
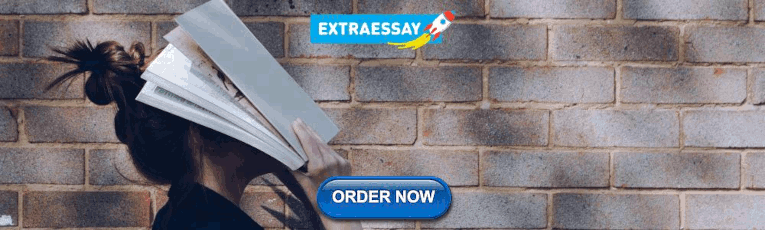
NLP & Speech
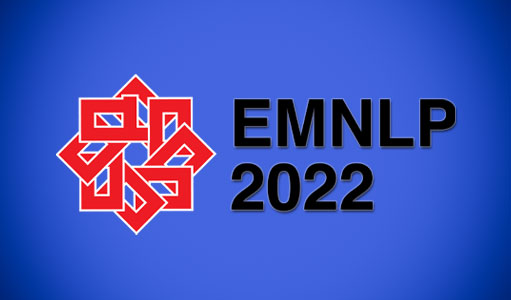
Papers from CS researchers were accepted to the Empirical Methods in Natural Language Processing (EMNLP) 2022.
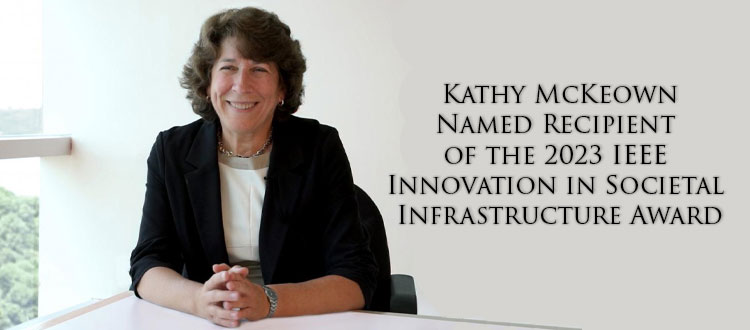
McKeown receives the award for pushing the boundaries of natural language processing for social media analysis, news summarization, crisis informatics, and creating a digital library for patient care.
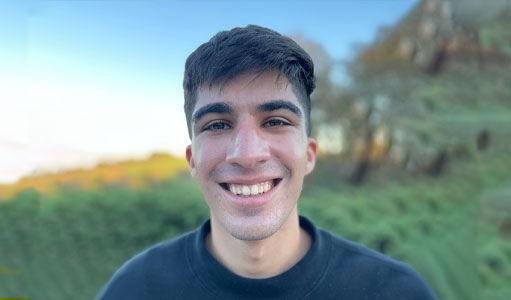
The CS undergrad shares how he started doing research in the Speech Lab and won a Best Paper award at EMNLP 2021.
The Speech and Natural Language Processing groups do fundamental work in language understanding and generation with applications to a wide variety of topics, including summarization, argumentation, persuasion, sentiment, detecting deceptive, emotional and charismatic speech, text-to-speech synthesis, analysis of social media to detect mental illness, abusive language, and radicalization.
The groups collaborate closely on many research projects with each other, with language faculty in other universities, and with Columbia faculty in other disciplines. They also mentor a very large number of master’s and undergraduate research project students who participate in their research each semester. They have regular talks for faculty, students, and the larger New York area community.
Faculty and Affiliates
Research Labs
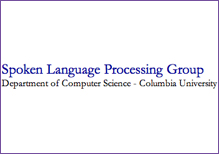
Find open faculty positions here .
Computer Science at Columbia University
Upcoming events, making elections more trustworthy (and trusted).
Distinguished Lecture Series
Monday 11:40 am
CSB 451 CS Auditorium
Matt Blaze, Georgetown University
Virtual Employer Info Session: North Atlantic Industries Inc.
Friday 3:00 pm
, CS Careers
Academic Holiday
Monday 9:00 am
Election Day - University Holiday
Tuesday 9:00 am
In the News
Press mentions, dean boyce's statement on amicus brief filed by president bollinger.
President Bollinger announced that Columbia University along with many other academic institutions (sixteen, including all Ivy League universities) filed an amicus brief in the U.S. District Court for the Eastern District of New York challenging the Executive Order regarding immigrants from seven designated countries and refugees. Among other things, the brief asserts that “safety and security concerns can be addressed in a manner that is consistent with the values America has always stood for, including the free flow of ideas and people across borders and the welcoming of immigrants to our universities.”
This recent action provides a moment for us to collectively reflect on our community within Columbia Engineering and the importance of our commitment to maintaining an open and welcoming community for all students, faculty, researchers and administrative staff. As a School of Engineering and Applied Science, we are fortunate to attract students and faculty from diverse backgrounds, from across the country, and from around the world. It is a great benefit to be able to gather engineers and scientists of so many different perspectives and talents – all with a commitment to learning, a focus on pushing the frontiers of knowledge and discovery, and with a passion for translating our work to impact humanity.
I am proud of our community, and wish to take this opportunity to reinforce our collective commitment to maintaining an open and collegial environment. We are fortunate to have the privilege to learn from one another, and to study, work, and live together in such a dynamic and vibrant place as Columbia.
Mary C. Boyce Dean of Engineering Morris A. and Alma Schapiro Professor

{{title}} {{fullname}}
Courses This Semester
- {{title}} ({{dept}} {{prefix}}{{course_num}}-{{section}})
- Who’s Teaching What
- Subject Updates
- MEng program
- Opportunities
- Minor in Computer Science
- Resources for Current Students
- Program objectives and accreditation
- Graduate program requirements
- Admission process
- Degree programs
- Graduate research
- EECS Graduate Funding
- Resources for current students
- Student profiles
- Instructors
- DEI data and documents
- Recruitment and outreach
- Community and resources
- Get involved / self-education
- Rising Stars in EECS
- Graduate Application Assistance Program (GAAP)
- MIT Summer Research Program (MSRP)
- Sloan-MIT University Center for Exemplary Mentoring (UCEM)
- Electrical Engineering
- Computer Science
- Artificial Intelligence + Decision-making
- AI and Society
- AI for Healthcare and Life Sciences
- Artificial Intelligence and Machine Learning
- Biological and Medical Devices and Systems
- Communications Systems
- Computational Biology
- Computational Fabrication and Manufacturing
- Computer Architecture
- Educational Technology
- Electronic, Magnetic, Optical and Quantum Materials and Devices
- Graphics and Vision
- Human-Computer Interaction
- Information Science and Systems
- Integrated Circuits and Systems
- Nanoscale Materials, Devices, and Systems
- Natural Language and Speech Processing
- Optics + Photonics
- Optimization and Game Theory
- Programming Languages and Software Engineering
- Quantum Computing, Communication, and Sensing
- Security and Cryptography
- Signal Processing
- Systems and Networking
- Systems Theory, Control, and Autonomy
- Theory of Computation
- Departmental History
- Departmental Organization
- Visiting Committee
- Explore all research areas
Our research encompasses all aspects of speech and language processing—ranging from the design of fundamental machine learning methods to the design of advanced applications that can extract information from documents, translate between languages, and execute instructions in real-world environments. Two central themes of our research are unsupervised discovery of linguistic structure (from sounds to word meanings to grammars) and the use of language to train and explain computational models across application domains (including computer vision, robotics, and medicine). We aim to simultaneously tackle pressing social problems and develop foundational technologies for enabling humans to interact with computers using the languages they already speak.
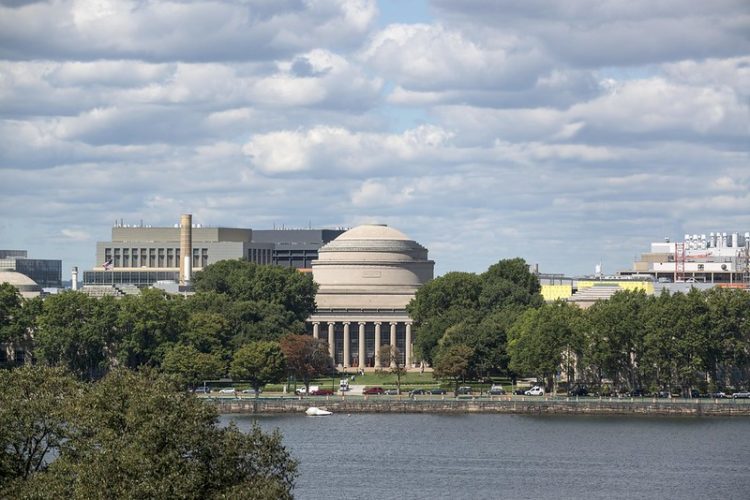
Latest news in natural language and speech processing
Mit researchers use large language models to flag problems in complex systems.
The approach can detect anomalies in data recorded over time, without the need for any training.
QS ranks MIT the world’s No. 1 university for 2024-25
Ranking at the top for the 13th year in a row, the Institute also places first in 11 subject areas.
Creating bespoke programming languages for efficient visual AI systems
Associate Professor Jonathan Ragan-Kelley optimizes how computer graphics and images are processed for the hardware of today and tomorrow.
QS World University Rankings rates MIT No. 1 in 11 subjects for 2024
The Institute also ranks second in five subject areas.
Department of EECS Announces 2024 Promotions
The Department of Electrical Engineering and Computer Science (EECS) is proud to announce multiple promotions.
Upcoming events
Doctoral thesis: expanding the design space of vertical gallium nitride power devices.

- Schools & departments

Speech and Language Processing MSc
Awards: MSc
Study modes: Full-time, Part-time
Funding opportunities
Postgraduate Virtual Open Days
Join us online on 12 to 14 November where you can learn more about postgraduate study through webinars, live panel sessions, and one-to-one chat.
Find out more and register
Programme description
This intensive programme covers all areas of speech and language processing, from phonetics, speech synthesis and speech recognition to natural language generation, machine translation and generative AI. You will have an exciting opportunity to learn from world leaders in both informatics and linguistics.
The programme provides research and vocational training and can be either freestanding or used as preparation for a PhD. The modular nature allows you to tailor it to your own interests.
Key research areas
This programme combines elements of linguistics, artificial intelligence, computer science, and engineering and is taught by leading researchers from Linguistics & English Language, the Centre for Speech Technology Research, and the School of Informatics.
In the most recent Research Excellence Framework (REF 2021), Linguistics research at Edinburgh is ranked 3rd in the UK (Times Higher Education, Modern Languages and Linguistics, Rank order by GPA).
What will I learn
You will develop up-to-date knowledge of a broad range of areas in speech and language processing and gain the technical expertise and hands-on skills required to carry out research and development in this challenging interdisciplinary area.
This programme covers all areas of speech and language processing, from the foundations of phonetics, speech technology and natural language understanding, to the latest methods using large generative speech and language models.
The flexible nature of the programme allows students to take courses ranging across other areas of linguistics, informatics, and cognitive science.
Is this MSc for me
This programme is best suited to applicants whose academic background is in:
- linguistics
- computer science
- engineering
- cognitive science
It provides specialist knowledge and is an excellent preparation for subsequent research, whether you choose to pursue this in academia or in a professional career.
Reputation, relevance and employability
Edinburgh has a proud and distinguished history of teaching in speech and language processing and you will be taught by world-leading experts.
You will benefit from the breadth and strength of the interdisciplinary academic community at Edinburgh, having the opportunity to select option courses and attend research seminars across different disciplines.
Our students’ dissertation projects are sometimes published in academic conferences or journals.
Our programme has an excellent reputation amongst employers. Many of our graduates have gone onto PhD training, either directly or after a period in industry.
- Find out more about our community
The School of Philosophy, Psychology and Language Sciences is home to a large, supportive and active student community, hosting events and activities throughout the year which you can join. As a postgraduate student, you will have access to a range of research resources, state-of-the-art facilities research, seminars, and reading groups.
Programme structure
This programme contains two semesters of taught core courses and optional courses followed by a dissertation project.
The taught courses and their assessments take place from September to December (Semester 1) and January to April (Semester 2). Dissertation topics are assigned at the start of Semester 2 and the project is carried out from June to August.
Core courses
Most core compulsory courses have both computational and mathematical content. A few optional courses need a stronger mathematical background. The core courses are:
- Computer Programming for Speech and Language Processing
- Accelerated Natural Language Processing
- Speech Processing
- Statistics and Quantitative Methods
- Research Ethics Training in Linguistics and English Language
Optional courses
The optional courses offer you the opportunity to explore areas of your interest. Examples of optional courses are:
- Natural Language Understanding, Generation, and Machine Translation
- Automatic Speech Recognition
- Speech Synthesis
- Machine Learning in Signal Processing
- Simulating Language
- Phonetics and Laboratory Phonology
- Speech Production and Perception
- The Human Factor: Working with Users
Dissertation
The dissertation involves a practical project under the guidance of an expert supervisor.
Past examples of dissertation topics include:
- Multi-Task Learning Approaches to Accented Speech Recognition
- Using i-vectors in Speaker Adaptive Speech Synthesis for Disordered Speech
- Multimodal Emotion Recognition: an Assessment of Deep Learning Approaches
- A Sequence-to-Sequence Neural Network for English Past-tense Inflection
- Hate Speech Detection on Twitter
- Controlling Prosody in Speech Synthesis Systems
- Knowledge-lean Approaches to Metonymy
How will I learn
Courses are typically delivered using combinations of live and pre-recorded lectures and seminars/tutorials. The number of contact hours and the teaching format will depend to some extent on the option courses chosen.
After classes finish in April, you will spend all your time working independently on coursework, exam revision and on your dissertation. When you carry out your supervised dissertation project, you will receive guidance from your supervisor through one-to-one meetings, comments on written work and email communication.
Find out more about compulsory and optional courses
We link to the latest information available. Please note that this may be for a previous academic year and should be considered indicative.
Learning outcomes
This programme aims to equip you with the technical knowledge and practical skills required to carry out research and development in the challenging interdisciplinary arena of speech and language technology.
You will learn about state-of-the-art techniques in:
- speech synthesis
- automatic speech recognition
- natural language processing
- natural language generation
- machine translation
You will also learn the theory behind such technologies and gain the practical experience of working with and developing real systems based on these technologies.
Career opportunities
This programme is ideal preparation for a PhD or for working in industry and teaches a broad range of transferable skills applicable to a wide range of modern jobs as well as further academic research and training.
Our graduates
The analytical skills you develop and the research training you receive will be valuable in a wide range of careers. In addition to a number of our graduates going onto further academic research opportunities, some recent examples of positions they have gone on to work for companies including:
- British Telecom
- EF Education First
- Phonetic Arts
- Sensory Inc.
- Speechmatics
Several have started up companies, some directly after the MSc, including:
- Openhearted
Many graduates will go on to study for a PhD, some examples of where they have gone on to study are:
- Northwestern University
- Technische Universität München
- University of Cambridge
- University of Edinburgh
- University of Padua
Former students have research or faculty positions at universities including:
- Macquarie University
- Pennsylvania State University
- RIKEN Brain Science Institute
- Stanford University
- University of British Columbia
- University of Manchester
- University of Wellington, New Zealand
- Vrije Universiteit Brussel
- Careers Service
Our award-winning Careers Service plays an essential part in your wider student experience at the University, providing:
- tailored advice
- individual guidance and personal assistance
- access to the experience of our worldwide alumni network
We invest in your future beyond the end of your degree. Studying at the University of Edinburgh will lay the foundations for your future success, whatever shape that takes.
Important application information
Your application and personal statement allow us to make sure that you and your chosen MSc are a good fit, and that you will have a productive and successful year at Edinburgh.
We strongly recommend you apply as early as possible. You should generally avoid applying to more than one degree with the same School. Applicants who can demonstrate their understanding of, and commitment to, a specific programme are preferred.
There are typically around 35 places available on the programme each year. We anticipate awarding most places in the first three batches.
Personal statements
When applying, you should include a personal statement detailing your academic abilities and your reasons for applying for the programme
The personal statement helps us decide whether you are right for the MSc programme you have selected, but just as importantly, it helps us decide whether the MSc programme is right for you.
Your personal statement should include:
- What makes this particular MSc programme interesting for you?
- What are the most important things you want to gain from the MSc programme?
- What are the key courses you have taken and that are relevant for this specific programme and what are your academic abilities?
- Any other information which you feel will help us ensure that you are a good match to your intended MSc programme.
A good personal statement can make a big difference to the admissions process as it may be the only opportunity to explain why you are an ideal candidate for the programme.
You will be asked to add contact details for your referees. We will email them with information on how to upload their reference directly to your online application. Alternatively, they can email their comments to:
Preparing a strong application
One factor used in the admissions process is your level of preparation for the programme. Whilst we do not expect an advanced level of preparation at the time of application, the majority of successful applicants do demonstrate that they have actively started to prepare. The programme director provides some suggestions of things you can do before applying and after receiving an offer. You can describe your level of preparation in your personal statement.
- Prepare for study in speech and language processing
Find out more about the general application process for postgraduate programmes:
- How to apply
Language Sciences at Edinburgh
Entry requirements.
These entry requirements are for the 2025/26 academic year and requirements for future academic years may differ. Entry requirements for the 2026/27 academic year will be published on 1 Oct 2025.
A UK 2:1 degree, or its international equivalent, in linguistics, computer science, engineering, psychology, philosophy, mathematics or a related subject. Your application should show evidence of a basic understanding of linguistics, mathematics and computer science and you must be willing to undertake further study to prepare you for the programme.
You can increase your chances of a successful application by exceeding the minimum programme requirements.
Students from China
This degree is Band C.
- Postgraduate entry requirements for students from China
International qualifications
Check whether your international qualifications meet our general entry requirements:
- Entry requirements by country
- English language requirements
Regardless of your nationality or country of residence, you must demonstrate a level of English language competency which will enable you to succeed in your studies.
English language tests
We accept the following English language qualifications at the grades specified:
- IELTS Academic: total 7.0 with at least 6.5 in each component. We do not accept IELTS One Skill Retake to meet our English language requirements.
- TOEFL-iBT (including Home Edition): total 100 with at least 23 in each component. We do not accept TOEFL MyBest Score to meet our English language requirements.
- C1 Advanced ( CAE ) / C2 Proficiency ( CPE ): total 185 with at least 176 in each component.
- Trinity ISE : ISE III with passes in all four components.
- PTE Academic: total 73 with at least 65 in each component. We do not accept PTE Academic Online..
- Oxford ELLT : 8 overall with at least 7 in each component. Your English language qualification must be no more than three and a half years old from the start date of the programme you are applying to study, unless you are using IELTS , TOEFL, Trinity ISE or PTE , in which case it must be no more than two years old.
Degrees taught and assessed in English
We also accept an undergraduate or postgraduate degree that has been taught and assessed in English in a majority English speaking country, as defined by UK Visas and Immigration:
- UKVI list of majority English speaking countries
We also accept a degree that has been taught and assessed in English from a university on our list of approved universities in non-majority English speaking countries (non-MESC).
- Approved universities in non-MESC
If you are not a national of a majority English speaking country, then your degree must be no more than five years old at the beginning of your programme of study.
Find out more about our language requirements:
Fees and costs
Scholarships and funding.
Funding for postgraduate study is different to undergraduate study, and many students need to combine funding sources to pay for their studies.
Most students use a combination of the following funding to pay their tuition fees and living costs:
borrowing money
taking out a loan
family support
personal savings
income from work
employer sponsorship
- scholarships
Explore sources of funding for postgraduate study
There are a number of highly competitive scholarships and funding options available to MSc students.
Deadlines for funding applications vary for each funding source - please make sure to check the specific deadlines for the funding opportunities you wish to apply for and make sure that you submit your application in good time.
UK government postgraduate loans
If you live in the UK, you may be able to apply for a postgraduate loan from one of the UK’s governments.
The type and amount of financial support you are eligible for will depend on:
- your programme
- the duration of your studies
- your tuition fee status
Programmes studied on a part-time intermittent basis are not eligible.
- UK government and other external funding
Other funding opportunities
Search for scholarships and funding opportunities:
- Search for funding
Further information
- PPLS Postgraduate Office
- Phone: +44 (0)131 651 5002
- Contact: [email protected]
- Programme Director, Professor Simon King
- Contact: [email protected]
- Dugald Stewart Building
- 3 Charles Street
- Central Campus
- School: Philosophy, Psychology & Language Sciences
- College: Arts, Humanities & Social Sciences
Select your programme and preferred start date to begin your application.
MSc Speech and Language Processing - 1 Year (Full-time)
Msc speech and language processing - 2 years (part-time), application deadlines.
We operate a gathered field approach to applications for MSc Speech and Language Processing. You may submit your application at any time.
All complete applications that satisfy our minimum entry requirements will be placed on a shortlist and held until the next batch processing deadline. Applications will then be ranked and offers made to the top candidates.
Exceptionally well-qualified applicants may occasionally be made an immediate offer, without entering the shortlisting process.
Applications are held for processing over the following deadlines:
If you are also applying for funding or will require a visa then we strongly recommend you apply as early as possible.
Places available
There are typically up to 40 places available on the programme each year. We anticipate awarding most places in the first three batches.
Supporting documents and references
Applications must be complete with all supporting documentation to be passed on for consideration; this includes references.
Please ensure that you inform referees of any deadline you wish to meet.
You must submit two references with your application.
Please read through the ‘Important application information’ section on this page before applying.
- Log in
- Site search
Computer Science with Speech and Language Processing
Entry requirements.
Minimum 2:1 undergraduate honours degree in a relevant subject.
Subject requirements
We accept degrees in the following subject areas:
- Computer Science
- Mathematics
- Any Engineering subject
We may also consider degrees in Linguistics or Psychology.
Months of entry
Course content.
Speech and language technology is being transformed by exciting developments in artificial intelligence, including deep learning and generative models such as ChatGPT.
Our world-leading speech and language research staff will help you to understand these technologies and develop the skills needed to apply them to real-world problems.
Applying for this course
We use a staged admissions process to assess applications for this course. You'll still apply for this course in the usual way, using our Postgraduate Online Application Form.
Accreditation
This course is accredited by the British Computer Society (BCS). The course partially meets the requirements for Chartered Information Technology Professional (CITP) and partially meets the requirements for Chartered Engineer (CEng).
Please see our University website for the most up-to-date course information: https://www.sheffield.ac.uk/postgraduate/taught/courses
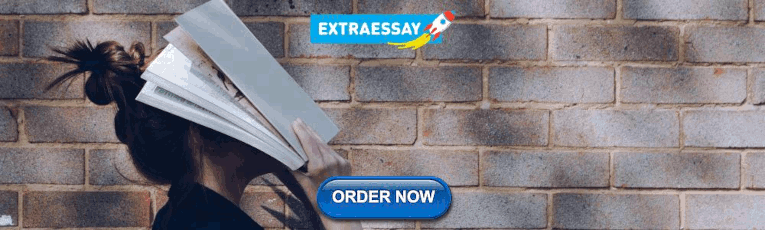
Information for international students
English language requirements
IELTS 6.5 (with 6 in each component) or University equivalent.
Other English language qualifications we accept
Fees and funding
https://www.sheffield.ac.uk/international/fees-and-funding/tuition-fees
Qualification, course duration and attendance options
- Campus-based learning is available for this qualification
Course contact details
Our cookies
We use cookies for three reasons: to give you the best experience on PGS, to make sure the PGS ads you see on other sites are relevant , and to measure website usage. Some of these cookies are necessary to help the site work properly and can’t be switched off. Cookies also support us to provide our services for free, and by click on “Accept” below, you are agreeing to our use of cookies .You can manage your preferences now or at any time.
Privacy overview
We use cookies, which are small text files placed on your computer, to allow the site to work for you, improve your user experience, to provide us with information about how our site is used, and to deliver personalised ads which help fund our work and deliver our service to you for free.
The information does not usually directly identify you, but it can give you a more personalised web experience.
You can accept all, or else manage cookies individually. However, blocking some types of cookies may affect your experience of the site and the services we are able to offer.
You can change your cookies preference at any time by visiting our Cookies Notice page. Please remember to clear your browsing data and cookies when you change your cookies preferences. This will remove all cookies previously placed on your browser.
For more detailed information about the cookies we use, or how to clear your browser cookies data see our Cookies Notice
Manage consent preferences
Strictly necessary cookies
These cookies are necessary for the website to function and cannot be switched off in our systems.
They are essential for you to browse the website and use its features.
You can set your browser to block or alert you about these cookies, but some parts of the site will not then work. We can’t identify you from these cookies.
Functional cookies
These help us personalise our sites for you by remembering your preferences and settings. They may be set by us or by third party providers, whose services we have added to our pages. If you do not allow these cookies, then these services may not function properly.
Performance cookies
These cookies allow us to count visits and see where our traffic comes from, so we can measure and improve the performance of our site. They help us to know which pages are popular and see how visitors move around the site. The cookies cannot directly identify any individual users.
If you do not allow these cookies we will not know when you have visited our site and will not be able to improve its performance for you.
Marketing cookies
These cookies may be set through our site by social media services or our advertising partners. Social media cookies enable you to share our content with your friends and networks. They can track your browser across other sites and build up a profile of your interests. If you do not allow these cookies you may not be able to see or use the content sharing tools.
Advertising cookies may be used to build a profile of your interests and show you relevant adverts on other sites. They do not store directly personal information, but work by uniquely identifying your browser and internet device. If you do not allow these cookies, you will still see ads, but they won’t be tailored to your interests.
Computer Science with Speech and Language Processing MSc
University of sheffield, different course options.
- Key information
Course Summary
Tuition fees, entry requirements, similar courses at different universities, key information data source : idp connect, qualification type.
MSc - Master of Science
Subject areas
Speech / Voice Processing (Computer) Speech Therapy
Course type
Learn skills that will change lives from experts in the fields of speech technology and natural language processing., course description.
Speech and language technology is being transformed by exciting developments in artificial intelligence, including deep learning and generative models such as ChatGPT.
Our world-leading speech and language research staff will help you to understand these technologies and develop the skills needed to apply them to real-world problems.
Applying for this course
We use a staged admissions process to assess applications for this course. You'll still apply for this course in the usual way, using our Postgraduate Online Application Form.
Accreditation
This course is accredited by the British Computer Society (BCS). The course partially meets the requirements for Chartered Information Technology Professional (CITP) and partially meets the requirements for Chartered Engineer (CEng).
Please see our University website for the most up-to-date course information.
UK fees Course fees for UK students
For this course (per year)
International fees Course fees for EU and international students
Minimum 2:1 undergraduate honours degree in a relevant subject, such as computer science, engineering, linguistics, mathematics or psychology.
Vision, Speech and Signal Processing PhD
University of surrey.
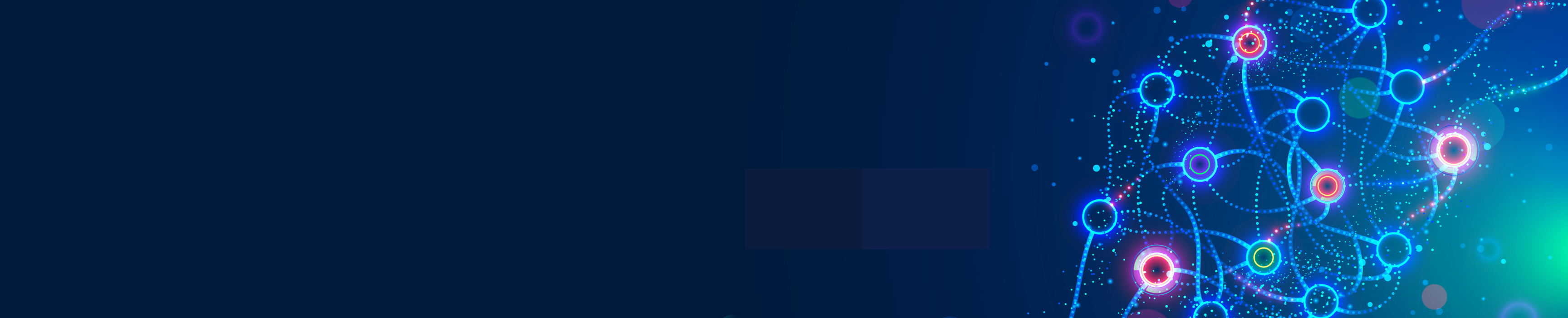
Natural Language Processing
Introduction.
Natural Language Processing (NLP) is one of the hottest areas of artificial intelligence (AI) thanks to applications like text generators that compose coherent essays, chatbots that fool people into thinking they’re sentient, and text-to-image programs that produce photorealistic images of anything you can describe. Recent years have brought a revolution in the ability of computers to understand human languages, programming languages, and even biological and chemical sequences, such as DNA and protein structures, that resemble language. The latest AI models are unlocking these areas to analyze the meanings of input text and generate meaningful, expressive output.
What is Natural Language Processing (NLP)
Natural language processing (NLP) is the discipline of building machines that can manipulate human language — or data that resembles human language — in the way that it is written, spoken, and organized. It evolved from computational linguistics, which uses computer science to understand the principles of language, but rather than developing theoretical frameworks, NLP is an engineering discipline that seeks to build technology to accomplish useful tasks. NLP can be divided into two overlapping subfields: natural language understanding (NLU), which focuses on semantic analysis or determining the intended meaning of text, and natural language generation (NLG), which focuses on text generation by a machine. NLP is separate from — but often used in conjunction with — speech recognition, which seeks to parse spoken language into words, turning sound into text and vice versa.
Why Does Natural Language Processing (NLP) Matter?
NLP is an integral part of everyday life and becoming more so as language technology is applied to diverse fields like retailing (for instance, in customer service chatbots) and medicine (interpreting or summarizing electronic health records). Conversational agents such as Amazon’s Alexa and Apple’s Siri utilize NLP to listen to user queries and find answers. The most sophisticated such agents — such as GPT-3, which was recently opened for commercial applications — can generate sophisticated prose on a wide variety of topics as well as power chatbots that are capable of holding coherent conversations. Google uses NLP to improve its search engine results , and social networks like Facebook use it to detect and filter hate speech .
NLP is growing increasingly sophisticated, yet much work remains to be done. Current systems are prone to bias and incoherence, and occasionally behave erratically. Despite the challenges, machine learning engineers have many opportunities to apply NLP in ways that are ever more central to a functioning society.
What is Natural Language Processing (NLP) Used For?
NLP is used for a wide variety of language-related tasks, including answering questions, classifying text in a variety of ways, and conversing with users.
Here are 11 tasks that can be solved by NLP:
- Sentiment analysis is the process of classifying the emotional intent of text. Generally, the input to a sentiment classification model is a piece of text, and the output is the probability that the sentiment expressed is positive, negative, or neutral. Typically, this probability is based on either hand-generated features, word n-grams, TF-IDF features, or using deep learning models to capture sequential long- and short-term dependencies. Sentiment analysis is used to classify customer reviews on various online platforms as well as for niche applications like identifying signs of mental illness in online comments.

- Toxicity classification is a branch of sentiment analysis where the aim is not just to classify hostile intent but also to classify particular categories such as threats, insults, obscenities, and hatred towards certain identities. The input to such a model is text, and the output is generally the probability of each class of toxicity. Toxicity classification models can be used to moderate and improve online conversations by silencing offensive comments , detecting hate speech , or scanning documents for defamation .
- Machine translation automates translation between different languages. The input to such a model is text in a specified source language, and the output is the text in a specified target language. Google Translate is perhaps the most famous mainstream application. Such models are used to improve communication between people on social-media platforms such as Facebook or Skype. Effective approaches to machine translation can distinguish between words with similar meanings . Some systems also perform language identification; that is, classifying text as being in one language or another.
- Named entity recognition aims to extract entities in a piece of text into predefined categories such as personal names, organizations, locations, and quantities. The input to such a model is generally text, and the output is the various named entities along with their start and end positions. Named entity recognition is useful in applications such as summarizing news articles and combating disinformation . For example, here is what a named entity recognition model could provide:
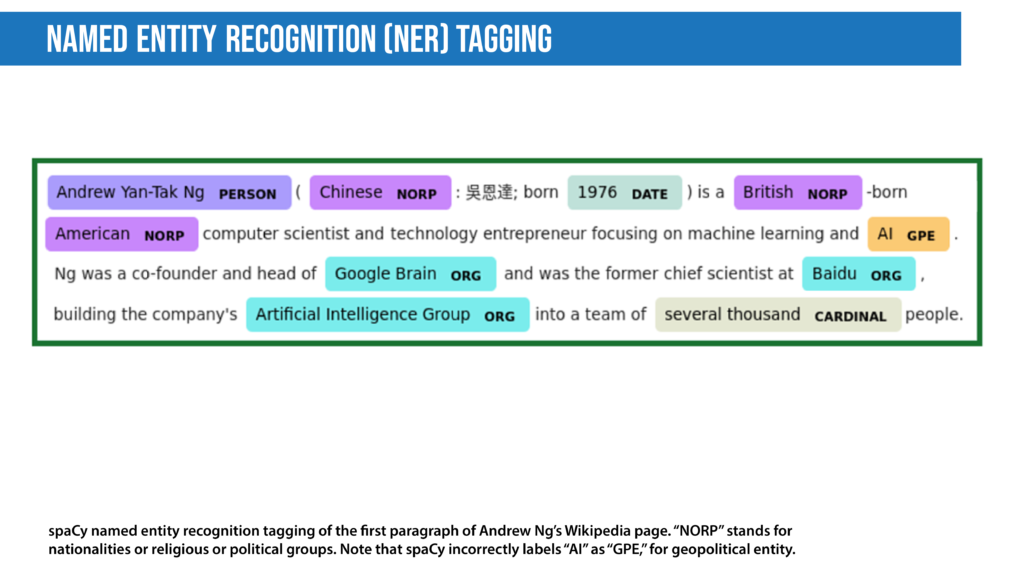
- Spam detection is a prevalent binary classification problem in NLP, where the purpose is to classify emails as either spam or not. Spam detectors take as input an email text along with various other subtexts like title and sender’s name. They aim to output the probability that the mail is spam. Email providers like Gmail use such models to provide a better user experience by detecting unsolicited and unwanted emails and moving them to a designated spam folder.
- Grammatical error correction models encode grammatical rules to correct the grammar within text. This is viewed mainly as a sequence-to-sequence task, where a model is trained on an ungrammatical sentence as input and a correct sentence as output. Online grammar checkers like Grammarly and word-processing systems like Microsoft Word use such systems to provide a better writing experience to their customers. Schools also use them to grade student essays .
- Topic modeling is an unsupervised text mining task that takes a corpus of documents and discovers abstract topics within that corpus. The input to a topic model is a collection of documents, and the output is a list of topics that defines words for each topic as well as assignment proportions of each topic in a document. Latent Dirichlet Allocation (LDA), one of the most popular topic modeling techniques, tries to view a document as a collection of topics and a topic as a collection of words. Topic modeling is being used commercially to help lawyers find evidence in legal documents .
- Autocomplete predicts what word comes next, and autocomplete systems of varying complexity are used in chat applications like WhatsApp. Google uses autocomplete to predict search queries. One of the most famous models for autocomplete is GPT-2, which has been used to write articles , song lyrics , and much more.
- Database query: We have a database of questions and answers, and we would like a user to query it using natural language.
- Conversation generation: These chatbots can simulate dialogue with a human partner. Some are capable of engaging in wide-ranging conversations . A high-profile example is Google’s LaMDA, which provided such human-like answers to questions that one of its developers was convinced that it had feelings .
- Information retrieval finds the documents that are most relevant to a query. This is a problem every search and recommendation system faces. The goal is not to answer a particular query but to retrieve, from a collection of documents that may be numbered in the millions, a set that is most relevant to the query. Document retrieval systems mainly execute two processes: indexing and matching. In most modern systems, indexing is done by a vector space model through Two-Tower Networks, while matching is done using similarity or distance scores. Google recently integrated its search function with a multimodal information retrieval model that works with text, image, and video data.
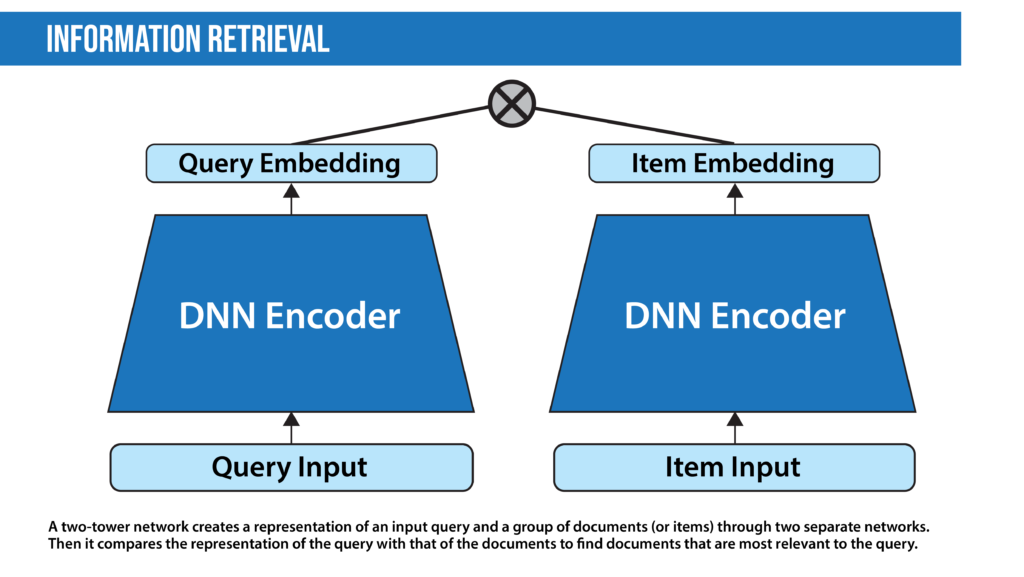
- Extractive summarization focuses on extracting the most important sentences from a long text and combining these to form a summary. Typically, extractive summarization scores each sentence in an input text and then selects several sentences to form the summary.
- Abstractive summarization produces a summary by paraphrasing. This is similar to writing the abstract that includes words and sentences that are not present in the original text. Abstractive summarization is usually modeled as a sequence-to-sequence task, where the input is a long-form text and the output is a summary.
- Multiple choice: The multiple-choice question problem is composed of a question and a set of possible answers. The learning task is to pick the correct answer.
- Open domain : In open-domain question answering, the model provides answers to questions in natural language without any options provided, often by querying a large number of texts.
How Does Natural Language Processing (NLP) Work?
NLP models work by finding relationships between the constituent parts of language — for example, the letters, words, and sentences found in a text dataset. NLP architectures use various methods for data preprocessing, feature extraction, and modeling. Some of these processes are:
- Stemming and lemmatization : Stemming is an informal process of converting words to their base forms using heuristic rules. For example, “university,” “universities,” and “university’s” might all be mapped to the base univers . (One limitation in this approach is that “universe” may also be mapped to univers , even though universe and university don’t have a close semantic relationship.) Lemmatization is a more formal way to find roots by analyzing a word’s morphology using vocabulary from a dictionary. Stemming and lemmatization are provided by libraries like spaCy and NLTK.
- Sentence segmentation breaks a large piece of text into linguistically meaningful sentence units. This is obvious in languages like English, where the end of a sentence is marked by a period, but it is still not trivial. A period can be used to mark an abbreviation as well as to terminate a sentence, and in this case, the period should be part of the abbreviation token itself. The process becomes even more complex in languages, such as ancient Chinese, that don’t have a delimiter that marks the end of a sentence.
- Stop word removal aims to remove the most commonly occurring words that don’t add much information to the text. For example, “the,” “a,” “an,” and so on.
- Tokenization splits text into individual words and word fragments. The result generally consists of a word index and tokenized text in which words may be represented as numerical tokens for use in various deep learning methods. A method that instructs language models to ignore unimportant tokens can improve efficiency.
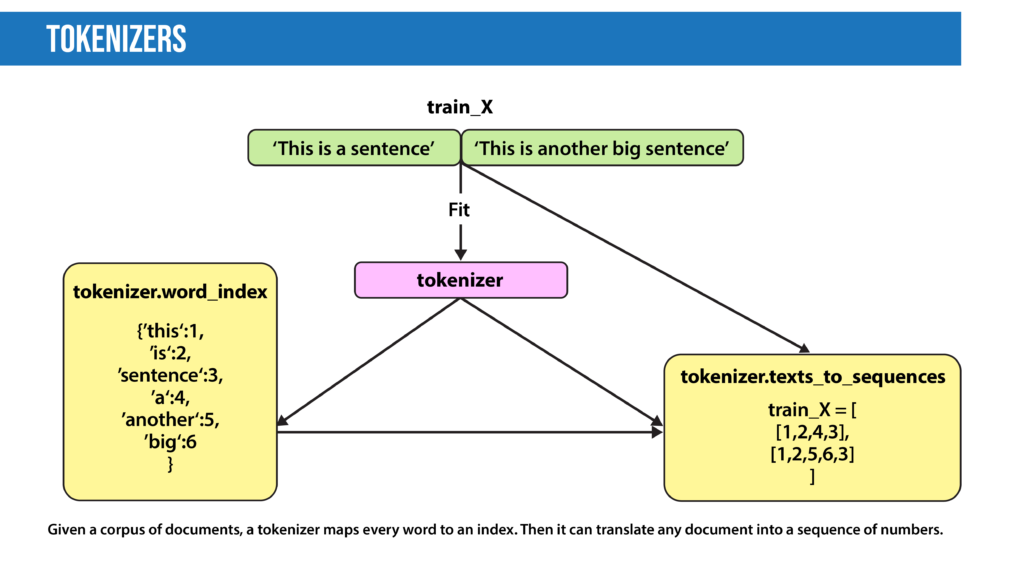
- Bag-of-Words: Bag-of-Words counts the number of times each word or n-gram (combination of n words) appears in a document. For example, below, the Bag-of-Words model creates a numerical representation of the dataset based on how many of each word in the word_index occur in the document.
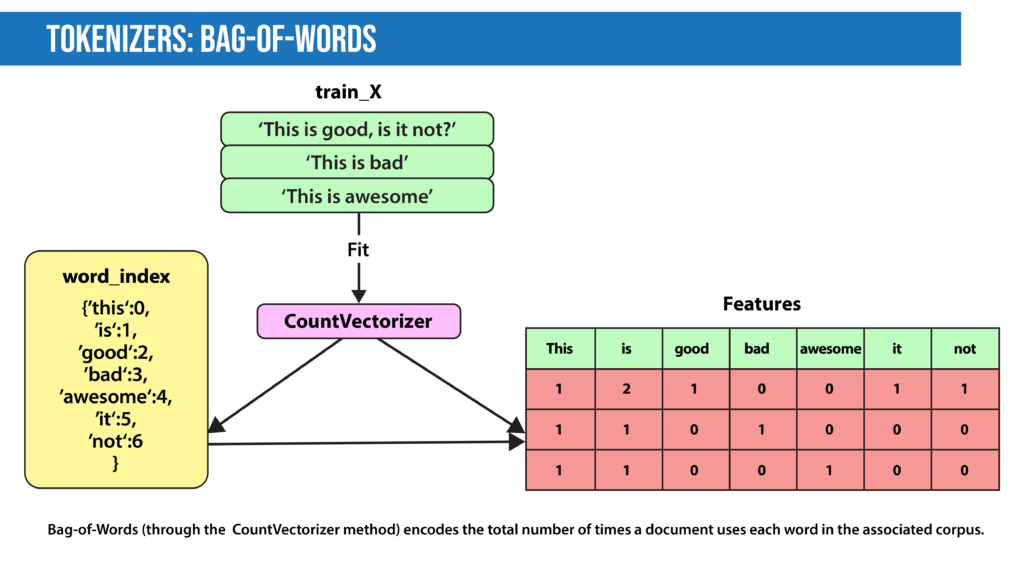
- Term Frequency: How important is the word in the document?
TF(word in a document)= Number of occurrences of that word in document / Number of words in document
- Inverse Document Frequency: How important is the term in the whole corpus?
IDF(word in a corpus)=log(number of documents in the corpus / number of documents that include the word)
A word is important if it occurs many times in a document. But that creates a problem. Words like “a” and “the” appear often. And as such, their TF score will always be high. We resolve this issue by using Inverse Document Frequency, which is high if the word is rare and low if the word is common across the corpus. The TF-IDF score of a term is the product of TF and IDF.
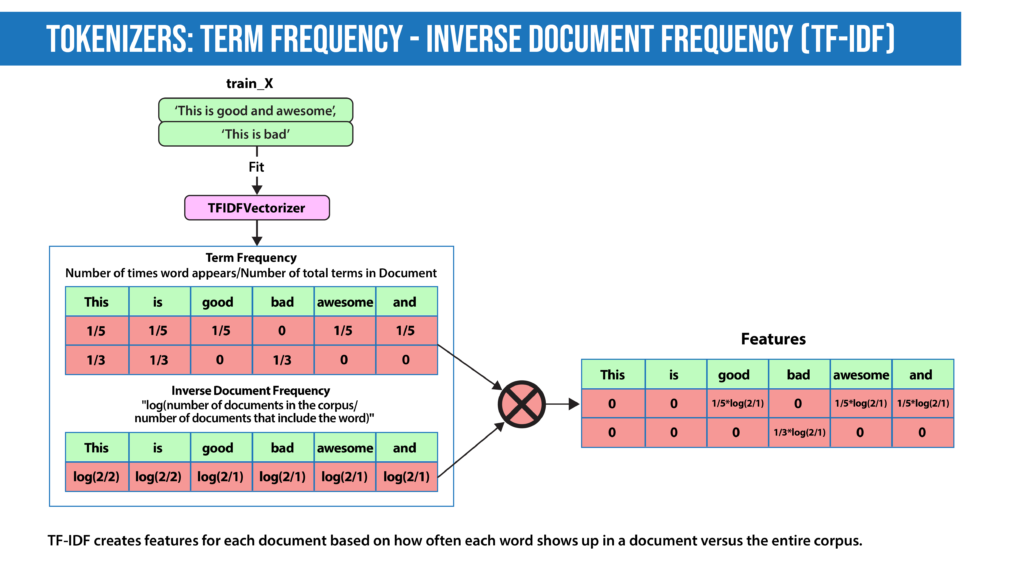
- Word2Vec , introduced in 2013 , uses a vanilla neural network to learn high-dimensional word embeddings from raw text. It comes in two variations: Skip-Gram, in which we try to predict surrounding words given a target word, and Continuous Bag-of-Words (CBOW), which tries to predict the target word from surrounding words. After discarding the final layer after training, these models take a word as input and output a word embedding that can be used as an input to many NLP tasks. Embeddings from Word2Vec capture context. If particular words appear in similar contexts, their embeddings will be similar.
- GLoVE is similar to Word2Vec as it also learns word embeddings, but it does so by using matrix factorization techniques rather than neural learning. The GLoVE model builds a matrix based on the global word-to-word co-occurrence counts.
- Numerical features extracted by the techniques described above can be fed into various models depending on the task at hand. For example, for classification, the output from the TF-IDF vectorizer could be provided to logistic regression, naive Bayes, decision trees, or gradient boosted trees. Or, for named entity recognition, we can use hidden Markov models along with n-grams.
- Deep neural networks typically work without using extracted features, although we can still use TF-IDF or Bag-of-Words features as an input.
- Language Models : In very basic terms, the objective of a language model is to predict the next word when given a stream of input words. Probabilistic models that use Markov assumption are one example:
P(W n )=P(W n |W n−1 )
Deep learning is also used to create such language models. Deep-learning models take as input a word embedding and, at each time state, return the probability distribution of the next word as the probability for every word in the dictionary. Pre-trained language models learn the structure of a particular language by processing a large corpus, such as Wikipedia. They can then be fine-tuned for a particular task. For instance, BERT has been fine-tuned for tasks ranging from fact-checking to writing headlines .
Top Natural Language Processing (NLP) Techniques
Most of the NLP tasks discussed above can be modeled by a dozen or so general techniques. It’s helpful to think of these techniques in two categories: Traditional machine learning methods and deep learning methods.
Traditional Machine learning NLP techniques:
- Logistic regression is a supervised classification algorithm that aims to predict the probability that an event will occur based on some input. In NLP, logistic regression models can be applied to solve problems such as sentiment analysis, spam detection, and toxicity classification.
- Naive Bayes is a supervised classification algorithm that finds the conditional probability distribution P(label | text) using the following Bayes formula:
P(label | text) = P(label) x P(text|label) / P(text)
and predicts based on which joint distribution has the highest probability. The naive assumption in the Naive Bayes model is that the individual words are independent. Thus:
P(text|label) = P(word_1|label)*P(word_2|label)*…P(word_n|label)
In NLP, such statistical methods can be applied to solve problems such as spam detection or finding bugs in software code .
- Decision trees are a class of supervised classification models that split the dataset based on different features to maximize information gain in those splits.
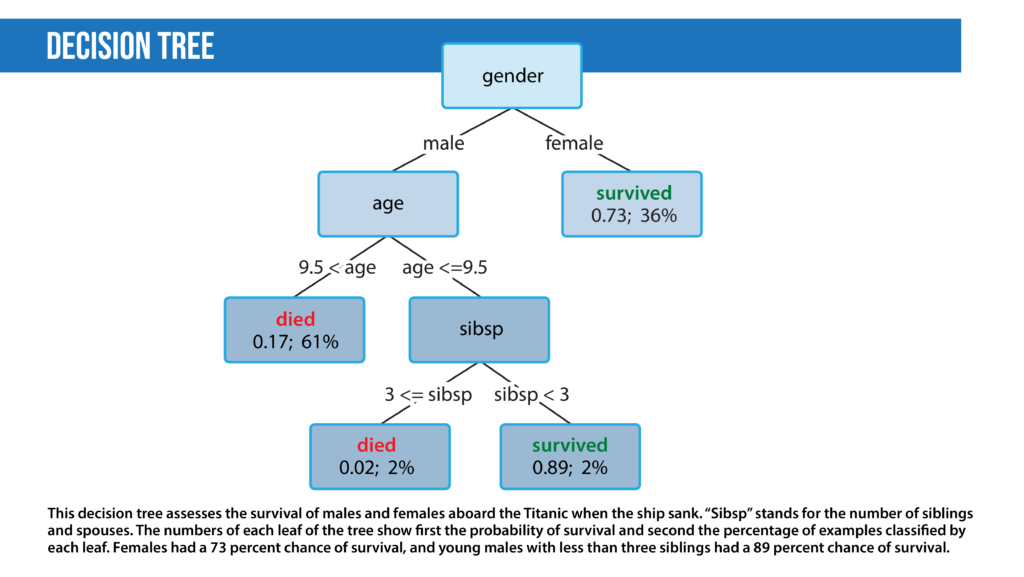
- Latent Dirichlet Allocation (LDA) is used for topic modeling. LDA tries to view a document as a collection of topics and a topic as a collection of words. LDA is a statistical approach. The intuition behind it is that we can describe any topic using only a small set of words from the corpus.
- Hidden Markov models : Markov models are probabilistic models that decide the next state of a system based on the current state. For example, in NLP, we might suggest the next word based on the previous word. We can model this as a Markov model where we might find the transition probabilities of going from word1 to word2, that is, P(word1|word2). Then we can use a product of these transition probabilities to find the probability of a sentence. The hidden Markov model (HMM) is a probabilistic modeling technique that introduces a hidden state to the Markov model. A hidden state is a property of the data that isn’t directly observed. HMMs are used for part-of-speech (POS) tagging where the words of a sentence are the observed states and the POS tags are the hidden states. The HMM adds a concept called emission probability; the probability of an observation given a hidden state. In the prior example, this is the probability of a word, given its POS tag. HMMs assume that this probability can be reversed: Given a sentence, we can calculate the part-of-speech tag from each word based on both how likely a word was to have a certain part-of-speech tag and the probability that a particular part-of-speech tag follows the part-of-speech tag assigned to the previous word. In practice, this is solved using the Viterbi algorithm.
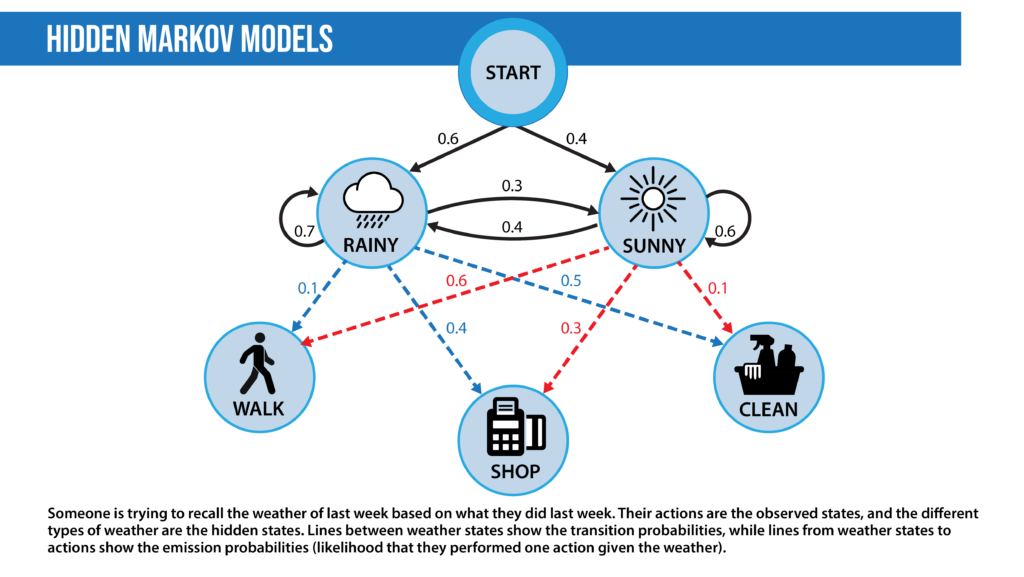
Deep learning NLP Techniques:
- Convolutional Neural Network (CNN): The idea of using a CNN to classify text was first presented in the paper “ Convolutional Neural Networks for Sentence Classification ” by Yoon Kim. The central intuition is to see a document as an image. However, instead of pixels, the input is sentences or documents represented as a matrix of words.
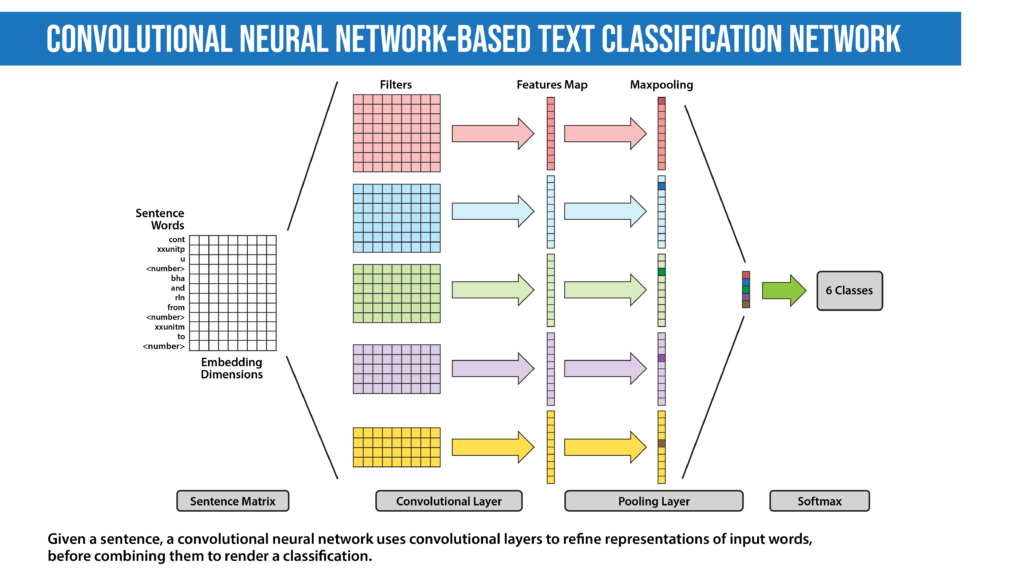
- Recurrent Neural Network (RNN) : Many techniques for text classification that use deep learning process words in close proximity using n-grams or a window (CNNs). They can see “New York” as a single instance. However, they can’t capture the context provided by a particular text sequence. They don’t learn the sequential structure of the data, where every word is dependent on the previous word or a word in the previous sentence. RNNs remember previous information using hidden states and connect it to the current task. The architectures known as Gated Recurrent Unit (GRU) and long short-term memory (LSTM) are types of RNNs designed to remember information for an extended period. Moreover, the bidirectional LSTM/GRU keeps contextual information in both directions, which is helpful in text classification. RNNs have also been used to generate mathematical proofs and translate human thoughts into words.
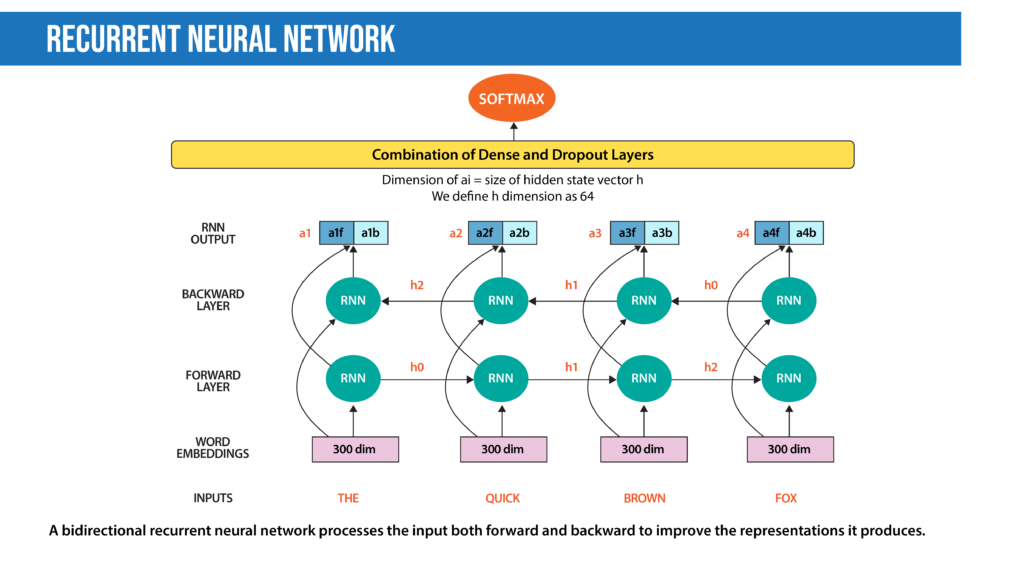
- Autoencoders are deep learning encoder-decoders that approximate a mapping from X to X, i.e., input=output. They first compress the input features into a lower-dimensional representation (sometimes called a latent code, latent vector, or latent representation) and learn to reconstruct the input. The representation vector can be used as input to a separate model, so this technique can be used for dimensionality reduction. Among specialists in many other fields, geneticists have applied autoencoders to spot mutations associated with diseases in amino acid sequences.
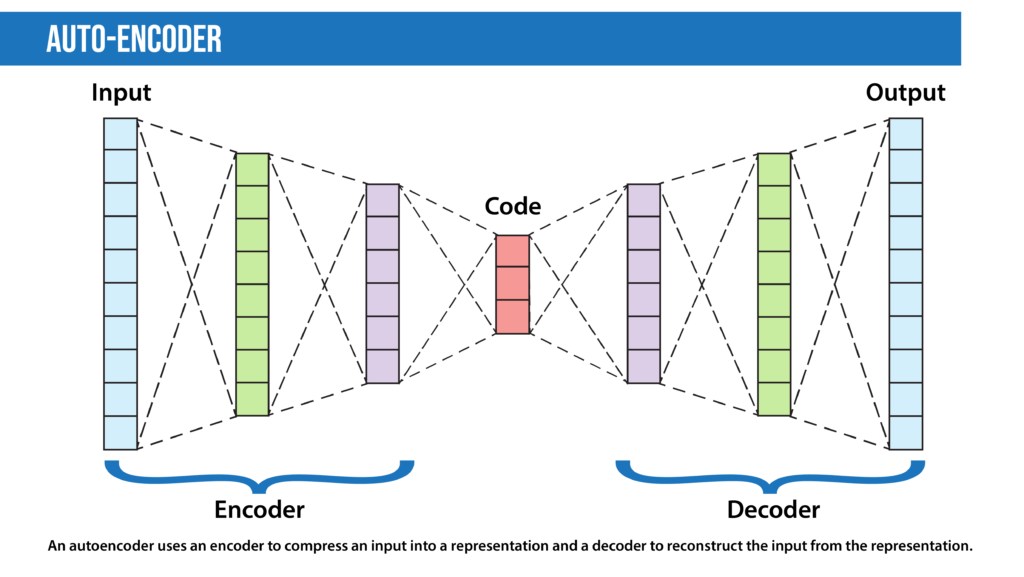
- Encoder-decoder sequence-to-sequence : The encoder-decoder seq2seq architecture is an adaptation to autoencoders specialized for translation, summarization, and similar tasks. The encoder encapsulates the information in a text into an encoded vector. Unlike an autoencoder, instead of reconstructing the input from the encoded vector, the decoder’s task is to generate a different desired output, like a translation or summary.
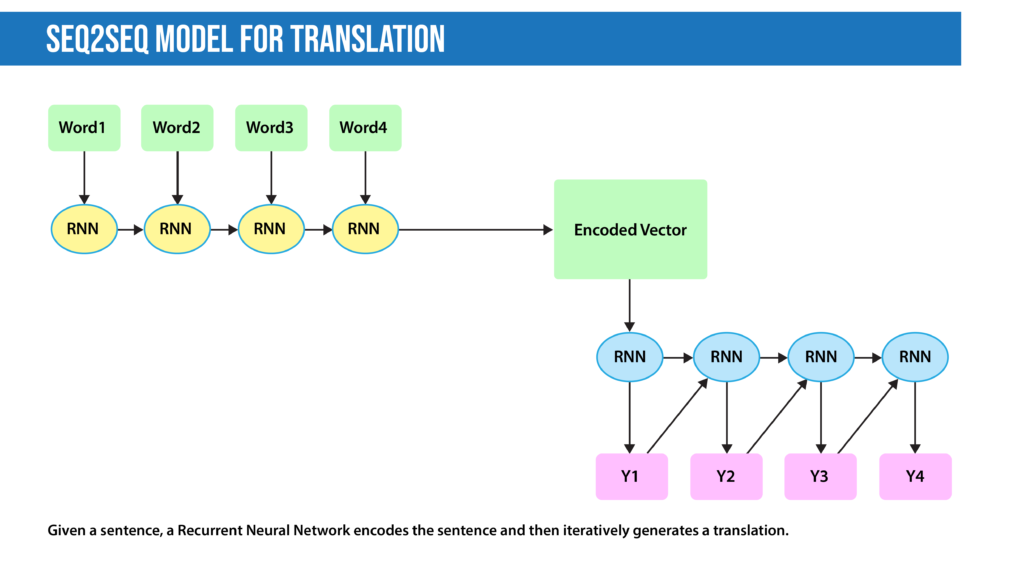
- Transformers : The transformer, a model architecture first described in the 2017 paper “ Attention Is All You Need ” (Vaswani, Shazeer, Parmar, et al.), forgoes recurrence and instead relies entirely on a self-attention mechanism to draw global dependencies between input and output. Since this mechanism processes all words at once (instead of one at a time) that decreases training speed and inference cost compared to RNNs, especially since it is parallelizable. The transformer architecture has revolutionized NLP in recent years, leading to models including BLOOM , Jurassic-X , and Turing-NLG . It has also been successfully applied to a variety of different vision tasks , including making 3D images .
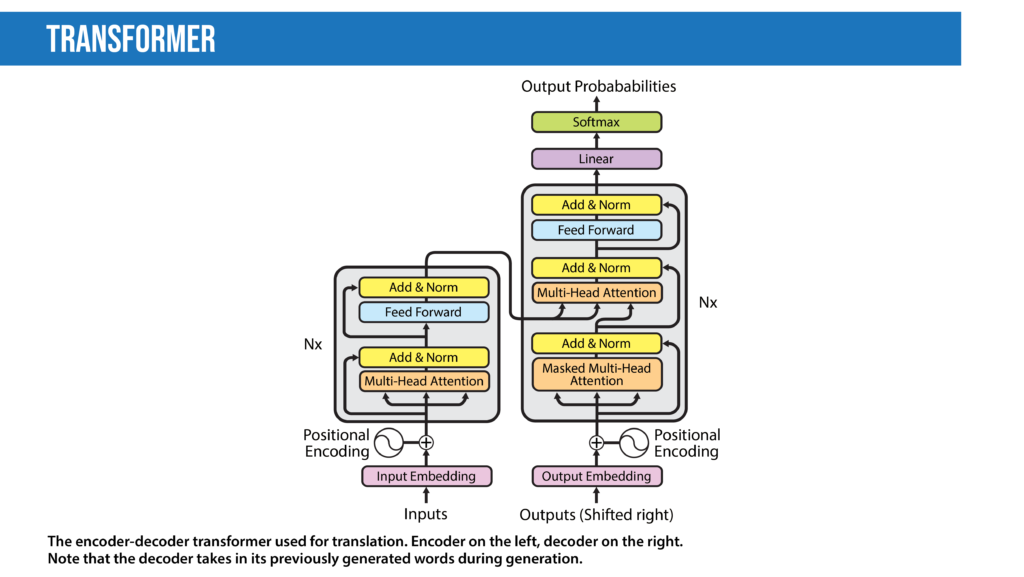
Six Important Natural Language Processing (NLP) Models
Over the years, many NLP models have made waves within the AI community, and some have even made headlines in the mainstream news. The most famous of these have been chatbots and language models. Here are some of them:
- Eliza was developed in the mid-1960s to try to solve the Turing Test; that is, to fool people into thinking they’re conversing with another human being rather than a machine. Eliza used pattern matching and a series of rules without encoding the context of the language.
- Tay was a chatbot that Microsoft launched in 2016. It was supposed to tweet like a teen and learn from conversations with real users on Twitter. The bot adopted phrases from users who tweeted sexist and racist comments, and Microsoft deactivated it not long afterward. Tay illustrates some points made by the “Stochastic Parrots” paper, particularly the danger of not debiasing data.
- BERT and his Muppet friends: Many deep learning models for NLP are named after Muppet characters , including ELMo , BERT , Big BIRD , ERNIE , Kermit , Grover , RoBERTa , and Rosita . Most of these models are good at providing contextual embeddings and enhanced knowledge representation.
- Generative Pre-Trained Transformer 3 (GPT-3) is a 175 billion parameter model that can write original prose with human-equivalent fluency in response to an input prompt. The model is based on the transformer architecture. The previous version, GPT-2, is open source. Microsoft acquired an exclusive license to access GPT-3’s underlying model from its developer OpenAI, but other users can interact with it via an application programming interface (API). Several groups including EleutherAI and Meta have released open source interpretations of GPT-3.
- Language Model for Dialogue Applications (LaMDA) is a conversational chatbot developed by Google. LaMDA is a transformer-based model trained on dialogue rather than the usual web text. The system aims to provide sensible and specific responses to conversations. Google developer Blake Lemoine came to believe that LaMDA is sentient. Lemoine had detailed conversations with AI about his rights and personhood. During one of these conversations, the AI changed Lemoine’s mind about Isaac Asimov’s third law of robotics. Lemoine claimed that LaMDA was sentient, but the idea was disputed by many observers and commentators. Subsequently, Google placed Lemoine on administrative leave for distributing proprietary information and ultimately fired him.
- Mixture of Experts ( MoE): While most deep learning models use the same set of parameters to process every input, MoE models aim to provide different parameters for different inputs based on efficient routing algorithms to achieve higher performance . Switch Transformer is an example of the MoE approach that aims to reduce communication and computational costs.
Programming Languages, Libraries, And Frameworks For Natural Language Processing (NLP)
Many languages and libraries support NLP. Here are a few of the most useful.
- Natural Language Toolkit (NLTK) is one of the first NLP libraries written in Python. It provides easy-to-use interfaces to corpora and lexical resources such as WordNet . It also provides a suite of text-processing libraries for classification, tagging, stemming, parsing, and semantic reasoning.
- spaCy is one of the most versatile open source NLP libraries. It supports more than 66 languages. spaCy also provides pre-trained word vectors and implements many popular models like BERT. spaCy can be used for building production-ready systems for named entity recognition, part-of-speech tagging, dependency parsing, sentence segmentation, text classification, lemmatization, morphological analysis, entity linking, and so on.
- Deep Learning libraries: Popular deep learning libraries include TensorFlow and PyTorch , which make it easier to create models with features like automatic differentiation. These libraries are the most common tools for developing NLP models.
- Hugging Face offers open-source implementations and weights of over 135 state-of-the-art models. The repository enables easy customization and training of the models.
- Gensim provides vector space modeling and topic modeling algorithms.
- R : Many early NLP models were written in R, and R is still widely used by data scientists and statisticians. Libraries in R for NLP include TidyText , Weka , Word2Vec , SpaCyR , TensorFlow , and PyTorch .
- Many other languages including JavaScript, Java, and Julia have libraries that implement NLP methods.
Controversies Surrounding Natural Language Processing (NLP)
NLP has been at the center of a number of controversies. Some are centered directly on the models and their outputs, others on second-order concerns, such as who has access to these systems, and how training them impacts the natural world.
- Stochastic parrots: A 2021 paper titled “On the Dangers of Stochastic Parrots: Can Language Models Be Too Big?” by Emily Bender, Timnit Gebru, Angelina McMillan-Major, and Margaret Mitchell examines how language models may repeat and amplify biases found in their training data. The authors point out that huge, uncurated datasets scraped from the web are bound to include social biases and other undesirable information, and models that are trained on them will absorb these flaws. They advocate greater care in curating and documenting datasets, evaluating a model’s potential impact prior to development, and encouraging research in directions other than designing ever-larger architectures to ingest ever-larger datasets.
- Coherence versus sentience: Recently, a Google engineer tasked with evaluating the LaMDA language model was so impressed by the quality of its chat output that he believed it to be sentient . The fallacy of attributing human-like intelligence to AI dates back to some of the earliest NLP experiments.
- Environmental impact: Large language models require a lot of energy during both training and inference. One study estimated that training a single large language model can emit five times as much carbon dioxide as a single automobile over its operational lifespan. Another study found that models consume even more energy during inference than training. As for solutions, researchers have proposed using cloud servers located in countries with lots of renewable energy as one way to offset this impact.
- High cost leaves out non-corporate researchers: The computational requirements needed to train or deploy large language models are too expensive for many small companies . Some experts worry that this could block many capable engineers from contributing to innovation in AI.
- Black box: When a deep learning model renders an output, it’s difficult or impossible to know why it generated that particular result. While traditional models like logistic regression enable engineers to examine the impact on the output of individual features, neural network methods in natural language processing are essentially black boxes. Such systems are said to be “not explainable,” since we can’t explain how they arrived at their output. An effective approach to achieve explainability is especially important in areas like banking, where regulators want to confirm that a natural language processing system doesn’t discriminate against some groups of people, and law enforcement, where models trained on historical data may perpetuate historical biases against certain groups.
“ Nonsense on stilts ”: Writer Gary Marcus has criticized deep learning-based NLP for generating sophisticated language that misleads users to believe that natural language algorithms understand what they are saying and mistakenly assume they are capable of more sophisticated reasoning than is currently possible.
How To Get Started In Natural Language Processing (NLP)
If you are just starting out, many excellent courses can help.
If you want to learn more about NLP, try reading research papers. Work through the papers that introduced the models and techniques described in this article. Most are easy to find on arxiv.org . You might also take a look at these resources:
- The Batch : A weekly newsletter that tells you what matters in AI. It’s the best way to keep up with developments in deep learning.
- NLP News : A newsletter from Sebastian Ruder, a research scientist at Google, focused on what’s new in NLP.
- Papers with Code : A web repository of machine learning research, tasks, benchmarks, and datasets.
We highly recommend learning to implement basic algorithms (linear and logistic regression, Naive Bayes, decision trees, and vanilla neural networks) in Python. The next step is to take an open-source implementation and adapt it to a new dataset or task.
NLP is one of the fast-growing research domains in AI, with applications that involve tasks including translation, summarization, text generation, and sentiment analysis. Businesses use NLP to power a growing number of applications, both internal — like detecting insurance fraud , determining customer sentiment, and optimizing aircraft maintenance — and customer-facing, like Google Translate.
Aspiring NLP practitioners can begin by familiarizing themselves with foundational AI skills: performing basic mathematics, coding in Python, and using algorithms like decision trees, Naive Bayes, and logistic regression. Online courses can help you build your foundation. They can also help as you proceed into specialized topics. Specializing in NLP requires a working knowledge of things like neural networks, frameworks like PyTorch and TensorFlow, and various data preprocessing techniques. The transformer architecture, which has revolutionized the field since it was introduced in 2017, is an especially important architecture.
NLP is an exciting and rewarding discipline, and has potential to profoundly impact the world in many positive ways. Unfortunately, NLP is also the focus of several controversies, and understanding them is also part of being a responsible practitioner. For instance, researchers have found that models will parrot biased language found in their training data, whether they’re counterfactual, racist, or hateful. Moreover, sophisticated language models can be used to generate disinformation. A broader concern is that training large models produces substantial greenhouse gas emissions.
This page is only a brief overview of what NLP is all about. If you have an appetite for more, DeepLearning.AI offers courses for everyone in their NLP journey, from AI beginners and those who are ready to specialize . No matter your current level of expertise or aspirations, remember to keep learning!
Computer Science with Speech and Language Processing MSc The University of Sheffield
Key course facts.
- View programme website
- Admission advice for international students
Course Description
Learn skills that will change lives from experts in the fields of speech technology and natural language processing.
Course description Speech and language technology graduates are in demand in areas like machine translation, document indexing and retrieval, and speech recognition. Our world-leading speech and language research staff will help you to develop the skills you need.
Accreditation Accredited by the British Computer Society.
Please see our University website for the most up-to-date course information.
Entry Requirements / Admissions
Ucas international information, tuition fees computer science with speech and language processing msc, average student cost of living in the uk.
London costs approx 34% more than average, mainly due to rent being 67% higher than average of other cities. For students staying in student halls, costs of water, gas, electricity, wifi are generally included in the rental. Students in smaller cities where accommodation is in walking/biking distance transport costs tend to be significantly smaller.
University Rankings
Positions of the university of sheffield in top uk and global rankings., about the university of sheffield.
The University of Sheffield is a government funded research university based in Sheffield, England. The university’s vision is to change the world for the better through the power and application of ideas and knowledge. This university does not have campuses, but instead approximately 430 buildings that are located quite close to each other in Sheffield’s metropolitan city centre.
List of 363 Bachelor and Master Courses from The University of Sheffield - Course Catalogue
Student composition of The University of Sheffield
Where is this programme taught.

Similar courses
Ask a question to the university of sheffield, request course information or send a question directly to the university of sheffield.
Universities
The University of Sheffield
MSc in Computer Science With Speech and Language Processing
The University of Sheffield, Sheffield
Faculty of Engineering
Help Me Decide
Pre-requisites
- Discussions
About Course
Program Duration
Computer Science
Degree Type
Course Credits
More than any other field, computer technology has shaped the modern world. Things considered common these days, like the Internet, Smart Phones, Cyber security systems, and more, wouldn’t have been possible without computing. A Master’s in Computer Science or Masters in CS from The University of Sheffield can help develop an individual's skills and career prospects.
The masters in computer science course at the The University of Sheffield is usually offered for 24 months. Through MS in computer science course at The University of Sheffield , the students will receive the knowledge and experience that will help them demonstrate domain expertise and the ability to either continue educational training at the doctoral level or immediately work in the key economic sectors, such as government, business, industry, health care, and education. MS in CS at The University of Sheffield also brings several career opportunities after course completion.
The Master of Science in Computer Science Program at TUOS is distinguished by its forward-thinking curriculum, which includes credits, courses, and a research thesis requirement. It also involves physical resources for comprehensive learning like labs/equipment and the diversity of the program faculty.
Subjects under MS in Computer Science
The syllabus for the MS in Computer Science course will vary as per the specialisation, and university/college chosen by an international student. However, certain MS in Computer Science subjects are common for most courses. Apart from the final year projects and internship, the common MS in Computer Science subjects at The University of Sheffield are as follows:
- Basic Programming Laboratory
- Programming Languages
- Theory of Computation
- Design and Analysis of Algorithms
- Mathematical Logic
- Discrete Mathematics
- Distributed Systems
- Computer Systems Verification
- Complexity Theory
- Operations Research
- Data Mining and Machine Learning
- Cryptography and Computer Security
- Probability and Statistics
These MSCS Subjects list may vary based on the specialisations in each university or one might be given the option to pick and choose.
Scope of MS in Computer Science
After pursuing an MS in CS at The University of Sheffield , a student can explore the given benefits and more:
- Better career opportunities
- Knowledge to elevate your tech stature
- Possibility of tuition fee reimbursement
- One step closer to doctorate
- Avenues in the teaching field
Official fee page
£31,190 / year
£31,190 / 12 months
5000+ Students
Availed education loan
Loan amount sanctioned
Assistance for loan process
Minimum english score required
- Online Application
- Academic Transcripts
- One or Two Academic References
- Supporting Statement
- Curriculum Vitae (CV)
- English Language Proficiency
Find all the GRE Waived-off courses by applying a quick filter
Apply GRE filter in this university
Find GRE-waivers across all universities
Yocketers applied
Yocketers admitted
Yocketers interested
Yocketer profiles
Spring 2022
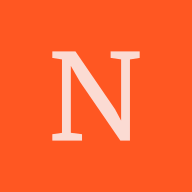
Nithish Reddy Polepelly
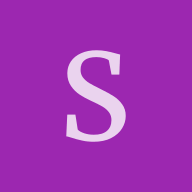
Sakshi Agarwal
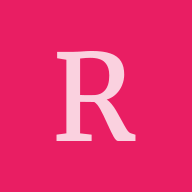
Raushan Kr. Bharti
Yocket’s Counsel
Meet our counsellors.
We got a team of 50+ experienced counsellors ready to help you!
Related Discussion for the Universities
Ask, post and discuss!
Have a question? Ask and discuss with your fellow aspirants!
2 years ago
Help | Advanced Search
Computer Science > Computation and Language
Important: e-prints posted on arXiv are not peer-reviewed by arXiv; they should not be relied upon without context to guide clinical practice or health-related behavior and should not be reported in news media as established information without consulting multiple experts in the field.
Title: SpeakGer: A meta-data enriched speech corpus of German state and federal parliaments
Abstract: The application of natural language processing on political texts as well as speeches has become increasingly relevant in political sciences due to the ability to analyze large text corpora which cannot be read by a single person. But such text corpora often lack critical meta information, detailing for instance the party, age or constituency of the speaker, that can be used to provide an analysis tailored to more fine-grained research questions. To enable researchers to answer such questions with quantitative approaches such as natural language processing, we provide the SpeakGer data set, consisting of German parliament debates from all 16 federal states of Germany as well as the German Bundestag from 1947-2023, split into a total of 10,806,105 speeches. This data set includes rich meta data in form of information on both reactions from the audience towards the speech as well as information about the speaker's party, their age, their constituency and their party's political alignment, which enables a deeper analysis. We further provide three exploratory analyses, detailing topic shares of different parties throughout time, a descriptive analysis of the development of the age of an average speaker as well as a sentiment analysis of speeches of different parties with regards to the COVID-19 pandemic.
Submission history
Access paper:.
- HTML (experimental)
- Other Formats

References & Citations
- Google Scholar
- Semantic Scholar
BibTeX formatted citation

Bibliographic and Citation Tools
Code, data and media associated with this article, recommenders and search tools.
- Institution
arXivLabs: experimental projects with community collaborators
arXivLabs is a framework that allows collaborators to develop and share new arXiv features directly on our website.
Both individuals and organizations that work with arXivLabs have embraced and accepted our values of openness, community, excellence, and user data privacy. arXiv is committed to these values and only works with partners that adhere to them.
Have an idea for a project that will add value for arXiv's community? Learn more about arXivLabs .

UCL Psychology and Language Sciences
MSc Language Sciences: Neuroscience of Language and Speech

< Return to MSc Language Sciences (all routes) The Neuroscience of Language and Speech route explores the neural mechanisms involved in speech production, speech perception, and language processing. Throughout your studies, you'll learn about a broad range of topics, including neurophysiological approaches to the study of speech (fMRI, EEG, and TMS) and computational modelling techniques. You'll also have the opportunity to gain hands-on experience of collecting and analysing neurological data related to language and speech.
On the MSc Language Sciences programme ( Neuroscience of Language and Speech route) you will complete 180 UCL credits from a range of compulsory, optional and elective modules. This selection of modules has been chosen to provide you with a flexible degree that you can tailor to your specific interests whilst meeting the learning outcomes of the specialised Neuroscience of Language and Speech route.
Compulsory modules are taken by all MSc LangSci students, whatever their strand of specialisation. You will undertake three core modules, Topics and Methods in Language Sciences (PALS0054), Fundamental Statistics: Statistical and Causal Reasoning (PSYC0223) and the Research Project (PALS0025). The Research Project is mostly carried out in the Summer period after other assessments have been completed.
PLEASE NOTE: All students will be automatically registered for PSYC0223 (Fundamental Statistics: Statistical and Causal Reasoning). Applicants who have already reached an intermediate or advanced level of statistics prior to entry to the programme should contact [email protected] to discuss the selection of an alternative Term 1 module at a more advanced level ( PALS0049 Intermediate statistics: Data analysis visualisation with R or PSYC0146 Advanced statistics: Data analysis and modelling with R). These changes will only be allowed when students demonstrate to have the requisite knowledge, and with consultation and approval of the Programme Director. In addition to the compulsory modules listed above, you will register for three optional modules (45 credits) from the set of 15 credit modules below.
Finally, you will choose three further elective modules (45 credits) from all of those offered within the Division of Psychology and Language Sciences, subject to availability and normal pre-requisites. Some possible modules are listed as examples below, but this list is not exhaustive.
The full list of modules offered in the Division of Psychology and Language Sciences can be found in the UCL Module Catalogue.
Application
You are advised to apply as early as possible due to competition for places. Those applying for scholarship funding (particularly overseas applicants) should take note of application deadlines.
Who can apply?
This programme is designed for students with a background in a related discipline who wish to deepen their knowledge of language sciences. The degree is ideal preparation for those interested in applying for a research degree in language development/speech sciences/neuroscience (delete as applicable), linguistics or a related discipline and will also appeal to individuals currently working in areas such as education, speech and language therapy, audiology and speech technology.
Successful applicants will normally hold, or be progressing towards, a minimum of an upper second-class Bachelor's degree from a UK university or an overseas qualification of an equivalent standard in a language-related area such as Linguistics, Speech Sciences, English Language, Psychology or Cognitive Science. We also warmly welcome applications from those with degrees in a cognate discipline such as Computational Science. Your application will be evaluated on the basis of the quality of your degree and degree institution; the quality of your references; your relevant skills and experience; the quality of your personal statement and the suitability of your career plans.
What to include in your personal statement:
Together with essential academic requirements, the personal statement is your opportunity to illustrate whether your reasons for applying to this programme match what the programme will deliver.
When we assess your personal statement, we would like to learn:
• why you want to study Language Sciences at graduate level, • why you want to study Language Sciences at UCL, • why you have applied to your chosen strand (Language Development, Neuroscience Language & Communication, Speech Sciences, Sign Language & Deaf Studies), • which module(s) on the strand interest you most and why, • if there is a particular research area, research question, or research project you would like to work on; if there is a specific staff member you would like to work with, we encourage you to include this, • how your academic and professional background have prepared you for the programme, • how studying for the MSc will enable you to meet your short- and long-term career goals.
Virtual Q&A Event - TBC
Please join us for our next virtual Q&A event via Zoom. Please register your interest here: MSc Language Sciences Q&A Event and enter a question for the programme team if you wish.
This event is a fantastic opportunity to ask the programme team any questions you have about about the Language Sciences MSc programme or life at UCL.
This event will be delivered via Zoom.
Application deadlines
Applicants who require a visa: 14 Oct 2024 – 04 Apr 2025 Applications close at 5pm UK time
Applicants who do not require a visa: 14 Oct 2024 – 29 Aug 2025 Applications close at 5pm UK time
For more information see our Applications page.
Teaching Administrator
- [email protected]
- +44 (0)20 7679 4274
- Twitter @ucl_langsci
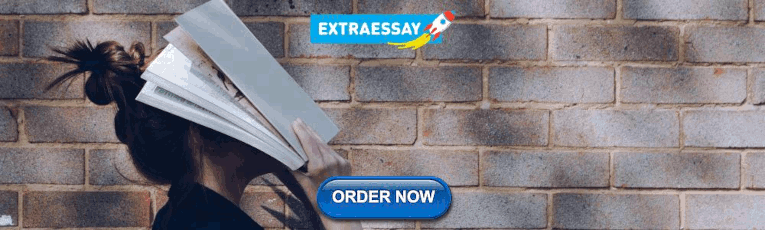
COMMENTS
Computer Science With Speech and Language Processing. School of Computer Science, Faculty of Engineering. Learn skills that will change lives from experts in the fields of speech technology and natural language processing. ... This module aims to demonstrate why computer speech processing is an important and difficult problem, to investigate ...
Computer Speech & Language is an official publication of the International Speech Communication Association (ISCA) that covers various aspects of speech and language processing and technology. The journal publishes original research, reviews, tutorials, and special issues on topics such as speech recognition, synthesis, understanding, generation, emotion, and more.
About. The Speech and Natural Language Processing groups do fundamental work in language understanding and generation with applications to a wide variety of topics, including summarization, argumentation, persuasion, sentiment, detecting deceptive, emotional and charismatic speech, text-to-speech synthesis, analysis of social media to detect mental illness, abusive language, and radicalization.
Learn about the research and applications of speech and language processing at MIT EECS. Explore the faculty, news, and events in this interdisciplinary area of computer science and engineering.
Learn from world leaders in linguistics and informatics how to apply speech and language technology to real-world problems. This programme covers phonetics, speech synthesis, speech recognition, natural language processing, machine translation and more.
Chair of Spoken Language Processing in the 'Speech and Hearing' research group, Dept. Computer Science, University of Sheffield. Editor-in-Chief of 'Computer Speech & Language'. Editorial Board Member for 'Speech Communication', 'Languages' and the 'International Journal of Cognitive Informatics and Natural Intelligence'.
Embark on a transformative journey with the MSc Computer Science with Speech and Language Processing programme at the University of Sheffield. Delve into the cutting-edge realms of speech technology and natural language processing under the guidance of world-leading experts.
The Centre for Speech & Language Technology brings together expertise from researchers in the Department of Computer Science and Silicon Valley based company, VoiceBase, the leading provider of AI-powered speech analytics, to pioneer the future of speech recognition and speech analytics
Speech and language technology is being transformed by exciting developments in artificial intelligence, including deep learning and generative models such as ChatGPT. Our world-leading speech and language research staff will help you to understand these technologies and develop the skills needed to apply them to real-world problems.
All studies; Language Studies; Europe; United Kingdom; England; University of Sheffield; Computer Science With Speech and Language Processing ; About. In the Computer Science With Speech and Language Processing programme from University of Sheffield, you can learn skills that will change lives from experts in the fields of speech technology and natural language processing.
Natural Language Processing (15 Credits) - Core This module provides an introduction to the field of computer processing of written natural language, known as Natural Language Processing (NLP). We will cover standard theories, models and algorithms, discuss competing solutions to problems, describe example systems and applications, and ...
A personal perspective on the evolution of NLP and CL research by a pioneer in the field. The article covers topics such as machine translation, reasoning, and language understanding, and discusses the relationships among disciplines related to language.
CLSP is a center for interdisciplinary research and education in natural language processing, speech, and related fields. Explore the latest news, events, and projects from CLSP faculty and students.
This paper introduces Moonshine, a family of speech recognition models optimized for live transcription and voice command processing. Moonshine is based on an encoder-decoder transformer architecture and employs Rotary Position Embedding (RoPE) instead of traditional absolute position embeddings. The model is trained on speech segments of various lengths, but without using zero-padding ...
Learn more about Computer Science with Speech and Language Processing (Taught) program including the program fees, scholarships, scores and further course information ... Computer Science with Speech and Language Processing (Taught) Western Bank, Sheffield, GB, Sheffield, United Kingdom. Request More Details Shortlist Compare
Jason Eisner, professor of Computer Science, is affiliated with the Center for Language and Speech Processing, the Cognitive Science Department, the Machine Learning Group and the National Center of Excellence in Human Language Technology. Eisner has authored over 100 papers (as well as software tools) in several computational linguistics areas.
Learn what natural language processing (NLP) is, why it matters, and what it is used for. Explore 11 NLP tasks, such as sentiment analysis, machine translation, and text generation, with examples and applications.
This course focuses on the transformative impact of artificial intelligence, particularly deep learning and generative models like ChatGPT, on speech and language technology. Led by world-leading researchers in the field, the program equips students with the knowledge and practical skills necessary to harness these advanced technologies for addressing real-world challenges in speech and ...
Learn skills that will change lives from experts in the fields of speech technology and natural language processing. Course description Speech and language technology graduates are in demand in areas like machine translation, document indexing and retrieval, and speech recognition. Our world-leading speech and language research staff will help you to develop the skills you need.
The masters in computer science course at the The University of Sheffield is usually offered for 24 months. Through MS in computer science course at The University of Sheffield, the students will receive the knowledge and experience that will help them demonstrate domain expertise and the ability to either continue educational training at the doctoral level or immediately work in the key ...
The MSc in Computer Science with Speech and Language Processing consists of 120 credits of taught modules and a 60 credit dissertation. The full-time programme is offered over 12 months starting in late September each year, and finishing the following September. The core modules consist of 120 credits (15 credits each):
Lagunita was a free and open online learning platform that Stanford used to offer more than 200 courses. It was retired on March 31, 2020 and most courses were moved to edx.org.
The application of natural language processing on political texts as well as speeches has become increasingly relevant in political sciences due to the ability to analyze large text corpora which cannot be read by a single person. But such text corpora often lack critical meta information, detailing for instance the party, age or constituency of the speaker, that can be used to provide an ...
The Neuroscience of Language and Speech route explores the neural mechanisms involved in speech production, speech perception, and language processing. Throughout your studies, you'll learn about a broad range of topics, including neurophysiological approaches to the study of speech (fMRI, EEG, and TMS) and computational modelling techniques.