11 fully funded PhD student positions in the computational & experimental plant sciences
The graduate school.
Our International Max Planck Research School on Understanding Complex Plant Traits using Computational and Evolutionary Approaches is a local collaboration between the Max Planck Institute for Plant Breeding Research and the University of Cologne, Germany. International students are closely supervised by an advisory committee, receive training in professional skills and are supported to share their scientific insights. All training is in English.
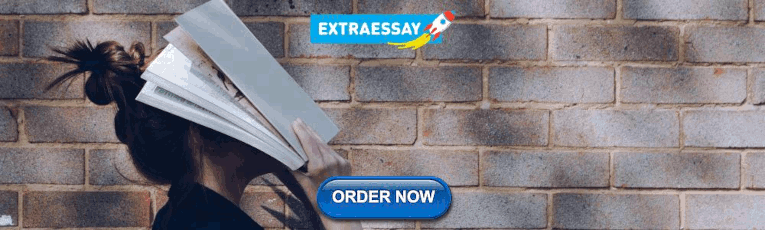
The research
The mission of our IMPRS is to study fundamental biological processes in plants and to create knowledge and material that can empower innovative plant breeding. More than 30 research groups study regulatory networks controlling fundamental traits such as reproductive development, organ geometry and growth, innate immunity and microbiome effects on plant performance. They apply and develop a broad range of interdisciplinary technologies ranging from genetics and genomics to structural biochemistry, advanced imaging and computational modelling in various plant species. All available projects are detailed at www.mpipz.mpg.de/imprs/proposals where applicants will also find more information about the host groups.
Your profile
You are a highly qualified and motivated student from any nationality and hold, or expect to hold, a Master’s degree in a related subject. You have a proved track record of academic and research excellence and are fluent in written and spoken English.
We provide an excellent, international and interdisciplinary research environment with state-of-the-art facilities and a renowned faculty. Positions are fully funded for 3 years with possible extension. Salary is based upon the framework of a Max Planck Society doctoral funding contract (TVöD 13, 65%). We are committed to increasing the number of individuals with disabilities in the workforce and encourage applications from such qualified individuals. Further, we seek to increase the number of women in those areas where they are underrepresented and therefore explicitly encourage women to apply.
Your application
- Apply online by January 6 th 2024 at gradschool.mpipz.mpg.de
- Shortlisted applicants will be invited for interviews on March 19 th –25 th 2024
- Selected applicants will start the PhD between summer and October 1 ST 2024
- Dr. Monika Schlosser at [email protected]
- Find more information at www.mpipz.mpg.de/imprs/
Freie Universität Berlin
Service navigation.
- Legal Notice
- Data Protection Policy
- Emergencies
- Accessibility Statement
- DE: Deutsch
- EN: English
- Prospective Students
- Students and Doctorate
- Researchers
- Alumni and Supporters
- Journalists
- Continuing Education
Path Navigation
- International Max Planck Research School for Computational Biology and Scientific Computing
International Max Planck Research School for Computational Biology & Scientific Computing (IMPRS-CBSC)
The International Max Planck Research School for Computational Biology and Scientific Computing (IMPRS-CBSC) originated in a joint initiative of the Max Planck Institute for Molecular Genetics and Freie Universität Berlin. Building on the existing master’s degree programs in bioinformatics and scientific computing at Freie Universität Berlin, it is integrated into a number of scientific working groups that work in the fields where life sciences (molecular biology, genome research) and theoretical sciences (mathematics, computer science) overlap. The IMPRS-CBSC focuses on issues of mathematics and computer science with regard to sequential analysis, theoretical structural biology, theoretical chemistry and drug design, molecular evolution, genome analysis, and methods of data analysis for functional genome research.
Berlin-Dahlem offers a unique concentration of academic and scientific excellence in this field. This enables participating students to get to know the full spectrum of formal methods and put them into practice in tackling a variety of applied issues.
Read more: www.imprs-cbsc.mpg.de
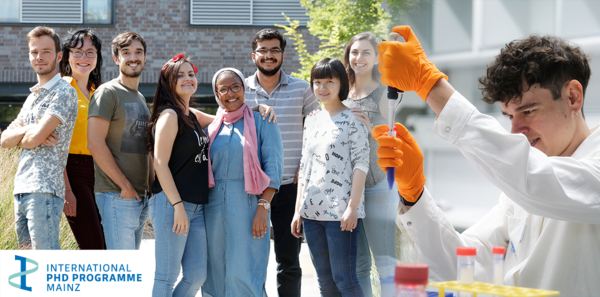
Are you a talented and enthusiastic student with a Master’s degree in molecular biology or a related field? Join us and become a researcher in one of more than 50 labs working on cutting-edge research in Mainz!
As a PhD student in our International PhD Programme (IPP), you can work on exciting projects related to our overarching theme of Gene Regulation, Epigenetics & Genome Stability , as well as join our lively IPP community of 200 students from all over the world!
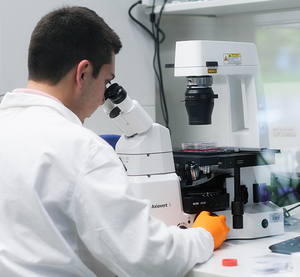
The IPP has participating labs from the Institute of Molecular Biology (IMB), Johannes Gutenberg University (JGU) and the University Medical Center (UMC). Our research groups offer projects in:
- Ageing & disease
- RNA biology
- DNA repair & genome stability
- Epigenetics & nuclear dynamics
- Bioinformatics & computational biology
- Gene regulation & evolution
- Proteostasis & phase separation
Within these projects, you will have the chance to work closely with top scientists, learn valuable skills and get training in the latest techniques like CRISPR/Cas genome editing, next-generation sequencing (NGS), gene network modelling, mass spectrometry and super-resolution microscopy. See our research topics page for more information.
In addition, the IPP has many scientific and social events for you to meet and get to know your fellow students! Find out more about what the IPP offers you here .
For more facts and figures about the IPP, check out the IPP at a Glance .
If you have any additional questions, please check out our FAQs or feel free to drop us an email .
Computational Biology
Computational Biology is concerned with the development and application of computer-based methods for the analysis of biological data and the creation of mathematical and statistical models that can be used to describe and simulate biological processes. Here, both data-driven approaches from the field of machine learning are applied and hypothesis-driven models are adapted to existing data. A particular challenge is the integration of different types of biological data in order to create a holistic picture of biological processes. This interdisciplinary subject is at the interface between biology, mathematics and computer science, and offers numerous areas of application, e.g. in biomedical research or the pharmaceutical industry.
Supervising professors
Prof. dr. carl herrmann, prof. dr. ursula kummer, pd dr. karl rohr.
Contact person for PhD questions

Links und Funktionen
Sprachumschaltung, navigationspfad.
- Career and jobs
PhD student positions in the computational and experimental plant sciences (Cologne, Germany)
Hauptnavigation.
- News & Events
- Serviceeinheiten
- Organisation
- Für Mitarbeiter*Innen
- Für Student*Innen
- Für BioAlumni
- Für die Öffentlichkeit
- Nachhaltigkeit an der Fakultät
- Tag der offenen Tür am 3. Juli 2024
- Anfahrt und Adresse
Inhaltsbereich
11 fully funded PhD students positions
The Max Planck Institute for Plant Breeding Research in Cologne, Germany and the University of Cologne, Germany have opened 11 fully funded PhD students positions in the computational and experimental plant sciences embedded in an International Max Planck Research School (IMPRS).
The call closes on January 6th 2024 and successful candidates are expected to start the PhD positions between summer and winter 2024 .
Further information is available at https://www.mpipz.mpg.de/imprs-2021 and in the PDF document ( click here ).
- phd_cologne (182 KByte)
- Datenschutzerklärung
- Barrierefreiheit
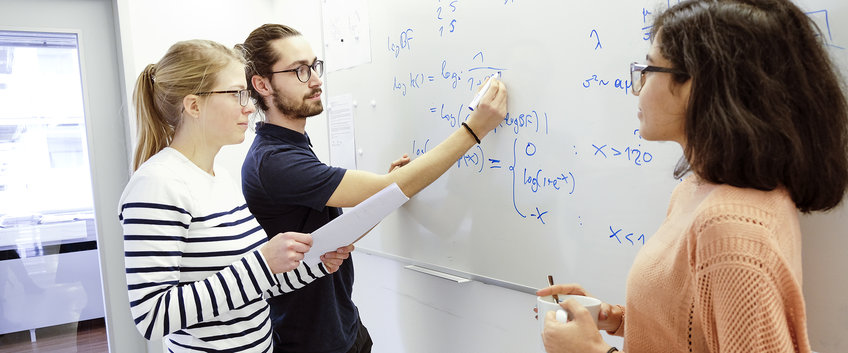
Programs for Graduate and Master Students
Do you study life sciences or medicine?
Are you interested in a bachelor's, master's , or doctoral thesis at our institute?
Then you are welcome to apply directly to our directors and research group leaders.
You can find information on the various doctoral programs at the Göttingen Campus , in which our institute participates, here. If you have general questions regarding your application, please contact our coordinator Antje Erdmann (see contacts on the left).
All our students doing research at the institute for a thesis or doctoral thesis must be enrolled at a university at the same time. With a few exceptions, academic titles in Germany are only awarded by the nationwide universities. The institute, therefore, cooperates closely with the University of Göttingen in the IMPRS doctoral programs listed below, where our directors and research group leaders also teach and give seminars.
International Max Planck Research Schools (IMPRS)

Max Planck School Matter to Life
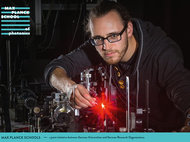
Max Planck School of Photonics
There are also programs for young scientists within the framework of further cooperations between the institute and the University of Göttingen, the Max Planck Institutes for Dynamics and Self-Organization and for Experimental Medicine as well as the German Primate Center. These include:
- the European Neuroscience Institute Göttingen (ENI)
- the Bernstein Center for Computational Neuroscience Göttingen (BCCN Göttingen)
- the Göttingen Excellence Cluster nad DFG Research Center Nanoscale Microscopy and Molecular Physiology of the Brain (CNMPB)
86 Best universities for Bioinformatics and Computational biology in Germany
Updated: February 29, 2024
- Art & Design
- Computer Science
- Engineering
- Environmental Science
- Liberal Arts & Social Sciences
- Mathematics
Below is a list of best universities in Germany ranked based on their research performance in Bioinformatics and Computational biology. A graph of 7.07M citations received by 171K academic papers made by 86 universities in Germany was used to calculate publications' ratings, which then were adjusted for release dates and added to final scores.
We don't distinguish between undergraduate and graduate programs nor do we adjust for current majors offered. You can find information about granted degrees on a university page but always double-check with the university website.
1. Heidelberg University - Germany
For Bioinformatics and Computational biology
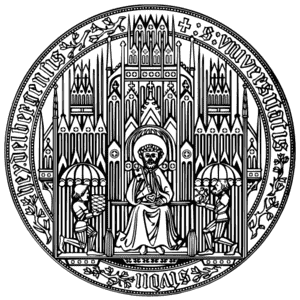
2. Technical University of Munich
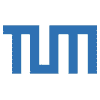
3. Charite - Medical University of Berlin
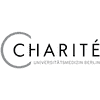
4. University of Munich
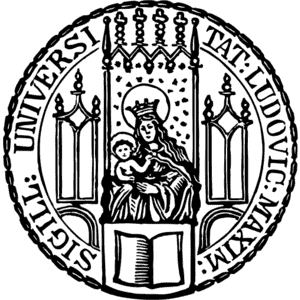
5. Goethe University of Frankfurt am Main
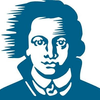
6. University of Tubingen
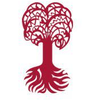
7. Heinrich Heine University of Dusseldorf
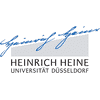
8. University of Freiburg
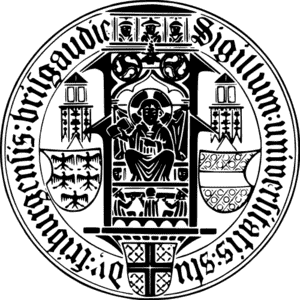
9. University of Bonn
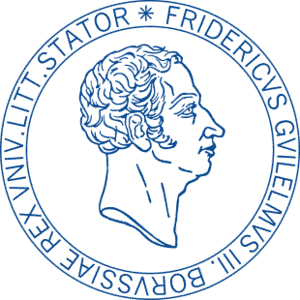
10. University of Hamburg
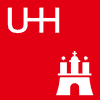
11. Hannover Medical School
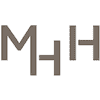
12. Free University of Berlin
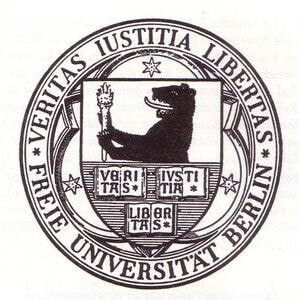
13. University of Wurzburg
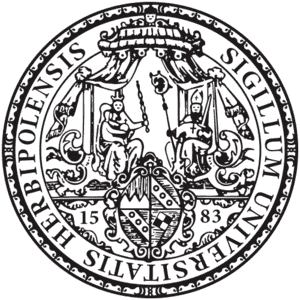
14. University of Cologne
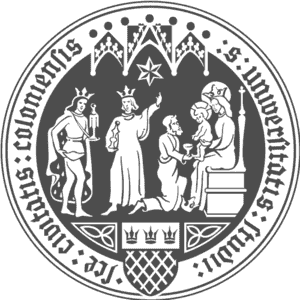
15. Johannes Gutenberg University Mainz
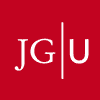
16. University of Gottingen
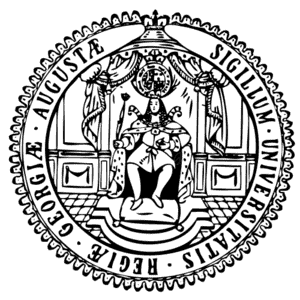
17. University of Leipzig
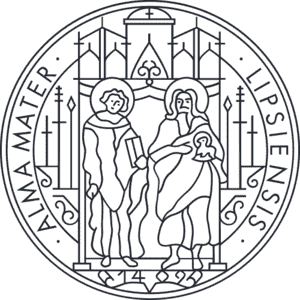
18. Humboldt University of Berlin
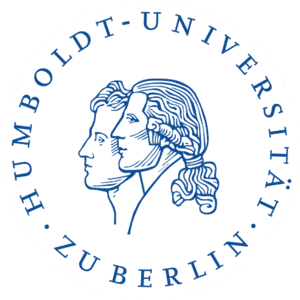
19. University of Erlangen Nuremberg
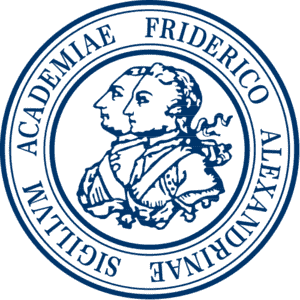
20. Saarland University
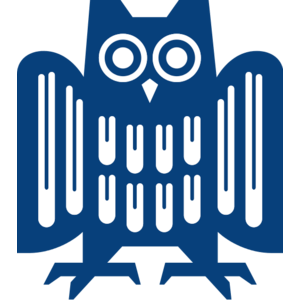
21. University of Marburg
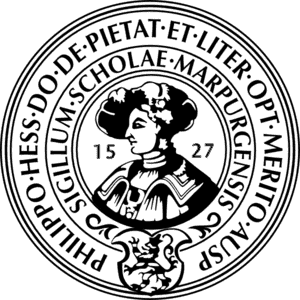
22. RWTH Aachen University
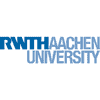
23. University of Munster
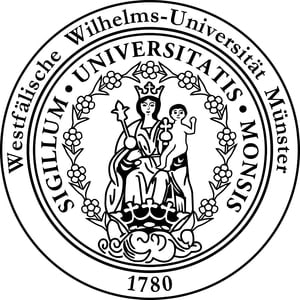
24. Dresden University of Technology
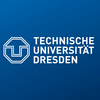
25. University of Lubeck
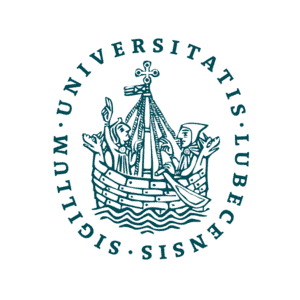
26. Kiel University
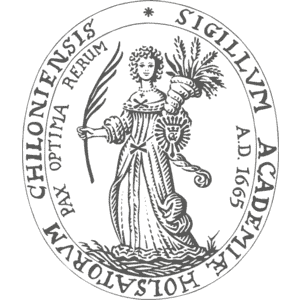
27. University of Regensburg
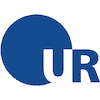
28. Ruhr University Bochum
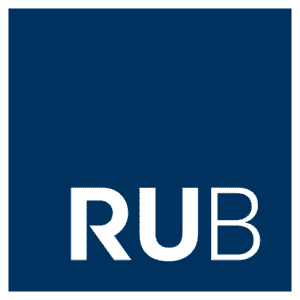
29. University of Ulm
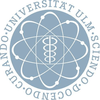
30. Friedrich Schiller University of Jena
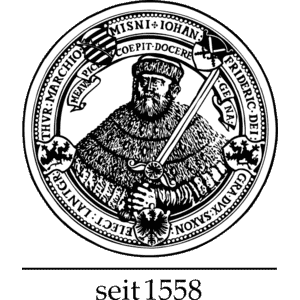
31. University of Bielefeld
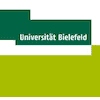
32. Martin Luther University of Halle-Wittenberg
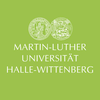
33. University of Giessen
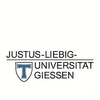
34. University of Greifswald
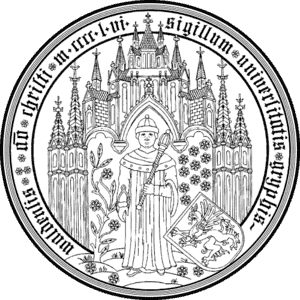
35. Braunschweig University of Technology
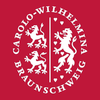
36. University of Duisburg - Essen
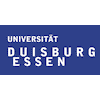
37. TU Dortmund University
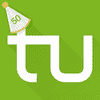
38. Jacobs University Bremen
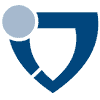
39. University of Konstanz
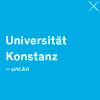
40. Karlsruhe Institute of Technology
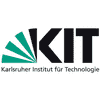
41. University of Stuttgart
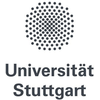
42. University of Rostock
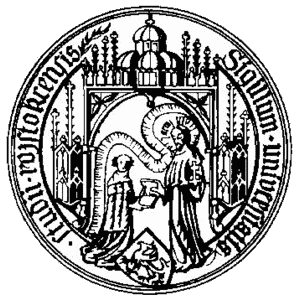
43. University of Potsdam
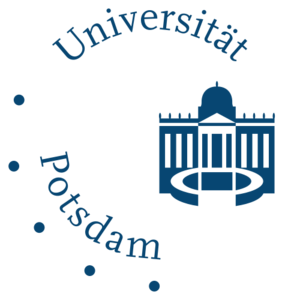
44. Technical University of Berlin
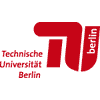
45. Otto von Guericke University of Magdeburg
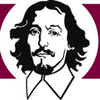
46. University of Bremen
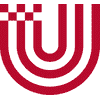
47. Darmstadt University of Technology
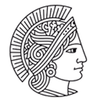
48. University of Bayreuth
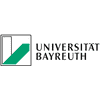
49. Leibniz University of Hanover
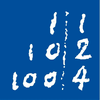
50. University of Hohenheim
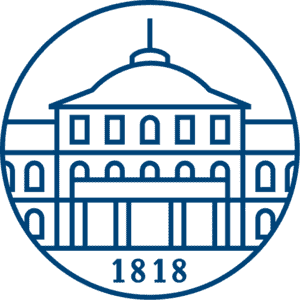
51. Witten/Herdecke University
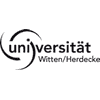
52. Technical University of Kaiserslautern
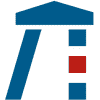
53. University of Veterinary Medicine Hannover
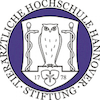
54. Weihenstephan-Triesdorf University of Applied Sciences
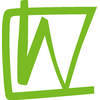
55. Carl von Ossietzky University of Oldenburg
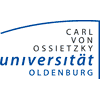
56. University of Paderborn
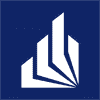
57. University of Mannheim
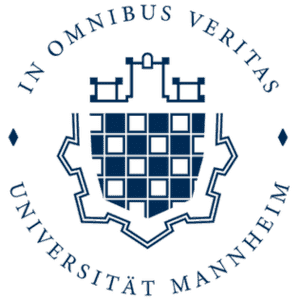
58. Osnabruck University
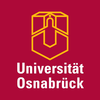
59. University of Wuppertal
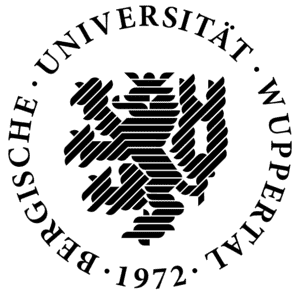
60. University of Augsburg
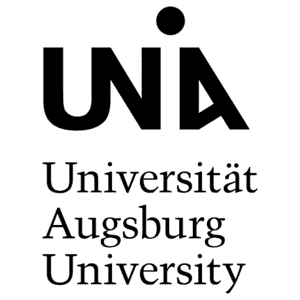
61. German Sport University Cologne
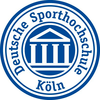
62. Hamburg University of Technology
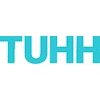
63. University of Trier
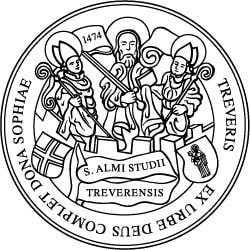
64. University of Kassel
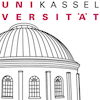
65. Aachen University of Applied Sciences
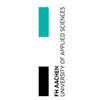
66. Freiberg University of Technology
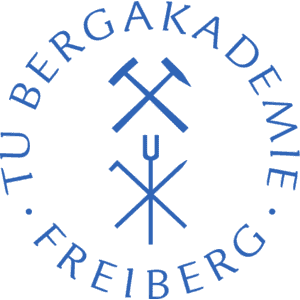
67. University of Siegen
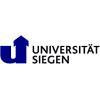
68. Hannover University of Applied Sciences
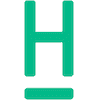
69. Munich University of the Federal Armed Forces
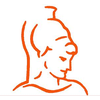
70. Darmstadt University of Applied Sciences
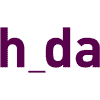
71. University of Hagen
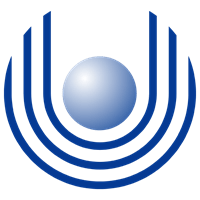
72. Biberach University of Applied Sciences
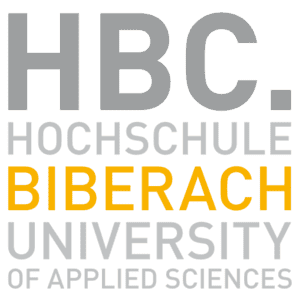
73. Brandenburg University of Technology Cottbus - Senftenberg
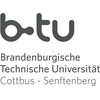
74. University of Passau
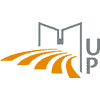
75. Mannheim University of Applied Sciences
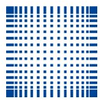
76. Leuphana University of Luneburg
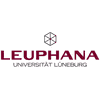
77. Mainz University of Applied Sciences
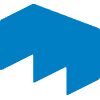
78. Ilmenau University of Technology
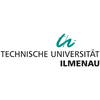
79. University of Applied Sciences Wildau
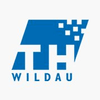
80. Furtwangen University of Applied Sciences
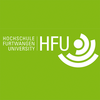
81. Berlin Technical University of Applied Sciences
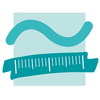
82. University of Koblenz-Landau
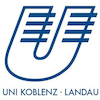
83. Chemnitz University of Technology
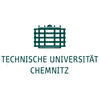
84. University of Applied Sciences Mittweida
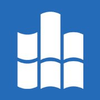
85. University of Hildesheim
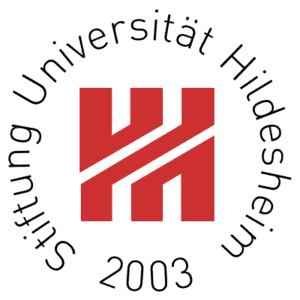
86. University of Applied Sciences Landshut
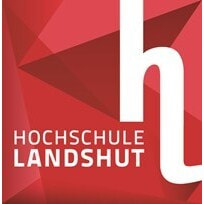
The best cities to study Bioinformatics and Computational biology in Germany based on the number of universities and their ranks are Heidelberg , Munich , Berlin , and Frankfurt .
Biology subfields in Germany

Position: PhD Student Position in Computational Biology – Somatic Evolution of Cancer Development
Department: “Somatic Evolution and Early Detection” and “Experimental Hepatology, Inflammation and Cancer”
Code number: 2024-0119
The Junior Research Groups of Dr. Angela Goncalves (Somatic Evolution and Early Detection) and Dr. Dr. Michael Dill (Experimental Hepatology, Inflammation and Cancer) are looking for a highly motivated and skilled PhD student to join a collaborative project with the goal of investigating the somatic evolution of cancer development in primary sclerosing cholangitis (PSC) by integrating large scale genomics analyses with single-cell transcriptomics with access to one of the largest organoid biobank from PSC patients.
Our research groups are committed to advancing the understanding of cancer development in chronically inflamed organs, such as liver. Our work involves cutting-edge organoid technology, genomics, single-cell transcription analysis, and computational modelling to unravel the complexities of somatic evolution during carcinogenesis.
You will be jointly affiliated with the laboratory of Dr. Goncalves and Dr. Dill to improve our understanding of somatic evolution in early carcinogenesis and will be working with an interdisciplinary team consisting of physician-scientists, biologists and bioinformaticians.
Your activities will comprise:
- Designing and analyzing novel single-cell transcription experiments using cutting-edge bioinformatics tools
- Analyzing genomic data and computational modelling of somatic mutations
- Master’s degree or equivalent qualification in computer science, mathematics or physics, or a degree in biological sciences with demonstrated experience in computational biology
- Programming skills in R or Python
- Motivation and dedication to lead a scientific project
- Excellent communication and collaboration skills, including a good command of written and oral English
- Ability to work independently and be a supportive and responsible team member
- Experience with next-generation sequencing techniques is a plus
Applications should consist of a single PDF file including a curriculum vitae, a cover letter stating research and career interests, transcripts of records of both bachelor and master studies, letter of recommendation and contact details of two referees.
- Excellent framework conditions: state-of-the-art equipment and opportunities for international networking at the highest level
- Access to international research networks
- Doctoral salary with the usual social benefits
- 30 days of vacation per year
- Flexible working hours
- Possibility of part-time work
- Family-friendly working environment, e.g. parent-child room, advisory services
- Sustainable travel to work: subsidized Germany job ticket
- Unleash your full potential: targeted training and mentoring through the DKFZ International PhD Program and DKFZ Career Service
- Our Corporate Health Management Program offers a holistic range of services for your well-being
Important notice:
The DKFZ is subject to the regulations of the Infection Protection Act (IfSG). As a consequence, only persons who present proof of immunity against measles may work at the DKFZ.
Earliest Possible Start Date: as soon as possible
Duration: The position is initially limited to 3 years.
Application Deadline: 21.05.2024
Dr. Dr. Michael Dill Phone +49 (0)6221/42-1520
Please note that we do not accept applications submitted via email.
The DKFZ is committed to increase the proportion of women in all areas and positions in which women are underrepresented. Qualified female applicants are therefore particularly encouraged to apply.
Among candidates of equal aptitude and qualifications, a person with disabilities will be given preference.
To apply for a position please use our online application portal (https://www.dkfz.de/en/stellenangebote/index.php).
We ask for your understanding that we cannot return application documents that are sent to us by post (Deutsches Krebsforschungszentrum, Personalabteilung, Im Neuenheimer Feld 280, 69120 Heidelberg) and that we do not accept applications submitted via email. We apologize for any inconvenience this may cause.
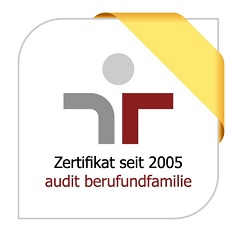

- General Information
- Tuition fees
Application & Admission
Language requirements, program features.
- List of Universities
2716 Study programs
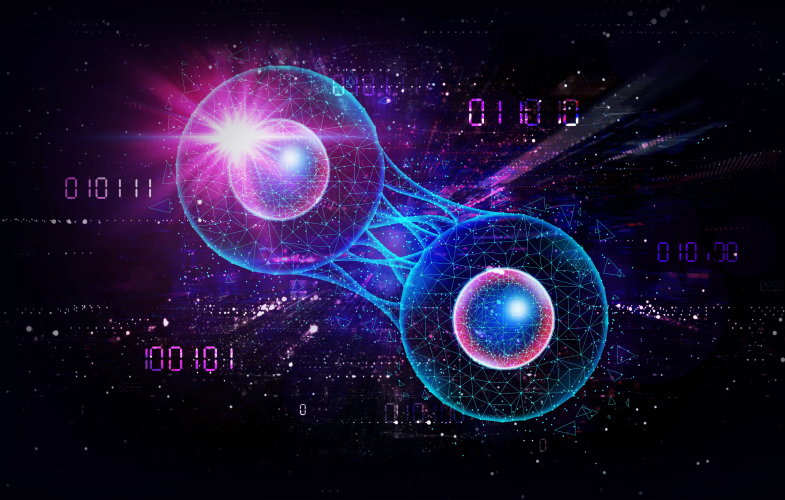
Study Computational Biology in Germany: 11 Universities with 12 English Degree Programs
All important info for international students in germany (2024/2025).
Computational Biology is the exciting intersection of biology and digital computation, decoding life's mysteries with tech's precision. This dynamic field uses algorithms, modeling, and computational simulations to analyze and visualize biological data, from DNA sequences to intricate cell behaviors. Students dive deep into bioinformatics, genomics, and systems biology, becoming fluent in both biological nuances and computing prowess. Careers springboard to roles like bioinformatics analysts, genomic data scientists, and molecular modelers. As we race to unlock nature's codes, Computational Biology stands at the forefront, producing tech-savvy biologists who revolutionize our understanding of life.
Study Programs in English
Universities
Universities in International Rankings
€ 0 (12 programs for EU citizens/Non-EU)
€ 0 per semester (12 programs for EU citizens/Non-EU)
Winter Semester
between May 31 and May 15
Summer Semester
between July 15 and May 15
Top-ranked German Universities in Computational Biology

public University
No. of Students: approx. 38,000 students
Program Fees: € 0 (per semester)

No. of Students: approx. 27,000 students
Tuition Fees
3 english degree programs for computational biology in germany.
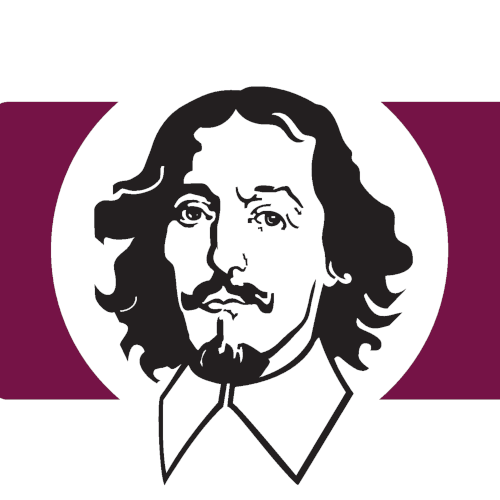
Otto von Guericke University Magdeburg Magdeburg
Advanced semiconductor nanotechnologies.
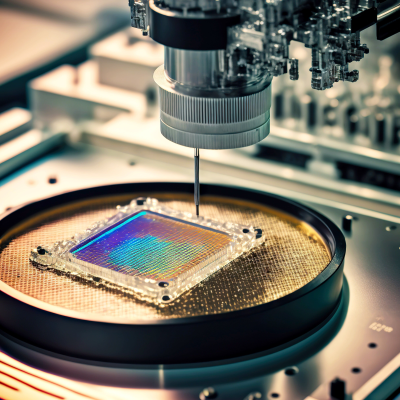
University of Cologne Cologne
Computational sciences.

Deggendorf Institute of Technology Deggendorf
Life science informatics.
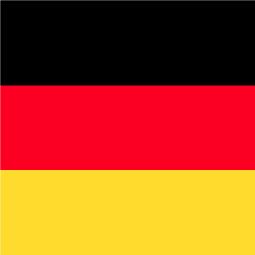
Application Deadlines
Winter Semester 2024/2025
Summer Semester 2025
Winter Semester 2025/2026
Open Programs
10 programs
12 programs
Application Modes
Application process.
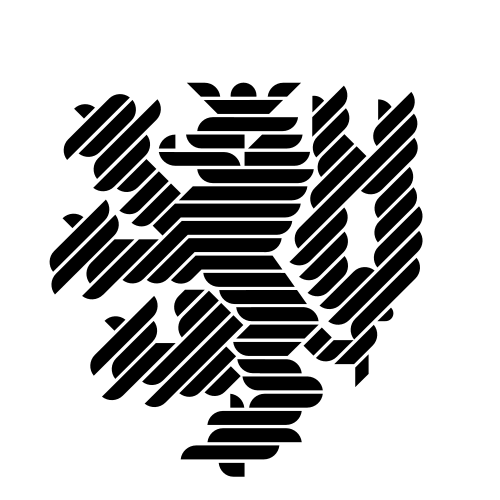
University of Wuppertal Wuppertal
Computer simulation in science (csis).
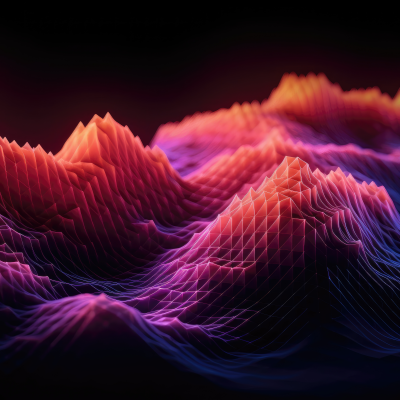
Technische Universität Dresden Dresden
Physics of life.
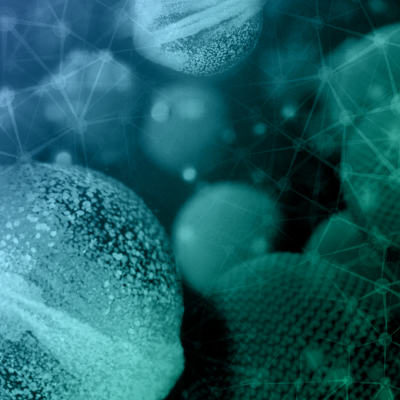
Justus Liebig University Giessen Giessen
Mind, brain and behavior.
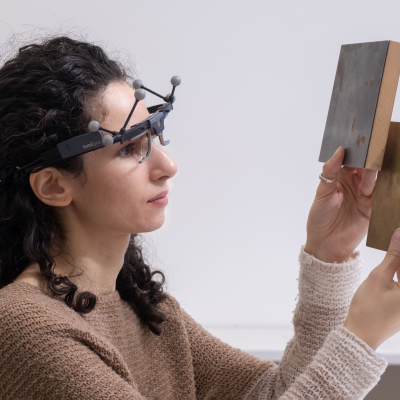
TOEFL Scores
Cambridge Levels
5.5 (1 program )
72 (1 program )
B2 First (FCE) (2 programs )
7 (1 program )
95 (2 programs )
C1 Advanced (CAE) (4 programs )
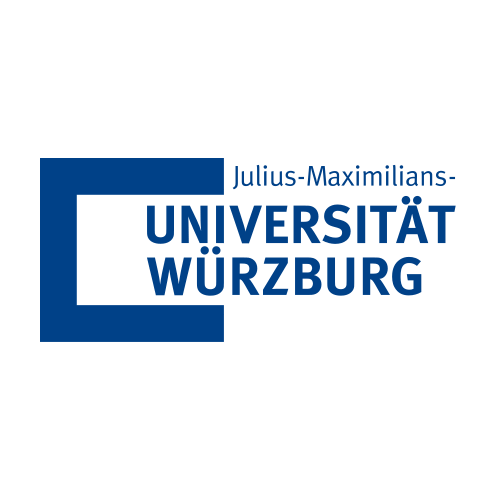
Julius-Maximilians-Universität Würzburg Würzburg
Molecular and computational biology.
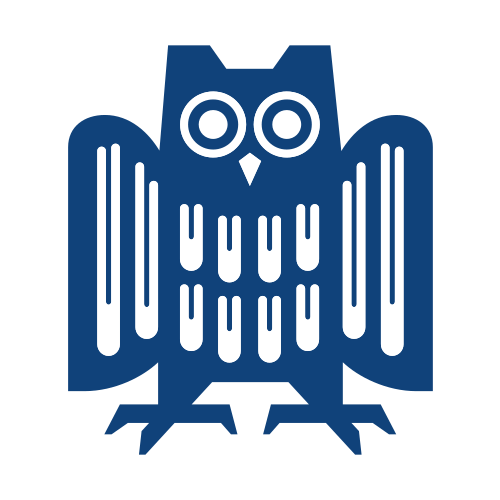
Saarland University Saarbrücken
Bioinformatics.

University of Göttingen Göttingen
Computational biology and bioinformatics.
3-4 semesters
→ View all programs with online courses
Master of Science
Winter intake
Summer intake
Winter & Summer intake
List of all German Universities offering English-taught Study Programs in Computational Biology

Deggendorf Institute of Technology
Program Fees: € 0
M.Sc. (Master of Science)

Freie Universität Berlin
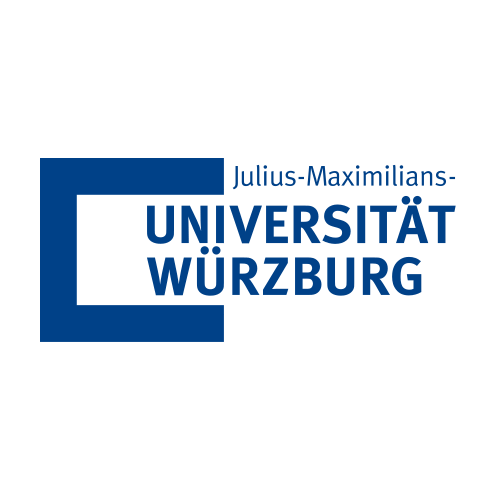
Julius-Maximilians-Universität Würzburg

Justus Liebig University Giessen
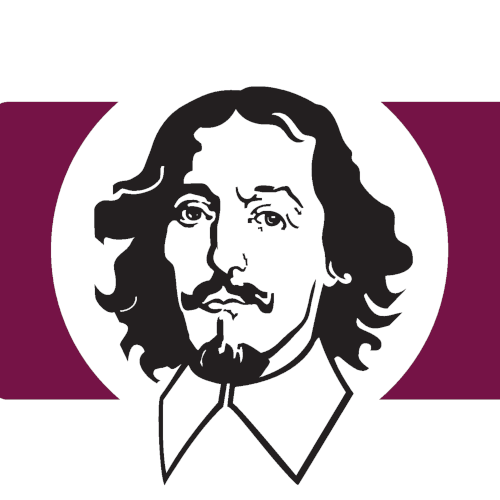
Otto von Guericke University Magdeburg
← Prev page
Next Page →
News & Articles
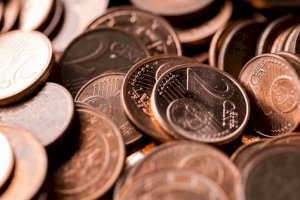
Tuition-free Universities in Germany in English
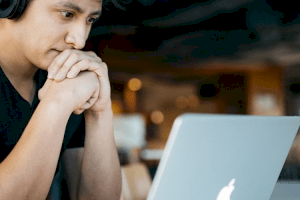
Master's Requirements in Germany
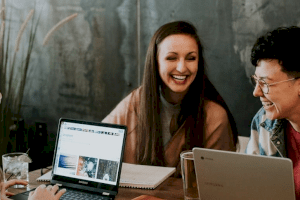
Scholarships for international students (2024/25)
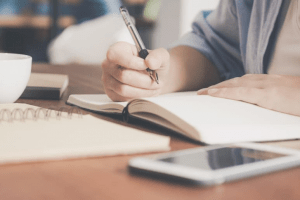
Uni-assist: A guide for international students (2024)
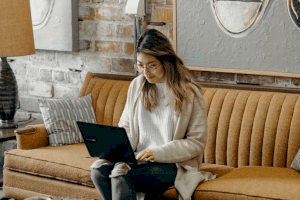
How Much Does it Cost to Live in Germany?
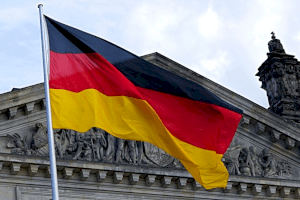
Germany in University Rankings

DAAD Scholarships: Guide
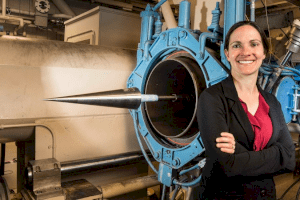
Engineering Universities in Germany: A Guide 2024/25
- Postdoc India
- Postdoc Abroad
- Postdoc (SS)
- RESEARCHERSJOB
- Post a position
- JRF/SRF/Project
- Science News
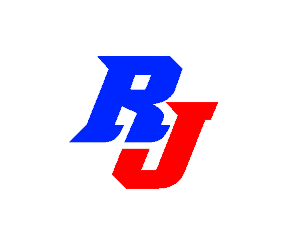
International PhD Positions: Computational Biology, Leibniz Institute, Germany
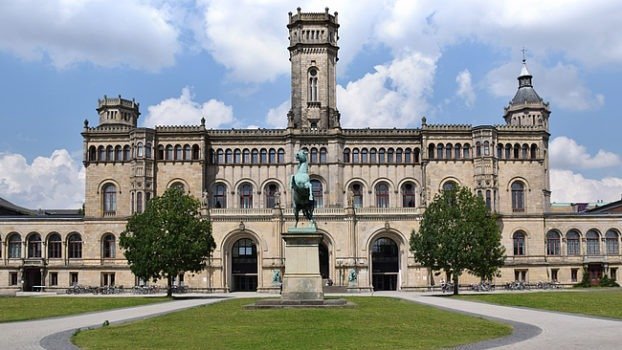
PhD Positions: Computational Biology: Join our dynamic team in Computational Biology! We are currently seeking motivated candidates for various positions, including a bioinformatician role and international PhD openings. Additionally, if you have your own funding or are interested in applying for fellowships, we welcome collaborations in developing hybrid projects. Explore the intersection of aging, microbiome, and computational biology with us and contribute to cutting-edge research in an innovative environment.
Study Area: Computational Biology, Bioinformatics, Computer Science, Biology, Wetlab-Drylab Hybrid Projects
Location: Leibniz Institute, Germany
Eligibility/Qualification: For the bioinformatician position, candidates should hold a PhD in computational biology, bioinformatics, computer science, biology, or related fields. Exceptional candidates without a PhD but with significant hands-on experience will also be considered. Proficiency in R, Python, Bash, and version control is required, along with experience in high-throughput data preprocessing and analysis. Excellent communication skills in English are essential.
For international PhD positions, interested candidates should refer to the DAAD-supported openings and submit applications according to the institute’s guidelines. Candidates with backgrounds in computational biology or mixed wet-dry backgrounds are encouraged to apply. PhD and postdoc candidates with their own funding or interest in applying for fellowships are welcome to explore opportunities for collaboration.
Description: As a bioinformatician in our team, you will be responsible for establishing and running computational pipelines for preprocessing and integrating datasets from various high-throughput experiments. You will collaborate with other computational biologists and experimentalists to support research data management and collaborative projects. For international PhD applicants, positions are available with DAAD support across multiple groups, including ours. We encourage applicants with computational biology backgrounds or mixed wet-dry backgrounds to apply and list our group as a preferred choice.
If you have your own funding or are interested in applying for fellowships, we invite you to collaborate with us on developing hybrid projects at the intersection of aging, microbiome, and computational biology. Prestigious fellowship opportunities such as MSCA Postdoctoral Fellowships, EMBO Postdoctoral Fellowships, and HFSP LTF are available, requiring high knowledge transfer. We welcome postdoctoral candidates from non-biological disciplines as well.
How to Apply: Interested candidates for the bioinformatician position should check the ad on our institute website and submit their applications according to the provided instructions. For international PhD positions, applicants should refer to the institute’s website for more information and submit applications accordingly. Candidates with their own funding or interested in fellowships should contact Melike directly with their CV, cover letter, references, Google Scholar link, and a brief project description.
- For the bioinformatician position, the application deadline is May 5th, 2024.
- For international PhD positions, the application deadline is May 12th, 2024.
- For fellowship opportunities, please refer to specific fellowship deadlines mentioned in the description.
RELATED ARTICLES MORE FROM AUTHOR
Phd position: pathogenic bacteria, rub, germany, 8 phd positions in economics – 40th cycle, università degli studi di verona, italy, phd position: quantum materials, universität zu köln, germany, phd position: multiscale modelling, university of amsterdam (uva), amsterdam, netherlands, phd/postdoc position, tu/e, netherlands, 51 phd positions (40th cycle) at scuola normale superiore, italy, leave a reply cancel reply.
Save my name, email, and website in this browser for the next time I comment.
Follow us on Instagram @researchersjob_rj
- Terms Of Service
- Privacy Policy
HFSP Postdoctoral Fellowships in Life Sciences

319 computational-biology-phd positions in Germany
Filtered by.
- computational-biology-phd
Refine Your Search
- Last-3-days 10
- Last-7-days 9
- Last-30-days 53
- Scholarship 198
- Research Job 72
- Postdoctoral 61
- Postgraduate 6
- Fellowship 3
- International PhD Programme (IPP) Mainz 24
- Technical University of Munich 20
- University of Göttingen • 15
- Forschungszentrum Jülich 10
- Heidelberg University 9
- Nature Careers 8
- Helmholtz Zentrum München - Deutsches Forschungszentrum für Gesundheit und Umwelt 7
- Alfred-Wegener-Institut Helmholtz-Zentrum für Polar- und Meeresforschung 5
- Helmholtz-Zentrum Dresden-Rossendorf - HZDR - Helmholtz Association 5
- Jena School for Microbial Communication (JSMC) 5
- Johannes Gutenberg University Mainz 5
- Ludwig-Maximilians-Universität München • 5
- Academic Europe 4
- Helmholtz 4
- Helmholtz-Zentrum für Infektionsforschung 4
- Leibniz-Institut für Agrartechnik und Bioökonomie e.V. 4
- University of Tübingen • 4
- Deutsches Zentrum für Neurodegenerative Erkrankungen e. V. (DZNE) 3
- HBIGS Heidelberg Biosciences International Graduate School 3
- Helmholtz-Zentrum Potsdam - Deutsches GeoForschungsZentrum GFZ 3
- Max Planck Institute of Animal Behavior, Radolfzell / Constance 3
- Max Planck Institutes 3
- Constructor University Bremen gGmbH 2
- Deutsches Elektronen-Synchrotron DESY 2
- Deutsches Krebsforschungszentrum 2
- Dresden University of Technology • 2
- Fraunhofer-Gesellschaft 2
- Free University of Berlin 2
- Georg August University of Göttingen 2
- Hannover Medical School • 2
- Heidelberg University • 2
- Leibniz Institute of Vegetable and Ornamental Crops 2
- Max Planck Institute for Multidisciplinary Sciences, Göttingen 2
- Max-Delbrück-Centrum für Molekulare Medizin in der Helmholtz-Gemeinschaft 2
- Ruhr-Universität Bochum • 2
- University of Bonn 2
- University of Cologne • 2
- University of Goettingen 2
- University of Greifswald 2
- University of Konstanz • 2
- University of Luebeck 2
- University of Tübingen 2
- Universität Hamburg • 2
- ; German Cancer Research Center (DKFZ) 1
- Berliner Hochschule für Technik 1
- Biano GMP 1
- Carl von Ossietzky University of Oldenburg • 1
- Charité - Universitätsmedizin Berlin • 1
- Chemie HU Berlin 1
- Christian-Albrechts-Universitaet zu Kiel 1
- Clausthal University of Technology 1
- Cologne Graduate School of Ageing Research 1
- DKFZ (German Cancer Research Center) 1
- Deutsches Elektronen-Synchrotron DESY • 1
- Deutsches Zentrum für Luft - und Raumfahrt (DLR) 1
- Experimental Physics I, University of Bayreuth, Germany 1
- FAU Erlangen-Nürnberg • 1
- FBN Dummerstorf 1
- Free University Berlin 1
- Friedrich Schiller University Jena • 1
- GSI Helmholtzzentrum für Schwerionenforschung 1
- Georg-August-Universität Göttigen 1
- Heidelberg University Hospital 1
- Heinrich Heine University Düsseldorf 1
- Heinrich Heine University Düsseldorf • 1
- Helmholtz Munich 1
- Helmholtz-Centre for Environmental Research, UFZ, Multiple Stressor Ecology group of the Department of Ecotoxicology 1
- Helmholtz-Zentrum für Umweltforschung - UFZ 1
- Humboldt-Universität zu Berlin 1
- Institute of Freshwater Ecology and Inland Fisheries 1
- Interherence GmbH 1
- Johannes Gutenberg University Mainz • 1
- Justus Liebig University 1
- Justus Liebig University Giessen • 1
- Justus Liebig University Giessen, Institute of Anatomy and Cell Biology 1
- Karlsruhe Institute of Technology • 1
- Karlsruher Institut für Technologie (KIT) 1
- LIMES Institute, University of Bonn 1
- LMU Klinikum 1
- Leibniz Institute for Food Systems Biology at the Technical University of MunichFood Systems Biology at the Technical University of Munich 1
- Leibniz Institute for Natural Product Research and Infection Biology - Hans Knöll Institute • 1
- Leibniz Institute for Natural Products Research and Infection Immunology 1
- Leibniz Institute on Aging – Fritz Lipmann Institute (FLI) 1
- Leibniz-Forschungsinstitut für Molekulare Pharmakologie (FMP) im Forschungsverbund Berlin 1
- Leibniz-Institut für Alternsforschung – Fritz-Lipmann-Institut e.V. (FLI) 1
- Leibniz-Institut für Analytische Wissenschaften - ISAS - e.V. 1
- Max Planck Institute for Biogeochemistry, Jena 1
- Max Planck Institute for Biological Intelligence • 1
- Max Planck Institute for Biology of Ageing, Köln 1
- Max Planck Institute for Chemical Ecology • 1
- Max Planck Institute for Chemical Ecology, Jena 1
- Max Planck Institute for Evolutionary Biology, Plön 1
- Max Planck Institute for Infection Biology • 1
- Max Planck Institute for Infection Biology, Berlin 1
- Max Planck Institute for Plant Breeding Research • 1
- Max Planck Institute for Software Systems • 1
- Biology 154
- Computer Science 42
- Medical Sciences 31
- Linguistics 17
- Chemistry 9
- Economics 7
- Mathematics 7
- Environment 5
- Earth Sciences 3
- Arts and Literature 2
- Materials Science 2
- Engineering 1
- Psychology 1
- Social Sciences 1
PhD student computational biology / bioinformatics (m/f/d) (ID: 24264)
programme Is the Job related to staff position within a Research Infrastructure? No Offer Description PhD student computational biology / bioinformatics (m/f/d) (fulltime) The position will be hosted
PhD Student Position in Computational Biology – Somatic Evolution of Cancer Development
https://jobs.dkfz.de/en/jobs/166580/ phd -student-position-in- computational -biolo… Work Location(s) Number of offers available1Company/InstituteDeutsches
PhD student
within a Research Infrastructure? No Offer Description PhD Project PhD position in biology and virulence of the human malaria parasite Plasmodium falciparum (Lanzer_0124) - Heidelberg University. Details
PhD positions in Structural Biology /Protein Biochemistry
related to staff position within a Research Infrastructure? No Offer Description Two PhD positions in Structural Biology /Protein Biochemistry are available in the research group of Prof. Eva Wolf
(Africa/Abidjan) Type of Contract Not Applicable Job Status Not Applicable Is the job funded through the EU Research Framework Programme ? Not funded by an EU programme Is the Job related to staff position
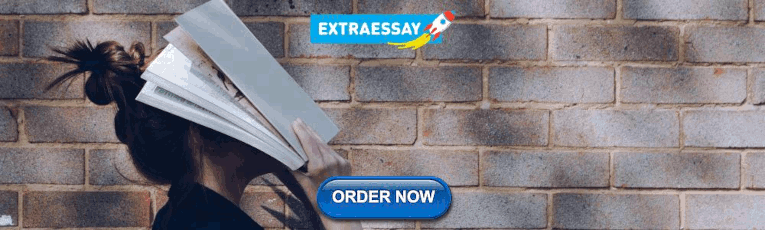
DAAD PhD Scholarship in novel drug delivery to cancer cells for Students from Developing Countries
13 May 2024 Job Information Organisation/Company University of Luebeck Department Hematology and Oncology Research Field Biological sciences » Biology Researcher Profile First Stage Researcher (R1
PhD Positions in Cancer Research
School Scholarship Program . Research at the DKFZ is organized into the following programs with a lot of interaction and interdisciplinary PhD projects available across different topics: Cell Biology and
PhD Researcher Positions (f/m/d)
PhD Positions (f/m/d) Yeast Synthetic Biology Humboldt-Universität zu Berlin - Germany Location: Institute for Biology , Humboldt-Universität Zu Berlin, Berlin The newly established Emmy Noether
PhD position in the Biochemistry of Gene Regulation Group “Exploring the Molecular Basis of Transcription Factor Evolution"
leveraging the experimental versatility of yeast and the recent advances in computational biology . Experimentally, we combine classical genetics/ cloning with CRISPR-Cas9-based gene editing as well as omics
PhD Position - Bacterial antiviral immunity
Your Job: The MOI-V Manchot Graduate School`s research programme focuses on the functional characterisation of molecules that are essential for infections with a wide variety of pathogens
Searches related to computational biology phd
- phd computational biology
- bioinformatics
- computational biology
- bioinformatics phd
- phd bioinformatics
- machine learning phd
- system biology phd
- structural bioinformatics
By using the Google™ Search you agree to Google's privacy policy
Computational Biology and Bioinformatics (M.Sc.)
- Application
This international study programme provides research-oriented, interdisciplinary training at the interface between computer science and biology. Core fields are bioinformatics and systems biology, meaning the analysis and modelling of data in molecular biology and biochemistry, in particular from genomics, transcriptomics, proteomics and metabolomics. Graduates will have a strong biological background as well as being able to understand and develop software for the handling and analysis of biological data.
Get to know us

Programme homepage

Customise your degree
Programmes and options

Student life
Living, finances and culture

Study and internships abroad
Expand your horizons
The master‘s programme comprises the subject areas "Algorithms in Bioinformatics“, „Biological Data Mining“, „Computational and Functional Gemonics“, "Evolutionary Bioinformatics and Phylogeny“, „Bayesian Statistics and Infomation Theory“ as well as „Machine Learning“.
Based on their previous education students attend basic courses in biology or informatics during the first semester and receive an overview of the different areas of application for bioinformatics. Computational methods are supported by practical trainings in the wet lab. Advanced courses will focus on how these methods are used to answer current biological questions.
Related programmes
- Molecular Life Sciences: Microbiology, Biotechnology and Biochemistry (M.Sc.)
- Computer Science (M.Sc.)
- Applied Data Science (M.Sc.)
The research-oriented master’s programme typically follows a bachelor’s degree in Biology, Bioinformatics or Informatics. Fundamental knowledge of the subject and its methods are first conveyed in bridging modules. Later on students select courses within the core disciplines of the programme. Key competence modules additionally convey further occupational qualifying competencies.
Core Studies (54 ECTS)
- Bridging Coursese (10 C)
- Bioinformatics and its areas of application (8 C)
- Elective Courses ‚Bioinformatics‘ (24 C)
- Elective Courses ‚Biology‘ (12 C)
Professionalization (36 ECTS)
- Advanced Course in Computational Biology (12 C)
- Elective Courses ‚Informatics‘ (12 C)
- Key competencies (12 C)
Master’s Thesis (30 ECTS)
Regulations and module directory.
- Current und older versions
Qualifications
- major in Biology: at least 50 C in Biology and 40 C in Bioinformatics, Informatics, Mathematics or Physics
- major in in Bioinformatics or Computer Sciences: at least 30 C in Biology and Bioinformatics 60 C in Informatics, Mathematics, Physics or Bioinformatics
- proficiency in English level C1 or higher
- succesful completion of the assesment test
Study and examination advice
Office of Studies
Wilhelm-Weber-Str. 2 37073 Göttingen
Modal title
Computational Health Center Institute of Computational Biology
The Institute of Computational Biology (ICB) develops models and methods for data analysis in systems biology and systems medicine. We integrate information on multiple scales, ranging from single-cell time series to cohort omics data. Heterogeneities in the data are modeled using statistical and dynamical approaches, both on the cellular and on the population level.
Click & View
We develop innovative methods and tools in
- data sciences
- dynamical modeling
- integrative omics and
- bioimage informatics
and apply them collaboratively with our experimental partners to
- modeling stem cell decisions
- modeling transcriptional heterogeneities and
- quantifying gene environment effects in diabetes and lung disease
to jointly advance our understanding and treatment of human diseases.
Ahmidi Group
Colomé-tatché group, fuchs group, gagneur group, hasenauer group, heinig group, jüstel group, kastenmüller group, knauer-arloth group, marsico group, menden group, c.l. müller group, schubert group, scialdone group, talavera-lópez group, operations & scientific management.
Dr. Anna Sacher
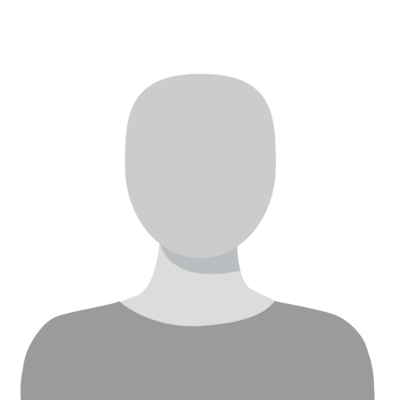
Dr. Julia Schlehe
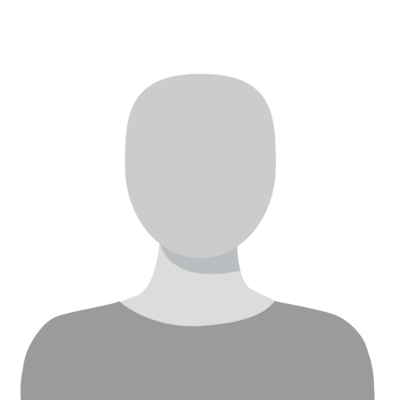
Daniela Herrmann
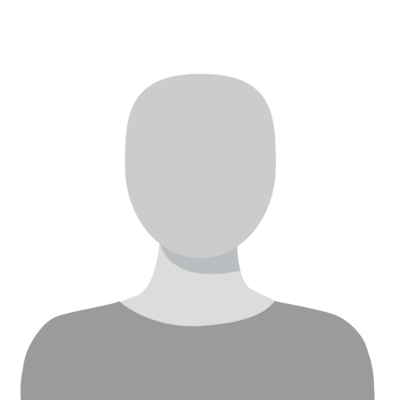
Karina Kahl
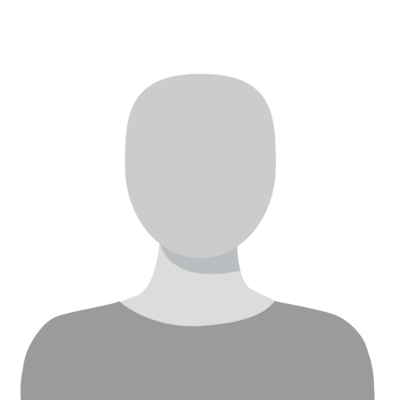
Sabine Kunz
Director of Operations
- Telephone: +49 89 3187-2926
- E-mail: Email me
Privacy settings
We use cookies to improve your experience on our Website. We need cookies to continually improve our services, enable certain features, and when we embed third-party services or content, such as the Vimeo video player. In such cases, information may also be transferred to third parties. By using our website, you agree to the use of cookies. We use different types of cookies. You can personalize your cookie settings here:
PhD student computational biology / bioinformatics (m/f/d) (ID: 24264)
Job information, offer description.
PhD student computational biology / bioinformatics (m/f/d) (fulltime)
The position will be hosted at the Institute of Human Genetics, in the workgroup "Regulatory Genomics" of Prof. Martin Kircher. The bioinformatics group performs research in the fields of sequence analysis, data mining, machine learning and functional genomics ( https://kircherlab.github.io/ ). Our research focuses on computational approaches of identifying functionally relevant genetic changes in disease and adaptation as well as developing more sensitive methods in diagnostics (especially exome, genome and cell-free DNA sequencing). For example, we devise computational approaches (e.g. CADD, CADD-SV, ReMM) to score and identify functional genetic changes in the human genome through Massively Parallel Reporter Assays and genome pertubation. Understanding how gene regulation is encoded in our genomes across development and the diversity of cell-types is one of our fundamental research questions.
Start in our team
The position is immediately available. The full-time contract will be issued for 3 years with the prospect of extension to finish the thesis work and preparation of related publications.
- A collaborative research environment that encourages and supports scientific curiosity, innovation, and development of its team members
- Training in computational biology and independent research, as well as receiving guidance on PhD requirements and thesis work
- Career development and collaboration with internal and external partners
- Participation in international meetings and scientific conferences
- Salary based full-time payment on the German E13 TV-L scale, if terms and conditions under collective bargaining law are fulfilled. This for example also includes 30 paid vacation days per year.
- Flexible working hours and a family-friendly environment
Your responsibilities:
- Work on cutting-edge projects using state-of-the-art technology and gain insights into the sequence encoding of molecular function and its relation to disease as well as epigenetic and 3D genome annotations
- Develop deep neural nets or classical machine learning models that integrate sequence features and molecular measures
- Develop or improve methods to infer disease and regulatory mechanisms and develop data standards as well as prepare data for analysis or computational modeling
- Analyze high-throughput sequencing data and develop custom computational tools,
- Apply and improve methods for model interpretation, and actively contribute to the lab, institute and international collaborations
Requirements:
- Master degree or equivalent in bioinformatics, computational biology or similar, alternatively in computer sciences with experience in molecular biology and large-scale data analysis
- Basic knowledge of molecular biology, gene regulation and epigenetics as well as deep understanding of sequence analysis, statistical concepts and machine learning
- Highly skilled in a programming language such as Python, R, C++, or Java and intermediate skills in a statistical modeling or analysis environment like R or Python scipy/scikit learn
- Prior experience with Unix and bash scripting, previous exposure to HPC environments is an advantage
- Exposure to reproducible work and FAIR principles
- Highly motivated to expand knowledge and develop innovative approaches as well as diligent, goal-oriented and independent work attitude
- Team-oriented with interdisciplinary communication skills and very good English language skills
We are looking forward to your application under the following Link: PhD student computational biology / bioinformatics (m/f/d) (uksh.de) . Please submit your application until 05.06.2024, indicating your earliest possible starting date as well as the reference number 24264.
If you have any questions, do not hesitate to contact Prof. Martin Kircher: [email protected]
We live diversity and strongly encourage qualified female scientists to apply. UKSH and the University of Lübeck aim to increase the number of women among faculty and staff. Applications from people with an immigrant background who meet the hiring requirements are encouraged. Disabled candidates who are equally qualified will receive preference.
Requirements
Additional information, work location(s), where to apply.
UNIVERSITY OF COLOGNE
change language Deutsch Deutsch
Faculty of Mathematics and Natural Sciences Department of Biology
Computational biology.
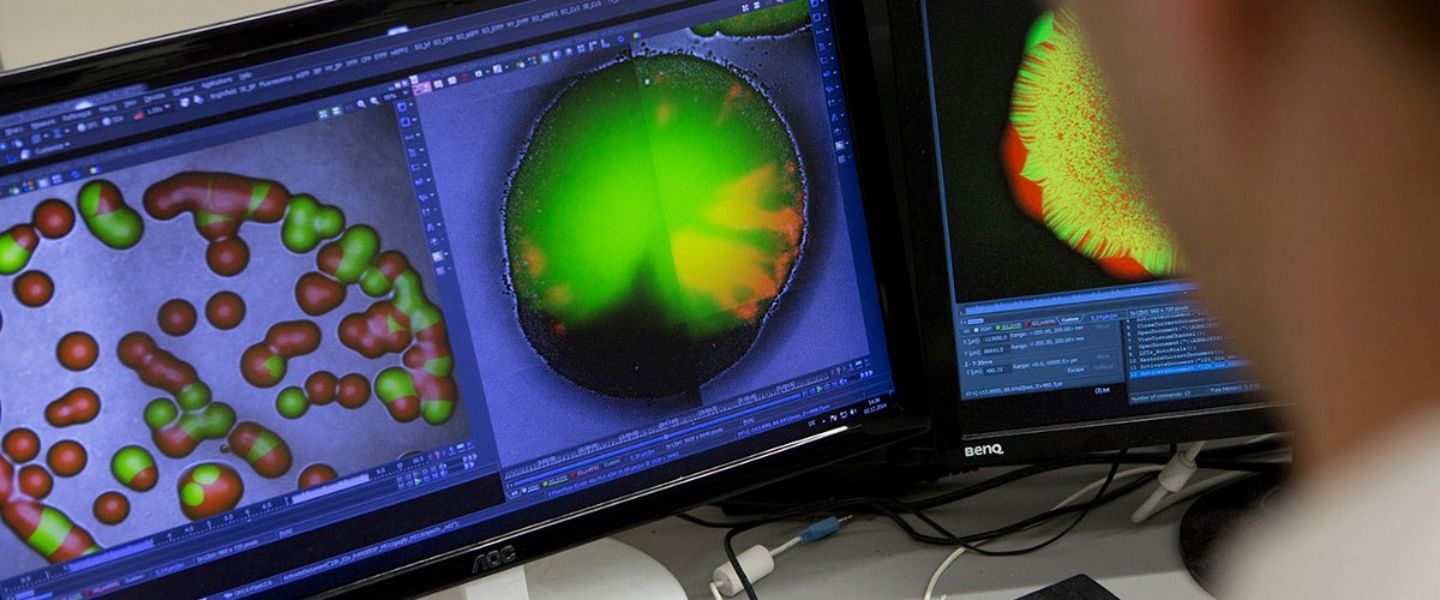
Graduate competencies:
Graduates will be able to identify open questions in the areas of computational and quantitative biology, conduct own or collaborative research projects, and communicate their findings in written reports or oral presentations. The master degree in computational biology will offer a wide range of career opportunities within or outside of academia. These range from continued education in doctoral programs to professional careers in the life science industry, non-governmental organizations, science management or communication and scientific outreach.
Contact Information
For detailed information about the study program "Computational Biology", please contact:
For general information about the M.Sc. program, please contact: [email protected]
Biological Sciences
- Mellon College of Science
M.S. in Quantitative Biology and Bioinformatics
The study of Biology is undergoing a revolution driven by new technologies that enable scientists to generate extensive amounts of data. For example, the costs of sequencing nucleic acids have dropped dramatically, resulting in unprecedented amounts of genomic, transcriptomic, and proteomic data. Advances in imaging extend from the nano to the macro scale to probe function and generate enormous amounts of data that describe behaviours of cells from subcellular to organ-levels. The new datasets cut across all subdisciplines in biology and enable scientists to ask questions in new ways to reveal the fundamental rules of life.
The M.S. in Quantitative Biology and Bioinformatics (MS-QBB) will prepare students for new careers bioinformatics and related fields. Our mission is to provide students who have background in life sciences skills to prepare for careers in bioinformatics. This program allows student to choose a 2-semester or a 3-semester program of study. If you are interested in applying, learn more about the application process on our admissions page or e-mail us .
Program Mission
To provide students who have a background in biology and other sciences with a practical and focused educational experience to prepare them for careers in bioinformatics and quantitative biological science.
2-semester M.S. in QBB
Our 2-semester option allows students to quickly gain the most relevant skills in bioinformatics. Students will begin study in late August and graduate in late May.
3-semester M.S. in QBB - Advanced Study
The 3-semester option allows students to spend a third semester gaining additional experience and some more advanced coursework. Students will begin study in late August, have the option to earn course credit with optional summer internships (interested students may apply to these in the first year), then students will complete their third semester in the following Fall and graduate in late December.
Students are encouraged to seek external internships after their first year and pursue this degree full-time, completing the program in 3 semesters.
Related programs
Students who are interested in this program may also want to consider the M.S. in Computational Biology and M.S. in Automated Science programs . Those programs expect a higher level of quantitative background & skills to enter and are designed to engage students with a more in-depth focus computational machine learning competencies and the application of machine learning to biological research.
How to Apply
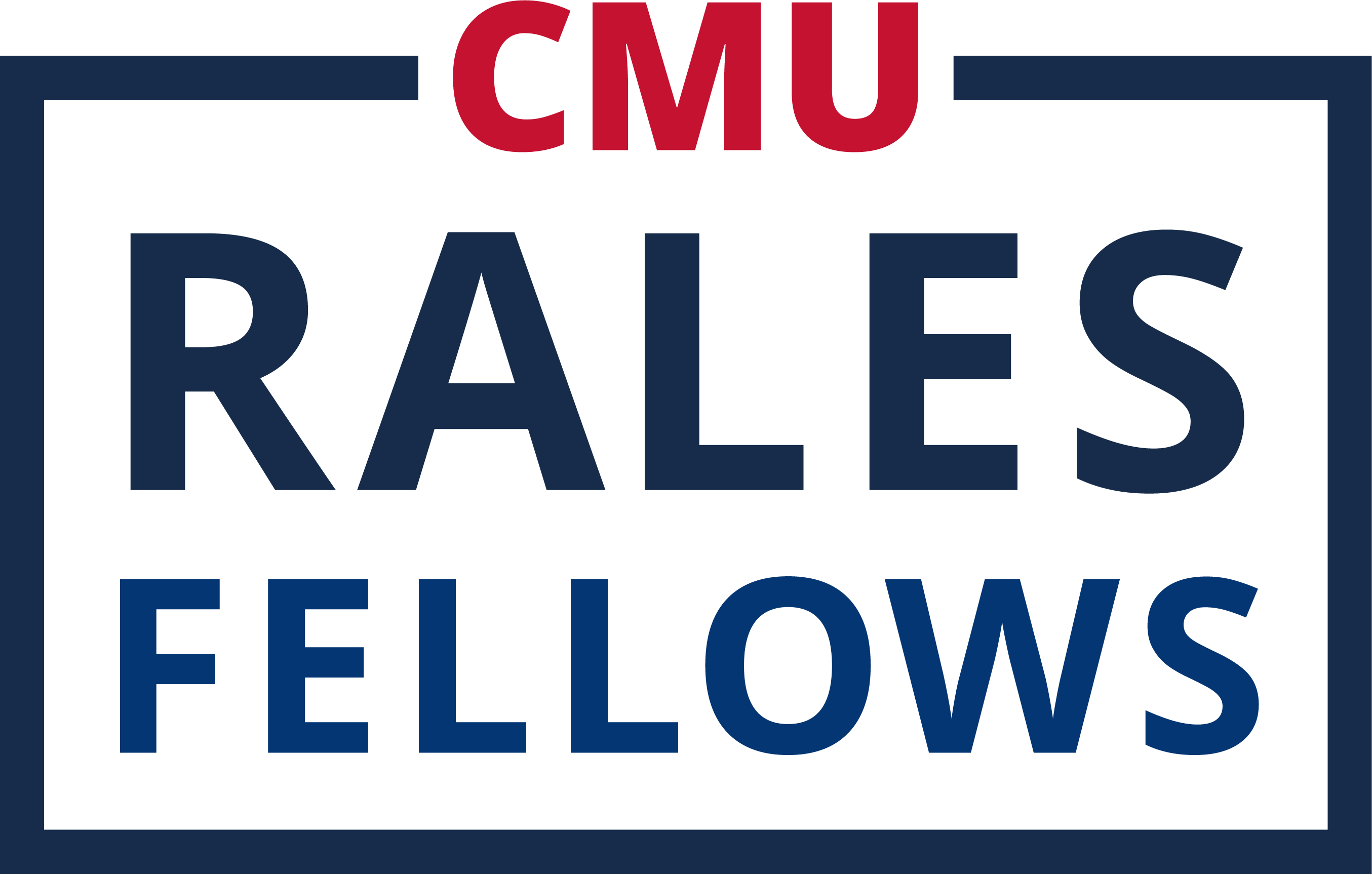
The CMU Rales Fellow Program is dedicated to developing a diverse community of STEM leaders from underrepresented and underresourced backgrounds by eliminating cost as a barrier to education. Learn more about this program for master's and Ph.D. students. Learn more
Join our growing network of prospective students!
Fill in the form below to connect with a program advisor
- Graduate Students
- Administration
Facility for Rare Isotope Beams
At michigan state university, international research team uses wavefunction matching to solve quantum many-body problems, new approach makes calculations with realistic interactions possible.
FRIB researchers are part of an international research team solving challenging computational problems in quantum physics using a new method called wavefunction matching. The new approach has applications to fields such as nuclear physics, where it is enabling theoretical calculations of atomic nuclei that were previously not possible. The details are published in Nature (“Wavefunction matching for solving quantum many-body problems”) .
Ab initio methods and their computational challenges
An ab initio method describes a complex system by starting from a description of its elementary components and their interactions. For the case of nuclear physics, the elementary components are protons and neutrons. Some key questions that ab initio calculations can help address are the binding energies and properties of atomic nuclei not yet observed and linking nuclear structure to the underlying interactions among protons and neutrons.
Yet, some ab initio methods struggle to produce reliable calculations for systems with complex interactions. One such method is quantum Monte Carlo simulations. In quantum Monte Carlo simulations, quantities are computed using random or stochastic processes. While quantum Monte Carlo simulations can be efficient and powerful, they have a significant weakness: the sign problem. The sign problem develops when positive and negative weight contributions cancel each other out. This cancellation results in inaccurate final predictions. It is often the case that quantum Monte Carlo simulations can be performed for an approximate or simplified interaction, but the corresponding simulations for realistic interactions produce severe sign problems and are therefore not possible.
Using ‘plastic surgery’ to make calculations possible
The new wavefunction-matching approach is designed to solve such computational problems. The research team—from Gaziantep Islam Science and Technology University in Turkey; University of Bonn, Ruhr University Bochum, and Forschungszentrum Jülich in Germany; Institute for Basic Science in South Korea; South China Normal University, Sun Yat-Sen University, and Graduate School of China Academy of Engineering Physics in China; Tbilisi State University in Georgia; CEA Paris-Saclay and Université Paris-Saclay in France; and Mississippi State University and the Facility for Rare Isotope Beams (FRIB) at Michigan State University (MSU)—includes Dean Lee , professor of physics at FRIB and in MSU’s Department of Physics and Astronomy and head of the Theoretical Nuclear Science department at FRIB, and Yuan-Zhuo Ma , postdoctoral research associate at FRIB.
“We are often faced with the situation that we can perform calculations using a simple approximate interaction, but realistic high-fidelity interactions cause severe computational problems,” said Lee. “Wavefunction matching solves this problem by doing plastic surgery. It removes the short-distance part of the high-fidelity interaction, and replaces it with the short-distance part of an easily computable interaction.”
This transformation is done in a way that preserves all of the important properties of the original realistic interaction. Since the new wavefunctions look similar to that of the easily computable interaction, researchers can now perform calculations using the easily computable interaction and apply a standard procedure for handling small corrections called perturbation theory. A team effort
The research team applied this new method to lattice quantum Monte Carlo simulations for light nuclei, medium-mass nuclei, neutron matter, and nuclear matter. Using precise ab initio calculations, the results closely matched real-world data on nuclear properties such as size, structure, and binding energies. Calculations that were once impossible due to the sign problem can now be performed using wavefunction matching.
“It is a fantastic project and an excellent opportunity to work with the brightest nuclear scientist s in FRIB and around the globe,” said Ma. “As a theorist , I'm also very excited about programming and conducting research on the world's most powerful exascale supercomputers, such as Frontier , which allows us to implement wavefunction matching to explore the mysteries of nuclear physics.”
While the research team focused solely on quantum Monte Carlo simulations, wavefunction matching should be useful for many different ab initio approaches, including both classical and quantum computing calculations. The researchers at FRIB worked with collaborators at institutions in China, France, Germany, South Korea, Turkey, and United States.
“The work is the culmination of effort over many years to handle the computational problems associated with realistic high-fidelity nuclear interactions,” said Lee. “It is very satisfying to see that the computational problems are cleanly resolved with this new approach. We are grateful to all of the collaboration members who contributed to this project, in particular, the lead author, Serdar Elhatisari.”
This material is based upon work supported by the U.S. Department of Energy, the U.S. National Science Foundation, the German Research Foundation, the National Natural Science Foundation of China, the Chinese Academy of Sciences President’s International Fellowship Initiative, Volkswagen Stiftung, the European Research Council, the Scientific and Technological Research Council of Turkey, the National Natural Science Foundation of China, the National Security Academic Fund, the Rare Isotope Science Project of the Institute for Basic Science, the National Research Foundation of Korea, the Institute for Basic Science, and the Espace de Structure et de réactions Nucléaires Théorique.
Michigan State University operates the Facility for Rare Isotope Beams (FRIB) as a user facility for the U.S. Department of Energy Office of Science (DOE-SC), supporting the mission of the DOE-SC Office of Nuclear Physics. Hosting what is designed to be the most powerful heavy-ion accelerator, FRIB enables scientists to make discoveries about the properties of rare isotopes in order to better understand the physics of nuclei, nuclear astrophysics, fundamental interactions, and applications for society, including in medicine, homeland security, and industry.
The U.S. Department of Energy Office of Science is the single largest supporter of basic research in the physical sciences in the United States and is working to address some of today’s most pressing challenges. For more information, visit energy.gov/science.
- News & Media
- Chemical Biology
- Computational Biology
- Ecosystem Science
- Cancer Biology
- Exposure Science & Pathogen Biology
- Metabolic Inflammatory Diseases
- Advanced Metabolomics
- Mass Spectrometry-Based Measurement Technologies
- Spatial and Single-Cell Proteomics
- Structural Biology
- Biofuels & Bioproducts
- Human Microbiome
- Soil Microbiome
- Synthetic Biology
- Computational Chemistry
- Chemical Separations
- Chemical Physics
- Atmospheric Aerosols
- Human-Earth System Interactions
- Modeling Earth Systems
- Coastal Science
- Plant Science
- Subsurface Science
- Terrestrial Aquatics
- Materials in Extreme Environments
- Precision Materials by Design
- Science of Interfaces
- Friction Stir Welding & Processing
- Dark Matter
- Flavor Physics
- Fusion Energy Science
- Neutrino Physics
- Quantum Information Sciences
- Emergency Response
- AGM Program
- Tools and Capabilities
- Grid Architecture
- Grid Cybersecurity
- Grid Energy Storage
- Earth System Modeling
- Energy System Modeling
- Transmission
- Distribution
- Appliance and Equipment Standards
- Building Energy Codes
- Advanced Building Controls
- Advanced Lighting
- Building-Grid Integration
- Building and Grid Modeling
- Commercial Buildings
- Federal Performance Optimization
- Resilience and Security
- Grid Resilience and Decarbonization
- Building America Solution Center
- Energy Efficient Technology Integration
- Home Energy Score
- Electrochemical Energy Storage
- Flexible Loads and Generation
- Grid Integration, Controls, and Architecture
- Regulation, Policy, and Valuation
- Science Supporting Energy Storage
- Chemical Energy Storage
- Waste Processing
- Radiation Measurement
- Environmental Remediation
- Subsurface Energy Systems
- Carbon Capture
- Carbon Storage
- Carbon Utilization
- Advanced Hydrocarbon Conversion
- Fuel Cycle Research
- Advanced Reactors
- Reactor Operations
- Reactor Licensing
- Solar Energy
- Wind Resource Characterization
- Wildlife and Wind
- Community Values and Ocean Co-Use
- Wind Systems Integration
- Wind Data Management
- Distributed Wind
- Energy Equity & Health
- Environmental Monitoring for Marine Energy
- Marine Biofouling and Corrosion
- Marine Energy Resource Characterization
- Testing for Marine Energy
- The Blue Economy
- Environmental Performance of Hydropower
- Hydropower Cybersecurity and Digitalization
- Hydropower and the Electric Grid
- Materials Science for Hydropower
- Pumped Storage Hydropower
- Water + Hydropower Planning
- Grid Integration of Renewable Energy
- Geothermal Energy
- Algal Biofuels
- Aviation Biofuels
- Waste-to-Energy and Products
- Hydrogen & Fuel Cells
- Emission Control
- Energy-Efficient Mobility Systems
- Lightweight Materials
- Vehicle Electrification
- Vehicle Grid Integration
- Contraband Detection
- Pathogen Science & Detection
- Explosives Detection
- Threat-Agnostic Biodefense
- Discovery and Insight
- Proactive Defense
- Trusted Systems
- Nuclear Material Science
- Radiological & Nuclear Detection
- Nuclear Forensics
- Ultra-Sensitive Nuclear Measurements
- Nuclear Explosion Monitoring
- Global Nuclear & Radiological Security
- Disaster Recovery
- Global Collaborations
- Legislative and Regulatory Analysis
- Technical Training
- Additive Manufacturing
- Deployed Technologies
- Rapid Prototyping
- Systems Engineering
- 5G Security
- RF Signal Detection & Exploitation
- Climate Security
- Internet of Things
- Maritime Security
- Artificial Intelligence
- Graph and Data Analytics
- Software Engineering
- Computational Mathematics & Statistics
- High-Performance Computing
- Visual Analytics
- Lab Objectives
- Publications & Reports
- Featured Research
- Diversity, Equity, Inclusion & Accessibility
- Lab Leadership
- Lab Fellows
- Staff Accomplishments
- Undergraduate Students
- Graduate Students
- Post-graduate Students
- University Faculty
- University Partnerships
- K-12 Educators and Students
- STEM Workforce Development
- STEM Outreach
- Meet the Team
- Internships
- Regional Impact
- Philanthropy
- Volunteering
- Available Technologies
- Industry Partnerships
- Licensing & Technology Transfer
- Entrepreneurial Leave
- Atmospheric Radiation Measurement User Facility
- Electricity Infrastructure Operations Center
- Energy Sciences Center
- Environmental Molecular Sciences Laboratory
- Grid Storage Launchpad
- Institute for Integrated Catalysis
- Interdiction Technology and Integration Laboratory
- PNNL Portland Research Center
- PNNL Seattle Research Center
- PNNL-Sequim (Marine and Coastal Research)
- Radiochemical Processing Laboratory
- Shallow Underground Laboratory
Connecting Computational and Systems Biology for Biodefense
Retooling security with bioagent-agnostic signatures
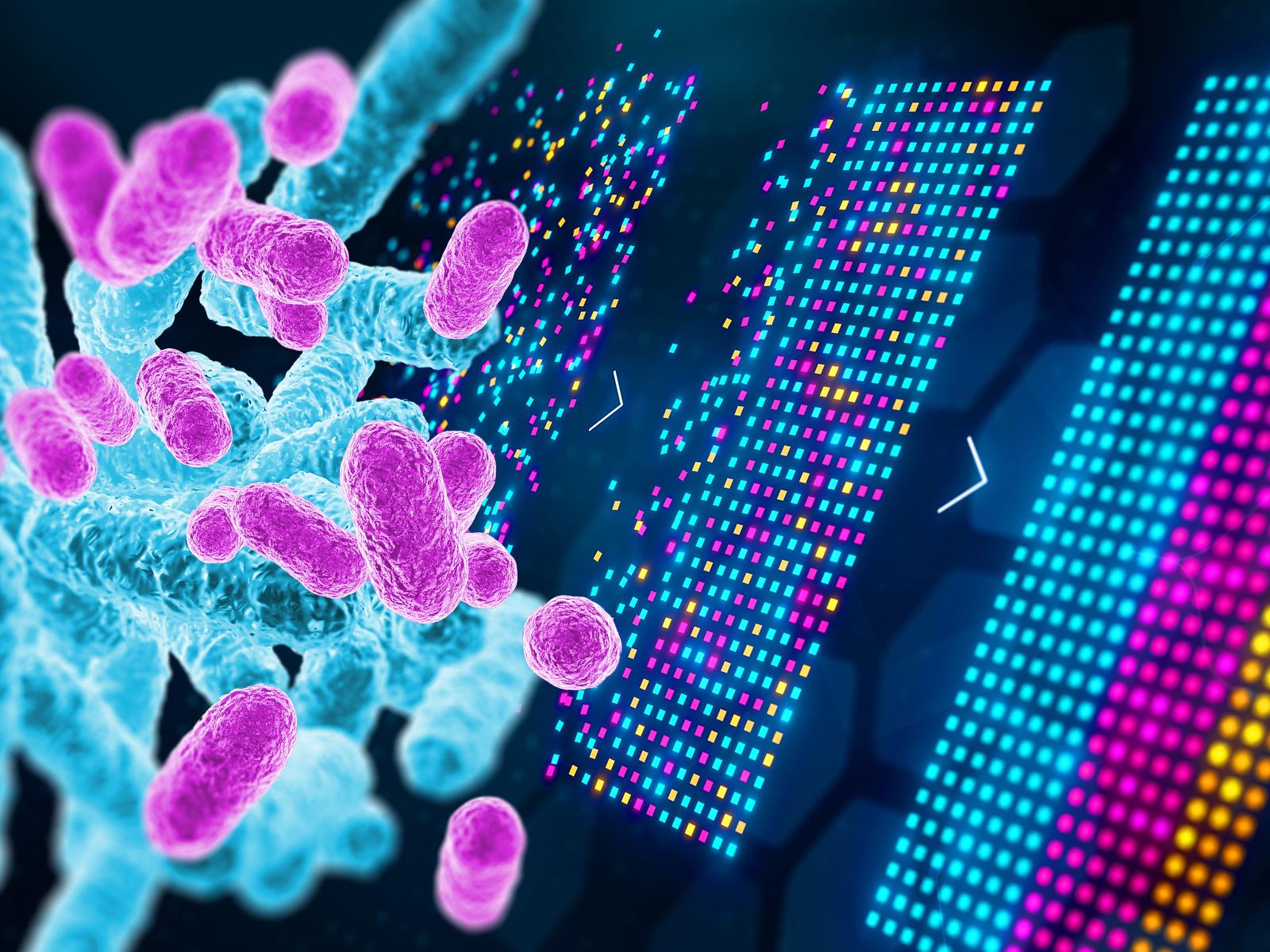
Researchers at Pacific Northwest National Laboratory and the University of Texas at El Paso are exploring the computational challenges of thinking beyond the list and developing bioagent-agnostic signatures to assess threats.
(Composite image by Derek Munson | Pacific Northwest National Laboratory)
Historically, the biodefense community relies on lists of known agents—pathogens and biotoxins like anthrax and ricin—that have been identified and prioritized as threats. In Health Security , a team of researchers at Pacific Northwest National Laboratory (PNNL) and the University of Texas at El Paso (UTEP) discuss the computational challenges of thinking beyond the list and developing bioagent-agnostic signatures to assess threats.
“As biological threats evolve, we face more unknowns and an increased sense of urgency to quickly detect and characterize disease agents to increase our biopreparedness and drive rapid responses. By shifting from an identification-based approach to a characterization-based one—with the right computational and data capabilities—we can accurately and reproducibly assess impacts without prior knowledge of an agent,” said Andy Lin, data scientist at PNNL.
The article “Computational and Systems Biology Advances to Enable Bioagent Agnostic Signatures” explores the computational data challenges of threat-agnostic biodefense , or the ability to characterize an unknown agent’s likely impact to human, animal, and plant health. The researchers discuss how the biodefense community can make the shift to a dual list-based and bioagent-agnostic signatures approach—but it will not be without its challenges. The shift will require policy changes, technological improvements, and improved data analytics.
The research brought together PNNL’s Lin, Errett Hobbs, Karen Taylor , Tony Chiang , and Jay Bardhan , with UTEP’s Cameron Torres, Stephen Aley, and Charles Spencer—convening diverse expertise across data science, computational systems biology, cytometry, immunology, and more. The research showcases the power of a cross-institution collaboration building on PNNL and UTEP’s long-standing partnership and joint appointment program to accelerate the science mission of both institutions. The UTEP team performed experiments that helped demonstrate the challenges discussed in the paper.
The research also builds on PNNL’s previous effort, highlighted in a 2021 Pathogens publication, “ Beyond the List: Bioagent-Agnostic Signatures Could Enable a More Flexible and Resilient Biodefense Posture Than an Approach Based on Priority Agent Lists Alone ,” which addressed how traditional list-based approaches are ill-equipped to accommodate threats posed by emergent, reemergent, or novel pathogens. A threat-agnostic model could present a means to more effectively surveil for and treat known and novel agents alike.
“While the biodefense community may have only just begun to develop technologies for a threat-agnostic approach, we’re highlighting promising new immunological approaches and the data challenges that need to be overcome to build exciting new possible paths forward,” said Lin.
The article was published in the March 2024 issue of Health Security and featured in the March 17 issue of the Global Biodefense headlines .
Published: May 15, 2024
Lin, A., C. M. Torres, E. C. Hobbs, J. Bardhan, S. B. Aley, C. T. Spencer, K. L. Taylor, and T. Chiang. 2024. “Computational and Systems Biology Advances to Enable Bioagent Agnostic Signatures.” Health Security . https://doi.org/10.1089/hs.2023.0076
Research topics
Lab-level communications priority topics.
Thank you for visiting nature.com. You are using a browser version with limited support for CSS. To obtain the best experience, we recommend you use a more up to date browser (or turn off compatibility mode in Internet Explorer). In the meantime, to ensure continued support, we are displaying the site without styles and JavaScript.
- View all journals
- My Account Login
- Explore content
- About the journal
- Publish with us
- Sign up for alerts
- Open access
- Published: 09 May 2024
Computational design of non-porous pH-responsive antibody nanoparticles
- Erin C. Yang ORCID: orcid.org/0000-0002-1305-9066 1 , 2 , 3 ,
- Robby Divine 1 , 2 , 4 , 5 ,
- Marcos C. Miranda ORCID: orcid.org/0000-0002-2839-6997 1 , 6 ,
- Andrew J. Borst ORCID: orcid.org/0000-0003-4297-7824 1 , 2 ,
- Will Sheffler 1 ,
- Jason Z. Zhang ORCID: orcid.org/0000-0003-0091-6273 1 , 2 ,
- Justin Decarreau 1 , 2 ,
- Amijai Saragovi 1 , 2 ,
- Mohamad Abedi 1 , 2 ,
- Nicolas Goldbach ORCID: orcid.org/0000-0001-5401-3892 1 , 7 ,
- Maggie Ahlrichs ORCID: orcid.org/0000-0002-4793-3890 1 , 2 ,
- Craig Dobbins 1 , 2 ,
- Alexis Hand 1 , 2 ,
- Suna Cheng 1 , 2 ,
- Mila Lamb 1 , 2 ,
- Paul M. Levine 1 , 2 ,
- Sidney Chan ORCID: orcid.org/0000-0002-1617-0464 1 , 2 ,
- Rebecca Skotheim 1 , 2 ,
- Jorge Fallas 1 , 2 ,
- George Ueda ORCID: orcid.org/0000-0002-9792-7149 1 , 2 ,
- Joshua Lubner ORCID: orcid.org/0000-0003-1506-0949 1 , 2 ,
- Masaharu Somiya ORCID: orcid.org/0000-0002-5607-8128 1 , 8 ,
- Alena Khmelinskaia 1 , 9 , 10 ,
- Neil P. King ORCID: orcid.org/0000-0002-2978-4692 1 , 2 &
- David Baker ORCID: orcid.org/0000-0001-7896-6217 1 , 2 , 11
Nature Structural & Molecular Biology ( 2024 ) Cite this article
6202 Accesses
35 Altmetric
Metrics details
- Biochemistry
- Cell biology
- Structural biology
Programming protein nanomaterials to respond to changes in environmental conditions is a current challenge for protein design and is important for targeted delivery of biologics. Here we describe the design of octahedral non-porous nanoparticles with a targeting antibody on the two-fold symmetry axis, a designed trimer programmed to disassemble below a tunable pH transition point on the three-fold axis, and a designed tetramer on the four-fold symmetry axis. Designed non-covalent interfaces guide cooperative nanoparticle assembly from independently purified components, and a cryo-EM density map closely matches the computational design model. The designed nanoparticles can package protein and nucleic acid payloads, are endocytosed following antibody-mediated targeting of cell surface receptors, and undergo tunable pH-dependent disassembly at pH values ranging between 5.9 and 6.7. The ability to incorporate almost any antibody into a non-porous pH-dependent nanoparticle opens up new routes to antibody-directed targeted delivery.
Similar content being viewed by others
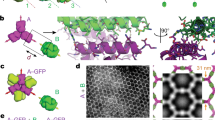
Design of biologically active binary protein 2D materials
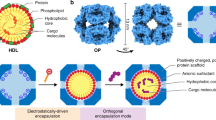
Two-tier supramolecular encapsulation of small molecules in a protein cage
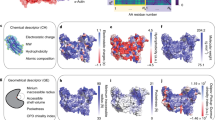
Unifying structural descriptors for biological and bioinspired nanoscale complexes
There is considerable interest in tailoring nanoparticle platforms for targeted delivery of therapeutic molecules. Effective nanoparticle platforms for targeted delivery require in vitro cargo encapsulation, followed by target recognition, triggered nanoparticle disassembly and controlled cargo release once inside the cell 1 , 2 , 3 , 4 , 5 , 6 , 7 , 8 , 9 , 10 , 11 , 12 , 13 . Although several self-assembling protein nanoparticles with customized structures have been designed, they are composed of just one or two static building blocks, and efforts to adapt them for cargo-packaging and delivery applications are still in their infancy 1 , 14 , 15 , 16 , 17 , 18 , 19 , 20 . Antibodies are particularly attractive targeting moieties for delivery applications, and several previous studies have described various ways in which antibodies can be incorporated into nanoparticle delivery platforms 21 , 22 , 23 , 24 , 25 . We recently reported the computational design of antibody-incorporating nanoparticles in which a designed homo-oligomer drives the assembly of the antibody of interest into bounded, multivalent, polyhedral architectures 26 (Fig. 1a ). Although such antibody nanoparticles can activate signaling through a variety of cell surface receptors, they have large pores, complicating the packaging and retention of molecular cargoes.
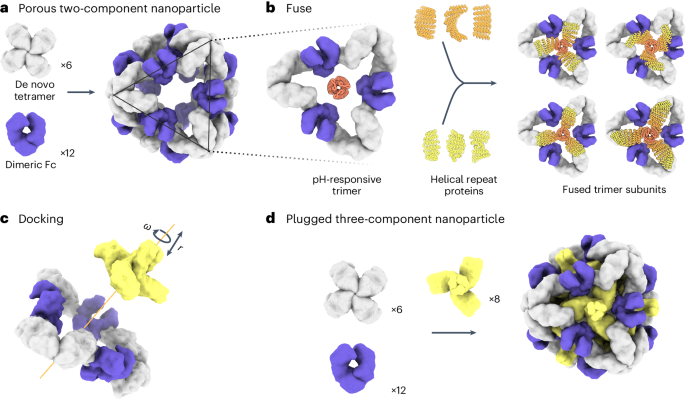
a , Six de novo tetramers (gray) and 12 dimeric Fc domains (purple) assemble into a porous octahedral O42 nanoparticle. The tetramers are aligned along the four-fold symmetry axis, and the Fc domains along the two-fold symmetry axis. b , Combinations of helical repeat proteins are fused to each other and to the pH trimer subunit at regions of high backbone overlap between pairs of helices to generate fused trimer subunits large enough to fully occupy the void along the three-fold axis in the original nanoparticle. c , The three-fold symmetry axes of the resulting pH-dependent trimeric fusions and the nanoparticle are aligned. Favorable docked arrangements are then generated by sampling rotations and translations along this axis. d , The resulting docked three-component nanoparticles have eight new trimeric plug subunits (yellow) that occupy the three-fold symmetry axes of the octahedral architecture. UCSF ChimeraX 1.6 (ref. 54 ) and the PyMOL Molecular Graphics System version 2.5 (Schrödinger) were used to create a – d .
Design and biophysical characterization
To enable packaging and pH-dependent release of molecular cargoes, we sought to computationally design pH-dependent ‘plugs’ for antibody nanoparticles that, in environments above pH 7, close off the apertures in the original designs, but dissociate at the lower pH values that are characteristic of the endosome and tumor microenvironment 27 . We focused on octahedral antibody nanoparticles (O42.1) constructed from a C 4 -symmetric designed tetramer and C 2 -symmetric IgG dimers 26 (Fig. 1a ). The eight C 3 axes in the nanoparticle are unoccupied and feature triangular pores that are 13 nm in length. On these C 3 axes, we aimed to incorporate a designed pH-dependent C 3 trimer 28 and tune it to disassemble at the pH of the endosomal environment (Fig. 1b ). We reasoned that such three-component nanoparticles could (1) encapsulate molecular cargoes without leakage, (2) selectively enter target cells and (3) disassemble in the acidic environment of the endosome.
The previously designed pH-dependent trimer is much smaller than the aperture along the C 3 axis of the octahedral nanoparticle (Fig. 1b ); hence, filling the C 3 axis with the pH-dependent trimer requires extending the backbone such that it makes shape-complementary interactions with the designed tetramer. To enable this, we combined helical fusion 29 , 30 and protein-docking 31 approaches into a single design pipeline. We extended the pH-dependent trimer by fusing combinations of helical-repeat-protein building blocks onto each subunit to generate more than 80,000 distinct C 3 fusions with helical repeats of variable geometry extending outwards from the C 3 axis (Fig. 1b and Methods ). The resultant diverse set of C 3 building blocks was docked into the three-fold-symmetric pore by aligning both C 3 axes and sampling translational and rotational degrees of freedom along this axis 31 and varying the lengths of the repeat protein arms (Fig. 1c and Methods ). The resulting ‘plugged’ octahedral assemblies (O432) contain 12 IgG1 crystallizable fragment (Fc) domains along the octahedral two-fold axes, six tetramers along the octahedral four-fold axes and eight trimeric plugs along the octahedral three-fold axes (Fig. 1d ).
The newly generated interfaces between the pH-dependent plugs and the octahedral assembly were evaluated for designability using a combination of the residue pair transform (rpx) score—a prediction of interaction energy following sequence design 31 , 32 —and overall shape complementarity at the interface 33 . For 6,000 docks predicted to have high designability and shape complementarity, the amino acid sequences at the newly formed fusion junctions and at the interface between the trimeric plug and antibody nanoparticle were optimized using Rosetta sequence design calculations 34 , generating substitutions on both the trimer and the tetramer subunits. Designed interfaces were evaluated for secondary structure contacts and chemical complementarity, and 45 designed trimeric plug and nanoparticle tetramer pairs were selected for experimental characterization.
Designed trimers and tetramers were expressed bicistronically and co-purified by immobilized metal affinity chromatography (IMAC) purification. SDS–polyacrylamide gel electrophoresis (SDS–PAGE) revealed that 16 out of 45 trimer designs co-eluted with the tetramer, suggesting that the trimeric and tetrameric components are associated (Extended Data Fig. 1 ). To form the three-component nanoparticle, a superfolder green fluorescent protein (sfGFP)-Fc fusion protein 26 was added to the clarified lysates of co-expressed trimers and tetramers, and the resulting mixtures were subjected to IMAC. For 5 out of the 16 designs, all three components co-purified, as assessed by SDS–PAGE and native PAGE (Extended Data Fig. 2a,b ). To enable controllable nanoparticle assembly in vitro, the genes in these designs that encode the trimers and tetramers were subcloned into separate expression vectors containing an amino- or carboxy-terminal 6×-histidine tag, and the independently expressed oligomers were purified separately by size-exclusion chromatography (SEC) (Extended Data Fig. 3a ). The SEC elution profiles of the redesigned tetramers were similar to those of the parent O42.1 tetramer, O42.1 C4 (Extended Data Fig. 3b ) 26 . Three-component assemblies were prepared by mixing the three purified proteins—the trimeric plug, designed tetramer and Fc of human IgG1—in a 1:1:1 stoichiometric ratio, followed by overnight incubation and SEC purification (Extended Data Fig. 3c ; owing to tetramer insolubility, insufficient material was produced to prepare a three-component assembly reaction for one design, O432-43). Mixing the three components in a 1:1:1 stoichiometric ratio yielded elution peaks in the void volume, with a shoulder peak between 9 and 11 ml at the elution volume of the original O42.1 antibody nanoparticle 26 (Extended Data Fig. 3c,d ).
For one of the designed assemblies, O432-17, both peaks contained all three protein components (Extended Data Fig. 3e,f ). Optimization of the stoichiometric ratios of the components during in vitro assembly increased the yield at the expected O42.1 elution volume (Fig. 2b ; the optimal assembly ratio per protomer of purified trimeric plug, tetramer and Fc was 1.1:1:1). Assembly of the designed nanoparticle was cooperative and required all three components: mixing any two of the three O432-17 components stoichiometrically did not result in fully assembled nanoparticles (Extended Data Fig. 3g–i ). This cooperativity simplifies preparation of the three-component nanoparticles because it prevents incomplete assembly or assembly hysteresis of nanoparticles containing two out of the three components, thus eliminating the need for further purification steps to separate these species from the intended three-component assembly. Dynamic light scattering (DLS) of the optimized O432 peak indicated that the hydrodynamic diameter was 34 nm (Fig. 2c ), and negative-stain electron microscopy (NS-EM) revealed the presence of monodisperse nanoparticles (Fig. 2d ). Two-dimensional (2D) class averages of negatively stained micrographs revealed plug-like density in the three-fold views compared with the original two-component antibody nanoparticle.
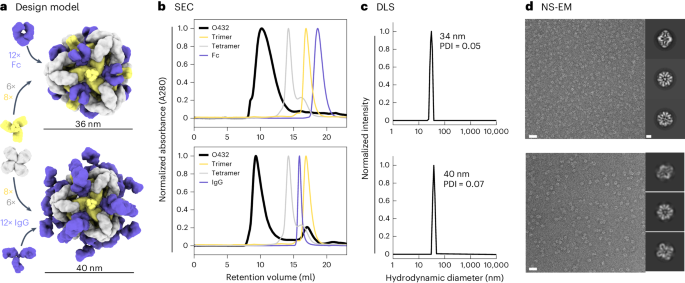
a , Design models with Fc or IgG (purple), designed nanoparticle-forming tetramers (gray) and pH-dependent plug-forming trimers (yellow). b , Overlay of representative SEC traces of the full assembly formed by mixing designed tetramers, trimers and Fc or IgG (black) with those of the single components in gray (tetramer), yellow (trimeric plug) or purple (Fc or IgG). c , Representative DLS of fractions collected from the O432 assembly peak, showing average hydrodynamic diameters of 34 nm (polydispersity index (PDI), 0.05) and 40 nm (PDI, 0.07) for the O432 assemblies with Fc and full-length IgG, respectively. d , Negatively stained electron micrographs with reference-free 2D class averages along each axis of symmetry in inset; electron microscopy images were collected prior to SEC purification. Scale bars, 100 nm and 10 nm for the micrograph and 2D averages, respectively. The PyMOL Molecular Graphics System version 2.5 (Schrödinger) was used to create a .
Source data
For downstream delivery applications, we tested whether the O432-17 nanoparticle would assemble when the designed trimer and tetramer were co-incubated with full-length α-EGFR IgG antibodies containing both Fc and Fab domains (Fig. 2a ). The O432-17 design eluted in the void volume, owing to the increased diameter from the additional Fab domains (Fig. 2b ). DLS of this void volume peak revealed a monodisperse hydrodynamic diameter of 40 nm, in line with the expected diameter of the IgG-containing O432-17 assembly (Fig. 2c ). NS-EM micrographs and 2D class averages of the peak fraction exhibited plug-like density in the three-fold view following 2D classification, as well as Fab-like density in the 2-fold, 3-fold and 4-fold views (Fig. 2d ). Owing to the inherent flexibility of the antibody Fc–Fab junction 35 , the Fab domain density was not well resolved (Fig. 2d ).
Cryo-EM analysis of O432-17
We sought to determine the structure of the O432-17 nanoparticles using single-particle cryogenic electron microscopy (cryo-EM) (Fig. 3a–d ). Following data collection and preprocessing of raw micrographs, a subset of the 2D averages with fully assembled O432-17 nanoparticles (Extended Data Fig. 4a,b ) was used to generate an ab initio three-dimensional (3D) reconstruction in the absence of applied symmetry (Extended Data Fig. 4c ). 3D heterogeneous refinement yielded four classes (Extended Data Fig. 4d ), all corresponding to fully plugged O432 antibody nanoparticles, with six designed tetramers at the four-fold octahedral symmetry axes forming interfaces with eight designed trimers at the three-fold symmetry axes and 12 dimeric Fc fragments at the two-fold symmetry axes (Table 1 ). A subsequent 3D refinement containing particles from all four classes was generated after applying octahedral symmetry, resulting in a final map with an estimated global resolution of 7 Å (Extended Data Fig. 4e ).
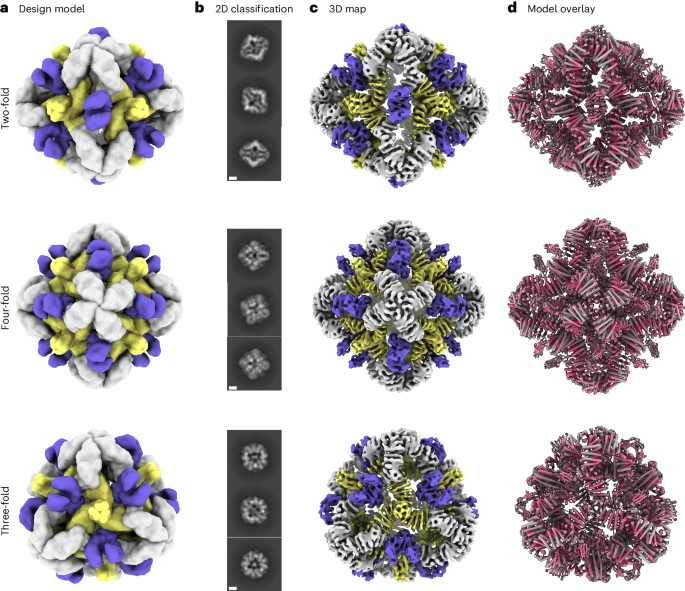
a , Cryo-EM characterization of the O432 assembly with Fc before SEC purification. Computational design models viewed along each axis of symmetry of the octahedral architecture are shown. b , Representative 2D class averages along each axis of symmetry. Scale bars, 10 nm. c , 3D EM density map with 7 Å resolution, reconstructed from the collected dataset. d , Overlay of the design model (gray) and the model refined into the 3D EM density map (pink), showing high agreement. UCSF ChimeraX 1.6 (ref. 54 ) was used to create a .
We fit the O432-17 design model into the experimentally determined cryo-EM map using backbone refinement in Rosetta, yielding a density-refined model. The model contains the plug, tetramer and Fc, and is in close agreement with the original design (Fig. 3d ). The helices of the tetramers at the four-fold axes are clearly evident in the cryo-EM density, with each helical repeat extending from the four-fold axis of the octahedral architecture to bind the Fc, which exhibits little or no structural distortion. The designed trimeric plugs are also clearly evident in the cryo-EM density, with helices along the three-fold axis of the octahedral architecture that extend to form an interface with the tetramers. The addition of the trimeric plug reduces the porosity of the antibody nanoparticle, as intended: the largest diameter pore is 3 nm, compared with 13 nm in the original O42.1 nanoparticle 26 . The helices forming the trimer and tetramer interface are largely consistent between the design and the model refined into density (Extended Data Fig. 4f ). The density-refined model of the plugged O432-17 nanoparticle is substantially closer to the original O42.1 design model than to the previously determined O42.1 cryo-EM structure: the average Cα root mean squared deviation (r.m.s.d.) of the asymmetric unit was 1.6 Å between the O432-17 density-refined model and the design model, 1.9 Å between the O432-17 design model and O42.1 density-refined model, and 4.2 Å between the O42.1 density-refined model and the O42.1 design model (Extended Data Fig. 5a–c ). These results suggest that the addition of the trimeric plug buttresses the tetramer into a conformation that more closely matches that of the original design model, and could also reduce the overall flexibility of the system, as compared with the original O42.1 design.
Trimeric-plug-dependent packaging of molecular cargoes
We next set out to redesign the nanoparticles to package and protect molecular cargoes through electrostatic interactions 17 , 36 , 37 . We generated variants with either highly positively or highly negatively charged interiors, and focused on changing interior surface residues of the trimeric plug. Substitutions to amino acids with the desired charge (or no charge) were preferred.
We screened for packaging of nucleic acids by assembling positively charged trimeric plug variants, the designed tetramer and cetuximab (CTX), an antibody to EGFR, with a 154-nucleotide (nt) prime editing guide RNA (pegRNA) cargo 38 , 39 , 40 (Fig. 4a ). Packaging and protection of nucleic acid cargo in the presence or absence of nuclease was assessed through non-denaturing electrophoresis, staining with SYBR Gold and Coomassie to detect RNA and protein, respectively. One variant, O432-17(+), showed co-migration of the nucleic acid and nanoparticle, both with and without treatment with the Benzonase nuclease (Fig. 4b ). The RNA-packaging three-component assembly migrated similarly to an assembly reaction lacking RNA cargo, and negatively stained electron micrographs and corresponding 2D class averages also closely resembled those of empty three-component nanoparticles (Extended Data Figs. 6a,b and 7a–f ). Excess nucleic acid that did not co-migrate with the protein was degraded in the Benzonase-treated sample, demonstrating that the nanoparticles protected the nucleic acid that co-migrated with O432-17(+). We did not observe co-migration of O432-17(+) and pegRNA after RNAse A treatment (Fig. 4b,c ); the remaining pore size after the addition of the plug is likely still large enough to admit the 14-kDa enzyme but small enough to exclude the 60-kDa Benzonase 19 . Nucleic acids that co-migrated with the plugless O42.1 nanoparticle were degraded after both Benzonase and RNAse A treatment, demonstrating that the trimeric plug is required for packaging and protection of nucleic acid cargo (Fig. 4b,c ).
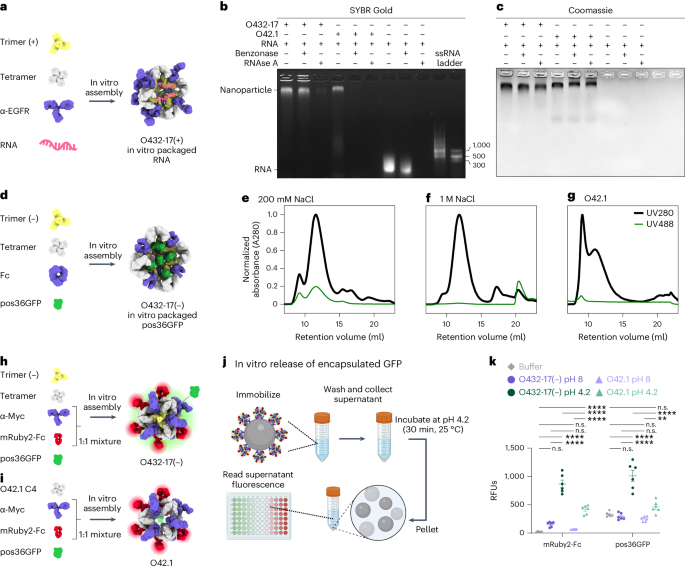
a , Designed positively charged trimer variants were assembled by incorporating the designed tetramer, pegRNA and antibody to EGFR (α-EGFR) to form O432-17(+) nucleocapsids. b , c , O432-17(+) nucleocapsids and O42.1 assembled in vitro with RNA were treated with Benzonase or RNAse A for 1 h, electrophoresed on non-denaturing 0.8% agarose gels and stained with SYBR Gold ( b ; nucleic acid) and Coomassie ( c ; protein). d , Designed negatively charged trimer variants were assembled with designed tetramer, pos36GFP and human Fc to form O432-17(–) nanoparticles with in vitro-packaged pos36GFP. e , f , SEC chromatograms of in vitro packaging reactions involving O432-17(–) and pos36GFP were performed in either 200 mM NaCl ( e ) or 1 M NaCl ( f ). g , As a comparison, SEC chromatograms of in vitro-assembled O42.1 with pos36GFP in 200 mM NaCl showed no co-migration of pos36GFP. Absorbance was monitored at 280 nm (black) and 488 nm (green). h , O432-17(–) nanoparticles for in vitro release of pos36GFP were assembled with negatively charged trimer, designed tetramer, pos36GFP and a 1:1 mixture of a Myc-targeted monoclonal antibody (α-Myc) and mRuby2-Fc. i , As a comparison, O42.1 was assembled in vitro with O42.1 C4, pos36GFP and a 1:1 mixture of antibody to Myc and mRuby2-Fc. j , Experimental design for in vitro release of encapsulated pos36GFP in acidic conditions. Assembled nanoparticles were immobilized on Myc-peptide-coated S. cerevisiae yeast cells. Cells and supernatant were collected by centrifugation and resuspended and incubated in acidic conditions. The supernatant containing released components and cargo was buffer exchanged to pH 8, and fluorescence intensity was analyzed. k , Fluorescence intensity of the supernatant of O432-17(–) and O42.1 assembled with pos36GFP and mRuby2-Fc before and after acidic incubation. mRuby2-Fc fluorescence was used as an indicator of nanoparticle assembly; a positive fluorescence signal indicates nanoparticle disassembly. Positive pos36GFP fluorescence indicates release of pos36GFP cargo. * P ≤ 0.05, ** P ≤ 0.01, *** P ≤ 0.001, **** P < 0.0001, two-way ANOVA with Tukey’s correction for multiple comparisons. n.s., not significant. Data are presented as mean values ± s.e.m. and measured over three independent samples in duplicate (Supplementary Table 6 ). UCSF ChimeraX 1.6 (ref. 54 ) and the PyMOL Molecular Graphics System version 2.5 (Schrödinger) were used to create a , d , h and i . j was created using BioRender . GraphPad Prism version 9.3.1 (GraphPad Software) was used to create k . RFUs, relative fluorescence units.
We also designed negatively charged plug variants to package positively charged protein cargoes. We screened for packaging of positively charged GFP (pos36GFP) 41 by assembling negatively charged trimeric plug variants with different interior surface charges with tetramer, Fc and pos36GFP (Fig. 4d ). For one variant, O432-17(–), both the 488 nm GFP and the 280 nm nanocage signals were detected in the elution profile during SEC, and NS-EM confirmed that three-component nanoparticle assembly had occurred (Fig. 4e and Extended Data Fig. 8c,d ). On the basis of the fluorescence and absorbance of the assemblies, approximately nine to ten pos36GFPs are packaged per nanoparticle in 200 mM NaCl, occupying roughly 40% of the interior volume. SEC revealed that GFP packaging did not occur in the presence of 1 M NaCl (Fig. 4f ), indicating that packaging is largely driven by electrostatic interactions between the cargo and nanoparticle interior. In vitro assembly of the unplugged O42.1 (ref. 26 ) with pos36GFP did not result in cargo packaging (Fig. 4g ). SEC, DLS and NS-EM confirmed O432-17(–) assembly in the absence of cargo, suggesting that assembly stability is cargo-independent (Extended Data Fig. 8a–d ). Thus, our designed three-component nanoparticles efficiently encapsulate and protect molecular cargoes.
We next sought to release encapsulated pos36GFP cargo through pH-mediated disassembly of O432-17(–) nanoparticles. O432-17(–) trimer, tetramer and pos36GFP were assembled in vitro using a 50:50 mixture of mouse antibody to Myc and mRuby2-Fc, the latter of which enabled monitoring of nanoparticle disassembly (Fig. 4h ). As a comparison, we also assembled O42.1 with the same antibody cocktail and pos36GFP (Fig. 4i ). Assembled nanoparticles were immobilized on Saccharomyces cerevisiae cells expressing the Myc peptide. The cells were collected by centrifugation and then resuspended in citrate–phosphate buffer at low or high pH (4.2 and 8, respectively) for 30 min, after which the supernatant was collected and buffer exchanged to neutral pH before fluorescence was measured (Fig. 4j ). Although both O42.1 and O432-17(–) nanoparticles remain intact at high pH and disassemble at low pH, we observed considerably more pH-dependent pos36GFP cargo release from the plugged O432-17(–) nanoparticles than from the O42.1 nanoparticles, because the absence of the plug precluded cargo packaging and retention (Fig. 4k ). The designed trimeric plug on O432-17(–) nanoparticles thus enables packaging, retention and pH-mediated release of encapsulated cargo.
Tuning pH-dependent plug dissociation
We next explored the potential for disassembly of O432-17(–) and O432-17(+) nanoparticles in the pH range of the endosome and lysosome (pH 4.5–6.5) 42 . We monitored the dissociation of fluorescently labeled plug trimers and antibody Fc fused to GFP (sfGFP-Fc) from the nanoparticles at low pH through flow cytometry 43 , 44 , 45 . O432-17 nanoparticles assembled with tetramer, Alexa Fluor 647 (AF647)-conjugated trimeric plug and a 50:50 mixture of mouse monoclonal antibody to Myc and sfGFP-Fc (Fig. 5a ) were immobilized onto 3 μm Myc-tag-coated polystyrene beads and incubated in citrate–phosphate buffers ranging from pH 4.2 to 7.5. The beads were collected by centrifugation and resuspended in buffer at pH 8, and then were analyzed using flow cytometry (Fig. 5b ). Loss of AF647 and sfGFP signals indicates disassembly and release of plug trimers and sfGFP-Fc from the nanoparticles, respectively (Extended Data Fig. 8a–d ).
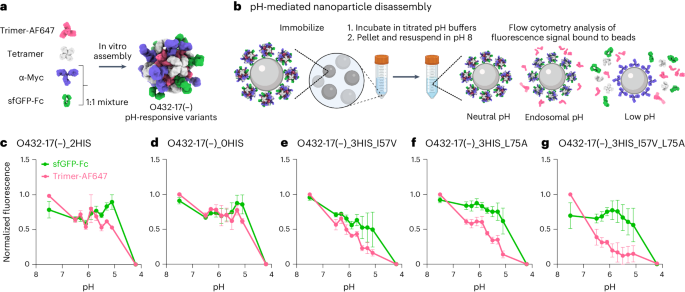
a , O432-17 nanoparticles were assembled with AF647-conjugated trimeric plug variants, designed tetramer, sfGFP-Fc and α-Myc antibody. b , O432-17 nanoparticles were immobilized on Myc-peptide-coated beads and incubated in titrated pH buffers. Beads were washed by centrifugation and resuspended in 25 mM Tris and 500 mM NaCl, pH 8, and the remaining trimer and sfGFP-Fc fluorescence on the beads was analyzed by flow cytometry. c – g , AF647 and sfGFP fluorescence levels were normalized to the minimum and maximum values across the titration and analyzed as a function of the pH for each nanoparticle variant (O432-17(–)_2HIS ( c ), O432-17(–)_0HIS ( d ), O432-17(–)_3HIS_I57V ( e ), O432-17(–)_3HIS_L75A ( f ), O432-17(–)_3HIS_I57V_L75A ( g )). Data are normalized to the minimum and maximum fluorescence per titration and presented as mean ± s.e.m. for three biologically independent replicates. UCSF ChimeraX 1.6 (ref. 54 ) and the PyMOL Molecular Graphics System version 2.5 (Schrödinger) were used to create a ; b was created using BioRender ; GraphPad Prism version 9.3.1 (GraphPad Software) was used to create c – g .
We found that by varying the number of histidine-containing hydrogen-bond networks and the hydrophobic packing with the trimer, the pH of plug disassembly could be tuned over a remarkably wide range. The O432-17(–) plug design (O432-17(–)_2HIS) with two histidine hydrogen-bond networks disassembled at pH 5.1, similar to O432-17(–)_0HIS, a negative control trimer variant in which all histidines are substituted with asparagine (Fig. 5c,d ). O432-17(–)_3HIS_I57V and O432-17(–)_3HIS_L75A, which have three histidine networks and additional core packaging destabilization, assembled robustly (Extended Data Fig. 9a–d ) and showed clear pH-dependent release of the pH trimer at pH of above 5.1 (Fig. 5e,f ). The apparent dissociation constant (p K a ) values of trimer dissociation (the pH at which the fluorescence was 50% of the maximum signal) were pH 6.1 and pH 5.9 for O432-17(–)_3HIS_I57V and O432-17(–)_3HIS_L75A, respectively; nanoparticle disassembly, as measured by release of sfGFP-Fc, occurred only at lower pH (pH 5.3 and 4.7, respectively). For plug release, O432-17(–)_3HIS_I57V_L75A had a remarkably high apparent p K a of pH 6.7 (Fig. 5g ; the nanoparticle only disassembled at pH 5). pH-mediated release of encapsulated cargo was observed for O432-17(–)_2HIS (Fig. 4k ), but could not be investigated for the other O432-17(–) variants, because pos36GFP was not well packaged, perhaps owing to altered interactions with the plug. Like O432-17(–), histidine-containing variants of O432-17(+) disassembled at a more basic pH than did the parental construct (Extended Data Figs. 7a–d and 8e,f ). These results demonstrate that nanoparticle dissociation can be tuned over a wide pH range.
Entering target cells through receptor-mediated endocytosis
We next tested the ability of the O432-17 nanoparticles to enter cells through receptor-mediated endocytosis. We assembled targeted nanoparticles by incubating a 1:1 stoichiometric mixture of CTX and Fc fused to mRuby2 (mRuby2-Fc) with the tetramer and the 3HIS_I57V_L75A trimeric plug variant labeled with AF647 (named O432-17-CTX). We assembled a non-EGFR-targeting nanoparticle (named O432-17-Fc) as a negative control by mixing the tetramer and trimer-AF647 with mRuby2-Fc (Extended Data Fig. 10a ). Assembled O432-17-CTX and O432-17-Fc were incubated with A431 cells, which have high levels of EGFR expression, and imaged with epifluorescence microscopy. The percentage of A431 cells expressing O432-17-CTX (44%) was substantially higher than the percentage expressing O432-17-Fc (5%), suggesting that the O432-17(–) nanoparticles require EGFR binding for efficient endocytosis (Extended Data Fig. 10b,c ). The EGFR-targeted nanoparticle bound to 45% and 68% of wild-type HeLa cells, which express moderate levels of EGFR with and without serum, respectively; EGFR-knockout HeLa cells were not efficiently labeled in either condition (10% with serum and 7% without serum) (Extended Data Fig. 10f,g ; the reduced labeling in the presence of serum likely reflects a slight instability in the nanoparticle structure in the presence of high concentrations of exogenous antibody 26 ). Together, these data establish that the antibody component of O432-17 nanoparticles enables targeting of cells expressing a specific receptor.
We describe a general approach for reducing the porosity of protein nanomaterials by designing custom symmetric plugs that fill pores along unoccupied symmetry axes. We used this approach to generate the first designed non-covalently-associated protein nanoparticles with distinct structural components on three axes of symmetry. In contrast to functionalized homomeric and designed two-component protein nanoparticles 1 , 8 , 14 , 17 , 18 , 26 , 36 , 46 , 47 , 48 , 49 , each of the three components in our designs has a specific functional role. The trimeric plug enables pH-responsive disassembly and packaging (and release) of cargoes, the antibody provides cell-targeting functionality and the tetramer drives assembly of the three-component nanoparticle by interacting with both the trimer and Fc domain. This division of labor makes our designed system highly modular: targeting specificity can be altered simply by switching the antibody component to target cells of interest, and the pH of disassembly and cargo-packaging specificity can be programmed by choosing the appropriate trimeric plug. Assembly of the nanoparticles in vitro from independently purified components enables facile inclusion of multiple variants of each component, such as multiple distinct antibodies.
Engineering drug-delivery platforms to induce nanoparticle disassembly at a biologically relevant pH is a critical challenge. Our results represent a test of our understanding of protein disassembly and assembly dynamics 50 , 51 , 52 , 53 . We finely tuned the apparent p K a of disassembly of our O432 system through a combination of histidine-containing hydrogen-bonding networks and cavity-introducing substitutions that weaken hydrophobic interactions at the trimeric component’s oligomeric interface. Through this approach, we raised the apparent p K a of disassembly to a remarkably high pH of 6.7, well above the pH of the endosome and in the range of many tumor microenvironments, and close to the maximum achievable value, given the p K a of histidine.
Our O432 nanoparticle system is capable of packaging both protein and nucleic acid cargoes, protects nucleic acid cargoes from degradation, disassembles at biologically relevant pH with precise tunability, incorporates a variety of targeting moieties and is readily internalized by target cells. In performing these functions, the O432 nanoparticles resemble viruses. They also resemble the smallest viruses in size, with an internal diameter (25 nm) that is slightly larger than that of adeno-associated viruses (18 nm). However, the architecture of the O432 nanoparticles differs greatly from small icosahedral viruses, many of which are constructed from multiple copies of a single capsid protein that assumes several different conformations and performs several distinct functions at different stages of the viral life cycle. By contrast, the O432 nanoparticles are constructed from three modular protein components that each perform a specific function. The tunable pH dependence makes this system a particularly attractive platform for engineering release and delivery of drugs during early stages of endosomal maturation. However, to be broadly useful, future designs for intracellular biologics-delivery systems will need to incorporate endosomal escape machinery. The nanoparticles are well suited in their current form for conditional delivery of cytotoxic tumor-killing or tumor-modulating drugs into the tumor microenvironment with tumor-specific antibodies; by providing an additional checkpoint on proper localization, the pH-dependent release of cargo in the lower pH tumor microenvironment could minimize off-tumor toxicity and systemic exposure compared with classic direct antibody-conjugation approaches. Overall, the pH-dependent disassembly, programmability and versatility of the O432 platform provide multiple exciting paths forward for biologics delivery.
Extension of pH-responsive trimeric building blocks
We used the WORMS helical fusion software 29 to generate fusions between the base pH-trimer helical bundle and pairs of 82 helical repeat proteins, designed to cover a broad range of axial and radial displacement between repeat units 55 . Scaffold information was prepared in a json file, which is used as an input parameter in the WORMS software through the --dbfiles option. For each scaffold, the pdb file path ('file'), a geometric class specification ('class') and a connections dictionary ('connections') specifying the chain ('chain'), terminus direction ('direction') and residue positions that were available for fusion ('residues') were included in the json file. Geometric class specification for oligomeric scaffolds required either an N or C following the oligomeric architecture, to define the terminus available for subsequent fusions. A connections dictionary specifying each chain, the terminus direction and residue positions allowed for fusion was required for each chain and terminus direction. The oligomeric scaffold asymmetric unit required one connections dictionary, specifying fusion to one terminus and one chain. As monomers, helical repeat proteins require a list of two connections dictionaries, because they allow fusions to either the N or C terminus. An example json file example is shown below, where the connections dictionary specifies allowable fusions on the C terminus of the pH trimer from residue 50 and over, and anywhere along the helical repeat proteins:
{ {"file": "/path/to/ph_trimer.pdb", "class": ["C3_C"], "connections":[ {"chain": 1, "direction": "C", "residues":["50:"]} ] }, {"file": "/path/to/helicalrepeatprotein1.pdb", "class": ["Monomer"], "connections": [ {"chain": 1, "direction": "N", "residues":[":210"]}, {"chain": 1, "direction": "C", "residues":["-210:"]} ] }, {"file": "/path/to/helicalrepeatprotein2.pdb", "class": ["Monomer"], "connections": [ {"chain": 1, "direction": "N", "residues":[":160"]}, {"chain": 1, "direction": "C", "residues":["-160:"]} ] } }
To prepare the WORMS software for fusions, we also included a map for backbone connections (--bbconn), which specifies the order in which scaffolds of each class can be fused. We used the following backbone connection map, which specifies that the N terminus of the fusion of two helical repeat proteins in the monomer class is fused to the C terminus of an oligomer with C 3 symmetry:
--bbconn C3 C3_C \ NC Monomer \ N_ Monomer \
Finally, we specified a --geometry NullCriteria() option, which allowed generation of arbitrary fusions of varying radius and length to the C terminus of the pH trimer.
Computational docking of plugged antibody nanoparticles
The generated fusions served as docking inputs into the antibody nanoparticle through the --inputs1 and --inputs2 options. To prepare the antibody nanoparticle for axle docking along its C 3 axis, we generated a context structure PDB file by isolating the chains forming the C 3 symmetric aperture. We fed this context structure and each trimer fusion separately into the RPXDock application 31 using the axle docking protocol (--architecture AXLE_C3). To enable trimming, we set a maximum number of trimmed residues (--max_trim) and specified the chains representing the fusion to be trimmed (--trimmable_components). Finally, to enable greater sampling, we allowed the RPXDock application to sample the entire search space (--docking_method) at a cartesian and angular resolution of 0.25 angstroms and degrees, respectively (--grid_resolution_cart_angstroms and --grid_resolution_ori_degrees). An example axle docking executable is shown below.
PYTHONPATH = /path/to/rpxdock/site-packages /path/to/python/environment /path/to/rpxdock/dock.py \
––architecture AXLE_C3 \ ––inputs1 /path/to/plugfusion.pdb \ ––inputs2 /path/to/contextstructure.pdb \ ––max_trim 400 \ ––cart_bounds -300 300 \ ––docking_method grid \ ––grid_resolution_cart_angstroms 0.25 \ ––grid_resolution_ori_degrees 0.25 \ ––hscore_files ailv_h \ ––hscore_data_dir /path/to/rpxdock/hscore \ ––trimmable_components 'A' \ ––function stnd \
We evaluated the docked interfaces between each fusion and antibody nanoparticle using the RPX score and NContact metrics, which are predictive of geometric complementarity and designability. Empirically, we deemed docks with an RPX score greater than 50 and with more than one residue pair contact to be suitable for sequence optimization.
Computational design of plugged antibody nanoparticles
We used the Rosetta Macromolecular Modeling suite to optimize the sequence residues contributing to either the fusion domains or interface between plug and nanoparticle. With residue selectors, we identified clashing residue positions as a result of the WORMS fusion and residues contributing to the interface between the plug and antibody nanoparticle. The sequence of the selected residues was optimized on the basis of the local positions of each residue defined by secondary structure, solvent accessible surface area (SASA) of the backbone atom and the Cβ atom and the number of amino acid side chains in a cone extending along the Cα–Cβ vector side chain neighbors. An example of the fusion, docking and design of O432-17 is included at https://github.com/erincyang/plug_design.git .
Small-scale bicistronic bacterial protein expression
Synthetic genes encoding designed trimeric-plug and tetramer sequences bicistronically were purchased from Genscript in pET-29b+ vectors. They were connected by an intergenic region (5′-TAAAGAAGGAGATATCATATG-3′) and a C-terminal 6×histidine tag on the tetramer. Expression plasmids were transformed into BL21(DE3) Escherichia coli cells and were grown in LB medium supplemented with 50 mg l –1 kanamycin at 37 °C overnight. The overnight culture was diluted into autoinduction medium and incubated at 37 °C overnight. Cells were lysed chemically in BugBuster supplemented with 1 mM PMSF and 20 mM imidazole, and were cleared by centrifugation. Clarified lysates were purified using IMAC with Ni-NTA magnetic beads. The soluble fractions were washed with 25 mM Tris pH 8.0, 300 mM NaCl and 60 mM imidazole before elution with 25 mM Tris pH 8.0, 300 mM NaCl and 300 mM imidazole. Elution fractions from bicistronic expression plasmids were subsequently subjected to native PAGE and SDS–PAGE to identify slow migrating species that co-eluted two proteins of different molecular weights.
Small-scale bicistronic bacterial protein expression for trimeric-plug and tetrameric designs for sfGFP-Fc lysate assembly
Synthetic genes encoding designed trimeric plug and tetramer sequences bicistronically were purchased from Genscript in pET-29b+ vectors and connected by an intergenic region (5′-TAAAGAAGGAGATATCATATG-3′) and a C-terminal 6×Histidine tag on the tetramer. Expression plasmids were transformed into BL21(DE3) E. coli cells and grown in LB medium supplemented with 50 mg l –1 kanamycin at 37 °C overnight. The overnight culture was diluted into autoinduction medium and incubated at 37 °C overnight. Cells were lysed chemically in BugBuster supplemented with 1 mM PMSF and 20 mM imidazole, and were cleared by centrifugation. Clarified lysates were incubated with a 3 μM final concentration of sfGFP-Fc and purified by IMAC with Ni-NTA magnetic beads. The soluble fractions were washed with 25 mM Tris pH 8.0, 300 mM NaCl and 60 mM imidazole before elution with 25 mM Tris pH 8.0, 300 mM NaCl and 300 mM imidazole. Elution fractions from bicistronic expression plasmids were subsequently subjected to native PAGE and SDS–PAGE to identify slow migrating species that co-eluted three proteins of different molecular weights.
Production of Fc and Fc fusions
Fc, sfGFP-Fc, mRuby2-Fc and CTX IgG were purchased from Genscript in CMVR vectors and transfected into in ExpiHEK293F cells (Thermo Fisher Scientific) and purified by IMAC with 50 mM Tris pH 8.0, 300 mM NaCl and 500 mM imidazole elution buffer, and were then purified further through SEC over a Superdex 200 10/300 GL FPLC column (Cytiva) into 50 mM Tris pH 8.0, 300 mM NaCl and 0.75% CHAPS (Fc) or 50 mM Tris, pH 8.0, 300 mM NaCl and 0.05% glycerol (Cetuximab IgG, sfGFP-Fc, mRuby2-Fc). Stocks were frozen at −80 °C for subsequent analyses.
Large-scale expression and purification of O432-17 components
Designs that co-purified and yielded slowly migrating species, as determined by native PAGE, were subsequently subcloned into pET-29b+ vectors (Genscript). Each vector encoded either a trimeric plug or a tetramer variant—both with C-terminal hexahistidine tags—that was expressed at a larger scale (1–12 l of culture). Cells were lysed by microfluidization in 25 mM Tris pH 8.0, 300 mM NaCl, 1 mM DTT, 1 mM PMSF and 0.1 mg ml –1 DNAse and cleared by centrifugation. Clarified lysates were filtered through 0.7-μm filters and purified by IMAC using gravity columns with nickel-NTA resin or HisTrap HP columns (Cytiva) using 25 mM Tris pH 8.0, 300 mM NaCl and 60 mM imidazole wash buffer and 25 mM Tris pH 8.0, 300 mM NaCl and 300 mM imidazole elution buffer. Elution fractions containing pure proteins were concentrated using centrifugal filter devices (Millipore) and further purified on a Superdex 200 10/300 GL (for large-scale purification) or Superose 6 10/300 GL (for comparison to assembly) gel-filtration column (Cytiva) using 25 mM Tris pH 8.0, 150 mM NaCl and 0.75% CHAPS. Gel filtration fractions containing pure protein in the desired oligomeric state were pooled, concentrated and frozen in aliquots at −80 °C for subsequent analyses.
In vitro assembly of O432-17 nanoparticles
Purified tetramer and Fc or IgG from gel filtration fractions were assembled in a 1:1 molar ratio and with 1.1× excess trimeric plug. Molar ratios were calculated on the basis of the monomeric extinction coefficient. Assemblies were assembled in a total volume between 100 and 500 μl and a total concentration of each monomeric component between 5 and 50 μM, dialyzed overnight at 25 °C in 25 mM Tris pH 8.0 and 150 mM NaCl, and purified on a Superose 6 10/300 GL gel filtration column (Cytiva) using 25 mM Tris pH 8.0 and 150 mM NaCl as the running buffer.
Dynamic light scattering
DLS measurements were performed using the default sizing and polydispersity method on the UNcle (Unchained Labs). O432-17 variants (8.8 μl) were pipetted into glass cuvettes. DLS measurements were run in triplicate at 25 °C with an incubation time of 1 s; results were averaged across ten runs and plotted using Python3.7.
Negative-stain electron microscopy preparation and data collection of O432-17 nanoparticles and variants
Between 0.1 and 0.2 mg ml –1 of pre- or post-SEC O432-17 assemblies in 25 mM Tris pH 8.0 and 150 mM NaCl were applied onto 400- or 200-mesh carbon-coated copper grids and glow discharged for 20 s, followed by three applications of 3.04 μl 2% nano-W or Uranyless stain.
Micrographs were recorded using EPU software (Thermo Fisher Scientific) on a 120 kV Talos L120C transmission electron microscope (Thermo Fisher Scientific) at a nominal magnification of ×45,000 (pixel size, 3.156 Å per pixel) and a defocus range of 1.0–2.5 μm.
Negative-stain electron microscopy data analysis of O432-17 nanoparticles
Negative-stain electron microscopy datasets were processed using Relion3.0 software 56 . Micrographs were imported into the Relion3.0 software, and a contrast transfer function was estimated using GCTF 57 . Around 500 particles were manually picked and 2D classified, and selected classes were used as templates for particle picking in all images. Approximately 100,000 picked particles were 2D classified for 25 iterations into 50 classes.
Negative-stain electron microscopy data analysis of O432-17 nanoparticle variants
Negative-stain electron microscopy datasets were processed by CryoSPARC software v4.0.3 (ref. 58 ). Micrographs were imported into the CryoSparc software. Particles were picked using the blob picker in CryoSPARC and 2D classified, or 2D classes from blob picking were used as templates for particle picking in all images. All the picked particles were 2D classified for 20 iterations into 100 classes.
Cryo-electron microscopy preparation and data collection of O432-17 nanoparticles
Two microliters of pre-SEC-purified O432-17-Fc sample at 0.5 mg ml –1 in 25 mM Tris pH 8.0 and 150 mM NaCl were applied onto C-flat 1.2/1.3 holey carbon grids. Grids were then plunge-frozen into liquid ethane and cooled with liquid nitrogen using a Thermo Fisher Vitrobot Mk IV, with a 6.5-s blotting time and blot force of 0. The blotting process took place inside the vitrobot chamber at 22 °C and 100% humidity. Data acquisition was performed with Leginon on a Titan Krios electron microscope operating at 300 kV using a K3 summit direct electron detector equipped with an energy filter and operating in super-resolution mode. The nominal magnification for data collection was ×105,000 with a calculated pixel size of 0.42 Å per pixel. The final dose was 63.775 e – per A 2 for 2,223 videos.
Cryo-electron microscopy data analysis of O432-17 nanoparticles
All data processing was done using CryoSPARC v3.0.0 (ref. 58 ). Alignment of video frames was performed using Patch Motion with an estimated B factor of 500 Å 2 , with the maximum alignment resolution set to 5. During alignment, all videos were Fourier cropped by one-half. Defocus and astigmatism values were estimated using patch CTF with default parameters. An initial 1,391 nanoparticle particles were manually picked and extracted with a box size of 576 pixels. This was followed by a round of 2D classification and subsequent template-picking using the best 2D class averages low-pass filtered to 20 Å. Particles were next picked with Template Picker and were manually inspected before extraction with a box size of 660 pixels, and were then further Fourier cropped to a final box size of 330 pixels, for a total of 66,904 particles. A round of reference-free 2D classification was performed in CryoSPARC, with a maximum alignment resolution of 6 Å. The best classes that revealed visibly assembled nanoparticles were used for 3D ab initio determination using the C 1 symmetry operator. This was followed by a round of 3D heterogeneous refinement using C 1 symmetry and sorting into four distinct classes, all of which revealed complete plugging of the octahedral three-component nanoparticle. Thus, all 50,017 of the best particles selected from 2D classification were subjected to non-uniform 3D refinement with octahedral symmetry applied, yielding a final map with an estimated global resolution of 7.06 Å, following per-particle defocus refinement. The final maps were deposited in the EMDB under accession code EMD- 29602 . Refined models were generated by rigid-body docking followed by refinement of the backbone of the design model into the final cryo-EM density map in Rosetta 59 .
Computational design of O432-17 electrostatically charged variants
A consensus design approach was used to first identify interior surface positions predicted to be the most robust to surface substitutions. These positions were divided into three tiers on the basis of the predicted enhancement to the design’s stability and/or solubility. Using the Rosetta modeling suite, the trimeric plug design model was redesigned, allowing optimization of the identities of interior surface residues that did not contribute to the interface between plug monomers or between the trimeric plug and antibody nanoparticle. We deployed three tiers of sequence-optimization strategies per charged variant. The first strategy enabled optimization of residue positions toward only charged amino acids (arginine or lysine for the positively charged variants, glutamate and aspartate for the negatively charged variants). The second strategy included the charged amino acids but also polar, non-charged amino acids, such as asparagine, glutamine and alanine, to maintain helical propensity. The third strategy included the residue identities in the first and second strategy, but also enabled reversion to the original identity in the trimeric plug design model. Substitutions that resulted in substantial losses of atomic packing interactions or side chain–side chain or side chain–backbone hydrogen bonds were discarded. The top-scoring designs for each design strategy and surface position tier were selected for inclusion as variant proteins.
Expression and purification of electrostatically charged trimeric plug variants
Plasmids encoding electrostatically charged trimeric plug variants and containing a C-terminal hexahistidine tag were cloned into pET-29b+ vectors (Genscript) and expressed overnight at 37 °C in autoinduction medium 60 . Cells were lysed by microfluidization or sonication in 25 mM Tris pH 8.0, 500 mM NaCl, 1 mM DTT, 1 mM PMSF and 0.1 mg ml –1 DNAse and cleared by centrifugation. Clarified lysates were filtered through 0.7-μm filters and purified by IMAC using gravity columns with nickel-NTA resin or HisTrap HP columns (Cytiva) using 25 mM Tris pH 8.0, 500 mM NaCl and 60 mM imidazole wash buffer and 25 mM Tris pH 8.0, 500 mM NaCl and 300 mM imidazole elution buffer. Elution fractions containing pure proteins were concentrated using centrifugal filter devices (Millipore) and further purified on a Superdex 200 10/300 GL (for large-scale purification) or Superose 6 10/300 GL (for comparison with assembly) gel-filtration column (Cytiva) using 25 mM Tris pH 8.0, 500 mM NaCl and 0.75% CHAPS. Gel filtration fractions containing pure protein in the desired oligomeric state were pooled, concentrated and frozen in aliquots at −80 °C for subsequent analyses.
Expression and purification of pos36GFP
pos36GFP plasmid (Addgene no. 62937) was transformed into BL21(DE3) bacteria cells in lysogeny broth and induced with isopropyl ß- d -1-thiogalactopyranoside (IPTG) at an optical density of 0.6 and grown for 16 h at 20 °C. Cells were lysed by microfluidization or sonication in 25 mM Tris pH 8.0, 500 mM NaCl, 1 mM DTT, 1 mM PMSF and 0.1 mg ml –1 DNAse and cleared by centrifugation. Clarified lysates were filtered through 0.7-μm filters and purified by IMAC using gravity columns with nickel-NTA resin or HisTrap HP columns (Cytiva) with 25 mM Tris pH 8.0, 500 mM NaCl and 60 mM imidazole wash buffer and 25 mM Tris pH 8.0, 500 mM NaCl and 300 mM imidazole elution buffer. Elution fractions containing pure proteins were concentrated using centrifugal filter devices (Millipore) and further purified on a Superdex 200 10/300 GL gel filtration columns (Cytiva) using 25 mM Tris pH 8.0, 500 mM NaCl and 0.75% CHAPS. Gel filtration fractions containing pure protein were pooled, concentrated and frozen in aliquots at −80 °C for subsequent analyses.
In vitro packaging of pos36GFP
O432-17(–) nanoparticles with pos36GFP cargoes were assembled in the same molar ratio of purified 1× tetramer, 1× Fc and 1.1× negatively charged trimeric plug from gel filtration fractions with 1× purified pos36GFP. Mixtures were dialyzed at 25 °C for 16 h into assembly buffer containing 25 mM Tris pH 8.0 and 200 mM NaCl, or 25 mM Tris pH 8.0 and 1 M NaCl. The ability of nanoparticles to package pos36GFP cargo was assessed using SEC on a Superose 6 10/300 GL column (Cytiva) in either buffer. Packaged GFP was quantified using the absorbance measurements at 280 and 488 nm obtained using a NanoDrop 8000 spectrophotometer. The absorbance of pure pos36GFP at 280 and 488 nm in 200 mM NaCl was used to calculate the absorbance at 280 nm due to pos36GFP in gel filtration fractions containing pos36GFP packaged in O432-17(–) nanoparticles. The relative absorbance due to pos36GFP and O432-17(–) nanoparticles was used to calculate the molar ratio of each protein using calculated extinction coefficients and was quantified against a standard curve generated using pure pos36GFP 36 .
In vitro packaging of pegRNA
O432-17(+) nucleocapsids with pegRNA (Integrated DNA Technologies) were assembled in a molar ratio of purified 1× tetramer, 1× CTX IgG and 1.1× positively charged trimeric plug from gel filtration fractions with 3× pegRNA per nucleocapsid. Mixtures were dialyzed at 25 °C for 16 h into 25 mM Tris pH 8.0 and 150 mM NaCl. Nucleocapsids were screened for in vitro encapsidation by native gel electrophoresis before and after a 1 h treatment with Benzonase or RNAse A.
Gel electrophoresis
Native agarose gels were prepared using 0.8% ultrapure agarose (Invitrogen) in TAE buffer (Thermo Fisher Scientific) containing SYBR Gold (Invitrogen). Nine microliters of O432-17(+) nucleocapsids were treated with 1 µl Benzonase (Invitrogen, diluted to 10 units per µl) or 1 µl RNAse A (Thermo Fisher Scientific, diluted to 10 units per µl) at 25 °C for 60 min, followed by mixing with 2 μl 6× loading dye (New England Biosciences, no SDS), and electrophoresed at 120 V for 30 min 17 , 19 . RNA in gels was imaged, and gels were subsequently stained with Gelcode Blue to analyze protein (Thermo Fisher Scientific).
Protein SDS–PAGE gels were performed using anyKD polyacrylamide gels (Bio-Rad) in Tris-glycine buffer and were electrophoresed at 180 V for 120 min.
In vitro assembly of O42.1 with pos36GFP
For each component, 1× O42.1 C4, 1× Fc and 1× pos36GFP per monomeric concentration were assembled and dialyzed for 16 h at 25 °C into 25 mM Tris pH 8.0 and 200 mM NaCl. The resulting assembly was screened using SEC on a Superose 6 10/300 GL column (Cytiva) in 25 mM Tris pH 8.0 and 200 mM NaCl.
Yeast display of Myc peptide
EBY100 S. cerevisiae cells were grown at 30 °C for 16 h in C-Trp-Ura medium supplemented with 2% (w/v) glucose, and were allowed to grow at 30 °C for 16 h in SGCAA expression medium to display myc-3×YPG peptide, followed by two washes in PBS + 3% BSA before incubation with O432-17 nanoparticle variants targeting the Myc peptide.
In vitro release of pos36GFP
O432-17(–) or O42.1 nanoparticles that had been in vitro assembled with pos36GFP were incubated with EBY100 S. cerevisiae cells expressing Myc-3×YPG peptide for 1 h at 25 °C. The cells were washed in 500 μl 25 mM Tris pH 8.0 and 500 mM NaCl until the supernatant fluorescence plateaued (after seven wash cycles) and were resuspended into citrate–phosphate buffer, pH 4.2, for 30 min at 25 °C. The supernatant was then collected and buffer exchanged using 25 mM Tris pH 8.0 and 500 mM NaCl before a fluorescence readout was generated using a Synergy Neo2 plate reader, with excitation wavelengths of 460 and 559 nm and emission wavelengths of 509 and 600 nm, and a gain of 120. Fluorescence was analyzed using GraphPad Prism version 9.3.1 for Windows, GraphPad Software ( www.graphpad.com ) and statistical significance was determined by two-way ANOVA with a 95% confidence interval and an alpha of 0.5.
Rational design of O432-17 pH-responsive variants
Bulky hydrophobic residues such as isoleucine and leucine amino acid positions within the pH-responsive trimeric interface were selected for optimization to either alanine or valine using the Rosetta software suite. The point substitution with the best scoring interface energy (ddG) at each position was selected as a trimeric plug variant. Combinatorial pairs of each point substitution variant were included as trimeric plug variants. We generated four O432-17 pH-responsive variants for both O432-17(–) and O432-17(+), two containing a third histidine hydrogen-bond network and either the p.I57V or p.L75A point substitution (3HIS_I57V and 3HIS_L75A), one containing a third histidine hydrogen-bond network and both p.I57V and p.L75A point substitutions (3HIS_ I57V_L75A) and one negative control containing zero histidine hydrogen-bond networks (0HIS), in which all histidines were mutated to asparagine.
AF647 conjugation to O432-17 trimeric plug variants
Trimeric plug variants containing a p.T359C substitution were generated by site-directed mutagenesis PCR. Alexa Fluor 647 C2 maleimide (Thermo Fisher Scientific) dissolved in DMSO and trimeric plug variants containing 10× TCEP were incubated in a 5:1 molar ratio with respect to the trimeric plug monomer for 16 h (overnight) at 4 °C in PBS + 0.75% CHAPS titrated to pH 7.2. The final reaction mixture contained 75 μM Alexa Fluor, 15 μM trimeric plug and 150 μM TCEP. The maleimide reaction was quenched with 1 mM DTT and buffer exchanged into 25 mM Tris pH 8.0 and 150 mM NaCl using PD-10 desalting columns with Sephadex-25 resin (Cytiva), according to the manufacturer’s protocol. It was then dialyzed for 3–4 days in 25 mM Tris pH 8.0, 150 mM NaCl and 0.75% CHAPS at 4 °C. Positively charged trimeric plug variants were buffer exchanged and dialyzed into 25 mM Tris pH 8.0, 500 mM NaCl and 0.75% CHAPS. The degree of labeling was estimated from a fluorophore extinction coefficient of 265,000 M −1 cm −1 , and absorbance measurements were taken at 280 and 650 nm using a NanoDrop 8000 spectrophotometer.
Flow-cytometry-based pH titration
Linear Myc peptide with an N-terminal lysine side chain and 3× glycine linker (KGGGEQKLISEEDL) was produced through solid-phase peptide synthesis and biotinylated through amide formation. The resulting biotinylated Myc peptide was purified by reversed-phase high-performance liquid chromatography and quality checked for the proper molecular weight using liquid chromatography–mass spectrometry, lyophilized and dissolved in 100% DMSO for long-term storage. Then, 3.0–3.4 μm streptavidin-coated polystyrene particles (Spherotech) were incubated with biotinylated Myc peptide diluted in PBS to 5% DMSO 1 h at 25 °C. The antibody to Myc was purchased from Cell Signaling Technologies (cat. no. 9B11). The coated particles were washed in PBS + 3% BSA twice and split equally into pH-titrated citrate–phosphate buffer for 30 min at 25 °C. Coated particles were washed twice with PBS + 3% BSA and resuspended for flow cytometry. For O432-17(+) experiments, we prevented non-specific association with the polystyrene particles by using EBY100 S. cerevisiae cells.
All flow-cytometry experiments were performed on a LSR II Flow Cytometer (BD Biosciences). Lasers calibrated with coated particles were stained with either FITC-labeled anti-Myc-tag antibody (Abcam cat. no. 9E10), APC-labeled anti-Myc-tag antibody (Abcam cat. no. 9E10) or no antibody. Ten thousand events were collected per sample on three biological replicates. All flow-cytometry results were analyzed using FlowJo. Singlet beads were first isolated before analysis for AF647 or sfGFP signal. Normalization to the minimum and maximum fluorescence of each channel with each titration sample was performed in Python3.7, and the apparent p K a of AF647 and sfGFP fluorescence was estimated with a four-parameter non-linear logistic regression fit in GraphPad Prism version 9.3.1 for Windows (GraphPad Software).
Wild-type HeLa (ATCC CCL-2), EGFR-knockout HeLa (Abcam ab255385) and A431 cells (ATCC CRL-1555) were cultured at 37 °C with 5% CO 2 in flasks with Dulbecco’s modified Eagle medium (DMEM) (Gibco) supplemented with 1 mM l -glutamine (Gibco), 4.5 g l –1 d -glucose (Gibco), 10% fetal bovine serum (FBS) (Hyclone) and 1% penicillin–streptomycin (PenStrep) (Gibco). Wild-type HeLa and EGFR-knockout HeLa cells were also cultured with 1× nonessential amino acids (Gibco) supplemented in the medium. Cells were passaged twice per week. For passaging, cells were dissociated using 0.05% trypsin EDTA (Gibco) and split 1:5 or 1:10 into a new tissue-culture-treated T75 flask (Thermo Fisher Scientific cat. no. 156499). For serum starvation, cells were cultured in their respective cell medium without FBS for 16 h.
Immunostaining
For immunostaining, 35-mm glass-bottom dishes were seeded at a density of 20,000 cells per dish. A final monomeric concentration of 10 nM of O432-17-CTX or O432-17-Fc nanoparticles (417 pM final nanoparticle concentration) were incubated with cultured cells in DMEM or DMEM supplemented with 10% FBS. Cells were fixed in 4% paraformaldehyde, permeabilized with 100% methanol and blocked with PBS + 1% BSA. Cells were immunostained with anti-LAMP2A antibody (Abcam cat. no. ab18528) and/or anti-NaK ATPase (Abcam cat. no. ab76020, Abcam cat. no. ab283318), followed by Alexa Fluor 488-conjugated goat anti-rabbit-IgG secondary antibody (Thermo Fisher Scientific cat. no. A-11034) or Alexa Fluor 488-conjugated goat anti-mouse-IgG secondary antibody (Thermo Fisher Scientific cat. no. A-11029) and DAPI (Thermo Fisher Scientific cat. no. D1306), and were stored in the dark at 4 °C until imaging.
Nanoparticle uptake epifluorescence microscopy (A431 cells)
Cells were washed twice with FluoroBrite DMEM imaging medium and subsequently imaged in the same medium in the dark at room temperature. Epifluorescence imaging was performed on a Yokogawa CSU-X1 spinning dish confocal microscope with either a Lumencor Celesta light engine with seven laser lines (408, 445, 473, 518, 545, 635 and 750 nm) or a Nikon LUN-F XL laser launch with four solid state lasers (405, 488, 561 and 640 nm). A 40× objective with a numerical aperture (NA) of 0.95 and a Hamamatsu ORCA-Fusion scientific CMOS camera, both controlled by NIS Elements software (Nikon), were used. The following excitation (EX)/emission (EM) filter combinations (center/bandwidth in nm) were used: BFP, EX408 EM443/38; GFP, EX473 EM525/36; RFP, EX545 EM605/52; far red, EX635 EM705/72. The exposure times were 100 ms for the acceptor direct channel and 500 ms for all other channels, with no EM gain set and no neutral density filter added.
Nanoparticle uptake image acquisition in wild-type and EGFR-knockout HeLa cells
Four-color, 3D images were acquired with a commercial OMX-SR system (GE Healthcare). Toptica diode lasers with excitations of 405 nm, 488 nm and 640 nm were used. Emission was collected on three separate PCO.edge sCMOS cameras using an Olympus 60× 1.420-NA PlanApochromat oil immersion lens; 1024 × 1024 images (pixel size, 6.5 μm) were captured with no binning. Acquisition was controlled with AcquireSR Acquisition control software. Z -stacks were collected with a step size of 125 nm. Images were deconvolved in SoftWoRx 7.0.0 (GE Healthcare) using the enhanced ratio method and 200 nm noise filtering. Images from different color channels were registered in SoftWoRx using parameters generated from a gold-grid registration slide (GE Healthcare).
Nanoparticle uptake quantification
To quantify the percentage of cells that were bound to nanocages, the Multi-point tool in Fiji (ImageJ) 61 was used to count nuclei in each image. The mRuby2 channel was used to identify and count cells, again using the multi-point tool, showing nanoparticle localization above the background signal using the identified nuclei as a guide for cell position. For cells positive for the LAMP2A nanoparticle signal, staining was used to aid in determining cell shape, and the freehand selection tool was used to draw manual regions of interest around the cell circumference. All regions of interest for positive cells were measured for cell area and integrated intensity in the mRuby2 channel for integrated intensity–cell area quantification. Cellular data were plotted and statistical tests were done in GraphPad Prism version 9.3.1 for Windows, GraphPad Software.
Statistics and reproducibility
Details on electron microscopy experiments can be found in Supplementary Table 4 . All gel electrophoresis experiments were replicated in at least two biologically independent experiments.
Reporting summary
Further information on research design is available in the Nature Portfolio Reporting Summary linked to this article.
Data availability
Source data for all images and data generated and analyzed by the authors are provided with this paper. Density maps have been deposited in the Electron Microscopy Data Bank under the accession number EMD- 29602 . Source data are provided with this paper.
Code availability
Source code for the fusion, docking and design of non-porous pH-responsive antibody nanoparticles is available at https://github.com/erincyang/plug_design . The protocol requires compilation of the worms and rpxdock repositories, which have been made available at https://github.com/willsheffler/worms (ref. 29 ) and https://github.com/willsheffler/rpxdock (ref. 31 ), respectively. Source code for generation of the figures in this manuscript was written by the authors and is provided in the Supplementary Information .
Edwardson, T. G. W., Mori, T. & Hilvert, D. Rational engineering of a designed protein cage for siRNA delivery. J. Am. Chem. Soc. 140 , 10439–10442 (2018).
Article CAS PubMed Google Scholar
Mitchell, M. J. et al. Engineering precision nanoparticles for drug delivery. Nat. Rev. Drug Discov. 20 , 101–124 (2021).
Banskota, S. et al. Engineered virus-like particles for efficient in vivo delivery of therapeutic proteins. Cell 185 , 250–265 (2022).
Article CAS PubMed PubMed Central Google Scholar
Wang, D., Tai, P. W. L. & Gao, G. Adeno-associated virus vector as a platform for gene therapy delivery. Nat. Rev. Drug Discov. 18 , 358–378 (2019).
Hou, X., Zaks, T., Langer, R. & Dong, Y. Lipid nanoparticles for mRNA delivery. Nat. Rev. Mater. 6 , 1078–1094 (2021).
Douglas, T. & Young, M. Viruses: making friends with old foes. Science 312 , 873–875 (2006).
Seo, J. et al. Fibrinolytic nanocages dissolve clots in the tumor microenvironment, improving the distribution and therapeutic efficacy of anticancer drugs. Exp. Mol. Med. 53 , 1592–1601 (2021).
Van de Steen, A. et al. Bioengineering bacterial encapsulin nanocompartments as targeted drug delivery system. Synth. Syst. Biotechnol. 6 , 231–241 (2021).
Article PubMed PubMed Central Google Scholar
Azuma, Y., Edwardson, T. G. W. & Hilvert, D. Tailoring lumazine synthase assemblies for bionanotechnology. Chem. Soc. Rev. 47 , 3543–3557 (2018).
Sigl, C. et al. Programmable icosahedral shell system for virus trapping. Nat. Mater. 20 , 1281–1289 (2021).
Ijäs, H., Hakaste, I., Shen, B., Kostiainen, M. A. & Linko, V. Reconfigurable DNA origami nanocapsule for pH-controlled encapsulation and display of cargo. ACS Nano 13 , 5959–5967 (2019).
Hu, Y. et al. Dynamic DNA assemblies in biomedical applications. Adv. Sci. 7 , 2000557 (2020).
Article CAS Google Scholar
Ried, M. U., Girod, A., Leike, K., Büning, H. & Hallek, M. Adeno-associated virus capsids displaying immunoglobulin-binding domains permit antibody-mediated vector retargeting to specific cell surface receptors. J. Virol. 76 , 4559–4566 (2002).
Cannon, K. A., Nguyen, V. N., Morgan, C. & Yeates, T. O. Design and characterization of an icosahedral protein cage formed by a double-fusion protein containing three distinct symmetry elements. ACS Synth. Biol. 9 , 517–524 (2020).
Lai, Y.-T., King, N. P. & Yeates, T. O. Principles for designing ordered protein assemblies. Trends Cell Biol. 22 , 653–661 (2012).
King, N. P. et al. Accurate design of co-assembling multi-component protein nanomaterials. Nature 510 , 103–108 (2014).
Butterfield, G. L. et al. Evolution of a designed protein assembly encapsulating its own RNA genome. Nature 552 , 415–420 (2017).
Votteler, J. et al. Designed proteins induce the formation of nanocage-containing extracellular vesicles. Nature 540 , 292–295 (2016).
Tetter, S. et al. Evolution of a virus-like architecture and packaging mechanism in a repurposed bacterial protein. Science 372 , 1220–1224 (2021).
Levasseur, M. D. et al. Cell-specific delivery using an engineered protein nanocage. ACS Chem. Biol. 16 , 838–843 (2021).
Iijima, Y. et al. Cell-specific targeting of a thymidine kinase/ganciclovir gene therapy system using a recombinant Sindbis virus vector. Int. J. Cancer 80 , 110–118 (1999).
Volpers, C. et al. Antibody-mediated targeting of an adenovirus vector modified to contain a synthetic immunoglobulin G-binding domain in the capsid. J. Virol. 77 , 2093–2104 (2003).
Kickhoefer, V. A. et al. Targeting vault nanoparticles to specific cell surface receptors. ACS Nano 3 , 27–36 (2009).
Kim, H., Kang, Y. J., Min, J., Choi, H. & Kang, S. Development of an antibody-binding modular nanoplatform for antibody-guided targeted cell imaging and delivery. RSC Adv. 6 , 19208–19213 (2016).
Rujas, E. et al. Multivalency transforms SARS-CoV-2 antibodies into ultrapotent neutralizers. Nat. Commun. 12 , 3661 (2021).
Divine, R. et al. Designed proteins assemble antibodies into modular nanocages. Science 372 , eabd9994 (2021).
Yang, E. C. De Novo Design of Protein Nanoparticles With Programmable and Tunable Function. PhD thesis, Univ. Washington (2023).
Boyken, S. E. et al. De novo design of tunable, pH-driven conformational changes. Science 364 , 658–664 (2019).
Hsia, Y. et al. Design of multi-scale protein complexes by hierarchical building block fusion. Nat. Commun. 12 , 2294 (2021).
Padilla, J. E., Colovos, C. & Yeates, T. O. Nanohedra: using symmetry to design self assembling protein cages, layers, crystals, and filaments. Proc. Natl Acad. Sci. USA 98 , 2217–2221 (2001).
Sheffler, W. et al. Fast and versatile sequence-independent protein docking for nanomaterials design using RPXDock. PLoS Comput. Biol. 19 , e1010680 (2023).
Fallas, J. A. et al. Computational design of self-assembling cyclic protein homo-oligomers. Nat. Chem. 9 , 353–360 (2017).
Lawrence, M. C. & Colman, P. M. Shape complementarity at protein/protein interfaces. J. Mol. Biol. 234 , 946–950 (1993).
Leman, J. K. et al. Macromolecular modeling and design in Rosetta: recent methods and frameworks. Nat. Methods 17 , 665–680 (2020).
Roux, K. H., Strelets, L. & Michaelsen, T. E. Flexibility of human IgG subclasses. J. Immunol. 159 , 3372–3382 (1997).
Bale, J. B. et al. Accurate design of megadalton-scale two-component icosahedral protein complexes. Science 353 , 389–394 (2016).
Edwardson, T. G. W., Levasseur, M. D. & Hilvert, D. The OP protein cage: a versatile molecular delivery platform. Chimia 75 , 323–328 (2021).
Hsu, J. Y. et al. PrimeDesign software for rapid and simplified design of prime editing guide RNAs. Nat. Commun. 12 , 1034 (2021).
Anzalone, A. V. et al. Search-and-replace genome editing without double-strand breaks or donor DNA. Nature 576 , 149–157 (2019).
Nelson, J. W. et al. Engineered pegRNAs improve prime editing efficiency. Nat. Biotechnol. 40 , 402–410 (2022).
Zuris, J. A. et al. Cationic lipid-mediated delivery of proteins enables efficient protein-based genome editing in vitro and in vivo. Nat. Biotechnol. 33 , 73–80 (2015).
Hu, Y.-B., Dammer, E. B., Ren, R.-J. & Wang, G. The endosomal-lysosomal system: from acidification and cargo sorting to neurodegeneration. Transl. Neurodegener. 4 , 18 (2015).
Meanor, J. N., Keung, A. J., Rao, B. M. & Gera, N. Yeast display guided selection of pH-dependent binders. Methods Mol. Biol . 2491 , 293–311 (2022).
Schröter, C. et al. A generic approach to engineer antibody pH-switches using combinatorial histidine scanning libraries and yeast display. mAbs 7 , 138–151 (2015).
Gaggero, S. et al. IL-2 is inactivated by the acidic pH environment of tumors enabling engineering of a pH-selective mutein. Sci. Immunol. 7 , eade5686 (2022).
Sun, X., Hong, Y., Gong, Y., Zheng, S. & Xie, D. Bioengineered ferritin nanocarriers for cancer therapy. Int. J. Mol. Sci. 22 , 7023 (2021).
Sutter, M. et al. Structural basis of enzyme encapsulation into a bacterial nanocompartment. Nat. Struct. Mol. Biol. 15 , 939–947 (2008).
Steinmetz, N. F., Lim, S. & Sainsbury, F. Protein cages and virus-like particles: from fundamental insight to biomimetic therapeutics. Biomater. Sci. 8 , 2771–2777 (2020).
Hsia, Y. et al. Design of a hyperstable 60-subunit protein icosahedron. Nature 535 , 136–139 (2016).
Lavelle, L., Michel, J.-P. & Gingery, M. The disassembly, reassembly and stability of CCMV protein capsids. J. Virol. Methods 146 , 311–316 (2007).
Chen, H., Zhang, S., Xu, C. & Zhao, G. Engineering protein interfaces yields ferritin disassembly and reassembly under benign experimental conditions. Chem. Commun. 52 , 7402–7405 (2016).
Dalmau, M., Lim, S. & Wang, S.-W. pH-triggered disassembly in a caged protein complex. Biomacromolecules 10 , 3199–3206 (2009).
Kim, M. et al. pH-dependent structures of ferritin and apoferritin in solution: disassembly and reassembly. Biomacromolecules 12 , 1629–1640 (2011).
Pettersen, E. F. et al. UCSF ChimeraX: structure visualization for researchers, educators, and developers. Protein Sci. 30 , 70–82 (2021).
Brunette, T. J. et al. Exploring the repeat protein universe through computational protein design. Nature 528 , 580–584 (2015).
Zivanov, J. et al. New tools for automated high-resolution cryo-EM structure determination in RELION-3. eLife 7 , e42166 (2018).
Zhang, K.Gctf: real-time CTF determination and correction. J. Struct. Biol. 193 , 1–12 (2016).
Punjani, A., Rubinstein, J. L., Fleet, D. J. & Brubaker, M. A. cryoSPARC: algorithms for rapid unsupervised cryo-EM structure determination. Nat. Methods 14 , 290–296 (2017).
Tyka, M. D. et al. Alternate states of proteins revealed by detailed energy landscape mapping. J. Mol. Biol. 405 , 607–618 (2011).
Studier, F. W. Protein production by auto-induction in high density shaking cultures. Protein Expr. Purif. 41 , 207–234 (2005).
Schindelin, J. et al. Fiji: an open-source platform for biological-image analysis. Nat. Methods 9 , 676–682 (2012).
Download references
Acknowledgements
We thank J. Lazarovitz, W. L. White, S. Boyken, C. Richardson, D. Chmielewski, I. Yousif, H. Choi, S. J. Hendel, Y. Hsia, M. Wu, G. Reggiano, A. Olshefsky, K. Van Wormer, R. Krishnamurty, K. Herrera, L. Goldschmidt and L. Stewart for helpful discussions and general tasks related to the study, L. Carter and M. Miranda for help with SEC-MALS, M. Murphy and C. Ogohara for assistance with mammalian proteins and H. Bai and K. Wu for providing EBY100 cells displaying Myc-3×YPG. This research was funded by the NSF Grant CHE-1629214 (N.P.K. and D.B.), Defense Threat Reduction Agency Grants HDTRA1-18-1-0001 and HDTRA1-19-1-0003 (N.P.K. and D.B.), the grant DE-SC0018940 funded by the U.S. Department of Energy, Office of Science (D.B.), National Institutes of Health’s National Institute on Aging grants R01AG063845 and 1R01CA240339 (N.P.K. and D.B.), the Bill and Melinda Gates Foundation no. INV-010680 (N.P.K. and D.B.), the Audacious Project at the Institute for Protein Design, Washington Research Foundation and Translational Research Fund, National Science Foundation Graduate Research Fellowship program under the grant number DGE-1762114 (E.C.Y.), the Helen Hay Whitney Foundation (J.Z.Z.), the DAAD PROMOS program (N.G.) and the Howard Hughes Medical Institute (D.B.).
Author information
Authors and affiliations.
Institute for Protein Design, University of Washington, Seattle, WA, USA
Erin C. Yang, Robby Divine, Marcos C. Miranda, Andrew J. Borst, Will Sheffler, Jason Z. Zhang, Justin Decarreau, Amijai Saragovi, Mohamad Abedi, Nicolas Goldbach, Maggie Ahlrichs, Craig Dobbins, Alexis Hand, Suna Cheng, Mila Lamb, Paul M. Levine, Sidney Chan, Rebecca Skotheim, Jorge Fallas, George Ueda, Joshua Lubner, Masaharu Somiya, Alena Khmelinskaia, Neil P. King & David Baker
Department of Biochemistry, University of Washington, Seattle, WA, USA
Erin C. Yang, Robby Divine, Andrew J. Borst, Jason Z. Zhang, Justin Decarreau, Amijai Saragovi, Mohamad Abedi, Maggie Ahlrichs, Craig Dobbins, Alexis Hand, Suna Cheng, Mila Lamb, Paul M. Levine, Sidney Chan, Rebecca Skotheim, Jorge Fallas, George Ueda, Joshua Lubner, Neil P. King & David Baker
Graduate Program in Biological Physics, Structure & Design, University of Washington, Seattle, WA, USA
Erin C. Yang
Graduate Program in Biochemistry, University of Washington, Seattle, WA, USA
Robby Divine
Department of Chemistry, University of California, Davis, Davis, CA, USA
Department of Medicine Solna, Division of Immunology and Allergy, Karolinska Institutet and Karolinska University Hospital, Stockholm, Sweden
Marcos C. Miranda
Technical University of Munich, Munich, Germany
Nicolas Goldbach
SANKEN, Osaka University, Osaka, Japan
Masaharu Somiya
Transdisciplinary Research Area ‘Building Blocks of Matter and Fundamental Interactions (TRA Matter)’, University of Bonn, Bonn, Germany
Alena Khmelinskaia
Life and Medical Sciences Institute, University of Bonn, Bonn, Germany
Howard Hughes Medical Institute, University of Washington, Seattle, WA, USA
David Baker
You can also search for this author in PubMed Google Scholar
Contributions
E.C.Y., R.D., N.P.K. and D.B. designed the research. E.C.Y., R.D., M.C.M, A.J.B., W.S., J.Z.Z., J.D., A.S., M. Abedi, N.G., M. AAhlrichs, C.D., A.H., S. Cheng, M.L., P.M.L., S. Chan, R.S., J.F., G.U., J.L., M.S. and A.K acquired, analyzed or interpreted the data. E.C.Y., W.S. and D.B. created software for the research. E.C.Y., N.P.K. and D.B. wrote the paper.
Corresponding authors
Correspondence to Neil P. King or David Baker .
Ethics declarations
Competing interests.
A provisional patent application has been filed (63/493,252) on the plugged antibody nanoparticle sequences by the University of Washington, listing D.B., E.C.Y., N.P.K., R.D., J.L., W.S., G.U., and J.F. as inventors. The other authors declare no competing interests.
Peer review
Peer review information.
Nature Structural & Molecular Biology thanks Tom Edwardson, Stefan Howorka and the other, anonymous, reviewer(s) for their contribution to the peer review of this work. Peer reviewer reports are available. Primary Handling Editor: Sara Osman, in collaboration with the Nature Structural & Molecular Biology team.
Additional information
Publisher’s note Springer Nature remains neutral with regard to jurisdictional claims in published maps and institutional affiliations.
Extended data
Extended data fig. 1 reducing sds–page screening gels of co-expressed trimer-tetramer variants 1–45..
Tetramer protein band is marked (right), designs where the designed trimers and tetramers co-eluted are marked with pink stars next to the trimer band. Gel samples were derived from the same experiment and electrophoresis was processed in parallel.
Extended Data Fig. 2 Example experimental screen of O432 three−component nanoparticles.
a , Clarified lysates of 16 designs where the designed trimer co-eluted with the tetramer were supplemented with a purified sfGFP-Fc fusion protein, purified by IMAC, and subject to reducing SDS-PAGE. Trimer protein bands selected for subsequent characterization are marked with pink stars. b , Non-denaturing native PAGE gel electrophoresis of clarified lysates from 16 designs, supplemented with purified sfGFP-Fc fusion protein and purified by IMAC. All designs were compared against the previously designed, two-component antibody nanoparticle (O42.1), O42.1 tetrameric component (O42.1 C4) and sfGFP-Fc (Divine et al. 26 ). Gel samples were derived from the same experiment and electrophoresis was processed in parallel.
Extended Data Fig. 3 Large-scale assembly competency screen of O432 three-component nanoparticles.
a , Subcloned components from putative three-component assemblies were purified separately. Peaks marked with a pink star were collected for stoichiometric in vitro assembly of three-component O432 nanoparticles. b , Elution profiles of tetrameric components were compared to the original O42.1 tetramer (O42.1 C4). c , Material permitting, stoichiometric in vitro assembly of trimer, tetramer, and Fc were purified by SEC, d , Elution profiles of resulting assemblies were compared to the SEC elution profile of previously designed O42.1 (Divine et al. 26 ). e , Non-reducing SDS-PAGE of the void (V) and shoulder (S) peaks from SEC of O432-17 with purified trimer, tetramer, and Fc components as controls. f , Non-reducing SDS-PAGE of the void (V) and shoulder (S) peaks from SEC purification of O432-23, O432-7, and O432-34 in vitro assemblies. g , 6× designed tetramers form protein-protein interfaces with both 12× Fc and 8× designed trimers. A schematic depicts a hypothetical nanoparticle assembly from the designed tetramer with only the Fc (top) or only the trimer (bottom). h , Representative SEC traces on the Superose 6 10/300 GL of assembly reactions containing only the designed tetramer and Fc (pink), compared to the full three-component assembly (black) and the individual components (gray and purple). i , Representative SEC traces on the Superdex 200 10/300 GL of assembly reactions containing only the designed tetramer and trimer at concentrations of 50 µM, 100 µM, 200 µM, 300 µM, and 400 µM, compared to the individual components (gray and yellow). UCSF ChimeraX 1.6 and the PyMOL Molecular Graphics System, version 2.5 (Schrödinger) was used to create g .
Extended Data Fig. 4 Cryo-EM processing shows newly designed trimeric plug occupying all 3-fold symmetry axes of the nanoparticle octahedral architecture.
a , Representative micrograph of cryo-EM sample. Scale bar, 100 nm. b , Reference-free two-dimensional class averages. Scale bar, 10 nm. c , Ab initio three-dimensional reconstruction without applied octahedral symmetry. d , 3D reconstructions generated following a heterogeneous refinement in the absence of applied octahedral symmetry. All four classes show trimeric plugs occupying all facets of the designed nanoparticle. e , Gold-standard Fourier shell correlation curves for the O432-Fc EM density map with octahedral symmetry applied. f , Close up of plug interface between design model (light gray and yellow) and model built from the 3D reconstruction (dark gray). UCSF ChimeraX 1.6 and the PyMOL Molecular Graphics System, version 2.5 (Schrödinger) was used to create f .
Extended Data Fig. 5 Comparison of design models of O42.1 and O432-17 with models fit into Cryo-EM density.
a , The three-component O432-17 design model (light gray, purple, and yellow) overlaid on the two-component O42.1 density-refined model (dark gray) shows an RMSD of 1.9 Å. b , The O432-17 design model (light gray, purple, and yellow) deviates from its density-refined model (dark gray) by 1.6 Å. c , The O42.1 design model deviates from its density-refined model (dark gray) by 4.2 Å. The PyMOL Molecular Graphics System, version 2.5 (Schrödinger) was used to create a-c .
Extended Data Fig. 6 O432-17 nanoparticles form assemblies in the presence of protein and nucleic acid cargo.
a , Representative negative stain electron micrograph of O432-17(+) in the presence of RNA. Scale bar, 100 nm. b , Reference-free two-dimensional class averages showing multiple views of O432-17(+) nanoparticles. Scale bar, 10 nm. c , Representative negative-stain electron micrograph of O432-17(-) in the presence of pos36GFP. Scale bar, 100 nm. d , Reference-free two-dimensional class averages showing multiple views of O432-17(-) nanoparticles. Scale bar, 10 nm.
Extended Data Fig. 7 Assembly competency of O432-17(+) trimer variants.
a , SEC elution profile of the trimer component on a Superdex 200 GL 10/300 chromatography column. b , SEC elution profile of the full O432-17 assembly containing each trimer variant assembled with tetramer and Fc and purified on a Superose 6 GL 10/300 chromatography column. c , DLS profile of the resulting assembly as normalized intensity. d , Representative nsEM and reference-free two-dimensional class averages of each assembly variant. Scale bar 100 nm and 10 nm, respectively.
Extended Data Fig. 8 Flow cytometry analysis of O432-17 nanoparticle variants.
a , Forward and side scattering intensity isolated all beads. b , Isolated beads were selected for singlet scattering. c , Singlet beads were gated for both AF647 and sfGFP-Fc signal with reference to negative controls: beads only (left), beads incubated with sfGFP-Fc (middle), and beads incubated with AF647-labeled trimeric plug (right). d , The mean fluorescence intensity of beads positive for AF647-labeled trimer and sfGFP-Fc signal was taken at each pH. This gating strategy was applied across all trimeric plug variants: 0HIS, 2HIS, 3HIS_I57V, 3HIS_L75A, 3HIS_I57V_L75A. e-f , Mean fluorescence intensity of O432-17(+) nanoparticles was measured as a function of pH for the trimeric plug variants and sfGFP-Fc. Histidine-containing O432-17(+) variants: O432-17(+)_3HIS_I57V, O432-17(+)_3HIS_L75A, O432-17(+)_3HIS_I57V_L75A); negative control: O432-17(+)_0HIS. Apparent pKas for O432-17(+)_3HIS_I57V, O432-17(+)_3HIS_L75A, and O432-17(+)_3HIS_I57V_L75A: pH 6.1 (AF647); pH 5.8 (sfGFP). O432-17(+)_0HIS apparent pKa: pH 5.1 (AF647); pH 5.0 (sfGFP). Data are presented as mean values +/- SEM over 3 biologically independent replicates. FlowJo v10.8.1(BD Biosciences) was used to create a, b, c , and d . GraphPad Prism version 9.3.1 (GraphPad Software) was used to create e and f .
Extended Data Fig. 9 Assembly competency of O432-17(-) trimer variants.
Extended data fig. 10 quantification of targeted receptor-mediated uptake of o432 nanoparticles..
a , Targeted and non-targeted variants of O432-17(-) nanoparticles were assembled in vitro with AF647-conjugated trimeric plug, designed tetramer, and either premixed mRuby2-Fc and α-EGFR mAb (top) or mRuby2-Fc alone (bottom). b , Cellular uptake of O432-17-CTX and O432-17-Fc nanoparticles was measured by the AF647 and mRuby2 fluorescence within the cell area after 3 hours of incubation in A431 cells. Single confocal plane images; grayscale panels for lysosomal membranes (green), mRuby2-Fc (red), and Trimer-AF647 (gray). Scale bar, 10 µm. c , Percentage of A431 cells containing nanoparticles. Statistics: *P = 0.0306, two-tailed unpaired t-test. Data are presented as mean values +/- SEM over 3 images per condition. d , Integrated intensity per cell area among A431 cells containing nanoparticles. Statistics: P = 0.6025, ns = not significant, two-tailed, unpaired T-test. Data are presented as mean values +/- SEM across all cells in 3 images per condition. e , Targeted receptor-mediated uptake of O432-17-CTX nanoparticles in WT and EGFR KO HeLa cells after 3 hours. Scale bar, 10 µm. f , Percentage of HeLa WT and HeLa EGFR KO cells containing O432-17-CTX nanoparticles with and without serum. Statistics: *P = 0.0116, ***P = 0.001, ****P < 0.0001, ns (P = 0.9349) = not significant, One-way ANOVA with Tukey’s correction for multiple comparisons. Data are presented as mean values +/- SEM over 3 images per condition. g , Integrated intensity per cell area among HeLa WT and HeLa EGFR KO cells containing O432-17-CTX nanoparticles with and without serum. Statistics: *P = 0.0359 (HeLa WT without serum vs. HeLa EGFR KO without serum), *P = 0.0161 (HeLa EGFR KO with serum vs. HeLa EGFR KO without serum), P = 0.9598 (HeLa WT with serum vs. HeLa WT without serum), P = 0.9298 (HeLa WT with serum vs. HeLa EGFR KO with serum), One-way ANOVA with Tukey’s correction for multiple comparisons. Data are presented as mean values +/- SEM across all cells in 3 images per condition; ImageJ v2 was used to create b,e ; GraphPad Prism version 9.3.1 (GraphPad Software) was used to create c-d, f-g .
Supplementary information
Supplementary information.
Supplementary Tables 1–9 and Figs. 1–4 containing uncropped gels for Extended Data figures.
Reporting Summary
Peer review file, source data fig. 2.
Plotting source data.
Source Data Fig. 4
Umodified gels.
Source Data Fig. 5
Statistical source data.
Source Data Extended Data Fig. 3
Source data extended data fig. 7, source data extended data fig. 8, source data extended data fig. 9, source data extended data fig. 10, rights and permissions.
Open Access This article is licensed under a Creative Commons Attribution 4.0 International License, which permits use, sharing, adaptation, distribution and reproduction in any medium or format, as long as you give appropriate credit to the original author(s) and the source, provide a link to the Creative Commons licence, and indicate if changes were made. The images or other third party material in this article are included in the article’s Creative Commons licence, unless indicated otherwise in a credit line to the material. If material is not included in the article’s Creative Commons licence and your intended use is not permitted by statutory regulation or exceeds the permitted use, you will need to obtain permission directly from the copyright holder. To view a copy of this licence, visit http://creativecommons.org/licenses/by/4.0/ .
Reprints and permissions
About this article
Cite this article.
Yang, E.C., Divine, R., Miranda, M.C. et al. Computational design of non-porous pH-responsive antibody nanoparticles. Nat Struct Mol Biol (2024). https://doi.org/10.1038/s41594-024-01288-5
Download citation
Received : 24 February 2023
Accepted : 22 March 2024
Published : 09 May 2024
DOI : https://doi.org/10.1038/s41594-024-01288-5
Share this article
Anyone you share the following link with will be able to read this content:
Sorry, a shareable link is not currently available for this article.
Provided by the Springer Nature SharedIt content-sharing initiative
Quick links
- Explore articles by subject
- Guide to authors
- Editorial policies
Sign up for the Nature Briefing newsletter — what matters in science, free to your inbox daily.

Nicholas Hatsopoulos receives the Quantrell Awards for Excellence in Undergraduate Teaching
May 17, 2024
The University annually recognizes faculty for their incredible teaching and mentoring of undergraduate and graduate students through the Llewellyn John and Harriet Manchester Quantrell Awards , believed to be the nation’s oldest prize for undergraduate teaching.
In 2024, five faculty members received this award. Professor Nicholas Hatsopoulos, PhD from the Department of Organismal Biology and Anatomy is among them. Learn more below:
Nicholas Hatsopoulos, Professor, Organismal Biology and Anatomy
Nicholas Hatsopoulos teaches a course titled "Neuroscience of Consciousness," delving into a subject that has intrigued him since his undergraduate days, when he minored in philosophy.
He has always been fascinated by questions surrounding free will, which propelled him into this field of study. Though consciousness is not his primary area of research as a neuroscience professor, Hatsopoulos said he finds immense joy in engaging with his students and the lively discussions that ensue during his lectures.
"I love the interactions I have with the students and all the questions they ask during my lectures,” he said. “The students here are really smart and inquisitive. They genuinely want to learn and not just get a good grade."
Hatsopoulos fosters an environment of active participation in his classroom. He encourages interruptions and questions, believing that dialogue is essential for deep learning. If he doesn’t know the answer to a question, he is not afraid to say he doesn’t know but says he’ll try to get an answer by the next class.
He assigns students the task of critiquing two papers they read each week, promptly discussing some of the submissions in the following class. Throughout the course, debates on consciousness-related topics stimulate further exploration and critical thinking.
“I want them to interrupt me and ask questions,” he said. "The message I give them at the beginning of the course is telling them that we won't ultimately answer the question as to how consciousness arises from the brain, but hopefully they will learn about some of the experiments and theories and learn some neuroscience in the process.”
Suggestions or feedback?
MIT News | Massachusetts Institute of Technology
- Machine learning
- Social justice
- Black holes
- Classes and programs
Departments
- Aeronautics and Astronautics
- Brain and Cognitive Sciences
- Architecture
- Political Science
- Mechanical Engineering
Centers, Labs, & Programs
- Abdul Latif Jameel Poverty Action Lab (J-PAL)
- Picower Institute for Learning and Memory
- Lincoln Laboratory
- School of Architecture + Planning
- School of Engineering
- School of Humanities, Arts, and Social Sciences
- Sloan School of Management
- School of Science
- MIT Schwarzman College of Computing
Four from MIT named 2024 Knight-Hennessy Scholars
Press contact :.
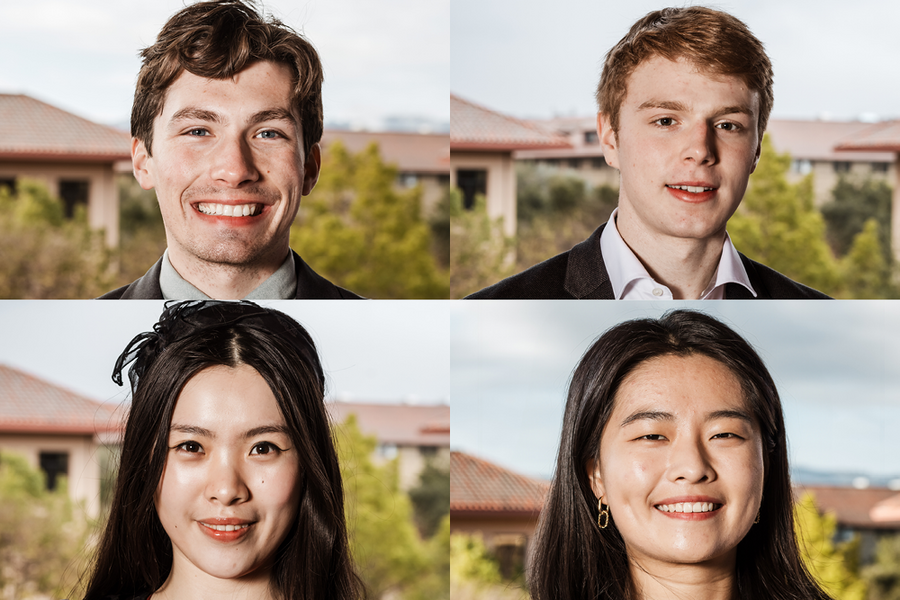
Previous image Next image
MIT senior Owen Dugan, graduate student Vittorio Colicci ’22, predoctoral research fellow Carine You ’22, and recent alumna Carina Letong Hong ’22 are recipients of this year’s Knight-Hennessy Scholarships. The competitive fellowship, now in its seventh year, funds up to three years of graduate studies in any field at Stanford University. To date, 22 MIT students and alumni have been awarded Knight-Hennessy Scholarships.
“We are excited for these students to continue their education at Stanford with the generous support of the Knight Hennessy Scholarship,” says Kim Benard, associate dean of distinguished fellowships in Career Advising and Professional Development. “They have all demonstrated extraordinary dedication, intellect, and leadership, and this opportunity will allow them to further hone their skills to make real-world change.”
Vittorio Colicci ’22
Vittorio Colicci, from Trumbull, Connecticut, graduated from MIT in May 2022 with a BS in aerospace engineering and physics. He will receive his master’s degree in planetary sciences this spring. At Stanford, Colicci will pursue a PhD in earth and planetary sciences at the Stanford Doerr School of Sustainability. He hopes to investigate how surface processes on Earth and Mars have evolved through time alongside changes in habitability. Colicci has worked largely on spacecraft engineering projects, developing a monodisperse silica ceramic for electrospray thrusters and fabricating high-energy diffraction gratings for space telescopes. As a Presidential Graduate Fellow at MIT, he examined the influence of root geometry on soil cohesion for early terrestrial plants using 3D-printed reconstructions. Outside of research, Colicci served as co-director of TEDxMIT and propulsion lead for the MIT Rocket Team. He is also passionate about STEM engagement and outreach, having taught educational workshops in Zambia and India.
Owen Dugan, from Sleepy Hollow, New York, is a senior majoring in physics. As a Knight-Hennessy Scholar, he will pursue a PhD in computer science at the Stanford School of Engineering. Dugan aspires to combine artificial intelligence and physics, developing AI that enables breakthroughs in physics and using physics techniques to design more capable and safe AI systems. He has collaborated with researchers from Harvard University, the University of Chicago, and DeepMind, and has presented his first-author research at venues including the International Conference on Machine Learning, the MIT Mechanistic Interpretability Conference, and the American Physical Society March Meeting. Among other awards, Dugan is a Hertz Finalist, a U.S. Presidential Scholar, an MIT Outstanding Undergraduate Research Awardee, a Research Science Institute Scholar, and a Neo Scholar. He is also a co-founder of VeriLens, a funded startup enabling trust on the internet by cryptographically verifying digital media.
Carina Letong Hong ’22
Carina Letong Hong, from Canton, China, is currently pursuing a JD/PhD in mathematics at Stanford. A first-generation college student, Hong graduated from MIT in May 2022 with a double major in mathematics and physics and was inducted into Sigma Pi Sigma, the physics honor society. She then earned a neuroscience master’s degree with dissertation distinctions from the University of Oxford, where she conducted artificial intelligence and machine learning research at Sainsbury Wellcome Center’s Gatsby Unit. At Stanford Law School, Hong provides legal aid to low-income workers and uses economic analysis to push for law enforcement reform. She has published numerous papers in peer-reviewed journals, served as an expert referee for journals and conferences, and spoken at summits in the United States, Germany, France, the U.K., and China. She was the recipient of the AMS-MAA-SIAM Morgan Prize for Outstanding Research, the highest honor for an undergraduate in mathematics in North America; the AWM Alice T. Schafer Prize for Mathematical Excellence, given annually to an undergraduate woman in the United States; the Maryam Mirzakhani Fellowship; and a Rhodes Scholarship.
Carine You ’22
Carine You, from San Diego, California, graduated from MIT in May 2022 with bachelor’s degrees in electrical engineering and computer science and in mathematics. Since graduating, You has worked as a predoctoral research assistant with Professor Amy Finkelstein in the MIT Department of Economics, where she has studied the quality of Medicare nursing home care and the targeting of medical screening technologies. This fall, You will embark on a PhD in economic analysis and policy at the Stanford Graduate School of Business. She wishes to address pressing issues in environmental and health-care markets, with a particular focus on economic efficiency and equity. You previously developed audio signal processing algorithms at Bose, refined mechanistic models to inform respiratory monitoring at the MIT Research Laboratory of Electronics, and analyzed corruption in developmental projects in India at the World Bank. Through Middle East Entrepreneurs of Tomorrow, she taught computer science to Israeli and Palestinian students in Jerusalem and spearheaded an online pilot expansion for the organization. At MIT, she was named a Burchard Scholar.
Share this news article on:
Related links.
- Knight-Hennessy Scholars
Related Topics
- Awards, honors and fellowships
- Undergraduate
- Graduate, postdoctoral
- Aeronautical and astronautical engineering
- Electrical Engineering & Computer Science (eecs)
- Mathematics
- Research Laboratory of Electronics
- School of Humanities Arts and Social Sciences
Related Articles
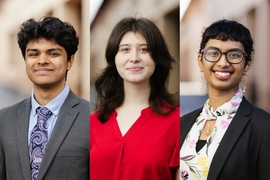
Three from MIT named 2023 Knight-Hennessy Scholars
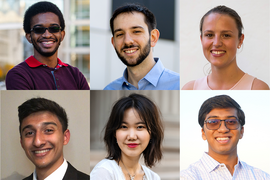
Six from MIT Named 2022 Knight-Hennessy Scholars
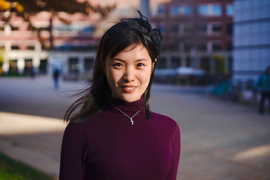
Carina Letong Hong named a 2022 Rhodes Scholar for China
Previous item Next item
More MIT News
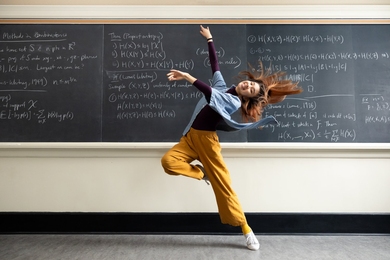
Janabel Xia: Algorithms, dance rhythms, and the drive to succeed
Read full story →
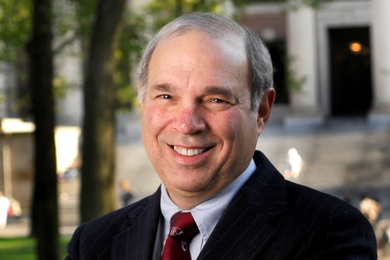
Jonathan Byrnes, MIT Center for Transportation and Logistics senior lecturer and visionary in supply chain management, dies at 75
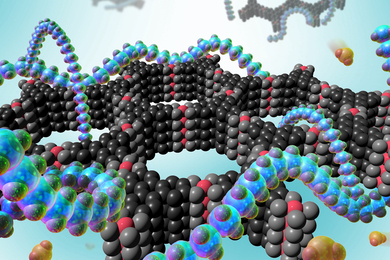
Researchers develop a detector for continuously monitoring toxic gases
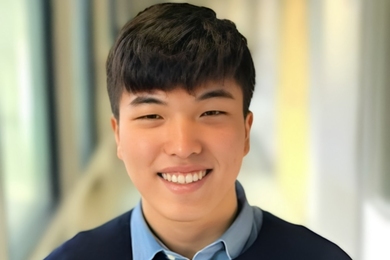
The beauty of biology
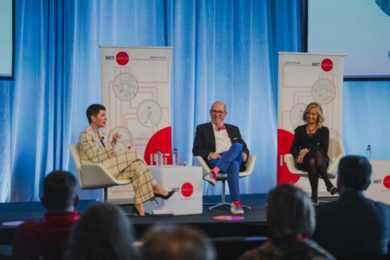
Navigating longevity with industry leaders at MIT AgeLab PLAN Forum
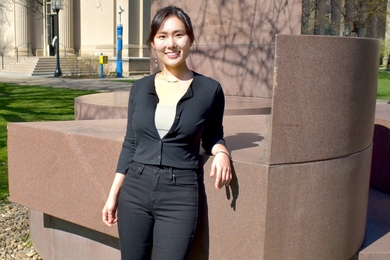
Jeong Min Park earns 2024 Schmidt Science Fellowship
- More news on MIT News homepage →
Massachusetts Institute of Technology 77 Massachusetts Avenue, Cambridge, MA, USA
- Map (opens in new window)
- Events (opens in new window)
- People (opens in new window)
- Careers (opens in new window)
- Accessibility
- Social Media Hub
- MIT on Facebook
- MIT on YouTube
- MIT on Instagram
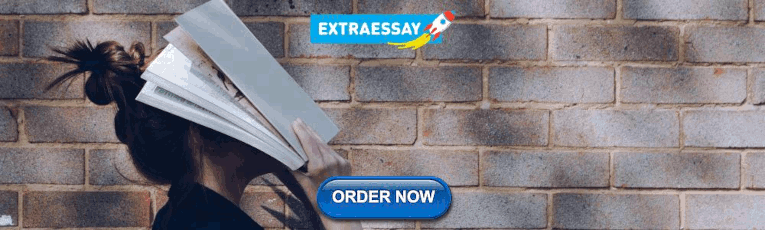
IMAGES
VIDEO
COMMENTS
We provide an excellent, international and interdisciplinary research environment with state-of-the-art facilities and a renowned faculty. Positions are fully funded for 3 years with possible extension. Salary is based upon the framework of a Max Planck Society doctoral funding contract (TVöD 13, 65%). We are committed to increasing the number ...
Applicants should possess. a Bachelor's degree (6 semester) in Biology, Bioinformatics or in Computational Sciences (with a minor in the field of Bioinformatics or Biology). Application is also possible for students who passed at least 3/4 of their Bachelor (150 of 180 C). conditions for applicants with - major in Biology: at least 50 C in Biology and 40 C in Bioinformatics, Informatics ...
The International Max Planck Research School for Computational Biology and Scientific Computing (IMPRS-CBSC) originated in a joint initiative of the Max Planck Institute for Molecular Genetics and Freie Universität Berlin. Building on the existing master's degree programs in bioinformatics and scientific computing at Freie Universität ...
IMB's International PhD Programme / Program gives talented and enthusiastic students the opportunity to undertake PhD research at the cutting edge of modern biology. ... Bioinformatics & computational biology; Gene regulation & evolution; ... Germany. E-Mail: info(at)imb.de Phone: +49-6131-39-21501 Sitemap; Impressum;
About. Computational Biology at TU Dresden addresses problems in biology, biomedicine and ecology through image analysis, theory, computer simulations and data visualization. TU Dresden. Dresden , Germany. Top 1% worldwide. Studyportals University Meta Ranking. 4.3 Read 46 reviews.
PhD subject areas; Computational Biology. Computational Biology is concerned with the development and application of computer-based methods for the analysis of biological data and the creation of mathematical and statistical models that can be used to describe and simulate biological processes. Here, both data-driven approaches from the field ...
The Max Planck Institute for Plant Breeding Research in Cologne, Germany and the University of Cologne, Germany have opened 11 fully funded PhD students positions in the computational and experimental plant sciences embedded in an International Max Planck Research School (IMPRS).. The call closes on January 6th 2024 and successful candidates are expected to start the PhD positions between ...
PhD Studentship Opportunities in the overall Field of Cognition Research. The Max Planck School of Cognition (MPS-Cog) is a joint graduate program of the Max Planck Society and leading German Universities together with Fraunhofer-Gesellschaft, Helmholtz Association, and University College London, and is additionally funded by the Federal ...
PhD position in Computational and Developmental Biology in Berlin (m/f/d) job in Berlin with Helmholtz Association of German Research Centres. Apply Today.
The Max Planck Institutes for Multidisciplinary Sciences and for Dynamics and Self-Organization have teamed up with the University of Göttingen to establish the scientific programs Genome Science , Molecular Biology, Neurosciences , and Physics of Complex and Biological Systems. The structured education and training offers excellent research ...
Below is a list of best universities in Germany ranked based on their research performance in Bioinformatics and Computational biology. A graph of 7.07M citations received by 171K academic papers made by 86 universities in Germany was used to calculate publications' ratings, which then were adjusted for release dates and added to final scores.
PhD student. between the human organism and food ingredients. The working group "Molecular Modeling" at Leibniz-LSB@TUM is looking for a PhD student to start on 01/07/2024. Project: Advancements in structural biology. Enter an email to receive alerts for phd-computational-biology positions.
Position: PhD Student Position in Computational Biology - Somatic Evolution of Cancer Development Department: "Somatic Evolution and Early Detection" and "Experimental Hepatology, Inflammation and Cancer" Code number: 2024-0119 The German Cancer Research Center is the largest biomedical research institution in Germany. With more than 3,000 employees, we operate an extensive ...
Top-ranked German Universities in Computational Biology. Top 100 Worldwide. Top 250 Worldwide. National Ranking. #83 Times Higher Education Ranking. Freie Universität Berlin. public University. No. of Students: approx. 38,000 students. Program Fees: € 0 (per semester)
For international PhD applicants, positions are available with DAAD support across multiple groups, including ours. We encourage applicants with computational biology backgrounds or mixed wet-dry backgrounds to apply and list our group as a preferred choice. If you have your own funding or are interested in applying for fellowships, we invite ...
390 computational-biology-phd positions in Germany. Filters Search Sort by. relevance listed; Filtered by; Germany ... From 01.09.2023 until 31.08.2026 the BIH is looking for a PhD Student Computational Biology/Bioinformatics (f/m/d) The bioinformatics group "Computational Genome Biology" of. Research Assistant (m/f/d) ...
Graduates will have a strong biological background as well as being able to understand and develop software for the handling and analysis of biological data. Programme: Computational Biology and Bioinformatics. Degree: Master of Science (M.Sc.) Standard period of study: 4 semesters. Start: winter semester.
Heterogeneities in the data are modeled using statistical and dynamical approaches, both on the cellular and on the population level. The Institute of Computational Biology (ICB) develops models and methods for data analysis in systems biology and systems medicine. We integrate information on multiple scales, ranging from single-cell time ...
International Max Planck Research School "From Molecules to Organisms". The International Max Planck Research School (IMPRS) "From Molecules to Organisms" provides excellent interdisciplinary training across the disciplines of structural, molecular, cellular and developmental biology as well as bioinformatics, genomics and evolutionary biology.
PhD student computational biology / bioinformatics (m/f/d) (fulltime) The position will be hosted at the Institute of Human Genetics, in the workgroup "Regulatory Genomics" of Prof. Martin Kircher. The bioinformatics group performs research in the fields of sequence analysis, data mining, machine learning and functional genomics ( https ...
Computational Biology. The Master of Science in Computational Biology is being offered since winter term 2023/2024 at the University of Cologne. It provides students with a solid background in computational and quantitative methods to analyze, integrate and interpret biological data, covering a spectrum from single cells to entire ecosystems ...
Students will begin study in late August and graduate in late May. 3-semester M.S. in QBB - Advanced Study. ... Students who are interested in this program may also want to consider the M.S. in Computational Biology and M.S. in Automated Science programs. Those programs expect a higher level of quantitative background & skills to enter and are ...
New approach makes calculations with realistic interactions possibleFRIB researchers are part of an international research team solving challenging computational problems in quantum physics using a new method called wavefunction matching. The new approach has applications to fields such as nuclear physics, where it is enabling theoretical calculations of atomic nuclei that were previously not ...
The article "Computational and Systems Biology Advances to Enable Bioagent Agnostic Signatures" explores the computational data challenges of threat-agnostic biodefense, or the ability to characterize an unknown agent's likely impact to human, animal, and plant health. The researchers discuss how the biodefense community can make the ...
Graduate Program in Biochemistry, University of Washington, Seattle, WA, USA Robby Divine Department of Chemistry, University of California, Davis, Davis, CA, USA
The University annually recognizes faculty for their incredible teaching and mentoring of undergraduate and graduate students through the Llewellyn John and Harriet Manchester Quantrell Awards, believed to be the nation's oldest prize for undergraduate teaching. In 2024, five faculty members received this award.
MIT senior Owen Dugan, graduate student Vittorio Colicci '22, predoctoral research fellow Carine You '22, and recent alumna Carina Letong Hong '22 are recipients of this year's Knight-Hennessy Scholarships. The competitive fellowship, now in its seventh year, funds up to three years of graduate studies in any field at Stanford University.