Channel Assignment Strategies in Mobile Communication Explained
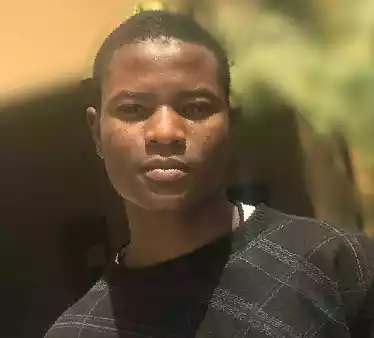
Mohammad Jamiu
Engineering Contents
Read Time 🕠 - 3mins
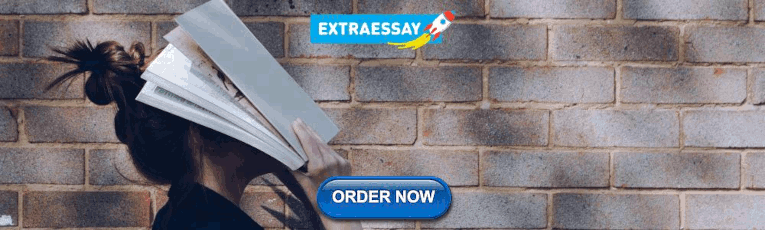
Table of Contents ↬
What are channel assignment strategies.
Channel assignment strategies in mobile communication are used to allocate available radio channels to mobile users in a way that maximizes spectrum utilization and minimizes interference.
This is important because the radio spectrum is a limited resource, and there is a growing demand for mobile communication services.
Types of Channel Assignment Strategies
There are three main types of channel assignment strategies:
Fixed channel assignment (FCA)
Dynamic channel assignment (dca), hybrid channel assignment (hca).
In fixed channel assignment, each cell is allocated a fixed or predetermined set of channels (voice channels).
If all channels in a cell are occupied, the call from a mobile user is blocked and the user won’t receive service.
This strategy is simple to implement, but it can lead to inefficient spectrum utilization and increased interference if the traffic load is not evenly distributed across the cells.
Advantages of FCA:
- Simple to implement and manage
- Reduces co-channel interference
Disadvantages of FCA:
- Can lead to inefficient spectrum utilization
- Can lead to increased call blocking if traffic load is not evenly distributed across the cells
In dynamic channel assignment, channels are assigned to cells on demand, based on the current traffic load. i.e., there is no allocation of predetermined set of channels (voice channels).
This strategy is more efficient than FCA, but it is also more complex to implement.
Advantages of DCA:
- Improves spectrum utilization
- Reduces likelihood of blocking since all available channels are accessible to all cells
Disadvantages of DCA:
- More complex to implement and manage than FCA
- Can lead to increased call blocking if traffic load is high
HCA is a combination of FCA and DCA. In HCA, each cell is allocated a fixed set of channels, but additional channels can be dynamically assigned to cells if needed.
This strategy offers a good balance between simplicity and efficiency.
Advantages of HCA:
- Improves spectrum utilization compared to FCA
- Simpler to implement and manage than DCA
Disadvantages of HCA:
- Can be more complex to implement than FCA
- Can lead to increased co-channel interference compared to DCA
Channel Borrowing
Channel borrowing is a technique that can be used with any channel assignment strategy.
In channel borrowing, a cell can borrow a channel from a neighboring cell if all of its own channels are occupied.
This process is carried out by the Mobile Switching Center (MSC) which supervises the borrowing procedures and ensures that the borrowing of a channel does not interrupt or interfere with any of the calls in progress in the donor cell.
This technique can help to reduce call blocking and improve spectrum utilization.
Advantages of channel borrowing:
- Reduces call blocking
Disadvantages of channel borrowing:
- Can increase co-channel interference
Comparison of Channel Assignment Strategies (FCA, DCA and HCA)
More for you ☄.
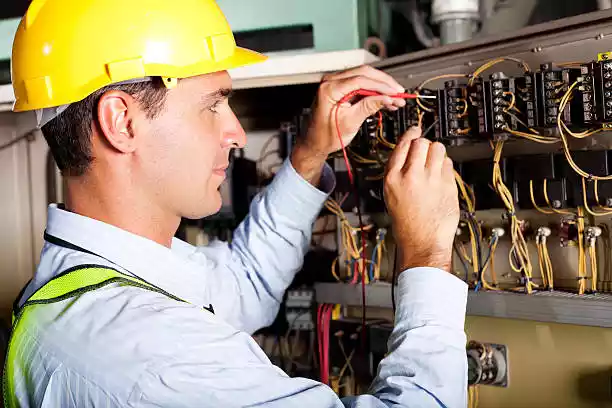

DCA (Dynamic channel allocation)
Dynamic channel allocation (DCA) is a technique used in wireless communication networks to optimize the allocation of radio frequency (RF) channels to different users in real-time. The main goal of DCA is to minimize the interference between users while maximizing the capacity of the wireless network. In this article, we will explain the basics of DCA, its advantages, and its limitations.
Wireless communication networks are becoming increasingly important in our daily lives. They are used for cellular phones, wireless local area networks (WLANs), satellite communication, and many other applications. These networks are based on radio frequency (RF) signals that are transmitted through the air. RF signals are electromagnetic waves that propagate through the air and are subject to attenuation, reflection, and interference.
In wireless communication networks, there are a limited number of RF channels that can be used by multiple users. Each channel has a specific frequency range and bandwidth. When multiple users share the same channel, interference can occur, which can reduce the quality of the communication and decrease the overall capacity of the network. Therefore, the allocation of RF channels to different users is an important task in wireless communication networks.
Static Channel Allocation
In early wireless communication networks, the allocation of RF channels to different users was done using a static channel allocation (SCA) scheme. In SCA, each user is assigned a fixed RF channel, and the channel assignment does not change over time. SCA is simple and easy to implement, but it has several limitations. For example, SCA does not adapt to changes in the traffic load, user mobility, or interference conditions. Therefore, SCA can result in inefficient use of the RF spectrum and reduced network performance.
Dynamic Channel Allocation
To overcome the limitations of SCA, dynamic channel allocation (DCA) schemes were developed. DCA is a technique that dynamically assigns RF channels to users based on real-time conditions in the network. DCA can adapt to changes in the traffic load, user mobility, and interference conditions. DCA can be classified into three categories: centralized DCA, decentralized DCA, and distributed DCA.
Centralized DCA
In centralized DCA, a central entity called the channel allocator (CA) is responsible for allocating RF channels to different users. The CA collects information about the network conditions, such as the traffic load, user mobility, and interference conditions, and uses this information to allocate channels. The CA can also optimize the channel allocation based on various metrics, such as the signal-to-interference ratio (SIR), the bit error rate (BER), or the capacity of the network.
Centralized DCA has several advantages. It can provide a global view of the network and optimize the channel allocation based on the network-wide conditions. It can also be used to enforce various policies, such as fairness, priority, or quality of service (QoS). However, centralized DCA also has some limitations. It requires a high degree of coordination between the CA and the users, which can result in high overhead and delay. It can also be vulnerable to single point failures, such as the failure of the CA.
Decentralized DCA
In decentralized DCA, each user is responsible for selecting its own RF channel based on the local conditions. Each user measures the quality of the RF channels and selects the best available channel. Decentralized DCA can be based on simple algorithms, such as the round-robin or the first-come-first-served (FCFS) algorithm, or more advanced algorithms, such as the interference-aware channel selection (IACS) algorithm or the channel quality-based channel selection (CQCS) algorithm.
Decentralized DCA has several advantages. It is simple and easy to implement. It does not require a central entity, which can reduce overhead and delay. It can also be more robust to single point failures, as each user can operate independently. However, decentralized DCA also has some limitations. It can result in suboptimal channel allocation, as each user selects the channel based only on the local conditions. It can also result in unfair channel allocation, as some users may have better channel conditions than others.
Distributed DCA
In distributed DCA, the channel allocation is done through a distributed negotiation process between the users. Each user broadcasts its channel request to the neighboring users and receives the channel allocation from the best available user. The negotiation process can be based on various algorithms, such as the auction-based algorithm, the game-theoretic algorithm, or the coalition formation algorithm.
Distributed DCA has several advantages. It can provide a flexible and scalable channel allocation scheme, as each user can negotiate with its neighbors independently. It can also result in efficient channel allocation, as the negotiation process can converge to the optimal solution in a distributed manner. However, distributed DCA also has some limitations. It can result in high overhead and delay, as each user needs to broadcast its request to its neighbors. It can also be vulnerable to collusion and malicious attacks, as users may collude to manipulate the negotiation process.
Advantages of DCA
DCA has several advantages over SCA. First, DCA can adapt to changes in the traffic load, user mobility, and interference conditions. This can result in efficient use of the RF spectrum and increased network capacity. Second, DCA can provide various performance guarantees, such as fairness, priority, or QoS. This can improve the user experience and satisfaction. Third, DCA can support various types of wireless communication networks, such as cellular phones, WLANs, and satellite communication. Fourth, DCA can provide a flexible and scalable channel allocation scheme, as it can be implemented using various algorithms and architectures.
Limitations of DCA
DCA also has some limitations. First, DCA can result in suboptimal channel allocation, especially in decentralized and distributed DCA schemes. This can reduce the network capacity and performance. Second, DCA can result in increased overhead and delay, especially in centralized and distributed DCA schemes. This can reduce the network efficiency and responsiveness. Third, DCA can be vulnerable to collusion and malicious attacks, especially in distributed DCA schemes. This can compromise the network security and privacy.
Dynamic channel allocation (DCA) is a technique used in wireless communication networks to optimize the allocation of RF channels to different users in real-time. DCA can adapt to changes in the traffic load, user mobility, and interference conditions. DCA can provide various performance guarantees, such as fairness, priority, or QoS. DCA can also support various types of wireless communication networks, such as cellular phones, WLANs, and satellite communication. However, DCA also has some limitations, such as suboptimal channel allocation, increased overhead and delay, and vulnerability to collusion and malicious attacks. Therefore, the selection of DCA schemes should be based on the specific requirements and constraints of the wireless communication network.
Channel Assignment Schemes
Contributed by John S. Davis, II , U.C. Berkeley
One main issue in cellular system design reduces to one of economics. Essentially we have a limited resource transmission spectrum, that must be shared by several users. Unlike wired communications which benefits from isolation provided by cables, wireless users within close proximity of one another can cause significant interference to one another. To address this issue, the concept of cellular communications was introduced around in 1968 by researchers at AT&T Bell Labs. The basic concept being that a given geography is divided into polygons called cells.
Each cell is allocated a portion of the total frequency spectrum. As users move into a given cell, they are then permitted to utilize the channel allocated to that cell. The virtue of the cellular system is that different cells can use the same channel given that the cells are separated by a minimum distance according to the system propagation characteristics; otherwise, intercellular or cochannel interference occurs. The minimum distance necessary to reduce cochannel interference is called the reuse distance. The reuse distance is defined as the ratio of the distance, D , between cells that can use the same channel without causing interference and the cell radius, R . Note that R is the distance from the center of a cell to the outermost point of the cell in cases when the cells are not circular.
Channel Allocation
- Fixed Channel Allocation,
- Dynamic Channel Allocation and
- Hybrid Channel Allocation which is a combination of the first two methods.
Fixed Channel Allocation
Dynamic channel allocation.
- First, DCA methods typically have a degree of randomness associated with them and this leads to the fact that frequency reuse is often not maximized unlike the case for FCA systems in which cells using the same channel are separated by the minimum reuse distance.
- Secondly, DCA methods often involve complex algorithms for deciding which available channel is most efficient. These algorithms can be very computationally intensive and may require large computing resources in order to be real-time.
Hybrid Channel Allocation Schemes
The third category of channel allocation methods includes all systems that are hybrids of fixed and dynamic channel allocation systems. Several methods have been presented that fall within this category and in addition, a great deal of comparison has been made with corresponding simulations and analyses [Cox, Elnoubi, Jiang, Katzela, Yue, Zhang]. We will present several of the more developed hybrid methods below.
Channel Borrowing is one of the most straightforward hybrid allocation schemes. Here, channels are assigned to cells just as in fixed allocation schemes. If a cell needs a channel in excess of the channels previously assigned to it, that cell may borrow a channel from one of its neighboring cells given that a channel is available and use of this channel won't violate frequency reuse requirements. Note that since every channel has a predetermined relationship with a specific cell, channel borrowing (without the extensions mentioned below) is often categorized as a subclass of fixed allocation schemes. The major problem with channel borrowing is that when a cell borrows a channel from a neighboring cell, other nearby cells are prohibited from using the borrowed channel because of co-channel interference. This can lead to increased call blocking over time. To reduce this call blocking penalty, algorithms are necessary to ensure that the channels are borrowed from the most available neighboring cells; i.e., the neighboring cells with the most unassigned channels.
- The ratio of fixed to dynamic channels varies with traffic load.
- Nominal channels are ordered such that the first nominal channel of a cell has the highest priority of being applied to a call within the cell.
The last nominal channel is most likely to be borrowed by neighboring channels. Once a channel is borrowed, that channel is locked in the co-channel cells within the reuse distance of the cell in question. To be "locked" means that a channel can not be used or borrowed. Zhang and Yum [Zhang] presented the BDCL scheme as an improvement over the BCO method. From a frequency reuse standpoint, in a BCO system, a channel may be borrowed only if it is free in the neighboring cochannel cells. This criteria is often too strict.
- In Borrowing with Directional Channel Locking, borrowed channels are only locked in nearby cells that are affected by the borrowing. This differs from the BCO scheme in which a borrowed channel is locked in every cell within the reuse distance. The benefit of BDCL is that more channels are available in the presence of borrowing and subsequent call blocking is reduced. A disadvantage of BDCL is that the statement "borrowed channels are only locked in nearby cells that are affected by the borrowing" requires a clear understanding of the term "affected." This may require microscopic analysis of the area in which the cellular system will be located. Ideally, a system can be general enough that detailed analysis of specific propagation measurements is not necessary for implementation.
A natural extension of channel borrowing is to set aside a portion of the channels in a system as dynamic channels with the remaining (nominal) channels being fixed to specified cells. If a cell requires an extra channel, instead of borrowing the channel from a neighboring cell, the channel is borrowed from the common "bank" of dynamic channels. An important consideration in hybrid systems of this type is the ratio of dynamic channels to fixed channels. Analysis by Cox and Reudlink [Cox - 1973] showed that given ten channels per cell, an optimum ratio was 8 fixed channels and 2 dynamic channels. In general, the optimum ratio depends upon the traffic load [Zhang]. In addition to BDCL, a second channel allocation method was presented by Yum and Zhang [Zhang]. Referred to as Locally Optimized Dynamic Assignment Strategy (LODA), this method is best described as a purely dynamic channel allocation procedure as opposed to a hybrid method. In this strategy there are no nominal channels; all channels are dynamic. When a given cell needs to accommodate a call, it chooses from among the bank of available channels according to some cost criteria. The channel with minimum cost is assigned. In a general sense, the cost is a measure of the future blocking probability in the vicinity of the cell given that the candidate channel is assigned. A more detailed description of the cost function will be addressed below.
Dynamic Channel Reassignment
Similar to the goals of dynamic channel assignment is the process of Dynamic Channel Reassignment (DCR). Whereas a DCA scheme allocates a channel to an initial call or handover , a DCR system switches a cell's channel (that is currently being used) to another channel which is closer to the optimum according to frequency reuse or other cost criteria. Thus, for example, a user communicating with channel n may be switched to channel m during the middle of her/his call if channel m is a more efficient use of the available bandwidth from a frequency reuse point of view. Philosophically, DCR is equivalent to DCA.
Simulation and Comparison of Channel Allocation Schemes
A great deal of work is available comparing various realizations of channel allocation schemes [Cox, Elnoubi, Jiang, Katzela, Yue, Zhang]. In comparing performance, typical system metrics include blocking probability of new calls and blocking probability of handover calls. These metrics are written as functions of offered traffic (where the traffic may be written in a variety of forms). It is generally assumed that a blocked new call is preferred over a blocked hand-off call. The idea being that with a blocked hand-off, users are forced to terminate communication in the middle of their session. If this blocking happens at a particularly inopportune time, the results could be disastrous (e.g., business partners cut off in the middle of a vital negotiation). In the case of a blocked new call, at least the business negotiation hasn't started and the involved parties aren't interrupted. Blocking probability is an important metric throughout the field of queueing theory and in the case of M/M/ 1 queues, the Erlang-B formula is often used for analysis of blocking probability. Because blocked calls can be very disconcerting, systems are typically designed to have blocking probabilities of no more than 1% or 2%. This is consistent with the assumption of small offered traffic loads.
Cox and Reudink were the first researchers to present published comparisons of different channel allocation schemes. Their comparison was based on simulation of an outdoor vehicular wireless communication system [Cox - 1971, Cox - 1972, Jakes]. The simulation divided a region into a grid of square cells. The movement of vehicles had a two dimensional normal distribution with 0 mean and 30 mph standard deviation in each of the two orthogonal directions. Poisson arrivals were assumed for the rate of calls per vehicle and call durations were assume to have a truncated normal distribution (truncated on the left at zero) with a "mean" 90 seconds (true mean of 103.5 seconds).
Cox and Reudink's study considered uniform and non-uniform distributions of spatial traffic. In the uniform case, all cells had approximately the same call arrival rate while in the non-uniform case, some cells had a significantly higher call arrival rate. With both the uniform and non-uniform spatial distributions, fixed channel allocation schemes were optimally matched so that the cells with the greatest numbers of calls had the greatest number of channels to deal with those calls. In both cases of uniform and non-uniform traffic, results showed that for low blocking probabilities, dynamic channel allocation schemes could handle more calls than fixed channel allocation schemes. More specifically, in the case of uniform traffic, the DCA approach outperformed the FCA approach when the blocking probability was lower than 10%. At a blocking probability of 1%, the DCA approach could handle over 10% more calls than the FCA approach. In the case of non-uniform traffic, the DCA approach outperformed FCA for blocking rates up to 60%. At a blocking rate of 1%, DCA could handle almost 70% more calls per cell than FCA. Cox and Reudink performed another comparison involving dynamic channel reassignment in [Cox - 1973]. In this hybrid procedure, the total number of available channels is broken into two groups: fixed and dynamic channels. When a cell requires a channel, it first searches for an available fixed channel that is preassigned to the cell. If none of the fixed channels are available, a dynamic channel is searched for from the common bank of dynamic channels. If this search is in vain, the call is blocked. When users who were assigned fixed channels end their calls, these freed fixed channels are then assigned to users in the same cell who are currently using dynamic channels. This frees the dynamic channel for future use and ensures that a large number of channels being used are the optimally-spaced, fixed channels. Results from Cox and Reudink's study of dynamic channel reassignment showed that channel use was increased by over 60% compared to fixed channel allocation for a blocking rate of 1%. This result corresponds to uniform offered traffic.
- Fixed Channel Assignment (FCA),
- Borrowing with Channel Ordering (BCO),
- Borrowing with Directional Channel Locking (BDCL) and
- Locally Optimized Dynamic Assignment (LODA).
With respect to uniform offered traffic, their results showed that BDCL had the lowest blocking probability followed by BCO, LODA and FCA. With non-uniform offered traffic, the relative performance of the four methods was the same with the exception that in this case, LODA performed better than BCO. It makes sense that the ordering for BDCL, BCO and FCA was as found. Indeed, BDCL was specifically designed as an improvement over BCO and BCO was designed as an improvement over FCA [Zhang, Elnoubi]. The fact that the performance of LODA varies under uniform versus non-uniform traffic is rather interesting however. The reason behind this phenomenon is that LODA provides optimal channel allocation only in local regions. Given non-uniform traffic which consists of dense regions in certain local areas, LODA will accommodate these regions of high traffic offering. However, in a global sense, the LODA algorithm will not necessarily provide the optimal allocation. With uniform offered traffic, LODA does not have any regions with peak traffic to optimize; i.e., no local regions within which the benefits of LODA can be realized. Furthermore, with respect to the entire region, the optimization is generally not optimal in a global sense. The result is that with uniform traffic, LODA does not have any advantage to offer over BCO. From the previous discussion we see that one general result of all of the comparisons is that dynamic channel allocation outperforms fixed channel allocation for low blocking rates (below 10% in most cases). Blocking rates above 1% or 2% are generally not tolerated. This is generally an accepted guideline throughout the telecommunications industry and we will adhere to this design constraint as well.
Common Principles of Channel Allocation Schemes
- Channel allocation schemes must not violate minimum frequency reuse conditions.
- Channel allocation schemes should adapt to changing traffic conditions.
- Channel allocation schemes should approach (from above) the minimum frequency reuse constraints so as to efficiently utilize available transmission resources.
As the first requirement suggests, all channel allocation schemes adhere to condition 1. From a frequency reuse standpoint, a fixed channel allocation system distributes frequency (or other transmission) resources to the cells in an optimum manner; i.e., common channels are separated by the minimum frequency reuse distance. Thus, a fixed channel allocation scheme perfectly satisfies condition 3 as well. However, a fixed allocation scheme does not satisfy condition 2.
Philosophically, any dynamic channel allocation scheme will meet the requirements of all of the above three conditions to some degree. At the system architecture level dynamic channel allocation schemes may differ widely, but fundamentally, their only difference is in the degree to which they satisfy condition 3. Different DCA schemes attempt to satisfy condition 3 (in addition to conditions 1 and 2) by approaching the minimum frequency reuse constraint arbitrarily closely, and by doing so in as short a time period as possible. The above three conditions point to the fact that design of dynamic channel allocation schemes falls within the general class of optimization problems. Furthermore, since we can always assume that the available number of base stations is finite and the transmission resources will always be countable (due to FCC requirements if nothing else) then our problem can be reduced to the subclass of combinatorial optimization problems. As with all combinatorial optimization problems, there will exist a solution space and a cost function [Aarts & Korst]. A typical element of the solution space could be a particular layout of frequency channels among the base-stations. The cost function can be loosely characterized as the difference between the frequency reuse of an arbitrary solution and the frequency reuse of the optimized solution. The error associated with a non-optimized cost is realized as a future increased blocking probability or an otherwise unwarranted lack of channel availability. It is typically assumed that the solution to the wireless dynamic channel allocation problem is NP-complete [Yue, Cox - 1971]. The definition of np-completeness follows from the conjecture made in the late 1960's that there exists a class of combinatorial optimization problems of such inherent complexity that any algorithm, solving each instance of such a problem to optimality, requires a computational effort that grows superpolynomially with the size of the problem. In the case of dynamic channel allocation, the complexity is generally attributed to the required inclusion of cochannel interference in any analysis of dynamic channel allocation schemes [Yue]. The author is aware of one published article to date offering an analytical method (approximate) for calculating the performance of dynamic channel allocation [see Yue]. Recently, several approximation techniques have been proposed as methods for solving condition 3 of the dynamic channel allocation problem. In particular there has been interest in applying simulated annealing techniques [Duque-Anton] and neural network methods [Chan, Kunz, Funabiki] to dynamic channel allocation.
Channel and Base-Station Allocation Schemes
- [1] Chan, P. T. H., Palaniswami, M. & Everitt, D., "Neural Network-Based Dynamic Channel Assignment for Cellular Mobile Communication Systems," IEEE Transactions on Vehicular Technology, vol. 43, no. 2, May 1994.
- [2] Cox, D.C. and Reudink, D.O., "Dynamic Channel Assignment in High-Capacity Mobile Communications Systems," Bell System Technical Journal, vol. 50, no. 6, July-August 1971.
- [3] Cox, D.C. and Reudink, D.O., "Dynamic Channel Assignment in Two-Dimensional Large -Scale Mobile Radio Systems," Bell System Technical Journal, vol. 51, no. 7, September 1972.
- [4] Cox, D.C. and Reudink, D.O., "Increasing Channel Occupancy in Large-Scale Mobile Radio Systems: Dynamic Channel Reassignment," IEEE Transactions on Communications, vol. 21, no. 11, November 1973.
- [5] Duque-Anton, M., Kunz, D., & Ruber, B., "Channel Assignment for Cellular Radio Using Simulated Annealing," IEEE Transactions on Vehicular Technology, vol. 42, no. 1, February 1993.
- [6] Elnoubi, S. M., Singh, R., and Gupta, S.C., "A New Frequency Channel Assignment Algorithm in High Capacity Mobile Communication Systems," IEEE Transactions on Vehicular Technology, vol. 31, no. 3, August 1982.
- [7] Funabiki N. & Takefuji, Y., "A Neural Network Parallel Algorithm for Channel Assignment Problems in Cellular Radio Networks," IEEE Transactions on Vehicular Technology, vol. 41, no. 4, November 1992.
- [8] Gamst, A., "Homogeneous Distribution of Frequencies in a Regular Hexagonal Cell System," IEEE Transactions on Vehicular Technology, vol. 31, no. 3, August 1982.
- [9] Jakes, W. C., Micr wave Mobile Communication ns, IEEE Press: New Jersey, c. 1993.
- [10] Jiang, H. and Rappaport, S. S., "CBWL: A New Channel Assignment and Sharihng Method for Cellular Communication Systems," IEEE Transactions on Vehicular Technology, vol. 43., no. 2, May 1994.
- [11] Katzela, I., "Channel Assignment Schemes for Cellular Mobile Telecommunication Systems," Unpublished work, Columbia University, December 15, 1992.
- [12] Kunz, D., "Channel Assignment for Cellular Radio Using Neural Networks," IEEE Transactions on Vehicular Technology, vol. 40, no. 1, February 1991.
- [13] Yue, W., "Analytical Methods to Calculate the Performance of a Cellular Mobile Radio Communication with Hybrid Channel Assignment," IEEE Transactions on Vehicular Technology, vol. 40, now. 2, May 1991.
- [14] Zhang, M. and Yum, TS. P., "Comparisons of Channel-Assignment Strategies in Cellular Mobile Telephone Systems", IEEE Transactions on Vehicular Technology, vol. 38, no. 4, November 1989.
Combinatorial Optimization
- [15] Aarts, E. and Korst, J., Simulated Annealing and Boltzmann Machines: A Stochastic Approach to Combinatorial Optimization and Neural Computing, John Wiley & Sons: Chichester, c. 1989.
- [16] Garey, M. R. & Johnson, D. S., Computers and Intractability: A Guide to the Theory of NP-Completeness, W. H. Freeman and Company: New York, c. 1979.
Try you luck as a frequency assigner in the Dynamic Channel Allocation game .
JPL's Wireless Communication Reference Website � John S. Davis, II and 1993, 1995.
- Engineering Mathematics
- Discrete Mathematics
- Operating System
- Computer Networks
- Digital Logic and Design
- C Programming
- Data Structures
- Theory of Computation
- Compiler Design
- Computer Org and Architecture
- Difference Between Go-Back-N and Selective Repeat Protocol
- Difference between Ring Topology and Tree Topology
- Difference between Hub and Bridge
- Difference between Flow Control and Error Control
- Difference between Encryption and Decryption
- Difference Between Modem and Router
- Difference between Switch and Bridge
- Difference between Ethernet and LAN
- Difference between 1-persistent, p-persistent and Non-persistent CSMA
- Difference between Steganography and Cryptography
- Difference between Star topology and Bus topology
- Difference between Hub and Switch
- Difference between Mesh Topology and Bus Topology
- Difference between Adaptive and Non-Adaptive Routing algorithms
- Difference between SMTP and POP3
- Difference between ARP and RARP
- Difference between DNS and DHCP
- Difference between FTP and TFTP
- Difference between Flow Control and Congestion Control
Difference between Fixed and Dynamic Channel Allocations
Prerequisite – Channel Allocation Problem in Computer Network Fixed Channel Allocation (FCA): Fixed Channel Allocation is a strategy in which fixed number of channels or voice channels are allocated to the cells. Once the channels are allocated to the specific cells then they cannot be changed. In FCA channels are allocated in a manner that maximize Frequency reuse. If all channels are occupied and user make a call then the call is blocked. Borrowing Channels handles this type of problem. In this cell borrow channels from other cells. Dynamic Channel Allocation (DCA): Dynamic Channel Allocation is a strategy in which channels are not permanently allocated to the cells. When a User makes a call request then Base Station(BS) send that request to the Mobile Station Center(MSC) for the allocation of channels or voice channels. This way the likelihood of blocking calls is reduced. As traffic increases more channels are assigned and vice-versa. Difference between Fixed Channel Allocation(FCA) and Dynamic Channel Allocation(DCA):
Please Login to comment...
Similar reads.
- Difference Between
- Otter.ai vs. Fireflies.ai: Which AI Transcribes Meetings More Accurately?
- Google Chrome Will Soon Let You Talk to Gemini In The Address Bar
- AI Interior Designer vs. Virtual Home Decorator: Which AI Can Transform Your Home Into a Pinterest Dream Faster?
- Top 10 Free Webclipper on Chrome Browser in 2024
- 30 OOPs Interview Questions and Answers (2024)
Improve your Coding Skills with Practice
What kind of Experience do you want to share?
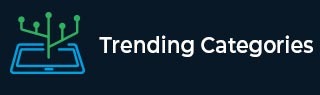
- Selected Reading
- UPSC IAS Exams Notes
- Developer's Best Practices
- Questions and Answers
- Effective Resume Writing
- HR Interview Questions
- Computer Glossary
Dynamic Channel Allocation in computer network
When there are more than one user who desire to access a shared network channel, an algorithm is deployed for channel allocation among the competing users. Dynamic channel allocation encompasses the channel allocation schemes where channels are allotted to users dynamically as per their requirements, from a central pool.
Working Principle
In dynamic channel allocation schemes, frequency channels are not permanently allotted to any user. Channels are assigned to the user as needed depending upon the network environment. The available channels are kept in a queue or a spool. The allocation of the channels is temporary. Distribution of the channels to the contending users is based upon distribution of the users in the network and offered traffic load. The allocation is done so that transmission interference is minimized.
Dynamic Channel Allocation Schemes
The dynamic channel allocation schemes can be divided into three categories −
- Interference Adaptive Dynamic Channel Allocation (IA-DCA)
- Location Adaptive Dynamic Channel Allocation (LA-DCA)
- Traffic Adaptive Dynamic Channel Allocation (TA-DCA)
All these schemes evaluate the cost of using each available channel and allocates the channel with the optimum cost.
Dynamic channel allocation schemes allots channels as needed. This results in optimum utilization of network resources. There are less chances of denial of services and call blocking in case of voice transmission. These schemes adjust bandwidth allotment according to traffic volume, and so are particularly suitable for bursty traffic.
Disadvantages
Dynamic channel allocation schemes increases the computational as well as storage load on the system.

I love programming (: That's all I know
Related Articles
- Static Channel Allocation in computer network
- What is channel allocation in computer network?
- What is a Dynamic channel allocation in computer networks?
- Assumptions for Dynamic Channel Allocation
- Multiplexing (Channel Sharing) In Computer Network
- What is a static channel allocation in computer networks?
- Network Allocation Vector (NAV)
- What is Dynamic Memory Allocation in C?
- Difference between Fixed Channel Allocations and Dynamic Channel Allocations.
- How to restrict dynamic allocation of objects in C++?
- Example program on Dynamic memory allocation in C language
- Explain dynamic memory allocation in C with an example
- Basic Network Attacks in Computer Network
- Example program on Dynamic memory allocation function in C language
- Flooding in Computer Network
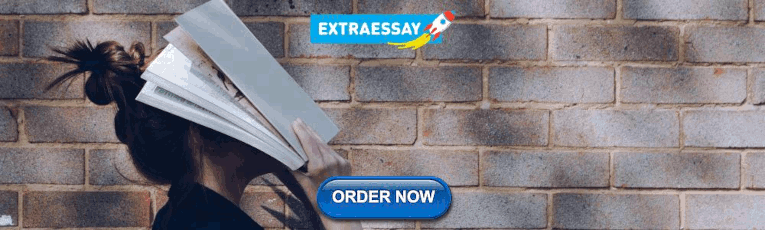
Kickstart Your Career
Get certified by completing the course
Dynamic channel assignment in clustered multihop CDMA/TDMA ad hoc networks
Ieee account.
- Change Username/Password
- Update Address
Purchase Details
- Payment Options
- Order History
- View Purchased Documents
Profile Information
- Communications Preferences
- Profession and Education
- Technical Interests
- US & Canada: +1 800 678 4333
- Worldwide: +1 732 981 0060
- Contact & Support
- About IEEE Xplore
- Accessibility
- Terms of Use
- Nondiscrimination Policy
- Privacy & Opting Out of Cookies
A not-for-profit organization, IEEE is the world's largest technical professional organization dedicated to advancing technology for the benefit of humanity. © Copyright 2024 IEEE - All rights reserved. Use of this web site signifies your agreement to the terms and conditions.

An official website of the United States government
The .gov means it’s official. Federal government websites often end in .gov or .mil. Before sharing sensitive information, make sure you’re on a federal government site.
The site is secure. The https:// ensures that you are connecting to the official website and that any information you provide is encrypted and transmitted securely.
- Publications
- Account settings
Preview improvements coming to the PMC website in October 2024. Learn More or Try it out now .
- Advanced Search
- Journal List
- Scientific Reports

Efficient dynamic channel assignment through laser chaos: a multiuser parallel processing learning algorithm
Zengjing chen.
Zhongtai Securities Institute for Financial Studies, Shandong University, Jinan, 250100 China
Chengzhi Xing
Associated data.
The datasets generated during this study are available from the corresponding author upon reasonable request.
As laser chaos has been proven to be a robust tool to solve the multi-armed bandit (MAB) problem, this study investigates the problem of multiuser dynamic channel assignment using laser chaos in cognitive radio networks with K -orthogonal channels and M secondary users. A novel dynamic channel assignment algorithm with laser chaos series for multiple users, named parallel processing learning with laser chaos (PPL-LC) algorithm, is proposed to efficiently address two main objectives: stable channel assignment and fuzzy stable channel assignment. The latter objective accounts for the realistic scenario where users have fuzzy preferences and do not necessarily pursue the best preference. The PPL-LC algorithm uses the randomness properties of laser chaos to learn the assignment of channels to multiple users without any limitations on the number of channels, which has not been considered in existing laser chaos algorithms. Moreover, the PPL-LC is equipped with parallel processing channel selections, resulting in higher throughput and stronger adaptability with environmental changes over time than comparison algorithms, such as distributed stable strategy learning and coordinated stable marriage MAB algorithms. Finally, numerical examples are presented to demonstrate the performance of the PPL-LC algorithm.
Introduction
Since cognitive radio networks (CRNs) were proposed 1 , multiuser dynamic channel assignment has been a significant focus. Advances in terminology suggest improvements in the performance of CRNs based on resource utilization and other factors. As primary users have priority over secondary users in spectrum utilization, secondary users should be aware of primary users’ behaviors. For efficient resource utilization, corresponding issues, such as which channel primary users choose and what time to sense, should be considered by secondary users. Existing spectrum allocation and sharing approaches are discussed in detail 2 . Zhao and Sadler 3 reviewed various models with dynamic spectrum access. Using the Gale–Shapley stable matching algorithm, the stable marriage problem of multiuser dynamic channel assignment was first solved under the assumption that the expected rates are known and a distributed opportunistic CSMA protocol that solves the problem was proposed 4 . This approach has been shown to have a range of advantages and has received significant attention recently.
The combination of the multi-armed bandit (MAB) problem and cognitive radio access was initially studied with independent channels 5 . Channel selection is considered as a type of MAB problem; that is, the amount of information passed through a channel is considered as the gain obtained by a robot arm. Lai, Jiang, and Poor 6 discussed the solution to the cognitive media access problem and considered various scenarios using the upper confidence bound (UCB) algorithm to explore the optimal choice of numerous users and channels in this problem. Further, they proved that the UCB algorithm achieves an expected sum of regrets of near- O ( log T ) . Avner and Mannor 7 proposed the concept of stable marriage configuration (SMC) and proposed the coordinated stable marriage MAB (CSM-MAB) algorithm to reach the SMC state. The CSM-MAB algorithm requires communication and exchange of channels between different users, but it does not give the approximate order achieved by the regret. Bistritz and Leshem 8 proposed The game of thrones (GoT) algorithm, which does not require user communication, and proved that it achieves an expected sum of regrets of near- O ( log 2 T ) . Gafni and Cohen 9 introduced a fully distributed algorithm—distributed stable strategy learning (DSSL)—to solve a multiuser channel assignment problem and achieved a stable state through a large number of exchanges between users with the upper boundary of the regret given in the theoretical proof.
Several recent observations on laser chaos motivate its application to dynamic channel assignment 10 . In physics, chaos has always been closely related to randomness and is extremely sensitive to the initial parameter values. The chaotic oscillation of a laser signal level is generated by the injection of delayed light into the cavity of a diode laser emitter, which is accomplished by directing the output of the semiconductor laser through a beam splitter toward a laser reflector. The resulting chaotic oscillation of the laser signal level can be harnessed for various applications, such as secure communication, chaos-based sensing, and chaos-based control. Based on this, random numbers are obtained by the fast sampling of the signal level 11 . Random numbers generated by laser chaos are generally in the GHz or higher level, indicating the high quality of these random numbers. They seem natural and have passed the National Institute of Standards and Technology (NIST) random number test. Considering these advantages, research has been conducted on potential applications of laser chaos. Naruse et al. 12 proposed a MAB algorithm using chaotically oscillating waveforms. Subsequently, the algorithm was further improved to consider the order correct probability of MAB 13 . The major work inspiring this study is the use of a laser chaos algorithm to solve the problem of dynamic channel selection for a single user 14 .
Although laser chaos has been proven to be effective in the aforementioned literature, there still exists some engaging questions that need to be addressed. One of these questions is whether laser chaos can perform well in CRNs with multiple users. In other words, it is worth investigating whether it is possible to achieve higher throughput by laser chaos to attain a suitable stable configuration. Focusing on stable configuration, it should be noted that the preferences of different users in the real world are not consistently precise, which prompts contemplation on how to dynamically select channels in CRNs based on the fuzzy preferences of users. Therefore, this study investigates dynamic channel selection in CRNs using laser chaos series based on a proper stable aim.
Based on the above starting points, a novel dynamic channel assignment algorithm with laser chaos series for multiple users, named parallel processing learning with laser chaos (PPL-LC) algorithm, is proposed to enhance throughput and improve operational efficiency. To increase the verisimilitude of the proposed model, consider a CRN comprising multiple users and multiple channels. The probability of a given channel may differ among the various users, adding a layer of complexity and realism to the model. The stable channel assignment (SCA) has aroused widespread interest. As a result, a new definition for the stable arrangement of channels based on users’ fuzzy preferences, called fuzzy stable channel assignment (FSCA), has been proposed. This described the reality of situations where users have their recognition interval 15 , 16 , and all channels in this interval are equally possible to be chosen. Therefore, the channel selection problem is studied to achieve SCA and FSCA separately. The PPL-LC algorithm includes two stages with the users’ channel preference updating process, and the parallel channel exchange process. As MAB problems require a balance between exploration and exploitation, laser chaos provides an efficient tool for sufficient exploration. Based on this, in the process of updating users’ preferences, the PPL-LC algorithm applies laser chaos to achieve equilibrium for multiple users. During the channel exchange process, a parallel method that selected users simultaneously perform the procedure is employed.
In the following, detailed comparisons with related results are provided. First, the previous work only proposed a stable state similar to SMC, whereas this paper considers FSCA state. The performance of the proposed algorithm is evaluated by targeting these two states. Based on the experiment, the PPL-LC algorithm has a higher throughput than the CSM-MAB and DSSL algorithms. Second, the restriction of existing laser chaos algorithms based on channels (arms) that are integer powers of 2 is eliminated and the algorithm is generalized to support an arbitrary number of channels. Third, to the best of our knowledge, this is the first time laser chaos is applied to dynamic channel selection with multiple users. In addition, to attain higher throughput and perform more processes, this work introduces a parallel channel exchange processing to reach two target stable states. The principles of this processing approach are presented in detail below.
Characteristics of CRNs
This paper considers the following scenario to investigate the CRN problem. In an information communication network system with K channels and N users, it is assumed that each user can only use one channel and that each channel allows only a single user to transmit information. If two or more users occupy the same channel simultaneously, the information passed through the channel becomes null. Users can communicate with each other to exchange channels. Without loss of generality, the assumption K ≤ N is taken in this study. Otherwise, the number of users transmitting information concurrently can be restricted through the use of split time to satisfy the condition K ≤ N .
When user n selects the channel k for information transmission, two random outcomes may occur: complete transmission or rejection. Complete transmission signifies that all information passes through channel k , whereas rejection denotes the state in which no information passes through channel k . For convenience, the symbols used in this paper are defined as follows.
- U = { 1 , 2 , … , N } indicates the set of users and C = { 1 , 2 , … , K } is the set of channels.
- X n , k indicates the binary random variable of whether user n can completely transmit 1 bit of information using channel k , which is consistent regardless of time step.
- μ n , k indicates the expectation of X n , k , i.e., E [ X n , k ] .
- π n , t indicates the channel assignment of the user n at time t .
- T t n , k indicates the number of times the user n selects the channel k before time t .
- R t n , k indicates the total benefit of the user n choosing channel k before time t .
- μ ^ t n , k indicates the average benefit of the user n choosing channel k before time t , i.e, μ ^ t n , k = R t n , k T t n , k .
As mentioned in Introduction, the existing laser chaos algorithms usually assume that the number of arms (channels) satisfies the condition K = 2 M for M ∈ N ∗ . However, real situations rarely satisfy this assumption. To be coincident with reality, this study removes this restriction such that K can take any number. For any number of channels K > 1 , a positive integer M can be found such that 2 M < K ≤ 2 M + 1 . A sequence of binary codes { S 1 S 2 … S M + 1 } can be used to represent each channel k ∈ C with S i ∈ { 0 , 1 } for i = 1 , … , M + 1 . The laser chaos algorithm uses 2 M + 1 as the number of channels and sets μ n , k a to zero for all k a among the last 2 M + 1 - N channels with n = 1 , … , N . For example, consider the case where K = 5 and all channels can be represented as a set of binary codes { 000 , 001 , … , 111 } . The last three channels { 101 , 110 , 111 } are designated as virtual channels (Fig. (Fig.1 1 ).

Extension of number of channel by adding virtual channels with five channels as an example.
For channel distributions, a general model is considered where reward differs for various users in the same channel. In other words, the probability that a channel exhibits a fully transmitted state depends on the users. This assumption fits the situation, in complex electromagnetic environments, that the channel environment changes according to distinct users. Each user has B bits information waiting to be delivered. According to the additivity of distribution, the amount of information that user n transmits from the B bits information through channel k is B X n , k . If two or more users choose the same channel simultaneously, the collision results in X n , k = 0 . An allocation strategy π n for user n is defined as a vector of time { π n , t , n ∈ U , t = 1 , 2 , … } . Therefore, the total amount of information R t transmitted by all users at time t is defined as
Unlike the single-user model, optimal decisions of multiuser dynamic channel selection are determined by target state setting. This study consider two matching objectives: SCA 4 , which is widespread in existing literature, and FSCA, which takes vague choice preferences into account. The following provides detailed descriptions of these two definitions.
Definition 1
SCA indicates the strategy mapping M S from U to C that for any n ∈ U and k ∈ C satisfying M S ( n ) ≠ k , if μ n , k - μ n , M S ( n ) > 0 , then there exists some user n 0 ∈ U such that M S ( n 0 ) = k and μ n 0 , k - μ n , k > 0 .
Definition 2
FSCA indicates the strategy mapping M F from U to C that for any n ∈ U and k ∈ C satisfying M F ( n ) ≠ k , if | μ n , k - μ n , M F ( n ) | ≤ δ , then there exists some user n 0 ∈ U such that M F ( n 0 ) = k , | μ n 0 , M F ( n ) - μ n 0 , k | > δ or | μ n 0 , k - μ n , k | > δ .
The difference between SCA (Fig. (Fig.2a) 2 a) and FSCA (Fig. (Fig.2b) 2 b) is users’ subjective choice, which reflects that FSCA is a weaker match than SCA. From the definitions, it is noticed that a strategy is FSCA if it satisfies Conditions A and B, including the two alternatives Condition B1 and B2 (Fig. (Fig.2b). 2 b). In other words, FSCA will be reached if one of Condition B is satisfied. A more intuitive representation of fuzzy preferences is presented in Fig. Fig.2c,d. 2 c,d. If the user has a very clear acknowledgment of a channel, the good or bad preference can be seen through the shade of blue. The darker the color, the more satisfied the user is. It can be seen that, in this case, the color of blue changes a lot, and many level divisions exist (Fig. (Fig.2c). 2 c). With the same data in Fig. Fig.2c, 2 c, the user’s preferences are blurred and orange has less color change and less grading (Fig. (Fig.2d). 2 d). In the next subsection, a brief overview of the laser chaos algorithm is presented.

SCA and FSCA instructions for two users and eleven channels. ( a ) The SCA state where user 1 is willing to exchange, and user 2 is reluctant to exchange. ( b ) The SCA state where Conditions A and B1 are satisfied but Condition B2 is not. ( c ) The users’ preferences with specific cognition ranging from 0 to 1. ( d ) The users’ preferences with vague cognition obtained from (c) .
Laser chaos algorithm
In previous research, the laser chaos algorithm was experimentally proven to have a correct higher-order probability compared with other algorithms 12 . A correct higher-order probability helps the selection preference to be close to the true channel gain order, which is vital for the subsequent channel exchange. This is also the main reason the laser chaos algorithm is used in this study. As shown in Fig. Fig.3, 3 , the semiconductor laser emits laser light through the beam splitter to the laser reflector, and the delayed light injects into the diode laser emitter cavity. Laser chaos is a result of delayed laser feedback, converted to an electrical signal by a photodetector and illustrated by an oscilloscope. Through ultrafast frequency sampling, high-quality random numbers are generated.

The generation process of laser chaos where a semiconductor laser emits laser light through the beam splitter to a laser reflector, and the delayed light injects into a diode laser emitter cavity.
Previous studies used the laser chaos algorithm to find the optimal arm (channel) in MAB problems, whereas this study only uses the laser chaos algorithm to update selection preference instead of channel selection. Updating a user’s preference with the laser chaos algorithm is divided into three steps as follows.
Step 1: Select the channel according to the random number generated by laser chaos.
Throughout the previous discussions, any channel number can be converted into 2 M . Thus, use { S 1 S 2 … S M } to represent all channels. The channel can be selected by M -times comparisons between the signal levels at different times and the corresponding threshold. Denote s ( t i ) as the signal level value generated by the laser chaos at time t i and t 1 as the initial time point of the first channel selection. T i , S 1 … S i - 1 H indicates the threshold of selecting S i on the basis that S 1 … S i - 1 has been determined.
If s ( t i ) is no less than T i , S 1 … S i - 1 H , set S i to 1. Otherwise, S i is set to 0 for i = 1 , … , M . In this round of channel selection, Δ S is the time interval to select S i for i = 1 , … , M in sequence. Naturally, the initial time point is t 1 + Δ S in the next round of channel assignment and a similar procedure can be performed in the subsequence.
Step 2: Send a message and receive feedback.
Send the message through the channel k ′ = S 1 ′ … S M ′ selected in the previous step and obtain the feedback. The feedback value is equivalent to the observation of random variables. Then, record T t n , k ′ and R t n , k ′ and update μ ^ t n , k ′ of channel k ′ at time slot t .
Step 3: Adjust the threshold according to the feedback obtained in Step 2 .
This last step is to adjust the threshold according to the input obtained in the previous step. If the return is greater than 0, the threshold is adjusted to the direction that k ′ is more likely to be chosen. Otherwise, the threshold is adjusted to the direction where k ′ is less likely to be chosen. The adjustment of the threshold is performed by adding and subtracting the two parameters Λ and Ω . The parameter which will be adjusted is determined by the size of the arm gain. If the arm gain is greater than 0, the threshold is adjusted using the parameter Λ and vice versa. The parameters are divided into fixed and flexible types. The values of Λ and Ω under the fixed type are both 1. For the flexible type, Λ is set to be 1 and Ω is continuously adjusted according to the selection as follows 17 :
where L i , S 1 S 2 … S i = j indicates the selection times of observing positive return when S 1 S 2 … S i - 1 have been determined with S i = j , j ∈ { 0 , 1 } and T j , S 1 S 2 … S i = j indicates the selection times when S 1 S 2 … S i - 1 have been determined, and S i = j . The two parameter types are suitable for different distribution situations; thus, an appropriate parameter type is chosen according to the channel distribution. In more detail, we present the process of using laser chaos series to select a channel with M = 2 (Fig. (Fig.4 4 ).

The process of using the laser chaos series to select the corresponding channel when M =2.
Parallel exchange process
The basic thought of this exchange model is derived from the marriage matching model, which can be described by the following scenario (Fig. (Fig.5). 5 ). Suppose that there are two sets A , B , each of which contains L individuals. Every person has his/her unique preferences. Considering L = 3 as example, the sets of individuals are given by A : = { a , b , c } and B : = { e , f , g } . The selection preference of individuals in A is given by the strictly ordered sequence [ f , e , g ], in which the level of attraction decreases constantly. At the beginning of the first round, the individuals in A sequentially select their preferred individual from B . If an individual in B is chosen by a single individual in A , the combination is tentatively established. If an individual in B is chosen by multiple individuals in A , the individual with higher priority, as determined by the preference of the individual in B , is selected. The remaining individuals in A proceed to the next round and repeat the process until each individual in A is paired with a single individual in B .

Users { a , b , c } and users { e , f , g } are paired based on the marriage matching model with their selection preference.
The multiuser channel selection model is similar to the marriage matching model. The individuals in A can be analogized to users, whereas the individuals in B can be likened to channels. The phenomenon that one person in A can only be paired with an unmatched person in B agrees with previous assumptions in dynamic channel selection issues. Therefore, this study uses this principle to handle each user’s selection. In addition, this paper proposes a parallel processing method where all users perform channel selection (stable marriage model) simultaneously. Compared with a single initiator at a given time, the simultaneous operation of multiple users intends to get more effective information throughput. The detailed parallel exchange process is discussed below.
The exchange phase at every time slot t is divided into two parts. First, initiators are selected randomly from users, which differs from the marriage matching model with only initiators exchanging channels, not all users. Second, the randomly selected initiators will launch requests to channels in parallel. Notably, the exchange steps are closely related to the target states. For the exchange step with SCA as the target state, the selected initiators send requests to channels cyclically according to their preferences. If a channel is empty and no other requests are received, the channel is occupied by the request sender. If channel k 1 receives multiple requests from initiators, compare all μ ^ t i n i t , k 1 (including μ ^ t n 1 , k 1 if user n 1 occupies channel k 1 at time slot t ), where init indicates all initiators sending requests to channel k 1 . The user with the largest value occupies channel k 1 . In addition, if the largest one is the initiator, the channels are exchanged with user n 1 and the current preference cycle is terminated. Otherwise, the cycle continues. For the exchange step with FSCA as the target state, the process is similar to that of SCA, with the major difference being the exchange condition. The detailed steps for SCA and FSCA are shown in Fig. Fig.6 6 and 7, respectively.

Exchange steps with FSCA as the target state.

Exchange steps with SCA as the target state.
The performance of PPL-LC with SCA
The PPL-LC algorithm is initially tested using SCA as the target state. Ten channels and eight users, designated as { a , b , … , h } , are configured. The initial and subsequent channel distributions of each user are set as follows (Fig. (Fig.8). 8 ). The size of each black block represents the initial channel distribution set by each user. In other words, the larger the black block, the greater the user’s satisfaction with the channel and the higher the probability of successful data transmission. Notably, these data settings will be used throughout the experiments.

Initial channel distribution settings. ( a ) The distributions of users for each channel during 0–3000 iterations. ( b ) The distributions after 3000 iterations.
A small number of channel exchanges may prevent the algorithm from achieving the maximum information throughput. When there is a small number of channel exchanges, the information throughput may reach equilibrium more quickly, resulting in a local optimum and an inability to achieve the maximum information throughput. However, an excessive number of channel exchanges can also cause resource waste between users. Therefore, it is crucial to balance the relationship between the number of channel exchanges and throughput with two aspects. On the one hand, it is necessary to ensure sufficient exchange times so that the information throughput achieved by all users will not fall into the local optimum. On the other hand, it is also necessary to control the number of channel exchanges so as not to waste resources.
When the channel distribution does not change over time t , the relationship between the exchange amount and time, as well as the transmitted information bits and time, are examined separately. In Fig. Fig.9, 9 , the performances of the PPL-LC, DSSL, and CSM-MAB algorithms with respect to the aforementioned metrics are compared. Fig. Fig.9a 9 a shows that the DSSL and CSM-MAB algorithms reach equilibrium faster, but the information throughput under the SCA state is lower (namely, the SCA state is not reached). Although it takes the PPL-LC algorithm longer (around 100 time slots) to reach the SCA state, it ultimately achieves higher information throughput. From Fig. Fig.9b, 9 b, the number of channel exchanges for the CSM-MAB algorithm is extremely lower than that of the DSSL algorithm, close to the exchange times of the PPL-LC algorithm, which may contribute to its inability to reach the SCA state. In addition, the number of channel exchanges for the DSSL algorithm is significantly higher than those of the other two algorithms, resulting in inefficient resource utilization in practical implementation.

The performance comparison of the PPL-LC, DSSL, and CSM algorithms. ( a ) The trend of transmitted information bits over time. ( b ) The performance exchange times over time.
After 3000 iterations, the channel distribution environment changes. As shown in Fig. Fig.10, 10 , the SCA state has been achieved before the 3000th iteration. However, the information throughput experiences a sharp decline after the 3000th iteration. As a result, the channel exchange process is restarted, re-establishing of the SCA state under the new distribution. In conjunction with this, the number of channel exchanges, which had remained stable, also increased after the 3000th iteration.

The performance of PPL-LC. ( a ) The relationship between transmitted information bits and time when distribution changes. ( b ) The relationship between the number of channel exchanges and the time when distribution changes.
The performance of PPL-LC with FSCA
In the following, the FSCA state is considered the target state. Similar to the SCA state, the performances of the three algorithms in terms of throughput and change times are considered (Fig. (Fig.11). 11 ). Under the FSCA target, the PPL-LC algorithm fluctuates after reaching a high information throughput, corresponding to the user’s overall selection switching back and forth between several fuzzy states. With more observations, the PPL-LC algorithm can result in the throughput tending to be stable. Meanwhile, the DSSL and CSM-MAB algorithms still cannot reach the FSCA state, and the number of channel exchanges of the CSM-MAB algorithm quickly reaches equilibrium, whereas the number of channel exchanges of the DSSL algorithm gradually increases as users continue to exchange between empty channels.

The performance of PPL-LC with FSCA. ( a ) The relationship between total information transmitted and time, which also exhibits the comparison with the DSSL and CSM algorithms. ( b ) The relationship between exchanges and time.
Previous research has demonstrated that laser chaos is effective at random number generation and can have a good effect on MAB problems. Considering these facts, the usefulness of ultrafast chaos sequences for real wireless applications was confirmed 14 . Despite this, there is inadequate research on the potential applications of the laser chaos series. In this study, we explored the high performance of ultrafast laser chaos for CRNs with multiple users. We proposed a parallel processing algorithm using laser chaos to attain two stable states: the classical SCA and FSCA. We discovered that the proposed algorithm provides improved performance, specifically in terms of throughput, in dynamic spectrum access. In addition, the algorithm demonstrates good adaptability to changing environments, which is a key feature in dynamic spectrum access scenarios where the channel conditions may vary over time. These combined capabilities make the proposed algorithm a valuable tool for improving the performance of CRNs with multiple users.
These findings of this study extend those of Takeuchi 14 with multiple users and unlimited channel numbers, confirming that ultrafast laser chaos gives high performance in wireless communication networks. In addition, this study expands the classical SCA and describes the circumstance where users have vague preferences. Therefore, this study indicates that laser chaos has positive effects on wireless communication networks. Particularly, to the best of our knowledge, the proposed algorithm is the first to apply laser chaos to channel selection with multiple users. Although this study uses laser-chaos-based decision-making for dynamic channel selection, future work can focus on more general channel assignment scenarios.
In this section, the proposed PPL-LC algorithm is presented, along with the parameter adjustment of the laser chaos algorithm.
Brief description of the PPL-LC algorithm
With detailed discussions in previous sections, the PPL-LC algorithm is described in detail (Fig. (Fig.12). 12 ). It comprises three parts: initializing, updating and exchanging. In the initializing part, it employs the Communication-free learning (CFL) algorithm 18 to reach an initial orthogonal configuration (line 1). The CFL algorithm convergence quickly to an orthogonal configuration, allowing users to proceed with the updating and exchanging parts.

The PPL-LC algorithm.
Next, in the updating part, users select the channel according to the random number generated by laser chaos (lines 5–10). Then,users send a message and obtain feedback. Based on this, the return and corresponding parameters are adjusted (lines 11–12). The above steps are all supported by the principle introduced in the laser chaos algorithm. Therefore, users obtain a list of channels they prefer over their current action.
Upon arriving at the exchanging part, randomly select initiators among users (line 15). All the initiators choose a channel based on their preference list simultaneously. Every initiator will then observe whether the channel is empty and only receiving one initiator’s request (line 19). If this occurs, he/she can occupy this channel immediately (lines 20–21). Otherwise, further observations are reqquired for the selection. The initiator proceeds to signal whether the desired channel is being occupied by a user who is not an initiator. If it is, this responding user will consider whether exchanging channels with the initiator will improve his/her osituation based on his/her preference ranking. If the responder agrees to the swap, it will be done (lines 24–26). If the responder refuses, the initiator will approach the next best channel on the list.
If the desired channel is not occupied by a user, but initiators, all the initiators who have sent requests to this channel will compare their estimated returns. The initiator with the largest estimated return will occupy the channel. If multiple initiators have the same highest estimated return, they will all move on to the next best channel on their list. All users will continue the process of seeking a partner who is willing to exchange channels with them until they either find one or exhaust their list of potential swaps. This part of the algorithm is illustrated in Fig. Fig.10 10 .
Parameter adjustment
When user n occupies channel k to transmit information and receives a positive return, the parameters of the laser chaos algorithm are adjusted as follows:
If the feedback return is equal to zero, it is updated with
Data analysis
For laser chaos generation, there are two ways to simulate: optical system and computer simulation. This study adopts computer simulation to obtain random numbers and the simulation with real optical systems will be studied in later papers. This simulation is mainly completed through the example-DiodeWithFeedbackSimulation in Matlab Simulate and the chaotic waveform is sampled to form a random sequence. Compared with building an optical system, this method is more convenient. The simulation is performed on a personal computer (R9000X 2021, R7 5800H 3.2GHz, 16GB RAM, Windows 11, Matlab R2016b).
Parameters setting
In this study, the initial parameters of the laser chaos algorithm are selected as follows: Λ = 1 , Ω = 1 , α = 0.99 . The values of Δ S and Δ L are the same as in the previous experiments, and we convert the signal level value obtained by laser chaos to 8 bits, namely, - 128 ≤ s ( t ) ≤ 127 19 .
Acknowledgements
The authors would like to thank two anonymous referees for the extensive and constructive suggestions that helped to improve this paper considerably. The work is supported by National Key R &D Program of China (No. 2018YFA0703900), Shandong Provincial Natural Science Foundation (No.ZR2021MA098).
Author contributions
Z.J.C., L.W. and C.Z.X. designed and conducted the experiments, and wrote the paper.
Data availability
Competing interests.
The authors declare no competing interests.
Publisher's note
Springer Nature remains neutral with regard to jurisdictional claims in published maps and institutional affiliations.
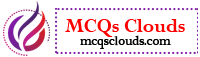
Advantage of using Dynamic channel assignment is
A. Blocking is reduced
B. Capacity of the system is increased
C. Both a & b
D. None of the above
Answer & Explanation
Related Questions on Mobile Communication Test Questions
The modulation technique used for mobile communication systems during world war II was
A. Amplitude modulation
B. Frequency modulation
----------- introduced Frequency Modulation for mobile communication systems in 1935.
A. Edwin Armstrong
B. Albert Einstein
C. Galileo Galilei
D. David Bohm
The early FM push-to-talk telephone systems were used in
A. Simplex mode
B. Half duplex mode
C. Full duplex mode
DECT stands for
A. Digital European Cellular Telex
B. Digitized Emergency Cellular Telephone
C. Digital European Cordless Telephone
D. Digital European Cellular Telephone
World's first cellular system was developed by
A. Nippon Telephone and Telegraph (NTT)
B. Bellcore and Motorola
C. AT&T Bell Laboratories
D. Qualcomm
More Related Questions on Mobile Communication Test Questions
Read More: MCQ Type Questions and Answers
- Aerospace & Aeronautical Mcqs
- Agriculture Mcqs
- Banking Exam Questions
- Biology Mcqs
- Career Aptitude Test
- Central Superior Service Test Mcqs (CSS)
- Chemical Engineering Mcqs
- Chemistry Mcqs
- Civil Engineering Mcqs
- Computer Science MCQs
- ECAT & MCAT Entry Test Mcqs
- Economics Mcqs
- Electrical Engineering Questions
- Electronic Engineering Questions
- English for Competitive Exams
- English Literature Mcqs
- English MCQs
- General Knowledge Quiz
- Geography Mcqs
- Graduate Assessment Test Mcqs (GAT)
- Health & Physical MCQs
- Islamic Studies Mcqs
- Judiciary & Law Mcqs
- Mathematics Quizzes
- MBA Questions
- Mechanical Engineering Questions
- Medical Subjects Mcqs
- Pedagogy Mcqs
- Physics MCQs
- Placement Tests
- Science Mcqs
- Sociology Mcqs
- Software Engineering Mcqs
- Statistics Mcqs
Optimized channel allocation in emerging mobile cellular networks
- Methodologies and Application
- Published: 20 May 2020
- Volume 24 , pages 16361–16382, ( 2020 )
Cite this article
- Daniel Asuquo ORCID: orcid.org/0000-0002-0189-737X 1 ,
- Moses Ekpenyong ORCID: orcid.org/0000-0001-6774-5259 1 ,
- Samuel Udoh 1 ,
- Samuel Robinson 1 &
- Kingsley Attai 2
234 Accesses
7 Citations
Explore all metrics
The task of optimizing service quality in wireless networks is a continuous research that requires the design of efficient channel allocation schemes. The problem is how limited channel resources can be maximally utilized, to guarantee seamless communication while maintaining excellent service quality. Whereas, fixed channel allocation (FCA) schemes treat new and handoff calls equally without preference to normally prioritized handoff calls; dynamic channel allocation (DCA) schemes accommodate users mobility in randomly changing network conditions. However, classical Erlang-B models are deficient and do not consider users mobility and dynamically changing traffic of the mobile network environment. A modified Erlang-B dynamic channel allocation (MEB-DCA) scheme is therefore introduced in this paper, for improved network performance. The MEB-DCA algorithm introduces a conditional threshold for handoff request assignment to ensure that communication systems do not unnecessarily prioritize handoff calls at the detriment of new calls. Deriving knowledge from imprecise network data is difficult when developing functional relationships between parameters, requiring advanced modeling techniques with cognitive experience. Soft computing techniques have been shown to handle this challenge given its ability to represent precisely, both data and expert knowledge. An adaptive neuro-fuzzy inference system-based dynamic channel allocation (ANFIS-DCA) framework was proposed to automate the learning of communication parameters for optimized channel allocation decisions. Network parameters considered were received signal strength indication impacted by user mobility, number of guard and general channels, carried traffic, and handoff blocking threshold. The performance of the proposed ANFIS-DCA model was found to outsmart the static FCA and back propagation neural network-based DCA (NN-DCA) schemes using mean square error and root mean square error as performance measures. Our approach can be effectively deployed to improve channel allocation, resource utilization, network capacity, and satisfy users experience.
This is a preview of subscription content, log in via an institution to check access.
Access this article
Price includes VAT (Russian Federation)
Instant access to the full article PDF.
Rent this article via DeepDyve
Institutional subscriptions
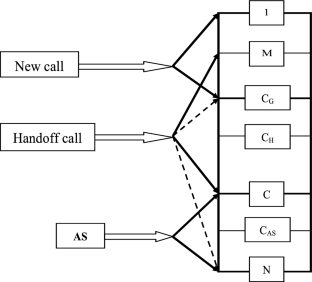
Similar content being viewed by others
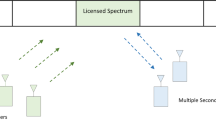
A review of spectrum sensing in modern cognitive radio networks
Muhammad Umair Muzaffar & Rula Sharqi
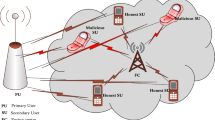
Discrimination of primary user emulation attack on cognitive radio networks using machine learning based spectrum sensing scheme
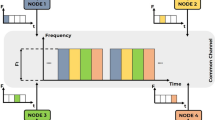
Multiple channel access techniques in industrial IoT: evaluation case of time-slotted channel hopping
Abdelhadi Eloudrhiri Hassani, Aicha Sahel, … Ilham El Mourabit
Adiego D, Cordier C (2002) Multiservice radio dimensioning for UMTS packet-switched services. Proc IEEE Pers Indoor Mob Radio Commun 5:2409–2413
Article Google Scholar
Ahmed RE (2006) A hybrid channel allocation algorithm using hot-spot notification for wireless cellular networks. In: Proceedings of IEEE Canadian conference on electrical and computer engineering, Canada, pp 891–894. https://doi.org/10.1109/CCECE.2006.277671
Alagu S, Meyyappan T (2012) A novel handoff decision algorithm in call admission control strategy to utilize the scarce spectrum in wireless mobile networks. Int J Wirel Mob Netw 4(6):99–113. https://doi.org/10.5121/ijwmn.2012.4608
Ali SH (2013) Novel approach for generating the key of stream cipher system using random forest data mining algorithm. In: 2013 sixth international conference on developments in esystems engineering, pp 259–269. IEEE. https://doi.org/10.1109/DeSE.2013.54
Al-Janabi S, Alkaim AF (2019) A nifty collaborative analysis to predicting a novel tool (DRFLLS) for missing values estimation. Soft Comput 24(1):555–569. https://doi.org/10.1007/s00500-019-03972-x
Al-Janabi S, Alwan E (2017) Soft mathematical system to solve black box problem through development the FARB based on hyperbolic and polynomial functions. In: 2017 10th international conference on developments in esystems engineering (DeSE), pp 37–42. IEEE. https://doi.org/10.1109/DeSE.2017.23
Al-Janabi S, Hussein NY (2019) The reality and future of the secure mobile cloud computing (SMCC): survey. In: International Conference on big data and networks technologies, pp 231–261. Springer, Cham. https://doi.org/10.1007/978-3-030-23672-4_18
Al-Janabi S, Patel A, Fatlawi H, Kalajdzic K, Al Shourbaji I (2014) Empirical rapid and accurate prediction model for data mining tasks in cloud computing environments. In: 2014 international congress on technology, communication and knowledge (ICTCK), pp 1–8. IEEE. https://doi.org/10.1109/ICTCK.2014.7033495
Al-Janabi S, Al-Shourbaji I, Shojafar M, Abdelhag M (2017) Mobile cloud computing: challenges and future research directions. In: 2017 10th international conference on developments in esystems engineering (DeSE), pp 62–67. IEEE. https://doi.org/10.1109/DeSE.2017.21
Alkaim AF, Al-Janabi S (2019) Multi objectives optimization to gas flaring reduction from oil production. In: International conference on big data and networks technologies, pp 117–139. Springer, Cham. https://doi.org/10.1007/978-3-030-23672-4_10
Alshanyour A, Baroudi U (2010) A simulation study: the impact of random and realistic mobility models on the performance of bypass-AODV in ad hoc wireless networks. EURASIP J Wirel Commun Netw 2010:1–10
Amaldi E, Capone A, Malucelli F, Mannino C (2006) Optimization problems and models for planning cellular networks. In: Resende M, Pardalos P (eds) Handbook of optimization in telecommunication, vol 31. Springer, New York, pp 879–901
MATH Google Scholar
Amaldi E, Capone A, Malucelli F (2008) Radio planning and coverage optimization of 3G cellular networks. Wirel Netw 14(4):435–447. https://doi.org/10.1007/s11276-006-0729-3
Asuquo DE, Williams EE, Nwachukwu EO, Inyang UG (2013) An intelligent call admission control scheme for quality of service provisioning in a multi-traffic CDMA network. Int J Sci Eng Res 4(2):152–161
Google Scholar
Deng W, Zhao H, Zou L, Li G, Yang X, Wu D (2017) A novel collaborative optimization algorithm in solving complex optimization problems. Soft Comput 21:4387–4398. https://doi.org/10.1007/s00500-016-2071-8
Deng W, Xu J, Zhao H (2019) An improved ant colony optimization algorithm based on hybrid strategies for scheduling problem. IEEE Access 7:20281–20292. https://doi.org/10.1109/ACCESS.2019.2897580
Doo-Won L, Gye-Tae G, Dong-Hoi K (2010) A cost-based adaptive handover hysteresis scheme to minimize the handover failure rate in 3GPP LTE system. EURASIP J Wirel Commun Netw 10:1–7. https://doi.org/10.1155/2010/750173
Ekpenyong ME, Asuquo DE (2017) HMM-based quality of service survivability in mobile cellular networks. J Niger Math Soc 35:191–217
MathSciNet Google Scholar
Ekpenyong M, Isabona J (2011) An enhanced SINR-based call admission control in 3G networks. Int J Wirel Mob Netw 3(5):49–64. https://doi.org/10.5121/ijwmn.2011.3505
Ekpenyong M, Isabona J, Isong E (2015) Handoffs decision optimization of mobile cellular networks. In: Proceedings of international conference on computational science and computational intelligence, pp 697–702. https://doi.org/10.1109/CSCI.2015.155
Ekpenyong ME, Inyang UG, Asuquo DE, Ekong UO, Usip PU, Umoh UA, Aniekpeno J, Isabona J, Tom A (2018) Intelligent test bed tuning for improved wireless local area networks service quality in academic environments. Int J Artif Intell 16(1):60–87
Ekpenyong ME, Asuquo DE, Umoren IJ (2019) Evolutionary optimisation of energy-efficient communication in wireless sensor networks. Int J Wirel Inf Netw. https://doi.org/10.1007/s10776-019-00450-x
Gangwar AK, Singh VV (2014) Quality of service improvement, handoff prioritization and channel utilization for cellular network. Int J Eng Res Appl 4(10):46–49
Gehlod L, Jain V, Sharma GJ (2013) Handover management using adaptive and prioritization scheme in cellular mobile systems. Int J Adv Res Comput Commun Eng 2(7):2689–2692
Ghaderi M, Boutaba R (2006) Call admission control in mobile cellular network: a comprehensive survey. Wirel Commun Mob Comput 6(1):69–93. https://doi.org/10.1002/wcm.246
Gharbi N (2016) Using GSPNs for performance analysis of a new admission control strategy with retrials and guard channels. In: Kim K, Wattanapongsakorn N, Joukov N (eds) 2016 mobile and wireless technologies lecture notes in electrical engineering, vol 391. Springer, Singapore
Hamid NIB, Kawser MT, Hoque MA (2012) Coverage and capacity analysis of LTE radio network planning considering Dhaka city. Int J Comput Appl 46(15):49–56. https://doi.org/10.5120/6989-9604
Huang J, Huang CY, Chou CM (2004) Soft-blocking based resource dimensioning for CDMA systems. Proc IEEE Veh Technol Conf 6:4306–4309
Huang R, Zhang C, Zhao H, Fang Y (2010) Enhancing handoff performance by introducing Ad-hoc mode into cellular networks. In: Proceedings of IEEE global telecommunications conference, Miami, pp 1–5
Isabona J, Ekpenyong M (2009) Performance evaluation of coverage capacity interaction in CDMA wireless networks. J Niger Math Soc 28:153–167
ITU-D (2007) Telecom network planning for evolving network architectures: reference manual, draft version 4.1, document NPM/4.1, Geneva
Iversen VB, Stepanov SN, Kostrov AV (2006) Dimensioning of multiservice links taking account of soft blocking. In: International conference on next generation wired/wireless networking, pp 3–10. Springer, Berlin
Kar RR, Nayak SS (2014) An efficient adaptive channel allocation scheme for cellular networks. IOSR J Comput Eng 16(2):75–79
Katzis K, Pearce DAJ, Grace D (2004) Fixed channel allocation techniques exploiting cell overlap for high altitude platforms. In: Proceedings of 5th European wireless conference mobile and wireless systems beyond 3G, Barcelona, Spain, Academic Press
Kolate VS, Patil GI, Bhide AS (2012) Call admission control schemes and handoff prioritization in 3G wireless mobile networks. Int J Eng Innov Technol 1(3):92–97
Kumar S, Kumar K, Pandey AK (2016) Dynamic channel allocation in mobile multimedia networks using error back propagation and hopfield neural network (EHP-HOP). In: Proceedings of 12th international multi-conference on information processing, procedia computer science, vol 89, pp 107–116
Liu Z-H, Chen J-C (2012) Design and analysis of the gateway relocation and admission control algorithm in mobile WiMax networks. IEEE Trans Mob Comput. https://doi.org/10.1109/TMC.2010.265
Mahalungkar SP, Sambare SS (2013) Improved call blocking probability reducing technique using auxiliary stations. Int J Sci Eng Res 4(3):1–5
Nie S, Wu D, Zhao M, Gu X, Zhang L, Lu L (2015) An enhanced mobility state estimation based handover optimization algorithm in LTE-A self-organizing network. In: Proceedings of 6th international conference on ambient systems, networks and technologies, vol 52, pp 270–277. https://doi.org/10.1016/j.procs.2015.05.078
Nyangaresi VO, Abeka SO, Rodgrigues A (2018) Security evaluation of cellular networks handover techniques. Int J Comput Netw Inf Secur 5:45–59. https://doi.org/10.5815/ijcnis.2018.05.06
Parija S, Nanda SK, Sahu PK, Singh SS (2013) Location prediction of mobility management using soft computing techniques in cellular network. Int J Comput Netw Inf Secur 6:27–33
Prakash R, Shivaratri NG, Singhal M (2015) Distributed dynamic fault-tolerant channel allocation for mobile computing. IEEE Trans Veh Technol 52:47–56
Premkumar N, Kannan M (2015) Optimal fast handover algorithm to reduce handover latency in wireless communication. Int J Adv Eng 1(3):378–381
Shamar A, Kumar D (2011) Call blocking performance of new reservation-based channel assignment scheme in cellular networks. In: Proceedings of IJCA special issue on 2nd national conference on computing, communication and sensor network, pp 6–9
Sriwastava VP, Singh J, Verma VK (2014) Decreasing call blocking rate by using optimization technique. Int J Sci Res Publ 4(6):1–5
Udoh SS, Akinyokun OC, Inyang UG, Olabode O, Iwasokun GB (2017) Discrete event based hybrid framework for petroleum products pipeline activities classification. J Artif Intell Res 6(2):39–50
Walia N, Singh H, Sharma A (2015) ANFIS: adaptive neuro-fuzzy inference system—a survey. Int J Comput Appl 123(13):32–38
Zeng Q-A, Agrawal DP (2003) Modeling and efficient handling of handoff in integrated wireless mobile networks. IEEE Trans Veh Technol 51(6):1469–1478
Zhang J, Guo L, Yang J, Wu TY (2004) Automation of 3G/4G cellular network planning. In: Proceedings of IEEE 5th international conference on 3G mobile communications technologies, London, pp 412–416
Zhao C, Gan L (2011) Dynamic channel assignment for large-scale cellular networks using noisy chaotic neural network. IEEE Trans Neural Netw 22:222–232. https://doi.org/10.1109/TNN.2010.2091653
Zhao X, Liang H, Gu Z (2008) A Markov chain-based capacity dimensioning method for wireless communication system with AMC, HARQ and packet multimedia traffic source. IEEE Int 1:2. https://doi.org/10.1109/ICC.2008.86
Zhao H, Liu H, Xu J, Deng W (2019) Performance prediction using high-order differential mathematical morphology gradient spectrum entropy and extreme learning machine. IEEE Trans Instrum Meas. https://doi.org/10.1109/TIM.2019.2948414
Download references
Acknowledgements
The authors would like to thank all the reviewers for their constructive comments. This research was supported by the Tertiary Education Trust Fund (TETFund)-Nigeria, research Grant. The program for the study, training and simulation of the proposed algorithm in this article was written in the toolbox of MATLAB R2015a produced by the Math-Works, Inc.
Author information
Authors and affiliations.
Department of Computer Science, Faculty of Science University of Uyo, Uyo, Nigeria
Daniel Asuquo, Moses Ekpenyong, Samuel Udoh & Samuel Robinson
Department of Mathematics and Computer Science, Ritman University, Ikot Ekpene, Nigeria
Kingsley Attai
You can also search for this author in PubMed Google Scholar
Corresponding author
Correspondence to Daniel Asuquo .
Ethics declarations
Conflict of interest.
The authors declare that there is no conflict of interest for any of them in this article.
Additional information
Communicated by V. Loia.
Publisher's Note
Springer Nature remains neutral with regard to jurisdictional claims in published maps and institutional affiliations.
Rights and permissions
Reprints and permissions
About this article
Asuquo, D., Ekpenyong, M., Udoh, S. et al. Optimized channel allocation in emerging mobile cellular networks. Soft Comput 24 , 16361–16382 (2020). https://doi.org/10.1007/s00500-020-04947-z
Download citation
Published : 20 May 2020
Issue Date : November 2020
DOI : https://doi.org/10.1007/s00500-020-04947-z
Share this article
Anyone you share the following link with will be able to read this content:
Sorry, a shareable link is not currently available for this article.
Provided by the Springer Nature SharedIt content-sharing initiative
- Network optimization
- Erlang-B model
- Service quality
- Find a journal
- Publish with us
- Track your research
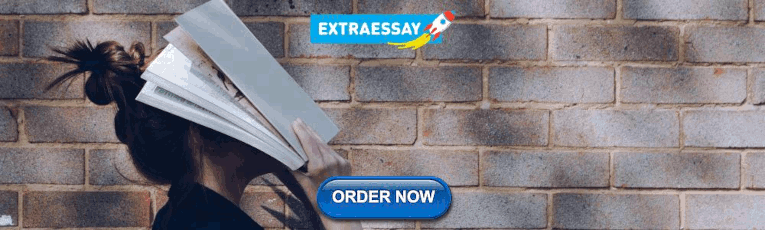
COMMENTS
Dynamic Channel Assignment adjustments will subsequently occur as needed. Any time that a controller in the RF Group enters or departs the RF group (a reboot for instance) Start-up mode is assumed. ... Higher Data Rates, better multipath immunity, and Client Link are three examples of big benefits that can be enjoyed by legacy as well as 802 ...
Dynamic channel assignment (DCA) In dynamic channel assignment, channels are assigned to cells on demand, based on the current traffic load. i.e., there is no allocation of predetermined set of channels (voice channels). This strategy is more efficient than FCA, but it is also more complex to implement. Advantages of DCA: Improves spectrum ...
The Dynamic Channel Assignment (DCA) Algorithm. • Can dynamically determine best bandwidth for each AP (DBS v.8.1) Figure 1: When a new AP is added, it's radio conflicts with an existing AP's radio causing contention. DCA adjusts the channel plan for the best solution for the new AP. DCA's job is to monitor the available channels for the RF ...
Dynamic channel assignment approach using CRNs is implemented in [89], where the authors developed a dynamic channel assignment problem as an optimization problem by using the MILP approach, and proposed an ant colony optimization (ACO) algorithm to address the dynamic channel assignment problem in CRNs. 4.3 Non-linear programming
Conclusion. Dynamic channel allocation (DCA) is a technique used in wireless communication networks to optimize the allocation of RF channels to different users in real-time. DCA can adapt to changes in the traffic load, user mobility, and interference conditions. DCA can provide various performance guarantees, such as fairness, priority, or QoS.
distributed dynamic channel assignment (DCA) , where the nature of the strategy permits adaptation to spatial and temporal traffic variations while the distribution of control reduces the required computation and commu ... often yield an inefficient arrangement of channels. Although DCA has clear benefits, the cost can be quite high because ...
Dynamic Channel Assignment (DCA) assigns the channels to the cells dynamically according to traffic demand, and hence, can provide higher capacity (or lower call blocking probability), fidelity and quality of service than the fixed assignment schemes. Devising a DCA, that is practical, efficient, and which can generate high quality allocations ...
In general, dynamic channel allocation methods can generate better capacity and better handoff performance (lesser forced terminations). Previous studies of dynamic methods are largely simulation based, and theoretical studies aimed at a better understanding of the benefits are relatively few.
Fixed Channel Allocation (FCA): Fixed Channel Allocation is a strategy in which fixed number of channels or voice channels are allocated to the cells. Once the channels are allocated to the specific cells then they cannot be changed. In FCA channels are allocated in a manner that maximize Frequency reuse. In cell A 20 Channels or Voice channels ...
Similar to the goals of dynamic channel assignment is the process of Dynamic Channel Reassignment (DCR). Whereas a DCA scheme allocates a channel to an initial call or handover , a DCR system switches a cell's channel (that is currently being used) to another channel which is closer to the optimum according to frequency reuse or other cost ...
Fixed Channel Allocation Strategy is less costly than the DCA. Dynamic Channel Allocation Strategy is costly because lot of computation is required in real-time. In FCA allocated channels remains to the cell, once the call is completed. In DCA once the call is completed then the channel or the voice channel return to the MSC.
Dynamic channel assignment is an effective approach to decrease inter-cell interference and improve link quality. Due to flat beam coverage property, link performance is degraded if conventional dynamic channel assignment schemes are directly employed in satellite network. To solve this problem, this study proposes the beam cooperation based ...
The advantage of using a fixed ordering is that the channels higher in the ordering are used more frequently and, hence, have higher interference levels. ... K. Okada, A dynamic channel assignment strategy using information of speed and moving direction in microcellular systems, in International Symposium on Circuits and Systems, Chicago, IL, ...
In dynamic channel allocation schemes, frequency channels are not permanently allotted to any user. Channels are assigned to the user as needed depending upon the network environment. The available channels are kept in a queue or a spool. The allocation of the channels is temporary. Distribution of the channels to the contending users is based ...
The performance advantages of using adaptive modulation are investigated in terms of the overall network performance, mean transmitted power, and the average network throughput. Adaptive ...
A dynamic channel assignment (DCA) strategy called Hybrid-DCA is proposed in a clustered ad hoc network. This DCA strategy is designed to make the best use of available channels by taking advantage of the spatial reuse concept.
An effective and efficient Modified Genetic Algorithm (MGA) is presented for the solution of DCA Problem, which can provide higher capacity, fidelity and quality of service than the fixed assignment schemes. In GSM Telecommunication networks it is important to efficiently utilize the scarce radio spectrum using a proper channel assignment scheme. The objective of a Channel Assignment problem ...
Two dynamic channel assignment (DCA) strategies, called greedy-based DCA (GB-DCA) and channel segregation DCA (CS-DCA) are proposed in a clustered wireless multihop ad hoc network. These DCA strategies are designed to make better use of available channels by taking advantage of the spatial reuse concept.
dynamic channel allocation methods. There is a considerable literature on dynamic channel allocation. How ever, most of these studies use simulation tools for analysis, and in addition, handoff considerations are usually ignored. Theoretical studies aimed at a better understanding of the benefits of dynamic methods are relatively few.
The Dynamic Channel Assignment (DCA) Algorithm. The Group Leader maintains the neighbor lists for all AP's in the RF Group, and organizes these neighbors into RF Neighborhoods. The following metrics are also tracked for each AP in the RF Group. Same Channel Contention —other AP's/clients on the same channel - also known as Co-Channel ...
Using the Gale-Shapley stable matching algorithm, the stable marriage problem of multiuser dynamic channel assignment was first solved under the assumption that the expected rates are known and a distributed opportunistic CSMA protocol that solves the problem was proposed 4. This approach has been shown to have a range of advantages and has ...
World's first cellular system was developed by. A. Nippon Telephone and Telegraph (NTT) B. Bellcore and Motorola. C. AT&T Bell Laboratories. D. Qualcomm. View Answer. MCQs: Advantage of using Dynamic channel assignment is - (A) Blocking is reduced - (B) Capacity of the system is increased.
A modified Erlang-B dynamic channel allocation (MEB-DCA) scheme is therefore introduced in this paper, for improved network performance. The MEB-DCA algorithm introduces a conditional threshold for handoff request assignment to ensure that communication systems do not unnecessarily prioritize handoff calls at the detriment of new calls.