlated with gestational age; ranging from 13.6% in those born at <24 weeks gestational age to 3.7% of those born at 31 weeks gestational age. The majority (82%) of these PICU admissions were unplanned, and hence unexpected for the families of these children.
Between a quarter and third of babies born extremely preterm develop BPD, 21 which can result in respiratory impairment 22 and susceptibility to respiratory infections into childhood. 5 We found that BPD was associated with unplanned PICU admission. Given that the largest percentage of PICU admissions and readmissions were for respiratory disease, the continued high rates of BPD in very preterm survivors is concerning. 23 Unplanned PICU admission was also associated with neonatal brain injury and severe NEC. This is consistent with previous studies that have demonstrated that the number of neonatal morbidities is associated with death and disability in preterm-born children. 24 25
Respiratory admissions to PICU are most commonly due to viral illnesses, such as bronchiolitis. 26 Selective passive immunisation to respiratory syncytial virus (RSV) has been recommended since 2010 in the UK, 27 however our results may prompt neonatal services to consider whether additional measures around neonatal discharge planning may help families prepare for and potentially prevent respiratory viral infections. This may include guidance on how best to access healthcare, 28 open access to paediatric assessment units or neonatal outreach nurse services. The first unplanned PICU admission tended to occur relatively soon after neonatal discharge; healthcare professionals and families may wish to know that the first few months after going home are the highest risk for very preterm-born children.
We observed that a relatively high proportion of children admitted to PICU required subsequent PICU readmission before the age of 2 years (22.0%), and this percentage increased further for the most preterm children. Previous studies have described readmission rates of 15.5% of children readmitted to PICU within 1 year, 29 increasing to 21.7% for children with complex chronic disease, 30 which was similar to that observed in our study.
The mortality rate within PICU was relatively low (2.4%), and similar to the rate previously observed using PICANet data (2.8%) for preterm-born children admitted with respiratory failure. 26
The majority of PICU admissions (82% of first admissions) after neonatal discharge were unplanned. Both neonatal and PICU care are associated with symptoms of post-traumatic stress in parents, 31–33 and unexpected admissions to another intensive care environment may exacerbate this. Moreover, families may find the PICU environment very different to the neonatal environment they had been used to. 34 We are not aware of any literature examining the effects of preparing families for the possibility of PICU admission, however information from our work regarding the potential for PICU admission could be made available to clinicians to share with families who wish to know this.
Strengths and limitations
The major strength of this study is the use of a novel, large, linked national dataset. Given the data quality and completeness of the NNRD and PICANet, and the use of NHS numbers, this should allow high levels of linkage success, 8 35 although we cannot quantify this.
Limited research has explored this area previously. Aggregate data from NNRD and PICANet been used to estimate PICU admission during the neonatal stay for children born very preterm (1.7%–5.5% of children), 36 however PICU admissions after neonatal discharge were not examined. A conference abstract reported estimates of extreme preterm-born children requiring PICU admission between birth and 2 years using PICANet and ONS summary data (up to 20%–25% of children born <25 weeks). 6 However, 30% of PICU admissions had missing data for gestation, leading to a high degree of uncertainty regarding these estimates, in addition this study included children admitted to PICU during their neonatal stay, unlike our study. The use of patient-level data linkage in our study enables us to identify patient journeys of preterm-born children so provides more robust estimates, and allows adjustment for neonatal factors.
While the datasets we have used cover a wide geographical area, there may be some children whose PICU admission was outside of England or Wales and therefore missed. In addition, a small number of children (0.4%) died outside of neonatal care or PICUs within England and Wales.
While tests of model fit and multicollinearity were satisfactory, our model had only moderate predictive ability for unplanned PICU admission despite a large dataset and selection of significant neonatal morbidity. Therefore, there may have been unmeasured factors which affect the risk of respiratory infections requiring PICU admission, such as the season of discharge, individuals’ RSV prophylaxis or smoking within the home. 37
Implications for future research
Our dataset does not include babies born since the 2019 changes in UK neonatal management of babies born 22–23 weeks. 38 Future work using more recent data may increase understanding of the intensive care needs of this relatively small population, and any effect on overall PICU admissions.
This study did not examine children who were discharged directly from neonatal units to ongoing care in inpatient wards, high dependency units or PICUs (n=2065). These children, particularly those requiring ongoing critical care, are likely to have a greater degree of morbidity, and we intend to study this important group further.
Future research could also examine the impact of early neonatal discharge, and the season of discharge, particularly with regard to subsequent respiratory admissions, and assess the effectiveness of interventions to prevent such illnesses such as RSV prophylaxis for high-risk children.
Conclusions
The majority of babies born <32 weeks and discharged home from neonatal care do not require PICU admission in the first 2 years of life. However, unexpected admissions to PICU are more common in the most preterm-born children, and especially those with brain injury, severe NEC or BPD. More work is required to understand the impact of morbidity and multimorbidity in the very preterm population.
The main driver of PICU admissions is respiratory illness, mostly occurring in the first few months following neonatal discharge, while a considerable proportion of children require multiple PICU admissions for respiratory disease in the first 2 years.
Our results provide data to support neonatal healthcare professionals in identifying babies with the greatest risk of PICU admission after neonatal discharge. We plan to work with families and healthcare professionals to develop resources to aid discussions around this risk.
Ethics statements
Patient consent for publication.
Not applicable.
Ethics approval
Research ethical approval was provided by the East of England committee (reference: 20/EE/0220) and the Confidentiality Advisory Group (20/CAG/0110). This study made use of routinely collected data and therefore no participants were actively recruited.
Acknowledgments
The authors thank all UK Neonatal Collaborative neonatal units that agreed to the inclusion of data in the National Neonatal Research Database (NNRD) from their patients to be used in this work. The NNRD is a UK Health Research Authority-approved research database; the Chief Investigator for the NNRD is Professor Neena Modi. The authors would like to thank all paediatric intensive care units in England and Wales who allowed their data to be used in this study. PICANet is commissioned by the Healthcare Quality Improvement Partnership (HQIP) as part of the National Clinical Audit and Patient Outcomes Programme (NCAPOP). HQIP is led by a consortium of the Academy of Medical Royal Colleges, the Royal College of Nursing and National Voices. Its aim is to promote quality improvement in patient outcomes, and in particular, to increase the impact that clinical audit, outcome review programmes and registries have on healthcare quality in England and Wales. HQIP holds the contract to commission, manage and develop the NCAPOP, comprising around 40 projects covering care provided to people with a wide range of medical, surgical and mental health conditions. The programme is funded by NHS England, the Welsh Government and, with some individual projects, other devolved administrations and crown dependencies www.hqip.org.uk/national-programmes. Support with the data extraction and linkage was kindly provided by Kayleigh Ougham (NNRD), Lee Norman (PICANet) and NHS Digital. The authors would like to thank the other members of the study advisory group (Professor Jennifer J Kurinczuk, Dr Jonathan Cusack, Dr Patrick Davies, Dr Nicola Mackintosh and Dr Joseph Manning). The authors would also like to acknowledge and thank the support of Bliss, the charity for babies born premature or sick, ADAPT Prem Babies—Leicestershire, the local charity supporting parents and families with premature and poorly babies and The Smallest Things, the volunteer-run charity promoting health and well-being for preterm babies and their families. In particular, the authors would like to acknowledge the children who have contributed data to our research and their families; and the support and input from the families who have contributed so much to this project during the patient and public involvement meetings.
- Santhakumaran S ,
- Statnikov Y ,
- Gray D , et al
- Helenius K ,
- Shah PS , et al
- Stensvold HJ ,
- Klingenberg C ,
- Stoen R , et al
- Coathup V ,
- Carson C , et al
- Ghazaly M ,
- Paediatric Intensive Care National Audit - Universities of Leicester and Leeds
- Battersby C ,
- Longford N ,
- Mandalia S , et al
- Jawad S , et al
- Webbe JWH ,
- Duffy JMN ,
- Afonso E , et al
- Manktelow BN ,
- Seaton SE ,
- Field DJ , et al
- Manktelow BN , et al
- Williams AF ,
- Wright CM , et al
- Burnham KP ,
- Anderson DR
- Hosmer DW ,
- Lemeshow S ,
- Sturdivant RX
- Sarkar SK ,
- Sjörs G , et al
- Moschino L ,
- Bonadies L ,
- Avila-Alvarez A ,
- Pértega-Diaz S , et al
- Bassler D ,
- Schmidt B , et al
- Farooqi A ,
- Hägglöf B ,
- Sedin G , et al
- O’Donnell DR ,
- Parslow RC ,
- Joint Committee on Vaccination and Immunisation
- Ingram JC ,
- Powell JE ,
- Blair PS , et al
- Cecil C , et al
- Richardson T , et al
- Bronner MB ,
- Knoester H ,
- Bos AP , et al
- Zelkowitz P ,
- Cormier C , et al
- Harrison S ,
- Burton HAL , et al
- Santhakumaran S , et al
- Coathup V , et al
- McKeever T , et al
- British Association of Perinatal Medicine
Supplementary materials
Supplementary data.
This web only file has been produced by the BMJ Publishing Group from an electronic file supplied by the author(s) and has not been edited for content.
- Data supplement 1
X @DrCGale, @DrCBattersby
Collaborators Matthew Babirecki (Airedale General Hospital), Rebecca Kettle (Alder Hey), Anand Kamalanathan (Arrowe Park Hospital), Clare Cane (Barnet Hospital), Kavi Aucharaz (Barnsley District General Hospital), Rathod Poorva (Basildon Hospital), Maninder Bal (Basingstoke & North Hampshire Hospital), L M Wong (Bassetlaw District General Hospital), Anita Mittal (Bedford Hospital), Penny Broggio (Birmingham City Hospital), Pinki Surana (Birmingham Heartlands Hospital), Matt Nash (Birmingham Women's Hospital), Sam Wallis (Bradford Royal Infirmary), Ahmed Hassan (Broomfield Hospital, Chelmsford), Karin Schwarz (Calderdale Royal Hospital), Shu-Ling Chuang (Chelsea & Westminster Hospital), Penelope Young (Chesterfield & North Derbyshire Royal Hospital), Ramona Onita (Colchester General Hospital), Mani Kandasamy (Conquest Hospital), Stephen Brearey (Countess of Chester Hospital), Morris/Siramhatia (Croydon University Hospital), Yee Aung (Cumberland Infirmary), Bharath Gowda (Darent Valley Hospital), Mehdi Garbash (Darlington Memorial Hospital), Alex Allwood (Derriford Hospital), Pauline Adiotomre (Diana Princess of Wales Hospital), Nigel Brooke (Doncaster Royal Infirmary), Claire Hollinsworh (Dorset County Hospital), Toria Klutse (East Surrey Hospital), Sonia Spathis (Epsom General Hospital), Sathish Krishnan (Frimley Park Hospital), Samar Sen (Furness General Hospital), Alaa Ghoneem (George Eliot Hospital), Jennifer Holman (Gloucester Royal Hospital), Daniel Dogar (Good Hope Hospital), Girish Gowda (Great Western Hospital), Karen Turnock (Guy's & St Thomas' Hospital), Sobia Balal (Harrogate District Hospital), Cath Seagrave (Hereford County Hospital), Tristan Bate (Hillingdon Hospital), Hilary Dixon (Hinchingbrooke Hospital), Narendra Aladangady (Homerton Hospital), Hassan Gaili (Hull Royal infirmary), Matthew James (Ipswich Hospital), M Lal (James Cook University Hospital), Oluseun Tayo (James Paget Hospital), Abraham Isaac (Kettering General Hospital), Carolina Zorro (Kings College Hospital), Dhaval Dave (King's Mill Hospital), Jonathan Filkin (Kingston Hospital), Savi Sivashankar (Lancashire Women and Newborn Centre), Hannah Shore (Leeds General Infirmary), Jo Behrsin (Leicester General Hospital), Jo Behrsin (Leicester Royal Infirmary), Michael Grosdenier (Leighton Hospital), Ruchika Gupta (Lincoln County Hospital), Ather Ahmed (Lister Hospital), Alison Bedford Russell (Liverpool Women's Hospital), Jennifer Birch (Luton & Dunstable Hospital), Surendran Chandrasekaran (Macclesfield District General Hospital), Ashok Karupaiah (Manor Hospital), Ghada Ramadan (Medway Maritime Hospital), I Misra (Milton Keynes General Hospital), Chris Knight (Musgrove Park Hospital), Richard Heaver (New Cross Hospital), Mohammad Alam (Newham General Hospital), Prakash Thiagarajan (Nobles Hospital), Muthukumar (Norfolk & Norwich University Hospital), Tiziana Fragapane (North Devon District Hospital), Bivan Saha (North Manchester General Hospital), Cheentan Singh (North Middlesex University Hospital), Nick Barnes (Northampton General Hospital), Sangeeta Tiwary (Northumbria Specialist Emergency Care Hospital), Richard Nicholl (Northwick Park Hospital), Dush Batra (Nottingham City Hospital), Dush Batra (Nottingham University Hospital (QMC)), Victoria Nesbitt (Ormskirk District General Hospital), Amit Gupta (Oxford University Hospitals, John Radcliffe Hospital), Katharine McDevitt (Peterborough City Hospital), Ruchika Gupta (Pilgrim Hospital), David Gibson (Pinderfields General Hospital), Peter Mcewan (Poole General Hospital), Sanath Reddy (Princess Alexandra Hospital), Mark Johnson (Princess Anne Hospital), Aesha Mohammedi (Princess Royal Hospital), Patrica Cowley (Princess Royal Hospital), Rashmi Gandhi (Princess Royal University Hospital), Charlotte Groves (Queen Alexandra Hospital), Lidia Tyszcuzk (Queen Charlotte's Hospital), Shilpa Ramesh (Queen Elizabeth Hospital, Gateshead), Salamatu Jalloh (Queen Elizabeth Hospital, King's Lynn), Julia Croft (Queen Elizabeth Hospital, Woolwich), Bushra Abdul-Malik (Queen Elizabeth the Queen Mother Hospital), Dominic Muogbo (Queen's Hospital, Burton on Trent), Ambalika Das (Queen's Hospital, Romford), Khalid Mannan (Queen's Hospital, Romford), Rajiv Chaudhary (Rosie Maternity Hospital, Addenbrookes), Soma Sengupta (Rotherham District General Hospital), Christos Zipitis (Royal Albert Edward Infirmary), Kemy Naidoo (Royal Berkshire Hospital), Archana Mishra (Royal Bolton Hospital), Chris Warren (Royal Cornwall Hospital), Nigel Ruggins (Royal Derby Hospital), Chrissie Oliver (Royal Devon & Exeter Hospital), Lucinda Winckworth (Royal Hampshire County Hospital), Joanne Fedee (Royal Lancaster Infirmary), Anitha Vayalakkad (Royal Oldham Hospital), Richa Gupta (Royal Preston Hospital), Lee Abbott (Royal Stoke University Hospital), Ben Obi (Royal Surrey County Hospital), Aesha Mohammedi (Royal Sussex County Hospital), Rebecca Winterson (Royal United Hospital), Naveen Athiraman (Royal Victoria Infirmary), Anjali Pektar (Russells Hall Hospital), Jim Baird (Salisbury District Hospital), Adedayo Owoeye (Scarborough General Hospital), Umapathee Majuran (Scunthorpe General Hospital), Richard Lindley (Sheffield Children's Hospital), Vineet Gupta (Southend Hospital), Faith Emery (Southmead Hospital), Donovan Duffy (St George's Hospital), Salim Yasin (St Helier Hospital), Hannah Shore (St James University Hospital), Akinsola Ogundiya (St Mary's Hospital, IOW), Lidia Tyszcuzk (St Mary's Hospital, London), Ngozi Edi-Osagie (St Mary's Hospital, Manchester), Pamela Cairns (St Michael's Hospital), Vennila Ponnusamy (St Peter's Hospital), Victoria Sharp (St Richard's Hospital), Carrie Heal (Stepping Hill Hospital), Sanjay Salgia (Stoke Mandeville Hospital), Imran Ahmed (Sunderland Royal Hospital), Helen Purves (Tameside General Hospital), Porus Bastani (The Jessop Wing, Sheffield), Eleanor Bond (The Royal Free Hospital), Divyen Shah (The Royal London Hospital—Constance Green), Esther Morris (Torbay Hospital), Se-Yeon Park (Tunbridge Wells Hospital), Giles Kendall (University College Hospital), Puneet Nath (University Hospital Coventry), Igor Fierens (University Hospital Lewisham), Mehdi Garbash (University Hospital of North Durham), Hari Kumar (University Hospital of North Tees), Peter Curtis (Victoria Hospital, Blackpool), Delyth Webb (Warrington Hospital), Bird (Warwick Hospital), Sankara Narayanan (Watford General Hospital), Yee Aung (West Cumberland Hospital), Eleanor Hulse (West Middlesex University Hospital), Tayyaba Aamir (West Suffolk Hospital), Angela Yannoulias (Wexham Park Hospital), Caroline Sullivan (Whipps Cross University Hospital), Ros Garr (Whiston Hospital), Wynne Leith (Whittington Hospital), Shaveta Mulla (William Harvey Hospital), Anna Gregory (Worcestershire Royal Hospital), Edward Yates (Worthing Hospital), Abijeet Godhamgaonkar (Wythenshawe Hospital), Megan Eaton (Yeovil District Hospital), Sundeep Sandhu (York District Hospital), Arun Ramachandran (Singleton Hospital), Abby Parish (Princess of Wales Hospital), Anitha James (The Grange University Hospital), Ian Barnard (Glan Clwyd Hospital), Artur Abelian (Wrexham Maelor Hospital), Shakir Saeed (Ysbyty Gwynedd), Nitin Goel (University Hospital of Wales), David Deekollu (Prince Charles Hospital), Prem Pitchaikani (Glangwili General Hospital).
Contributors TJvH designed the study, and undertook analysis under the supervision of SES, CG and ED. CB and PJD provided clinical interpretation and review of manuscript. All authors contributed to the interpretation, revised the manuscript critically and approved the final version for submission. SES, as supervisor of TJvH, had access to all data and responsibility for the project including decision for publication, and is the guarantor for this paper.
Funding SES (Advanced Fellowship: NIHR300579), CB (Advanced Fellowship: NIHR300617) and TJvH (Doctoral Research Fellowship: NIHR301761) are funded by the National Institute for Health Research (NIHR) for this research project. The views expressed in this publication are those of the authors and not necessarily those of the NIHR, NHS or the UK Department of Health and Social Care.CG is supported by the Medical Research Council through a Clinician Scientist Fellowship (MR/N008405/1, MR/V036866/1) and this supported his salary over the time spent on this study. He has also received grants and funding from the NIHR, Action Medical Research, Chiesi Pharmaceuticals and the Canadian Institute for Health Research (CIHR).
Disclaimer The views expressed in this publication are those of the authors and not necessarily those of the NIHR, NHS or the UK Department of Health and Social Care.
Competing interests None declared.
Provenance and peer review Not commissioned; externally peer reviewed.
Supplemental material This content has been supplied by the author(s). It has not been vetted by BMJ Publishing Group Limited (BMJ) and may not have been peer-reviewed. Any opinions or recommendations discussed are solely those of the author(s) and are not endorsed by BMJ. BMJ disclaims all liability and responsibility arising from any reliance placed on the content. Where the content includes any translated material, BMJ does not warrant the accuracy and reliability of the translations (including but not limited to local regulations, clinical guidelines, terminology, drug names and drug dosages), and is not responsible for any error and/or omissions arising from translation and adaptation or otherwise.
Linked Articles
- Highlights from this issue Fantoms Ben J Stenson Archives of Disease in Childhood - Fetal and Neonatal Edition 2024; 109 229-229 Published Online First: 18 Apr 2024. doi: 10.1136/archdischild-2024-327233
Read the full text or download the PDF:
Advertisement
Intensive Care Medicine – Paediatric and Neonatal ( ICM-PN ): creating a journal to fill a gap and strengthen our domain
- Open access
- Published: 02 February 2023
- Volume 1 , article number 1 , ( 2023 )
Cite this article
You have full access to this open access article
- Daniele De Luca 1 , 2 ,
- Paulien Raymakers 1 ,
- Monique van Dijk 3 &
- Dick Tibboel 3
1764 Accesses
3 Altmetric
Explore all metrics
Avoid common mistakes on your manuscript.
Today, we celebrate a landmark event in our history with the launch of Intensive Care Medicine – Paediatric and Neonatal ( ICM-PN ), the official journal of the European Society for Paediatric and Neonatal Intensive Care (ESPNIC). This fills a dual gap.
First, in Europe, paediatric and neonatal intensive care did not have a dedicated journal so far. Certainly, we represent a relatively small specialty but we treat and care special patients: neonates, infants, toddlers and children suffering from a life-threatening condition admitted to specialised intensive care units. Saving them is what drives us and we desperately need high-quality academic research to improve our results both from a medical and a nursing perspective. So far, publications of our research have been divided between adult critical care medicine journals and those dedicated to neonatology but not specifically to neonatal and paediatric intensive care. None of these solutions was ideal to foster research and development in our field, although we share some aspects with adult intensive care and neonatology. In fact, we also have many relevant differences related to changes in organ development and maturation, disease patterns and pathophysiology. Ours is a cross-disciplinary specialty that can only develop by learning from adult experience and from other paediatric areas. Moreover, our domain is becoming more and more multi-professional, as in neonatal (NICU) and paediatric intensive care units (PICU), physicians, nurses and allied healthcare professionals closely work together. Our work is highly technology-based, as medical devices are needed to monitor patients’ vital parameters, understand the changing pathophysiology over time and sustain organ functions in the most efficacious and personalised way. Both doctors and nurses aim to maintain and enhance the quality of life in the long run even into adulthood. ICM-PN is the place where any advancement, produced by any NICU/PICU professional and obtained in a methodologically sound way, can be published with the right priority that cannot be given by adult intensive care or general neonatal journals. From the bench to bedside, from animal and translational to clinical research, high-quality data are welcome, as well as systematic reviews and position papers having the potential to improve NICU/PICU care. Special attention is also paid to mutual education and gain of knowledge represented by papers written by paediatric and adult specialists together under the heading: Crossing Borders.
Second, ESPNIC is one of the oldest European scientific societies, the largest paediatric one and the only uniquely focused on intensive care, but it did not have its entirely own journal. This was identified as a major shortcoming hampering the spread of academic research and advancement in our field. Research is the base of every specialty in medicine, as it enables to be a “real” contributor to science and evidence-based care. Creating a journal is not an easy task but we were able to do so thanks to the collaboration with the European Society for Intensive Care Medicine (ESICM) and the Intensive Care Medicine ( ICM ) team. ICM-PN represents the ESPNIC official journal with a clear multi-professional background. With its launch, the “ ICM family” welcomes a new member: in fact, the ICM family is now a group of high-quality academic journals that covers the whole spectrum of research in the field of intensive care medicine. ICM represents the “mother journal”, dedicated to ground-breaking clinical research of extremely high importance (including paediatric and neonatal ones [ 1 ]); ICM-experimental ( ICM-X ) is dedicated to translational and animal research, while ICM-PN specifically covers all paediatric and neonatal research. The three journals are interconnected and editors work closely together. They have the same editorial style and can transfer articles between the three. There is no similar example of a whole journal family dedicated to those taking care of the most critically ill patients. They are, in a broader sense, our journals and we are happy for this and grateful to ESICM for the strong support and collaboration.
A new era just started, and we are sure that European paediatric and neonatal intensive care will take giant steps. Although our research is of high quality, often, NICU/PICU-based articles are unfunded or only partially funded especially at a European level [ 2 ]. ESPNIC is highly committed to support members and this translates in covering article processing charges as much as possible for the first years of ICM-PN life. Together with ESICM colleagues, we foresee a very good impact factor in 4 years, but to achieve this, we must all commit in publishing our manuscripts in ICM-PN , as well as to cite articles published in the ICM family journals as much as possible. We all have the same goal: together, we are intensive care and we are so for neonates and children.
MCJ K, on behalf of the section Respiratory Failure of the European Society for Paediatric and Neonatal Intensive Care (2017) Recommendations for mechanical ventilation of critically ill children from the Paediatric Mechanical Ventilation Consensus Conference (PEMVECC). Intensive Care Med 43:1764–1780. https://doi.org/10.1007/s00134-017-4920-z
Article Google Scholar
Shankar-Aguilera S, Taveira M, De Luca D (2014) Neonatal ventilation trials need specific funding. Lancet Respir Med 2:867–869. https://doi.org/10.1016/S2213-2600(14)70194-8
Article PubMed Google Scholar
Download references
Acknowledgements
We are extremely grateful to ESICM colleagues and friends who have helped to make this possible and particularly to Prof. Maurizio Cecconi (MD, PhD), Past-President of ESICM; Prof. Elie Azoulay (MD, PhD), President of ESICM and Past-Editor-in-Chief of ICM; Prof. Giuseppe Citerio (MD, PhD), Editor-in-Chief of ICM; and Mr. Alexander Joel . Finally, we are extremely grateful to Prof. Anne Sylvie Ramelet (RN, PhD), Dr. Martin Kneyber (MD, PhD) and Prof. Akash Deep (MD, PhD), respectively, ESPNIC Treasurer, President-Elect and Chief of Scientific Affairs for their precious advices and support.
Author information
Authors and affiliations.
European Society for Paediatric and Neonatal Intensive Care and University Medical Center Utrecht Wilhelmina Children’s Hospital, Utrecht, the Netherlands
Daniele De Luca & Paulien Raymakers
Service de Pédiatrie et Réanimation Néonatale, Hôpital “A. Béclère” - Université Paris Saclay, APHP, 157 rue de la Porte de Trivaux, 92140, Clamart (Paris-IDF), France
Daniele De Luca
Intensive Care Erasmus Medical Center, Rotterdam, The Netherlands
Monique van Dijk & Dick Tibboel
You can also search for this author in PubMed Google Scholar
Contributions
DDL and DT conceived the editorial and wrote the manuscript draft. PR, MVD, DT helped in the literature and data interpertation and critically reviewed the manuscript for important intellectual content. All authors read and approved the final manuscript.
Corresponding author
Correspondence to Daniele De Luca .
Ethics declarations
Competing interests.
The authors declare that they have no competing interests.
Additional information
Publisher’s note.
Springer Nature remains neutral with regard to jurisdictional claims in published maps and institutional affiliations.
Rights and permissions
Open Access This article is licensed under a Creative Commons Attribution 4.0 International License, which permits use, sharing, adaptation, distribution and reproduction in any medium or format, as long as you give appropriate credit to the original author(s) and the source, provide a link to the Creative Commons licence, and indicate if changes were made. The images or other third party material in this article are included in the article's Creative Commons licence, unless indicated otherwise in a credit line to the material. If material is not included in the article's Creative Commons licence and your intended use is not permitted by statutory regulation or exceeds the permitted use, you will need to obtain permission directly from the copyright holder. To view a copy of this licence, visit http://creativecommons.org/licenses/by/4.0/ .
Reprints and permissions
About this article
De Luca, D., Raymakers, P., van Dijk, M. et al. Intensive Care Medicine – Paediatric and Neonatal ( ICM-PN ): creating a journal to fill a gap and strengthen our domain. Intensive Care Med. Paediatr. Neonatal 1 , 1 (2023). https://doi.org/10.1007/s44253-023-00001-6
Download citation
Published : 02 February 2023
DOI : https://doi.org/10.1007/s44253-023-00001-6
Share this article
Anyone you share the following link with will be able to read this content:
Sorry, a shareable link is not currently available for this article.
Provided by the Springer Nature SharedIt content-sharing initiative
- Critical care
- Find a journal
- Publish with us
- Track your research
Academia.edu no longer supports Internet Explorer.
To browse Academia.edu and the wider internet faster and more securely, please take a few seconds to upgrade your browser .
- We're Hiring!
- Help Center
Pediatric intensive care
- Most Cited Papers
- Most Downloaded Papers
- Newest Papers
- Save to Library
- Last »
- Polio Follow Following
- Pulmonology Follow Following
- Pediatric Nutrition Follow Following
- Pediatric emergency Follow Following
- Pediatrics and Child Health Follow Following
- Biosciences Follow Following
- Drug Delivery Systems Follow Following
- Pediatric Gastroenterology Follow Following
- Pediatrics Follow Following
- Cardiac and Thoracic Surgery Follow Following
Enter the email address you signed up with and we'll email you a reset link.
- Academia.edu Publishing
- We're Hiring!
- Help Center
- Find new research papers in:
- Health Sciences
- Earth Sciences
- Cognitive Science
- Mathematics
- Computer Science
- Academia ©2024
- Data Dashboards
- Regenstrief Data Services

- Dental Health Informatics
- Global Health Informatics
- Learning Health Informatics
- Public Health Informatics
- Center for Health Services Research
- LOINC & Health Data Standards
- Regenstrief Data Services (RDS)
- Real World Solutions
- Impact / Results
- Our Investigators
- Affiliated Scientists
- Research and Administrative Staff
In The News
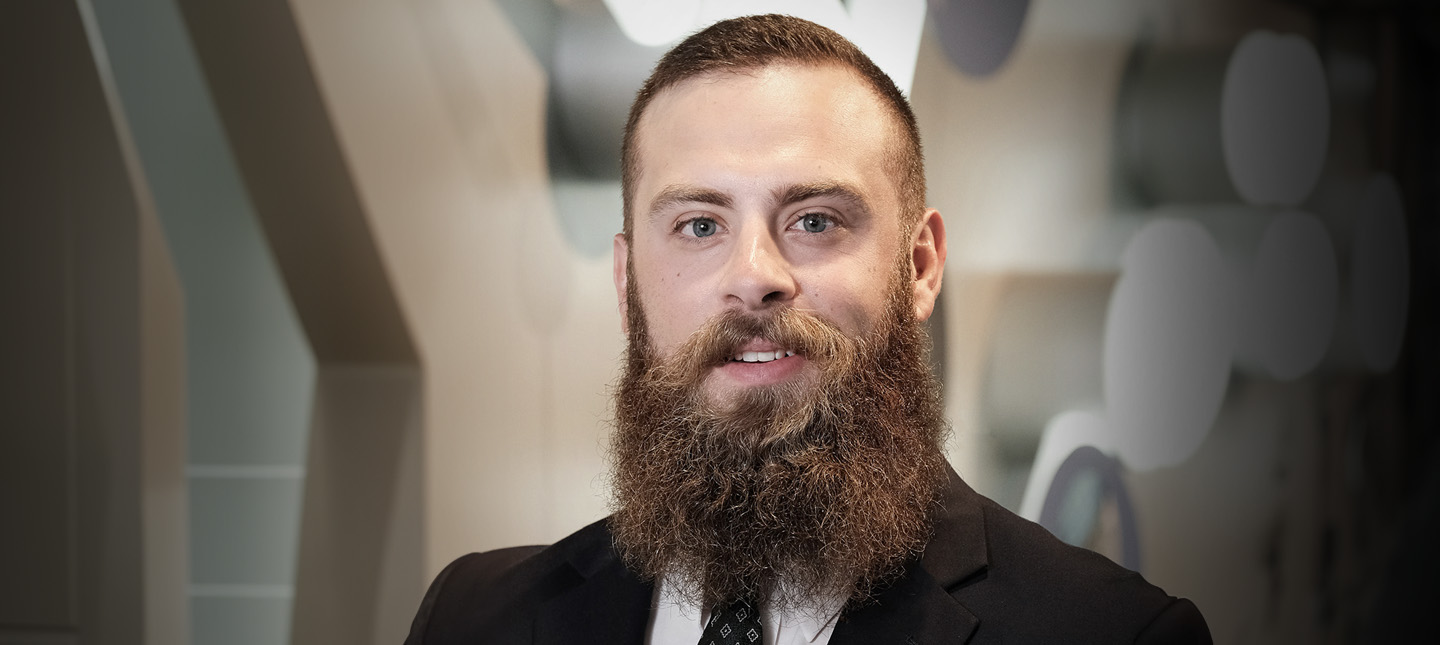
MedicalXpress quoted Dr. Eric Vachon
Eric Vachon, PhD, R.N., was quoted in MedicalXpress about his...
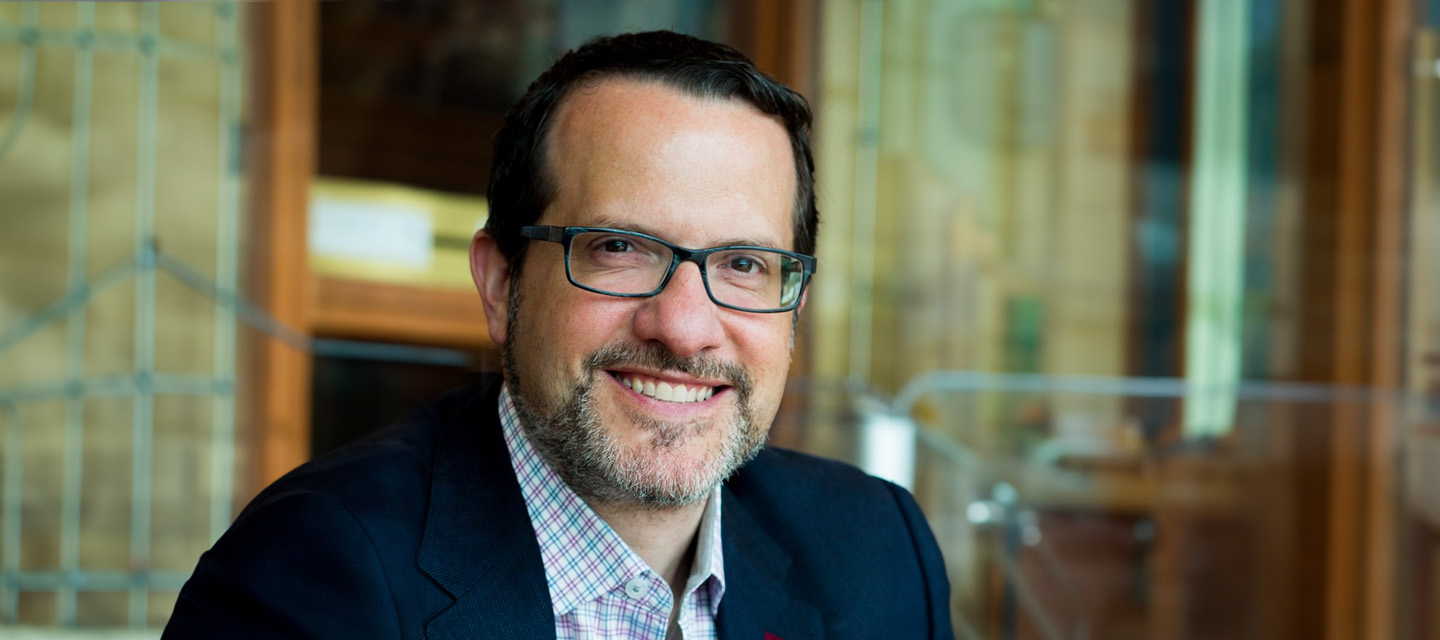
Call for Papers on Artificial Intelligence Applied to Pediatric Care
Published in JAMA Pediatrics. Here is a link to the...
- Our Partners
- Partner With Us
- Fellowships
- Clinical Research Degrees
- Summer Scholars
Featured Fellowship
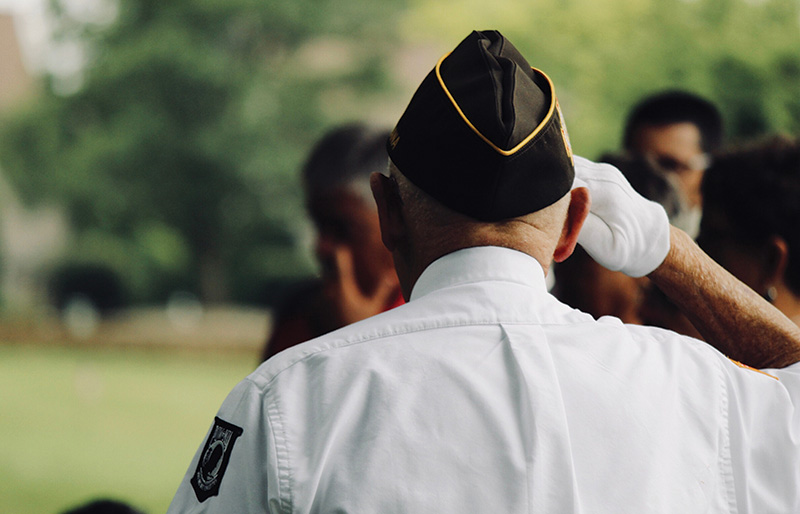
Health Services Research (HSR) Fellowships & Training
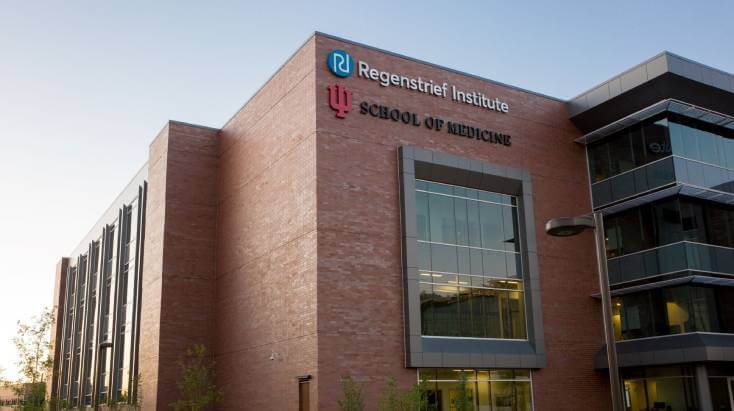
Regenstrief envisions a world where better information empowers people to end disease and realize true health.
- Strategic Plan
- Our History
- Our Facilities
- Centers & Programs
- Executive Leadership
- Investigators
- Diversity, Equity & Inclusion
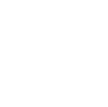
News Regenstrief Institute will host collaborative conference to improve public health data
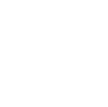
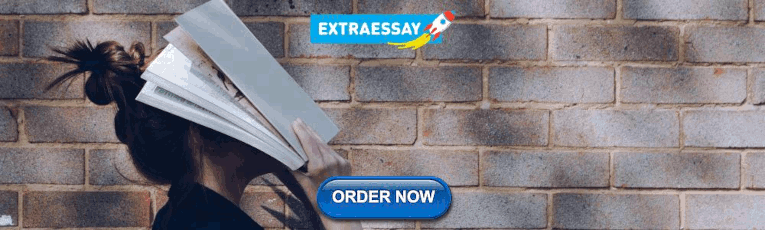
News Study reports chlamydia and gonorrhea more likely to be treated per CDC guidelines in males, younger patients and individuals identifying as Black or multiracial
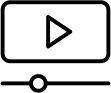
Video Dr. Vachon on how partner satisfaction impacts breast cancer survivors’ well-being
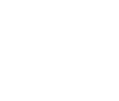
Influence Call for Papers on Artificial Intelligence Applied to Pediatric Care
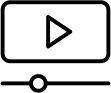
Video Dr. Maratt on closing screening gaps for colorectal cancer
Regenstrief institute will host collaborative conference to....
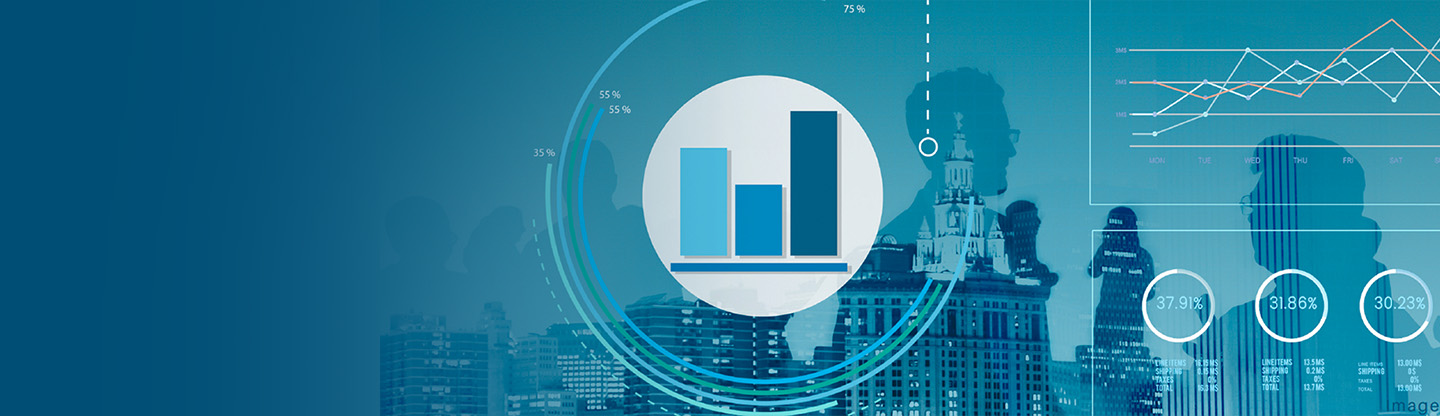
- Annual Reports
- Newsletters
Published in JAMA Pediatrics. Here is a link to the article.
Regenstrief Institute author: Aaron E. Carroll, M.D., M.S.
Artificial intelligence (AI) has long been discussed as a transformative tool in health care, promising to revolutionize all aspects of patient care and even our broader health care systems. Nowhere is this potential more profound than in the realm of pediatrics, where early detection and intervention can significantly impact life trajectories.
AI is evolving rapidly, with potential to transform health care diagnosis and treatment for children and adolescents. 1 It can improve outcomes while making many facets of the health care ecosystem more efficient. 2 It can also assist with imaging analysis, research, documentation, workflow efficiency, and more. 3
In recent years, we have witnessed AI transition from a theoretical promise to a practical tool. Machine learning algorithms have been used in diagnosing skin cancer with comparable accuracy to dermatologists. AI’s predictive modeling capabilities have been tapped into to forecast patient readmission rates, 4 disease progression, and even to anticipate epidemic outbreaks. 5 Deep learning techniques are enhancing the interpretation of medical imaging, and natural language processing is streamlining the review and analysis of electronic health records.
However, many challenges and ethical issues also exist that need to be addressed when applying AI to the care of pediatric populations. 6 These include ensuring the quality, validity, safety, and fairness of AI systems; protecting the privacy, security, and consent of patients and health care professionals; balancing the human and machine roles and responsibilities; and evaluating the impact of AI on health care costs, access, equity, and outcomes. 7 As AI systems are only as good as the inputs they rely on, there is the potential that they could increase rather than decrease disparities.
JAMA Pediatrics now invites submissions of original research articles on the topic of AI applied to pediatric health. We are interested in high-quality studies and viewpoints that explore the benefits, risks, limitations, and implications of AI for pediatric care across various settings and domains.
We welcome submissions that address any aspect of AI applied to the health of children, including:
- Development and validation of AI models or tools for pediatric diagnosis, prognosis, screening, prevention, intervention, or monitoring 8
- Evaluation of the clinical effectiveness, cost-effectiveness, safety, usability, or acceptability of AI interventions or systems in pediatric care 9
- Comparison of AI-based approaches with conventional methods or human experts for pediatric care 10
- Identification and mitigation of potential biases, errors, or harms of AI for pediatric populations 11
- Ethical, legal, social, or policy issues related to the use of AI for pediatric care 12
- Patient, family, or clinician perspectives on the use of AI for pediatric care 13
- Implementation and dissemination strategies for AI interventions or systems in pediatric setting 14
We encourage submissions that use rigorous methods, report transparent and reproducible results, and adhere to relevant reporting standards and guidelines. We also encourage submissions that involve multidisciplinary collaboration and stakeholder engagement.
All submissions will undergo the journal’s usual rigorous peer review to ensure quality and relevance. Accepted papers will be published on a rolling basis and will be promoted extensively to reach a wide audience globally. Guidelines for manuscript preparation and submission can be found on in our Instructions for Authors. 15 Please indicate in your cover letter that your manuscript is intended for this call for papers.
We look forward to your valuable contributions as we embark on this exciting exploration of AI’s potential within pediatric health. AI will undoubtedly play a large role in pediatric health and health care in the future, and we seek to publish the best available science to guide it.
References:
- Liang H, Tsui BY, Ni H, et al. Evaluation and accurate diagnoses of pediatric diseases using artificial intelligence. Nat Med. 2019;25(3):433-438. doi:10.1038/s41591-018-0335-9
2.Ramgopal S, Sanchez-Pinto LN, Horvat CM, Carroll MS, Luo Y, Florin TA. Artificial intelligence-based clinical decision support in pediatrics. Pediatr Res. 2023;93(2):334-341. doi:10.1038/s41390-022-02226-1
3.Shah N, Arshad A, Mazer MB, Carroll CL, Shein SL, Remy KE. The use of machine learning and artificial intelligence within pediatric critical care. Pediatr Res. 2023;93(2):405-412. doi:10.1038/s41390-022-02380-6
4.Romero-Brufau S, Wyatt KD, Boyum P, Mickelson M, Moore M, Cognetta-Rieke C. Implementation of artificial intelligence-based clinical decision support to reduce hospital readmissions at a regional hospital. Appl Clin Inform. 2020;11(4):570-577. doi:10.1055/s-0040-1715827
5.Malik YS, Sircar S, Bhat S, et al. How artificial intelligence may help the Covid-19 pandemic: pitfalls and lessons for the future. Rev Med Virol. 2021;31(5):1-11. doi:10.1002/rmv.2205
6.Mittelstadt BD, Floridi L. The ethics of big data: current and foreseeable issues in biomedical contexts. Sci Eng Ethics. 2016;22(2):303-341. doi:10.1007/s11948-015-9652-2
7.Artificial Intelligence in Health Care. Benefits and Challenges of Technologies to Augment Patient Care (710920). Government Accountability Office; 2020.
8.Rajkomar A, Dean J, Kohane I. Machine learning in medicine: reply. N Engl J Med. 2019;380(26):2589-2590.
9.Wilkinson J, Arnold KF, Murray EJ, et al. Time to reality check the promises of machine learning-powered precision medicine. Lancet Digit Health. 2020;2(12):e677-e680. doi:10.1016/S2589-7500(20)30200-4
10.Ayers JW, Poliak A, Dredze M, et al. Comparing physician and artificial intelligence chatbot responses to patient questions posted to a public social media forum. JAMA Intern Med. 2023;e231838. doi:10.1001/jamainternmed.2023.1838
11.Tschandl P. Risk of Bias and Error From Data Sets Used for Dermatologic Artificial Intelligence. JAMA Dermatol. 2021;157(11):1271-1273. doi:10.1001/jamadermatol.2021.3128
12.Fiske A, Henningsen P, Buyx A. Your robot therapist will see you now: ethical implications of embodied artificial intelligence in psychiatry, psychology, and psychotherapy. J Med Internet Res. 2019;21(5):e13216. doi:10.2196/13216
13.Chen YW, Stanley K, Att W. Artificial intelligence in dentistry: current applications and future perspectives. Quintessence Int. 2020;51(3):248-257.
14.Svedberg P, Reed J, Nilsen P, Barlow J, Macrae C, Nygren J. Toward successful implementation of artificial intelligence in health care practice: protocol for a research program. JMIR Res Protoc. 2022;11(3):e34920. doi:10.2196/34920
15.Instructions for authors. JAMA Pediatrics. Updated September 28, 2022. Accessed May 16, 2023. https://jamanetwork.com/journals/jamapediatrics/pages/instructions-for-authors
Authors and Affiliations:
Aaron E. Carroll, M.D., M.S. 1,2 , Dimitri A. Christakis, M.D., MPH 3,4
1 Center for Pediatric and Adolescent Comparative Effectiveness Research, Indiana University School of Medicine, Indianapolis
2 Web and Social Media Editor, JAMA Pediatrics
3 Center for Child Health, Behavior, and Development, Seattle Children’s Research Institute, Seattle, Washington
4 Editor, JAMA Pediatrics
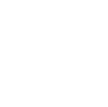
Regenstrief Institute will host collaborative conference to improve public health data
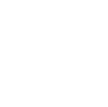
Study reports chlamydia and gonorrhea more likely to be treated per CDC guidelines in males, younger patients and individuals identifying as Black or multiracial
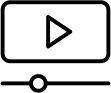
Dr. Vachon on how partner satisfaction impacts breast cancer survivors’ well-being
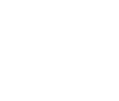
Dr. Maratt on closing screening gaps for colorectal cancer
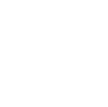
Critical gap in colorectal cancer screening process: follow-up after positive stool test
Get the latest Regenstrief news and events.
Related News
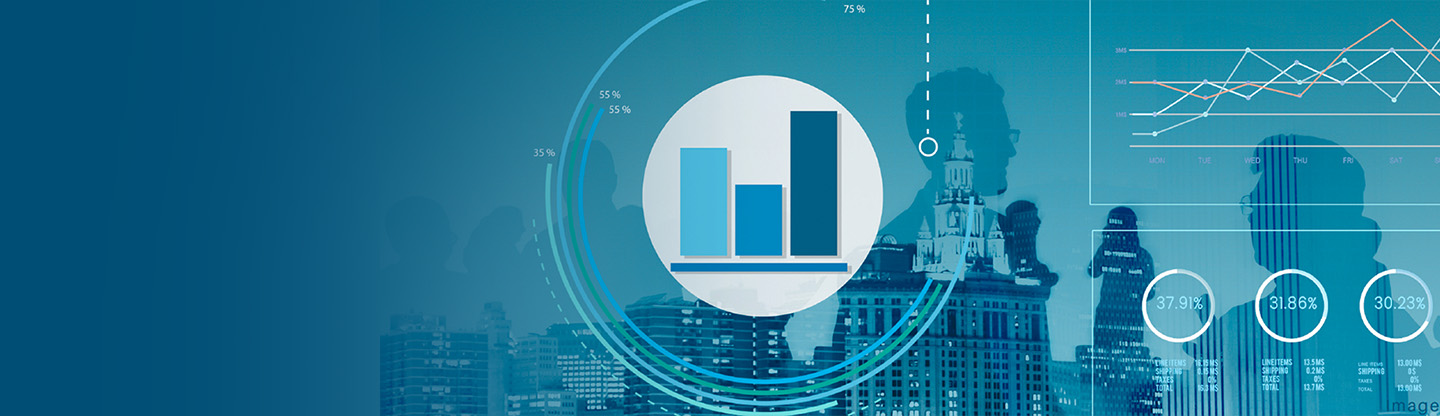
Due to previous year’s high demand, virtual attendance option added The Public Health Informatics Program in the Center for
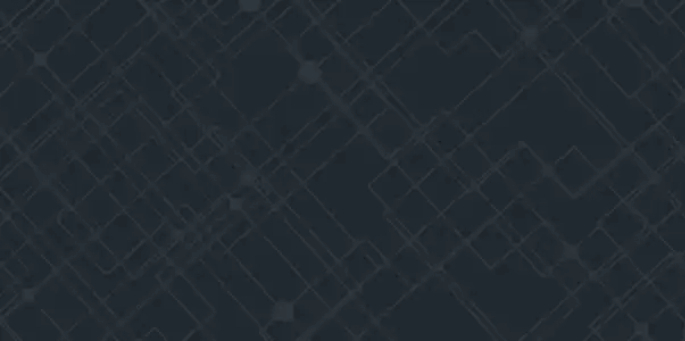
But significant numbers of those living with these diseases don’t receive this treatment INDIANAPOLIS – Chlamydia and gonorrhea are
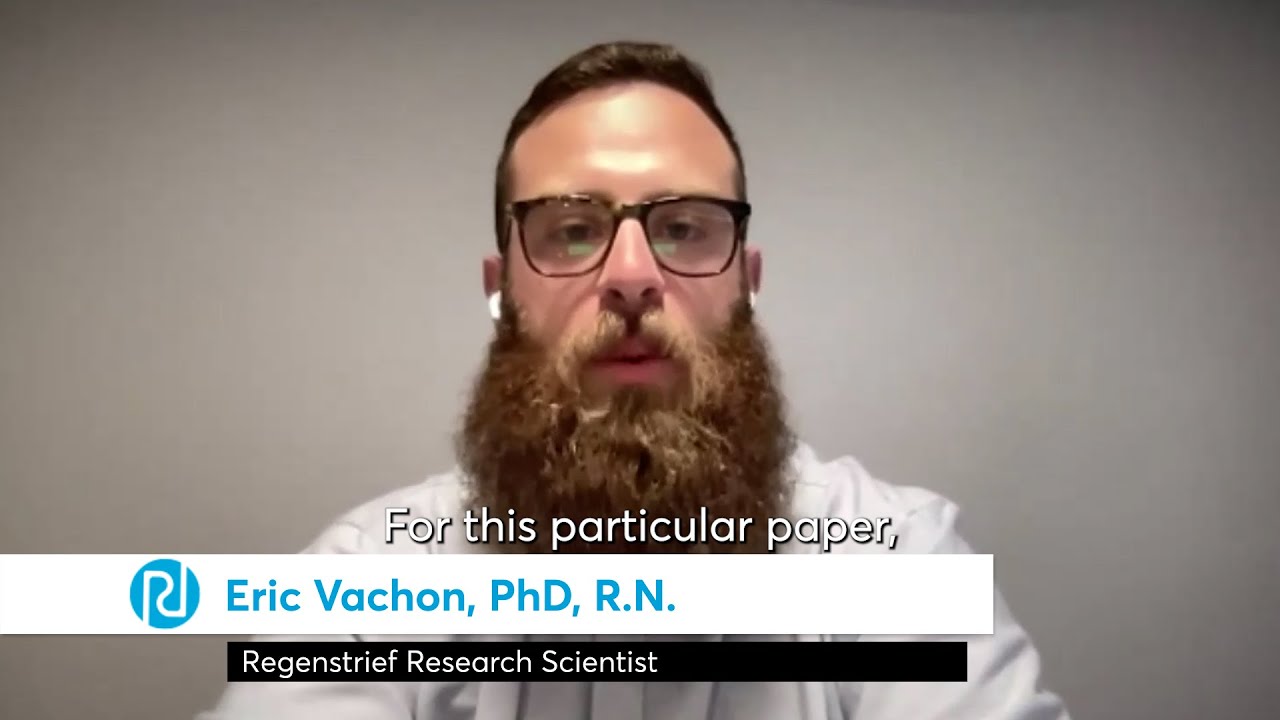
" * " indicates required fields
Please vote for no more than four candidates.
When the paediatric intensive care unit becomes home: A hermeneutic-phenomenological study
Affiliations.
- 1 McGill University Ingram School of Nursing, Montreal, Quebec, Canada.
- 2 Quebec Integrated University Centre for Health and Social Services of Western Central Montreal Island, Montreal, Quebec, Canada.
- PMID: 31799741
- DOI: 10.1111/nicc.12491
Background: Family-centred care is the dominant model for providing nursing care in paediatrics. Unit layout has been shown to impact nurses' ability to provide family-centred care. Little is known about the meanings and experiences of paediatric intensive care unit nurses concerning the care they provide to families within their unique physical setting.
Aim: This study examined paediatric intensive care unit nurses' lived experience of caring for families following a major hospital transformation project, which included the construction of a new unit and quality improvement changes.
Study design: A hermeneutic-phenomenological design was selected to study a paediatric intensive care unit in a large Canadian paediatric teaching hospital.
Methods: Data were collected over a 6-month period through individual interviews, photographs, participant observation, and document review. The sample consisted of 15 paediatric intensive care unit nurses who experienced the unit both pre- and post-transformation. Data were analysed in an ongoing fashion using the method described by Benner to identify common and divergent meanings.
Results: Despite pride in offering a family-friendly environment, nurses' practice prejudiced a family focus in favour of patient-centred care. Nurses in this study negotiated physical and practice spaces with families by interpreting that nurses do not belong in the home-like patient room and exhibiting gatekeeping comportments.
Conclusion: Although similar nurse comportments have been identified in prior works, no previous studies have identified these as forming a pattern of negotiating spaces with families.
Relevance to clinical practice: This study provides insights into the lived experience of paediatric intensive care unit nurses in relation to family care, which can stimulate reflections at an organizational level about creating environments where nurses and families can both feel at home.
Keywords: family nursing; hermeneutics; intensive care units-Paediatric; paediatrics.
© 2019 British Association of Critical Care Nurses.
Publication types
- Research Support, Non-U.S. Gov't
- Critical Care Nursing*
- Family Nursing*
- Hermeneutics*
- Hospitals, Teaching / organization & administration
- Intensive Care Units, Pediatric / organization & administration*
- Interviews as Topic
- Patient-Centered Care
- Qualitative Research
Grants and funding
- McGill University/International
- Institut universitaire en santé mentale de Montréal-Research Center/International
- Mitacs/International
- Quebec Network on Nursing Intervention Research (RRISIQ)/International
- Fonds de recherche du Québec-Santé (FRQ-S)/International
This paper is in the following e-collection/theme issue:
Published on 25.4.2024 in Vol 26 (2024)
Large Language Models and User Trust: Consequence of Self-Referential Learning Loop and the Deskilling of Health Care Professionals
Authors of this article:

- Avishek Choudhury, PhD ;
- Zaira Chaudhry, MD
Industrial and Management Systems Engineering, West Virginia University, Morgantown, WV, United States
Corresponding Author:
Avishek Choudhury, PhD
Industrial and Management Systems Engineering
West Virginia University
321 Engineering Sciences Bdlg
1306 Evansdale Drive
Morgantown, WV, 26506
United States
Phone: 1 5156080777
Email: [email protected]
As the health care industry increasingly embraces large language models (LLMs), understanding the consequence of this integration becomes crucial for maximizing benefits while mitigating potential pitfalls. This paper explores the evolving relationship among clinician trust in LLMs, the transition of data sources from predominantly human-generated to artificial intelligence (AI)–generated content, and the subsequent impact on the performance of LLMs and clinician competence. One of the primary concerns identified in this paper is the LLMs’ self-referential learning loops, where AI-generated content feeds into the learning algorithms, threatening the diversity of the data pool, potentially entrenching biases, and reducing the efficacy of LLMs. While theoretical at this stage, this feedback loop poses a significant challenge as the integration of LLMs in health care deepens, emphasizing the need for proactive dialogue and strategic measures to ensure the safe and effective use of LLM technology. Another key takeaway from our investigation is the role of user expertise and the necessity for a discerning approach to trusting and validating LLM outputs. The paper highlights how expert users, particularly clinicians, can leverage LLMs to enhance productivity by off-loading routine tasks while maintaining a critical oversight to identify and correct potential inaccuracies in AI-generated content. This balance of trust and skepticism is vital for ensuring that LLMs augment rather than undermine the quality of patient care. We also discuss the risks associated with the deskilling of health care professionals. Frequent reliance on LLMs for critical tasks could result in a decline in health care providers’ diagnostic and thinking skills, particularly affecting the training and development of future professionals. The legal and ethical considerations surrounding the deployment of LLMs in health care are also examined. We discuss the medicolegal challenges, including liability in cases of erroneous diagnoses or treatment advice generated by LLMs. The paper references recent legislative efforts, such as The Algorithmic Accountability Act of 2023, as crucial steps toward establishing a framework for the ethical and responsible use of AI-based technologies in health care. In conclusion, this paper advocates for a strategic approach to integrating LLMs into health care. By emphasizing the importance of maintaining clinician expertise, fostering critical engagement with LLM outputs, and navigating the legal and ethical landscape, we can ensure that LLMs serve as valuable tools in enhancing patient care and supporting health care professionals. This approach addresses the immediate challenges posed by integrating LLMs and sets a foundation for their maintainable and responsible use in the future.
Introduction
Integration of existing artificial intelligence (AI) models into health care—a field where the trust in AI is crucial due to the significant impact of decision-making—is still a work in progress [ 1 ]. At the same time, efforts to develop standardized protocols for the deployment of AI in health care are underway, yet they have not reached a point of completion [ 2 ]. This endeavor is critical for ensuring AI’s safe and effective use in health care settings. Additionally, the challenge of evaluating AI in health care is exacerbated by a lack of comprehensive and standardized metrics [ 3 ]. This void is something that researchers and policymakers are actively working to address by creating robust evaluation frameworks that could be applied universally. The regulatory landscape has been focusing on policies around ethical considerations, data privacy, transparency, and patient safety, alongside frameworks that hold AI systems and their developers accountable for the outcomes of their use in patient care [ 1 ].
Advent of Generative AI—Large Language Models in Health Care
Despite these ongoing challenges and developments, generative AI like large language models (LLM) is already being deployed in the public sphere [ 4 , 5 ], used by health care workers, researchers, and the public for a variety of health care–related tasks. Although LLMs have shown promise in medical assessments [ 6 - 10 ], scientific writing, eHealth care, and patient classification [ 11 - 13 ], their integration marks a shift in paradigm introducing new AI complexities [ 14 - 17 ]. Its rapid and early adoption highlights the critical need for continued discourse ensuring the safe and effective integration of LLM into health care. Additionally, LLM characters such as—stochasticity, emergent indeterminacy, and lack of consciousness—reinforces the need for cautiousness.
One fundamental aspect of LLMs that prompts special attention is their stochastic paradigm, which means that these models operate based on probabilities and randomness, allowing the model to generate varied outputs for a given input. It exhibits a level of indeterminacy and unpredictability. LLMs can produce different responses under seemingly similar conditions, complicating their reliability. Such LLM behaviors can lead to unexpected results, which, while sometimes beneficial in generating creative solutions or insights, can also pose risks when applied to critical domains like health care, where accuracy and predictability are paramount.
Another critical risk characteristic of LLM is the lack of inherent understanding of the context they parse and generate. Despite their ability to produce human-like text, LLMs do not possess consciousness, comprehension, or the ability to discern the truthfulness of their outputs. In other words, LLMs might generate plausible but incorrect content, presenting significant challenges in contexts where the veracity and relevance of information are critical [ 18 , 19 ].
Approaching AI integration in health care with a critical mindset is important. It is crucial for users to have a clear understanding of a technology’s actual performance, distinguishing it from the exaggerated expectations set by media hype. These risks underscore the importance of asking the question: are we and our health care system ready to integrate LLMs? If yes, is there a policy in place explicitly stating in what capacity it could be used to reduce clinical workload before its dissemination?
In this paper, we conceptually investigate the dynamics between clinicians’ growing trust in LLMs, the evolving sources of training data, and the resultant implications for both clinician competency and LLM performance over time. Our discussion highlights a potential feedback loop where LLMs, increasingly trained on narrower data sets dominated by their own outputs, may experience a decline in output quality coinciding with a reduction in user skills. While these phenomena are not yet fully realized, they represent anticipated challenges that coincide with the deeper integration of LLMs into the health care domain. We call for preemptive, focused dialogues concerning the integration of LLMs in medical settings, underscoring the importance of maintaining patient safety and the standard of care.
Presently, LLMs are developing at an accelerated pace, heavily reliant on human-generated data sets that are integral to their accuracy and the consequent trust placed in them, particularly in the health care sector. This burgeoning dependency, although seemingly beneficial in terms of efficiency and productivity, may lead to an unintended erosion of clinician skills due to the habitual delegation of tasks to AI—as noted in the academic context [ 20 , 21 ]. This trend raises the possibility of an overreliance on LLM outputs, potentially diminishing the variety and depth of human insights within these models. The risk is a self-perpetuating cycle where LLMs, learning mostly from their creations, could see a degradation in their effectiveness and a narrowing of the breadth of human knowledge they were designed to emulate. Such an outcome would be counterproductive, possibly leading to a decline in both LLM effectiveness and human expertise.
Figure 1 illustrates our core arguments. The first panel reveals a timeline that shows an inverse correlation between clinicians’ escalating trust in AI and the preservation of clinical skills over successive time points (T1 to Tn), signaling an increase in AI reliance and a decrease in skill retention. The middle panel demonstrates the shift in training data for LLMs from predominantly human-generated to a growing proportion of AI-generated data, which in turn affects LLM performance and contributes to the feedback loop. The final panel plots LLM accuracy against time, displaying an initial increase as LLMs leverage a mix of data sources. However, upon reaching a tipping point—marked as the self-referential zone—accuracy declines in tandem with the onset of the user deskilling zone, emphasizing the dilemma of increased AI reliance degrading user capabilities. We underscore the need for strategic measures to address these impending challenges in the health care sector.

User Expertise and Trust in LLMs
User trust in LLMs is deeply intertwined with the individual’s subject matter expertise and their willingness to engage critically with AI outcomes. Expert users, with a robust understanding of their domain, are more likely to approach LLMs with a discerning mindset and preparedness to review and validate its suggestions. Thus, trust in LLMs can be seen as a spectrum influenced by the user’s expertise, and the effort they are willing to invest in ensuring the accuracy of the outcomes.
User Expertise: Ability to Detect Errors in LLMs
The use of LLMs presents a range of possibilities and challenges that vary depending on the user’s expertise and intent, delineating into 2 primary user categories—subject matter experts and those seeking assistance due to a lack of knowledge.
Subject matter experts (doctors) may use LLMs to handle routine, time-consuming tasks, enabling them to allocate more time to complex or urgent issues like seeking a second opinion on complex medical diagnoses, or patient triage. They have the advantage of being able to critically evaluate the LLM’s output, verify its accuracy (deviation from clinical standards), and make necessary corrections. The expertise of such users acts as a safeguard against potential errors, ensuring that the AI’s assistance enhances productivity without introducing risk.
On the other hand, individuals who turn to LLMs due to a lack of expertise in a particular area face a different set of challenges [ 22 ]. The ability of LLMs to generate fake but persuasive responses further exacerbates the risks making users vulnerable to accepting erroneous information as fact [ 23 ]. For instance, a general practitioner faced with a dermatological case, such as an atypical presentation of psoriasis, can use an LLM to access detailed diagnostic criteria and treatment protocols. This capability can significantly assist in the management of the patient, particularly when the LLM’s suggestions are accurate and relevant. However, the inherent risk of LLMs generating incorrect suggestions cannot be overlooked. Such inaccuracies pose a heightened risk to patient safety, especially in scenarios where the clinician may lack the specialized dermatological knowledge required to critically evaluate the validity of the LLM’s output [ 24 ]—constituting an environment where trust becomes critical.
The crux of the problem lies in the user’s ability to verify the accuracy and relevance of the AI-generated content. However, the pivotal consideration here is whether the verification of LLM outcomes by health care staff negates the purported reduction in workload. If health care professionals are required to meticulously check each AI-generated output for accuracy, the time saved through automation may be offset by the time spent on verification. Maintaining the balance between productivity and accuracy is pivotal. For instance, LLMs can analyze vast data sets to identify patterns or treatment outcomes that may not be immediately apparent to human clinicians, thereby offering insights that can lead to more accurate diagnoses and personalized treatment plans. This capability, even if it requires additional time for verification of AI-generated recommendations, may be deemed a worthy trade-off for reducing long-term health care costs and improving care quality. However, this trade-off must be carefully managed to ensure that the pursuit of improved health outcomes does not lead to unmaintainable decreases in productivity. Excessive time spent verifying AI recommendations could strain health care resources, leading to longer patient wait times and potentially overburdening health care staff.
To navigate this trade-off, health care systems might adopt strategies such as targeted use of LLMs in high-impact areas where they are most likely to enhance outcomes and the development of systems that prioritize clarity and actionability in their recommendations to minimize verification time. By carefully weighing the benefits of improved patient outcomes against the costs in terms of productivity, health care providers can make informed decisions about how best to integrate LLMs into their practices, ensuring that these technologies serve to enhance rather than hinder the delivery of patient care.
User Trust: Willingness to Review LLM Output
Trust in user engagement with LLMs, particularly in health care, is a multifaceted construct influenced by sociotechnical and psychological factors. We acknowledge that user trust in LLMs, in health care, can substantially depend on the context. Depending on the stakes (risk) the level of trust required may differ; for instance, LLMs used for diagnosis and treatment recommendations necessitate a higher trust level compared to applications for patient note summarization. Additionally, the degree of autonomy granted to the LLMs, and the extent of clinical oversight are crucial determinants of trust.
Clinicians bring their own norms and expectations to the evaluation of trust in these systems, further complicating the landscape. Individual and cultural perspectives on risk tolerance and acceptance also play pivotal roles. Together, these factors create a complex environment where trust in LLMs is dynamic, varying according to the specific context of use and the interplay of diverse elements. In this section, we focus on user willingness to scrutinize LLM output as a precursor to trust.
A user may have the ability and necessary expertise but may not be willing to review LLM-generated outcomes due to factors including prior trust in the technology or biases. A doctor with high trust (blind trust) in the LLM, might be more inclined to accept its suggestion without extensive further verification [ 25 , 26 ], exhibiting automation bias [ 27 ]. Automation bias, particularly in the context of clinicians’ interactions with LLMs can manifest when clinicians exhibit an undue level of trust in the systems, based on past experiences of accuracy and reliability.
Blind trust in LLMs can introduce 2 critical cognitive biases, precautionary [ 28 ] and confirmation bias [ 29 ], both of which alter clinician behavior in the presence of agreement or disagreement between human judgment and LLM outputs. When LLM recommendations align with a clinician’s initial diagnosis or treatment plan (agreement), confirmation bias can be reinforced. Clinicians may overlook or undervalue subsequent information that contradicts the LLM-supported decision, even if this new information is critical to patient care. This confirmation bias can lead to a narrowed diagnostic vision, where alternative diagnoses or treatments are not sufficiently considered. Conversely, in cases where there is a disagreement, precautionary bias can occur. The clinician, having developed a reliance on the LLM due to positive past experiences, might doubt their own expertise and perceive LLM to be the safer alternative for decision-making. Such problems associated with blind trust might persist unchallenged until a point of failure or harm, which can have serious implications in health care.
Future Risk Considerations
As we delve deeper into the dynamics between technology and human expertise, the concepts of the LLM Paradox of Self-Referential Loop and the Risk of Deskilling emerge as pivotal to our discourse. As Figure 1 illustrates the projected trajectory of clinician reliance on LLMs but also hints at the potentially cyclic nature of knowledge and skills within the health care industry. Concurrently, the risk of deskilling looms over the horizon, particularly for upcoming generations of health care professionals who might become overly reliant on LLMs, possibly at the expense of their diagnostic acumen and critical thinking abilities. This section explores these challenges and the strategies needed to mitigate them. Additionally, this section discusses the LLM accountability concern.
LLM Paradox of Self-Referential Loop (Learning From Itself)
In a scenario where LLMs become widely adopted in the health care industry for tasks like paper writing, educational material creation, clinical text summarization, and risk identification, the possibility of a self-referential loop does emerge as a significant concern. This paradox occurs when AI-generated human-like content becomes so widespread that the AI begins to reference its own generated content, potentially leading to an echo chamber effect where original, human-generated insights become diluted or harder to distinguish from AI-generated content. While this problem of a self-referential loop in AI-generated content, particularly in the health care industry, has not yet materialized, it represents a likely challenge as generative AI continues to proliferate. The consequence of a self-referential loop in LLMs can lead to several problematic outcomes, including the propagation of biases [ 30 ], increased homogeneity in generated data, and ultimately, hindered performance. AI systems learn from the data they are fed, and if these data include biases, the AI is likely to replicate and even amplify these biases in its outputs [ 31 ]. In a self-referential loop, the problem becomes compounded. As the AI references its own biased outputs to generate new content, these biases can become more entrenched, making them harder to identify and correct.
The issue of self-referential loops and the potential degradation of information quality are indeed significant concerns; however, when these LLMs, such as the Medical Pathways Language Model (Med-PaLM) [ 32 ], are specifically fine-tuned and tailored for health care applications, the severity of these issues can be mitigated through stringent quality assurance measures. This approach reduces the risk associated with the indiscriminate use of a broader corpus that may contain inaccuracies, outdated information, or irrelevant content. Despite these precautions, the risk of self-referential loops in health care contexts can shift toward a different concern, the reinforcement and entrenchment of specific clinical approaches and schools of thought. This occurs as a reflection of the biases present in the curated data sets, which are inherently influenced by the prevailing medical practices, research focus, and therapeutic approaches at the time of data collection.
Addressing this challenge requires a nuanced approach to developing and integrating LLMs technologies into societal frameworks. It involves fostering a symbiotic relationship between human intellect and LLM capabilities, ensuring that AI serves as a tool for augmenting human intellect rather than replacing it. Strategies for maintaining the diversity and quality of training data, including the deliberate inclusion of varied and novel human-generated content, will be critical.
Risk of Deskilling
As individuals come to rely more on LLMs for routine tasks, such as the synthesis of patient information or the interpretation of medical data, there is a possibility that their skills in these critical areas may diminish over time due to reduced practice [ 33 ]. This situation is compounded by the AI’s ability to quickly furnish answers to medical inquiries, which might decrease the motivation for in-depth research and learning, consequently affecting the professionals’ knowledge depth and critical thinking capabilities.
It is crucial to note that the discussion here does not assert that LLMs will definitively lead to the deskilling of current practitioners in the health care sector. These professionals have developed their expertise through extensive experience and rigorous academic training, establishing a solid foundation that is not readily compromised by the integration of AI tools. Instead, the concern is more pronounced for the next generation of health care professionals, particularly medical students who might increasingly use AI for educational tasks and learning activities where over delegating tasks to AI could attenuate the development of critical analytical skills and a comprehensive understanding of medical concepts, traditionally cultivated through deep engagement with the material [ 33 , 34 ]. The critical question emerges “will the ease of generating content with AI stifle the development of creativity and critical thinking in younger generations accustomed to technology providing immediate solutions?”
If future generations of clinicians grow accustomed to AI doing the bulk of diagnostic review and analysis, there is a risk that their own diagnostic skills might not develop as fully. More critically, should they be required to review patient charts manually—due to AI failures—they may find the task daunting, or lack the detailed insight that manual review processes help to cultivate. The crux of the issue lies in ensuring that reliance on technology should not come at the expense of fundamental skills and knowledge. The challenge is to ensure that the deployment of AI technologies complements human abilities without diminishing the need for critical thinking, reasoning, and creativity.
What is needed is to adapt to the paradigm shift—failing to do so can adversely impact health care industry. A dual focus on harnessing AI capabilities while enhancing unique human skills is pivotal for advancing patient care in the modern medical landscape. The advent of human-AI collaboration in health care prompts a shift in the skill set emphasis within medical disciplines. The transformation accentuates the value of unique human skills—such as problem-solving, critical thinking, creativity, and fostering patient rapport—over traditional reliance on memory and knowledge base tasks. As LLMs undertake roles in diagnostic assistance, literature synthesis, and treatment optimization, the medical profession should evolve to leverage AI for data-driven insights while prioritizing human-centric skills for patient care. The paradigm shift underscores the growing importance of critical engagement with AI outputs, necessitating medical professionals to adeptly interpret and apply AI-generated information within the complex context of individual patient needs.
LLM Accountability
The integration of LLMs in health care introduces medicolegal challenges concerning the allocation and apportionment of liability for outcomes, particularly in instances of negligent diagnoses and treatment. The complexity arises from the interaction between clinicians, health care institutions, and AI providers, each contributing differently to the health care delivery process.
Legal Framework and Liability Allocation
In the legal domain, traditional frameworks for medical liability often center on direct human actions, with established principles guiding negligence and malpractice claims. The introduction of LLMs used for diagnostic support or task delegation complicates these frameworks. Clinicians, operating at the interface of LLM recommendations and patient care, are generally seen as the final decision-makers, thus bearing the primary moral and legal responsibility for the outcomes of those decisions. This perspective is grounded in the principle that clinicians must integrate LLM outputs into a broader clinical judgment context, considering patient-specific factors and adhering to professional standards.
Shared Liability and AI Providers
However, the role of LLM providers in developing, deploying, and maintaining LLMs introduces questions about shared liability, especially when system errors or deficiencies contribute to adverse outcomes. Determining the extent of LLM provider liability hinges on factors such as the accuracy of the LLM’s training data, transparency regarding the system’s capabilities and limitations, and the adequacy of user training and support provided.
Institutional Responsibility
Health care institutions also play a critical role in mediating the use of LLMs, responsible for ensuring that these systems are integrated into clinical workflows in a manner that upholds patient safety and complies with regulatory standards. Institutional policies and practices, including the selection of AI tools, clinician training, and oversight mechanisms, are pivotal in mitigating risks associated with LLM use.
Algorithmic Accountability Act of 2023
The Algorithmic Accountability Act of 2023 and Artificial Intelligence Accountability Act [ 35 , 36 ] represent a critical legislative step toward ensuring the responsible use of algorithms. The act calls for the creation of standardized procedures and assessment frameworks to evaluate the effectiveness and consequences of these systems, reflecting an understanding of the complex ethical and regulatory challenges posed by AI in decision-making processes, particularly in health care. The act is in dialogue with the wider conversation on the ethics of AI, advocating for an approach that emphasizes response-ability—the capacity to respond ethically to the challenges posed by algorithmic decision-making. This perspective is crucial for developing impact assessments and frameworks aimed at promoting fairness and preventing discriminatory practices within algorithmic systems.
The implications of this act on the integration of LLMs in health care are profound and ensuring transparency in LLM can further enhance trust in the system. Transparency can allow clinicians to verify errors and review outputs effectively. For example, an LLM providing a diagnostic suggestion would detail the medical literature and patient data informing its analysis, enhancing clinician trust by making the AI’s reasoning processes visible and understandable. This transparency combats algorithmic deference by encouraging health care professionals to critically assess LLM outputs against their expertise and patient-specific contexts. Moreover, transparency reduces the perceived infallibility of LLMs by highlighting their reliance on input data quality and inherent limitations, promoting a balanced use of LLMs as supportive tools in patient care.
Conclusions
It’s important to acknowledge that the performance of LLMs like ChatGPT (OpenAI) today does not guarantee their performance tomorrow. LLMs have the potential to be a substantial boon to the health care industry, offering to streamline workflows, enhance the accuracy of patient data processing, and even support diagnostic and treatment planning processes. Its value, however, is contingent upon a systematic and informed integration into health care systems. Recognizing that LLMs, like any technology, is fallible is crucial to its successful adoption. Its performance is temporal and will change as new data are fed to its algorithm. This acknowledgment underpins the necessity for robust oversight mechanisms, ongoing evaluation of AI-driven outputs for accuracy and relevance, and clear guidelines on its role as an assistive tool rather than a stand-alone decision-maker.
A thoughtful, deliberate approach to integrating generative AI into health care can mitigate risks associated with overreliance and deskilling, ensuring that it complements rather than compromises the quality of care. By leveraging AI’s strengths and compensating for its limitations through human oversight, health care can harness the benefits of this technology to improve outcomes, enhance patient care, and support health care professionals in their vital work. Thus, the path forward involves embracing generative AI’s potential while remaining vigilant about its limitations, ensuring that its integration enhances rather than diminishes the human element in health care.
Acknowledgments
This study is not funded by any internal or external agency.
Conflicts of Interest
None declared.
- Harris LA. Artificial intelligence: background, selected issues, and policy considerations. Congressional Research Service. 2021. URL: https://crsreports.congress.gov/product/pdf/R/R46795 [accessed 2024-04-02]
- DoC. AI accountability policy request for comment. Natonal Archives. 2023. URL: https://www.federalregister.gov/documents/2023/04/13/2023-07776/ai-accountability-policy-request-for-comment [accessed 2024-04-02]
- Shinners L, Aggar C, Grace S, Smith S. Exploring healthcare professionals' understanding and experiences of artificial intelligence technology use in the delivery of healthcare: an integrative review. Health Informatics J. 2020;26(2):1225-1236. [ FREE Full text ] [ CrossRef ] [ Medline ]
- Hegde N, Vardhan M, Nathani D, Rosenzweig E, Speed C, Karthikesalingam A, et al. Infusing behavior science into large language models for activity coaching. PLOS Digit Health. Apr 2024;3(4):e0000431-e0000413. [ FREE Full text ] [ CrossRef ] [ Medline ]
- Firaina R, Sulisworo D. Exploring the usage of ChatGPT in higher education: frequency and impact on productivity. Bul Edukasi Indones. 2023;2(01):39-46. [ CrossRef ]
- Sedaghat S. Early applications of ChatGPT in medical practice, education and research. Clin Med (Lond). 2023;23(3):278-279. [ FREE Full text ] [ CrossRef ] [ Medline ]
- Hung YC, Chaker SC, Sigel M, Saad M, Slater ED. Comparison of patient education materials generated by chat generative pre-trained transformer versus experts: an innovative way to increase readability of patient education materials. Ann Plast Surg. 2023;91(4):409-412. [ CrossRef ] [ Medline ]
- Galido PV, Butala S, Chakerian M, Agustines D. A case study demonstrating applications of ChatGPT in the clinical management of treatment-resistant schizophrenia. Cureus. 2023;15(4):e38166. [ FREE Full text ] [ CrossRef ] [ Medline ]
- Morrison M, Nobles V, Johnson-Agbakwu CE, Bailey C, Liu L. Classifying refugee status using common features in EMR. Chem Biodivers. 2022;19(10):e202200651. [ CrossRef ] [ Medline ]
- Yeo YH, Samaan JS, Ng WH, Ting PS, Trivedi H, Vipani A, et al. Assessing the performance of ChatGPT in answering questions regarding cirrhosis and hepatocellular carcinoma. Clin Mol Hepatol. 2023;29(3):721-732. [ FREE Full text ] [ CrossRef ] [ Medline ]
- Chen TJ. ChatGPT and other artificial intelligence applications speed up scientific writing. J Chin Med Assoc. 2023;86(4):351-353. [ FREE Full text ] [ CrossRef ] [ Medline ]
- Malamas N, Papangelou K, Symeonidis AL. Upon improving the performance of localized healthcare virtual assistants. Healthcare (Basel). 2022;10(1):99. [ FREE Full text ] [ CrossRef ] [ Medline ]
- Wang DQ, Feng LY, Ye JG, Zou JG, Zheng YF. Accelerating the integration of ChatGPT and other large‐scale AI models into biomedical research and healthcare. MedComm—Future Medicine. 2023;2(2):1-28. [ FREE Full text ] [ CrossRef ]
- Hoffmann J, Borgeaud S, Mensch A, Buchatskaya E, Cai T, Rutherford E, et al. Training compute-optimal large language models. ArXiv. preprint posted online on March 29, 2022. [ FREE Full text ] [ CrossRef ]
- Seth I, Kenney PS, Bulloch G, Hunter-Smith DJ, Thomsen JB, Rozen WM. Artificial or augmented authorship? A conversation with a chatbot on base of thumb arthritis. Plast Reconstr Surg Glob Open. 2023;11(5):e4999. [ FREE Full text ] [ CrossRef ] [ Medline ]
- Yeung JA, Kraljevic Z, Luintel A, Balston A, Idowu E, Dobson RJ, et al. AI chatbots not yet ready for clinical use. Front Digit Health. 2023;5:1161098. [ FREE Full text ] [ CrossRef ] [ Medline ]
- Cloesmeijer ME, Janssen A, Koopman SF, Cnossen MH, Mathôt RAA, SYMPHONY consortium. ChatGPT in pharmacometrics? Potential opportunities and limitations. Br J Clin Pharmacol. Jan 2024;90(1):360-365. [ CrossRef ] [ Medline ]
- De Angelis L, Baglivo F, Arzilli G, Privitera GP, Ferragina P, Tozzi AE, et al. ChatGPT and the rise of large language models: the new AI-driven infodemic threat in public health. Front Public Health. 2023;11:1166120. [ FREE Full text ] [ CrossRef ] [ Medline ]
- Gravel J, D'Amours-Gravel M, Osmanlliu E. Learning to fake it: limited responses and fabricated references provided by ChatGPT for medical questions. MCP: Digital Health. 2023;1(3):226-234. [ FREE Full text ] [ CrossRef ]
- Sison AJG, Daza MT, Gozalo-Brizuela R, Garrido-Merchán EC. ChatGPT: more than a "weapon of mass deception" ethical challenges and responses from the Human-Centered Artificial Intelligence (HCAI) perspective. Int J Hum Comput. 2023.:1-20. [ CrossRef ]
- Birenbaum M. The chatbots' challenge to education: disruption or destruction? Educ Sci (Basel). 2023;13(7):711. [ FREE Full text ] [ CrossRef ]
- Dwivedi YK, Kshetri N, Hughes L, Slade EL, Jeyaraj A, Kar AK, et al. Opinion paper: "So what if ChatGPT wrote it?" Multidisciplinary perspectives on opportunities, challenges and implications of generative conversational AI for research, practice and policy. Int J Inf Manag. 2023;71:102642. [ FREE Full text ] [ CrossRef ]
- Shahsavar Y, Choudhury A. User intentions to use ChatGPT for self-diagnosis and health-related purposes: cross-sectional survey study. JMIR Hum Factors. 2023;10:e47564. [ FREE Full text ] [ CrossRef ] [ Medline ]
- Dempere J, Modugu K, Hesham A, Ramasamy LK. The impact of ChatGPT on higher education. Front Educ. 2023;8:1206936. [ FREE Full text ] [ CrossRef ]
- Choudhury A, Shamszare H. Investigating the impact of user trust on the adoption and use of ChatGPT: survey analysis. J Med Internet Res. 2023;25:e47184. [ FREE Full text ] [ CrossRef ] [ Medline ]
- Asan O, Bayrak AE, Choudhury A. Artificial intelligence and human trust in healthcare: focus on clinicians. J Med Internet Res. 2020;22(6):e15154. [ FREE Full text ] [ CrossRef ] [ Medline ]
- Goddard K, Roudsari A, Wyatt JC. Automation bias: a systematic review of frequency, effect mediators, and mitigators. J Am Med Inform Assoc. 2012;19(1):121-127. [ FREE Full text ] [ CrossRef ] [ Medline ]
- Vlassov VV. Precautionary bias. Eur J Public Health. 2017;27(3):389. [ FREE Full text ] [ CrossRef ] [ Medline ]
- Oswald ME, Grosjean S. Confirmation bias. In: Cognitive Illusions: A Handbook on Fallacies and Biases in Thinking, Judgement and Memory. Hove, East Sussex, United Kingdom. Psychology Press; 2004;79-96.
- Meyer JG, Urbanowicz RJ, Martin PCN, O'Connor K, Li R, Peng PC, et al. ChatGPT and large language models in academia: opportunities and challenges. BioData Min. 2023;16(1):20. [ FREE Full text ] [ CrossRef ] [ Medline ]
- Nazir A, Wang Z. A comprehensive survey of ChatGPT: advancements, applications, prospects, and challenges. Meta Radiol. 2023;1(2):100022. [ FREE Full text ] [ CrossRef ] [ Medline ]
- Singhal K, Azizi S, Tu T, Mahdavi SS, Wei J, Chung HW, et al. Large language models encode clinical knowledge. Nature. 2023;620(7972):172-180. [ FREE Full text ] [ CrossRef ] [ Medline ]
- Lam K. ChatGPT for low- and middle-income countries: a Greek gift? Lancet Reg Health West Pac. 2023;41:100906. [ FREE Full text ] [ CrossRef ] [ Medline ]
- Lo CK. What is the impact of ChatGPT on education? A rapid review of the literature. Educ Sci (Basel). 2023;13(4):410. [ FREE Full text ] [ CrossRef ]
- H.R.3369—118th congress (2023-2024). Congress. 2023. URL: https://www.congress.gov/bill/118th-congress/house-bill/3369 [accessed 2024-04-02]
- Algorithmic accountability act of 2023. Office of U.S. Senator Ron Wyden. 2023. URL: https://www.congress.gov/bill/118th-congress/senate-bill/2892/text [accessed 2024-04-02]
Abbreviations
Edited by T de Azevedo Cardoso, G Eysenbach; submitted 25.01.24; peer-reviewed by M Saremi, D Hua; comments to author 08.03.24; revised version received 12.03.24; accepted 20.03.24; published 25.04.24.
©Avishek Choudhury, Zaira Chaudhry. Originally published in the Journal of Medical Internet Research (https://www.jmir.org), 25.04.2024.
This is an open-access article distributed under the terms of the Creative Commons Attribution License (https://creativecommons.org/licenses/by/4.0/), which permits unrestricted use, distribution, and reproduction in any medium, provided the original work, first published in the Journal of Medical Internet Research, is properly cited. The complete bibliographic information, a link to the original publication on https://www.jmir.org/, as well as this copyright and license information must be included.

An official website of the United States government
The .gov means it’s official. Federal government websites often end in .gov or .mil. Before sharing sensitive information, make sure you’re on a federal government site.
The site is secure. The https:// ensures that you are connecting to the official website and that any information you provide is encrypted and transmitted securely.
- Publications
- Account settings
Preview improvements coming to the PMC website in October 2024. Learn More or Try it out now .
- Advanced Search
- Journal List
- BMJ - PMC COVID-19 Collection

Organisation of care in paediatric intensive care units during the first 18 months of the COVID-19 pandemic: a scoping review protocol
1 School of Nursing, Midwifery & Health Systems, University College Dublin, Dublin, Ireland
Catherine McCabe
2 School of Nursing & Midwifery, Trinity College Dublin, Dublin, Ireland
Maria Brenner
Associated data, introduction.
The emergence of COVID-19 has had a significant impact on hospital services, particularly care delivered to those in intensive care units (ICUs) and paediatric ICUs (PICUs) across the world. Although much has been written about healthcare delivery and the healthcare setting since COVID-19 began, to the authors’ knowledge, this is the first scoping review to investigate the organisation of care and changes implemented in PICUs during the COVID-19 pandemic. The aim is to conduct a scoping review of the literature to map out the existing studies about care delivery in PICUs during the COVID-19 pandemic and the changes made to the organisation of care in these units during the first 18 months of the pandemic. This review will also identify gaps in current knowledge in this area.
Methods and analysis
This study will be guided by the Joanna Briggs Institute’s methodology for scoping reviews, using Arksey and O’Malley’s six-stage scoping review framework: (1) identifying the research question; (2) identifying relevant studies; (3) selecting the studies; (4) data charting; (5) collating, summarising and reporting results; and (6) consulting with experts. A comprehensive search will be conducted using the following databases: CINAHL Complete; MEDLINE; PsycINFO; PsycARTICLES and EMBASE. A search strategy with predefined inclusion and exclusion criteria will be used to uncover relevant research in this area. This study will include quantitative, qualitative and mixed research methods studies published in English from 2019 to May 2021.
Ethics and dissemination
Ethical approval is not required for this scoping review. The results from this study will be disseminated through conferences and in peer-reviewed academic journals for those working in the healthcare arena.
Strengths and limitations of this study
- This scoping review protocol will provide the basis for a scoping review exploring this critical area of the organisation of care in paediatric intensive care units (PICUs) during the first 18 months of the COVID-19 pandemic; to the authors’ knowledge, this is the first of its kind.
- This scoping review will conform to the rigorous Joanna Briggs Institute methodology manual.
- Limitations of the review include: inclusion of English texts only, due to non-availability of translators for this review; and potential bias towards high-income countries, although the search of grey literature limits this risk.
The first human cases of COVID-19 were reported from Wuhan, China in December 2019; and since then, COVID-19 has spread rapidly across the globe. 1 The outbreak was declared a Public Health Emergency of International Concern in January 2020, and a pandemic in March 2020 due to the worldwide spread of this new disease. 2 As a result of COVID-19, there has been a substantial impact on hospital services, particularly care delivered to those in intensive care units (ICUs) and paediatric ICUs (PICUs) across the world. Globally, children are admitted to PICUs for respiratory and or haemodynamic monitoring for a variety of reasons including post-surgery for continuous monitoring 3 ; with exacerbation of medical conditions 4 5 ; post-severe accident or injury 6 ; and children with complex conditions. 7 8
PICUs provide an increased level of clinical observation, invasive monitoring, specialised interventions and technical support to care for critically ill children over an indefinite period of time. 9 PICUs care for children from birth, typically to their 18th birthday, although some children from the age of 16 years will be cared for in an adult ICU. 10 A multidisciplinary team works within a PICU and comprises of professionals such as paediatric intensivists, nurses, pharmacists, physiotherapists, dietitians, speech and language therapists, occupational therapists, social workers and psychologists. 11
Since the beginning of the pandemic in December 2019, there have been significant adjustments in some PICUs worldwide, including transitioning into adult ICUs to meet the increasing demands of patient needs. 12–19 Lynn et al 20 discovered in Ireland and the UK that the COVID-19 pandemic has brought additional challenges to healthcare for children ranging from delays to presenting to the emergency department for fear of contracting COVID-19 and decline in presenting for scheduled hospital appointments leading to serious health consequences for children. Similar findings have been reported in additional studies in Ireland 21–23 and the UK, 24–28 and across numerous other countries including Italy, 29–32 the Netherlands, 33 Germany, 34 Canada, 35 the USA 36–38 and Australia. 39
COVID-19 has impacted all aspects of healthcare delivery, including the care delivered in PICU, both for children admitted with COVID-19 and associated complications, alongside the general adjustments required for effective care delivery during a pandemic. Recent research has begun to explore the psychological impact of COVID-19 on healthcare staff working within critical care, 40–45 the challenges faced as a result of COVID-19 46 and their experiences of working during the pandemic. 47–50
Aims and objectives
The aim of this scoping review is to:
- Identify the existing studies and explore what is known about the organisation of care in PICUs during the first 18 months of the COVID-19 pandemic.
This review also aims to explore and summarise the evidence available and the diversity of the studies published. This review will also identify any gaps in the literature to identify areas for future research.
Knowledge regarding the effects of COVID-19 pandemic within the healthcare system is dynamic, with new research studies rapidly emerging. A scoping review was chosen as the most appropriate approach to collating and critiquing the current research on the transitions in the organisation of care occurring in PICUs as a result of the pandemic. The organisation of care within PICU will consider factors including resources, staffing, equipment and technology. 51 52 As scoping reviews are useful for examining emerging evidence, this was selected as the most appropriate method for reviewing the evidence in this area. 53 This scoping review will follow Arksey and O’Malley’s 54 six-stage scoping review framework: (1) identifying the research question; (2) identifying relevant studies; (3) selecting the studies; (4) data charting; (5) collating, summarising and reporting results; and (6) consulting with experts. This scoping review protocol will outline how each stage will be addressed.
Stage 1: identification of the scoping review research question
The research question and focus of the scoping review was clearly identified through an initial search using the key search terms to capture the most appropriate literature. As recommended by Anderson et al 55 and Joanna Briggs Institute (JBI), 56 57 the population, concept and context framework was applied to form the research question.
The research question for this scoping review is exploring:
How was care organised in PICUs during the first 18 months of the COVID-19 pandemic?
Stage 2: identifying relevant studies
Three researchers identified the databases for the literature search process. A comprehensive search will be conducted in the following databases: CINAHL Complete; MEDLINE; PsycINFO; PsycARTICLES and EMBASE. These sources include journals in the area of healthcare. Grey literature will be included in the data searching to ensure all relevant scientific evidence in this arena will be explored. The inclusion criteria for this review will be based on the population–concept–context framework recommended by the JBI. 56 Discussion among the three researchers regarding inclusion and exclusion criteria at the start of the review process occurred, with each researcher agreeing with the final criteria. The eligibility criteria for this study is outlined in table 1 .
Eligibility criteria for this study
Stage 3: selecting studies
There is debate in the literature about the need for additional researchers to undertake the initial screening of reviewing titles and abstracts from the search results. 58 59 The researchers have agreed that two reviewers will independently undertake all steps in the process as outlined below. Three reviewers will collaborate to create search keywords to uncover relevant research using Arksey and O’Malley’s framework. 54 A brief literature search in journals of relevance will be conducted to identify any additional keywords, in addition to consultation with experts in the area and healthcare professionals working within PICU. These provisional keywords are outlined in table 2 .
Keywords for the literature search strategy
Arksey and O’Malley 54 advocate that scoping reviews should retrieve all relevant studies of all design types, thus all design types will be included in this review. Two reviewers will independently screen the results for inclusion based on title and abstract to ensure transparency. Full-text reviews of the selected studies will be conducted based on the prespecified inclusion and exclusion criteria relevant to the research question. The reviewers will meet at each stage of the review process to debate challenges, clarify any inconsistencies and make refinements to the search. 58 If there are any discrepancies with any of the decisions, an additional reviewer who is an expert in the field will be consulted. A Preferred Reporting Items for Systematic Reviews and Meta-Analyses (PRISMA) flow chart will be produced after the completed searches to ensure transparency of reporting 56 and detail the search strategy and how decisions were made. 60 Rationale for exclusion of articles will be clearly documented.
Stage 4: data charting
Data charting will be carried out independently by two reviewers and focuses on details of the individual studies. Data extraction will occur using a checklist or data charting form influenced by the JBI Reviewer’s Manual. 56 58 The charting characteristics and associated data extraction details are outlined in table 3 . This process will be flexible to integrate new characteristics of the included studies if this will improve the data analysis, for example, any additional characteristics about the participants within the studies. This stage will support the next stage of reporting results and identifying themes. As recommended by Daudt et al, 61 each data charting form will be given a unique code to assist with identification and discussion with the review team. These charts, which have been completed independently by two reviewers, will be compared to pilot the tool, while assessing if the results are consistent with the research question. Daudt et al 61 advocate this approach to improve the data charting phase to ensure the review question can be answered. Scoping review processes are iterative processes, and this systematic repetition of tasks will be completed for each study and discussed with the review team. If there are any changes made to the data charting form, these will be noted in addition to any decisions made regarding screening, as a result of meetings with the review team, reflections and actions taken. 62
Characteristics of the studies identified
Stage 5: collating, summarising and reporting the results
This scoping review will be reported following the PRISMA Extension for Scoping Reviews 53 and the guidelines outlined by JBI Reviewer’s Manual for Scoping Reviews. 56 Quality appraisal and risk of bias of the included articles are not consistent within the conduct of a scoping review. 58 Subsequently, the methodological quality of each article will be outlined not appraised in detail, and the researcher will report this throughout the discussion and synthesis of findings. As scoping reviews are not aimed at producing critically appraised results to the review question, but more so provide an overview of the available evidence, methodological assessments will not be completed on the studies included in this review. 63 Thematic analysis will be used to analyse the findings of the studies, using qualitative descriptive methods to review the literature as advised by Levac et al . 58 Findings will be grouped into thematic categories and the key findings will be presented.
The general characteristics of the studies, relevant to the scoping review aim, will be identified; the data will be extracted and charted and the findings will be described and summarised. 56 The conclusions, strengths and limitations identified within this review will be documented. This review will illustrate the changes occurring within PICUs during the COVID-19 pandemic.
Stage 6: consultation
Two of the reviewers are very experienced paediatric nurses, with one having a background in children’s intensive care nursing. Consultation will be also made with relevant experts working in the PICUs at the outset of the review for selecting keywords to ensure all relevant areas are covered, for example, a paediatric nurse working in PICU during the pandemic. Networking with relevant individuals will continue to occur virtually, due to the current restrictions associated with COVID-19. As advocated by Levac et al, 58 a cross-disciplinary consultation will occur in the planning of this study, selecting keywords and identification of inclusion and exclusion criteria if required. Further consultation will be sought if necessary at any stage during the review and will be explained in the review where required.
Patient and public involvement
No patient involvement will be sought for this review, but there is patient and public involvement engagement in the wider programme of research of which this review is a part of.
A scoping review protocol has been explained in relation to the current literature available. 56–58 61 This scoping review will contribute to knowledge in this area and inform a research project in this area. The rationale for choosing a scoping review over other reviews is to map the scientific evidence in this area to inform further research as the knowledge base in this area is still being created and evaluated.
This pandemic is not unprecedented, with other pandemics occurring previously including the Spanish Influenza in 1918–1919, 64 the Asian Influenza in 1957–1958 65 and the SARS pandemic in 2002. 66 Previous research has highlighted some guidelines for the management of children with COVID-19 in PICU, although a number of these are acknowledged as weak or having insufficient evidence to make recommendations. 67 This review offers an opportunity to learn from this pandemic and provide recommendations for future global pandemics in a PICU setting. A scoping review will contribute to ensuring that future research in this area can be planned appropriately to address any gaps in the scientific knowledge.
The authors believe that the information gained through this scoping review will:
- Contribute to the knowledge in the field about adaptations and transitions occurring in PICUs during a global pandemic.
- Assist in providing recommendations and planning for the practical implications that may be required in future during another global pandemic.
- Offer an opportunity for mitigating the negative impact of COVID-19 on the health services and support change and growth in PICUs across the world.
- Contribute beneficial knowledge in the event of further waves of COVID-19, but also in the face of other inevitable, future healthcare crises through the findings and recommendations resulting from this review.
Research ethics
Ethical approval is not required for this scoping review. However, this study is part of the TechChild Project; ‘Just because we can, should we? An anthropological perspective on the initiation of technology dependence to sustain a child’s life’. TechChild, funded by the European Research Council, is a programme of research exploring international influences on the initiation of technological support for children. Ethical approval was previously obtained from the relevant academic and clinical Research Ethics Committees, nationally and internationally for the TechChild Project.
Dissemination
The results from this study will be disseminated through conferences and in peer-reviewed academic journals for those working in the healthcare arena.
Supplementary Material
Contributors: KH provided the original idea and drafted the first manuscript. All authors (KH, CM, MB) made revisions and contributed to the final manuscript.
Funding: This project has received funding from the European Research Council (ERC) under the European Union’s Horizon 2020 research and innovation programme (grant agreement no. 803051).
Competing interests: None declared.
Patient and public involvement: Patients and/or the public were not involved in the design, or conduct, or reporting, or dissemination plans of this research.
Provenance and peer review: Not commissioned; externally peer reviewed.
Ethics statements
Patient consent for publication.
Not required.
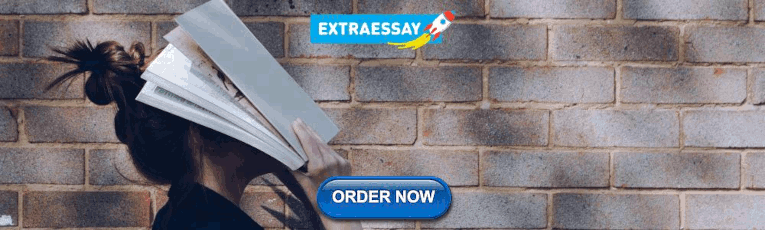
IMAGES
VIDEO
COMMENTS
Introduction. Paediatric critical and intensive care medicine have rapidly evolved into an extraordinarily complex and intricate medical field. 1 Paediatric intensive care units (PICUs) are high-acuity units that provide an increased level of clinical observation, invasive monitoring, specialised interventions and technical support to care for critically ill children with a range of medical ...
The exponential growth in pediatric intensive care research (1 citation under PICU in 1979 in PubMed compared with 904 in 2020), the development of training programmes, and advancements in technology, diagnostics and surgical/anaesthetic techniques have contributed to a remarkable reduction in unadjusted and case-mix adjusted PICU mortality, but challenges and unmet needs still exist in the ...
Pediatric Critical Care Medicine | Society of Critical Care Medicine Subscribe to the Pediatric Critical Care Medicine Journal, written for the entire critical care team and for those who deal with pediatric patients. ... Pediatric Critical Care Medicine and Cardiac Critical Care Research. Checchia, Paul A. Pediatric Critical Care Medicine. 24 ...
Journal of Pediatric Critical Care. 10(5):186-192, Sep-Oct 2023. Abstract. Favorite; PDF; Permissions Open. Review Article Integrated care in cardiac intensive care unit for noncardiovascular complications in children after open-heart surgery: A comprehensive review ...
Clinical research in pediatric intensive care has increased in quantity and quality over the past several decades. 1. National and international research networks, large electronic registries, sophisticated statistical software, innovative trial designs, shifts in funding priorities toward pragmatic clinical trials, and other advancements have ...
The very existence of this study, the participation of medical and paramedical teams for more than 5 years now in the Ethics of Invasive Care in Pediatric Intensive Care Unit (EICPICU) study [1], but also the strong involvement of parents themselves in the research similarly show to what extent the role of parents in hospital services has ...
Editorial on the Research Topic Case reports in pediatric critical care 2022. While critical illness in children has existed since the dawn of humankind, the ability to understand these illnesses and provide therapies in dire circumstances is relatively new. The fledgling field began with the technological advances of the mid-20th century ...
Conclusions: This paper has identified important consensus-derived priorities for clinical research in pediatric critical care nutrition. Future studies should determine topics that are a priority for patients and parents. Research funding should target these priority areas and promote an international collaborative approach to research in this ...
Objective Survival of babies born very preterm (<32 weeks gestational age) has increased, although preterm-born children may have ongoing morbidity. We aimed to investigate the risk of admission to paediatric intensive care units (PICUs) of children born very preterm following discharge home from neonatal care. Design Retrospective cohort study, using data linkage of National Neonatal Research ...
To compare the experiences of parents and children during inpatient admission to either a paediatric intensive care unit (PICU) or a general paediatric ward (GPW) with a specific focus on identifying factors which may influence psychological outcome ... This meta-synthesis of 15 primary research papers has provided novel insights into the ...
cically to neonatal and paediatric intensive care. None of these solutions was ideal to foster research and devel- ... gain of knowledge represented by papers written by pae-diatric and adult specialists together under the heading: ... spectrum of research in the eld of intensive care medi-cine. ICM represents the "mother journal", ...
The objective is to identify the available literature on parents' experiences in caring for children in the Paediatric Intensive Care Unit (PICU). Original research on parents experience in caring their child in PICU were identified from five online databases, namely CINAHL, Science Direct, Proquest, Cochrane, and Pubmed (2008-2018) using ...
Pediatric intensive care units (PICUs) are highly specialized medical facilities dealing with both conditions that are unique to children and others that are also found in adults but require a different, age-related approach. ... In addition to reviews of relevant PICU topics, all types of research papers, from laboratory studies to pro- and ...
Focus on paediatrics. Since a wise man or woman proportions his or her belief to the evidence [ 1 ], this focus on paediatrics discusses interesting and influential papers that have been published in important journals in 2018, related to paediatric intensive care unit (PICU) clinical research.
A new era just started, and we are sure that European paediatric and neonatal intensive care will take giant steps. Although our research is of high quality, often, NICU/PICU-based articles are unfunded or only partially funded especially at a European level [].ESPNIC is highly committed to support members and this translates in covering article processing charges as much as possible for the ...
Pediatric intensive care, Paediatric Intensive Care, Fluid Therapy Pediatric and Congenital Cardiac Care: Volume 2: Patient Safety and Quality Improvement This book is the second in a two-volume set of textbooks and focuses on quality improvement and patient safety, supporting the coverage of outcomes analysis in its sister title.
An editorial published in JAMA Pediatrics about the call for papers centered around AI applied to pediatric care. Skip to content. Search. Data Dashboards; Regenstrief Data Services; Careers; Donate; Search. ... Mazer MB, Carroll CL, Shein SL, Remy KE. The use of machine learning and artificial intelligence within pediatric critical care ...
Aim: This study examined paediatric intensive care unit nurses' lived experience of caring for families following a major hospital transformation project, which included the construction of a new unit and quality improvement changes. Study design: A hermeneutic-phenomenological design was selected to study a paediatric intensive care unit in a ...
As the health care industry increasingly embraces large language models (LLMs), understanding the consequence of this integration becomes crucial for maximizing benefits while mitigating potential pitfalls. This paper explores the evolving relationship among clinician trust in LLMs, the transition of data sources from predominantly human-generated to artificial intelligence (AI)-generated ...
Paediatric intensive care units and healthcare staff working in this area. Other departments within the hospital. ... Studies from peer-reviewed journals including papers using qualitative, quantitative, mixed methods and case study examples; grey literature. ... Patient and public involvement in paediatric intensive care research ...