- Privacy Policy

Home » What is a Hypothesis – Types, Examples and Writing Guide
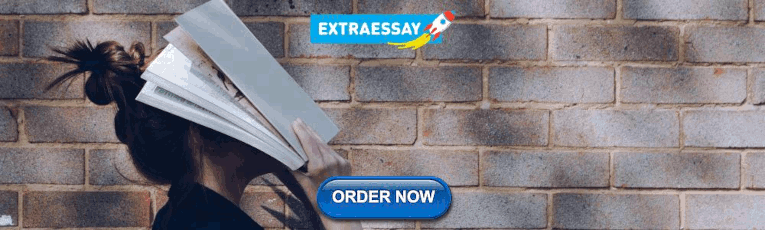
What is a Hypothesis – Types, Examples and Writing Guide
Table of Contents

Definition:
Hypothesis is an educated guess or proposed explanation for a phenomenon, based on some initial observations or data. It is a tentative statement that can be tested and potentially proven or disproven through further investigation and experimentation.
Hypothesis is often used in scientific research to guide the design of experiments and the collection and analysis of data. It is an essential element of the scientific method, as it allows researchers to make predictions about the outcome of their experiments and to test those predictions to determine their accuracy.
Types of Hypothesis
Types of Hypothesis are as follows:
Research Hypothesis
A research hypothesis is a statement that predicts a relationship between variables. It is usually formulated as a specific statement that can be tested through research, and it is often used in scientific research to guide the design of experiments.
Null Hypothesis
The null hypothesis is a statement that assumes there is no significant difference or relationship between variables. It is often used as a starting point for testing the research hypothesis, and if the results of the study reject the null hypothesis, it suggests that there is a significant difference or relationship between variables.
Alternative Hypothesis
An alternative hypothesis is a statement that assumes there is a significant difference or relationship between variables. It is often used as an alternative to the null hypothesis and is tested against the null hypothesis to determine which statement is more accurate.
Directional Hypothesis
A directional hypothesis is a statement that predicts the direction of the relationship between variables. For example, a researcher might predict that increasing the amount of exercise will result in a decrease in body weight.
Non-directional Hypothesis
A non-directional hypothesis is a statement that predicts the relationship between variables but does not specify the direction. For example, a researcher might predict that there is a relationship between the amount of exercise and body weight, but they do not specify whether increasing or decreasing exercise will affect body weight.
Statistical Hypothesis
A statistical hypothesis is a statement that assumes a particular statistical model or distribution for the data. It is often used in statistical analysis to test the significance of a particular result.
Composite Hypothesis
A composite hypothesis is a statement that assumes more than one condition or outcome. It can be divided into several sub-hypotheses, each of which represents a different possible outcome.
Empirical Hypothesis
An empirical hypothesis is a statement that is based on observed phenomena or data. It is often used in scientific research to develop theories or models that explain the observed phenomena.
Simple Hypothesis
A simple hypothesis is a statement that assumes only one outcome or condition. It is often used in scientific research to test a single variable or factor.
Complex Hypothesis
A complex hypothesis is a statement that assumes multiple outcomes or conditions. It is often used in scientific research to test the effects of multiple variables or factors on a particular outcome.
Applications of Hypothesis
Hypotheses are used in various fields to guide research and make predictions about the outcomes of experiments or observations. Here are some examples of how hypotheses are applied in different fields:
- Science : In scientific research, hypotheses are used to test the validity of theories and models that explain natural phenomena. For example, a hypothesis might be formulated to test the effects of a particular variable on a natural system, such as the effects of climate change on an ecosystem.
- Medicine : In medical research, hypotheses are used to test the effectiveness of treatments and therapies for specific conditions. For example, a hypothesis might be formulated to test the effects of a new drug on a particular disease.
- Psychology : In psychology, hypotheses are used to test theories and models of human behavior and cognition. For example, a hypothesis might be formulated to test the effects of a particular stimulus on the brain or behavior.
- Sociology : In sociology, hypotheses are used to test theories and models of social phenomena, such as the effects of social structures or institutions on human behavior. For example, a hypothesis might be formulated to test the effects of income inequality on crime rates.
- Business : In business research, hypotheses are used to test the validity of theories and models that explain business phenomena, such as consumer behavior or market trends. For example, a hypothesis might be formulated to test the effects of a new marketing campaign on consumer buying behavior.
- Engineering : In engineering, hypotheses are used to test the effectiveness of new technologies or designs. For example, a hypothesis might be formulated to test the efficiency of a new solar panel design.
How to write a Hypothesis
Here are the steps to follow when writing a hypothesis:
Identify the Research Question
The first step is to identify the research question that you want to answer through your study. This question should be clear, specific, and focused. It should be something that can be investigated empirically and that has some relevance or significance in the field.
Conduct a Literature Review
Before writing your hypothesis, it’s essential to conduct a thorough literature review to understand what is already known about the topic. This will help you to identify the research gap and formulate a hypothesis that builds on existing knowledge.
Determine the Variables
The next step is to identify the variables involved in the research question. A variable is any characteristic or factor that can vary or change. There are two types of variables: independent and dependent. The independent variable is the one that is manipulated or changed by the researcher, while the dependent variable is the one that is measured or observed as a result of the independent variable.
Formulate the Hypothesis
Based on the research question and the variables involved, you can now formulate your hypothesis. A hypothesis should be a clear and concise statement that predicts the relationship between the variables. It should be testable through empirical research and based on existing theory or evidence.
Write the Null Hypothesis
The null hypothesis is the opposite of the alternative hypothesis, which is the hypothesis that you are testing. The null hypothesis states that there is no significant difference or relationship between the variables. It is important to write the null hypothesis because it allows you to compare your results with what would be expected by chance.
Refine the Hypothesis
After formulating the hypothesis, it’s important to refine it and make it more precise. This may involve clarifying the variables, specifying the direction of the relationship, or making the hypothesis more testable.
Examples of Hypothesis
Here are a few examples of hypotheses in different fields:
- Psychology : “Increased exposure to violent video games leads to increased aggressive behavior in adolescents.”
- Biology : “Higher levels of carbon dioxide in the atmosphere will lead to increased plant growth.”
- Sociology : “Individuals who grow up in households with higher socioeconomic status will have higher levels of education and income as adults.”
- Education : “Implementing a new teaching method will result in higher student achievement scores.”
- Marketing : “Customers who receive a personalized email will be more likely to make a purchase than those who receive a generic email.”
- Physics : “An increase in temperature will cause an increase in the volume of a gas, assuming all other variables remain constant.”
- Medicine : “Consuming a diet high in saturated fats will increase the risk of developing heart disease.”
Purpose of Hypothesis
The purpose of a hypothesis is to provide a testable explanation for an observed phenomenon or a prediction of a future outcome based on existing knowledge or theories. A hypothesis is an essential part of the scientific method and helps to guide the research process by providing a clear focus for investigation. It enables scientists to design experiments or studies to gather evidence and data that can support or refute the proposed explanation or prediction.
The formulation of a hypothesis is based on existing knowledge, observations, and theories, and it should be specific, testable, and falsifiable. A specific hypothesis helps to define the research question, which is important in the research process as it guides the selection of an appropriate research design and methodology. Testability of the hypothesis means that it can be proven or disproven through empirical data collection and analysis. Falsifiability means that the hypothesis should be formulated in such a way that it can be proven wrong if it is incorrect.
In addition to guiding the research process, the testing of hypotheses can lead to new discoveries and advancements in scientific knowledge. When a hypothesis is supported by the data, it can be used to develop new theories or models to explain the observed phenomenon. When a hypothesis is not supported by the data, it can help to refine existing theories or prompt the development of new hypotheses to explain the phenomenon.
When to use Hypothesis
Here are some common situations in which hypotheses are used:
- In scientific research , hypotheses are used to guide the design of experiments and to help researchers make predictions about the outcomes of those experiments.
- In social science research , hypotheses are used to test theories about human behavior, social relationships, and other phenomena.
- I n business , hypotheses can be used to guide decisions about marketing, product development, and other areas. For example, a hypothesis might be that a new product will sell well in a particular market, and this hypothesis can be tested through market research.
Characteristics of Hypothesis
Here are some common characteristics of a hypothesis:
- Testable : A hypothesis must be able to be tested through observation or experimentation. This means that it must be possible to collect data that will either support or refute the hypothesis.
- Falsifiable : A hypothesis must be able to be proven false if it is not supported by the data. If a hypothesis cannot be falsified, then it is not a scientific hypothesis.
- Clear and concise : A hypothesis should be stated in a clear and concise manner so that it can be easily understood and tested.
- Based on existing knowledge : A hypothesis should be based on existing knowledge and research in the field. It should not be based on personal beliefs or opinions.
- Specific : A hypothesis should be specific in terms of the variables being tested and the predicted outcome. This will help to ensure that the research is focused and well-designed.
- Tentative: A hypothesis is a tentative statement or assumption that requires further testing and evidence to be confirmed or refuted. It is not a final conclusion or assertion.
- Relevant : A hypothesis should be relevant to the research question or problem being studied. It should address a gap in knowledge or provide a new perspective on the issue.
Advantages of Hypothesis
Hypotheses have several advantages in scientific research and experimentation:
- Guides research: A hypothesis provides a clear and specific direction for research. It helps to focus the research question, select appropriate methods and variables, and interpret the results.
- Predictive powe r: A hypothesis makes predictions about the outcome of research, which can be tested through experimentation. This allows researchers to evaluate the validity of the hypothesis and make new discoveries.
- Facilitates communication: A hypothesis provides a common language and framework for scientists to communicate with one another about their research. This helps to facilitate the exchange of ideas and promotes collaboration.
- Efficient use of resources: A hypothesis helps researchers to use their time, resources, and funding efficiently by directing them towards specific research questions and methods that are most likely to yield results.
- Provides a basis for further research: A hypothesis that is supported by data provides a basis for further research and exploration. It can lead to new hypotheses, theories, and discoveries.
- Increases objectivity: A hypothesis can help to increase objectivity in research by providing a clear and specific framework for testing and interpreting results. This can reduce bias and increase the reliability of research findings.
Limitations of Hypothesis
Some Limitations of the Hypothesis are as follows:
- Limited to observable phenomena: Hypotheses are limited to observable phenomena and cannot account for unobservable or intangible factors. This means that some research questions may not be amenable to hypothesis testing.
- May be inaccurate or incomplete: Hypotheses are based on existing knowledge and research, which may be incomplete or inaccurate. This can lead to flawed hypotheses and erroneous conclusions.
- May be biased: Hypotheses may be biased by the researcher’s own beliefs, values, or assumptions. This can lead to selective interpretation of data and a lack of objectivity in research.
- Cannot prove causation: A hypothesis can only show a correlation between variables, but it cannot prove causation. This requires further experimentation and analysis.
- Limited to specific contexts: Hypotheses are limited to specific contexts and may not be generalizable to other situations or populations. This means that results may not be applicable in other contexts or may require further testing.
- May be affected by chance : Hypotheses may be affected by chance or random variation, which can obscure or distort the true relationship between variables.
About the author
Muhammad Hassan
Researcher, Academic Writer, Web developer
You may also like

Data Collection – Methods Types and Examples

Delimitations in Research – Types, Examples and...

Research Process – Steps, Examples and Tips

Research Design – Types, Methods and Examples

Institutional Review Board – Application Sample...

Evaluating Research – Process, Examples and...
Have a language expert improve your writing
Run a free plagiarism check in 10 minutes, automatically generate references for free.
- Knowledge Base
- Methodology
- How to Write a Strong Hypothesis | Guide & Examples
How to Write a Strong Hypothesis | Guide & Examples
Published on 6 May 2022 by Shona McCombes .
A hypothesis is a statement that can be tested by scientific research. If you want to test a relationship between two or more variables, you need to write hypotheses before you start your experiment or data collection.
Table of contents
What is a hypothesis, developing a hypothesis (with example), hypothesis examples, frequently asked questions about writing hypotheses.
A hypothesis states your predictions about what your research will find. It is a tentative answer to your research question that has not yet been tested. For some research projects, you might have to write several hypotheses that address different aspects of your research question.
A hypothesis is not just a guess – it should be based on existing theories and knowledge. It also has to be testable, which means you can support or refute it through scientific research methods (such as experiments, observations, and statistical analysis of data).
Variables in hypotheses
Hypotheses propose a relationship between two or more variables . An independent variable is something the researcher changes or controls. A dependent variable is something the researcher observes and measures.
In this example, the independent variable is exposure to the sun – the assumed cause . The dependent variable is the level of happiness – the assumed effect .
Prevent plagiarism, run a free check.
Step 1: ask a question.
Writing a hypothesis begins with a research question that you want to answer. The question should be focused, specific, and researchable within the constraints of your project.
Step 2: Do some preliminary research
Your initial answer to the question should be based on what is already known about the topic. Look for theories and previous studies to help you form educated assumptions about what your research will find.
At this stage, you might construct a conceptual framework to identify which variables you will study and what you think the relationships are between them. Sometimes, you’ll have to operationalise more complex constructs.
Step 3: Formulate your hypothesis
Now you should have some idea of what you expect to find. Write your initial answer to the question in a clear, concise sentence.
Step 4: Refine your hypothesis
You need to make sure your hypothesis is specific and testable. There are various ways of phrasing a hypothesis, but all the terms you use should have clear definitions, and the hypothesis should contain:
- The relevant variables
- The specific group being studied
- The predicted outcome of the experiment or analysis
Step 5: Phrase your hypothesis in three ways
To identify the variables, you can write a simple prediction in if … then form. The first part of the sentence states the independent variable and the second part states the dependent variable.
In academic research, hypotheses are more commonly phrased in terms of correlations or effects, where you directly state the predicted relationship between variables.
If you are comparing two groups, the hypothesis can state what difference you expect to find between them.
Step 6. Write a null hypothesis
If your research involves statistical hypothesis testing , you will also have to write a null hypothesis. The null hypothesis is the default position that there is no association between the variables. The null hypothesis is written as H 0 , while the alternative hypothesis is H 1 or H a .
Hypothesis testing is a formal procedure for investigating our ideas about the world using statistics. It is used by scientists to test specific predictions, called hypotheses , by calculating how likely it is that a pattern or relationship between variables could have arisen by chance.
A hypothesis is not just a guess. It should be based on existing theories and knowledge. It also has to be testable, which means you can support or refute it through scientific research methods (such as experiments, observations, and statistical analysis of data).
A research hypothesis is your proposed answer to your research question. The research hypothesis usually includes an explanation (‘ x affects y because …’).
A statistical hypothesis, on the other hand, is a mathematical statement about a population parameter. Statistical hypotheses always come in pairs: the null and alternative hypotheses. In a well-designed study , the statistical hypotheses correspond logically to the research hypothesis.
Cite this Scribbr article
If you want to cite this source, you can copy and paste the citation or click the ‘Cite this Scribbr article’ button to automatically add the citation to our free Reference Generator.
McCombes, S. (2022, May 06). How to Write a Strong Hypothesis | Guide & Examples. Scribbr. Retrieved 6 May 2024, from https://www.scribbr.co.uk/research-methods/hypothesis-writing/
Is this article helpful?
Shona McCombes
Other students also liked, operationalisation | a guide with examples, pros & cons, what is a conceptual framework | tips & examples, a quick guide to experimental design | 5 steps & examples.
Research Hypothesis In Psychology: Types, & Examples
Saul Mcleod, PhD
Editor-in-Chief for Simply Psychology
BSc (Hons) Psychology, MRes, PhD, University of Manchester
Saul Mcleod, PhD., is a qualified psychology teacher with over 18 years of experience in further and higher education. He has been published in peer-reviewed journals, including the Journal of Clinical Psychology.
Learn about our Editorial Process
Olivia Guy-Evans, MSc
Associate Editor for Simply Psychology
BSc (Hons) Psychology, MSc Psychology of Education
Olivia Guy-Evans is a writer and associate editor for Simply Psychology. She has previously worked in healthcare and educational sectors.
On This Page:
A research hypothesis, in its plural form “hypotheses,” is a specific, testable prediction about the anticipated results of a study, established at its outset. It is a key component of the scientific method .
Hypotheses connect theory to data and guide the research process towards expanding scientific understanding
Some key points about hypotheses:
- A hypothesis expresses an expected pattern or relationship. It connects the variables under investigation.
- It is stated in clear, precise terms before any data collection or analysis occurs. This makes the hypothesis testable.
- A hypothesis must be falsifiable. It should be possible, even if unlikely in practice, to collect data that disconfirms rather than supports the hypothesis.
- Hypotheses guide research. Scientists design studies to explicitly evaluate hypotheses about how nature works.
- For a hypothesis to be valid, it must be testable against empirical evidence. The evidence can then confirm or disprove the testable predictions.
- Hypotheses are informed by background knowledge and observation, but go beyond what is already known to propose an explanation of how or why something occurs.
Predictions typically arise from a thorough knowledge of the research literature, curiosity about real-world problems or implications, and integrating this to advance theory. They build on existing literature while providing new insight.
Types of Research Hypotheses
Alternative hypothesis.
The research hypothesis is often called the alternative or experimental hypothesis in experimental research.
It typically suggests a potential relationship between two key variables: the independent variable, which the researcher manipulates, and the dependent variable, which is measured based on those changes.
The alternative hypothesis states a relationship exists between the two variables being studied (one variable affects the other).
A hypothesis is a testable statement or prediction about the relationship between two or more variables. It is a key component of the scientific method. Some key points about hypotheses:
- Important hypotheses lead to predictions that can be tested empirically. The evidence can then confirm or disprove the testable predictions.
In summary, a hypothesis is a precise, testable statement of what researchers expect to happen in a study and why. Hypotheses connect theory to data and guide the research process towards expanding scientific understanding.
An experimental hypothesis predicts what change(s) will occur in the dependent variable when the independent variable is manipulated.
It states that the results are not due to chance and are significant in supporting the theory being investigated.
The alternative hypothesis can be directional, indicating a specific direction of the effect, or non-directional, suggesting a difference without specifying its nature. It’s what researchers aim to support or demonstrate through their study.
Null Hypothesis
The null hypothesis states no relationship exists between the two variables being studied (one variable does not affect the other). There will be no changes in the dependent variable due to manipulating the independent variable.
It states results are due to chance and are not significant in supporting the idea being investigated.
The null hypothesis, positing no effect or relationship, is a foundational contrast to the research hypothesis in scientific inquiry. It establishes a baseline for statistical testing, promoting objectivity by initiating research from a neutral stance.
Many statistical methods are tailored to test the null hypothesis, determining the likelihood of observed results if no true effect exists.
This dual-hypothesis approach provides clarity, ensuring that research intentions are explicit, and fosters consistency across scientific studies, enhancing the standardization and interpretability of research outcomes.
Nondirectional Hypothesis
A non-directional hypothesis, also known as a two-tailed hypothesis, predicts that there is a difference or relationship between two variables but does not specify the direction of this relationship.
It merely indicates that a change or effect will occur without predicting which group will have higher or lower values.
For example, “There is a difference in performance between Group A and Group B” is a non-directional hypothesis.
Directional Hypothesis
A directional (one-tailed) hypothesis predicts the nature of the effect of the independent variable on the dependent variable. It predicts in which direction the change will take place. (i.e., greater, smaller, less, more)
It specifies whether one variable is greater, lesser, or different from another, rather than just indicating that there’s a difference without specifying its nature.
For example, “Exercise increases weight loss” is a directional hypothesis.
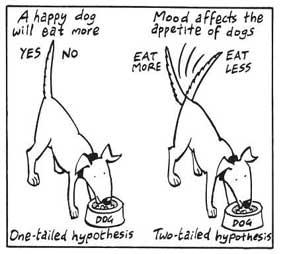
Falsifiability
The Falsification Principle, proposed by Karl Popper , is a way of demarcating science from non-science. It suggests that for a theory or hypothesis to be considered scientific, it must be testable and irrefutable.
Falsifiability emphasizes that scientific claims shouldn’t just be confirmable but should also have the potential to be proven wrong.
It means that there should exist some potential evidence or experiment that could prove the proposition false.
However many confirming instances exist for a theory, it only takes one counter observation to falsify it. For example, the hypothesis that “all swans are white,” can be falsified by observing a black swan.
For Popper, science should attempt to disprove a theory rather than attempt to continually provide evidence to support a research hypothesis.
Can a Hypothesis be Proven?
Hypotheses make probabilistic predictions. They state the expected outcome if a particular relationship exists. However, a study result supporting a hypothesis does not definitively prove it is true.
All studies have limitations. There may be unknown confounding factors or issues that limit the certainty of conclusions. Additional studies may yield different results.
In science, hypotheses can realistically only be supported with some degree of confidence, not proven. The process of science is to incrementally accumulate evidence for and against hypothesized relationships in an ongoing pursuit of better models and explanations that best fit the empirical data. But hypotheses remain open to revision and rejection if that is where the evidence leads.
- Disproving a hypothesis is definitive. Solid disconfirmatory evidence will falsify a hypothesis and require altering or discarding it based on the evidence.
- However, confirming evidence is always open to revision. Other explanations may account for the same results, and additional or contradictory evidence may emerge over time.
We can never 100% prove the alternative hypothesis. Instead, we see if we can disprove, or reject the null hypothesis.
If we reject the null hypothesis, this doesn’t mean that our alternative hypothesis is correct but does support the alternative/experimental hypothesis.
Upon analysis of the results, an alternative hypothesis can be rejected or supported, but it can never be proven to be correct. We must avoid any reference to results proving a theory as this implies 100% certainty, and there is always a chance that evidence may exist which could refute a theory.
How to Write a Hypothesis
- Identify variables . The researcher manipulates the independent variable and the dependent variable is the measured outcome.
- Operationalized the variables being investigated . Operationalization of a hypothesis refers to the process of making the variables physically measurable or testable, e.g. if you are about to study aggression, you might count the number of punches given by participants.
- Decide on a direction for your prediction . If there is evidence in the literature to support a specific effect of the independent variable on the dependent variable, write a directional (one-tailed) hypothesis. If there are limited or ambiguous findings in the literature regarding the effect of the independent variable on the dependent variable, write a non-directional (two-tailed) hypothesis.
- Make it Testable : Ensure your hypothesis can be tested through experimentation or observation. It should be possible to prove it false (principle of falsifiability).
- Clear & concise language . A strong hypothesis is concise (typically one to two sentences long), and formulated using clear and straightforward language, ensuring it’s easily understood and testable.
Consider a hypothesis many teachers might subscribe to: students work better on Monday morning than on Friday afternoon (IV=Day, DV= Standard of work).
Now, if we decide to study this by giving the same group of students a lesson on a Monday morning and a Friday afternoon and then measuring their immediate recall of the material covered in each session, we would end up with the following:
- The alternative hypothesis states that students will recall significantly more information on a Monday morning than on a Friday afternoon.
- The null hypothesis states that there will be no significant difference in the amount recalled on a Monday morning compared to a Friday afternoon. Any difference will be due to chance or confounding factors.
More Examples
- Memory : Participants exposed to classical music during study sessions will recall more items from a list than those who studied in silence.
- Social Psychology : Individuals who frequently engage in social media use will report higher levels of perceived social isolation compared to those who use it infrequently.
- Developmental Psychology : Children who engage in regular imaginative play have better problem-solving skills than those who don’t.
- Clinical Psychology : Cognitive-behavioral therapy will be more effective in reducing symptoms of anxiety over a 6-month period compared to traditional talk therapy.
- Cognitive Psychology : Individuals who multitask between various electronic devices will have shorter attention spans on focused tasks than those who single-task.
- Health Psychology : Patients who practice mindfulness meditation will experience lower levels of chronic pain compared to those who don’t meditate.
- Organizational Psychology : Employees in open-plan offices will report higher levels of stress than those in private offices.
- Behavioral Psychology : Rats rewarded with food after pressing a lever will press it more frequently than rats who receive no reward.

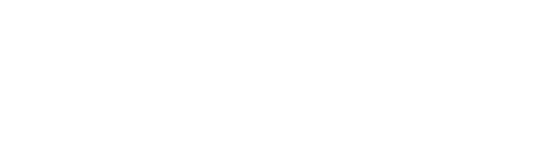
- Peterborough
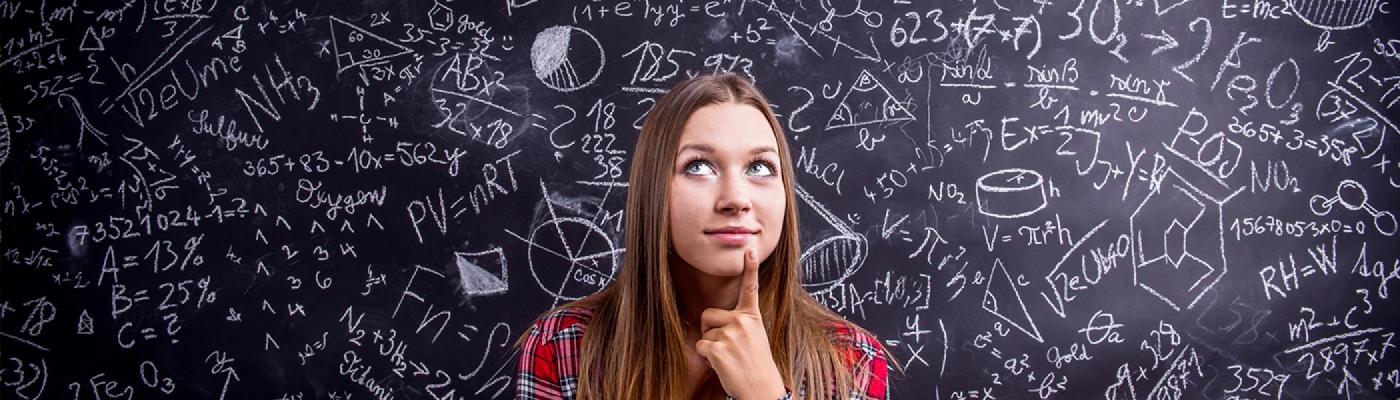
Understanding Hypotheses and Predictions
Hypotheses and predictions are different components of the scientific method. The scientific method is a systematic process that helps minimize bias in research and begins by developing good research questions.
Research Questions
Descriptive research questions are based on observations made in previous research or in passing. This type of research question often quantifies these observations. For example, while out bird watching, you notice that a certain species of sparrow made all its nests with the same material: grasses. A descriptive research question would be “On average, how much grass is used to build sparrow nests?”
Descriptive research questions lead to causal questions. This type of research question seeks to understand why we observe certain trends or patterns. If we return to our observation about sparrow nests, a causal question would be “Why are the nests of sparrows made with grasses rather than twigs?”
In simple terms, a hypothesis is the answer to your causal question. A hypothesis should be based on a strong rationale that is usually supported by background research. From the question about sparrow nests, you might hypothesize, “Sparrows use grasses in their nests rather than twigs because grasses are the more abundant material in their habitat.” This abundance hypothesis might be supported by your prior knowledge about the availability of nest building materials (i.e. grasses are more abundant than twigs).
On the other hand, a prediction is the outcome you would observe if your hypothesis were correct. Predictions are often written in the form of “if, and, then” statements, as in, “if my hypothesis is true, and I were to do this test, then this is what I will observe.” Following our sparrow example, you could predict that, “If sparrows use grass because it is more abundant, and I compare areas that have more twigs than grasses available, then, in those areas, nests should be made out of twigs.” A more refined prediction might alter the wording so as not to repeat the hypothesis verbatim: “If sparrows choose nesting materials based on their abundance, then when twigs are more abundant, sparrows will use those in their nests.”
As you can see, the terms hypothesis and prediction are different and distinct even though, sometimes, they are incorrectly used interchangeably.
Let us take a look at another example:
Causal Question: Why are there fewer asparagus beetles when asparagus is grown next to marigolds?
Hypothesis: Marigolds deter asparagus beetles.
Prediction: If marigolds deter asparagus beetles, and we grow asparagus next to marigolds, then we should find fewer asparagus beetles when asparagus plants are planted with marigolds.
A final note
It is exciting when the outcome of your study or experiment supports your hypothesis. However, it can be equally exciting if this does not happen. There are many reasons why you can have an unexpected result, and you need to think why this occurred. Maybe you had a potential problem with your methods, but on the flip side, maybe you have just discovered a new line of evidence that can be used to develop another experiment or study.

What Is A Research (Scientific) Hypothesis? A plain-language explainer + examples
By: Derek Jansen (MBA) | Reviewed By: Dr Eunice Rautenbach | June 2020
If you’re new to the world of research, or it’s your first time writing a dissertation or thesis, you’re probably noticing that the words “research hypothesis” and “scientific hypothesis” are used quite a bit, and you’re wondering what they mean in a research context .
“Hypothesis” is one of those words that people use loosely, thinking they understand what it means. However, it has a very specific meaning within academic research. So, it’s important to understand the exact meaning before you start hypothesizing.
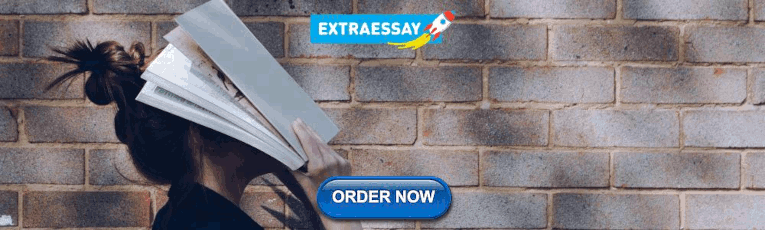
Research Hypothesis 101
- What is a hypothesis ?
- What is a research hypothesis (scientific hypothesis)?
- Requirements for a research hypothesis
- Definition of a research hypothesis
- The null hypothesis
What is a hypothesis?
Let’s start with the general definition of a hypothesis (not a research hypothesis or scientific hypothesis), according to the Cambridge Dictionary:
Hypothesis: an idea or explanation for something that is based on known facts but has not yet been proved.
In other words, it’s a statement that provides an explanation for why or how something works, based on facts (or some reasonable assumptions), but that has not yet been specifically tested . For example, a hypothesis might look something like this:
Hypothesis: sleep impacts academic performance.
This statement predicts that academic performance will be influenced by the amount and/or quality of sleep a student engages in – sounds reasonable, right? It’s based on reasonable assumptions , underpinned by what we currently know about sleep and health (from the existing literature). So, loosely speaking, we could call it a hypothesis, at least by the dictionary definition.
But that’s not good enough…
Unfortunately, that’s not quite sophisticated enough to describe a research hypothesis (also sometimes called a scientific hypothesis), and it wouldn’t be acceptable in a dissertation, thesis or research paper . In the world of academic research, a statement needs a few more criteria to constitute a true research hypothesis .
What is a research hypothesis?
A research hypothesis (also called a scientific hypothesis) is a statement about the expected outcome of a study (for example, a dissertation or thesis). To constitute a quality hypothesis, the statement needs to have three attributes – specificity , clarity and testability .
Let’s take a look at these more closely.
Need a helping hand?
Hypothesis Essential #1: Specificity & Clarity
A good research hypothesis needs to be extremely clear and articulate about both what’ s being assessed (who or what variables are involved ) and the expected outcome (for example, a difference between groups, a relationship between variables, etc.).
Let’s stick with our sleepy students example and look at how this statement could be more specific and clear.
Hypothesis: Students who sleep at least 8 hours per night will, on average, achieve higher grades in standardised tests than students who sleep less than 8 hours a night.
As you can see, the statement is very specific as it identifies the variables involved (sleep hours and test grades), the parties involved (two groups of students), as well as the predicted relationship type (a positive relationship). There’s no ambiguity or uncertainty about who or what is involved in the statement, and the expected outcome is clear.
Contrast that to the original hypothesis we looked at – “Sleep impacts academic performance” – and you can see the difference. “Sleep” and “academic performance” are both comparatively vague , and there’s no indication of what the expected relationship direction is (more sleep or less sleep). As you can see, specificity and clarity are key.
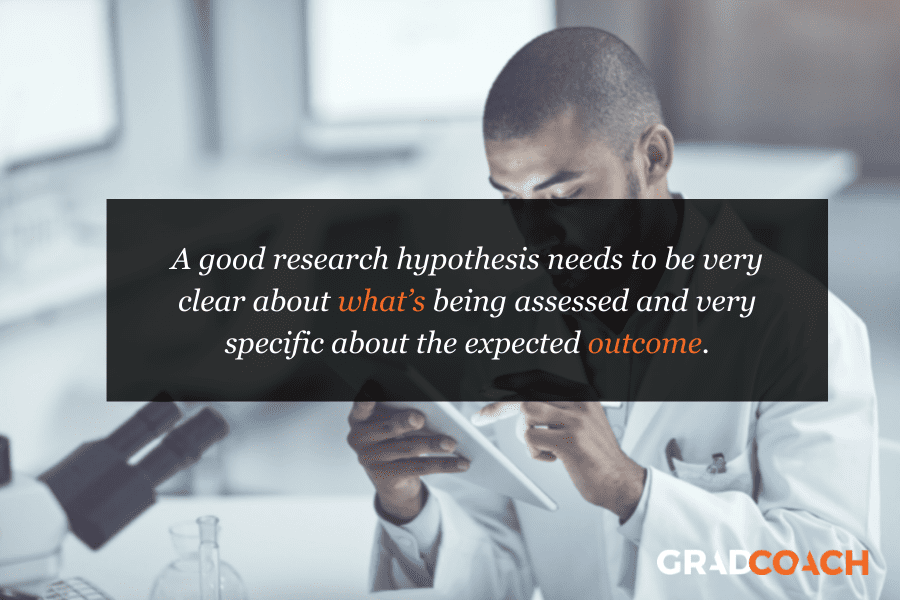
Hypothesis Essential #2: Testability (Provability)
A statement must be testable to qualify as a research hypothesis. In other words, there needs to be a way to prove (or disprove) the statement. If it’s not testable, it’s not a hypothesis – simple as that.
For example, consider the hypothesis we mentioned earlier:
Hypothesis: Students who sleep at least 8 hours per night will, on average, achieve higher grades in standardised tests than students who sleep less than 8 hours a night.
We could test this statement by undertaking a quantitative study involving two groups of students, one that gets 8 or more hours of sleep per night for a fixed period, and one that gets less. We could then compare the standardised test results for both groups to see if there’s a statistically significant difference.
Again, if you compare this to the original hypothesis we looked at – “Sleep impacts academic performance” – you can see that it would be quite difficult to test that statement, primarily because it isn’t specific enough. How much sleep? By who? What type of academic performance?
So, remember the mantra – if you can’t test it, it’s not a hypothesis 🙂
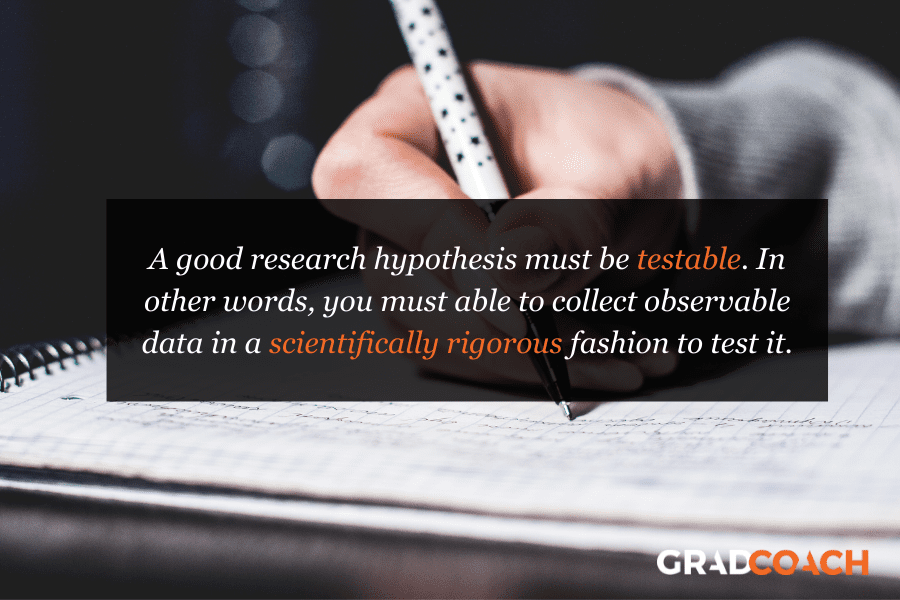
Defining A Research Hypothesis
You’re still with us? Great! Let’s recap and pin down a clear definition of a hypothesis.
A research hypothesis (or scientific hypothesis) is a statement about an expected relationship between variables, or explanation of an occurrence, that is clear, specific and testable.
So, when you write up hypotheses for your dissertation or thesis, make sure that they meet all these criteria. If you do, you’ll not only have rock-solid hypotheses but you’ll also ensure a clear focus for your entire research project.
What about the null hypothesis?
You may have also heard the terms null hypothesis , alternative hypothesis, or H-zero thrown around. At a simple level, the null hypothesis is the counter-proposal to the original hypothesis.
For example, if the hypothesis predicts that there is a relationship between two variables (for example, sleep and academic performance), the null hypothesis would predict that there is no relationship between those variables.
At a more technical level, the null hypothesis proposes that no statistical significance exists in a set of given observations and that any differences are due to chance alone.
And there you have it – hypotheses in a nutshell.
If you have any questions, be sure to leave a comment below and we’ll do our best to help you. If you need hands-on help developing and testing your hypotheses, consider our private coaching service , where we hold your hand through the research journey.
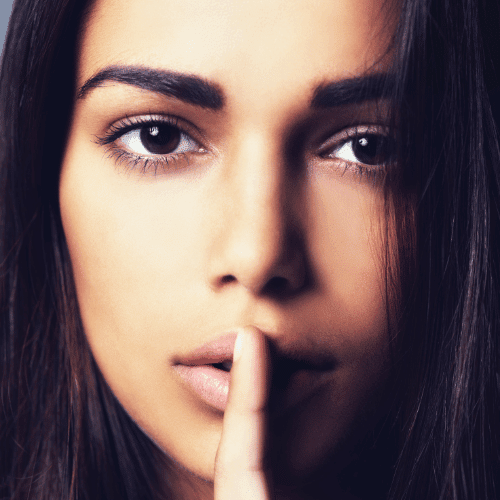
Psst... there’s more!
This post was based on one of our popular Research Bootcamps . If you're working on a research project, you'll definitely want to check this out ...
You Might Also Like:
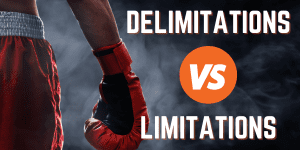
16 Comments
Very useful information. I benefit more from getting more information in this regard.
Very great insight,educative and informative. Please give meet deep critics on many research data of public international Law like human rights, environment, natural resources, law of the sea etc
In a book I read a distinction is made between null, research, and alternative hypothesis. As far as I understand, alternative and research hypotheses are the same. Can you please elaborate? Best Afshin
This is a self explanatory, easy going site. I will recommend this to my friends and colleagues.
Very good definition. How can I cite your definition in my thesis? Thank you. Is nul hypothesis compulsory in a research?
It’s a counter-proposal to be proven as a rejection
Please what is the difference between alternate hypothesis and research hypothesis?
It is a very good explanation. However, it limits hypotheses to statistically tasteable ideas. What about for qualitative researches or other researches that involve quantitative data that don’t need statistical tests?
In qualitative research, one typically uses propositions, not hypotheses.
could you please elaborate it more
I’ve benefited greatly from these notes, thank you.
This is very helpful
well articulated ideas are presented here, thank you for being reliable sources of information
Excellent. Thanks for being clear and sound about the research methodology and hypothesis (quantitative research)
I have only a simple question regarding the null hypothesis. – Is the null hypothesis (Ho) known as the reversible hypothesis of the alternative hypothesis (H1? – How to test it in academic research?
this is very important note help me much more
Trackbacks/Pingbacks
- What Is Research Methodology? Simple Definition (With Examples) - Grad Coach - […] Contrasted to this, a quantitative methodology is typically used when the research aims and objectives are confirmatory in nature. For example,…
Submit a Comment Cancel reply
Your email address will not be published. Required fields are marked *
Save my name, email, and website in this browser for the next time I comment.
- Print Friendly
- Search Menu
- Browse content in Arts and Humanities
- Browse content in Archaeology
- Anglo-Saxon and Medieval Archaeology
- Archaeological Methodology and Techniques
- Archaeology by Region
- Archaeology of Religion
- Archaeology of Trade and Exchange
- Biblical Archaeology
- Contemporary and Public Archaeology
- Environmental Archaeology
- Historical Archaeology
- History and Theory of Archaeology
- Industrial Archaeology
- Landscape Archaeology
- Mortuary Archaeology
- Prehistoric Archaeology
- Underwater Archaeology
- Urban Archaeology
- Zooarchaeology
- Browse content in Architecture
- Architectural Structure and Design
- History of Architecture
- Residential and Domestic Buildings
- Theory of Architecture
- Browse content in Art
- Art Subjects and Themes
- History of Art
- Industrial and Commercial Art
- Theory of Art
- Biographical Studies
- Byzantine Studies
- Browse content in Classical Studies
- Classical History
- Classical Philosophy
- Classical Mythology
- Classical Literature
- Classical Reception
- Classical Art and Architecture
- Classical Oratory and Rhetoric
- Greek and Roman Epigraphy
- Greek and Roman Law
- Greek and Roman Archaeology
- Greek and Roman Papyrology
- Late Antiquity
- Religion in the Ancient World
- Digital Humanities
- Browse content in History
- Colonialism and Imperialism
- Diplomatic History
- Environmental History
- Genealogy, Heraldry, Names, and Honours
- Genocide and Ethnic Cleansing
- Historical Geography
- History by Period
- History of Agriculture
- History of Education
- History of Emotions
- History of Gender and Sexuality
- Industrial History
- Intellectual History
- International History
- Labour History
- Legal and Constitutional History
- Local and Family History
- Maritime History
- Military History
- National Liberation and Post-Colonialism
- Oral History
- Political History
- Public History
- Regional and National History
- Revolutions and Rebellions
- Slavery and Abolition of Slavery
- Social and Cultural History
- Theory, Methods, and Historiography
- Urban History
- World History
- Browse content in Language Teaching and Learning
- Language Learning (Specific Skills)
- Language Teaching Theory and Methods
- Browse content in Linguistics
- Applied Linguistics
- Cognitive Linguistics
- Computational Linguistics
- Forensic Linguistics
- Grammar, Syntax and Morphology
- Historical and Diachronic Linguistics
- History of English
- Language Acquisition
- Language Variation
- Language Families
- Language Evolution
- Language Reference
- Lexicography
- Linguistic Theories
- Linguistic Typology
- Linguistic Anthropology
- Phonetics and Phonology
- Psycholinguistics
- Sociolinguistics
- Translation and Interpretation
- Writing Systems
- Browse content in Literature
- Bibliography
- Children's Literature Studies
- Literary Studies (Asian)
- Literary Studies (European)
- Literary Studies (Eco-criticism)
- Literary Studies (Modernism)
- Literary Studies (Romanticism)
- Literary Studies (American)
- Literary Studies - World
- Literary Studies (1500 to 1800)
- Literary Studies (19th Century)
- Literary Studies (20th Century onwards)
- Literary Studies (African American Literature)
- Literary Studies (British and Irish)
- Literary Studies (Early and Medieval)
- Literary Studies (Fiction, Novelists, and Prose Writers)
- Literary Studies (Gender Studies)
- Literary Studies (Graphic Novels)
- Literary Studies (History of the Book)
- Literary Studies (Plays and Playwrights)
- Literary Studies (Poetry and Poets)
- Literary Studies (Postcolonial Literature)
- Literary Studies (Queer Studies)
- Literary Studies (Science Fiction)
- Literary Studies (Travel Literature)
- Literary Studies (War Literature)
- Literary Studies (Women's Writing)
- Literary Theory and Cultural Studies
- Mythology and Folklore
- Shakespeare Studies and Criticism
- Browse content in Media Studies
- Browse content in Music
- Applied Music
- Dance and Music
- Ethics in Music
- Ethnomusicology
- Gender and Sexuality in Music
- Medicine and Music
- Music Cultures
- Music and Religion
- Music and Culture
- Music and Media
- Music Education and Pedagogy
- Music Theory and Analysis
- Musical Scores, Lyrics, and Libretti
- Musical Structures, Styles, and Techniques
- Musicology and Music History
- Performance Practice and Studies
- Race and Ethnicity in Music
- Sound Studies
- Browse content in Performing Arts
- Browse content in Philosophy
- Aesthetics and Philosophy of Art
- Epistemology
- Feminist Philosophy
- History of Western Philosophy
- Metaphysics
- Moral Philosophy
- Non-Western Philosophy
- Philosophy of Science
- Philosophy of Action
- Philosophy of Law
- Philosophy of Religion
- Philosophy of Language
- Philosophy of Mind
- Philosophy of Perception
- Philosophy of Mathematics and Logic
- Practical Ethics
- Social and Political Philosophy
- Browse content in Religion
- Biblical Studies
- Christianity
- East Asian Religions
- History of Religion
- Judaism and Jewish Studies
- Qumran Studies
- Religion and Education
- Religion and Health
- Religion and Politics
- Religion and Science
- Religion and Law
- Religion and Art, Literature, and Music
- Religious Studies
- Browse content in Society and Culture
- Cookery, Food, and Drink
- Cultural Studies
- Customs and Traditions
- Ethical Issues and Debates
- Hobbies, Games, Arts and Crafts
- Lifestyle, Home, and Garden
- Natural world, Country Life, and Pets
- Popular Beliefs and Controversial Knowledge
- Sports and Outdoor Recreation
- Technology and Society
- Travel and Holiday
- Visual Culture
- Browse content in Law
- Arbitration
- Browse content in Company and Commercial Law
- Commercial Law
- Company Law
- Browse content in Comparative Law
- Systems of Law
- Competition Law
- Browse content in Constitutional and Administrative Law
- Government Powers
- Judicial Review
- Local Government Law
- Military and Defence Law
- Parliamentary and Legislative Practice
- Construction Law
- Contract Law
- Browse content in Criminal Law
- Criminal Procedure
- Criminal Evidence Law
- Sentencing and Punishment
- Employment and Labour Law
- Environment and Energy Law
- Browse content in Financial Law
- Banking Law
- Insolvency Law
- History of Law
- Human Rights and Immigration
- Intellectual Property Law
- Browse content in International Law
- Private International Law and Conflict of Laws
- Public International Law
- IT and Communications Law
- Jurisprudence and Philosophy of Law
- Law and Politics
- Law and Society
- Browse content in Legal System and Practice
- Courts and Procedure
- Legal Skills and Practice
- Primary Sources of Law
- Regulation of Legal Profession
- Medical and Healthcare Law
- Browse content in Policing
- Criminal Investigation and Detection
- Police and Security Services
- Police Procedure and Law
- Police Regional Planning
- Browse content in Property Law
- Personal Property Law
- Study and Revision
- Terrorism and National Security Law
- Browse content in Trusts Law
- Wills and Probate or Succession
- Browse content in Medicine and Health
- Browse content in Allied Health Professions
- Arts Therapies
- Clinical Science
- Dietetics and Nutrition
- Occupational Therapy
- Operating Department Practice
- Physiotherapy
- Radiography
- Speech and Language Therapy
- Browse content in Anaesthetics
- General Anaesthesia
- Neuroanaesthesia
- Browse content in Clinical Medicine
- Acute Medicine
- Cardiovascular Medicine
- Clinical Genetics
- Clinical Pharmacology and Therapeutics
- Dermatology
- Endocrinology and Diabetes
- Gastroenterology
- Genito-urinary Medicine
- Geriatric Medicine
- Infectious Diseases
- Medical Oncology
- Medical Toxicology
- Pain Medicine
- Palliative Medicine
- Rehabilitation Medicine
- Respiratory Medicine and Pulmonology
- Rheumatology
- Sleep Medicine
- Sports and Exercise Medicine
- Clinical Neuroscience
- Community Medical Services
- Critical Care
- Emergency Medicine
- Forensic Medicine
- Haematology
- History of Medicine
- Browse content in Medical Dentistry
- Oral and Maxillofacial Surgery
- Paediatric Dentistry
- Restorative Dentistry and Orthodontics
- Surgical Dentistry
- Medical Ethics
- Browse content in Medical Skills
- Clinical Skills
- Communication Skills
- Nursing Skills
- Surgical Skills
- Medical Statistics and Methodology
- Browse content in Neurology
- Clinical Neurophysiology
- Neuropathology
- Nursing Studies
- Browse content in Obstetrics and Gynaecology
- Gynaecology
- Occupational Medicine
- Ophthalmology
- Otolaryngology (ENT)
- Browse content in Paediatrics
- Neonatology
- Browse content in Pathology
- Chemical Pathology
- Clinical Cytogenetics and Molecular Genetics
- Histopathology
- Medical Microbiology and Virology
- Patient Education and Information
- Browse content in Pharmacology
- Psychopharmacology
- Browse content in Popular Health
- Caring for Others
- Complementary and Alternative Medicine
- Self-help and Personal Development
- Browse content in Preclinical Medicine
- Cell Biology
- Molecular Biology and Genetics
- Reproduction, Growth and Development
- Primary Care
- Professional Development in Medicine
- Browse content in Psychiatry
- Addiction Medicine
- Child and Adolescent Psychiatry
- Forensic Psychiatry
- Learning Disabilities
- Old Age Psychiatry
- Psychotherapy
- Browse content in Public Health and Epidemiology
- Epidemiology
- Public Health
- Browse content in Radiology
- Clinical Radiology
- Interventional Radiology
- Nuclear Medicine
- Radiation Oncology
- Reproductive Medicine
- Browse content in Surgery
- Cardiothoracic Surgery
- Gastro-intestinal and Colorectal Surgery
- General Surgery
- Neurosurgery
- Paediatric Surgery
- Peri-operative Care
- Plastic and Reconstructive Surgery
- Surgical Oncology
- Transplant Surgery
- Trauma and Orthopaedic Surgery
- Vascular Surgery
- Browse content in Science and Mathematics
- Browse content in Biological Sciences
- Aquatic Biology
- Biochemistry
- Bioinformatics and Computational Biology
- Developmental Biology
- Ecology and Conservation
- Evolutionary Biology
- Genetics and Genomics
- Microbiology
- Molecular and Cell Biology
- Natural History
- Plant Sciences and Forestry
- Research Methods in Life Sciences
- Structural Biology
- Systems Biology
- Zoology and Animal Sciences
- Browse content in Chemistry
- Analytical Chemistry
- Computational Chemistry
- Crystallography
- Environmental Chemistry
- Industrial Chemistry
- Inorganic Chemistry
- Materials Chemistry
- Medicinal Chemistry
- Mineralogy and Gems
- Organic Chemistry
- Physical Chemistry
- Polymer Chemistry
- Study and Communication Skills in Chemistry
- Theoretical Chemistry
- Browse content in Computer Science
- Artificial Intelligence
- Computer Architecture and Logic Design
- Game Studies
- Human-Computer Interaction
- Mathematical Theory of Computation
- Programming Languages
- Software Engineering
- Systems Analysis and Design
- Virtual Reality
- Browse content in Computing
- Business Applications
- Computer Security
- Computer Games
- Computer Networking and Communications
- Digital Lifestyle
- Graphical and Digital Media Applications
- Operating Systems
- Browse content in Earth Sciences and Geography
- Atmospheric Sciences
- Environmental Geography
- Geology and the Lithosphere
- Maps and Map-making
- Meteorology and Climatology
- Oceanography and Hydrology
- Palaeontology
- Physical Geography and Topography
- Regional Geography
- Soil Science
- Urban Geography
- Browse content in Engineering and Technology
- Agriculture and Farming
- Biological Engineering
- Civil Engineering, Surveying, and Building
- Electronics and Communications Engineering
- Energy Technology
- Engineering (General)
- Environmental Science, Engineering, and Technology
- History of Engineering and Technology
- Mechanical Engineering and Materials
- Technology of Industrial Chemistry
- Transport Technology and Trades
- Browse content in Environmental Science
- Applied Ecology (Environmental Science)
- Conservation of the Environment (Environmental Science)
- Environmental Sustainability
- Environmentalist Thought and Ideology (Environmental Science)
- Management of Land and Natural Resources (Environmental Science)
- Natural Disasters (Environmental Science)
- Nuclear Issues (Environmental Science)
- Pollution and Threats to the Environment (Environmental Science)
- Social Impact of Environmental Issues (Environmental Science)
- History of Science and Technology
- Browse content in Materials Science
- Ceramics and Glasses
- Composite Materials
- Metals, Alloying, and Corrosion
- Nanotechnology
- Browse content in Mathematics
- Applied Mathematics
- Biomathematics and Statistics
- History of Mathematics
- Mathematical Education
- Mathematical Finance
- Mathematical Analysis
- Numerical and Computational Mathematics
- Probability and Statistics
- Pure Mathematics
- Browse content in Neuroscience
- Cognition and Behavioural Neuroscience
- Development of the Nervous System
- Disorders of the Nervous System
- History of Neuroscience
- Invertebrate Neurobiology
- Molecular and Cellular Systems
- Neuroendocrinology and Autonomic Nervous System
- Neuroscientific Techniques
- Sensory and Motor Systems
- Browse content in Physics
- Astronomy and Astrophysics
- Atomic, Molecular, and Optical Physics
- Biological and Medical Physics
- Classical Mechanics
- Computational Physics
- Condensed Matter Physics
- Electromagnetism, Optics, and Acoustics
- History of Physics
- Mathematical and Statistical Physics
- Measurement Science
- Nuclear Physics
- Particles and Fields
- Plasma Physics
- Quantum Physics
- Relativity and Gravitation
- Semiconductor and Mesoscopic Physics
- Browse content in Psychology
- Affective Sciences
- Clinical Psychology
- Cognitive Neuroscience
- Cognitive Psychology
- Criminal and Forensic Psychology
- Developmental Psychology
- Educational Psychology
- Evolutionary Psychology
- Health Psychology
- History and Systems in Psychology
- Music Psychology
- Neuropsychology
- Organizational Psychology
- Psychological Assessment and Testing
- Psychology of Human-Technology Interaction
- Psychology Professional Development and Training
- Research Methods in Psychology
- Social Psychology
- Browse content in Social Sciences
- Browse content in Anthropology
- Anthropology of Religion
- Human Evolution
- Medical Anthropology
- Physical Anthropology
- Regional Anthropology
- Social and Cultural Anthropology
- Theory and Practice of Anthropology
- Browse content in Business and Management
- Business Strategy
- Business History
- Business Ethics
- Business and Government
- Business and Technology
- Business and the Environment
- Comparative Management
- Corporate Governance
- Corporate Social Responsibility
- Entrepreneurship
- Health Management
- Human Resource Management
- Industrial and Employment Relations
- Industry Studies
- Information and Communication Technologies
- International Business
- Knowledge Management
- Management and Management Techniques
- Operations Management
- Organizational Theory and Behaviour
- Pensions and Pension Management
- Public and Nonprofit Management
- Strategic Management
- Supply Chain Management
- Browse content in Criminology and Criminal Justice
- Criminal Justice
- Criminology
- Forms of Crime
- International and Comparative Criminology
- Youth Violence and Juvenile Justice
- Development Studies
- Browse content in Economics
- Agricultural, Environmental, and Natural Resource Economics
- Asian Economics
- Behavioural Finance
- Behavioural Economics and Neuroeconomics
- Econometrics and Mathematical Economics
- Economic Systems
- Economic Methodology
- Economic History
- Economic Development and Growth
- Financial Markets
- Financial Institutions and Services
- General Economics and Teaching
- Health, Education, and Welfare
- History of Economic Thought
- International Economics
- Labour and Demographic Economics
- Law and Economics
- Macroeconomics and Monetary Economics
- Microeconomics
- Public Economics
- Urban, Rural, and Regional Economics
- Welfare Economics
- Browse content in Education
- Adult Education and Continuous Learning
- Care and Counselling of Students
- Early Childhood and Elementary Education
- Educational Equipment and Technology
- Educational Strategies and Policy
- Higher and Further Education
- Organization and Management of Education
- Philosophy and Theory of Education
- Schools Studies
- Secondary Education
- Teaching of a Specific Subject
- Teaching of Specific Groups and Special Educational Needs
- Teaching Skills and Techniques
- Browse content in Environment
- Applied Ecology (Social Science)
- Climate Change
- Conservation of the Environment (Social Science)
- Environmentalist Thought and Ideology (Social Science)
- Natural Disasters (Environment)
- Social Impact of Environmental Issues (Social Science)
- Browse content in Human Geography
- Cultural Geography
- Economic Geography
- Political Geography
- Browse content in Interdisciplinary Studies
- Communication Studies
- Museums, Libraries, and Information Sciences
- Browse content in Politics
- African Politics
- Asian Politics
- Chinese Politics
- Comparative Politics
- Conflict Politics
- Elections and Electoral Studies
- Environmental Politics
- European Union
- Foreign Policy
- Gender and Politics
- Human Rights and Politics
- Indian Politics
- International Relations
- International Organization (Politics)
- International Political Economy
- Irish Politics
- Latin American Politics
- Middle Eastern Politics
- Political Methodology
- Political Communication
- Political Philosophy
- Political Sociology
- Political Theory
- Political Behaviour
- Political Economy
- Political Institutions
- Politics and Law
- Public Administration
- Public Policy
- Quantitative Political Methodology
- Regional Political Studies
- Russian Politics
- Security Studies
- State and Local Government
- UK Politics
- US Politics
- Browse content in Regional and Area Studies
- African Studies
- Asian Studies
- East Asian Studies
- Japanese Studies
- Latin American Studies
- Middle Eastern Studies
- Native American Studies
- Scottish Studies
- Browse content in Research and Information
- Research Methods
- Browse content in Social Work
- Addictions and Substance Misuse
- Adoption and Fostering
- Care of the Elderly
- Child and Adolescent Social Work
- Couple and Family Social Work
- Developmental and Physical Disabilities Social Work
- Direct Practice and Clinical Social Work
- Emergency Services
- Human Behaviour and the Social Environment
- International and Global Issues in Social Work
- Mental and Behavioural Health
- Social Justice and Human Rights
- Social Policy and Advocacy
- Social Work and Crime and Justice
- Social Work Macro Practice
- Social Work Practice Settings
- Social Work Research and Evidence-based Practice
- Welfare and Benefit Systems
- Browse content in Sociology
- Childhood Studies
- Community Development
- Comparative and Historical Sociology
- Economic Sociology
- Gender and Sexuality
- Gerontology and Ageing
- Health, Illness, and Medicine
- Marriage and the Family
- Migration Studies
- Occupations, Professions, and Work
- Organizations
- Population and Demography
- Race and Ethnicity
- Social Theory
- Social Movements and Social Change
- Social Research and Statistics
- Social Stratification, Inequality, and Mobility
- Sociology of Religion
- Sociology of Education
- Sport and Leisure
- Urban and Rural Studies
- Browse content in Warfare and Defence
- Defence Strategy, Planning, and Research
- Land Forces and Warfare
- Military Administration
- Military Life and Institutions
- Naval Forces and Warfare
- Other Warfare and Defence Issues
- Peace Studies and Conflict Resolution
- Weapons and Equipment

- < Previous chapter
- Next chapter >

7 The Predictive Processing Hypothesis
Jakob Hohwy Cognition & Philosophy Lab, Department of Philosophy, Faculty of Arts, Monash University, Melbourne, Australia
- Published: 09 October 2018
- Cite Icon Cite
- Permissions Icon Permissions
Prediction may be a central concept for understanding perceptual and cognitive processing. Contemporary theoretical neuroscience formalizes the role of prediction in terms of probabilistic inference. Perception, action, attention, and learning may then be unified as aspects of predictive processing in the brain. This chapter first explains the sense in which predictive processing is inferential and representational. Then follows an exploration of how the predictive processing framework relates to a series of considerations in favor of enactive, embedded, embodied, and extended cognition (4E cognition). The initial impression may be that predictive processing is too representational and inferential to fit well to 4E cognition. But, in fact, predictive processing encompasses many phenomena prevalent in 4E approaches, while remaining both inferential and representational.
Introduction
A millennium ago the great polymath Ibn al Haytham (Alhazen) (ca. 1030; 1989) developed the view that “many visible properties are perceived by judgment and inference” (II.3.16). He knew that there are optical distortions and omissions of the image hitting the eye, which without inference would make perception as we know it impossible ( Lindberg 1976 ; Hatfield 2002 ). Al Haytham was aware it is counterintuitive to say perception depends on typically intellectual activities of judgment and inference and so remarks that “the shape and size of a body . . . and such like properties of visible objects are in most cases perceived extremely quickly, and because of this speed one is not aware of having perceived them by inference and judgment” (II.3.26).
Since al Haytham, many in optics, psychology, neuroscience, and philosophy have advocated the role of inference in perception, and have insisted too that this inference is somehow unconscious (for review, see Hatfield 2002 ). With characteristic clarity, Hermann von Helmholtz coined the phrase unconscious perceptual inference and said that the “psychical activities” leading to perception:
are in general not conscious, but rather unconscious. In their outcomes they are like inferences insofar as we from the observed effect on our senses arrive at an idea of the cause of this effect. This is so even though we always in fact only have direct access to the events at the nerves, that is, we sense the effects, never the external objects. ( Helmholtz 1867 , p. 430)
The starting point for this inferential view is the conviction that perception can be explained only if a particular, fundamental problem of perception is solved, namely, how the brain can construct our familiar perceptual experience on the basis only of the imperfect data delivered to the senses, and without ever having unfettered access to the true hidden causes of that input. This type of problem is also at the heart of massive scientific endeavors in contemporary artificial intelligence and machine learning.
Recently, the notion of unconscious perceptual inference has been embedded in a vast probabilistic theoretical framework covering cognitive science, theoretical neurobiology, and machine learning. The basic idea is that unconscious perceptual inference is a matter of Bayesian inference, such that the brain in some manner follows Bayes’s rule and thereby can overcome the problem of perception. The most comprehensive, ambitious, and fascinating of these probabilistic theories build on the notion of prediction error minimization (PEM) (this notion arose in machine learning research, with versions of it going back to 1950s; for recent philosophical overviews, see Clark 2013 ; Hohwy 2013 ).
Several aspects of unconscious perceptual inference are anathema to many versions of enactive, embedded, embodied, and extended (4E) cognition. If perception is a matter of Bayesian inference, then perception seems a very passive, intellectualist, neurocentric phenomenon of receiving sensory input and performing inferential operations on them in order to build internal representations. This process is divorced from action and active interaction with the environment; it appears insensitive to the situation in which the system is embedded; it leaves no foundational role for the body in cognitive and perceptual processes; and it makes perceptual processes a matter of what happens behind the sensory veil with no possibility of extension to mental states beyond the brain, let alone the body (4E cognition is now a vast and varied area of research; the types of approaches that stress anti-representational and anti-inferential elements are, for example, Varela et al. 1991 ; Clark 1997 ; Noë 2004; Gallagher 2005 ; Thompson 2007 ; Clark 2008 ; Hutto and Myin 2013 ).
The tension between perceptual inference and 4E cognition matters because both are influential attempts at explaining the same range of phenomena. Having noticed the initial tension between them, there are three main options: (1) perceptual inference and 4E cognition are incompatible as foundational accounts of perception and cognition, which means one must be false ( Anderson and Chemero 2013 ; Barrett 2015 ); this option appears unattractive because key aspects of both seem believable and important. The next two options are more discursive: (2) perceptual inference and 4E cognition should be considered compatible, but only because perceptual inference, rightly understood, is not a matter of neurocentric, representationalist inference but yields just the kinds of processes necessary for 4E cognition (Clark 2013 , 2015 , 2016 ). (3) Perceptual inference and 4E cognition should be considered compatible, but only because 4E cognition, rightly understood, is nothing but representation and inference ( Hohwy 2016b ). Options 2 and 3 deflate perceptual inference and 4E cognition, respectively, that is, they achieve reconciliation by recasting one of the sides of the debate in terms of the other.
This chapter aims to show that Option 3 is reasonable. Perceptual inference, in the shape of PEM, is tremendously resourceful and can therefore encompass phenomena highlighted in debates on 4E cognition. Reconciliation with somewhat deflated 4E notions is achieved without compromising PEM’s representationalist and inferentialist essence. This advances the debate about 4E cognition because, in the context of PEM, inference and representation are both shown to have several surprising aspects, such that, perhaps, 4E cognition need not abhor these notions altogether.
The chapter first explains PEM and lays out its specific notion of inference. Then action is subsumed under PEM’s inferential scheme, and the role of representation in perception and action is explained. Finally, select aspects of 4E cognition are incorporated into the PEM fold.
Predictive Processing and Inference
In many approaches to unconscious perceptual inference, the notion of inference is left unspecified; as Helmholtz says, our psychical activities are “like” inference. Here, the notion of inference captures the idea that the perceptual and cognitive systems need to draw conclusions about the true hidden causes of sensory input vicariously, working only from the incomplete information given in the sensory input.
On modern approaches, this is given shape in terms of Bayesian inference. This yields a concrete sense of “inference” where Bayes’s rule is used to update internal models of the causes of the input in the light of new evidence. A Bayesian system will arrive at new probabilistically optimal “conclusions” about the hidden causes by weighting its prior expectations about the causes against the likelihood that the current evidence was caused by those causes (there are useful textbook sources on machine learning such as Bishop 2007 ; philosophical reviews such as Rescorla 2015 ; see also recent treatments of hierarchical Bayes and volatility such as Payzan-LeNestour and Bossaerts 2011 ; Mathys et al. 2014 ).
Consider a series of sensory samples, for example, auditory inputs drawn from a sound source. The question for the perceiver is where the sound source is located (somewhere on a 180° space in front of the perceiver). Assume the samples are normally distributed and that the true source is 80°. Before any samples come in, the perceiver expects—predicts—samples to be distributed around 90°. The first sample comes in indicating 77°, and thereby suggests a prediction error of 13°. Which probabilistic inference should the perceiver make? Inferring that the source is at 77° would disregard prior knowledge and lead to a model overfitted to noise. Ignoring the prediction error would prevent perceptual learning altogether. So the right weight to assign to the prediction error in updating the prior belief of 90° ought to reflect an optimal, rational balance between the prior and the likelihood, and this is indeed what Bayes’s rule delivers. So probabilistic inference should be determined by Bayes’s rule. In other words, the learning rate in Bayesian inference is determined by how much is already known and how much is being learned by the current evidence, reflected in the likelihood. (In this toy example, I set aside the question how the perceiver knows not to add the weighted prediction error to 90°, moving toward 103° and away from 80°; notice that if the system does this, then prediction error will tend to grow over time).
The correct weights to give to the prior and the prediction error can be considered transparently through the variance of their probability distributions. The more the variance, the less the weight. A strong prior will have little variance and should be weighted highly, and a precise input, which fits well the expected values of the model in question, should be weighted highly. The inverse of the variance is called the precision , and it is a mathematically expedient convention to operate with precisions in discussions of inference: the learning rate in Bayesian inference therefore depends on the precisions of the priors and prediction errors. As will become apparent later, precisions are important to PEM and its ability to engage 4E-type issues.
So far, only one inferential step is described. For subsequent samples, Bayes’s rule should also be applied, but for the old inferred posterior as the new prior. Since there is an optimal mix of prior and likelihood, the model will converge on the true mean (80°) in the long run. Critically, in this process, the average prediction error is minimized over the long run. Even for quite noisy samples (imprecise distributions, or probability density functions), a Bayesian inference system will eventually settle on an expectation for the mean that keeps prediction error low. This can be turned around such that, subject to a number of assumptions about the shape of the probability distributions and the context in which they are considered, a system that minimizes prediction error in the long run will approximate Bayesian inference.
The heart of PEM is then the idea that a system need not explicitly know or calculate Bayes’s rule to approximate Bayesian inference. All the system needs is the ability to minimize prediction error in the long run. This is the sense in which unconscious perceptual inference is inference: internal models are refined through prediction error minimization such that Bayesian inference is approximated. The notion of inference is therefore nothing to do with propositional logic or deduction, nor with overly intellectual application of theorems of probability theory.
It would be misguided to withdraw the label “inference” from unconscious perceptual inference, or from PEM, just because it is an approximation to Bayes, or because the process is not an explicit application of a mathematical formalism by the brain. If the inferential aspect is not kept in focus, then it would appear to be a coincidence, or somehow an optional aspect of perceptual and cognitive processes that conform to what Bayes’s rule dictate. Put differently, anyone who subscribes to the notion of predictive processing must also accept the inferential aspect. If it is thrown out, then the “prediction error minimization” part becomes a meaningless, unconstrained notion.
PEM thus says that perceivers harbor internal models that give rise to precision-weighted predictions of what the sensory input should be, and that these predictions can be compared to the actual sensory input. The ensuing prediction error guides the updates of the internal model such that prediction error in the long run is minimized and Bayesian inference approximated.
However, this description of PEM is still too sparse. In any given situation, a PEM system will not know how much or how little to weight prediction error even if it can assess the precisions of the prior and of the current prediction error. In essence, a system that operates with only those precisions will be assuming the world is more simple and persistent than it really is. For example, different sensory modalities have different precisions in different contexts, and without prior knowledge of these precisions, the system can make no informed decisions about how to weight prediction error. For example, similarly sized prediction errors in the auditory and visual modalities should not be weighted the same, since the precisions of each should be expected to be different. Therefore a PEM system would need to have and shape expectations about the precisions as well as the means of probability distributions. The need for such expected precisions is also driven by the occurrence of multiple interacting causes of sensory input within and across sensory modalities. In the example of the location of the auditory source, variability in the sensory sampling might be due to a new cause interfering with the original sound source (e.g., a moving screen intermittently obscures the location of the sound). If the system does not have robust expectations for the precision of the sound source, then it will be unable to make the right inferences about the input (i.e., is it one cause with varying precisions or is it two interacting causes that gives rise to the nonlinear evolution in the auditory sensory input?).
A PEM system must model expectations of precisions, and this part of the PEM system itself needs to be Bayes-optimal. Models will harbor priors for precisions; they will predict precisions and generate precision prediction errors. Moreover, they will need to do this across all the hidden causes modeled such that their interactions can be taken into account. This calls for a hierarchical structure where the occurrence of various causes over many different time scales can impact on the predictions of the sensory input received at any given time. For example, the interaction of relatively slow time scale regularities (e.g., the trains driving past your house two or three times an hour) need to influence the predictions of faster time scale regularities (e.g., the words heard in a conversation in your lounge room), and vice versa.
A PEM system that operates in a complex environment, with levels of uncertainty that depend on the current state of the world and many interacting causes at many different time scales, will thus build up a vast internal model with many interacting, hierarchically ordered levels, which all pass messages to each other in an attempt to minimize average prediction error over the long term.
Consider finally what happens over time to the models harbored in the brain, on the basis of which predictions are made and prediction errors minimized. The parameters of these models will be shaped by the Bayesian inferential process to mirror the causes of the sensory input. In the example earlier, by minimizing prediction error over time for the location of the cause of auditory input, the model will revise its initial false belief that the location is at 90°, and come to expect it to be at its true position of 80°. Further, by minimizing precision prediction error, the model may be able to anticipate interacting causes, such as a moving screen intermittently blocking the sound. This means that, by approximating Bayesian inference, the models of a PEM system must represent its world.
Here, the notion of representation is not just a matter of receptor covariance, where the states of neural populations covary with the occurrence of certain environmental causes. The hierarchical model is highly structured, and performs operations over the parameters. For example, there will be model selection. In our example, the system might ask whether there is another cause interacting with the sound source, or if the signal itself is becoming noisier. In addition, there are convolutions of separate expected signals generated on the basis of the models; for example, when a cat and a fence are detected, the expected sensory signals from both hidden causes are convolved into one stream by the brain to take the occlusion of the cat by the fence into account. As will become clear, the representational aspects of PEM are critical when it comes to incorporating action, too.
The representational nature of a PEM system is not optional. The ability to minimize prediction error over time depends on building better and better representations of the causes of its sensory input. This is encapsulated in the very notion of model revision in Bayesian inference. (There is extensive discussion of what it takes for perception to be representational; for examples of relevance to Bayesian inference, see Ramsey 2007 ; Orlandi 2013 , 2014 ; Gładziejewski 2015; Ramsey 2015 .)
So far, it appears that predictive processing is inferential and representational in a specific Bayesian sense. Traditionally, 4E approaches have rejected both notions. Next, PEM will be shown to have explanatory reach into 4E cognition too.
PEM and Action
A representationalist and inferentialist account of cognition and perception may appear divorced from the concerns and activities of a real, embodied agent operating in its environment. Thus enactive and embodied accounts have de-emphasized classic representationalist understandings of cognition and perception and with it much semblance to inference (there are many versions and much discussion of embodiment; see, e.g., Brooks 1991 ; Noë 2004; Gallagher 2005 ; Alsmith and Vignemont 2012 ; Hutto and Myin 2013 ; Orlandi 2014 ).
Perhaps the basic sentiment could be summed up in the strong intuition that embodied action is not inference, and yet the body and its actions are crucial to gain any kind of understanding of perception and cognition. PEM can, however, easily cast action as a kind of inference—as active inference (Friston, Samothrakis, et al. 2012).
Recall that any system that minimizes prediction error over time will approximate Bayesian inference; that is, such a system will be inferential in the Bayesian sense that it increases the evidence for its internal model. Using the example from earlier again, by minimizing prediction error the system could accumulate evidence for the model that represents the sound source as located at 80°. In that case, the internal model is revised from the initial 90° to the new estimate of 80°.
It is trivial to observe that the perceiver could also have minimized prediction error by turning the head 10° to the left and thereby have accumulated evidence for the prediction that the sound source is located at 90°. Prediction error can be minimized both through passive updating of the internal model and through active changes to the sensory input. Action, such as turning one’s head, can therefore minimize prediction error. Since, as argued earlier, minimizing prediction error is inference, and action is inference. There is then no hindrance to incorporating action into an inferentialist framework.
In active inference, representations are central to guiding action. This is because action only occurs when a hypothesis—in this case a representation of a state that is yet to occur—has accumulated sufficient evidence relative to other hypotheses to become the target of PEM. This yields two aspects that are sometimes seen as hallmarks of representations: they are action-guiding and they are somehow detached from what they stand for (for discussion and review, see Orlandi 2014 ). Active inference therefore has a good claim to be both inferential and representational.
For perceptual inference, precisions were shown to be critical. Without precisions, the PEM system would not be able to minimize error in a world with state-dependent uncertainty and interacting causes. The same holds for active inference. Without any notion of how levels of prediction error tend to shift over many interacting time scales, the system would pick the action that minimizes most error here and now—for example, by entering and remaining in a dark room (for discussion, see Friston, Thornton, et al. 2012). This would be analogous to overfitting, and would come at the cost of increasing prediction error over the longer term. For example, even though the perceiver might minimize prediction error by forcing the sound to come at the 90° midline, this might make it difficult to ascertain the true source of a potentially moving cause such as the trajectory of a mosquito buzzing about (since direction detection is harder over the midline due to minimal interaural time difference). This calls for even more hierarchical model-building, namely, in terms of the precisions expected in the evolution of the prediction error landscape as a result of the agent’s active intervention in the world. These self-involving, modeled regularities are, however, not fundamentally different from the regularities involved in perceptual inference. They simply concern the sensory input the agent should expect to result from the interaction of one particular cause in the world—the agent itself—with all the other causes of sensory input (for discussion of self-models, see, e.g., Synofzik et al. 2008 ; Metzinger 2009 ).
There is thus room for a notion of action within PEM. But this possibility alone does not imply that a PEM system is likely to actually be an agent. If the system is endowed with a body such that it could act, then the imperative for minimization of prediction error will make actual action highly likely.
If the system has accumulated strong evidence for, say, an association between two sounds, it may still be unable to distinguish several hypotheses, for example, whether the sounds are related as cause and effect or if they are effects of some common cause. It is standard in the causal inference literature that intervention is required to acquire evidence for or against these hypotheses ( Pearl 2000 ; Woodward 2003 ). For example, if variation in one sound persists even if the other sound is actively switched off, then that is evidence the latter sound is not the cause of the first. The necessity of action is generalized in the observation from earlier that the system needs to learn differences in precisions and patterns of interactions among causes, such as occlusions and other causal relations that change the sensory input in nonlinear ways. Such learning thus requires action. The price of not engaging the body plant to intervene in the environment is that prediction error will tend to increase since predictions will be unable to distinguish between several different hypotheses. A PEM system that can act will therefore be best served to actually act.
This simple account of agency has profound consequences. It will be a learnable pattern in nature that inaction will tend to increase prediction error in the longer term (due to the inaccuracy of the hypotheses the system can accumulate evidence for by using only passive inference). Conversely, the system can learn that action tends to allow minimization of prediction error at reasonable time scales. Overall, this teaches the system that, on balance, its model will accumulate more precise evidence through action than through inaction. This will bias it to minimize prediction error through active inference. Of course, a system that only ever acts on the basis of unchanging models will never be able to learn new patterns, which is detrimental in a changing world. Therefore action must be interspersed with perceptual inference where models are updated, before new action takes place.
The mechanism by which this switching between perception and action takes place is best conceived in terms of precision optimization. Recall that the PEM system will build up expectations for precisions, which are crucial for dealing with state-dependent noise in a world with interacting causes. The role of expected precisions in inference is to optimally adjust weights for expected sensory input: input that is expected to be precise is favored in Bayesian inference whereas input that is expected to be imprecise is not favored. Mechanistically, this calls for a neuronal gating mechanism that inhibits or excites sensory inputs according to their expected precisions. This gating mechanism serves as a kind of probabilistic searchlight and thus plays the functional role of attention ( Feldman and Friston 2010 ; Brown et al. 2011 ; Hohwy 2012 , 2016a ).
As the system gates its sensory input according to where it expects the most precise sensory input will occur, across several time scales, it may switch between perception and action. For example, if more precision is expected by the agent having its hand at the position of the coffee cup rather than at the current position at the laptop, then it will begin gating the current sensory input, which suggests the hand is at the laptop. This in turn allows the coffee hypothesis to gain relative weight over the laptop hypothesis, and the prediction error generated by that hypothesis can easily by minimized by moving the hand. Since the gain is high on this prediction error, the new hypothesis quickly accumulates evidence for its truth, and the hand will find itself at the coffee cup (for more on the dynamics of action and perception in relation to temporal phenomenology, see Hohwy et al. 2015 ; for the formal background, see Friston, Trujillo-Barreto et al. 2008).
Embodied, Embedded, and Inferential and Representational
When all the elements described in the last section are combined, a wholly inferential conception of agency begins to take shape. If action and agency are moments of PEM, then desires are just beliefs (or priors) about states that happen to be future, with a focus on their anticipated levels of prediction error, and where reward is the absence of prediction error. This suggests a neat continuity with perceptual inference, which also relies on priors and the imperative to minimize prediction error.
The idea that action is driven by PEM relative to a model does raise a question about the content of the model relative to which error is minimized. This model is what defines what we would normally describe as the agent’s desires. In the wider PEM framework—which, as shall be described later, relies on notions of free energy minimization —the expected states that anchor active inference relate to set points in terms of the organism’s homeostasis. This immediately evokes an evolutionary perspective, where expected bodily states are central to behavior. Apart from the specific evolutionary aspects, this suggests an embodiment perspective, because all aspects of perception and cognition then have a foundation in bodily states, and movement and purposeful behavior have a foundation in the environment. This element of embodiment makes it more likely that contact can be made between probabilistic theories of perception and action and embodied cognition approaches (such as, e.g., Varela et al. 1991 ; Gallagher 2005 ; Thompson 2007 ; for recent treatments that relate to PEM, see Bruineberg and Rietveld 2014 ; Fazelpour and Thompson 2015 ).
However, even this foundational embodiment is conceived probabilistically in PEM. A set of expectations for bodily states (relating to homeostasis) is essentially a model. In probabilistic terms, this model gives the probability of finding the organism in some subset of the overall set of states it could be in. The model is specified in terms of internal states, as signaled in interoception, but is tied to the overall setting of the organism in a subset of environmental states. The expected states defined in interoceptive terms would, in real organisms traversing actual environments, be mirrored in the expected states described in environmental terms, or in terms of their sensory input or exteroception. For example, fish are most likely to find their sensory organs impinged upon from watery states and this is associated strongly with the homeostatic needs specified in their model. In general, within this probabilistic reading of the foundational embodiment of a PEM organism, there is thus a tight coupling between the interoceptive and exteroceptive prediction error landscapes for any PEM system.
Not only does PEM provide a notion of embodiment, it also speaks to elements of embedded or situated cognition (see van Gelder 1995 ; Clark 1997 ; Aydede and Robbins 2009 ). With the tight coupling of the organism’s expected states in terms of interoception and exteroception, perception and cognition cannot be separated from bodily or environmental aspects of the PEM system.
Crucially, this reading of embodiment and embedding leads directly to inferential processing and PEM. The model specifies the probability of finding the organism in any one of all the possible states. To know this model directly would require the agent averaging over all possible states and ascertaining the occurrence of itself in them. This is not possible for a finite organism to learn directly. Instead, the organism must essentially guess what its expected states are and minimize the ensuing error through perceptual and active inference. In slightly more formal terms, the organism needs to minimize surprise; that is, it needs to avoid finding itself in states that are surprising given its model. The sum of prediction error is always equal to or larger than the surprise, so minimizing prediction error will implicitly minimize surprise. This bound on surprise is also known in probabilistic terms as the free energy, and so this challenging idea is enshrined in the so-called free energy principle ( Friston 2010 ).
When viewed in this larger context of the free energy principle, promising notions of embodied and embedded cognition present themselves. More research is needed on the extent to which they capture facets of the wide-ranging and heterogeneous 4E body of research. However, for the conception of embodiment and embedding mooted here, an inferential conception is inescapable.
Hierarchical Inference for a Changing World
In much 4E research there is a focus on fluid interactions with the world, characterized by non-inferential, nonrepresentational, “quick and dirty” processing. This picture is set up to contrast with inferential, representational, “slow and clean” processing (Clark 1997 , 2013 , 2015 ). Often, this kind of quick and dirty, situated cognition is discussed in terms of affordances : salient elements of the environment that are in some sense perceived directly and are immediately action-guiding. Affordances in quick and dirty processing are thought to evade the computational bottleneck that a traditional representational system would have trying to passively encode the entire sensory input presented at any given time. For some types of action and at some stages of learning, performance is rather plodding and sluggish, but there is an important insight in how the notion of situated cognition highlights the fluid swiftness with which organisms can perform some complex actions in their environment.
In a PEM system there is no bottleneck problem in the first place, however. There is never an issue of starting from scratch and encoding an entire natural scene in order to be able to perceive it. Hierarchical Bayesian inference is based on prior learning, which over time has shaped priors at many levels. Given priors, the sensory input is no longer something that needs to be encoded here and now. Instead the sensory input is, functionally speaking, the feedback to the forward predictive signal generated by the brain’s internal model ( Friston 2005 ). The model predicts what will happen and gets confirmation or disconfirmation on these predictions from the sensory input. There is thus no encoding of the entire sensory input in each perceptual instance. This means the PEM system has no need to resort to quick and dirty processing tricks to overcome a computational bottleneck. Instead, the system relies on slow and clean learning in order to facilitate swift and fluid perception of and interaction with the world. This learning is “slow” because is relies on meticulous accumulation of evidence for hypotheses at multiple time scales. It is “clean” because the learning slots into a hierarchy with clearly defined, general functional roles for time scales, for predictions of values, and for predictions of precisions.
The difference between swift and fluid processing and plodding and sluggish processing can easily be accommodated within a PEM system. Affordances are just causes of sensory input that, on the basis of prior learning, are strongly expected to give rise to high precision prediction error. To maintain Bayes optimality, the system gates sensory input accordingly, and strongly focuses both perceptual and active inference on these affordances. In this setting, PEM happens quickly, since highly precise distributions are easier to deal with computationally than imprecise ones. This means that the agent in question will obtain its expected states swiftly and fluidly.
Typically, the 4E preference for quick and dirty processing and affordances comes with a rejection of rich representational states (Clark 2008 , 2015 ). The point is that such representations cannot come about due to the bottleneck problem. Moreover, the appeal to affordance-based quick and dirty processing is thought to obviate the need for rich internal representations altogether as the world’s affordances in some sense are its own representation ( Brooks 1991 ).
On the PEM-based account of swift and fluid processing, internal representations are, however, necessary. Over time, multilayered representations are constructed and shaped, and Bayesian model selection picks the model with the best evidence as the representation of the world relative to which prediction error is minimized in active inference (this kind of approach is developed in more detail for PEM in Seth 2014 , 2015 ). Again, we get the result that PEM has the resources to speak to typical 4E discussions, but that it happens on the basis of representation and inference.
It could be that the brain builds rich representations as it learns about the world, and then gradually substitutes these much sparser and representation-poor, purpose-made representations that more directly tie in with and engage the environment. One argument here derives from Occam’s razor, in the sense that there are simplicity gains from opting for a simple over a complex, rich model ( Clark 2015 ). However, simplicity is not something additional to inference. Complex models are to be avoided because they are overfitted and thereby incur a prediction error cost in the longer run. How rich or simple a model should be is thus fully given by PEM in the first place.
In fact, there is reason to think the PEM account is preferable to the affordance-based account. It is true that swift and fluid processing is a salient and impressive aspect of human cognition. But so is the flexible way we shift between contexts, projects, beliefs, and actions. We might engage in attentive, fluid, and swift interaction for a period of time, but other beliefs and concerns always creep in and make it imperative to shift to another behavior. On the affordance-based account, it is not readily explained how the agent might disengage from a given set of affordances; the focus is at best on how representation-rich learning is needed before swift and fluid processing is possible, rather than the role of rich representation during swift and fluid processing. The agent seems tightly knitted to its environment, and it is not clear how the agent can step back and reconsider its current course of action.
In contrast, flexible cognition is a central motivation for adopting PEM’s hierarchical Bayesian inference in the first place. Active inference is driven by the most probable hypothesis at any given time. The system will have built up expectations not just for what the most likely causes of sensory input might be but also for the typical evolution of prediction error precision. In particular, there will be accumulated evidence that any given hypothesis under which prediction error is minimized at a certain time will have a limited life span—in essence, the system will know that it lives in a changing world where precise evidence for any given hypothesis will soon begin to be hard to find. For example, as the agent fluidly and swiftly catches baseballs, it will know that the sun will soon set and make the visual input imprecise. It will therefore begin accumulating evidence for the next hypothesis (e.g., “I am eating dinner”) under which evidence will soon begin to be accumulated and prediction error minimized.
This speaks to a crucial balance, which a PEM system must obtain. As prediction error is minimized in active inference, the hypothesis relative to which error is minimized is held stable. This means that, as prediction error is minimized, the world can in fact change “behind the scenes” to such an extent that it would eventually be better to abandon the current hypothesis and adopt a new one. Anticipating such change in the environment matters greatly to the agent because it should never engage in any behavior, no matter how swift and fluid, for so long that when it ceases the behavior, the world has changed in other respects and predictive error will be very large. A PEM agent therefore will be inclined to believe that the current state of affairs will change, and therefore the agent will intersperse active inference with perceptual inference, where the internal model is checked and the size of the overall prediction error is adjusted and tightened up before a new hypothesis is selected for active inference (see Hohwy 2013 ; Hohwy et al. 2015 ).
A hierarchical system operating with slow and clean processing can thus economically explain both swift and fluid, affordance-based cognition as well as flexible cognition. This is an important point to make in the context of PEM’s affinity to 4E cognition. The motivation for PEM is, in the end, the simple observation that we live in a changing world. Our world presents many different causes of our sensory input, and these causes interact with each other to create nonlinearities in the input; moreover, these interactions happen concurrently at many different time scales (e.g., “The setting sun makes the balls hard to see, but this time of the year the janitor often turns on the floodlights at the far pitch”). This complexity is what creates the need for hierarchical Bayesian inference in the first place: a rich internal model that keeps track of all these contingencies and can mix the various causes in the right way to anticipate the sensory input. This has a 4E-type ring to it: the cognitive system is the way it is because the agent’s world and body are the way they are. In particular, PEM is not the best solution for non-ecological, lab-style model environments where typically context and interactions between hidden causes are kept to a minimum. In other words, a machine learning researcher who never tests their system against the real world will have little impetus to build a PEM system. On 4E approaches, there is also a strong focus on real-world settings, but the response is typically to tie the agent very closely to its environment. This, however, makes it harder to see how the real world, and also that fact that the real world is a changing place, can be taken into consideration. PEM, in contrast, makes room for the changing world by retracting farther away from the world, into a vast internal model that seeks to represent the full richness of the world and the way it changes over many time scales. On the PEM conception of the agent’s place in the world, cognition is not a matter of being closely in tune with and driven by the sensory input. Rather, cognition is a matter of having richly represented expectations for the world and the body and seeking confirming feedback on those expectations through the senses.
The Mind and Things Without It
Both perception and action are inferential and representational. The PEM system’s process of minimizing prediction error implies that the sensory input is explained away on the basis of the evolving hypotheses of an internal model. The more the system can minimize its prediction error, the more it will accumulate evidence for its own model. This is a trivial observation: if I can minimize prediction error for my theory that my hamster has escaped, the more evidence I have for that theory. If we consider the PEM system an agent, then it acquires evidence for its own existence through its activities ( Friston 2010 ). Borrowing a term from philosophy of science, the PEM system can thus be said to be self-evidencing ( Hempel 1965 ; Hohwy 2016b ).
A self-evidencing system creates a sensory boundary between itself (i.e., the model) and the causes of its sensory input. This again is a trivial consequence of self-evidencing: there is something that garners evidence and then there is what the evidence is evidence of. Or again, in both perceptual and active inference there is something doing the inference and something being inferred. This boundary can also be described in terms of causal nets, where a set of inner states (i.e., brain states) can be said to have a “Markov blanket” ( Pearl 1988 ) consisting of the inner states’ parents (i.e., the sensory states) and their children and other parents of the children (i.e., the active states driving active inference) ( Friston 2013 ; Hohwy 2015 , 2017 ; causal Bayes nets must be acyclic, but brains have recurrent (cyclic) states; there are technical ways, such as dynamical Bayes nets, to deal with such problems). The activity of the states within a Markov blanket is wholly determined by the states of the blanket. In principle, nothing about the environmental states beyond the blanket need be known to know what the system is doing. By extension, in principle, only the states of the sensory organs need be known to know everything the mind does.
PEM then comes with a principled way of drawing a boundary between the mind and the outside world. If a particular state is part of what is doing the inference, then it must be within the sensory boundary, as a part of what approximates inference about outside causes of sensory input. This may relate to the vigorous debate about extended cognition ( Clark and Chalmers 1998 ; Clark 2008 ), which is the last member of 4E cognition to discuss.
Extended cognition is the idea that some objects, such as notebooks and smartphones, play such an integrated, memory-like function in the mental economy of some agents that, by parity of reasoning, they should be considered part of the agent’s mental states even though they reside outside the central nervous system. There is much discussion of this idea (see, e.g., Menary 2007 ; Adams and Aizawa 2008 ; Anderson et al. 2012 ; Spaulding 2012 ). PEM brings with it a new way of thinking about the role of such external objects. On the one hand, these objects are inferred (e.g., on the basis of the sensory input from the notebook) and as such they are outside the mental states of the system. On the other hand, if the extended cognition hypothesis is correct, they are within the sensory boundary, forming part of the inner states behind a Markov blanket inferring the hidden causes beyond it.
Interpreting purported cases of extended cognition according to PEM thus leaves two main options. There might be contradiction, since something cannot be both within and beyond the same boundary at the same time. Or, there might be multiple coexisting sensory boundaries. The second option is very interesting and very likely to be true, since Markov blankets occur easily. There is an associated cost, however: we have identified the inner states (or the model) with the agent, and if there are multiple Markov blankets then there are multiple agents coexisting at the same time. Though this may be true in a weak sense of agent, it is explanatorily messy. When asking which agent is acting, there would then be a multitude of correct answers, depending on how many nested Markov blankets are involved in the same action. This speaks in favor of using inference to the best explanation to identify the agent whose relatively invariant involvement accounts for most of observed behavior over time. It seems likely this more pragmatically identified agent would be the agent as specified by the model harbored just in the nervous system. This is the agent relative to which prediction error is minimized over the longer time scale, which as we saw is central to understanding predictive processing accounts in the first place (for discussion, see Hohwy 2016b ). Bringing this discussion back to extended cognition, the pragmatic method of identifying the agent suggests that there is no extended cognition, since the special objects in question are beyond the one Markov blanket. The more lax way of identifying agents suggests that extended cognition ambiguous, since the special objects are beyond some blankets and within others.
The existence of the sensory boundary or Markov blanket implies that perception and agency are confined to the inner states of the PEM system (wherever the boundary or boundaries of the system are located). Those inner states will mirror the states outside the boundary: the inner states will, through PEM, come to represent the worldly causes of the sensory input impinging at the system’s periphery. Conversely, through active inference, the outside states will come to conform to the expectations harbored in the internal states.
There is then an intriguing duality to this sensory boundary between mind and world. On the one hand, the boundary is epistemic (cf. self-evidencing): the worldly causes can only be known vicariously, through inference on sensory input. On the other hand, the boundary is characterized in causal terms (cf. Markov blanket): there is a dynamic coupling between mind and world, enabled through both perception and action.
This duality summarizes well why PEM is a good fit for many of the issues in 4E debates: PEM is able to throw light on embodied agents dynamically interacting with the environment in which they are embedded. This good fit with 4E cognition is, however, made possible precisely because PEM is inferential and representational.
Adams, F. and Aizawa, K. ( 2008 ). The bounds of cognition . Oxford: Blackwell.
Google Scholar
Google Preview
Alsmith, A.J.T. and Vignemont, F. ( 2012 ). Embodying the mind and representing the body. Review of Philosophy and Psychology , 3(1), 1–13.
Anderson, M. and Chemero, A. ( 2013 ). The problem with brain GUTs: conflation of different senses of “prediction” threatens metaphysical disaster. Behavioral and Brain Sciences , 36, 204–5.
Anderson, M.L. , Richardson, M.J. , and Chemero, A. ( 2012 ). Eroding the boundaries of cognition: implications of embodiment. Topics in Cognitive Science , 4(4), 717–30.
Aydede, M. and Robbins, P. ( 2009 ). The Cambridge handbook of situated cognition . New York: Cambridge University Press
Barrett, L. ( 2015 ). A better kind of continuity. The Southern Journal of Philosophy , 53, 28–49.
Bishop, C.M. ( 2007 ). Pattern recognition and machine learning . Dordrecht: Springer.
Brooks, R.A. ( 1991 ). Intelligence without representation. Artificial Intelligence , 47(1–3), 139–59.
Brown, H. , Friston, K.J. , and Bestmann, S. ( 2011 ). Active inference, attention and motor preparation. Frontiers in Psychology , 2, 218.
Bruineberg, J. and Rietveld, E. ( 2014 ). Self-organization, free energy minimization, and optimal grip on a field of affordances. Frontiers in Human Neuroscience , 8, 599.
Clark, A. ( 1997 ). Being there. Cambridge, MA: MIT Press.
Clark, A. ( 2008 ). Supersizing the mind: embodiment, action, and cognitive extension . New York: Oxford University Press.
Clark, A. ( 2013 ). Whatever next? Predictive brains, situated agents, and the future of cognitive science. Behavioral and Brain Sciences , 36(3), 181–204.
Clark, A. ( 2015 ). Radical predictive processing. The Southern Journal of Philosophy , 53, 3–27.
Clark, A. ( 2016 ). Surfing uncertainty . New York: Oxford University Press.
Clark, A. and Chalmers, D. ( 1998 ). The extended mind. Analysis 58(1), 7–19.
Fazelpour, S. and Thompson, E. ( 2015 ). The Kantian brain: brain dynamics from a neurophenomenological perspective. Current Opinion in Neurobiology , 31, 223–9.
Feldman, H. and Friston, K. ( 2010 ). Attention, uncertainty and free-energy. Frontiers in Human Neuroscience , 4, 215.
Friston, K. ( 2010 ). The free-energy principle: a unified brain theory? Nature Reviews Neuroscience , 11(2), 127–38.
Friston, K. ( 2013 ). Life as we know it. Journal of The Royal Society Interface , 10, 20130475.
Friston, K. , Samothrakis, S. , and Montague, R. ( 2012 ). Active inference and agency: optimal control without cost functions. Biological Cybernetics , 106(8), 523–41.
Friston, K. , Thornton, C. , and Clark, A. ( 2012 ). Free-energy minimization and the dark room problem. Frontiers in Psychology , 3, 130.
Friston, K.J. ( 2005 ). A theory of cortical responses. Philosophical transactions of the Royal Society of London. Series B, Biological sciences , 369(1456), 815–36.
Friston, K.J. , Trujillo-Barreto, N. , and Daunizeau, J. ( 2008 ). DEM: a variational treatment of dynamic systems. NeuroImage , 41(3), 849–85.
Gallagher, S. ( 2005 ). How the body shapes the mind . Oxford: Oxford University Press.
Gładziejewski, P. ( 2015 ). Predictive coding and representationalism. Synthese , 193(2), 559–82.
Hatfield, G. ( 2002 ). Perception as unconscious inference. In: D. Heyer and R. Mausfeld (eds.), Perception and the physical world . Chichester: John Wiley & Sons, Ltd., pp. 113–43.
Helmholtz, H.v. ( 1867 ). Handbuch der Physiologishen Optik . Leipzig: Leopold Voss.
Hempel, C.G. ( 1965 ). Aspects of scientific explanation and other essays in the philosophy of science . New York: Free Press.
Hohwy, J. ( 2012 ). Attention and conscious perception in the hypothesis testing brain. Frontiers in Psychology , 3, 96.
Hohwy, J. ( 2013 ). The predictive mind . Oxford: Oxford University Press.
Hohwy, J. ( 2015 ). The neural organ explains the mind. In: T. Metzinger and J.M. Windt (eds.), Open MIND . Frankfurt am Main: MIND Group, pp. 1–23.
Hohwy, J. ( 2016 a). Prediction, agency, and body ownership. In: A.K. Engel , K.J. Friston , and D. Kragic (eds.), The pragmatic turn: toward action-oriented view in cognitive science . Cambridge, MA: MIT Press.
Hohwy, J. ( 2016 b). The self-evidencing brain. Noûs , 50(2), 259–85.
Hohwy, J. ( 2017 ). How to entrain your evil demon. In: T.K. Metzinger and W. Wiese (eds.), Philosophy and predictive processing . Frankfurt am Main: MIND Group. doi:10.15502/9783958573048
Hohwy, J. , Paton, B. , and Palmer, C. ( 2015 ). Distrusting the present. Phenomenology and the Cognitive Sciences , 15(3), 315–35.
Hutto, D. and Myin, E. ( 2013 ). Radicalizing enactivism: basic minds without content . Cambridge, MA: MIT Press.
Lindberg, D.C. ( 1976 ). Theories of vision: from Al Kindi to Kepler . Chicago: University of Chicago Press.
Mathys, C.D. , Lomakina, E.I. , Daunizeau, J. , Iglesias, S. , Brodersen, K.H. , Friston, K.J. et al. ( 2014 ). Uncertainty in perception and the hierarchical Gaussian filter. Frontiers in Human Neuroscience , 8, 825.
Menary, R. ( 2007 ). Cognitive integration: mind and cognition unbounded . Basingstoke, UK: Palgrave Macmillan.
Metzinger, T. ( 2009 ). The ego tunnel . New York: Basic Books.
Noë, A. ( 2004 ). Action in perception . Cambridge, MA: MIT Press.
Orlandi, N. ( 2013 ). Embedded seeing: vision in the natural world. Noûs , 47(4), 727–47.
Orlandi, N. ( 2014 ). The innocent eye: why vision is not a cognitive process . Oxford: Oxford University Press.
Payzan-LeNestour, E. and Bossaerts, P. ( 2011 ). Risk, unexpected uncertainty, and estimation uncertainty: Bayesian learning in unstable settings. PLoS Comput Biol , 7(1), e1001048.
Pearl, J. ( 1988 ). Probabilistic reasoning in intelligent systems: networks of plausible inference . San Fransisco: Morgan Kaufmann Publishers.
Pearl, J. ( 2000 ). Causality . Cambridge: Cambridge University Press.
Ramsey, W. ( 2007 ). Representation reconsidered . Cambridge: Cambridge University Press.
Ramsey, W. ( 2015 ). Must cognition be representational? Synthese , 1–18.
Rescorla, M. ( 2015 ). Bayesian perceptual psychology. In: M. Matthen (ed.), The Oxford handbook of the philosophy of perception . Oxford: Oxford University Press, pp. 694–716.
Seth, A.K. ( 2014 ). A predictive processing theory of sensorimotor contingencies: explaining the puzzle of perceptual presence and its absence in synesthesia. Cognitive Neuroscience , 5(2), 97–118.
Seth, A.K. ( 2015 ). The cybernetic Bayesian brain. In: T.K. Metzinger and J.M. Windt (eds.), Open MIND . Frankfurt am Main: MIND Group, pp. 1–24.
Spaulding, S. ( 2012 ). Overextended cognition. Philosophical Psychology , 25(4), 469–90.
Synofzik, M. , Vosgerau, G. , and Newen, A. ( 2008 ). I move, therefore I am: a new theoretical framework to investigate agency and ownership. Consciousness and Cognition , 17(2), 411–24.
Thompson, E. ( 2007 ). Mind in life: biology, phenomenology, and the sciences of mind . Harvard: Harvard University Press.
van Gelder, T. ( 1995 ). What might cognition be, if not computation? Journal of Philosophy , 91, 345–81.
Varela, F. , Thompson, E. , and Rosch, E. ( 1991 ). The embodied mind . Cambridge, MA: MIT Press.
Woodward, J. ( 2003 ). Making things happen . New York: Oxford University Press.
- About Oxford Academic
- Publish journals with us
- University press partners
- What we publish
- New features
- Open access
- Institutional account management
- Rights and permissions
- Get help with access
- Accessibility
- Advertising
- Media enquiries
- Oxford University Press
- Oxford Languages
- University of Oxford
Oxford University Press is a department of the University of Oxford. It furthers the University's objective of excellence in research, scholarship, and education by publishing worldwide
- Copyright © 2024 Oxford University Press
- Cookie settings
- Cookie policy
- Privacy policy
- Legal notice
This Feature Is Available To Subscribers Only
Sign In or Create an Account
This PDF is available to Subscribers Only
For full access to this pdf, sign in to an existing account, or purchase an annual subscription.
Scientific Hypothesis, Model, Theory, and Law
Understanding the Difference Between Basic Scientific Terms
Hero Images / Getty Images
- Chemical Laws
- Periodic Table
- Projects & Experiments
- Scientific Method
- Biochemistry
- Physical Chemistry
- Medical Chemistry
- Chemistry In Everyday Life
- Famous Chemists
- Activities for Kids
- Abbreviations & Acronyms
- Weather & Climate
- Ph.D., Biomedical Sciences, University of Tennessee at Knoxville
- B.A., Physics and Mathematics, Hastings College
Words have precise meanings in science. For example, "theory," "law," and "hypothesis" don't all mean the same thing. Outside of science, you might say something is "just a theory," meaning it's a supposition that may or may not be true. In science, however, a theory is an explanation that generally is accepted to be true. Here's a closer look at these important, commonly misused terms.
A hypothesis is an educated guess, based on observation. It's a prediction of cause and effect. Usually, a hypothesis can be supported or refuted through experimentation or more observation. A hypothesis can be disproven but not proven to be true.
Example: If you see no difference in the cleaning ability of various laundry detergents, you might hypothesize that cleaning effectiveness is not affected by which detergent you use. This hypothesis can be disproven if you observe a stain is removed by one detergent and not another. On the other hand, you cannot prove the hypothesis. Even if you never see a difference in the cleanliness of your clothes after trying 1,000 detergents, there might be one more you haven't tried that could be different.
Scientists often construct models to help explain complex concepts. These can be physical models like a model volcano or atom or conceptual models like predictive weather algorithms. A model doesn't contain all the details of the real deal, but it should include observations known to be valid.
Example: The Bohr model shows electrons orbiting the atomic nucleus, much the same way as the way planets revolve around the sun. In reality, the movement of electrons is complicated but the model makes it clear that protons and neutrons form a nucleus and electrons tend to move around outside the nucleus.
A scientific theory summarizes a hypothesis or group of hypotheses that have been supported with repeated testing. A theory is valid as long as there is no evidence to dispute it. Therefore, theories can be disproven. Basically, if evidence accumulates to support a hypothesis, then the hypothesis can become accepted as a good explanation of a phenomenon. One definition of a theory is to say that it's an accepted hypothesis.
Example: It is known that on June 30, 1908, in Tunguska, Siberia, there was an explosion equivalent to the detonation of about 15 million tons of TNT. Many hypotheses have been proposed for what caused the explosion. It was theorized that the explosion was caused by a natural extraterrestrial phenomenon , and was not caused by man. Is this theory a fact? No. The event is a recorded fact. Is this theory, generally accepted to be true, based on evidence to-date? Yes. Can this theory be shown to be false and be discarded? Yes.
A scientific law generalizes a body of observations. At the time it's made, no exceptions have been found to a law. Scientific laws explain things but they do not describe them. One way to tell a law and a theory apart is to ask if the description gives you the means to explain "why." The word "law" is used less and less in science, as many laws are only true under limited circumstances.
Example: Consider Newton's Law of Gravity . Newton could use this law to predict the behavior of a dropped object but he couldn't explain why it happened.
As you can see, there is no "proof" or absolute "truth" in science. The closest we get are facts, which are indisputable observations. Note, however, if you define proof as arriving at a logical conclusion, based on the evidence, then there is "proof" in science. Some work under the definition that to prove something implies it can never be wrong, which is different. If you're asked to define the terms hypothesis, theory, and law, keep in mind the definitions of proof and of these words can vary slightly depending on the scientific discipline. What's important is to realize they don't all mean the same thing and cannot be used interchangeably.
- Theory Definition in Science
- Hypothesis, Model, Theory, and Law
- What Is a Scientific or Natural Law?
- Scientific Hypothesis Examples
- The Continental Drift Theory: Revolutionary and Significant
- What 'Fail to Reject' Means in a Hypothesis Test
- What Is a Hypothesis? (Science)
- Hypothesis Definition (Science)
- Definition of a Hypothesis
- Processual Archaeology
- The Basics of Physics in Scientific Study
- What Is the Difference Between Hard and Soft Science?
- Tips on Winning the Debate on Evolution
- Geological Thinking: Method of Multiple Working Hypotheses
- 5 Common Misconceptions About Evolution
- Deductive Versus Inductive Reasoning
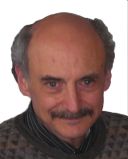
Hypotheses Versus Predictions
Hypotheses and predictions are not the same thing..
Posted January 12, 2018
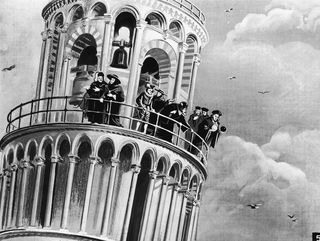
Blogs are not typically places where professors post views about arcane matters. But blogs have the advantage of providing places to convey quick messages that may be of interest to selected parties. I've written this blog to point students and others to a spot where a useful distinction is made that, as far as I know, hasn't been made before. The distinction concerns two words that are used interchangeably though they shouldn't be. The words are hypothesis (or hypotheses) and prediction (or predictions).
It's not uncommon to see these words swapped for each other willy-nilly, as in, "We sought to test the hypothesis that the two groups in our study would remember the same number of words," or "We sought to test the prediction that the two groups in our study would remember the same number of words." Indifference to the contrast in meaning between "hypothesis" and "prediction" is unfortunate, in my view, because "hypothesis" and "prediction" (or "hypotheses" and "predictions") mean very different things. A student proposing an experiment, or an already-graduated researcher doing the same, will have more gravitas if s/he states a hypothesis from which a prediction follows than if s/he proclaims a prediction from thin air.
Consider the prediction that the time for two balls to drop from the Tower Pisa will be the same if the two balls have different mass. This is the famous prediction tested (or allegedly tested) by Galileo. This experiment — one of the first in the history of science — was designed to test two contrasting predictions. One was that the time for the two balls to drop would be the same. The other was that the time for the heavier ball to drop would be shorter. (The third possibility, that the lighter ball would drop more quickly, was logically possible but not taken seriously.) The importance of the predictions came from the hypotheses on which they were based. Those hypotheses couldn't have been more different. One stemmed from Aristotle and had an entire system of assumptions about the world's basic elements, including the idea that motion requires a driving force, with the force being greater for a heavier object than a lighter one, in which case the heavier object would land first. The other hypothesis came from an entirely different conception which made no such assumptions, as crystallized (later) by Newton. It led to the prediction of equivalent drop times. Dropping two balls and seeing which, if either, landed first was a more important experiment if it was motivated by different hypotheses than if it was motivated by two different off-the-cuff predictions. Predictions can be ticked off by a monkey at a typewriter, so to speak. Anyone can list possible outcomes. That's not good (interesting) science.
Let me say this, then, to students or colleagues reading this (some of whom might be people to whom I give the URL for this blog): Be cognizant of the distinction between "hypotheses" and "predictions." Hypotheses are claims or educated guesses about the world or the part of it you are studying. Predictions are derived from hypotheses and define opportunities for seeing whether expected consequences of hypotheses are observed. Critically, if a prediction is confirmed — if the data agree with the prediction — you can say that the data are consistent with the prediction and, from that point onward you can also say that the data are consistent with the hypothesis that spawned the prediction. You can't say that the data prove the hypothesis, however. The reason is that any of an infinite number of other hypotheses might have caused the outcome you obtained. If you say that a given data pattern proves that such-and-such hypothesis is correct, you will be shot down, and rightly so, for any given data pattern can be explained by an infinite number of possible hypotheses. It's fine to say that the data you have are consistent with a hypothesis, and it's fine for you to say that a hypothesis is (or appears to be) wrong because the data you got are inconsistent with it. The latter outcome is the culmination of the hypothetico-deductive method, where you can say that a hypothesis is, or seems to be, incorrect if you have data that violates it, but you can never say that a hypothesis is right because you have data consistent with it; some other hypothesis might actually correspond to the true explanation of what you found. By creating hypotheses that lead to different predictions, you can see which prediction is not supported, and insofar as you can make progress by rejecting hypotheses, you can depersonalize your science by developing hypotheses that are worth disproving. The worth of a hypothesis will be judged by how resistant it is to attempts at disconfirmation over many years by many investigators using many methods.
Some final comments.... First, hypotheses don't predict; people do. You can say that a prediction arose from a hypothesis, but you can't say, or shouldn't say, that a hypothesis predicts something.
Second, beware of the admonition that hypotheses are weak if they predict no differences. Newtonian mechanics predicts no difference in the landing times of heavy and light objects dropped from the same height at the same time. The fact that Newtonian mechanics predicts no difference hardly means that Newtonian mechanics is lightweight. Instead, the prediction of no difference in landing times demands creation of extremely sensitive experiments. Anyone can get no difference with sloppy experiments. By contrast, getting no difference when a sophisticated hypothesis predicts none and when one has gone to great lengths to detect even the tiniest possible difference ... now that's good science.
Third and finally, according to the hypothesis that a blog about hypotheses versus predictions will prove informative, the prediction that follows is that those who read and heed this blog will exhibit less confusion about which term to use when. More important, they will exhibit greater gravitas and deeper thoughtfulness as they generate their hypotheses and subsequent predictions. I hope this blog will prove useful. Its utility will be judged by how long it takes to disconfirm the prediction I have just advanced.
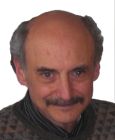
David A. Rosenbaum, Ph.D. , is a cognitive psychologist and a Distinguished Professor of Psychology at the University of California, Riverside.
- Find a Therapist
- Find a Treatment Center
- Find a Psychiatrist
- Find a Support Group
- Find Online Therapy
- United States
- Brooklyn, NY
- Chicago, IL
- Houston, TX
- Los Angeles, CA
- New York, NY
- Portland, OR
- San Diego, CA
- San Francisco, CA
- Seattle, WA
- Washington, DC
- Asperger's
- Bipolar Disorder
- Chronic Pain
- Eating Disorders
- Passive Aggression
- Personality
- Goal Setting
- Positive Psychology
- Stopping Smoking
- Low Sexual Desire
- Relationships
- Child Development
- Therapy Center NEW
- Diagnosis Dictionary
- Types of Therapy
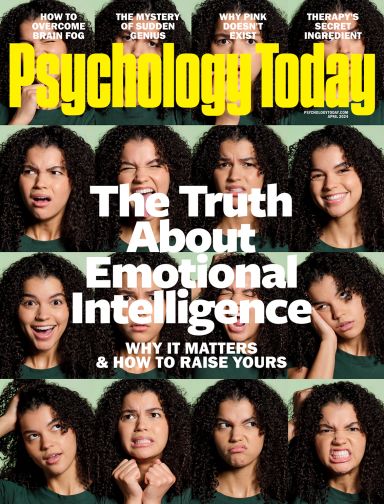
Understanding what emotional intelligence looks like and the steps needed to improve it could light a path to a more emotionally adept world.
- Emotional Intelligence
- Gaslighting
- Affective Forecasting
- Neuroscience
- Maths Notes Class 12
- NCERT Solutions Class 12
- RD Sharma Solutions Class 12
- Maths Formulas Class 12
- Maths Previous Year Paper Class 12
- Class 12 Syllabus
- Class 12 Revision Notes
- Physics Notes Class 12
- Chemistry Notes Class 12
- Biology Notes Class 12
- Null Hypothesis
- Hypothesis Testing Formula
- Difference Between Hypothesis And Theory
- Real-life Applications of Hypothesis Testing
- Permutation Hypothesis Test in R Programming
- Bayes' Theorem
- Hypothesis in Machine Learning
- Current Best Hypothesis Search
- Understanding Hypothesis Testing
- Hypothesis Testing in R Programming
- Jobathon | Stats | Question 10
- Jobathon | Stats | Question 17
- Testing | Question 1
- Difference between Null and Alternate Hypothesis
- ML | Find S Algorithm
- Python - Pearson's Chi-Square Test
Hypothesis is a testable statement that explains what is happening or observed. It proposes the relation between the various participating variables. Hypothesis is also called Theory, Thesis, Guess, Assumption, or Suggestion. Hypothesis creates a structure that guides the search for knowledge.
In this article, we will learn what is hypothesis, its characteristics, types, and examples. We will also learn how hypothesis helps in scientific research.
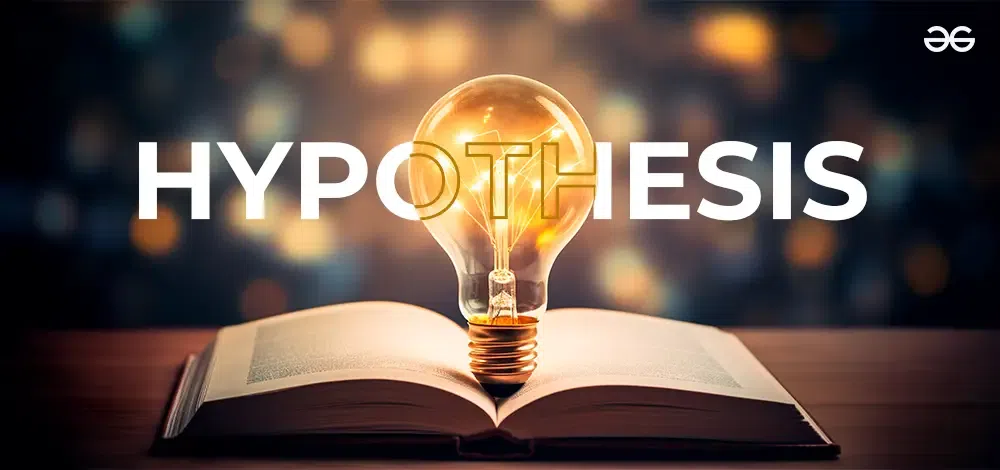
What is Hypothesis?
A hypothesis is a suggested idea or plan that has little proof, meant to lead to more study. It’s mainly a smart guess or suggested answer to a problem that can be checked through study and trial. In science work, we make guesses called hypotheses to try and figure out what will happen in tests or watching. These are not sure things but rather ideas that can be proved or disproved based on real-life proofs. A good theory is clear and can be tested and found wrong if the proof doesn’t support it.
Hypothesis Meaning
A hypothesis is a proposed statement that is testable and is given for something that happens or observed.
- It is made using what we already know and have seen, and it’s the basis for scientific research.
- A clear guess tells us what we think will happen in an experiment or study.
- It’s a testable clue that can be proven true or wrong with real-life facts and checking it out carefully.
- It usually looks like a “if-then” rule, showing the expected cause and effect relationship between what’s being studied.
Characteristics of Hypothesis
Here are some key characteristics of a hypothesis:
- Testable: An idea (hypothesis) should be made so it can be tested and proven true through doing experiments or watching. It should show a clear connection between things.
- Specific: It needs to be easy and on target, talking about a certain part or connection between things in a study.
- Falsifiable: A good guess should be able to show it’s wrong. This means there must be a chance for proof or seeing something that goes against the guess.
- Logical and Rational: It should be based on things we know now or have seen, giving a reasonable reason that fits with what we already know.
- Predictive: A guess often tells what to expect from an experiment or observation. It gives a guide for what someone might see if the guess is right.
- Concise: It should be short and clear, showing the suggested link or explanation simply without extra confusion.
- Grounded in Research: A guess is usually made from before studies, ideas or watching things. It comes from a deep understanding of what is already known in that area.
- Flexible: A guess helps in the research but it needs to change or fix when new information comes up.
- Relevant: It should be related to the question or problem being studied, helping to direct what the research is about.
- Empirical: Hypotheses come from observations and can be tested using methods based on real-world experiences.
Sources of Hypothesis
Hypotheses can come from different places based on what you’re studying and the kind of research. Here are some common sources from which hypotheses may originate:
- Existing Theories: Often, guesses come from well-known science ideas. These ideas may show connections between things or occurrences that scientists can look into more.
- Observation and Experience: Watching something happen or having personal experiences can lead to guesses. We notice odd things or repeat events in everyday life and experiments. This can make us think of guesses called hypotheses.
- Previous Research: Using old studies or discoveries can help come up with new ideas. Scientists might try to expand or question current findings, making guesses that further study old results.
- Literature Review: Looking at books and research in a subject can help make guesses. Noticing missing parts or mismatches in previous studies might make researchers think up guesses to deal with these spots.
- Problem Statement or Research Question: Often, ideas come from questions or problems in the study. Making clear what needs to be looked into can help create ideas that tackle certain parts of the issue.
- Analogies or Comparisons: Making comparisons between similar things or finding connections from related areas can lead to theories. Understanding from other fields could create new guesses in a different situation.
- Hunches and Speculation: Sometimes, scientists might get a gut feeling or make guesses that help create ideas to test. Though these may not have proof at first, they can be a beginning for looking deeper.
- Technology and Innovations: New technology or tools might make guesses by letting us look at things that were hard to study before.
- Personal Interest and Curiosity: People’s curiosity and personal interests in a topic can help create guesses. Scientists could make guesses based on their own likes or love for a subject.
Types of Hypothesis
Here are some common types of hypotheses:
Simple Hypothesis
Complex hypothesis, directional hypothesis.
- Non-directional Hypothesis
Null Hypothesis (H0)
Alternative hypothesis (h1 or ha), statistical hypothesis, research hypothesis, associative hypothesis, causal hypothesis.
Simple Hypothesis guesses a connection between two things. It says that there is a connection or difference between variables, but it doesn’t tell us which way the relationship goes.
Complex Hypothesis tells us what will happen when more than two things are connected. It looks at how different things interact and may be linked together.
Directional Hypothesis says how one thing is related to another. For example, it guesses that one thing will help or hurt another thing.
Non-Directional Hypothesis
Non-Directional Hypothesis are the one that don’t say how the relationship between things will be. They just say that there is a connection, without telling which way it goes.
Null hypothesis is a statement that says there’s no connection or difference between different things. It implies that any seen impacts are because of luck or random changes in the information.
Alternative Hypothesis is different from the null hypothesis and shows that there’s a big connection or gap between variables. Scientists want to say no to the null hypothesis and choose the alternative one.
Statistical Hypotheis are used in math testing and include making ideas about what groups or bits of them look like. You aim to get information or test certain things using these top-level, common words only.
Research Hypothesis comes from the research question and tells what link is expected between things or factors. It leads the study and chooses where to look more closely.
Associative Hypotheis guesses that there is a link or connection between things without really saying it caused them. It means that when one thing changes, it is connected to another thing changing.
Causal Hypothesis are different from other ideas because they say that one thing causes another. This means there’s a cause and effect relationship between variables involved in the situation. They say that when one thing changes, it directly makes another thing change.
Hypothesis Examples
Following are the examples of hypotheses based on their types:
Simple Hypothesis Example
- Studying more can help you do better on tests.
- Getting more sun makes people have higher amounts of vitamin D.
Complex Hypothesis Example
- How rich you are, how easy it is to get education and healthcare greatly affects the number of years people live.
- A new medicine’s success relies on the amount used, how old a person is who takes it and their genes.
Directional Hypothesis Example
- Drinking more sweet drinks is linked to a higher body weight score.
- Too much stress makes people less productive at work.
Non-directional Hypothesis Example
- Drinking caffeine can affect how well you sleep.
- People often like different kinds of music based on their gender.
- The average test scores of Group A and Group B are not much different.
- There is no connection between using a certain fertilizer and how much it helps crops grow.
Alternative Hypothesis (Ha)
- Patients on Diet A have much different cholesterol levels than those following Diet B.
- Exposure to a certain type of light can change how plants grow compared to normal sunlight.
- The average smarts score of kids in a certain school area is 100.
- The usual time it takes to finish a job using Method A is the same as with Method B.
- Having more kids go to early learning classes helps them do better in school when they get older.
- Using specific ways of talking affects how much customers get involved in marketing activities.
- Regular exercise helps to lower the chances of heart disease.
- Going to school more can help people make more money.
- Playing violent video games makes teens more likely to act aggressively.
- Less clean air directly impacts breathing health in city populations.
Functions of Hypothesis
Hypotheses have many important jobs in the process of scientific research. Here are the key functions of hypotheses:
- Guiding Research: Hypotheses give a clear and exact way for research. They act like guides, showing the predicted connections or results that scientists want to study.
- Formulating Research Questions: Research questions often create guesses. They assist in changing big questions into particular, checkable things. They guide what the study should be focused on.
- Setting Clear Objectives: Hypotheses set the goals of a study by saying what connections between variables should be found. They set the targets that scientists try to reach with their studies.
- Testing Predictions: Theories guess what will happen in experiments or observations. By doing tests in a planned way, scientists can check if what they see matches the guesses made by their ideas.
- Providing Structure: Theories give structure to the study process by arranging thoughts and ideas. They aid scientists in thinking about connections between things and plan experiments to match.
- Focusing Investigations: Hypotheses help scientists focus on certain parts of their study question by clearly saying what they expect links or results to be. This focus makes the study work better.
- Facilitating Communication: Theories help scientists talk to each other effectively. Clearly made guesses help scientists to tell others what they plan, how they will do it and the results expected. This explains things well with colleagues in a wide range of audiences.
- Generating Testable Statements: A good guess can be checked, which means it can be looked at carefully or tested by doing experiments. This feature makes sure that guesses add to the real information used in science knowledge.
- Promoting Objectivity: Guesses give a clear reason for study that helps guide the process while reducing personal bias. They motivate scientists to use facts and data as proofs or disprovals for their proposed answers.
- Driving Scientific Progress: Making, trying out and adjusting ideas is a cycle. Even if a guess is proven right or wrong, the information learned helps to grow knowledge in one specific area.
How Hypothesis help in Scientific Research?
Researchers use hypotheses to put down their thoughts directing how the experiment would take place. Following are the steps that are involved in the scientific method:
- Initiating Investigations: Hypotheses are the beginning of science research. They come from watching, knowing what’s already known or asking questions. This makes scientists make certain explanations that need to be checked with tests.
- Formulating Research Questions: Ideas usually come from bigger questions in study. They help scientists make these questions more exact and testable, guiding the study’s main point.
- Setting Clear Objectives: Hypotheses set the goals of a study by stating what we think will happen between different things. They set the goals that scientists want to reach by doing their studies.
- Designing Experiments and Studies: Assumptions help plan experiments and watchful studies. They assist scientists in knowing what factors to measure, the techniques they will use and gather data for a proposed reason.
- Testing Predictions: Ideas guess what will happen in experiments or observations. By checking these guesses carefully, scientists can see if the seen results match up with what was predicted in each hypothesis.
- Analysis and Interpretation of Data: Hypotheses give us a way to study and make sense of information. Researchers look at what they found and see if it matches the guesses made in their theories. They decide if the proof backs up or disagrees with these suggested reasons why things are happening as expected.
- Encouraging Objectivity: Hypotheses help make things fair by making sure scientists use facts and information to either agree or disagree with their suggested reasons. They lessen personal preferences by needing proof from experience.
- Iterative Process: People either agree or disagree with guesses, but they still help the ongoing process of science. Findings from testing ideas make us ask new questions, improve those ideas and do more tests. It keeps going on in the work of science to keep learning things.
People Also View:
Mathematics Maths Formulas Branches of Mathematics
Summary – Hypothesis
A hypothesis is a testable statement serving as an initial explanation for phenomena, based on observations, theories, or existing knowledge. It acts as a guiding light for scientific research, proposing potential relationships between variables that can be empirically tested through experiments and observations. The hypothesis must be specific, testable, falsifiable, and grounded in prior research or observation, laying out a predictive, if-then scenario that details a cause-and-effect relationship. It originates from various sources including existing theories, observations, previous research, and even personal curiosity, leading to different types, such as simple, complex, directional, non-directional, null, and alternative hypotheses, each serving distinct roles in research methodology. The hypothesis not only guides the research process by shaping objectives and designing experiments but also facilitates objective analysis and interpretation of data, ultimately driving scientific progress through a cycle of testing, validation, and refinement.
FAQs on Hypothesis
What is a hypothesis.
A guess is a possible explanation or forecast that can be checked by doing research and experiments.
What are Components of a Hypothesis?
The components of a Hypothesis are Independent Variable, Dependent Variable, Relationship between Variables, Directionality etc.
What makes a Good Hypothesis?
Testability, Falsifiability, Clarity and Precision, Relevance are some parameters that makes a Good Hypothesis
Can a Hypothesis be Proven True?
You cannot prove conclusively that most hypotheses are true because it’s generally impossible to examine all possible cases for exceptions that would disprove them.
How are Hypotheses Tested?
Hypothesis testing is used to assess the plausibility of a hypothesis by using sample data
Can Hypotheses change during Research?
Yes, you can change or improve your ideas based on new information discovered during the research process.
What is the Role of a Hypothesis in Scientific Research?
Hypotheses are used to support scientific research and bring about advancements in knowledge.
Please Login to comment...
Similar reads.
- Geeks Premier League 2023
- Maths-Class-12
- Geeks Premier League
- Mathematics
- School Learning
Improve your Coding Skills with Practice
What kind of Experience do you want to share?
Projective Hypothesis
- Living reference work entry
- First Online: 13 April 2017
- Cite this living reference work entry
- Jayanti Basu 3
792 Accesses
1 Citations
7 Altmetric
Synonyms/Related Terms
Projection ; Projective tests ; Underlying principles
Projective hypothesis refers to the notion that when confronted with ambiguous and unstructured stimuli, the responses elicited by a person reflect one’s unconscious needs, feelings, anxieties, motives, thoughts, conflicts, and prior behavioral conditioning.
Introduction
The concept of projective hypothesis lies behind the development of the stimuli of projective tests and also touches upon the validity-related concern for such tests. It is assumed that unstructured and ambiguous stimuli have the power to bypass both conscious suppression and unconscious defenses that might otherwise result in faked, distorted, or falsified responses. Therefore, assessment techniques using such stimuli may have the ability to unearth one’s core traits, irrespective of culture and training. Evidently, the idea is linked to the psychodynamic understanding of human behavior and specifically to the mechanism of projection.
This is a preview of subscription content, log in via an institution to check access.
Access this chapter
Institutional subscriptions
Basu, J. (2014). Psychologists’ ambivalence toward ambiguity: Relocating the projective test debate for multiple interpretative hypotheses. SIS Journal of Projective Psychology and Mental Health, 21 (1), 25–36.
Google Scholar
Frank, L. K. (1939). Projective methods for the study of personality. Journal of Psychology, 8 , 389–413.
Article Google Scholar
Frick, P. J., Barry, C. T., & Kamphaus, R. W. (2010). Clinical assessment of child and adolescent personality and behavior . New York: Springer.
Book Google Scholar
Gregory, R. J. (2000). Psychological testing: History, principles, and applications . Boston: Allyn & Bacon.
Hughes, T. L., Gacono, C. G., & Owen, P. F. (2007). Current status of Rorschach assessment: Implications for the school psychologist. Psychology in the Schools, 44 (3), 281–291.
Knoff, H. M. (1983). Projective/personality assessment in the schools (Special issue). School Psychology Review, 12 (4), 446–451.
Koppitz, E. M. (1982). Personality assessment in the schools. In C. R. Reynolds & T. B. Gutkin (Eds.), The handbook of school psychology (pp. 273–295). New York: Wiley.
LIlienfeld, S. O., Wood, J. M., & Garb, H. N. (2000). The scientific status of projective techniques. Psychological Science in the Public Interest, 1 , 27–66.
Article PubMed Google Scholar
Piotrowski, C. (2015). Projective techniques usage worldwide: A review of applied settings 1995–2015. Journal of the Indian Academy of Applied Psychology, 41 (3), 9–19. (Special issue).
Winter, D. G., John, O. P., Stewart, A. J., Klohnen, E. C., & Duncan, L. E. (1998). Traits and motives: Toward an integration of two traditions in personality research. Psychological Review, 105 , 230–251.
Download references
Author information
Authors and affiliations.
Department of Applied Psychology, University of Calcutta, 92 APC Road, Kolkata, 700009, India
Jayanti Basu
You can also search for this author in PubMed Google Scholar
Corresponding author
Correspondence to Jayanti Basu .
Editor information
Editors and affiliations.
Oakland University, Rochester, USA
Virgil Zeigler-Hill
Todd K. Shackelford
Section Editor information
Wichita State University, Wichita, KS, USA
Brendan Clark
Rights and permissions
Reprints and permissions
Copyright information
© 2017 Springer International Publishing AG
About this entry
Cite this entry.
Basu, J. (2017). Projective Hypothesis. In: Zeigler-Hill, V., Shackelford, T. (eds) Encyclopedia of Personality and Individual Differences. Springer, Cham. https://doi.org/10.1007/978-3-319-28099-8_64-1
Download citation
DOI : https://doi.org/10.1007/978-3-319-28099-8_64-1
Received : 13 March 2017
Accepted : 10 April 2017
Published : 13 April 2017
Publisher Name : Springer, Cham
Print ISBN : 978-3-319-28099-8
Online ISBN : 978-3-319-28099-8
eBook Packages : Springer Reference Behavioral Science and Psychology Reference Module Humanities and Social Sciences Reference Module Business, Economics and Social Sciences
- Publish with us
Policies and ethics
- Find a journal
- Track your research
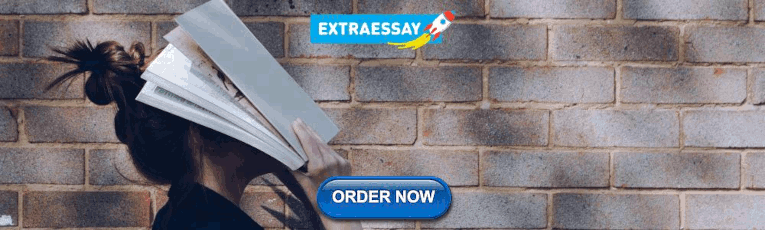
IMAGES
VIDEO
COMMENTS
Hypothesis. Definition: Hypothesis is an educated guess or proposed explanation for a phenomenon, based on some initial observations or data. It is a tentative statement that can be tested and potentially proven or disproven through further investigation and experimentation. ... Predictive power: A hypothesis makes predictions about the outcome ...
4. Refine your hypothesis. You need to make sure your hypothesis is specific and testable. There are various ways of phrasing a hypothesis, but all the terms you use should have clear definitions, and the hypothesis should contain: The relevant variables; The specific group being studied; The predicted outcome of the experiment or analysis; 5.
It seeks to explore and understand a particular aspect of the research subject. In contrast, a research hypothesis is a specific statement or prediction that suggests an expected relationship between variables. It is formulated based on existing knowledge or theories and guides the research design and data analysis. 7.
Scientific hypotheses writing promoting and predicting is a skill that can help researchers to formulate and test their ideas about schizophrenia and its symptoms. This article provides a guide on how to write clear, testable, and falsifiable hypotheses, and how to use them to generate predictions and implications for future research.
The Royal Society - On the scope of scientific hypotheses (Apr. 24, 2024) scientific hypothesis, an idea that proposes a tentative explanation about a phenomenon or a narrow set of phenomena observed in the natural world. The two primary features of a scientific hypothesis are falsifiability and testability, which are reflected in an "If ...
Step 5: Phrase your hypothesis in three ways. To identify the variables, you can write a simple prediction in if … then form. The first part of the sentence states the independent variable and the second part states the dependent variable. If a first-year student starts attending more lectures, then their exam scores will improve.
Examples. A research hypothesis, in its plural form "hypotheses," is a specific, testable prediction about the anticipated results of a study, established at its outset. It is a key component of the scientific method. Hypotheses connect theory to data and guide the research process towards expanding scientific understanding.
A hypothesis is a prediction of what will be found at the outcome of a research project and is typically focused on the relationship between two different variables studied in the research. It is usually based on both theoretical expectations about how things work and already existing scientific evidence. Within social science, a hypothesis can ...
On the other hand, a prediction is the outcome you would observe if your hypothesis were correct. Predictions are often written in the form of "if, and, then" statements, as in, "if my hypothesis is true, and I were to do this test, then this is what I will observe.". Following our sparrow example, you could predict that, "If sparrows ...
A hypothesis is an idea about how something works that can be tested using experiments. A prediction says what will happen in an experiment if the hypothesis is correct. Presenter 1: We are going ...
Table of contents. Step 1: State your null and alternate hypothesis. Step 2: Collect data. Step 3: Perform a statistical test. Step 4: Decide whether to reject or fail to reject your null hypothesis. Step 5: Present your findings. Other interesting articles. Frequently asked questions about hypothesis testing.
Simple hypothesis. A simple hypothesis is a statement made to reflect the relation between exactly two variables. One independent and one dependent. Consider the example, "Smoking is a prominent cause of lung cancer." The dependent variable, lung cancer, is dependent on the independent variable, smoking. 4.
A research hypothesis (also called a scientific hypothesis) is a statement about the expected outcome of a study (for example, a dissertation or thesis). To constitute a quality hypothesis, the statement needs to have three attributes - specificity, clarity and testability. Let's take a look at these more closely.
DEVELOPING HYPOTHESES & RESEARCH QUESTIONS. Nature of Hypothesis. It can be tested - verifiable or falsifiable Hypotheses are not moral or ethical questions It is neither too specific nor to general It is a prediction of consequences It is considered valuable even if proven false. DEVELOPING HYPOTHESES & RESEARCH QUESTIONS.
Formulating Strong Hypotheses. Before you write your research hypothesis, make sure to do some reading in your area of interest; good resources will include scholarly papers, articles, books, and other academic research. Because your research hypothesis will be a specific, testable prediction about what you expect to happen in a study, you will ...
Abstract. The last two decades of neuroscience research has produced a growing number of studies that suggest the various psychological phenomena are produced by predictive processes in the brain. When considered together, these studies form a coherent, neurobiologically-inspired research program for guiding psychological research about the ...
hypothetico-deductive, or hypohetico-predictive approach) that comprises the following steps: 1. Asking a causal question about a puzzling observation. 2. Advancing a causal hypothesis (defined as a proposed explanation) for what has been observed (e.g., "the grass grows better on this side of the building because it is exposed to
Formulating Hypotheses for Different Study Designs. Generating a testable working hypothesis is the first step towards conducting original research. Such research may prove or disprove the proposed hypothesis. Case reports, case series, online surveys and other observational studies, clinical trials, and narrative reviews help to generate ...
The difference between hypothesis and prediction is explained through explanations & examples. Use our simple table for hypothesis vs prediction reference. ... Therefore, by definition, a prediction is making a statement of what will happen in the future. In science, a prediction is what you expect to happen if your hypothesis is true. So ...
Prediction may be a central concept for understanding perceptual and cognitive processing. Contemporary theoretical neuroscience formalizes the role of prediction in terms of probabilistic inference. Perception, action, attention, and learning may then be unified as aspects of predictive processing in the brain.
Hypothesis. A hypothesis is an educated guess, based on observation. It's a prediction of cause and effect. Usually, a hypothesis can be supported or refuted through experimentation or more observation. A hypothesis can be disproven but not proven to be true. Example: If you see no difference in the cleaning ability of various laundry ...
First, hypotheses don't predict; people do. You can say that a prediction arose from a hypothesis, but you can't say, or shouldn't say, that a hypothesis predicts something. Second, beware of the ...
Hypothesis is a testable statement that explains what is happening or observed. It proposes the relation between the various participating variables. Hypothesis is also called Theory, Thesis, Guess, Assumption, or Suggestion. Hypothesis creates a structure that guides the search for knowledge. In this article, we will learn what is hypothesis ...
The concept of projective hypothesis lies behind the development of the stimuli of projective tests and also touches upon the validity-related concern for such tests. It is assumed that unstructured and ambiguous stimuli have the power to bypass both conscious suppression and unconscious defenses that might otherwise result in faked, distorted ...