- Privacy Policy
Buy Me a Coffee

Home » Research Objectives – Types, Examples and Writing Guide
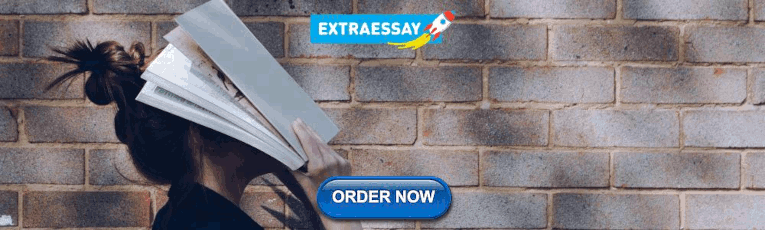
Research Objectives – Types, Examples and Writing Guide
Table of Contents

Research Objectives
Research objectives refer to the specific goals or aims of a research study. They provide a clear and concise description of what the researcher hopes to achieve by conducting the research . The objectives are typically based on the research questions and hypotheses formulated at the beginning of the study and are used to guide the research process.
Types of Research Objectives
Here are the different types of research objectives in research:
- Exploratory Objectives: These objectives are used to explore a topic, issue, or phenomenon that has not been studied in-depth before. The aim of exploratory research is to gain a better understanding of the subject matter and generate new ideas and hypotheses .
- Descriptive Objectives: These objectives aim to describe the characteristics, features, or attributes of a particular population, group, or phenomenon. Descriptive research answers the “what” questions and provides a snapshot of the subject matter.
- Explanatory Objectives : These objectives aim to explain the relationships between variables or factors. Explanatory research seeks to identify the cause-and-effect relationships between different phenomena.
- Predictive Objectives: These objectives aim to predict future events or outcomes based on existing data or trends. Predictive research uses statistical models to forecast future trends or outcomes.
- Evaluative Objectives : These objectives aim to evaluate the effectiveness or impact of a program, intervention, or policy. Evaluative research seeks to assess the outcomes or results of a particular intervention or program.
- Prescriptive Objectives: These objectives aim to provide recommendations or solutions to a particular problem or issue. Prescriptive research identifies the best course of action based on the results of the study.
- Diagnostic Objectives : These objectives aim to identify the causes or factors contributing to a particular problem or issue. Diagnostic research seeks to uncover the underlying reasons for a particular phenomenon.
- Comparative Objectives: These objectives aim to compare two or more groups, populations, or phenomena to identify similarities and differences. Comparative research is used to determine which group or approach is more effective or has better outcomes.
- Historical Objectives: These objectives aim to examine past events, trends, or phenomena to gain a better understanding of their significance and impact. Historical research uses archival data, documents, and records to study past events.
- Ethnographic Objectives : These objectives aim to understand the culture, beliefs, and practices of a particular group or community. Ethnographic research involves immersive fieldwork and observation to gain an insider’s perspective of the group being studied.
- Action-oriented Objectives: These objectives aim to bring about social or organizational change. Action-oriented research seeks to identify practical solutions to social problems and to promote positive change in society.
- Conceptual Objectives: These objectives aim to develop new theories, models, or frameworks to explain a particular phenomenon or set of phenomena. Conceptual research seeks to provide a deeper understanding of the subject matter by developing new theoretical perspectives.
- Methodological Objectives: These objectives aim to develop and improve research methods and techniques. Methodological research seeks to advance the field of research by improving the validity, reliability, and accuracy of research methods and tools.
- Theoretical Objectives : These objectives aim to test and refine existing theories or to develop new theoretical perspectives. Theoretical research seeks to advance the field of knowledge by testing and refining existing theories or by developing new theoretical frameworks.
- Measurement Objectives : These objectives aim to develop and validate measurement instruments, such as surveys, questionnaires, and tests. Measurement research seeks to improve the quality and reliability of data collection and analysis by developing and testing new measurement tools.
- Design Objectives : These objectives aim to develop and refine research designs, such as experimental, quasi-experimental, and observational designs. Design research seeks to improve the quality and validity of research by developing and testing new research designs.
- Sampling Objectives: These objectives aim to develop and refine sampling techniques, such as probability and non-probability sampling methods. Sampling research seeks to improve the representativeness and generalizability of research findings by developing and testing new sampling techniques.
How to Write Research Objectives
Writing clear and concise research objectives is an important part of any research project, as it helps to guide the study and ensure that it is focused and relevant. Here are some steps to follow when writing research objectives:
- Identify the research problem : Before you can write research objectives, you need to identify the research problem you are trying to address. This should be a clear and specific problem that can be addressed through research.
- Define the research questions : Based on the research problem, define the research questions you want to answer. These questions should be specific and should guide the research process.
- Identify the variables : Identify the key variables that you will be studying in your research. These are the factors that you will be measuring, manipulating, or analyzing to answer your research questions.
- Write specific objectives: Write specific, measurable objectives that will help you answer your research questions. These objectives should be clear and concise and should indicate what you hope to achieve through your research.
- Use the SMART criteria: To ensure that your research objectives are well-defined and achievable, use the SMART criteria. This means that your objectives should be Specific, Measurable, Achievable, Relevant, and Time-bound.
- Revise and refine: Once you have written your research objectives, revise and refine them to ensure that they are clear, concise, and achievable. Make sure that they align with your research questions and variables, and that they will help you answer your research problem.
Example of Research Objectives
Examples of research objectives Could be:
Research Objectives for the topic of “The Impact of Artificial Intelligence on Employment”:
- To investigate the effects of the adoption of AI on employment trends across various industries and occupations.
- To explore the potential for AI to create new job opportunities and transform existing roles in the workforce.
- To examine the social and economic implications of the widespread use of AI for employment, including issues such as income inequality and access to education and training.
- To identify the skills and competencies that will be required for individuals to thrive in an AI-driven workplace, and to explore the role of education and training in developing these skills.
- To evaluate the ethical and legal considerations surrounding the use of AI for employment, including issues such as bias, privacy, and the responsibility of employers and policymakers to protect workers’ rights.
When to Write Research Objectives
- At the beginning of a research project : Research objectives should be identified and written down before starting a research project. This helps to ensure that the project is focused and that data collection and analysis efforts are aligned with the intended purpose of the research.
- When refining research questions: Writing research objectives can help to clarify and refine research questions. Objectives provide a more concrete and specific framework for addressing research questions, which can improve the overall quality and direction of a research project.
- After conducting a literature review : Conducting a literature review can help to identify gaps in knowledge and areas that require further research. Writing research objectives can help to define and focus the research effort in these areas.
- When developing a research proposal: Research objectives are an important component of a research proposal. They help to articulate the purpose and scope of the research, and provide a clear and concise summary of the expected outcomes and contributions of the research.
- When seeking funding for research: Funding agencies often require a detailed description of research objectives as part of a funding proposal. Writing clear and specific research objectives can help to demonstrate the significance and potential impact of a research project, and increase the chances of securing funding.
- When designing a research study : Research objectives guide the design and implementation of a research study. They help to identify the appropriate research methods, sampling strategies, data collection and analysis techniques, and other relevant aspects of the study design.
- When communicating research findings: Research objectives provide a clear and concise summary of the main research questions and outcomes. They are often included in research reports and publications, and can help to ensure that the research findings are communicated effectively and accurately to a wide range of audiences.
- When evaluating research outcomes : Research objectives provide a basis for evaluating the success of a research project. They help to measure the degree to which research questions have been answered and the extent to which research outcomes have been achieved.
- When conducting research in a team : Writing research objectives can facilitate communication and collaboration within a research team. Objectives provide a shared understanding of the research purpose and goals, and can help to ensure that team members are working towards a common objective.
Purpose of Research Objectives
Some of the main purposes of research objectives include:
- To clarify the research question or problem : Research objectives help to define the specific aspects of the research question or problem that the study aims to address. This makes it easier to design a study that is focused and relevant.
- To guide the research design: Research objectives help to determine the research design, including the research methods, data collection techniques, and sampling strategy. This ensures that the study is structured and efficient.
- To measure progress : Research objectives provide a way to measure progress throughout the research process. They help the researcher to evaluate whether they are on track and meeting their goals.
- To communicate the research goals : Research objectives provide a clear and concise description of the research goals. This helps to communicate the purpose of the study to other researchers, stakeholders, and the general public.
Advantages of Research Objectives
Here are some advantages of having well-defined research objectives:
- Focus : Research objectives help to focus the research effort on specific areas of inquiry. By identifying clear research questions, the researcher can narrow down the scope of the study and avoid getting sidetracked by irrelevant information.
- Clarity : Clearly stated research objectives provide a roadmap for the research study. They provide a clear direction for the research, making it easier for the researcher to stay on track and achieve their goals.
- Measurability : Well-defined research objectives provide measurable outcomes that can be used to evaluate the success of the research project. This helps to ensure that the research is effective and that the research goals are achieved.
- Feasibility : Research objectives help to ensure that the research project is feasible. By clearly defining the research goals, the researcher can identify the resources required to achieve those goals and determine whether those resources are available.
- Relevance : Research objectives help to ensure that the research study is relevant and meaningful. By identifying specific research questions, the researcher can ensure that the study addresses important issues and contributes to the existing body of knowledge.
About the author
Muhammad Hassan
Researcher, Academic Writer, Web developer
You may also like

How to Cite Research Paper – All Formats and...

Data Collection – Methods Types and Examples

Delimitations in Research – Types, Examples and...

Research Paper Format – Types, Examples and...

Research Process – Steps, Examples and Tips

Research Design – Types, Methods and Examples

Research Aims, Objectives & Questions
The “Golden Thread” Explained Simply (+ Examples)
By: David Phair (PhD) and Alexandra Shaeffer (PhD) | June 2022
The research aims , objectives and research questions (collectively called the “golden thread”) are arguably the most important thing you need to get right when you’re crafting a research proposal , dissertation or thesis . We receive questions almost every day about this “holy trinity” of research and there’s certainly a lot of confusion out there, so we’ve crafted this post to help you navigate your way through the fog.
Overview: The Golden Thread
- What is the golden thread
- What are research aims ( examples )
- What are research objectives ( examples )
- What are research questions ( examples )
- The importance of alignment in the golden thread
What is the “golden thread”?
The golden thread simply refers to the collective research aims , research objectives , and research questions for any given project (i.e., a dissertation, thesis, or research paper ). These three elements are bundled together because it’s extremely important that they align with each other, and that the entire research project aligns with them.
Importantly, the golden thread needs to weave its way through the entirety of any research project , from start to end. In other words, it needs to be very clearly defined right at the beginning of the project (the topic ideation and proposal stage) and it needs to inform almost every decision throughout the rest of the project. For example, your research design and methodology will be heavily influenced by the golden thread (we’ll explain this in more detail later), as well as your literature review.
The research aims, objectives and research questions (the golden thread) define the focus and scope ( the delimitations ) of your research project. In other words, they help ringfence your dissertation or thesis to a relatively narrow domain, so that you can “go deep” and really dig into a specific problem or opportunity. They also help keep you on track , as they act as a litmus test for relevance. In other words, if you’re ever unsure whether to include something in your document, simply ask yourself the question, “does this contribute toward my research aims, objectives or questions?”. If it doesn’t, chances are you can drop it.
Alright, enough of the fluffy, conceptual stuff. Let’s get down to business and look at what exactly the research aims, objectives and questions are and outline a few examples to bring these concepts to life.
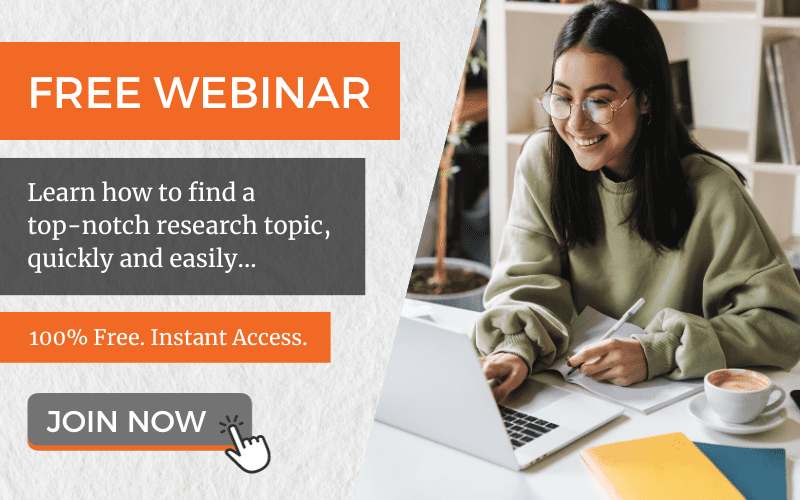
Research Aims: What are they?
Simply put, the research aim(s) is a statement that reflects the broad overarching goal (s) of the research project. Research aims are fairly high-level (low resolution) as they outline the general direction of the research and what it’s trying to achieve .
Research Aims: Examples
True to the name, research aims usually start with the wording “this research aims to…”, “this research seeks to…”, and so on. For example:
“This research aims to explore employee experiences of digital transformation in retail HR.” “This study sets out to assess the interaction between student support and self-care on well-being in engineering graduate students”
As you can see, these research aims provide a high-level description of what the study is about and what it seeks to achieve. They’re not hyper-specific or action-oriented, but they’re clear about what the study’s focus is and what is being investigated.
Need a helping hand?
Research Objectives: What are they?
The research objectives take the research aims and make them more practical and actionable . In other words, the research objectives showcase the steps that the researcher will take to achieve the research aims.
The research objectives need to be far more specific (higher resolution) and actionable than the research aims. In fact, it’s always a good idea to craft your research objectives using the “SMART” criteria. In other words, they should be specific, measurable, achievable, relevant and time-bound”.
Research Objectives: Examples
Let’s look at two examples of research objectives. We’ll stick with the topic and research aims we mentioned previously.
For the digital transformation topic:
To observe the retail HR employees throughout the digital transformation. To assess employee perceptions of digital transformation in retail HR. To identify the barriers and facilitators of digital transformation in retail HR.
And for the student wellness topic:
To determine whether student self-care predicts the well-being score of engineering graduate students. To determine whether student support predicts the well-being score of engineering students. To assess the interaction between student self-care and student support when predicting well-being in engineering graduate students.
As you can see, these research objectives clearly align with the previously mentioned research aims and effectively translate the low-resolution aims into (comparatively) higher-resolution objectives and action points . They give the research project a clear focus and present something that resembles a research-based “to-do” list.
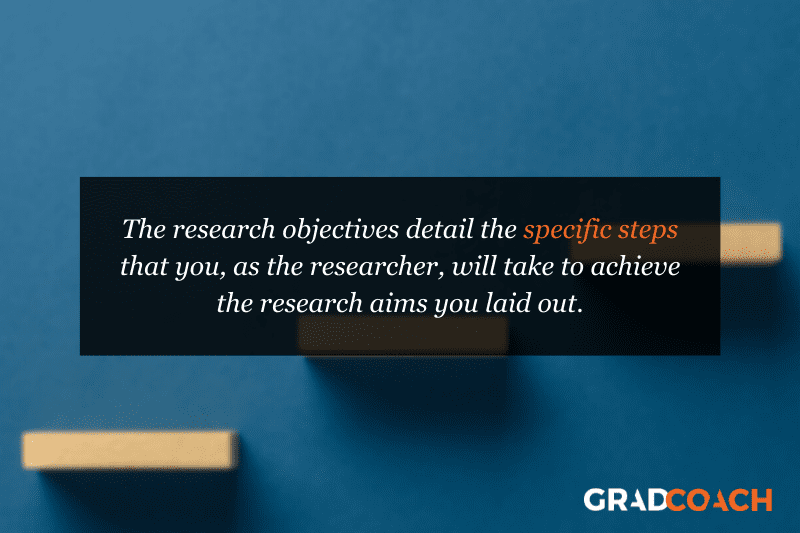
Research Questions: What are they?
Finally, we arrive at the all-important research questions. The research questions are, as the name suggests, the key questions that your study will seek to answer . Simply put, they are the core purpose of your dissertation, thesis, or research project. You’ll present them at the beginning of your document (either in the introduction chapter or literature review chapter) and you’ll answer them at the end of your document (typically in the discussion and conclusion chapters).
The research questions will be the driving force throughout the research process. For example, in the literature review chapter, you’ll assess the relevance of any given resource based on whether it helps you move towards answering your research questions. Similarly, your methodology and research design will be heavily influenced by the nature of your research questions. For instance, research questions that are exploratory in nature will usually make use of a qualitative approach, whereas questions that relate to measurement or relationship testing will make use of a quantitative approach.
Let’s look at some examples of research questions to make this more tangible.
Research Questions: Examples
Again, we’ll stick with the research aims and research objectives we mentioned previously.
For the digital transformation topic (which would be qualitative in nature):
How do employees perceive digital transformation in retail HR? What are the barriers and facilitators of digital transformation in retail HR?
And for the student wellness topic (which would be quantitative in nature):
Does student self-care predict the well-being scores of engineering graduate students? Does student support predict the well-being scores of engineering students? Do student self-care and student support interact when predicting well-being in engineering graduate students?
You’ll probably notice that there’s quite a formulaic approach to this. In other words, the research questions are basically the research objectives “converted” into question format. While that is true most of the time, it’s not always the case. For example, the first research objective for the digital transformation topic was more or less a step on the path toward the other objectives, and as such, it didn’t warrant its own research question.
So, don’t rush your research questions and sloppily reword your objectives as questions. Carefully think about what exactly you’re trying to achieve (i.e. your research aim) and the objectives you’ve set out, then craft a set of well-aligned research questions . Also, keep in mind that this can be a somewhat iterative process , where you go back and tweak research objectives and aims to ensure tight alignment throughout the golden thread.
The importance of strong alignment
Alignment is the keyword here and we have to stress its importance . Simply put, you need to make sure that there is a very tight alignment between all three pieces of the golden thread. If your research aims and research questions don’t align, for example, your project will be pulling in different directions and will lack focus . This is a common problem students face and can cause many headaches (and tears), so be warned.
Take the time to carefully craft your research aims, objectives and research questions before you run off down the research path. Ideally, get your research supervisor/advisor to review and comment on your golden thread before you invest significant time into your project, and certainly before you start collecting data .
Recap: The golden thread
In this post, we unpacked the golden thread of research, consisting of the research aims , research objectives and research questions . You can jump back to any section using the links below.
As always, feel free to leave a comment below – we always love to hear from you. Also, if you’re interested in 1-on-1 support, take a look at our private coaching service here.
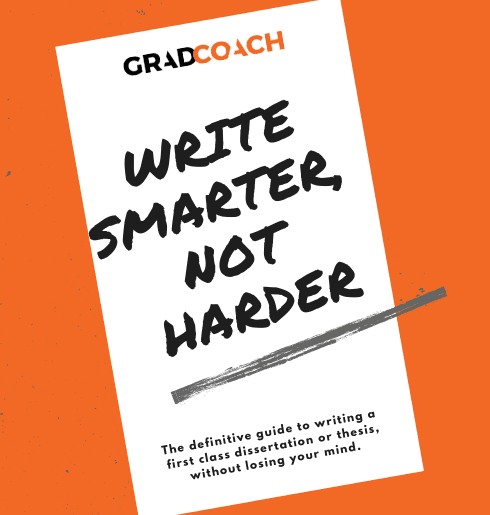
Psst… there’s more (for free)
This post is part of our dissertation mini-course, which covers everything you need to get started with your dissertation, thesis or research project.
You Might Also Like:
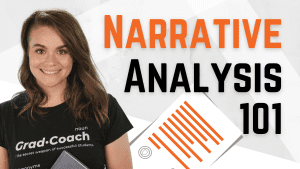
38 Comments
Thank you very much for your great effort put. As an Undergraduate taking Demographic Research & Methodology, I’ve been trying so hard to understand clearly what is a Research Question, Research Aim and the Objectives in a research and the relationship between them etc. But as for now I’m thankful that you’ve solved my problem.
Well appreciated. This has helped me greatly in doing my dissertation.
An so delighted with this wonderful information thank you a lot.
so impressive i have benefited a lot looking forward to learn more on research.
I am very happy to have carefully gone through this well researched article.
Infact,I used to be phobia about anything research, because of my poor understanding of the concepts.
Now,I get to know that my research question is the same as my research objective(s) rephrased in question format.
I please I would need a follow up on the subject,as I intends to join the team of researchers. Thanks once again.
Thanks so much. This was really helpful.
I know you pepole have tried to break things into more understandable and easy format. And God bless you. Keep it up
i found this document so useful towards my study in research methods. thanks so much.
This is my 2nd read topic in your course and I should commend the simplified explanations of each part. I’m beginning to understand and absorb the use of each part of a dissertation/thesis. I’ll keep on reading your free course and might be able to avail the training course! Kudos!
Thank you! Better put that my lecture and helped to easily understand the basics which I feel often get brushed over when beginning dissertation work.
This is quite helpful. I like how the Golden thread has been explained and the needed alignment.
This is quite helpful. I really appreciate!
The article made it simple for researcher students to differentiate between three concepts.
Very innovative and educational in approach to conducting research.
I am very impressed with all these terminology, as I am a fresh student for post graduate, I am highly guided and I promised to continue making consultation when the need arise. Thanks a lot.
A very helpful piece. thanks, I really appreciate it .
Very well explained, and it might be helpful to many people like me.
Wish i had found this (and other) resource(s) at the beginning of my PhD journey… not in my writing up year… 😩 Anyways… just a quick question as i’m having some issues ordering my “golden thread”…. does it matter in what order you mention them? i.e., is it always first aims, then objectives, and finally the questions? or can you first mention the research questions and then the aims and objectives?
Thank you for a very simple explanation that builds upon the concepts in a very logical manner. Just prior to this, I read the research hypothesis article, which was equally very good. This met my primary objective.
My secondary objective was to understand the difference between research questions and research hypothesis, and in which context to use which one. However, I am still not clear on this. Can you kindly please guide?
In research, a research question is a clear and specific inquiry that the researcher wants to answer, while a research hypothesis is a tentative statement or prediction about the relationship between variables or the expected outcome of the study. Research questions are broader and guide the overall study, while hypotheses are specific and testable statements used in quantitative research. Research questions identify the problem, while hypotheses provide a focus for testing in the study.
Exactly what I need in this research journey, I look forward to more of your coaching videos.
This helped a lot. Thanks so much for the effort put into explaining it.
What data source in writing dissertation/Thesis requires?
What is data source covers when writing dessertation/thesis
This is quite useful thanks
I’m excited and thankful. I got so much value which will help me progress in my thesis.
where are the locations of the reserch statement, research objective and research question in a reserach paper? Can you write an ouline that defines their places in the researh paper?
Very helpful and important tips on Aims, Objectives and Questions.
Thank you so much for making research aim, research objectives and research question so clear. This will be helpful to me as i continue with my thesis.
Thanks much for this content. I learned a lot. And I am inspired to learn more. I am still struggling with my preparation for dissertation outline/proposal. But I consistently follow contents and tutorials and the new FB of GRAD Coach. Hope to really become confident in writing my dissertation and successfully defend it.
As a researcher and lecturer, I find splitting research goals into research aims, objectives, and questions is unnecessarily bureaucratic and confusing for students. For most biomedical research projects, including ‘real research’, 1-3 research questions will suffice (numbers may differ by discipline).
Awesome! Very important resources and presented in an informative way to easily understand the golden thread. Indeed, thank you so much.
Well explained
The blog article on research aims, objectives, and questions by Grad Coach is a clear and insightful guide that aligns with my experiences in academic research. The article effectively breaks down the often complex concepts of research aims and objectives, providing a straightforward and accessible explanation. Drawing from my own research endeavors, I appreciate the practical tips offered, such as the need for specificity and clarity when formulating research questions. The article serves as a valuable resource for students and researchers, offering a concise roadmap for crafting well-defined research goals and objectives. Whether you’re a novice or an experienced researcher, this article provides practical insights that contribute to the foundational aspects of a successful research endeavor.
A great thanks for you. it is really amazing explanation. I grasp a lot and one step up to research knowledge.
I really found these tips helpful. Thank you very much Grad Coach.
I found this article helpful. Thanks for sharing this.
Submit a Comment Cancel reply
Your email address will not be published. Required fields are marked *
Save my name, email, and website in this browser for the next time I comment.
- Print Friendly

- Aims and Objectives – A Guide for Academic Writing
- Doing a PhD
One of the most important aspects of a thesis, dissertation or research paper is the correct formulation of the aims and objectives. This is because your aims and objectives will establish the scope, depth and direction that your research will ultimately take. An effective set of aims and objectives will give your research focus and your reader clarity, with your aims indicating what is to be achieved, and your objectives indicating how it will be achieved.
Introduction
There is no getting away from the importance of the aims and objectives in determining the success of your research project. Unfortunately, however, it is an aspect that many students struggle with, and ultimately end up doing poorly. Given their importance, if you suspect that there is even the smallest possibility that you belong to this group of students, we strongly recommend you read this page in full.
This page describes what research aims and objectives are, how they differ from each other, how to write them correctly, and the common mistakes students make and how to avoid them. An example of a good aim and objectives from a past thesis has also been deconstructed to help your understanding.
What Are Aims and Objectives?
Research aims.
A research aim describes the main goal or the overarching purpose of your research project.
In doing so, it acts as a focal point for your research and provides your readers with clarity as to what your study is all about. Because of this, research aims are almost always located within its own subsection under the introduction section of a research document, regardless of whether it’s a thesis , a dissertation, or a research paper .
A research aim is usually formulated as a broad statement of the main goal of the research and can range in length from a single sentence to a short paragraph. Although the exact format may vary according to preference, they should all describe why your research is needed (i.e. the context), what it sets out to accomplish (the actual aim) and, briefly, how it intends to accomplish it (overview of your objectives).
To give an example, we have extracted the following research aim from a real PhD thesis:
Example of a Research Aim
The role of diametrical cup deformation as a factor to unsatisfactory implant performance has not been widely reported. The aim of this thesis was to gain an understanding of the diametrical deformation behaviour of acetabular cups and shells following impaction into the reamed acetabulum. The influence of a range of factors on deformation was investigated to ascertain if cup and shell deformation may be high enough to potentially contribute to early failure and high wear rates in metal-on-metal implants.
Note: Extracted with permission from thesis titled “T he Impact And Deformation Of Press-Fit Metal Acetabular Components ” produced by Dr H Hothi of previously Queen Mary University of London.
Research Objectives
Where a research aim specifies what your study will answer, research objectives specify how your study will answer it.
They divide your research aim into several smaller parts, each of which represents a key section of your research project. As a result, almost all research objectives take the form of a numbered list, with each item usually receiving its own chapter in a dissertation or thesis.
Following the example of the research aim shared above, here are it’s real research objectives as an example:
Example of a Research Objective
- Develop finite element models using explicit dynamics to mimic mallet blows during cup/shell insertion, initially using simplified experimentally validated foam models to represent the acetabulum.
- Investigate the number, velocity and position of impacts needed to insert a cup.
- Determine the relationship between the size of interference between the cup and cavity and deformation for different cup types.
- Investigate the influence of non-uniform cup support and varying the orientation of the component in the cavity on deformation.
- Examine the influence of errors during reaming of the acetabulum which introduce ovality to the cavity.
- Determine the relationship between changes in the geometry of the component and deformation for different cup designs.
- Develop three dimensional pelvis models with non-uniform bone material properties from a range of patients with varying bone quality.
- Use the key parameters that influence deformation, as identified in the foam models to determine the range of deformations that may occur clinically using the anatomic models and if these deformations are clinically significant.
It’s worth noting that researchers sometimes use research questions instead of research objectives, or in other cases both. From a high-level perspective, research questions and research objectives make the same statements, but just in different formats.
Taking the first three research objectives as an example, they can be restructured into research questions as follows:
Restructuring Research Objectives as Research Questions
- Can finite element models using simplified experimentally validated foam models to represent the acetabulum together with explicit dynamics be used to mimic mallet blows during cup/shell insertion?
- What is the number, velocity and position of impacts needed to insert a cup?
- What is the relationship between the size of interference between the cup and cavity and deformation for different cup types?
Difference Between Aims and Objectives
Hopefully the above explanations make clear the differences between aims and objectives, but to clarify:
- The research aim focus on what the research project is intended to achieve; research objectives focus on how the aim will be achieved.
- Research aims are relatively broad; research objectives are specific.
- Research aims focus on a project’s long-term outcomes; research objectives focus on its immediate, short-term outcomes.
- A research aim can be written in a single sentence or short paragraph; research objectives should be written as a numbered list.
How to Write Aims and Objectives
Before we discuss how to write a clear set of research aims and objectives, we should make it clear that there is no single way they must be written. Each researcher will approach their aims and objectives slightly differently, and often your supervisor will influence the formulation of yours on the basis of their own preferences.
Regardless, there are some basic principles that you should observe for good practice; these principles are described below.
Your aim should be made up of three parts that answer the below questions:
- Why is this research required?
- What is this research about?
- How are you going to do it?
The easiest way to achieve this would be to address each question in its own sentence, although it does not matter whether you combine them or write multiple sentences for each, the key is to address each one.
The first question, why , provides context to your research project, the second question, what , describes the aim of your research, and the last question, how , acts as an introduction to your objectives which will immediately follow.
Scroll through the image set below to see the ‘why, what and how’ associated with our research aim example.
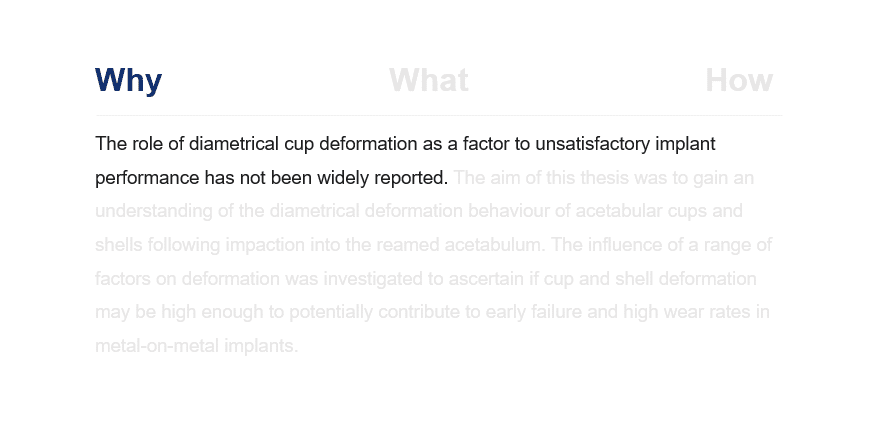
Note: Your research aims need not be limited to one. Some individuals per to define one broad ‘overarching aim’ of a project and then adopt two or three specific research aims for their thesis or dissertation. Remember, however, that in order for your assessors to consider your research project complete, you will need to prove you have fulfilled all of the aims you set out to achieve. Therefore, while having more than one research aim is not necessarily disadvantageous, consider whether a single overarching one will do.
Research Objectives
Each of your research objectives should be SMART :
- Specific – is there any ambiguity in the action you are going to undertake, or is it focused and well-defined?
- Measurable – how will you measure progress and determine when you have achieved the action?
- Achievable – do you have the support, resources and facilities required to carry out the action?
- Relevant – is the action essential to the achievement of your research aim?
- Timebound – can you realistically complete the action in the available time alongside your other research tasks?
In addition to being SMART, your research objectives should start with a verb that helps communicate your intent. Common research verbs include:
Table of Research Verbs to Use in Aims and Objectives
Last, format your objectives into a numbered list. This is because when you write your thesis or dissertation, you will at times need to make reference to a specific research objective; structuring your research objectives in a numbered list will provide a clear way of doing this.
To bring all this together, let’s compare the first research objective in the previous example with the above guidance:
Checking Research Objective Example Against Recommended Approach
Research Objective:
1. Develop finite element models using explicit dynamics to mimic mallet blows during cup/shell insertion, initially using simplified experimentally validated foam models to represent the acetabulum.
Checking Against Recommended Approach:
Q: Is it specific? A: Yes, it is clear what the student intends to do (produce a finite element model), why they intend to do it (mimic cup/shell blows) and their parameters have been well-defined ( using simplified experimentally validated foam models to represent the acetabulum ).
Q: Is it measurable? A: Yes, it is clear that the research objective will be achieved once the finite element model is complete.
Q: Is it achievable? A: Yes, provided the student has access to a computer lab, modelling software and laboratory data.
Q: Is it relevant? A: Yes, mimicking impacts to a cup/shell is fundamental to the overall aim of understanding how they deform when impacted upon.
Q: Is it timebound? A: Yes, it is possible to create a limited-scope finite element model in a relatively short time, especially if you already have experience in modelling.
Q: Does it start with a verb? A: Yes, it starts with ‘develop’, which makes the intent of the objective immediately clear.
Q: Is it a numbered list? A: Yes, it is the first research objective in a list of eight.
Mistakes in Writing Research Aims and Objectives
1. making your research aim too broad.
Having a research aim too broad becomes very difficult to achieve. Normally, this occurs when a student develops their research aim before they have a good understanding of what they want to research. Remember that at the end of your project and during your viva defence , you will have to prove that you have achieved your research aims; if they are too broad, this will be an almost impossible task. In the early stages of your research project, your priority should be to narrow your study to a specific area. A good way to do this is to take the time to study existing literature, question their current approaches, findings and limitations, and consider whether there are any recurring gaps that could be investigated .
Note: Achieving a set of aims does not necessarily mean proving or disproving a theory or hypothesis, even if your research aim was to, but having done enough work to provide a useful and original insight into the principles that underlie your research aim.
2. Making Your Research Objectives Too Ambitious
Be realistic about what you can achieve in the time you have available. It is natural to want to set ambitious research objectives that require sophisticated data collection and analysis, but only completing this with six months before the end of your PhD registration period is not a worthwhile trade-off.
3. Formulating Repetitive Research Objectives
Each research objective should have its own purpose and distinct measurable outcome. To this effect, a common mistake is to form research objectives which have large amounts of overlap. This makes it difficult to determine when an objective is truly complete, and also presents challenges in estimating the duration of objectives when creating your project timeline. It also makes it difficult to structure your thesis into unique chapters, making it more challenging for you to write and for your audience to read.
Fortunately, this oversight can be easily avoided by using SMART objectives.
Hopefully, you now have a good idea of how to create an effective set of aims and objectives for your research project, whether it be a thesis, dissertation or research paper. While it may be tempting to dive directly into your research, spending time on getting your aims and objectives right will give your research clear direction. This won’t only reduce the likelihood of problems arising later down the line, but will also lead to a more thorough and coherent research project.
Finding a PhD has never been this easy – search for a PhD by keyword, location or academic area of interest.
Browse PhDs Now
Join thousands of students.
Join thousands of other students and stay up to date with the latest PhD programmes, funding opportunities and advice.
- Link to facebook
- Link to linkedin
- Link to twitter
- Link to youtube
- Writing Tips
How to Write Research Objectives
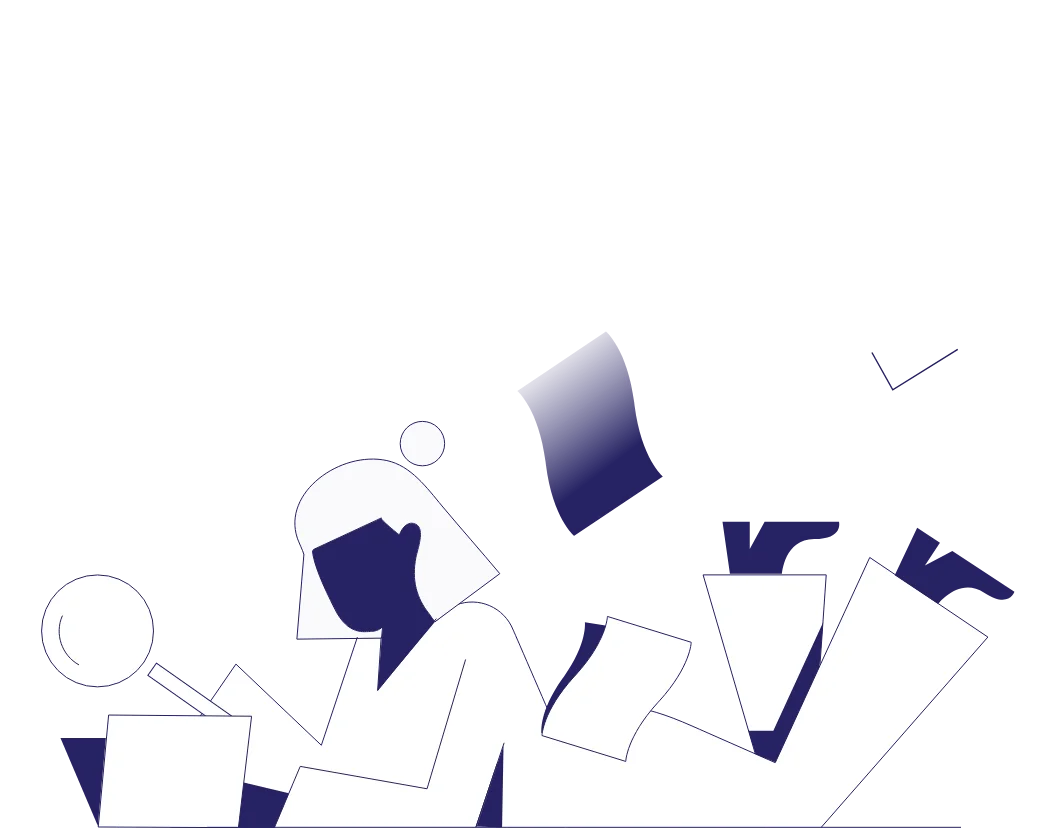
3-minute read
- 22nd November 2021
Writing a research paper, thesis, or dissertation ? If so, you’ll want to state your research objectives in the introduction of your paper to make it clear to your readers what you’re trying to accomplish. But how do you write effective research objectives? In this post, we’ll look at two key topics to help you do this:
- How to use your research aims as a basis for developing objectives.
- How to use SMART criteria to refine your research objectives.
For more advice on how to write strong research objectives, see below.
Research Aims and Objectives
There is an important difference between research aims and research objectives:
- A research aim defines the main purpose of your research. As such, you can think of your research aim as answering the question “What are you doing?”
- Research objectives (as most studies will have more than one) are the steps you will take to fulfil your aims. As such, your objectives should answer the question “How are you conducting your research?”
For instance, an example research aim could be:
This study will investigate the link between dehydration and the incidence of urinary tract infections (UTIs) in intensive care patients in Australia.
To develop a set of research objectives, you would then break down the various steps involved in meeting said aim. For example:
This study will investigate the link between dehydration and the incidence of urinary tract infections (UTIs) in intensive care patients in Australia. To achieve this, the study objectives w ill include:
- Replicat ing a small Singaporean study into the role of dehydration in UTIs in hospital patients (Sepe, 2018) in a larger Australian cohort.
- Trialing the use of intravenous fluids for intensive care patients to prevent dehydration.
- Assessing the relationship between the age of patients and quantities of intravenous fluids needed to counter dehydration.
Find this useful?
Subscribe to our newsletter and get writing tips from our editors straight to your inbox.
Note that the objectives don’t go into any great detail here. The key is to briefly summarize each component of your study. You can save details for how you will conduct the research for the methodology section of your paper.
Make Your Research Objectives SMART
A great way to refine your research objectives is to use SMART criteria . Borrowed from the world of project management, there are many versions of this system. However, we’re going to focus on developing specific, measurable, achievable, relevant, and timebound objectives.
In other words, a good research objective should be all of the following:
- S pecific – Is the objective clear and well-defined?
- M easurable – How will you know when the objective has been achieved? Is there a way to measure the thing you’re seeking to do?
- A chievable – Do you have the support and resources necessary to undertake this action? Are you being overly ambitious with this objective?
- R elevant – Is this objective vital for fulfilling your research aim?
- T imebound – Can this action be realistically undertaken in the time you have?
If you follow this system, your research objectives will be much stronger.
Expert Research Proofreading
Whatever your research aims and objectives, make sure to have your academic writing proofread by the experts!
Our academic editors can help you with research papers and proposals , as well as any other scholarly document you need checking. And this will help to ensure that your academic writing is always clear, concise, and precise.
Submit a free sample document today to trial our services and find out more.
Share this article:
Post A New Comment
Got content that needs a quick turnaround? Let us polish your work. Explore our editorial business services.
What is a content editor.
Are you interested in learning more about the role of a content editor and the...
4-minute read
The Benefits of Using an Online Proofreading Service
Proofreading is important to ensure your writing is clear and concise for your readers. Whether...
2-minute read
6 Online AI Presentation Maker Tools
Creating presentations can be time-consuming and frustrating. Trying to construct a visually appealing and informative...
What Is Market Research?
No matter your industry, conducting market research helps you keep up to date with shifting...
8 Press Release Distribution Services for Your Business
In a world where you need to stand out, press releases are key to being...
How to Get a Patent
In the United States, the US Patent and Trademarks Office issues patents. In the United...
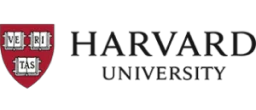
Make sure your writing is the best it can be with our expert English proofreading and editing.

21 Research Objectives Examples (Copy and Paste)
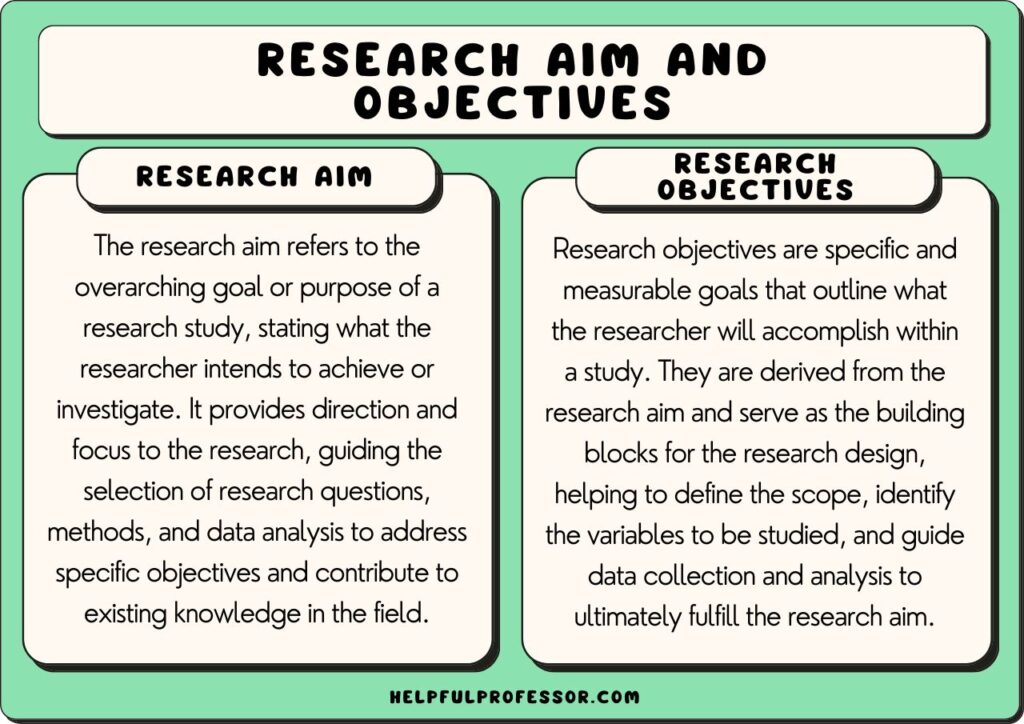
Research objectives refer to the definitive statements made by researchers at the beginning of a research project detailing exactly what a research project aims to achieve.
These objectives are explicit goals clearly and concisely projected by the researcher to present a clear intention or course of action for his or her qualitative or quantitative study.
Research objectives are typically nested under one overarching research aim. The objectives are the steps you’ll need to take in order to achieve the aim (see the examples below, for example, which demonstrate an aim followed by 3 objectives, which is what I recommend to my research students).
Research Objectives vs Research Aims
Research aim and research objectives are fundamental constituents of any study, fitting together like two pieces of the same puzzle.
The ‘research aim’ describes the overarching goal or purpose of the study (Kumar, 2019). This is usually a broad, high-level purpose statement, summing up the central question that the research intends to answer.
Example of an Overarching Research Aim:
“The aim of this study is to explore the impact of climate change on crop productivity.”
Comparatively, ‘research objectives’ are concrete goals that underpin the research aim, providing stepwise actions to achieve the aim.
Objectives break the primary aim into manageable, focused pieces, and are usually characterized as being more specific, measurable, achievable, relevant, and time-bound (SMART).
Examples of Specific Research Objectives:
1. “To examine the effects of rising temperatures on the yield of rice crops during the upcoming growth season.” 2. “To assess changes in rainfall patterns in major agricultural regions over the first decade of the twenty-first century (2000-2010).” 3. “To analyze the impact of changing weather patterns on crop diseases within the same timeframe.”
The distinction between these two terms, though subtle, is significant for successfully conducting a study. The research aim provides the study with direction, while the research objectives set the path to achieving this aim, thereby ensuring the study’s efficiency and effectiveness.
How to Write Research Objectives
I usually recommend to my students that they use the SMART framework to create their research objectives.
SMART is an acronym standing for Specific, Measurable, Achievable, Relevant, and Time-bound. It provides a clear method of defining solid research objectives and helps students know where to start in writing their objectives (Locke & Latham, 2013).
Each element of this acronym adds a distinct dimension to the framework, aiding in the creation of comprehensive, well-delineated objectives.
Here is each step:
- Specific : We need to avoid ambiguity in our objectives. They need to be clear and precise (Doran, 1981). For instance, rather than stating the objective as “to study the effects of social media,” a more focused detail would be “to examine the effects of social media use (Facebook, Instagram, and Twitter) on the academic performance of college students.”
- Measurable: The measurable attribute provides a clear criterion to determine if the objective has been met (Locke & Latham, 2013). A quantifiable element, such as a percentage or a number, adds a measurable quality. For example, “to increase response rate to the annual customer survey by 10%,” makes it easier to ascertain achievement.
- Achievable: The achievable aspect encourages researchers to craft realistic objectives, resembling a self-check mechanism to ensure the objectives align with the scope and resources at disposal (Doran, 1981). For example, “to interview 25 participants selected randomly from a population of 100” is an attainable objective as long as the researcher has access to these participants.
- Relevance : Relevance, the fourth element, compels the researcher to tailor the objectives in alignment with overarching goals of the study (Locke & Latham, 2013). This is extremely important – each objective must help you meet your overall one-sentence ‘aim’ in your study.
- Time-Bound: Lastly, the time-bound element fosters a sense of urgency and prioritization, preventing procrastination and enhancing productivity (Doran, 1981). “To analyze the effect of laptop use in lectures on student engagement over the course of two semesters this year” expresses a clear deadline, thus serving as a motivator for timely completion.
You’re not expected to fit every single element of the SMART framework in one objective, but across your objectives, try to touch on each of the five components.
Research Objectives Examples
1. Field: Psychology
Aim: To explore the impact of sleep deprivation on cognitive performance in college students.
- Objective 1: To compare cognitive test scores of students with less than six hours of sleep and those with 8 or more hours of sleep.
- Objective 2: To investigate the relationship between class grades and reported sleep duration.
- Objective 3: To survey student perceptions and experiences on how sleep deprivation affects their cognitive capabilities.
2. Field: Environmental Science
Aim: To understand the effects of urban green spaces on human well-being in a metropolitan city.
- Objective 1: To assess the physical and mental health benefits of regular exposure to urban green spaces.
- Objective 2: To evaluate the social impacts of urban green spaces on community interactions.
- Objective 3: To examine patterns of use for different types of urban green spaces.
3. Field: Technology
Aim: To investigate the influence of using social media on productivity in the workplace.
- Objective 1: To measure the amount of time spent on social media during work hours.
- Objective 2: To evaluate the perceived impact of social media use on task completion and work efficiency.
- Objective 3: To explore whether company policies on social media usage correlate with different patterns of productivity.
4. Field: Education
Aim: To examine the effectiveness of online vs traditional face-to-face learning on student engagement and achievement.
- Objective 1: To compare student grades between the groups exposed to online and traditional face-to-face learning.
- Objective 2: To assess student engagement levels in both learning environments.
- Objective 3: To collate student perceptions and preferences regarding both learning methods.
5. Field: Health
Aim: To determine the impact of a Mediterranean diet on cardiac health among adults over 50.
- Objective 1: To assess changes in cardiovascular health metrics after following a Mediterranean diet for six months.
- Objective 2: To compare these health metrics with a similar group who follow their regular diet.
- Objective 3: To document participants’ experiences and adherence to the Mediterranean diet.
6. Field: Environmental Science
Aim: To analyze the impact of urban farming on community sustainability.
- Objective 1: To document the types and quantity of food produced through urban farming initiatives.
- Objective 2: To assess the effect of urban farming on local communities’ access to fresh produce.
- Objective 3: To examine the social dynamics and cooperative relationships in the creating and maintaining of urban farms.
7. Field: Sociology
Aim: To investigate the influence of home offices on work-life balance during remote work.
- Objective 1: To survey remote workers on their perceptions of work-life balance since setting up home offices.
- Objective 2: To conduct an observational study of daily work routines and family interactions in a home office setting.
- Objective 3: To assess the correlation, if any, between physical boundaries of workspaces and mental boundaries for work in the home setting.
8. Field: Economics
Aim: To evaluate the effects of minimum wage increases on small businesses.
- Objective 1: To analyze cost structures, pricing changes, and profitability of small businesses before and after minimum wage increases.
- Objective 2: To survey small business owners on the strategies they employ to navigate minimum wage increases.
- Objective 3: To examine employment trends in small businesses in response to wage increase legislation.
9. Field: Education
Aim: To explore the role of extracurricular activities in promoting soft skills among high school students.
- Objective 1: To assess the variety of soft skills developed through different types of extracurricular activities.
- Objective 2: To compare self-reported soft skills between students who participate in extracurricular activities and those who do not.
- Objective 3: To investigate the teachers’ perspectives on the contribution of extracurricular activities to students’ skill development.
10. Field: Technology
Aim: To assess the impact of virtual reality (VR) technology on the tourism industry.
- Objective 1: To document the types and popularity of VR experiences available in the tourism market.
- Objective 2: To survey tourists on their interest levels and satisfaction rates with VR tourism experiences.
- Objective 3: To determine whether VR tourism experiences correlate with increased interest in real-life travel to the simulated destinations.
11. Field: Biochemistry
Aim: To examine the role of antioxidants in preventing cellular damage.
- Objective 1: To identify the types and quantities of antioxidants in common fruits and vegetables.
- Objective 2: To determine the effects of various antioxidants on free radical neutralization in controlled lab tests.
- Objective 3: To investigate potential beneficial impacts of antioxidant-rich diets on long-term cellular health.
12. Field: Linguistics
Aim: To determine the influence of early exposure to multiple languages on cognitive development in children.
- Objective 1: To assess cognitive development milestones in monolingual and multilingual children.
- Objective 2: To document the number and intensity of language exposures for each group in the study.
- Objective 3: To investigate the specific cognitive advantages, if any, enjoyed by multilingual children.
13. Field: Art History
Aim: To explore the impact of the Renaissance period on modern-day art trends.
- Objective 1: To identify key characteristics and styles of Renaissance art.
- Objective 2: To analyze modern art pieces for the influence of the Renaissance style.
- Objective 3: To survey modern-day artists for their inspirations and the influence of historical art movements on their work.
14. Field: Cybersecurity
Aim: To assess the effectiveness of two-factor authentication (2FA) in preventing unauthorized system access.
- Objective 1: To measure the frequency of unauthorized access attempts before and after the introduction of 2FA.
- Objective 2: To survey users about their experiences and challenges with 2FA implementation.
- Objective 3: To evaluate the efficacy of different types of 2FA (SMS-based, authenticator apps, biometrics, etc.).
15. Field: Cultural Studies
Aim: To analyze the role of music in cultural identity formation among ethnic minorities.
- Objective 1: To document the types and frequency of traditional music practices within selected ethnic minority communities.
- Objective 2: To survey community members on the role of music in their personal and communal identity.
- Objective 3: To explore the resilience and transmission of traditional music practices in contemporary society.
16. Field: Astronomy
Aim: To explore the impact of solar activity on satellite communication.
- Objective 1: To categorize different types of solar activities and their frequencies of occurrence.
- Objective 2: To ascertain how variations in solar activity may influence satellite communication.
- Objective 3: To investigate preventative and damage-control measures currently in place during periods of high solar activity.
17. Field: Literature
Aim: To examine narrative techniques in contemporary graphic novels.
- Objective 1: To identify a range of narrative techniques employed in this genre.
- Objective 2: To analyze the ways in which these narrative techniques engage readers and affect story interpretation.
- Objective 3: To compare narrative techniques in graphic novels to those found in traditional printed novels.
18. Field: Renewable Energy
Aim: To investigate the feasibility of solar energy as a primary renewable resource within urban areas.
- Objective 1: To quantify the average sunlight hours across urban areas in different climatic zones.
- Objective 2: To calculate the potential solar energy that could be harnessed within these areas.
- Objective 3: To identify barriers or challenges to widespread solar energy implementation in urban settings and potential solutions.
19. Field: Sports Science
Aim: To evaluate the role of pre-game rituals in athlete performance.
- Objective 1: To identify the variety and frequency of pre-game rituals among professional athletes in several sports.
- Objective 2: To measure the impact of pre-game rituals on individual athletes’ performance metrics.
- Objective 3: To examine the psychological mechanisms that might explain the effects (if any) of pre-game ritual on performance.
20. Field: Ecology
Aim: To investigate the effects of urban noise pollution on bird populations.
- Objective 1: To record and quantify urban noise levels in various bird habitats.
- Objective 2: To measure bird population densities in relation to noise levels.
- Objective 3: To determine any changes in bird behavior or vocalization linked to noise levels.
21. Field: Food Science
Aim: To examine the influence of cooking methods on the nutritional value of vegetables.
- Objective 1: To identify the nutrient content of various vegetables both raw and after different cooking processes.
- Objective 2: To compare the effect of various cooking methods on the nutrient retention of these vegetables.
- Objective 3: To propose cooking strategies that optimize nutrient retention.
The Importance of Research Objectives
The importance of research objectives cannot be overstated. In essence, these guideposts articulate what the researcher aims to discover, understand, or examine (Kothari, 2014).
When drafting research objectives, it’s essential to make them simple and comprehensible, specific to the point of being quantifiable where possible, achievable in a practical sense, relevant to the chosen research question, and time-constrained to ensure efficient progress (Kumar, 2019).
Remember that a good research objective is integral to the success of your project, offering a clear path forward for setting out a research design , and serving as the bedrock of your study plan. Each objective must distinctly address a different dimension of your research question or problem (Kothari, 2014). Always bear in mind that the ultimate purpose of your research objectives is to succinctly encapsulate your aims in the clearest way possible, facilitating a coherent, comprehensive and rational approach to your planned study, and furnishing a scientific roadmap for your journey into the depths of knowledge and research (Kumar, 2019).
Kothari, C.R (2014). Research Methodology: Methods and Techniques . New Delhi: New Age International.
Kumar, R. (2019). Research Methodology: A Step-by-Step Guide for Beginners .New York: SAGE Publications.
Doran, G. T. (1981). There’s a S.M.A.R.T. way to write management’s goals and objectives. Management review, 70 (11), 35-36.
Locke, E. A., & Latham, G. P. (2013). New Developments in Goal Setting and Task Performance . New York: Routledge.
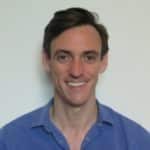
Chris Drew (PhD)
Dr. Chris Drew is the founder of the Helpful Professor. He holds a PhD in education and has published over 20 articles in scholarly journals. He is the former editor of the Journal of Learning Development in Higher Education. [Image Descriptor: Photo of Chris]
- Chris Drew (PhD) https://helpfulprofessor.com/author/chris-drew-phd/ 5 Top Tips for Succeeding at University
- Chris Drew (PhD) https://helpfulprofessor.com/author/chris-drew-phd/ 50 Durable Goods Examples
- Chris Drew (PhD) https://helpfulprofessor.com/author/chris-drew-phd/ 100 Consumer Goods Examples
- Chris Drew (PhD) https://helpfulprofessor.com/author/chris-drew-phd/ 30 Globalization Pros and Cons
Leave a Comment Cancel Reply
Your email address will not be published. Required fields are marked *
Research Objectives: Meaning, Types

A research objective addresses the purpose of the investigation and the types of knowledge generated from one’s investigation. It provides a framework for what is to be achieved by the study
What is the Research Objective?
A research objective addresses the purpose of the investigation and the types of knowledge generated from one’s investigation. Looking at the objectives of the research , one can anticipate what is to be achieved by the study.
A research objective indicates the population of interest and independent and dependent variables.
Many researchers state their research objective in the declarative form as a broad statement of purpose, such as the objective of this study is to examine the relationship between the initial salary (dependent variable) of those who are employed in NGOs (population of interest) and their previous job experience (independent variable).
The descriptive study does not always have variables that can be designated as independent or dependent.
In such a case, the objective indicates the nature of the inquiry, the study variables, and the population under study, as we find in the example.
This study aims to assess the women’s decision-making autonomy regarding their health care, their child’s health care, large household purchases, household purchases for daily needs, and visits to the women’s family or relatives.
In causal studies, the objectives are usually stated in the form of hypotheses.
Here is an example: Participation of women in household decision-making increases with age, their level of education, and the number of surviving children.
We can enumerate three major reasons for formulating the objectives of the research;
- Focus the study on narrowing it down to essentials;
- Avoid collection of data that are not strictly necessary for understanding and solving the problem at hand;
- Organize the study in clearly defined components or phases.
While formulating the research objectives, we should keep in mind that the results will be compared to the objectives when the study is evaluated.
If the objectives have not been formulated clearly, the study cannot be evaluated as desired. It is because of this reason; we should take care that the objectives fulfill certain criteria;
- They are realistic to fit the local environment.
- They cover the different aspects of the problem.
- They consider the contributing factors in a coherent way and logical sequence.
- They consider ethical issues, if any.
- They are phrased in operational terms.
Objectives should be closely related to the research problem statement, giving the sponsor specific, concrete, and achievable goals.
It is best to state the objectives of a study in general terms first and then move down to specific terms.
4 Types of Research Objectives
From this point of view, objectives are of two types: general and specific. We elaborate on these two concepts below, along with two more objectives: immediate objective and ultimate objective.
General Objective
The general objective of a study states what is expected to be achieved by the study in general terms.
For example, if the problem identified is the low utilization of Child Welfare Clinics (CWC), the general objective of the study could be:
- Identify the reasons for the low utilization of Child Welfare Clinics to find solutions.
Similarly, in a study on anemia in pregnancy, the general objective could be stated as:
- To study the changes in the hemoglobin level with an increase in the duration of pregnancy.
Or in a study to examine the contribution of goat farming to poverty alleviation, the general objective may be framed as follows:
- To assess the impact of investment in goat farming for poverty alleviation in rural Bangladesh.
Specific Objectives
Given that we have rightly stated the general objectives, it is advisable to break it down into several smaller, logically connected parts. These are normally referred to as specific objectives.
Specific objectives should systematically address the various aspects of the problems defined under the problem statement and the key factors that are assumed to influence or cause the problems.
They should specify what you will do in your study, where this study will be done, and for what purpose.
If formulated properly, specific objectives will facilitate the development of the research methodology and help the researcher orient the collection, analysis, interpretation, and utilization of data.
Thus in the anemia survey just cited above, the specific objectives could be
- To determine through history, the duration of pregnancy, parity, and the last birth interval of pregnant women in the study;
- To assess the hemoglobin level of pregnant women using Sahli’s method;
- To determine the changes in hemoglobin level with the duration of pregnancy, controlling for birth and parity.
Immediate Objectives
In addition to general objectives and specific objectives, a few studies, particularly evaluative studies, attempt to specify immediate objectives.
The immediate objective serves to indicate the focus of the proposed research in behavioral terms. The objective should specify the following points:
- Why are we going to do the study?
- Who will conduct the study?
- When will the study be conducted?
- What are we going to study?
- Whom will the study cover?
- How will the study be conducted?
The ‘why’ question addresses the rationale and objectives of the study.
The ‘whose’ question is designed to identify the individuals, firms, or organizations responsible for implementing the study.
The ‘when’ question seeks to know the study period.
The ‘what’ question addresses the issue of a statement of the problem, including the key variables.
The ‘ whom’ question seeks to answer the population to be studied.
The ‘ how’ question seeks to know the methodology to be followed, including the research design and sampling strategy to be employed.
Ultimate Objective
Most applied research studies have a statement of ultimate objective that focuses on how the results will be used to motivate the program managers and policymakers to implement and execute the recommendations from the survey results.
In the anemia survey, the ultimate objective may be stated as follows:
It is expected that the study’s findings will help enhance understanding of the effect of pregnancy on hemoglobin levels of mothers and thereby guide the physician’s incorrect iron therapy for pregnant women during the different gestational periods.
In the child nutrition survey cited above, the ultimate objectives were to highlight issues that policymakers and program managers need to address to improve the nutrition status of children in the country.
How are research objectives typically stated in causal studies?
What are the main reasons for formulating research objectives.
The main reasons for formulating research objectives are to focus the study, avoid unnecessary data collection , and organize the study in clearly defined components or phases.
What is the difference between general and specific research objectives?
The general objective of a study states the expected outcome in broad terms, while specific objectives break down the general objective into smaller, logically connected parts that address various aspects of the research problem.
What are the immediate objectives in research?
Immediate objectives indicate the focus of the proposed research in behavioral terms, specifying why, who, when, what, whom, and how the study will be conducted.
How does an ultimate objective differ from other research objectives?
The ultimate objective focuses on how the results of the research will be used, aiming to motivate program managers and policymakers to implement the recommendations derived from the study’s findings.
Why is it essential to state research objectives clearly?
Clear research objectives are crucial because the study’s results will be compared to these objectives when evaluating the study. If the objectives are not clearly formulated, the study cannot be evaluated as desired.

Your email address will not be published. Required fields are marked *
Writing the Research Objectives: 5 Straightforward Examples
The research objective of a research proposal or scientific article defines the direction or content of a research investigation. Without the research objectives, the proposal or research paper is in disarray. It is like a fisherman riding on a boat without any purpose and with no destination in sight. Therefore, at the beginning of any research venture, the researcher must be clear about what he or she intends to do or achieve in conducting a study.
How do you define the objectives of a study? What are the uses of the research objective? How would a researcher write this essential part of the research? This article aims to provide answers to these questions.
Table of Contents
Definition of a research objective.
A research objective describes, in a few words, the result of the research project after its implementation. It answers the question,
“ What does the researcher want or hope to achieve at the end of the research project.”
The research objective provides direction to the performance of the study.
What are the Uses of the Research Objective?
The uses of the research objective are enumerated below:
- serves as the researcher’s guide in identifying the appropriate research design,
- identifies the variables of the study, and
- specifies the data collection procedure and the corresponding analysis for the data generated.
The research design serves as the “blueprint” for the research investigation. The University of Southern California describes the different types of research design extensively. It details the data to be gathered, data collection procedure, data measurement, and statistical tests to use in the analysis.
The variables of the study include those factors that the researcher wants to evaluate in the study. These variables narrow down the research to several manageable components to see differences or correlations between them.
Specifying the data collection procedure ensures data accuracy and integrity . Thus, the probability of error is minimized. Generalizations or conclusions based on valid arguments founded on reliable data strengthens research findings on particular issues and problems.
In data mining activities where large data sets are involved, the research objective plays a crucial role. Without a clear objective to guide the machine learning process, the desired outcomes will not be met.
How is the Research Objective Written?
A research objective must be achievable, i.e., it must be framed keeping in mind the available time, infrastructure required for research, and other resources.
Before forming a research objective, you should read about all the developments in your area of research and find gaps in knowledge that need to be addressed. Readings will help you come up with suitable objectives for your research project.
5 Examples of Research Objectives
The following examples of research objectives based on several published studies on various topics demonstrate how the research objectives are written:
- This study aims to find out if there is a difference in quiz scores between students exposed to direct instruction and flipped classrooms (Webb and Doman, 2016).
- This study seeks to examine the extent, range, and method of coral reef rehabilitation projects in five shallow reef areas adjacent to popular tourist destinations in the Philippines (Yeemin et al ., 2006).
- This study aims to investigate species richness of mammal communities in five protected areas over the past 20 years (Evans et al ., 2006).
- This study aims to clarify the demographic, epidemiological, clinical, and radiological features of 2019-nCoV patients with other causes of pneumonia (Zhao et al ., 2020).
- This research aims to assess species extinction risks for sample regions that cover some 20% of the Earth’s terrestrial surface.
Finally, writing the research objectives requires constant practice, experience, and knowledge about the topic investigated. Clearly written objectives save time, money, and effort.
Once you have a clear idea of your research objectives, you can now develop your conceptual framework which is a crucial element of your research paper as it guides the flow of your research. The conceptual framework will help you develop your methodology and statistical tests.
I wrote a detailed, step-by-step guide on how to develop a conceptual framework with illustration in my post titled “ Conceptual Framework: A Step by Step Guide on How to Make One. “
Evans, K. L., Rodrigues, A. S., Chown, S. L., & Gaston, K. J. (2006). Protected areas and regional avian species richness in South Africa. Biology letters , 2 (2), 184-188.
Thomas, C. D., Cameron, A., Green, R. E., Bakkenes, M., Beaumont, L. J., Collingham, Y. C., … & Hughes, L. (2004). Extinction risk from climate change. Nature, 427(6970), 145-148.
Webb, M., & Doman, E. (2016). Does the Flipped Classroom Lead to Increased Gains on Learning Outcomes in ESL/EFL Contexts?. CATESOL Journal, 28(1), 39-67.
Yeemin, T., Sutthacheep, M., & Pettongma, R. (2006). Coral reef restoration projects in Thailand. Ocean & Coastal Management , 49 (9-10), 562-575.
Zhao, D., Yao, F., Wang, L., Zheng, L., Gao, Y., Ye, J., Guo, F., Zhao, H. & Gao, R. (2020). A comparative study on the clinical features of COVID-19 pneumonia to other pneumonias, Clinical Infectious Diseases , ciaa247, https://doi.org/10.1093/cid/ciaa247
© 2020 March 23 P. A. Regoniel Updated 17 November 2020 | Updated 18 January 2024
Related Posts
Do you know that the computer can disturb your sleeping patterns.
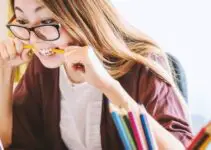
Conceptual Framework: A Step-by-Step Guide on How to Make One
Simplified explanation of probability in statistics, about the author, patrick regoniel.
Dr. Regoniel, a faculty member of the graduate school, served as consultant to various environmental research and development projects covering issues and concerns on climate change, coral reef resources and management, economic valuation of environmental and natural resources, mining, and waste management and pollution. He has extensive experience on applied statistics, systems modelling and analysis, an avid practitioner of LaTeX, and a multidisciplinary web developer. He leverages pioneering AI-powered content creation tools to produce unique and comprehensive articles in this website.
thank you for clarification
This is excellent
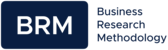
Formulating Research Aims and Objectives
Formulating research aim and objectives in an appropriate manner is one of the most important aspects of your thesis. This is because research aim and objectives determine the scope, depth and the overall direction of the research. Research question is the central question of the study that has to be answered on the basis of research findings.
Research aim emphasizes what needs to be achieved within the scope of the research, by the end of the research process. Achievement of research aim provides answer to the research question.
Research objectives divide research aim into several parts and address each part separately. Research aim specifies WHAT needs to be studied and research objectives comprise a number of steps that address HOW research aim will be achieved.
As a rule of dumb, there would be one research aim and several research objectives. Achievement of each research objective will lead to the achievement of the research aim.
Consider the following as an example:
Research title: Effects of organizational culture on business profitability: a case study of Virgin Atlantic
Research aim: To assess the effects of Virgin Atlantic organizational culture on business profitability
Following research objectives would facilitate the achievement of this aim:
- Analyzing the nature of organizational culture at Virgin Atlantic by September 1, 2022
- Identifying factors impacting Virgin Atlantic organizational culture by September 16, 2022
- Analyzing impacts of Virgin Atlantic organizational culture on employee performances by September 30, 2022
- Providing recommendations to Virgin Atlantic strategic level management in terms of increasing the level of effectiveness of organizational culture by October 5, 2022
Figure below illustrates additional examples in formulating research aims and objectives:

Formulation of research question, aim and objectives
Common mistakes in the formulation of research aim relate to the following:
1. Choosing the topic too broadly . This is the most common mistake. For example, a research title of “an analysis of leadership practices” can be classified as too broad because the title fails to answer the following questions:
a) Which aspects of leadership practices? Leadership has many aspects such as employee motivation, ethical behaviour, strategic planning, change management etc. An attempt to cover all of these aspects of organizational leadership within a single research will result in an unfocused and poor work.
b) An analysis of leadership practices in which country? Leadership practices tend to be different in various countries due to cross-cultural differences, legislations and a range of other region-specific factors. Therefore, a study of leadership practices needs to be country-specific.
c) Analysis of leadership practices in which company or industry? Similar to the point above, analysis of leadership practices needs to take into account industry-specific and/or company-specific differences, and there is no way to conduct a leadership research that relates to all industries and organizations in an equal manner.
Accordingly, as an example “a study into the impacts of ethical behaviour of a leader on the level of employee motivation in US healthcare sector” would be a more appropriate title than simply “An analysis of leadership practices”.
2. Setting an unrealistic aim . Formulation of a research aim that involves in-depth interviews with Apple strategic level management by an undergraduate level student can be specified as a bit over-ambitious. This is because securing an interview with Apple CEO Tim Cook or members of Apple Board of Directors might not be easy. This is an extreme example of course, but you got the idea. Instead, you may aim to interview the manager of your local Apple store and adopt a more feasible strategy to get your dissertation completed.
3. Choosing research methods incompatible with the timeframe available . Conducting interviews with 20 sample group members and collecting primary data through 2 focus groups when only three months left until submission of your dissertation can be very difficult, if not impossible. Accordingly, timeframe available need to be taken into account when formulating research aims and objectives and selecting research methods.
Moreover, research objectives need to be formulated according to SMART principle,
where the abbreviation stands for specific, measurable, achievable, realistic, and time-bound.
Examples of SMART research objectives
At the conclusion part of your research project you will need to reflect on the level of achievement of research aims and objectives. In case your research aims and objectives are not fully achieved by the end of the study, you will need to discuss the reasons. These may include initial inappropriate formulation of research aims and objectives, effects of other variables that were not considered at the beginning of the research or changes in some circumstances during the research process.
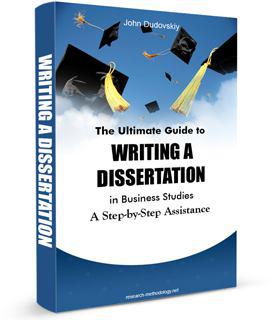
John Dudovskiy
Have a language expert improve your writing
Run a free plagiarism check in 10 minutes, generate accurate citations for free.
- Knowledge Base
Methodology
- What Is a Research Design | Types, Guide & Examples
What Is a Research Design | Types, Guide & Examples
Published on June 7, 2021 by Shona McCombes . Revised on November 20, 2023 by Pritha Bhandari.
A research design is a strategy for answering your research question using empirical data. Creating a research design means making decisions about:
- Your overall research objectives and approach
- Whether you’ll rely on primary research or secondary research
- Your sampling methods or criteria for selecting subjects
- Your data collection methods
- The procedures you’ll follow to collect data
- Your data analysis methods
A well-planned research design helps ensure that your methods match your research objectives and that you use the right kind of analysis for your data.
Table of contents
Step 1: consider your aims and approach, step 2: choose a type of research design, step 3: identify your population and sampling method, step 4: choose your data collection methods, step 5: plan your data collection procedures, step 6: decide on your data analysis strategies, other interesting articles, frequently asked questions about research design.
- Introduction
Before you can start designing your research, you should already have a clear idea of the research question you want to investigate.
There are many different ways you could go about answering this question. Your research design choices should be driven by your aims and priorities—start by thinking carefully about what you want to achieve.
The first choice you need to make is whether you’ll take a qualitative or quantitative approach.
Qualitative research designs tend to be more flexible and inductive , allowing you to adjust your approach based on what you find throughout the research process.
Quantitative research designs tend to be more fixed and deductive , with variables and hypotheses clearly defined in advance of data collection.
It’s also possible to use a mixed-methods design that integrates aspects of both approaches. By combining qualitative and quantitative insights, you can gain a more complete picture of the problem you’re studying and strengthen the credibility of your conclusions.
Practical and ethical considerations when designing research
As well as scientific considerations, you need to think practically when designing your research. If your research involves people or animals, you also need to consider research ethics .
- How much time do you have to collect data and write up the research?
- Will you be able to gain access to the data you need (e.g., by travelling to a specific location or contacting specific people)?
- Do you have the necessary research skills (e.g., statistical analysis or interview techniques)?
- Will you need ethical approval ?
At each stage of the research design process, make sure that your choices are practically feasible.
Receive feedback on language, structure, and formatting
Professional editors proofread and edit your paper by focusing on:
- Academic style
- Vague sentences
- Style consistency
See an example
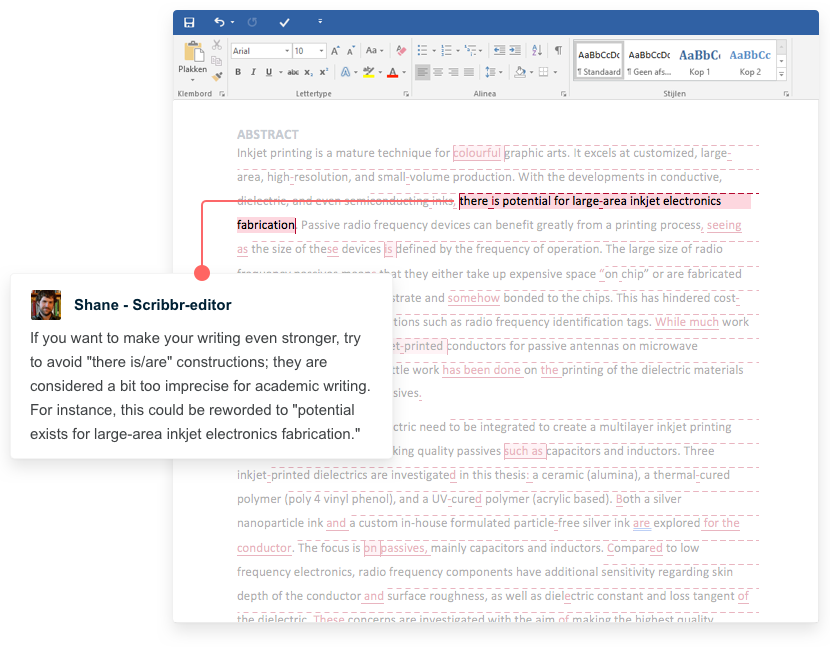
Within both qualitative and quantitative approaches, there are several types of research design to choose from. Each type provides a framework for the overall shape of your research.
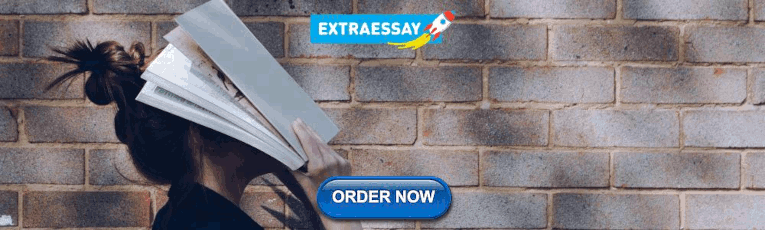
Types of quantitative research designs
Quantitative designs can be split into four main types.
- Experimental and quasi-experimental designs allow you to test cause-and-effect relationships
- Descriptive and correlational designs allow you to measure variables and describe relationships between them.
With descriptive and correlational designs, you can get a clear picture of characteristics, trends and relationships as they exist in the real world. However, you can’t draw conclusions about cause and effect (because correlation doesn’t imply causation ).
Experiments are the strongest way to test cause-and-effect relationships without the risk of other variables influencing the results. However, their controlled conditions may not always reflect how things work in the real world. They’re often also more difficult and expensive to implement.
Types of qualitative research designs
Qualitative designs are less strictly defined. This approach is about gaining a rich, detailed understanding of a specific context or phenomenon, and you can often be more creative and flexible in designing your research.
The table below shows some common types of qualitative design. They often have similar approaches in terms of data collection, but focus on different aspects when analyzing the data.
Your research design should clearly define who or what your research will focus on, and how you’ll go about choosing your participants or subjects.
In research, a population is the entire group that you want to draw conclusions about, while a sample is the smaller group of individuals you’ll actually collect data from.
Defining the population
A population can be made up of anything you want to study—plants, animals, organizations, texts, countries, etc. In the social sciences, it most often refers to a group of people.
For example, will you focus on people from a specific demographic, region or background? Are you interested in people with a certain job or medical condition, or users of a particular product?
The more precisely you define your population, the easier it will be to gather a representative sample.
- Sampling methods
Even with a narrowly defined population, it’s rarely possible to collect data from every individual. Instead, you’ll collect data from a sample.
To select a sample, there are two main approaches: probability sampling and non-probability sampling . The sampling method you use affects how confidently you can generalize your results to the population as a whole.
Probability sampling is the most statistically valid option, but it’s often difficult to achieve unless you’re dealing with a very small and accessible population.
For practical reasons, many studies use non-probability sampling, but it’s important to be aware of the limitations and carefully consider potential biases. You should always make an effort to gather a sample that’s as representative as possible of the population.
Case selection in qualitative research
In some types of qualitative designs, sampling may not be relevant.
For example, in an ethnography or a case study , your aim is to deeply understand a specific context, not to generalize to a population. Instead of sampling, you may simply aim to collect as much data as possible about the context you are studying.
In these types of design, you still have to carefully consider your choice of case or community. You should have a clear rationale for why this particular case is suitable for answering your research question .
For example, you might choose a case study that reveals an unusual or neglected aspect of your research problem, or you might choose several very similar or very different cases in order to compare them.
Data collection methods are ways of directly measuring variables and gathering information. They allow you to gain first-hand knowledge and original insights into your research problem.
You can choose just one data collection method, or use several methods in the same study.
Survey methods
Surveys allow you to collect data about opinions, behaviors, experiences, and characteristics by asking people directly. There are two main survey methods to choose from: questionnaires and interviews .
Observation methods
Observational studies allow you to collect data unobtrusively, observing characteristics, behaviors or social interactions without relying on self-reporting.
Observations may be conducted in real time, taking notes as you observe, or you might make audiovisual recordings for later analysis. They can be qualitative or quantitative.
Other methods of data collection
There are many other ways you might collect data depending on your field and topic.
If you’re not sure which methods will work best for your research design, try reading some papers in your field to see what kinds of data collection methods they used.
Secondary data
If you don’t have the time or resources to collect data from the population you’re interested in, you can also choose to use secondary data that other researchers already collected—for example, datasets from government surveys or previous studies on your topic.
With this raw data, you can do your own analysis to answer new research questions that weren’t addressed by the original study.
Using secondary data can expand the scope of your research, as you may be able to access much larger and more varied samples than you could collect yourself.
However, it also means you don’t have any control over which variables to measure or how to measure them, so the conclusions you can draw may be limited.
Here's why students love Scribbr's proofreading services
Discover proofreading & editing
As well as deciding on your methods, you need to plan exactly how you’ll use these methods to collect data that’s consistent, accurate, and unbiased.
Planning systematic procedures is especially important in quantitative research, where you need to precisely define your variables and ensure your measurements are high in reliability and validity.
Operationalization
Some variables, like height or age, are easily measured. But often you’ll be dealing with more abstract concepts, like satisfaction, anxiety, or competence. Operationalization means turning these fuzzy ideas into measurable indicators.
If you’re using observations , which events or actions will you count?
If you’re using surveys , which questions will you ask and what range of responses will be offered?
You may also choose to use or adapt existing materials designed to measure the concept you’re interested in—for example, questionnaires or inventories whose reliability and validity has already been established.
Reliability and validity
Reliability means your results can be consistently reproduced, while validity means that you’re actually measuring the concept you’re interested in.
For valid and reliable results, your measurement materials should be thoroughly researched and carefully designed. Plan your procedures to make sure you carry out the same steps in the same way for each participant.
If you’re developing a new questionnaire or other instrument to measure a specific concept, running a pilot study allows you to check its validity and reliability in advance.
Sampling procedures
As well as choosing an appropriate sampling method , you need a concrete plan for how you’ll actually contact and recruit your selected sample.
That means making decisions about things like:
- How many participants do you need for an adequate sample size?
- What inclusion and exclusion criteria will you use to identify eligible participants?
- How will you contact your sample—by mail, online, by phone, or in person?
If you’re using a probability sampling method , it’s important that everyone who is randomly selected actually participates in the study. How will you ensure a high response rate?
If you’re using a non-probability method , how will you avoid research bias and ensure a representative sample?
Data management
It’s also important to create a data management plan for organizing and storing your data.
Will you need to transcribe interviews or perform data entry for observations? You should anonymize and safeguard any sensitive data, and make sure it’s backed up regularly.
Keeping your data well-organized will save time when it comes to analyzing it. It can also help other researchers validate and add to your findings (high replicability ).
On its own, raw data can’t answer your research question. The last step of designing your research is planning how you’ll analyze the data.
Quantitative data analysis
In quantitative research, you’ll most likely use some form of statistical analysis . With statistics, you can summarize your sample data, make estimates, and test hypotheses.
Using descriptive statistics , you can summarize your sample data in terms of:
- The distribution of the data (e.g., the frequency of each score on a test)
- The central tendency of the data (e.g., the mean to describe the average score)
- The variability of the data (e.g., the standard deviation to describe how spread out the scores are)
The specific calculations you can do depend on the level of measurement of your variables.
Using inferential statistics , you can:
- Make estimates about the population based on your sample data.
- Test hypotheses about a relationship between variables.
Regression and correlation tests look for associations between two or more variables, while comparison tests (such as t tests and ANOVAs ) look for differences in the outcomes of different groups.
Your choice of statistical test depends on various aspects of your research design, including the types of variables you’re dealing with and the distribution of your data.
Qualitative data analysis
In qualitative research, your data will usually be very dense with information and ideas. Instead of summing it up in numbers, you’ll need to comb through the data in detail, interpret its meanings, identify patterns, and extract the parts that are most relevant to your research question.
Two of the most common approaches to doing this are thematic analysis and discourse analysis .
There are many other ways of analyzing qualitative data depending on the aims of your research. To get a sense of potential approaches, try reading some qualitative research papers in your field.
If you want to know more about the research process , methodology , research bias , or statistics , make sure to check out some of our other articles with explanations and examples.
- Simple random sampling
- Stratified sampling
- Cluster sampling
- Likert scales
- Reproducibility
Statistics
- Null hypothesis
- Statistical power
- Probability distribution
- Effect size
- Poisson distribution
Research bias
- Optimism bias
- Cognitive bias
- Implicit bias
- Hawthorne effect
- Anchoring bias
- Explicit bias
A research design is a strategy for answering your research question . It defines your overall approach and determines how you will collect and analyze data.
A well-planned research design helps ensure that your methods match your research aims, that you collect high-quality data, and that you use the right kind of analysis to answer your questions, utilizing credible sources . This allows you to draw valid , trustworthy conclusions.
Quantitative research designs can be divided into two main categories:
- Correlational and descriptive designs are used to investigate characteristics, averages, trends, and associations between variables.
- Experimental and quasi-experimental designs are used to test causal relationships .
Qualitative research designs tend to be more flexible. Common types of qualitative design include case study , ethnography , and grounded theory designs.
The priorities of a research design can vary depending on the field, but you usually have to specify:
- Your research questions and/or hypotheses
- Your overall approach (e.g., qualitative or quantitative )
- The type of design you’re using (e.g., a survey , experiment , or case study )
- Your data collection methods (e.g., questionnaires , observations)
- Your data collection procedures (e.g., operationalization , timing and data management)
- Your data analysis methods (e.g., statistical tests or thematic analysis )
A sample is a subset of individuals from a larger population . Sampling means selecting the group that you will actually collect data from in your research. For example, if you are researching the opinions of students in your university, you could survey a sample of 100 students.
In statistics, sampling allows you to test a hypothesis about the characteristics of a population.
Operationalization means turning abstract conceptual ideas into measurable observations.
For example, the concept of social anxiety isn’t directly observable, but it can be operationally defined in terms of self-rating scores, behavioral avoidance of crowded places, or physical anxiety symptoms in social situations.
Before collecting data , it’s important to consider how you will operationalize the variables that you want to measure.
A research project is an academic, scientific, or professional undertaking to answer a research question . Research projects can take many forms, such as qualitative or quantitative , descriptive , longitudinal , experimental , or correlational . What kind of research approach you choose will depend on your topic.
Cite this Scribbr article
If you want to cite this source, you can copy and paste the citation or click the “Cite this Scribbr article” button to automatically add the citation to our free Citation Generator.
McCombes, S. (2023, November 20). What Is a Research Design | Types, Guide & Examples. Scribbr. Retrieved April 5, 2024, from https://www.scribbr.com/methodology/research-design/
Is this article helpful?
Shona McCombes
Other students also liked, guide to experimental design | overview, steps, & examples, how to write a research proposal | examples & templates, ethical considerations in research | types & examples, "i thought ai proofreading was useless but..".
I've been using Scribbr for years now and I know it's a service that won't disappoint. It does a good job spotting mistakes”

The Importance Of Research Objectives
Imagine you’re a student planning a vacation in a foreign country. You’re on a tight budget and need to draw…

Imagine you’re a student planning a vacation in a foreign country. You’re on a tight budget and need to draw up a pocket-friendly plan. Where do you begin? The first step is to do your research.
Before that, you make a mental list of your objectives—finding reasonably-priced hotels, traveling safely and finding ways of communicating with someone back home. These objectives help you focus sharply during your research and be aware of the finer details of your trip.
More often than not, research is a part of our daily lives. Whether it’s to pick a restaurant for your next birthday dinner or to prepare a presentation at work, good research is the foundation of effective learning. Read on to understand the meaning, importance and examples of research objectives.
Why Do We Need Research?
What are the objectives of research, what goes into a research plan.
Research is a careful and detailed study of a particular problem or concern, using scientific methods. An in-depth analysis of information creates space for generating new questions, concepts and understandings. The main objective of research is to explore the unknown and unlock new possibilities. It’s an essential component of success.
Over the years, businesses have started emphasizing the need for research. You’ve probably noticed organizations hiring research managers and analysts. The primary purpose of business research is to determine the goals and opportunities of an organization. It’s critical in making business decisions and appropriately allocating available resources.
Here are a few benefits of research that’ll explain why it is a vital aspect of our professional lives:
Expands Your Knowledge Base
One of the greatest benefits of research is to learn and gain a deeper understanding. The deeper you dig into a topic, the more well-versed you are. Furthermore, research has the power to help you build on any personal experience you have on the subject.
Keeps You Up To Date
Research encourages you to discover the most recent information available. Updated information prevents you from falling behind and helps you present accurate information. You’re better equipped to develop ideas or talk about a topic when you’re armed with the latest inputs.
Builds Your Credibility
Research provides you with a good foundation upon which you can develop your thoughts and ideas. People take you more seriously when your suggestions are backed by research. You can speak with greater confidence because you know that the information is accurate.
Sparks Connections
Take any leading nonprofit organization, you’ll see how they have a strong research arm supported by real-life stories. Research also becomes the base upon which real-life connections and impact can be made. It even helps you communicate better with others and conveys why you’re pursuing something.
Encourages Curiosity
As we’ve already established, research is mostly about using existing information to create new ideas and opinions. In the process, it sparks curiosity as you’re encouraged to explore and gain deeper insights into a subject. Curiosity leads to higher levels of positivity and lower levels of anxiety.
Well-defined objectives of research are an essential component of successful research engagement. If you want to drive all aspects of your research methodology such as data collection, design, analysis and recommendation, you need to lay down the objectives of research methodology. In other words, the objectives of research should address the underlying purpose of investigation and analysis. It should outline the steps you’d take to achieve desirable outcomes. Research objectives help you stay focused and adjust your expectations as you progress.
The objectives of research should be closely related to the problem statement, giving way to specific and achievable goals. Here are the four types of research objectives for you to explore:
General Objective
Also known as secondary objectives, general objectives provide a detailed view of the aim of a study. In other words, you get a general overview of what you want to achieve by the end of your study. For example, if you want to study an organization’s contribution to environmental sustainability, your general objective could be: a study of sustainable practices and the use of renewable energy by the organization.
Specific Objectives
Specific objectives define the primary aim of the study. Typically, general objectives provide the foundation for identifying specific objectives. In other words, when general objectives are broken down into smaller and logically connected objectives, they’re known as specific objectives. They help define the who, what, why, when and how aspects of your project. Once you identify the main objective of research, it’s easier to develop and pursue a plan of action.
Let’s take the example of ‘a study of an organization’s contribution to environmental sustainability’ again. The specific objectives will look like this:
To determine through history how the organization has changed its practices and adopted new solutions
To assess how the new practices, technology and strategies will contribute to the overall effectiveness
Once you’ve identified the objectives of research, it’s time to organize your thoughts and streamline your research goals. Here are a few effective tips to develop a powerful research plan and improve your business performance.
Set SMART Goals
Your research objectives should be SMART—Specific, Measurable, Achievable, Realistic and Time-constrained. When you focus on utilizing available resources and setting realistic timeframes and milestones, it’s easier to prioritize objectives. Continuously track your progress and check whether you need to revise your expectations or targets. This way, you’re in greater control over the process.
Create A Plan
Create a plan that’ll help you select appropriate methods to collect accurate information. A well-structured plan allows you to use logical and creative approaches towards problem-solving. The complexity of information and your skills are bound to influence your plan, which is why you need to make room for flexibility. The availability of resources will also play a big role in influencing your decisions.
Collect And Collate
After you’ve created a plan for the research process, make a list of the data you’re going to collect and the methods you’ll use. Not only will it help make sense of your insights but also keep track of your approach. The information you collect should be:
Logical, rigorous and objective
Can be reproduced by other people working on the same subject
Free of errors and highlighting necessary details
Current and updated
Includes everything required to support your argument/suggestions
Analyze And Keep Ready
Data analysis is the most crucial part of the process and there are many ways in which the information can be utilized. Four types of data analysis are often seen in a professional environment. While they may be divided into separate categories, they’re linked to each other.
Descriptive Analysis:
The most commonly used data analysis, descriptive analysis simply summarizes past data. For example, Key Performance Indicators (KPIs) use descriptive analysis. It establishes certain benchmarks after studying how someone has been performing in the past.
Diagnostic Analysis:
The next step is to identify why something happened. Diagnostic analysis uses the information gathered through descriptive analysis and helps find the underlying causes of an outcome. For example, if a marketing initiative was successful, you deep-dive into the strategies that worked.
Predictive Analysis:
It attempts to answer ‘what’s likely to happen’. Predictive analysis makes use of past data to predict future outcomes. However, the accuracy of predictions depends on the quality of the data provided. Risk assessment is an ideal example of using predictive analysis.
Prescriptive Analysis:
The most sought-after type of data analysis, prescriptive analysis combines the insights of all of the previous analyses. It’s a huge organizational commitment as it requires plenty of effort and resources. A great example of prescriptive analysis is Artificial Intelligence (AI), which consumes large amounts of data. You need to be prepared to commit to this type of analysis.
Review And Interpret
Once you’ve collected and collated your data, it’s time to review it and draw accurate conclusions. Here are a few ways to improve the review process:
Identify the fundamental issues, opportunities and problems and make note of recurring trends if any
Make a list of your insights and check which is the most or the least common. In short, keep track of the frequency of each insight
Conduct a SWOT analysis and identify the strengths, weaknesses, opportunities and threats
Write down your conclusions and recommendations of the research
When we think about research, we often associate it with academicians and students. but the truth is research is for everybody who is willing to learn and enhance their knowledge. If you want to master the art of strategically upgrading your knowledge, Harappa Education’s Learning Expertly course has all the answers. Not only will it help you look at things from a fresh perspective but also show you how to acquire new information with greater efficiency. The Growth Mindset framework will teach you how to believe in your abilities to grow and improve. The Learning Transfer framework will help you apply your learnings from one context to another. Begin the journey of tactful learning and self-improvement today!
Explore Harappa Diaries to learn more about topics related to the THINK Habit such as Learning From Experience , Critical Thinking & What is Brainstorming to think clearly and rationally.
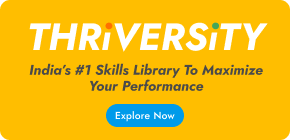
- Open access
- Published: 03 April 2024
Capturing artificial intelligence applications’ value proposition in healthcare – a qualitative research study
- Jasmin Hennrich 1 ,
- Eva Ritz 2 ,
- Peter Hofmann 1 , 4 &
- Nils Urbach 1 , 3
BMC Health Services Research volume 24 , Article number: 420 ( 2024 ) Cite this article
430 Accesses
Metrics details
Artificial intelligence (AI) applications pave the way for innovations in the healthcare (HC) industry. However, their adoption in HC organizations is still nascent as organizations often face a fragmented and incomplete picture of how they can capture the value of AI applications on a managerial level. To overcome adoption hurdles, HC organizations would benefit from understanding how they can capture AI applications’ potential.
We conduct a comprehensive systematic literature review and 11 semi-structured expert interviews to identify, systematize, and describe 15 business objectives that translate into six value propositions of AI applications in HC.
Our results demonstrate that AI applications can have several business objectives converging into risk-reduced patient care, advanced patient care, self-management, process acceleration, resource optimization, and knowledge discovery.
We contribute to the literature by extending research on value creation mechanisms of AI to the HC context and guiding HC organizations in evaluating their AI applications or those of the competition on a managerial level, to assess AI investment decisions, and to align their AI application portfolio towards an overarching strategy.
Peer Review reports
Applications based on artificial intelligence (AI) have the potential to transform the healthcare (HC) industry [ 1 ]. AI applications can be characterized as applications or agents with capabilities that typically demand intelligence [ 2 , 3 ]. In our context, we understand AI as a collection of technological solutions from the field of applied computer science, in which algorithms are trained on medical and HC data to perform tasks that are normally associated with human intelligence (i.e., medical decision-making) [ 4 ]. AI is not a single type of technology, instead, it encompasses a diverse array of technologies spread across various application areas in HC, such as diagnostics (e.g., [ 5 ], biomedical research (e.g., [ 6 ], clinical administration (e.g., [ 7 ], therapy (e.g., [ 8 ], and intelligent robotics (e.g., [ 9 ]. These areas are expected to benefit from AI applications’ capabilities, such as accuracy, objectivity, rapidity, data processing, and automation [ 10 , 11 ]. Accordingly, AI applications are said to have the potential to drive business value and enhance HC [ 12 ], paving the way for transformative innovations in the HC industry [ 13 ]. There are already many promising AI use cases in HC that are expected to improve patient care and create value for HC organizations. For instance, AI applications can advance the quality of patient care by supporting radiologists with more accurate and rapid diagnosis, compensating for humans’ limitations (e.g., data processing speeds) and weaknesses (e.g., inattention, distraction, and fatigue) [ 10 , 14 ]. Klicken oder tippen Sie hier, um Text einzugeben.While the use of AI applications in HC has the overarching goal of creating significant value for patients through improved care, they also come with the potential for business value creation and the opportunity for HC organizations to gain a competitive edge (e.g., [ 15 , 16 ]).
Despite the promised advantages, AI applications’ implementation is slow, and the full realization of their potential within the HC industry is yet to be achieved [ 11 , 17 ]. With just a handful of practical examples of AI applications in the HC industry [ 13 , 18 ], the adoption of AI applications is still in its infancy. The AI in Healthcare Survey Report stated that in 2021, only 9% of respondents worldwide have reached a sophisticated adoption of AI Models, while 32% of respondents are still in the early stages of adopting AI models. According to the survey, the majority of HC organizations (60%) are not actively considering AI as a solution, or they are currently evaluating AI use cases and experimenting with the implementation [ 19 ]. Nevertheless, HC startups are increasingly entering the market [ 20 ], pressuring incumbent HC organizations to evaluate and adopt AI applications. Existing studies already investigate AI technologies in various use cases in HC and provide insights on how to design AI-based services [ 21 ], explain in detail the technical functions and capabilities of AI technologies [ 10 , 11 ], or take on a practical perspective with a focus on concrete examples of AI applications [ 14 ]. However, to foster the adoption of AI applications, HC organizations should understand how they can unfold AI applications’ capabilities into business value to ensure effective investments. Previous studies on the intersection of information systems and value creation have expressed interest into how organizations can actually gain value through the use of technology and thus, enhance their adoption [ 22 , 23 ]. However, to the best of our knowledge, a comprehensive investigation of the value creation of AI applications in the context of HC from a managerial level is currently missing. Thus, our study aims to investigate AI applications’ value creation and capture mechanisms in the specific HC context by answering the following question: How can HC organizations create and capture AI applications’ value?
We conduct a systematic literature analysis and semi structured expert interviews to answer this research question. In the systematic literature analysis, we identify and analyze a heterogeneous set of 21 AI use cases across five different HC application fields and derive 15 business objectives and six value propositions for HC organizations. We then evaluate and refine the categorized business objectives and value propositions with insights from 11 expert interviews. Our study contributes to research on the value creation mechanism of AI applications in the HC context. Moreover, our results have managerial implications for HC organizations since they can draw on our results to evaluate AI applications, assess investment decisions, and align their AI application portfolio toward an overarching strategy.
In what follows, this study first grounds on relevant work to gain a deeper understanding of the underlying constructs of AI in HC. Next, we describe our qualitative research method by describing the process of data collection and analysis, followed by our derived results on capturing AI applications’ value proposition in HC. Afterward, we discuss our results, including this study’s limitations and pathways for further research. Finally, we summarize our findings and their contribution to theory and practice in the conclusion.
Relevant work
In the realm of AI, a thorough exploration of its key subdiscipline, machine learning (ML), is essential [ 24 , 25 ]. ML is a computational model that learns from data without explicitly programming the data [ 24 ] and can be further divided into supervised, unsupervised, and reinforcement learning [ 26 ]. In supervised learning, the machine undergoes training with labeled data, making it well-suited for tasks involving regression and classification problems [ 27 ]. In contrast, unsupervised learning is designed to automatically identify patterns within unlabeled datasets [ 28 ], with its primary utility lying in the extraction of features [ 11 ]. Reinforcement learning, characterized as a method of systematic experimentation or trial and error, involves a situated agent taking specific actions and observing the rewards it gains from those actions, facilitating the learning of behavior in a given environment [ 29 ]. The choice of which type of ML will be used in the different application areas depends on the specific problem, the availability of labeled data, and the nature of the desired outcome.
In recent years, the rapid advances in AI have triggered a revolution in various areas, with numerous impressive advantages. In the financial sector, AI applications can significantly improve security by detecting anomalies and preventing fraud [ 30 ]. Within education, AI has emerged as a powerful tool for tailoring learning experiences, aiming to enhance engagement, understanding, and retention [ 31 ]. In the energy market, the efficacy of AI extends to fault detection and diagnosis in building energy systems, showcasing its robust capabilities in ensuring system integrity [ 32 ]. Moreover, the HC industry is expected to be a promising application area for AI applications. The HC sector is undergoing a significant transformation due to the increasing adoption of digital technologies, with AI technologies at the forefront of this shift. The increasing relevance of AI technologies in HC is underlined by a growing and multidisciplinary stream of AI research, as highlighted by Secinaro et al. [ 33 ]. Taking a closer look at the different application areas in HC, AI applications offer promising potential, as demonstrated by the following exemplary AI use cases. In diagnosis, AI applications can identify complex patterns in medical image data more accurately, resulting in precise and objective disease recognition. This can improve patient safety by reducing the risks of misinterpretation [ 5 ]. Another use case can be found in biomedical research. For example, AI technology is commonly used for de novo drug design. AI can rapidly browse through molecule libraries to detect nearly \({10}^{60}\) drug-like molecules, accelerating the drug development process [ 6 ]. Furthermore, AI applications are used in clinical administration. They enable optimized operation room capacities by automating the process and by including information about absence or waiting times, as well as predicting interruptions [ 34 ]. Furthermore, AI applications are used in therapy by predicting personalized medication dosages. As this helps to reduce the mortality risk, it leads to enhanced patient outcomes and quality of care [ 35 ]. Intelligent prostheses by which patients can improve interactions are another use case. The AI algorithm continuously detects and classifies myoelectric signal patterns to predict movements, leading to reduced training expenditure and more self-management by the patient [ 36 ]. In summary, envisioning that AI applications successfully address persisting challenges, such as lack of transparency (e.g., [ 37 ], bias (e.g., [ 38 ], privacy concerns, and trust issues (e.g., [ 39 ], the potential of AI applications is vast. The conceivable benefits extend to individual practitioners and HC organizations, including hospitals, enabling them to harness AI applications for creating business value and ultimately enhancing competitiveness. Thereby, we follow Schryen’s (p. 141) revisited definition of business value of technologies: “the impact of investments on the multidimensional performance and capabilities of economic entities at various levels, complemented by the ultimate meaning of performance in the economic environment” [ 40 ]. His perspective includes all kinds of tangible value (such as an increase in productivity or reduced costs) to intangible value (such as service innovation or customer satisfaction), as well as internal value for the HC organizations and external value for stakeholders, shareholders, and customers. To create business value, it is essential to have a clear understanding of how the potential of AI applications can be captured. The understanding of how information systems, in general, create value is already covered in the literature. For example, Badakhshan et al. [ 31 ] focus on how process mining can pave the way to create business value. Leidner et al. [ 32 ] examine how enterprise social media adds value for new employees, and Lehrer et al. [ 33 ] answer the question of how big data analytics can enable service. There are also studies focusing on the value creation of information systems in the context of HC. For instance, the study by Haddad and Wickramasinghe [ 41 ] shows that information technology in HC can capture value by improving the quality of HC delivery, increasing safety, or offering additional services. Strong et al. [ 42 ] analyze how electronic health records afford value for HC organizations and determine goal-oriented actions to capture this potential. There is even literature on how machine learning adds value within the discipline of radiology (e.g., [ 43 ].
However, these studies either do not address the context of HC, consider technologies other than AI or information systems in general, or focus only on a small area of HC (e.g., radiology) and a subset of AI technology (e.g., machine learning). Although these studies deliver valuable insights into the value creation of information systems, a comprehensive picture of how HC organizations can capture business value with AI applications is missing.
To answer our research question, we adopted a qualitative inductive research design. This research design is consistent with studies that took a similar perspective on how technologies can create business value [ 44 ]. In conducting our structured literature review, we followed the approach of Webster and Watson [ 45 ] and included recommendations of Wolfswinkel et al. [ 46 ] when considering the inclusion and exclusion criteria. We started by collecting relevant data on different successful AI use cases across five application areas in HC. Siggelkow [ 47 ] argued that use cases are able to provide persuasive arguments for causal relationships. In an initial literature screening, we identified five promising application domains focusing on AI applications for patients and HC providers: disease diagnostics (DD) (e.g., [ 5 ], biomedical research (BR) (e.g., [ 6 ], clinical administration (CA) (e.g., [ 7 ], therapy (T) (e.g., [ 8 ], and intelligent robotics (IR) (e.g., [ 9 ]. Second, to sample AI use cases, we aimed to collect a heterogeneous set of AI use cases within these application domains and consider the heterogeneity in AI applications, underlying data, innovation types, and implementation stages when selecting 21 AI use cases for our in-depth analysis. The AI use case and an exemplary study for each use case are listed in Table 1 .
After sampling the AI use cases, we used PubMed to identify papers for each use case. PubMed is recognized as a common database for biomedical and medical research for HC topics in the information systems domain (e.g., [ 62 , 63 ]. Our search included journal articles, clinical conferences, clinical studies, and comparative studies in English as of 2010. Based on the AI use case sample, we derived a search string based on keywords [ 45 ] considering titles and abstracts by following Shepherd et al. [ 62 ] guidelines. It was aimed to narrow and specific selection to increase data collection replicability for the use cases. Boolean operators (AND, OR) are used to improve results by combining search terms [ 62 ].
((artificial intelligence AND (radiology OR (cancer AND imaging) OR (radiology AND error) OR (cancer AND genomics) OR (speech AND cognitive AND impairment) OR (voice AND parkinson) OR EEG OR (facial AND analysis) OR (drug AND design) OR (Drug AND Biomarker) OR De-identification OR Splicing OR (emergency AND triage) OR (mortality AND prediction) OR (operating AND room) OR text summarization OR (artificial AND pancreas) OR vasopressor OR Chatbot OR (myoelectric prosthesis) OR (automated surgery task) OR (surgery AND workflow)))
The initial search led to 877 results (see Fig. 1 ). After title screening, we eliminated 516 papers that are not relevant (i.e., not covering a specific AI application, only including the description of AI algorithm, or not including a managerial perspective and the value created by AI applications). We further excluded 162 papers because their abstract is not concurrent with any specific use case (e.g., because they were literature reviews on overarching topics and did not include a specific AI application). We screened the remaining 199 papers for eligibility through two content-related criteria. First, papers need to cover an AI use case’s whole value proposition creation path, including information on data, algorithms, functions, competitive advantage, and business value of a certain AI application. The papers often only examine how a certain application works but lack the value proposition perspective, which leads to the exclusion of 63 articles. Second, we removed 89 papers that do not match any of our use cases. This step led to a remaining set of 47 relevant papers. During a backward-forward search according to Webster and Watson [ 45 ] and Levy and Ellis [ 64 ], we additionally included 35 papers. We also incorporated previous and subsequent clinical studies of the same researcher, resulting in an additional six papers. The final set contains 88 relevant papers describing the identified AI use cases, whereby at least three papers describe each AI use case.
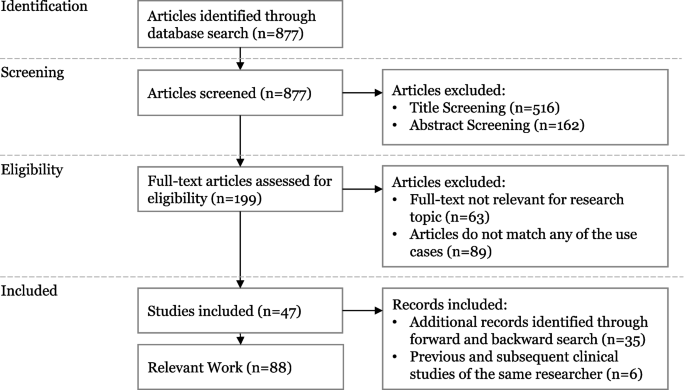
Search strategy
In the second step, we engaged in open, axial, and selective coding of the AI use cases following analysis techniques of grounded theory [ 65 ]. We focused on extracting business objectives, detailing how each AI application drives value. We documented these for each AI use case by recording codes of business objectives and value propositions and assigning relationships among the open codes. For example, from the following text passage of Berlyand et al. [ 56 ], who investigate the use case CA1: “Rapidly interpreting clinical data to classify patients and predict outcomes is paramount to emergency department operations, with direct impacts on cost, efficiency, and quality of care”, we derived the code rapid task execution.
After analyzing the AI use cases, we revised the documented tuples to foster consistency and comparability. Then, we iteratively coded the identified tuples by relying on selective coding techniques which is a process to identify and refine categories at a highly generalizable degree [ 65 ]. In all 14 coding iterations, one author continuously compares, relates, and associates categories and properties and discusses the coding results with another author. We modified some tuples during the coding process in two ways. First, we equalized small phrasing disparities for homogenous and refined wording. Second, we carefully adjusted the tuples regarding coherency. Finally, we reviewed the coding schema for internal validity through a final comparison with the data [ 66 ]. Then, we set the core variables “business objectives” and “value propositions”. We refer to business objectives as improvements through implementing the technology that drives a value proposition. We define value proposition as the inherent commitment to deliver reciprocal value to the organization, its customers, and/or partners [ 67 ].
In the third step following Schultze and Avital [ 68 ], we conducted semi structured expert interviews to evaluate and refine the value propositions and business objectives. We developed and refined an interview script following the guidelines of Meyers and Newman [ 69 ] for qualitative interviews. An additional file shows the used interview script (see Additional file 1 ). We conducted expert sampling to select suitable interviewees [ 70 ]. Due to the interdisciplinarity of the research topic, we chose experts in the two knowledge areas, AI and HC. In the process of expert selection, we ensured that interviewees possessed a minimum of two years of experience in their respective fields. We aimed for a well-balanced mix of diverse professions and positions among the interviewees. Additionally, for those with a primary background in HC, we specifically verified their proficiency and understanding of AI, ensuring a comprehensive perspective across the entire expert panel. Table 2 provides an overview of our expert sample. The interviewees were recruited in the authors’ networks and by cold calling. Identified experts were first contacted by email, including some brief information regarding the study. If there was no response within two weeks, they were contacted again by telephone to arrange an interview date. In total, we conducted 11 interviews that took place in a time range between 40 and 75 min. The expert interviews are transcribed verbatim using the software f4. As a coding aid, we use the software MAXQDA—a tool for qualitative data analysis which is frequently used in the analyses of qualitative data in the HC domain (e.g., [ 38 , 71 , 72 ]).
To systematically decompose how HC organizations can realize value propositions from AI applications, we identified 15 business objectives and six value propositions (see Fig. 2 ). These business objectives and value propositions resulted from analyzing the collected data, which we derived from the literature and refined through expert interviews. In the following, we describe the six value propositions and elaborate on how the specific AI business objectives can result in value propositions. This will be followed by a discussion of the results in the discussion of the paper.
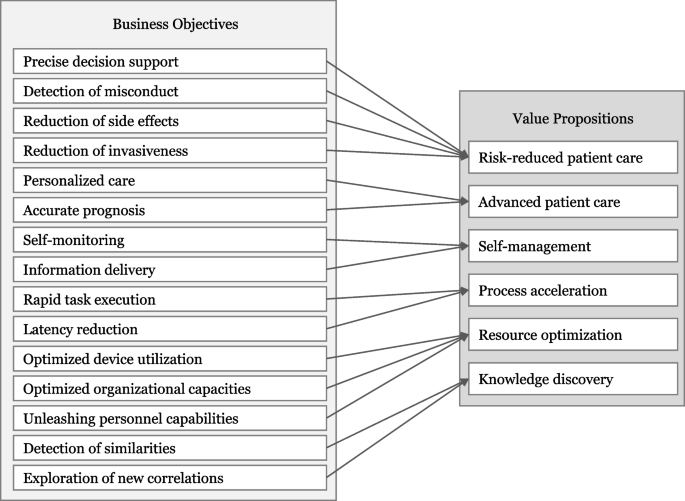
Business objectives and value propositions risk-reduced patient care
This value proposition follows business objectives that may identify and reduce threats and adverse factors during medical procedures. HC belongs to a high-risk domain since there are uncertain external factors (E4), including physicians’ fatigue, distractions, or cognitive biases [ 73 , 74 ]. AI applications can reduce certain risks by enabling precise decision support, detecting misconduct, reducing emergent side effects, and reducing invasiveness.
Precise decision support stems from AI applications’ capability to integrate various data types into the decision-making process, gaining a sophisticated overview of a phenomenon. Precise knowledge about all uncertainty factors reduces the ambiguity of decision-making processes [ 49 ]. E5 confirms that AI applications can be seen as a “perceptual enhancement”, enabling more comprehensive and context-based decision support. Humans are naturally prone to innate and socially adapted biases that also affect HC professionals [ 14 ]. Use Case CA1 highlights how rapid decision-making by HC professionals during emergency triage may lead to overlooking subtle yet crucial signs. AI applications can offer decision support based on historical data, enhancing objectivity and accuracy [ 56 ].
Detection of misconduct is possible since AI applications can map and monitor clinical workflows and recognize irregularities early. In this context, E10 highlights that “one of the best examples is the interception of abnormalities.” For instance, AI applications can assist in allocating medications in hospitals (Use case T2). Since HC professionals can be tired or distracted in medication preparation, AI applications may avoid serious consequences for patients by monitoring allocation processes and patients’ reactions. Thus, AI applications can reduce abuse and increase safety.
Reduction of emergent side effects is enabled by AI applications that continuously monitor and process data. If different treatments and medications are combined during a patient’s clinical pathway, it may cause overdosage or evoke co-effects and comorbidities, causing danger for the patient [ 75 ]. AI applications can prevent these by detecting and predicting these effects. For instance, AI applications can calculate the medication dosage for the individual and predict contraindications (Use case T2) [ 76 ]. E3 adds that the reduction of side effects also includes “cross-impacts between medications or possible symptoms that only occur for patients of a certain age or disease.” Avoidable side effects can thus be detected at an early stage, resulting in better outcomes.
Reduction of invasiveness of medical treatments or surgeries is possible by allowing AI applications to compensate for and overcome human weaknesses and limitations. During surgery, AI applications can continuously monitor a robot’s position and accurately predict its trajectories [ 77 ]. Intelligent robots can eliminate human tremors and access hard-to-reach body parts [ 60 ]. E2 validates, “a robot does not tremble; a robot moves in a perfectly straight line.” The precise AI-controlled movement of surgical robots minimizes the risk of injuring nearby vessels and organs [ 61 ]. Use cases DD5 and DD7 elucidate how AI applications enable new methods to perform noninvasive diagnoses. Reducing invasiveness has a major impact on the patient’s recovery, safety, and outcome quality.
Advanced patient care
Advanced patient care follows business objectives that extend patient care to increase the quality of care. One of HC’s primary goals is to provide the most effective treatment outcome. AI applications can advance patient care as they enable personalized care and accurate prognosis.
Personalized care can be enabled by the ability of AI technologies to integrate and process individual structured and unstructured patient data to increase the compatibility of patient and health interventions. For instance, by analyzing genome mutations, AI applications precisely assess cancer, enabling personalized therapy and increasing the likelihood of enhancing outcome quality (Use case DD4). E11 sums up that “we can improve treatment or even make it more specific for the patient. This is, of course, the dream of healthcare”. Use case T1 exemplifies how the integration of AI applications facilitates personalized products, such as an artificial pancreas. The pancreas predicts glucose levels in real time and adapts insulin supplementation. Personalized care allows good care to be made even better by tailoring care to the individual.
Accurate prognosis is achieved by AI applications that track, combine, and analyze HC data and historical data to make accurate predictions. For instance, AI applications can precisely analyze tumor tissue to improve the stratification of cancer patients. Based on this result, the selection of adjuvant therapy can be refined, improving the effectiveness of care [ 48 ]. Use case DD6 shows how AI applications can predict seizure onset zones to enhance the prognosis of epileptic seizures. In this context, E10 adds that an accurate prognosis fosters early and preventive care.
Self-management
Self-management follows the business objectives that increase disease controllability through the support of intelligent medical products. AI applications can foster self-management by self-monitoring and providing a new way of delivering information.
Self-monitoring is enhanced by AI applications, which can automatically process frequently measured data. There are AI-based chatbots, mobile applications, wearables, and other medical products that gather periodic data and are used by people to monitor themselves in the health context (e.g., [ 78 , 79 ]. Frequent data collection of these products (e.g., using sensors) enables AI applications to analyze periodic data and become aware of abnormalities. While the amount of data rises, the applications can improve their performance continuously (E2). Through continuous tracking of heartbeats via wearables, AI applications can precisely detect irregularities, notify their users in the case of irregularities, empower quicker treatment (E2), and may reduce hospital visits (E9). Self-monitoring enhances patient safety and allows the patient to be more physician-independent and involved in their HC.
Information delivery to the patient is enabled by AI applications that give medical advice adjusted to the patient’s needs. Often, patients lack profound knowledge about their anomalies. AI applications can contextualize patients’ symptoms to provide anamnesis support and deliver interactive advice [ 59 ]. While HC professionals must focus on one diagnostic pathway, AI applications can process information to investigate different diagnostic branches simultaneously (E5). Thus, these applications can deliver high-quality information based on the patient’s feedback, for instance, when using an intelligent conversational agent (use case T3). E4 highlights that this can improve doctoral consultations because “the patient is already informed and already has information when he comes to talk to doctors”.
Process acceleration
Process acceleration comprises business objectives that enable speed and low latencies. Speed describes how fast one can perform a task, while latency specifies how much time elapses from an event until a task is executed. AI applications can accelerate processes by rapid task execution and reducing latency.
Rapid task execution can be achieved by the ability of AI applications to process large amounts of data and identify patterns in a short time. In this context, E4 mentions that AI applications can drill diagnosis down to seconds. For instance, whereas doctors need several minutes for profound image-based detection, AI applications have a much faster report turnaround time (use case DD1). Besides, rapid data processing also opens up new opportunities in drug development. AI applications can rapidly browse through molecule libraries to detect nearly 10^60 molecules, which are synthetically available (use case BR1). This immense speed during a discovery process has an essential influence on the business potential and can enormously decrease research costs (E10).
Latency reduction can be enabled by AI technologies monitoring and dynamically processing information and environmental factors. By continuously evaluating vital signs and electrocardiogram records, AI applications can predict the in-house mortality of patients in real time [ 57 ]. The AI application can detect an increased mortality risk faster than HC professionals, enabling a more rapid emergency intervention. In this case, AI applications decrease the time delay between the cause and the reaction, which positively impacts patient care. E7 emphasizes the importance of short latencies: “One of the most important things is that the timeframe between the point when all the data is available, and a decision has been made, […] must be kept short.”
Resource optimization
Resource optimization follows the business objectives that manage limited resources and capacities. The HC industry faces a lack of sufficient resources, especially through a shortage of specialists (E8), which in turn negatively influences waiting times. AI applications can support efficient resource allocation by optimizing device utilization, organizational capacities and unleashing personnel capabilities.
Optimized device utilization can be enhanced by AI applications that track, analyze, and precisely predict load of times of medical equipment in real-time. For instance, AI applications can maximize X-Ray or magnetic resonance tomography device utilization (use case CA3). Besides, AI applications can enable a dynamic replanning of device utilization by including absence or waiting times and predicting interruptions. Intelligent resource optimization may include various key variables (e.g., the maximized lifespan of a radiation scanner) [ 48 ]. Optimized device utilization reduces the time periods when the device is not utilized, and thus, losses are made.
Optimized organizational capacities are possible due to AI applications breaking up static key performance indicators and finding more dynamic measuring approaches for the required workflow changes (E5, E10). The utilization of capacities in hospitals relies on various known and unknown parameters, which are often interdependent [ 80 ]. AI applications can detect and optimize these dependencies to manage capacity. An example is the optimization of clinical occupancy in the hospital (use case CA3), which has a strong impact on cost. E5 adds that the integration of AI applications may increase the reliability of planning HC resources since they can predict capacity trends from historical occupancy rates. Optimized planning of capacities can prevent capacities from remaining unused and fixed costs from being offset by no revenue.
Unleashing personnel capabilities is enabled by AI applications performing analytical and administrative tasks, relieving caregivers’ workload (E8, E10, E11). E7 validates that “our conviction is […] that administrational tasks generate the greatest added value and benefit for doctors and caregivers.” Administrative tasks include the creation of case summaries (use case CA4) or automated de-identification of private health information in electronic health records (use case BR2) [ 54 ]. E8 says that resource optimization enables “more time for direct contact with patients.”
Knowledge discovery
Knowledge discovery follows the business objectives that increase perception and access to novel and previously unrevealed information. AI applications might synthesize and contextualize medical knowledge to create uniform or equalized semantics of information (E5, E11). This semantics enables a translation of knowledge for specific users.
Detection of similarities is enabled by AI applications identifying entities with similar features. AI applications can screen complex and nonlinear databases to identify reoccurring patterns without any a priori understanding of the data (E3). These similarities generate valuable knowledge, which can be applied to enhance scientific research processes such as drug development (use case BR1). In drug development, AI applications can facilitate ligand-based screening to detect new active molecules based on similarities compared with already existing molecular properties. This increases the effectiveness of drug design and reduces risks in clinical trials [ 6 ].
Exploration of new correlations is facilitated by AI applications identifying relationships in data. In diagnostics, AI applications can analyze facial photographs to accurately identify genotype–phenotype correlations and, thus, increase the detection rate of rare diseases (use case DD7). E8 states the potential of AI applications in the field of knowledge discovery: “Well, if you are researching in any medical area, then everybody aims to understand and describe phenomena because science always demands a certain causation.” However, it is crucial to develop transparent and intelligible inferences that are comprehensible for HC professionals and researchers. Exploring new correlations improves diagnoses of rare diseases and ensures earlier treatment.
After describing each business objective and value proposition, we summarize the AI use cases’ contributions to the value propositions in Table 3 .
By revealing 15 business objectives that translate into six value propositions, we contribute to the academic discourse on the value creation of AI (e.g. [ 81 ] and provide prescriptive knowledge on AI applications' value propositions in the HC domain. Our discourse also emphasizes that our findings are not only relevant to the field of value creation research but can also be helpful for adoption research. The value propositions we have identified can be a good starting point to accelerate the adoption of AI in HC, as the understanding of potential value propositions that we foster could mitigate some of the current obstacles to the adoption of AI applications in HC. For example, our findings may help to mitigate the obstacle “added value”, which is presented in the study by Hennrich et al.38 [ 38 ] as users’ concerns that AI might create more burden than benefits.
Further, we deliver valuable implications for practice and provide a comprehensive picture of how organizations in the context of HC can achieve business value with AI applications from a managerial level, which has been missing until now. We guide HC organizations in evaluating their AI applications or those of the competition to assess AI investment decisions and align their AI application portfolio toward an overarching strategy. These results will foster the adoption of AI applications as HC organizations can now understand how they can unfold AI applications’ capabilities into business value. In case a hospital’s major strategy is to reduce patient risks due to limited personal capacities, it might be beneficial for them to invest in AI applications that reduce side effects by calculating medication dosages (use case T2). If an HC organization currently faces issues with overcrowded emergency rooms, the HC organization might acquire AI applications that increase information delivery and help patients decide if and when they should visit the hospital (use case T3) to increase patients’ self-management and, in turn, improve triage. Besides, our findings also offer valuable insights for AI developers. Addressing issues such as transparency and the alignment of AI applications with the needs of HC professionals is crucial. Adapting AI solutions to the specific requirements of the HC sector ensures responsible integration and thus the realization of the expected values.
A closer look at the current challenges in the HC sector reveals that new solutions to mitigate them and improve value creation are needed. Given that a nurse, for example, dedicates a substantial 25% of their working hours to administrative tasks [ 17 ], the rationale behind the respondents’ (E7) recognition of “the greatest added value” in utilizing AI applications for administrative purposes becomes evident. The potential of AI applications in streamlining administrative tasks lies in creating additional time for meaningful patient interactions. Acknowledging the significant impact of the doctor-patient interpersonal relationship on both the patient’s well-being and the processes of diagnosis and healing, as elucidated by Buck et al. [ 82 ] in their interview study, the physicians interviewed emphasized that the mere presence of the doctor in the same room often alleviates the patient’s problems. Consequently, it becomes apparent that the intangible value of AI applications plays a crucial role in the context of HC and is an important factor in the investment decision as to where an AI application should be deployed.
The interviews also indicate that the special context of the HC sector leads to concerns regarding the use of AI applications. For example, one interviewee emphasized a fundamental characteristic of medical staff by pointing out that physicians have a natural desire to understand all phenomena (E8). AI applications, however, are currently struggling with the challenge of transparency. This challenge is described by the so-called black box problem, a phenomenon that makes it impossible to decipher the underlying algorithms that lead to a particular recommendation [ 37 ]. The lack of transparency and the resulting lack of intervention options for medical staff can lead to incorrect decisions by the AI application, which may cause considerable damage. Aware of these risks, physicians are currently struggling with trust issues in AI applications [ 72 ]. The numerous opportunities for value creation through AI applications in HC are offset by the significant risk of causing considerable harm to patients if the technology is not yet fully mature. Ultimately, it remains essential to keep in mind that there are many ethical questions to be answered [ 83 ], and AI applications are still facing many obstacles [ 38 ] that must be overcome in order to realize the expected values and avoid serious harm. One important first step in mitigating the obstacles is disseminating the concerns and risks to relevant stakeholders, emphasizing the urgency for collaborative scientific and public monitoring efforts [ 84 ]. However, keeping these obstacles in mind, by providing prescriptive knowledge, we enhance the understanding of AI’s value creation paths in the HC industry and thus help to drive AI integration forward. For example, looking at the value proposition risk reduced patient care , we demonstrate that this value proposition is determined by four business objectives: precise decision support , detection of misconduct, reduction of side effects, and reduction of invasiveness . Similarly, the AI application’s capability to analyze data more accurately in diagnosis (use case DD1) enables the business objective precise decision support , thereby reducing risks in patient care. Another mechanism can be seen, for example, considering the business objective task execution , which leads to the value proposition process acceleration . The ability of AI applications to rapidly analyze large amounts of data and recognize patterns in biomedical research (use case BR1) allows a faster drug development process.
Further research
By investigating the value creation mechanism of AI applications for HC organizations, we not only make an important contribution to research and practice but also create a valuable foundation for future studies. While we have systematically identified the relations between the business objectives and value propositions, further research is needed to investigate how the business objectives themselves are determined. While the examination of AI capabilities was not the primary research focus, we found first evidence in the use cases that indicates AI technology’s unique capabilities (e.g., to make diagnoses accurate, faster, and more objective) that foster one or several business objectives (e.g., rapid task execution, precise decision support) and unlock one or several value propositions (e.g., Risk-reduced patient care, process acceleration ). In subsequent research, we aim to integrate these into the value creation mechanism by identifying which specific AI capabilities drive business objectives, thereby advancing the understanding of how AI applications in HC create value propositions.
Limitations
This study is subject to certain limitations of methodological and conceptual nature. First, while our methodological approach covers an in-depth analysis of 21 AI use cases, extending the sample of AI use cases would foster the generalizability of the results. This is especially important regarding the latest developments on generative AI and its newcoming use cases. However, our results demonstrate that these AI use cases already provide rich information to derive 15 business objectives, which translate into six value propositions. Second, while many papers assume the potential of AI applications to create value propositions, only a few papers explicitly focus on the value creation and capture mechanisms. To compensate for this paucity of appropriate papers, we used 11 expert interviews to enrich and evaluate the results. Besides, these interviews ensured the practical relevance and reliability of the derived results. Third, we acknowledge limitations of conceptual nature. Our study predominantly takes an optimistic perspective on AI applications in medicine. While we discuss the potential benefits and value propositions in detail, it is important to emphasize that there are still significant barriers and risks currently associated with AI applications that need to be addressed before the identified values can be realized. Furthermore, our investigation is limited because we derive the expected value of AI applications without having extensive real-world use cases to evaluate. It is important to emphasize that our findings are preliminary, and critical reassessment will be essential as the broader implementation of AI applications in medical practice progresses. These limitations emphasize the need for ongoing research and monitoring to understand the true value of AI applications in HC fully.
Conclusions
This study aimed to investigate how AI applications can create value for HC organizations. After elaborating on a diverse and comprehensive set of AI use cases, we are confident that AI applications can create value by making HC, among others, more precise, individualized, self-determined, faster, resource-optimized, and data insight-driven. Especially with regard to the mounting challenges of the industry, such as the aging population and the resulting increase in HC professionals’ workloads, the integration of AI applications and the expected benefits have become more critical than ever. Based on the systematic literature review and expert interviews, we derived 15 business objectives that translate into the following six value propositions that describe how HC organizations can capture the value of AI applications: risk-reduced patient care, advanced patient care, self-management, process acceleration, resource optimization, and knowledge discovery .
By presenting and discussing our results, we enhance the understanding of how HC organizations can unlock AI applications’ value proposition. We provide HC organizations with valuable insights to help them strategically assess their AI applications as well as those deployed by competitors at a management level. Our goal is to facilitate informed decision-making regarding AI investments and enable HC organizations to align their AI application portfolios with a comprehensive and overarching strategy. However, even if various value proposition-creating scenarios exist, AI applications are not yet fully mature in every area or ready for widespread use. Ultimately, it remains essential to take a critical look at which AI applications can be used for which task at which point in time to achieve the promised value. Nonetheless, we are confident that we can shed more light on the value proposition-capturing mechanism and, therefore, support AI application adoption in HC.
Availability of data and materials
The datasets analyzed during the current study are available from the corresponding author on reasonable request.
Abbreviations
Artificial Intelligence
Machine Learning
Fogel AL, Kvedar JC. Artificial intelligence powers digital medicine. NPJ Digit Med. 2018;1:5.
Article PubMed PubMed Central Google Scholar
Rai A, Constantinides P, Sarker S. Next-Generation Digital Platforms: Toward Human–AI Hybrid. Manag Inf Syst Q. 2019;43(1):iii–ix.
Google Scholar
Russell S, Norvig P. Artificial Intelligence: A Modern Approach. Pearson Education Limited; 2016.
He J, Baxter SL, Xu J, Xu J, Zhou X, Zhang K. The practical implementation of artificial intelligence technologies in medicine. Nat Med. 2019;25(1):30–6.
Article CAS PubMed PubMed Central Google Scholar
Hosny A, Parmar C, Quackenbush J, Schwartz LH, Aerts HJWL. Artificial intelligence in radiology. Nat Rev Cancer. 2018;18(8):500–10.
Kadurin A, Aliper A, Kazennov A, Mamoshina P, Vanhaelen Q, Khrabrov K, et al. The cornucopia of meaningful leads: Applying deep adversarial autoencoders for new molecule development in oncology. Oncotarget. 2017;8(7):10883–90.
Article PubMed Google Scholar
Rezazade Mehrizi MH, van Ooijen P, Homan M. Applications of artificial intelligence (AI) in diagnostic radiology: a technography study. Eur Radiol. 2020;31:1805–11.
Dankwa-Mullan I, Rivo M, Sepulveda M, Park Y, Snowdon J, Rhee K. Transforming Diabetes Care Through Artificial Intelligence: The Future Is Here. Popul Health Manag. 2019;22(3):229–42.
Bohr A, Memarzadeh K. The rise of artificial intelligence in healthcare applications. In: Artificial Intelligence in Healthcare. Elsevier; 2020. p. 25–60.
Yu K-H, Beam AL, Kohane IS. Artificial Intelligence in Healthcare. Nat Biomed Eng. 2018;2(10):719–31.
Jiang F, Jiang Y, Zhi H, Dong Y, Li H, Ma S, et al. Artificial intelligence in healthcare: past, present and future. Stroke Vasc Neurol. 2017;2(4):230–43.
Gilvary C, Madhukar N, Elkhader J, Elemento O. The Missing Pieces of Artificial Intelligence in Medicine. Trends Pharmacol Sci. 2019;40(8):555–64.
Article CAS PubMed Google Scholar
Fernández E. Innovation in Healthcare: Harnessing New Technologies. Journal of the Midwest Association for Information Systems 2017; (2). Available from: http://aisel.aisnet.org/jmwais/vol2017/iss2/8 .
Topol EJ. Deep medicine: How artificial intelligence can make healthcare human again. 1st ed. New York: Basic Books; 2019.
Ngiam KY, Khor IW. Big data and machine learning algorithms for health-care delivery. Lancet Oncol. 2019;20(5):e262–73.
Plastino E, Purdy M. Game changing value from artificial intelligence: eight strategies. Strategy Leadership. 2018;46(1):16–22.
Article Google Scholar
Davenport T, Kalakota R. The potential for artificial intelligence in healthcare. Future Healthcare J. 2019;6(2):94–8.
Meskó B, Görög M. A short guide for medical professionals in the era of artificial intelligence. NPJ Digit Med. 2020;3:126.
Statista. What is the stage of AI adoption in your organization?; 2021. Available from: https://www.statista.com/statistics/1225955/stage-of-ai-adoption-in-healthcare-worldwide/ . Cited 2024 Feb. 8
Garbuio M, Lin N. Artificial Intelligence as a Growth Engine for Health Care Startups: Emerging Business Models. Calif Manage Rev. 2019;61(2):59–83.
Väänänen A, Haataja K, Vehviläinen-Julkunen K, Toivanen P. AI in healthcare A narrative review. F1000Res. 2021;10:6.
Kim H-W, Chan HC, Gupta S. Value-based Adoption of Mobile Internet: An empirical investigation. Decis Support Syst. 2007;43(1):111–26.
Lin TC, Wu S, Hsu JSC, Chou YC. The integration of value-based adoption and expectation–confirmation models An example of IPTV continuance intention. Decision Support Systems. 2012;54(1):63–75.
Jordan MI, Mitchell TM. Machine learning: Trends, perspectives, and prospects. Science. 2015;349(6245):255–60.
LeCun Y, Bengio Y, Hinton G. Deep learning. Nature. 2015;521(7553):436–44.
Hamet P, Tremblay J. Artificial intelligence in medicine. Metab Clin Exp. 2017;69S:S36–40.
Lidströmer N, Aresu F, Ashrafian H. Basic Concepts of Artificial Intelligence Primed for Clinicians In Artificial Intelligence in Medicine. Cham: Springer; 2022. p. 3–20.
Choi RY, Coyner AS, Kalpathy-Cramer J, Chiang MF, Campbell JP. Introduction to machine learning, neural networks, and deep learning. Transl Vis Sci Technol. 2020;9(2):1–12.
Lorenz U. Reinforcement Learning: Aktuelle Ansätze verstehen - mit Beispielen in Java und Greenfoot. Berlin, Heidelberg: Springer Berlin Heidelberg; 2020.
Kunduru AR. Artificial intelligence advantages in cloud fintech application security. Central Asian J Mathematical Theory Comp Sci. 2023;4(8):48–53.
Zhai X, Chu X, Chai CS, Jong MSY, Istenic A, Spector M, et al. A Review of Artificial Intelligence (AI) in Education from 2010 to 2020. Complexity. 2021;2021:1–18.
Zhao Y, Li T, Zhang X, Zhang C. Artificial intelligence-based fault detection and diagnosis methods for building energy systems: Advantages, challenges and the future. Renew Sustain Energy Rev. 2019;109:85–101.
Secinaro S, Calandra D, Secinaro A, Muthurangu V, Biancone P. The role of artificial intelligence in healthcare: a structured literature review. BMC Med Inform Decis Mak. 2021;21(1):125.
Bellini V, Guzzon M, Bigliardi B, Mordonini M, Filippelli S, Bignami E. Artificial intelligence a new tool in operating room management role of machine learning models in operating room optimization. J Med Syst. 2019;44(1):20.
Komorowski M, Celi LA, Badawi O, Gordon AC, Faisal AA. The Artificial Intelligence Clinician learns optimal treatment strategies for sepsis in intensive care. Nat Med. 2018;24(11):1716–20.
Roland T. Motion Artifact Suppression for Insulated EMG to Control Myoelectric Prostheses. Sensors (Basel). 2020;20(4):1031.
Amann J, Blasimme A, Vayena E, Frey D, Madai VI. Explainability for artificial intelligence in healthcare: a multidisciplinary perspective. BMC Med Inform Decis Mak. 2020;20(1):310.
Hennrich J, Fuhrmann H, Eymann T. Accelerating the Adoption of Artificial Intelligence Technologies in Radiology: A Comprehensive Overview on Current Obstacles. Proceedings of the 57th Hawaii International Conference on System Sciences 2024.
Asan O, Bayrak AE, Choudhury A. Artificial Intelligence and Human Trust in Healthcare: Focus on Clinicians. J Med Internet Res. 2020;22(6):e15154.
Schryen G. Revisiting IS business value research: what we already know, what we still need to know, and how we can get there. Eur J Inf Syst. 2013;22(2):139–69.
Haddad P, Wickramasinghe N. Conceptualizing Business Value of IT in Healthcare to Design Sustainable e-Health Solutions. Proceedings of Americas Conference on Information Systems 2014.
Strong DM, Volkoff O, Johnson SA, Pelletier LR, Tulu B, Bar-On I, et al. A Theory of Organization-EHR Affordance Actualization. J Assoc Inf Syst. 2014;15(2):53–85.
Hofmann P, Oesterle S, Rust P, Urbach N. Machine Learning Approaches Along the Radiology Value Chain - Rethinking Value Propositions. Proceedings of the European Conference on Information Systems 2019.
Badakhshan P, Wurm B, Grisold T, Geyer-Klingeberg J, Mendling J, Vom Brocke J. Creating business value with process mining. J Strateg Inf Syst. 2022;31(4):101745.
Webster J, Watson RT. Analyzing the Past to Prepare for the Future Writing a Literature Review. MIS Quarterly. 2002;26(2):xiii–xxiii.
Wolfswinkel JF, Furtmueller E, Wilderom CPM. Using Grounded Theory as a Method for Rigorously Reviewing Literature. Eur J Inf Syst. 2013;22(1):45–55.
Siggelkow N. Persuasion With Case Studies. AMJ. 2007;50(1):20–4.
Lakhani P, Prater AB, Hutson RK, Andriole KP, Dreyer KJ, Morey J, et al. Machine Learning in Radiology: Applications Beyond Image Interpretation. J Am Coll Radiol. 2018;15(2):350–9.
Low S-K, Zembutsu H, Nakamura Y. Breast cancer: The translation of big genomic data to cancer precision medicine. Cancer Sci. 2018;109(3):497–506.
Altay EV, Alatas B. Association analysis of Parkinson disease with vocal change characteristics using multi-objective metaheuristic optimization. Med Hypotheses. 2020;141:109722.
Abbasi B, Goldenholz DM. Machine learning applications in epilepsy. Epilepsia. 2019;60(10):2037–47.
Qin B, Quan Q, Wu J, Liang L, Li D. Diagnostic performance of artificial intelligence to detect genetic diseases with facial phenotypes: A protocol for systematic review and meta analysis. Medicine (Baltimore). 2020;99(27):e20989.
Zhavoronkov A, Mamoshina P, Vanhaelen Q, Scheibye-Knudsen M, Moskalev A, Aliper A. Artificial intelligence for aging and longevity research: Recent advances and perspectives. Ageing Res Rev. 2019;49:49–66.
Meystre SM, Friedlin FJ, South BR, Shen S, Samore MH. Automatic de-identification of textual documents in the electronic health record - a review of recent research. BMC Med Res Methodol. 2010;10(70):1–6.
Rhine CL, Neil C, Glidden DT, Cygan KJ, Fredericks AM, Wang J, et al. Future directions for high-throughput splicing assays in precision medicine. Hum Mutat. 2019;40(9):1225–34.
Berlyand Y, Raja AS, Dorner SC, Prabhakar AM, Sonis JD, Gottumukkala RV, et al. How artificial intelligence could transform emergency department operations. Am J Emerg Med. 2018;36(8):1515–7.
Kwon J-M, Kim K-H, Jeon K-H, Park J. Deep learning for predicting in-hospital mortality among heart disease patients based on echocardiography. Echocardiography. 2019;36(2):213–8.
Yang M, Li C, Shen Y, Wu Q, Zhao Z, Chen X. Hierarchical Human-Like Deep Neural Networks for Abstractive Text Summarization. IEEE Trans Neural Netw Learn Syst. 2021;32(6):2744–57.
Hernandez JPT. Network diffusion and technology acceptance of a nurse chatbot for chronic disease self-management support a theoretical perspective. J Med Invest. 2019;66(1.2):24–30.
Bhandari M, Zeffiro T, Reddiboina M. Artificial Intelligence and Robotic Surgery: Current Perspective and Future Directions. Curr Opin Urol. 2020;30(1):48–54.
Padoy N. Machine and deep learning for workflow recognition during surgery. Minim Invasive Ther Allied Technol. 2019;28(2):82–90.
Shepherd M, Abidi SSR, Gao Q, Chen Z, Qi Q, Finley GA. Information Systems and Health Care IX: Accessing Tacit Knowledge and Linking It to the Peer-Reviewed Literature. CAIS 2006; 17.
Wilson EV, Wang W, Sheetz SD. Underpinning a Guiding Theory of Patient-Centered E-Health. CAIS. 2014;34(1):16.
Levy Y, Ellis TJ. A systems approach to conduct an effective literature review in support of information systems research. Informing Sci J. 2006;9:181–211.
Corbin JM, Strauss AL. Basics of qualitative research: Techniques and procedures for developing grounded theory. Fourth edition. Thousand Oaks, Kalifornien: SAGE; 2015.
Glaser BG, Strauss A. Discovery of grounded theory: Strategies for qualitative research. Routledge; 1967.
Feldman S, Horan T. The dynamics of information collaboration: a case study of blended IT value propositions for health information exchange in disability determination. J Assoc Inf Syst. 2011;12(2):189–207.
Schultze U, Avital M. Designing interviews to generate rich data for information systems research. Inf Organ. 2011;21(1):1–16.
Myers MD, Newman M. The qualitative interview in IS research: examining the craft. Inf Organ. 2007;17(1):2–26.
Bhattacherjee A. Social Science Research: Principles, Methods, and Practices. Textbooks Collection 2012; 3.
Moulaei K, Sheikhtaheri A, Fatehi F, Shanbehzadeh M, Bahaadinbeigy K. Patients’ perspectives and preferences toward telemedicine versus in-person visits: a mixed-methods study on 1226 patients. BMC Med Inform Decis Mak. 2023;23(1):261.
Buck C, Hennrich J, Kauffmann A-L. Artificial Intelligence in Radiology – A Qualitative Study on Imaging Specialists’ Perspectives. Proceedings of the 42nd International Conference on Information Systems 2021.
Degnan AJ, Ghobadi EH, Hardy P, Krupinski E, Scali EP, Stratchko L, et al. Perceptual and interpretive error in diagnostic radiology-causes and potential solutions. Acad Radiol. 2019;26(6):833–45.
Pesapane F, Codari M, Sardanelli F. Artificial intelligence in medical imaging: threat or opportunity? Radiologists again at the forefront of innovation in medicine. Eur Radiol Exp. 2018;2(1):35.
Schinkel M, Paranjape K, Nannan Panday RS, Skyttberg N, Nanayakkara PWB. Clinical applications of artificial intelligence in sepsis: A narrative review. Comput Biol Med. 2019;115:103488.
Shamim Nemati, Mohammad Ghassemi, Gari Clifford. Optimal medication dosing from suboptimal clinical examples: A deep reinforcement learning approach. International Conference of the IEEE Engineering in Medicine and Biology Society 2016. Available from: http://ieeexplore.ieee.org/servlet/opac?punumber=7580725 .
Padoy N, Hager GD. Human-Machine Collaborative Surgery Using Learned Methods. Proceedings of IEEE International Conference on Robotics and Automation 2011:5285–92.
Nadarzynski T, Miles O, Cowie A, Ridge D. Acceptability of artificial intelligence (AI)-led chatbot services in healthcare: A mixed-methods study. Digit Health. 2019;5:2055207619871808.
PubMed PubMed Central Google Scholar
Trevitt S, Simpson S, Wood A. Artificial Pancreas device systems for the closed-loop control of type 1 diabetes: what systems are in development? J Diabetes Sci Technol. 2016;10(3):714–23.
Luo L, Zhang F, Yao Y, Gong R, Fu M, Xiao J. Machine learning for identification of surgeries with high risks of cancellation. Health Informatics J. 2018;26(1):141–55.
Shollo A, Hopf K, Thiess T, Müller O. Shifting ML value creation mechanisms: a process model of ML value creation. J Strateg Inf Syst. 2022;31(3):101734.
Buck C, Doctor E, Hennrich J, Jöhnk J, Eymann T. General Practitioners’ Attitudes Toward Artificial Intelligence-Enabled Systems: Interview Study. J Med Internet Res. 2022;24(1):e28916.
Bennett SJ. Transmuting values in artificial intelligence: investigating the motivations and contextual constraints shaping the ethics of artificial intelligence practitioners; 2023.
Baumgartner R, Arora P, Bath C, Burljaev D, Ciereszko K, Custers B, et al. Fair and equitable AI in biomedical research and healthcare: Social science perspectives. Artif Intell Med. 2023;144:102658.
Download references
Acknowledgements
The authors thank all physicians who participated in this study.
Open Access funding enabled and organized by Projekt DEAL.
Author information
Authors and affiliations.
FIM Research Institute for Information Management, University of Bayreuth, Branch Business and Information Systems Engineering of the Fraunhofer FIT, Wittelsbacherring 10, 95444, Bayreuth, Germany
Jasmin Hennrich, Peter Hofmann & Nils Urbach
University St. Gallen, Dufourstrasse 50, 9000, St. Gallen, Switzerland
Faculty Business and Law, Frankfurt University of Applied Sciences, Nibelungenplatz 1, 60318, Frankfurt Am Main, Germany
Nils Urbach
appliedAI Initiative GmbH, August-Everding-Straße 25, 81671, Munich, Germany
Peter Hofmann
You can also search for this author in PubMed Google Scholar
Contributions
JH initially composed the introduction, background, and discussion sections; PH contributed to the analysis design, supported data analysis, and critically reviewed the manuscript; ER conducted data collection and analysis and produced the initial draft of the methodology section; NU edited and critically reviewed the manuscript and provided guidance. All authors read and approved the final manuscript.
Corresponding author
Correspondence to Jasmin Hennrich .
Ethics declarations
Ethics approval and consent to participate.
The study was based on original data. The authors confirm that all methods were carried out in accordance with relevant guidelines and regulations and confirm that informed consent was obtained from all participants. Ethics approval was granted by the Ethics Committee of the University of Bayreuth (Application-ID 23–032).
Consent for publication
Not applicable.
Competing interests
The authors declare no competing interests.
Additional information
Publisher’s note.
Springer Nature remains neutral with regard to jurisdictional claims in published maps and institutional affiliations.
Supplementary Information
Supplementary material 1., rights and permissions.
Open Access This article is licensed under a Creative Commons Attribution 4.0 International License, which permits use, sharing, adaptation, distribution and reproduction in any medium or format, as long as you give appropriate credit to the original author(s) and the source, provide a link to the Creative Commons licence, and indicate if changes were made. The images or other third party material in this article are included in the article's Creative Commons licence, unless indicated otherwise in a credit line to the material. If material is not included in the article's Creative Commons licence and your intended use is not permitted by statutory regulation or exceeds the permitted use, you will need to obtain permission directly from the copyright holder. To view a copy of this licence, visit http://creativecommons.org/licenses/by/4.0/ . The Creative Commons Public Domain Dedication waiver ( http://creativecommons.org/publicdomain/zero/1.0/ ) applies to the data made available in this article, unless otherwise stated in a credit line to the data.
Reprints and permissions
About this article
Cite this article.
Hennrich, J., Ritz, E., Hofmann, P. et al. Capturing artificial intelligence applications’ value proposition in healthcare – a qualitative research study. BMC Health Serv Res 24 , 420 (2024). https://doi.org/10.1186/s12913-024-10894-4
Download citation
Received : 26 October 2023
Accepted : 25 March 2024
Published : 03 April 2024
DOI : https://doi.org/10.1186/s12913-024-10894-4
Share this article
Anyone you share the following link with will be able to read this content:
Sorry, a shareable link is not currently available for this article.
Provided by the Springer Nature SharedIt content-sharing initiative
- Artificial intelligence
- Value propositions
- Business objectives
BMC Health Services Research
ISSN: 1472-6963
- General enquiries: [email protected]

An official website of the United States government
The .gov means it's official. Federal government websites often end in .gov or .mil. Before sharing sensitive information, make sure you're on a federal government site.
The site is secure. The https:// ensures that you are connecting to the official website and that any information you provide is encrypted and transmitted securely.
- Publications
- Account settings
- Browse Titles
NCBI Bookshelf. A service of the National Library of Medicine, National Institutes of Health.
Davidson D, Ellis Paine A, Glasby J, et al. Analysis of the profile, characteristics, patient experience and community value of community hospitals: a multimethod study. Southampton (UK): NIHR Journals Library; 2019 Jan. (Health Services and Delivery Research, No. 7.1.)

Analysis of the profile, characteristics, patient experience and community value of community hospitals: a multimethod study.
Chapter 2 research objectives, questions and methodology.
In the light of the unfolding policy context and gaps within the existing literature outlined in Chapter 1 , and informed by conversations with key stakeholders (see Patient and public involvement ), this study aimed to provide a comprehensive analysis of the profile, characteristics, patient and carer experience and community engagement and value of community hospitals in contrasting local contexts. The specific objectives were to:
- construct a national database and develop a typology of community hospitals
- explore and understand the nature and extent of patients’ and carers’ experiences of community hospital care and services
- investigate the value of the interdependent relationship between community hospitals and their communities through in-depth case studies of community value (qualitative study) and analysis of Charity Commission data (quantitative study).
In meeting these aims and objectives, the study addressed three overarching research questions (each with an associated set of more specific subquestions as summarised in Table 1 ):
Research questions and objectives
- What is a community hospital? In addressing this question, we drew on existing definitions and conceptualisations of ‘community hospitals’ as outlined in Chapter 1 , Research on community hospitals . Although our emphasis here was primarily empirical and descriptive, we were nevertheless guided by, and sought to contribute to, theoretical debates on definitions of community hospitals and their place within wider health and care systems, drawing on concepts of rural health care, chronic disease and complex care burden, integrated care and clinical leadership.
- What are patients’ (and carers’) experiences of community hospitals? This element of the study was designed to contribute to the conceptualisation of the distinctive elements of community hospitals as understood through the ‘lived experiences’ of patients, rather than just satisfaction ratings. Here, we were influenced by prior analysis of the functional, technical and relational components of patient experience (e.g. environment and facilities, delivery of care, staff) alongside a more theoretical interest in the interpersonal, psychological and social dimensions of patient experience. Very early on in our study, through conversations with patient and public involvement (PPI) stakeholders, we recognised the importance of exploring and understanding the experience not only of patients but also of family carers, and hence we extended our initial question to include both patients’ and carers’ experiences.
- What does the community do for its community hospital, and what does the community hospital do for its community? In addressing this question, we drew on notions of voluntarism and participation and brought together thinking from the separate bodies of literature on volunteering, philanthropy and co-production. This led us to question not just the level of voluntary support for community hospitals but also the different forms it took, how this varies between and within communities, how it is encouraged, organised and managed, and what difference it makes (outcomes). We also drew on notions of social value, including existing typologies, that encouraged us to question different forms of value (e.g. economic, social, human, symbolic) and different stakeholder groups (e.g. staff, patients, communities).
Given the diversity of the questions, we do not set out to provide an over-riding hypothesis or unified theoretical framework for the study as a whole. Instead, these concepts, frameworks and debates served as ‘sensitising categories’, shaping our approach to study design as well as data collection and analysis. 71 We return to these in Chapter 8 and augment them with new concepts that emerged from our analysis.
In addressing these diverse questions, we adopted a multimethod approach with a convergent design. Quantitative methods were employed to provide breadth of understanding relating to the questions concerning ‘what’, ‘where’ and ‘how much’, whereas qualitative methods provided depth of understanding, particularly in relation to questions of ‘how’, ‘why’ and ‘to what effect’.
The research was conducted in three distinct (although temporally overlapping) phases, each with a number of different associated elements and research methods: (1) mapping (database construction and analysis through data set reconciliation and verification), (2) qualitative case studies (semistructured interviews, discovery interviews, focus groups) and (3) quantitative analysis of charity commission data. Table 1 summarises the study objectives, questions and research methods. Each of the three phases of research are discussed in turn through the following sections of this chapter, before the final sections discuss data integration, PPI and ethics.
- Phase 1: mapping and profiling community hospitals
Phase 1 of the research involved a national mapping exercise to address the first study question ‘what is a community hospital?’. It aimed to map the number and location of all hospitals in England to then provide a profile and definition of community hospitals. A database of characteristics would enable the profiling of community hospitals, inform a typology and support a sampling strategy for subsequent case studies. Data were collected from all four UK countries but, in accordance with the brief of the study, this report focuses on England. Reference is made to Scotland’s data as they were important in developing the methodology. The structure of the mapping comprised five elements:
- literature review – constructing a working definition: (see Chapter 1 )
- data set reconciliation – building a new database from multiple data sets
- database analysis – developing an initial classification of community hospitals with beds
- rapid telephone enquiry – refining the classification
- verification – checking and refining the database through internet searches.
The flow of activities is depicted in Figure 1 .
Structure of the national mapping exercise.
Literature review: constructing a working definition
We developed a working definition of a community hospital as drawn from the literature (and as outlined in Chapter 1 ):
- A hospital with < 100 beds serving a local population of up to 100,000 and providing direct access to GPs and local community staff.
- Typically GP led, or nurse led with medical support from local GPs.
- Services provided are likely to include inpatient care for older people, rehabilitation and maternity services, outpatient clinics and day care as well as minor injury and illness units, diagnostics and day surgery. The hospital may also be a base for the provision of outreach services by MDTs.
- Will not have a 24-hour A&E nor provide complex surgery. In addition, a specialist hospital (e.g. a children’s hospital, a hospice or a specialist mental health or learning disability hospital) would not be classified as a community hospital.
The initial enquiry was framed around a ‘classic’ community hospital. The term was drawn directly from the Community Hospital Association 2008 classification, 72 describing classic community hospitals as ‘local community hospitals with inpatient facilities’ (i.e. with beds) and as distinct from community care resource centres (without beds), community care homes (integrated health and social care campus) or rehabilitation units. Although the term ‘classic’ was initially helpful in setting the boundaries of the study, it presented ongoing problems, such as whether it described all community hospitals with beds or a subset within that. Throughout the study, therefore, we have adopted the term ‘community hospital’ and omitted the adjective ‘classic’. Our focus, however, has remained on community hospitals with beds.
Data reconciliation: building a new database from multiple data sets
There was no up-to-date comprehensive database of community hospitals in England. The NHS Benchmarking Network [URL: www.nhsbenchmarking.nhs.uk (accessed 8 October 2018)] membership database was not comprehensive and could not be used to populate our hospital-level database because the data were anonymised. For this reason, one of our first tasks was to compile a new database, by bringing together existing health-care data sets, each of which provided different fields of information needed to test our working definition and to map and profile community hospitals.
Two types of data sets were collected. Centrally available data sets formed the starting point for the mapping study, providing codified data (see Appendix 1 ). As none of these centrally available data sets provided a comprehensive picture, it was necessary to supplement them through extensive internet searching and by talking to people in the field, as well as drawing on the expertise of research team members.
The base year for major data sets was 2012/13. Data were difficult to access, not comprehensive and spread across a greater number of sources. Four data sets were used:
- Community Hospital Association databases of community hospitals (one from 2008 and another from 2013)
- Patient-Led Assessments of the Care Environment (PLACE) 2013 [replacing the former Patient Environment Action Team programme]
- Estates database – Estates Returns Information Collection (ERIC) 2012
- NHS Digital activity by site of treatment 2012/13.
Barriers to obtaining site and activity data included (1) specific difficulties in the period 2012/13 when primary care trusts (PCTs) were being disbanded and clinical commissioning groups (CCGs) were being established (with effect from 31 March 2013) and (2) processes and caution in NHS Digital associated with releasing patient-sensitive data (even though we had not requested patient-based data). Quality problems were associated with the ‘location of treatment’ code, which was central to our enquiry identifying community hospitals but did not appear to be well used in England, leading to examples of missing data and inconsistent labels (described under reconciliation and duplication). The code also lacked stability as it changed with each new NHS reconfiguration in England.
The core data set for England, supplied by NHS Digital, was a list of all hospitals in England, based on ‘site of treatment code.’ Figure 2 shows the relationship between national data sets.
The relationship between four England data sets. CHA, Community Hospitals Associations.
The new database, populated through our reconciliation of these various data sets, provided a census of community hospitals at 2012/13, which was updated to August 2015 (e.g. when a hospital closed and then redeveloped, formed a new hospital replacing two old community hospitals, closed beds on a temporary basis and changed its name).
Database analysis: developing an initial classification of community hospitals with beds
Although the focus of this report is on England, it is important to mention our work on mapping community hospitals in Scotland, as this was instrumental in developing our approach to classifying data for England. Data sets on community hospitals in Scotland [Information Services Division (ISD) and government community hospital data sets: community hospital, general hospital, long-stay/psychiatric hospital, small long-stay hospital] were both more accessible and more comprehensive, lending themselves to early analysis (see Appendix 2 ).
An initial classification of hospitals in England was developed, informed by categories set out by Estates (community hospital, general acute hospital, long-stay hospital, multiservice hospital, short-term non-acute hospital, specialist hospital, support facility, treatment centre) and PLACE (acute/specialist, community, mental health only, mixed acute and mental health/mental health, treatment centre). It was combined with specialty classifications based mainly on NHS Digital inpatient activity data and developed further through analysis of Community Hospitals Association (CHA) data and discussions within the study team ( Table 2 ).
Classification of all hospitals in England
Rapid telephone enquiry: refining the classification
Analysis of the Scotland data suggested that the code ‘GP specialty’ was a defining feature of community hospitals, but early analysis of the England data showed that this was less transferable. If we relied on GP specialty coding alone, many known community hospitals would be excluded from our database: not all community hospital inpatient beds in England were coded to GPs.
A short piece of empirical data collection was undertaken to understand the link between the specialty codes and practice and to test the working definition (based on the literature and on the Scottish data) that community hospitals were predominantly GP led. A telephone questionnaire was designed by the study team (see Appendix 3 ) and piloted through the CHA.
Seven hospitals from five specialty category codes (≥ 80% GP, < 80% GP and mixed specialties, general medicine, geriatric medicine, geriatric mixed specialties) were randomly selected. The test sample of 35 was reduced by four as a result of closure or conversion to nursing homes. The research team called the hospitals to gain contact details of the matron or ward manager ( n = 20; the small sample size highlighting the difficulty of identifying leadership, especially when the community hospital is represented by a single ward), e-mailed the questionnaire, conducted telephone interviews with staff to complete the questionnaire (taking 10–20 minutes each), transcribed notes and returned the completed questionnaire to respondents ( n = 12). Analysis of these telephone interviews gave us confidence in the specialty coding, while also confirming the need to be more expansive in our working definitions and categorisations.
Verification: checking and refining the database through internet searches
The mapping enquiry was finalised through five iterations of searching and checking. The CHA consulted its database and membership list (from both 2008 and 2013). A full internet search took place at two points, in February 2015 and August 2015, taking account of hospital closures and changes of function up to 2014/15, with further validation and amendments up to August 2015. By the end of the study, the 2012/13 data set, based on the NHS Digital Spine using ‘site of treatment code’, had been validated through a check of every potential community hospital. A total of 366 sites were examined through web-based and telephone enquiries, including 60 that were not present on the NHS Digital database (see Appendix 4 for the list of community hospitals with beds).
- Phase 2: case studies
In order to explore patient and carer experience of community hospitals and aspects of community engagement and value, we undertook qualitative case studies. Although the initial aim of the case studies was to address the second and third research questions, the findings also enabled new insights into the first study question of ‘what is a community hospital’.
The decision to adopt a comparative case study design 73 across multiple community hospital sites was influenced by three factors. First, given the gaps in the literature highlighted in Chapter 1 , it would be useful to uncover different aspects of the patient experience, community engagement and value of community hospitals and enable the identification and analysis of common themes (looking for similarities, differences and patterns) both within and across cases. 74 – 76 Second, it provides a suitable way of ‘exemplifying’ sites, 77 given the variety of ownership models and locations. Third, it is useful in enabling an examination of ‘complex social phenomena’, 78 and, in particular, the social, functional, interpersonal and psychological factors that shape patient experiences, as well as those that influence community engagement and value. Below, we summarise the approach to case study selection for work packages 2 and 3, before moving on to discuss the research elements used.
Selection of case study sites
In selecting case study sites, we adopted a ‘realist’ approach to sampling, 79 moving back and forth between categories identified from the literature as being important for patient experience and community value and our learning about the characteristics of community hospitals identified from the mapping exercise. In order to reflect the diversity of community hospitals (highlighted in the literature and mapping), we selected cases in contrasting locations with different numbers of beds, ranges of services, ownership/provision and levels of voluntary income and deprivation.
To allow for a particular focus on variations in voluntary support for community hospitals, hinted at through the national mapping exercise and identified as a particular gap in the existing literature, we selected pairs of hospitals across four Clinical Commissioning Group (CCG) areas with contrasting levels of voluntary income but similar levels of deprivation. This would allow for a good comparison within and between cases (e.g. why two community hospitals within one CCG area, with similar levels of deprivation, have contrasting levels of voluntary support, given that previous research has tended to suggest a strong negative correlation between deprivation and voluntary activity).
Using these criteria, we selected eight case studies of hospitals of different sizes, ages and service profiles located across England (although mostly concentrated in the south, reflecting the national pattern of community hospital development; see Figure 7 ) in areas of contrasting levels of deprivation. Six of the buildings were owned by, and their main inpatient service was provided by, the NHS. Two were owned by the NHS but their main inpatient services were provided by a community interest company (CIC). We added a ninth case study, owned by a charity, to increase diversity in terms of ownership/provision (as there were very few examples of independently owned community hospitals, it was not possible to identify a matched pair). Table 3 provides a summary of the nine case studies selected, according to the data that were available from the mapping exercise. Fuller qualitative descriptions are provided in Chapter 4 and Appendix 5 .
Profile of selected case studies
Case study data collection
The case studies involved seven research elements, as summarised in Table 4 . All elements were conducted over five visits to each case study. Across all case study sites and research methods, 241 people participated in the study through interviews and 130 people participated through 22 focus groups; a small number of people who participated in individual interviews also participated in focus groups (see Appendix 6 for full details).
Research elements and focus
Scoping visits were made to each of the case studies in order to build relationships with key stakeholders (primarily matrons and chairpersons of Leagues of Friends), gather background information on the hospitals and local communities, identify potential study participants and collect key documents and data. Documents selected included hospital histories, annual reports, local service information (when available) and media coverage. Reviewing these helped to provide a basic understanding of the cases prior to the main fieldwork visits and added to our profiling of each of the case study hospitals.
We also aimed to gather hospital-level data from patient-reported experience measures (PREMs) 80 and the revised Friends and Family Test (FFT). 81 However, none of the case study community hospitals collected PREMs data, as this had only recently been required of community providers. Although all sites collected FFT scores, we were able to access data for only seven of the nine case studies because, in the remaining two cases, the trust compiled data at trust rather than hospital level and it was not possible to disaggregate the data. Furthermore, the FFT data were not strictly comparable as some scores covered inpatient care only, whereas others covered both inpatient and outpatient care.
Local reference group
We established a local reference group (LRG) in each of our case studies to bring local people together to steer, support and inform the research at the local level. These LRGs comprised key members of hospital staff, the League of Friends, volunteers and local voluntary and community groups, some of whom had also been patients and/or carers. Their role was to help build a picture of the local context to inform subsequent data collection elements, build support for the study within the local community and reflect on emerging findings and their implications for local practice. There were two LRG meetings per case study during the local fieldwork stage: one at the start of the fieldwork period (which focused on mapping the community hospital services and community links) and one at the end (which focused on discussing the emerging findings and their potential implications). The first LRG meeting for CH3 and CH4 was joint (for convenience) but the second meeting was separate. Following completion of the fieldwork and analysis, each LRG received a report of the findings relating to their specific case study (i.e. alongside this national report, we produced nine local reports).
Semistructured interviews with staff, volunteers and community representatives
We conducted semistructured interviews with staff ( n = 89 staff across the nine cases), community stakeholders ( n = 20) and volunteers ( n = 35). Although most of the interviews were with single respondents, some were with two or, very occasionally, three people (depending on respondent preferences). Respondents were selected through purposive sampling 79 guided by the scoping visits, the initial LRG and snowballing. Each of the interviews explored the profile of the hospital and the local context, perceptions of patient and carer experience, and community engagement and value. The emphasis placed on the different sets of questions, however, varied between the groups of respondents (e.g. more time was spent on community engagement and value within the community stakeholder interviews, although we still asked questions relating to hospital profile and perceptions of patient/carer experience). Interviews were nearly all conducted face to face, although a small number were conducted via telephone, at respondent preference. Interviews with staff, volunteers and stakeholders lasted, on average, 60 minutes. All were digitally recorded and later transcribed verbatim.
Discovery interviews with patients
Rather than focusing on satisfaction levels, or other quantifiable measures of experience, the study was concerned with exploring the lived experience of being a patient using community hospital services. Lessons from previous studies show that gathering experiences in the form of stories enhances their power and richness, 36 so we selected an experience-centred interview method 82 that drew on the principles of narrative approaches 83 and, particularly, discovery interviewing. 84 Narrative approaches invite respondents to tell their stories uninterrupted, rather than respond to predetermined questions, giving control to the ‘storyteller’. This approach can elicit richer and more complete accounts than other methods 85 , 86 because reflection enables respondents to contextualise, and connect to, different aspects of their experiences. Discovery interviewing helps to capture patients’ experiences of health care when there may be pathways or clinical interventions central to patient experience. 87 As such, after a general opening question, our interviews focused around a very open question inviting respondents to tell their story of being a patient at the community hospital. We followed this by asking respondents to consider a visual representation we had developed of factors found in previous research to have shaped patient experience, to prompt people’s memories and thoughts (see Appendix 7 for an example of the discovery interview).
Our aim was to interview six patients from each case study. Our final sample across all sites was 60 patients. The small sample size reflected the in-depth nature of the interviews. We sought, as far as possible, to select patients with a mix of demographics (particularly in terms of gender), care pathways (particularly in terms of step up/step down) and services used (inpatient/outpatient). Potential participants were identified by the hospital matron and/or lead clinician and/or service leads. Each was written to by the hospital with a request to participate in the study and was sent an information sheet and an opt-in consent form. Patients who were willing to participate sent their replies directly to the study team. Written consent was provided prior to the commencement of the interview. In line with the Mental Capacity Act 2005 Code of Practice, 88 we made provision for the appointment of consultees when potential respondents lacked the capacity to consent to participation in the study, although this was not utilised.
Although many of our respondents were current inpatients, we also spoke to some inpatients who had been recently discharged and to outpatients from a range of different clinics. Outpatients who agreed to participate tended to be those using services several times a week (e.g. renal patients) or over a longer period of time (e.g. those with chronic conditions), rather than one-off users. Interviews with patients lasted between 30 and 90 minutes, were digitally recorded (in all cases except for two because of respondent preference/requirements) and transcribed verbatim. At the end of the interviews, we asked respondents to complete a short pro forma to gather basic demographic and service information for analysis purposes.
Semistructured interviews with carers
Semistructured interviews were conducted with carers in order to explore their experience of using the community hospital as a carer of an inpatient. Our aim was to interview three carers per case study; in total we spoke to 28 carers across the nine sites. Carers were either related to, or close friends of, patients (either current or recent) at the hospital. In most cases, we interviewed carers of patients who had also been interviewed, but in some cases carers were not directly linked to patients involved in the study (indeed, some carers were reflecting on the experience of caring for a patient who had recently died).
The main focus of the interviews was on the experience of being a carer of someone at the hospital, with our initial question reflecting the narrative approach adopted for patients by asking respondents to tell us their story of using the hospital. In addition, as the respondents were typically local residents, we also asked questions about their perceptions of patient experience, about local support for, and engagement with, the hospital and of value. Interviews with carers lasted, on average, 60 minutes. All were digitally recorded and later transcribed verbatim.
Focus groups
We conducted focus groups with members of MDTs, volunteers and community stakeholders. Although we had anticipated conducting each of the three focus groups in each of the case study sites, this was not always possible owing to practical reasons; for example, in some of the case study sites there were very few volunteers, making it difficult to organise a focus group. We ran focus groups with MDTs in eight of the nine case studies, involving a total of 43 respondents; with volunteers in six of the case studies, involving a total of 33 respondents; and with community stakeholders in eight of the cases, involving 54 respondents. Individual focus group respondents were selected through purposive sampling. We worked with LRGs and other key contacts to identify potential participants, each of whom was written to and asked to participate.
The focus groups complemented the interviews, enabling the inclusion of a wider range of perspectives in the study and, in particular, allowing us to observe the emergence of discussion, consensus and dissonance among groups of participants. They lasted, on average, 90 minutes and were digitally recorded and transcribed in full.
Telephone interviews with managers and commissioners
We conducted telephone interviews to explore the views of senior managers of provider organisations and commissioners of community hospitals. The nine case studies were based in five CCG areas where the main inpatient services were provided by four NHS trusts and one integrated health and social care CIC. Our aim was to interview one respondent from each of the providers and CCGs. In total, we spoke to five provider and four CCG representatives. The interviews explored the strategic context for the community hospitals involved in the study, alongside the perceptions of these senior stakeholders of patient experience and the value of community hospitals. The interviews lasted, on average, 60 minutes and were digitally recorded and later transcribed in full.
Qualitative case study data analysis
We adopted a thematic approach to qualitative data analysis, aided by the use of NVivo 11 (QSR International, Warrington, UK) for data management and exploration. Our approach was both inductive, with themes emerging from the data, and deductive, framed by our research questions and ongoing reading of the literature. Initial themes and codes were developed after three members of the team (AEP, DD and NLM), who collectively had been responsible for the case study data collection, reviewed the transcripts. The emerging themes, codes and associated findings were discussed at wider study team meetings, at the LRG meetings for individual case studies and at annual learning events that brought together participants from across the case studies. A refined coding frame was then tested by the same three members of the research team each coding a sample of transcripts; this led to a further refinement of the codes, while also helping to ensure that each of the researchers was adopting a similar approach.
In this report, we focus in particular on across-case comparisons, highlighting themes that emerged across the case studies, emphasising key points of similarity and difference between the cases, as relevant. In addition, we have produced individual reports for each of the local case study sites that have shared findings from our within-case analysis, as relevant for each individual hospital. Comparative analysis, including of the paired cases, will be developed further in future research articles, in which a focus on more specific aspects of the study will allow more space for presentation of such work.
Throughout the analysis, unique identifiers were used for the transcripts/respondents to help ensure confidentiality and anonymity. Sites were assigned a number (e.g. CH1) and respondents given a letter: patient (P), family carer (CA), staff (S), volunteer (V), community stakeholder (CY) and senior manager or commissioner (T), with sequential numbering, date of interview and initials of researcher added to provide an audit trail. This basic coding method is used throughout the report (e.g. CH1, S01 represents the first staff member to be interviewed at the first community hospital case study site). It is worth noting, however, that, although respondents were identified by a key characteristic (e.g. patient or staff) and their transcripts labelled as such, the boundaries between these categories were not discrete: many community stakeholders, for example, had also been patients or carers, and many staff were also members of the local community.
- Phase 3: quantitative analysis of Charity Commission data
Collating data on charitable finance and volunteering support
The third phase of our research involved the quantitative analysis of data from the Charity Commission on voluntary income and volunteering for community hospitals across England. The aim of this activity was to examine charitable financial and volunteering support for community hospitals by investigating:
- variations in the likelihood that hospitals receive support through a formal organisational structure such as a League of Friends, and if so, variations in its scale (in financial terms) between communities
- uses of the funds raised (e.g. capital development, equipment, patient amenities).
We captured financial and volunteering data for registered charities from the Charity Commission (the Commission). The Commission holds details of organisations that have been recognised as charitable in law and that hold most of their assets in England, or have all or the majority of their trustees normally resident in England, or are companies incorporated in England. The data are described more fully in Appendix 9 .
Subject to a small number of exceptions, all charities in England with incomes of > £5000 must register with the Commission and submit financial statements consisting of trustees’ annual reports (returns) and annual accounts. The accounts of those charities whose income or expenditure exceeds a threshold of £25,000 are made available on the Commission’s website. 89 Charities that have income and expenditure of < £5000 a year have (since 2009) been exempted from the need to register. We identified 274 hospitals in England that satisfied the inclusion criteria for this research project ( Figure 3 ). We used the Charity Commission’s data to identify charities that support each of these hospitals, matching by name or through examining lists of charities registered in the locality where the hospital is based.
Community hospital and charities sampling frame.
We also directly approached eight non-registered charities (usually those with an income of < £5000 a year) that were known to have been established to support specific community hospitals, but received no usable data relating to them. Four hospitals in our data set were registered as charities themselves but were excluded from the analysis because they are exceptional cases of charitable action.
We found that 247 of these charities were registered in their own right (labelled ‘individual associated charities’ in Figure 3 ). The remainder were what is known as ‘linked’ charities, that is, entities associated with larger charitable organisations serving a NHS trust comprising several institutions. These ‘linked’ charities were excluded because it was not possible to disaggregate the support they provide to individual components of the trust. Financial information was available for the period from 1995 to 2014 (only small numbers of observations were available for years prior to that because digitisation of the register began only in the early 1990s).
Measurements
Financial information for at least 1 year between 1995 and 2014 was available for 245 charities in England, and this information formed the final sample for this part of the study. The number of non-zero financial reports to the Commission in each year ranged from 181 (1996) to 226 (2007). The data, covering the period to 2014, were the latest available at the time of analysis (2016). See Appendix 9 for full details of available charity reports by year. All financial information in this paper is presented at constant 2014 prices.
Using the Charity Commission website, we obtained copies of these accounts for those selected charities whose expenditure or income exceeded £25,000 in any one year. This gave data covering 358 separate financial years; the number of accounts available is shown in Table 5 .
Accounts for larger charities (income of > £25,000)
We focused on the period from 2008 to 2013, when between 41 and 91 charities of interest generated at least one such financial return. Numbers vary because an individual charity may or may not exceed the £25,000 threshold at which its accounts are presented via the Charity Commission’s website, depending on fluctuations in its finances.
Charity accounts aggregate income and expenditure figures into a small number of general categories. These provide relatively little detail on income and expenditure and may even aggregate quite different sources of expenditure within the same funding stream. As such, to probe income sources and the application of expenditure in more detail, data were captured from the notes to the accounts of these charities. The extensive income data that were generated (21,733 items) were categorised to provide useful insights into sources of income. Classifying the expenditure of charities was not undertaken because of the complexity of the data and the limits to the usefulness of such an exercise. Appendix 9 provides further details of the extraction, classification and analysis of income and expenditure data.
Contribution: number of volunteers and estimates of input
The Charity Commission guidelines 90 require charities to record their best estimates of the number of individual UK volunteers involved in the charity during the financial year, excluding trustees (see Appendix 9 ).
Before 2013, data on volunteer numbers were often sparse, but, since that date, efforts have been made to gather more detailed information. Approximately 73,000 charities had supplied between one and three non-zero returns of their volunteer counts in the three years between 2013 and 2015, including > 90% of our charities. We calculated the average number of volunteers for the period in question. To provide an upper-bound estimate, we also take the maximum value returned for each charity.
Volunteer hours were estimated using regular survey data (Home Office Citizenship Survey, 2001–10; Community Life survey, 2012 onwards). We take the average number of hours per week reported by those who say they have given unpaid help to organisations during the previous year. This is approximately 2.2 hours. This is a minimum estimate and it may be that the actual numbers are larger than these survey data would imply. If we make the assumption that these are probably fairly regular volunteers, a higher figure of 3.05 hours per week is given if we take the average number of hours reported by those who say they volunteer either at least once a week or more frequently, or at least monthly but less frequently than once a week.
There are no studies that would tell us with any certainty whether or not volunteers in these kinds of organisations put in more or fewer hours than the volunteering population generally. We then multiply these two estimates of time inputs by the average and maximum volunteer numbers, respectively, to give the number of hours contributed by volunteers over the course of the year (assuming 46 weeks of volunteering a year). These can be converted to full-time equivalent numbers by dividing by 37.5 (hours per working week) and 46 (weeks per working year).
Opinions differ on the best method for calculating a cash equivalent for the value of volunteer labour. The lowest is to use the national minimum wage; others might include an estimate of the replacement cost (i.e. what it would cost the organisation to employ people to do the same tasks if they had to pay them), but this assumes knowledge of the tasks being undertaken. The national minimum wage for the period for which we have the most comprehensive volunteering data (2013–15) was £6.50 per hour. 91
Data convergence and integration
Although the quantitative (phases 1 and 3) and qualitative (phase 2) data were collected separately, they could nevertheless be considered ‘integrated’ because the different research elements were explicitly related to each other within a single study and in such a way ‘as to be mutually illuminating, thereby producing findings that are greater than the sum of the parts’. 92 Data triangulation, convergence and integration occurred in a number of different ways, at different stages of the research.
In phase 1 of the research, a revised definition and set of characteristics captured within the database was used to support development of a typology and informed the case study sampling for phase 2. For phase 3, the database informed the sample of charities selected for analysing voluntary income and volunteering data and providing additional data fields to be linked to the Charity Commission data.
Although the national quantitative data provided breadth to the study, these were limited and left questions unanswered. The local qualitative data brought depth to the question ‘what is a community hospital’, by helping to build a picture of the history, context and change over time. Qualitative interviews in work packages 2 and 3 were conducted concurrently, and triangulation of data between stakeholder, volunteer, staff, carer and patient interviews helped validate findings and strengthen our understanding of patient and carer experiences and community engagement and value.
In addition, the combination of researchers working on more than one work package, reflexive team meetings and the involvement of different representations in the team [CHA, University of Birmingham and Crystal Blue Consulting (London, UK)] allowed for healthy dialogue, debate and analysis. Emerging findings from each phase of the research were, for example, shared through internal working papers and discussed regularly at whole project team meetings.
- Patient and public involvement
Our commitment to PPI ensured that patients, carers and the public were involved in this study before and during its conduct. PPI involvement in the study design was facilitated by one of the researchers (HT), who first consulted with 10 PPI members of the Swanage Health Forum, representing the League of Friends; a GP practice Patient Participation Group; Swanage Carers; Partnership for Older People’s Programme; Wayfinders; the Senior Forum; the Health and Wellbeing Board; Cancare; a public Governor for Dorset Healthcare NHS Trust; and a retired GP. This group provided an endorsement of the study’s proposed focus and methodology.
At the national level, 13 board members of CHA (four GPs, six nurses, two managers and one League of Friends member) co-produced the initial research proposal. Two members then became part of the study steering group, which met regularly throughout the study, supported the development of research materials and supporting documentation, helped facilitate access to potential case studies, contributed to the local and national reports and reviewed several drafts. We also engaged with approximately 100 delegates at three CHA annual conferences (presentations and workshops focused on working with findings) that included not only practitioners but members of community hospital Leagues of Friends.
In addition, a cross-study steering group, chaired by Professor Sir Lewis Ritchie, University of Aberdeen, provided guidance across all three Health Services and Delivery Research community hospital studies, with representation from the CHA, Attend (National League of Friends) and the Patients Association, alongside the three study teams. The steering group met seven times over the period of this study, offering opportunities to share findings and explore experiences between the studies.
As described in Local reference group , at the local level we established LRGs within each of our case study sites to bring local people together (hospital staff, volunteers and community members, a number of whom were patients and/or carers) to steer, support and inform the case study research. To facilitate cross-case learning, we brought together representatives from each of the LRGs three times to share experiences, identify best practice and network. Event themes reflected each of the three research questions, and the days offered time for case study representatives to work together, share across sites, hear from national experts, contribute to the ongoing development of the study and reflect on emerging findings and their implications.
- Ethics approval
Ethics approval was provided by the University of Birmingham, in line with the Department of Health and Social Care’s Research Governance Framework, for work package 1 (national mapping) and elements of work package 3 (quantitative charitable finance and volunteering support data). The university also provided sponsorship for the whole study. The qualitative case studies required full ethics review through the National Research Ethics Service as they involved interviews with patients and carers and interviews and focus groups with NHS staff, volunteers and community stakeholders. The Wales Research Ethics Committee 6 reviewed this research and provided a favourable ethics opinion (study reference number: 16/WA/0021).
Informed by key stakeholder engagement and a review of the policy context and existing literature, this study explored the profile, characteristics, patient and carer experience, community engagement and value of community hospitals in England through a multimethod approach. The research was conducted in three overlapping phases – mapping, case studies and Charity Commission data analysis – that, together, involved a range of qualitative and quantitative methods. Data for each phase were collected and analysed separately but iteratively, with emerging findings discussed regularly through a range of mechanisms, including whole project team meetings and internal working papers. We involved key national and local stakeholders throughout the study, from design, through to data collection and analysis, and reporting and dissemination.
Having framed the study (see Chapter 1 ) and described our research methodology (see Chapter 2 ), we now move on to share the findings. Chapters 3 – 7 describe the findings emerging from different elements of the study, and Chapter 8 brings those findings together and discusses them in relation to the wider literature and their significance for knowledge and practice.
- Cite this Page Davidson D, Ellis Paine A, Glasby J, et al. Analysis of the profile, characteristics, patient experience and community value of community hospitals: a multimethod study. Southampton (UK): NIHR Journals Library; 2019 Jan. (Health Services and Delivery Research, No. 7.1.) Chapter 2, Research objectives, questions and methodology.
- PDF version of this title (6.1M)
In this Page
Other titles in this collection.
- Health Services and Delivery Research
Recent Activity
- Research objectives, questions and methodology - Analysis of the profile, charac... Research objectives, questions and methodology - Analysis of the profile, characteristics, patient experience and community value of community hospitals: a multimethod study
Your browsing activity is empty.
Activity recording is turned off.
Turn recording back on
Connect with NLM
National Library of Medicine 8600 Rockville Pike Bethesda, MD 20894
Web Policies FOIA HHS Vulnerability Disclosure
Help Accessibility Careers
Title: Disengaged: the problem of employee engagement in gig workers
Authors : Rebecca Wason
Addresses : Algoma University, 1520 Queen St E, Sault Ste. Marie, Ontario, P6A 2G4, Canada
Abstract : The objective of this study is to examine the levels of disengagement among remotely working independent gig workers with their client teams and organisations. The researcher distributed a structured questionnaire anchored on Kahn's three facets of employee engagement to various freelancers and consultants, also known as gig workers. Based on the results of the study, the respondents were adequately engaged in the work for which they were contracted, but their level of organisational and client team engagement was lower in comparison. Additionally, many respondents felt that the management had not clearly stated the significance and purpose of their work and/or had not guided them on organisational culture and norms. Thus, many of the respondents felt excluded and were unable to form social bonds with the client team that they were working with.
Keywords : employee disengagement; employee job satisfaction; gig workers; gig workers' job motivation; gig workers' job satisfaction; remote employees; organisational engagement; remote workers.
DOI : 10.1504/IJMCP.2024.137637
International Journal of Management Concepts and Philosophy, 2024 Vol.17 No.2, pp.149 - 160
Received: 28 Dec 2022 Accepted: 20 Feb 2023 Published online: 02 Apr 2024 *
Keep up-to-date
- Our Newsletter ( subscribe for free )
- New issue alerts
- Inderscience is a member of publishing organisations including:
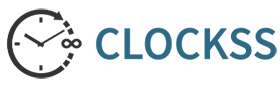
Public Health Sciences Learning Objectives
Public health sciences students graduate with the knowledge and skills necessary to be successful in the workplace, public health sciences major - learning objectives.
Students in the Public Health Sciences major graduate with the following skills and abilities:
- Describe the concept of population health and the basic processes, approaches, and interventions specific to the needs and concerns of populations.
- Evaluate the source and quality of health information and data as related to individual and community health.
- Demonstrate mastery in public health communication skills including oral communication and written communication for both lay and expert audiences.
- Assess the values and perspectives of diverse individuals, communities, and cultures, and describe how these factors influence health behaviors, choices, and practices.
- Engage in collaborative, team-based, and interdisciplinary approaches for improving population health.
- Identify public health tools and evidence-based strategies to respond to public health issues in a global world.
- Gain awareness of and exposure to the broad and diverse range of areas of study within the field of public health, and identify and explore areas that are of personal interest.
The Public Health Sciences major is accredited by the Council for Education on Public Health
Courses in the Public Health Sciences major provide instruction in the following domains and competencies, developed by the Council for Education on Public Health.
Foundational Domains
- The concepts and applications of basic statistics
- The foundations of biological and life sciences
- The history and philosophy of public health as well as its core values, concepts, and functions across the globe and in society
- The basic concepts, methods, and tools of public health data collection, use, and analysis and why evidence-based approaches are an essential part of public health practice
- The concepts of population health, and the basic processes, approaches and interventions that identify and address the major health-related needs and concerns of populations
- The underlying science of human health and disease, including opportunities for promoting and protecting health across the life course
- The socioeconomic, behavioral, biological, environmental, and other factors that impact human health and contribute to health disparities
- The fundamental concepts and features of project implementation, including planning, assessment, and evaluation
- The fundamental characteristics and organizational structures of the U.S. health system as well as the differences between systems in other countries
- Basic concepts of legal, ethical, economic, and regulatory dimensions of health care and public health policy and the roles, influences, and responsibilities of the different agencies and branches of government
- Basic concepts of public health-specific communication, including technical and professional writing and the use of mass media and electronic technology
Foundational Competencies
- Communicate public health information, in both oral and written forms, through a variety of media and to diverse audiences
- Locate, use, evaluate, and synthesize public health information
Accreditation
The Public Health Sciences major at UMass Amherst is accredited by the Council on Education for Public Health, which is an independent agency recognized by the U.S. Department of Education to accredit schools of public health, and public health programs outside schools of public health.
Global footer
- ©2024 University of Massachusetts Amherst
- Site policies
- Non-discrimination notice
- Accessibility
- Terms of use
Prevention of Child Maltreatment: Primary Care Interventions
About this resource:.
Source: U.S. Preventive Services Task Force
The last reviewed date indicates when the evidence for this resource last underwent a comprehensive review.
Workgroups: Injury and Violence Prevention Workgroup
The U.S. Preventive Services Task Force (USPSTF) found that the current evidence is insufficient to assess the balance of benefits and harms of primary care interventions to prevent child maltreatment in children and adolescents younger than 18 years who don’t have signs or symptoms of, or known exposure to, maltreatment.
More research is needed to help primary care clinicians accurately identify families who might benefit from supportive interventions to prevent child maltreatment. Studies are also needed to:
- Determine whether intervention effectiveness or child maltreatment reporting differs by social factors and race and ethnicity
- Evaluate the effectiveness of interventions, using more accurate outcome measures that limit bias
- Establish consistency in outcome measure definitions, outcome types, and outcome timing
- Identify the most effective ways to prevent child maltreatment, including interventions that address the social determinants of health that can negatively affect families
USPSTF recommends clinicians should use their judgment to decide if and when to provide interventions to help prevent child maltreatment in children without signs or symptoms.
Objectives related to this resource (2)
Suggested citation.
U.S. Preventive Services Task Force. (2024). Final Recommendation Statement: Prevention of Child Maltreatment: Primary Care Interventions. Retrieved from https://www.uspreventiveservicestaskforce.org/uspstf/recommendation/child-maltreatment-primary-care-interventions .
The Office of Disease Prevention and Health Promotion (ODPHP) cannot attest to the accuracy of a non-federal website.
Linking to a non-federal website does not constitute an endorsement by ODPHP or any of its employees of the sponsors or the information and products presented on the website.
You will be subject to the destination website's privacy policy when you follow the link.
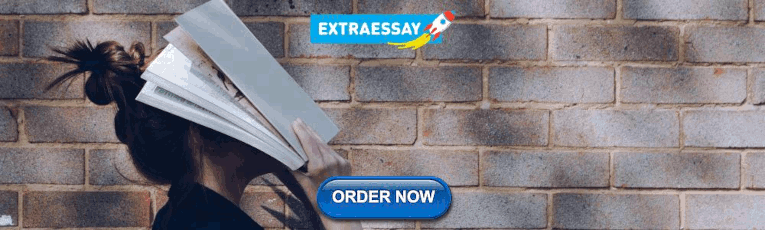
IMAGES
VIDEO
COMMENTS
Formulating research objectives has the following five steps, which could help researchers develop a clear objective: 8. Identify the research problem. Review past studies on subjects similar to your problem statement, that is, studies that use similar methods, variables, etc.
Research objectives describe what your research project intends to accomplish. They should guide every step of the research process, including how you collect data, build your argument, and develop your conclusions. Your research objectives may evolve slightly as your research progresses, but they should always line up with the research carried ...
Research Objectives. Research objectives refer to the specific goals or aims of a research study. They provide a clear and concise description of what the researcher hopes to achieve by conducting the research.The objectives are typically based on the research questions and hypotheses formulated at the beginning of the study and are used to guide the research process.
A research objective is defined as a clear and concise statement of the specific goals and aims of a research study. It outlines what the researcher intends to accomplish and what they hope to learn or discover through their research. Research objectives are crucial for guiding the research process and ensuring that the study stays focused and ...
The research aims, objectives and research questions (collectively called the "golden thread") are arguably the most important thing you need to get right when you're crafting a research proposal, dissertation or thesis.We receive questions almost every day about this "holy trinity" of research and there's certainly a lot of confusion out there, so we've crafted this post to help ...
The research aim focus on what the research project is intended to achieve; research objectives focus on how the aim will be achieved. Research aims are relatively broad; research objectives are specific. Research aims focus on a project's long-term outcomes; research objectives focus on its immediate, short-term outcomes.
Research objectives are how researchers ensure that their study has direction and makes a significant contribution to growing an industry or niche. Research objectives provide a clear and concise statement of what the researcher wants to find out. As a researcher, you need to clearly outline and define research objectives to guide the research ...
Part 1 The research process. 1 Develop the research objectives. 2 Design and plan the study. 3 Write the proposal. 4 Obtain financial support for the research. 5 Manage the research. 6 Draw conclusions and make recommendations. 7 Write the report. 8 Disseminate the results.
To develop a set of research objectives, you would then break down the various steps involved in meeting said aim. For example: This study will investigate the link between dehydration and the incidence of urinary tract infections (UTIs) in intensive care patients in Australia. To achieve this, the study objectives w ill include:
A research aim is a broad statement indicating the general purpose of your research project. It should appear in your introduction at the end of your problem statement, before your research objectives. Research objectives are more specific than your research aim. They indicate the specific ways you'll address the overarching aim.
Here are three simple steps that you can follow to identify and write your research objectives: 1. Pinpoint the major focus of your research. The first step to writing your research objectives is to pinpoint the major focus of your research project. In this step, make sure to clearly describe what you aim to achieve through your research.
<button>Click to continue</button>
Examples of Specific Research Objectives: 1. "To examine the effects of rising temperatures on the yield of rice crops during the upcoming growth season.". 2. "To assess changes in rainfall patterns in major agricultural regions over the first decade of the twenty-first century (2000-2010).". 3.
Answer: Research objectives describe concisely what the research is trying to achieve. They summarize the accomplishments a researcher wishes to achieve through the project and provides direction to the study. A research objective must be achievable, i.e., it must be framed keeping in mind the available time, infrastructure required for ...
A research objective addresses the purpose of the investigation and the types of knowledge generated from one's investigation. Looking at the objectives of the research, one can anticipate what is to be achieved by the study. A research objective indicates the population of interest and independent and dependent variables.
5 Examples of Research Objectives. The following examples of research objectives based on several published studies on various topics demonstrate how the research objectives are written: This study aims to find out if there is a difference in quiz scores between students exposed to direct instruction and flipped classrooms (Webb and Doman, 2016).
The development of research questions and the subsequent hypotheses are prerequisites to defining the main research purpose and specific objectives of a study. Consequently, these objectives determine the study design and research outcome. The development ...
Formulating research aim and objectives in an appropriate manner is one of the most important aspects of your thesis. This is because research aim and objectives determine the scope, depth and the overall direction of the research. Research question is the central question of the study that has to be answered on the basis of research findings.
Research objective. The primary objective should be coupled with the hypothesis of the study. Study objectives define the specific aims of the study and should be clearly stated in the introduction of the research protocol. 7 From our previous example and using the investigative hypothesis that there is a difference in functional outcomes ...
A research design is a strategy for answering your research question using empirical data. Creating a research design means making decisions about: Your overall research objectives and approach. Whether you'll rely on primary research or secondary research. Your sampling methods or criteria for selecting subjects. Your data collection methods.
An in-depth analysis of information creates space for generating new questions, concepts and understandings. The main objective of research is to explore the unknown and unlock new possibilities. It's an essential component of success. Over the years, businesses have started emphasizing the need for research.
Moreover, the research objectives should be framed by the researcher before the initiation of the study: a specific, measurable, achievable, realistic, and time-defined (SMART) approach is utilized to devise the objectives based on the research question. It is preferable to have a single primary objective whereas the secondary objectives can be ...
While the examination of AI capabilities was not the primary research focus, we found first evidence in the use cases that indicates AI technology's unique capabilities (e.g., to make diagnoses accurate, faster, and more objective) that foster one or several business objectives (e.g., rapid task execution, precise decision support) and unlock ...
Arthritis Care & Research is a rheumatology journal from the American College of Rheumatology and the Association of Rheumatology Health Professionals. Objective The purpose of this study was to determine the causal effect of statins on osteoarthritis (OA) risk using Mendelian randomization (MR). Methods Single-nucleotide polymorphisms (SNP ...
The primary objective of the study was local failure-free survival (LFFS); which was calculated from the date of diagnosis to failure to achieve remission at the local site of CRT, detection of local progression/date of death or date of last follow up. The secondary objectives were event-free survival, overall survival (OS) and patterns of ...
Table 1 summarises the study objectives, questions and research methods. Each of the three phases of research are discussed in turn through the following sections of this chapter, before the final sections discuss data integration, PPI and ethics. Phase 1: mapping and profiling community hospitals.
Abstract: The objective of this study is to examine the levels of disengagement among remotely working independent gig workers with their client teams and organisations. The researcher distributed a structured questionnaire anchored on Kahn's three facets of employee engagement to various freelancers and consultants, also known as gig workers.
We formulate a multi-objective optimisation problem and implement the NSGA-II algorithm to obtain Pareto optimal solutions. Finally, three regression models are proposed to determine the minimum cost and the associated optimal service rates, given the maximum acceptable value of the expected number of rush orders. ... His research interests ...
Public Health Sciences Major - Learning Objectives. Students in the Public Health Sciences major graduate with the following skills and abilities: Describe the concept of population health and the basic processes, approaches, and interventions specific to the needs and concerns of populations.
The U.S. Preventive Services Task Force (USPSTF) found that the current evidence is insufficient to assess the balance of benefits and harms of primary care interventions to prevent child maltreatment in children and adolescents younger than 18 years who don't have signs or symptoms of, or known exposure to, maltreatment. More research is needed to help primary care clinicians accurately ...