
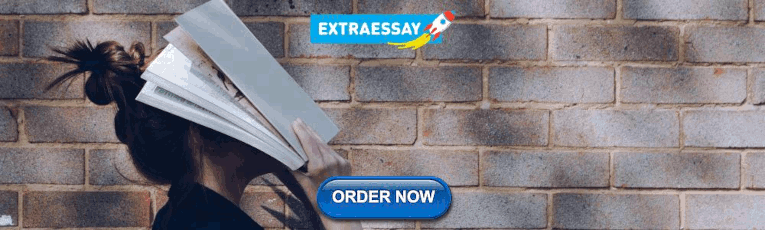
Hypothesis Testing: A Complete Guide for Beginners
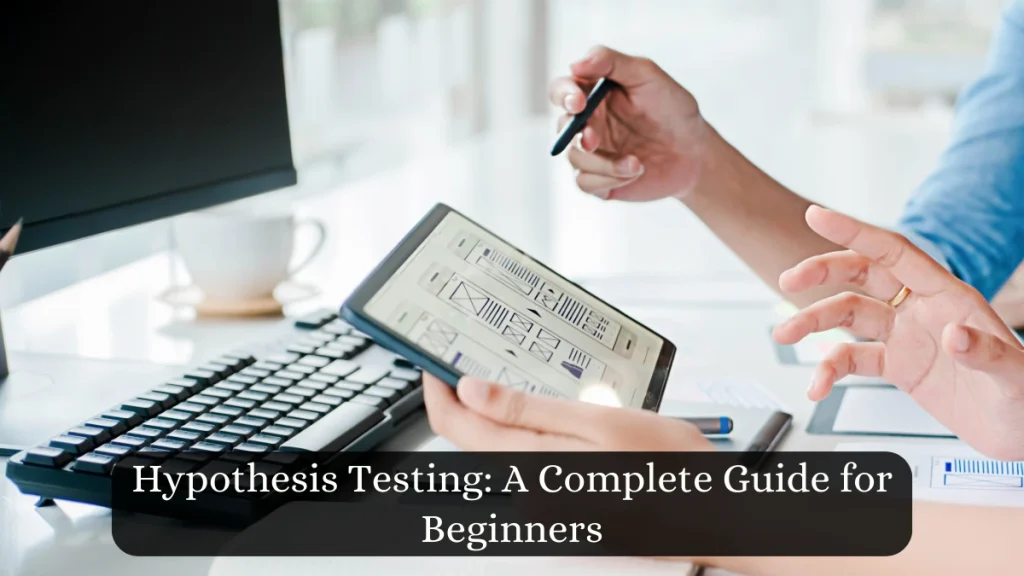
Statistical hypothesis testing is a key concept in statistics. It helps researchers, data analysts, and scientists make decisions based on data. Hypothesis testing allows you to determine whether your results are meaningful when analyzing experiments, surveys, or other data.
In this blog, we’ll explain statistical hypothesis testing from the basics to more advanced ideas, making it easy to understand even for 10th-grade students.
By the end of this blog, you’ll be able to understand hypothesis testing and how it’s used in research.
What is a Hypothesis?
Table of Contents
A hypothesis is a statement that can be tested. It’s like a guess you make after observing something, and you want to see if that guess holds when you collect more data.
For example:
- “Eating more vegetables improves health.”
- “Students who study regularly perform better in exams.”
These statements are testable because we can gather data to check if they are true or false.
What is Hypothesis Testing?
Hypothesis testing is a statistical process that helps us make decisions based on data. Suppose you collect data from an experiment or survey. Hypothesis testing helps you decide whether the results are significant or could have happened by chance.
For example, if you believe a new teaching method helps students score better, hypothesis testing can help you decide if the improvement is real or just a random fluctuation.
Null and Alternative Hypothesis
Hypothesis testing usually involves two competing hypotheses:
- Example: “There is no difference in exam scores between students using the new method and those who don’t.”
- Example: “Students using the new method perform better in exams than those who don’t.”
Key Terms in Hypothesis Testing
Before diving into the details, let’s understand some important terms used in hypothesis testing:
1. Test Statistic
The test statistic is a number calculated from your data that is compared against a known distribution (like the normal distribution) to test the null hypothesis. It tells you how much your sample data differs from what’s expected under the null hypothesis.
The p-value is the probability of observing the sample data or something more extreme, assuming the null hypothesis is true. A smaller p-value suggests that the null hypothesis is less likely to be true. In many studies, a p-value of 0.05 or less is considered statistically significant.
3. Significance Level (α)
The significance level is the threshold at which you decide to reject the null hypothesis. Commonly, this level is set at 5% (α = 0.05), meaning there’s a 5% chance of rejecting the null hypothesis even when it is true.
4. Critical Value
The critical value is the boundary that defines the region where we reject the null hypothesis. It is calculated based on the significance level and tells us how extreme the test statistic needs to be to reject the null hypothesis.
5. Type I and Type II Errors
- Type I Error (False Positive): Rejecting the null hypothesis when it’s true.
- Type II Error (False Negative): Failing to reject the null hypothesis when it’s false.
In simpler terms:
- Type I error is like thinking something has changed when it hasn’t.
- Type II error is like thinking nothing has changed when it actually has.
Types of Hypothesis Testing
1. one-tailed test.
A one-tailed test checks for an effect in a single direction. For example, if you are only interested in testing whether students who study 2 hours daily score higher than those who don’t, that’s a one-tailed test.
2. Two-Tailed Test
A two-tailed test checks for an effect in both directions. This means you’re testing if the scores are different , regardless of whether they are higher or lower. For example, “Do students who study 2 hours daily score differently than those who don’t?” That’s a two-tailed test.
Steps in Hypothesis Testing
Step 1: define hypotheses.
Start by defining the:
- Null Hypothesis (H₀): The status quo or no change.
- Alternative Hypothesis (H₁): The hypothesis you believe in, suggesting that something has changed.
Step 2: Set the Significance Level (α)
Next, set the significance level, typically 0.05 . This means you’re willing to accept a 5% risk of incorrectly rejecting the null hypothesis.
Step 3: Collect and Analyze Data
Conduct your experiment or survey and collect data. Then, analyze this data to calculate the test statistic. The formula you use depends on the type of test you’re conducting (e.g., Z-test, T-test).
Step 4: Calculate the P-value or Critical Value
Compare the test statistic to a standard distribution (such as the normal distribution). If you calculate a p-value , compare it to the significance level. If the p-value is less than the significance level, reject the null hypothesis.
Alternatively, you can compare your test statistic to a critical value from statistical tables to determine if you should reject the null hypothesis.
Step 5: Make a Decision
Based on your calculations:
- If the p-value is less than the significance level (e.g., p < 0.05), reject the null hypothesis.
- If the p-value is greater than the significance level, do not reject the null hypothesis.
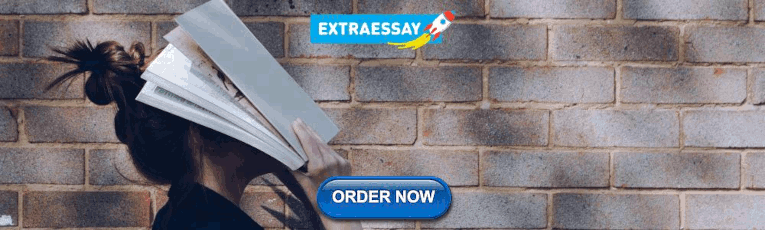
Step 6: Interpret the Results
Finally, interpret the results in context. If you reject the null hypothesis, you have evidence to support the alternative hypothesis. If not, the data does not provide enough evidence to reject the null.
P-Value and Significance
The p-value is a key part of hypothesis testing. It tells us the likelihood of getting results as extreme as the observed data, assuming the null hypothesis is true. In simple terms:
- A low p-value (≤ 0.05) suggests strong evidence against the null hypothesis, so you reject it.
- A high p-value (> 0.05) means the data is consistent with the null hypothesis, and you don’t reject it.
Here’s a table to summarize:
Common Hypothesis Tests
There are different types of hypothesis tests depending on the data and what you are testing for.
Example of Hypothesis Testing
Let’s say a nutritionist claims that a new diet increases the average weight loss for people by 5 kg in a month.
- Null Hypothesis (H₀): The average weight loss is not 5 kg (no difference).
- Alternative Hypothesis (H₁): The average weight loss is greater than 5 kg.
Suppose we collect data from 30 people and find that the average weight loss is 5.5 kg. Now we follow these steps:
- Significance level : Set α = 0.05 (5%).
- Calculate the test statistic: Using the T-test formula.
- Find the p-value : Calculate the p-value for the test statistic.
- Make a decision : Compare the p-value to the significance level.
If the p-value is less than 0.05, we reject the null hypothesis and conclude that the new diet results in more than 5 kg of weight loss.
Statistical hypothesis testing is an essential method in statistics for making informed decisions based on data. By understanding the basics of null and alternative hypotheses, test statistics, p-values, and the steps in hypothesis testing, you can analyze experiments and surveys effectively.
Hypothesis testing is a powerful tool for everything from scientific research to everyday decisions, and mastering it can lead to better data analysis and decision-making.
Also Read: Step-by-step guide to hypothesis testing in statistics
What is the difference between the null hypothesis and the alternative hypothesis?
The null hypothesis (H₀) is the default assumption that there is no effect or no difference. It’s what we try to disprove. The alternative hypothesis (H₁) is what you want to prove. It suggests that there is a significant effect or difference.
What is the difference between a one-tailed test and a two-tailed test?
A one-tailed test looks for evidence of an effect in one direction (either greater or smaller). A two-tailed test checks for evidence of an effect in both directions (whether greater or smaller), making it a more conservative test.
Can we always reject the null hypothesis if the p-value is less than 0.05?
Yes, if the p-value is less than 0.05 , we typically reject the null hypothesis. However, this does not guarantee that the alternative hypothesis is true; it simply indicates that the data provide strong evidence against it.
Related Posts
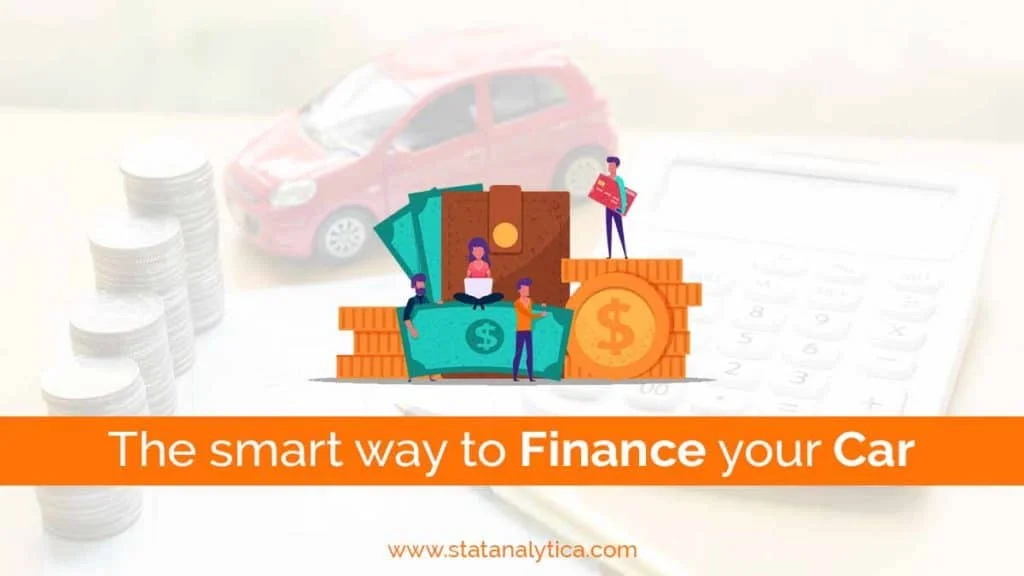
Step by Step Guide on The Best Way to Finance Car
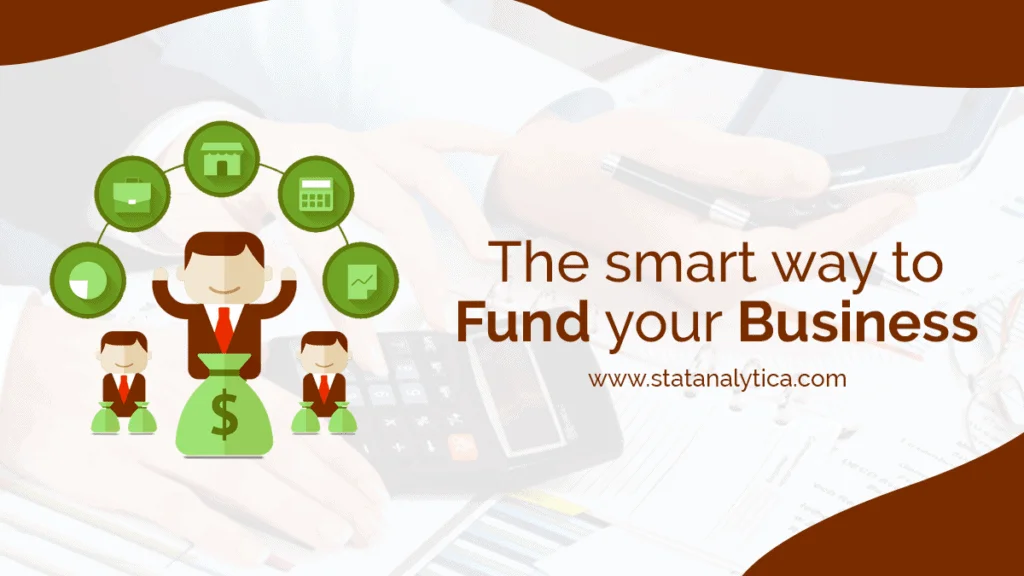
The Best Way on How to Get Fund For Business to Grow it Efficiently
Leave a comment cancel reply.
Your email address will not be published. Required fields are marked *
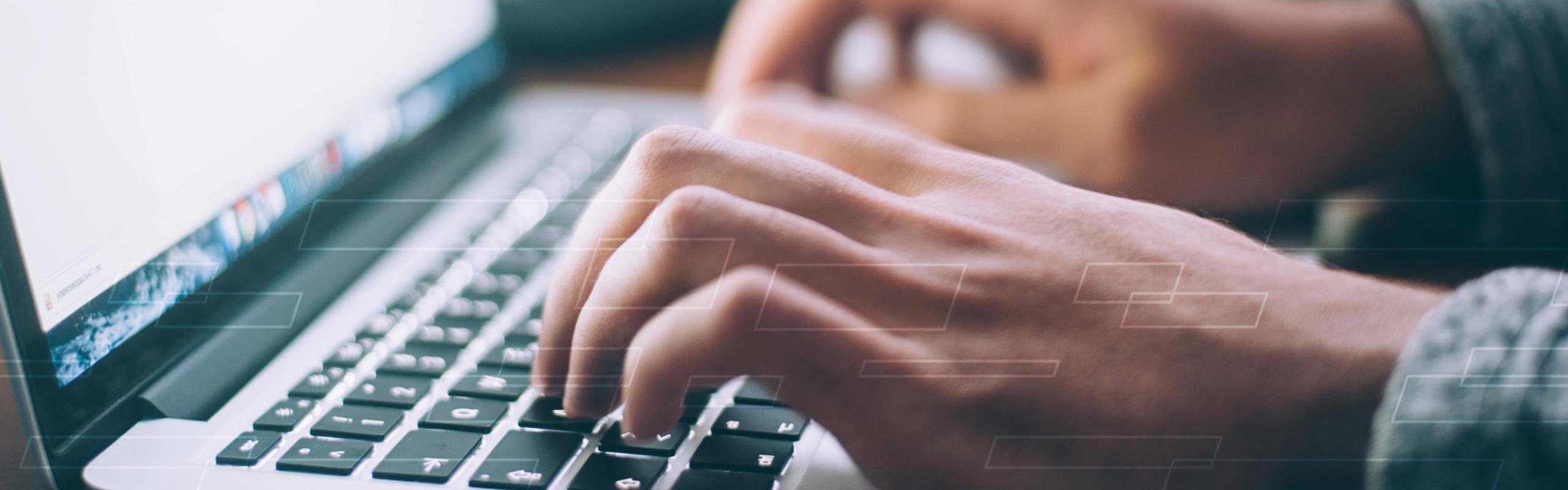
User Preferences
Content preview.
Arcu felis bibendum ut tristique et egestas quis:
- Ut enim ad minim veniam, quis nostrud exercitation ullamco laboris
- Duis aute irure dolor in reprehenderit in voluptate
- Excepteur sint occaecat cupidatat non proident
Keyboard Shortcuts
S.3 hypothesis testing.
In reviewing hypothesis tests, we start first with the general idea. Then, we keep returning to the basic procedures of hypothesis testing, each time adding a little more detail.
The general idea of hypothesis testing involves:
- Making an initial assumption.
- Collecting evidence (data).
- Based on the available evidence (data), deciding whether to reject or not reject the initial assumption.
Every hypothesis test — regardless of the population parameter involved — requires the above three steps.
Example S.3.1
Is normal body temperature really 98.6 degrees f section .
Consider the population of many, many adults. A researcher hypothesized that the average adult body temperature is lower than the often-advertised 98.6 degrees F. That is, the researcher wants an answer to the question: "Is the average adult body temperature 98.6 degrees? Or is it lower?" To answer his research question, the researcher starts by assuming that the average adult body temperature was 98.6 degrees F.
Then, the researcher went out and tried to find evidence that refutes his initial assumption. In doing so, he selects a random sample of 130 adults. The average body temperature of the 130 sampled adults is 98.25 degrees.
Then, the researcher uses the data he collected to make a decision about his initial assumption. It is either likely or unlikely that the researcher would collect the evidence he did given his initial assumption that the average adult body temperature is 98.6 degrees:
- If it is likely , then the researcher does not reject his initial assumption that the average adult body temperature is 98.6 degrees. There is not enough evidence to do otherwise.
- either the researcher's initial assumption is correct and he experienced a very unusual event;
- or the researcher's initial assumption is incorrect.
In statistics, we generally don't make claims that require us to believe that a very unusual event happened. That is, in the practice of statistics, if the evidence (data) we collected is unlikely in light of the initial assumption, then we reject our initial assumption.
Example S.3.2
Criminal trial analogy section .
One place where you can consistently see the general idea of hypothesis testing in action is in criminal trials held in the United States. Our criminal justice system assumes "the defendant is innocent until proven guilty." That is, our initial assumption is that the defendant is innocent.
In the practice of statistics, we make our initial assumption when we state our two competing hypotheses -- the null hypothesis ( H 0 ) and the alternative hypothesis ( H A ). Here, our hypotheses are:
- H 0 : Defendant is not guilty (innocent)
- H A : Defendant is guilty
In statistics, we always assume the null hypothesis is true . That is, the null hypothesis is always our initial assumption.
The prosecution team then collects evidence — such as finger prints, blood spots, hair samples, carpet fibers, shoe prints, ransom notes, and handwriting samples — with the hopes of finding "sufficient evidence" to make the assumption of innocence refutable.
In statistics, the data are the evidence.
The jury then makes a decision based on the available evidence:
- If the jury finds sufficient evidence — beyond a reasonable doubt — to make the assumption of innocence refutable, the jury rejects the null hypothesis and deems the defendant guilty. We behave as if the defendant is guilty.
- If there is insufficient evidence, then the jury does not reject the null hypothesis . We behave as if the defendant is innocent.
In statistics, we always make one of two decisions. We either "reject the null hypothesis" or we "fail to reject the null hypothesis."
Errors in Hypothesis Testing Section
Did you notice the use of the phrase "behave as if" in the previous discussion? We "behave as if" the defendant is guilty; we do not "prove" that the defendant is guilty. And, we "behave as if" the defendant is innocent; we do not "prove" that the defendant is innocent.
This is a very important distinction! We make our decision based on evidence not on 100% guaranteed proof. Again:
- If we reject the null hypothesis, we do not prove that the alternative hypothesis is true.
- If we do not reject the null hypothesis, we do not prove that the null hypothesis is true.
We merely state that there is enough evidence to behave one way or the other. This is always true in statistics! Because of this, whatever the decision, there is always a chance that we made an error .
Let's review the two types of errors that can be made in criminal trials:
Table S.3.2 shows how this corresponds to the two types of errors in hypothesis testing.
Note that, in statistics, we call the two types of errors by two different names -- one is called a "Type I error," and the other is called a "Type II error." Here are the formal definitions of the two types of errors:
There is always a chance of making one of these errors. But, a good scientific study will minimize the chance of doing so!
Making the Decision Section
Recall that it is either likely or unlikely that we would observe the evidence we did given our initial assumption. If it is likely , we do not reject the null hypothesis. If it is unlikely , then we reject the null hypothesis in favor of the alternative hypothesis. Effectively, then, making the decision reduces to determining "likely" or "unlikely."
In statistics, there are two ways to determine whether the evidence is likely or unlikely given the initial assumption:
- We could take the " critical value approach " (favored in many of the older textbooks).
- Or, we could take the " P -value approach " (what is used most often in research, journal articles, and statistical software).
In the next two sections, we review the procedures behind each of these two approaches. To make our review concrete, let's imagine that μ is the average grade point average of all American students who major in mathematics. We first review the critical value approach for conducting each of the following three hypothesis tests about the population mean $\mu$:
In Practice
- We would want to conduct the first hypothesis test if we were interested in concluding that the average grade point average of the group is more than 3.
- We would want to conduct the second hypothesis test if we were interested in concluding that the average grade point average of the group is less than 3.
- And, we would want to conduct the third hypothesis test if we were only interested in concluding that the average grade point average of the group differs from 3 (without caring whether it is more or less than 3).
Upon completing the review of the critical value approach, we review the P -value approach for conducting each of the above three hypothesis tests about the population mean \(\mu\). The procedures that we review here for both approaches easily extend to hypothesis tests about any other population parameter.
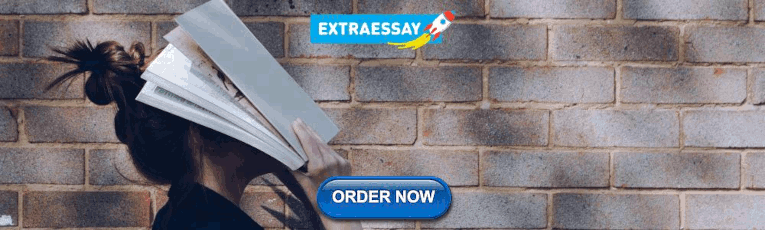
IMAGES
VIDEO
COMMENTS
A hypothesis test is a formal statistical test we use to reject or fail to reject a statistical hypothesis. The Two Types of Statistical Hypotheses. To test whether a statistical hypothesis about a population parameter is true, we obtain a random sample from the population and perform a hypothesis test on the sample data.
Learn how to test your ideas about the world using statistics. Follow the 5 steps of hypothesis testing: state your null and alternate hypothesis, collect data, perform a statistical test, decide whether to reject or fail to reject your null hypothesis, and present your findings.
Hypothesis testing is a method of evaluating the validity of a theory by comparing it to empirical data. It involves formulating null and alternative hypotheses, collecting data, conducting a test, making a decision, and reporting the results.
In hypothesis testing, the goal is to see if there is sufficient statistical evidence to reject a presumed null hypothesis in favor of a conjectured alternative hypothesis. The null hypothesis is usually denoted \(H_0\) while the alternative hypothesis is usually denoted \(H_1\).
Statistical hypothesis testing is an essential method in statistics for making informed decisions based on data. By understanding the basics of null and alternative hypotheses, test statistics, p-values, and the steps in hypothesis testing, you can analyze experiments and surveys effectively.
A statistical hypothesis test is a method of statistical inference used to decide whether the data sufficiently supports a particular hypothesis. A statistical hypothesis test typically involves a calculation of a test statistic.
4. Calculate the test statistic. This is the step of the scientific method (and, thus, also in the process of hypothesis testing) in which data are analyzed. In this step, the statistician uses the inferential test that was chosen in step 2 to analyze the data and yield a result.
Hypothesis testing is a statistical analysis that uses sample data to assess two mutually exclusive theories about the properties of a population. Statisticians call these theories the null hypothesis and the alternative hypothesis.
Learn the general idea and procedures of hypothesis testing in statistics, with examples and analogies. Find out how to make decisions based on evidence, avoid errors, and use critical values or P-values.
Statistical tests are used in hypothesis testing. They can be used to: determine whether a predictor variable has a statistically significant relationship with an outcome variable. estimate the difference between two or more groups. Statistical tests assume a null hypothesis of no relationship or no difference between groups.