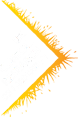
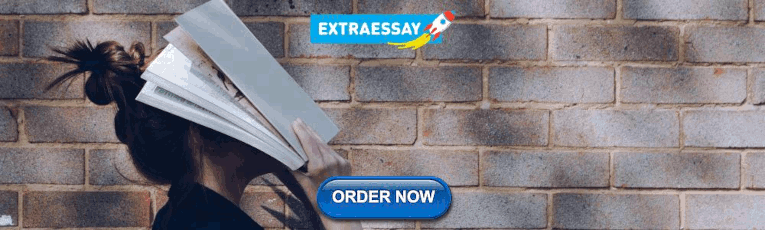
HRCS ONLINE
- 2018redirect
- 2014public_tableau
- 2014 redirect
- 2009redirect
- Use of the HRCS
- Conditions of use
- Assigning health categories
- Assigning research activities
- Common mistakes
- Trainer Resources
- Cancer and neoplasms
- Cardiovascular
- Congenital disorders
- Inflammatory and immune system
- Injuries and accidents
- Mental health
- Metabolic and Endocrine
- Musculoskeletal
- Neurological
- Oral and Gastrointestinal
- Renal and Urogenital
- Reproductive health and childbirth
- Respiratory
- Generic health relevance
- Disputed Aetiology and Other
- 1.1 Normal biological development and functioning
- 1.2 Psychological and socioeconomic process
- 1.3 Chemical and physical sciences
- 1.4 Methodologies and measurements
- 1.5 Resources and infrastructure
- 2.1 Biological and endogenous factors
- 2.2 Factors relating to physical environment
- 2.3 Psychological, social and economic factors
- 2.4 Surveillance and distribution
- 2.5 Research design and methodologies (aetiology)
- 2.6 Resources and infrastructure (aetiology)
- 3.1 Primary prevention interventions to modify behaviours or promote well-being
- 3.2 Interventions to alter physical and biological environmental risks
- 3.3 Nutrition and chemoprevention
- 3.4 Vaccines
- 3.5 Resources and infrastructure (prevention)
- 4.1 Discovery and preclinical testing of markers and technologies
- 4.2 Evaluation of markers and technologies
- 4.3 Influences and impact
- 4.4 Population screening
- 4.5 Resources and infrastructure (detection)
- 5.1 Pharmaceuticals
- 5.2 Cellular and gene therapies
- 5.3 Medical devices
- 5.4 Surgery
- 5.5 Radiotherapy and other non-invasive therapies
- 5.6 Psychological and behavioural
- 5.7 Physical
- 5.8 Complementary
- 5.9 Resources and infrastructure (treatment development)
- 6.1 Pharmaceuticals
- 6.2 Cellular and gene therapies
- 6.3 Medical devices
- 6.4 Surgery
- 6.5 Radiotherapy and other non-invasive therapies
- 6.6 Psychological and behavioural
- 6.7 Physical
- 6.8 Complementary
- 6.9 Resources and infrastructure (treatment evaluation)
- 7.1 Individual care needs
- 7.2 End of life care
- 7.3 Management and decision making
- 7.4 Resources and infrastructure (disease management)
- 8.1 Organisation and delivery of services
- 8.2 Health and welfare economics
- 8.3 Policy, ethics and research governance
- 8.4 Research design and methodologies
- 8.5 Resources and infrastructure (health services)
- Alcohol consumption
- Animal welfare and replacing animals in research
- Atherosclerosis
- Biomarkers and screening
- Cachexia in chronic diseases and chemotherapy
- Cancer and conditions predisposing to cancer
- Case studies and tracer examples
- Cell cycle and DNA repair
- Cerebral palsy and spina bifida
- Cholesterol
- Co-morbidities
- Congenital disorders and inherited syndromes
- Developmental biology
- Diet, obesity and nutrition
- Ear and hearing
- Economic evaluation components in trials
- Eye and vision
- Foetal development, pregnancy and ‘in utero’ studies
- Immunology and immune system
- Infectious diseases
- Informatics, Bioinformatics and ‘Big Data’
- Methodologies, measurements and research designs
- Multiple diseases and categories
- Muscle and neuromuscular junction
- Neuropsychology
- Nutritional and vitamin supplements
- Pharmaceuticals outside the therapeutic context
- Physical activity and exercise
- Pollution, radiation and environmental hazards
- Prevention – differentiating primary and secondary
- Prions – studies of TSEs, BSE, CJD and prion protein
- Pulmonary hypertension
- Quality of life components in trials
- Resources and infrastructure
- Sequelae, consequences and side effects
- Sexual health
- Sleep and studies of sleep disorders
- Smoking and tobacco
- Stratified Medicine, Precision Medicine and Personalised Medicine
- Substance misuse and addiction
- Surgical procedures
- Systematic reviews
- Thromboses and embolisms
- Treatment effectiveness
- Vertigo and disorders of balance
- Wounds and healing
- Analysis 2022 – Submission Guidance
- Analysis 2022 – Frequently Asked Questions
- Anaylsis 2022 – Data Publicity Statement
- Analysis 2022 – Qualitative submissions
- Analysis 2018 – news and wider use
- 2014 analysis – news and wider use
- UK Health Research Analysis 2009/10
- HRCS International Workshop 2009
- From Donation to Innovation (2007)
- UK Health Research Analysis 2004/05
- Analysis data
- Analysis tools
- Additional resources
- Terms and Conditions
- Data protection
- Privacy notice
- Cookies policy
- Accessibility
UK Health Research Analysis 2022
The fifth in our uk analysis series is officially published.
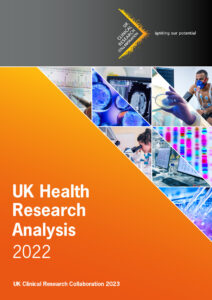
The UK Health Research Analysis 2022 – the fifth iteration of our nationwide health research landscaping report series – has been published .
Begun in 2023, t he UK Health Research Analysis 2022 provides the most detailed overview yet of UK health research funding from all public sectors, including the governments of the four nations of the UK, charities, societies, and professional bodies.
The analysis consists of more than 23,500 health and biomedical research awards from 173 organisations accounting for an estimated expenditure of just over £5.0 billion in the calendar year 2022.
As with previous analyses, our aim is to provide a high-quality overview of the UK’s health research landscape; the conditions of focus, the types of research being conducted, where that research is being done, and how this compares to the wider UK R&D ecosystem and health research internationally.
As the fifth report in our series, we now provide an 18-year overview of the changes in the UK’s health research landscape since 2004.
- The report summarised the main findings from the analysis, including estimations of total UK health R&D expenditure and an assessment of international funding
- The report’s tables and figures are also available as a separate .xlsx file , to minimise the need for extracting data directly from the report itself
- The report’s cover is also available as JPEG file for use in presentations, if desired
- This public version of the dataset which underpins the analysis is freely available for further analysis – see our data section for more details
- Please note that, due to file size, we have also made the data available as a ZIP file for ease of download
- To aid further analyses of the 2022 data, we have provided a Tableau dashboard to allow your own interrogation of the report
- The dashboard is available further down this page , although this may load more effectively if viewed directly in Tableau Public
Citing the UK Health Research Analysis 2022
When citing the report and/or onward use of the dataset please use the following acknowledgement:
“UK Health Research Analysis 2022 (UK Clinical Research Collaboration , 2023) https://hrcsonline.net/reports/analysis-reports/uk-health-research-analysis-2022/ ”.
Note that this report was in early access from December 2023, and formally published on 1st of February 2024.
The reports in the UK Health Research Analysis series, their associated data and the HRCS coding system are available for onward use as part of our creative commons licence . We encourage replication and further examination of the wealth of data this series provides to participating organisations, stakeholders in UK health research and the wider public.
If you have any questions at all regarding this or previous analyses then please contact us .
Data visualisation
Note – You may find the visualisation works best by accessing it directly from the Tableau Public website: https://public.tableau.com/views/UKHRA2022PublicDataset/HRCS2022
To provide an alternative to the static report – and an opportunity for individual organisations to view their own data – we have provided an interactive Tableau dashboard which consists of two pages:
- Funder level information providing details of 2022 expenditure by funding organisation, HRCS Health Category and Research Activity
- A geographical distribution of the 2022 expenditure by UK region and city
You can also find additional details (‘tool tips’, such as exact values) by hovering over with your cursor.
Background to the UK Health Research Analysis 2022
Work on this analysis began in earnest in April 2023, to ensure data from the latest financial year end was ready for inclusion. To lay the ground work and facilitate participation in this analysis, we produced the following detailed documentation:
- Submission guidance – which gives an overview of what we ask of participating organisations and why
- Frequently asked questions (FAQ) – which clarifies and builds on the submission guidance
- Data submission Excel spreadsheet – our pro forma for providing your research award information
- Data publicity statement – which clarifies our data protection stance
If you are interested in learning more about the efforts made across the many participating funders in this analysis, please contact us .
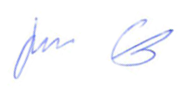
Dr Jim Carter, MRC (part of UKRI)
Chair of the Health Research Analysis Forum
if you are viewing this on Internet Explorer 8 (or older), it will not look optimal and you are running unnecessary security risks in your browsing. It is suggested you upgrade your browser to the latest version of Internet Explorer or try the free Google Chrome or Mozilla Firefox.
News Events
Search form.

Public Health Agency - Research & Development in Northern Ireland
The uk health research analysis 2022 has been published..
The UK Health Research Analysis 2022 provides the most detailed overview yet of UK health research funding from all public sectors, including the governments of the four nations of the UK, charities, societies, and professional bodies.
The analysis consists of more than 23,500 health and biomedical research awards from 173 organisations accounting for an estimated expenditure of just over £5.0 billion in the calendar year 2022.
The aim of the analysis is to provide a high-quality overview of the UK’s health research landscape; the conditions of focus, the types of research being conducted, where that research is being done, and how this compares to the wider UK R&D ecosystem and health research internationally.
To read more see the HRCS website or click on the image below to download the report.
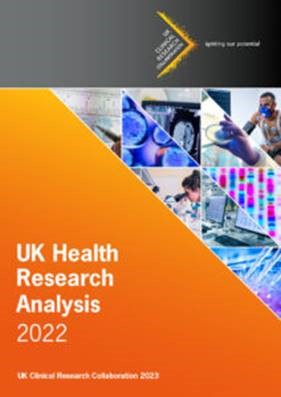
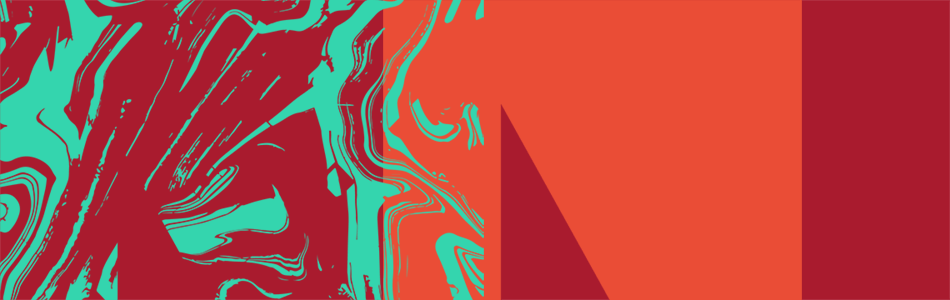
Health research: who funds what and where?
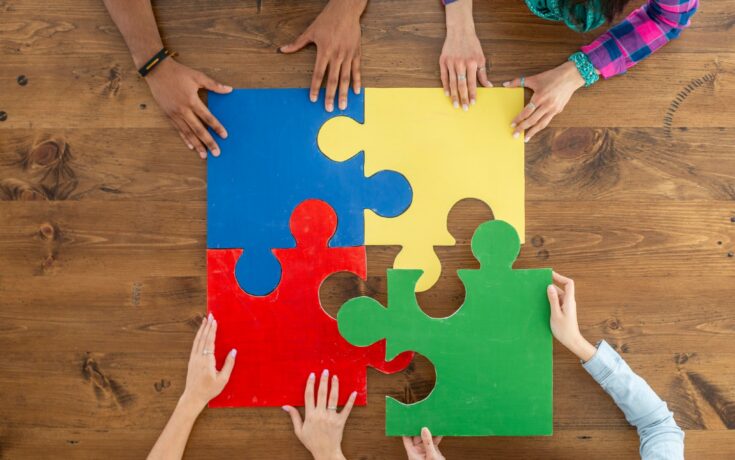
Evaluation Officer, Medical Research Council (MRC)
28 February 2024
Looking at funding trends can help build the case for future funding. Find out how you can make use of the largest ever analysis of UK health research funding.
Having a referenceable figure on how much the UK spends on a given research topic is handy for all sorts of publications. Charities find it particularly useful; ‘Only X% of UK health research is spent on Y’ is a great introduction when seeking support for your cause. I’ve even found a reference to UK Health Research Analysis reports in adverts for Nurofen.
UK Health Research Analysis reports are the largest analyses of UK health research funding. Our new report on 23,500 projects from 173 funding organisations, is the largest to date and shows over £5 billion of spend within the UK in 2022.
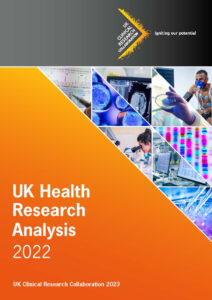
Front cover of the new UK Health Research Analysis report
Looking across the whole 18-year period of our reporting, we’ve seen increases for most areas of research. This includes long term upwards trends for prevention and translational research, which aims to turn fundamental discoveries into improvements in human health. But it also includes the signs of near-flat funding since 2009 and, for the first time, a small decrease in real terms health research funding since 2018.
But the reports aren’t just a handy source for quoting stats. They have real impact. Government policies often quote our data. Our findings can influence where public money is spent and show that policies have been delivered.
Getting a consistent picture
The Medical Research Council (MRC) leads on the creation of these reports on behalf of the UK Clinical Research Collaboration (UKCRC), which coordinates the efforts of major stakeholders who conduct research in the UK. Before this report series, individual organisations were able to say how much they spent on cancer research but the definitions, methodology or timescales varied, making a messy and inconsistent picture.
To fix this, in 2004 we designed the Health Research Classification System (HRCS). It aimed to unify the approach with enough detail to be useful but without unnecessary complexities.
We apply the system to every UK health research award, to classify them by health categories and research activities. This means that for every health research award that gets funded, I can tell you what type of research it is, how many fund it and who gets the funding.
Open access to everyone
I firmly believe the power of this work lies in keeping our reports and the underlying data public. It means that anyone can replicate the findings. It also allows for re-use of the dataset for other analyses. This saves countless resource-intensive hours for others interested in funding data and research policy.
While the reports’ main audience is policymakers, they have a much wider potential appeal. A researcher could use our data to find which funders are supporting work in their field. A patient could see how many charities support research into their disease. All in one place.
They also support the principles of publicly funded research being accessible to everyone that funds it. I like to say the UK Health Research Analysis reports are not just for data nerds like me, they are for everyone.
Ways to create collaboration
As you can imagine, reaching out to hundreds of organisations to collect the data for the reports can be daunting. However, I have three good ways to get organisations to work together:
Being part of these reports is an acknowledgement of being part of a wider ecosystem.
In my talks with other organisations, I emphasise the holistic aims and sense of community this brings. It helps convince organisations to take part, even if they are not health research specialists.
I’m doing what I can to make the process simple.
For organisations who can’t publish their award data we have an option for a narrative submission, so their contribution to the whole is still recognised. For those who can provide data, we keep requests simple and flexible but within a template format that can maintain consistency.
It’s a team effort.
I work closely with representatives from the 12 largest health funders to coordinate this work. This includes the fantastic Association of Medical Research Charities , who coordinate a combined submission from their entire membership, which cuts my job in half!
Building on two decades of analysis
I joined MRC as a data analyst, straight out of a research lab, to lead the third analysis in 2014. It was great to work with other organisations to not only produce the report but also review the HRCS itself. We revamped it with revised guidance and a new website in 2017.
Next year is the 20th anniversary for the HRCS and its use is expanding. We plan to use it to assess grants funded by our UK Research and Innovation ‘Securing better health, ageing and wellbeing’ strategic theme .
If I could oversee what happens next, I’d push for a publicly funded national database where research award data can be easily added by funders of any size. It would have a tool like HRCS built in, where automation is the default but with the opportunity to fill the gaps manually where an algorithm fails. That to me feels like the natural extension of the UK Health Research Analysis. An open, community-led and semi-automated way to provide the big picture view of the UK biomedical research landscape.
Find out more
Explore what compiling the UK Health Research Analysis involves and read the new report .
Top image: Credit: FatCamera, E+, via Getty Images.
Share this page
- Share this page on Twitter
- Share this page on LinkedIn
- Share this page on Facebook
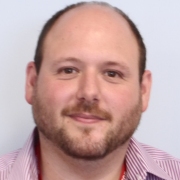
Dr Jim Carter began working in the MRC Evaluation and Analysis Team to manage the UK Health Research Analysis 2014, and has led both the 2018 and latest 2022 analyses too. Outside of the UK Clinical Research Collaboration reports, Jim provides both evaluation and analytical expertise to a range of MRC programmes and published reports.
Before joining MRC, Jim’s medical research career began working in the Bristol Eye Hospital labs investigating hereditary eye disease. He completed a PhD in the genetics behind neovascularisation, the growth of new blood vessels, in both eye disease and cancer at Bristol. He then joined the University of Nottingham Centre for Cancer Sciences.
This is the website for UKRI: our seven research councils, Research England and Innovate UK. Let us know if you have feedback or would like to help improve our online products and services .
- COP Climate Change
- Coronavirus (COVID-19)
- Cancer Research
- Diseases & Conditions
- Mental Health
- Women’s Health
- Circular Economy
- Sustainable Development
- Agriculture
- Research & Innovation
- Digital Transformation
- Publications
- Academic Articles
- Health & Social Care
- Environment
- HR & Training
- Health Research
- North America Analysis
- Asia Analysis
- Our Audience
- Marketing Information Pack
- Prestige Contributors
- Testimonials
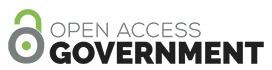
- North America
- Open Access News
- Research & Innovation News
UK health research funding plateaus
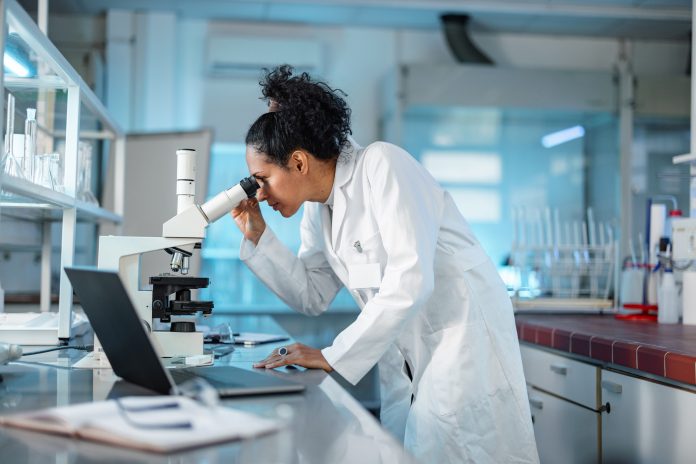
UK health research funding reached £5 billion in 2022 but is now plateauing, according to the UK Health Research Analysis 2022 , but why?
The report, commissioned by the UK Clinical Research Collaboration (UKCRC), provides the most detailed overview yet of UK health research funding from 2004 to 2022 from all public sectors.
During this 18-year period, annual funding had increased overall reaching £5 billion in 2022; however, since 2018, this growth has slowed to just 0.2% annually, resulting in a real terms decrease of £110 million. The decrease has been attributed to high inflation and a reduction in the charity sector’s contribution to health research due to the impact of COVID-19.
23,500 projects supported by 173 funding organisations
The Health Research Classification System was applied to over 23,500 projects supported by 173 funding organisations, corresponding to just over £5 billion of spend within the UK in 2022.
The analysis showed that of the £5 billion spent: £2.8 billion was invested directly on research projects; £1.4 billion was spent on infrastructure and other indirect support; and £865 million of health-relevant support funding was added from other sources not directly captured in the analysis.
The report also noted that funding for research projects over the study period was stable for most areas of health but with some notable trends:
- Translational research, in detection and diagnosis, treatment development, and treatment evaluation, spends were up 13.0% from 22% to 35% between 2004 and 2022, resulting in a real-term increase of £676 million
- Prevention research expenditure was up 4.6% from 2.5% in 2004 to 7.1% in 2022, conveying a real-term increase of £163 million since 2004
- Research on discovery science remains strong, accounting for almost half of all funding, but growth in spending has been prioritised to other research activities
- The largest growth has been in infections research (up 6.4%, from 9.0% in 2004 to 15.4% in 2022) as funders sought to address the challenges of antimicrobial resistance, COVID-19 and post-pandemic recovery
- Almost a fifth of health funding was spent on cancer research, but this has declined in proportion to overall funding (from 20.3% in 2004 to 16.8% in 2022, down by 2.1% since 2018)
£5 billion annual investment
Professor Lucy Chappell, Chair of UKCRC, and Chief Scientific Adviser, Department of Health and Social Care, said:
“The UK health funding ecosystem successfully brings together government spending with the charity and private sector to drive world-leading discovery research and innovation that is having impact for patients and the public, the NHS, and for the UK life sciences industry.
“This report shows how the £5 billion annual investment made by public and charitable funders is being spent, including substantial growth in patient-focussed research.
“We have seen this ecosystem discover and deliver new technologies for early detection and diagnosis alongside new treatments and vaccines for prevention.
“This was only possible through sustained investment over many decades in discovery science and our translational infrastructure.
“The health funders across the public, charity and private sectors will continue to work together closely to address the current and future health challenges.
UK health research is world-class
“UK health research is world-class; we should continue to see increasing research and development funding in order to drive health and economic impact.”
Editor's Recommended Articles
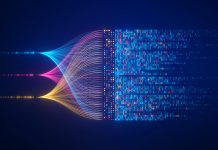
RELATED ARTICLES MORE FROM AUTHOR
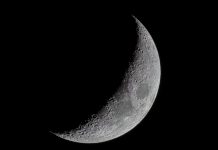
The moons transformation: How it turned itself inside out
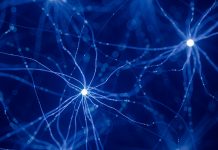
Insights into autism development, treatment and management
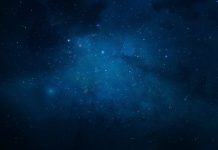
DESI telescope unveils cosmic secrets: Mapping the universe’s evolution
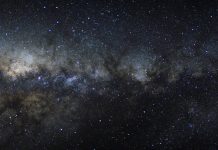
BREAD experiment takes on dark matter
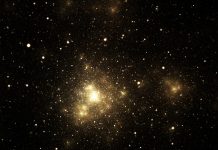
Curtin University discover rare dust particles in ancient extraterrestrial meteorites
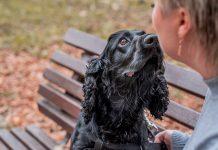
Dogs trained to smell out PTSD flashbacks
Leave a reply cancel reply.
Save my name, email, and website in this browser for the next time I comment.
Related Academic Articles
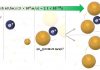
Thermodynamics of hadronization: The rotating lepton model explains key CERN experiments
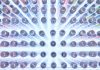
The future of sustainable electrified catalyst recycling
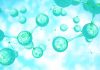
Independent research experiences in sustainable chemistry
Follow open access government, latest research reports.
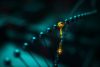
Karl von Frisch and Konrad Lorenz: Two biologists in Nazi Germany
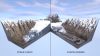
Science speaks out! INTERACT bridge the gap between researchers and the...
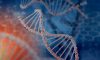
Biocolloidy and Epigenetics – Is “scientific revolution” a useful concept in...
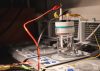
The role of synthetic biology in Microbial electrosynthesis
Latest research ebooks.
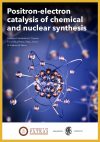
Positron-electron catalysis of chemical and nuclear synthesis
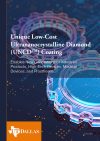
Unique Low-Cost Ultrananocrystalline Diamond Coating
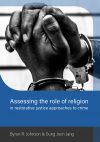
Assessing the role of religion in restorative justice approaches to crime
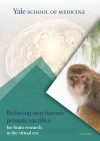
Reducing non-human primate sacrifice for brain research in the virtual era
Feature articles.
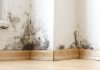
Damp and mould-related surveys: What is a healthy building?
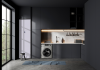
Heat pumps as a renewable energy source to stabilise the electricity...
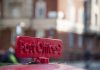
Lessons learned from Post Office’s Horizon IT scandal
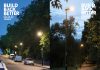
Smart connected lighting: Creating a sustainable urban ecosystem
Latest publication.
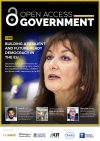
Open Access Government April 2024
Latest ebook.
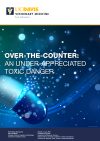
Are over-the-counter drugs an under-appreciated toxic danger?
- Terms & Conditions
- Privacy Policy
- GDPR Privacy Policy
- Marketing Info Pack
- Fee Schedule
- Health Research Classification System
UK Health Research Analysis
- Public Health Research
Health Research Analysis
An accurate picture of current research investment is important for planning research funding. The UKCRC has carried out analyses of the health research portfolios of government and charity organisations funding health related research in the UK, using a bespoke Health Research Classification System.
The first UK Health Research Analysis report, published in 2006, was the first ever national analysis of health research. It
Largest study of UK health research funding released today
UK health research funding has increased since 2004, reaching £5 billion in 2022 with growth in translational and infection research, but is now plateauing.
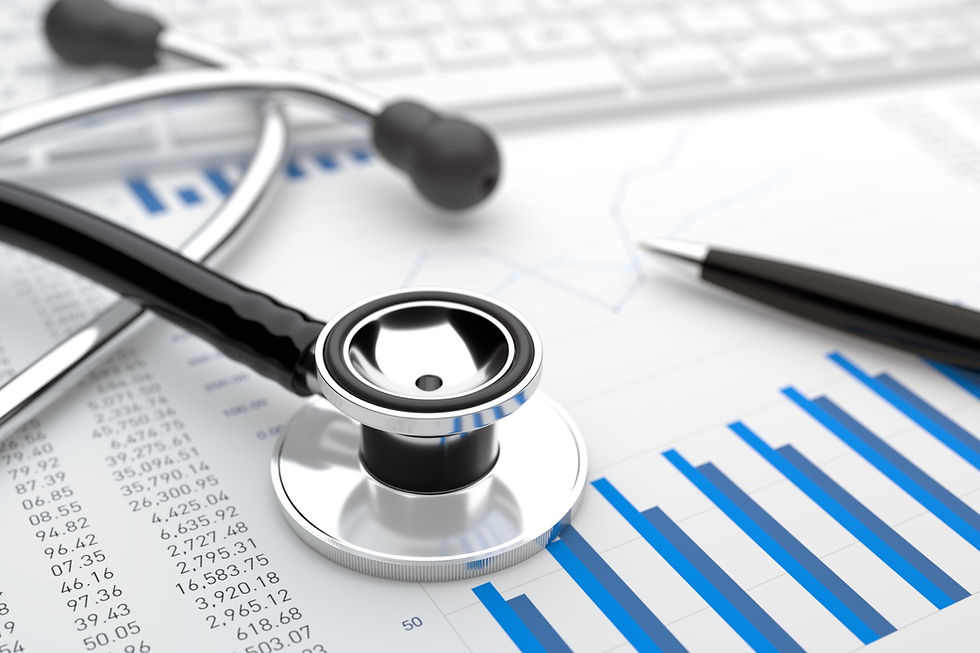
A report published today, the UK Health Research Analysis 2022, provides the most detailed overview yet of UK health research funding from 2004 to 2022 from all public sectors, including:
the governments of the four nations of the UK
professional bodies
This report is part of a series published every four to five years.
The report was commissioned by the UK Clinical Research Collaboration (UKCRC), a partnership of the main stakeholders that influence clinical research across the business, public and charitable sectors in the UK.
Read more here.
Comentarios
Other science, engineering & technology news.

Scientists identify gene variants linked to obesity risk

Celebrating 20 years of CPI: How one organisation helped generate billions for the UK economy

UK & United States announce partnership on science of AI safety
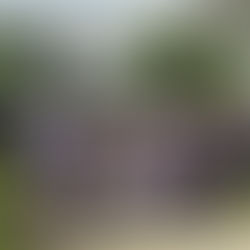
Navigating exciting horizons: Nat Edington assumes CEO role at Filtronic
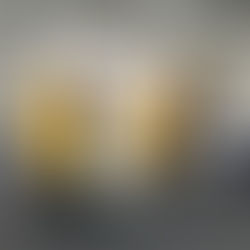
Princess Anne visits North East to open Pragmatic Park microchip factory

UK Healthcare Outlook Report, 2022

- January 2022
- Region: United Kingdom
- DelveInsight
- ID: 5526154
- Description
Table of Contents
- Companies Mentioned
Related Topics
Related reports.
- Purchase Options
- Ask a Question
- Recently Viewed Products
UK Healthcare Outlook
Uk industry overview chapter, pharmaceutical, medical device, legislation in this area has previously been updated at eu level, with the changes flowing through into uk law. the medicines and medical devices bill will allow the health secretary to take over that task. the bill proposes similar powers to amend or supplement the medical devices legislation. of course, the existing eu directives in this area are due to be replaced by two new regulations:.
- Regulation 2017/745 on medical devices (applicable from 26 May 2020)
- Regulation 2017/746 on in vitro diagnostic medical devices (applicable from 26 May 2022).
UK Healthcare Assessment Chapter
Uk: market access, uk: pestle analysis, report highlights.
- Overview of the pharmaceutical and medical device markets
- An insightful review of the reimbursement and regulatory landscape, covering the healthcare reimbursement process, regulatory agencies, and the approval processes for new drugs and medical devices
- Detailed analysis of the political and economic environment, including economic indicators, demographics, healthcare infrastructure and healthcare expenditure
- An overview of the opportunities for and challenges to growth in the healthcare market
UK Report Insights
- UK - Healthcare Outlook
- UK - Healthcare Assessment
- UK - Market Access
- UK - Healthcare Market Perspectives
- UK - Analyst View
Key Questions Answered
- What are business strategies and trends shaping and driving the UK healthcare market?
- What are reimbursement and regulatory policies, pharmaceutical and medical device market segments and companies likely to impact the UK healthcare market in the future?
- How to organize sales and marketing efforts by identifying the market categories and segments that present the best opportunities for consolidation, investment and strategic partnership?
- How to identify, understand and capitalize on the opportunities and challenges in the UK healthcare market?
Key Players
- The Harley Street Clinic
- Portland Hospital
- Ramsay Health Care UK
- Synergy Health
- Nuffield Health
- GlaxoSmithKline
- Oxford BioLabs
- Spire Healthcare
- Allied Healthcare
- Circle Health Ltd
Companies Mentioned (Partial List)
A selection of companies mentioned in this report includes, but is not limited to:
- Healthcare Services

Global Healthcare Contract Manufacturing Market by Manufacturing Type (Accessories Manufacturing, Assembly Manufacturing, Component Manufacturing), End-Users (Medical Device Companies, Pharmaceutical & Biopharmaceutical Companies) - Forecast 2024-2030
- Report

UK Healthcare Markets (without digital archive)
- Newsletter
- United Kingdom

Temporary Recruitment and Staffing: Healthcare 1ed
- January 2024

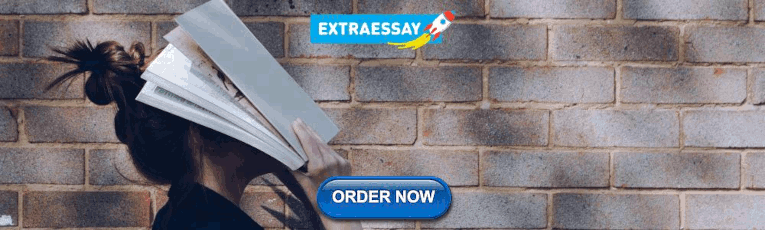
Opportunities in the Western Europe Consumer Health Industry 2023
- August 2023

Impact of Inflation on the Healthcare Sector by Physicians Perspective, Patient Treatment, Regional Analysis, Drug Prices and Treatment Costs - Thematic Intelligence
ASK A QUESTION
We request your telephone number so we can contact you in the event we have difficulty reaching you via email. We aim to respond to all questions on the same business day.
Request a Quote
YOUR ADDRESS
YOUR DETAILS
PRODUCT FORMAT
DOWNLOAD SAMPLE
Please fill in the information below to download the requested sample.
This site uses session cookies and persistent cookies to improve the content and structure of the site.
By clicking “ Accept All Cookies ”, you agree to the storing of cookies on this device to enhance site navigation and content, analyse site usage, and assist in our marketing efforts.
By clicking ' See cookie policy ' you can review and change your cookie preferences and enable the ones you agree to.
By dismissing this banner , you are rejecting all cookies and therefore we will not store any cookies on this device.
The Health Survey for England 2022 to 2025
Research type.
Research Study
The Health Survey for England 2022 to 2025 - pilot and main stage studies
Contact name
Jennifer Mindell
Contact email
Sponsor organisation
NatCen Social Research
Duration of Study in the UK
5 years, 8 months, 0 days
Research summary
The Health Survey for England (HSE) is part of a programme of surveys commissioned by NHS Digital. HSE has been conducted since 1994 by the Joint Health Surveys Unit of NatCen Social Research (NatCen) and the Research Department of Epidemiology and Public Health at UCL. The study provides information that cannot be obtained from other sources about the public’s health and many factors that affect health. The HSE monitors trends in the nation’s health, estimating the proportion of people in England with certain health conditions and how common certain risk factors and combinations of risk factors are, and monitors progress towards selected health targets.
HSE 2022, HSE 2023, HSE 2024, and HSE 2025 (referred to as HSE 2022-2025 hereafter) will each include a new, general population sample of around 8,000 adults (aged 16 and over) and 2,000 children (aged 0-15), representative of the general population. Participants will be recruited from January to December each year from everyone living at randomly selected private addresses in England. After an advance letter is sent to the address, an interviewer will visit to ask people living at that address to take part in the survey. Some who agree will also be visited afterwards by a nurse. The interview asks about the household, then individuals are asked about their general health and health risk factors. Additional modules vary each year.
Depending on the participant's age, measurements are taken of height, weight, waist and hip circumference, and blood pressure; a saliva or urine sample collected; and blood samples taken with consent, for laboratory analysis.
Core modules for HSE 2022-25 and additional modules for HSE 2022 are specified in this application. In HSE 2022, a sample of HSE participants aged 16-49y will also be invited to take part in the Human Biomonitoring sub-study.
East Midlands - Nottingham 2 Research Ethics Committee
REC reference
Date of rec opinion.
15 Jul 2021
REC opinion
Further Information Favourable Opinion
- Privacy notice
- Terms & conditions
- Accessibility statement
- Feedback or concerns
Cookies on GOV.UK
We use some essential cookies to make this website work.
We’d like to set additional cookies to understand how you use GOV.UK, remember your settings and improve government services.
We also use cookies set by other sites to help us deliver content from their services.
You have accepted additional cookies. You can change your cookie settings at any time.
You have rejected additional cookies. You can change your cookie settings at any time.

- Health and social care
- Technology in health and social care
Better, broader, safer: using health data for research and analysis
Professor Ben Goldacre’s review into how the efficient and safe use of health data for research and analysis can benefit patients and the healthcare sector.
Applies to England
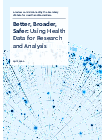
PDF , 2.85 MB , 112 pages
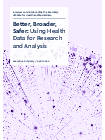
Better, broader, safer: using health data for research and analysis – executive summary
PDF , 192 KB , 5 pages
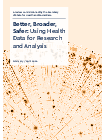
Better, broader, safer: using health data for research and analysis – summary
PDF , 517 KB , 18 pages
Professor Ben Goldacre was commissioned by the government in February 2021 to review how to improve safety and security in the use of health data for research and analysis. The report makes 185 recommendations that would benefit patients and the healthcare sector.
The report is aimed at policy makers in the NHS and government, research funders and those who use the data for service planning, public health management and medical research. Patient representatives and the wider public may also have an interest in the report’s recommendations.
The report is informed by interviews, open sessions and deep dives with more than 100 stakeholders throughout academia and healthcare. The government’s response will be included in our upcoming health and social care data strategy, which was published in draft form in June 2021 .
This review is technical in detail and because of this it is presented at 3 levels of detail:
executive summary – contains a short overview of high-level strategic opportunities
summary – contains a longer overview of the opportunities, challenges and recommendations
full text – contains a detailed explanation of the work with extensive findings from interviews and desk research, alongside practical descriptions of the mechanics of working with NHS data to help ensure informed discussion; and granular detail on the practical and technical aspects of recommendations where these are needed
Related content
Is this page useful.
- Yes this page is useful
- No this page is not useful
Help us improve GOV.UK
Don’t include personal or financial information like your National Insurance number or credit card details.
To help us improve GOV.UK, we’d like to know more about your visit today. We’ll send you a link to a feedback form. It will take only 2 minutes to fill in. Don’t worry we won’t send you spam or share your email address with anyone.
Internet Explorer is no longer supported by Microsoft. To browse the NIHR site please use a modern, secure browser like Google Chrome, Mozilla Firefox, or Microsoft Edge.

National Institute for Health and Care Research
We fund, enable and deliver world-leading health and social care research that improves people's health and wellbeing, and promotes economic growth.
Read NIHR news
Read NIHR blog
NIHR launches new climate health and sustainability commitments
These commitments set out key steps the NIHR will take to engage in climate, health and sustainability research and capacity building. The NIHR is also committing to reduce its carbon footprint and the footprint of the wider research community.
Read the full news story here
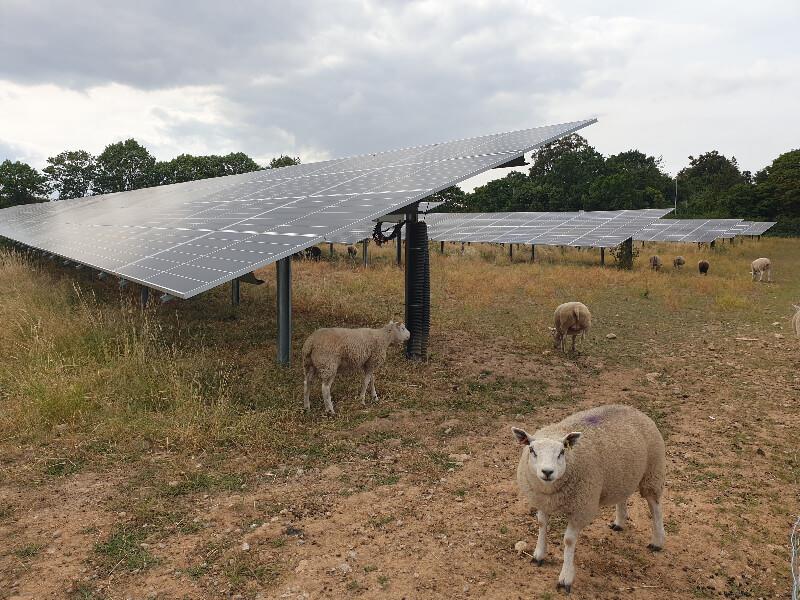
Project funding
We fund a variety of commissioned, researcher-led and themed call research:
- in health, social care and public health
- in Global Health
Search project funding opportunities
Career development
We support the development of professionals through:
- personal awards including Fellowships
- practitioner academic training
- Global Health Research careers
Find career development opportunities
Making a Difference
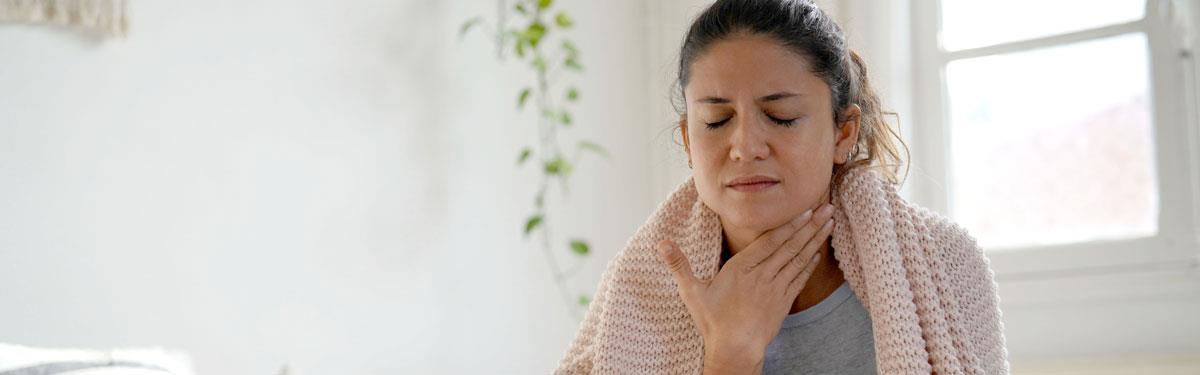
Test for sore throat cuts antibiotic use by nearly a third
A clinical scoring test developed by NIHR-funded researchers has cut the use of antibiotics prescribed for sore throats by nearly a third and a cost-effective approach to manage patients’ symptoms.
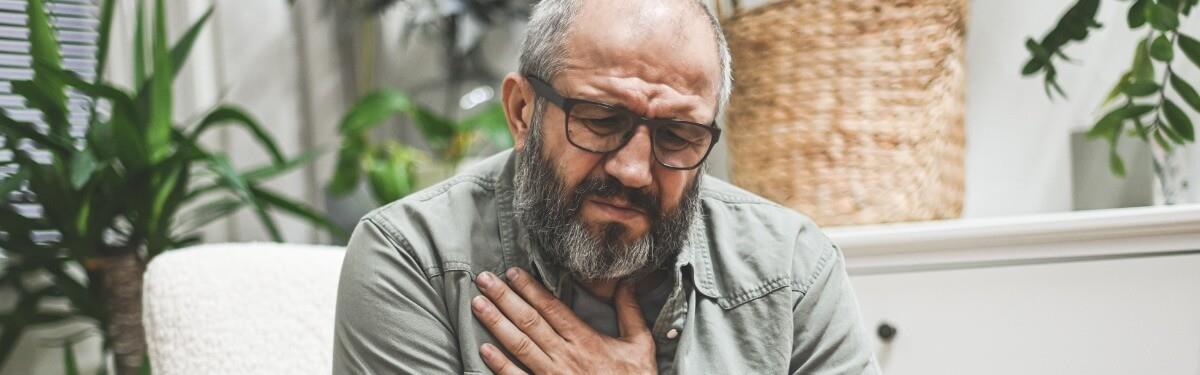
Preventing early heart disease in high-risk patients
Use of new software developed with NIHR funding is identifying more patients at high risk of early heart disease and heart attacks. Earlier diagnosis and treatment are saving lives and shaping healthcare policy.
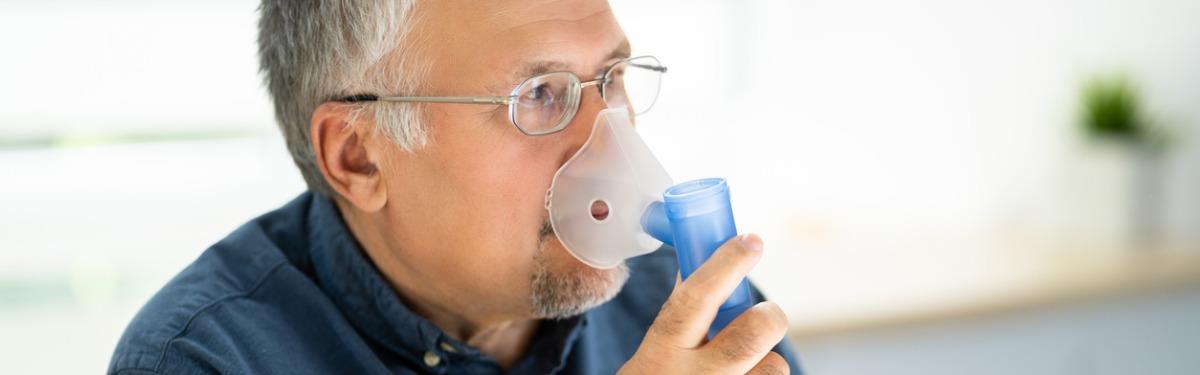
Breaking down barriers to effective cystic fibrosis treatment
In the biggest cystic fibrosis trial in the UK, NIHR-funded research has shown that a personalised web platform, CFHealthHub, could markedly increase adherence to treatment.
Read our case studies
Applied health and care research on both commissioned and researcher-identified topic areas.
Explore our Programmes
Research Infrastructure
Find out more about our expertise, specialist facilities, research delivery workforce and support services.
Explore our offers
Specialty therapy areas
The NIHR funds and supports research:
- in all specialty therapy areas
- delivered by expert clinical leaders and practitioners
- through effective collaboration
Discover our specialties
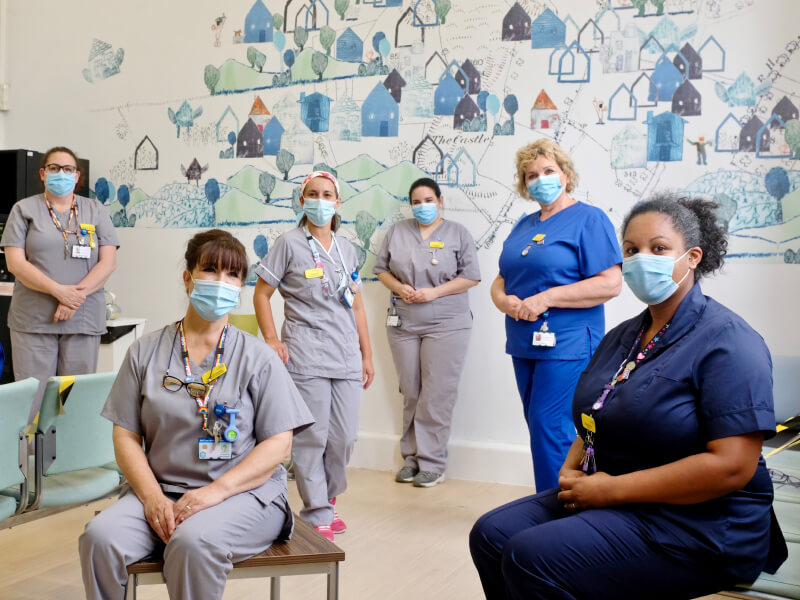
Global Health Research
NIHR is a major funder of high quality global health research that directly addresses the diverse health needs of people in low and middle income countries (LMICs).
Explore our Programme
Journals, publications and data
Explore NIHR-supported research projects, their findings and data from our range of resources:
- 5+ journals and publications for the public, care and research professionals
- 3+ open access health research databases
Explore our research
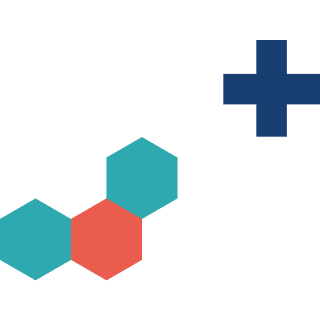
New figures suggest the public's appetite for taking part in research remains remarkably high after an unprecedented period in research history.
Find out more about the NIHR annual statistics
- Open access
- Published: 11 April 2024
Applying the UTAUT2 framework to patients’ attitudes toward healthcare task shifting with artificial intelligence
- Weiting Huang 1 , 2 ,
- Wen Chong Ong 6 ,
- Mark Kei Fong Wong 5 ,
- Eddie Yin Kwee Ng 5 ,
- Tracy Koh 1 , 2 ,
- Chanchal Chandramouli 1 , 2 ,
- Choon Ta Ng 1 , 2 ,
- Yoran Hummel 4 ,
- Feiqiong Huang 4 ,
- Carolyn Su Ping Lam 1 , 2 , 4 &
- Jasper Tromp 2 , 3
BMC Health Services Research volume 24 , Article number: 455 ( 2024 ) Cite this article
Metrics details
Increasing patient loads, healthcare inflation and ageing population have put pressure on the healthcare system. Artificial intelligence and machine learning innovations can aid in task shifting to help healthcare systems remain efficient and cost effective. To gain an understanding of patients’ acceptance toward such task shifting with the aid of AI, this study adapted the Unified Theory of Acceptance and Use of Technology 2 (UTAUT2), looking at performance and effort expectancy, facilitating conditions, social influence, hedonic motivation and behavioural intention.
This was a cross-sectional study which took place between September 2021 to June 2022 at the National Heart Centre, Singapore. One hundred patients, aged ≥ 21 years with at least one heart failure symptom (pedal oedema, New York Heart Association II-III effort limitation, orthopnoea, breathlessness), who presented to the cardiac imaging laboratory for physician-ordered clinical echocardiogram, underwent both echocardiogram by skilled sonographers and the experience of echocardiogram by a novice guided by AI technologies. They were then given a survey which looked at the above-mentioned constructs using the UTAUT2 framework.
Significant, direct, and positive effects of all constructs on the behavioral intention of accepting the AI-novice combination were found. Facilitating conditions, hedonic motivation and performance expectancy were the top 3 constructs. The analysis of the moderating variables, age, gender and education levels, found no impact on behavioral intention.
Conclusions
These results are important for stakeholders and changemakers such as policymakers, governments, physicians, and insurance companies, as they design adoption strategies to ensure successful patient engagement by focusing on factors affecting the facilitating conditions, hedonic motivation and performance expectancy for AI technologies used in healthcare task shifting.
Peer Review reports
Healthcare providers worldwide are constantly challenged to achieve the ‘quadruple aim’ in care delivery: Improving population health, enhancing patient experience, reducing healthcare worker burnout and dissatisfaction, and reducing costs [ 1 , 2 ]. There has been great progress in healthcare artificial intelligence (AI) and machine learning (ML) in the recent years [ 3 , 4 , 5 , 6 ], which hold promise.
Healthcare AI technologies has seen tremendous adoption especially in the medical imaging field [ 7 , 8 , 9 ]. AI’s performance is shown to match or exceed human experts in studies involving computed tomography [ 10 ], chest X-ray classification [ 11 ], ophthalmology [ 12 , 13 ], oncology [ 14 , 15 ] and dermatology [ 16 ]. Such advances demonstrate how AI can refine diagnostic accuracy and improve patient outcomes, while using healthcare resources more efficiency. However, there are still challenges and limitations in the data quality, ethical issues, regulatory frameworks, and user acceptance of AI technologies [ 17 ].
In our main study, a novice layperson with no prior sonography knowledge was trained and equipped with a point-of-care(POC) ultrasound device [ 18 ], which had real-time AI image guidance on probe repositioning to capture better image quality. We also used a United States Food and Drug Agency-approved deep learning-based software [ 19 ], which automatically analyses the acquired images and provides cardiac measurements such as the left ventricular ejection fraction (LVEF). We named this workflow the AI-novice combination. Our study showed that the AI-novice combination, compared with trained sonographer-performed and cardiologist-interpreted echocardiogram studies, was able to achieve an area under the curve (AUC) of 0.880 (95%CI 0.802, 0.958) in detecting patients with left ventricular dysfunction. Furthermore, the AI-novice combination had a median absolute deviation from the reference standard LVEF at 6.03%, which is comparable to human inter-reader variability [ 20 ].
Apart from the AI-novice combination’s accuracy, we wanted to study the patients’ attitudes and hence usage intention, given that AI taskshifting is a new model of care. There are several theories which look at technology usage intention behaviours. For our study, we chose the unified theory of acceptance and use of technology (UTAUT) and its extended version (UTAUT2) because it was the most comprehensive [ 21 , 22 ]. Among the UTAUT2 framework’s constructs, we examined the relationships between performance expectancy, effort expectancy, social influence, facilitating conditions, hedonic motivation and behavioral intention. We did not include questions on price and trust in the framework as the cost and reliability of the new AI-novice combination had not been established at the point of study. Also, the questions on habit pertain more towards voluntary consumerism, hence it was not applicable to a physician-ordered investigation. Additionally, the UTAUT2 has been one of the more important and versatile theoretical models used to predict consumer technology use and its effectiveness has been proven in different cultural backgrounds [ 23 ].
Prior studies largely looked at healthcare workers’ adoption intention of AI-assisted diagnosis and treatment [ 24 ]. In contrast, our study looks at the patients’ perspective, using the UTAUT2 framework. This has not been studied comprehensively, to our knowledge. Since patients are key stakeholders in new care pathways, this study will contribute to better understanding of factors influencing patient usage intention, therefore reduce barriers and facilitate implementation of AI-enabled taskshifting.
Study hypothesis and theoretical foundation
Hypothesis 1.
Performance expectancy influences the intention to accept task shifting of specialized scans to AI-novice combination.
Performance expectancy describes the perception that using the technology will benefit the patient and is therefore tied to the perception of usefulness [ 21 ]. In most studies, performance expectancy significantly influenced behavioural intention and was also often the strongest predictor [ 25 ].
Hypothesis 2
Effort expectancy influences the intention to accept task shifting of specialized scans to AI-novice combination.
Effort expectancy describes the expected ease of using the technology [ 21 ].
Hypothesis 3
Social influence affects the intention to accept task shifting of specialized scans to AI-novice combination.
Social influence is “the degree to which an individual perceives that important others believe he or she should use the new system” [ 21 ].
Hypothesis 4
Facilitating conditions influence the intention to accept task shifting of specialized scans to AI-novice combination.
Facilitating conditions refer to the perceptions “of the resources and support available to perform a behavior” [ 21 ].
Hypothesis 5
Hedonic motivation influences the intention to accept task shifting of specialized scans to AI-novice combination.
Hedonic motivation refers to gratification (fun, pleasure, and enjoyment) associated with using technology [ 21 ]. It is considered as the most important theoretical addition to the UTAUT2 as it shifted the focus from extrinsic motivation of an organization to intrinsic motivation of consumers toward usage of technologies [ 26 ].
Behavioural intention refers to the degree in which a person will perform a specified action, in this case accepting the task shifting of specialized scan to the AI-novice combination. It is the variable that responds to the above hypotheses.
Sample and data collection
In this cross-sectional study, patients aged ≥ 21 years with at least one heart failure symptom (pedal oedema, New York Heart Association (NYHA) II-III effort limitation, orthopnoea, breathlessness), were prospectively included. Consecutive patients presenting to the cardiac imaging laboratory at the National Heart Centre Singapore for physician-ordered clinical echocardiogram for investigation or follow-up for heart failure were approached. All patients underwent standard cart-based sonographer-performed, cardiologist-reported echocardiogram as per clinical practice. For the study, patients then underwent an additional experience of a novice-performed AI-supported POC echocardiogram on the same day for comparison. After the AI-novice combination echocardiogram was performed, a questionnaire was administered individually to each patient. The questionnaire comprised two portions; the first collected demographic information, while the second focused on the key constructs of performance and effort expectancy, facilitating conditions, social influence, hedonic motivation and behavioural intention. The second part of the questionnaire was adapted from the original version developed by Venkatesh et al. [ 21 ], see supplementary file 1 .
Ethics approvals
Ethics approvals were obtained from the local institutional review committee for this study, and this study conformed to the ethical guidelines in the Declaration of Helsinki.
Measurement and data analysis
The items measuring performance expectancy, effort expectancy, social influence, facilitating conditions, hedonic motivation and behavioral intention were adapted from Venkatesh et al. [ 21 ] to suit our study. We modelled our questionnaire as closely as possible to the original questionnaire by Venkatesh et al. [ 21 ], excluding only questions that did not suit our context. These items were measured using 5-point Likert scales anchored by 1(strongly disagree) and 5(strongly agree). Table 1 displays the finalized items with the mean scores of each question.
The analysis process was divided into two parts. As the questionnaire was adapted, the measurement quality was rigorously checked for reliability and validity. Only when the quality of the model was confirmed, the structural model was analyzed and interpreted.
Composite reliability (CR) and Cronbach’s α are used to evaluate internal consistency reliability. Both CR and Cronbach’s α values are recommended to be greater than 0.7 [ 27 ].
Convergent validity is supported if all the standardized item loadings are greater than 0.70, and if the average variance extracted (AVE) values for every construct exceed 0.5, the threshold value recommended by Fornell and Larcker [ 28 ]. Items which did not meet the criteria of > 0.70 were dropped from the final partial least squares structural equation modelling (PLS-SEM).
Discriminant validity is achieved if both AVE estimates of a given pair of constructs are greater than the square of the construct correlation [ 29 ].
PLS-SEM was used to explain causal relationships among constructs [ 30 ], as it could support both exploratory and confirmatory relationships [ 31 , 31 , 34 , 34 ]. This is suitable in this context as the questionnaire has been adapted from the original to suit our study. This regression analysis also allows for smaller sample sizes and permits estimation of models using ordinal scale data [ 35 ]. Additionally, prior studies of UTAUT2 with adapted questionnaires also used PLS-SEM as the preferred method [ 36 , 36 , 38 ]. PLS-SEM evaluations are comprised of an outer model (measurement model) and an inner model (structural model).
The structural model was validated using a nonparametric bootstrap procedure to test the statistical significance of path coefficients with 5,000 bootstrap samples [ 27 ].
All analyses were performed using Stata statistical software (version 14.0, Stata Corporation, College Station, Texas, USA). All statistical analyses were conducted at the significance level of 0.05, and all tests were two-tailed whenever appropriate.
The sample characteristics are presented in Table 2 . Of the respondents, 56% were male, the average age was 61.2 years, and 33% of respondents received tertiary or higher education.
Internal consistency reliability, convergent and discriminant reliability.
The internal consistency reliabilities for all constructs were greater than the threshold value (i.e. α ≥ 0.7; CR ≥ 0.7). However, the item loadings for Question 5 of Performance Expectancy and Question 4 of Effort Expectancy were < 0.70; hence these 2 items were excluded from the PLS-SEM. The other items had met criteria for being statistically significant ( p < 0.001) and greater than 0.70 (see Table 3 ).
After excluding Question 5 of Performance Expectancy and Question 4 of Effort Expectancy, the AVE values for all constructs were greater than 0.5. Thus, convergent validity was supported. The square of the correlation coefficients was smaller than the corresponding square roots of the AVE estimates for all pairs of constructs. Therefore, discriminant validity was achieved (see Table 4 ).
The structural model
The resulting path coefficients are depicted in Fig. 1 , after bootstrapping analysis.
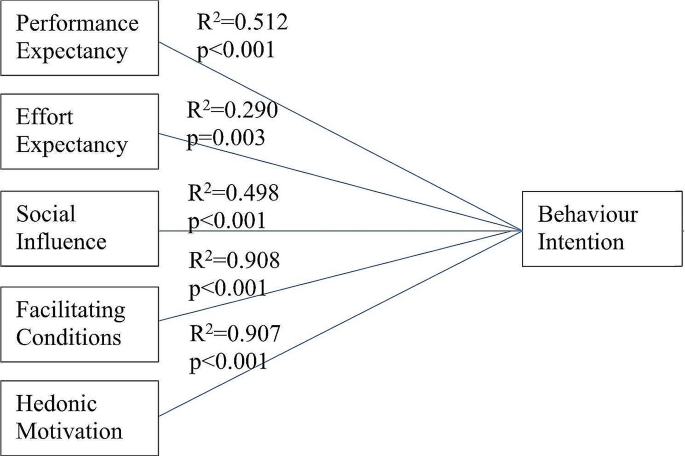
Resulting path coefficients after bootstrapping analysis for constructs affecting behvioural intention to accept task shifting of specialized scans to AI-novice combination in the extended UTAUT2 model
The results revealed that the extended UTAUT2 model could explain 85% of the variance in behavioural intention. All the hypothesized variables showed significant relationship to behavioral intention. Facilitating conditions, hedonic motivation and performance expectancy showed the strongest relationship to behavioural intention.
Patient factors such as age ( p = 0.181), education level( p = 0.218) and gender( p = 0.776) did not significantly affect behavioural intention.
Task shifting of specialized studies is one key area where AI is helping to revolutionize healthcare. Examples include interpreting medical imaging, such as chest x-rays [ 39 ], and implementing nationwide screening programs, such as retinal imaging [ 40 ]. While the medical community focuses on the accuracy and theoretical foundations of the AI, studies have shown that despite comparable or even superior accuracy of AI, patients have found it challenging to accept AI in medical care [ 41 , 42 ]. Hence in our study, apart from determining the accuracy of the AI-novice combination, we also sought to study the motivators and barriers from the patient’s perspective toward acceptance of such new models of care.
The UTAUT2 suggests that actual use of any technology is affected by one’s behavioural intention to use it. It proposes that four main constructs affect behavioural intention: usage, intention—performance expectancy, effort expectancy, social influence and facilitating conditions. It extends three more constructs (hedonic motivation, price value and habit) in its framework. The UTAUT2 provides the highest explanatory power of all standard acceptance models. Knowing these underlying important constructs will help organizations improve their technology implementation processes [ 21 , 22 ].
Our study showed that all four main constructs showed a significant relationship with behavioural intention, influencing 85.8% of variance to behavioural intention. The top 3 constructs influencing behavioural intention in our study were facilitating conditions, hedonic motivation, and performance expectancy. While medical performance expectancy was the most important construct in many other studies that focused on healthcare professionals [ 25 ], facilitating conditions may exert stronger influence from the patients’ perspective [ 43 , 44 ]. This does not mean that performance expectancy, which is the perception that using the technology will benefit the patient, is less important; rather, in a complex network like the healthcare system, facilitating conditions play an important and significant role in access. Hedonic motivation, which is the fun and pleasure derived from using the technology, represents an affective component of the UTAUT2 [ 26 ] and looks at the user’s intrinsic motivation [ 45 , 46 ] rather than the extrinsic motivation from the four main constructs. Our study shows that hedonic motivation is a significant factor in behavioural intention. Therefore, making the process fun and pleasurable is important towards patient adoption [ 47 ]. Facilitating conditions and hedonic motivation had a strong correlation ( r = 0.992, p -value < 0.001) in our study. This intuitively suggests that having the resources to improve access itself also improves overall patient experience and enjoyment, placing further importance on facilitating conditions in the successful implementation of this new pathway.
Age, gender and education levels were not significant modifiers of behavioural intention. This was congruent with prior studies on health apps, where age, gender and education levels were weakly correlated with behavioural intention [ 36 ].
Prior studies of UTAUT2 in healthcare largely looked at patients; acceptance toward digital health applications [ 36 , 37 , 48 , 49 ] and have largely found that hedonic motivation, social influence and habit, rather than utilitarian factors predicted usage intention. As the nature of digital health applications are different from AI-assisted taskshifting and diagnosis, we felt that it was important to apply the UTAUT2 framework in this new model of care. In the area of AI-assisted diagnosis, from the healthcare workers’ perspective, Cheng et al. [ 50 ] demonstrated that performance and effort expectancy directly influenced usage intention. Our study showed that patients have a different considerations, suggesting that it is important to look at factors affecting different stakeholders to ensure successful implementation.
The sustainability of new pathways and health programs requires understanding the implementation process and factors that impact adoption. The UTAUT2 is one of the most comprehensive theoretical frameworks developed to investigate factors related to future usage intention [ 47 ]. When detailing the implementation process, understanding these factors will allow changemakers to focus more on certain aspects, such as facilitating conditions, hedonic motivation and performance expectancy. Our study is the first to look at patients’ attitudes toward task shifting using AI in healthcare, using the UTAUT2 theory. This provides important information for the implementation of such pathways in future.
Limitations
Our study has several limitations. First, our study is based in Singapore, which has high levels of access to digital technologies. Based on the Singapore Digital Society Report 2023 [ 51 ], 97% of Singaporeans own a smartphone. The study also found that 65% of Singaporeans are keen to try out new digital technologies. This limits the generalizability of our study’s findings to settings that are less advantaged technologically, but it may still be used to make future predictions in these settings. Furthermore, we did not control for patients’ digital literacy, technology acceptance and attitudes towards AI in general in our study, which may be potential confounders for our study’s findings. In our study, we had chosen to omit items with item loadings < 0.70 for convergent validity, after ensuring that each construct had at least two questions for measurement. We recognize that by omitting these items, especially borderline cases, can result in missing out important information that can be measured by the question. Also, our study’s design offered patients the experience of both the standard cardiac sonography and AI-novice workflow for comparison on the same day. While the study team attempted to distinguish the two experiences by explanation and conducting the AI-novice workflow only after the standard echocardiogram as per protocol, we acknowledge that patients may not be fully able to distinguish their experiences and perceptions for each method clearly and this may limit the accuracy of our study’s findings.
This study shows that all constructs proposed by the UTAUT2 model are essential for patients’ successful acceptance of AI-enabled novice-performed echocardiogram. These results are important for stakeholders and changemakers such as policymakers, governments, physicians, and insurance companies, as they design adoption strategies to ensure successful patient engagement by focusing on factors affecting the facilitating conditions, hedonic motivation and performance expectancy for AI technologies used in healthcare task shifting. Future studies can look into the practical design of adoption strategies based on these key factors and to evaluate their impact on patients’ acceptance of similar AI technologies.
Data availability
The datasets used and/or analysed during the current study are available from the corresponding author on reasonable request.
Abbreviations
Artificial intelligence
Area under the curve
Average variance extracted
Composite reliability
Deep learning
- Machine learning
Left ventricular ejection fraction
SEM–partial least squares structural equation modelling
Unified theory of acceptance and use of technology, extended version
Bodenheimer T, Sinsky C. From triple to quadruple aim: care of the patient requires care of the provider. Ann Fam Med. 2014;12:573–6.
Article PubMed PubMed Central Google Scholar
Berwick DM, Nolan TW, Whittington J. The triple aim: care, health, and cost. Health Aff (Millwood). 2008;27:759–69.
Article PubMed Google Scholar
Bajwa J, Munir U, Nori A, Williams B. Artificial intelligence in healthcare: transforming the practice of medicine. Future Healthc J. 2021;8:e188.
Zhang A, Xing L, Zou J, Wu JC. Shifting machine learning for healthcare from development to deployment and from models to data. Nature Biomedical Engineering 2022;6:12 6;1330–1345 (2022).
Rajpurkar P, Chen E, Banerjee O, Topol E. J. AI in health and medicine. Nature Medicine 2022;28:1 28, 31–38 (2022).
Bohr A, Memarzadeh K. The rise of artificial intelligence in healthcare applications. Artif Intell Healthc. 2020;25. https://doi.org/10.1016/B978-0-12-818438-7.00002-2 .
Barragán-Montero A, et al. Artificial intelligence and machine learning for medical imaging: a technology review. Phys Med. 2021;83:242.
Zhou SK, Rueckert D, Fichtinger G. Handbook of medical image computing and computer assisted intervention.
Gupta M, Konar D, Bhattacharyya S, Biswas S, editors. Computer Vision and Machine Intelligence in Medical Image Analysis. International Symposium, ISCMM. Singapore: Springer Singapore; 2019.
Ardila D et al. End-to-end lung cancer screening with three-dimensional deep learning on low-dose chest computed tomography. Nature Medicine 2019;25:6 25, 954–961 (2019).
Novikov AA, et al. Fully Convolutional architectures for Multiclass segmentation in chest radiographs. IEEE Trans Med Imaging. 2017;37:1865–76.
Article Google Scholar
Gulshan V, et al. Development and validation of a deep learning algorithm for detection of Diabetic Retinopathy in Retinal Fundus photographs. JAMA. 2016;316:2402–10.
Lim JI et al. Artificial Intelligence Detection of Diabetic Retinopathy: Subgroup Comparison of the EyeArt System with Ophthalmologists’ Dilated Examinations. Ophthalmology science 2022, 3.
Zhou D et al. Diagnostic evaluation of a deep learning model for optical diagnosis of colorectal cancer. Nat Commun 2020, 11.
Lu MY, et al. AI-based pathology predicts origins for cancers of unknown primary. Nature. 2021;594:106–10.
Article CAS PubMed Google Scholar
Esteva A, et al. Dermatologist-level classification of skin cancer with deep neural networks. Nature. 2017;542:115–8.
Article CAS PubMed PubMed Central Google Scholar
Giordano C, et al. Accessing Artificial Intelligence for clinical decision-making. Front Digit Health. 2021;3:645232.
AI POCUS. https://echonous.com/product/kosmos-ai/ .
Tromp J et al. A formal validation of a deep learning-based automated workflow for the interpretation of the echocardiogram. Nat Commun 13, (2022).
Johri AM, et al. Can a teaching intervention reduce Interobserver Variability in LVEF Assessment: a Quality Control Exercise in the Echocardiography Lab. JACC Cardiovasc Imaging. 2011;4:821–9.
Venkatesh V, Thong JYL, Xu X. Consumer Acceptance and Use of Information Technology: extending the Unified Theory of Acceptance and Use of Technology. Behav Mark eJournal. 2012;36:157–78.
Google Scholar
Venkatesh V, Morris MG, Davis GB, Davis F. D. user acceptance of information technology: toward a unified view. MIS Q. 2003;27:425–78.
Al Qeisi K, Al-Abdallah G. Internet Banking Adoption in Jordan: a behavioral Approach. Int J Mark Stud 5, (2013).
Cheng M, Li X, Xu J. Promoting Healthcare workers’ adoption intention of Artificial-Intelligence-assisted diagnosis and treatment: the Chain Mediation of Social Influence and Human-Computer Trust. Int J Environ Res Public Health 19, (2022).
Castanha J, Pillai SKB. Indrawati. What influences Consumer Behavior toward Information and Communication Technology Applications: a systematic literature review of UTAUT2 model. Adv Intell Syst Comput. 2021;1270:317–27.
Tamilmani K, Rana NP, Prakasam N, Dwivedi YK. The battle of Brain vs. Heart: a literature review and meta-analysis of hedonic motivation use in UTAUT2. Int J Inf Manage. 2019;46:222–35.
Hair J, Hollingsworth CL, Randolph AB, Chong AY. L. An updated and expanded assessment of PLS-SEM in information systems research. Industrial Manage Data Syst. 2017;117:442–58.
Fornell C, Larcker DF. Evaluating Structural equation models with unobservable variables and measurement error. J Mark Res. 1981;18:39.
Henseler J, Ringle CM, Sarstedt M. A new criterion for assessing discriminant validity in variance-based structural equation modeling. J Acad Mark Sci. 2015;43:115–35.
Hair JF, Ringle CM, Sarstedt M. PLS-SEM: indeed a silver bullet. J Mark Theory Pract. 2011;19:139–52.
Diño MJS, De Guzman AB. Using partial least squares (PLS) in Predicting behavioral intention for Telehealth Use among Filipino Elderly. Educ Gerontol. 2015;41:53–68.
Schaper LK, Pervan GP. ICT and OTs: a model of information and communication technology acceptance and utilisation by occupational therapists. Int J Med Inf. 2006;76(Suppl 1):S212–21.
Falk R. Frank. & Miller, N. B. A primer for soft modeling. 103 (1992).
Marcoulides GA. The partial least squares Approach to Structural equation modeling. 295–336 (1998) https://doi.org/10.4324/9781410604385-10 .
Kock N, Hadaya P. Minimum sample size estimation in PLS-SEM: the inverse square root and gamma-exponential methods. Inform Syst J. 2018;28:227–61.
Schomakers E-M, Lidynia C, Vervier LS, Valdez AC, Ziefle M. Applying an extended UTAUT2 model to explain user Acceptance of Lifestyle and Therapy Mobile Health apps: Survey Study. JMIR Mhealth Uhealth. 2022;10:e27095.
Chang YT, Chao CM, Yu CW, Lin FC. Extending the Utility of UTAUT2 for Hospital Patients’ Adoption of Medical Apps: Moderating Effects of e-Health Literacy. Mobile Information Systems 2021, (2021).
Schretzlmaier P, Hecker A, Ammenwerth E. Extension of the Unified Theory of Acceptance and Use of Technology 2 model for predicting mHealth acceptance using diabetes as an example: a cross-sectional validation study. BMJ Health Care Inf. 2022;29:e100640.
Born J et al. On the role of artificial intelligence in medical imaging of COVID-19. Patterns (N Y) 2, (2021).
Ta AWA, et al. Two Singapore public healthcare AI applications for national screening programs and other examples. Health Care Sci. 2022;1:41–57.
Longoni C, Bonezzi A, Morewedge CK. Resistance to Medical Artificial Intelligence. J Consum Res. 2019;46:629–50.
Asan O, Bayrak AE, Choudhury A. Artificial Intelligence and Human Trust in Healthcare: Focus on clinicians. J Med Internet Res. 2020;22:e15154.
Alam MZ, Hoque MR, Hu W, Barua Z. Factors influencing the adoption of mHealth services in a developing country: a patient-centric study. Int J Inf Manage. 2020;50:128–43.
Chang Y-T, Chao C-M, Yu C-W, Lin F-C. Extending the utility of UTAUT2 for hospital patients’ adoption of medical apps: moderating effects of e-Health literacy. (2021) https://doi.org/10.1155/2021/8882317 .
Sarani A et al. Emotional intelligence and self-efficacy among deputy’s administrative staff of Kerman University of Medical Sciences. J Educ Health Promot 9, (2020).
Sheikhbardsiri H, Sheikhasadi H, Mahani S, Mohamadi M. Emotional intelligence and learning strategies of postgraduate students at Kerman University of Medical Sciences in the southeast of Iran. J Educ Health Promot 9, (2020).
Sułkowski Ł, Kaczorowska-Spychalska D. Determinants of the adoption of AI wearables - practical implications for marketing. Hum Technol. 2021;17:294–320.
Palas JU, Sorwar G, Hoque MR, Sivabalan A. Factors influencing the elderly’s adoption of mHealth: an empirical study using extended UTAUT2 model. BMC Med Inf Decis Mak. 2022;22:1–21.
Schretzlmaier P, Hecker A, Ammenwerth E. Suitability of the Unified Theory of Acceptance and Use of Technology 2 Model for Predicting mHealth Acceptance using diabetes as an example: qualitative methods Triangulation Study. JMIR Hum Factors 9, (2022).
Cheng M, Li X, Xu J. Promoting Healthcare Workers’ Adoption Intention of Artificial-Intelligence-Assisted Diagnosis and Treatment: The Chain Mediation of Social Influence and Human–Computer Trust. IJERPH 19, 1–19 (2022).
Singapore Digital Society Report | IMDA - Infocomm Media Development Authority. https://www.imda.gov.sg/about-imda/research-and-statistics/singapore-digital-society-report .
Download references
Acknowledgements
Not applicable.
The PANES-HF is supported by a research grants from Singapore Ministry of Health’s National Medical Research Council under its OF-YIRG ( MOH-000941-00) and AstraZeneca. The funders had no role in the design and conduct of the study; collection, management, analysis, and interpretation of the data; and preparation, review or approval of the manuscript; and decision to submit the manuscript for publication.
Author information
Authors and affiliations.
National Heart Centre Singapore, 5 Hospital Drive, Singapore, 169609, Singapore
Weiting Huang, Tracy Koh, Chanchal Chandramouli, Choon Ta Ng & Carolyn Su Ping Lam
Duke-NUS Medical School, Singapore, Singapore
Weiting Huang, Tracy Koh, Chanchal Chandramouli, Choon Ta Ng, Carolyn Su Ping Lam & Jasper Tromp
Saw Swee Hock School of Public Health, National University of Singapore, National University Health System Singapore, Singapore, Singapore
Jasper Tromp
Us2.ai, Singapore, Singapore
Yoran Hummel, Feiqiong Huang & Carolyn Su Ping Lam
School of Mechanical and Aerospace Engineering, Nanyang Technological University, Singapore, Singapore
Mark Kei Fong Wong & Eddie Yin Kwee Ng
National Healthcare Group Polyclinics, Singapore, Singapore
Wen Chong Ong
You can also search for this author in PubMed Google Scholar
Contributions
WTH had full access to all the data in the study and takes responsibility for the integrity of the data and the accuracy of the data analysis.All authors critically reviewed and contributed to the intellectual content of the manuscript. WTH, JT, CC, TK and CSPL were involved with the conception of the study. Initial data preparation was done by WTH. WTH, WO and TK performed the statistical analyses, supported by JT, FH, YH, CC, CN, MW and EN and drafted the manuscript. CSPL and CN provided the clinical expertise. MW and EN provided input on the translation of AI. All authors have read and approved the final version of the manuscript.
Corresponding author
Correspondence to Weiting Huang .
Ethics declarations
Ethics approval and consent to participate.
This study was approved by the SingHealth Centralised Institutional Review Board (Ref. No. 2021/2361, approved 06/01/2021.) All patients gave written informed consent. The first patient was recruited on 09/28/2021.
Consent for publication
Not Applicable.
Competing interests
The authors declare no competing interests.
Additional information
Publisher’s note.
Springer Nature remains neutral with regard to jurisdictional claims in published maps and institutional affiliations.
Electronic supplementary material
Below is the link to the electronic supplementary material.
Supplementary Material 1
Rights and permissions.
Open Access This article is licensed under a Creative Commons Attribution 4.0 International License, which permits use, sharing, adaptation, distribution and reproduction in any medium or format, as long as you give appropriate credit to the original author(s) and the source, provide a link to the Creative Commons licence, and indicate if changes were made. The images or other third party material in this article are included in the article’s Creative Commons licence, unless indicated otherwise in a credit line to the material. If material is not included in the article’s Creative Commons licence and your intended use is not permitted by statutory regulation or exceeds the permitted use, you will need to obtain permission directly from the copyright holder. To view a copy of this licence, visit http://creativecommons.org/licenses/by/4.0/ . The Creative Commons Public Domain Dedication waiver ( http://creativecommons.org/publicdomain/zero/1.0/ ) applies to the data made available in this article, unless otherwise stated in a credit line to the data.
Reprints and permissions
About this article
Cite this article.
Huang, W., Ong, W.C., Wong, M.K.F. et al. Applying the UTAUT2 framework to patients’ attitudes toward healthcare task shifting with artificial intelligence. BMC Health Serv Res 24 , 455 (2024). https://doi.org/10.1186/s12913-024-10861-z
Download citation
Received : 22 December 2023
Accepted : 13 March 2024
Published : 11 April 2024
DOI : https://doi.org/10.1186/s12913-024-10861-z
Share this article
Anyone you share the following link with will be able to read this content:
Sorry, a shareable link is not currently available for this article.
Provided by the Springer Nature SharedIt content-sharing initiative
- Healthcare artificial intelligence
- Task shifting
- Point-of-care ultrasound
- Echocardiography
- Technology acceptance
- Patient attitudes
BMC Health Services Research
ISSN: 1472-6963
- General enquiries: [email protected]
The independent source for health policy research, polling, and news.
Americans’ Challenges with Health Care Costs
Lunna Lopes , Alex Montero , Marley Presiado , and Liz Hamel Published: Mar 01, 2024
This issue brief was updated on March 1, 2024 to include the latest KFF polling data.
For many years, KFF polling has found that the high cost of health care is a burden on U.S. families, and that health care costs factor into decisions about insurance coverage and care seeking. These costs and the prospect of unexpected medical bills also rank as the top financial worries for adults and their families, and recent polling shows that lowering out-of-pocket health care costs is by and large the public’s top health care priority. Health care affordability is also one of the top issues that voters want to hear presidential candidates talk about during the 2024 election. This data note summarizes recent KFF polling on the public’s experiences with health care costs. Main takeaways include:
- About half of U.S. adults say it is difficult to afford health care costs, and one in four say they or a family member in their household had problems paying for health care in the past 12 months. Younger adults, those with lower incomes, adults in fair or poor health, and the uninsured are particularly likely to report problems affording health care in the past year.
- The cost of health care can lead some to put off needed care. One in four adults say that in the past 12 months they have skipped or postponed getting health care they needed because of the cost. Notably six in ten uninsured adults (61%) say they went without needed care because of the cost.
- The cost of prescription drugs prevents some people from filling prescriptions. About one in five adults (21%) say they have not filled a prescription because of the cost while a similar share say they have instead opted for over-the-counter alternatives. About one in ten adults say they have cut pills in half or skipped doses of medicine in the last year because of the cost.
- Those who are covered by health insurance are not immune to the burden of health care costs. About half (48%) of insured adults worry about affording their monthly health insurance premium and large shares of adults with employer-sponsored insurance (ESI) and those with Marketplace coverage rate their insurance as “fair” or “poor” when it comes to their monthly premium and to out-of-pocket costs to see a doctor.
- Health care debt is a burden for a large share of Americans. About four in ten adults (41%) report having debt due to medical or dental bills including debts owed to credit cards, collections agencies, family and friends, banks, and other lenders to pay for their health care costs, with disproportionate shares of Black and Hispanic adults, women, parents, those with low incomes, and uninsured adults saying they have health care debt.
- Notable shares of adults still say they are worried about affording medical costs such as unexpected bills, the cost of health care services (including out-of-pocket costs not covered by insurance, such as co-pays and deductibles), prescription drug costs, and long-term care services for themselves or a family member. About three in four adults say they are either “very” or “somewhat worried” about being able to afford unexpected medical bills (74%) or the cost of health care services (73%) for themselves and their families. Additionally, about half of adults would be unable to pay an unexpected medical bill of $500 in full without going into debt.
Difficulty Affording Medical Costs
Many U.S. adults have trouble affording health care costs. While lower income and uninsured adults are the most likely to report this, those with health insurance and those with higher incomes are not immune to the high cost of medical care. About half of U.S. adults say that it is very or somewhat difficult for them to afford their health care costs (47%). Among those under age 65, uninsured adults are much more likely to say affording health care costs is difficult (85%) compared to those with health insurance coverage (47%). Additionally, at least six in ten Black adults (60%) and Hispanic adults (65%) report difficulty affording health care costs compared to about four in ten White adults (39%). Adults in households with annual incomes under $40,000 are more than three times as likely as adults in households with incomes over $90,000 to say it is difficult to afford their health care costs (69% v. 21%). (Source: KFF Health Care Debt Survey: Feb.-Mar. 2022 )
When asked specifically about problems paying for health care in the past year, one in four adults say they or a family member in their household had problems paying for care, including three in ten adults under age 50 and those with lower household incomes (under $40,000). Affording health care is particularly a problem for those who may need it the most as one-third of adults who describe their physical health as “fair” or “poor” say they or a family member had problems paying for health care in the past 12 months. Among uninsured adults, half (49%) say they or a family member in their household had problems paying for health care, including 51% of uninsured adults who say they are in fair or poor health.
The cost of care can also lead some adults to skip or delay seeking services. One-quarter of adults say that in the past 12 months, they have skipped or postponed getting health care they needed because of the cost. The cost of care can also have disproportionate impacts among different groups of people; for instance, women are more likely than men to say they have skipped or postponed getting health care they needed because of the cost (28% vs. 21%). Adults ages 65 and older, most of whom are eligible for health care coverage through Medicare, are much less likely than younger age groups to say they have not gotten health care they needed because of cost.
One in four immigrant adults (22%) say they have skipped or postponed care in the past year, rising to about a third (36%) among those who are uninsured. Seven in ten (69%) of immigrant adults who skipped or postponed care (15% of all immigrant adults) said they did so due to cost or lack of health coverage. (Source: The 2023 KFF/LA Times Survey of Immigrants: Apr.-June 2023 )
Six in ten uninsured adults (61%) say they have skipped or postponed getting health care they needed due to cost. Health insurance, however, does not offer ironclad protection as one in five adults with insurance (21%) still report not getting health care they needed due to cost.
KFF health polling from March 2022 also looked at the specific types of care adults are most likely to report putting off and found that dental services are the most common type of medical care that people report delaying or skipping, with 35% of adults saying they have put it off in the past year due to cost. This is followed by vision services (25%), visits to a doctor’s offices (24%), mental health care (18%), hospital services (14%), and hearing services, including hearing aids (10%). (Source: KFF Health Tracking Poll: March 2022 )
A 2022 KFF report found that people who already have debt due to medical or dental care are disproportionately likely to put off or skip medical care. Half (51%) of adults currently experiencing debt due to medical or dental bills say in the past year, cost has been a probititor to getting the medical test or treatment that was recommended by a doctor. (Source: KFF Health Care Debt Survey: Feb.-Mar. 2022 )
Prescription Drug Costs
For many U.S. adults, prescription drugs are a component of their routine care. More than one in four (28%) adults say it is either “somewhat” or “very difficult” for them to afford to pay for prescription drugs. Affording prescription drugs is particularly difficult for adults who take four or more prescription medications (37%) and those in households with annual incomes under $40,000 (40%). Black and Hispanic adults are also more likely than White adults to say it is difficult for them to afford to pay for prescription drugs. (Source: KFF Health Tracking Poll: July 2023 )
The high cost of prescription drugs also leads some people to cut back on their medications in various ways. About one in five adults (21%) say in the past 12 months they have not filled a prescription because of the cost. A similar share (21%) say they have taken an over-the-counter drug instead of getting a prescription filled – rising to about one third of Hispanic adults (32%) and more than one in four adults (27%) with annual household incomes under $40,000. About one in ten adults say that in the past 12 months they have cut pills in half or skipped doses of medicine due to cost. (Source: KFF Health Tracking Poll: July 2023 )
Health Insurance Cost Ratings
Overall, most insured adults rate their health insurance as “excellent” or “good” when it comes to the amount they have to pay out-of-pocket for their prescriptions (61%), the amount they have to pay out-of-pocket to see a doctor (53%), and the amount they pay monthly for insurance (54%). However, at least three in ten rate their insurance as “fair” or “poor” on each of these metrics, and affordability ratings vary depending on the type of coverage people have.
Adults who have private insurance through employer-sponsored insurance or Marketplace coverage are more likely than those with Medicare or Medicaid to rate their insurance negatively when it comes to their monthly premium, the amount they have to pay out of pocket to see a doctor, and their prescription co-pays. About one in four adults with Medicare give negative ratings to the amount they have to pay each month for insurance and to their out-of-pocket prescription costs, while about one in five give their insurance a negative rating when it comes to their out-of-pocket costs to see a doctor.
Medicaid enrollees are less likely than those with other coverage types to give their insurance negative ratings on these affordability measures (Medicaid does not charge monthly premiums in most states, and copays for covered services, where applied, are required to be nominal.) (Source: KFF Survey of Consumer Experiences with Health Insurance )
Health Care Debt
In June 2022, KFF released an analysis of the KFF Health Care Debt Survey , a companion report to the investigative journalism project on health care debt conducted by KFF Health News and NPR, Diagnosis Debt . This project found that health care debt is a wide-reaching problem in the United States and that 41% of U.S. adults currently have some type of debt due to medical or dental bills from their own or someone else’s care, including about a quarter of adults (24%) who say they have medical or dental bills that are past due or that they are unable to pay, and one in five (21%) who have bills they are paying off over time directly to a provider. One in six (17%) report debt owed to a bank, collection agency, or other lender from loans taken out to pay for medical or dental bills, while similar shares say they have health care debt from bills they put on a credit card and are paying off over time (17%). One in ten report debt owed to a family member or friend from money they borrowed to pay off medical or dental bills.
While four in ten U.S. adults have some type of health care debt, disproportionate shares of lower income adults, the uninsured, Black and Hispanic adults, women, and parents report current debt due to medical or dental bills.
Vulnerabilities and Worries About Health Care and Long-Term Care Costs
A February 2024 KFF Health Tracking Poll shows unexpected medical bills and the cost of health care services are at the top of the list of people’s financial worries, with about three-quarters of the public – and similar shares of insured adults younger than 65 – saying they are at least somewhat worried about affording unexpected medical bills (74%) or the cost of health care services (73%) for themselves and their families. Just over half (55%) of the public say they are “very” or “somewhat worried” about being able to afford their prescription drug costs, while about half (48%) of insured adults say they are worried about affording their monthly health insurance premium.
Worries about health care costs pervade among a majority of adults regardless of their financial situation . Among adults who report difficulty affording their monthly bills, more than eight in ten say they are worried about the cost of health care services (86%) or unexpected medical bills (83%). Among those who report being just able to afford their bills, about eight in ten say they are worried about being able to afford unexpected medical bills (84%) or health care services (83%). And even among adults who say they can afford their bills with money left over, six in ten nonetheless say they are “very” or “somewhat worried” about being able to afford unexpected medical bills (62%) or the cost of health care services (60%) for themselves and their family. (Source: KFF Health Tracking Poll: February 2024 )
Many U.S. adults may be one unexpected medical bill from falling into debt. About half of U.S. adults say they would not be able to pay an unexpected medical bill that came to $500 out of pocket. This includes one in five (19%) who would not be able to pay it at all, 5% who would borrow the money from a bank, payday lender, friends or family to cover the cost, and one in five (21%) who would incur credit card debt in order to pay the bill. Women, those with lower household incomes, Black and Hispanic adults are more likely than their counterparts to say they would be unable to afford this type of bill. (Source: KFF Health Care Debt Survey: Feb.-Mar. 2022 )
Among older adults, the costs of long-term care and support services are also a concern. Almost six in ten (57%) adults 65 and older say they are at least “somewhat anxious” about affording the cost of a nursing home or assisted living facility if they needed it, and half say they feel anxious about being able to afford support services such as paid nurses or aides. These concerns also loom large among those between the ages of 50 and 64, with more than seven in ten saying they feel anxious about affording residential care (73%) and care from paid nurses or aides (72%) if they were to need these services. See The Affordability of Long-Term Care and Support Services: Findings from a KFF Survey for a deeper dive into concerns about the affordability of nursing homes and support services.
- Health Costs
- Racial Equity and Health Policy
- Private Insurance
- Affordability
- High Deductible Plans
- Tracking Poll
Also of Interest
- Health Care Debt In The U.S.: The Broad Consequences Of Medical And Dental Bills
- KFF Health Tracking Poll – March 2022: Economic Concerns and Health Policy, The ACA, and Views of Long-term Care Facilities
- KFF’s Kaiser Health News and NPR Launch Diagnosis: Debt, a Yearlong Reporting Partnership Exploring the Scale, Impact, and Causes of the Health Care Debt Crisis in America
- How Financially Vulnerable are People with Medical Debt?
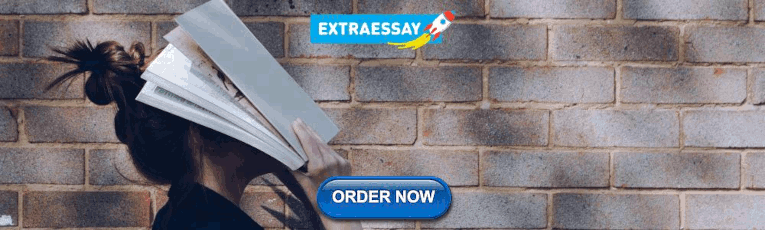
IMAGES
COMMENTS
The analysis consists of more than 23,500 health and biomedical research awards from 173 organisations accounting for an estimated expenditure of just over £5.0 billion in the calendar year 2022. As with previous analyses, our aim is to provide a high-quality overview of the UK's health research landscape; the conditions of focus, the types ...
The UK Health Research Analysis 2022 dataset has been made publicly available so that other funders can perform their own analyses and better support health and biomedical funding in the future. World-leading research and innovation. Professor Lucy Chappell, Chair of UKCRC, and Chief Scientific Adviser, Department of Health and Social Care ...
The analysis consists of more than 23,500 health and biomedical research awards from 173 organisations accounting for an estimated expenditure of just over £5.0 billion in the calendar year 2022. The aim of the analysis is to provide a high-quality overview of the UK's health research landscape; the conditions of focus, the types of research ...
The third report of the Health Research Analysis (2014) is now available to download. This report builds on the basis of the previous analyses in 2004/05 and 2009/10 and has expanded this third volume to include 64 charitable and public funders of medical research in the UK. As with previous reports, the data relates to a 'snapshot' of ...
UK Health Research Analysis reports are the largest analyses of UK health research funding. Our new report on 23,500 projects from 173 funding organisations, is the largest to date and shows over £5 billion of spend within the UK in 2022. Front cover of the new UK Health Research Analysis report. Looking across the whole 18-year period of our ...
The Health Research Classification System was applied to over 23,500 projects supported by 173 funding organisations, corresponding to just over £5 billion of spend within the UK in 2022. The analysis showed that of the £5 billion spent: £2.8 billion was invested directly on research projects; £1.4 billion was spent on infrastructure and ...
The Future of UK Clinical Research Delivery: 2021 to 2022 Implementation Plan was published and the HRA continues to play a central role in enabling UK research sector developments including our work leading strands of the Recovery, Resilience and Growth programme with DHSC in addition to our research systems transformation programme.
The UKCRC has carried out analyses of the health research portfolios of government and charity organisations funding health related research in the UK, using a bespoke Health Research Classification System. The first UK Health Research Analysis report, published in 2006, was the first ever national analysis of health research. It.
UK health research funding has increased since 2004, reaching £5 billion in 2022 with growth in translational and infection research, but is now plateauing.A report published today, the UK Health Research Analysis 2022, provides the most detailed overview yet of UK health research funding from 2004 to 2022 from all public sectors, including: • the governments of the four nations of the UK ...
This "UK Healthcare Outlook 2022" provides comprehensive insights about healthcare scenario, regulatory policies and reimbursement landscape in UK. ... As per the analysis, UK has a globally unique health and life sciences ecosystem. NHS offers a national research and evidence gathering platform with unique data and clinical insights. Track ...
In 2023 the UK Clinical Research Collaboration published its analysis of the 2022 Health Research funding allocations, which gives us an opportunity to see if there are any early indicators of levelling up since the last data release from 2018. Levelling Up assessment One of the UK's flagship policies 2019 to 2022, Levelling Up promised to ...
HSE 2022, HSE 2023, HSE 2024, and HSE 2025 (referred to as HSE 2022-2025 hereafter) will each include a new, general population sample of around 8,000 adults (aged 16 and over) and 2,000 children (aged 0-15), representative of the general population. Participants will be recruited from January to December each year from everyone living at ...
Nicola is a Civil Servant in the UK Health Security ... April 2022. Executive summary ... code audits of all organisations that have received public funding for health data research or analysis ...
Research and analysis Close; Research and analysis overview Global Burden of Disease (GBD) Global Burden of Disease (GBD) GBD Collaborator Network Diseases and injuries Diseases and injuries COVID-19 Disease, injury, and risk factsheets HIV/AIDS Health risks and issues Health risks and issues Air pollution Alcohol use Antimicrobial resistance (AMR)
Professor Ben Goldacre was commissioned by the government in February 2021 to review how to improve safety and security in the use of health data for research and analysis. The report makes 185 ...
The nation's largest funder of health and care research, providing the people, facilities and technology for research to thrive. ... In the biggest cystic fibrosis trial in the UK, NIHR-funded research has shown that a personalised web platform, CFHealthHub, could markedly increase adherence to treatment. Read our case studies.
Published: 11 January 2023. Today we kicked off our 2023 annual data collection. Each year we ask our charities to provide us with their research expenditure and grants portfolio data. This data helps us create a strong, collective, evidence-based voice for the sector that is fundamental for our policy work. Formal reps were sent an email with ...
Increasing patient loads, healthcare inflation and ageing population have put pressure on the healthcare system. Artificial intelligence and machine learning innovations can aid in task shifting to help healthcare systems remain efficient and cost effective. To gain an understanding of patients' acceptance toward such task shifting with the aid of AI, this study adapted the Unified Theory of ...
In June 2022, KFF released an analysis of the KFF Health Care Debt Survey, a companion report to the investigative journalism project on health care debt conducted by KFF Health News and NPR ...
41%. Percentage of teens with the highest social media use who rate their overall mental health as poor or very poor, compared with 23% of those with the lowest use. For example, 10% of the highest use group expressed suicidal intent or self-harm in the past 12 months compared with 5% of the lowest use group, and 17% of the highest users expressed poor body image compared with 6% of the lowest ...
Last month, the World Trade Organization's (WTO) 13th Ministerial Conference (MC13) tried to advance further rules to strengthen the existing Agreement on Fisheries Subsidies. That agreement, adopted by WTO members in June 2022 and still awaiting final ratification, prohibits subsidies that enable illegal fishing, fishing of overfished stocks and fishing of unmanaged stocks on the high seas.