Have a language expert improve your writing
Run a free plagiarism check in 10 minutes, generate accurate citations for free.
- Knowledge Base
- Interval Data and How to Analyze It | Definitions & Examples
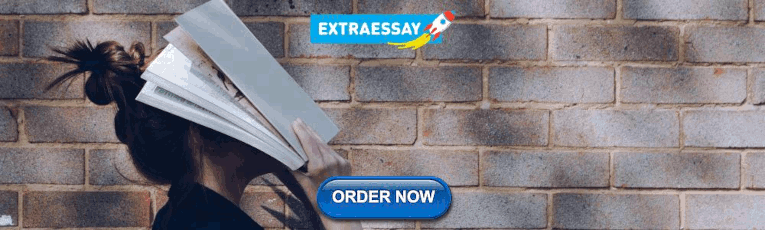
Interval Data and How to Analyze It | Definitions & Examples
Published on August 28, 2020 by Pritha Bhandari . Revised on June 21, 2023.
Interval data is measured along a numerical scale that has equal distances between adjacent values. These distances are called “intervals.”
There is no true zero on an interval scale, which is what distinguishes it from a ratio scale . On an interval scale, zero is an arbitrary point, not a complete absence of the variable .
Common examples of interval scales include standardized tests, such as the SAT, and psychological inventories.
Table of contents
Levels of measurement, interval vs ratio scales, examples of interval data, interval data analysis, other interesting articles, frequently asked questions about interval data.
Interval is one of four hierarchical levels of measurement . The levels of measurement indicate how precisely data is recorded. The higher the level, the more complex the measurement is.
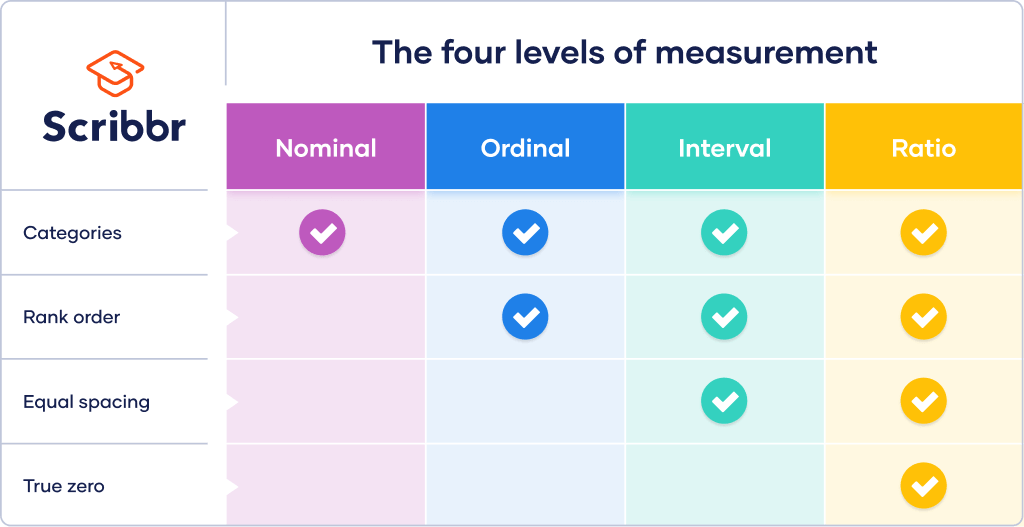
While nominal and ordinal variables are categorical, interval and ratio variables are quantitative. Many more statistical tests can be performed on quantitative than categorical data.
Prevent plagiarism. Run a free check.
Interval and ratio scales both have equal intervals between values. However, only ratio scales have a true zero that represents a total absence of the variable.
Celsius and Fahrenheit are examples of interval scales . Each point on these scales differs from neighboring points by intervals of exactly one degree. The difference between 20 and 21 degrees is identical to the difference between 225 and 226 degrees.
However, these scales have arbitrary zero points – zero degrees isn’t the lowest possible temperature.
Because there’s no true zero, you can’t multiply or divide scores on interval scales. 30°C is not twice as hot as 15°C. Similarly, -5°F is not half as cold as -10°F.
In contrast, the Kelvin temperature scale is a ratio scale . In the Kelvin scale, nothing can be colder than 0 K. Therefore, temperature ratios in Kelvin are meaningful: 20 K is twice as hot as 10 K.
Psychological concepts like intelligence are often quantified through operationalization in tests or inventories. These tests have equal intervals between scores, but they do not have true zeros because they cannot measure “zero intelligence” or “zero personality.”
To identify whether a scale is interval or ordinal , consider whether it uses values with fixed measurement units, where the distances between any two points are of known size. For example:
- A pain rating scale from 0 (no pain) to 10 (worst possible pain) is interval.
- A pain rating scale that goes from no pain, mild pain, moderate pain, severe pain, to the worst pain possible is ordinal.
Treating your data as interval data allows for more powerful statistical tests to be performed.
To get an overview of your data, you can first gather the following descriptive statistics :
- the frequency distribution in numbers or percentages,
- the mode , median , or mean to find the central tendency ,
- the range , standard deviation and variance to indicate the variability .
Distribution
Tables and graphs can be used to organize your data and visualize its distribution.
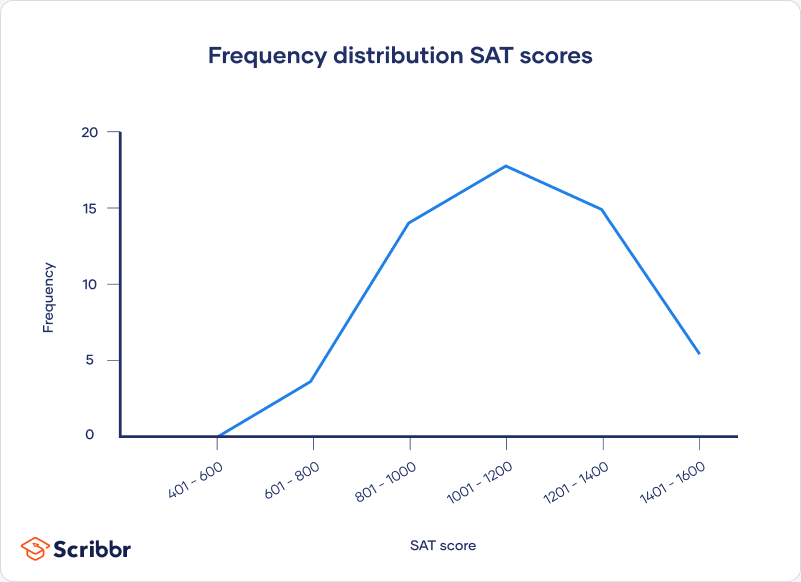
Central tendency
From your graph, you can see that your data is fairly normally distributed . Since there is no skew , to find where most of your values lie, you can use all 3 common measures of central tendency : the mode, median and mean.
( n +1)/2 = (59+1)/2 = 30
The median is in the 30th position, which has a value of 1120 . The mean uses all values to give you a single number for the central tendency of your data. To find the mean, use the formula of ⅀x/ n . Sum up all values (⅀x) and divide the sum by n.
⅀x = 65850 n = 59 ⅀x/ n = 65850/59 = 1116.1
The mean is usually considered the best measure of central tendency when you have normally distributed quantitative data. That’s because it uses every single value in your data set for the computation, unlike the mode or the median.
Variability
The range, standard deviation and variance describe how spread your data is. The range is the easiest to compute while the standard deviation and variance are more complicated, but also more informative.
- Standard deviation
Range = 1500 – 620 = 880
s 2 = 44279.36
Statistical tests
Now that you have an overview of your data, you can select appropriate tests for making statistical inferences. With a normal distribution of interval data, both parametric and non-parametric tests are possible.
Parametric tests are more statistically powerful than non-parametric tests and let you make stronger conclusions regarding your data. However, your data must meet several requirements for parametric tests to apply.
The following parametric tests are some of the most common ones applied to test hypotheses about interval data.
If you want to know more about statistics , methodology , or research bias , make sure to check out some of our other articles with explanations and examples.
- Student’s t -distribution
- Normal distribution
- Null and Alternative Hypotheses
- Chi square tests
- Confidence interval
Methodology
- Cluster sampling
- Stratified sampling
- Data cleansing
- Reproducibility vs Replicability
- Peer review
- Likert scale
Research bias
- Implicit bias
- Framing effect
- Cognitive bias
- Placebo effect
- Hawthorne effect
- Hindsight bias
- Affect heuristic
Levels of measurement tell you how precisely variables are recorded. There are 4 levels of measurement, which can be ranked from low to high:
- Nominal : the data can only be categorized.
- Ordinal : the data can be categorized and ranked.
- Interval : the data can be categorized and ranked, and evenly spaced.
- Ratio : the data can be categorized, ranked, evenly spaced and has a natural zero.
While interval and ratio data can both be categorized, ranked, and have equal spacing between adjacent values, only ratio scales have a true zero.
For example, temperature in Celsius or Fahrenheit is at an interval scale because zero is not the lowest possible temperature. In the Kelvin scale, a ratio scale, zero represents a total lack of thermal energy.
Individual Likert-type questions are generally considered ordinal data , because the items have clear rank order, but don’t have an even distribution.
Overall Likert scale scores are sometimes treated as interval data. These scores are considered to have directionality and even spacing between them.
The type of data determines what statistical tests you should use to analyze your data.
Cite this Scribbr article
If you want to cite this source, you can copy and paste the citation or click the “Cite this Scribbr article” button to automatically add the citation to our free Citation Generator.
Bhandari, P. (2023, June 21). Interval Data and How to Analyze It | Definitions & Examples. Scribbr. Retrieved April 2, 2024, from https://www.scribbr.com/statistics/interval-data/
Is this article helpful?
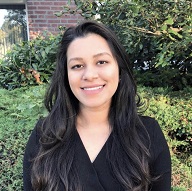
Pritha Bhandari
Other students also liked, levels of measurement | nominal, ordinal, interval and ratio, nominal data | definition, examples, data collection & analysis, ordinal data | definition, examples, data collection & analysis, what is your plagiarism score.
Helping math teachers bring statistics to life

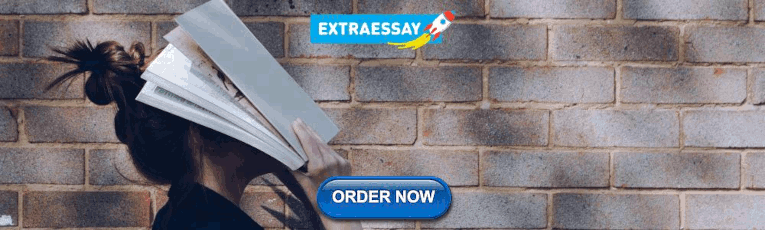
Interpreting a Confidence Level
Chapter 8 - day 1 - lesson 8.1, all chapters, learning targets.
Identify an appropriate point estimator and calculate the value of a point estimate.
Interpret a confidence interval in context.
Determine the point estimate and margin of error from a confidence interval.
Use a confidence interval to make a decision about the value of a parameter.
Activity: Guess the Mystery Mean?

Answer Key:
In this Activity, students will be trying to estimate the mean score for the midterm exam, using a sample of 5 scores. The teacher will need to prepare several samples of 5 scores to distribute to students during the Activity (we just wrote them down on index cards to pass out). We could have had students actually take a sample of 5 scores from the population of all scores, but we didn’t want to reveal all of the scores. Additionally, when we construct confidence intervals in the real world, we only see the data from the sample (not the whole population).
Possible extension: If you have time, you could also prepare samples of size 10. After students are done with the Activity, give them the opportunity to create a new confidence interval using a sample of size 10, rather than a sample of size 5. What happens to their margin of error?
Where are we headed?
Notice the organization of this Chapter, which parallels the structure in Chapter 7 (and will parallel Chapter 9).
Section 8.1 is an introduction to confidence intervals, which includes confidence intervals for a proportion and confidence intervals for a mean.
Section 8.2, we learn how to construct and interpret a confidence interval for a proportion.
Section 8.3, we learn how to construct and interpret a confidence interval for a mean.
The Activity has students thinking about a confidence interval for a mean. The Check Your Understanding problem has students thinking about a confidence interval for a proportion.
Notation matters
Continue to be diligent about using the correct notation. Get students in the habit of thinking about the population, parameter, sample, and statistic for each new context.

Interpreting a confidence interval
We are _____% confident that the interval from _____ to _____ captures the true (parameter with context).
The structure of this interpretation will work for any confidence interval calculated in AP Statistics. Students must become comfortable with producing this interpretation. We feel the word “capture” is most appropriate, and the reason why will become clear in tomorrow’s lesson when we interpret a confidence level.
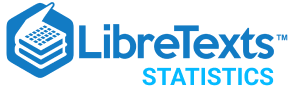
- school Campus Bookshelves
- menu_book Bookshelves
- perm_media Learning Objects
- login Login
- how_to_reg Request Instructor Account
- hub Instructor Commons
- Download Page (PDF)
- Download Full Book (PDF)
- Periodic Table
- Physics Constants
- Scientific Calculator
- Reference & Cite
- Tools expand_more
- Readability
selected template will load here
This action is not available.
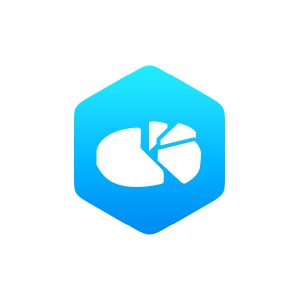
8.1: Introduction to Confidence Intervals
- Last updated
- Save as PDF
- Page ID 14685
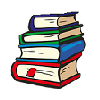
Suppose you were trying to determine the mean rent of a two-bedroom apartment in your town. You might look in the classified section of the newspaper, write down several rents listed, and average them together. You would have obtained a point estimate of the true mean. If you are trying to determine the percentage of times you make a basket when shooting a basketball, you might count the number of shots you make and divide that by the number of shots you attempted. In this case, you would have obtained a point estimate for the true proportion the parameter \(p\) in the binomial probability density function.
We use sample data to make generalizations about an unknown population. This part of statistics is called inferential statistics . The sample data help us to make an estimate of a population parameter . We realize that the point estimate is most likely not the exact value of the population parameter, but close to it. After calculating point estimates, we construct interval estimates, called confidence intervals. What statistics provides us beyond a simple average, or point estimate, is an estimate to which we can attach a measure of accuracy, what we will call a confidence level. We make inferences with a known level of confidence.
In this chapter, you will learn to construct and interpret confidence intervals. You will also learn a new distribution, the Student's- t , and how it is used with these intervals. Throughout the chapter, it is important to keep in mind that the confidence interval is a random variable (until a specifi interval is calculated). It is the population parameter that is fixed.
If you worked in the marketing department of an entertainment company, you might be interested in the mean number of songs a consumer downloads a month from iTunes. If so, you could conduct a survey and calculate the sample mean, \(\overline x\), and the sample standard deviation, \(s\). You would use \(\overline x\) to estimate the population mean and \(s\) to estimate the population standard deviation. The sample mean, \(\overline x\), is the point estimate for the population mean, \(\mu\). The sample standard deviation, \(s\), is the point estimate for the population standard deviation, \(\sigma\).
\(\overline x\) and \(s\) are each called a statistic .
A confidence interval is another type of estimate but, instead of being just one number, it is an interval of numbers. The interval of numbers is a range of values calculated from a given set of sample data. The confidence interval is likely to include the unknown population parameter.
Suppose, for the iTunes example, we do not know the population mean \(\mu\), but we do know that the population standard deviation is \(\sigma = 1\) and our sample size is 100. Then, by the Central Limit Theorem, the standard deviation of the sampling distribution of the sample means is
\[\frac{\sigma}{\sqrt{n}}=\frac{1}{\sqrt{100}}=0.1.\nonumber\]
The Empirical Rule , which applies to the normal distribution, says that in approximately 95% of the samples, the sample mean, \(\overline x\), will be within two standard deviations of the population mean \(\mu\). For our iTunes example, two standard deviations is \((2)(0.1) = 0.2\). The sample mean \(\overline x\) is likely to be within 0.2 units of \(\mu\).
Because \(\overline x\) is within 0.2 units of \(\mu\), which is unknown, then \(\mu\) is likely to be within 0.2 units of \(\overline x\) with 95% probability. The population mean \(\mu\) is contained in an interval whose lower number is calculated by taking the sample mean and subtracting two standard deviations \((2)(0.1)\) and whose upper number is calculated by taking the sample mean and adding two standard deviations. In other words, \(\mu\) is between \(\overline{x}-0.2\) and \(\overline{x}+0.2\) in 95% of all the samples.
For the iTunes example, suppose that a sample produced a sample mean \(\overline{x}=2\). Then with 95% confidence the unknown population mean \(\mu\) is between
\[\overline{x}-0.2=2-0.2=1.8 \text { and } \overline{x}+0.2=2+0.2=2.2 \nonumber\]
We say that we are 95% confident that the unknown population mean number of songs downloaded from iTunes per month is between 1.8 and 2.2. The 95% confidence interval is (1.8, 2.2). Please note that we talked in terms of 95% confidence using the Empirical Rule. The Empirical Rule for two standard deviations is only approximately 95% of the probability under the normal distribution. To be precise, two standard deviations under a normal distribution is actually 95.44% of the probability. To calculate the exact 95% confidence level we would use 1.96 standard deviations.
The 95% confidence interval implies two possibilities. Either the interval (1.8, 2.2) contains the true mean \(\mu\), or our sample produced an \(\overline x\) that is not within 0.2 units of the true mean \(\mu\). The second possibility happens for only 5% of all the samples (100% minus 95% = 5%).
Remember that a confidence interval is created for an unknown population parameter like the population mean, \(\mu\).
For the confidence interval for a mean the formula would be:
\[\mu=\overline{X} \pm z_{\alpha/2} \sigma / \sqrt{n}\nonumber\]
Or written another way as:
\[\overline{X}-z_{\alpha/2} \frac{\sigma}{{\sqrt{n}} \leq \mu \leq \overline{X}+z_{\alpha/2} \frac{\sigma}{\sqrt{n}}\nonumber\]
Where \(\overline X\) is the sample mean. The critical value , \(z_{\alpha/2}\), is determined by the level of confidence desired by the analyst, and \(\sigma / \sqrt{n}\) is the standard deviation of the sampling distribution for means given to us by the Central Limit Theorem.

- school Campus Bookshelves
- menu_book Bookshelves
- perm_media Learning Objects
- login Login
- how_to_reg Request Instructor Account
- hub Instructor Commons
- Download Page (PDF)
- Download Full Book (PDF)
- Periodic Table
- Physics Constants
- Scientific Calculator
- Reference & Cite
- Tools expand_more
- Readability
selected template will load here
This action is not available.
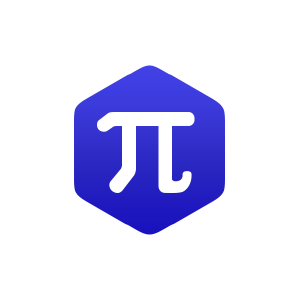
7.1: Process of Estimation - Introduction to Confidence Intervals
- Last updated
- Save as PDF
- Page ID 125710
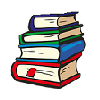
Learning Objectives
By the end of this chapter, the student should be able to:
- Calculate and interpret confidence intervals for estimating a population mean and a population proportion.
- Interpret the Student's t probability distribution as the sample size changes.
- Discriminate between problems applying the normal and the Student's t distributions.
- Calculate the sample size required to estimate a population mean and a population proportion given a desired confidence level and margin of error.
Suppose you were trying to determine the mean rent of a two-bedroom apartment in your town. You might look in the classified section of the newspaper, write down several rents listed, and average them together. You would have obtained a point estimate of the true mean. If you are trying to determine the percentage of times you make a basket when shooting a basketball, you might count the number of shots you make and divide that by the number of shots you attempted. In this case, you would have obtained a point estimate for the true proportion.
We use sample data to make generalizations about an unknown population. This part of statistics is called inferential statistics . The sample data help us to make an estimate of a population parameter. We realize that the point estimate is most likely not the exact value of the population parameter, but close to it. After calculating point estimates, we construct interval estimates, called confidence intervals.
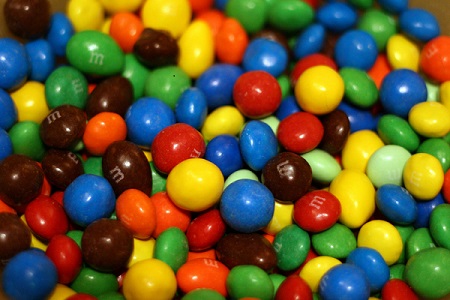
In this chapter, you will learn to construct and interpret confidence intervals. You will also learn a new distribution, the Student's-t, and how it is used with these intervals. Throughout the chapter, it is important to keep in mind that the confidence interval is a random variable. It is the population parameter that is fixed.
If you worked in the marketing department of an entertainment company, you might be interested in the mean number of songs a consumer downloads a month from iTunes. If so, you could conduct a survey and calculate the sample mean, \(\bar{x}\), and the sample standard deviation, \(s\). You would use \(\bar{x}\) to estimate the population mean and \(s\) to estimate the population standard deviation. The sample mean, \(\bar{x}\), is the point estimate for the population mean, \(\mu\). The sample standard deviation, \(s\), is the point estimate for the population standard deviation, \(\sigma\).
Each of \(\bar{x}\) and \(s\) is called a statistic.
A confidence interval is another type of estimate but, instead of being just one number, it is an interval of numbers. The interval of numbers is a range of values calculated from a given set of sample data. The confidence interval is likely to include an unknown population parameter.
Suppose, for the iTunes example, we do not know the population mean \(\mu\), but we do know that the population standard deviation is \(\sigma = 1\) and our sample size is 100. Then, by the central limit theorem, the standard deviation for the sample mean is
\[\dfrac{\sigma}{\sqrt{n}} = \dfrac{1}{\sqrt{100}} = 0.1.\]
The empirical rule, which applies to bell-shaped distributions, says that in approximately 95% of the samples, the sample mean, \(\bar{x}\), will be within two standard deviations of the population mean \(\mu\). For our iTunes example, two standard deviations is (2)(0.1) = 0.2. The sample mean \(\bar{x}\) is likely to be within 0.2 units of \(\mu\).
Because \(\bar{x}\) is within 0.2 units of \(\mu\), which is unknown, then \(\mu\) is likely to be within 0.2 units of \(\bar{x}\) in 95% of the samples. The population mean \(\mu\) is contained in an interval whose lower number is calculated by taking the sample mean and subtracting two standard deviations (2)(0.1) and whose upper number is calculated by taking the sample mean and adding two standard deviations. In other words, \(\mu\) is between \(\bar{x} - 0.2\) and \(\bar{x} + 0.2\) in 95% of all the samples.
For the iTunes example, suppose that a sample produced a sample mean \(\bar{x} = 2\). Then the unknown population mean \(\mu\) is between
\[\bar{x} - 0.2 = 2 - 0.2 = 1.8\]
\[\bar{x} + 0.2 = 2 + 0.2 = 2.2\]
We say that we are 95% confident that the unknown population mean number of songs downloaded from iTunes per month is between 1.8 and 2.2. The 95% confidence interval is (1.8, 2.2). This 95% confidence interval implies two possibilities. Either the interval (1.8, 2.2) contains the true mean \(\mu\) or our sample produced an \(\bar{x}\) that is not within 0.2 units of the true mean \(\mu\). The second possibility happens for only 5% of all the samples (95–100%).
Remember that a confidence interval is created for an unknown population parameter like the population mean, \(\bar{x}\). Confidence intervals for some parameters have the form:
(point estimate – margin of error, point estimate + margin of error)
The margin of error depends on the confidence level or percentage of confidence and the standard error of the mean.
When you read newspapers and journals, some reports will use the phrase "margin of error." Other reports will not use that phrase, but include a confidence interval as the point estimate plus or minus the margin of error. These are two ways of expressing the same concept.
Although the text only covers symmetrical confidence intervals, there are non-symmetrical confidence intervals (for example, a confidence interval for the standard deviation).
Collaborative Exercise
Have your instructor record the number of meals each student in your class eats out in a week. Assume that the standard deviation is known to be three meals. Construct an approximate 95% confidence interval for the true mean number of meals students eat out each week.
- Calculate the sample mean.
- Let \(\sigma = 3\) and \(n\) = the number of students surveyed.
- Construct the interval \(\left(\bar{x} - 2 \cdot \frac{\sigma}{\sqrt{n}}, \bar{x} + 2 \cdot \frac{\sigma}{\sqrt{n}}\right)\).
We say we are approximately 95% confident that the true mean number of meals that students eat out in a week is between __________ and ___________.
- the desired confidence level,
- information that is known about the distribution (for example, known standard deviation),
- the sample and its size.
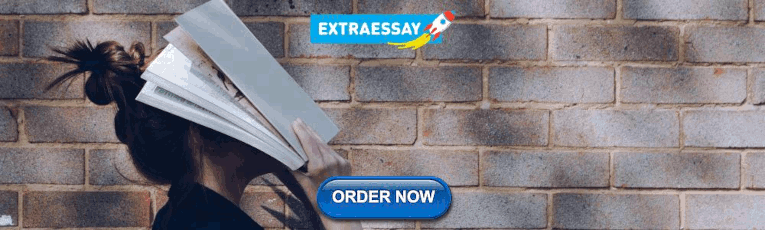
IMAGES
VIDEO
COMMENTS
View 7.1.7 Practice - Creating Intervals.docx from MATH 1 at American Online High School. Statistics Assignment Creating Intervals Page 1 of 2 1. Define confidence interval and confidence level. (2
A statistics Worksheet: The student will calculate the 90% confidence interval for the mean cost of a home in the area in which this school is located. The student will interpret confidence intervals. The student will determine the effects of changing conditions on the confidence interval. 8.6: Confidence Interval -Place of Birth (Worksheet)
Interval data is measured along a numerical scale that has equal distances between adjacent values. These distances are called “intervals.”. There is no true zero on an interval scale, which is what distinguishes it from a ratio scale. On an interval scale, zero is an arbitrary point, not a complete absence of the variable.
8.7: Confidence Interval (Women's Heights) This page titled 9.8: Confidence Intervals (Exercises) is shared under a CC BY license and was authored, remixed, and/or curated by Chau D Tran. These are homework exercises to accompany the Textmap created for "Introductory Statistics" by OpenStax.
Statistical Intervals 7 (One sample) Is called the 95% confidence interval for the mean. This interval varies from sample to sample, as the sample mean varies. So, the interval itself is a random interval. The CI interval is centered at the sample mean X extends 1.96 to each side of X.
The confidence interval X 1:96SE definitely (100%) contains the sample mean X, not just with probability 95%. True or False and explain: 95% of college students have been in 2.7 to 3.7 exclusive relationships. False. The confidence interval is for covering the population mean , not for covering 95% of the entire population.
7. 1. A frequency is the number of times a value of the data occurs. According to Table Table 2.1.1, there are three students who work two hours, five students who work three hours, and so on. The sum of the values in the frequency column, 20, represents the total number of students included in the sample.
In this Activity, students will be trying to estimate the mean score for the midterm exam, using a sample of 5 scores. The teacher will need to prepare several samples of 5 scores to distribute to students during the Activity (we just wrote them down on index cards to pass out). We could have had students actually take a sample of 5 scores from ...
The 95% confidence interval implies two possibilities. Either the interval (1.8, 2.2) contains the true mean μ μ, or our sample produced an x¯¯¯ x ¯ that is not within 0.2 units of the true mean μ μ. The second possibility happens for only 5% of all the samples (100% minus 95% = 5%). Remember that a confidence interval is created for an ...
The interval of numbers is a range of values calculated from a given set of sample data. The confidence interval is likely to include an unknown population parameter. Suppose, for the iTunes example, we do not know the population mean μ μ, but we do know that the population standard deviation is σ = 1 σ = 1 and our sample size is 100.