Big Data Analytics: Applications, Challenges & Future Directions
Ieee account.
- Change Username/Password
- Update Address
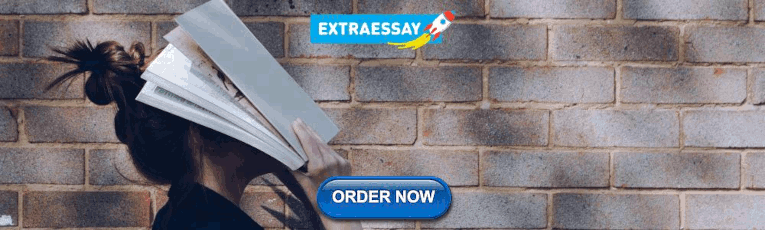
Purchase Details
- Payment Options
- Order History
- View Purchased Documents
Profile Information
- Communications Preferences
- Profession and Education
- Technical Interests
- US & Canada: +1 800 678 4333
- Worldwide: +1 732 981 0060
- Contact & Support
- About IEEE Xplore
- Accessibility
- Terms of Use
- Nondiscrimination Policy
- Privacy & Opting Out of Cookies
A not-for-profit organization, IEEE is the world's largest technical professional organization dedicated to advancing technology for the benefit of humanity. © Copyright 2024 IEEE - All rights reserved. Use of this web site signifies your agreement to the terms and conditions.
IEEE BigData 2016
IEEE BigData 2020 Now Taking Place Virtually
IEEE Big Data 2020 Accepted Papers
Main conference, 1. big data science and foundations, 2. big data infrastructure, 3. big data management, 4. big data search and mining, 5. big data security, privacy and trust, 6. hardware/os acceleration for big data, 7. big data applications, industry & government regular papers, industry & government short papers.
- Email Alert
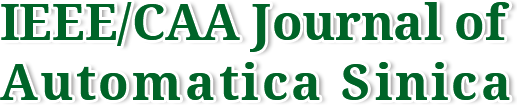
论文 全文 图 表 新闻
- Abstracting/Indexing
- Journal Metrics
- Current Editorial Board
- Early Career Advisory Board
- Previous Editor-in-Chief
- Past Issues
- Current Issue
- Special Issues
- Early Access
- Online Submission
- Information for Authors
- Share facebook twitter google linkedin
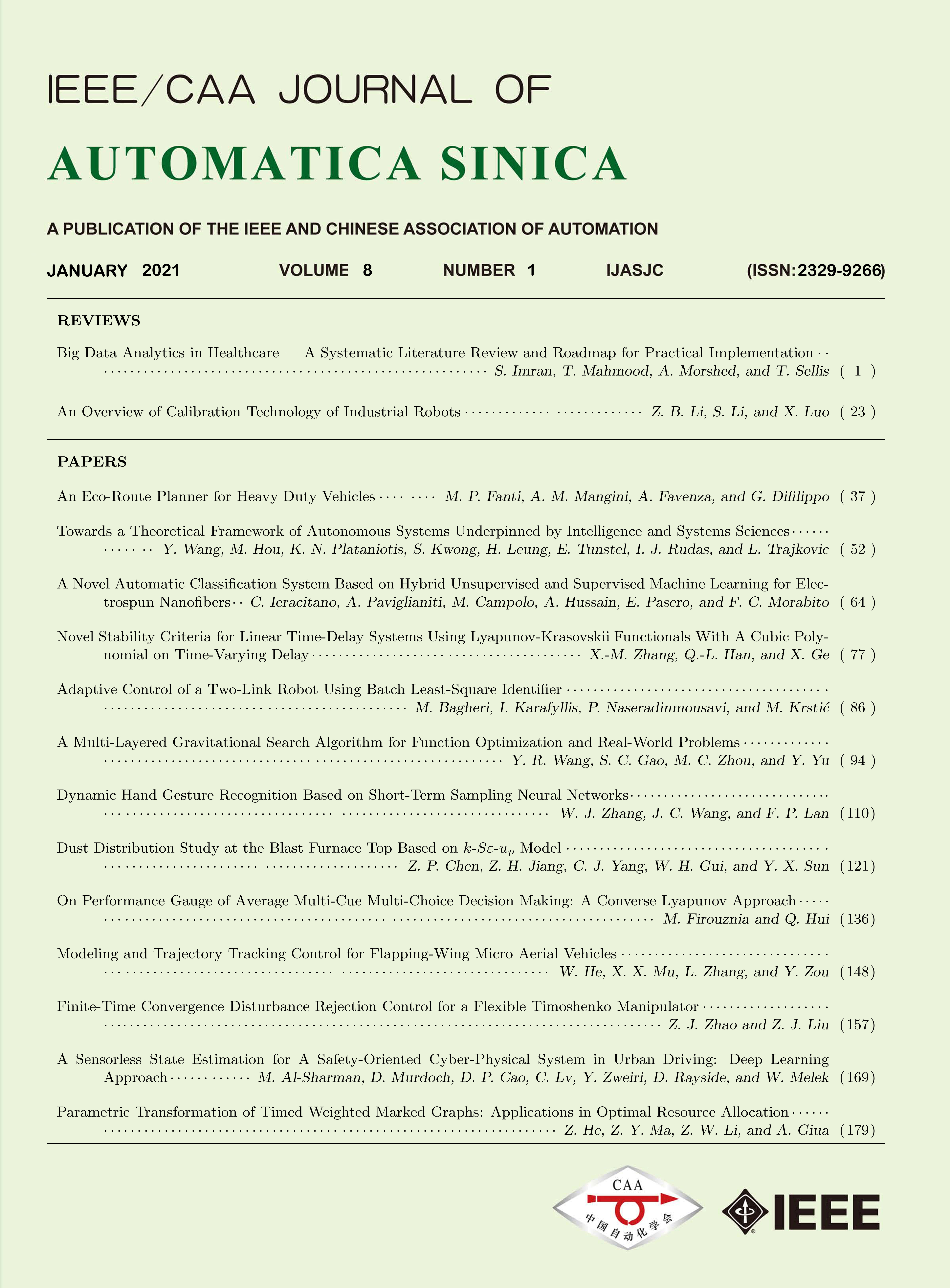
IEEE/CAA Journal of Automatica Sinica
- JCR Impact Factor: 11.8 , Top 4% (SCI Q1) CiteScore: 17.6 , Top 3% (Q1) Google Scholar h5-index: 77, TOP 5
Big Data Analytics in Healthcare — A Systematic Literature Review and Roadmap for Practical Implementation
Doi: 10.1109/jas.2020.1003384.
- Sohail Imran ,
- Tariq Mahmood , ,
- Ahsan Morshed ,
- Timos Sellis
Sohail Imran is an Assistant Professor and a doctoral candidate at the PAF-Karachi Institute of Economics and Technology, Pakistan. He has more than 15 years teaching experience in databases, data science, and big data analytics, and more than 10 years of training experience in databases (SQL and NoSQL), big data infrastructure, and data science for different institutes, universities, and the corporate sector. His research work is focused on mapping OLAP data warehousing schema into the distributed Hadoop environment. Specifically, he has developed a framework which creates dimension and fact tables over Hbase and Hive in a NoSQL schema-less manner and computes aggregates through SQL-overHadoop technologies (Presto, Drill, Spark SQL). This functionality is made scalable through containerization and more efficient through the use of Apache Spark
Tariq Mahmood is an Associate Professor at the Faculty of Computer Science, Institute of Business Administration (IBA), Pakistan. He received the Ph.D. degree in machine learning from University of Trento, Italy, and the M.S. degree in statistical machine learning from Universite Pierre et Marie Curie (Paris 6), France. He has published around 20 international journal and 35 conference publications with total 691 citations and h-index of 12 (Google Scholar). His research interests include BDA, deep learning and machine learning/data science. He heads the Big Data Analytics Laboratory at IBA, with the focus on imparting data science and big data certifications to students and industry professionals, implementing BDA-related industrial projects and researching in BDA technology stack, particularly to develop BDA architectures for different types of streaming and non-streaming data. He also consults in various local industries regarding business intelligence, data governance, BDA, and machine learning
Ahsan Morshed is a Lecturer in ICT at CQ University, Australia. Previously, he was a Research Fellow in Data Analytics at Swinburne University of Technology and a Senior Project Officer at RMIT University. He was also a Postdoctoral Fellow at CSIRO (Australia) on sensor data integration and machine learning, and an Information Management Specialist in the OEKC division at Food and Agriculture Organization (FAO) of UN in Rome, Italy. During his time in FAO, he acquired extensive skills in metadata standards, knowledge organization systems, ontologies, Linked Open Data management and information management tools. His research interests are the big data, data science, semantic web, linked open data and semantic machine learning. He holds the Ph.D. degree from the University of Trento, Italy. Dr. Morshed has 50 peer-reviewed publications (book, book chapter, journals, conference and workshop papers), with 229 citations and an h-index of 6 (Google Scholar)
Timos Sellis (F’09) is a Professor at Swinburne University of Technology, Australia. He holds the diploma from National Technical University of Athens (NTUA), Greece, the M.Sc. degree from Harvard University, USA, and the Ph.D. degree from the University of California at Berkeley, USA. Timos has a significant international research reputation in big data, data analytics, data integration and spatiotemporal database systems. He is a Fellow of the Association for Computing Machinery (ACM) for his contributions to database query optimisation, spatial data management and data warehousing and also an Institute of Electrical and Electronics Engineers (IEEE) Fellow for his contributions to database query optimisation and spatial data management. In 2018 he was awarded the IEEE TCDE Impact Award, in recognition of his impact in the field and for contributions to database systems research and broadening the reach of data engineering research. Before joining Swinburne, Timos was the Director of the Institute for Management of Information Systems and Professor at the National Technical University of Athens. He has also held the role of Director, Big Data Lab at RMIT University
- Corresponding author: T. Mahmood is with the Faculty of Computer Science, Institute of Business Administration, Karachi 75270, Pakistan (e-mail: [email protected] )
- 1 https://neo4j.com
- 2 http://www.hl7.org/implement/standards/fhir/)
- 3 A group of graduate students participated in this activity over a period of 3 months. For the sake of brevity, the details are outside the scope of this paper.
- 4 To the best of our knowledge, this list is complete as of June 2020.
- 5 A detailed discussion of the nine compared papers is outside the scope of this work; we invite the reader to go through these papers for more required information.
- Revised Date: 2020-07-21
- Accepted Date: 2020-07-22
- Big data analytics (BDA) ,
- big data architecture ,
- healthcare ,
- NoSQL data stores ,
- patient care ,
- roadmap ,
- systematic literature review
Proportional views
通讯作者: 陈斌, [email protected].
沈阳化工大学材料科学与工程学院 沈阳 110142
Figures( 13 ) / Tables( 5 )
Article Metrics
- PDF Downloads( 323 )
- Abstract views( 4143 )
- HTML views( 909 )
- The most thorough systematic literature review on big data analytics applications to healthcare
- Focus on healthcare applications for NoSQL databases and Apache Hadoop ecosystem
- Proposes the first-ever Zeta architecture called Med-BDA for big healthcare data analytics
- Med-BDA has the potential to solve ALL current limitations for big healthcare data analytics
- We present business strategies to successfully implement Med-BDA in any clinical organization
- Copyright © 2022 IEEE/CAA Journal of Automatica Sinica
- 京ICP备14019135号-24
- E-mail: [email protected] Tel: +86-10-82544459, 10-82544746
- Address: 95 Zhongguancun East Road, Handian District, Beijing 100190, China
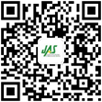
Export File

- Figure 1. Year-wise distribution of selected 99 articles
- Figure 2. Digital source distribution for six basic search queries
- Figure 3. Digital source distribution for six basic search queries + healthcare (HC)
- Figure 4. Digital source distribution for six basic search queries + healthcare analytics (HA)
- Figure 5. Hadoop components and ecosystem
- Figure 6. Data generators for an HIMS
- Figure 7. The 4 V’s big data identified in healthcare research literature
- Figure 8. The Challenges in Application of Big Data Analytics to Healthcare
- Figure 9. A snapshot of key-value store from healthcare domain
- Figure 10. A snapshot of columnar store from healthcare domain
- Figure 11. A snapshot of a document store from healthcare domain
- Figure 12. A snapshot of a graph store from healthcare domain
- Figure 13. Med-BDA: A state-of-the-art BDA architecture for healthcare

An official website of the United States government
The .gov means it’s official. Federal government websites often end in .gov or .mil. Before sharing sensitive information, make sure you’re on a federal government site.
The site is secure. The https:// ensures that you are connecting to the official website and that any information you provide is encrypted and transmitted securely.
- Publications
- Account settings
Preview improvements coming to the PMC website in October 2024. Learn More or Try it out now .
- Advanced Search
- Journal List
- Elsevier - PMC COVID-19 Collection

Big data analytics meets social media: A systematic review of techniques, open issues, and future directions
Sepideh bazzaz abkenar.
a Department of Computer Engineering, Science and Research Branch, Islamic Azad University, Tehran, Iran
Mostafa Haghi Kashani
b Department of Computer Engineering, Shahr-e-Qods Branch, Islamic Azad University, Tehran, Iran
Ebrahim Mahdipour
Seyed mahdi jameii.
- • A comprehensive systematic review on social big data analytic approaches is provided.
- • The main methods, pros, cons, evaluation methods, and parameters are discussed.
- • A scientific taxonomy of social big data analytic approaches is presented.
- • A detailed list of challenges and future research directions is outlined.
Social Networking Services (SNSs) connect people worldwide, where they communicate through sharing contents, photos, videos, posting their first-hand opinions, comments, and following their friends. Social networks are characterized by velocity, volume, value, variety, and veracity, the 5 V’s of big data. Hence, big data analytic techniques and frameworks are commonly exploited in Social Network Analysis (SNA). By the ever-increasing growth of social networks, the analysis of social data, to describe and find communication patterns among users and understand their behaviors, has attracted much attention. In this paper, we demonstrate how big data analytics meets social media, and a comprehensive review is provided on big data analytic approaches in social networks to search published studies between 2013 and August 2020, with 74 identified papers. The findings of this paper are presented in terms of main journals/conferences, yearly distributions, and the distribution of studies among publishers. Furthermore, the big data analytic approaches are classified into two main categories: Content-oriented approaches and network-oriented approaches. The main ideas, evaluation parameters, tools, evaluation methods, advantages, and disadvantages are also discussed in detail. Finally, the open challenges and future directions that are worth further investigating are discussed.
1. Introduction
Social networking services (or social networking sites) are online platforms distributed across various computers over long distances. Millions of people all around the world use SNSs to upload photos, videos, update their current status, and post daily comments ( Arora et al., 2019 , Lai et al., 2020 , Alalwan et al., 2017 ). They can join social networks in two ways: People may sign in by searching the network or may be invited by friends ( Kumar et al., 2010 ). After being accepted by contacts, the inviter often invites the invitee’s contacts, so the network expands in this way. The rapid growth of online social network relationship sites (e.g., Facebook, Myspace), media sharing networks (e.g., YouTube, Instagram), microblogging (e.g., Tumbler, Twitter), have encouraged researchers to investigate the published contents and analyse users’ behaviors ( Feng et al., 2018 , Heidemann et al., 2012 ). A social network refers to structures among people or other social entities with the edges related to their associations ( Busalim, 2016 ). In this structure, nodes are considered as people (or things) in the network, and interactions are expressed via the edges or links among them. A social network originated in mathematical graph theory, which is defined as a graph, G= (V, E), in which V is a set of vertices or nodes that refers to people or objects, and E denotes a set of edges or ties indicating the relationships that connect the respective people ( Bello-Orgaz et al., 2016 ).
Traditionally, data about users’ interests and behaviors were collected by questionnaires. While this is still a prominent way in social science, the emergence and popularity of social networks have allowed us to collect data regarding users’ behaviors in an unprecedented way, where we collect social data directly from users’ social platform accounts ( Jamali and Abolhassani, 2006 ). By collecting data from Online Social Networks (OSNs) and analyzing them, researchers can study a different aspect of users’ behaviors and get valuable information ( Martinez-Rojas et al., 2018 , Cetto et al., 2018 , Go and You, 2016 ). Now social science researchers send relevant queries to online social networks with the Application Programming Interface (API) to extract a large amount of users’ data ( Manovich, 2011 ). Further, most popular social networks provide API that allows researchers to gather and assess data from a given social media service ( Lomborg and Bechmann, 2014 ). When the data is not accessible through API, researchers develop web crawlers to crawl OSN website, collect and extract data by using HTTP requests, manipulating, and responding to them ( Abdesslem et al., 2012 ).
Thus, SNA is a scientific approach to extract data and analyse the structural characteristics of networks both quantitatively and qualitatively. In other words, the challenge of SNA is to study and extract the relationships among individuals, different organizations, and communities, which is essential for managing and reducing the complexities of social networks ( Otte and Rousseau, 2002 ). Social network is an efficient way to collaborate and share knowledge among the core groups of the organization, research, and development units ( Cross et al., 2002 , Parveen et al., 2015 ). In this respect, the emergence of new social networks and an increasing number of social media users led to the explosion of user-generated contents (UGCs). Thus, it is crucial to know what this big data is and what insight can be gained from it ( Boyd and Crawford, 2012 ). Big data refers to a massive volume and complicated amount of data that traditional tools are not able to manage and process effectively ( Katal et al., 2013 , Terrazas et al., 2019 , Canito et al., 2018 ). Big data is different from “a large dataset” by the fact that the former is complex and has unique attributes, while the latter is a dataset with many records ( di Bella et al., 2018 ).
In order to find out the role and influence of big data in social networks, the features of big data are described by using 5 V’s, volume, velocity, variety, veracity, and value ( Hadi et al., 2018 ). Volume means a vast amount of data that can be produced every second ( Gandomi and Haider, 2015 ). Velocity stands for rapid generation of data, often referred to as streaming data ( Kitchin, 2014 ). Variety represents various types of data, including structured, unstructured, and semi-structured data like images, videos, and texts ( Sagiroglu and Sinanc, 2013 , Pei et al., 2018 ). Veracity deals with the truthfulness of the data analysed and the accuracy behind any information ( Bello-Orgaz et al., 2016 ). The value refers to the valuable information extracted for business and real values of the data ( Peng et al., 2017 ). All these five features are available on social networks, so the most important application of big data is in the field of social media, which refers to big social data (or social big data) ; the data are obtained from social networks. Big data technologies have produced new and exciting challenges in social networks ( Duan et al., 2019 ).
Till today, with our observation and scrutiny, some surveys and Systematic Literature Reviews (SLRs) were performed on social big data analytics, but no comprehensive SLR has been written on social big data analytics that complicates the identification and assessment of the existing approaches, challenges, and gaps precisely. Moreover, due to the importance of big data analytics in social networks, this study aims at providing a systematic and comprehensive review to identify the challenges, potential future directions, merits, and demerits of this field. On the other hand, the association between SNA and big data analytic approaches is shown in particular and a research plan is investigated. An SLR presents a comprehensive review of state-of-the-art to reveal existing methods, challenges, and potential future research directions for research communities ( Brereton et al., 2007 ). We conduct this SLR with the intention of identifying , classifying , comparing social big data analytic approaches, evaluating the methods of existing papers systematically, and offering a reasonable taxonomy . Additionally, to attain this intension and to answer the following research questions, this methodological review is conducted:
- • Q1: What are the existing big data analytic approaches applied in social networks?
- • Q2: What parameters do the researchers employ to evaluate the big data analytics in social networks?
- • Q3: What are the tools used in social network analysis and big data areas?
- • Q4: What are the social big data analysis applications in the studied papers?
- • Q5: What are the datasets and case studies used in social big data analysis?
- • Q6: What evaluation methods are applied to measure the big data analytic approaches in social networks?
- • Q7: What are the challenges and future perspectives of big data analytic approaches in social networks?
We followed the guidelines in ( Brereton et al., 2007 , Kitchenham and Charters, 2007 , Jamshidi et al., 2013 , Jatoth et al., 2015 ) with the intension of exploring systematically, categorizing available social big data analytic approaches, and presenting a precise comparison analysis of approaches along with their potential challenges and limitations. This SLR presents a systematic review of the current studies on big data analytic approaches in social networks. For this purpose, 74 papers are chosen and compared to introduce a scientific taxonomy for the classification of big data analytic approaches in social networks. We summarize available methods, main ideas, applied tools, advantages, disadvantages, and evaluation parameters, and then provide statistical and analytical reports on them. Furthermore, this review identifies the motivation for presenting an SLR, outlines an abreast list of the primary challenges and open issues, and defines the significant areas where future research can improve the methods in the selected papers.
The remainder of this SLR is organized as can be seen in Fig. 1 . Section 2 discusses some related works and motivation. The research questions, the details of the selection process, and the research methodology are documented in Section 3 . Following, Section 4 provides a classification and a detailed study of the selected papers and demonstrates their main ideas, advantages, disadvantages, evaluation methods, tools, and evaluation parameters. 5 , 5.2 , respectively, disclose the analysis of the results, open issues, and future directions. Threats to validity and limitations are presented in Section 7 . At last, the conclusion is explained in Section 8 .

The structure of this SLR.
2. Related works and motivation
So far, there have been many reviews in the field of big data or social networks. However, the literature reviews conducted on this subject have some drawbacks. This section refers to several review studies that discussed social big data approaches.
2.1. The related studies on social big data analytic approaches
We explore the similarities and differences of the current reviews on this topic according to a systematic research, and the related works are summarized in surveys, and SLRs in 2.1.1 , 2.1.2 , respectively. Consequently, the weak points of these reviews are outlined in Section 2.1.3 . In Table 1 , a summary of the related works is illustrated in which such parameters as the main ideas, the review types, the paper selection processes, the taxonomies, open issues, evaluation parameters, applied tools, and the publication year of each study are represented.
Summary of the related works.
2.1.1. Surveys
Yaqoob ( 2016 ) surveyed the possible applications of Information Fusion (IF) in social media. They also discussed social big data processing technologies, similarities, and differences based on relevant parameters. Moreover, the challenges of applying IF and future research directions were presented. The authors reviewed several potential applications of IF, such as advanced marketing, fraud detection, social context-based recommendation systems, and an advanced feasibility study was performed for new businesses and optimal decision making. Findings showed that applying fusion increases the accuracy, reliability, and confidence. However, business intelligence, integration, sharing, security, and data sharing were not touched in the paper. Besides, this research did not mention a systematic structure and the paper selection process was not clearly indicated.
Furthermore, di Bella et al. ( 2018 ) analysed the metadata for Scopus database papers in the field of big data in 1957–2017. The authors found that actual tendencies in academic big data literature were not enough in the building of real-time indicators considering this massive volume of productions. This study was written in a non-systematic manner and there was a gap among its discussions in big data quality measures, privacy, transparency, and big data diffusion. Moreover, recently published papers in the years 2018–2019 were not considered.
In another study, Ghani et al. ( 2018 ) provided a survey on social network analysis and classified the literature based on data sources, characteristics, computational intelligence, techniques of analysis, and the quality of features from the published papers between 2011 and 2017. The characteristics of big data analysis were summarized into descriptive, diagnostic, predictive, and prescriptive analytics. The authors classified the big data analytic techniques into modeling, sentiment analysis, SNA, and text mining. The papers were categorized according to approaches, techniques, and qualitative features by authors. Although, the paper selection process was not mentioned, they provided a comprehensive perspective of the big social media analytic research topics, and several challenges such as data quality, data locality, velocity, data availability, and natural language processing remained unaddressed.
Many other researchers perused several social big data papers such as ( Bukovina, 2016 ) by reviewing technical analysis of social media to examine the behavior of capital markets, ( Martin and Schuurman, 2019 ) by surveying social media data for qualitative geographic analysis, ( Arnaboldi et al., 2017 ) by surveying the relationship between social big data analysis and the accounting function, ( Bello-Orgaz et al., 2016 ) by reviewing the big data analytic algorithms in social media and their applications, ( Peng et al., 2016 ) by conducting a survey to explore the architecture of influence analysis in social big data, and ( Guellil and Boukhalfa, 2015 , Gole and Tidke, 2015 , Paul et al., 2017 ) by surveying big data mining in social media.
2.1.2. SLRs
Moreover, Sebei et al. ( 2018 ) presented an SLR by considering journal and conference papers published between the years 2008 and 2018 to provide a clear description of the social network analysis process applicable to big data technologies. In addition to suggesting solutions, the authors identified the challenges encountered during big data analysis. The social network analytic processes, challenges, solutions, and big data tools related to each step were studied, but the relevant parameters for comparing big data-related technologies were not specified.
Finally, other social big data SLRs are conducted such as ( Al-Garadi, 2019 ) to detect cyber-attacks on social media via the aid of Machine Learning (ML) approaches, and ( Lerena et al., 2019 ) by reviewing firm-level innovations based on text-mining and social network analysis.
2.1.3. Concluding remark
Considering the overviewed papers, some weaknesses have been noticed as described below:
- • Some studies have not mentioned the periods of reviewed papers explicitly. In this paper, besides mentioning the scope of the study and the time range of articles, recently published articles have also been considered.
- • The lack of a systematic construction in the related papers made the selection process unclear.
- • Some papers have not been properly classified or have not presented any taxonomies. However, this paper not only provides a lucid and visual classification, but also defines a subclass for each of them.
- • Some studies have not analysed the assessment parameters and evaluation tools. This SLR presents applied tools, evaluation parameters, and evaluation methods of the studied papers.
- • Some of the related papers have not concentrated open issues explicitly, and future challenges have been enumerated briefly and implicitly. The presented literature is intended to highlight open issues well and precisely.
2.2. The motivation for an SLR on social big data analytic approaches
The need for an SLR is to identify , classify , and compare the existing research reviews on big data analytics in social networks. In order to show that a comprehensive SLR has not been already proposed, we searched Google Scholar with the following search string:
According to the reasons mentioned in Section 2.1.3 , and considering Table 1 , most of the retrieved reviews were not conducted systematically, their paper selection processes were unclear, and they did not propose any lucid classification in their papers. To the best of our scrutiny, only three SLRs have been conducted on this topic ( Sebei et al., 2018 , Al-Garadi, 2019 , Lerena et al., 2019 ) none of which has provided a complete systematic review to investigate SNA techniques, tools, strengths, weaknesses, open issues, evaluation parameters, and the application and critical role of big data in social networks. The two most similar efforts are in ( Ghani et al., 2018 ), which is a survey not an SLR; It only covers journal papers between 2011 and 2017 and excludes conferences, and ( Sebei et al., 2018 ), which is an SLR, covers the works between 2008 and 2018, but does not present evaluation parameters used in each studied paper. In ( Al-Garadi, 2019 ), researchers only examined cyber-attacks and security issues in social big data, which differed from our paper, and the time range of studied papers was not specified. Additionally, open issues were not specified in ( Lerena et al., 2019 ) and researchers in ( Al-Garadi, 2019 , Lerena et al., 2019 ) did not investigate the evaluation parameters and applied tools; therefore, writing an SLR that covers these weaknesses and highlights open issues and future research directions precisely is timely.
3. Research methodology
Researchers have conducted various studies on social networks and big data , their applications, and their challenges. In order to accomplish a comprehensive study of big data analytic approaches, this section presents an SLR method of big data analytic approaches in social networks. An SLR is a methodology to identify, classify, assess, and synthesize a comparative overview of the state-of-the-art in a specific subject ( Brereton et al., 2007 , Kitchenham et al., 2009 ). In contrast to other types of review papers, an SLR is a process of presenting a taxonomical review and performing a methodological analysis of the research literature to find the answers to problems and the given research questions related to specific research topics. The SLR has been used for the first time in medical fields ( Aznoli and Navimipour, 2017 ) and can be conducted in any field of study for an accurate understanding, reducing bias, and identifying open issues and future directions ( Rahimi et al., 2020 , Haghi Kashani et al., 2020 ). Since most review articles on big data analytic approaches in social networks were written in unstructured procedures, the purpose of this paper is to provide a rigorous process of the methodological steps for researching the literature in this scope.
In this systematic process, a three-phase guideline, namely planning , conducting , and documenting ( Brereton et al., 2007 ) is adopted, as depicted in Fig. 2 . The review is accompanied by an external evaluation of the outcome of each phase. We first identify the questions and the needs that are the motivation of this SLR in the planning phase. Then the articles in this subject are selected based on inclusion/exclusion criteria in the conducting phase. Ultimately, in the documenting phase, the observations are documented, and the results are analysed, compared, and visualized, which yields the answers to the research questions, then the final reports are represented. The three phases of the research methodology that are followed in this SLR are discussed below:

Overview of research methodology.
3.1. Planning phase
Planning begins with the determination of the research motivation for this SLR and finishes in a review protocol as follows:
Stage 1- Specifying the research motivation. According to the contribution of this SLR that is justified by comparing the available reviews explained in Section 2.2 , the motivation is specified at the first stage.
Stage 2- Defining research questions. In the second stage, according to the motivation of this paper, the research questions are defined that assists the development and validation of the review protocol. The research questions are stated below. By finding the answers to the questions, available gaps on this subject can be found, which can facilitate reaching new ideas in documenting phase.
Stage 3- Determining the review protocol. According to the goals of this SLR, in the previous stage, the research questions and the review scope were identified to adjust search strings for literature extraction ( Brereton et al., 2007 ). Moreover, a protocol was developed by following ( Calero et al., 2013 ) and our previous experience with SLR ( Haghi Kashani et al., 2020 , Rahimi et al., 2020 ). To evaluate the defined protocol before its execution, we requested an external specialist for feedback, who was experienced in conducting SLRs in this era. His feedback was applied in the upgraded protocol. A pilot study (approximately 25%) of the included papers was performed to reduce the bias between researchers and to enhance the data extraction process. We also enhanced the review scope, search strategies, and inclusion/exclusion during the pilot stage.
3.2. Conducting phase
The second phase of the research methodology is conducting, starting with paper selection, and culminating in data extraction. This section aims to represent the process of searching and selecting papers conducted in the second phase of the SLR. The process of selecting papers consists of a three-step guideline as depicted in Fig. 3 .
- • First step. The first step of the research process was searching through Google Scholar 1 as the dominant search engine based on well-known academic publishers such as Springer 2 , IEEE Explorer 3 , ScienceDirect 4 , SAGE 5 , Taylor&Francis 6 , Wiley 7 , Emerald 8 , ACM 9 , and Inderscience 10 based on titles and keywords. The search strings were defined as follows:
Inclusion/Exclusion criteria.

Paper selection process.
- • Third step. Finally, in the third step , the full texts of all selected papers were reviewed, and for further detailed analysis, 74 relevant papers were chosen, which could answer our research questions and fully describe the methods and challenges. Investigating 74 relevant papers assists us in proposing a classification on social big data analysis approaches in Section 4 and revealing the pros and cons of these approaches.
3.3. Documenting phase
As determined in Fig. 2 , in documenting phase, after documenting the observations, threats to validity and limitations are explored which is presented in Section 7 . Then the results are analysed, visualized, and reported in Section 5 .
4. Classification of the selected papers
In this section, 74 chosen papers are explored to examine social big data analysis objectives, techniques, and innovations; a review of the advantages and disadvantages of each approach is also presented. A taxonomy of the related literature is given in this paper, and the pictorial description of the proposed taxonomy for the reviewed papers is shown in Fig. 4 . Offering a taxonomy for social big data analysis is not a trivial and easy task. As researchers look at the problems in this area from various perspectives, each researcher performs this classification differently. By using this categorization, the reader can easily refer to each of these papers as a categorical reference. The selected papers use big data analytic techniques for analyzing social networks. These techniques are categorized into two major groups: Content-oriented approaches, and network-oriented approaches.

Taxonomy of social big data analysis.
Content-oriented approaches are classified into two subgroups, namely topical learning and opinion/sentiment learning. Topical learning can be performed in a single modal or a multimodal approach. Opinion/sentiment learning can be carried out in lexicon-based, learning-based, or hybrid approaches. Further, network-oriented approaches are classified into two groups: Embedding learning and community learning. Embedding learning has graph-based, non-graph based, and explanatory models, while, community learning is node-based or group-based. The papers relevant to content-oriented approaches and network-oriented approaches are reviewed in 4.1 , 4.2 , respectively. In this study, the methods of big data analysis on social networks are examined and evaluated with a list of important evaluation parameters. Further, the definition associated with evaluation parameters of the reviewed papers, as well as their formulas, is presented in Appendix A .
4.1. Content-oriented approaches
Nowadays, with the explosion of data in social networks that provides the researchers with a different type of contents instead of the traditional books and libraries, it is essential to analyse this immense volume of data. In this paper, the selected papers with topical learning and opinion/sentiment learning are reviewed in 4.1.1 , 4.1.2 , respectively. In 4.1.1 , 4.1.2 , classification of techniques, the definition of methods, and the related papers are discussed.
4.1.1. Overview of the topical learning approaches
In content-oriented approaches, topical learning focuses on the communication contents of social networks, consisting of text mining, video content analysis, and image analysis. It is the process of analyzing various types of unstructured data, like images, audio and video files, or different types of text including word, PDF files, PowerPoint slides, posts of weblogs and social network sites, or semi-structured data such as XML, HTML, JSON, and CSV files with the purpose of uncovering underlying similarities and hidden associations and transforming them into structured data for further analysis. The topical learning may be either performed “single modal” or “multi-modal” in which a “single modal” collects and analyses one modality (text OR audio OR image OR video) whereas “multi-modal” analyse a combination of various types of datasets such as text, audio, image, and video. According to the reviewed papers, the comparison between the specification and evaluation parameters is illustrated in Tables 3 and and4 . 4 . Table 3 summarizes the main ideas, advantages, disadvantages, evaluation methods, tools, and case studies along with their categories related to the papers in this approach. Table 4 presents a side by side comparison of the evaluation parameters in papers related to topical learning approaches.
Reviewing and comparing papers with topical learning approaches.
An overview of the evaluation parameters in papers with topical learning approaches.
In order to investigate the effects of social media on Eating Disorders (ED), Moessner et al. ( 2018 ) applied texts, linguistics, and lexical analysis with an unsupervised, bottom-up method to identify harmful posts. They did not investigate social media data in real-time, otherwise, the safety of ED-related communication could have been improved. Further, to execute the balance policies in the business application of social networks, Huo et al. ( 2018 ) presented a new logic Datalog. TS_u_Datalog was presented as the most appropriate logic Datalogs and a new programming language with both Active_U_Datalog and Distributed Temporal Logic (DTL) was introduced to implement contractual policies in a dynamic social media. The results of the time evaluation parameter of TS_u_Datalog could have been improved and used for blockchain systems, privacy-preserving of smartphones, and as a fault tolerance technique for wireless sensor networks.
To enhance health monitoring systems to detect infectious disease and to take preventive actions, Zadeh et al. ( 2019 ) presented a spatio-temporal platform to check out whether social posts could discover flu outbreaks in a particular area during the flu season. As some people do not activate their GPS or do not express their geographic locations in a social network profile, the geographic analysis cannot be done more deeply and accurately. More efficient ML techniques were needed to perform more in-depth analysis and to identify noise and unrelated social network posts. To recognize all repetitive and non-repetitive substring in passwords, Xylogiannopoulos et al. ( 2020 ) designed an efficient pattern detection system that can be embedded in social network platforms to generate a more robust and valid password. The results indicated that, contrary to common belief, long passwords are not safe, but passwords that are a combination of small/capital numbers and symbols are stronger than the others. This methodology did not have a limitation on the length and the type of characters. However, the proposed system could have been tested on other datasets, leading to different results.
In order to prevent the death caused by Adverse Drug Reactions (ADRs), Yang et al. ( 2015 ) used text classification to propose an automated framework to filter ADR related posts. A supervised learning method was applied to classify the extracted posts into positive/negative examples. The results of classification were used as an input to build an early warning system to prohibit future ADRs. Although the presented method generally outperformed in precision, recall, and F-measure, they did not extend their framework for various types of drugs. Furthermore, Cheung et al. ( 2015 ) presented a connection discovery system for follower/followee recommendations instead of user-generated tags and social graphs. They used Bag-of-Features Tagging (BoFT) to label user-shared images with BoFT labels, and a computer vision approach was employed to model the characteristics of user-shared images. In addition to the identification of user’s gender in the proposed system, the image classification performance was higher than K-mean, and there was no need to know K (the number of clusters in the clustering) in advance. However, the runtime of clustering and feature extraction was high. Subsequently, for more users and user-shared images, a big data system is required to manage and discover data.
Furthermore, to identify mental disorders in advance, Thorstad and Wolff ( 2019 ) scrutinized people’s every day mental and non-mental health topic posts on Reddit website. The outcome of the accuracy assessment indicated that people’s posts on clinical and non-clinical subreddits were highly and moderate predictive of mental disease, respectively. Also, it revealed that the predictions were more precise on recent past posts compared to distant past posts. The limitation was that posting a clinical post may not be a significant criterion for early diagnosis of psychological disorders, as some people may be affected by mental illnesses before posting. Besides, to identify vulnerabilities, Subroto and Apriyana ( 2019 ) offered an algorithmic model applying social media analytics and ML algorithms to protect cyber-attacks. Despite the highest accuracy of the model created by artificial neural networks, it was not scalable, having hardware limitations, and was tested only on a small sample of Twitter dataset, but the authors claimed that it did not affect the accuracy of the model.
Moreover, many other studies adopted clustering and ML algorithms in text mining and trending topics on big data of social platforms ( Straton et al., 2017 , Makaroğlu et al., 2019 , Vakali et al., 2016 , Aa et al., 2015 ). Also, researchers in ( Singh and Kaur, 2019 , Sachar and Khullar, 2017 ) proposed hybrid models by applying a metaheuristic approach to enhance the classification performance in the content analysis of social big data. Nowadays, as millions of users produce and share videos in various social media, Panarello et al. ( 2020 ) developed a framework for video transcoding processing in a short time. They applied Hadoop in their cloud federation framework to transcode videos to be compatible with sharing of users with different hardware/software devices. The evaluation results on real testbed demonstrated performance enhancement in terms of speed, scalability, and transcoding time, but security and privacy issues were neglected.
Alomari et al. ( 2020 ) developed a methodology based on text mining by using big data technologies for road traffic detection from Arabic tweets. The authors applied three machine learning algorithms, namely Logistic Regression, Support Vector Machine, and Naïve Bayes for classifying eight types of events. The evaluation results showed enhancement in text processing, leading to more accurate event detection with no prior knowledge about those events. However, this methodology could also be used to identify events other than road transportation. They did not focus on improving scalability and data management of the proposed method.
Zhou et al. ( 2016 ) proposed a private video recommendation system based on distributed online learning. Multimedia such as images, audios, and videos produced by users were sent and stored in remote and decentralized data centers. The user’s context vectors were extracted by BOFT (bag-of-features tagging) and converted into distributed video service servers. At last, the recommended video was transferred to multimedia applications in online social networks. The evaluation results on real datasets in Sina microblog and Youku, a video sharing site (VSS) in China, achieved sublinear regret bound and established a trade-off between the performance loss and the privacy protection level. However, for simplicity, a small dataset was chosen in those social networks, so it suffered from low scalability. In another study, Feng et al. ( 2018 ) proposed a Content-Centric Networking (CCN) architecture based on the Monte Carlo Tree Search (MCTS) algorithm. Since the volume and variety of both users and contents are rapidly growing, the MCTS algorithm solved the accurate content push problem in big data. Their algorithm outperformed in the experimental results of push accuracy, scalability, and robustness of users’ arrivals in Sina Weibo on an offline dataset. Although the proposed architecture could evaluate the performance in a real-world CCN-based social media, energy efficiency was neglected.
Sahoo and Gupta ( 2020 ) proposed a framework to distinguish fake profiles on Facebook. The authors applied various ML algorithms along with content analysis and account-based features to detect suspicious accounts from genuine ones. The evaluation results indicated that the presented framework gave the best outcome in terms of accuracy, precision, recall, F-measure, and Matthews’s correlation coefficient, but they did not evaluate the responding time of the presented approach. Moreover, applying this approach on other platforms such as Twitter and Google + or adding an aggregator module for comparing various account features and their activities may lead to different results. Since various microblogs contain videos, emoticons, and pictures as well as texts, Zhang et al. ( 2019 ) proposed a multi-modal emotion analyzer based on deep learning. The authors applied a two-way Long and Short Term Memory network (LSTM) model to integrate contents and user’s features. The offered model attained a higher accuracy, precision, and F-measure compared to previous models, but users’ personalities were not considered and in the proposed model, user-based emotions could not be classified.
4.1.2. Overview of the opinion/sentiment learning approaches
In this section, the selected papers with opinion/sentiment learning approaches are reviewed. Opinion/sentiment learning approaches entail Natural Language Processing (NLP) to extract opinions from the text and classify the polarity of subjects into positive, negative, or neutral to determine what they are talking about and to identify the public group perception. With the help of sentiment analysis, opinions about products, services, brands, politics, or any topic that people care about are extracted. These data can be used in many applications like marketing analysis, product reviews and feedback, emotion detection, intent analysis, customer support and services, social media monitoring, and brand monitoring ( Shirdastian et al., 2019 ).
By reviewing papers relevant to opinion/sentiment learning, we recognized three methods, namely lexicon-based, learning-based, and hybrid approaches, employed to extract and analyse opinion/sentiment in social media contents. In lexicon-based approaches, a set of predefined lexical wordlist, corpus, and dictionaries are used to extract subjectivity, the orientation, and the polarity of opinions and sentiments. Learning-based approaches utilize various ML algorithms (supervised or unsupervised) to classify text into positive or negative classes. Moreover, some of the reviewed papers combine both learning-based and lexicon-based approaches that mentioned hybrid approaches. Table 5 depicts a comparison of the selected papers with opinion/sentiment learning approaches. It includes main ideas, advantages, disadvantages, evaluation methods, tools, and case studies along with their categories. In some studies, the applied tools for analyzing and implementing approaches have not been mentioned. Table 6 shows the parameters used by papers relevant to opinion/sentiment learning approaches to evaluate the intended methods.
Reviewing and comparing papers with opinion/sentiment learning approaches.
An overview of the evaluation parameters in papers with opinion/sentiment learning approaches.
Kauffmann et al. ( 2019 ) offered a modular framework for qualitative interpretation of UGC by employing NLP techniques and applying cosine similarity measures to recognize fake reviews. Their Fake Review Detections Framework (FRDF) utilized NLP techniques to discover similarities between reviews and eliminate fake and unreliable reviews of a product. The major weakness was that FRDF set a threshold in the cosine similarity measure to detect fake reviews; other thresholds or other sentiment analysis tools, except for lexicon Afinn, may produce different outcomes. Furthermore, Jiang et al. ( 2017 ) suggested a method for performing sentiment computing of the news event in social big data. First, a Word Emotion Association Network (WEAN) was constructed to compute both word and text emotions at a specific time. After dividing emotions, a questionnaire was designed to collect ideas about the six-dimensional sentiment emotion of emoticons, and emoticons were used to calculate the emotions of each sentence. Second, based on WEAN, a word emotion computation algorithm was presented to get the primary word emotions. Then an emotional refinement algorithm was offered by employing the standard emotional thesaurus to improve the sentiments of news with high accuracy, but emotion distance and word emotion patterns were not considered into text sentiment computations.
Moreover, Dalla Valle and Kenett ( 2018 ) presented a new approach to integrate online review data with customer survey data. The sentiments of online users were calibrated with customer surveys by resampling and merging data via Bayesian networks in their method. This approach was used in various areas, and the data integration between online blogs and customer satisfaction led to enhancement in sentiment analysis. However, it did not consider methods for integrating vast data sources to enhance the accuracy of results. In addition, Jimenez-Marquez et al. ( 2019 ) presented a two-stage framework to analyse UGC in social media. The first stage, which aimed at managing big data and processing UGC, built a Machine Learning Model (MLM). The second stage, which took MLM of stage one, involved a series of layers to build a big data architecture that analysed unstructured and heterogeneous data. The proposed framework was superior to its competitors in both quantitative and qualitative analysis. Despite high accuracy, better results may be obtained by applying the integration of advanced ML algorithms on different domains.
Despite the advancement and development of medical science, COVID-19 is the most perilous disease of the 21st century around the world, which is a critical threat to the physical and mental health of individuals. In this respect, Zhu et al. ( 2020 ) analysed the topics about COVID-19 in Weibo from January 24 to February 25, 2020. The authors tried to grasp the opinions of users about the epidemic from a temporal and spatial perspective in China. However, the study had some drawbacks. The spatial perspective of opinion analysis was limited to a provincial region. The age and gender of Weibo users were not considered, so they were not reflected in the analysis results. Moreover, since some users did not apply Sina Weibo to express their opinions, the result cannot be generalized. Thus, employing a high volume of data may lead to more predictive and accurate opinion analysis for relevant organizations in emergency conditions.
Fan et al. ( 2020 ) introduced a novel method for exploring real-time sentiments, team identification, and national identification of tweets during the 2018 FIFA world cup. The authors observed how the sentiments of fans’ tweets in two matches (England vs. Croatia and England vs. Colombia) fluctuated during the match. They applied python and ensemble methods not only to design a model with high accuracy for sentiment analysis at different temporal points during the match, but also to analyse emojis as well as their valence. However, since 4% of the collected tweets were in Spanish and Croatian and the ML approaches cannot perform properly on Multilingual datasets, their method had low reliability. Moreover, they only analysed two English competitions, other international matches or other countries were not considered. Finally, all ML techniques did not have the ability to analyse the available sarcasm in tweets, so the results attained a low level of precision, recall, and F-measure.
Shirdastian et al. ( 2019 ) presented a framework to explore brand validity and their sentiment polarity both qualitatively and quantitatively. The authors explored opinion and sentiment polarity towards brand validity on Twitter dataset in terms of uniqueness, heritage, quality commitment, and symbolism. The study results indicate the enhancement of the proposed framework in precision and accuracy to find out the brand authenticity by exploring the related brand sentiments. The main drawback of this study was that neither was the variation of sentiments over time explored, and nor was the sentiment mining of bot-created brands excluded. Sayed et al. ( 2020 ) presented a hybrid approach that applied a combination of ML and lexicon techniques for sentiment analysis of tweets. The authors suggested a new metaheuristic approach based on Particle Swarm Optimization (PSO) and K-means to optimize data clustering. They evaluated their approach on four Twitter datasets with various topics employing spark streaming, leading to better accuracy in real-time analytics compared to previous approaches, but deep learning methods probably may lead to more accurate predictions.
To examine the relationship between volatility in the stock markets and UGCs, van Dieijen et al. ( 2020 ) presented a framework through the use of multivariate regression analysis and Generalized Autoregressive Conditional Heteroscedasticity (GARCH) model. The results showed the asymmetric impact of UGC on volatility, which means negative comments, compared to positive ones, increased volatility and had a significant effect on customers. For future research, scaling up may lead to practical implementation. Spruce et al. ( 2020 ) presented a new methodology for exploring the impact of social sensing and social data sentiment analysis of real-world events on named storms in the United Kingdom and Ireland. The authors collected tweets posted in winters 2017 and 2018. Then time zone, bot, and weather-related filters were applied to extract data related to weather incidents. By analyzing the sentiments of tweets during extreme climate events, the effects of weather incidents and their social impacts in terms of physical, emotional, spatial, and temporal perspectives were revealed and enhanced. The main limitation of this study was low scalability due to the small number of tweets retained in filtering weather-related tweets after the collecting phase. Further, the results were somewhat unreliable due to applying the python’s sentiment analysis package (TextBlob) which has a training corpus based on movie review datasets.
Um et al. ( 2013 ) introduced a distributed and parallel parsing system based on MapReduce to analyse users’ sentences in social sensor networks. To conduct the study, a Stanford parser with loose coupling was applied, which led to high scalability. Due to the parallel environment, the parsing time was low, the proposed system had high precision and high portability. The main limitation was that the actual data of social sensor networks like Twitter was not considered, and technical sentences were not analysed in the same way as ordinary users’ phrases were. Moreover, researchers in ( Baltas et al., 2016 , Lee and Paik, 2017 , Moise, 2016 ) employed ML along with NLP for opinion and polarity mining of social big data in sentiment analysis that were applied for various decision-making purposes including marketing or health care issues like reporting drug side effects. In order to conduct sentiment analysis on a microblog big data platform, Sun et al. ( 2018 ) presented a model called Convolutional Neural Network-Long-Short Term Memory (CNN-LSTM). Each type of emotion was modeled through a Single Gaussian Model (SGM). The authors used CNN for extracting local attributes and LSTM as a global attribute extractor. The findings indicated that the sentiment of social language performed through CNN-LSTM model achieved high accuracy, but time was neglected in their model, and threshold selection was still taking too much time.
Also, BalaAnand et al. ( 2019 ) presented a mechanism to collect contents from social media by utilizing big sheets, big vision schemes, and sentiment assessment. In addition to Deep Learning Modified Neural Network (DMNN), which was used to investigate sentiments, the Modified Threshold-based Cuckoo Search Algorithm (MTCSA) was applied as a heuristic search algorithm for weight optimization. The experimental results revealed that the proposed Deep MNN outperformed in terms of reliability, robustness, scalability, accuracy, precision, recall, F-measure, and computational time in comparison with other algorithms, but the cost of the proposed method was not assessed. For topic classification and sentiment analysis of social big data, Rodrigues and Chiplunkar ( 2019 ) presented a distributed Hadoop framework. Additionally, the Bag-of-words method was used to classify the relevant tweets into six different groups. Then four various NLP methods, namely Lexicon uni-gram, bi-gram Lexicon, uni-gram NB, bi-gram NB, and Hybrid Lexicon-Naive Bayesian Classifier (HL-NBC), were employed. HL-NBC was more effective and outperformed other classifiers in terms of accuracy, execution, and response time. However, separating and classifying sarcastic sentences and cross-lingual opinions for sentiment analysis were still unsolved challenges.
4.2. Network-oriented approaches
Network-oriented approaches analyse big social data based on nodes or entities and their relations within social networks. Network-oriented approaches are classified into two groups: Embedding learning and community learning. We review the selected papers with embedding learning and community learning approaches in 4.2.1 , 4.2.2 , respectively. In 4.2.1 , 4.2.2 , the classification of techniques, the definition of methods, and the related papers are discussed.
4.2.1. Overview of the embedding learning approaches
Some of the reviewed papers presented embedding learning that focused on extracting valuable information about users and nodes inside a network for link prediction, influence analysis, and information diffusion in social networks. Social influence means an individual’s ability to influence another user in a network; the more influential a person is, the more followers he will have ( Kumaran and Chitrakala, 2017 ). The embedding learning approach aims to analyse a network based on users and their features and model the process of information diffusion on online social networks through learning user’s characteristics and dissemination of information among users. Embedding learning approaches try to find the influence of different nodes in a network by identifying the position of a node in a path or a number of paths in which it occurs; the node that is most often in the center of a network and has more paths is more influential.
In the aspect of predicting the underlying diffusion process, three categories are distinguished in embedding learning approaches: Graph-based, non-graph based, and explanatory. Graph-based and non-graph based are kinds of predictive models in which, by investigating the previous information propagation, the information dissemination is predicted from spatial or/and temporal points of view. Graph-based approaches focus on the static and graphical structure of a network in which information is transmitted and predicts who influences whom. In this approach, each node can be activated or deactivated, such as Independent Cascades (IC) and Linear Threshold (LT), while in non-graph based approaches, the topology and structure of a network are not taken into account and each node is randomly connected to other nodes in the network with an equal probability such as epidemic models, Linear Influence Model (LIM) and Partial Differential Equations (PDEs). The main goal of explanatory models is to infer the information propagation path and to show how the information is propagated in social networks. Propagation characteristics such as pairwise transmission rate, pairwise transmission probability, and cascade properties are explored in this model whereas the network in which information diffusion takes place is unknown.
This section presents the selected papers with embedding learning approaches. In addition, the selected papers that use this approach in social big data analysis are reviewed. Finally, they are compared and summarized in Table 7 , Table 8 . Table 7 compares them in terms of main ideas, advantages, disadvantages, evaluation methods, tools, and case studies along with their categories. In some studies, the applied tools for analyzing and implementing the intended approach were not mentioned. The evaluation parameters are also specified in Table 8 .
Reviewing and comparing papers with embedding learning approaches.
An overview of the evaluation parameters in papers with embedding learning approaches.
Kumaran and Chitrakala ( 2017 ) offered a social influence method based on rank-sampling approach. After collecting Twitter’s data, parallel information diffusion modelling, which took the users’ queries as input, determined forwarding nodes and calculated the path of information flow. The next portion was influential spreader ranking, which took a search query and applied topological and users’ attributes to calculate users’ feature scores. At last, two solutions were provided for an influence maximization problem. Ranking-based sampling, MapReduce, and parallel processing were applied to ensure accuracy and time reduction, respectively. Despite scalability, the sample size was considered fixed, so an approach that could define the most appropriate sample size was needed to be performed.
In another research, Persico et al. ( 2018 ) analysed the efficiency of two big data architectures, namely Lambda and Kappa. Although the size of the dataset affects the performance, both architectures provided good scalability, but in case of increasing input size, Lambda had higher performance than Kappa due to its in-memory computation. Findings indicated that the deployment for Kappa with the same number of executors was more expensive than Lambda. Besides, in both architectures, the performance was improved when the algorithm was executed on more massive clusters. In case of virtual machines (VMs) characteristic enhancement (or with resource-richer nodes), Kappa significantly improved the performance (vertical scaling). In general, reports showed that Lambda performed better, and both architectures supported social network applications properly. To predict information diffusion in the content of social big data, Gao et al. ( 2017 ) offered an efficient Information-dependent Embedding Based Diffusion Prediction (IEDP) model. They also extended a typical margin-based optimization algorithm and presented an efficient learning algorithm based on Stochastic Gradient Descent (SGD). The complexity of the proposed model was significantly reduced, but the social structure was not considered in their proposed embedding model.
Additionally, for illness control and prediction in advance, Elkin et al. ( 2017 ) introduced a network-based approach for modeling illness activity and generated predictions about ILI based (Influenza-Like Illnesses) across geographical locations. This prediction model could help with illness control and provided predictions for one week in advance. Meanwhile, it was unsuccessful with airline traffic data in predicting ILI activity across geographies and had a low level of scalability, and except for geographical locations, other factors such as weather patterns or low population density were not considered. By discovering more factors, the model could have been stronger. Moreover, a heuristic model called PRDiscount was proposed in ( Wang et al., 2014 ) to select the first seeds for maximizing the influence diffusion in social networks. On the contrary, Talukder and Hong ( 2019 ) introduced a heuristic mixed approach to minimize and optimize viral marketing costs in social media.
Since nowadays social networks have a great impact on the dissemination of information and users’ comments and on individuals’ daily lives, Chen et al. ( 2020 ) suggested a topic-aware influence maximization model based on cloud computing. They employed a sketching technique along with a greedy algorithm to discover the optimal top-k seed users that maximize the influence of information being spread within a network. Compared with available influence maximization approaches, the proposed approach achieved low running time and low storage, but a limited number of evaluation parameters were applied to verify the accuracy of the model.
Moreover, to discover the influential users, Wu et al. ( 2020 ) offered a Protection and Recovery Strategy model (PRS) to study the propagation of the virus in social networks. In the proposed mechanism, the users were divided into five groups based on their reactions to the virus: Susceptible, Contagious, Doubt, Immune, and Recoverable (SCDIR). The PRS model made it possible to control viruses and to reduce infected users. Despite the low running time and low cost of the model, a fixed number of nodes and connections were assumed; the dynamic changes in a number of nodes and their connections may lead to different results. Wu et al. ( 2018 ) suggested a model to search small data and to compute the effect of small data nodes to use them instead of big data. They believed that obtaining small data leads to a reduction in the complexity of big data. Results showed that 1% of small data could connect 15% of communication nodes, and 20% of small data could broadcast 80% of data packets, so the other nodes were in waiting status. Although complexity was decreased and the delivery ratio was improved, a new algorithm was needed to establish a trade-off between reliability, delivery ratio, delay, and the use of limited network resources.
Wu et al. ( 2018 ) presented a developed model to recognize and restrict the process of rumor dissemination among users by considering all the users’ behaviors. A time threshold was dedicated to each user to indicate the delays in users’ reactions. The authors suggested a mobile node to propagate authorized information to decrease the penetration of rumors. They simulated the proposed model on the Facebook dataset to investigate the influence of speed, arrival time, and strategies of the mobile node on rumors. The speed and the strategy of mobile nodes could not reduce the spread time point of rumors earlier, but in general, it reduced the spread time of rumor; therefore, the best solution to detect rumors is to send mobile nodes to neighbor nodes with the highest degree.
Furthermore, to prevent the spread of malwares, Peng et al. ( 2017 ) presented a big data-based framework in which social interactions were transformed into a bidirectional weighted graph that displayed people’s daily SMSs/MMSs. Moreover, social influence, involving direct and indirect influence, was measured. Then a set of immunization algorithms were designed, and the Susceptible Infectious Recovery (SIR) model was developed because the top k influential nodes had more influence on the distribution of malware propagation. Thus, based on the presented immunization strategy, the top k influential nodes were minimized; meanwhile, it did not detect social media malware in real-time.
In order to improve the statistical and economic performance of credit scoring applications both, Óskarsdóttir et al. ( 2019 ) employed personalized Page Rank (PR) and SPreading Activation (SPA) methods on Call-Detail Records (CDR), credit and debit account information. The results showed that the features of calling behavior were most effective, and the information extracted from CDR data in terms of “value” facilitated financial prediction. The major challenge was how to maintain privacy-preserving of customer’s data. Moreover, only one type of credit was analysed; other types of credits may lead to different results.
Furthermore, Raj and Babu ( 2015 ) proposed Firefly Inspired Algorithm for Establishing Connections (FIAEC) and mathematical models for computing the probability of staying in social networks. The goal of this algorithm was to maximize the number of connections concerning n individual in social network sites. By using the proposed algorithm, the number of connections was increased, and so did the interaction between connections. On the other hand, FIAEC was not scalable, and it was only tested for a sample size of 10,200 and 600.
Su et al. ( 2016 ) studied the characteristics of mobile big data and presented a new framework to spread these data over content-centric Mobile Social Networks (MSNs). To resolve volume, variety, control, and manage mobile big data challenges, the framework was delivered over CCNs. Findings showed that a low value of weight coefficient for a data packet led to a low delay. As their proposed framework was based on static characteristics, it did not consider dynamic mobile social users and was tested on a limited number of users, so it was not scalable. The limited resource allocation, such as bandwidth and buffer space, was not considered, and security was not maintained for the data stored out of their own mobile devices. In addition, to recognize the influential users, Kumar et al. ( 2016 ) developed a methodology by applying the number of friends and followers of accounts. In another study, Zhang et al. ( 2017 ) analysed an offline device-to-device dataset in mobile social big data and pushed interesting contents to the most influential users.
Besides, Xu et al. ( 2015 ) investigated the impact of various sampling approaches on the distribution of tweets and measured retweets to identify the influence diffusion in social network analysis. Since a notable amount of data in social networks are related to people who declare their opinions and thoughts, Yang et al. ( 2020 ) offered a social big data analysis framework to diagnose depression efficiently. The authors applied a large Facebook dataset to evaluate the proposed framework by investigating the effect of both friendship influence and users’ intentions and interactions on users’ mental health. They evaluated the performance of the framework with a various subset of social and user-level features to indicate that the users' social interactions with their friends on social networks could show their mental states. Unlike other researchers, to analyse friendships’ influence, both indirect and direct neighbors of a user were investigated; however, the topics of users’ posts were not considered as well as various genders, age groups, and their depression risk level.
Additionally, in order to investigate the diffusion structure of networks, Maireder et al. ( 2017 ) presented two new social network measures, namely Audience Diversity Score (ADS) and Communication Connector Bridging Score (CCBS). ADS identified the diversity of a particular actor’s followers, and CCBS highlighted the account that bridge and diffuse information throughout the entire network. The results demonstrated that the network was not divided by a unique factor but by a set of influential ones, like language, geo-identity, and political trends. Despite the advancement in communication patterns, the contents and types of tweets broadcast across the network were not analysed. Moreover, ADS and CCBD measures were not combined to detect the two-factor interaction in the spread of information.
4.2.2. Overview of the community learning approaches
As we stated earlier, social networks comprise a set of vertices or nodes in which nodes stand for users and individuals, which are associated with one another through numerous edges that represent their relations and interactions ( Leung and Zhang, 2016 ). “Community” is referred to as groups of individuals who have similar interests, attitudes, or common characteristics ( Wu et al., 2018 ). From the social aspect, detecting groups of individuals in a network on structural and topological properties is known as community learning which is crucial for various perspectives in society such as business and recommendation systems. Thus, it leads to innovative approaches for identification of communities that can be carried out in micro (micro-communities) or macro (macro-communities) network structural features. In community detection, the assumption is that people in one community interact more with one another because of the similarity of interests among them compared with other communities, so the network is divided into various communities.
In community learning, after identifying clusters of nodes, the number of clusters is determined. A cluster is mapped into a community, then the probability distribution over interactions among users and also within and among clusters is estimated. Community learning approaches can be categorized into node-based or group-based approaches to recognize the communities. Node-based approaches are carried out based on the properties of network nodes. Since similar nodes belong to the same communities, node degree, node similarity, or node reachability are considered in this approach. While group-based approaches do not regard characteristics at the node-level and consider the characteristics and the connections of the whole group and network by recognizing balanced, robust, modular, dense, or hierarchical communities.
In this section, the selected papers with community learning approaches are reviewed. Table 9 depicts a comparison of the selected papers with community learning approaches. It includes the main ideas, advantages, disadvantages, evaluation methods, tools, and case studies along with their categories. Table 10 shows the parameters that these papers with community learning approaches have used to evaluate their methods.
Reviewing and comparing papers with community learning approaches.
An overview of the evaluation parameters in papers with community learning approaches.
Aksu et al. ( 2013 ) presented a multi K-core and multi-resolution solution for social network community detection. The authors offered a distributed and scalable algorithm that ran on Apache HBase to compute K-core subgraphs for both client and server-side. The experimental results on dynamic networks indicated that despite such advantages as robustness, parallel, and distributed processing, the proposed algorithm was very costly in case of inserting and deleting edges. Wu, et al. ( Wu et al., 2018 ) presented a hash-based approach along with graph mining to discover interactions and communities among users in social media in which a trade-off between efficiency and effectiveness of incremental and time slices-based approaches was guaranteed.
Since the result of SNA helps managers in decision making for their markets, Dabas ( 2017 ) considered an electronic store with 98 employees, who were responsible for selling, maintaining, and installing mobile phones, tablets, and so on. For experiments, Pajek and different metrics of SNA like degree centrality, betweenness centrality, stress centrality, Power Centrality (PC), Information Centrality (IC), reachability matrix, and clustering coefficient were used. The social analysis informed executive managers of customers’ reactions in real-time to respond quickly if necessary, but it suffered from inadequate security of sensitive and personal data. While Yousfi et al. ( 2016 ) proposed a solution to construct the graph of social big data to enhance the semantic extraction by graph analysis.
As finding the right researcher with the best experience and knowledge is time-consuming and critical in research communities, Sun et al. ( 2015 ) presented an expert recommendation method based on topic relevance, expert quality, and researcher connectivity for experts in scientific communities. The architecture of this expert finder system contained three phases (profiling, modeling, and ranking). Large-scale computation task was supported as well as linear speed up and high accuracy. In their method, except for AHP in the ranking phase, the authors did not use other techniques as the rank aggregation model. In another study, to enhance the quality of vehicle localization in vehicular networks, Lin et al. ( 2016 ) proposed an Overlapping and Hierarchical Social Clustering (OHSC) model. The OHSC model explored the social relations between vehicles, and then classified the vehicles into different social clusters. As a result of OHSC, a Social based Localization Algorithm (SBL) was presented to support the global localization through vehicle location prediction even without the GPS devices. Although SBL had a high overall performance in the vehicle localization, the SBL algorithm had low stability and the worst performance in location error.
By increasing active users and daily tweets, users are faced with a severe problem of overloading information. To overcome ranking and recommending challenge, most micro-blogging services organize tweets in a timely order that place newer tweets at the top, but all these tweets may not be attractive to users. Kuang et al. ( 2016 ) proposed a new tweet ranking model considered three main aspects, consisting of the popularity of a tweet itself, the intimacy between the user and the tweet publisher, and the user’s interest areas. This ranking model improved tweet ranking performance; however, more indicators for ranking in analysing users’ behaviors were not considered. In order to identify all hidden communities in social media networks, Jin et al. ( 2015 ) designed a framework for community structure mining in which network partitioning process was avoided, and map equation process ran directly on MapReduce in the new framework. Instead of PageRank, the authors employed local information of nodes and their neighbors for calculating the distribution probability related to each node. The framework outperformed the previous algorithms, such as Radetal and FastGN, in accuracy, velocity, and scalability. However, the greedy search method that was applied to find an appropriate node for combining had some limitations that needed to be improved.
Additionally, Li et al. ( 2016 ) offered a distributed algorithm for data centers to handle social data to ensure privacy and guarantee the prediction accuracy improvement in real-time. Further, Paik et al. ( 2017 ) presented an effective service discovery through the creation of a graph-based algorithm based on MapReduce and parallel programming. In ( Karimi et al., 2018 ), Twitter data were analysed, and the degree centrality was calculated to investigate deceiving information based on a parallel approach. Leung and Zhang ( 2016 ) offered a novel method to represent and manage social big data. They employed graph mining approaches in directed, bi-directed, undirected, and bipartite graphs for analyzing and mining social big data in distributed settings. In ( Sharma, 2018 ), researchers designed a framework to analyse real-time Twitter hashtags by employing hashtag co-occurrence graph and connected components algorithm. Moreover, Du ( 2018 ) developed a high-frequency pair trading algorithm to perform semantic analysis on a weighted undirected graph by employing SNA approaches along calculating centrality parameters in a stock market.
Since similar nodes are usually placed in the same cluster, in ( Wang et al., 2017 ), a U-model was introduced for directed and undirected graphs based on similarity, which could define social big data characteristics, clustering coefficient, degree, and distance distribution accurately. In order to analyse the conversation in a social network, Ghosh et al. ( 2016 ) offered a new algorithm utilizing fuzzy methodology and density-based clustering on social clouds. This study was applied to examine the rate of users’ participations to find the popularity of the subject under discussion. Besides, this algorithm could have been developed towards more heuristic-based graph mining and put a benchmark towards heuristic optimization. Further, to represent the structures of network communities, Wang et al. ( 2017 ) digitally analysed Twitter’s data about diverse actors involved in entrepreneurial networks by applying the Clauset-Newman-Moore algorithm. The counties that were in the same cluster had stronger internal interactions than those in different clusters, but this research did not analyse entrepreneurial networks on Twitter data and in case of lacking the participation of users in low population regions of the country.
5. Analysis of results
The results of this systematic review are analysed in this section. Section 5.1 presents an overview of the selected papers. Since the goal of this review is to highlight the differences, advantages, and disadvantages of various big data analytic approaches in social networks, a discussion of the mentioned classification is outlined in Section 5.2 .
5.1. Overview of the selected studies
The following complementary questions are defined to explore the state-of-the-art on big data analytic approaches applied in social networks.
- • Which publishers have published most papers on big data analytic approaches applied in social networks?
- • How was the distribution of publishers and studies per year on big data analytic approaches applied in social networks?
- • How was the distribution of studies per publication channel on big data analytic approaches applied in social networks?
In this section, the distribution of 74 papers reviewed in Section 4 —categorized by publishers, the year of publication, the number of papers by year, and the percentage of papers classified by publishers—is shown in Fig. 5 , Fig. 6 , Fig. 7 , respectively. Fig. 5 , which states the papers over time, indicates that ScienceDirect, and Inderscience, have published papers in this field since 2015. IEEE, Springer, and ScienceDirect have provided the highest number of papers in this area, respectively. Also, Emerald and Taylor&Francis have presented the least number of papers. Fig. 6 shows that most papers in this subject were published in 2017 and 2019. Fig. 7 illustrates the classification of papers among nine publishers, out of which IEEE and Springer have provided 37% and 27% of the papers, respectively. 19% of the total papers were related to ScienceDirect, while, ACM, Inderscience, and SAGE publishers had 4% of the papers each. Also, 3% of the papers were published by Wiley. Additionally, Taylor&Francis, and Emerald, had 1% of the reviewed papers each.

The number of the studied papers categorized by publishers and years.

The number of the studied papers by years.

Percentage of the studied papers categorized by the publishers.
In Table 11 , we demonstrate the distribution of publication channel that published more than one paper among 74 studied papers. Table 11 depicts that 23 papers were published in IEEE Access (IF = 3.745), TMM (IF = 5.452), IJIM (IF = 8.210), IMMGT (IF = 4.695), FGCS (IF = 6.125), MTAP (IF = 2.313), WPC (IF = 1.061), I4C, and IEEE Big Data.
Distribution of the studies per publication channel.
5.2. Research objectives, approaches, and evaluation parameters
The reviewed studies were studied and classified according to various characteristics to answer some of the research questions listed in Section 3.1 , as explained below:
Big data analysis has many applications in social networks and is performed in various ways. As it was stated earlier, selected papers were reviewed, and big data analytic approaches in social networks were described in two main categories based on their analysis method: Content-oriented approaches, and network-oriented approaches. In content-oriented approaches, user-generated posts are analysed with the aid of lexical codes, linguistic codes, and statistical tools. Meanwhile, network-oriented approaches considered nodes or users and their relations for big social analysis. Also, the interaction between social group members and the relationship between group members and people outside the group are discovered. We categorized content-oriented approaches into two groups, topical learning and opinion/sentiment learning, and network-oriented approaches into two groups: Embedding learning and community learning.
Fig. 8 represents the percentage of social big data analytic techniques in reviewed papers based on Fig. 4 . Fig. 8 shows that the content-oriented approaches have the highest percentage (51%) in which topical learning and opinion/sentiment learning comprise 27% and 24% of the studied papers in the literature, respectively. Further, 49% of the papers are network-oriented approaches out of which 26% and 23% of the papers are related to embedding learning and community learning, respectively. The main properties of the selected papers reviewed were shown in Table 3 , Table 5 , Table 7 , Table 9 . The selected papers were evaluated based on critical parameters such as accuracy, scalability, precision, recall, F-measure, cost, and time. The advantages and disadvantages of the discussed taxonomy are summarized in Table 12 based on Table 3 , Table 5 , Table 7 , Table 9 . As specified in Table 12 , the main focus of researchers in content-oriented approaches are on some parameters such as accuracy, precision, recall, and time. This table also illustrates that accuracy and scalability are enhanced in network-oriented approaches, but privacy and security are not considered by most researchers. Moreover, findings have shown that since manipulating community-based features is challenging and not user-controlled, and extracting these features requires an in-depth analysis of a large and complex social community, which has high complexity and requires plenty of resources, community learning approaches have high costs. Besides, according to Table 12 , security and privacy-preserving are still the main drawbacks of community learning approaches.

Percentage of social big data analytic techniques in the selected papers.
A summarization of the advantages and disadvantages of the discussed taxonomy.
In this study, reviewed papers have been evaluated by various evaluation parameters, which were presented in Table 4 , Table 6 , Table 8 , Table 10 . Fig. 9 , illustrates the parameters used by researchers to evaluate the techniques and methods applied in reviewed papers. The results of the provided comparison in Fig. 9 show that 20% of the studies have enhanced accuracy, 16% of them have reduced time, and 12% of the studies have assessed scalability. Recall, precision, F-measure, and cost were also important among parameters. Based on the mentioned parameters, the percentage of each parameter was computed using (1) ( Hamzei and Navimipour, 2018 ). This equation means that the number of each occurrence was counted and divided by the sum of the whole number of occurrences, then the answer was multiplied by 100 (Eq. (1) ).

Percentage of evaluation parameters in the selected papers.
Fig. 10 indicates that in topical learning approaches, researchers focused on accuracy (23%) and recall (15%), while in opinion/sentiment learning approaches, accuracy (31%) and F-measure (18%) are the crucial ones. The significant parameters in embedding learning approaches were time and cost by 23% and 16%, respectively. To say more, 20% of the papers with community learning approaches have optimized scalability and 18% of them have reduced time, so the results showed that accuracy is essential in most approaches; however, privacy, reliability, and security are somewhat neglected in these approaches.

Percentage of evaluation parameters in each approach of the selected papers.
Some of the papers did not mention any tools for analyzing and implementing the intended approaches. According to tool columns in Table 3 , Table 5 , Table 7 , Table 9 , along with python programming language, Hadoop was the top used tool in 74 research studies of social network analysis. The high frequent application of Hadoop is due to its open-source libraries for distributed and parallel processing of large datasets, cost-effective, big storage, reliability, scalability, and handling unstructured and semi-structured data.
Fig. 11 demonstrates the social big data analysis applications of the reviewed papers, along with their percentage of repetitions. The results showed that, in the reviewed papers, the business and decision making, and parsing and sentiment analysis platform had the highest applications with 19% each. Along with these two applications, health care (15%) was a significant application of big social data analysis in studied papers.

Percentage of social big data analysis applications in the studied papers.
Selected studies have used various datasets to evaluate their approaches for analyzing the results of experiments. Based on the findings shown in Fig. 12 , most of the researchers used Twitter. In addition to Twitter, the most significant percentage of the usage of datasets belongs to Sina microblog and Facebook.

Repetition of used datasets and case studies in the selected papers.
Based on Table 3 , Table 5 , Table 7 , Table 9 , which have depicted the evaluation methods applied in each approach, there were five evaluation methods in the reviewed papers: Simulation, prototype, data sets, real testbed, and example application. As shown in Fig. 13 , 42% of assessments were related to data sets, while 35% of them were associated with real testbed. Lucidly, simulation dedicated 19% in itself. Fig. 14 , displays the repetition of evaluation methods in each learning approach. The comparative results illustrate that in topical and opinion/sentiment learning, most evaluation methods are data sets. ML algorithms and data sets were widely used in semantic analysis and incorporated many ideas and innovations into social networks, welcoming virtual world users and social network growth; however, in community learning approaches, the real testbed has the highest usage in most evaluations. Finally, real testbed and simulation cover most of the evaluations for embedding learning approaches.

Percentage of evaluation methods in the selected papers.

Repetition of evaluation methods in each approach in the selected papers.
6. Open issues and future directions
Given the vast quantity of live social media streams and their impact on society, many techniques have been proposed to collect and analyse live UGC to support various applications. The techniques studied in this paper assist us in gaining insights into social data via big data analytics. The presented systematic literature is a good starting point to reveal open challenges. However, content-oriented and network-oriented approaches still face many vital challenges as mentioned below:
- • The extensive usage of social media has resulted in the advancement of many disciplines and industries in which healthcare is one vertical application that has attracted much attention. Fig. 11 demonstrates an increasing tendency towards healthcare systems along with other domains. Patients join different social media groups, sharing experiences, describing their illness, and the treatment process. Social platforms provide patients with emotional supports from peers with similar conditions. The first-hand experiences and comments from other members in the network are invaluable sources for making informed decisions, especially for those with chronic conditions ( Akbari et al., 2019 , Akbari et al., 2018 ). Further, healthcare professionals also utilize social media to share healthcare, psychology, and medical information and to interact with their peers as well as patients ( Nie et al., 2014 ).
In this respect, public care organizations can start-up social health networks for diagnosing and preventing the spread of contagious disease in various geographical locations at different times by exploring public health posts in various social networks ( Elkin et al., 2017 ). On the other hand, by analyzing the graph of interactions between users on social networks and examining influential users, nodes with multiple edges have been identified, so by limiting and quarantining them, the transmission rate of contagious disease can be forecasted, which allows us in better decision making to control infectious ailments. This would ultimately lead to a notable reduction in healthcare costs ( Zadeh et al., 2019 ).
They can also track the origin of diseases, the transmission of diseases from generation to generation, the effects of drugs, and their interactions in different diseases ( Thorstad and Wolff, 2019 ). This helps the pharmaceutical industry as well as healthcare promotion and health disorder diagnosis. One of the limitations of the current work in this area is that the nodes and their relations were considered static over time. Considering and analyzing the network in real-time and the dynamic interaction among nodes are still open issues that can achieve more accurate predictions. Most researchers also have studied social influence and information diffusion in a particular platform; analyzing information diffusion and social influence across multiple platforms simultaneously can also be a challenge in the future. However, among the reviewed literature, there were few papers on political and e-commerce applications, so these two issues are good topics for future research.
- • In case of a vast number of data sources, another challenge is enhancing accuracy to improve services and predictions in various social network applications. For example, in social networking services, users frequently publish about themselves via status updates, photos, videos, self-description, and interests. Some of the recommendation and prediction systems predict the users’ personalities by considering the users’ profile data. On the other hand, some people keep some of their personal information private, or some users deliberately create fake accounts or fake information such as birth date, location, occupation, and status to increase the number of followers or get more likes; the available data may be fake or cannot be achieved due to privacy concerns, so the result of prediction is not accurate. Further, user profiling would be an essential aspect of social networking services to attest accurate prediction and recommendation ( Akbari and Chua, 2017 , Akbari et al., 2017 ).
- • The ever-increasing volume of social media data has led to the distribution of files in various physical locations. A key future direction is to investigate factors such as network traffic, data locality, latency, high-level runtime of feature extraction, and clustering users . Despite the fact that enhancing the speed of feature extraction has been considered by a limited number of papers ( Hsu et al., 2017 ), other challenges are still unsolved.
- • Conspicuously, due to the high volume of data and the rapid growth of contents produced in social platforms, scalability is still a key factor to determine the effectiveness of social network analysis frameworks. The scalability issue includes handling an immense number of users, updating users’ profiles and status, internal network traffic, as well as data storage and database management, so the expanding infrastructure, infrastructure management, and operational costs can affect the scalability challenge. Although some papers have proposed algorithms or methods to increase scalability in their approaches ( Sachar and Khullar, 2017 , Feng et al., 2018 , Aa et al., 2015 ), others implement their approaches on small scale datasets; hence, it is still a significant challenge.
- • The Internet has increased the growth of social networks to connect people and make it easier for them to find friends and share multimedia information, such as photos, videos, which are considered big data in social networks. With the increasing likelihood of cyber-attacks or malicious users, there is a risk of personal data being misused. A limited number of studies made efforts to solve this issue ( Zhou et al., 2016 ); hence, offering novel approaches to ensure the privacy-preserving of social network users to secure photos, videos, sensitive personal data, and profiles, without crippling the utility of social media data, is a crucial challenge for future research.
- • Due to the streaming nature of social data, both collecting and analyzing real-time data from various sources can assist the organization of customers’ tweets, blog posts, and status updates. It allows organizations to track and answer customers’ updates and comments as soon as possible. Some papers debated this challenge ( Sayed et al., 2020 , Lee and Paik, 2017 , Rodrigues and Chiplunkar, 2019 ), but, unlike Twitter Streaming API, Facebook’s graph API does not provide any real-time streaming access. The analytic approach should be able to investigate social media platforms in real-time, which leads to real-time results, so the real-time nature of social data is still an appealing challenge.
- • Predictive analytics is another interesting direction that still remains as an open and challenging task. The key challenges which were focused on in ( Yang et al., 2015 ) are aggregating data, extracting high dimensionality features, and building a model that can predict future events. Considerable amounts of data that are produced by users in social networks represent the views, suggestions, and thoughts of users in the form of texts, images, and videos, which may be high-quality or low-quality. As these data come from grass roots users with informal and unconstructed formats, social data are popular as noisy sources of information. Low quality, out of date, or incorrect data can lead to wrong or inaccurate analytics results; therefore, in addition to extracting high-quality information from a variety of sources, it is also essential to prevent the flow of misinformation. Although ML and data mining permit us to reduce the impact of low-quality data, it still cannot assure the proper quality of data. Besides, the modeling process should be repeatable to ensure and extract meaningful relationships among data. Without a useful model, the predictive system cannot produce satisfactory results; therefore, data quality and modeling are two engrossing directions for future works in predictive analysis.
- • From the sentiment analysis aspect, the following key challenges are still open to be addressed:
- o Domain dependency : As sentiment analysis is a domain-dependent task in which the polarity of some words and phrases vary from one domain to the other; thus, a classifier trained for a specific domain may fail to perform well on other domains.
- o The rare-resource languages : Most of the resources of sentiment analysis are only built for English language. There is no sufficient corpus for such languages as Chines, French, Hindi, Spanish, and so on. The bottleneck of performing opinion/sentiment analysis is the scarcity of predefined dictionaries and tools for various languages.
- o Detecting sarcasm : Since sentiment analysis classifies texts as positive, negative, or neutral, another challenging issue in sentiment analysis is detecting sarcasm. It refers to sentences that have negative meanings despite the use of positive sentiment-bearing words. In other words, the meaning is just the opposite. It is a challenging task for a system to identify sarcastic sentences. Researchers should allocate their attention to find innovative approaches to analyse sarcasm in the sentiment of social big data analysis.
- o Detecting slang : Most of the people use slang to express their feelings and, as slang words contain extreme sentiments, detecting slang words is a serious problem.
- o Heterogeneous nature of data : The sentiment classifiers should work effectively and handle the diverse types of data from various data sources.
- o Unreliable and incomplete data : Users usually use abbreviations in a social network. Social network data may contain a lot of noise and misspellings; the sentiment classification of these data is not accurate; therefore, sentiment classifiers should be able to predict incomplete information to have a more accurate prediction.
- o Semantic relations in multiple data sources : Different social networks such as Twitter, Facebook, Instagram, and YouTube may discuss the same topic. Researchers in studied papers, investigate data only on a single social media, so the analysis of an event from various social media is a challenge that can offer better insights for the task of sentiment analysis and its model creation.
- o Subjectivity detection : Regarding the personality of a user or his political views, a text may be neutral to one person, but not for the other, so a sentence may have a different interpretation.
- o Spam detection : Spammers or fake users try to post fake reviews and to mislead other readers, so detecting these spams among posts is a significant challenge.
Many researchers try to mitigate a limited number of these challenges ( Sun et al., 2018 , Kauffmann et al., 2019 , Jimenez-Marquez et al., 2019 ), but they failed to achieve high accuracy, so most of these challenges in sentiment analysis have not yet been resolved, and further research is needed.
- • Finally, a few number of the studied papers did not test their approaches on real datasets of social networks. Unlike users’ typical sentences, specialized sentences were not technically analysed. Also, specific vocabulary is used for particular platforms, e.g., the use of slang terms, which makes analysis very specific to each platform; therefore, it is another research direction, and further studies may test various social networks and real datasets of social networks. More experiments can be performed to increase the performance of social big data analytic approaches in the future.
7. Threats to validity and limitations
This SLR presents a taxonomy and a comparison of big data analytics in social networks. These types of review papers usually have constraints ( Brereton et al., 2007 ), but the results of SLRs are mainly reliable ( Zhang and Babar, 2013 ). The major limitations and threats to the validity of this SLR are discussed below.
- • The scope of the research: In the paper selection process, only academic journals and conferences were considered. Furthermore, national conferences and journals, non-English papers, book chapters, and review papers were neglected.
- • Study and publication bias: These nine electronic publishers offer the most related and valid papers; some of them were neglected via the paper selection process; therefore, the selection of all related papers cannot be guaranteed.
- • Study queries: This paper is proposed according to seven questions, which were defined to find their answers. Other researchers may add some other questions.
- • Taxonomy: The reviewed papers were classified into two main categories based on analysis methods: Content-oriented and network-oriented approaches, but it can be categorized otherwise.
- • Simulation: The reviewed papers were not simulated.
- • Time range: Only papers from 2013 to August 2020 were reviewed, and those before 2013 were not considered.
As a matter of fact, by defining a review protocol, following a systematic procedure, and the involvement of various researchers, this SLR has high validity.
8. Conclusion
This paper presents a systematic review of big data analytics in social networks. We explained the research methodology, paper selection process, and selected 74 papers between 2013 and August 2020, from among 785 papers in our search query. A significant number of the studied papers were related to IEEE, Springer, and ScienceDirect journals, with 37%, 27%, and 19%, respectively. On the other hand, each of Taylor&Francis and Emerald publishers with 1% had the lowest number of published papers. From these studies, 74 papers were categorized into two approaches: Content-oriented approaches (51%) and network-oriented approaches (49%). Besides, the main ideas, advantages, disadvantages, evaluation methods, tools, and evaluation parameters of each studied paper were discussed. It was found that the most widely considered evaluation parameters were accuracy (20%), time (16%), and scalability (12%), but privacy, reliability, and security measures were somewhat neglected. Considering the applied tools, it is observed that, in the selected studies, along with Python programming language, Hadoop was used more than other tools. Concerning the outcome of this SLR, the existing social big data analytic approaches have inadequate capability to guarantee privacy-preserving and scalability and have faced several open issues such as latency, real-time processing, and high run-time of feature selection. Lucidly, the most unresolved challenges are various aspects of opinion/sentiment analysis such as domain dependency, the rare resource languages, detecting sarcasm and slangs, subjectivity detection, and multiple data sources. We hope that the findings of this paper will assist researchers to propose novel contributions to overcome social big data challenges.
Declaration of Competing Interest
The authors declare that they have no known competing financial interests or personal relationships that could have appeared to influence the work reported in this paper.
Acknowledgement
We are grateful for the insightful and constructive comments offered by Dr. Mohammad Akbari, and also appreciate anonymous reviewers for their precious comments which improved the final version.
1 https://scholar.google.com
2 https://link.springer.com
3 https://ieeeexplore.ieee.org
4 https://www.sciencedirect.com
5 https://online.sagepub.com
6 https://www.tandfonline.com
7 https://onlinelibrary.wiley.com
8 https://www.emeraldinsight.com
9 https://dl.acm.org
10 https://www.inderscienceonline.com
Appendix A. List of evaluation parameters and their description
- Arora A., Bansal S., Kandpal C., Aswani R., Dwivedi Y. Measuring social media influencer index-insights from facebook, Twitter and Instagram. J. Retail. Cons. Serv. 2019; 49 :86–101. [ Google Scholar ]
- Lai W.K., Chen Y.U., Wu T.-Y. Analysis and evaluation of random-based message propagation models on the social networks. Comput. Netw. 2020; 170 [ Google Scholar ]
- Alalwan A.A., Rana N.P., Dwivedi Y.K., Algharabat R. Social media in marketing: A review and analysis of the existing literature. Telematics Inform. 2017; 34 (7):1177–1190. [ Google Scholar ]
- R. Kumar, J. Novak, and A. Tomkins, Structure and evolution of online social networks. In Link mining: models, algorithms, and applications: Springer, 2010, pp. 337–357.
- Feng Y., Zhou P., Wu D., Hu Y. Accurate content push for content-centric social networks: A big data support online learning approach. IEEE Trans. Emerg. Top. Comput. Intell. 2018; 99 :1–13. [ Google Scholar ]
- Heidemann J., Klier M., Probst F. Online social networks: A survey of a global phenomenon. Comput. Netw. 2012; 56 (18):3866–3878. [ Google Scholar ]
- Busalim A.H. Understanding social commerce: A systematic literature review and directions for further research. Int. J. Inf. Manage. 2016; 36 (6):1075–1088. [ Google Scholar ]
- Bello-Orgaz G., Jung J.J., Camacho D. Social big data: Recent achievements and new challenges. Inf. Fusion. 2016; 28 :45–59. [ PMC free article ] [ PubMed ] [ Google Scholar ]
- M. Jamali and H. Abolhassani, Different aspects of social network analysis. In Web Intelligence, 2006. WI 2006. IEEE/WIC/ACM International Conference on, 2006, pp. 66–72: IEEE.
- Martinez-Rojas M., del Carmen Pardo-Ferreira M., Rubio-Romero J.C. Twitter as a tool for the management and analysis of emergency situations: A systematic literature review. Int. J. Inf. Manage. 2018; 43 :196–208. [ Google Scholar ]
- Cetto A., Klier M., Richter A., Zolitschka J.F. “Thanks for sharing”—Identifying users’ roles based on knowledge contribution in Enterprise Social Networks. Comput. Netw. 2018; 135 :275–288. [ Google Scholar ]
- Go E., You K.H. But not all social media are the same: Analyzing organizations’ social media usage patterns. Telematics Inform. 2016; 33 (1):176–186. [ Google Scholar ]
- [13] L. Manovich, Trending: The promises and the challenges of big social data. In Debates in the digital humanities, vol. 2, pp. 460–475, 2011.
- Lomborg S., Bechmann A. Using APIs for data collection on social media. Inf. Soc. 2014; 30 (4):256–265. [ Google Scholar ]
- F. B. Abdesslem, I. Parris, and T. Henderson, Reliable online social network data collection. In Computational Social Networks: Springer, 2012, pp. 183–210.
- Otte E., Rousseau R. Social network analysis: a powerful strategy, also for the information sciences. J. Inf. Sci. 2002; 28 (6):441–453. [ Google Scholar ]
- Cross R., Borgatti S.P., Parker A. Making invisible work visible: Using social network analysis to support strategic collaboration. Calif. Manage. Rev. 2002; 44 (2):25–46. [ Google Scholar ]
- Parveen F., Jaafar N.I., Ainin S. Social media usage and organizational performance: Reflections of Malaysian social media managers. Telematics Inform. 2015; 32 (1):67–78. [ Google Scholar ]
- Boyd D., Crawford K. Critical questions for big data: Provocations for a cultural, technological, and scholarly phenomenon. Inf. Commun. Soc. 2012; 15 (5):662–679. [ Google Scholar ]
- A. Katal, M. Wazid, and R. Goudar, Big data: Issues, challenges, tools and good practices. In Contemporary Computing (IC3), 2013 Sixth International Conference on, 2013, pp. 404–409: IEEE.
- Terrazas G., Ferry N., Ratchev S. A cloud-based framework for shop floor big data management and elastic computing analytics. Comput. Ind. 2019; 109 :204–214. [ Google Scholar ]
- Canito J., Ramos P., Moro S., Rita P. Unfolding the relations between companies and technologies under the Big Data umbrella. Comput. Ind. 2018; 99 :1–8. [ Google Scholar ]
- di Bella E., Leporatti L., Maggino F. Big data and social indicators: Actual trends and new perspectives. Soc. Indic. Res. 2018; 135 (3):869–878. [ Google Scholar ]
- Hadi M.S., Lawey A.Q., El-Gorashi T.E., Elmirghani J.M. Big data analytics for wireless and wired network design: A survey. Comput. Netw. 2018; 132 :180–199. [ Google Scholar ]
- Gandomi A., Haider M. Beyond the hype: Big data concepts, methods, and analytics. Int. J. Inf. Manage. 2015; 35 (2):137–144. [ Google Scholar ]
- Kitchin R. The real-time city? Big data and smart urbanism. GeoJournal. 2014; 79 (1):1–14. [ Google Scholar ]
- S. Sagiroglu and D. Sinanc, Big data: A review. In Collaboration Technologies and Systems (CTS), 2013 International Conference on, 2013, pp. 42–47: IEEE.
- Pei F.-Q., Li D.-B., Tong Y.-F. Double-layered big data analytics architecture for solar cells series welding machine. Comput. Ind. 2018; 97 :17–23. [ Google Scholar ]
- Peng S., Wang G., Zhou Y., Wan C., Wang C., Yu S. An immunization framework for social networks through big data based influence modeling. IEEE Trans. Dependable Secure Comput. 2017 [ Google Scholar ]
- Duan Y., Edwards J.S., Dwivedi Y.K. Artificial intelligence for decision making in the era of Big Data–Evolution, challenges and research agenda. Int. J. Inf. Manage. 2019; 48 :63–71. [ Google Scholar ]
- Brereton P., Kitchenham B.A., Budgen D., Turner M., Khalil M. Lessons from applying the systematic literature review process within the software engineering domain. J. Syst. Softw. 2007; 80 (4):571–583. [ Google Scholar ]
- B. Kitchenham and S. Charters, Guidelines for performing systematic literature reviews in software engineering, 2007.
- Jamshidi P., Ahmad A., Pahl C. Cloud migration research: A systematic review. IEEE Trans. Cloud Comput. 2013; 1 (2):142–157. [ Google Scholar ]
- Jatoth C., Gangadharan G., Buyya R. Computational intelligence based QoS-aware web service composition: A systematic literature review. IEEE Trans. Serv. Comput. 2015; 10 (3):475–492. [ Google Scholar ]
- Yaqoob I. TEMPORARY REMOVAL: Information fusion in social big data: Foundations, state-of-the-art, applications, challenges, and future research directions. Int. J. Inf. Manage. 2016 [ Google Scholar ]
- Ghani N.A., Hamid S., Hashem I.A.T., Ahmed E. Social media big data analytics: A survey. Comput. Hum. Behav. 2018 [ Google Scholar ]
- Bukovina J. Social media big data and capital markets—An overview. J. Behav. Exp. Finance. 2016; 11 :18–26. [ Google Scholar ]
- M. E. Martin and N. Schuurman, Social media big data acquisition and analysis for qualitative GIScience: challenges and opportunities. Ann. Am. Assoc. Geogr., pp. 1–18, 2019.
- M. Arnaboldi, C. Busco, and S. Cuganesan, Accounting, accountability, social media and big data: revolution or hype? Acc. Audit. Account. J., 2017.
- Peng S., Wang G., Xie D. Social influence analysis in social networking big data: Opportunities and challenges. IEEE Netw. 2016; 31 (1):11–17. [ Google Scholar ]
- I. Guellil and K. Boukhalfa, Social big data mining: A survey focused on opinion mining and sentiments analysis. In 2015 12th International Symposium on Programming and Systems (ISPS), 2015, pp. 1–10: IEEE.
- S. Gole and B. Tidke, A survey of big data in social media using data mining techniques. In 2015 International Conference on Advanced Computing and Communication Systems, 2015, pp. 1–6: IEEE.
- P. V. Paul, K. Monica, and M. Trishanka, A survey on big data analytics using social media data. In 2017 Innovations in Power and Advanced Computing Technologies (i-PACT), 2017, pp. 1–4: IEEE.
- Sebei H., Taieb M.A.H., Aouicha M.B. Review of social media analytics process and Big Data pipeline. Social Netw. Anal. Min. 2018; 8 (1):30. [ Google Scholar ]
- Al-Garadi M.A. Predicting cyberbullying on social media in the big data era using machine learning algorithms: Review of literature and open challenges. IEEE Access. 2019; 7 :70701–70718. [ Google Scholar ]
- O. Lerena, F. Barletta, F. Fiorentin, D. Suárez, and G. Yoguel, Big data of innovation literature at the firm level: a review based on social network and text mining techniques. Econ. Innov. New Technol., pp. 1–17, 2019.
- Kitchenham B., Brereton O.P., Budgen D., Turner M., Bailey J., Linkman S. Systematic literature reviews in software engineering–A systematic literature review. Inf. Softw. Technol. 2009; 51 (1):7–15. [ Google Scholar ]
- Rahimi M., Songhorabadi M., Kashani M.H. Fog-based smart homes: A systematic review. J. Netw. Comput. Appl. 2020 [ Google Scholar ]
- Haghi Kashani M., Rahmani A.M., Jafari Navimipour N. Quality of service-aware approaches in fog computing. Int. J. Commun. Syst. 2020 [ Google Scholar ]
- C. Calero, M. F. Bertoa, and M. Á. Moraga, A systematic literature review for software sustainability measures. In 2013 2nd international workshop on green and sustainable software (GREENS), 2013, pp. 46–53: IEEE.
- Aznoli F., Navimipour N.J. Deployment strategies in the wireless sensor networks: systematic literature review, classification, and current trends. Wireless Pers. Commun. 2017; 95 (2):819–846. [ Google Scholar ]
- Yang M., Kiang M., Shang W. Filtering big data from social media–Building an early warning system for adverse drug reactions. J. Biomed. Inform. 2015; 54 :230–240. [ PubMed ] [ Google Scholar ]
- Aa V., Shekhara V.S., Jb R., Aggrawalb T., Balasubramanya K., Murthya S.N. Cloud based big data analytics framework for face recognition in social networks using machine learning. Procedia Comput. Sci. 2015; 50 :623–630. [ Google Scholar ]
- Moessner M., Feldhege J., Wolf M., Bauer S. Analyzing big data in social media: Text and network analyses of an eating disorder forum. Int. J. Eat. Disord. 2018 [ PubMed ] [ Google Scholar ]
- Cheung M., She J., Jie Z. Connection discovery using big data of user-shared images in social media. IEEE Trans. Multimedia. 2015; 17 (9):1417–1428. [ Google Scholar ]
- N. Straton, R. R. Mukkamala, and R. Vatrapu, Big social data analytics for public health: Predicting facebook post performance using artificial neural networks and deep learning. In 2017 IEEE International Congress on Big Data (BigData Congress), 2017, pp. 89–96: IEEE.
- P. Sachar and V. Khullar, Social media generated big data clustering using genetic algorithm. In 2017 International Conference on Computer Communication and Informatics (ICCCI), 2017, pp. 1–6: IEEE.
- A. Vakali, N. Kitmeridis, and M. Panourgia, A distributed framework for early trending topics detection on big social networks data threads. In INNS Conference on Big Data, 2016, pp. 186–194: Springer.
- Huo Y., Ma L., Zhong Y. A Big Data privacy respecting dissemination method for social network. J. Signal Process. Syst. 2018; 90 (4):467–475. [ Google Scholar ]
- A. H. Zadeh, H. M. Zolbanin, R. Sharda, and D. Delen, Social media for nowcasting flu activity: Spatio-temporal big data analysis. Inf. Syst. Front., pp. 1–18, 2019.
- Xylogiannopoulos K.F., Karampelas P., Alhajj R. A password creation and validation system for social media platforms based on big data analytics. J. Ambient Intell. Hum. Comput. 2020; 11 (1):53–73. [ Google Scholar ]
- Subroto A., Apriyana A. Cyber risk prediction through social media big data analytics and statistical machine learning. J. Big Data. 2019; 6 (1):50. [ Google Scholar ]
- D. Makaroğlu, A. Çakır, and K. Kocabaş, Social Media and Clickstream Analysis in Turkish News with Apache Spark. In International Conference on Intelligent and Fuzzy Systems, 2019, pp. 221–228: Springer.
- Singh A., Kaur M. Intelligent content-based cybercrime detection in online social networks using cuckoo search metaheuristic approach. J. Supercomput. 2019:1–23. [ Google Scholar ]
- R. Thorstad and P. Wolff, Predicting future mental illness from social media: A big-data approach. Behav. Res. Methods, pp. 1–15, 2019. [ PubMed ]
- E. Alomari, I. Katib, and R. Mehmood, Iktishaf: A Big Data road-traffic event detection tool using twitter and spark machine learning. Mob. Netw. Appl., pp. 1–16, 2020.
- Panarello A., Celesti A., Fazio M., Puliafito A., Villari M. A big video data transcoding service for social media over federated clouds. Multimedia Tools Appl. 2020; 79 (13):9037–9061. [ Google Scholar ]
- Sahoo S.R., Gupta B. Fake profile detection in multimedia big data on online social networks. Int. J. Inf. Comput. Secur. 2020; 12 (2–3):303–331. [ Google Scholar ]
- Zhou P., Zhou Y., Wu D., Jin H. Differentially private online learning for cloud-based video recommendation with multimedia big data in social networks. IEEE Trans. Multimedia. 2016; 18 (6):1217–1229. [ Google Scholar ]
- Zhang C., Xie L., Aizezi Y., Gu X. User multi-modal emotional intelligence analysis method based on deep learning in social network Big Data environment. IEEE Access. 2019; 7 :181758–181766. [ Google Scholar ]
- Kauffmann E., Peral J., Gil D., Ferrández A., Sellers R., Mora H. A framework for big data analytics in commercial social networks: A case study on sentiment analysis and fake review detection for marketing decision-making. Ind. Mark. Manage. 2019 [ Google Scholar ]
- Jiang D., Luo X., Xuan J., Xu Z. Sentiment computing for the news event based on the social media big data. IEEE Access. 2017; 5 :2373–2382. [ Google Scholar ]
- Dalla Valle L., Kenett R. Social media big data integration: A new approach based on calibration. Expert Syst. Appl. 2018; 111 :76–90. [ Google Scholar ]
- Jimenez-Marquez J.L., Gonzalez-Carrasco I., Lopez-Cuadrado J.L., Ruiz-Mezcua B. Towards a big data framework for analyzing social media content. Int. J. Inf. Manage. 2019; 44 :1–12. [ Google Scholar ]
- Shirdastian H., Laroche M., Richard M.-O. Using big data analytics to study brand authenticity sentiments: The case of Starbucks on Twitter. Int. J. Inf. Manage. 2019; 48 :291–307. [ Google Scholar ]
- Zhu B., Zheng X., Liu H., Li J., Wang P. Analysis of spatiotemporal characteristics of big data on social media sentiment with COVID-19 epidemic topics. Chaos, Solitons Fractals. 2020; 140 [ PMC free article ] [ PubMed ] [ Google Scholar ]
- Fan M., Billings A., Zhu X., Yu P. Twitter-based BIRGing: Big Data analysis of English national team fans during the 2018 FIFA World Cup. Commun. Sport. 2020; 8 (3):317–345. [ Google Scholar ]
- C. Lee and I. Paik, Stock market analysis from Twitter and news based on streaming big data infrastructure. In 2017 IEEE 8th International Conference on Awareness Science and Technology (iCAST), 2017, pp. 312–317: IEEE.
- A. A. Sayed, M. M. Abdallah, A. M. Zaki, and A. A. Ahmed, Big Data analysis using a metaheuristic algorithm: Twitter as Case Study. In 2020 International Conference on Innovative Trends in Communication and Computer Engineering (ITCE), 2020, pp. 20–26: IEEE.
- van Dieijen M., Borah A., Tellis G.J., Franses P.H. Big data analysis of volatility spillovers of brands across social media and stock markets. Ind. Mark. Manage. 2020; 88 :465–484. [ Google Scholar ]
- Spruce M., Arthur R., Williams H. Using social media to measure impacts of named storm events in the United Kingdom and Ireland. Meteorol. Appl. 2020; 27 (1) [ Google Scholar ]
- Um J.-H., Jeong C.-H., Choi S.-P., Lee S., Kim H.-M., Jung H. Distributed and parallel big textual data parsing for social sensor network. Int. J. Distrib. Sens. Netw. 2013; 9 (12) [ Google Scholar ]
- I. Moise, The technical hashtag in Twitter data: A hadoop experience. In 2016 IEEE International Conference on Big Data (Big Data), 2016, pp. 3519–3528: IEEE.
- D. Hsu, M. Moh, and T.-S. Moh, Mining frequency of drug side effects over a large twitter dataset using apache spark. In Proceedings of the 2017 IEEE/ACM International Conference on Advances in Social Networks Analysis and Mining 2017, 2017, pp. 915–924.
- A. Baltas, A. Kanavos, and A. K. Tsakalidis, An apache spark implementation for sentiment analysis on twitter data. In International Workshop of Algorithmic Aspects of Cloud Computing, 2016, pp. 15–25: Springer.
- X. Sun, C. Zhang, S. Ding, and C. Quan, Detecting anomalous emotion through big data from social networks based on a deep learning method. Multimedia Tools Appl., pp. 1–22, 2018.
- BalaAnand M., Karthikeyan N., Karthik S. Envisioning social media information for big data using big vision schemes in wireless environment. Wireless Pers. Commun. 2019:1–20. [ Google Scholar ]
- A. P. Rodrigues and N. N. Chiplunkar, A new big data approach for topic classification and sentiment analysis of Twitter data. Evol. Intell., pp. 1–11, 2019.
- Persico V., Pescapé A., Picariello A., Sperlí G. Benchmarking big data architectures for social networks data processing using public cloud platforms. Future Gener. Comput. Syst. 2018; 89 :98–109. [ Google Scholar ]
- Elkin L.S., Topal K., Bebek G. Network based model of social media big data predicts contagious disease diffusion. Inf. Disc. Del. 2017; 45 (3):110–120. [ PMC free article ] [ PubMed ] [ Google Scholar ]
- Gao S., Pang H., Gallinari P., Guo J., Kato N. A novel embedding method for information diffusion prediction in social network big data. IEEE Trans. Ind. Inf. 2017; 13 (4):2097–2105. [ Google Scholar ]
- A. Talukder and C. S. Hong, A heuristic mixed model for viral marketing cost minimization in social networks. In 2019 International Conference on Information Networking (ICOIN), 2019, pp. 141–146: IEEE.
- Chen S., Yin X., Cao Q., Li Q., Long H. Targeted influence maximization based on cloud computing over big data in social networks. IEEE Access. 2020; 8 :45512–45522. [ Google Scholar ]
- Y. Wang, B. Zhang, A. V. Vasilakos, and J. Ma, PRDiscount: A heuristic scheme of initial seeds selection for diffusion maximization in social networks. In International Conference on Intelligent Computing, 2014, pp. 149–161: Springer.
- Kumaran P., Chitrakala S. Social influence determination on big data streams in an online social network. Multimedia Tools Appl. 2017; 76 (21):22133–22167. [ Google Scholar ]
- Wu Y., Huang H., Wu N., Wang Y., Bhuiyan M.Z.A., Wang T. An incentive-based protection and recovery strategy for secure big data in social networks. Inf. Sci. 2020; 508 :79–91. [ Google Scholar ]
- Wu Y., Huang H., Zhao J., Wang C., Wang T. Using mobile nodes to control rumors in big data based on a new rumor propagation model in vehicular social networks. IEEE Access. 2018; 6 :62612–62621. [ Google Scholar ]
- Wu J., Zhao M., Chen Z. Small data: Effective data based on big communication research in social networks. Wireless Pers. Commun. 2018; 99 (3):1391–1404. [ Google Scholar ]
- Óskarsdóttir M., Bravo C., Sarraute C., Vanthienen J., Baesens B. The value of big data for credit scoring: Enhancing financial inclusion using mobile phone data and social network analytics. Appl. Soft Comput. 2019; 74 :26–39. [ Google Scholar ]
- Yang X., McEwen R., Ong L.R., Zihayat M. A big data analytics framework for detecting user-level depression from social networks. Int. J. Inf. Manage. 2020; 54 [ Google Scholar ]
- Raj E.D., Babu L.D. A firefly swarm approach for establishing new connections in social networks based on big data analytics. Int. J. Commun. Netw. Distrib.Syst. 2015; 15 (2–3):130–148. [ Google Scholar ]
- K. Xu, F. Wang, X. Jia, and H. Wang, The impact of sampling on big data analysis of social media: A case study on flu and ebola. In 2015 IEEE Global Communications Conference (GLOBECOM), 2015, pp. 1–6: IEEE.
- Su Z., Xu Q., Qi Q. Big data in mobile social networks: A QoE-oriented framework. IEEE Network. 2016; 30 (1):52–57. [ Google Scholar ]
- K. S. Kumar, D. E. Geetha, N. Nagesh, and T. S. Manoj, Identify the influential user in online social networks using R, Hadoop and Python. In 2016 International Conference on Circuits, Controls, Communications and Computing (I4C), 2016, pp. 1–6: IEEE.
- Y. Zhang, Z. Huang, S. Wang, X. Wang, and T. Jiang, “Spark-based measurement and analysis on offline mobile application market over device-to-device sharing in mobile social networks. in 2017 IEEE 23rd International Conference on Parallel and Distributed Systems (ICPADS), 2017, pp. 545–552: IEEE.
- Maireder A., Weeks B.E., Gil de Zúñiga H., Schlögl S. Big data and political social Networks: Introducing audience diversity and communication connector bridging measures in social network theory. Social Sci. Comput. Rev. 2017; 35 (1):126–141. [ Google Scholar ]
- Dabas C. Big data analytics for exploratory social network analysis. Int. J. Inf. Technol. Manage. 2017; 16 (4):348–359. [ Google Scholar ]
- H. Aksu, M. Canim, Y.-C. Chang, I. Korpeoglu, and Ö. Ulusoy, Multi-resolution social network community identification and maintenance on big data platform. In Big Data (BigData Congress), 2013 IEEE International Congress on, 2013, pp. 102–109: IEEE.
- Z. Wu, J. Chen, and Y. Zhang, An incremental community detection method in social big data. In 2018 IEEE/ACM 5th International Conference on Big Data Computing Applications and Technologies (BDCAT), 2018, pp. 136–141: IEEE.
- S. Yousfi, D. Chiadmi, F. Nafis, Toward a Big Data-as-a-service for social networks graphs analysis. In Proceedings of the Mediterranean Conference on Information & Communication Technologies 2015, 2016, pp. 593–598: Springer.
- Sun J., Xu W., Ma J., Sun J. Leverage RAF to find domain experts on research social network services: A big data analytics methodology with MapReduce framework. Int. J. Prod. Econ. 2015; 165 :185–193. [ Google Scholar ]
- Ghosh G., Banerjee S., Yen N.Y. State transition in communication under social network: An analysis using fuzzy logic and density based clustering towards big data paradigm. Future Gener. Comput. Syst. 2016; 65 :207–220. [ Google Scholar ]
- Wang F., Mack E.A., Maciewjewski R. Analyzing entrepreneurial social networks with big data. Ann. Am. Assoc. Geogr. 2017; 107 (1):130–150. [ Google Scholar ]
- K. Lin, J. Luo, L. Hu, M. S. Hossain, and A. Ghoneim, Localization based on social big data analysis in the vehicular networks. IEEE Trans. Ind. Inform, 99(1), 2016.
- C. Li, P. Zhou, Y. Zhou, K. Bian, T. Jiang, and S. Rahardja, Distributed private online learning for social big data computing over data center networks. In 2016 IEEE International Conference on Communications (ICC), 2016, pp. 1–6: IEEE.
- I. Paik, Y. Koshiba, and T. A. S. Siriweera, Efficient service discovery using social service network based on big data infrastructure. In 2017 IEEE 11th International Symposium on Embedded Multicore/Many-core Systems-on-Chip (MCSoC), 2017, pp. 166–173: IEEE.
- J. Wang, C. Jiang, S. Guan, L. Xu, and Y. Ren, Big data driven similarity based U-model for online social networks. In GLOBECOM 2017-2017 IEEE Global Communications Conference, 2017, pp. 1–6: IEEE.
- S. Sharma, Building Real-time knowledge in Social Media on Focus Point: An Apache Spark Streaming Implementation. In 2018 IEEE Punecon, pp. 1–6: IEEE.
- H. F. Karimi, S. U. Masruroh, F. Mintarsih, The influence of iteration calculation manipulation on social network analysis toward twitter's users against hoax in Indonesia with single cluster multi-node method using apache Hadoop Hortonworkstm distribution. In 2018 6th International Conference on Cyber and IT Service Management (CITSM), 2018, pp. 1–6: IEEE.
- W. Du, Toward semantic social network analysis for business big data. In 2018 14th International Conference on Semantics, Knowledge and Grids (SKG), 2018, pp. 1–8: IEEE.
- C. K. Leung and H. Zhang, Management of distributed big data for social networks. In 2016 16th IEEE/ACM International Symposium on Cluster, Cloud and Grid Computing (CCGrid), 2016, pp. 639–648: IEEE.
- Jin S., Lin W., Yin H., Yang S., Li A., Deng B. Community structure mining in big data social media networks with MapReduce. Cluster computing. 2015; 18 (3):999–1010. [ Google Scholar ]
- Kuang L., Tang X., Yu M., Huang Y., Guo K. A comprehensive ranking model for tweets big data in online social network. EURASIP J. Wire. Commun. Netw. 2016; 2016 (1):46. [ Google Scholar ]
- Hamzei M., Navimipour N.J. Toward efficient service composition techniques in the Internet of things. IEEE Internet Things J. 2018; 5 (5):3774–3787. [ Google Scholar ]
- M. Akbari, X. Hu, and T.-S. Chua, Learning wellness profiles of users on social networks: The case of diabetes. In Social Web and Health Research: Springer, 2019, pp. 139–169.
- M. Akbari, K. Relia, A. Elghafari, R. Chunara, From the user to the medium: Neural profiling across web communities. In Twelfth International AAAI Conference on Web and Social Media, 2018.
- Nie L., Zhao Y.-L., Akbari M., Shen J., Chua T.-S. Bridging the vocabulary gap between health seekers and healthcare knowledge. IEEE Trans. Knowl. Data Eng. 2014; 27 (2):396–409. [ Google Scholar ]
- M. Akbari and T.-S. Chua, Leveraging behavioral factorization and prior knowledge for community discovery and profiling. Presented at the Proceedings of the Tenth ACM International Conference on Web Search and Data Mining, Cambridge, United Kingdom, 2017.
- Akbari M., Hu X., Wang F., Chua T. Wellness representation of users in social media: Towards joint modelling of heterogeneity and temporality. IEEE Trans. Knowl. Data Eng. 2017; 29 (10):2360–2373. [ Google Scholar ]
- Zhang H., Babar M.A. Systematic reviews in software engineering: An empirical investigation. Inf. Softw. Technol. 2013; 55 (7):1341–1354. [ Google Scholar ]
- Casciaro T., Carley K.M., Krackhardt D. Positive affectivity and accuracy in social network perception. Motiv. Emotion. 1999; 23 (4):285–306. [ Google Scholar ]
- Kalna G., Higham D.J. A clustering coefficient for weighted networks, with application to gene expression data. AI Commun. 2007; 20 (4):263–271. [ Google Scholar ]
- Zhang P., Wang J., Li X., Li M., Di Z., Fan Y. Clustering coefficient and community structure of bipartite networks. Physica A. 2008; 387 (27):6869–6875. [ Google Scholar ]
- Holland P.W., Leinhardt S. Transitivity in structural models of small groups. Comp. Group Stud. 1971; 2 (2):107–124. [ Google Scholar ]
- Watts D.J., Strogatz S.H. Collective dynamics of ‘small-world’networks. Nature. 1998; 393 (6684):440. [ PubMed ] [ Google Scholar ]
- L. A. Cutillo, M. Manulis, T. Strufe, Security and privacy in online social networks. In Handbook of Social Network Technologies and Applications .Springer, 2010, pp. 497–522.
- Amelio A., Pizzuti C. Correction for closeness: Adjusting normalized mutual information measure for clustering comparison. Comput. Intell. 2017; 33 (3):579–601. [ Google Scholar ]
- X. Wang, L. Tang, H. Gao, H. Liu. Discovering overlapping groups in social media. In Data Mining (ICDM), 2010 IEEE 10th International Conference on, 2010, pp. 569–578: IEEE.
- V. Junquero-Trabado, N. Trench-Ribes, M. A. Aguila-Lorente, D. Dominguez-Sal, Comparison of influence metrics in information diffusion networks. In Computational Aspects of Social Networks (CASoN), 2011 International Conference on, 2011, pp. 31–36: IEEE.
- Getoor L., Diehl C.P. Link mining: A survey. Acm Sigkdd Explor. News. 2005; 7 (2):3–12. [ Google Scholar ]
- Abbasi A., Altmann J., Hossain L. Identifying the effects of co-authorship networks on the performance of scholars: A correlation and regression analysis of performance measures and social network analysis measures. J. Inf. 2011; 5 (4):594–607. [ Google Scholar ]
- Everett M.G. Centrality and the dual-projection approach for two-mode social network data. Methodol. Innovations. 2016; 9 [ Google Scholar ]
- Kim Y., Choi T.Y., Yan T., Dooley K. Structural investigation of supply networks: A social network analysis approach. J. Oper. Manage. 2011; 29 (3):194–211. [ Google Scholar ]
- D. G. Luenberger, Introduction to Dynamic Systems: Theory, Models, and Applications. Wiley New York, 1979.
- Newman M.E. Analysis of weighted networks. Phys. Rev. E. 2004; 70 (5) [ PubMed ] [ Google Scholar ]
- S. A. Catanese, P. De Meo, E. Ferrara, G. Fiumara, A. Provetti, Crawling facebook for social network analysis purposes. In Proceedings of the International Conference on Web Intelligence, Mining and Semantics, 2011, p. 52: ACM.
- L. Page, S. Brin, R. Motwani, T. Winograd, The pagerank citation ranking: Bringing order to the web. Stanford InfoLab1999.
- Open access
- Published: 06 January 2022
The use of Big Data Analytics in healthcare
- Kornelia Batko ORCID: orcid.org/0000-0001-6561-3826 1 &
- Andrzej Ślęzak 2
Journal of Big Data volume 9 , Article number: 3 ( 2022 ) Cite this article
70k Accesses
95 Citations
28 Altmetric
Metrics details
The introduction of Big Data Analytics (BDA) in healthcare will allow to use new technologies both in treatment of patients and health management. The paper aims at analyzing the possibilities of using Big Data Analytics in healthcare. The research is based on a critical analysis of the literature, as well as the presentation of selected results of direct research on the use of Big Data Analytics in medical facilities. The direct research was carried out based on research questionnaire and conducted on a sample of 217 medical facilities in Poland. Literature studies have shown that the use of Big Data Analytics can bring many benefits to medical facilities, while direct research has shown that medical facilities in Poland are moving towards data-based healthcare because they use structured and unstructured data, reach for analytics in the administrative, business and clinical area. The research positively confirmed that medical facilities are working on both structural data and unstructured data. The following kinds and sources of data can be distinguished: from databases, transaction data, unstructured content of emails and documents, data from devices and sensors. However, the use of data from social media is lower as in their activity they reach for analytics, not only in the administrative and business but also in the clinical area. It clearly shows that the decisions made in medical facilities are highly data-driven. The results of the study confirm what has been analyzed in the literature that medical facilities are moving towards data-based healthcare, together with its benefits.
Introduction
The main contribution of this paper is to present an analytical overview of using structured and unstructured data (Big Data) analytics in medical facilities in Poland. Medical facilities use both structured and unstructured data in their practice. Structured data has a predetermined schema, it is extensive, freeform, and comes in variety of forms [ 27 ]. In contrast, unstructured data, referred to as Big Data (BD), does not fit into the typical data processing format. Big Data is a massive amount of data sets that cannot be stored, processed, or analyzed using traditional tools. It remains stored but not analyzed. Due to the lack of a well-defined schema, it is difficult to search and analyze such data and, therefore, it requires a specific technology and method to transform it into value [ 20 , 68 ]. Integrating data stored in both structured and unstructured formats can add significant value to an organization [ 27 ]. Organizations must approach unstructured data in a different way. Therefore, the potential is seen in Big Data Analytics (BDA). Big Data Analytics are techniques and tools used to analyze and extract information from Big Data. The results of Big Data analysis can be used to predict the future. They also help in creating trends about the past. When it comes to healthcare, it allows to analyze large datasets from thousands of patients, identifying clusters and correlation between datasets, as well as developing predictive models using data mining techniques [ 60 ].
This paper is the first study to consolidate and characterize the use of Big Data from different perspectives. The first part consists of a brief literature review of studies on Big Data (BD) and Big Data Analytics (BDA), while the second part presents results of direct research aimed at diagnosing the use of big data analyses in medical facilities in Poland.
Healthcare is a complex system with varied stakeholders: patients, doctors, hospitals, pharmaceutical companies and healthcare decision-makers. This sector is also limited by strict rules and regulations. However, worldwide one may observe a departure from the traditional doctor-patient approach. The doctor becomes a partner and the patient is involved in the therapeutic process [ 14 ]. Healthcare is no longer focused solely on the treatment of patients. The priority for decision-makers should be to promote proper health attitudes and prevent diseases that can be avoided [ 81 ]. This became visible and important especially during the Covid-19 pandemic [ 44 ].
The next challenges that healthcare will have to face is the growing number of elderly people and a decline in fertility. Fertility rates in the country are found below the reproductive minimum necessary to keep the population stable [ 10 ]. The reflection of both effects, namely the increase in age and lower fertility rates, are demographic load indicators, which is constantly growing. Forecasts show that providing healthcare in the form it is provided today will become impossible in the next 20 years [ 70 ]. It is especially visible now during the Covid-19 pandemic when healthcare faced quite a challenge related to the analysis of huge data amounts and the need to identify trends and predict the spread of the coronavirus. The pandemic showed it even more that patients should have access to information about their health condition, the possibility of digital analysis of this data and access to reliable medical support online. Health monitoring and cooperation with doctors in order to prevent diseases can actually revolutionize the healthcare system. One of the most important aspects of the change necessary in healthcare is putting the patient in the center of the system.
Technology is not enough to achieve these goals. Therefore, changes should be made not only at the technological level but also in the management and design of complete healthcare processes and what is more, they should affect the business models of service providers. The use of Big Data Analytics is becoming more and more common in enterprises [ 17 , 54 ]. However, medical enterprises still cannot keep up with the information needs of patients, clinicians, administrators and the creator’s policy. The adoption of a Big Data approach would allow the implementation of personalized and precise medicine based on personalized information, delivered in real time and tailored to individual patients.
To achieve this goal, it is necessary to implement systems that will be able to learn quickly about the data generated by people within clinical care and everyday life. This will enable data-driven decision making, receiving better personalized predictions about prognosis and responses to treatments; a deeper understanding of the complex factors and their interactions that influence health at the patient level, the health system and society, enhanced approaches to detecting safety problems with drugs and devices, as well as more effective methods of comparing prevention, diagnostic, and treatment options [ 40 ].
In the literature, there is a lot of research showing what opportunities can be offered to companies by big data analysis and what data can be analyzed. However, there are few studies showing how data analysis in the area of healthcare is performed, what data is used by medical facilities and what analyses and in which areas they carry out. This paper aims to fill this gap by presenting the results of research carried out in medical facilities in Poland. The goal is to analyze the possibilities of using Big Data Analytics in healthcare, especially in Polish conditions. In particular, the paper is aimed at determining what data is processed by medical facilities in Poland, what analyses they perform and in what areas, and how they assess their analytical maturity. In order to achieve this goal, a critical analysis of the literature was performed, and the direct research was based on a research questionnaire conducted on a sample of 217 medical facilities in Poland. It was hypothesized that medical facilities in Poland are working on both structured and unstructured data and moving towards data-based healthcare and its benefits. Examining the maturity of healthcare facilities in the use of Big Data and Big Data Analytics is crucial in determining the potential future benefits that the healthcare sector can gain from Big Data Analytics. There is also a pressing need to predicate whether, in the coming years, healthcare will be able to cope with the threats and challenges it faces.
This paper is divided into eight parts. The first is the introduction which provides background and the general problem statement of this research. In the second part, this paper discusses considerations on use of Big Data and Big Data Analytics in Healthcare, and then, in the third part, it moves on to challenges and potential benefits of using Big Data Analytics in healthcare. The next part involves the explanation of the proposed method. The result of direct research and discussion are presented in the fifth part, while the following part of the paper is the conclusion. The seventh part of the paper presents practical implications. The final section of the paper provides limitations and directions for future research.
Considerations on use Big Data and Big Data Analytics in the healthcare
In recent years one can observe a constantly increasing demand for solutions offering effective analytical tools. This trend is also noticeable in the analysis of large volumes of data (Big Data, BD). Organizations are looking for ways to use the power of Big Data to improve their decision making, competitive advantage or business performance [ 7 , 54 ]. Big Data is considered to offer potential solutions to public and private organizations, however, still not much is known about the outcome of the practical use of Big Data in different types of organizations [ 24 ].
As already mentioned, in recent years, healthcare management worldwide has been changed from a disease-centered model to a patient-centered model, even in value-based healthcare delivery model [ 68 ]. In order to meet the requirements of this model and provide effective patient-centered care, it is necessary to manage and analyze healthcare Big Data.
The issue often raised when it comes to the use of data in healthcare is the appropriate use of Big Data. Healthcare has always generated huge amounts of data and nowadays, the introduction of electronic medical records, as well as the huge amount of data sent by various types of sensors or generated by patients in social media causes data streams to constantly grow. Also, the medical industry generates significant amounts of data, including clinical records, medical images, genomic data and health behaviors. Proper use of the data will allow healthcare organizations to support clinical decision-making, disease surveillance, and public health management. The challenge posed by clinical data processing involves not only the quantity of data but also the difficulty in processing it.
In the literature one can find many different definitions of Big Data. This concept has evolved in recent years, however, it is still not clearly understood. Nevertheless, despite the range and differences in definitions, Big Data can be treated as a: large amount of digital data, large data sets, tool, technology or phenomenon (cultural or technological.
Big Data can be considered as massive and continually generated digital datasets that are produced via interactions with online technologies [ 53 ]. Big Data can be defined as datasets that are of such large sizes that they pose challenges in traditional storage and analysis techniques [ 28 ]. A similar opinion about Big Data was presented by Ohlhorst who sees Big Data as extremely large data sets, possible neither to manage nor to analyze with traditional data processing tools [ 57 ]. In his opinion, the bigger the data set, the more difficult it is to gain any value from it.
In turn, Knapp perceived Big Data as tools, processes and procedures that allow an organization to create, manipulate and manage very large data sets and storage facilities [ 38 ]. From this point of view, Big Data is identified as a tool to gather information from different databases and processes, allowing users to manage large amounts of data.
Similar perception of the term ‘Big Data’ is shown by Carter. According to him, Big Data technologies refer to a new generation of technologies and architectures, designed to economically extract value from very large volumes of a wide variety of data by enabling high velocity capture, discovery and/or analysis [ 13 ].
Jordan combines these two approaches by identifying Big Data as a complex system, as it needs data bases for data to be stored in, programs and tools to be managed, as well as expertise and personnel able to retrieve useful information and visualization to be understood [ 37 ].
Following the definition of Laney for Big Data, it can be state that: it is large amount of data generated in very fast motion and it contains a lot of content [ 43 ]. Such data comes from unstructured sources, such as stream of clicks on the web, social networks (Twitter, blogs, Facebook), video recordings from the shops, recording of calls in a call center, real time information from various kinds of sensors, RFID, GPS devices, mobile phones and other devices that identify and monitor something [ 8 ]. Big Data is a powerful digital data silo, raw, collected with all sorts of sources, unstructured and difficult, or even impossible, to analyze using conventional techniques used so far to relational databases.
While describing Big Data, it cannot be overlooked that the term refers more to a phenomenon than to specific technology. Therefore, instead of defining this phenomenon, trying to describe them, more authors are describing Big Data by giving them characteristics included a collection of V’s related to its nature [ 2 , 3 , 23 , 25 , 58 ]:
Volume (refers to the amount of data and is one of the biggest challenges in Big Data Analytics),
Velocity (speed with which new data is generated, the challenge is to be able to manage data effectively and in real time),
Variety (heterogeneity of data, many different types of healthcare data, the challenge is to derive insights by looking at all available heterogenous data in a holistic manner),
Variability (inconsistency of data, the challenge is to correct the interpretation of data that can vary significantly depending on the context),
Veracity (how trustworthy the data is, quality of the data),
Visualization (ability to interpret data and resulting insights, challenging for Big Data due to its other features as described above).
Value (the goal of Big Data Analytics is to discover the hidden knowledge from huge amounts of data).
Big Data is defined as an information asset with high volume, velocity, and variety, which requires specific technology and method for its transformation into value [ 21 , 77 ]. Big Data is also a collection of information about high-volume, high volatility or high diversity, requiring new forms of processing in order to support decision-making, discovering new phenomena and process optimization [ 5 , 7 ]. Big Data is too large for traditional data-processing systems and software tools to capture, store, manage and analyze, therefore it requires new technologies [ 28 , 50 , 61 ] to manage (capture, aggregate, process) its volume, velocity and variety [ 9 ].
Undoubtedly, Big Data differs from the data sources used so far by organizations. Therefore, organizations must approach this type of unstructured data in a different way. First of all, organizations must start to see data as flows and not stocks—this entails the need to implement the so-called streaming analytics [ 48 ]. The mentioned features make it necessary to use new IT tools that allow the fullest use of new data [ 58 ]. The Big Data idea, inseparable from the huge increase in data available to various organizations or individuals, creates opportunities for access to valuable analyses, conclusions and enables making more accurate decisions [ 6 , 11 , 59 ].
The Big Data concept is constantly evolving and currently it does not focus on huge amounts of data, but rather on the process of creating value from this data [ 52 ]. Big Data is collected from various sources that have different data properties and are processed by different organizational units, resulting in creation of a Big Data chain [ 36 ]. The aim of the organizations is to manage, process and analyze Big Data. In the healthcare sector, Big Data streams consist of various types of data, namely [ 8 , 51 ]:
clinical data, i.e. data obtained from electronic medical records, data from hospital information systems, image centers, laboratories, pharmacies and other organizations providing health services, patient generated health data, physician’s free-text notes, genomic data, physiological monitoring data [ 4 ],
biometric data provided from various types of devices that monitor weight, pressure, glucose level, etc.,
financial data, constituting a full record of economic operations reflecting the conducted activity,
data from scientific research activities, i.e. results of research, including drug research, design of medical devices and new methods of treatment,
data provided by patients, including description of preferences, level of satisfaction, information from systems for self-monitoring of their activity: exercises, sleep, meals consumed, etc.
data from social media.
These data are provided not only by patients but also by organizations and institutions, as well as by various types of monitoring devices, sensors or instruments [ 16 ]. Data that has been generated so far in the healthcare sector is stored in both paper and digital form. Thus, the essence and the specificity of the process of Big Data analyses means that organizations need to face new technological and organizational challenges [ 67 ]. The healthcare sector has always generated huge amounts of data and this is connected, among others, with the need to store medical records of patients. However, the problem with Big Data in healthcare is not limited to an overwhelming volume but also an unprecedented diversity in terms of types, data formats and speed with which it should be analyzed in order to provide the necessary information on an ongoing basis [ 3 ]. It is also difficult to apply traditional tools and methods for management of unstructured data [ 67 ]. Due to the diversity and quantity of data sources that are growing all the time, advanced analytical tools and technologies, as well as Big Data analysis methods which can meet and exceed the possibilities of managing healthcare data, are needed [ 3 , 68 ].
Therefore, the potential is seen in Big Data analyses, especially in the aspect of improving the quality of medical care, saving lives or reducing costs [ 30 ]. Extracting from this tangle of given association rules, patterns and trends will allow health service providers and other stakeholders in the healthcare sector to offer more accurate and more insightful diagnoses of patients, personalized treatment, monitoring of the patients, preventive medicine, support of medical research and health population, as well as better quality of medical services and patient care while, at the same time, the ability to reduce costs (Fig. 1 ).
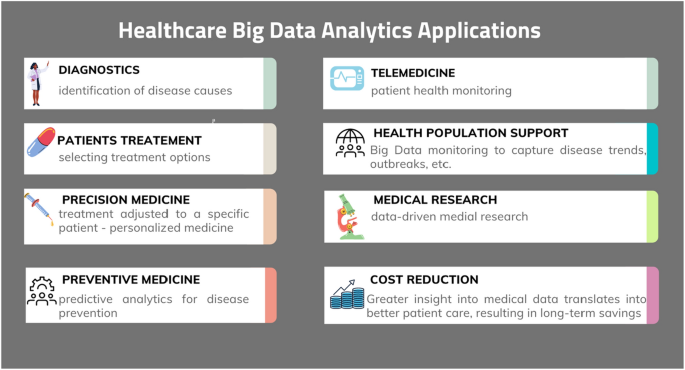
(Source: Own elaboration)
Healthcare Big Data Analytics applications
The main challenge with Big Data is how to handle such a large amount of information and use it to make data-driven decisions in plenty of areas [ 64 ]. In the context of healthcare data, another major challenge is to adjust big data storage, analysis, presentation of analysis results and inference basing on them in a clinical setting. Data analytics systems implemented in healthcare are designed to describe, integrate and present complex data in an appropriate way so that it can be understood better (Fig. 2 ). This would improve the efficiency of acquiring, storing, analyzing and visualizing big data from healthcare [ 71 ].
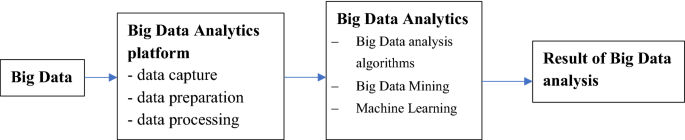
Process of Big Data Analytics
The result of data processing with the use of Big Data Analytics is appropriate data storytelling which may contribute to making decisions with both lower risk and data support. This, in turn, can benefit healthcare stakeholders. To take advantage of the potential massive amounts of data in healthcare and to ensure that the right intervention to the right patient is properly timed, personalized, and potentially beneficial to all components of the healthcare system such as the payer, patient, and management, analytics of large datasets must connect communities involved in data analytics and healthcare informatics [ 49 ]. Big Data Analytics can provide insight into clinical data and thus facilitate informed decision-making about the diagnosis and treatment of patients, prevention of diseases or others. Big Data Analytics can also improve the efficiency of healthcare organizations by realizing the data potential [ 3 , 62 ].
Big Data Analytics in medicine and healthcare refers to the integration and analysis of a large amount of complex heterogeneous data, such as various omics (genomics, epigenomics, transcriptomics, proteomics, metabolomics, interactomics, pharmacogenetics, deasomics), biomedical data, talemedicine data (sensors, medical equipment data) and electronic health records data [ 46 , 65 ].
When analyzing the phenomenon of Big Data in the healthcare sector, it should be noted that it can be considered from the point of view of three areas: epidemiological, clinical and business.
From a clinical point of view, the Big Data analysis aims to improve the health and condition of patients, enable long-term predictions about their health status and implementation of appropriate therapeutic procedures. Ultimately, the use of data analysis in medicine is to allow the adaptation of therapy to a specific patient, that is personalized medicine (precision, personalized medicine).
From an epidemiological point of view, it is desirable to obtain an accurate prognosis of morbidity in order to implement preventive programs in advance.
In the business context, Big Data analysis may enable offering personalized packages of commercial services or determining the probability of individual disease and infection occurrence. It is worth noting that Big Data means not only the collection and processing of data but, most of all, the inference and visualization of data necessary to obtain specific business benefits.
In order to introduce new management methods and new solutions in terms of effectiveness and transparency, it becomes necessary to make data more accessible, digital, searchable, as well as analyzed and visualized.
Erickson and Rothberg state that the information and data do not reveal their full value until insights are drawn from them. Data becomes useful when it enhances decision making and decision making is enhanced only when analytical techniques are used and an element of human interaction is applied [ 22 ].
Thus, healthcare has experienced much progress in usage and analysis of data. A large-scale digitalization and transparency in this sector is a key statement of almost all countries governments policies. For centuries, the treatment of patients was based on the judgment of doctors who made treatment decisions. In recent years, however, Evidence-Based Medicine has become more and more important as a result of it being related to the systematic analysis of clinical data and decision-making treatment based on the best available information [ 42 ]. In the healthcare sector, Big Data Analytics is expected to improve the quality of life and reduce operational costs [ 72 , 82 ]. Big Data Analytics enables organizations to improve and increase their understanding of the information contained in data. It also helps identify data that provides insightful insights for current as well as future decisions [ 28 ].
Big Data Analytics refers to technologies that are grounded mostly in data mining: text mining, web mining, process mining, audio and video analytics, statistical analysis, network analytics, social media analytics and web analytics [ 16 , 25 , 31 ]. Different data mining techniques can be applied on heterogeneous healthcare data sets, such as: anomaly detection, clustering, classification, association rules as well as summarization and visualization of those Big Data sets [ 65 ]. Modern data analytics techniques explore and leverage unique data characteristics even from high-speed data streams and sensor data [ 15 , 16 , 31 , 55 ]. Big Data can be used, for example, for better diagnosis in the context of comprehensive patient data, disease prevention and telemedicine (in particular when using real-time alerts for immediate care), monitoring patients at home, preventing unnecessary hospital visits, integrating medical imaging for a wider diagnosis, creating predictive analytics, reducing fraud and improving data security, better strategic planning and increasing patients’ involvement in their own health.
Big Data Analytics in healthcare can be divided into [ 33 , 73 , 74 ]:
descriptive analytics in healthcare is used to understand past and current healthcare decisions, converting data into useful information for understanding and analyzing healthcare decisions, outcomes and quality, as well as making informed decisions [ 33 ]. It can be used to create reports (i.e. about patients’ hospitalizations, physicians’ performance, utilization management), visualization, customized reports, drill down tables, or running queries on the basis of historical data.
predictive analytics operates on past performance in an effort to predict the future by examining historical or summarized health data, detecting patterns of relationships in these data, and then extrapolating these relationships to forecast. It can be used to i.e. predict the response of different patient groups to different drugs (dosages) or reactions (clinical trials), anticipate risk and find relationships in health data and detect hidden patterns [ 62 ]. In this way, it is possible to predict the epidemic spread, anticipate service contracts and plan healthcare resources. Predictive analytics is used in proper diagnosis and for appropriate treatments to be given to patients suffering from certain diseases [ 39 ].
prescriptive analytics—occurs when health problems involve too many choices or alternatives. It uses health and medical knowledge in addition to data or information. Prescriptive analytics is used in many areas of healthcare, including drug prescriptions and treatment alternatives. Personalized medicine and evidence-based medicine are both supported by prescriptive analytics.
discovery analytics—utilizes knowledge about knowledge to discover new “inventions” like drugs (drug discovery), previously unknown diseases and medical conditions, alternative treatments, etc.
Although the models and tools used in descriptive, predictive, prescriptive, and discovery analytics are different, many applications involve all four of them [ 62 ]. Big Data Analytics in healthcare can help enable personalized medicine by identifying optimal patient-specific treatments. This can influence the improvement of life standards, reduce waste of healthcare resources and save costs of healthcare [ 56 , 63 , 71 ]. The introduction of large data analysis gives new analytical possibilities in terms of scope, flexibility and visualization. Techniques such as data mining (computational pattern discovery process in large data sets) facilitate inductive reasoning and analysis of exploratory data, enabling scientists to identify data patterns that are independent of specific hypotheses. As a result, predictive analysis and real-time analysis becomes possible, making it easier for medical staff to start early treatments and reduce potential morbidity and mortality. In addition, document analysis, statistical modeling, discovering patterns and topics in document collections and data in the EHR, as well as an inductive approach can help identify and discover relationships between health phenomena.
Advanced analytical techniques can be used for a large amount of existing (but not yet analytical) data on patient health and related medical data to achieve a better understanding of the information and results obtained, as well as to design optimal clinical pathways [ 62 ]. Big Data Analytics in healthcare integrates analysis of several scientific areas such as bioinformatics, medical imaging, sensor informatics, medical informatics and health informatics [ 65 ]. Big Data Analytics in healthcare allows to analyze large datasets from thousands of patients, identifying clusters and correlation between datasets, as well as developing predictive models using data mining techniques [ 65 ]. Discussing all the techniques used for Big Data Analytics goes beyond the scope of a single article [ 25 ].
The success of Big Data analysis and its accuracy depend heavily on the tools and techniques used to analyze the ability to provide reliable, up-to-date and meaningful information to various stakeholders [ 12 ]. It is believed that the implementation of big data analytics by healthcare organizations could bring many benefits in the upcoming years, including lowering health care costs, better diagnosis and prediction of diseases and their spread, improving patient care and developing protocols to prevent re-hospitalization, optimizing staff, optimizing equipment, forecasting the need for hospital beds, operating rooms, treatments, and improving the drug supply chain [ 71 ].
Challenges and potential benefits of using Big Data Analytics in healthcare
Modern analytics gives possibilities not only to have insight in historical data, but also to have information necessary to generate insight into what may happen in the future. Even when it comes to prediction of evidence-based actions. The emphasis on reform has prompted payers and suppliers to pursue data analysis to reduce risk, detect fraud, improve efficiency and save lives. Everyone—payers, providers, even patients—are focusing on doing more with fewer resources. Thus, some areas in which enhanced data and analytics can yield the greatest results include various healthcare stakeholders (Table 1 ).
Healthcare organizations see the opportunity to grow through investments in Big Data Analytics. In recent years, by collecting medical data of patients, converting them into Big Data and applying appropriate algorithms, reliable information has been generated that helps patients, physicians and stakeholders in the health sector to identify values and opportunities [ 31 ]. It is worth noting that there are many changes and challenges in the structure of the healthcare sector. Digitization and effective use of Big Data in healthcare can bring benefits to every stakeholder in this sector. A single doctor would benefit the same as the entire healthcare system. Potential opportunities to achieve benefits and effects from Big Data in healthcare can be divided into four groups [ 8 ]:
Improving the quality of healthcare services:
assessment of diagnoses made by doctors and the manner of treatment of diseases indicated by them based on the decision support system working on Big Data collections,
detection of more effective, from a medical point of view, and more cost-effective ways to diagnose and treat patients,
analysis of large volumes of data to reach practical information useful for identifying needs, introducing new health services, preventing and overcoming crises,
prediction of the incidence of diseases,
detecting trends that lead to an improvement in health and lifestyle of the society,
analysis of the human genome for the introduction of personalized treatment.
Supporting the work of medical personnel
doctors’ comparison of current medical cases to cases from the past for better diagnosis and treatment adjustment,
detection of diseases at earlier stages when they can be more easily and quickly cured,
detecting epidemiological risks and improving control of pathogenic spots and reaction rates,
identification of patients who are predicted to have the highest risk of specific, life-threatening diseases by collating data on the history of the most common diseases, in healing people with reports entering insurance companies,
health management of each patient individually (personalized medicine) and health management of the whole society,
capturing and analyzing large amounts of data from hospitals and homes in real time, life monitoring devices to monitor safety and predict adverse events,
analysis of patient profiles to identify people for whom prevention should be applied, lifestyle change or preventive care approach,
the ability to predict the occurrence of specific diseases or worsening of patients’ results,
predicting disease progression and its determinants, estimating the risk of complications,
detecting drug interactions and their side effects.
Supporting scientific and research activity
supporting work on new drugs and clinical trials thanks to the possibility of analyzing “all data” instead of selecting a test sample,
the ability to identify patients with specific, biological features that will take part in specialized clinical trials,
selecting a group of patients for which the tested drug is likely to have the desired effect and no side effects,
using modeling and predictive analysis to design better drugs and devices.
Business and management
reduction of costs and counteracting abuse and counseling practices,
faster and more effective identification of incorrect or unauthorized financial operations in order to prevent abuse and eliminate errors,
increase in profitability by detecting patients generating high costs or identifying doctors whose work, procedures and treatment methods cost the most and offering them solutions that reduce the amount of money spent,
identification of unnecessary medical activities and procedures, e.g. duplicate tests.
According to research conducted by Wang, Kung and Byrd, Big Data Analytics benefits can be classified into five categories: IT infrastructure benefits (reducing system redundancy, avoiding unnecessary IT costs, transferring data quickly among healthcare IT systems, better use of healthcare systems, processing standardization among various healthcare IT systems, reducing IT maintenance costs regarding data storage), operational benefits (improving the quality and accuracy of clinical decisions, processing a large number of health records in seconds, reducing the time of patient travel, immediate access to clinical data to analyze, shortening the time of diagnostic test, reductions in surgery-related hospitalizations, exploring inconceivable new research avenues), organizational benefits (detecting interoperability problems much more quickly than traditional manual methods, improving cross-functional communication and collaboration among administrative staffs, researchers, clinicians and IT staffs, enabling data sharing with other institutions and adding new services, content sources and research partners), managerial benefits (gaining quick insights about changing healthcare trends in the market, providing members of the board and heads of department with sound decision-support information on the daily clinical setting, optimizing business growth-related decisions) and strategic benefits (providing a big picture view of treatment delivery for meeting future need, creating high competitive healthcare services) [ 73 ].
The above specification does not constitute a full list of potential areas of use of Big Data Analysis in healthcare because the possibilities of using analysis are practically unlimited. In addition, advanced analytical tools allow to analyze data from all possible sources and conduct cross-analyses to provide better data insights [ 26 ]. For example, a cross-analysis can refer to a combination of patient characteristics, as well as costs and care results that can help identify the best, in medical terms, and the most cost-effective treatment or treatments and this may allow a better adjustment of the service provider’s offer [ 62 ].
In turn, the analysis of patient profiles (e.g. segmentation and predictive modeling) allows identification of people who should be subject to prophylaxis, prevention or should change their lifestyle [ 8 ]. Shortened list of benefits for Big Data Analytics in healthcare is presented in paper [ 3 ] and consists of: better performance, day-to-day guides, detection of diseases in early stages, making predictive analytics, cost effectiveness, Evidence Based Medicine and effectiveness in patient treatment.
Summarizing, healthcare big data represents a huge potential for the transformation of healthcare: improvement of patients’ results, prediction of outbreaks of epidemics, valuable insights, avoidance of preventable diseases, reduction of the cost of healthcare delivery and improvement of the quality of life in general [ 1 ]. Big Data also generates many challenges such as difficulties in data capture, data storage, data analysis and data visualization [ 15 ]. The main challenges are connected with the issues of: data structure (Big Data should be user-friendly, transparent, and menu-driven but it is fragmented, dispersed, rarely standardized and difficult to aggregate and analyze), security (data security, privacy and sensitivity of healthcare data, there are significant concerns related to confidentiality), data standardization (data is stored in formats that are not compatible with all applications and technologies), storage and transfers (especially costs associated with securing, storing, and transferring unstructured data), managerial skills, such as data governance, lack of appropriate analytical skills and problems with Real-Time Analytics (health care is to be able to utilize Big Data in real time) [ 4 , 34 , 41 ].
The research is based on a critical analysis of the literature, as well as the presentation of selected results of direct research on the use of Big Data Analytics in medical facilities in Poland.
Presented research results are part of a larger questionnaire form on Big Data Analytics. The direct research was based on an interview questionnaire which contained 100 questions with 5-point Likert scale (1—strongly disagree, 2—I rather disagree, 3—I do not agree, nor disagree, 4—I rather agree, 5—I definitely agree) and 4 metrics questions. The study was conducted in December 2018 on a sample of 217 medical facilities (110 private, 107 public). The research was conducted by a specialized market research agency: Center for Research and Expertise of the University of Economics in Katowice.
When it comes to direct research, the selected entities included entities financed from public sources—the National Health Fund (23.5%), and entities operating commercially (11.5%). In the surveyed group of entities, more than a half (64.9%) are hybrid financed, both from public and commercial sources. The diversity of the research sample also applies to the size of the entities, defined by the number of employees. Taking into account proportions of the surveyed entities, it should be noted that in the sector structure, medium-sized (10–50 employees—34% of the sample) and large (51–250 employees—27%) entities dominate. The research was of all-Poland nature, and the entities included in the research sample come from all of the voivodships. The largest group were entities from Łódzkie (32%), Śląskie (18%) and Mazowieckie (18%) voivodships, as these voivodships have the largest number of medical institutions. Other regions of the country were represented by single units. The selection of the research sample was random—layered. As part of medical facilities database, groups of private and public medical facilities have been identified and the ones to which the questionnaire was targeted were drawn from each of these groups. The analyses were performed using the GNU PSPP 0.10.2 software.
The aim of the study was to determine whether medical facilities in Poland use Big Data Analytics and if so, in which areas. Characteristics of the research sample is presented in Table 2 .
The research is non-exhaustive due to the incomplete and uneven regional distribution of the samples, overrepresented in three voivodeships (Łódzkie, Mazowieckie and Śląskie). The size of the research sample (217 entities) allows the authors of the paper to formulate specific conclusions on the use of Big Data in the process of its management.
For the purpose of this paper, the following research hypotheses were formulated: (1) medical facilities in Poland are working on both structured and unstructured data (2) medical facilities in Poland are moving towards data-based healthcare and its benefits.
The paper poses the following research questions and statements that coincide with the selected questions from the research questionnaire:
From what sources do medical facilities obtain data? What types of data are used by the particular organization, whether structured or unstructured, and to what extent?
From what sources do medical facilities obtain data?
In which area organizations are using data and analytical systems (clinical or business)?
Is data analytics performed based on historical data or are predictive analyses also performed?
Determining whether administrative and medical staff receive complete, accurate and reliable data in a timely manner?
Determining whether real-time analyses are performed to support the particular organization’s activities.
Results and discussion
On the basis of the literature analysis and research study, a set of questions and statements related to the researched area was formulated. The results from the surveys show that medical facilities use a variety of data sources in their operations. These sources are both structured and unstructured data (Table 3 ).
According to the data provided by the respondents, considering the first statement made in the questionnaire, almost half of the medical institutions (47.58%) agreed that they rather collect and use structured data (e.g. databases and data warehouses, reports to external entities) and 10.57% entirely agree with this statement. As much as 23.35% of representatives of medical institutions stated “I agree or disagree”. Other medical facilities do not collect and use structured data (7.93%) and 6.17% strongly disagree with the first statement. Also, the median calculated based on the obtained results (median: 4), proves that medical facilities in Poland collect and use structured data (Table 4 ).
In turn, 28.19% of the medical institutions agreed that they rather collect and use unstructured data and as much as 9.25% entirely agree with this statement. The number of representatives of medical institutions that stated “I agree or disagree” was 27.31%. Other medical facilities do not collect and use structured data (17.18%) and 13.66% strongly disagree with the first statement. In the case of unstructured data the median is 3, which means that the collection and use of this type of data by medical facilities in Poland is lower.
In the further part of the analysis, it was checked whether the size of the medical facility and form of ownership have an impact on whether it analyzes unstructured data (Tables 4 and 5 ). In order to find this out, correlation coefficients were calculated.
Based on the calculations, it can be concluded that there is a small statistically monotonic correlation between the size of the medical facility and its collection and use of structured data (p < 0.001; τ = 0.16). This means that the use of structured data is slightly increasing in larger medical facilities. The size of the medical facility is more important according to use of unstructured data (p < 0.001; τ = 0.23) (Table 4 .).
To determine whether the form of medical facility ownership affects data collection, the Mann–Whitney U test was used. The calculations show that the form of ownership does not affect what data the organization collects and uses (Table 5 ).
Detailed information on the sources of from which medical facilities collect and use data is presented in the Table 6 .
The questionnaire results show that medical facilities are especially using information published in databases, reports to external units and transaction data, but they also use unstructured data from e-mails, medical devices, sensors, phone calls, audio and video data (Table 6 ). Data from social media, RFID and geolocation data are used to a small extent. Similar findings are concluded in the literature studies.
From the analysis of the answers given by the respondents, more than half of the medical facilities have integrated hospital system (HIS) implemented. As much as 43.61% use integrated hospital system and 16.30% use it extensively (Table 7 ). 19.38% of exanimated medical facilities do not use it at all. Moreover, most of the examined medical facilities (34.80% use it, 32.16% use extensively) conduct medical documentation in an electronic form, which gives an opportunity to use data analytics. Only 4.85% of medical facilities don’t use it at all.
Other problems that needed to be investigated were: whether medical facilities in Poland use data analytics? If so, in what form and in what areas? (Table 8 ). The analysis of answers given by the respondents about the potential of data analytics in medical facilities shows that a similar number of medical facilities use data analytics in administration and business (31.72% agreed with the statement no. 5 and 12.33% strongly agreed) as in the clinical area (33.04% agreed with the statement no. 6 and 12.33% strongly agreed). When considering decision-making issues, 35.24% agree with the statement "the organization uses data and analytical systems to support business decisions” and 8.37% of respondents strongly agree. Almost 40.09% agree with the statement that “the organization uses data and analytical systems to support clinical decisions (in the field of diagnostics and therapy)” and 15.42% of respondents strongly agree. Exanimated medical facilities use in their activity analytics based both on historical data (33.48% agree with statement 7 and 12.78% strongly agree) and predictive analytics (33.04% agrees with the statement number 8 and 15.86% strongly agree). Detailed results are presented in Table 8 .
Medical facilities focus on development in the field of data processing, as they confirm that they conduct analytical planning processes systematically and analyze new opportunities for strategic use of analytics in business and clinical activities (38.33% rather agree and 10.57% strongly agree with this statement). The situation is different with real-time data analysis, here, the situation is not so optimistic. Only 28.19% rather agree and 14.10% strongly agree with the statement that real-time analyses are performed to support an organization’s activities.
When considering whether a facility’s performance in the clinical area depends on the form of ownership, it can be concluded that taking the average and the Mann–Whitney U test depends. A higher degree of use of analyses in the clinical area can be observed in public institutions.
Whether a medical facility performs a descriptive or predictive analysis do not depend on the form of ownership (p > 0.05). It can be concluded that when analyzing the mean and median, they are higher in public facilities, than in private ones. What is more, the Mann–Whitney U test shows that these variables are dependent from each other (p < 0.05) (Table 9 ).
When considering whether a facility’s performance in the clinical area depends on its size, it can be concluded that taking the Kendall’s Tau (τ) it depends (p < 0.001; τ = 0.22), and the correlation is weak but statistically important. This means that the use of data and analytical systems to support clinical decisions (in the field of diagnostics and therapy) increases with the increase of size of the medical facility. A similar relationship, but even less powerful, can be found in the use of descriptive and predictive analyses (Table 10 ).
Considering the results of research in the area of analytical maturity of medical facilities, 8.81% of medical facilities stated that they are at the first level of maturity, i.e. an organization has developed analytical skills and does not perform analyses. As much as 13.66% of medical facilities confirmed that they have poor analytical skills, while 38.33% of the medical facility has located itself at level 3, meaning that “there is a lot to do in analytics”. On the other hand, 28.19% believe that analytical capabilities are well developed and 6.61% stated that analytics are at the highest level and the analytical capabilities are very well developed. Detailed data is presented in Table 11 . Average amounts to 3.11 and Median to 3.
The results of the research have enabled the formulation of following conclusions. Medical facilities in Poland are working on both structured and unstructured data. This data comes from databases, transactions, unstructured content of emails and documents, devices and sensors. However, the use of data from social media is smaller. In their activity, they reach for analytics in the administrative and business, as well as in the clinical area. Also, the decisions made are largely data-driven.
In summary, analysis of the literature that the benefits that medical facilities can get using Big Data Analytics in their activities relate primarily to patients, physicians and medical facilities. It can be confirmed that: patients will be better informed, will receive treatments that will work for them, will have prescribed medications that work for them and not be given unnecessary medications [ 78 ]. Physician roles will likely change to more of a consultant than decision maker. They will advise, warn, and help individual patients and have more time to form positive and lasting relationships with their patients in order to help people. Medical facilities will see changes as well, for example in fewer unnecessary hospitalizations, resulting initially in less revenue, but after the market adjusts, also the accomplishment [ 78 ]. The use of Big Data Analytics can literally revolutionize the way healthcare is practiced for better health and disease reduction.
The analysis of the latest data reveals that data analytics increase the accuracy of diagnoses. Physicians can use predictive algorithms to help them make more accurate diagnoses [ 45 ]. Moreover, it could be helpful in preventive medicine and public health because with early intervention, many diseases can be prevented or ameliorated [ 29 ]. Predictive analytics also allows to identify risk factors for a given patient, and with this knowledge patients will be able to change their lives what, in turn, may contribute to the fact that population disease patterns may dramatically change, resulting in savings in medical costs. Moreover, personalized medicine is the best solution for an individual patient seeking treatment. It can help doctors decide the exact treatments for those individuals. Better diagnoses and more targeted treatments will naturally lead to increases in good outcomes and fewer resources used, including doctors’ time.
The quantitative analysis of the research carried out and presented in this article made it possible to determine whether medical facilities in Poland use Big Data Analytics and if so, in which areas. Thanks to the results obtained it was possible to formulate the following conclusions. Medical facilities are working on both structured and unstructured data, which comes from databases, transactions, unstructured content of emails and documents, devices and sensors. According to analytics, they reach for analytics in the administrative and business, as well as in the clinical area. It clearly showed that the decisions made are largely data-driven. The results of the study confirm what has been analyzed in the literature. Medical facilities are moving towards data-based healthcare and its benefits.
In conclusion, Big Data Analytics has the potential for positive impact and global implications in healthcare. Future research on the use of Big Data in medical facilities will concern the definition of strategies adopted by medical facilities to promote and implement such solutions, as well as the benefits they gain from the use of Big Data analysis and how the perspectives in this area are seen.
Practical implications
This work sought to narrow the gap that exists in analyzing the possibility of using Big Data Analytics in healthcare. Showing how medical facilities in Poland are doing in this respect is an element that is part of global research carried out in this area, including [ 29 , 32 , 60 ].
Limitations and future directions
The research described in this article does not fully exhaust the questions related to the use of Big Data Analytics in Polish healthcare facilities. Only some of the dimensions characterizing the use of data by medical facilities in Poland have been examined. In order to get the full picture, it would be necessary to examine the results of using structured and unstructured data analytics in healthcare. Future research may examine the benefits that medical institutions achieve as a result of the analysis of structured and unstructured data in the clinical and management areas and what limitations they encounter in these areas. For this purpose, it is planned to conduct in-depth interviews with chosen medical facilities in Poland. These facilities could give additional data for empirical analyses based more on their suggestions. Further research should also include medical institutions from beyond the borders of Poland, enabling international comparative analyses.
Future research in the healthcare field has virtually endless possibilities. These regard the use of Big Data Analytics to diagnose specific conditions [ 47 , 66 , 69 , 76 ], propose an approach that can be used in other healthcare applications and create mechanisms to identify “patients like me” [ 75 , 80 ]. Big Data Analytics could also be used for studies related to the spread of pandemics, the efficacy of covid treatment [ 18 , 79 ], or psychology and psychiatry studies, e.g. emotion recognition [ 35 ].
Availability of data and materials
The datasets for this study are available on request to the corresponding author.
Abouelmehdi K, Beni-Hessane A, Khaloufi H. Big healthcare data: preserving security and privacy. J Big Data. 2018. https://doi.org/10.1186/s40537-017-0110-7 .
Article Google Scholar
Agrawal A, Choudhary A. Health services data: big data analytics for deriving predictive healthcare insights. Health Serv Eval. 2019. https://doi.org/10.1007/978-1-4899-7673-4_2-1 .
Al Mayahi S, Al-Badi A, Tarhini A. Exploring the potential benefits of big data analytics in providing smart healthcare. In: Miraz MH, Excell P, Ware A, Ali M, Soomro S, editors. Emerging technologies in computing—first international conference, iCETiC 2018, proceedings (Lecture Notes of the Institute for Computer Sciences, Social-Informatics and Telecommunications Engineering, LNICST). Cham: Springer; 2018. p. 247–58. https://doi.org/10.1007/978-3-319-95450-9_21 .
Bainbridge M. Big data challenges for clinical and precision medicine. In: Househ M, Kushniruk A, Borycki E, editors. Big data, big challenges: a healthcare perspective: background, issues, solutions and research directions. Cham: Springer; 2019. p. 17–31.
Google Scholar
Bartuś K, Batko K, Lorek P. Business intelligence systems: barriers during implementation. In: Jabłoński M, editor. Strategic performance management new concept and contemporary trends. New York: Nova Science Publishers; 2017. p. 299–327. ISBN: 978-1-53612-681-5.
Bartuś K, Batko K, Lorek P. Diagnoza wykorzystania big data w organizacjach-wybrane wyniki badań. Informatyka Ekonomiczna. 2017;3(45):9–20.
Bartuś K, Batko K, Lorek P. Wykorzystanie rozwiązań business intelligence, competitive intelligence i big data w przedsiębiorstwach województwa śląskiego. Przegląd Organizacji. 2018;2:33–9.
Batko K. Możliwości wykorzystania Big Data w ochronie zdrowia. Roczniki Kolegium Analiz Ekonomicznych. 2016;42:267–82.
Bi Z, Cochran D. Big data analytics with applications. J Manag Anal. 2014;1(4):249–65. https://doi.org/10.1080/23270012.2014.992985 .
Boerma T, Requejo J, Victora CG, Amouzou A, Asha G, Agyepong I, Borghi J. Countdown to 2030: tracking progress towards universal coverage for reproductive, maternal, newborn, and child health. Lancet. 2018;391(10129):1538–48.
Bollier D, Firestone CM. The promise and peril of big data. Washington, D.C: Aspen Institute, Communications and Society Program; 2010. p. 1–66.
Bose R. Competitive intelligence process and tools for intelligence analysis. Ind Manag Data Syst. 2008;108(4):510–28.
Carter P. Big data analytics: future architectures, skills and roadmaps for the CIO: in white paper, IDC sponsored by SAS. 2011. p. 1–16.
Castro EM, Van Regenmortel T, Vanhaecht K, Sermeus W, Van Hecke A. Patient empowerment, patient participation and patient-centeredness in hospital care: a concept analysis based on a literature review. Patient Educ Couns. 2016;99(12):1923–39.
Chen H, Chiang RH, Storey VC. Business intelligence and analytics: from big data to big impact. MIS Q. 2012;36(4):1165–88.
Chen CP, Zhang CY. Data-intensive applications, challenges, techniques and technologies: a survey on big data. Inf Sci. 2014;275:314–47.
Chomiak-Orsa I, Mrozek B. Główne perspektywy wykorzystania big data w mediach społecznościowych. Informatyka Ekonomiczna. 2017;3(45):44–54.
Corsi A, de Souza FF, Pagani RN, et al. Big data analytics as a tool for fighting pandemics: a systematic review of literature. J Ambient Intell Hum Comput. 2021;12:9163–80. https://doi.org/10.1007/s12652-020-02617-4 .
Davenport TH, Harris JG. Competing on analytics, the new science of winning. Boston: Harvard Business School Publishing Corporation; 2007.
Davenport TH. Big data at work: dispelling the myths, uncovering the opportunities. Boston: Harvard Business School Publishing; 2014.
De Cnudde S, Martens D. Loyal to your city? A data mining analysis of a public service loyalty program. Decis Support Syst. 2015;73:74–84.
Erickson S, Rothberg H. Data, information, and intelligence. In: Rodriguez E, editor. The analytics process. Boca Raton: Auerbach Publications; 2017. p. 111–26.
Fang H, Zhang Z, Wang CJ, Daneshmand M, Wang C, Wang H. A survey of big data research. IEEE Netw. 2015;29(5):6–9.
Fredriksson C. Organizational knowledge creation with big data. A case study of the concept and practical use of big data in a local government context. 2016. https://www.abo.fi/fakultet/media/22103/fredriksson.pdf .
Gandomi A, Haider M. Beyond the hype: big data concepts, methods, and analytics. Int J Inf Manag. 2015;35(2):137–44.
Groves P, Kayyali B, Knott D, Van Kuiken S. The ‘big data’ revolution in healthcare. Accelerating value and innovation. 2015. http://www.pharmatalents.es/assets/files/Big_Data_Revolution.pdf (Reading: 10.04.2019).
Gupta V, Rathmore N. Deriving business intelligence from unstructured data. Int J Inf Comput Technol. 2013;3(9):971–6.
Gupta V, Singh VK, Ghose U, Mukhija P. A quantitative and text-based characterization of big data research. J Intell Fuzzy Syst. 2019;36:4659–75.
Hampel HOBS, O’Bryant SE, Castrillo JI, Ritchie C, Rojkova K, Broich K, Escott-Price V. PRECISION MEDICINE-the golden gate for detection, treatment and prevention of Alzheimer’s disease. J Prev Alzheimer’s Dis. 2016;3(4):243.
Harerimana GB, Jang J, Kim W, Park HK. Health big data analytics: a technology survey. IEEE Access. 2018;6:65661–78. https://doi.org/10.1109/ACCESS.2018.2878254 .
Hu H, Wen Y, Chua TS, Li X. Toward scalable systems for big data analytics: a technology tutorial. IEEE Access. 2014;2:652–87.
Hussain S, Hussain M, Afzal M, Hussain J, Bang J, Seung H, Lee S. Semantic preservation of standardized healthcare documents in big data. Int J Med Inform. 2019;129:133–45. https://doi.org/10.1016/j.ijmedinf.2019.05.024 .
Islam MS, Hasan MM, Wang X, Germack H. A systematic review on healthcare analytics: application and theoretical perspective of data mining. In: Healthcare. Basel: Multidisciplinary Digital Publishing Institute; 2018. p. 54.
Ismail A, Shehab A, El-Henawy IM. Healthcare analysis in smart big data analytics: reviews, challenges and recommendations. In: Security in smart cities: models, applications, and challenges. Cham: Springer; 2019. p. 27–45.
Jain N, Gupta V, Shubham S, et al. Understanding cartoon emotion using integrated deep neural network on large dataset. Neural Comput Appl. 2021. https://doi.org/10.1007/s00521-021-06003-9 .
Janssen M, van der Voort H, Wahyudi A. Factors influencing big data decision-making quality. J Bus Res. 2017;70:338–45.
Jordan SR. Beneficence and the expert bureaucracy. Public Integr. 2014;16(4):375–94. https://doi.org/10.2753/PIN1099-9922160404 .
Knapp MM. Big data. J Electron Resourc Med Libr. 2013;10(4):215–22.
Koti MS, Alamma BH. Predictive analytics techniques using big data for healthcare databases. In: Smart intelligent computing and applications. New York: Springer; 2019. p. 679–86.
Krumholz HM. Big data and new knowledge in medicine: the thinking, training, and tools needed for a learning health system. Health Aff. 2014;33(7):1163–70.
Kruse CS, Goswamy R, Raval YJ, Marawi S. Challenges and opportunities of big data in healthcare: a systematic review. JMIR Med Inform. 2016;4(4):e38.
Kyoungyoung J, Gang HK. Potentiality of big data in the medical sector: focus on how to reshape the healthcare system. Healthc Inform Res. 2013;19(2):79–85.
Laney D. Application delivery strategies 2011. http://blogs.gartner.com/doug-laney/files/2012/01/ad949-3D-Data-Management-Controlling-Data-Volume-Velocity-and-Variety.pdf .
Lee IK, Wang CC, Lin MC, Kung CT, Lan KC, Lee CT. Effective strategies to prevent coronavirus disease-2019 (COVID-19) outbreak in hospital. J Hosp Infect. 2020;105(1):102.
Lerner I, Veil R, Nguyen DP, Luu VP, Jantzen R. Revolution in health care: how will data science impact doctor-patient relationships? Front Public Health. 2018;6:99.
Lytras MD, Papadopoulou P, editors. Applying big data analytics in bioinformatics and medicine. IGI Global: Hershey; 2017.
Ma K, et al. Big data in multiple sclerosis: development of a web-based longitudinal study viewer in an imaging informatics-based eFolder system for complex data analysis and management. In: Proceedings volume 9418, medical imaging 2015: PACS and imaging informatics: next generation and innovations. 2015. p. 941809. https://doi.org/10.1117/12.2082650 .
Mach-Król M. Analiza i strategia big data w organizacjach. In: Studia i Materiały Polskiego Stowarzyszenia Zarządzania Wiedzą. 2015;74:43–55.
Madsen LB. Data-driven healthcare: how analytics and BI are transforming the industry. Hoboken: Wiley; 2014.
Manyika J, Chui M, Brown B, Bughin J, Dobbs R, Roxburgh C, Hung BA. Big data: the next frontier for innovation, competition, and productivity. Washington: McKinsey Global Institute; 2011.
Marconi K, Dobra M, Thompson C. The use of big data in healthcare. In: Liebowitz J, editor. Big data and business analytics. Boca Raton: CRC Press; 2012. p. 229–48.
Mehta N, Pandit A. Concurrence of big data analytics and healthcare: a systematic review. Int J Med Inform. 2018;114:57–65.
Michel M, Lupton D. Toward a manifesto for the ‘public understanding of big data.’ Public Underst Sci. 2016;25(1):104–16. https://doi.org/10.1177/0963662515609005 .
Mikalef P, Krogstie J. Big data analytics as an enabler of process innovation capabilities: a configurational approach. In: International conference on business process management. Cham: Springer; 2018. p. 426–41.
Mohammadi M, Al-Fuqaha A, Sorour S, Guizani M. Deep learning for IoT big data and streaming analytics: a survey. IEEE Commun Surv Tutor. 2018;20(4):2923–60.
Nambiar R, Bhardwaj R, Sethi A, Vargheese R. A look at challenges and opportunities of big data analytics in healthcare. In: 2013 IEEE international conference on big data; 2013. p. 17–22.
Ohlhorst F. Big data analytics: turning big data into big money, vol. 65. Hoboken: Wiley; 2012.
Olszak C, Mach-Król M. A conceptual framework for assessing an organization’s readiness to adopt big data. Sustainability. 2018;10(10):3734.
Olszak CM. Toward better understanding and use of business intelligence in organizations. Inf Syst Manag. 2016;33(2):105–23.
Palanisamy V, Thirunavukarasu R. Implications of big data analytics in developing healthcare frameworks—a review. J King Saud Univ Comput Inf Sci. 2017;31(4):415–25.
Provost F, Fawcett T. Data science and its relationship to big data and data-driven decisionmaking. Big Data. 2013;1(1):51–9.
Raghupathi W, Raghupathi V. An overview of health analytics. J Health Med Inform. 2013;4:132. https://doi.org/10.4172/2157-7420.1000132 .
Raghupathi W, Raghupathi V. Big data analytics in healthcare: promise and potential. Health Inf Sci Syst. 2014;2(1):3.
Ratia M, Myllärniemi J. Beyond IC 4.0: the future potential of BI-tool utilization in the private healthcare, conference: proceedings IFKAD, 2018 at: Delft, The Netherlands.
Ristevski B, Chen M. Big data analytics in medicine and healthcare. J Integr Bioinform. 2018. https://doi.org/10.1515/jib-2017-0030 .
Rumsfeld JS, Joynt KE, Maddox TM. Big data analytics to improve cardiovascular care: promise and challenges. Nat Rev Cardiol. 2016;13(6):350–9. https://doi.org/10.1038/nrcardio.2016.42 .
Schmarzo B. Big data: understanding how data powers big business. Indianapolis: Wiley; 2013.
Senthilkumar SA, Rai BK, Meshram AA, Gunasekaran A, Chandrakumarmangalam S. Big data in healthcare management: a review of literature. Am J Theor Appl Bus. 2018;4:57–69.
Shubham S, Jain N, Gupta V, et al. Identify glomeruli in human kidney tissue images using a deep learning approach. Soft Comput. 2021. https://doi.org/10.1007/s00500-021-06143-z .
Thuemmler C. The case for health 4.0. In: Thuemmler C, Bai C, editors. Health 4.0: how virtualization and big data are revolutionizing healthcare. New York: Springer; 2017.
Tsai CW, Lai CF, Chao HC, et al. Big data analytics: a survey. J Big Data. 2015;2:21. https://doi.org/10.1186/s40537-015-0030-3 .
Wamba SF, Gunasekaran A, Akter S, Ji-fan RS, Dubey R, Childe SJ. Big data analytics and firm performance: effects of dynamic capabilities. J Bus Res. 2017;70:356–65.
Wang Y, Byrd TA. Business analytics-enabled decision-making effectiveness through knowledge absorptive capacity in health care. J Knowl Manag. 2017;21(3):517–39.
Wang Y, Kung L, Wang W, Yu C, Cegielski CG. An integrated big data analytics-enabled transformation model: application to healthcare. Inf Manag. 2018;55(1):64–79.
Wicks P, et al. Scaling PatientsLikeMe via a “generalized platform” for members with chronic illness: web-based survey study of benefits arising. J Med Internet Res. 2018;20(5):e175.
Willems SM, et al. The potential use of big data in oncology. Oral Oncol. 2019;98:8–12. https://doi.org/10.1016/j.oraloncology.2019.09.003 .
Williams N, Ferdinand NP, Croft R. Project management maturity in the age of big data. Int J Manag Proj Bus. 2014;7(2):311–7.
Winters-Miner LA. Seven ways predictive analytics can improve healthcare. Medical predictive analytics have the potential to revolutionize healthcare around the world. 2014. https://www.elsevier.com/connect/seven-ways-predictive-analytics-can-improve-healthcare (Reading: 15.04.2019).
Wu J, et al. Application of big data technology for COVID-19 prevention and control in China: lessons and recommendations. J Med Internet Res. 2020;22(10): e21980.
Yan L, Peng J, Tan Y. Network dynamics: how can we find patients like us? Inf Syst Res. 2015;26(3):496–512.
Yang JJ, Li J, Mulder J, Wang Y, Chen S, Wu H, Pan H. Emerging information technologies for enhanced healthcare. Comput Ind. 2015;69:3–11.
Zhang Q, Yang LT, Chen Z, Li P. A survey on deep learning for big data. Inf Fusion. 2018;42:146–57.
Download references
Acknowledgements
We would like to thank those who have touched our science paths.
This research was fully funded as statutory activity—subsidy of Ministry of Science and Higher Education granted for Technical University of Czestochowa on maintaining research potential in 2018. Research Number: BS/PB–622/3020/2014/P. Publication fee for the paper was financed by the University of Economics in Katowice.
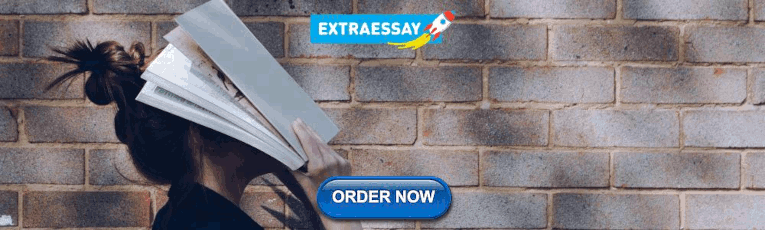
Author information
Authors and affiliations.
Department of Business Informatics, University of Economics in Katowice, Katowice, Poland
Kornelia Batko
Department of Biomedical Processes and Systems, Institute of Health and Nutrition Sciences, Częstochowa University of Technology, Częstochowa, Poland
Andrzej Ślęzak
You can also search for this author in PubMed Google Scholar
Contributions
KB proposed the concept of research and its design. The manuscript was prepared by KB with the consultation of AŚ. AŚ reviewed the manuscript for getting its fine shape. KB prepared the manuscript in the contexts such as definition of intellectual content, literature search, data acquisition, data analysis, and so on. AŚ obtained research funding. Both authors read and approved the final manuscript.
Corresponding author
Correspondence to Kornelia Batko .
Ethics declarations
Ethics approval and consent to participate.
Not applicable.
Consent for publication
Competing interests.
The author declares no conflict of interest.
Additional information
Publisher's note.
Springer Nature remains neutral with regard to jurisdictional claims in published maps and institutional affiliations.
Rights and permissions
Open Access This article is licensed under a Creative Commons Attribution 4.0 International License, which permits use, sharing, adaptation, distribution and reproduction in any medium or format, as long as you give appropriate credit to the original author(s) and the source, provide a link to the Creative Commons licence, and indicate if changes were made. The images or other third party material in this article are included in the article's Creative Commons licence, unless indicated otherwise in a credit line to the material. If material is not included in the article's Creative Commons licence and your intended use is not permitted by statutory regulation or exceeds the permitted use, you will need to obtain permission directly from the copyright holder. To view a copy of this licence, visit http://creativecommons.org/licenses/by/4.0/ .
Reprints and permissions
About this article
Cite this article.
Batko, K., Ślęzak, A. The use of Big Data Analytics in healthcare. J Big Data 9 , 3 (2022). https://doi.org/10.1186/s40537-021-00553-4
Download citation
Received : 28 August 2021
Accepted : 19 December 2021
Published : 06 January 2022
DOI : https://doi.org/10.1186/s40537-021-00553-4
Share this article
Anyone you share the following link with will be able to read this content:
Sorry, a shareable link is not currently available for this article.
Provided by the Springer Nature SharedIt content-sharing initiative
- Big Data Analytics
- Data-driven healthcare
Contemporary Issues in Communication, Cloud and Big Data Analytics
Proceedings of CCB 2020
- Conference proceedings
- © 2022
- Hiren Kumar Deva Sarma 0 ,
- Valentina Emilia Balas ORCID: https://orcid.org/0000-0003-0885-1283 1 ,
- Bhaskar Bhuyan 2 ,
- Nitul Dutta 3
Department of Information Technology, Sikkim Manipal Institute of Technology, Majitar, India
You can also search for this editor in PubMed Google Scholar
Department of Automatics and Applied Software, Aurel Vlaicu University of Arad, Arad, Romania
Department of computer science and engineering, marwadi university, rajkot, india.
- Presents research works in the field of communication, cloud and big data
- Provides original works presented at CCB 2020 held in Sikkim, India
- Serves as a reference for researchers and practitioners in academia and industry
Part of the book series: Lecture Notes in Networks and Systems (LNNS, volume 281)
31k Accesses
54 Citations
This is a preview of subscription content, log in via an institution to check access.
Access this book
- Available as EPUB and PDF
- Read on any device
- Instant download
- Own it forever
- Compact, lightweight edition
- Dispatched in 3 to 5 business days
- Free shipping worldwide - see info
Tax calculation will be finalised at checkout
Other ways to access
Licence this eBook for your library
Institutional subscriptions
Table of contents (38 papers)
Front matter, communication, reliable data delivery in software-defined networking: a survey.
- Prerna Rai, Hiren Kumar Deva Sarma
Phishing Websites, Detection and Analysis: A Survey
- Leena I. Sakri, Pushpalatha S. Nikkam, Madhuri Kulkarni, Priyanka Kamath, Shreedevi Subrahmanya Bhat, Swati Kamat
Analysis of Security Attacks in SDN Network: A Comprehensive Survey
- Ali Nadim Alhaj, Nitul Dutta
An Overview of 51% Attack Over Bitcoin Network
- Raja Siddharth Raju, Sandeep Gurung, Prativa Rai
An IPS Approach to Secure V-RSU Communication from Blackhole and Wormhole Attacks in VANET
- Gaurav Soni, Kamlesh Chandravanshi, Mahendra Ku. Jhariya, Arjun Rajput
BER Analysis of FBMC for 5G Communication
- Balwant Singh, Malay Ranjan Tripathy, Rishi Asthana
Impact of TCP-SYN Flood Attack in Cloud
- Anurag Sharma, Md. Ruhul Islam, Dhruba Ningombam
An Efficient Cooperative Caching with Request Forwarding Strategy in Information-Centric Networking
- Krishna Delvadia, Nitul Dutta
Instabilities of Consensus
- Priya Ranjan
Delay-Based Approach for Prevention of Rushing Attack in MANETs
- Ashwin Adarsh, Tshering Lhamu Tamang, Payash Pradhan, Vikash Kumar Singh, Biswaraj Sen, Kalpana Sharma
ASCTWNDN:A Simple Caching Tool for Wireless Named Data Networking
- Dependra Dhakal, Mohit Rathor, Sudipta Dey, Prantik Dey, Kalpana Sharma
Design of MIMO Cylindrical DRA’s Using Metalstrip for Enhanced Isolation with Improved Performance
- A. Jayakumar, K. Suresh Kumar, T. Ananth Kumar, S. Sundaresan
A Robust BSP Scheduler for Bioinformatics Application on Public Cloud
- Leena I. Sakri, K. S. Jagadeeshgowda
Mobile Cloud-Based Framework for Health Monitoring with Real-Time Analysis Using Machine Learning Algorithms
- Suman Mohanty, Ravi Anand, Ambarish Dutta, Venktesh Kumar, Utsav Kumar, Md. Ruhul Islam
- Big Data Analytics
Genomic Data and Big Data Analytics
- Hiren Kumar Deva Sarma
Image Processing
- Communication Networks
- Cloud Computing
- Network Security
- Cloud Computing Platform
- Big Data Open Platforms
About this book
This book presents the outcomes of the First International Conference on Communication, Cloud, and Big Data (CCB) held on December 18–19, 2020, at Sikkim Manipal Institute of Technology, Majitar, Sikkim, India. This book contains research papers and articles in the latest topics related to the fields like communication networks, cloud computing, big data analytics, and on various computing techniques. Research papers addressing security issues in above-mentioned areas are also included in the book. The research papers and articles discuss latest issues in the above-mentioned topics. The book is very much helpful and useful for the researchers, engineers, practitioners, research students, and interested readers.
Editors and Affiliations
Hiren Kumar Deva Sarma, Bhaskar Bhuyan
Valentina Emilia Balas
Nitul Dutta
About the editors
Dr. Hiren Kumar Deva Sarma is Professor in the Department of Information Technology, Sikkim Manipal Institute of Technology, Sikkim. He received Bachelor of Engineering in Mechanical Engineering from Assam Engineering College, Guwahati, Assam (1998). He completed Master of Technology in Information Technology from Tezpur University, Assam (2000). He received Doctor of Philosophy (in Computer Science & Engineering) from Jadavpur University, West Bengal (2013). He has co-authored two books, edited three book volumes, and published more than seventy research papers in different International Journals and referred International and National Conferences of repute. He is the recipient of Young Scientist Award from International Union of Radio Science (URSI) in the XVIII General Assembly 2005, held at New Delhi, India, and has received IEEE Early Adopter Award in 2014. His current research interests are networks, network security, robotics, and big data analytics.
Dr. Bhaskar Bhuyan is presently working as Associate Professor in the Department of Information Technology, Sikkim Manipal Institute of Technology affiliated to Sikkim Manipal University, Sikkim, India. He did his B.E. (1997) in Computer Science & Engineering from Motilal Nehru Regional Engineering College (now NIT), Allahabad, India. He did his M.Tech. (2000) in Information Technology and Ph.D. (2017) in Computer Science & Engineering from Tezpur University, Assam, India. He has 18+ years of professional experience in teaching as well as in industry. He has published several research papers in various conferences and journals of repute, and co-edited one book (conference proceedings). His research interests include computer networks, wireless sensor networks, mobile ad hoc networks, Internet of things, and cloud computing.
Bibliographic Information
Book Title : Contemporary Issues in Communication, Cloud and Big Data Analytics
Book Subtitle : Proceedings of CCB 2020
Editors : Hiren Kumar Deva Sarma, Valentina Emilia Balas, Bhaskar Bhuyan, Nitul Dutta
Series Title : Lecture Notes in Networks and Systems
DOI : https://doi.org/10.1007/978-981-16-4244-9
Publisher : Springer Singapore
eBook Packages : Engineering , Engineering (R0)
Copyright Information : The Editor(s) (if applicable) and The Author(s), under exclusive license to Springer Nature Singapore Pte Ltd. 2022
Softcover ISBN : 978-981-16-4243-2 Published: 02 December 2021
eBook ISBN : 978-981-16-4244-9 Published: 30 November 2021
Series ISSN : 2367-3370
Series E-ISSN : 2367-3389
Edition Number : 1
Number of Pages : XVIII, 476
Number of Illustrations : 41 b/w illustrations, 191 illustrations in colour
Topics : Communications Engineering, Networks , Professional Computing , Big Data , Computer Communication Networks
- Publish with us
Policies and ethics
- Find a journal
- Track your research
The Coolest Data Analytics Companies Of The 2024 Big Data 100
Part 1 of CRN’s Big Data 100 takes a look at the vendors solution providers should know in the data analytics and business intelligence space.

Gaining Business Insights
Data analytics, business intelligence and data visualization software are critical components of the big data technology stack. They are the tools that everyone from everyday business users to professional analysts use to gain understanding and insights from rapidly growing volumes of data and share that knowledge throughout an organization. They are, ultimately, the means of deriving value from big data.
Global business analytics software sales are expected to grow at a CAGR of more than 10 percent through 2030 when they will reach $172.59 billion, according to a Verified Market Research forecast .
As part of the CRN 2024 Big Data 100, we’ve put together the following list of data analytics and business intelligence software companies—from well-established vendors to those in startup mode—that solution providers should be familiar with.
These vendors offer everything from self-service reporting and data visualization tools for nontechnical managers and business users to high-performance data analytics software needed by analysts to tackle the most complex business intelligence tasks.
This week CRN is running the Big Data 100 list in a series of slide shows, organized by technology category, spotlighting vendors of data analytics software, database systems, data warehouse and data lake systems, data management and integration software, data observability tools, and big data systems and cloud platforms.
Some vendors have big data product portfolios that span multiple technology categories. They appear in the slideshow for the technology segment in which they are most prominent.

Top Executive: Paula Hansen, President and CRO
The flagship Alteryx AI Platform for Enterprise Analytics automates a range of data preparation, analytics and machine learning tasks with built-in data governance and security. Because of its high degree of automation, the system provides self-service capabilities to a broad range of business users.
In May 2023 the company launched Alteryx AiDIN, combining AI, generative AI, large language models and machine learning technology in one system that works with the Alteryx Analytics Cloud Platform to boost analytical productivity.
In March of this year Alteryx, previously a publicly traded company, was acquired by Clearlake Capital Group and Insight Partners in a deal valued at $4.4 billion.

Top Executive: CEO Chris Lynch
AtScale’s universal semantic layer technology sits between data sources and the tools – data science and business analytics software, spreadsheets, development tools, and AI and machine learning applications – used by “data consumers.”
By abstracting away data complexities, AtScale provides a business-oriented view of data that aids non-technical users, accelerates analytics workloads, and provides a platform for enforcing data governance and consistency.
In 2023 AtScale was named Emerging Partner of the Year by data lakehouse giant Databricks after the two companies expanded their alliance with AtScale support for Databricks’ Semantic Lakehouse architecture and Databricks Lakehouse for Manufacturing.

Top Executive: CEO James Li
CelerData offers a high-performance data lakehouse analytics system, based on the StarRocks SQL query engine, through its on-premises CelerData Enterprise software and CelerData Cloud managed service.
CelerData’s founders developed the StarRocks technology in 2020 and originally the company bore the StarRocks name. But the company changed its name to CelerData in late 2022 and in 2023 contributed the StarRocks technology to the Linux Foundation where it resides as an open-source project.

Top Executive: Josh James
The Domo Data Experience Platform is a cloud-native business intelligence and data visualization system with underlying data integration, data science and AI capabilities. Domo connects to an organization’s data sources, including cloud and on-premises operational applications, data lakes and data warehouses, to provide users with analytical reports, KPIs and data dashboards.
Domo, which reported fiscal 2024 revenue of $319 million, recently announced the general availability of App Studio, a low-code application builder for developing analysis-driven applications. The company also expanded the capabilities of its Workflows low-code automation engine.

Hex Technologies
Top Executive: CEO Barry McCardel
Startup Hex has been getting a lot of attention with the Hex platform, a modern data workspace system for collaborative analytics and data science tasks.
The company’s software includes AI-powered tools, collaborative data notebooks, tools for building applications with data visualizations, and data integration technology – all making it possible to connect and analyze data and share work using interactive data applications and stories.
Hex was founded in 2019 by McCardel, CTO Caitlin Colgrove and Chief Architect Glen Takahashi who previously worked together at Palantir. The company raised $52 million in Series B funding in March 2022.
In October Hex launched Hex 3.0 with new AI capabilities, a new compute engine, a new metadata engine and the App Builder tool for turning insights into interactive experiences. Earlier in the year the company debuted Hex Magic tools that bring the power of large language models directly into the Hex workspace.

Hitachi Vantara
Top Executive: CEO Sheila Rohra
Hitachi Vantara is the data storage, infrastructure and hybrid cloud management subsidiary of Hitachi Ltd.
Hitachi Vantara offers what it calls “intelligent data platforms” that include products for analytics, storage, data management, IoT, data operations and protection, and more. The DataOps Platform Software lineup includes Pentaho Data Integration & Analytics, Pentaho Data Catalog, and Pentaho Data Storage Optimizer.

Top Executive: CEO Osama Elkady
Incorta offers a unified data and analytics delivery platform for acquiring, processing, analyzing and presenting decision-ready data. More recently Incorta has positioned its technology as an operational data lakehouse system for data applications and AI and machine learning tasks. It provides data connectors for linking to operational applications and the company’s Direct Data Mapping tools for analytical queries.
In January the company debuted Incorta X, a data analytics system that integrates advanced AI and ML functions with organizational data from multiple sources to speed up analytical tasks.

Top Executive: CEO Luke Han
Kyligence offers data analytics products – including Kyligence Enterprise – powered by Apache Kylin, an open-source OLAP engine for interactive analytics of petabyte-scale data.
In 2023 the company expanded its analytics offerings with Kyligence Zen , an intelligent metrics platform for developing and centralizing all types of business management data metrics into a unified catalog system.

Kyvos Insights
Top Executive: CEO Praveen Kankariya
Kyvos provides a cloud-native, high-speed data analytics system that the company says enables sub-second querying on massive datasets.
A key component of the Kyvos Cloud offering is its universal semantic layer that provides a unified view of business data, no matter the source, standardizes data interpretation and delivers a single source of trusted data.
The platform’s GenAI capabilities provides users with self-service access and helps them interact with metrics in business language.

MicroStrategy
Top Executive: President and CEO Phong Lee
MicroStrategy is one of the long-time leaders in the business analytics space, going to market today with its flagship MicroStrategy One AI/BI platform.
In October the company launched MicroStrategy AI, an addition to its core platform that allows organizations to incorporate generative AI into their data applications. The new software includes Auto Answers self-service analytics, Auto Dashboard for designing dashboards through AI automation, Auto SQL for streamlining database query processes, and Auto Expert for accessing MicroStrategy resources and learning materials.
In September the company expanded its partner program with new partner training and enablement resources, enhanced sales motions and partner incentives, and partner development funds.
Publicly held MicroStrategy reported revenue of $496.3 million in 2023.

Top Executive: CEO Jordan Tigani
Startup MotherDuck launched the first release of its serverless MotherDuck Cloud Analytics Platform in June 2023, combining cloud and embedded database technology to make it easy to analyze data no matter where it resides.
MotherDuck’s software is based on the company’s DuckDB open-source, embeddable database. The cloud system simplifies the analysis of data of any size by combining the speed of an in-process database with the scalability of the cloud, according to the company.
MotherDuck makes the argument that most advances in data analysis in recent years have been geared toward large businesses and organizations with more than a petabyte of data while neglecting small and mid-size companies with like-sized data volumes.
MotherDuck, based in Seattle, was co-founded in 2022 by Google BigQuery founding engineer Jordan Tigani who today is the company’s CEO. In September the company raised $52.5 million in Series B funding, boosting its total financing to $100 million.

Top Executive: CEO Kaycee Lai
Promethium describes its software as the industry’s “first AI-native data fabric platform” that provides a single, unified, consistent view of – and access to – all data from across multiple sources.
Earlier this month the company shipped Promethium Revision18, a new release with new features and enhancements that streamline workflows, improve data governance and provide deeper insights for data engineers and chief data officers.

Pyramid Analytics
Top Executive: CEO Omri Kohl
Pyramid Analytics develops a business and decision intelligence platform that combines data preparation, business analytics and data science functionality in one system.
In March the company launched what it called the first “generative BI” solution through the combination of GenAI-based conversational analytics software – analytics initiated by speech – and its flagship platform. Conversational analytics provides non-technical users with “true self-service analytics” capabilities, the company said.
Headquartered in Amsterdam, The Netherlands, Pyramid also has offices in Tel Aviv, Israel and in New York and is looking to expand its presence in North America.

Top Executive: CEO Mike Capone
Qlik is a major player in both the data analytics and data integration technology spaces, allowing the company to offer a complete portfolio of tools for data preparation and analysis.
On the analytics side the company offers its flagship Qlik Sense on-premises analytics software, today targeted toward highly regulated industries. Qlik Cloud Analytics is the company’s popular cloud-based SaaS offering.
Data integration and data quality management have become a big part of Qlik’s technology portfolio – especially after its May 2023 acquisition of Talend . Qlik’s extensive product lineup today includes Qlik Cloud Data Integration and Qlik Replicate, Qlik Compose for building data lakes and data warehouses, and Talend’s tools for data preparation, inventory, catalog, stewardship, and more.
In January Qlik expanded its ability to work with unstructured data by acquiring patents and technology from Kyndi.

Top Executive: CEO Venkat Venkataramani
Rockset develops a data search and analytics database that the company says is built for the cloud, offers real-time streaming data ingest and indexing capabilities, with full-featured SQL on JSON, time series, geospatial and vector data functionality.
In November Rockset boosted its software’s AI capabilities by expanding its vector search capabilities with Approximate Nearest Neighbor (ANN) search and by supporting the LangChain and LlamaIndex development frameworks.
Rockset, headquartered in San Mateo, Calif., raised $44 million in funding in August 2023, bringing its total financing to $105 million.

Top Executive: CEO Marc Benioff
CRM application giant Salesforce acquired Tableau, one of the long-time leaders in data visual analytics, in 2019. Since then, Salesforce has been integrating Tableau with its other applications through the Data Cloud for Tableau.
But Salesforce continues to market individual Tableau products such as Tableau Desktop, Tableau Server and Tableau Prep.
On April 2 Salesforce announced the beta availability of Einstein Copilot for Tableau, an AI assistant that helps business users with self-service analytics and streamlines analyst workflows. (Einstein is a set of AI technologies for the Salesforce CRM platform.)

Top Executive: CEO Jim Goodnight
SAS is one of the biggest companies in the IT industry focused specifically on data analytics, AI and related technologies. SAS Viya is the company’s flagship analytics and AI platform and the company offers a broad range of analytical software for specific tasks (such as marketing, fraud detection and risk management) and for vertical industries (including banking, life science, public sector and retail/CPG).
SAS has been privately held since its 1976 founding, although the company has taken steps in recent years toward an IPO.
The company has been stepping up its channel efforts including expanding its alliance with Carahsoft in February to boost sales to U.S. government agencies.
Last week at the SAS Innovate conference the company expanded the Viya platform with new generative AI and large language model orchestration capabilities, announced the general availability of the SAS Viya Workbench developer environment for building AI models, and advanced its industry-specific solutions with packaged AI models.

Top Executive: CEO Ariel Katz
Sisense markets embedded analytics technology that software developers use to build data analytics and AI functionality directly into their applications. The company’s product portfolio includes the Sisense Platform and Sisense Fusion Embed for infusing analytics functionality into products or services.
In March Sisense announced the general availability of Compose SDK for Fusion, a developer toolkit that the company “enables the delivery of customized data experiences using a code-first, scalable and modular approach.” With the SDK developers can use Sisense’s API-first platform to create dynamic queries, charts and filters directly from application code.

ThoughtSpot
Top Executive: CEO Sudheesh Nair
ThoughtSpot has been one of the fastest growing data analytics companies in recent years, especially after it made a major pivot to the cloud in 2020 with a software-as-a-service version of its ThoughtSpot analytics platform.
The company’s AI-powered ThoughtSpot Analytics platform uses natural language capabilities and a search-based user interface to bring data analytics to a wide audience of users. The company’s product portfolio includes ThoughtSpot Everywhere for embedded analytics and ThoughtSpot Sage, which combines the company’s search technology with large language models.
In July 2023 ThoughtSpot acquired business intelligence software developer Mode Analytics in a move to expand its customer base, accelerate annual recurring revenue and deepen its technology portfolio for data analysts and data engineers.

Tibco, A Business Unit of Cloud Software Group
Top Executive: CEO Tom Krause
Cloud Software Group was created in September 2022 through the merger of Citrix Systems and Tibco Software.
Today the Tibco Platform offers a range of business intelligence, data management, integration and virtualization tools – products that Tibco assembled through a series of earlier acquisitions. The platform runs in the cloud, on-premises and at the edge.
Numbers, Facts and Trends Shaping Your World
Read our research on:
Full Topic List
Regions & Countries
- Publications
- Our Methods
- Short Reads
- Tools & Resources
Read Our Research On:
What the data says about crime in the U.S.
A growing share of Americans say reducing crime should be a top priority for the president and Congress to address this year. Around six-in-ten U.S. adults (58%) hold that view today, up from 47% at the beginning of Joe Biden’s presidency in 2021.
We conducted this analysis to learn more about U.S. crime patterns and how those patterns have changed over time.
The analysis relies on statistics published by the FBI, which we accessed through the Crime Data Explorer , and the Bureau of Justice Statistics (BJS), which we accessed through the National Crime Victimization Survey data analysis tool .
To measure public attitudes about crime in the U.S., we relied on survey data from Pew Research Center and Gallup.
Additional details about each data source, including survey methodologies, are available by following the links in the text of this analysis.
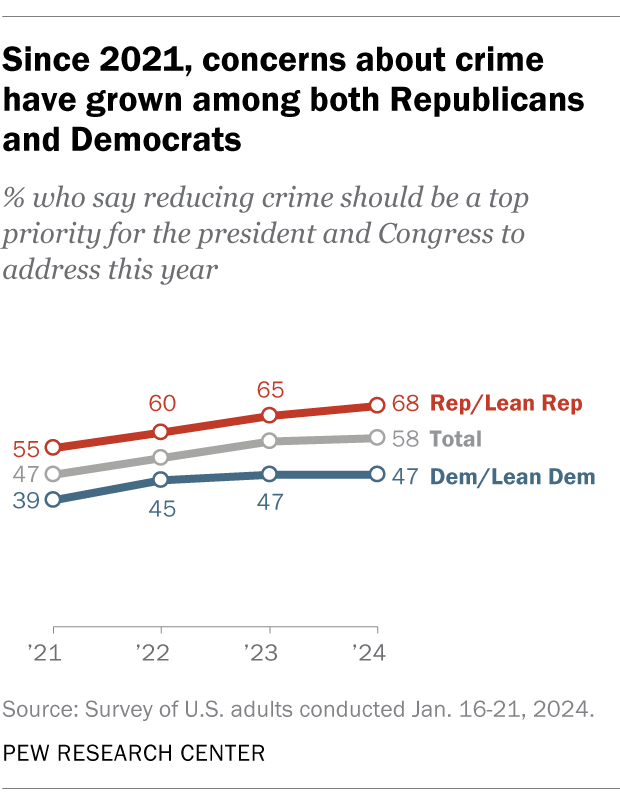
With the issue likely to come up in this year’s presidential election, here’s what we know about crime in the United States, based on the latest available data from the federal government and other sources.
How much crime is there in the U.S.?
It’s difficult to say for certain. The two primary sources of government crime statistics – the Federal Bureau of Investigation (FBI) and the Bureau of Justice Statistics (BJS) – paint an incomplete picture.
The FBI publishes annual data on crimes that have been reported to law enforcement, but not crimes that haven’t been reported. Historically, the FBI has also only published statistics about a handful of specific violent and property crimes, but not many other types of crime, such as drug crime. And while the FBI’s data is based on information from thousands of federal, state, county, city and other police departments, not all law enforcement agencies participate every year. In 2022, the most recent full year with available statistics, the FBI received data from 83% of participating agencies .
BJS, for its part, tracks crime by fielding a large annual survey of Americans ages 12 and older and asking them whether they were the victim of certain types of crime in the past six months. One advantage of this approach is that it captures both reported and unreported crimes. But the BJS survey has limitations of its own. Like the FBI, it focuses mainly on a handful of violent and property crimes. And since the BJS data is based on after-the-fact interviews with crime victims, it cannot provide information about one especially high-profile type of offense: murder.
All those caveats aside, looking at the FBI and BJS statistics side-by-side does give researchers a good picture of U.S. violent and property crime rates and how they have changed over time. In addition, the FBI is transitioning to a new data collection system – known as the National Incident-Based Reporting System – that eventually will provide national information on a much larger set of crimes , as well as details such as the time and place they occur and the types of weapons involved, if applicable.
Which kinds of crime are most and least common?
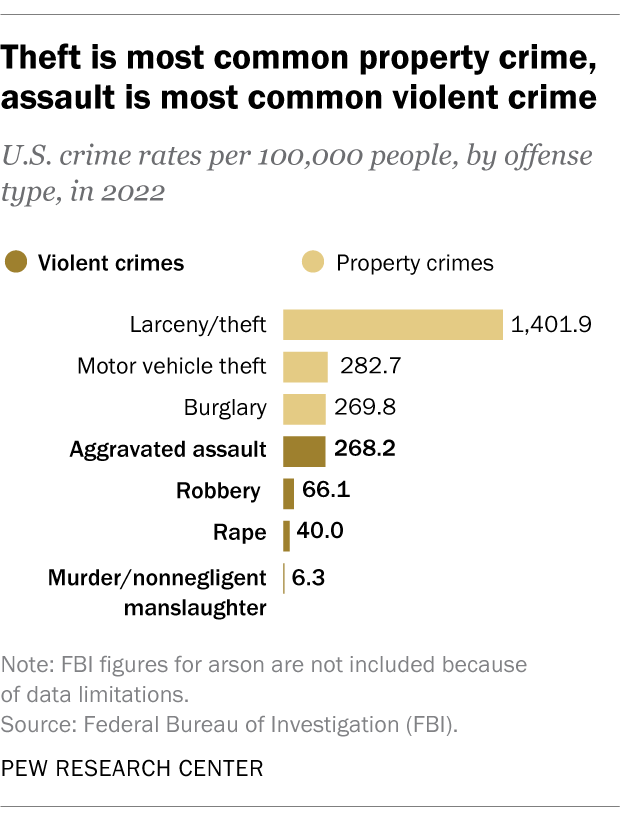
Property crime in the U.S. is much more common than violent crime. In 2022, the FBI reported a total of 1,954.4 property crimes per 100,000 people, compared with 380.7 violent crimes per 100,000 people.
By far the most common form of property crime in 2022 was larceny/theft, followed by motor vehicle theft and burglary. Among violent crimes, aggravated assault was the most common offense, followed by robbery, rape, and murder/nonnegligent manslaughter.
BJS tracks a slightly different set of offenses from the FBI, but it finds the same overall patterns, with theft the most common form of property crime in 2022 and assault the most common form of violent crime.
How have crime rates in the U.S. changed over time?
Both the FBI and BJS data show dramatic declines in U.S. violent and property crime rates since the early 1990s, when crime spiked across much of the nation.
Using the FBI data, the violent crime rate fell 49% between 1993 and 2022, with large decreases in the rates of robbery (-74%), aggravated assault (-39%) and murder/nonnegligent manslaughter (-34%). It’s not possible to calculate the change in the rape rate during this period because the FBI revised its definition of the offense in 2013 .
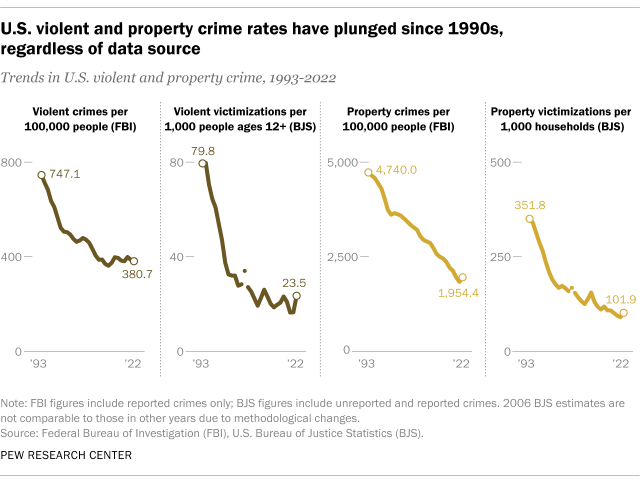
The FBI data also shows a 59% reduction in the U.S. property crime rate between 1993 and 2022, with big declines in the rates of burglary (-75%), larceny/theft (-54%) and motor vehicle theft (-53%).
Using the BJS statistics, the declines in the violent and property crime rates are even steeper than those captured in the FBI data. Per BJS, the U.S. violent and property crime rates each fell 71% between 1993 and 2022.
While crime rates have fallen sharply over the long term, the decline hasn’t always been steady. There have been notable increases in certain kinds of crime in some years, including recently.
In 2020, for example, the U.S. murder rate saw its largest single-year increase on record – and by 2022, it remained considerably higher than before the coronavirus pandemic. Preliminary data for 2023, however, suggests that the murder rate fell substantially last year .
How do Americans perceive crime in their country?
Americans tend to believe crime is up, even when official data shows it is down.
In 23 of 27 Gallup surveys conducted since 1993 , at least 60% of U.S. adults have said there is more crime nationally than there was the year before, despite the downward trend in crime rates during most of that period.
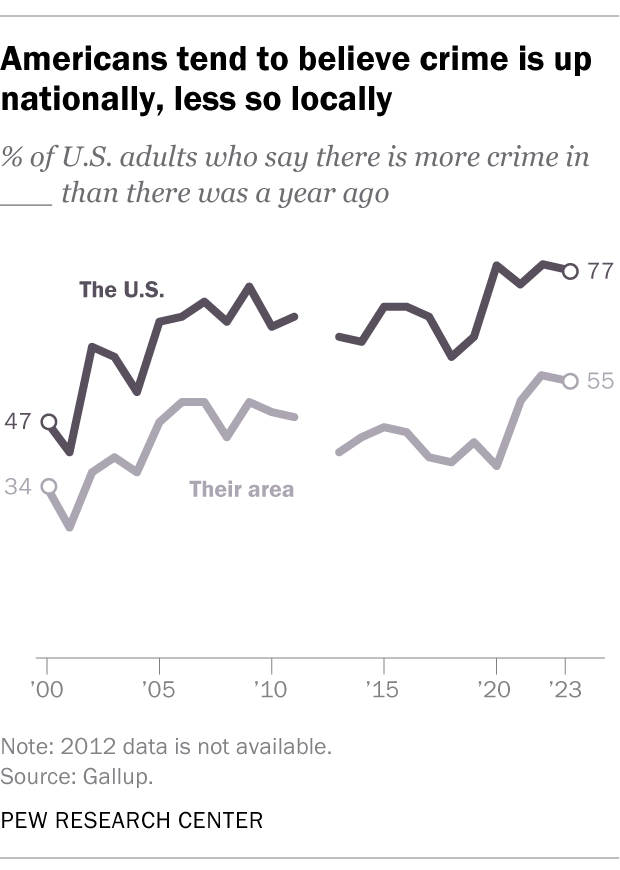
While perceptions of rising crime at the national level are common, fewer Americans believe crime is up in their own communities. In every Gallup crime survey since the 1990s, Americans have been much less likely to say crime is up in their area than to say the same about crime nationally.
Public attitudes about crime differ widely by Americans’ party affiliation, race and ethnicity, and other factors . For example, Republicans and Republican-leaning independents are much more likely than Democrats and Democratic leaners to say reducing crime should be a top priority for the president and Congress this year (68% vs. 47%), according to a recent Pew Research Center survey.
How does crime in the U.S. differ by demographic characteristics?
Some groups of Americans are more likely than others to be victims of crime. In the 2022 BJS survey , for example, younger people and those with lower incomes were far more likely to report being the victim of a violent crime than older and higher-income people.
There were no major differences in violent crime victimization rates between male and female respondents or between those who identified as White, Black or Hispanic. But the victimization rate among Asian Americans (a category that includes Native Hawaiians and other Pacific Islanders) was substantially lower than among other racial and ethnic groups.
The same BJS survey asks victims about the demographic characteristics of the offenders in the incidents they experienced.
In 2022, those who are male, younger people and those who are Black accounted for considerably larger shares of perceived offenders in violent incidents than their respective shares of the U.S. population. Men, for instance, accounted for 79% of perceived offenders in violent incidents, compared with 49% of the nation’s 12-and-older population that year. Black Americans accounted for 25% of perceived offenders in violent incidents, about twice their share of the 12-and-older population (12%).
As with all surveys, however, there are several potential sources of error, including the possibility that crime victims’ perceptions about offenders are incorrect.
How does crime in the U.S. differ geographically?
There are big geographic differences in violent and property crime rates.
For example, in 2022, there were more than 700 violent crimes per 100,000 residents in New Mexico and Alaska. That compares with fewer than 200 per 100,000 people in Rhode Island, Connecticut, New Hampshire and Maine, according to the FBI.
The FBI notes that various factors might influence an area’s crime rate, including its population density and economic conditions.
What percentage of crimes are reported to police? What percentage are solved?
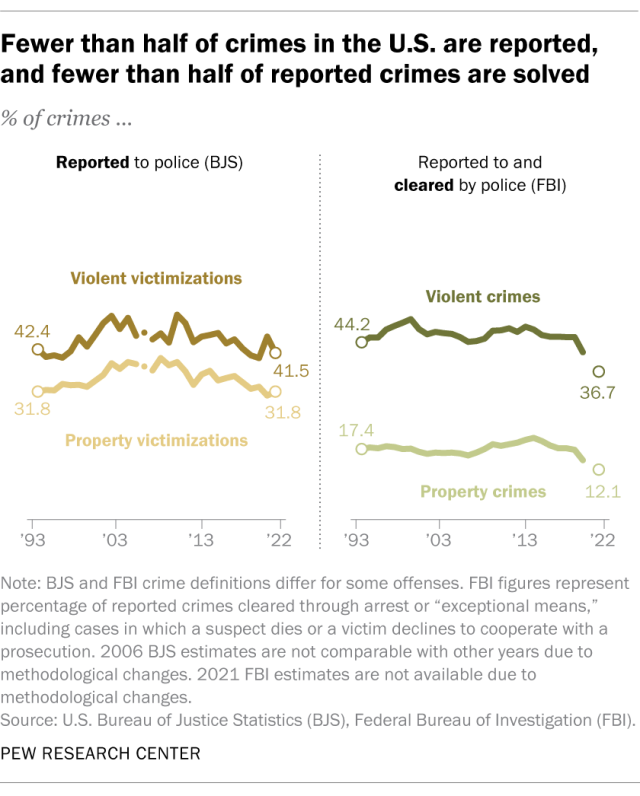
Most violent and property crimes in the U.S. are not reported to police, and most of the crimes that are reported are not solved.
In its annual survey, BJS asks crime victims whether they reported their crime to police. It found that in 2022, only 41.5% of violent crimes and 31.8% of household property crimes were reported to authorities. BJS notes that there are many reasons why crime might not be reported, including fear of reprisal or of “getting the offender in trouble,” a feeling that police “would not or could not do anything to help,” or a belief that the crime is “a personal issue or too trivial to report.”
Most of the crimes that are reported to police, meanwhile, are not solved , at least based on an FBI measure known as the clearance rate . That’s the share of cases each year that are closed, or “cleared,” through the arrest, charging and referral of a suspect for prosecution, or due to “exceptional” circumstances such as the death of a suspect or a victim’s refusal to cooperate with a prosecution. In 2022, police nationwide cleared 36.7% of violent crimes that were reported to them and 12.1% of the property crimes that came to their attention.
Which crimes are most likely to be reported to police? Which are most likely to be solved?
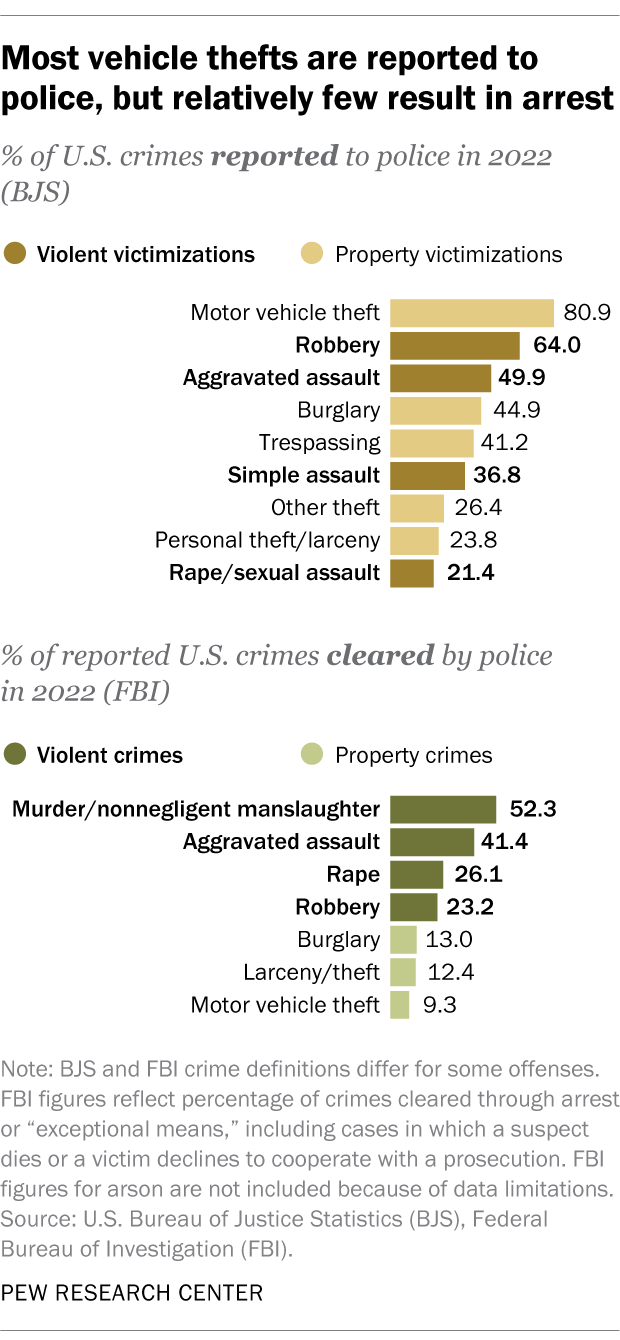
Around eight-in-ten motor vehicle thefts (80.9%) were reported to police in 2022, making them by far the most commonly reported property crime tracked by BJS. Household burglaries and trespassing offenses were reported to police at much lower rates (44.9% and 41.2%, respectively), while personal theft/larceny and other types of theft were only reported around a quarter of the time.
Among violent crimes – excluding homicide, which BJS doesn’t track – robbery was the most likely to be reported to law enforcement in 2022 (64.0%). It was followed by aggravated assault (49.9%), simple assault (36.8%) and rape/sexual assault (21.4%).
The list of crimes cleared by police in 2022 looks different from the list of crimes reported. Law enforcement officers were generally much more likely to solve violent crimes than property crimes, according to the FBI.
The most frequently solved violent crime tends to be homicide. Police cleared around half of murders and nonnegligent manslaughters (52.3%) in 2022. The clearance rates were lower for aggravated assault (41.4%), rape (26.1%) and robbery (23.2%).
When it comes to property crime, law enforcement agencies cleared 13.0% of burglaries, 12.4% of larcenies/thefts and 9.3% of motor vehicle thefts in 2022.
Are police solving more or fewer crimes than they used to?
Nationwide clearance rates for both violent and property crime are at their lowest levels since at least 1993, the FBI data shows.
Police cleared a little over a third (36.7%) of the violent crimes that came to their attention in 2022, down from nearly half (48.1%) as recently as 2013. During the same period, there were decreases for each of the four types of violent crime the FBI tracks:
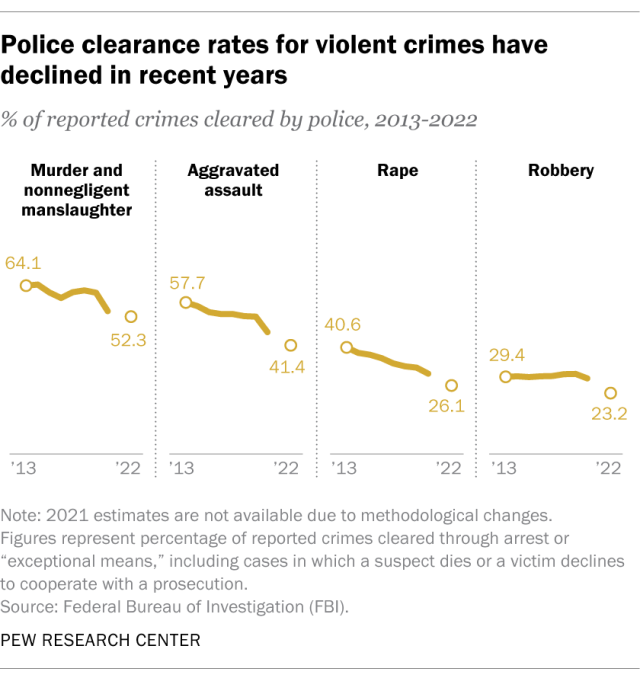
- Police cleared 52.3% of reported murders and nonnegligent homicides in 2022, down from 64.1% in 2013.
- They cleared 41.4% of aggravated assaults, down from 57.7%.
- They cleared 26.1% of rapes, down from 40.6%.
- They cleared 23.2% of robberies, down from 29.4%.
The pattern is less pronounced for property crime. Overall, law enforcement agencies cleared 12.1% of reported property crimes in 2022, down from 19.7% in 2013. The clearance rate for burglary didn’t change much, but it fell for larceny/theft (to 12.4% in 2022 from 22.4% in 2013) and motor vehicle theft (to 9.3% from 14.2%).
Note: This is an update of a post originally published on Nov. 20, 2020.
- Criminal Justice
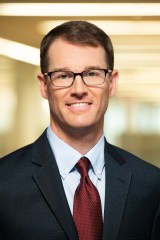
John Gramlich is an associate director at Pew Research Center
8 facts about Black Lives Matter
#blacklivesmatter turns 10, support for the black lives matter movement has dropped considerably from its peak in 2020, fewer than 1% of federal criminal defendants were acquitted in 2022, before release of video showing tyre nichols’ beating, public views of police conduct had improved modestly, most popular.
1615 L St. NW, Suite 800 Washington, DC 20036 USA (+1) 202-419-4300 | Main (+1) 202-857-8562 | Fax (+1) 202-419-4372 | Media Inquiries
Research Topics
- Age & Generations
- Coronavirus (COVID-19)
- Economy & Work
- Family & Relationships
- Gender & LGBTQ
- Immigration & Migration
- International Affairs
- Internet & Technology
- Methodological Research
- News Habits & Media
- Non-U.S. Governments
- Other Topics
- Politics & Policy
- Race & Ethnicity
- Email Newsletters
ABOUT PEW RESEARCH CENTER Pew Research Center is a nonpartisan fact tank that informs the public about the issues, attitudes and trends shaping the world. It conducts public opinion polling, demographic research, media content analysis and other empirical social science research. Pew Research Center does not take policy positions. It is a subsidiary of The Pew Charitable Trusts .
Copyright 2024 Pew Research Center
Terms & Conditions
Privacy Policy
Cookie Settings
Reprints, Permissions & Use Policy
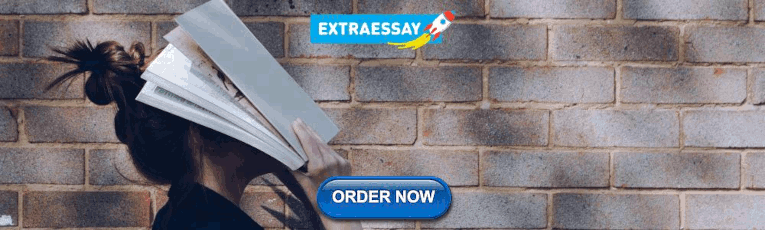
IMAGES
VIDEO
COMMENTS
Of the 70 papers satisfying our selection criteria, publication year and type (journal or conference paper) reveal an increasing trend in big data analytics over the last 6 years (Table 6). Additionally, journals produced more BDA papers than Conference proceedings (Fig. 2 ), which may be affected during 2020-2021 because of COVID, and fewer ...
In this paper, we demonstrate how big data analytics meets social media, and a comprehensive review is provided on big data analytic approaches in social networks to search published studies between 2013 and August 2020, with 74 identified papers. ... The need for an SLR is to identify, classify, and compare the existing research reviews on big ...
Big data is concerned with voluminous, complex, highly unstructured data produced from numerous sources. It is expanding at immense rate these days and is a crucial issue to handle and manage the data for the analysis of required information to save both time and cost. The data extracted can be useful for the organization in various aspects. A lot of decisions have to be taken by business ...
Big data analytics (BDA) in supply chain management (SCM) is receiving a growing attention. This is due to the fact that BDA has a wide range of applications in SCM, including customer behavior analysis, trend analysis, and demand prediction. In this survey, we investigate the predictive BDA applications in supply chain demand forecasting to propose a classification of these applications ...
2.1. Characteristics of big data. The concept of BDA overarches several data-intensive approaches to the analysis and synthesis of large-scale data (Galetsi, Katsaliaki, and Kumar Citation 2020; Mergel, Rethemeyer, and Isett Citation 2016).Such large-scale data derived from information exchange among different systems is often termed 'big data' (Bahri et al. Citation 2018; Khanra, Dhir ...
Initially, a descriptive analysis of the exported '.bib' file from 2006 to 2020 was conducted and is shown in Table 1. Fig. 2 illustrates the distribution of the corpus by article type. Out of 2160 journal papers, 1787 are research articles, 5 are both articles and book chapters, 49 are early access articles, 50 are both articles and conference proceedings, 1 Correction, 134 Editorial ...
The Journal of Big Data publishes open-access original research on data science and data analytics. Deep learning algorithms and all applications of big data are welcomed. Survey papers and case studies are also considered. The journal examines the challenges facing big data today and going forward including, but not limited to: data capture ...
The journal aims to promote and communicate advances in big data research by providing a fast and high quality forum for researchers, practitioners and policy makers from the very many different communities working on, and with, this topic. The journal will accept papers on foundational aspects in … View full aims & scope $2760
IEEE Big Data 2020 Accepted Papers. 1. Big Data Science and Foundations. Paper ID. Regular Papers. BigD273. "Connecting MapReduce Computations to Realistic Machine Models" Peter Sanders. BigD274. ""To Tell You the Truth" by Interval-Private Data" Jie Ding and Bangjun Ding.
The Internet of Things (IoT) is a communication paradigm and a collection of heterogeneous interconnected devices. It produces large-scale distributed, and diverse data called big data. Big Data Management (BDM) in IoT is used for knowledge discovery and intelligent decision-making and is one of the most significant research challenges today. There are several mechanisms and technologies for ...
The advent of healthcare information management systems (HIMSs) continues to produce large volumes of healthcare data for patient care and compliance and regulatory requirements at a global scale. Analysis of this big data allows for boundless potential outcomes for discovering knowledge. Big data analytics (BDA) in healthcare can, for instance, help determine causes of diseases, generate ...
Abstract. Big Data refers to a huge volume of data of various types, i.e., structured, semi structured, and unstructured. This data is generated through various digital channels such as mobile, Internet, social media, e-commerce websites, etc. Big Data has proven to be of great use since its inception, as companies started realizing its importance for various business purposes.
Particularly, each scientist summarizes his thoughts regarding the following two aspects: − the top future research challenges in Big Data visualiza-tion and analytics. − the top emerging applications in the context of Big Data visualization and analytics. We present their responses in the following sections, while the challenges are ...
For this special issue of the Journal of Business Analytics, we invited about a dozen papers from the track Business Analytics and Big Data at ECIS 2020. They comprised the best reviewed papers, the most suitable topics given the theme of the track and journal, as well as the most engaging discussion at the virtual conference.
The aim of Big Data analytics for safety is to obtain information that can be activated in real time. While Big Data analytics have a lot of promise, they still have a way to achieve full potential. Numerous security procedures were submitted for Big Data Analytics. A particular protocol should be used because of the safety issues and the ...
In brief, existing papers have neglected research on BDAC antecedents or restated generic resources from prior works as the majority of papers published in 2020, 2021, and 2022 do not examine this factor. ... Ramadan et al. (2020) "Big data analytics capabilities refer to the firm's ability to recognize and analyze different data sources to ...
Research papers related to descriptive analytical approach have implemented mathematical model techniques, data mining techniques, and descriptive statistic techniques. ... L. Xu, P. Dhamija, and Y. Kayikci. 2020. "Big Data Analytics as an Operational Excellence Approach to Enhance Sustainable Supply Chain Performance." Resources ...
Data science and big data analytics (DS &BDA) methodologies and tools are used extensively in supply chains and logistics (SC &L). However, the existing insights are scattered over different literature sources and there is a lack of a structured and unbiased review methodology to systematise DS &BDA application areas in the SC &L comprehensively covering efficiency, resilience and ...
The need for an SLR is to identify, classify, and compare the existing research reviews on big data analytics in social networks. In order to show that a comprehensive SLR has not been already proposed, we searched Google Scholar with the following search string: ... and selected 74 papers between 2013 and August 2020, from among 785 papers in ...
The introduction of Big Data Analytics (BDA) in healthcare will allow to use new technologies both in treatment of patients and health management. The paper aims at analyzing the possibilities of using Big Data Analytics in healthcare. The research is based on a critical analysis of the literature, as well as the presentation of selected results of direct research on the use of Big Data ...
This book contains research papers and articles in the latest topics related to the fields like communication networks, cloud computing, big data analytics, and on various computing techniques. Research papers addressing security issues in above-mentioned areas are also included in the book.
3. Issues and Challenges. Challenges in big data can be broadly alienated in to three types the first type is data challenges, the. second type is data process challenges, and t he third type are ...
The superiority of big data has led to ample research on big data analytics in the hospitality and tourism context. It is thus important to capture the overall intellectual landscape by reviewing extant relevant literature. ... (e.g., Li, Meng and Pan, 2020) - can come from the UGC data. ... Main data type Research theme Example paper ...
By publishing and sharing these papers, we are able to further expand the impact of our research, promote communication and collaboration among the academic community, and provide valuable references for future research. ... Big Data Analytics, Smart Grid, Electrical Traction Systems and Controls, Mechanical and Electrical Integration, etc. We ...
Domo. Top Executive: Josh James. The Domo Data Experience Platform is a cloud-native business intelligence and data visualization system with underlying data integration, data science and AI ...
The FBI data also shows a 59% reduction in the U.S. property crime rate between 1993 and 2022, with big declines in the rates of burglary (-75%), larceny/theft (-54%) and motor vehicle theft (-53%). Using the BJS statistics, the declines in the violent and property crime rates are even steeper than those captured in the FBI data.