- Open access
- Published: 01 September 2021
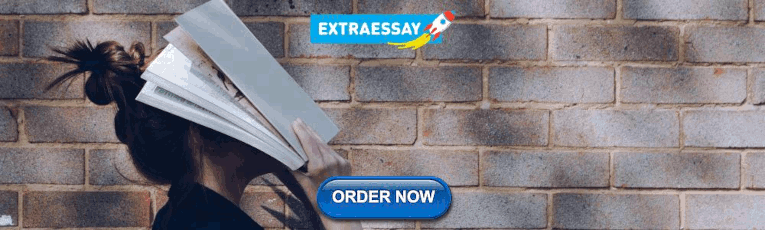
Perspectives in modeling and model validation during analytical quality by design chromatographic method evaluation: a case study
- Yongzhi Dong ORCID: orcid.org/0000-0003-4268-5952 1 ,
- Zhimin Liu 1 ,
- Charles Li 1 ,
- Emily Pinter 1 ,
- Alan Potts 1 ,
- Tanya Tadey 1 &
- William Weiser 1
AAPS Open volume 7 , Article number: 3 ( 2021 ) Cite this article
4255 Accesses
1 Citations
Metrics details
Design of experiments (DOE)-based analytical quality by design (AQbD) method evaluation, development, and validation is gaining momentum and has the potential to create robust chromatographic methods through deeper understanding and control of variability. In this paper, a case study is used to explore the pros, cons, and pitfalls of using various chromatographic responses as modeling targets during a DOE-based AQbD approach. The case study involves evaluation of a reverse phase gradient HPLC method by a modified circumscribed central composite (CCC) response surface DOE.
Solid models were produced for most responses and their validation was assessed with graphical and numeric statistics as well as chromatographic mechanistic understanding. The five most relevant responses with valid models were selected for multiple responses method optimization and the final optimized method was chosen based on the Method Operable Design Region (MODR). The final method has a much larger MODR than the original method and is thus more robust.
This study showcases how to use AQbD to gain deep method understanding and make informed decisions on method suitability. Discoveries and discussions in this case study may contribute to continuous improvement of AQbD chromatography practices in the pharmaceutical industry.
Introduction
Drug development using a quality by design (QbD) approach is an essential part of the Pharmaceutical cGMP Initiative for the twenty-first century (FDA Pharmaceutical cGMPs For The 21st Century — A Risk-Based Approach. 2004 ) established by the FDA. This initiative seeks to address unmet patient needs, unsustainable rise of healthcare costs, and the reluctance to adopt new technology in pharmaceutical development and manufacturing. These issues were the result of old regulations that are very rigid and made continuous improvement of previously approved drugs both challenging and costly. The International Council for Harmonization of Technical Requirements for Pharmaceuticals for Human Use (ICH) embraced this initiative and began issuing QbD relevant quality guidelines in 2005. The final versions of ICH Q8–Q12 [(ICH Q8 (R2) 2009 ) (ICH Q9 2005 ) (ICH Q10 2008 ) (ICH Q11 2012 ) (ICH Q12 2019 )] have been adopted by all ICH members. The in-progress version of ICH Q14 (ICH Q14 2018 ) will offer AQbD guidelines for analytical procedures and promote the use of QbD principles to achieve a greater understanding and control of testing methods and reduction of result variability.
Product development using a QbD approach emphasizes understanding of product and process variability, as well as control of process variability. It relies on analytical methods to measure, understand, and control the critical quality attributes (CQA) of raw materials and intermediates to optimize critical process parameters and realize the Quality Target Product Profile (ICH Q8 (R2) 2009 ). Nevertheless, part of the variability reported by an analytical test can originate from the variability of the analytical measurement itself. This can be seen from Eq. 1 .
The reported variability is the sum of intrinsic product variability and extrinsic analytical measurement variability (NIST/SEMATECH e-Handbook of statistical methods 2012a , 2012b , 2012c , 2012d ). The measurement variability can be minimized by applying QbD principles, concepts, and tools during method development to assure the quality and reliability of the analytical method can meet the target measurement uncertainty (TMU) (EURACHEM/CITAC 2015 ). High-quality analytical data truthfully reveal product CQAs and thus enables robust, informed decisions regarding drug development, manufacturing, and quality control.
ICH Q14 introduces the AQbD concepts, using a rational, systematic, and holistic approach to build quality into analytical methods. The Method Operable Design Region (MODR) (Borman et al. 2007 ) is a multidimensional space based on the critical method parameters and settings that provide suitable method performance. This approach begins with a predefined analytical target profile (ATP) (Schweitzer et al. 2010 ), which defines the method’s intended purpose and commands analytical technique selection and all other method development activities. This involves understanding of the method and control of the method variability based on sound science and risk management. It is generally agreed upon that systematic AQbD method development should include the following six consecutive steps (Tang 2011 ):
ATP determination
Analytical technique selection
Method risk assessment
MODR establishment
Method control strategy
Continuous method improvement through a life cycle approach
A multivariate MODR allows freedom to make method changes and maintain the method validation (Chatterjee S 2012 ). Changing method conditions within an approved MODR does not impact the results and offers an advantage for continuous improvement without submission of supplemental regulatory documentation. Establishment of the MODR is facilitated by multivariate design of experiments (DOE) (Volta e Sousa et al. 2021 ). Typically, three types of DOE may be involved in AQbD. The screening DOE further consolidates the potential critical method parameters determined from the risk assessment. The optimization DOE builds mathematical models and selects the appropriate critical method parameter settings to reach to the target mean responses. Finally, the robustness DOE further narrows down the critical method parameter settings to establish the MODR, within which the target mean responses are consistently realized. Based on this AQbD framework, it is very clear DOE models are essential to understanding and controlling method variability to build robustness into analytical methods. Although there have been extensive case studies published regarding AQbD (Grangeia et al. 2020 ), systematic and in-depth discussion of the fundamental AQbD modeling is still largely unexplored. Methodical evaluation of the pros, cons, and pitfalls of using various chromatographic responses as modeling targets is even more rare (Debrus et al. 2013 ) (Orlandini et al. 2013 ) (Bezerraa et al. 2019 ). The purpose of this case study is to investigate relevant topics such as data analysis and modeling principles, statistical and scientific validation of DOE models, method robustness evaluation and optimization by Monte Carlo simulation (Chatterjee S 2012 ), multiple responses method optimization (Leardi 2009 ), and MODR establishment. Discoveries and discussions in this case study may contribute to continuous improvement of chromatographic AQbD practices in the pharmaceutical industry.
Methods/experimental
Materials and methods.
C111229929-C, a third-generation novel synthetic tetracycline-class antibiotic currently under phase 1 clinical trial was provided by KBP Biosciences. A reverse phase HPLC purity and impurities method was also provided for evaluation and optimization using AQbD. The method was developed using a one factor at a time (OFAT) approach and used a Waters XBridge C18 column (4.6 × 150 mm, 3.5 μm) and a UV detector. Mobile phase A was composed of ammonium acetate/ethylenediaminetetraacetic acid (EDTA) buffer at pH 8.8 and mobile phase B was composed of 70:30 (v/v) acetonitrile/EDTA buffer at pH 8.5. Existing data from forced degradation and 24-month stability studies demonstrated that the method was capable of separating all six specified impurities/degradants with ≥ 1.5 resolution.
A 1.0 mg/mL C111229929-C solution was prepared by dissolving the aged C111229929-C stability sample into 10 mM HCl in methanol and used as the method evaluation sample. An agilent 1290 UPLC equipped with a DAD detector was used. In-house 18.2 MΩ Milli-Q Water was used for solution preparations. All other reagents were of ACS equivalent or higher grade. Waters Empower® 3 was used as the Chromatographic Data System. Fusion QbD v 9.9.0 software was used for DOE design, data analysis, modeling, Monte Carlo simulation, multiple responses mean, and robustness optimization. Empower® 3 and Fusion QbD were fully integrated and validated.
A method risk assessment was performed through review of the literature and existing validation and stability data to establish priorities for method inputs and responses. Based on the risk assessment, four method parameters with the highest risk priority numbers were selected as critical method parameters. Method evaluation and optimization was performed by a modified circumscribed central composite (CCC) response surface DOE design with five levels per parameter, for a total of 30 runs. The modifications were the extra duplicate replications at three factorial points. In addition to triplicate replications at the center point, the modified design had a total of nine replicates. See Table 1 for the detailed design matrix. A full quadratic model for the four-factor five-level CCC design has a total of fourteen potential terms. They include four main linear terms (A, B, C, D), four quadratic terms (A 2 , B 2 , C 2 , D 2 ), and six two-way interaction terms (A*B, A*C, A*D, B*C, B*D, and C*D).
Pre-runs executed at selected star (extreme) points verified that all expected analytes eluted within the 35 min run time. This mitigated the risk of any non-eluting peaks during the full DOE study, as a single unusable run may raise questions regarding the validity of the entire study. Based on the pre-runs, the concentration of the stock EDTA solution was decreased four-fold to mitigate inaccurate in-line mixing of mobile phase B caused by low volumes of a high concentration stock. The final ranges and levels for each of the four selected method parameters are also listed in Table 1 .
Each unique DOE run in Table 1 is a different method. As there were 25 unique running conditions, there were 25 different methods in this DOE study. The G-Efficiency and the average predicted variance (NIST/SEMATECH e-Handbook of statistical methods 2012a , 2012b , 2012c , 2012d ) (Myers and Montgomery 1995 ) of the design were 86.8% and 10.6%, respectively, meeting their respective design goals of ≥ 50% and ≤ 25%. Some of the major advantages of this modified CCC design include the following:
Established quadratic effects
Robust models that minimize effects of potential missing data
Good coverage of the design space by including the interior design points
Low predictive variances of the design points
Low model term coefficient estimation errors
The design also allows for implementation of a sequential approach, where trials from previously conducted factorial experiments can be augmented to form the CCC design. When there is little understanding about the method and critical method parameters, such as when developing a new method from scratch, direct application of an optimizing CCC design is generally not recommended. However, there was sufficient previous knowledge regarding this specific method, justifying the direct approach.
DOE data analysis and modeling principles
DOE software is one of the most important tools to facilitate efficient and effective AQbD chromatographic method development, validation, and transfer. Fusion QbD software was employed for DOE design and data analysis. Mathematical modeling of the physicochemical chromatographic separation process is essential for DOE to develop robust chromatographic methods through three phases: chemistry screening, mean optimization, and robustness optimization. The primary method parameters affecting separation (e.g., column packing, mobile phase pH, mobile phase organic modifier) are statistically determined with models during chemistry screening. The secondary method parameters affecting separation (e.g., column temperature, flow rate, gradient slope settings) are optimized during mean optimization using models to identify the method most capable of reaching all selected method response goals on average. During robustness optimization, robustness models for selected method responses are created with Monte Carlo simulation and used to further optimize method parameters such that all method responses consistently reach their goals, as reflected by a process capability value of ≥ 1.33, which is the established standard for a robust process (NIST/SEMATECH e-Handbook of statistical methods 2012a , 2012b , 2012c , 2012d ).
Models are critical to the AQbD approach and must be validated both statistically and scientifically. Statistical validation is performed using various statistical tests such as residual randomness and normality (NIST/SEMATECH e-Handbook of statistical methods 2012a , 2012b , 2012c , 2012d ), regression R-squared, adjusted regression R-squared, and prediction R-squared. Scientific validation is achieved by checking the terms in a statistical model against the relevant established scientific principles, which is described as mechanistic understanding in the relevant literature (ICH Q8 (R2) 2009 ).
Fusion uses data transformation analysis to decide whether data transformation is necessary before modeling, and then uses analysis of variance (ANOVA) and regression to generate method response models. ANOVA provides objective and statistical rationale for each consecutive modeling decision. Model residual plots are fundamental tools for validating the final method response models. When a model fits the DOE data well, the response residuals should be distributed randomly without any defined structure, and normally. A valid method response model provides the deepest understanding of how a method response, such as resolution, is affected by critical method parameters.
Since Fusion relies on models for chemistry screening, mean optimization, and robustness optimization, it is critical to holistically evaluate each method response model from all relevant model regression statistics to assure model validity before multiple method response optimization. Inappropriate models will lead to poor prediction and non-robust methods. This paper will describe the holistic evaluation approach used to develop a robust chromatographic method with Fusion QbD.
Representative chromatogram under nominal conditions
Careful planning and pre-runs executed at select star points allowed for successful execution of the DOE with all expected peaks eluting within the running time for all the 30 runs. A representative chromatogram at the nominal conditions is shown in Fig. 1 . The API peak (C1112299299-C) and the Epimer peak (C112299299-C-epimer) can be seen, as well as seven minor impurity peaks, among which impurity 2 and impurity 3 elute at 8.90 and 10.51 min, respectively. The inset shows the full-scale chromatogram.
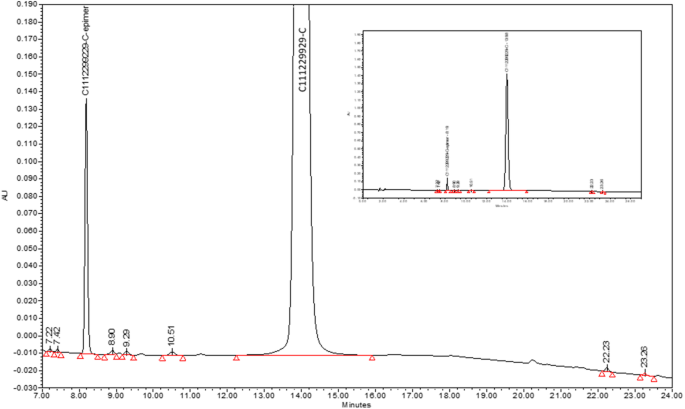
A representative chromatogram under nominal conditions
Results for statistical validation of the DOE models
ANOVA and regression data analysis revealed many DOE models for various peak responses. The major numeric regression statistics of the peak response models are summarized in Table 2 .
MSR (mean square regression), MSR adjusted, and MS-LOF (mean square lack of fit) are major numeric statistics for validating a DOE model. A model is statistically significant when the MSR ≥ the MSR significance threshold, which is the 0.0500 probability value for statistical significance. The lack of fit of a model is not statistically significant when the MS-LOF ≤ the MS-LOF significance threshold, which is also the 0.0500 probability value for statistical significance. The MSR adjusted statistic is the MSR adjusted with the number of terms in the model to assure a new term improves the model fit more than expected by chance alone. For a valid model, the MSR adjusted is always smaller than the MSR and the difference is usually very small, unless too many terms are used in the model or the sample size is too small.
Model Term Ranking Pareto Charts for scientific validation of DOE models
DOE models are calculated from standardized variable level settings. Scientific validation of a DOE model through mechanistic understanding can be challenging when data transformation before modeling ostensibly inverts the positive and negative nature of the model term effect. To overcome this challenge, Model Term Ranking Pareto Charts that provide the detailed effects of each term in a model were employed. See Fig. 2 for details.
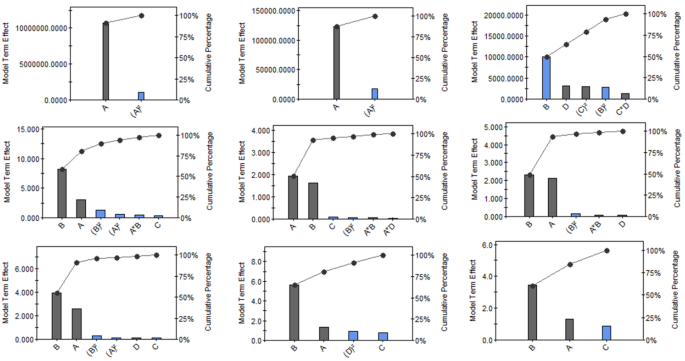
Model Term Ranking Pareto Charts. Top row from left to right: API area (default), Epimer area (default), API plate count. Middle row from left to right: API RT, Epimer RT, Impurity 2 RT. Bottom row from left to right: impurity 3 RT, # of peaks, # of peaks with ≥ 1.5 — resolution
The chart presents all terms of a model in descending order (left to right) based on the absolute magnitude of their effects. The primary y -axis (model term effect) gives the absolute magnitude of individual model terms, while the secondary y -axis (cumulative percentage) gives the cumulative relative percentage effects of all model terms. Blue bars correspond to terms with a positive effect, while gray bars correspond to those with a negative effect. The Model Term Ranking Pareto Charts for all models are summarized in Fig. 2 , except the two “customer” peak area models with a single term and the two C pk models.
AQbD relies on models for efficient and effective chemistry screening, mean optimization, and robustness optimization of chromatographic methods. It is critical to “validate” the models both statistically and scientifically, as inappropriate models may lead to impractical methods. As such, this section will discuss statistical and scientific validation of the DOE models. After the models were fully validated for all selected individual method responses, the method MODR was substantiated by balancing and compromising among the most important method responses.
Statistical validation of the DOE models
As shown in Table 2 , the MSR values ranged from 0.7928 to 0.9999. All MSR values were much higher than their respective MSR threshold, which ranged from 0.0006 to 0.0711, indicating that all models were statistically significant and explained the corresponding chromatographic response data. The MSR adjusted values were all smaller than their respective MSR values, and the differences between the two was always very small (the largest difference was 0.0195 for the API plate count model), indicating that there was no overfitting for the models. There was slight lack of fit for the two customer models due to very low pure errors, and the MS-LOF cannot be calculated for the two C pk model because the Monte Carlo simulation gives essentially zero pure error. Other than that, the MS-LOF ≤ the MS-LOF significance threshold for all other models, indicating the lack of fit was not statistically significant.
In addition to the above numeric statistical validation, various model residual plots were employed for graphical statistical model validation. The parameter–residual plots and the run number-residual plots for all models showed no defined structure, indicating random residual distribution. The normal probability plots showed all residual points lay in a nearly straight line for each single model, indicating normal residual distribution for all models. The randomly and normally distributed residuals provided the primary graphical statistical validation of the DOE models. See Fig. 3 for representative residuals plots for the “# of Peaks” model.
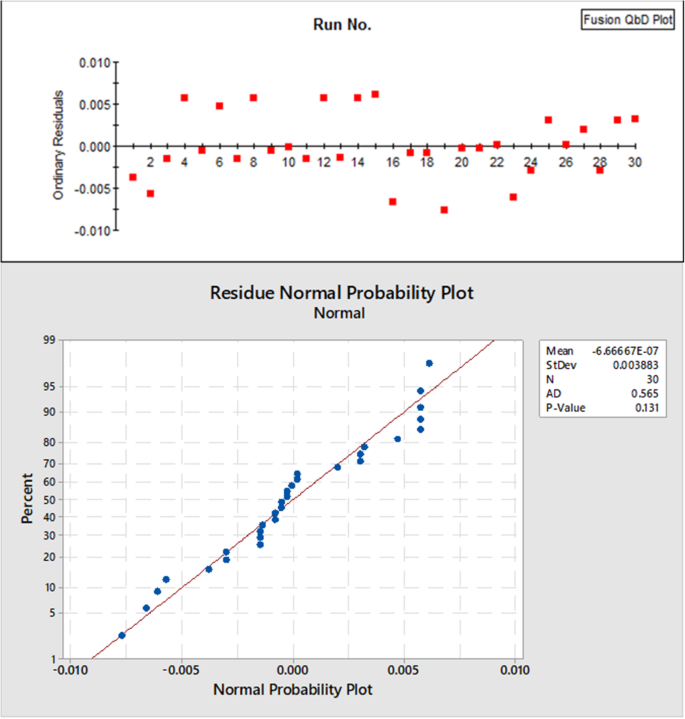
Representative residuals plot for the “# of Peaks” model. Upper: run no – residuals plot; lower: residues normal probability plot
Scientific validation of the DOE models
With all models statistically validated, the discussions below will focus on scientific validation of the models by mechanistic understanding.
Peak area models for API and Epimer peaks
Peak areas and peak heights have been used for chromatographic quantification. However, peak area was chosen as the preferred approach as it is less sensitive to peak distortions such as broadening, fronting, and tailing, which can cause significant variation in analyte quantitation. To use peak area to reliably quantify the analyte within the MODR of a robust chromatographic method, the peak area must remain stable with consistent analyte injections.
Peak area models can be critical to the method development and validation with multivariate DOE approach. Solid peak area models were revealed for the API and Epimer peaks in this study. See the “API (default)” and “Epimer (default)” rows in Table 2 for the detailed model regression statistics. See Fig. 2 for the Model Term Ranking Pareto Charts. See Eqs. 2 and 3 below for the detailed models.
Although a full quadratic model for the four-factor five-level CCC design has a total of fourteen potential terms, multivariate regression analyses revealed that only two of the fourteen terms are statistically significant for both the API and Epimer peak area models. In addition, the flow rate term and flow rate squared-terms are identical for the two models, indicating the other three parameters (final percentage strong solvent, oven temperature, and EDTA concentration) have no significant effect on peak area for both peaks.
Oven temperature and EDTA concentration have negligible effect on peak area and thus were not significant terms in the peak area models. The percentage of strong solvent was also not a significant term in the peak area models even though it did appear to influence peak height almost as much as flow rate, but not the peak area, as seen in Fig. 4 . It was hypothesized that the two flow rate terms in the model consisted of a strong negative first order term and a weak positive second order term, but more investigation was needed.
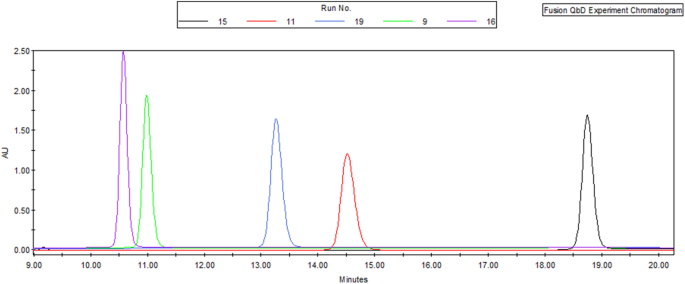
Effects of final percentage of strong solvent and flow rate on the API peak area and peak height: run 15 (black) = 31%/0.9 mL/min; run 11 (red) = 33%/1.0 mL/min; run 19 (blue) = 35%/0.9 mL/min; run 9 (green) = 37%/1.0 mL/min; run 16 (purple) = 39%/0.9 mL/min
Peak purity and peak integration are the primary factors affecting peak area. Partial or total peak overlap (resolution < 1.5) due to analyte co-elution can impact the peak purity resulting in inaccurate integration of both peaks. Peak integration may also be affected by unstable baseline and/or peak fronting and tailing due to uncertainty in determining peak start and end points. In this DOE study, the API and Epimer peaks were consistently well-resolved (resolution ≥ 2.0) and were also significantly higher than the limit of quantitation, contributing to the strong peak area models. In contrast, no appropriate peak area models could be developed for other impurity peaks as they were either not properly resolved or were too close to the limit of quantitation. For peaks with resolution ≤ 1.0 there will likely never be an area model with reliable predictivity as the peak area cannot be consistently and accurately measured.
The importance of a mechanistic understanding of the DOE models for AQbD has been extensively discussed. The API and Epimer peak area models were very similar in that they both contained a strong negative first order flow rate term and a weak positive second order flow rate term.
The strong negative first order term can be explained by the exposure time of the analyte molecules to the detector. The UV detector used in the LC method is non-destructive and concentration sensitive. Analyte molecules send signals to the detector when exposed to UV light while flowing through the fixed length detecting window in a band. As the molecules are not degraded by the UV light, the slower the flow rate, the longer the analyte molecules are exposed to the UV light, allowing for increased signal to the detector and thus increased analyte peak area. Simple direct linear regression of the peak area against inverse flow rate confirmed both the API and Epimer peak areas were proportional to the inverse flow rate, with R 2 values ≥ 0.99 (data not included).
As there was no obvious mechanistic explanation of the weak positive second order term in the models, more investigation was needed. Multivariate DOE customer models were pursued. The acquired customer models, listed in Eqs. 4 and 5 , used inverse flow rate “1/A” in place of the flow rate “A” for all pertinent terms among the fourteen terms. The major model regression statistics of the customer models are summarized in the “API (customer)” and “Epimer (customer)” rows in Table 2 . Both customer models contain a single inverse flow rate term, confirming the negative effect of flow rate on peak area for both peaks. The customer models in Eqs. 4 and 5 provide more intuitive understanding of the flow rate effects on peak area than the “default” models in Eqs. 2 and 3 . The weak positive second order flow rate term in Eqs. 2 and 3 contributes less than 15% effect to the peak area and is very challenging to explain mechanistically. This kind of model term replacing technique may be of general value when using DOE to explore and discover new scientific theory, including new chromatographic theory.
Additionally, the peak area models in Eqs. 2 – 5 revealed that the pump flow rate must be very consistent among all injections during a quantitative chromatographic sequence. Currently, the best-in-industry flow rate precision for a binary UPLC pump is “< 0.05% RSD or < 0.01 min SD” (Thermo Fisher Scientific, Vanquish Pump Specification. 2021 ).
API peak plate count model
Column plate count is potentially useful in DOE modeling as it is a key parameter used in all modes of chromatography for measuring and controlling column efficiency to assure separation of the analytes. The equation for plate count ( N ) is shown below. It is calculated using peak retention time ( t r ) and peak width at half height ( w 1/2 ) to mitigate any baseline effects and provide a more reliable response for modeling-based QbD chromatographic method development.
The peak plate count model for the API peak can be seen in Eq. 6 . It was developed by reducing the fourteen terms. The major model quality attributes are summarized in Table 2 .
The flow rate was not a critical factor in the plate count model. This seemingly goes against the Van Deemter equation (van Deemter et al. 1956 ), which states that flow rate directly affects column plate height and thus plate count. However, the missing flow rate term can be rationalized by the LC column that was used. According to the Van Deemter equation, plate height for the 150 × 4.6 mm, 3.5 μm column will remain flat at a minimum level within the 0.7–1.1 mL/min flow rate range used in this DOE study (Altiero 2018 ). As plate count is inversely proportional to the plate height, it will also remain flat at a maximal level within the 0.7–1.1 mL/min flow rate range.
The most dominating parameter in the API plate count model was the final percentage of strong solvent. Its two terms B and B 2 provided more than 60% positive effects to the plate count response (see the Model Term Ranking Pareto Chart in Fig. 2 ) and could be easily explained by the inverse relationship between plate count and peak width when the gradient slope is increased.
Retention time models
Retention time (RT) and peak width are the primary attributes for a chromatographic peak. They are used to calculate secondary attributes such as resolution, plate count, and tailing. These peak attributes together define the overall quality of separation and subsequently quantification of the analytes. RT is determined using all data points on a peak and is thus a more reliable measurand than peak width, which uses only some data points on a peak. As such, peak width cannot provide the same level of RT accuracy, especially for minor peaks, due to uncertainty in the determination of peak start, end, and height. Consequently, RT is the most reliably measured peak attribute.
The reliability of the RT measurement was confirmed in this DOE study. As listed in Table 2 , well-fitted RT models were acquired for the major API and Epimer peaks as well as the minor impurity 2 and impurity 3 peaks. The retention time models are listed in Eqs. 7 – 10 ( note : reciprocal square for the Epimer and impurity 2, and reciprocal for impurity 3 retention time data transformation before modeling inverted the positive and negative nature of the model term effect in Eqs. 8 – 10 , see the Model Term Ranking Pareto Charts in Fig. 2 for the actual effect). The four models shared three common terms: flow rate, final percentage of strong solvent, and the square of final percentage of strong solvent. These three terms contributed more than 90% of the effect in all four RT models. Furthermore, in all four models the flow rate and final percentage of strong solvent terms consistently produced a negative effect on RT, whereas the square of the final percentage of strong solvent term consistently produced positive effects. While the scientific rationale for the negative effects of the first two terms is well-established, the rationale for the positive effects of the third term lies beyond the scope of this study.
As RT is typically the most reliable measured peak response, therefore, it produces most reliable models. One potential shortcoming of RT modeling-based method optimization is that the resolution of two neighboring peaks is not only affected by the retention time, but also by peak width and peak shape, such as peak fronting and tailing.
Peak number models
A representative analytical sample is critical for AQbD to use DOE to develop a chromatographic method capable of resolving all potential related substances. Multivariate DOE chromatography of a forced degradation sample may contain many minor peaks, which may elute in different orders across the different runs of the study, making tracking of the individual peaks nearly impossible. One way to solve this problem is to focus on the number of peaks observed, instead of tracking of individual peaks. Furthermore, to avoid an impractical method with too many partially resolved peaks, the number of peaks with ≥ 1.5 resolution could be an alternative response for modeling.
Excellent models were acquired for both the number of peak responses and the number of peaks with ≥ 1.5 resolution in this DOE study. See Table 2 for the major model statistics, Fig. 2 for the Model Term Pareto Ranking Chart, and Eqs. 11 and 12 for the detailed models ( note : reciprocal square data transformation before modeling reversed the positive and negative nature of the model term effect in Eqs. 11 – 12 ; see the Model Term Ranking Pareto Charts in Fig. 2 for the actual effect). Of the 14 terms, only four were statistically significant for the peak number model and only three were statistically significant for the resolved peak number model. Additionally, it is notable that the two models share three common terms (final percentage of strong solvent ( B ), flow rate ( A ), and oven temperature ( C )) and the orders of impact for the three terms is maintained as ( B ) > ( A ) > ( C ), as seen in the Model Term Ranking Pareto Chart. The models indicated that within the evaluated ranges the final percentage of strong solvent and flow rate have negative effects on the overall separation, while column temperature has a positive effect. These observations align well with chromatographic scientific principles.
Challenges and solutions to peak resolution modeling
No appropriate model was found for the API peak resolution response in this study, possibly due to very high pure experimental error (34.2%) based on the replication runs. With this elevated level of resolution measurement error, only large effects of the experiment variables would be discernable from an analysis of the resolution data. There are many potential reasons for the high pure experimental error: (1) error in the resolution value determination in each DOE run, especially with small peak size or tailing of the reference impurity peaks; (2) the use of different reference peaks to calculate the resolution when elution order shifts between DOE runs; (3) the column is not sufficiently re-equilibrated between different conditions (note: Mention of column equilibration was hypothetical in this case and only to stress the importance of column conditioning during DOE in general. As Fusion QbD automatically inserts conditioning runs into the DOE sequence where needed, this was not found to be an issue in this case study). The respective solutions to overcome these challenges are (1) when reference materials are available, make a synthetic method-development sample composed of each analyte at concentrations at least ten times the limit of quantitation; (2) keep the concentration of analytes in the synthetic sample at distinguishably different levels so that the peaks can be tracked by size; and (3) allow enough time for the column to be sufficiently re-equilibrated between different conditions.
Method robustness evaluation and optimization by Monte Carlo simulation
The robustness of a method is a measure of its capacity to remain unaffected by small but deliberate variations in method parameters. It provides an indication of the method’s reliability during normal usage. Robustness was demonstrated for critical method responses by running system suitability checks, in which selected method parameters were changed one factor at a time. In comparison, the AQbD approach quantifies method robustness with process robustness indices, such as C P and C pk , through multivariate robustness DOE, in which critical method parameters are systematically varied, simultaneously. Process robustness indices are standard statistical process control matrices widely used to quantify and evaluate process and product variations. In this AQbD case study, method capability indices were calculated to compare the variability of a chromatographic method response to its specification limits. The comparison is made by forming the ratio between the spread of the response specifications and the spread of the response values, as measured by six times standard deviation of the response. The spread of the response values is acquired through tens of thousands of virtual Monte Carlo simulation runs of the corresponding response model, with all critical method parameters varied around their setting points randomly and simultaneously according to specified distributions. A method with a process capability of ≥ 1.33 is considered robust as it will only fail to meet the response specifications 63 times out of a million runs and thus is capable of providing much more reliable measurements for informed decisions on drug development, manufacturing, and quality control. Due to its intrinsic advantages over the OFAT approach, multivariate DOE robustness evaluation was recommended to replace the OFAT approach in the latest regulatory guidelines (FDA Guidance for industry-analytical procedures and methods validation for drugs and biologics. 2015 ).
In this DOE study, solid C pk models were produced for the “API Plate Count” and “Number of Peaks ≥ 1.5 USP Resolution”. See Table 2 for the detailed model regression statistics.
Multiple responses method optimization
Once models have been established for selected individual method responses, overall method evaluation and optimization can be performed. This is usually substantiated by balancing and compromising among multiple method responses. Three principles must be followed in selecting method responses to be included in the final optimization: (1) the selected response is critical to achieve the goal (see Table 4 ); (2) a response is included only when its model is of sufficiently high quality to meet the goals of validation; and (3) the total number of responses included should be kept to a minimum.
Following the above three principles, five method responses were selected for the overall method evaluation and optimization. Best overall answer search identified a new optimized method when the four critical method parameters were set at the specific values as listed in Table 3 . The cumulative desirability for the five desired method response goals reached the maximum value of 1.0. The desirability for each individual goal also reached the maximum value of 1.0, as listed in Table 4 .
Method Operable Design Region (MODR)
The critical method parameter settings in Table 3 define a single method that can simultaneously fulfill all five targeted method goals listed in Table 4 to the best extent possible. However, the actual operational values of the four critical parameters may drift around their set points during routine method executions. Based on the models, contour plots for method response can be created to reveal how the response value changes as the method parameters drift. Furthermore, overlaying the contour plots of all selected method responses reveal the MODR, as shown in Figs. 4 , 5 , and 6 . Note that for each response, a single unique color is used to shade the region of the graph where the response fails the criteria; thus, criteria for all responses are met in the unshaded area.
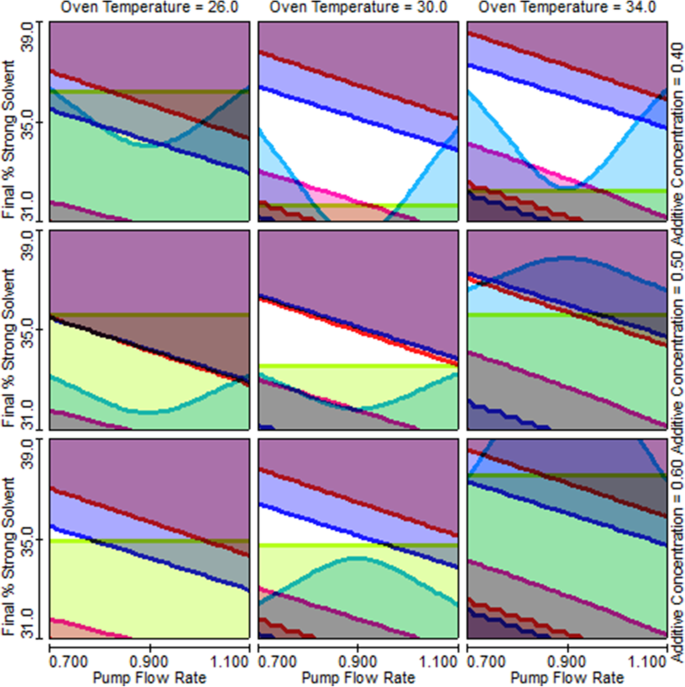
Trellis overlay graph shows how the size of the MODR (unshaded area) changes as the four method parameters change
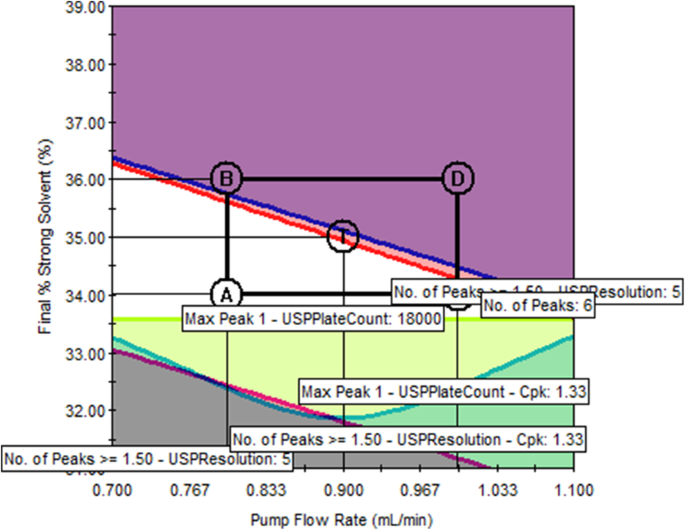
Single overlay graph shows the original as-is method at point T is not robust (pump flow rate = 0.90 mL/min; final % strong solvent = 35%; oven temperature = 30 °C; EDTA concentration = 0.50 mM)
The Trellis overlay graph in Fig. 5 reveals the MODR from the perspectives of all four critical method parameters, among which flow rate and final percentage of strong solvent change continuously while oven temperature and EDTA additive concentration were each set at three different levels. Figure 5 clearly demonstrates how the size of the MODR changes with the four method parameters. The single overlay graph in Fig. 6 shows that the original as-is method (represented by the center point T) is on the edge of failure for two method responses, number of peaks (red) and number of peaks ≥ 1.5 resolution (blue), indicating that the original method is not robust. Conversely, point T in the single overlay graph in Fig. 7 is at the center of a relatively large unshaded area, indicating that the method is much more robust than the original method.
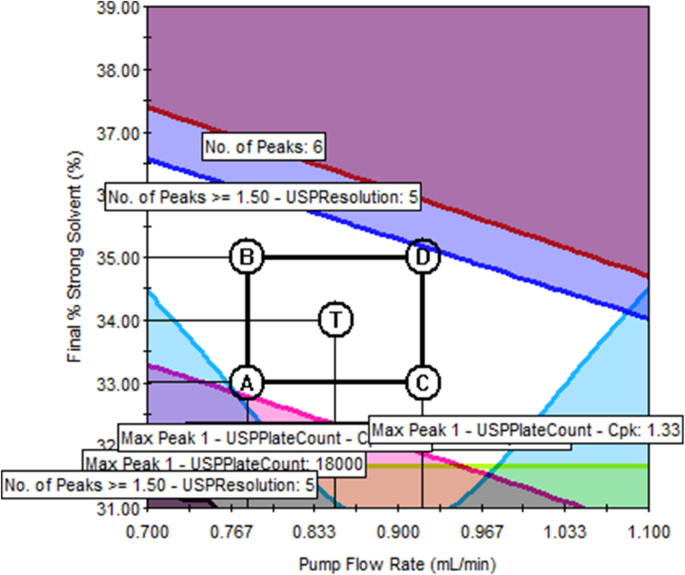
Single overlay graph shows a much more robust method at point T (pump flow rate = 0.78 mL/min; final % strong solvent = 34.2%; oven temperature = 30.8 °C; EDTA concentration = 0.42 mM)
Through the collaboration of regulatory authorities and the industry, AQbD is the new paradigm to develop robust chromatographic methods in the pharmaceutical industry. It uses a systematic approach to understand and control variability and build robustness into chromatographic methods. This ensures that analytical results are always close to the product true value and meet the target measurement uncertainty, thus enabling informed decisions on drug development, manufacturing, and quality control.
Multivariate DOE modeling plays an essential role in AQbD and has the potential to elevate chromatographic methods to a robustness level rarely achievable via the traditional OFAT approach. However, as demonstrated in this case study, chromatography science was still the foundation for prioritizing method inputs and responses for the most appropriate DOE design and modeling, and provided further scientific validation to the statistically validated DOE models. Once models were fully validated for all selected individual method responses, the MODR was substantiated by balancing and compromising among the most important method responses.
Developing a MODR is critical for labs that transfer in externally sourced chromatographic methods. In this case study, method evaluation using AQbD produced objective data that enabled a deeper understanding of method variability, upon which a more robust method with a much larger MODR was proposed. The in-depth method variability understanding through AQbD also paved the way for establishing a much more effective method control strategy. Method development and validation from a multivariate data driven exercise led to better and more informed decisions regarding the suitability of the method.
Availability of data and materials
The datasets used and/or analyzed during the current study are available from the corresponding author on reasonable request.
Abbreviations
Analytical quality by design
Design of experiments
Circumscribed central composite
Method Operable Design Region
Quality by design
The International Council for Harmonization of Technical Requirements for Pharmaceuticals for Human Use
Critical quality attributes
Target measurement uncertainty
Analytical target profile
Analysis of variance
Ethylenediaminetetraacetic acid
Mean square regression
Mean square lack of fit
One factor at a time
Altiero, P. Why they matter, an introduction to chromatography equations. Slide 21. https://www.agilent.com/cs/library/eseminars/public/Agilent_Webinar_Why_They_Matter_An_Intro_Chromatography_Equations_Nov262018.pdf . Accessed 13 May 2021. (2018).
Bezerraa MA, , Ferreirab SLC, Novaesa CG, dos Santoset AMP, Valasquesal GS, da Mata Cerqueira UMF, et al. Simultaneous optimization of multiple responses and its application in Analytical Chemistry – a review. Talanta; 194: 941-959. (2019).
Article Google Scholar
Borman P, Chatfield M, Nethercote P, Thompson D, Truman K (2007) The application of quality by design to analytical methods. Pharma.l Technol 31(12):142–152 (n.d.)
Google Scholar
Chatterjee S, CMC Lead for QbD, ONDQA/CDER/FDA. Design space considerations, AAPS Annual Meeting,2012. (n.d.).
Debrus B, Guillarme D, Rudaz S (2013) Improved quality-by-design compliant methodology for method development in reversed-phase liquid chromatography. J Pharm Biomed Anal 84:215–223 (n.d.)
Article CAS Google Scholar
EURACHEM / CITAC. Setting and using target uncertainty in chemical measurement. 2015. (n.d.).
FDA Guidance for industry-analytical procedures and methods validation for drugs and biologics. 2015.
FDA pharmaceutical cGMPs for the 21st century — a risk-based approach. 2004. (n.d.).
Grangeia HB, Silvaa C, Simões SP, Reis MS (2020) Quality by design in pharmaceutical manufacturing: a systematic review of current status, challenges and future perspectives. Eur J Pharm Biopharm 147:19–37 (n.d.)
ICH Q10 - Pharmaceutical quality system. 2008. (n.d.).
ICH Q11 - Development and manufacturing of drug substances (chemical entities and biotechnological/biological entities). 2012. (n.d.).
ICH Q12 - Technical and regulatory considerations for pharmaceutical product lifecycle management. 2019. (n.d.).
ICH Q14 - Analytical procedure development and revision of Q2(R1) analytical validation - final concept paper. 2018. (n.d.).
ICH Q8 (R2) - Pharmaceutical development. 2009. (n.d.).
ICH Q9 - Quality risk management. 2005. (n.d.).
Leardi R (2009) Experimental design in chemistry: a tutorial. Anal. Chim. Acta 652(1–2):161–172 (n.d.)
Myers RH, Montgomery DC (1995) Response surface methodology: process and product optimization using designed experiments, 2nd edition. John Wiley & Sons, New York, pp 366–404 (n.d.)
NIST/SEMATECH e-Handbook of statistical methods. 2012a. http://www.itl.nist.gov/div898/handbook/ppc/section1/ppc133.htm . Accessed May 13, 2021. (n.d.).
NIST/SEMATECH e-Handbook of statistical methods. 2012b. https://www.itl.nist.gov/div898/handbook/pmc/section1/pmc16.htm . Accessed May 13, 2021. (n.d.).
NIST/SEMATECH e-Handbook of statistical methods. 2012c. https://www.itl.nist.gov/div898/handbook/pmd/section4/pmd44.htm . Accessed May 13, 2021. (n.d.).
NIST/SEMATECH e-Handbook of statistical methods. 2012d. https://www.itl.nist.gov/div898/handbook/pri/section5/pri52.htm . Accessed May 13, 2021. (n.d.).
Orlandini S, Pinzauti S, Furlanetto S (2013) Application of quality by design to the development of analytical separation methods. Anal Bioanal Chem 2:443–450 (n.d.)
Schweitzer M, Pohl M, Hanna-Brown M, Nethercote P, Borman P, Hansen P, Smith K et al (2010) Implications and opportunities for applying QbD principles to analytical measurements. Pharma. Technol 34(2):52–59 (n.d.)
CAS Google Scholar
Tang YB, FDA/CDER/ONDQA (2011) Quality by design approaches to analytical methods -- FDA perspective. AAPS, Washington DC (n.d.)
Thermo Fisher Scientific, Vanquish pump specification. 2021. https://assets.thermofisher.com/TFS-Assets/CMD/Specification-Sheets/ps-73056-vanquish-pumps-ps73056-en.pdf . Accessed May 22, 2021. (n.d.).
van Deemter JJ, Zuiderweg FJ, Klinkenberg A. Longitudinal diffusion and resistance to mass transfer as causes of non ideality in chromatography. 1956. (n.d.).
Volta e Sousa L, Gonçalves R, Menezes JC, Ramos A (2021) Analytical method lifecycle management in pharmaceutical industry: a review. AAPS PharmSciTech 22(3):128–141. https://doi.org/10.1208/s12249-021-01960-9 (n.d.)
Article PubMed Google Scholar
Download references
Acknowledgements
The authors would like to thank KBP Biosciences for reviewing and giving permission to publish this case study. They would also like to thank Thermo Fisher Scientific and S Matrix for the Fusion QbD software, Lynette Bueno Perez for solution preparations, Dr. Michael Goedecke for statistical review, and both Barry Gujral and Francis Vazquez for their overall support.
Not applicable; authors contributed case studies based on existing company knowledge and experience.
Author information
Authors and affiliations.
Thermo Fisher Scientific Inc., Durham, NC, USA
Yongzhi Dong, Zhimin Liu, Charles Li, Emily Pinter, Alan Potts, Tanya Tadey & William Weiser
You can also search for this author in PubMed Google Scholar
Contributions
YD designed the study and performed the data analysis. ZL was the primary scientist that executed the study. CL, EP, AP, TT, and WW contributed ideas and information to the study and reviewed and approved the manuscript. The authors read and approved the final manuscript.
Corresponding author
Correspondence to Yongzhi Dong .
Ethics declarations
Competing interests.
The authors declare that they have no competing interests.
Additional information
Publisher’s note.
Springer Nature remains neutral with regard to jurisdictional claims in published maps and institutional affiliations.
Rights and permissions
Open Access This article is licensed under a Creative Commons Attribution 4.0 International License, which permits use, sharing, adaptation, distribution and reproduction in any medium or format, as long as you give appropriate credit to the original author(s) and the source, provide a link to the Creative Commons licence, and indicate if changes were made. The images or other third party material in this article are included in the article's Creative Commons licence, unless indicated otherwise in a credit line to the material. If material is not included in the article's Creative Commons licence and your intended use is not permitted by statutory regulation or exceeds the permitted use, you will need to obtain permission directly from the copyright holder. To view a copy of this licence, visit http://creativecommons.org/licenses/by/4.0/ .
Reprints and permissions
About this article
Cite this article.
Dong, Y., Liu, Z., Li, C. et al. Perspectives in modeling and model validation during analytical quality by design chromatographic method evaluation: a case study. AAPS Open 7 , 3 (2021). https://doi.org/10.1186/s41120-021-00037-y
Download citation
Received : 27 May 2021
Accepted : 29 July 2021
Published : 01 September 2021
DOI : https://doi.org/10.1186/s41120-021-00037-y
Share this article
Anyone you share the following link with will be able to read this content:
Sorry, a shareable link is not currently available for this article.
Provided by the Springer Nature SharedIt content-sharing initiative
- Statistical model validation
- Scientific model validation
- Multiple responses optimization
A Step-by-Step Guide to Analytical Method Development and Validation
Method development and validation are essential components of drug development and chemistry manufacturing and controls (CMC). The goal of method development and validation is to ensure that the methods used to measure the identity, purity, potency, and stability of drugs are accurate, precise, and reliable. Analytical methods are critical tools for ensuring the quality, safety, and efficacy of pharmaceutical products in the drug development process. Analytical development services performed at Emery Pharma are outlined below.
Analytical Method Development Overview:
Analytical method development is the process of selecting and optimizing analytical methods to measure a specific attribute of a drug substance or drug product. This process involves a systematic approach to evaluating and selecting suitable methods that are sensitive, specific, and robust, and can be used to measure the target attribute within acceptable limits of accuracy and precision.
Method Validation Overview:
Method validation is the process of demonstrating that an analytical method is suitable for its intended use, and that it is capable of producing reliable and consistent results over time. The validation process involves a set of procedures and tests designed to evaluate the performance characteristics of the method.
Components of method validation include:
- Specificity
- Limit of detection (LOD)
- Limit of quantification (LOQ)
Depending on the attribute being assayed, we use state-of-the-art instrumentation such as HPLC (with UV-Vis/DAD, IR, CAD, etc. detectors), LC-MS, HRMS, MS/MS, GC-FID/MS, NMR, plate readers, etc.
At Emery Pharma, we follow a prescribed set of key steps per regulatory (FDA, EMA, etc.) guidance, as well as instructions from the International Council for Harmonisation of Technical Requirements for Pharmaceuticals for Human Use (ICH) for any analytical method development and validation.
Step 1: Define the Analytical Method Objectives
The first step in analytical method development and validation is to define the analytical method objectives, including the attribute to be measured, the acceptance criteria, and the intended use of the method. This step involves understanding the critical quality attributes (CQAs) of the drug product or drug substance and selecting appropriate analytical methods to measure them.
For example, impurity profile of a drug substance assessment require suitable HPLC-based methods for small molecules, whereas host cell proteins (impurity-equivalent) for a Biologic drug substance require ligand binding assays (LBAs) such as ELISA for overview, and LC-HRMS-based analysis for thorough understanding.
Step 2: Conduct a Literature Review
Next, a literature review is conducted to identify existing methods and establish a baseline for the method development process. This step involves reviewing scientific literature, regulatory guidance, and industry standards to determine the current state of the art and identify potential methods that may be suitable for the intended purpose.
At Emery Pharma, we have worked on and have existing programs on virtually all type of drug modalities, thus we have access to many validated internal methods to tap into as well.
Step 3: Develop a Method Plan
The next step is to develop a method plan that outlines the methodology, instrumentation, and experimental design for method development and validation. The plan includes the selection of suitable reference standards, the establishment of performance characteristics, and the development of protocols for analytical method validation.
Step 4: Optimize the Method
Next, the analytical method is optimized to ensure that it is sensitive, specific, and robust. This step involves evaluating various parameters, such as sample preparation, column selection, detector selection, mobile phase composition, and gradient conditions, to optimize the method performance.
Step 5: Validate the Method
The critical next step is to validate the analytical method to ensure that it meets the performance characteristics established in the method plan. This step involves evaluating the method's accuracy, precision, specificity, linearity, range, LOD, LOQ, ruggedness, and robustness.
Depending on the stage of development, validation may be performed under Research and Development (R&D), however, most Regulatory submissions require method validation be conducted per 21 CFR Part 58 on Good Laboratory Practices (GLP). To that end, Emery Pharma has an in-house Quality Assurance department that ensures compliance and can play host to regulators/auditors.
Step 6: (Optional) Transfer the Method
In some instances, e.g., clinical trials with multiple international sites, the validated method may need to be transferred to another qualified laboratory. We routinely help our Clients get several parallel sites up to speed on new validated methods, and support with training analysts on the method, documenting the method transfer process, and conducting ongoing monitoring and maintenance of the method.
Step 7: Sample Analysis
The final step of an analytical method development Validation process is developing a protocol and initiate sample analysis.
At Emery Pharma, depending on the stage of development, sample analysis is conducted under R&D or in compliance with 21 CFR Part 210 and 211 for current Good Manufacturing Procedures (cGMP). We boast an impressive array of qualified instrumentation that can be deployed for cGMP sample analysis, which is overseen by our Quality Assurance Director for compliance and proper reporting.
Let us be a part of your success story
Emery Pharma has decades of experience in analytical method development and validation. We strive to implement procedures that help to ensure new drugs are manufactured to the highest quality standards and are safe and effective for patient use.
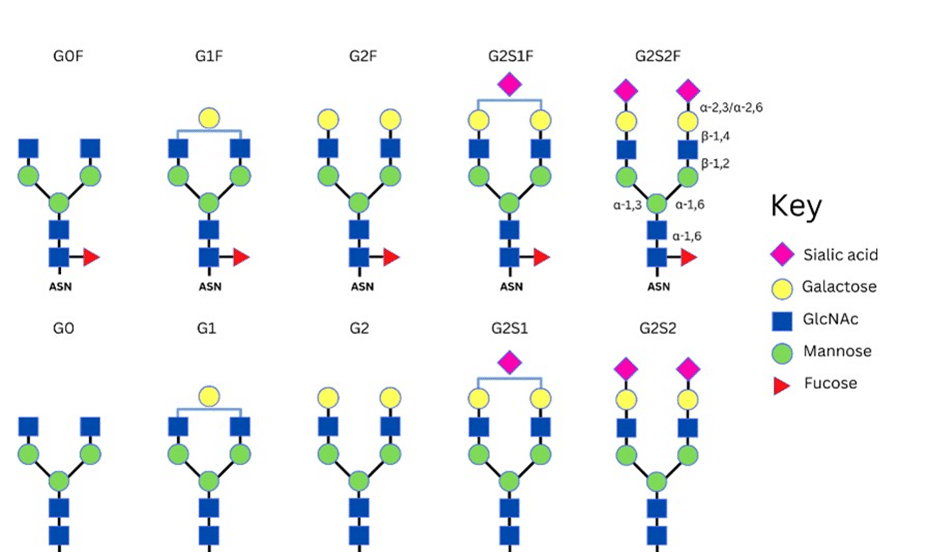
Emery Pharma
Request for proposal, let us be a part of your success story..
Do you have questions regarding a potential project? Or would you like to learn more about our services? Please reach out to a member of the Emery Pharma team via the contact form, and one of our experts will be in touch soon as possible. We look forward to working with you!
- My Shodhganga
- Receive email updates
- Edit Profile
Shodhganga : a reservoir of Indian theses @ INFLIBNET
- Shodhganga@INFLIBNET
- Jawaharlal Nehru Technological University, Anantapuram
- Department of Pharmaceutical Sciences
Items in Shodhganga are licensed under Creative Commons Licence Attribution-NonCommercial-ShareAlike 4.0 International (CC BY-NC-SA 4.0).

Thank you for visiting nature.com. You are using a browser version with limited support for CSS. To obtain the best experience, we recommend you use a more up to date browser (or turn off compatibility mode in Internet Explorer). In the meantime, to ensure continued support, we are displaying the site without styles and JavaScript.
- View all journals
- My Account Login
- Explore content
- About the journal
- Publish with us
- Sign up for alerts
- Open access
- Published: 10 May 2024
PermDroid a framework developed using proposed feature selection approach and machine learning techniques for Android malware detection
- Arvind Mahindru 1 ,
- Himani Arora 2 ,
- Abhinav Kumar 3 ,
- Sachin Kumar Gupta 4 , 5 ,
- Shubham Mahajan 6 ,
- Seifedine Kadry 6 , 7 , 8 , 9 &
- Jungeun Kim 10
Scientific Reports volume 14 , Article number: 10724 ( 2024 ) Cite this article
131 Accesses
1 Altmetric
Metrics details
- Electrical and electronic engineering
- Engineering
The challenge of developing an Android malware detection framework that can identify malware in real-world apps is difficult for academicians and researchers. The vulnerability lies in the permission model of Android. Therefore, it has attracted the attention of various researchers to develop an Android malware detection model using permission or a set of permissions. Academicians and researchers have used all extracted features in previous studies, resulting in overburdening while creating malware detection models. But, the effectiveness of the machine learning model depends on the relevant features, which help in reducing the value of misclassification errors and have excellent discriminative power. A feature selection framework is proposed in this research paper that helps in selecting the relevant features. In the first stage of the proposed framework, t -test, and univariate logistic regression are implemented on our collected feature data set to classify their capacity for detecting malware. Multivariate linear regression stepwise forward selection and correlation analysis are implemented in the second stage to evaluate the correctness of the features selected in the first stage. Furthermore, the resulting features are used as input in the development of malware detection models using three ensemble methods and a neural network with six different machine-learning algorithms. The developed models’ performance is compared using two performance parameters: F-measure and Accuracy. The experiment is performed by using half a million different Android apps. The empirical findings reveal that malware detection model developed using features selected by implementing proposed feature selection framework achieved higher detection rate as compared to the model developed using all extracted features data set. Further, when compared to previously developed frameworks or methodologies, the experimental results indicates that model developed in this study achieved an accuracy of 98.8%.
Similar content being viewed by others
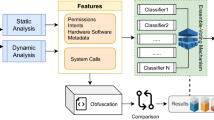
Evaluation and classification of obfuscated Android malware through deep learning using ensemble voting mechanism
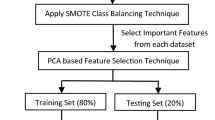
A study of dealing class imbalance problem with machine learning methods for code smell severity detection using PCA-based feature selection technique
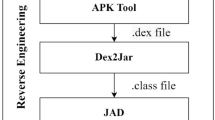
AndroMalPack: enhancing the ML-based malware classification by detection and removal of repacked apps for Android systems
Introduction.
Now-a-days, smartphones can do the same work as the computer has been doing. By the end of 2023, there will be around 6.64 billion smartphone users worldwide ( https://www.bankmycell.com/blog/how-many-phones-are-in-the-world ). According to the report ( https://www.statista.com/statistics/272307/market-share-forecast-for-smartphone-operating-systems/ ) at the end of 2023, Android operating systems captured 86.2% of the total segment. The main reason for its popularity is that its code is written in open source which attracts developers to develop Android apps on a daily basis. In addition to that it provides many valuable services such as process management, security configuration, and many more. The free apps that are provided in its official store are the second factor in its popularity. By the end of March 2023 data ( https://www.appbrain.com/stats/number-of-android-apps ), Android will have 2.6 billion apps in Google play store.
Nonetheless, the fame of the Android operating system has led to enormous security challenges. On the daily basis, cyber-criminals invent new malware apps and inject them into the Google Play store ( https://play.google.com/store?hl=en ) and third-party app stores. By using these malware-infected apps cyber-criminals steal sensitive information from the user’s phone and use that information for their own benefits. Google has developed the Google Bouncer ( https://krebsonsecurity.com/tag/google-bouncer/ ) and Google Play Protect ( https://www.android.com/play-protect/ ) for Android to deal with this unwanted malware, but both have failed to find out malware-infected apps 1 , 2 , 3 . According to the report published by Kaspersky Security Network, 6,463,414 mobile malware had been detected at the end of 2022 ( https://securelist.com/it-threat-evolution-in-q1-2022-mobile-statistics/106589/ ). Malware acts as a serious problem for the Android platform because it spreads through these apps. The challenging issue from the defender’s perspective is how to detect malware and enhance its performance. A traditional signature-based detection approach detects only the known malware whose definition is already known to it. Signature-based detection approaches are unable to detect unknown malware due to the limited amount of signatures present in its database. Hence, the solution is to develop a machine learning-based approach that dynamically learns the behavior of malware and helps humans in defending against malware attacks and enhancing mobile security.
Researchers and academicians have proposed different methods for analyzing and detecting malware from Android. Some of them have been proposed by using static analysis, for example, ANASTASIA 4 , DREBIN 5 , Droiddetector 6 and DroidDet 7 . On the other side, some researchers have proposed with the help of dynamic analysis, for example, IntelliDroid 8 , DroidScribe 9 , StormDroid 10 and MamaDroid 11 . But, the main constraints of these approaches are present in its implementation and time consumption because these models are developed with a number of features. On the other side, academicians and researchers 3 , 12 , 13 , 14 , 15 , 16 , 17 , 18 , 19 also proposed malware detection frameworks that are developed by using relevant features. But, they have restrictions too. They implemented only already proposed feature selection techniques in their work.
So, in this research paper, to overcome the hindrances a feature selection framework is proposed. This helps in the evaluation of appropriate feature sets with the goal of removing redundant features and enhances the effectiveness of the machine-learning trained model. Further, by selecting a significant features a framework named PermDroid is developed. The proposed framework is based on the principle of artificial neural network with six different machine learning techniques, i.e., Gradient descent with momentum (GDM), Gradient descent method with adaptive learning rate (GDA), Levenberg Marquardt (LM), Quasi-Newton (NM), Gradient descent (GD), and Deep Neural Network (DNN). These machine learning algorithms are considered on the basis of their performance in the literature 20 . In addition to this, three different ensemble techniques with three dissimilar combination rules are proposed in this research work to develop an effective malware detection framework. F-measure and Accuracy have been considered as performance parameters to evaluate the performance. From the literature review 21 , 22 , 23 , it is noticed that a number of authors have concentrated on bettering the functioning of the malware detection models. However, their study had a key flaw, they only used a small amount of data to develop and test the model. In order to address this issue, this study report takes into account 500,000 unique Android apps from various categories.

Steps are followed in developing Android malware detection framework.
The method for developing a reliable malware detection model is represented in Fig. 1 . The initial collection of Android application packages (.apk) comes from a variety of promised repositories (mentioned in “ Creation of experimental data set and extraction of features ” section). Anti-virus software is used to identify the class of .apk files at the next level (mentioned in “ Creation of experimental data set and extraction of features ” section). Then, features (such as API calls and permissions) are retrieved from the .apk file using various techniques described in the literature (mentioned in subsection 3.4). Additionally, a feature selection framework is applied to evaluate the extracted features (discussed in “ Proposed feature selection validation method ” section). Then, a model is developed using an artificial neural network using six different machine-learning techniques and three different ensemble models, employing the selected feature sets as input. Finally, F-measure and Accuracy are taken into consideration while evaluating the developed models. The following are the novel and distinctive contributions of this paper:
In this study, to develop efficient malware detection model half a million unique apps have been collected from different resources. Further, unique features are extracted by performing dynamic analysis in this study.
The methodology presented in this paper, is based on feature selection methodologies, which contributes in determining the significant features that are utilized to develop malware detection models.
In this study, we proposed three different ensemble techniques that are based on the principle of a heterogeneous approach.
Six different machine learning algorithms that are based on the principle of Artificial Neural Network (ANN) are trained by using relevant features.
When compared to previously developed frameworks and different anti-virus software in the market, the proposed Android malware detection framework can detect malware-infected apps in less time.
A cost-benefit analysis shows that the proposed Android malware detection framework is more effective in identifying malware-infected apps from the real world.
The remaining sections of this research paper are arranged as follows: “ Related work ” section presents the literature survey on Android malware detection as well as the creation of research questions. “ Research methodology ” section gives an overview of the research methodology used to create the Android malware detection framework. Different machine learning and ensemble techniques are addressed in “ Machine learning technique ” section. The proposed feature selection validation technique is discussed in “Proposed feature selection validation method” section. The experimental results are presented in “ Experimental setup and results ” section. Threats to validity are presented in “ Threats to validity ” section. Conclusion and the future scope are discussed in “ Conclusion and future work ” section.
Related work
The exploitation of the vulnerability is common these days to acquire higher privilege on Android platforms. Since 2008, cybercriminals have started targeting Android devices. An exploit app, from the perspective of Android security, can assist cyber-criminals in bypassing security mechanisms and gaining more access to users’ devices. Cybercriminals may exploit user data by selling their personal information for monetary gain if they took advantage of these privileges. The detection process, which has been used by researchers in the past and is based on Artificial Neural Networks (ANN) and feature selection techniques, is addressed in this subsection.
Androguard ( https://code.google.com/archive/p/androguard/ ) is a static analysis tool that detects malware on Android devices using the signature concept. Only malware that is already known to be present and whose definition is in the Androguard database is identified. It cannot, however, identify unidentified malware. Andromaly 23 , is developed on a dynamic analysis tool that uses a machine learning technique. It monitored CPU utilization, data transfer, the number of effective processes, and battery usage in real-time. The test was carried out on a few different types of simulated malware samples, but not on the applications that are present in the real-world. By using the semantics of the code in the form of code graphs collected from Android apps, Badhani et al. 24 developed malware detection methodology. Faruki et al. 21 introduced AndroSimilar, which is based on the principles of generated signatures that are developed from the extracted features, which are used to develop malware detection model.
Aurasium 25 takes control of an app’s execution by examining arbitrary security rules in real-time. It repackages Android apps with security policy codes and informs users of any privacy breaches. Aurasium has the problem of not being able to detect malicious behavior if an app’s signature changes. They performed dynamic analysis of Android apps and considered call-centric as a feature. The authors tested their method on over 2900 Android malware samples and found that it is effective at detecting malware activity. A web-based malware evaluation method has been proposed by Andrubis 26 , it operates on the premise that users can submit apps via a web service, and after examining their activity, it returns information on whether the app is benign or malicious. Ikram et al. 27 suggested an approach named as DaDiDroid based on weighted directed graphs of API calls to detect malware-infected apps. The experiment was carried out with 43,262 benign and 20,431 malware-infected apps, achieving a 91% accuracy rate. Shen et al. 28 developed an Android malware detection technique based on the information flow analysis principle. They implement N-gram analysis to determine common and unique behavioral patterns present in the complex flow. The experiment was carried out on 8,598 different Android apps with an accuracy of 82.0 percent. Yang et al. 29 proposed an approach named EnMobile that is based on the principle of entity characterization of the behavior of the Android app. The experiment was carried out on 6,614 different Android apps, and the empirical results show that their proposed approach outperformed four state-of-the-art approaches, namely Drebin, Apposcopy, AppContext, and MUDFLOW, in terms of recall and precision.
CrowDroid 34 , which is built using a behavior-based malware detection method, comprises of two components: a remote server and a crowdsourcing app that must both be installed on users’ mobile devices. CrowDroid uses a crowdsourcing app to send behavioral data to a remote server in the form of a log file. Further, they implemented 2-mean clustering approach to identify that the app belongs to malicious or benign class. But, the crowDroid app constantly depletes the device’s resources. Yuan et al. 52 proposed a machine learning approach named Droid-Sec that used 200 extracted static and dynamic features for developing the Android malware detection model. The empirical result suggests that the model built by using the deep learning technique achieved a 96% accuracy rate. TaintDroid 30 tracks privacy-sensitive data leakage in Android apps from third-party developers. Every time any sensitive data leaves the smartphone, TaintDroid records the label of the data, the app that linked with the data, as well as the data’s destination address.
Zhang et al. 53 proposed a malware detection technique based on the weighted contextual API dependency graph principle. An experiment was performed on 13500 benign samples and 2200 malware samples and achieved an acceptable false-positive rate of 5.15% for a vetting purpose.
AndroTaint 54 works on the principle of dynamic analysis. The features extracted were used to classify the Android app as dangerous, harmful, benign, or aggressive using a novel unsupervised and supervised anomaly detection method. Researchers have used numerous classification methods in the past, like Random forest 55 , J48 55 , Simple logistic 55 , Naïve Bayes 55 , Support Vector Machine 56 , 57 , K-star 55 , Decision tree 23 , Logistic regression 23 and k-means 23 to identify Android malware with a better percentage of accuracy. DroidDetector 6 , Droid-Sec 52 , and Deep4MalDroid 58 work on the convention of deep learning for identifying Android malware. Table 1 summarizes some of the existing malware detection frameworks for Android.
The artificial neural network (ANN) technique is used to identify malware on Android devices
Nix and Zhang 59 developed a deep learning algorithm by using a convolution neural network (CNN) and used API calls as a feature. They utilized the principle of Long Short-Term Memory (LSTM) and joined knowledge from its sequences. McLaughlin et al. 60 , implemented deep learning by using CNN and considered raw opcode as a feature to identify malware from real-world Android apps. Recently, researchers 6 , 58 used network parameters to identify malware-infected apps. Nauman et al. 61 , implemented connected, recurrent, and convolutional neural networks, and they also implemented DBN (Deep Belief Networks) to identify malware-infected apps from Android. Xiao et al. 62 , presented a technique that was based on the back-propagation of the neural networks on Markov chains and considered the system calls as a feature. They consider the system call sequence as a homogenous stationary Markov chain and employed a neural network to detect malware-infected apps. Martinelli et al. 63 , implemented a deep learning algorithm using CNN and consider the system call as a feature. They performed an experiment on a collection of 7100 real-world Android apps and identify that 3000 apps belong to distinct malware families. Xiao et al. 64 , suggested an approach that depends on the principle of LSTM (Long Short-Term Memory) and considers the system call sequence as a feature. They trained two LSTM models by the system call sequences for both the benign and malware apps and then compute the similarity score. Dimjas̈evic et al. 65 , evaluate several techniques for detecting malware apps at the repository level. The techniques worked on the tracking of system calls at the time the app is running in a sandbox environment. They performed an experiment on 12,000 apps and able to identify 96% malware-infected apps.
Using feature selection approaches, to detect Android malware
Table 2 shows the literature review for malware detection done by implementing feature selection techniques. Mas’ud et al. 66 proposed a functional solution to detect malware from the smartphone and can address the limitation of the environment of the mobile device. They implemented chi-square and information gain as feature selection techniques to select the best features from the extracted dataset. Further, with the help of selected best features, they employed K-Nearest Neighbour (KNN), Naïve Bayes (NB), Decision Tree (J48), Random Forest (RF), and Multi-Layer Perceptron (MLP) techniques to identify malware-infected apps. Mahindru and Sangal 3 developed a framework that works on the basis of feature selection approaches and used distinct semi-supervised, unsupervised, supervised, and ensemble techniques parallelly and identify 98.8% malware-infected apps. Yerima et al. 67 suggested an effective technique to detect malware from smartphones. They implemented mutual information as a feature selection approach to select the best features from the collected code and app characteristics that indicate the malicious activities of the app. To detect malware apps, from the wild, they trained selected features by using Bayesian classification and achieved an accuracy of 92.1%. Mahindru and Sangal 15 suggested a framework named as “PerbDroid” that is build by considering feature selection approaches and deep learning as a machine classifier. 2,00,000 Android apps in total were subjected to tests, with a detection rate of 97.8%. Andromaly 23 worked on the principle of the Host-based Malware Detection System that monitors features related to memory, hardware, and power events. After selecting the best features by implementing feature selection techniques, they employed distinct classification algorithms such as decision tree (J48), K-Means, Bayesian network, Histogram or Logistic Regression, Naïve Bayes (NB) to detect malware-infected apps. Authors 14 suggested a malware detection model based on semi-supervised machine learning approaches. They examined the proposed method on over 200,000 Android apps and found it to be 97.8% accurate. Narudin et al. 68 proposed a malware detection approach by considering network traffic as a feature. Further, they applied random forest, multi-layer perceptron, K-Nearest Neighbor (KNN), J48, and Bayes network machine learning classifiers out of which the K-Nearest Neighbor classifier attained an 84.57% true-positive rate for detection of the latest Android malware. Wang et al. 69 employed three different feature ranking techniques, i.e., t -test, mutual information, and correlation coefficient on 3,10,926, benign, and 4,868 malware apps using permission and detect 74.03% unknown malware. Previous researchers implement feature ranking approaches to select significant sets of features only. Authors 13 developed a framework named as “DeepDroid” based on deep learning algorithm. They use six different feature ranking algorithms on the extracted features dataset to select significant features. The tests involved 20,000 malware-infected apps and 100,000 benign ones. The detection rate of a framework proposed using Principal component analysis (PCA) was 94%. Researchers and Academicians 70 , 71 , 72 , 73 also implemented features selection techniques in the literature in different fields to select significant features for developing the models.
Research questions
To identify malware-infected apps and considering the gaps that are present in the literature following research questions are addressed in this research work:
RQ1 Does the filtering approach helps to identify that whether an app is a benign or malware-infected (first phase of the proposed feature selection framework)? To determine the statistical significance among malicious and benign apps, the t -test is used. After, determining significant features, a binary ULR investigation is applied to select more appropriate features. For analysis, all the thirty different feature data sets are assigned (shown in Table 5 ) as null hypotheses.
RQ2 Do already existing and presented work’s sets of features show an immense correlation with each other? To answer this question, both positive and negative correlations are examined to analyze the sets of features, which help in improving the detection rate.
RQ3 Can the identified features assist in determining whether the app is malware-infected or not? The primary objective of this question is to use the feature selection framework validation approach to determine the appropriate features. In this paper, four stages (i.e., ULR, t-test, Correlation analysis, and multivariate linear regression stepwise forward selection) are implemented to identify the appropriate features, that helps in identifying whether an app contains malicious behavior or not.
RQ4 Which classification algorithm among the implemented machine learning algorithms is most appropriate for identifying malware-infected apps? To answer to this question the efficiency of various machine learning approaches are evaluated. In this study, three different ensemble approaches and six different machine learning algorithms based on neural networks are considered.
RQ5 Is the feature collected (such as an app’s rating, API calls, permissions, and the number of people who have downloaded the app) sufficient for identifying a malicious app or not? This question helps in determining whether or not considering features can detect malware-infected apps in the real world. To answer this question, the performance of our suggested model is compared with previously published frameworks as well as several anti-virus scanners in the market.
Research methodology
Based on the research questions mentioned above, the methodology that is used in this research paper is mentioned in the following subsections. In order to improve the detection rate for malware, the obtained data set has been normalized, and dependent and independent variables have been selected.
Independent variables
In this study, the model is developed by applying the proposed feature selection approach, which helps in the detection of malware-infected apps. Additionally, as shown in Fig. 2 , five different strategies to select the best features are used. The best features are selected from other accessible features created on intermediate explore models at each level.
Dependent variables
The focus of this research is to find a link between Android apps and the features (such as app rating, API calls, permission, and the number of users who have downloaded an app) retrieved from the collected data set. The malware app characteristics are separated from the benign app features in the dependent variable of Android apps.
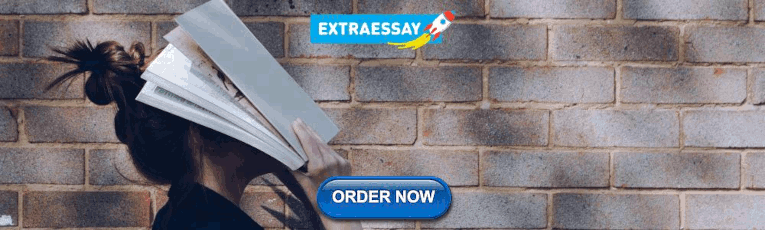
Creation of experimental data set and extraction of features
In this research paper, 70,000 .apk files from Google play store ( https://play.google.com/store?hl=en ), and more than 3 lacs .apk files from third-party app store i.e., Softonic ( https://en.softonic.com/android ), Android Authority ( https://www.androidauthority.com/apps/ ), CNET ( https://download.cnet.com/android/ ) belong to
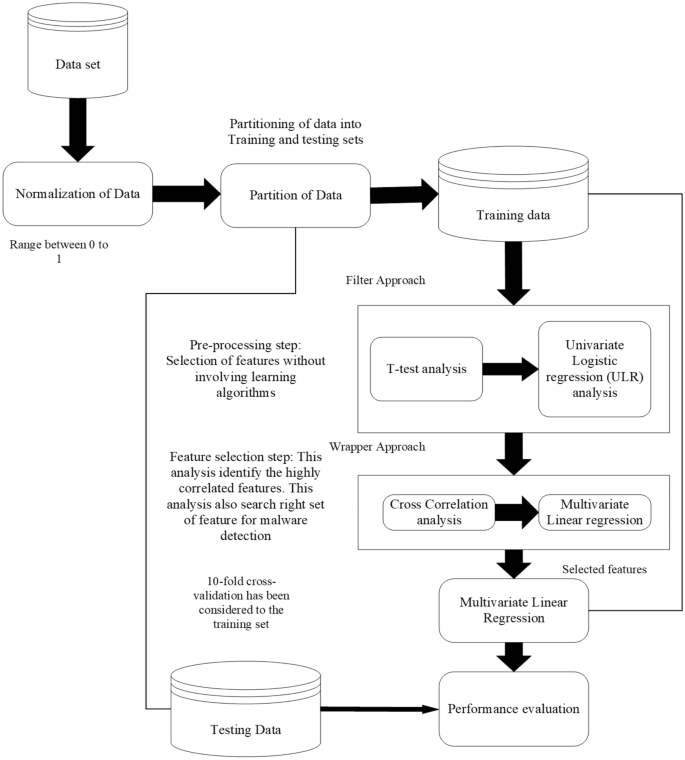
Proposed framework for feature selection and its validation.
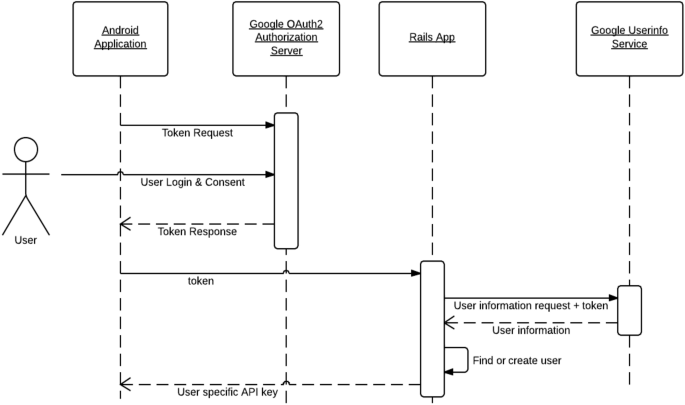
Sequence diagram showing reservation using Android app.
benign group and 70,000 malware-infected Android apps from 79 , 80 , 81 and Sanddroid ( http://sanddroid.xjtu.edu.cn:8080/ ) belongs to malicious group are collected to develop an effective malware detection framework. As seen in Table 3 , the .apk files we collected fall under thirty different categories. Collected malware-infected apps belong to ten different malware categories: AD (Adware), BA (Backdoor), HT (Hacker Tool), RA (Ransom), TR (Trojan), TB (Trojan-Banker), TC (Trojan-Clicker), TD (Trojan-Dropper), TS (Trojan-SMS) and TSY (Trojan-Spy). Classes are identified by using two distinct scanners i.e., VirusTotal ( https://www.virustotal.com/gui/ ) and Microsoft Windows Defender ( https://windows-defender.en.softonic.com/download ) and on the basis of its behavior defined in the study 82 .
To formulate an efficient malware detection framework, we extract 310 API calls and 1419 unique permissions ( https://github.com/ArvindMahindru66/Computer-and-security-dataset ), by implementing the procedure mentioned in the literature 3 , 13 , 15 , 83 . If an app requests the permission and API call during installation or runtime, we mark it as “1”; otherwise, we mark it as “0”. The following are some of the features of a certain app that have been extracted:
0,1,1,1,1,0,0,0,0,0,1,1,1,1,1,1,1,1,1,1,1,1,1,1,1,1,
0,0,0,0,0,0,0,0,0,0,1,1,1,1,1,1,1,1,1,1,1,1,1,0,0,0,
1,1,1,1,1,1,1,1,1,1,1,1,1,1,1,1,1,1,1,1,1,1,1,1,1,1,
1,1,1,1,1,1,1,1,1,1,0,0,0,0,0,0,0,0,0,0,0,0,0,0,0,0, and so on.
After extracting API calls and permissions from the collected data set from .apk files, it is divided into thirty different features data sets (Mahindru, Arvind (2024), “Android Benign and Malware Dataset”, Mendeley Data, V1, doi: 10.17632/rvjptkrc34.1). Table 4 illustrates the creation of various feature data sets as well as their explanations. These extracted features are divided into different sets on the basis of its behavior to which it belongs 3 , 13 , 15 , 83 . The main reasons to divide these extracted features into thirty different feature data sets are: to select significant features by using the proposed feature selection framework and to remove the complexity.
Figure 3 demonstrate the sequence diagram of an Android app by showing the example of an railway reservation app. How the process is started and how it is interact with other APIs and the permissions that are running in the background (Table 5 ).
Machine learning technique
ANN stands for artificial neural networks, and it is a computing system based on biological neural networks. These are able to perform certain tasks by utilizing certain examples, without using task-specific rules. Researchers are implementing ANN to solve different problems in malware detection, pattern recognition, classification, optimization, and associative memory 84 . In this paper, ANN is implemented to create a malware detection model. The structure of the ANN model is shown in Fig. 4 . ANN contains input nodes, hidden nodes, and output nodes.
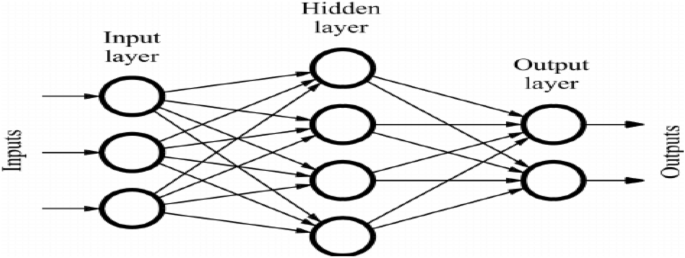
Artificial neural network.
The input layer employs a linear stimulation function, while the hidden and output layers employ squashed-S or sigmoidal functions. ANN can be presented as:
where B is the input vector, A is the weight vector and \(O^{'}\) denotes the desired output vector. In order to minimize the mean square error (MSE), the value of A is updated in each step. Mean square error can be calculated from the equation below:
Here, O is the actual output value, and \(O^{'}\) is the desired output value. Various methods were proposed by researchers 20 , 84 to train the neural network. In this research work, six different kinds of machine learning algorithms (namely, Gradient Descent approach, Quasi-Newton approach, Gradient Descent with Momentum approach, Levenber-Marquardt approach, Gradient Descent with Adaptive learning rate approach, and Deep neural network) are considered to develop malware detection model. These models are effective in the field of software fault prediction 20 , intrusion detection and desktop malware predictions 85 too.
Gradient descent with momentum approach
This approach accelerates the rate of convergence dramatically 20 , 84 . To obtain new weights, this approach combines the fraction diversity 20 , 84 , 86 . X is the updated weighed vector defined as:
where A denotes the momentum parameter value, \(X_k\) is the current weight vector and \(X_{k+1}\) is the update value of the weight vector and \((E_k)\) , used to identify the lower value in error space. Here, \(X_{k+1}\) relys on both the weight and the gradient. To determine the optimal value of A we implemented the cross-validation technique.
Gradient descent approach
This approach updates the weights to reduce the output error 20 , 84 , 86 . In Gradient descent (GD) approach, to identify the lower value in error space \((E_k)\) , the \(1^{st}\) - order derivative of the total error function is computed by considering, the following equation:
Redundancy weight vector X is modified by employing gradient vector G 20 , 84 , 86 . The up-dation of X is done through the following formula
where \(G_n\) is the gradient vector, \(O_{x+1}\) is the revised weight vector and \(\alpha\) is the gaining constant. To calculate the optimum value of \(\alpha\) , we implement cross-validation approach.
Gradient descent method with adaptive learning rate approach
In the GD approach, during training, the learning rate \((\alpha )\) remains stable. This approach is based on the concept that is quite perceptive to the approximation value of the learning rate. At the time of training, if the value of the learning rate is too high, the build model can be highly unstable and oscillate its value 20 . On the reverse of this, if the training value is too small, the procedure may take a long way to converge. Practically, it is not easy to find out the optimal value of \(\alpha\) before training. Actually, during the training process, the value of \(\alpha\) changes 20 . In each iteration, if the performance decline along with the required aim, the \(\alpha\) value is added by 1.05, and in reverse of this, if the performance increase by more than the factor of 1.04, then the \(\alpha\) value is incremented by 0.7 20 .
Levenberg Marquardt (LM) approach
The foundation of LM is an iterative technique that helps in locating the multivariate function’s minimal value. At the time of training, this value can be calculated as the sum of squares of real-valued with non-linera functions which helps in modifying the weights 20 , 87 . This method is quite stable and fast because it combines the Gauss Newton and the steepest descent approach. The iterative process for the same is given by
where \(X_{k+1}\) is the updated weight, \(X_k\) is the current weight, I is the identity matrix, \(\mu >0\) is named as combination coefficient and J is the Jacobian matrix. For a small value of \(\mu ,\) it becomes Gauss-Newton approach and for large, \(\mu ,\) it acts as GD approach. Representation of Jacobian matrix is : \(J=\) \(\begin{bmatrix} \frac{\partial E_{1,1}}{\partial X_1}&{} \frac{\partial E_{1,1}}{\partial X_2}&{}\cdots &{}\frac{\partial E_{1,1}}{\partial X_N}\\ \frac{\partial E_{1,2}}{\partial X_1}&{} \frac{\partial E_{1,2}}{\partial X_2}&{}\cdots &{}\frac{\partial E_{1,2}}{\partial X_N}\\ \vdots &{}\vdots &{}\vdots &{}\vdots \\ \frac{\partial E_{P,M}}{\partial X_1}&{} \frac{\partial E_{P,M}}{\partial X_2}&{}\cdots &{}\frac{\partial E_{P,M}}{\partial X_N}\\ \end{bmatrix}\) where P , N and M is the input patterns, weights and the output patterns.
Quasi-Newton approach
In order to compute the total error function, this approach requires the evaluation of the second order derivatives for each component of the gradient vector 20 , 84 . The iterative scheme for the Weight vector X is given as:
where \(X_k\) and \(X_{k+1}\) are the current and updated weight vectors, accordingly. H is the Hessian matrix given by \(H=\) \(\begin{bmatrix} \frac{\partial ^2E}{\partial X_1^2}&{} \frac{\partial ^2E}{\partial X_1X_2}&{}\cdots &{}\frac{\partial ^2E}{\partial X_1X_N}\\ \frac{\partial ^2E}{\partial X_1X_2}&{} \frac{\partial ^2E}{\partial X_2^2}&{}\cdots &{}\frac{\partial ^2E}{\partial X_2X_N}\\ \vdots &{}\vdots &{}\vdots &{}\vdots \\ \frac{\partial ^2E}{\partial X_1X_N}&{} \frac{\partial ^2E}{\partial X_2X_N}&{}\cdots &{}\frac{\partial ^2E}{\partial X_N^2} \end{bmatrix}\)
Deep learning neural network (DNN) approach
Convolutional Neural Networks (CNN) and Deep Belief Networks (DBN) are two deep architectures 88 that can be combined to create DNN. In this article, the DBN architecture to build our deep learning approach is implemented. The architecture of the deep learning method is demonstrated in Fig. 5 . The procedure is separated into two stages: supervised back-propagation and unsupervised pre-training. Restricted Boltzmann Machines (RBM) with a deep neural network is used to train the model with 100 epoches in the early stages of development. An iterative method is implemented to construct the model with unlabeled Android apps in the training step. Pre-trained DBN is fine-tuned with labeled Android apps in a supervised manner during the back-propagation step. In both stages of the training process, a model developed using deep learning methods uses an Android app.
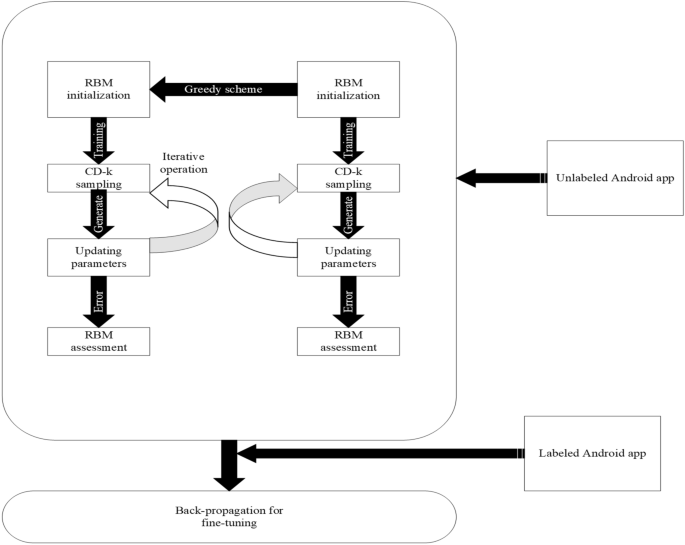
Deep learning neural network (DNN) method constructed with DBN.
Ensembles of classification models
In this study, three different ensemble models to detect malware from Android apps is also proposed. During development of the model, the outputs of all the classification models have been considered where the base machine learning algorithm allocated several priority levels and output is calculated by applying some combination rules. Ensemble approaches are divided into two types:
Homogenous ensemble approach: In this approach, all classification models, are of the same kinds, but the difference is in generating the training set.
Heterogenous ensemble approach: Here, all base classification approaches are of distinct types.
On the basis of combination rules, ensemble approaches are divided into two distinct categories:
Linear ensemble approach: While developing the model, with a linear ensemble approach an arbitrator combines the results that come from the base learners, i.e., selection of classification approach, average weighted, etc.
Nonlinear ensemble approach: While developing the model, with the nonlinear ensemble approach, it fed the result of the base classifier, which is a nonlinear malware detection model for example Decision tree (DT), Neural network (NN), etc.
In this work, a heterogenous ensemble approach having three distinct combination rules is adapted. The ensemble techniques are detailed in Table 6 .
BTE (best training ensemble) approach
The BTE technique is based on the observation that each classifier performs differently when the data set is partitioned 20 . Among the applied classifier, the best model is selected to train data set that are founded on the principles of certain performance parameters. In this research paper, accuracy is considered as a performance parameter. Algorithm 1 given below is considered to calculate the ensemble output \(E_{result}\) .
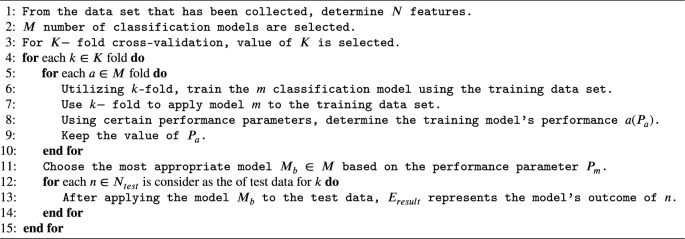
Best Training Ensemble (BTE) approach.
MVE (majority voting ensemble) approach
MVE approach, based on the principle to consider the output of the test data for each classifier, and the ensemble output \((E_{result})\) is concerned with the majority group differentiated by the base classifier 20 . Ensemble output \((E_{result})\) is calculated by implementing Algorithm 2.
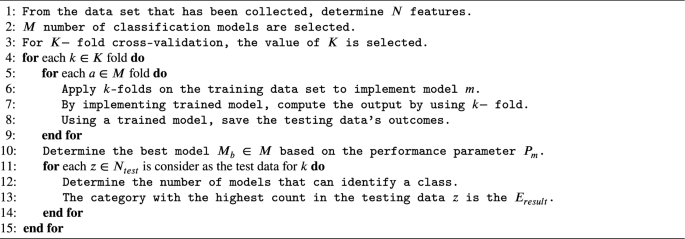
Majority Voting Ensemble (MVE) Approach.
NDTF (nonlinear ensemble decision tree forest) approach
In this study, to train the model with base leaner, is also considered. Further, the trained model is implemented the results on the corresponding testing data set to make the model for the final detection of malware apps. In this research paper, Decision tree forest (DTF) has been considered as a non-linear ensemble as a classifier which was suggested by Breiman in 2001. The developed model is based on the outcome of the collected results of the distinct decision trees. Algorithms 3 is used to calculate the result \((E_{result})\) .
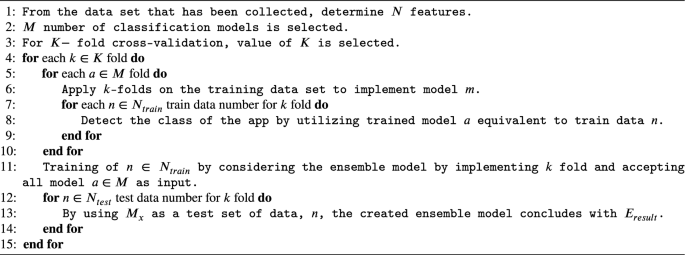
Nonlinear Ensemble Decision Tree Forest (NDTF) Approach.
Method for normalizing the data
In order to comply with the required diversity of input properties and prevent the saturation of the neurons, it is important to normalize the data prior to deploying a neural network spanning the range of 0 to 1 89 . The Min-max normalizing approach is used in this research study. This technique is work on the principle of a linear transformation, which brings each data point \(D_{q_i}\) of feature Q to a normalized value \(D_{q_i},\) that lies in between \(0-1.\)
To obtain the normalized value of \(D_{q_i}:\) , use the following equation:
The relative values of the relevance of the characteristic Q are min ( Q ) and max ( Q ).
Parameters considered for evaluation
This section provides definitions for the performance metrics needed to identify malicious apps. The confusion matrix is used to determine all of these characteristics. Actual and detected classification information is included in the confusion matrix, which was created using a detection approach. The constructed confusion matrix is shown in Table 7 . F-measure and accuracy are two performance parameters that are used to evaluate the performance of malware detection algorithms in this research. Formulas for evaluating the accuracy and F-measure are given below:
False positive (FP) A false positive occurs when the developed model identifies the positive class incorrectly.
False negative (FN) When the developed model successfully identifies the negative class, a false negative occurs.
True negative (TN) An accurate identification of the negative class by the developed model represents a true negative conclusion.
True positives (TP) An accurate identification of the positive class by the developed model represents a real positive conclusion.
Recall The data set’s positive classes that are made up of all other positive classes are identified.
where \(x= N_{Malware\rightarrow Malware},\) \(z= N_{Malware\rightarrow Benign}\)
Precision The accuracy measures the proportion of forecasts in the positive class that are indeed in the positive class.
where \(y= N_{Benign\rightarrow Malware}\)
Accuracy Accuracy is measured as 3 :
where \({N_{classes} = x+y+z+w}\) ,
\(w= N_{Benign\rightarrow Benign}\)
F-measure F-measure is measured as 3 :
Proposed feature selection validation method
The selection of relevant feature sets is an important challenge for data processing in various machine learning and data mining applications 90 , 91 , 92 . In the field of Android malware detection, a number of authors 13 , 14 , 15 , 69 , 93 , 94 applied only limited feature subset selection and feature ranking approaches i.e., Correlation, Goodman Kruskals, Information Gain, Chi-squared, Mutual Information, and t-test methods to detect malware. The first limitation of the previous studies is that they used a small data set (i.e., the number of malware or benign apps is less in number) to validate the proposed techniques. The additional significant disadvantage of the feature selection lies in the fact that after selecting the best features no comparison analyses were made among the classifiers model developed by reduced sets of features and by using all extracted feature sets. Mainly, the main reason for this is that the vast collection of features found in particular categories of the app (like books, entertainment, comics, game, etc.) makes it complex to produce a classifier by examining all the features as input. It is the best of our knowledge, that academicians and researchers were implemented these feature selection approaches individually; but no one selected features by combining all of these feature selection approaches. However, a framework for the feature selection approach has been given in this study, which helps in selecting the most appropriate features and enhance the effectiveness of the malware detection model. The suggested framework is applied to apps that have been gathered from the various repositories listed in section 2.4 and that fall under the thirty categories listed in Table 3 . Finally, we verified the framework by comparing the effectiveness of the models developed after implementing feature selection method with the efficiency of ones constructed using the whole data set initially formed.
Figure 2 demonstrates the phases of the proposed feature selection validation framework. Without using machine learning algorithms, this framework aims to determine whether the selected features are useful in detecting malicious apps. The wrapper strategy is used to pick the sets of features that are useful in identifying malware apps after all crucial components have been examined. It keeps track of the progress of the learning algorithm that was used to identify each feature subset. In this work, the selected features are investigated using linear discriminant analysis (LDA).
Data set Table 3 summarized the data set used in this research work. The considered data set belongs to 141 different malware families.
Normalization of data By using the Min-max normalizing approach, all features are normalized between the ranges of 0 and 1.
Partition of data We examined at the data set that wasn’t used for training in order to evaluate the proposed feature selection approach. Further, the data set is divided into two different parts one part is used for training, and the remaining is used for testing. The group ratios in the training and testing of the data sets are nearly identical.
Filter approach Pre-processing is the term that describes this technique because it eliminates extraneous features. In this step, the t -test and ULR analysis are implemented.
t-test analysis It examine the statistical significance of benign and malware apps using the t -test method. In a 2-class problem (malware apps and benign apps), analysis of the null hypothesis (H0) significant that the two populations are not equal, or it is seen that there is a noticeable variance among their mean values and features used by both of them are different 95 . Furthermore, it shows that the features affect the malware detection result. Hence, those features are considered, which have significant differences in their mean values, and others are excluded. Hence, it is essential to approve the null hypothesis (i.e., H0) and discard the alternative ones 95 . t -test is implemented on each of the attributes and then P value for each feature is calculated, which indicates how well it distinguishes the group of apps. According to research by 95 , features with an P value of < 0.05 show significant biases.
Univariate logistic regression (ULR) analysis After identifying features that make a significant difference between malware and benign apps, binary ULR analysis is implemented to test the correlation among features that helps in malware detection 95 . ULR analysis is implemented on each selected feature set, which helps in discovering whether the above-selected features were essential to detect the malware-infected apps or not. Only those features are considered, which are having P value < 0.05. From the results of the ULR analysis and t -test, the hypothesis are rejected and accepted mentioned in Table 5 .
Wrapper approach To determine optimum sets of the feature, cross-correlation analysis and multivariate linear regression stepwise forward selection is implemented in this stage.
Cross correlation analysis After finding the important features, the correlation analysis is implemented and then examination for both negative and positive correlation coefficients (i.e., r-value) between features is performed. If a feature has a value of r > = 0.7 or r-value < =0.7 with other features, i.e., have a higher correlation then the performance of these features is studied separately. Further, those features are selected, which perform better.
Multivariate linear regression stepwise forward selection It is not imply that, features that are achieved are relevant to develop malware detection framework. In this stage, ten-fold cross-validation technique is applied to determine the significant features.
Performance evaluation Further, to validate that proposed framework is able to identify malware-infected apps that were developed by implementing the steps mentioned above by using independent test data. Additionally, the efficiency of the essential feature sets used for malware detection is validated. On thirty different categories of Android apps, nine different machine learning classifiers were used to develop the investigation model. To evaluate the framework two separate performance parameters, are considered i.e., F-measure and Accuracy. The effectiveness of our detection model is then evaluated using the proposed malware detection methodology.
Evaluation of proposed framework
Three different approaches are used to evaluate our proposed framework:
Comparison with previously used classifiers Parameters like Accuracy and F-measure are compared with existing classifiers proposed by researchers in the literature to see if our suggested model is feasible or not.
Comparison with AV scanners To compare the effectiveness of our suggested work, ten different anti-virus scanners are considered and their performance is evaluated on the collected data set.
Detection of unknown and known malware families The proposed framework is also examined to see whether it can identify known and unknown malware families.
Experimental setup and results
The experimental setting used to develop the malware detection model is described in this portion of the paper. The model is developed using a Neural Network (NN) using six different types of machine learning algorithms, namely GD, NM, LM, GDA, GDM, DNN, and three ensemble techniques, including the best training, non-linear decision tree forest, and majority voting. These algorithms are applied on Android apps that were collected from different resources. Each category has a distinct number of benign and malicious apps (they are further separated into various families), which is sufficient for our analysis. Figure 6 presents PermDroid, our suggested framework.
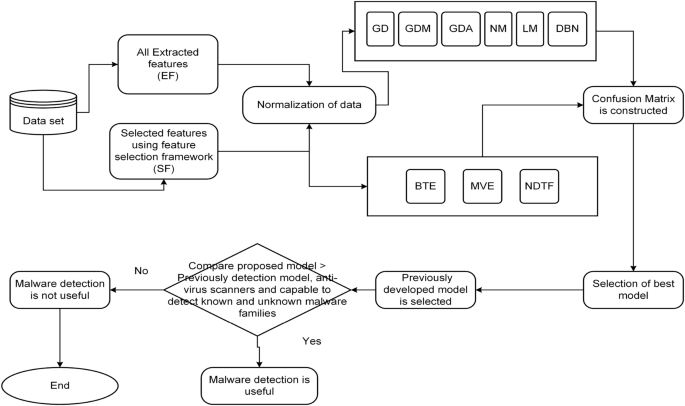
Proposed framework i.e., PermDroid.
Following are the phases that are pursued in this study, to develop an effective and efficient malware detection framework. The proposed feature selection framework is applied to all the extracted feature data sets, to select significant features. After that, six different machine learning algorithms based on the principle of neural network and three different ensemble algorithms are considered to develop a malware detection model. So, in this study, a total of 540 (30 different Android apps data sets * 9 different machine learning techniques * (one takes into account all extracted features, and another takes into account features identified using the suggested feature selection framework. )) different detection models are developed. The following are a detailed description of the model followed in this study:
Thirty different extracted feature data sets are used to implement the proposed feature selection framework.
The first stage, which involved identifying significant features, was employed as an input to train the model using various classification and ensemble machine learning approaches. In this research paper, ten-fold cross-validation technique is implemented to verify the develop model 16 . Further, outliers are eliminated, which effect the performance of the proposed framework. The performance of outliers is measured using the equation below:
The developed model using the aforementioned two processes is evaluated using the collected data set in order to determine whether or not the proposed framework is successful in identifying malicious apps.
Validation of the proposed feature selection framework
In this subsection, the selection of significant feature sets for malware detection is explained. Our analysis is started by using thirty different feature sets (mentioned in Table 4 ).
t-Test analysis
t -test analysis is used to determine the statistical significance of detecting the malware from Android apps. In this work, t -test is applied on extracted feature sets and calculated its P value. Further, in this study, the cut-off P value considered is 0.05, i.e., it denotes that feature sets that have P value < 0.05 has a strong prediction capability. Figure 7 illustrates the findings of a t -test performed on the thirty various categories of Android apps that comprise up our obtained data set. The P value is provided using two forms for simplicity of use (box with black circle \((\cdot)\) means P value < 0.05 and blank box \({}_ \Box\) means P value > than 0.05). The sets of features with emphasis P values of < 0.05 have a significant impact on identifying malicious or benign apps. Figure 7 shows how the S29, S27, S25, S23, S22, S21, S19, S18, S13, S10, S8, S5, S3, and S1 feature sets might help to detect malicious and benign apps in the Arcade and Action categories. As a result, in this study, we rule out the hypotheses H1, H3, H5, H8, H10, H13, H18, H19, H21, H22, H23, H25, H27, and H29, coming to the conclusion that these sets of features are capable of identifying apps in the Arcade and Action category that are malicious or benign.
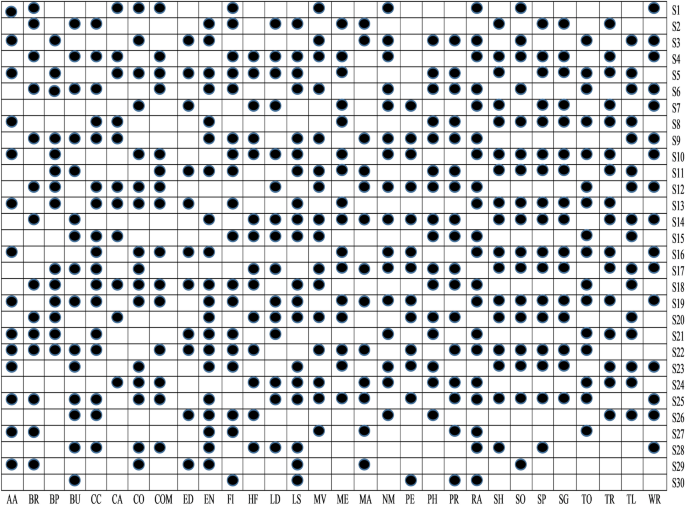
t -Test analysis.
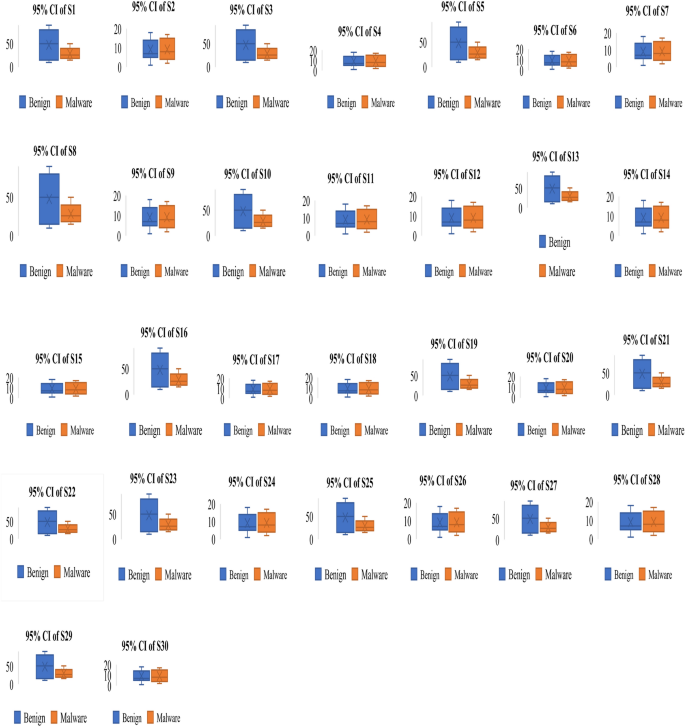
Error box-plots for all the set of permissions in Arcade and Action category apps.
To understand the relationship between malware and benign apps, we have drawn an error box-plot diagram. These box-plot diagrams verify the outcomes of the t -test analysis. If there is no overlapping in means and their confidence intervals (CI), then it means there will be a statistical difference between malware and benign apps else. There is no significant difference between them. An error box-plot of the 95% confidence intervals throughout the sets of features and the mean for Arcade and Action category apps is demonstrated in Fig. 8 . The outcomes of other categories of Android apps are of similar types. Based on Fig. 8 , we can observe that the boxes of S29, S27, S25, S23, S22, S21, S19, S18, S13, S10, S8, S5, S3, and S1 sets of feature do not overlap which means they are significantly different from each other. The mean value of the malware group is higher than the benign group apps. Based on error box-plots, we consider the hypotheses H1, H3, H5, H8, H10, H13, H18, H19, H21, H22, H23, H25, H27 and H29 concluding that these feature sets can able to identify the malware-infected apps for Arcade and Action category Android apps.
ULR analysis
To examine whether the selected sets of feature after implementing t -test analysis are significant to identify malware apps or not, in this study, ULR analysis is performed on selected sets of features. A set of features is considerably associated with malware detection if its P value is < 0.05. In every task, some sets of features are essential for the evolution of the malware detection model, while different sets of features do not seem to be appropriate for malware detection. The outcomes of the ULR approach are demonstrated in Fig. 9 . Equivalent to t-test analysis, the same representation is used as such in P values, i.e., blank box means P value > 0.05 and box having black square has P value \(\le\) to 0.05.
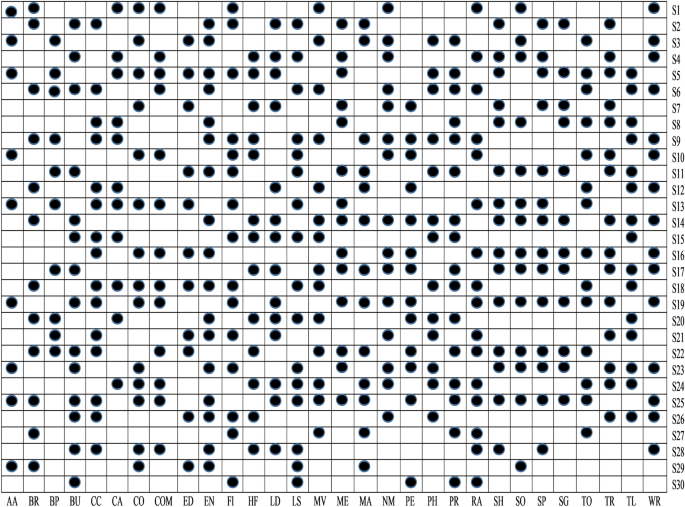
ULR analysis.
From Fig. 9 , it is clear that among thirty different categories of features, only S5, S3, S1, S13, S10, S23, S19, S29, and S25 sets of features are significant detectors of malware apps. As a result, we reject null hypotheses H1, H3, H5, H10, H13, H19, H23, H25, and H29 and conclude that these sets of features are directly related to the functioning of the apps. After implementing t -test and ULR analysis on our collected sets of features, rejection and acceptance of the hypotheses is done that is presented in the Table 5 . Figure 10 demonstrates the rejection and acceptance of the hypotheses for all of the thirty different categories of Android apps. The horizontal and vertical axes indicate the name of the hypothesis and the equivalent category of the Android app, accordingly. To represent the rejection and acceptance of the hypotheses, the cross symbol \((\times )\) and black circle \((\cdot )\) , are used respectively. Based on Fig. 10 , it is observed that only sixteen hypotheses out of thirty are accepted. Others are rejected for Arcade and Action category Android apps.
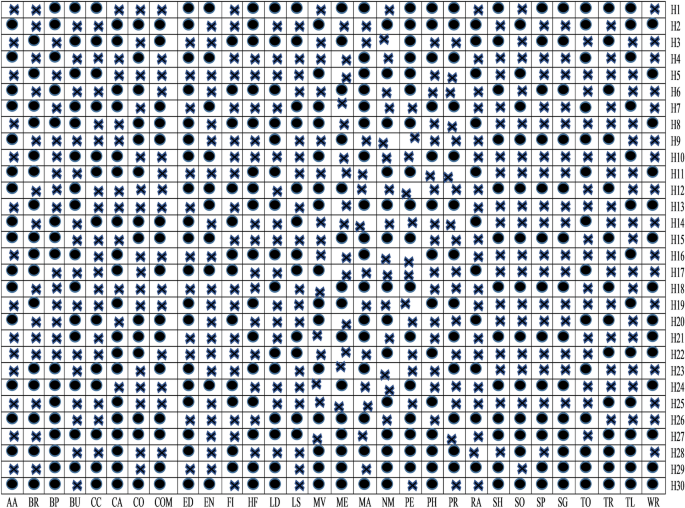
Hypothesis.
Cross correlation analysis
Figure 11 demonstrates the Pearson’s correlation between sets of features for all the categories of Android apps. The lower triangular (LT) and upper triangular (UT) matrices indicate the correlation in different sets of features for distinct Android app categories. The linear relation is evaluated by using the value of the correlation coefficient between distinct sets of extracted features from Android apps. In the present paper, Pearson’s correlation (r: Coefficient of correlation) is used to determine the linear relationship among distinct sets of features. The direction of the association is determined by whether the correlation coefficient, r , has a positive or negative sign. If the value of r is positive, it indicates that dependent and independent variables grow linearly or if the value of r is negative. Both the dependent and independent variables are inversely proportional to each other. Cross-correlation analysis is conducted only on the sets of features that were identified by implemented ULR and t -test analysis. If the relevant sets of features show a higher value of correlation (i.e., r -value \(\ge\) 0.7 or r -value \(\le -0.7\) ) with pertinent other sets of features, then the performance of these sets of feature separately and on the joint basis for malware detection is validated and consider those sets of feature which perform well. Figure 12 demonstrates the selected sets of the feature after implementing cross-correlation analysis. The selected sets of features are represented by utilizing a black circle \((\cdot)\) , demonstrating that equivalent sets of features are considered for this research paper.
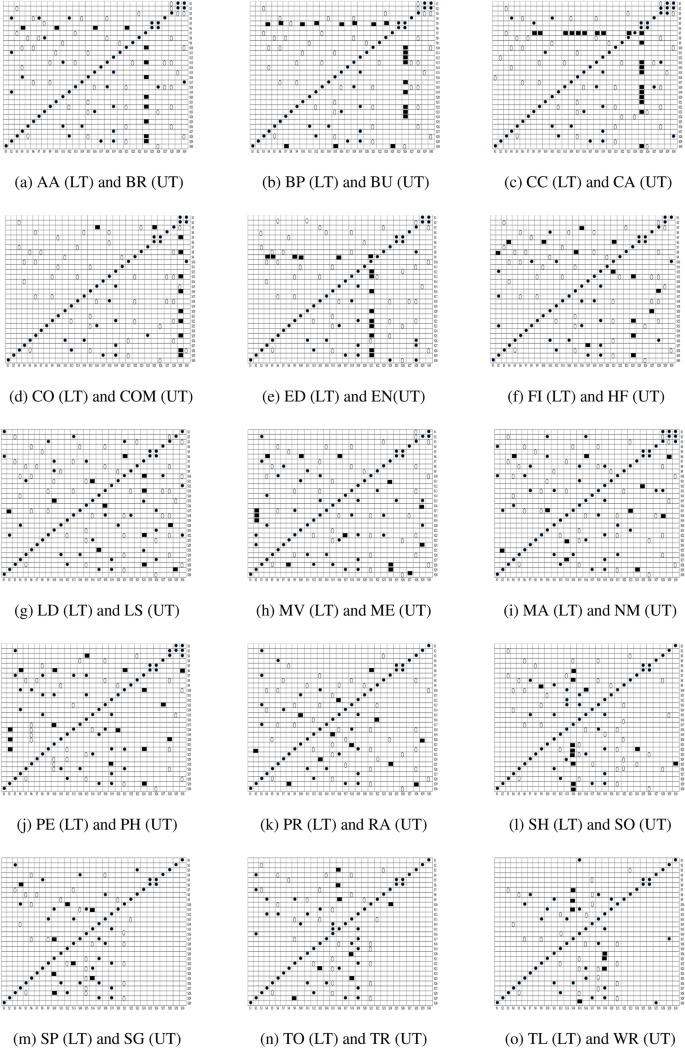
Correlation between set of features (here LT stands for lower triangle and UT stands for Upper triangle.
Stepwise forward selection for multivariate linear regression
After using cross-correlation analysis, the selected subset of features may or may not be important for creating the malware detection model. Further, a multivariate linear regression stepwise forward selection method is implemented in this study to discover the most important features for creating Android malware detection models. After applying multivariate linear regression stepwise on the retrieved feature data set, Fig. 13 shows a significant set of features. A set of features that were taken into account in this paper while building a malware detection model is represented by a black circle with the symbol \((\cdot )\) .
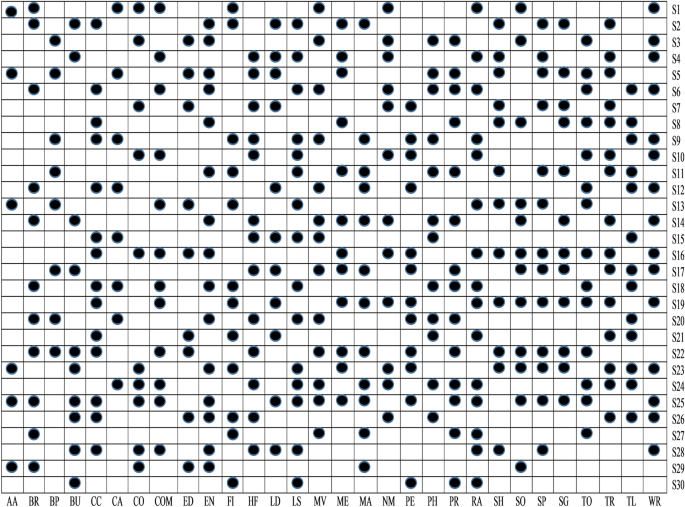
Features selected after implementing cross correlation analysis.
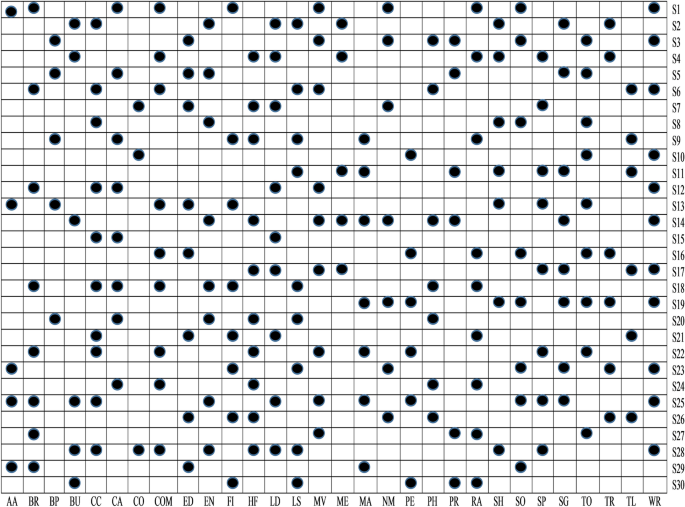
Features selected after implementing multivariate linear regression stepwise forward selection.
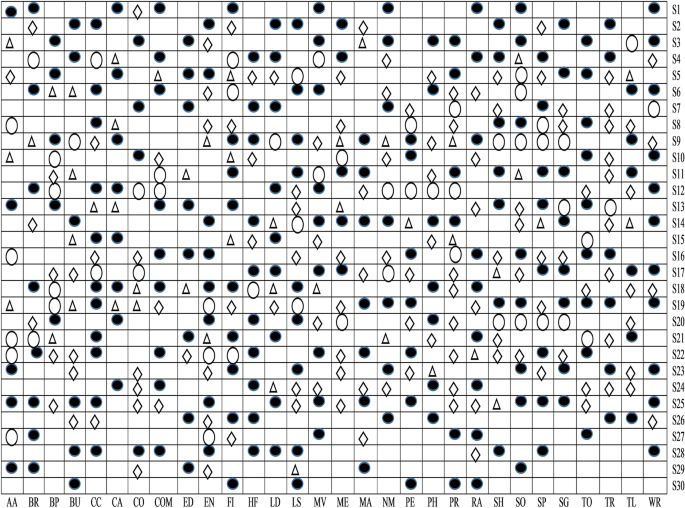
Selected sets of feature for malware detection.
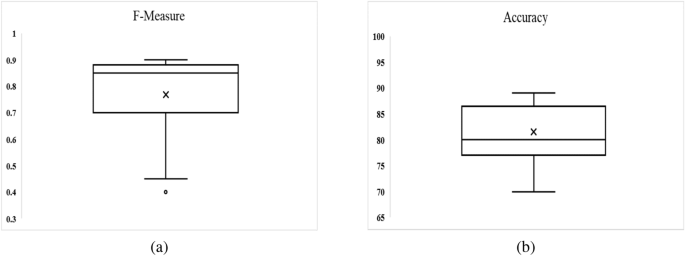
Results of testing data by considering performance parameters.
The overall outcome of the feature selection method
In this study, four distinct phases are used to identify relevant sets of features that will be taken into account while constructing the Android malware detection model. Some relevant sets of features are identified from the available sets of features in each stage based on the outcomes of the intermediate analysis. A selection of features from each of the thirty various categories of Android apps are shown in Fig. 14 . To make things easier, the selected feature sets are represented by four separate characters, as shown below:
Empty circle symbol: Features are relevant after implementing t -test analysis.
Triangle symbol: Features are relevant after implementing ULR analysis and t -test.
Diamond symbol: Features are relevant after applied cross-correlation analysis, ULR, and t -test.
Filled circle symbol: Features are relevant after implementing multivariate linear regression stepwise forward selection method, cross-correlation analysis, ULR, and t -test.
Evaluation on the basis of performance parameters
To examine set of features, a new data set is used that was not previously considered in this study. The model is originally built using ten-fold cross-validation, multivariate linear regression, and selected feature sets as input. Figure 15 illustrates the box-plot diagram for performance measures for all Android apps categories used in this study, including F-measure and Accuracy. It reveals that the outcome is computed as Accuracy of 82 percent and an average F-measure of 0.80.
Evaluation of the malware detection models developed using ANN
In this paper, we use a neural network to develop a model for malware detection using six different types of machine learning algorithms.
Two separate feature data sets are used as input to construct a model for identifying malware from Android apps (one comprises all extracted features (EF) and the other is used using the feature selection framework (SF). The following hardware was used to complete this task: a Core i7 processor with a 1 TB hard disc and 64 GB RAM. Each malware detection model’s performance is measured using two performance parameters: F-Measure and Accuracy. The outcomes of using a neural network with six different machine learning techniques to achieve performance metrics for various categories of Android apps are shown in Tables 8 and 9 . From Tables 8 and 9 , the following conclusions can be drawn:
The model developed by features selected using proposed framework (Model also developed by using distinct feature selection approaches are shown in Tables S1 to S14 in “Online Appendix A”) as an input produces better results when compared to a model constructed by taking into account all sets of features, presenting a significant value of F-measure and Accuracy for identifying malware.
In compared to the others, the neural network with Deep Neural Network (DNN) training method yields higher outcomes.
Figures 16 and 17 show the Accuracy and F-measure box-plot diagrams for each model built using classification methods. Each figure has two box plots, one containing all of the extracted features (EF) and the other containing only selected feature sets (SF).
The Box-plot diagram assists us in analyzing the performance of all the implemented approaches based on a single diagram. The line drawn in the middle of each box-plot diagram, i.e. the median, is used to determine its value. If a model’s median value is high, it’s regarded as the best model for detecting malware. It can be inferred from Figs. 16 and 17 that:
The models developed utilizing a significant set of features have high median values. The box-plot diagrams in Figs. 16 and 17 show that SF outperformed all extracted features in terms of detecting Android malware.
The DNN-based model yields the best results out of all the machine learning techniques for classification that have been used.
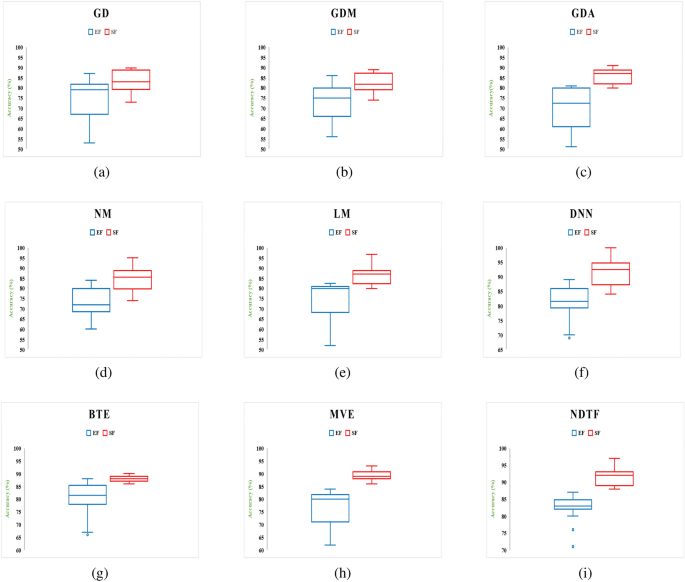
Box-plot diagram for measured performance parameter i.e., Accuracy.
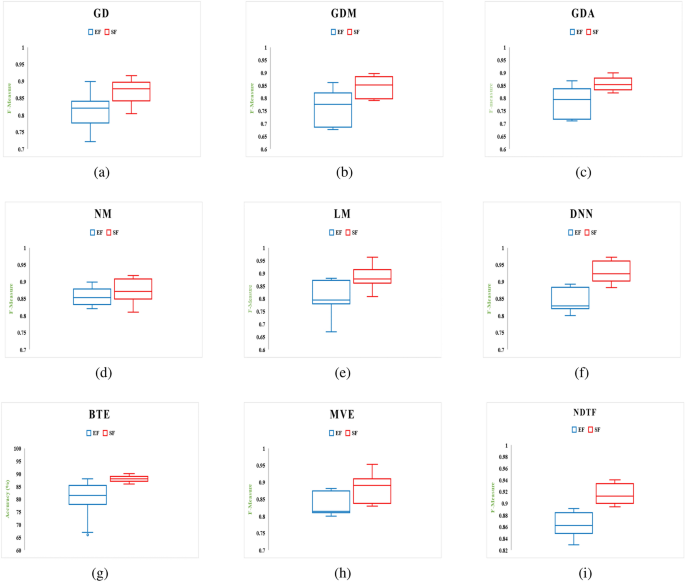
Box-plot diagram for measured performance parameter i.e., F-measure.
Evaluation of the malware detection models developed using ensemble techniques
In this study, three different heterogeneous ensemble approaches are considered for creating the Android malware detection model, each with a different combination rule (1 nonlinear and two linear). From Tables 8 and 9 and Figs. 16 and 17 , it can be revealed that the NDTF approach outperformed the BTE and MVE approaches. Further, it is also noticed that ensemble approaches detect more malware as compared to other implemented machine learning algorithms except DNN.
Comparison of the findings
In this study, paired Wilcoxon signed-rank tests to assess the relative performance of several feature sets and machine learning methods is employed. The Wilcoxon test with Bonferroni correction is used in this work for comparative review.
On the basis of detection approaches
To create a model that can determine whether an Android app is benign or malicious, nine different classification algorithms were evaluated. Two sets of features have been identified as inputs for developing malware detection models for thirty different categories of Android apps using two different performance parameters, namely F-Measure and Accuracy. One set of features takes into account all extracted features, and the other sets of selected features that are gained by implementing the framework of the feature selection method. Two sets of data are used for each strategy, each having 60 data points ((1 feature selection approach + 1 considering all retrieved features) * 30 Android app categories). The comparisons of pair-wise different machine learning techniques are shown in Table 10 .
There are two sections in Table 10 . The value of the significant difference between different pairings is shown in the second half of the table, and the calculated P value is shown in the first half. Using Bonferroni correction sets, the significant cutoff value is calculated. In this work, nine different machine learning algorithms were examined for creating malware detection models, resulting in a total of 36 potential pairs \({}^{9 techniques} C_2=36\) , with all results examined at a significance threshold of 0.05. We can rule out the null hypothesis if the P value is < 0.05/36 = 0.0013. According to the study, the null hypothesis for the test implies that no significant difference exists between the two procedures. Table 10 a shows that the P value is < 0.0013, indicating that there is a significant difference between the applied processes; out of 36 pairs of training techniques, 22 are offered as a significant outcome. By examining the mean difference value in Table 10 a, it can be seen that the DNN method outperformed the performance of other machine learning techniques. In addition, the value of the mean difference of ensemble techniques is better when compared to other models, with the exception of the model built using DNN.
On the basis of all selected sets of feature using proposed framework and extracted features
By taking into consideration each set of features, a total of 270 different data points ((3 ensemble techniques + neural network with six machine learning techniques) * 30 types of Android apps) are developed in this study (one for each performance measure). Wilcoxon signed-rank test performance was described in Table 10 b. It is seen from Table 10 b that there is a significant difference between the models developed because the P value is less than 0.05. Additionally, it is evident that the features taken into account employing the feature selection framework outperformed the model developed by using all extracted feature sets when comparing the mean difference values from Table 10 b to it.
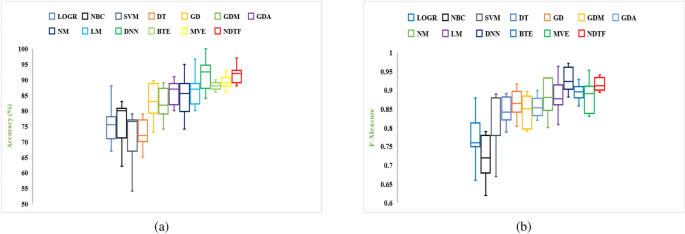
Measured performance parameters i.e., Accuracy and F-measure.
Proposed framework evaluation
Results comparison with previously employed classifiers.
In the present study, our newly developed malware detection model is also compared to the models developed using previously used classifiers such as decision tree analysis (DT), support vector machine (SVM), Naïve Bayes classifier (NBC), and logistic regression (LOGR). Two different sets of features (1 considering selected feature sets + 1 using all extracted features) are considered for 30 different categories of Android apps using two independent performance measures i.e., F-Measure and Accuracy. An aggregate of two sets i.e., 60 data points are employed for each classifier model are produced ((1 selected feature sets + 1 considering all extracted features)* 30 data sets). Figure 18 illustrates both the classifiers employed in this study and the most frequently used classifiers in the literature.
On the basis of Fig. 18 , it can be seen that the model produced using neural networks has a higher median value and achieves better results than the model developed using the literature’s used classifiers. Further, to decide that, which model produces better results, a pairwise Wilcoxon signed rank test is implemented. Table 11 summarizes the results of the Wilcoxon test with Bonferroni correction examination for accuracy outcomes. Further, the Table 11 is divided into two sections, the first of which indicates the P value and the second of which demonstrates the mean difference between different pairs of classifiers. We implemented thirteen different machine learning approaches in this research paper (4 previously applied classifier in the literature + 9 implemented classifier in this study); thus, an aggregate of seventy eight (78) individual pairs are possible \({}^{13techniques} C_2=78\) , and all classifier outcomes are examined at the 0.05 significance level. Only those null hypotheses with an P value is less than 0.05/78 = 0.000641 are rejected in this study. Table 11 shows that there is a significant difference between different implemented classifier approaches in a number of cases when the P value is less than 0.000641, i.e., 66 out of 78 pairs of classification approaches have significant outcomes. Table 11 demonstrates that the DNN approach outperforms other machine learning classifiers in terms of mean difference value.
Using cost-benefit analysis, comparison with previously employed classifiers
A cost-benefit analysis is used to evaluate the performance of developed model. Using the following equation, the cost-benefit analysis for each feature selection strategy is calculated:
In this case, \(Based_{cost}\) is determined by the correlation between the specified features set and the class error. The following equation can be used to compute \(Based_{cost}\) :
The multiple correlation coefficient between the error and the selected feature set is \(\rho _{SM.fault}\) , and the classification accuracy used to build a malware detection model using the selected feature set is \(Accuracy \ (SM)\) . The proposed model has a greater accuracy and a larger \(Based_{cost}\) since it has a higher multiple correlation coefficient. After adopting feature selection procedures, NAM stands for feature sets, while NSM stands for the number of selected features. The following equation can be used to determine \(Based_{cost}\) :
Instead of using the feature selection validation method, we use six other feature ranking approaches to evaluate PermDroid’s performance in this study. The naming standards used for the experiment are listed in Table 12 . The most important feature selection technique, as suggested in 96 , is the one that achieves a better value of cost-benefit. The cost-benefit analysis of different feature selection procedures is shown in Fig. 19 a,b. It is discovered that sets of features were selected after applying multivariate linear regression stepwise forward selection technique, cross-correlation analysis, ULR, and t -test to achieve a higher median Cost-benefit measure when compared to other feature selection techniques used by researchers in the literature.
In the literature academicians and researchers implemented different feature ranking and feature subset selection approaches i.e., Chi-squared test, Gain-ratio, Information-gain, Principal Component Analysis and Filtered subset evaluation. To evaluate the performance of our proposed feature selection approach, an experiment was performed by using Drebin data set and accuracy is measured and represented in Table 13 . Out of implemented six different feature selection techniques our proposed feature selection approach achieved an higher accuracy when compared to others.
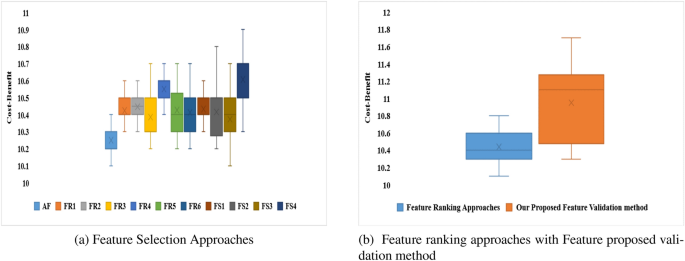
Calculated cost-benefit value.
Comparison of results based on the amount of time it takes to identify malware in real-world apps
In this section of the article, the performance of PermDroid is compared in terms of the time needed to identify malware in real-world apps. For this experiment, we download the data set from two different repositories Drebin ( https://www.sec.cs.tu-bs.de/~danarp/drebin/download.html ) and AMD ( http://amd.arguslab.org/ ) and experimented by implementing the individual frameworks. Table 14 shows that, when compared to the individual frameworks available in the literature, our suggested technique can identify malware in less time.
Comparison of the results on the basis of detection rate with different approaches or frameworks available in the literature
Furthermore, proposed malware detection model (i.e., PermDroid) is compared to previously developed techniques or frameworks present in the literature. The names, methodology, deployment, purpose, data collection, and detection rate of proposed methodologies or frameworks are listed in Table 15 . Empirical result revealed that our proposed framework produced a 3 percent greater detection rate. Experiment was performed by using Drebin data set ( https://www.sec.cs.tu-bs.de/~danarp/drebin/download.html ).
Comparison of results with different AV Scanners
Although PermDroid outperforms the classifiers used in the research, it should ultimately be similar to the results obtained using regular anti-virus software in the field for Android malware detection. For this study, ten different anti-virus softwares are selected from the market and used them on the data set that has been gathered in this study.
When compared to the various anti-viruses employed in the experiment, PermDroid performs significantly better. The results of the anti-virus scanner study are shown in Table 16 . The anti-virus scanners’ rates of virus detection vary widely. While the most effective scanners catch 97.1 percent of malware, some scanners only catch 82 percent of hazardous samples, which is probably a result of their inexperience with Android malware. PermDroid with DNN and NDTF outperform 1 out of 10 anti-virus scanners on the complete data set, with detection rates of 98.8% and 98.8%, respectively. Out of implemented different anti-virus scanners, it is discovered that at least two of them are capable of identifying every malware sample used in this study. As a result, it may conclude that PermDroid is more effective than many anti-virus scanners’ manually built signatures.
Identification of both well-known and new malware families
Detection of well-known malware families An experiment is also performed to identify whether or not our suggested framework, i.e., PermDroid, is capable of detecting malware from well-known families. The experiment is carried out on a sample of 20 families from each family (in our research paper, we collect 141 different malware families). According to empirical results, the suggested framework with DNN is capable of detecting an average of 98.8% of malware-infected apps, and the proposed framework with NDTF is likewise capable of doing the same. Table 17 lists the family names and the number of samples for each family, and Fig. 20 a,b show PermDroid’s detection performance for each family (Detection rates for some families are lower because of fewer samples in the data set).
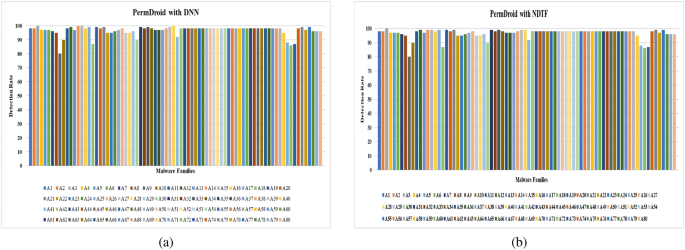
Detection rate of PermDroid with DNN and NDTF.
Detection of new malware families To examine if the suggested framework, is capable of identifying unknown malware families, PermDroid is trained with a random sample of 10 distinct families based on counting and then test is performed on the remaining families. Table 18 shows the outcomes in which PermDroid is trained with limited malware samples, which is required to generalize the characteristics of most malware families, and achieved a higher detection rate.
Experimental outcomes
The conclusions reached after conducting experimental work are presented in this section of the paper. The empirical work was done using a neural network and six different machine learning techniques, including GDA, NM, GD, GDM, LM, and DNN, as well as three ensemble approaches. The developed models outperform previously used classifiers in the literature (Table 11 ) and can detect malware from both known and unknown families (Table 18 , Fig. 20 ). Additionally, they increase the rate of detection by different Antivirus scanners (stated in Table 15 ). It is clear from Fig. 20 and Tables 14 , 15 , 16 , and 18 that:
PermDroid can detect 98.8% of Android malware, which is impossible for most AV scanners on the market.
With a detection rate of 98.8% for both known and unknown malware types, PermDroid is capable of finding malware.
The proposed framework is able to answer the research questions mentioned in “ Research questions ” section:
To verify the importance of the correlation between the feature sets and the malware detection model, the t -test and ULR analysis are used. It is discovered that there are several separate sets of features that are highly connected with the creation of malware detection models as a result of this research.
From Fig. 11 , it can be noticed that certain sets of features pass a high correlation with other sets of features (i.e., the case with a black square is having high negative correlation, and the case with a black circle is having a high positive correlation). It is essential to remove the collinearity among the features, for calculating the ability of each feature. In this manner, the models developed by selecting sets of the feature are capable to detect malware and do not suffer from the aspect of collinearity.
Forward stepwise selection process, ULR, correlation analysis, and t -test analysis are implemented to select features that are able to identify whether the app is malicious or not. The model built by applying the specified sets of features produces better outcomes when compared to the rest, according to t -test analysis.
Six various types of machine learning techniques based on neural network principles, such as NM, GD, LM, GDM, GDA, and DNN, as well as three ensemble approaches, are implemented in detecting whether an app is benign or malicious. From the Tables 8 and 9 , it is apparent that the model developed using an ANN and the Deep Neural Network (DNN) approach produces the best results when compared to other techniques.
Tables 8 and 9 and Figs. 18 , 19 and 20 show that our suggested model is effective in identifying malware from real-world apps when API calls, permissions, app rating, and the number of people that have downloaded the app are all considered features.
Threats to validity
In this section, threats to validity are discussed that are experienced while performing the experiment. Three different threats are mentioned below:
Construct validity The Android malware detection methodology in this research study is capable of detecting whether an app is benign or malicious, however it does not specify how many features are needed to find vulnerabilities in Android apps.
Internal validity The homogeneity of the data set employed in this research work is the second threat. Apps are collected from a variety of promised repositories. Any errors made while gathering data from these sources are not taken into account in this study. Although, it cannot promise that the data collected and retrieved for our analysis is 100 percent accurate, it can be believed that it assembled consistently.
External validity To train the Android malware detection algorithm, 141 different malware families are considered. Furthermore, the research can be extended to include other malware families in order to train the technique to identify malicious apps.
Conclusion and future work
This study suggests a framework for selecting small set of features that helps in detecting malware from Android apps. The following are our observations based on the basis of our proposed framework in this research paper:
Based on the feature selection method, it is discovered that there is a limited group of attributes that can detect malware or benign apps with greater accuracy and lower values of incorrectly classified errors.
Using our feature selection method sets S25, S28, S19, S14, S9, and S4 of features were discovered to be important malware detectors.
Based on the Wilcoxon signed-rank test, it is found that there is a significant difference between all extracted features and the selected feature sets. It is found that, after calculating the mean difference that the model developed with the input of the selected feature sets outperformed the model with the input of all extracted feature sets.
Different classification algorithms differ significantly, according to the Wilcoxon signed-rank test. By calculating the mean difference value, it is discovered that the model created by combining a neural network with the Deep-Learning machine-learning algorithm produced superior results than the other machine learning methods used in this study.
It may be inferred from the results of the experiments that the NDTF approach performed better than other ensemble methods.
Our used classifier outperformed the performance of the classifiers used in the literature, as shown in Fig. 20 and Tables 11 and 14 .
According to the results of the experiments (Tables 8 , 9 ), the malware detection model built was not significantly harmed after deleting 60% of the possible number of sets of features; in fact, in almost all cases, the results were better.
As shown in Table 18 and Fig. 20 , our proposed malware detection system can detect malware from both known and undiscovered malware families.
This study established that a malware detection method merely identifies whether an app is malicious or benign. Several avenues can be explored for future research. Firstly, a large amount of Android apps are required to develop the model, memorize and disclose information related to the data set. Second, it is also difficult to make a centralized system at the time of training and testing the model. Third, decentralized, privacy-preserving classifier model will be proposed for detecting Android malwares. Further, it is also be discovered how many permissions are necessary to evaluate whether an app is dangerous or not, more investigation may be done.
Data availibility
For materials should be addressed to corresponding authors.
Faruki, P. et al. Android security: A survey of issues, malware penetration, and defenses. IEEE Commun. Surv. Tutor. 17 (2), 998–1022 (2014).
Article Google Scholar
Gao, H., Cheng, S. & Zhang, W. Gdroid: Android malware detection and classification with graph convolutional network. Comput. Secur. 106 , 102264 (2021).
Mahindru, A. & Sangal, A. MLDroid—framework for android malware detection using machine learning techniques. Neural Comput. Appl. 33 , 1–58 (2020).
Google Scholar
Fereidooni, H., Conti, M., Yao, D. & Sperduti, A. Anastasia: Android malware detection using static analysis of applications. In 2016 8th IFIP International Conference on New Technologies, Mobility and Security (NTMS) , 1–5 (IEEE, 2016).
Arp, D. et al. Drebin: Effective and explainable detection of android malware in your pocket. Ndss 14 , 23–26 (2014).
Yuan, Z., Lu, Y. & Xue, Y. Droiddetector: Android malware characterization and detection using deep learning. Tsinghua Sci. Technol. 21 (1), 114–123 (2016).
Zhu, H. J. et al. Droiddet: Effective and robust detection of android malware using static analysis along with rotation forest model. Neurocomputing 272 , 638–646 (2018).
Wong, M. Y. & Lie, D. Intellidroid: A targeted input generator for the dynamic analysis of android malware. NDSS 16 , 21–24 (2016).
Dash, S. K., Suarez-Tangil, G., Khan, S., Tam, K., Ahmadi, M., Kinder, J. & Cavallaro, L. Droidscribe: Classifying android malware based on runtime behavior. In: 2016 IEEE Security and Privacy Workshops (SPW) , 252–261 (IEEE, 2016).
Chen, S., Xue, M., Tang, Z., Xu, L. & Zhu, H. Stormdroid: A streaminglized machine learning-based system for detecting android malware. In Proceedings of the 11th ACM on Asia Conference on Computer and Communications Security , 377–388 (2016).
Mariconti, E., Onwuzurike, L., Andriotis, P., Cristofaro, E. D., Ross, G. & Stringhini, G. Mamadroid: Detecting Android Malware by Building Markov Chains of Behavioral Models . arXiv:1612.04433 (2016)
Kabakus, A. T. DroidMalwareDetector: A novel android malware detection framework based on convolutional neural network. Expert Syst. Appl. 206 , 117833 (2022).
Mahindru, A. & Sangal, A. Deepdroid: Feature selection approach to detect android malware using deep learning. In: 2019 IEEE 10th International Conference on Software Engineering and Service Science (ICSESS) , 16–19 (IEEE, 2019).
Mahindru, A. & Sangal, A. Feature-based semi-supervised learning to detect malware from android. In Automated Software Engineering: A Deep Learning-Based Approach , 93–118 (Springer, 2020).
Mahindru, A. & Sangal, A. Perbdroid: Effective malware detection model developed using machine learning classification techniques. In A Journey Towards Bio-inspired Techniques in Software Engineering 103–139 (Springer, 2020).
Mahindru, A. & Sangal, A. Hybridroid: An empirical analysis on effective malware detection model developed using ensemble methods. J. Supercomput. 77 (8), 8209–8251 (2021).
Mahindru, A. & Sangal, A. Semidroid: A behavioral malware detector based on unsupervised machine learning techniques using feature selection approaches. Int. J. Mach. Learn. Cybern. 12 (5), 1369–1411 (2021).
Zhao, Y. et al. On the impact of sample duplication in machine-learning-based android malware detection. ACM Trans. Softw. Eng. Methodol. (TOSEM) 30 (3), 1–38 (2021).
Yumlembam, R., Issac, B., Jacob, S. M. & Yang L. IoT-based android malware detection using graph neural network with adversarial defense. IEEE Internet Things J. (2022).
Kumar, L., Misra, S. & Rath, S. K. An empirical analysis of the effectiveness of software metrics and fault prediction model for identifying faulty classes. Comput. Stand. Interfaces 53 , 1–32 (2017).
Faruki, P., Ganmoor, V., Laxmi, V., Gaur, M. S. & Bharmal, A. Androsimilar: Robust statistical feature signature for android malware detection. In Proceedings of the 6th International Conference on Security of Information and Networks , 152–159 (2013).
Milosevic, J., Malek, M. & Ferrante, A. Time, accuracy and power consumption tradeoff in mobile malware detection systems. Comput. Secur. 82 , 314–328 (2019).
Shabtai, A., Kanonov, U., Elovici, Y., Glezer, C. & Weiss, Y. Andromaly: A behavioral malware detection framework for android devices. J. Intell. Inf. Syst. 38 (1), 161–190 (2012).
Badhani, S. & Muttoo, S. K. Android malware detection using code graphs. In System Performance and Management Analytics , 203–215 (Springer, 2019).
Xu, R., Saïdi, H. & Anderson, R. Aurasium: Practical policy enforcement for android applications. In Presented as part of the 21st \(\{\) USENIX \(\}\) Security Symposium ( \(\{\) USENIX \(\}\) Security 12 ), 539–552 (2012).
Lindorfer, M., Neugschwandtner, M., Weichselbaum, L., Fratantonio, Y., Veen, V. V. D. & Platzer, C. (2014) Andrubis–1,000,000 apps later: A view on current android malware behaviors. In 2014 Third International Workshop on Building Analysis Datasets and Gathering Experience Returns for Security (BADGERS) , 3–17 (IEEE).
Ikram, M., Beaume, P. & Kâafar, M. A. Dadidroid: An Obfuscation Resilient Tool for Detecting Android Malware via Weighted Directed Call Graph Modelling . arXiv:1905.09136 (2019).
Shen, F., Vecchio, J. D., Mohaisen, A., Ko, S. Y. & Ziarek, L. Android malware detection using complex-flows. IEEE Trans. Mob. Comput. 18 (6), 1231–1245 (2018).
Yang, W., Prasad, M. R. & Xie, T. Enmobile: Entity-based characterization and analysis of mobile malware. In Proceedings of the 40th International Conference on Software Engineering , 384–394 (2018).
Enck, W. et al. Taintdroid: an information-flow tracking system for realtime privacy monitoring on smartphones. ACM Trans. Comput. Syst. (TOCS) 32 (2), 1–29 (2014).
Portokalidis, G., Homburg, P., Anagnostakis, K. & Bos, H. (2010) Paranoid android: Versatile protection for smartphones. In Proceedings of the 26th Annual Computer Security Applications Conference , 347–356.
Bläsing, T., Batyuk, L., Schmidt, A. D., Camtepe, S. A. & Albayrak, S. An android application sandbox system for suspicious software detection. In 2010 5th International Conference on Malicious and Unwanted Software , 55–62 (IEEE, 2010).
Aubery-Derrick, S. Detection of Smart Phone Malware . Unpublished Ph.D. Thesis, 1–211 (Electronic and Information Technology University, Berlin, 2011).
Burguera, I., Zurutuza, U. & Nadjm-Tehrani, S. Crowdroid: Behavior-based malware detection system for android. In Proceedings of the 1st ACM Workshop on Security and Privacy in Smartphones and Mobile Devices , 15–26 (2011).
Grace, M. C., Zhou, Y., Wang, Z. & Jiang, X. Systematic detection of capability leaks in stock android smartphones. In NDSS , vol 14, 19 (2012).
Grace, M., Zhou, Y., Zhang, Q., Zou, S. & Jiang, X. Riskranker: Scalable and accurate zero-day android malware detection. In Proceedings of the 10th International Conference on Mobile Systems, Applications, and Services , 281–294 (2012).
Zheng, C., Zhu, S., Dai, S., Gu, G., Gong, X., Han, X. & Zou, W. Smartdroid: An automatic system for revealing UI-based trigger conditions in android applications. In Proceedings of the Second ACM Workshop on Security and Privacy in Smartphones and Mobile Devices , 93–104 (2012).
Dini, G., Martinelli, F., Saracino, A. & Sgandurra, D. Madam: A multi-level anomaly detector for android malware. In International Conference on Mathematical Methods, Models, and Architectures for Computer Network Security , 240–253 (Springer, 2012).
Yan, L. K. & Yin, H. Droidscope: Seamlessly reconstructing the \(\{\) OS \(\}\) and Dalvik semantic views for dynamic android malware analysis. In Presented as part of the 21st \(\{\) USENIX \(\}\) Security Symposium ( \(\{\) USENIX \(\}\) Security 12 ), 569–584 (2012).
Backes, M., Gerling, S., Hammer, C., Maffei, M. & von Styp-Rekowsky, P. Appguard–enforcing user requirements on android apps. In International Conference on TOOLS and Algorithms for the Construction and Analysis of Systems , 543–548 (Springer, 2013).
Shahzad, F., Akbar, M., Khan, S. & Farooq, M. Tstructdroid: Realtime malware detection using in-execution dynamic analysis of kernel process control blocks on android . Tech Rep (National University of Computer and Emerging Sciences, Islamabad, 2013).
Rastogi, V., Chen, Y. & Enck, W. Appsplayground: Automatic security analysis of smartphone applications. In Proceedings of the third ACM Conference on Data and Application Security and Privacy , 209–220 (2013).
Rosen, S., Qian, Z. & Mao, Z. M. Appprofiler: A flexible method of exposing privacy-related behavior in android applications to end users. In Proceedings of the Third ACM Conference on Data and Application Security and Privacy , 221–232 (2013).
Desnos, A. et al . Androguard-reverse engineering, malware and goodware analysis of android applications. URL code google com/p/androguard 153 (2013).
Tam, K., Khan, S. J., Fattori, A. & Cavallaro, L. Copperdroid: Automatic reconstruction of android malware behaviors. In Ndss (2015).
Suarez-Tangil, G., Dash, S. K., Ahmadi, M., Kinder, J., Giacinto, G. & Cavallaro, L. Droidsieve: Fast and accurate classification of obfuscated android malware. In Proceedings of the Seventh ACM on Conference on Data and Application Security and Privacy , 309–320 (2017).
Idrees, F., Rajarajan, M., Conti, M., Chen, T. M. & Rahulamathavan, Y. Pindroid: A novel android malware detection system using ensemble learning methods. Comput. Secur. 68 , 36–46 (2017).
Martín, A., Menéndez, H. D. & Camacho, D. Mocdroid: Multi-objective evolutionary classifier for android malware detection. Soft. Comput. 21 (24), 7405–7415 (2017).
Karbab, E. B., Debbabi, M., Derhab, A. & Mouheb, D. Maldozer: Automatic framework for android malware detection using deep learning. Digit. Investig. 24 , S48–S59 (2018).
Lee, W. Y., Saxe, J. & Harang, R. Seqdroid: Obfuscated android malware detection using stacked convolutional and recurrent neural networks. In Deep Learning Applications for Cyber Security , 197–210 (Springer, 2019).
Alzaylaee, M. K., Yerima, S. Y. & Sezer, S. DL-Droid: Deep learning based android malware detection using real devices. Comput. Secur. 89 , 101663 (2020).
Yuan, Z., Lu, Y., Wang, Z. & Xue, Y. Droid-sec: Deep learning in android malware detection. In Proceedings of the 2014 ACM Conference on SIGCOMM , 371–372 (2014).
Zhang, M., Duan, Y., Yin, H. & Zhao, Z. Semantics-aware android malware classification using weighted contextual API dependency graphs. In Proceedings of the 2014 ACM SIGSAC Conference on Computer and Communications Security , 1105–1116 (2014).
Shankar, V. G., Somani, G., Gaur, M. S., Laxmi, V. & Conti, M. Androtaint: An efficient android malware detection framework using dynamic taint analysis. In 2017 ISEA Asia Security and Privacy (ISEASP) , 1–13 (IEEE, 2017).
Mahindru, A. & Singh, P. Dynamic permissions based android malware detection using machine learning techniques. In Proceedings of the 10th Innovations in Software Engineering Conference , 202–210 (2017).
Shi, B. et al. Prediction of recurrent spontaneous abortion using evolutionary machine learning with joint self-adaptive sime mould algorithm. Comput. Biol. Med. 148 , 105885 (2022).
Article PubMed Google Scholar
Zhang, Q., Wang, D. & Wang, Y. Convergence of decomposition methods for support vector machines. Neurocomputing 317 , 179–187 (2018).
Hou, S., Saas, A., Chen, L. & Ye, Y. Deep4maldroid: A deep learning framework for android malware detection based on linux kernel system call graphs. In 2016 IEEE/WIC/ACM International Conference on Web Intelligence Workshops (WIW) , 104–111 (IEEE, 2016).
Nix, R. & Zhang, J. Classification of android apps and malware using deep neural networks. In 2017 International Joint Conference on Neural Networks (IJCNN) , 1871–1878 (IEEE, 2017).
Zhang, X. A deep learning based framework for detecting and visualizing online malicious advertisement. Ph.D. Thesis, University of New Brunswick (2018)
Nauman, M., Tanveer, T. A., Khan, S. & Syed, T. A. Deep neural architectures for large scale android malware analysis. Clust. Comput. 21 (1), 569–588 (2018).
Xiao, X., Wang, Z., Li, Q., Xia, S. & Jiang, Y. Back-propagation neural network on Markov chains from system call sequences: a new approach for detecting android malware with system call sequences. IET Inf. Secur. 11 (1), 8–15 (2016).
Martinelli, F., Marulli, F. & Mercaldo, F. Evaluating convolutional neural network for effective mobile malware detection. Procedia Comput. Sci. 112 , 2372–2381 (2017).
Xiao, X., Zhang, S., Mercaldo, F., Hu, G. & Sangaiah, A. K. Android malware detection based on system call sequences and LSTM. Multim. Tools Appl. 78 (4), 3979–3999 (2019).
Dimjašević, M., Atzeni, S., Ugrina, I. & Rakamaric, Z. Evaluation of android malware detection based on system calls. In Proceedings of the 2016 ACM on International Workshop on Security and Privacy Analytics , 1–8 (2016).
Mas’ud, M. Z., Sahib, S., Abdollah, M. F., Selamat, S. R. & Yusof, R. Analysis of features selection and machine learning classifier in android malware detection. In 2014 International Conference on Information Science and Applications (ICISA) , 1–5 (IEEE, 2014).
Yerima, S. Y., Sezer, S., McWilliams, G. & Muttik, I. A new android malware detection approach using Bayesian classification. In 2013 IEEE 27th International Conference on Advanced Information Networking and Applications (AINA) , 121–128 (IEEE, 2013).
Narudin, F. A., Feizollah, A., Anuar, N. B. & Gani, A. Evaluation of machine learning classifiers for mobile malware detection. Soft. Comput. 20 (1), 343–357 (2016).
Wang, W. et al. Exploring permission-induced risk in android applications for malicious application detection. IEEE Trans. Inf. Forensics Secur. 9 (11), 1869–1882 (2014).
Ayar, M., Isazadeh, A., Gharehchopogh, F. S. & Seyedi, M. NSICA: Multi-objective imperialist competitive algorithm for feature selection in arrhythmia diagnosis. Comput. Biol. Med. 161 , 107025 (2023).
Article CAS PubMed Google Scholar
Hu, H. et al. Dynamic individual selection and crossover boosted forensic-based investigation algorithm for global optimization and feature selection. J. Bionic Eng. 20 , 1–27 (2023).
Zhong, C., Li, G., Meng, Z., Li, H. & He, W. A self-adaptive quantum equilibrium optimizer with artificial bee colony for feature selection. Comput. Biol. Med. 153 , 106520 (2023).
Zhou, P. et al. Unsupervised feature selection for balanced clustering. Knowl.-Based Syst. 193 , 105417 (2020).
Allix, K. et al. Empirical assessment of machine learning-based malware detectors for android. Empir. Softw. Eng. 21 (1), 183–211 (2016).
Narayanan, A., Chandramohan, M., Chen, L. & Liu, Y. A multi-view context-aware approach to android malware detection and malicious code localization. Empir. Softw. Eng. 23 (3), 1222–1274 (2018).
Azmoodeh, A., Dehghantanha, A. & Choo, K. K. R. Robust malware detection for internet of (battlefield) things devices using deep eigenspace learning. IEEE Trans. Sustain. Comput. 4 (1), 88–95 (2018).
Chen, K. Z., Johnson, N. M., D’Silva, V., Dai, S., MacNamara, K., Magrino, T. R., Wu, E. X., Rinard, M. & Song, D. X. Contextual policy enforcement in android applications with permission event graphs. In: NDSS , 234 (2013).
Yerima, S. Y., Sezer, S. & McWilliams, G. Analysis of Bayesian classification-based approaches for android malware detection. IET Inf. Secur. 8 (1), 25–36 (2014).
Gonzalez, H., Stakhanova, N. & Ghorbani, A. A. Droidkin: Lightweight detection of android apps similarity. In International Conference on Security and Privacy in Communication Networks , 436–453 (Springer, 2014) .
Kadir, A. F. A., Stakhanova, N. & Ghorbani, A. A. Android botnets: What urls are telling us. In International Conference on Network and System Security , 78–91 (Springer, 2015).
Zhou, Y. & Jiang, X. Android malware genome project. Disponibile a http://www.malgenomeproject.org (2012).
Garcia, J., Hammad, M. & Malek, S. Lightweight, obfuscation-resilient detection and family identification of android malware. ACM Trans. Softw. Eng. Methodol. (TOSEM) 26 (3), 1–29 (2018).
Mahindru, A. & Sangal, A. Parudroid: Validation of android malware detection dataset. J. Cybersecur. Inform. Manag. 3 (2), 42–52 (2020).
McCulloch, W. S. & Pitts, W. A logical calculus of the ideas immanent in nervous activity. Bull. Math. Biophys. 5 (4), 115–133 (1943).
Article MathSciNet Google Scholar
Faruk, M. J. H., Shahriar, H., Valero, M., Barsha, F. L., Sobhan, S., Khan, M. A., Whitman, M., Cuzzocrea, A., Lo, D., Rahman, A., et al . Malware detection and prevention using artificial intelligence techniques. In 2021 IEEE International Conference on Big Data (Big Data) , 5369–5377 (IEEE, 2021).
Battiti, R. First-and second-order methods for learning: Between steepest descent and newton’s method. Neural Comput. 4 (2), 141–166 (1992).
Levenberg, K. A method for the solution of certain non-linear problems in least squares. Q. Appl. Math. 2 (2), 164–168 (1944).
Bengio, Y. Learning deep architectures for AI. Found. Trends ® Mach. Learn. 2 (1), 1–127 (2009).
Kaur, J., Singh, S., Kahlon, K. S. & Bassi, P. Neural network-a novel technique for software effort estimation. Int. J. Comput. Theory Eng. 2 (1), 17 (2010).
Doraisamy, S., Golzari, S., Mohd, N., Sulaiman, M. N. & Udzir, N. I. A study on feature selection and classification techniques for automatic genre classification of traditional Malay music. In ISMIR , 331–336 (2008).
Forman, G. An extensive empirical study of feature selection metrics for text classification. J. Mach. Learn. Res. 3 (Mar), 1289–1305 (2003).
Furlanello, C., Serafini, M., Merler, S. & Jurman, G. Entropy-based gene ranking without selection bias for the predictive classification of microarray data. BMC Bioinform. 4 (1), 54 (2003).
Coronado-De-Alba, L. D., Rodríguez-Mota, A. & Escamilla-Ambrosio, P. J. Feature selection and ensemble of classifiers for android malware detection. In 2016 8th IEEE Latin-American Conference on Communications (LATINCOM) , 1–6 (IEEE, 2016).
Deepa, K., Radhamani, G. & Vinod, P. Investigation of feature selection methods for android malware analysis. Procedia Comput. Sci. 46 , 841–848 (2015).
Kothari, C. R. Research methodology: Methods and techniques. New Age International (2004).
Chaikla, N. & Qi, Y. Genetic algorithms in feature selection. In IEEE SMC’99 Conference Proceedings. 1999 IEEE International Conference on Systems, Man, and Cybernetics (Cat. No. 99CH37028) , vol 5, 538–540 (IEEE, 1999).
Onwuzurike, L. et al. Mamadroid: Detecting android malware by building Markov chains of behavioral models (extended version). ACM Trans. Privacy Secur. (TOPS) 22 (2), 1–34 (2019).
Hou, S., Ye, Y., Song, Y. & Abdulhayoglu, M. Hindroid: An intelligent android malware detection system based on structured heterogeneous information network. In Proceedings of the 23rd ACM SIGKDD International Conference on Knowledge Discovery and Data Mining , 1507–1515 (2017) .
Zhu, H. J. et al. HEMD: A highly efficient random forest-based malware detection framework for android. Neural Comput. Appl. 30 (11), 3353–3361 (2018).
Wang, W., Zhao, M. & Wang, J. Effective android malware detection with a hybrid model based on deep autoencoder and convolutional neural network. J. Ambient. Intell. Humaniz. Comput. 10 (8), 3035–3043 (2019).
Han, W., Xue, J., Wang, Y., Liu, Z. & Kong, Z. Malinsight: A systematic profiling based malware detection framework. J. Netw. Comput. Appl. 125 , 236–250 (2019).
Zou, D. et al. Intdroid: Android malware detection based on API intimacy analysis. ACM Trans. Softw. Eng. Methodol. (TOSEM) 30 (3), 1–32 (2021).
Mahindru, A. & Arora, H. Dnndroid: Android malware detection framework based on federated learning and edge computing. In International Conference on Advancements in Smart Computing and Information Security , 96–107 (Springer, 2022).
Mahindru, A. & Arora, H. Parudroid: Framework that enhances smartphone security using an ensemble learning approach. SN Comput. Sci. 4 (5), 630 (2023).
Mahindru, A., Sharma, S. K. & Mittal, M. Yarowskydroid: Semi-supervised based android malware detection using federation learning. In 2023 International Conference on Advancement in Computation & Computer Technologies (InCACCT) , 380–385 (IEEE, 2023).
Download references
Acknowlegment
This work was partly supported by the Technology Innovation Program funded by the Ministry of Trade, Industry & Energy (MOTIE) (No.20022899) and by the Technology Development Program of MSS (No.S3033853).
Author information
Authors and affiliations.
Department of Computer Science and applications, D.A.V. University, Sarmastpur, Jalandhar, 144012, India
Arvind Mahindru
Department of Mathematics, Guru Nanak Dev University, Amritsar, India
Himani Arora
Department of Nuclear and Renewable Energy, Ural Federal University Named after the First President of Russia Boris Yeltsin, Ekaterinburg, Russia, 620002
Abhinav Kumar
Department of Electronics and Communication Engineering, Central University of Jammu, Jammu, 181143, UT of J&K, India
Sachin Kumar Gupta
School of Electronics and Communication Engineering, Shri Mata Vaishno Devi University, Katra, 182320, UT of J&K, India
Department of Applied Data Science, Noroff University College, Kristiansand, Norway
Shubham Mahajan & Seifedine Kadry
Artificial Intelligence Research Center (AIRC), Ajman University, Ajman, 346, United Arab Emirates
Seifedine Kadry
MEU Research Unit, Middle East University, Amman 11831, Jordan
Applied Science Research Center, Applied Science Private University, Amman, Jordan
Department of Software, Department of Computer Science and Engineering, Kongju National University, Cheonan, 31080, Korea
Jungeun Kim
You can also search for this author in PubMed Google Scholar
Contributions
All the authors have contributed equally.
Corresponding authors
Correspondence to Arvind Mahindru , Sachin Kumar Gupta , Shubham Mahajan or Jungeun Kim .
Ethics declarations
Competing interests.
The authors declare no competing interests.
Additional information
Publisher's note.
Springer Nature remains neutral with regard to jurisdictional claims in published maps and institutional affiliations.
Supplementary Information
Supplementary information., rights and permissions.
Open Access This article is licensed under a Creative Commons Attribution 4.0 International License, which permits use, sharing, adaptation, distribution and reproduction in any medium or format, as long as you give appropriate credit to the original author(s) and the source, provide a link to the Creative Commons licence, and indicate if changes were made. The images or other third party material in this article are included in the article’s Creative Commons licence, unless indicated otherwise in a credit line to the material. If material is not included in the article’s Creative Commons licence and your intended use is not permitted by statutory regulation or exceeds the permitted use, you will need to obtain permission directly from the copyright holder. To view a copy of this licence, visit http://creativecommons.org/licenses/by/4.0/ .
Reprints and permissions
About this article
Cite this article.
Mahindru, A., Arora, H., Kumar, A. et al. PermDroid a framework developed using proposed feature selection approach and machine learning techniques for Android malware detection. Sci Rep 14 , 10724 (2024). https://doi.org/10.1038/s41598-024-60982-y
Download citation
Received : 14 October 2023
Accepted : 29 April 2024
Published : 10 May 2024
DOI : https://doi.org/10.1038/s41598-024-60982-y
Share this article
Anyone you share the following link with will be able to read this content:
Sorry, a shareable link is not currently available for this article.
Provided by the Springer Nature SharedIt content-sharing initiative
- Android apps
- Neural network
- Deep learning
- Feature selection
- Intrusion detection
- Permissions model
By submitting a comment you agree to abide by our Terms and Community Guidelines . If you find something abusive or that does not comply with our terms or guidelines please flag it as inappropriate.
Quick links
- Explore articles by subject
- Guide to authors
- Editorial policies
Sign up for the Nature Briefing newsletter — what matters in science, free to your inbox daily.


1. Introduction
2. results and discussion, 3. conclusions, 4. related literature, supporting information.

research papers \(\def\hfill{\hskip 5em}\def\hfil{\hskip 3em}\def\eqno#1{\hfil {#1}}\)

Analysis of COF-300 synthesis: probing degradation processes and 3D electron diffraction structure
a XStruct, Department of Chemistry, Ghent University, Krijgslaan 281–S3, 9000 Ghent, Belgium, b COMOC – Center for Ordered Materials, Organometallics and Catalysis – Department of Chemistry, Ghent University, Krijgslaan 281–S3, 9000 Ghent, Belgium, c Rigaku Corporation, Haijima, Tokyo, Japan, and d Rigaku Europe SE, Neu-Isenburg, Germany * Correspondence e-mail: [email protected]
Although COF-300 is often used as an example to study the synthesis and structure of (3D) covalent organic frameworks (COFs), knowledge of the underlying synthetic processes is still fragmented. Here, an optimized synthetic procedure based on a combination of linker protection and modulation was applied. Using this approach, the influence of time and temperature on the synthesis of COF-300 was studied. Synthesis times that were too short produced materials with limited crystallinity and porosity, lacking the typical pore flexibility associated with COF-300. On the other hand, synthesis times that were too long could be characterized by loss of crystallinity and pore order by degradation of the tetrakis(4-aminophenyl)methane (TAM) linker used. The presence of the degradation product was confirmed by visual inspection, Raman spectroscopy and X-ray photoelectron spectroscopy (XPS). As TAM is by far the most popular linker for the synthesis of 3D COFs, this degradation process might be one of the reasons why the development of 3D COFs is still lagging compared with 2D COFs. However, COF crystals obtained via an optimized procedure could be structurally probed using 3D electron diffraction (3DED). The 3DED analysis resulted in a full structure determination of COF-300 at atomic resolution with satisfying data parameters. Comparison of our 3DED-derived structural model with previously reported single-crystal X-ray diffraction data for this material, as well as parameters derived from the Cambridge Structural Database, demonstrates the high accuracy of the 3DED method for structure determination. This validation might accelerate the exploitation of 3DED as a structure determination technique for COFs and other porous materials.
Keywords: 3D electron diffraction ; 3DED ; microcrystal electron diffraction ; microED ; covalent organic frameworks ; Cambridge Structural Database ; porous organic solids ; crystallization and crystal growth .
CCDC reference: 2321626
In Figs. S6–S8, the effect of time on I 65°C (as discussed earlier) is compared with the effect on the other samples. Note that, as expected, I RT is slower to form a crystalline material compared with I 65°C due to the reduced error correction at room temperature, with reflections appearing after 1 d, and fully developed crystallinity after 5 d. However, the pore structure never fully establishes, as indicated by the broad, late and small second step in the N 2 -sorption isotherm. The appearance of crystallinity in C 65°C is even more delayed, with no crystalline reflections observed after 1 d of reaction time, indicating the superiority of the intermediate-assisted procedure. Here, maximal crystallinity is observed after 5 d, as peaks start to broaden significantly after 7 d. Surprisingly, the best N 2 -sorption behaviour was observed for the 7 d sample, indicating that the relationship between crystallinity and porosity is not always straightforward. Finally, using the conditions of C RT, we were unable to form any crystalline material, even after 7 d of reaction time. We also checked if the scale of the synthesis had any influence on the material. Therefore, a sample (I 65°C ×5) was prepared in an identical way to I 65°C but with every quantity used multiplied by 5. The resulting PXRD patterns and the N 2 -sorption isotherms are presented in Fig. S9 and show no significant influence on the crystallinity and a small decrease of porosity (with a BET surface area of 1180 m 2 g −1 and V p of 0.71 obtained for I 65°C ×5).
The response of COF-300 to an intermediate-assisted synthesis protocol was studied by careful evaluation of the evolution of both crystallinity and porosity as functions of reaction time and temperature. Kinetic studies among four different synthesis conditions revealed three distinct stages in the synthesis of COF-300, namely a network build-up phase at short synthetic times (≤1 d) with low crystallinity and no pore flexibility, followed by an optimal stage (3 d) characterized by high crystallinity and porosity before partial breakdown by TAM degradation (≥5 d). This degradation process could be confirmed in both control experiments as well as the obtained COF materials and can easily be estimated by the observation of magenta-coloured reaction mixtures. As a pronounced influence of this degradation reaction on both crystallinity and porosity was observed and most 3D COFs are based on the TAM linker, knowledge of TAM degradation in a acidic environment is of utmost importance for the synthesis of high-quality 3D COFs. Knowledge of this degradation process might help to increase the synthetic toolbox for 3D COFs (which are mainly based on the TAM linker), which is still lacking compared with 2D COFs. However, using the optimized conditions, a reliable crystal structure of COF-300 could be readily obtained via 3DED analysis, indicating single crystallinity of the synthesized materials. The structure model obtained showed high completeness and comparable resolution and R values. Comparison with an SCXRD structure model as well as with data for similar chemical functionalities in the CSD database showed no significant differences, supporting that 3DED is a reliable and fast technique for the structure solution of COFs. As SCXRD structure solution is hardly possible and PXRD models often show ambiguity in structure determination, 3DED might play an important role in the future of COFs with better accessibility of 3DED diffraction equipment and improving dynamic refinement algorithms.
Crystal structure: contains datablock 1. DOI: https://doi.org/10.1107/S2052252524003713/vq5005sup1.cif
Structure factors: contains datablock 1. DOI: https://doi.org/10.1107/S2052252524003713/vq5005sup2.hkl
Supporting Information - revised - highlighted. DOI: https://doi.org/10.1107/S2052252524003713/vq5005sup3.pdf
Acknowledgements
The authors thank Karen Leus for the XPS measurements and Dieter Buyst for the solid-state NMR measurements.
Funding information
LB acknowledges Ghent University (UGent) for funding. PVDV acknowledges financial support through UGent concerted action (grant No. 01G01017) and the Fonds Wetenschappelijk Onderzoek (FWO)–Vlaanderen project (grant Nos. 3G020521 awarded to PVDV; 1275221N awarded to SB and KVH). Gas sorption and powder X-ray diffraction were made possible through UGent (grant Nos. 01B00215; BOF20/BAS/015 awarded to PVDV). The spectrometer electronics, magnet and accessories used for solid-state NMR measurements, including the BBI and high-gradient diffusion probe, were funded by the Hercules foundation (grant No. AUGE/09/2006); the solid-state (CP-MAS) and HR-MAS expansion were made possible by FWO (grant No. I006920N).
This is an open-access article distributed under the terms of the Creative Commons Attribution (CC-BY) Licence , which permits unrestricted use, distribution, and reproduction in any medium, provided the original authors and source are cited.
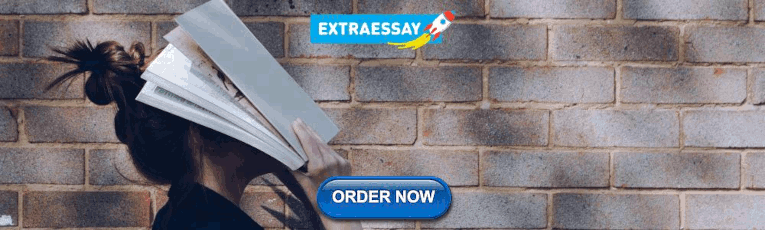
COMMENTS
The development and validation of analytical methods is a constant and interdependent effort that falls within the purview of the research and development, quality control, and quality assurance ...
integral part of any sensible analytical practice. Validation of analytical strategies is also needed by most rules and quality standards that impact laboratories [6]. Analytical method development When there are no definitive techniques are present, new methodologies are being progressed for evaluation of the novel product.
Chemistry Research Journal, 2020, 5(3):173-186 Chemistry Research Journal 173 Available online www.chemrj.org Re ISSN: 2455 view Article-8990 CODEN(USA): CRJHA5 Analytical Method Development and Validation: A Review Shashi Daksh*, Anju Goyal *B.N. Institute of Pharmaceutical Sciences, Faculty of Pharmacy, B N University, Udaipur, ...
An effective analytical method development and its validation can provide significant improvements in precision and a reduction in bias errors and can further help to avoid costly and time consuming exercises. Analytical method development and validation are the continuous and inter-dependent task associated with the research and development, quality control and quality assurance departments.
Approval of analytical method gives information about various stages and parameters like accuracy, precision, linearity, Limit Of Detection, Limit of Quantification, specificity, range and robustness. Development and validation of analytical method play an essential role in the discovery, development and manufacturing of pharmaceuticals. Every year, number of drugs entered into the market ...
The analytical method validation activity is not a one-time study. This is illustrated and summarized in the life cycle of an analytical procedure in Figure 1 . An analytical method will be developed and validated for use to analyze samples during the early development of an active pharmaceutical ingredient or drug product.
The development of sound Analytical method is of supreme importance during the process of drug discovery, release to market and development, culminating in a marketing approval. The objective of this paper is to review the method development, optimize and validation of the method for the drug product from the developmental stage of the ...
The common method for the development and validation of the analytical method is completed by the following process [1]. 1) Planning the appropriate method that must be developed. 2) The information related to the work should be collected. 3) Qualitative and quantitative analytical methods that can be performed in the lab should be developed.
Design of experiments (DOE)-based analytical quality by design (AQbD) method evaluation, development, and validation is gaining momentum and has the potential to create robust chromatographic methods through deeper understanding and control of variability. In this paper, a case study is used to explore the pros, cons, and pitfalls of using various chromatographic responses as modeling targets ...
analytical method development and its validation can provide significant improvements in precision and a reduction in bias errors. It can further help to avoid costly and time consuming exercises. Keyword: validation, cGMP, GLP, analytical method. Introduction: Quantification and qualification are examined in the analysis of chemical.
In order to get to the stage in method development where method robustness can be verified, multivariate design of experiments (DoE) should be used to systematically investigate cause-and-effect relationships between experimental method parameters and method performance. An enhanced development approach provides greater opportunities for ...
Given (1) the historical context for the terms verification and validation in software and hardware standards, regulations, and guidances, and (2) the separated concepts of analytical and clinical ...
Analytical method development and validation plays a crucial role in ensuring the accuracy, reliability, and fitness-for-purpose of ... Education and Research (DU), Porur, Chennai-600116. Article Received on 25 July 2023, Revised on 15 August 2023, Accepted on 05 Sept. 2023
and/or Office of Communication, Outreach and Development Center for Biologics Evaluation and Research Food and Drug Administration 10903 New Hampshire Ave., Bldg. 71, Room 3128 Silver Spring, MD ...
Step 1: Define the Analytical Method Objectives. The first step in analytical method development and validation is to define the analytical method objectives, including the attribute to be measured, the acceptance criteria, and the intended use of the method. This step involves understanding the critical quality attributes (CQAs) of the drug ...
The Shodhganga@INFLIBNET Centre provides a platform for research students to deposit their Ph.D. theses and make it available to the entire scholarly community in open access. ... Analytical method development and validation of selected drugs by rp hplc: Researcher: M.Deepa: Guide(s): Dr. K. Ravindra Reddy and Prof. S.V. Satyanarayana:
Analytical Method Development and Validation of Pharmaceutical Analysis Using Chromatographic Techniques - A Special Issue published by Hindawi ... - Research Article; Development and Validation of Selective High-Performance Liquid Chromatographic Method Using Photodiode Array Detection for Estimation of Aconitine in Polyherbal Ayurvedic Taila ...
Analytical method development and validation for the academic researcher. I. Krull, M. Swartz. Published 1999. Chemistry. Analytical Letters. INTRODUCTION During the course of our scientific careers, and after reading numerous original research papers and reviews submitted for publication to various journals or books, it has become apparent to ...
In this research paper, ten-fold cross-validation technique is implemented to verify the develop model 16. Further, outliers are eliminated, which effect the performance of the proposed framework.
Taxus, as a globally prevalent evergreen tree, contains a wealth of bioactive components that play a crucial role in the pharmaceutical field. Taxus extracts, defined as a collection of one or more bioactive compounds extracted from the genus Taxus spp., have become a significant focus of modern cancer treatment research. This review article aims to delve into the scientific background of ...
With the rapid development of artificial intelligence in recent years, intelligent evaluation of college students' growth by means of the monitoring data from training processes is becoming a promising technique in the field intelligent education. Current studies, however, tend to utilize course grades, which are objective, to predict students' grade-point averages (GPAs), but usually ...
The 3DED analysis resulted in a full structure determination of COF-300 at atomic resolution with satisfying data parameters. Comparison of our 3DED-derived structural model with previously reported single-crystal X-ray diffraction data for this material, as well as parameters derived from the Cambridge Structural Database, demonstrates the ...
A validated rapid high-performance liquid chromatography (HPLC)-refractive index (RI) method was developed for the identification and quantification of glucose and xylose in hydrolyzed bagasse extract. The separation of compounds was achieved on Eurokat® H column (300 × 8 mm, 10 μm) at 75°C, using 0.01 N sulfuric acid solution as mobile phase and 0.6 mL/min as flow rate. The method was ...
RT-qPCR analysis was performed and the results were converted into relative gene expression by delta-delta Ct method p test analysis was performed to verify the correlation between the relative gene expression and the transcriptome data (TPM). ... This work was supported by the Key-Area Research and Development Program of Guangdong Province (NO ...
Apart from that, new methods based on offline analytical techniques, to analyse trivalent and hexavalent chromium separately, are still under development. Therefore, one of the objectives of this paper is to review these recently published analytical methods and assess whether they are fit for chromium speciation analysis in foodstuffs.