- Privacy Policy

Home » Descriptive Research Design – Types, Methods and Examples
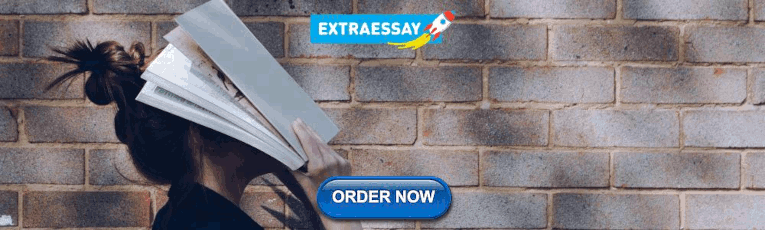
Descriptive Research Design – Types, Methods and Examples
Table of Contents

Descriptive Research Design
Definition:
Descriptive research design is a type of research methodology that aims to describe or document the characteristics, behaviors, attitudes, opinions, or perceptions of a group or population being studied.
Descriptive research design does not attempt to establish cause-and-effect relationships between variables or make predictions about future outcomes. Instead, it focuses on providing a detailed and accurate representation of the data collected, which can be useful for generating hypotheses, exploring trends, and identifying patterns in the data.
Types of Descriptive Research Design
Types of Descriptive Research Design are as follows:
Cross-sectional Study
This involves collecting data at a single point in time from a sample or population to describe their characteristics or behaviors. For example, a researcher may conduct a cross-sectional study to investigate the prevalence of certain health conditions among a population, or to describe the attitudes and beliefs of a particular group.
Longitudinal Study
This involves collecting data over an extended period of time, often through repeated observations or surveys of the same group or population. Longitudinal studies can be used to track changes in attitudes, behaviors, or outcomes over time, or to investigate the effects of interventions or treatments.
This involves an in-depth examination of a single individual, group, or situation to gain a detailed understanding of its characteristics or dynamics. Case studies are often used in psychology, sociology, and business to explore complex phenomena or to generate hypotheses for further research.
Survey Research
This involves collecting data from a sample or population through standardized questionnaires or interviews. Surveys can be used to describe attitudes, opinions, behaviors, or demographic characteristics of a group, and can be conducted in person, by phone, or online.
Observational Research
This involves observing and documenting the behavior or interactions of individuals or groups in a natural or controlled setting. Observational studies can be used to describe social, cultural, or environmental phenomena, or to investigate the effects of interventions or treatments.
Correlational Research
This involves examining the relationships between two or more variables to describe their patterns or associations. Correlational studies can be used to identify potential causal relationships or to explore the strength and direction of relationships between variables.
Data Analysis Methods
Descriptive research design data analysis methods depend on the type of data collected and the research question being addressed. Here are some common methods of data analysis for descriptive research:
Descriptive Statistics
This method involves analyzing data to summarize and describe the key features of a sample or population. Descriptive statistics can include measures of central tendency (e.g., mean, median, mode) and measures of variability (e.g., range, standard deviation).
Cross-tabulation
This method involves analyzing data by creating a table that shows the frequency of two or more variables together. Cross-tabulation can help identify patterns or relationships between variables.
Content Analysis
This method involves analyzing qualitative data (e.g., text, images, audio) to identify themes, patterns, or trends. Content analysis can be used to describe the characteristics of a sample or population, or to identify factors that influence attitudes or behaviors.
Qualitative Coding
This method involves analyzing qualitative data by assigning codes to segments of data based on their meaning or content. Qualitative coding can be used to identify common themes, patterns, or categories within the data.
Visualization
This method involves creating graphs or charts to represent data visually. Visualization can help identify patterns or relationships between variables and make it easier to communicate findings to others.
Comparative Analysis
This method involves comparing data across different groups or time periods to identify similarities and differences. Comparative analysis can help describe changes in attitudes or behaviors over time or differences between subgroups within a population.
Applications of Descriptive Research Design
Descriptive research design has numerous applications in various fields. Some of the common applications of descriptive research design are:
- Market research: Descriptive research design is widely used in market research to understand consumer preferences, behavior, and attitudes. This helps companies to develop new products and services, improve marketing strategies, and increase customer satisfaction.
- Health research: Descriptive research design is used in health research to describe the prevalence and distribution of a disease or health condition in a population. This helps healthcare providers to develop prevention and treatment strategies.
- Educational research: Descriptive research design is used in educational research to describe the performance of students, schools, or educational programs. This helps educators to improve teaching methods and develop effective educational programs.
- Social science research: Descriptive research design is used in social science research to describe social phenomena such as cultural norms, values, and beliefs. This helps researchers to understand social behavior and develop effective policies.
- Public opinion research: Descriptive research design is used in public opinion research to understand the opinions and attitudes of the general public on various issues. This helps policymakers to develop effective policies that are aligned with public opinion.
- Environmental research: Descriptive research design is used in environmental research to describe the environmental conditions of a particular region or ecosystem. This helps policymakers and environmentalists to develop effective conservation and preservation strategies.
Descriptive Research Design Examples
Here are some real-time examples of descriptive research designs:
- A restaurant chain wants to understand the demographics and attitudes of its customers. They conduct a survey asking customers about their age, gender, income, frequency of visits, favorite menu items, and overall satisfaction. The survey data is analyzed using descriptive statistics and cross-tabulation to describe the characteristics of their customer base.
- A medical researcher wants to describe the prevalence and risk factors of a particular disease in a population. They conduct a cross-sectional study in which they collect data from a sample of individuals using a standardized questionnaire. The data is analyzed using descriptive statistics and cross-tabulation to identify patterns in the prevalence and risk factors of the disease.
- An education researcher wants to describe the learning outcomes of students in a particular school district. They collect test scores from a representative sample of students in the district and use descriptive statistics to calculate the mean, median, and standard deviation of the scores. They also create visualizations such as histograms and box plots to show the distribution of scores.
- A marketing team wants to understand the attitudes and behaviors of consumers towards a new product. They conduct a series of focus groups and use qualitative coding to identify common themes and patterns in the data. They also create visualizations such as word clouds to show the most frequently mentioned topics.
- An environmental scientist wants to describe the biodiversity of a particular ecosystem. They conduct an observational study in which they collect data on the species and abundance of plants and animals in the ecosystem. The data is analyzed using descriptive statistics to describe the diversity and richness of the ecosystem.
How to Conduct Descriptive Research Design
To conduct a descriptive research design, you can follow these general steps:
- Define your research question: Clearly define the research question or problem that you want to address. Your research question should be specific and focused to guide your data collection and analysis.
- Choose your research method: Select the most appropriate research method for your research question. As discussed earlier, common research methods for descriptive research include surveys, case studies, observational studies, cross-sectional studies, and longitudinal studies.
- Design your study: Plan the details of your study, including the sampling strategy, data collection methods, and data analysis plan. Determine the sample size and sampling method, decide on the data collection tools (such as questionnaires, interviews, or observations), and outline your data analysis plan.
- Collect data: Collect data from your sample or population using the data collection tools you have chosen. Ensure that you follow ethical guidelines for research and obtain informed consent from participants.
- Analyze data: Use appropriate statistical or qualitative analysis methods to analyze your data. As discussed earlier, common data analysis methods for descriptive research include descriptive statistics, cross-tabulation, content analysis, qualitative coding, visualization, and comparative analysis.
- I nterpret results: Interpret your findings in light of your research question and objectives. Identify patterns, trends, and relationships in the data, and describe the characteristics of your sample or population.
- Draw conclusions and report results: Draw conclusions based on your analysis and interpretation of the data. Report your results in a clear and concise manner, using appropriate tables, graphs, or figures to present your findings. Ensure that your report follows accepted research standards and guidelines.
When to Use Descriptive Research Design
Descriptive research design is used in situations where the researcher wants to describe a population or phenomenon in detail. It is used to gather information about the current status or condition of a group or phenomenon without making any causal inferences. Descriptive research design is useful in the following situations:
- Exploratory research: Descriptive research design is often used in exploratory research to gain an initial understanding of a phenomenon or population.
- Identifying trends: Descriptive research design can be used to identify trends or patterns in a population, such as changes in consumer behavior or attitudes over time.
- Market research: Descriptive research design is commonly used in market research to understand consumer preferences, behavior, and attitudes.
- Health research: Descriptive research design is useful in health research to describe the prevalence and distribution of a disease or health condition in a population.
- Social science research: Descriptive research design is used in social science research to describe social phenomena such as cultural norms, values, and beliefs.
- Educational research: Descriptive research design is used in educational research to describe the performance of students, schools, or educational programs.
Purpose of Descriptive Research Design
The main purpose of descriptive research design is to describe and measure the characteristics of a population or phenomenon in a systematic and objective manner. It involves collecting data that describe the current status or condition of the population or phenomenon of interest, without manipulating or altering any variables.
The purpose of descriptive research design can be summarized as follows:
- To provide an accurate description of a population or phenomenon: Descriptive research design aims to provide a comprehensive and accurate description of a population or phenomenon of interest. This can help researchers to develop a better understanding of the characteristics of the population or phenomenon.
- To identify trends and patterns: Descriptive research design can help researchers to identify trends and patterns in the data, such as changes in behavior or attitudes over time. This can be useful for making predictions and developing strategies.
- To generate hypotheses: Descriptive research design can be used to generate hypotheses or research questions that can be tested in future studies. For example, if a descriptive study finds a correlation between two variables, this could lead to the development of a hypothesis about the causal relationship between the variables.
- To establish a baseline: Descriptive research design can establish a baseline or starting point for future research. This can be useful for comparing data from different time periods or populations.
Characteristics of Descriptive Research Design
Descriptive research design has several key characteristics that distinguish it from other research designs. Some of the main characteristics of descriptive research design are:
- Objective : Descriptive research design is objective in nature, which means that it focuses on collecting factual and accurate data without any personal bias. The researcher aims to report the data objectively without any personal interpretation.
- Non-experimental: Descriptive research design is non-experimental, which means that the researcher does not manipulate any variables. The researcher simply observes and records the behavior or characteristics of the population or phenomenon of interest.
- Quantitative : Descriptive research design is quantitative in nature, which means that it involves collecting numerical data that can be analyzed using statistical techniques. This helps to provide a more precise and accurate description of the population or phenomenon.
- Cross-sectional: Descriptive research design is often cross-sectional, which means that the data is collected at a single point in time. This can be useful for understanding the current state of the population or phenomenon, but it may not provide information about changes over time.
- Large sample size: Descriptive research design typically involves a large sample size, which helps to ensure that the data is representative of the population of interest. A large sample size also helps to increase the reliability and validity of the data.
- Systematic and structured: Descriptive research design involves a systematic and structured approach to data collection, which helps to ensure that the data is accurate and reliable. This involves using standardized procedures for data collection, such as surveys, questionnaires, or observation checklists.
Advantages of Descriptive Research Design
Descriptive research design has several advantages that make it a popular choice for researchers. Some of the main advantages of descriptive research design are:
- Provides an accurate description: Descriptive research design is focused on accurately describing the characteristics of a population or phenomenon. This can help researchers to develop a better understanding of the subject of interest.
- Easy to conduct: Descriptive research design is relatively easy to conduct and requires minimal resources compared to other research designs. It can be conducted quickly and efficiently, and data can be collected through surveys, questionnaires, or observations.
- Useful for generating hypotheses: Descriptive research design can be used to generate hypotheses or research questions that can be tested in future studies. For example, if a descriptive study finds a correlation between two variables, this could lead to the development of a hypothesis about the causal relationship between the variables.
- Large sample size : Descriptive research design typically involves a large sample size, which helps to ensure that the data is representative of the population of interest. A large sample size also helps to increase the reliability and validity of the data.
- Can be used to monitor changes : Descriptive research design can be used to monitor changes over time in a population or phenomenon. This can be useful for identifying trends and patterns, and for making predictions about future behavior or attitudes.
- Can be used in a variety of fields : Descriptive research design can be used in a variety of fields, including social sciences, healthcare, business, and education.
Limitation of Descriptive Research Design
Descriptive research design also has some limitations that researchers should consider before using this design. Some of the main limitations of descriptive research design are:
- Cannot establish cause and effect: Descriptive research design cannot establish cause and effect relationships between variables. It only provides a description of the characteristics of the population or phenomenon of interest.
- Limited generalizability: The results of a descriptive study may not be generalizable to other populations or situations. This is because descriptive research design often involves a specific sample or situation, which may not be representative of the broader population.
- Potential for bias: Descriptive research design can be subject to bias, particularly if the researcher is not objective in their data collection or interpretation. This can lead to inaccurate or incomplete descriptions of the population or phenomenon of interest.
- Limited depth: Descriptive research design may provide a superficial description of the population or phenomenon of interest. It does not delve into the underlying causes or mechanisms behind the observed behavior or characteristics.
- Limited utility for theory development: Descriptive research design may not be useful for developing theories about the relationship between variables. It only provides a description of the variables themselves.
- Relies on self-report data: Descriptive research design often relies on self-report data, such as surveys or questionnaires. This type of data may be subject to biases, such as social desirability bias or recall bias.
About the author
Muhammad Hassan
Researcher, Academic Writer, Web developer
You may also like

Questionnaire – Definition, Types, and Examples

Case Study – Methods, Examples and Guide

Observational Research – Methods and Guide

Quantitative Research – Methods, Types and...

Qualitative Research Methods

Explanatory Research – Types, Methods, Guide
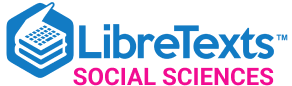
- school Campus Bookshelves
- menu_book Bookshelves
- perm_media Learning Objects
- login Login
- how_to_reg Request Instructor Account
- hub Instructor Commons
- Download Page (PDF)
- Download Full Book (PDF)
- Periodic Table
- Physics Constants
- Scientific Calculator
- Reference & Cite
- Tools expand_more
- Readability
selected template will load here
This action is not available.
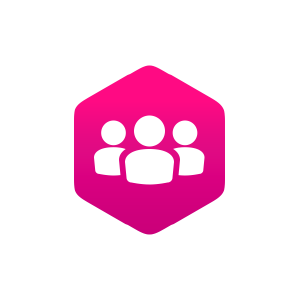
1.11: Developmental Research Designs
- Last updated
- Save as PDF
- Page ID 60388
- Lumen Learning
Learning Outcomes
- Compare advantages and disadvantages of developmental research designs (cross-sectional, longitudinal, and sequential)
Now you know about some tools used to conduct research about human development. Remember, research methods are tools that are used to collect information. But it is easy to confuse research methods and research design. Research design is the strategy or blueprint for deciding how to collect and analyze information. Research design dictates which methods are used and how. Developmental research designs are techniques used particularly in lifespan development research. When we are trying to describe development and change, the research designs become especially important because we are interested in what changes and what stays the same with age. These techniques try to examine how age, cohort, gender, and social class impact development.
Cross-sectional designs
The majority of developmental studies use cross-sectional designs because they are less time-consuming and less expensive than other developmental designs. Cross-sectional research designs are used to examine behavior in participants of different ages who are tested at the same point in time. Let’s suppose that researchers are interested in the relationship between intelligence and aging. They might have a hypothesis (an educated guess, based on theory or observations) that intelligence declines as people get older. The researchers might choose to give a certain intelligence test to individuals who are 20 years old, individuals who are 50 years old, and individuals who are 80 years old at the same time and compare the data from each age group. This research is cross-sectional in design because the researchers plan to examine the intelligence scores of individuals of different ages within the same study at the same time; they are taking a “cross-section” of people at one point in time. Let’s say that the comparisons find that the 80-year-old adults score lower on the intelligence test than the 50-year-old adults, and the 50-year-old adults score lower on the intelligence test than the 20-year-old adults. Based on these data, the researchers might conclude that individuals become less intelligent as they get older. Would that be a valid (accurate) interpretation of the results?
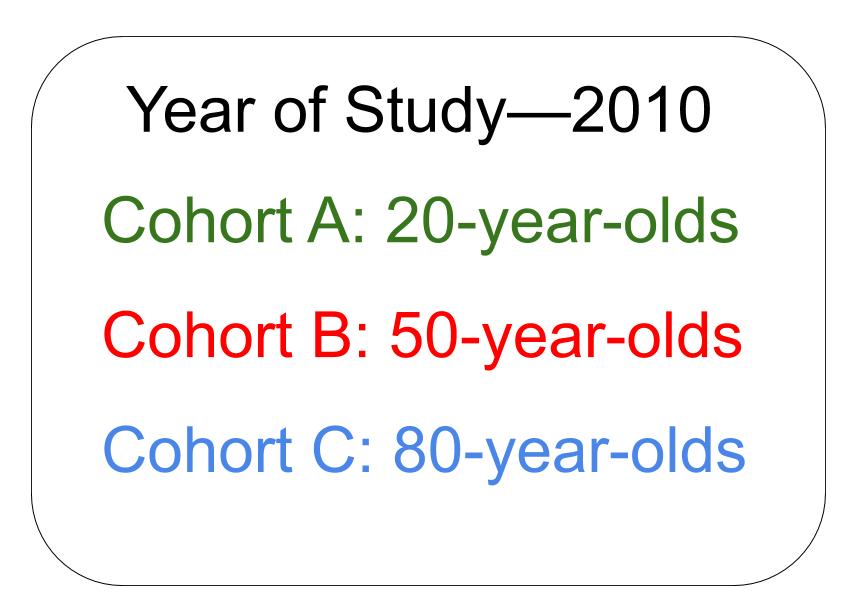
No, that would not be a valid conclusion because the researchers did not follow individuals as they aged from 20 to 50 to 80 years old. One of the primary limitations of cross-sectional research is that the results yield information about age differences not necessarily changes with age or over time. That is, although the study described above can show that in 2010, the 80-year-olds scored lower on the intelligence test than the 50-year-olds, and the 50-year-olds scored lower on the intelligence test than the 20-year-olds, the data used to come up with this conclusion were collected from different individuals (or groups of individuals). It could be, for instance, that when these 20-year-olds get older (50 and eventually 80), they will still score just as high on the intelligence test as they did at age 20. In a similar way, maybe the 80-year-olds would have scored relatively low on the intelligence test even at ages 50 and 20; the researchers don’t know for certain because they did not follow the same individuals as they got older.
It is also possible that the differences found between the age groups are not due to age, per se, but due to cohort effects. The 80-year-olds in this 2010 research grew up during a particular time and experienced certain events as a group. They were born in 1930 and are part of the Traditional or Silent Generation. The 50-year-olds were born in 1960 and are members of the Baby Boomer cohort. The 20-year-olds were born in 1990 and are part of the Millennial or Gen Y Generation. What kinds of things did each of these cohorts experience that the others did not experience or at least not in the same ways?
You may have come up with many differences between these cohorts’ experiences, such as living through certain wars, political and social movements, economic conditions, advances in technology, changes in health and nutrition standards, etc. There may be particular cohort differences that could especially influence their performance on intelligence tests, such as education level and use of computers. That is, many of those born in 1930 probably did not complete high school; those born in 1960 may have high school degrees, on average, but the majority did not attain college degrees; the young adults are probably current college students. And this is not even considering additional factors such as gender, race, or socioeconomic status. The young adults are used to taking tests on computers, but the members of the other two cohorts did not grow up with computers and may not be as comfortable if the intelligence test is administered on computers. These factors could have been a factor in the research results.
Another disadvantage of cross-sectional research is that it is limited to one time of measurement. Data are collected at one point in time and it’s possible that something could have happened in that year in history that affected all of the participants, although possibly each cohort may have been affected differently. Just think about the mindsets of participants in research that was conducted in the United States right after the terrorist attacks on September 11, 2001.
Longitudinal research designs
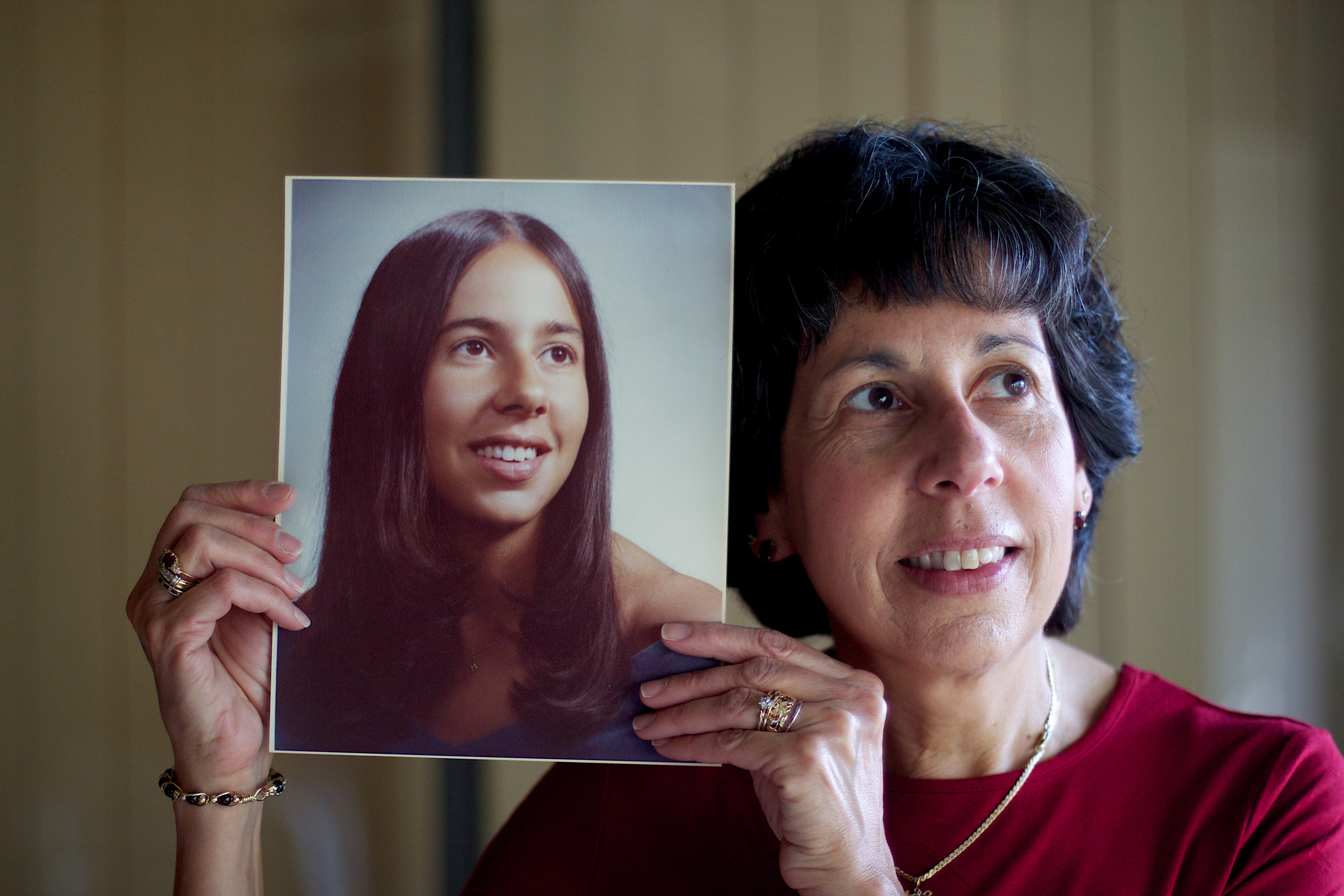
Longitudinal research involves beginning with a group of people who may be of the same age and background (cohort) and measuring them repeatedly over a long period of time. One of the benefits of this type of research is that people can be followed through time and be compared with themselves when they were younger; therefore changes with age over time are measured. What would be the advantages and disadvantages of longitudinal research? Problems with this type of research include being expensive, taking a long time, and subjects dropping out over time. Think about the film, 63 Up , part of the Up Series mentioned earlier, which is an example of following individuals over time. In the videos, filmed every seven years, you see how people change physically, emotionally, and socially through time; and some remain the same in certain ways, too. But many of the participants really disliked being part of the project and repeatedly threatened to quit; one disappeared for several years; another died before her 63rd year. Would you want to be interviewed every seven years? Would you want to have it made public for all to watch?
Longitudinal research designs are used to examine behavior in the same individuals over time. For instance, with our example of studying intelligence and aging, a researcher might conduct a longitudinal study to examine whether 20-year-olds become less intelligent with age over time. To this end, a researcher might give an intelligence test to individuals when they are 20 years old, again when they are 50 years old, and then again when they are 80 years old. This study is longitudinal in nature because the researcher plans to study the same individuals as they age. Based on these data, the pattern of intelligence and age might look different than from the cross-sectional research; it might be found that participants’ intelligence scores are higher at age 50 than at age 20 and then remain stable or decline a little by age 80. How can that be when cross-sectional research revealed declines in intelligence with age?

Since longitudinal research happens over a period of time (which could be short term, as in months, but is often longer, as in years), there is a risk of attrition. Attrition occurs when participants fail to complete all portions of a study. Participants may move, change their phone numbers, die, or simply become disinterested in participating over time. Researchers should account for the possibility of attrition by enrolling a larger sample into their study initially, as some participants will likely drop out over time. There is also something known as selective attrition— this means that certain groups of individuals may tend to drop out. It is often the least healthy, least educated, and lower socioeconomic participants who tend to drop out over time. That means that the remaining participants may no longer be representative of the whole population, as they are, in general, healthier, better educated, and have more money. This could be a factor in why our hypothetical research found a more optimistic picture of intelligence and aging as the years went by. What can researchers do about selective attrition? At each time of testing, they could randomly recruit more participants from the same cohort as the original members, to replace those who have dropped out.
The results from longitudinal studies may also be impacted by repeated assessments. Consider how well you would do on a math test if you were given the exact same exam every day for a week. Your performance would likely improve over time, not necessarily because you developed better math abilities, but because you were continuously practicing the same math problems. This phenomenon is known as a practice effect. Practice effects occur when participants become better at a task over time because they have done it again and again (not due to natural psychological development). So our participants may have become familiar with the intelligence test each time (and with the computerized testing administration).
Another limitation of longitudinal research is that the data are limited to only one cohort. As an example, think about how comfortable the participants in the 2010 cohort of 20-year-olds are with computers. Since only one cohort is being studied, there is no way to know if findings would be different from other cohorts. In addition, changes that are found as individuals age over time could be due to age or to time of measurement effects. That is, the participants are tested at different periods in history, so the variables of age and time of measurement could be confounded (mixed up). For example, what if there is a major shift in workplace training and education between 2020 and 2040 and many of the participants experience a lot more formal education in adulthood, which positively impacts their intelligence scores in 2040? Researchers wouldn’t know if the intelligence scores increased due to growing older or due to a more educated workforce over time between measurements.
Sequential research designs
Sequential research designs include elements of both longitudinal and cross-sectional research designs. Similar to longitudinal designs, sequential research features participants who are followed over time; similar to cross-sectional designs, sequential research includes participants of different ages. This research design is also distinct from those that have been discussed previously in that individuals of different ages are enrolled into a study at various points in time to examine age-related changes, development within the same individuals as they age, and to account for the possibility of cohort and/or time of measurement effects. In 1965, K. Warner Schaie [1] (a leading theorist and researcher on intelligence and aging), described particular sequential designs: cross-sequential, cohort sequential, and time-sequential. The differences between them depended on which variables were focused on for analyses of the data (data could be viewed in terms of multiple cross-sectional designs or multiple longitudinal designs or multiple cohort designs). Ideally, by comparing results from the different types of analyses, the effects of age, cohort, and time in history could be separated out.
Consider, once again, our example of intelligence and aging. In a study with a sequential design, a researcher might recruit three separate groups of participants (Groups A, B, and C). Group A would be recruited when they are 20 years old in 2010 and would be tested again when they are 50 and 80 years old in 2040 and 2070, respectively (similar in design to the longitudinal study described previously). Group B would be recruited when they are 20 years old in 2040 and would be tested again when they are 50 years old in 2070. Group C would be recruited when they are 20 years old in 2070 and so on.
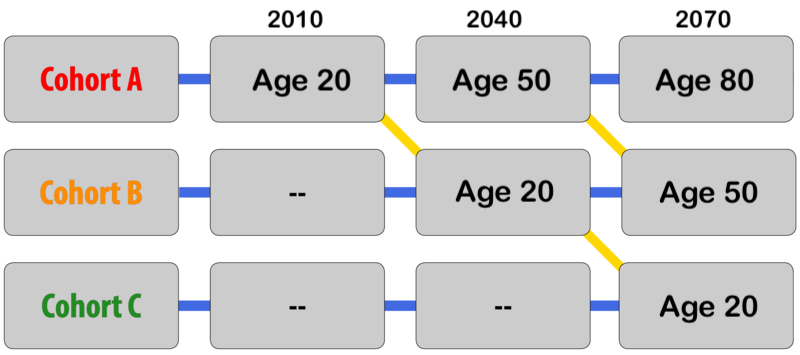
Studies with sequential designs are powerful because they allow for both longitudinal and cross-sectional comparisons—changes and/or stability with age over time can be measured and compared with differences between age and cohort groups. This research design also allows for the examination of cohort and time of measurement effects. For example, the researcher could examine the intelligence scores of 20-year-olds in different times in history and different cohorts (follow the yellow diagonal lines in figure 3). This might be examined by researchers who are interested in sociocultural and historical changes (because we know that lifespan development is multidisciplinary). One way of looking at the usefulness of the various developmental research designs was described by Schaie and Baltes (1975) [2] : cross-sectional and longitudinal designs might reveal change patterns while sequential designs might identify developmental origins for the observed change patterns.
Since they include elements of longitudinal and cross-sectional designs, sequential research has many of the same strengths and limitations as these other approaches. For example, sequential work may require less time and effort than longitudinal research (if data are collected more frequently than over the 30-year spans in our example) but more time and effort than cross-sectional research. Although practice effects may be an issue if participants are asked to complete the same tasks or assessments over time, attrition may be less problematic than what is commonly experienced in longitudinal research since participants may not have to remain involved in the study for such a long period of time.
When considering the best research design to use in their research, scientists think about their main research question and the best way to come up with an answer. A table of advantages and disadvantages for each of the described research designs is provided here to help you as you consider what sorts of studies would be best conducted using each of these different approaches.
https://assessments.lumenlearning.co...essments/16509
[glossary-page] [glossary-term]attrition:[/glossary-term] [glossary-definition]occurs when participants fail to complete all portions of a study[/glossary-definition]
[glossary-term]cross-sectional research:[/glossary-term] [glossary-definition]used to examine behavior in participants of different ages who are tested at the same point in time; may confound age and cohort differences[/glossary-definition]
[glossary-term]longitudinal research:[/glossary-term] [glossary-definition]studying a group of people who may be of the same age and background (cohort), and measuring them repeatedly over a long period of time; may confound age and time of measurement effects[/glossary-definition]
[glossary-term]research design:[/glossary-term] [glossary-definition]the strategy or blueprint for deciding how to collect and analyze information; dictates which methods are used and how[/glossary-definition]
[glossary-term]selective attrition:[/glossary-term] [glossary-definition]certain groups of individuals may tend to drop out more frequently resulting in the remaining participants longer being representative of the whole population[/glossary-definition]
[glossary-term]sequential research design:[/glossary-term] [glossary-definition]combines aspects of cross-sectional and longitudinal designs, but also adding new cohorts at different times of measurement; allows for analyses to consider effects of age, cohort, time of measurement, and socio-historical change[/glossary-definition] [/glossary-page]
- Schaie, K.W. (1965). A general model for the study of developmental problems. Psychological Bulletin, 64(2), 92-107. ↵
- Schaie, K.W. & Baltes, B.P. (1975). On sequential strategies in developmental research: Description or Explanation. Human Development, 18: 384-390. ↵
Contributors and Attributions
- Modification, adaptation, and original content. Authored by : Margaret Clark-Plaskie for Lumen Learning. Provided by : Lumen Learning. License : CC BY-NC-SA: Attribution-NonCommercial-ShareAlike
- Research Methods in Developmental Psychology. Authored by : Angela Lukowski and Helen Milojevich. Provided by : University of Calfornia, Irvine. Located at : https://nobaproject.com/modules/research-methods-in-developmental-psychology?r=LDcyNTg0 . Project : The Noba Project. License : CC BY-NC-SA: Attribution-NonCommercial-ShareAlike
- Woman holding own photograph. Provided by : Pxhere. Located at : https://pxhere.com/en/photo/221167 . License : CC0: No Rights Reserved
Using Science to Inform Educational Practices
Developmental Research Designs
Sometimes, especially in developmental research, the researcher is interested in examining changes over time and will need to consider a research design that will capture these changes. Remember, research methods are tools that are used to collect information, while r esearch design is the strategy or blueprint for deciding how to collect and analyze information. Research design dictates which methods are used and how. There are three types of developmental research designs: cross-sectional, longitudinal, and sequential.
Video 2.9.1. Developmental Research Design summarizes the benefits of challenges of the three developmental design models.
Cross-Sectional Designs
The majority of developmental studies use cross-sectional designs because they are less time-consuming and less expensive than other developmental designs. Cross-sectional research designs are used to examine behavior in participants of different ages who are tested at the same point in time. Let’s suppose that researchers are interested in the relationship between intelligence and aging. They might have a hypothesis that intelligence declines as people get older. The researchers might choose to give a particular intelligence test to individuals who are 20 years old, individuals who are 50 years old, and individuals who are 80 years old at the same time and compare the data from each age group. This research is cross-sectional in design because the researchers plan to examine the intelligence scores of individuals of different ages within the same study at the same time; they are taking a “cross-section” of people at one point in time. Let’s say that the comparisons find that the 80-year-old adults score lower on the intelligence test than the 50-year-old adults, and the 50-year-old adults score lower on the intelligence test than the 20-year-old adults. Based on these data, the researchers might conclude that individuals become less intelligent as they get older. Would that be a valid (accurate) interpretation of the results?
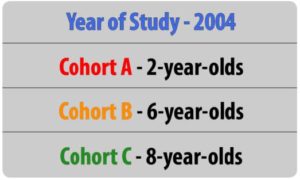
Figure 2.9.1. Example of cross-sectional research design
No, that would not be a valid conclusion because the researchers did not follow individuals as they aged from 20 to 50 to 80 years old. One of the primary limitations of cross-sectional research is that the results yield information about age differences not necessarily changes over time. That is, although the study described above can show that the 80-year-olds scored lower on the intelligence test than the 50-year-olds, and the 50-year-olds scored lower than the 20-year-olds, the data used for this conclusion were collected from different individuals (or groups). It could be, for instance, that when these 20-year-olds get older, they will still score just as high on the intelligence test as they did at age 20. Similarly, maybe the 80-year-olds would have scored relatively low on the intelligence test when they were young; the researchers don’t know for certain because they did not follow the same individuals as they got older.
With each cohort being members of a different generation, it is also possible that the differences found between the groups are not due to age, per se, but due to cohort effects. Differences between these cohorts’ IQ results could be due to differences in life experiences specific to their generation, such as differences in education, economic conditions, advances in technology, or changes in health and nutrition standards, and not due to age-related changes.
Another disadvantage of cross-sectional research is that it is limited to one time of measurement. Data are collected at one point in time, and it’s possible that something could have happened in that year in history that affected all of the participants, although possibly each cohort may have been affected differently.
Longitudinal Research Designs
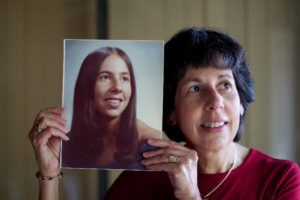
Longitudinal research designs are used to examine behavior in the same individuals over time. For instance, with our example of studying intelligence and aging, a researcher might conduct a longitudinal study to examine whether 20-year-olds become less intelligent with age over time. To this end, a researcher might give an intelligence test to individuals when they are 20 years old, again when they are 50 years old, and then again when they are 80 years old. This study is longitudinal in nature because the researcher plans to study the same individuals as they age. Based on these data, the pattern of intelligence and age might look different than from the cross-sectional research; it might be found that participants’ intelligence scores are higher at age 50 than at age 20 and then remain stable or decline a little by age 80. How can that be when cross-sectional research revealed declines in intelligence with age?

Figure 2.9.2. Example of a longitudinal research design
Since longitudinal research happens over a period of time (which could be short-term, as in months, but is often longer, as in years), there is a risk of attrition. Attrition occurs when participants fail to complete all portions of a study. Participants may move, change their phone numbers, die, or simply become disinterested in participating over time. Researchers should account for the possibility of attrition by enrolling a larger sample into their study initially, as some participants will likely drop out over time. There is also something known as selective attrition— this means that certain groups of individuals may tend to drop out. It is often the least healthy, least educated, and lower socioeconomic participants who tend to drop out over time. That means that the remaining participants may no longer be representative of the whole population, as they are, in general, healthier, better educated, and have more money. This could be a factor in why our hypothetical research found a more optimistic picture of intelligence and aging as the years went by. What can researchers do about selective attrition? At each time of testing, they could randomly recruit more participants from the same cohort as the original members to replace those who have dropped out.
The results from longitudinal studies may also be impacted by repeated assessments. Consider how well you would do on a math test if you were given the exact same exam every day for a week. Your performance would likely improve over time, not necessarily because you developed better math abilities, but because you were continuously practicing the same math problems. This phenomenon is known as a practice effect. Practice effects occur when participants become better at a task over time because they have done it again and again (not due to natural psychological development). So our participants may have become familiar with the intelligence test each time (and with the computerized testing administration).
Another limitation of longitudinal research is that the data are limited to only one cohort. As an example, think about how comfortable the participants in the 2010 cohort of 20-year-olds are with computers. Since only one cohort is being studied, there is no way to know if findings would be different from other cohorts. In addition, changes that are found as individuals age over time could be due to age or to time of measurement effects. That is, the participants are tested at different periods in history, so the variables of age and time of measurement could be confounded (mixed up). For example, what if there is a major shift in workplace training and education between 2020 and 2040, and many of the participants experience a lot more formal education in adulthood, which positively impacts their intelligence scores in 2040? Researchers wouldn’t know if the intelligence scores increased due to growing older or due to a more educated workforce over time between measurements.
Sequential Research Designs
Sequential research designs include elements of both longitudinal and cross-sectional research designs. Similar to longitudinal designs, sequential research features participants who are followed over time; similar to cross-sectional designs, sequential research includes participants of different ages. This research design is also distinct from those that have been discussed previously in that individuals of different ages are enrolled into a study at various points in time to examine age-related changes, development within the same individuals as they age, and to account for the possibility of cohort and/or time of measurement effects
Consider, once again, our example of intelligence and aging. In a study with a sequential design, a researcher might recruit three separate groups of participants (Groups A, B, and C). Group A would be recruited when they are 20 years old in 2010 and would be tested again when they are 50 and 80 years old in 2040 and 2070, respectively (similar in design to the longitudinal study described previously). Group B would be recruited when they are 20 years old in 2040 and would be tested again when they are 50 years old in 2070. Group C would be recruited when they are 20 years old in 2070, and so on.
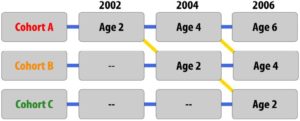
Figure 2.9.3. Example of sequential research design
Studies with sequential designs are powerful because they allow for both longitudinal and cross-sectional comparisons—changes and/or stability with age over time can be measured and compared with differences between age and cohort groups. This research design also allows for the examination of cohort and time of measurement effects. For example, the researcher could examine the intelligence scores of 20-year-olds at different times in history and different cohorts (follow the yellow diagonal lines in figure 2.9.3). This might be examined by researchers who are interested in sociocultural and historical changes (because we know that lifespan development is multidisciplinary). One way of looking at the usefulness of the various developmental research designs was described by Schaie and Baltes (1975): cross-sectional and longitudinal designs might reveal change patterns while sequential designs might identify developmental origins for the observed change patterns.
Since they include elements of longitudinal and cross-sectional designs, sequential research has many of the same strengths and limitations as these other approaches. For example, sequential work may require less time and effort than longitudinal research (if data are collected more frequently than over the 30-year spans in our example) but more time and effort than cross-sectional research. Although practice effects may be an issue if participants are asked to complete the same tasks or assessments over time, attrition may be less problematic than what is commonly experienced in longitudinal research since participants may not have to remain involved in the study for such a long period of time.
Comparing Developmental Research Designs
When considering the best research design to use in their research, scientists think about their main research question and the best way to come up with an answer. A table of advantages and disadvantages for each of the described research designs is provided here to help you as you consider what sorts of studies would be best conducted using each of these different approaches.
Table 2.9.1. Advantages and disadvantages of different research designs
Candela Citations
- Developmental Research Design. Authored by : Nicole Arduini-Van Hoose. Provided by : Hudson Valley Community College. Retrieved from : https://courses.lumenlearning.com/edpsy/chapter/developmental-research-designs/. License : CC BY-NC-SA: Attribution-NonCommercial-ShareAlike
- Developmental Research Designs. Authored by : Nicole Arduini-Van Hoose. Provided by : Hudson Valley Community College. Retrieved from : https://courses.lumenlearning.com/adolescent/chapter/developmental-research-design/. License : CC BY-NC-SA: Attribution-NonCommercial-ShareAlike
Educational Psychology Copyright © 2020 by Nicole Arduini-Van Hoose is licensed under a Creative Commons Attribution-NonCommercial-ShareAlike 4.0 International License , except where otherwise noted.
Share This Book

Want to create or adapt books like this? Learn more about how Pressbooks supports open publishing practices.
Descriptive and Explanatory Designs
Ellen Skinner; Julia Dancis; and The Human Development Teaching & Learning Group
Learning Objectives: Descriptive and Explanatory Designs
- Compare and contrast the three goals of lifespan developmental science.
- Define the three descriptive designs, namely, cross-sectional, longitudinal, and sequential, and identify their fatal flaws /confounds, strengths and limitations.
- Explain how sequential designs deal with the fatal flaws of the two basic designs, and how each design fits into a program of developmental research.
- Define the two explanatory designs, namely, naturalistic/correlational and experimental, show how they can be used in laboratory or field settings , and identify their strengths and limitations .
- Describe explanatory designs that extend these two and compensate for some of their limitations.
Goals of Lifespan Developmental Science
Research methods are tools that serve scientific ways of knowing, and their utility depends on the extent to which they can help researchers reach their scientific goals. From a lifespan perspective, developmental science has three primary goals: to describe, explain, and optimize human development (Baltes, Reese, & Nesselroade, 1977; see Table 1.1). Because these goals are embedded within the larger meta-theory created by the lifespan perspective, they target two kinds of development: (1) patterns of normative change and stability ; and (2) patterns of differential change and stability . When researchers say they are interested in understanding normative stability and change, they mean typical or regular age-graded patterns of individual change and constancy. When researchers want to understand differential development , they mean the different pathways that people can follow over time, including differences in the amount, nature, and direction of change. Moreover, researchers understand that some development entails quantitative changes (often called “trajectories”) and others involve qualitative shifts , such as the reorganization of existing forms or the emergence of new forms.
Description is the most basic task for all scientists. For developmental scientists, description involves depicting, portraying, or representing patterns of development in their target phenomena. This includes description of normative development, or typical quantitative and qualitative age-graded changes and continuities, as well as identifying the variety of different quantitative and qualitative pathways the phenomena can take. Explanations refer to explicit accounts of the factors that cause, influence, or produce the patterns of changes and stability that have been described. These are completely different from descriptions themselves. Descriptions answer questions like “what?” (i.e., the nature of the target phenomena), “how?” (i.e., the ways in which phenomena can change or remain the same), and “when?” (i.e., the ways in which these patterns appear as a function of age or time), whereas explanations focus on “why?”.
The goal of optimization refers to research and intervention activities designed to figure out how to promote healthy development (also referred to as flourishing or thriving) and the development of resilience. This task goes beyond description and explanation in two ways. First, in order to optimize development, trajectories and pathways must be identified as targets—targets that represent “optimal” development. These kinds of trajectories are often better than normative development, and so represent rare or even imaginary pathways, especially for groups living in highly risky environments. The search for optimal pathways reflects the assumption that individuals hold much more potential and plasticity in their development than is typically expressed or observed. The second way that optimization goes beyond description and explanation is that even when explanatory theories and research have identified all the conditions needed to promote optimal development, researchers and interventionists must still discover the strategies and levers that can consistently bring about these developmental conditions. One way to understand the difference between explanation and optimization is that, if explanations focus on the antecedents of a developmental phenomenon, then optimization efforts focus on the antecedents of these antecedents.
To learn more about these three goals, have a look at the expanded reading at this link.
Describe, Explain, Optimize [pdf]
- Normative stability and change : How do people typically develop and remain the same?
- Differential stability and change : What are the variety of different pathways that development (and stability) can follow?
- “What?”: the nature of the target phenomena.
- “How?”: the ways in which phenomena can change or remain the same.
- “When?”: the ways in which these patterns appear as a function of age or time.
- Normative stability and development: Why do people develop along a typical path?
- Differential stability and change : What accounts for different patterns of development?
- “Why?”: What are the underlying or overarching causes or factors that shape development?
- “Causality:” Influence, factors, promote, undermine, foster, nurture, support.
- Including changes and stability
- Typical and a variety of pathways
- Can be rare or imaginary
- Identify causal factors needed to support optimal development
- Locate “levers” to shift systems so that they sustain these positive causal factor
The term design refers to “when,” “where,” and “how” data are collected, so the term can be used in three different ways.
Descriptive developmental designs refer to when data are collected. These included cross-sectional, longitudinal, and sequential designs. Explanatory designs refer to how and where data are collected, so they include experimental vs. naturalistic (or correlational) designs, and data collected in laboratory vs. field settings. As a result, designs combine all three of these features, for example, researchers conduct longitudinal field experiments, cross-sectional lab experiments, and naturalistic longitudinal field studies. In the next sections, these kinds of designs are explained in more detail.
Describing Development: Cross-Sectional, Longitudinal, and Sequential Designs
Developmental designs are ways of collecting data (information) about people that allow the researcher to see how people differ or change with age. There are two simple developmental designs: cross-sectional and longitudinal.
Why is it challenging to figure how people differ or change with age?
It is challenging because people’s lives are always embedded in historical time. As soon as a person is born, he or she is inserted into a specific historical moment. And, as the person ages and changes, society is changing right along with them. For example, if you were born in 1990, I know exactly how old you were when the Twin Towers fell, when the Great Recession hit, and when iTunes opened. From a research design perspective, we can say that peoples’ development is confounded with historical changes (that is, with the specific historical events and general societal trends that occur during their lifetimes). The problem with either of the simple developmental designs is that they do not allow clear inferences about (1) whether differences between age groups are really due to age or to historical differences, or (2) whether changes in people over time are really due to age changes or to historical changes. That is why lifespan researchers need to use designs that give them more information than simple cross-sectional or longitudinal studies do, such as sequential designs.
Can you remind me about the cross-sectional design?
A CROSS-SECTIONAL DESIGN collects information (1) at one point in time (one time of measurement) on (2) groups of people who are different ages.
Design for a cross-sectional (CS) study : (1) conducted in 1960, using (2) six age groups:
The good news about cross-sectional studies is that we get information about a wide range of age differences in a short period of time (one time of measurement), and we get information about differences between groups of people of different ages.
From a developmental perspective, however, the bad news about cross-sectional studies is that we don’t get any information about the thing we are most interested in, that is, change . Cross sectional studies provide no information (1) about how people change, (2) about pathways (or trajectories) different people take; or (3) about how earlier events or experiences predict later functioning.
What is the problem with cross-sectional designs?
The problem is that you CANNOT infer that differences between the age groups are the same as age changes .
Because differences between age groups could be generational differences. What looks like age differences could really be cohort differences. Generational cohorts are groups of people who were born at the same time. They are sometimes just called “generations” and have been given labels, like the baby boomers, generation X, the millennial generation, and so on.
What are COHORT effects?
“Cohort effects” are the lifelong effects of belonging to a specific generation. They reflect the idea that being born at a specific time influences your development. Each of us has a series of experiences based on growing up during a specific historical period. Cohort effects also reflect the idea that specific historical events and trends have a different impact on people depending on how old they are. The Great Recession may have a very different effect on your development if you were 8 or 18 or 28 or 80. “Cohort effects” are the idea that people’s developmental pathways may differ based on the cumulative effects of these differences in experiences. If you want to learn more about different generations, you could watch this video about Generations throughout History (not required, bonus information).
What problem does that create for cross-sectional designs?
The problem is that the people in the different age groups also belong to different cohorts. In cross-sectional studies age is always confounded with cohort. Differences between the groups COULD be age differences or they COULD be cohort differences. You can see this confounding in the cross-sectional study depicted below. The participants who are 30 also belong to the 1930 birth cohort; the participants who are 40 also belong to the 1920 birth cohort, and so on. If we know the person’s age and the time of measurement, we can figure out their birth cohort.
Developmental researchers want to know if people differ according to their ages . But the fatal flaw with cross-sectional studies is that any differences between the groups in this design could be EITHER age differences OR generational (cohort) differences. Ages are completely confounded with cohorts (years of birth or generations). Any cross-sectional study could also be called an intergenerational study.
What is a LONGITUDINAL design?
It’s a study that examines (1) one group of people (2) repeatedly over multiple time points. Here is a longitudinal (LONG) study design that includes six times of measurement:
The good news about a longitudinal study is that it provides information about how people CHANGE or DEVELOP, as well as information about different people’s pathways or trajectories. It also allows researchers to see whether earlier experiences or events predict later outcomes.
BUT– are these really age changes?
Maybe, but they could also be historical changes. The fatal flaw with longitudinal studies is that changes in people over time could be EITHER due to age changes OR historical changes between times of measurement. As people age, the historical time they inhabit changes right along with them. Age and time of measurement are completely confounded , because all lives are embedded in historical time.
Can you see that age change is confounded with historical change in this longitudinal study? People who were 10 were also living in 1960, when they were 20, they were living in 1970, and so on. So, when people were changing from age 10 to age 20, the society they were living in also changed from 1960 to 1970. Therefore, people’s changes COULD be due to age (i.e., development), OR they could be due to historical changes over the times during which data were collected, or both.
If the simple developmental designs don’t work, what kinds of designs can developmental researchers use?
SEQUENTIAL designs are one good answer. They allow researchers to look at BOTH age changes and historical changes for multiple cohorts. There are many kinds of sequential designs. The one that we will learn about is called a Cross-sequential design. It provides the most developmental information in the shortest amount of time. It allows researchers to look at differences between people in terms of cohorts, and also to examine historical changes.
What is a CROSS-SEQUENTIAL design?
Cross-sequential designs combine cross-sectional and longitudinal designs. A cross-sequential study starts with a cross-sectional study that the researcher then follows up longitudinally for multiple measurement points. Here is a cross-sequential design (in green) that has 3 times of measurement, with 3 cross-sections (shown in the bottom row in yellow) and 8 longitudinal sequences (shown in the last column in blue).
The good news about a cross-sequential study is that this allows the researcher to compare longitudinal sequences across the same ages for different cohorts. So, for example, the researcher can compare what it’s like to go from age 20 to age 30 from 1960 to 1970 (LONG-2) with what it’s like to go from age 20 to age 30 from 1970 to 1980 (LONG-1). This way, the researcher can determine whether changes were due to age or to cohort differences.
This design also allows you to see if people who are the same age but from different cohorts are different from each other. For example, whether the 30-year-olds from the 1950 cohort are different from the 30-year-olds from the 1940 cohort or the 1930 cohort. If the age groups do not differ, you can zip the different longitudinal sequences together:
In this way, you can get imaginary long-term longitudinal information pretty quickly. That’s why the cross-sequential design is also called an “accelerated longitudinal design” or the “most efficient design.” The bad news about cross-sequential designs is that they are complex to conduct and to analyze.
Do SEQUENTIAL DESIGNS get rid of all the problems with developmental designs?
No, but they do allow you to look at them. It may be that age differences ARE generational differences or that age changes ARE shaped by historical changes.
If you are a contextualist, that’s the phenomenon you are actually interested in. However, you may need a historian to make sense of historical and cohort effects, and you may need to collect historical information as you go.
Explaining Development: Experimental and Naturalistic Designs
Description is the task of depicting, portraying, or representing patterns of development in the target phenomena, including patterns of normative age-graded changes and continuities, as well as the variety of different quantitative and qualitative pathways. In contrast, explanation refers to an account of the causes that together are sufficient to produce the patterns of changes and stability that we have described: What sets of factors cause, influence, or produce these different patterns of normative and differential change or stability over time? Explanation focuses on the weighty question of “Why?”.
Of the many features of research designs, the ones most relevant to explanation refer to the “where” and “how” of collecting data, so next we consider studies that use experimental and naturalistic designs conducted in the lab and field . In order to answer the causal questions of interest to lifespan developmental researchers, we want to create designs that allow us to make valid inferences about causes and effects as they unfold in the actual contexts of daily life. This is the information we will need to take with us into our optimization efforts. Let’s consider the four possible combinations of designs and settings (see Table below), one at a time.
LABORATORY EXPERIMENTS
What is an experimental design?
The defining features of an experimental design are twofold. The researcher (1) decides exactly what the causal agent (treatment) will be, and (2) determines who will get it (treatment group) and who will not (control group). So the challenges are also twofold: (1) to create a beautiful package of causal agents and (2) to ensure that the treatment and control groups are identical on everything but the treatment. Creating a plausible treatment that contains the causal ingredient is always an art, but researchers have become increasingly sophisticated in (1) isolating the putative cause by creating control groups who receive every single part of the experience of the treatment group except the specified causal agent; and (2) ensuring that control groups are identical to the treatment group on preexisting attributes, both known (which can be equated via matching or controlling for measured attributes) and unknown (which can be equated via random assignment; Campbell & Stanley, 1963; Shadish, Cook, & Campbell, 2002).
In many design classes, you will learn that the best design (and some will say the only design) for demonstrating causality is the experiment. So, for some researchers, the experiment (and not the time machine) is considered the “gold standard.” And, in the olden days, it was often assumed that experiments only happen in the laboratory, so experimental designs and laboratory settings are often merged in students’ minds. So let’s take a minute to consider experiments in labs.
Do labs provide advantages for detecting causality?
Indeed they do. They cannot be beat for settings in which the researcher has complete control over both key design features. Researchers can ensure that assignment to experimental or control conditions is completely random; and they can guarantee that the hypothesized causal variable is administered exactly as prescribed. Labs also provide very clear lines of sight on our phenomena—both potential causes and potential effects.
Do lifespan developmental researchers care about random assignment?
Yes. Huge problems are created by the fact that in the contexts of daily life people are not randomly assigned to causal conditions—there are particular personal characteristics that belong to the people who get in the way of particular causal forces, or who participate in them directly. And so, if we are going to distinguish pre-existing conditions that launched someone on a particular developmental trajectory from the causal factors that we are interested in discerning, we have to create groups that are “the same on everything” before we start our causal show. Randomized assignment is one strategy to accomplish this, as well as its more systematic options, such as block randomization (randomly assigning different categories of people), matching, propensity score matching, and so on.
Why are we so excited about exact control of the causal factor?
Well, that’s the magic of experimental designs. The researcher is like the fairy godmother who waves her wand and introduces the potentially new future for the treatment group. So the researcher knows that the treatment group got the potential causal factor, and how much of the factor, and so on. And then the researcher has approximately a bazgillion control groups, who got shades of everything but the hypothesized active ingredient. These can be very creative, the control group with nothing, with only attention, with a visit to the lab but no causal factor, with a causal factor that looks like the actual causal factor but really isn’t. Remember “placebos,” which were added to drug trials so that the control group even gets the experience of taking a pill?
NATURALISTIC LAB STUDIES
Are there lab studies that do not involve experiments?
Yes. They are called naturalistic lab studies because researchers bring participants into the lab setting, but do not administer a dose of a potential causal agent.
What would be some uses for naturalistic lab studies?
One important use is to measure constructs that you can’t capture outside of the lab. There are some phenomena of great interest that are not visible without specialized instrumentation or procedures that can be administered only in the lab setting. All manner of neurophysiological constructs can only be measured in the lab using complex equipment, like f MRI, as well as the assessment of internal states and capacities, like executive function or delay of gratification or implicit bias. Researchers can work with their participants in the lab in order to make these internal processes more visible. One great example is the “talk aloud protocol,” in which individuals (even children) are trained to narrate their mental processes as they work on a task or watch a demonstration. These protocols provide stream of consciousness information that would not be accessible in any other way.
A second important reason researchers might turn to lab settings is to create conditions where they can trigger and then observe interactions that are relatively rare in field settings. For example, research on learned helplessness often brings children into controlled settings where researchers can watch them work with solvable and then with unsolvable puzzles, mazes, and concept tasks, while monitoring their strategies, efforts, and actions over time. (And, of course they always end with success experiences.) Another example is the Strange Situation in which researchers trigger the attachment system in the living-room-like lab setting, by sending in a stranger and asking the caregiver to leave, and then observing the child’s actions.
These experiences are both rigorously standardized and intuitively compelling for participants. They can simulate transgressions (e.g., when a child hears a child in the next room knock over her block creation), competitions (e.g., when two children are playing a videogame and one messes up), exclusion (e.g., when a child is left out of a ball tossing game), and so on. Such standardization creates comparability among participants. It allows researchers to be sure that their differential responses are not due to differential provocations, but to their individual reactions to identical experiences.
In all these cases, naturalistic observation may seem more desirable, but social processes can be impossible to tease apart as they unfold in daily contexts. For example, mastery-oriented children run into fewer tasks they cannot solve than helpless-prone children and so it is harder to catch them in failure situations, and in schools teachers do not assign impossible tasks, and so observers could go for weeks without seeing their phenomena. And, by the way, after about five years of age, kids are busy trying to hide their true reactions to negative events (a phenomenon called “masking”), which makes it harder for observers to actually detect undesired states (like anxiety or boredom) in the field. In fact, precisely because people and their contexts are so intertwined, we sometimes bring our participants into the lab to see what they can do without the scaffolds or interference of social partners.
What are the main advantages of experimental and naturalistic lab studies?
Their claims to fame are control and precision . Because we have exact control of the causal factor and exact control over who receives it, we can make unambiguous causal inferences. These may be especially appreciated when we are trying to untangle the directions of effects in reciprocal proximal processes or transactions. Moreover, we have precision in our measurement or observation of the targets—both causes and effects. We can see below the surface (into neurophysiology or cognitive functioning). We can even trigger phenomena that are rare or hard to see in the wild, and record their details using our video cameras or talk aloud protocols.
What are the disadvantages of studies conducted in lab settings?
Well, for lifespan developmental researchers, they have some pretty serious limitations. Let’s think about three big ones. First, labs and fields are more than settings to us. They are contexts. And contexts are not just geographic and architectural locations, in the sense that you can simply pick people up and “set” them down in new places. Contexts have tentacles that reach out and wind themselves around people, and people have roots that reach down into places. They are connected, mutually created, interpenetrated even, so that our most likely causal forces, our proximal processes, cannot even be constituted when we look at only one without the other. When researchers split the child from his or her context, it destroys the phenomenon itself, like removing the heart from the body in order to see how it works. You can’t. Once you remove it, it doesn’t work anymore. So we worry that lab studies can alter or distort our phenomena.
So developmentalists don’t conduct research in laboratory settings?
Not at all. Contextualists are just very wary about the idea of the “setting” and very aware of what is lost by leaving the “scene of the crime,” that is, the contexts of daily life. The “field” is an intrinsic and crucial part of the target we are trying to understand, and if we are going to bring our whole phenomenon into the lab, we have to know all the relevant elements of the context and effectively simulate them in the lab. Otherwise, this kind of distortion can be a threat to internal validity.
What is the second limitation of laboratory studies?
Second, we assume that all our causal factors are embedded in contexts and shaped by them. Instead of thinking about the lab as a place where researchers can get more pristine information about their target phenomena (the child and their behavior), the lab has come to be regarded as one context with its own attributes (e.g., novelty) and set of social partners (i.e., the experimenter) that are exerting their own effects on the child. So if we are looking at the functioning of proximal processes in the lab, we can be sure that the lab context is shaping then, which means we can’t be sure that they actually operate the same way in the contexts of daily life. As a result, we always have to admit that any causal links we may have created in the lab have to be couched as “can cause” our target and not as “does cause” our target. We have to wait and see if these same processes are operating in the actual contexts that form the natural microsystems for our participants. Otherwise, this kind of artificiality can be a threat to external validity.
Third, the time span over which developmentalists assume that causal effects accumulate cannot be easily simulated in the lab. The causal processes of interest to developmentalists unfold over months and years and decades, across multiple contexts, so although we can use the lab to measure the long-term effects of causal factors by bringing our participants back to the lab as many times as we want to, if we want to actually look at the causal processes having their effects over months or years, it will be difficult to achieve that in the lab setting.
And are there corresponding disadvantages with experimental designs?
Well, for some developmentalists, they do have a fatal flaw. As noted by many methodologists, the seemingly insurmountable problem with experimental designs is that it is not possible to randomly assign or manipulate the causal forces that are of biggest interest to developmentalists. No one can randomly assign their participants to a particular age group (“I have flipped a coin and you will be in the five-year-old group” “Oh no, I wanted to be 10!”) or to a particular cohort or developmental history.
In fact, most of the causal factors that are of interest to us can’t ethically be manipulated at all—the happy single-parent family versus the unhappily married parents, the delinquent peer group versus theatre-obsessed friends, school failure versus success, peer rejection versus popularity, high stress reactivity, dangerous neighborhoods, or height. Before you ask, we will just add that this same issue applies to all areas of psychology. Many applied problems cannot be manipulated—divorce, PTSD, dangerous job conditions, psychopathology, work-family conflict, serious medical diagnosis, intimate partner violence, and so on. So there are serious limitations to how much experimental designs can help applied researchers study the conditions and causes that matter most to them.
FIELD EXPERIMENTS
Wait! What about field experiments designed to promote development?
Yes, indeed. And these field experiments can even be conducted as randomized controlled trials (RCTs; Shadish & Cook, 2009). And, yes, we can ethically study any old target we please as long as we are trying to promote development—to remediate unfavorable developmental trajectories, to maintain resilient ones, and in general to prevent adverse and promote healthy development.
What are randomized controlled trials?
As you may know, this methodology was borrowed from clinical trials of medical treatments, and it is cool in many ways. It has time in its design, which is always welcome news to developmentalists. RCTs compare (at least) two groups who should be equivalent to each other (based on random assignment), one of which has received the treatment and the other a placebo, so that researchers can examine the effects of the treatment over and above the effects of knowing that one is being treated. Then after a sufficient amount of time for the treatment to do its work, changes in the treatment and control group can be compared over however many time points the design includes. In recent decades, RCTs in field settings have become de rigueur for settling causal claims about “evidence-based” practices, programs, and policies. RCT field experiments provide a standard of proof that has made them essential to both the scientific community and to applied decision makers and stakeholders (e.g., see the What Works Clearinghouse ).
Do these kinds of studies have certain advantages?
Indeed, they do. Some researchers see field experiments as the best of both worlds. On the one hand, studies get to keep all the compelling features of experimental designs that are so helpful in making unambiguous causal inferences. But on the other hand, we have escaped from the lab setting and returned to the contexts of everyday life, so they generally evince higher authenticity and external validity. Often treatments are even administered by natural social partners in everyday contexts. Parents, teachers, bosses, coaches, or mentors receive systematic training and then bring their newly acquired attributes back into the settings of homes, classrooms, and workplaces, where researchers can determine whether they subsequently change the proximal processes and the development that takes place there.
Are there disadvantages to field experiments?
Many researchers consider RCTs to be “the gold standard,” but they have three important limitations for developmentalists. First, the very thing we like about them—getting out of the lab and into the field—can make the administration of the causal factor somewhat messy. We lose some of our beloved control and precision. Especially if researchers decide that the treatment (often an intervention program) will be administered through intermediaries (like teachers or caregivers or social workers), it can be a giant headache. A whole area of study, called “implementation research,” focuses on “implementation fidelity”—or how to make sure that participants actually make contact with the active ingredients of the potential causal factor.
Moreover, in the real world, participants can drop out of the treatment (e.g., the teacher or parent training) any time they want, but they do not drop out of the treatment group in our design. To maintain the equivalence between treatment and control groups accomplished by our initial random assignment, participants cannot voluntarily switch groups—or we have suddenly introduced bias. So the treatment group is often labeled the “intent to treat” group, meaning that participants were initially assigned to this group, even if they end up receiving no treatment whatsoever. It’s like doctors who send the treatment pills home with their patients and then hope for the best, but never get to count the pills that are left in the bottle at the end of the trial. If patients do not improve, they can’t really say whether the drug didn’t work or whether the patients just didn’t take their pills. Unsettling from a causal inference perspective.
A second limitation, and one that field experiments share with lab experiments, is that they cannot tell us what caused these unhealthy pathways of development in the first place, any more than studying aspirin can tell us what causes headaches or how to prevent them. So additional work will always be needed to fill in the causal puzzle of the factors that contribute to and maintain non-optimal development or lead to psychopathology. Such studies would be essential to prevention efforts.
Third, RCTs (in both lab and field) have inherent limitations in providing causal explanations . At the end of the day, the only thing that this design can tell you is “yes” or “no.” That is, the only information it yields is whether the two groups– treatment and control– are different. You can add many features, for example, many indicators of disease or health, you can measure dosage and its effects, over several time periods, and so on. However, developmentalists would say that, after all this work, the only thing we have in our hands is a causal description but not the thing that we most want, that is, a causal explanation . For the drug companies, everything they want to know about causal explanations is contained in the drug itself. To the extent that they care about how the drug works, its mechanisms of effects have already been studied (and of course, we take many drugs that are effective, but whose mechanisms of effects are unknown).
But as developmentalists, our interventions contain hundreds of potential active ingredients. And so we want to poke our heads under the hood and look all around, watching the cogs engage and the wheels turn. We want to watch the tennis game or the dance, and see who is hitting the ball the hardest and how the players adapt to each other’s style over time and who is playing the music. In other words, we are on the trail of causal explanation and so we can’t really be satisfied with “yes” or “no.” We will forever be asking “Why?” or “Why not?” and especially “How did that work?”. So we will always be supplementing experimental and lab studies, and even RCT studies, with studies using designs that can provide us with more complex process-oriented accounts of the multiple causes of differential developmental trajectories and transformations.
Take Home Messages about Different Explanatory Designs and Settings
All designs, including experimental lab and field designs, have advantages and disadvantages (see Table). Your job is to conduct each kind of study using the very best methods available (that’s the “looking” part of a scientist’s role) and then to keep straight on what you can and cannot see (that’s the “thinking” part of a scientist’s role). That way, you will learn what there really is to be learned using experimental designs, and then start longing for what can be seen using alternative designs that provide other lines of sight.
NATURALISTIC FIELD STUDIES
What we appreciate about naturalistic field studies is obvious from the limitations we encountered with lab and experimental designs: In naturalistic studies we can examine the effects of potential causes we can’t possibly administer (e.g., maltreatment, ability tracking, peer rejection). Moreover, we can watch these processes operate in their authentic multi-level contexts, and we can follow them for months and years.
But aren’t experiments the only way to show causality?
Yes, experiments can provide important evidence of causal processes. But let’s consider the kinds of causal evidence that can be provided by naturalistic studies.
Are we talking about correlational studies? Because we know for a fact that “correlation does not prove causation.”
Right, it is correct that correlation by itself does not prove causation. But let’s take a minute to understand why this is true, and then to see whether there are some things that researchers can do to improve the designs of their studies so that naturalistic studies, using more than correlations, can provide evidence about causes. Because, remember—correlation may not prove causation, but causal processes do generate correlations: Since causes produce effects, effects covary with their causes. In fact, this covariation is a defining condition of causality (see box). As a result, correlations (or covariation or contingencies, however you want to label them) may be the smoke that leads us to our causal fires. The problem is that many things besides causation lead to correlations, and so we have to work hard to decipher the causal evidence among all the other kinds of covariation information we are examining.
Okay, can you break down the reasons that correlations do not prove causation?
Yes, let’s start with a consideration of a typical correlation between two variables, and let’s pick variables that tap constructs we think could be causally connected, say, teacher involvement and student engagement (see figure below). Let’s say that in this research we get a robust correlation between good measures of both variables. Why can’t we conclude that teacher involvement influences student engagement? There are two main reasons. First, as also shown in the figure below, the connection between these two variables could be due to a reciprocal causal effect, in which student engagement influences teacher involvement. This direction of effects is conceptually plausible, since more engaged students could attract more positive teacher attention whereas more disaffected students could lead teachers to withdraw or treat students more harshly. Of course, the correlation could be due to both feedforward (i.e., teachers’ influences on students) and feedback (i.e., students’ influences on teachers) effects.
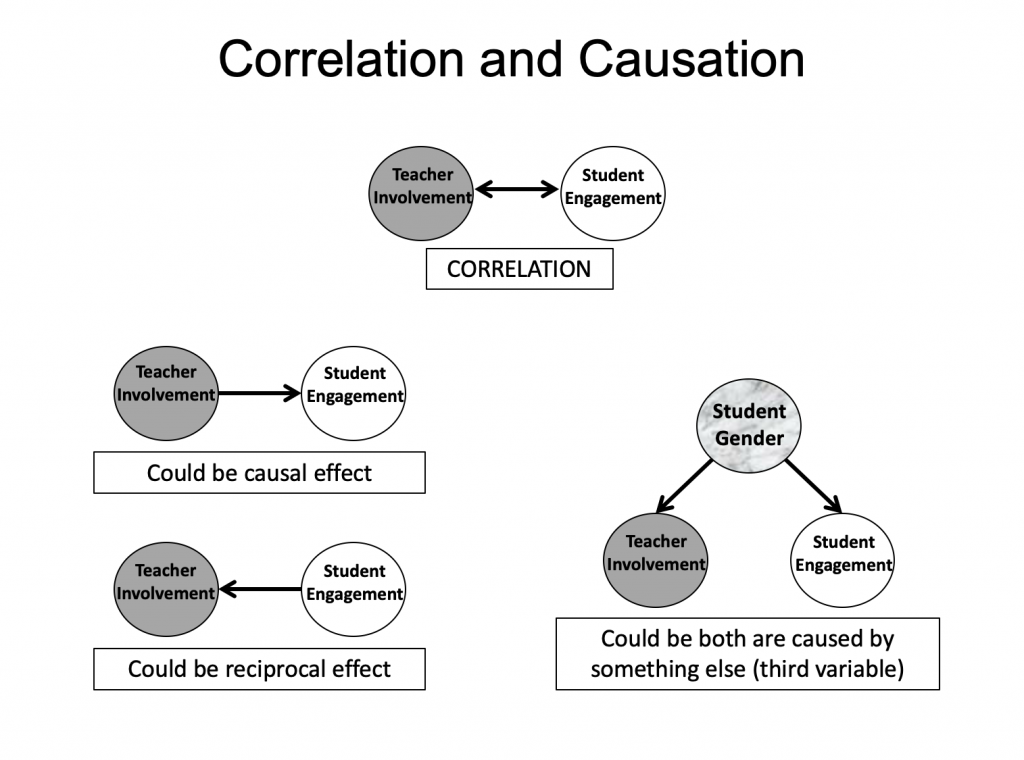
The second possibility is that there is no causal effect at all between these two variables (forward or backward). Instead, they are both actually produced by a completely different cause (the ominously named “third variable”); and they covary only because they are both effects from the same cause. In our example, also shown in the figure, we selected students’ gender as our third variable because gender is a plausible cause of both variables. In general, girls are more engaged and teachers show more involvement with them, whereas boys generally tend to be less engaged and teachers show less warmth toward them. In this scenario, as in all other scenarios involving concurrent correlations, there are a very large number of third variables (alternative causes of both) that could be in play—it could be achievement (engaged students perform better in school and teachers attend more to high performing students) or social class or student sense of relatedness—as well as a large number of third variables that we can’t immediately imagine. So in naturalistic designs, we use the term “third variable” as shorthand for all the alternative causal explanations that could underlie the connections between our hypothesized antecedent and its possible consequence.
Is there anything we can do to help solve these problems?
Yes, including time in the design of our naturalistic studies helps us out quite a bit.
What do you mean by “adding time” to a design?
When we say we are “adding time” to a design, we mean that we are adding “occasions” or “times of measurement” or “repeated measures” to a design.
Like in a longitudinal study?
Yes, but maybe the most general description is “time series” because the design includes a series of different times of measurement.
What are the advantages of adding time?
Let’s say that we add just one more time of measurement, so we have two waves in our study. The first advantage is that now we have a way to check the first condition of causality, namely, that causes precede their effects. So we can check out a time-ordered correlation. Continuing with our example, with time in the design, we can look at whether the potential cause at Time 1 predicts the potential outcome at Time 2. This is depicted in the figure below. So we are excited to be able to use the word “predict” correctly to describe our correlation. However, this is still just a zero-order bivariate correlation, so it does not allow a causal inference—it still has all the problems with those dreaded “third variables” or alternative causal explanations. But we can use our two time points to start looking at how our target outcome is changing from Time 1 to Time 2 and to see whether those changes can be predicted from where each person was on the potential cause at Time 1.
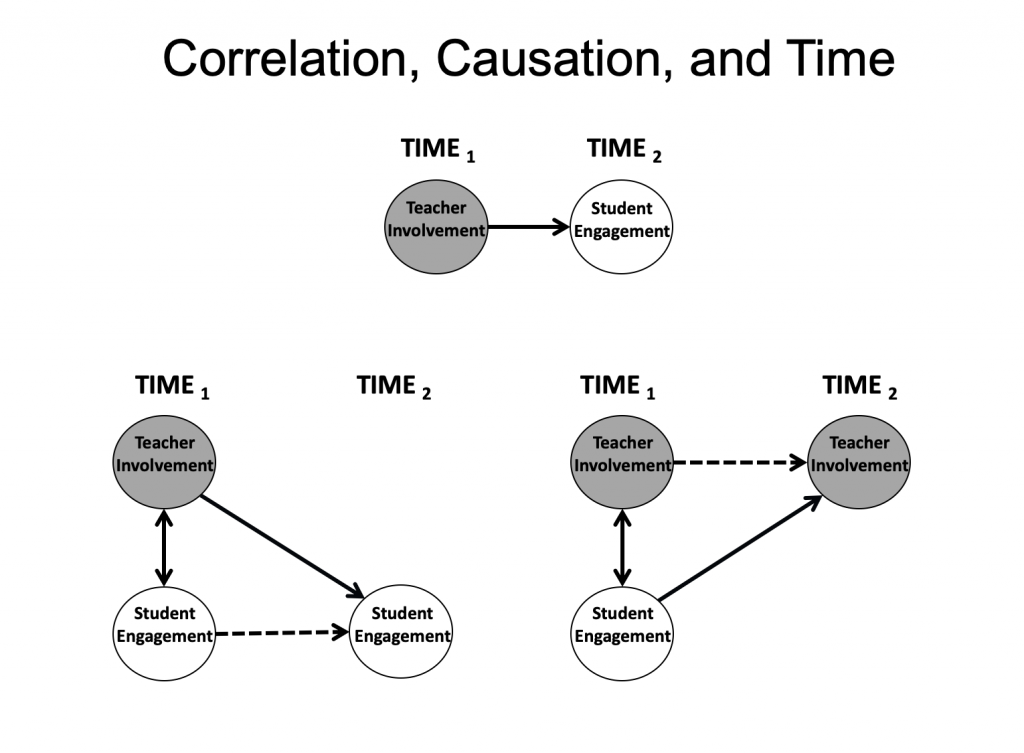
So what do we like about this kind of design?
We get to look at developmental trajectories as our outcomes (and if we add more time points, they will look more like trajectories), we are looking directly at individual differences in trajectories, and we are looking at predictors of individual differences in those trajectories. So, in our example, we can ask, “Does teacher involvement at the beginning of the school year predict changes in students’ engagement from the beginning to the end of the school year?”. And if the empirical answer is “yes” (i.e., the antecedent is a significant predictor of change from Time 1 to Time 2), we can say things like “Students whose teachers were warmer and more involved with them at the beginning of the school year, also showed increases in their engagement over the school year; whereas students whose teachers were less involved with them at the beginning of the school year, showed corresponding declines in their engagement as the year progressed.” This is a descriptive statement, but it is consistent with a causal hypothesis.
Any other advantages?
Yes. We can also, using the same design, look at the “reciprocal” predictions, in that we can take our antecedent variable and examine how it changes from Time 1 to Time 2, and see whether the variable we had been thinking of as a consequence (which we now consider as a possible antecedent) predicts these changes—see the figure above. In our example, we would be asking “Do students’ initial levels of engagement at the beginning of the year predict changes in how much involvement their teachers provide them over the year?” And, if the empirical answer is “yes,” we can say things like “Students who were more engaged in fall experienced increasing involvement from their teachers as the year progressed, whereas students who were initially higher in disaffection experienced declines in their teachers’ involvement from fall to spring.” One of the most important things about a design with two points of measurement (remember, we just added one more point) is that it allows researchers to begin to pull apart the different directions of effects. A concurrent correlation contains information about both directions of effects, which cannot logically be untangled, but the two analyses that we just ran can get the job done—the first looks at the feed forward prediction of teacher involvement on changes in subsequent student engagement, whereas the second looks at the feedback prediction of student engagement on changes in subsequent teacher involvement. So the answers to the questions posed by these two sets of analyses could be different—we could get two “yes”s or two “no”s or one of each. And if we get two “yes”s, we have the possibility of a feedback loop, which feels like we are getting some hints about potential dynamics in the system.
What about all those pesky third variables, those alternative explanations?
Well, we have good news and bad news about them.
What is the good news?
The good news is that we have reduced them some. If you start thinking about the third variables in the concurrent correlation in our illustration, that is, all the factors that are positively correlated with both teacher involvement and student engagement, an enormous number come to mind (e.g., achievement, SES, supportive caregivers, IQ, a sense of relatedness, and so on). And here is the kicker, these are only the ones we can imagine, there are also unknown confounders. However, when we include in our design and analyses intra-individual change over time, we are using people as their own controls. This means that out of our potential consequence at Time 2, we are taking each participant’s starting value of the consequence at Time 1, which has in it by definition everything (known and unknown) that led up to the consequence at Time 1 (e.g., achievement, SES, supportive caregivers, IQ, a sense of relatedness, and so on) as well as all the unknowns that created or predicted the consequence at Time 1.
So, for example, if we think that achievement is a possible alternative causal explanation for the zero-order correlation between teacher involvement at Time 1 and student engagement at Time 2 (meaning that high performing students are more engaged and teachers pay more attention to them), when we control for student engagement Time 1, we take out all of the achievement that was responsible for engagement up to that point, so we have controlled for that as a potential confounder. By controlling for the same variable at an earlier point in time, we have scraped off all the known and unknown predictors of engagement up until Time 1 that could be a potential confounder, or a plausible pre-existing difference, or an alternative causal chain.
So then what is the bad news?
The bad news is that the notorious third variables are not completely eliminated. Since we are still looking at a kind of correlation—specifically the correlation between teacher involvement at Time 1 and changes in student engagement from Time 1 to Time 2– we are still on the hunt for possible alternative causes of both. Remember, before we were looking for things that were correlated with both teacher involvement and student engagement, but now, with this design, we can narrow our candidates for third variables down to those that are correlated with both teacher involvement and changes in student engagement.
What should we be thinking about in adding time to our study design?
Let’s start with some basic questions that we almost never know the answers to—What are the right windows and the right time gaps between measurement points? We ran into this problem, called “time and timing” (Lerner, Schwartz, & Phelps, 2009), in the chapter on descriptive longitudinal designs, when we had to pick the developmental window (time) over which we thought our target change was likely to occur, and then decide on the spacing between measurement points (timing) needed to capture its hypothesized rate and pattern of change. In descriptive designs, we ask these questions about the developmental outcomes, but in explanatory designs, we also ask them about the causal process: “During what developmental window (time) are our target causal processes likely to be active?” and “What spacing (timing) should we use to capture the rate at which this causal process is likely to generate its effects (both feedforward and feedback)?”. For example, if we are thinking about teacher involvement and student engagement, it seems like the beginning of a new school year would be a good moment for them to be calibrating to each other (time), but how long would that take (timing)—a week, a month, six weeks? Who knows? One rule of thumb is to use more measurement points than you think you will need, so you can look over different time gaps for your possible process.
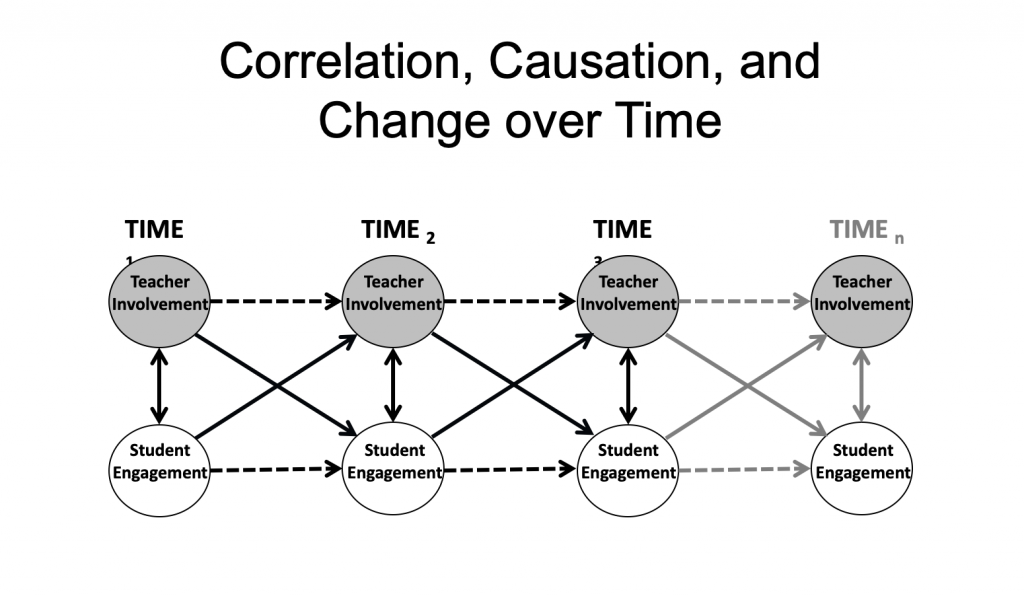
What do you mean “more”? Weren’t we excited to be adding just one more time of measurement?
Well, two is qualitatively better than one, so that’s good. But more really is merrier. Additional times of measurement allow researchers to look at these predictors of change across additional time gaps, for example, from a predictor at Time 2 to changes from Time 2 to Time 3 (as depicted in the figure). Multiple time points also open up a new world—one filled with trajectories of mean level change as the outcomes of our causal processes.
A first step in this direction has been referred to as a “launch” model because it tries to examine whether an individuals’ initial levels on an antecedent can predict the individuals’ trajectory on the target consequence. The term “launch” is used because such a model assumes that the initial levels of the potential causal variable may act like a catapult or rocket launcher to create the direction and angle of change in the object that is hurled, that is, the target outcome.
Can we even use these designs to look at how changes in our antecedents predict changes in our outcomes?
Yes, these have been called “change-to-change” models), but you can’t really use the word “predict” to describe these connections. They are actually like correlations between growth curves, and then we land back in our concurrent correlations puddle—where we still don’t know who is preceding whom. So it would probably be better to look at the connections between time-lagged growth curves, for example, the connections between a growth curve from Time 1 to Time n to predict an outcome from Time 2 to Time n+1. Then you could also look at reciprocal change-to-change effects by switching around your antecedents and consequences. Just like with the designs that incorporate only two measurement points, these two analyses can provide different estimates of the connections between the different portions of the growth curves.
What is happening with all our third variables in these analyses?
We can control for them, but it may be more interesting to look directly at their effects, by organizing our data into a “niche” study, where we look at the connections we are interested in for subgroups of children—for boys or girls separately or for students high or low in achievement. If the connection between teacher involvement and student engagement is due to gender, it will disappear when we look at boys and girls separately. As you know, looking directly at the developmental patterns of different groups is more consistent with a lifespan developmental systems perspective, which holds that development is differentially shaped by the characteristics of the people and their contexts.
These design ideas for naturalistic studies seem much less systematic than the strategies we learned about for experimental studies.
Yes, it can sometimes feel like the wild west out here on the frontier where we are trying to extract valid causal inferences from naturalistic studies. But keep in mind that you are not alone. Researchers from many other disciplines, like epidemiology, sociology, and economics (e.g., Gangl, 2010; Heckman, 2008), are also developing useful methods for tracking down causal processes, because they can no more create controlled experiments– in which they crash stock markets or start epidemics or shift social mores—than can developmental scientists. As part of this search, they are uncovering an important set of tools for causal inferences in the careful application of sound statistical methods (e.g., Madigan et al., 2014; see box).
Take Home Messages for Naturalistic Field Studies
We would highlight four.
- The first is a resounding “Yes!” to the question of whether naturalistic field studies can contribute to rich causal accounts of development . These are studies that contain everything you want to know about your target phenomenon, so it is worthwhile to figure out how to decipher that information in ways that yield valid causal accounts.
- The second take home is a resounding “Whoa!” because the ways that causes likely operate in the complex dynamic system of which our target phenomenon is a part can be truly mind-bending. So we need to pause and get our meta-theoretical glasses firmly on our noses before we start sifting through designs and strategies.
- Third, our most trusty tool is time itself —the times of measurement in longitudinal and time series designs, the time windows over which we choose to hover, and the timing (spacing) of our measurements so they map onto the pace of our causal processes.
- Our final take-home is that the search for design ideas for wresting causal information out of naturalistic field studies will take you into some beautiful and uncharted territory . When you encounter a complex problem (like historical embeddedness or cohort effects), your immediate reaction may “How do I avoid this problem?” or “How do I solve this problem?” But instead of resorting to coping, we would encourage you to react with curiosity. In other words, the best advice that lifespan developmental researchers can give themselves is always “Don’t ignore. Don’t evade. Turn around and look.” These are not methodological problems you have encountered. They are messages from your target developmental phenomena. And, if you have ears to hear, you can learn a great deal by walking directly towards them.
Take Home Messages for the Goals of Developmental Science and Descriptive and Explanatory Designs
Take home messages are also contained in the summary tables for the three goals of developmental science, and the tables describing (1) cross-sectional, longitudinal, and sequential designs, and for (2) experimental and correlational designs in the laboratory and the field. For the designs, we would like you to be able to define each one, identify the advantages and disadvantages of each, and discuss when it would make sense to use each of them in a program of research. Be sure to revisit the concept of converging operations — it helps pull together the idea that the strengths of each design can help compensate for the weaknesses of other designs!
Supplemental Materials
- This chapter discusses the use of qualitative methods in psychology and the ways in which qualitative inquiry has participated in a radical tradition.
Kidder, L.H., & Fine, M. (1997). Qualitative inquiry in psychology: A radical tradition. In D. Fox and I. Prilleltensky (Eds.) Critical psychology: An introduction (pp. 34-50). Thousand Oaks, CA: Sage.
- This article discusses the approach and method of Youth-led Participatory Action Research and implications for promoting healthy development.
Ozer, E. J. (2017). Youth‐led participatory action research: Overview and potential for enhancing adolescent development. Child Development Perspectives, 11(3), 173-177. doi:http://dx.doi.org/10.1111/cdep.12228
- The following article examines systematic racial inequality within the context of psychological research.
Roberts, S. O., Bareket-Shavit, C., Dollins, F. A., Goldie, P. D., & Mortenson, E. (2020). Racial inequality in psychological research: Trends of the past and recommendations for the future. Perspectives on Psychological Science , 1745691620927709.
Baltes, P. B., Reese, H. W., & Nesselroade, J. R. (1977). Life-span developmental psychology: Introduction to research methods . Oxford, England: Brooks/Cole.
Campbell, D. T. & Stanley, J. C. (1963). Experimental and quasi-experimental designs for research . Boston: Houghton Mifflin.
Case, R. (1985). Intellectual development . New York: Academic Press.
Eccles, J. S., & Midgley, C. (1989). Stage/environment fit: Developmentally appropriate classrooms for early adolescents. In R. Ames & C. Ames (Eds.), Research on motivation in education (Vol. 3, pp. 139-181). New York: Academic Press.
Eccles. J. S., Midgley, C., Wigfield, A., Buchanan, C. M., Reuman, D., Flanagan, C., & McIver, D. (1993). Development during adolescence: The impact of stage-environment fit on adolescents’ experiences in schools and families. American Psychologist, 48, 90-101.
Gangl, M. (2010). Causal inference in sociological research. Annual Review of Sociology , 36 , 21-47.
Heckman, J. J. (2008). Econometric causality. International Statistical Review , 76 (1), 1-27.
Lerner, R. M., Schwartz, S. J., & Phelps, E. (2009). Problematics of time and timing in the longitudinal study of human development: Theoretical and methodological issues. Human Development , 52 (1), 44-68.
Madigan, D., Stang, P. E., Berlin, J. A., Schuemie, M., Overhage, J. M., Suchard, M. A., … & Ryan, P. B. (2014). A systematic statistical approach to evaluating evidence from observational studies. Annual Review of Statistics and Its Application , 1 , 11-39.
Mill, J.S. (1843). A system of logic . London: Parker.
Shadish, W. R., & Cook, T. D. (2009). The renaissance of field experimentation in evaluating interventions. Annual Review of Psychology , 60 , 607-629.
Shadish, W. R., Cook, T. D., & Campbell, D. T. (2002). Experimental and quasi-experimental designs for generalized causal inference . Wadsworth Cengage learning.
Media Attributions
- correlation © Ellen Skinner
- correlation+time © Ellen Skinner
- chaneovertime © Ellen Skinner
Descriptive and Explanatory Designs Copyright © 2020 by Ellen Skinner; Julia Dancis; and The Human Development Teaching & Learning Group. All Rights Reserved.

Developmental Research Designs
Margaret Clark-Plaskie; Lumen Learning; Angela Lukowski; Helen Milojevich; and Diana Lang
- Compare advantages and disadvantages of developmental research designs (cross-sectional, longitudinal, and sequential)
- Describe challenges associated with conducting research in lifespan development
Now you know about some tools used to conduct research about human development. Remember, research methods are tools that are used to collect information. But it is easy to confuse research methods and research design. Research design is the strategy or blueprint for deciding how to collect and analyze information. Research design dictates which methods are used and how. Developmental research designs are techniques used particularly in lifespan development research. When we are trying to describe development and change, the research designs become especially important because we are interested in what changes and what stays the same with age. These techniques try to examine how age, cohort, gender, and social class impact development. [1]
Cross-sectional designs
The majority of developmental studies use cross-sectional designs because they are less time-consuming and less expensive than other developmental designs. Cross-sectional research designs are used to examine behavior in participants of different ages who are tested at the same point in time (Figure 1). Let’s suppose that researchers are interested in the relationship between intelligence and aging. They might have a hypothesis (an educated guess, based on theory or observations) that intelligence declines as people get older. The researchers might choose to give a certain intelligence test to individuals who are 20 years old, individuals who are 50 years old, and individuals who are 80 years old at the same time and compare the data from each age group. This research is cross-sectional in design because the researchers plan to examine the intelligence scores of individuals of different ages within the same study at the same time; they are taking a “cross-section” of people at one point in time. Let’s say that the comparisons find that the 80-year-old adults score lower on the intelligence test than the 50-year-old adults, and the 50-year-old adults score lower on the intelligence test than the 20-year-old adults. Based on these data, the researchers might conclude that individuals become less intelligent as they get older. Would that be a valid (accurate) interpretation of the results?
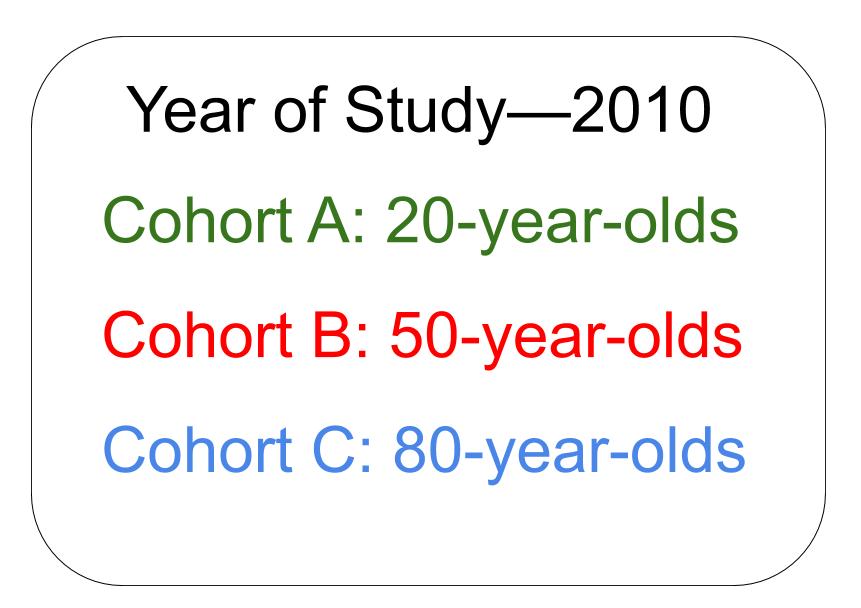
No, that would not be a valid conclusion because the researchers did not follow individuals as they aged from 20 to 50 to 80 years old. One of the primary limitations of cross-sectional research is that the results yield information about age differences not necessarily changes with age or over time. That is, although the study described above can show that in 2010, the 80-year-olds scored lower on the intelligence test than the 50-year-olds, and the 50-year-olds scored lower on the intelligence test than the 20-year-olds, the data used to come up with this conclusion were collected from different individuals (or groups of individuals). It could be, for instance, that when these 20-year-olds get older (50 and eventually 80), they will still score just as high on the intelligence test as they did at age 20. In a similar way, maybe the 80-year-olds would have scored relatively low on the intelligence test even at ages 50 and 20; the researchers don’t know for certain because they did not follow the same individuals as they got older.
It is also possible that the differences found between the age groups are not due to age, per se, but due to cohort effects. The 80-year-olds in this 2010 research grew up during a particular time and experienced certain events as a group. They were born in 1930 and are part of the Traditional or Silent Generation. The 50-year-olds were born in 1960 and are members of the Baby Boomer cohort. The 20-year-olds were born in 1990 and are part of the Millennial or Gen Y Generation. What kinds of things did each of these cohorts experience that the others did not experience or at least not in the same ways?
You may have come up with many differences between these cohorts’ experiences, such as living through certain wars, political and social movements, economic conditions, advances in technology, changes in health and nutrition standards, etc. There may be particular cohort differences that could especially influence their performance on intelligence tests, such as education level and use of computers. That is, many of those born in 1930 probably did not complete high school; those born in 1960 may have high school degrees, on average, but the majority did not attain college degrees; the young adults are probably current college students. And this is not even considering additional factors such as gender, race, or socioeconomic status. The young adults are used to taking tests on computers, but the members of the other two cohorts did not grow up with computers and may not be as comfortable if the intelligence test is administered on computers. These factors could have been a factor in the research results.
Another disadvantage of cross-sectional research is that it is limited to one time of measurement. Data are collected at one point in time and it’s possible that something could have happened in that year in history that affected all of the participants, although possibly each cohort may have been affected differently. Just think about the mindsets of participants in research that was conducted in the United States right after the terrorist attacks on September 11, 2001.
Longitudinal research designs
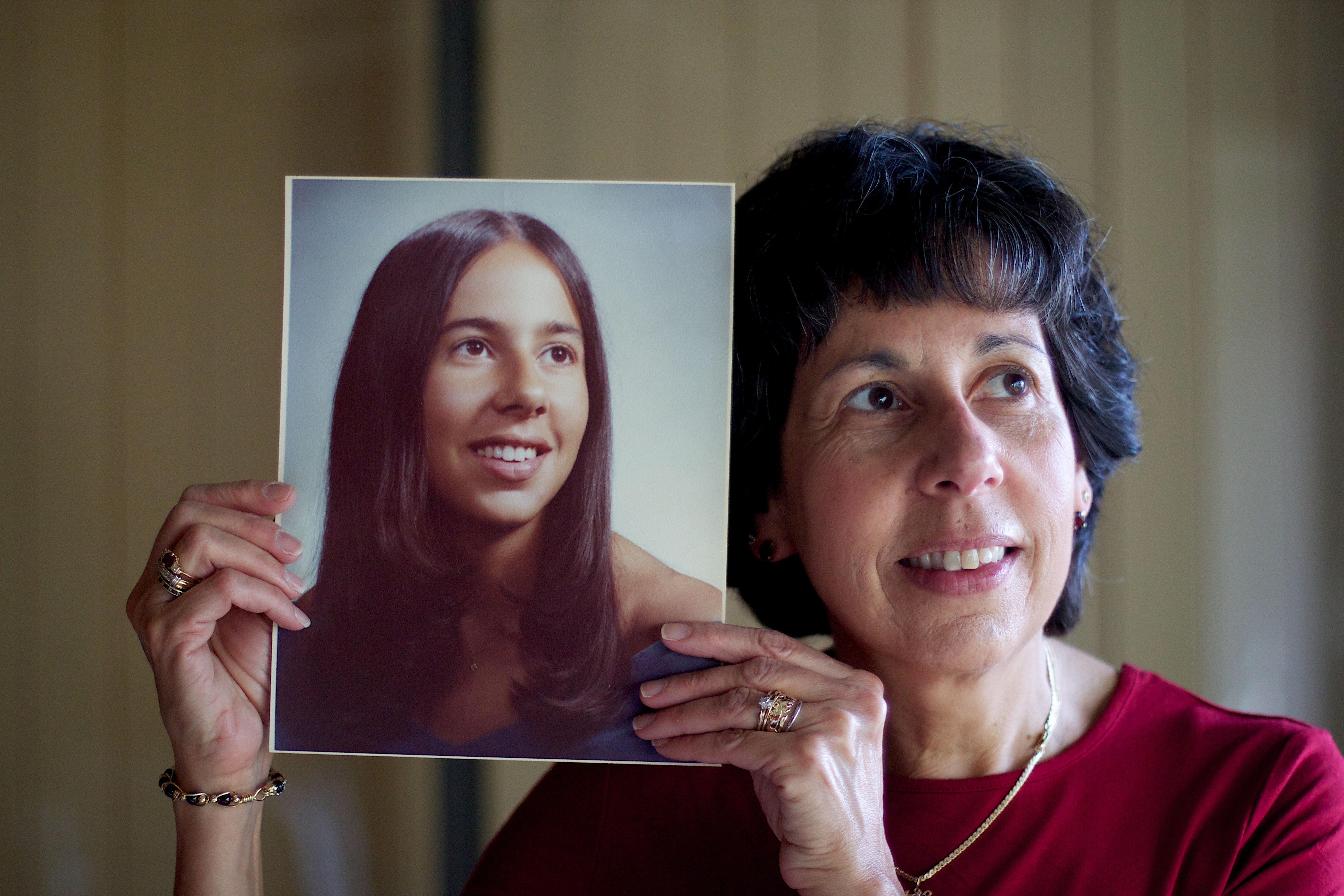
Longitudinal research involves beginning with a group of people who may be of the same age and background (cohort) and measuring them repeatedly over a long period of time (Figure 2 & 3). One of the benefits of this type of research is that people can be followed through time and be compared with themselves when they were younger; therefore changes with age over time are measured. What would be the advantages and disadvantages of longitudinal research? Problems with this type of research include being expensive, taking a long time, and subjects dropping out over time. Think about the film, 63 Up , part of the Up Series mentioned earlier, which is an example of following individuals over time. In the videos, filmed every seven years, you see how people change physically, emotionally, and socially through time; and some remain the same in certain ways, too. But many of the participants really disliked being part of the project and repeatedly threatened to quit; one disappeared for several years; another died before her 63rd year. Would you want to be interviewed every seven years? Would you want to have it made public for all to watch?
Longitudinal research designs are used to examine behavior in the same individuals over time. For instance, with our example of studying intelligence and aging, a researcher might conduct a longitudinal study to examine whether 20-year-olds become less intelligent with age over time. To this end, a researcher might give an intelligence test to individuals when they are 20 years old, again when they are 50 years old, and then again when they are 80 years old. This study is longitudinal in nature because the researcher plans to study the same individuals as they age. Based on these data, the pattern of intelligence and age might look different than from the cross-sectional research; it might be found that participants’ intelligence scores are higher at age 50 than at age 20 and then remain stable or decline a little by age 80. How can that be when cross-sectional research revealed declines in intelligence with age?

Since longitudinal research happens over a period of time (which could be short term, as in months, but is often longer, as in years), there is a risk of attrition. Attrition occurs when participants fail to complete all portions of a study. Participants may move, change their phone numbers, die, or simply become disinterested in participating over time. Researchers should account for the possibility of attrition by enrolling a larger sample into their study initially, as some participants will likely drop out over time. There is also something known as selective attrition— this means that certain groups of individuals may tend to drop out. It is often the least healthy, least educated, and lower socioeconomic participants who tend to drop out over time. That means that the remaining participants may no longer be representative of the whole population, as they are, in general, healthier, better educated, and have more money. This could be a factor in why our hypothetical research found a more optimistic picture of intelligence and aging as the years went by. What can researchers do about selective attrition? At each time of testing, they could randomly recruit more participants from the same cohort as the original members, to replace those who have dropped out.
The results from longitudinal studies may also be impacted by repeated assessments. Consider how well you would do on a math test if you were given the exact same exam every day for a week. Your performance would likely improve over time, not necessarily because you developed better math abilities, but because you were continuously practicing the same math problems. This phenomenon is known as a practice effect. Practice effects occur when participants become better at a task over time because they have done it again and again (not due to natural psychological development). So our participants may have become familiar with the intelligence test each time (and with the computerized testing administration).
Another limitation of longitudinal research is that the data are limited to only one cohort. As an example, think about how comfortable the participants in the 2010 cohort of 20-year-olds are with computers. Since only one cohort is being studied, there is no way to know if findings would be different from other cohorts. In addition, changes that are found as individuals age over time could be due to age or to time of measurement effects. That is, the participants are tested at different periods in history, so the variables of age and time of measurement could be confounded (mixed up). For example, what if there is a major shift in workplace training and education between 2020 and 2040 and many of the participants experience a lot more formal education in adulthood, which positively impacts their intelligence scores in 2040? Researchers wouldn’t know if the intelligence scores increased due to growing older or due to a more educated workforce over time between measurements.
Sequential research designs
Sequential research designs include elements of both longitudinal and cross-sectional research designs. Similar to longitudinal designs, sequential research features participants who are followed over time; similar to cross-sectional designs, sequential research includes participants of different ages. This research design is also distinct from those that have been discussed previously in that individuals of different ages are enrolled into a study at various points in time to examine age-related changes, development within the same individuals as they age, and to account for the possibility of cohort and/or time of measurement effects. In 1965, Schaie [2] (a leading theorist and researcher on intelligence and aging), described particular sequential designs: cross-sequential, cohort sequential, and time-sequential. The differences between them depended on which variables were focused on for analyses of the data (data could be viewed in terms of multiple cross-sectional designs or multiple longitudinal designs or multiple cohort designs). Ideally, by comparing results from the different types of analyses, the effects of age, cohort, and time in history could be separated out.
Consider, once again, our example of intelligence and aging. In a study with a sequential design, a researcher might recruit three separate groups of participants (Groups A, B, and C). Group A would be recruited when they are 20 years old in 2010 and would be tested again when they are 50 and 80 years old in 2040 and 2070, respectively (similar in design to the longitudinal study described previously). Group B would be recruited when they are 20 years old in 2040 and would be tested again when they are 50 years old in 2070. Group C would be recruited when they are 20 years old in 2070 and so on (Figure 4).
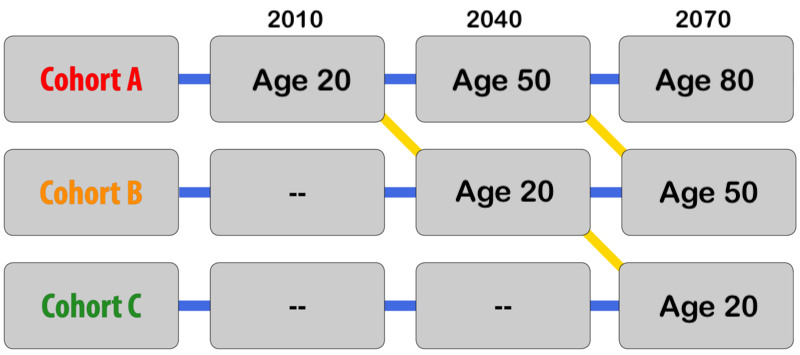
Studies with sequential designs are powerful because they allow for both longitudinal and cross-sectional comparisons—changes and/or stability with age over time can be measured and compared with differences between age and cohort groups. This research design also allows for the examination of cohort and time of measurement effects. For example, the researcher could examine the intelligence scores of 20-year-olds in different times in history and different cohorts (follow the yellow diagonal lines in figure 3). This might be examined by researchers who are interested in sociocultural and historical changes (because we know that lifespan development is multidisciplinary). One way of looking at the usefulness of the various developmental research designs was described by Schaie and Baltes [3] : cross-sectional and longitudinal designs might reveal change patterns while sequential designs might identify developmental origins for the observed change patterns.
Since they include elements of longitudinal and cross-sectional designs, sequential research has many of the same strengths and limitations as these other approaches. For example, sequential work may require less time and effort than longitudinal research (if data are collected more frequently than over the 30-year spans in our example) but more time and effort than cross-sectional research. Although practice effects may be an issue if participants are asked to complete the same tasks or assessments over time, attrition may be less problematic than what is commonly experienced in longitudinal research since participants may not have to remain involved in the study for such a long period of time.
When considering the best research design to use in their research, scientists think about their main research question and the best way to come up with an answer. A table of advantages and disadvantages for each of the described research designs is provided here to help you as you consider what sorts of studies would be best conducted using each of these different approaches.
Challenges Associated with Conducting Developmental Research
The previous sections describe research tools to assess development across the lifespan, as well as the ways that research designs can be used to track age-related changes and development over time. Before you begin conducting developmental research, however, you must also be aware that testing individuals of certain ages (such as infants and children) or making comparisons across ages (such as children compared to teens) comes with its own unique set of challenges. In the final section of this module, let’s look at some of the main issues that are encountered when conducting developmental research, namely ethical concerns, recruitment issues, and participant attrition.
Ethical Concerns
As a student of the social sciences, you may already know that Institutional Review Boards (IRBs) must review and approve all research projects that are conducted at universities, hospitals, and other institutions (each broad discipline or field, such as psychology or social work, often has its own code of ethics that must also be followed, regardless of institutional affiliation). An IRB is typically a panel of experts who read and evaluate proposals for research. IRB members want to ensure that the proposed research will be carried out ethically and that the potential benefits of the research outweigh the risks and potential harm (psychological as well as physical harm) for participants.
What you may not know though, is that the IRB considers some groups of participants to be more vulnerable or at-risk than others. Whereas university students are generally not viewed as vulnerable or at-risk, infants and young children commonly fall into this category. What makes infants and young children more vulnerable during research than young adults? One reason infants and young children are perceived as being at increased risk is due to their limited cognitive capabilities, which makes them unable to state their willingness to participate in research or tell researchers when they would like to drop out of a study. For these reasons, infants and young children require special accommodations as they participate in the research process. Similar issues and accommodations would apply to adults who are deemed to be of limited cognitive capabilities.
When thinking about special accommodations in developmental research, consider the informed consent process. If you have ever participated in scientific research, you may know through your own experience that adults commonly sign an informed consent statement (a contract stating that they agree to participate in research) after learning about a study. As part of this process, participants are informed of the procedures to be used in the research, along with any expected risks or benefits. Infants and young children cannot verbally indicate their willingness to participate, much less understand the balance of potential risks and benefits. As such, researchers are oftentimes required to obtain written informed consent from the parent or legal guardian of the child participant, an adult who is almost always present as the study is conducted. In fact, children are not asked to indicate whether they would like to be involved in a study at all (a process known as assent) until they are approximately seven years old. Because infants and young children cannot easily indicate if they would like to discontinue their participation in a study, researchers must be sensitive to changes in the state of the participant (determining whether a child is too tired or upset to continue) as well as to parent desires (in some cases, parents might want to discontinue their involvement in the research). As in adult studies, researchers must always strive to protect the rights and well-being of the minor participants and their parents when conducting developmental research.
This video from the US Department of Health and Human Services provides an overview of the Institutional Review Board process.
You can view the transcript for “How IRBs Protect Human Research Participants” here (opens in new window) .
Recruitment
An additional challenge in developmental science is participant recruitment. Recruiting university students to participate in adult studies is typically easy. Many colleges and universities offer extra credit for participation in research and have locations such as bulletin boards and school newspapers where research can be advertised. Unfortunately, young children cannot be recruited by making announcements in Introduction to Psychology courses, by posting ads on campuses, or through online platforms such as Amazon Mechanical Turk. Given these limitations, how do researchers go about finding infants and young children to be in their studies?
The answer to this question varies along multiple dimensions. Researchers must consider the number of participants they need and the financial resources available to them, among other things. Location may also be an important consideration. Researchers who need large numbers of infants and children may attempt to recruit them by obtaining infant birth records from the state, county, or province in which they reside. Some areas make this information publicly available for free, whereas birth records must be purchased in other areas (and in some locations birth records may be entirely unavailable as a recruitment tool). If birth records are available, researchers can use the obtained information to call families by phone or mail them letters describing possible research opportunities. All is not lost if this recruitment strategy is unavailable, however. Researchers can choose to pay a recruitment agency to contact and recruit families for them. Although these methods tend to be quick and effective, they can also be quite expensive. More economical recruitment options include posting advertisements and fliers in locations frequented by families, such as mommy-and-me classes, local malls, and preschools or daycare centers. Researchers can also utilize online social media outlets like Facebook, which allows users to post recruitment advertisements for a small fee. Of course, each of these different recruitment techniques requires IRB approval. And if children are recruited and/or tested in school settings, permission would need to be obtained ahead of time from teachers, schools, and school districts (as well as informed consent from parents or guardians).
And what about the recruitment of adults? While it is easy to recruit young college students to participate in research, some would argue that it is too easy and that college students are samples of convenience. They are not randomly selected from the wider population, and they may not represent all young adults in our society (this was particularly true in the past with certain cohorts, as college students tended to be mainly white males of high socioeconomic status). In fact, in the early research on aging, this type of convenience sample was compared with another type of convenience sample—young college students tended to be compared with residents of nursing homes! Fortunately, it didn’t take long for researchers to realize that older adults in nursing homes are not representative of the older population; they tend to be the oldest and sickest (physically and/or psychologically). Those initial studies probably painted an overly negative view of aging, as young adults in college were being compared to older adults who were not healthy, had not been in school nor taken tests in many decades, and probably did not graduate high school, let alone college. As we can see, recruitment and random sampling can be significant issues in research with adults, as well as infants and children. For instance, how and where would you recruit middle-aged adults to participate in your research?
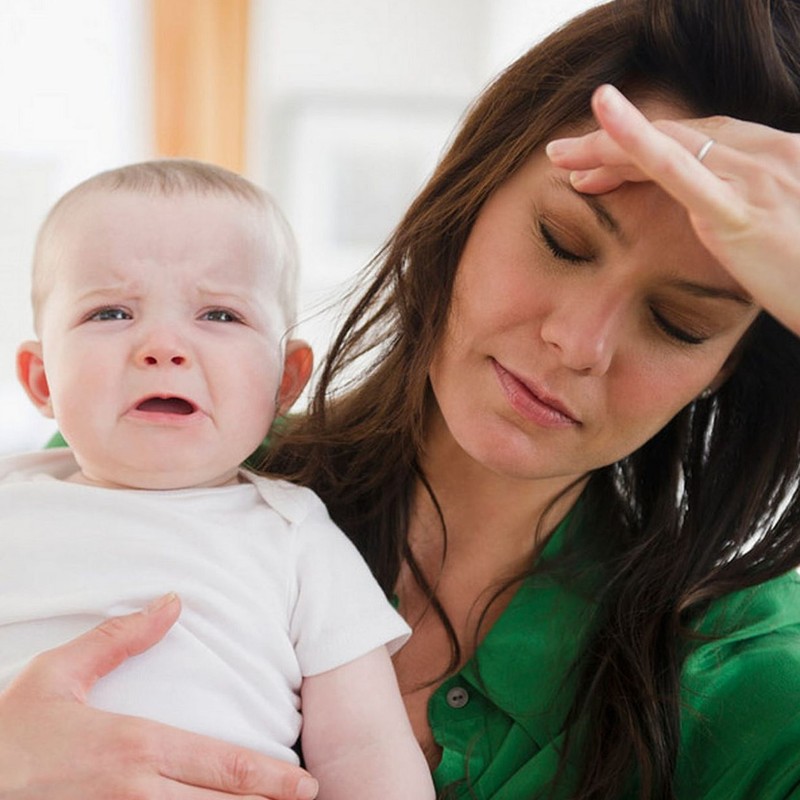
Another important consideration when conducting research with infants and young children is attrition . Although attrition is quite common in longitudinal research in particular (see the previous section on longitudinal designs for an example of high attrition rates and selective attrition in lifespan developmental research), it is also problematic in developmental science more generally, as studies with infants and young children tend to have higher attrition rates than studies with adults. For example, high attrition rates in ERP (event-related potential, which is a technique to understand brain function) studies oftentimes result from the demands of the task: infants are required to sit still and have a tight, wet cap placed on their heads before watching still photographs on a computer screen in a dark, quiet room (Figure 5).
In other cases, attrition may be due to motivation (or a lack thereof). Whereas adults may be motivated to participate in research in order to receive money or extra course credit, infants and young children are not as easily enticed. In addition, infants and young children are more likely to tire easily, become fussy, and lose interest in the study procedures than are adults. For these reasons, research studies should be designed to be as short as possible – it is likely better to break up a large study into multiple short sessions rather than cram all of the tasks into one long visit to the lab. Researchers should also allow time for breaks in their study protocols so that infants can rest or have snacks as needed. Happy, comfortable participants provide the best data.
Conclusions
Lifespan development is a fascinating field of study – but care must be taken to ensure that researchers use appropriate methods to examine human behavior, use the correct experimental design to answer their questions, and be aware of the special challenges that are part-and-parcel of developmental research. After reading this module, you should have a solid understanding of these various issues and be ready to think more critically about research questions that interest you. For example, what types of questions do you have about lifespan development? What types of research would you like to conduct? Many interesting questions remain to be examined by future generations of developmental scientists – maybe you will make one of the next big discoveries!
- attrition : occurs when participants fail to complete all portions of a study
- cross-sectional research : used to examine behavior in participants of different ages who are tested at the same point in time; may confound age and cohort differences
- i nformed consent : a process of informing a research participant what to expect during a study, any risks involved, and the implications of the research, and then obtaining the person’s agreement to participate
- Institutional Review Boards (IRBs) : a panel of experts who review research proposals for any research to be conducted in association with the institution (for example, a university)
- longitudinal research : studying a group of people who may be of the same age and background (cohort), and measuring them repeatedly over a long period of time; may confound age and time of measurement effects
- research design : the strategy or blueprint for deciding how to collect and analyze information; dictates which methods are used and how
- selective attrition : certain groups of individuals may tend to drop out more frequently resulting in the remaining participants no longer being representative of the whole population
- sequential research design : combines aspects of cross-sectional and longitudinal designs, but also adding new cohorts at different times of measurement; allows for analyses to consider effects of age, cohort, time of measurement, and socio-historical change
- This chapter was adapted from Lumen Learning's Lifespan Development , created by Margaret Clark-Plaskie for Lumen Learning and adapted from Research Methods in Developmental Psychology by Angela Lukowski and Helen Milojevich for Noba Psychology, available under a Creative Commons NonCommercial Sharealike Attribution license . ↵
- Schaie, K. W. (1965). A general model for the study of developmental problems. Psychological Bulletin, 64 (2), 92-107. ↵
- Schaie, K.W. & Baltes, B.P. (1975). On sequential strategies in developmental research: Description or Explanation. Human Development, 18, 384-390. ↵
Developmental Research Designs Copyright © 2022 by Margaret Clark-Plaskie; Lumen Learning; Angela Lukowski; Helen Milojevich; and Diana Lang is licensed under a Creative Commons Attribution-NonCommercial-ShareAlike 4.0 International License , except where otherwise noted.
Have a language expert improve your writing
Run a free plagiarism check in 10 minutes, automatically generate references for free.
- Knowledge Base
- Methodology
- Descriptive Research Design | Definition, Methods & Examples
Descriptive Research Design | Definition, Methods & Examples
Published on 5 May 2022 by Shona McCombes . Revised on 10 October 2022.
Descriptive research aims to accurately and systematically describe a population, situation or phenomenon. It can answer what , where , when , and how questions , but not why questions.
A descriptive research design can use a wide variety of research methods to investigate one or more variables . Unlike in experimental research , the researcher does not control or manipulate any of the variables, but only observes and measures them.
Table of contents
When to use a descriptive research design, descriptive research methods.
Descriptive research is an appropriate choice when the research aim is to identify characteristics, frequencies, trends, and categories.
It is useful when not much is known yet about the topic or problem. Before you can research why something happens, you need to understand how, when, and where it happens.
- How has the London housing market changed over the past 20 years?
- Do customers of company X prefer product Y or product Z?
- What are the main genetic, behavioural, and morphological differences between European wildcats and domestic cats?
- What are the most popular online news sources among under-18s?
- How prevalent is disease A in population B?
Prevent plagiarism, run a free check.
Descriptive research is usually defined as a type of quantitative research , though qualitative research can also be used for descriptive purposes. The research design should be carefully developed to ensure that the results are valid and reliable .
Survey research allows you to gather large volumes of data that can be analysed for frequencies, averages, and patterns. Common uses of surveys include:
- Describing the demographics of a country or region
- Gauging public opinion on political and social topics
- Evaluating satisfaction with a company’s products or an organisation’s services
Observations
Observations allow you to gather data on behaviours and phenomena without having to rely on the honesty and accuracy of respondents. This method is often used by psychological, social, and market researchers to understand how people act in real-life situations.
Observation of physical entities and phenomena is also an important part of research in the natural sciences. Before you can develop testable hypotheses , models, or theories, it’s necessary to observe and systematically describe the subject under investigation.
Case studies
A case study can be used to describe the characteristics of a specific subject (such as a person, group, event, or organisation). Instead of gathering a large volume of data to identify patterns across time or location, case studies gather detailed data to identify the characteristics of a narrowly defined subject.
Rather than aiming to describe generalisable facts, case studies often focus on unusual or interesting cases that challenge assumptions, add complexity, or reveal something new about a research problem .
Cite this Scribbr article
If you want to cite this source, you can copy and paste the citation or click the ‘Cite this Scribbr article’ button to automatically add the citation to our free Reference Generator.
McCombes, S. (2022, October 10). Descriptive Research Design | Definition, Methods & Examples. Scribbr. Retrieved 22 April 2024, from https://www.scribbr.co.uk/research-methods/descriptive-research-design/
Is this article helpful?
Shona McCombes
Other students also liked, a quick guide to experimental design | 5 steps & examples, correlational research | guide, design & examples, qualitative vs quantitative research | examples & methods.
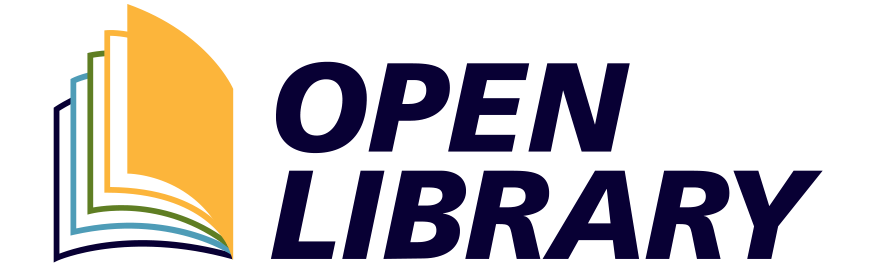
Want to create or adapt books like this? Learn more about how Pressbooks supports open publishing practices.
6 Developmental Research Designs
These designs examine what changes and what stays the same in a human life. Chronological age , cohort membership , and time of measurement are the basic elements of research designs looking at development. The frustrating thing about doing this kind of research is that you only can vary two of these three elements at a time. The two that you choose will determine the third element. Therefore no single study can definitively tell you about how human beings develop. However, combining results of multiple studies and using more complex designs, such as cross-sequential designs, can help us get closer to the truth.
Cross-sectional research involves beginning with a sample that represents a cross-section of the population. Respondents who vary in age, gender, ethnicity, and social class might be asked to complete a survey about television program preferences or attitudes toward the use of the Internet. The attitudes of males and females could then be compared as could attitudes based on age. In cross-sectional research, respondents are measured only once. This method is much less expensive than longitudinal research but does not allow the researcher to distinguish between the impact of age and the cohort effect. Different attitudes about the Internet, for example, might not be altered by a person’s biological age as much as their life experiences as members of a cohort.
Longitudinal research involves beginning with a group of people who may be of the same age and background, and measuring them repeatedly over a long period of time. One of the benefits of this type of research is that people can be followed through time and be compared with them when they were younger. A problem with this type of research is that it is very expensive and subjects may drop out over time. (The film 49 Up is a example of following individuals over time. You see how people change physically, emotionally, and socially through time.) What would be the drawbacks of being in a longitudinal study? What about 49 Up? Would you want to be filmed every 7 years? What would be the advantages and disadvantages? Can you imagine why some would continue and others drop out of the project?
Cross-sequential research involves combining aspects of the previous two techniques; beginning with a cross-sectional sample and measuring them through time. This is the perfect model for looking at age, gender, social class, and ethnicity. But here the drawbacks of high costs and attrition are here as well.
the amount of time elapsed since an individual’s birth, typically expressed in terms of months and years.
a group of individuals who share a similar characteristic or experience. The term usually refers to an age (or birth) cohort, that is, a group of individuals who are born in the same year and thus of similar age.
the moment in time when the participants' responses are recorded.
a research design in which individuals, typically of different ages or developmental levels, are compared at a single point in time. An example is a study that involves a direct comparison of 50-year-olds with 80-year-olds. Given its snapshot nature, however, it is difficult to determine causal relationships using a cross-sectional design. Moreover, a cross-sectional study is not suitable for measuring changes over time, for which a longitudinal design is required.
the study of a variable or group of variables in the same cases or participants over a period of time, sometimes several years. An example of a longitudinal design is a multiyear comparative study of the same children in an urban and a suburban school to record their cognitive development in depth. A longitudinal study that evaluates a group of randomly chosen individuals is referred to as a panel study, whereas a longitudinal study that evaluates a group of individuals possessing some common characteristic (usually age) is referred to as a cohort study.
a study in which two or more groups of individuals of different ages are directly compared over a period of time. It is thus a combination of a cross-sectional design and a longitudinal design. For example, an investigator using a cross-sequential design to evaluate children’s mathematical skills might measure a group of 5-year-olds and a group of 10-year-olds at the beginning of the research and then subsequently reassess the same children every 6 months for the next 5 years.
Always Developing Copyright © 2019 by Anne Baird is licensed under a Creative Commons Attribution-NonCommercial-ShareAlike 4.0 International License , except where otherwise noted.
Share This Book
- What is descriptive research?
Last updated
5 February 2023
Reviewed by
Cathy Heath
Descriptive research is a common investigatory model used by researchers in various fields, including social sciences, linguistics, and academia.
Read on to understand the characteristics of descriptive research and explore its underlying techniques, processes, and procedures.
Analyze your descriptive research
Dovetail streamlines analysis to help you uncover and share actionable insights
Descriptive research is an exploratory research method. It enables researchers to precisely and methodically describe a population, circumstance, or phenomenon.
As the name suggests, descriptive research describes the characteristics of the group, situation, or phenomenon being studied without manipulating variables or testing hypotheses . This can be reported using surveys , observational studies, and case studies. You can use both quantitative and qualitative methods to compile the data.
Besides making observations and then comparing and analyzing them, descriptive studies often develop knowledge concepts and provide solutions to critical issues. It always aims to answer how the event occurred, when it occurred, where it occurred, and what the problem or phenomenon is.
- Characteristics of descriptive research
The following are some of the characteristics of descriptive research:
Quantitativeness
Descriptive research can be quantitative as it gathers quantifiable data to statistically analyze a population sample. These numbers can show patterns, connections, and trends over time and can be discovered using surveys, polls, and experiments.
Qualitativeness
Descriptive research can also be qualitative. It gives meaning and context to the numbers supplied by quantitative descriptive research .
Researchers can use tools like interviews, focus groups, and ethnographic studies to illustrate why things are what they are and help characterize the research problem. This is because it’s more explanatory than exploratory or experimental research.
Uncontrolled variables
Descriptive research differs from experimental research in that researchers cannot manipulate the variables. They are recognized, scrutinized, and quantified instead. This is one of its most prominent features.
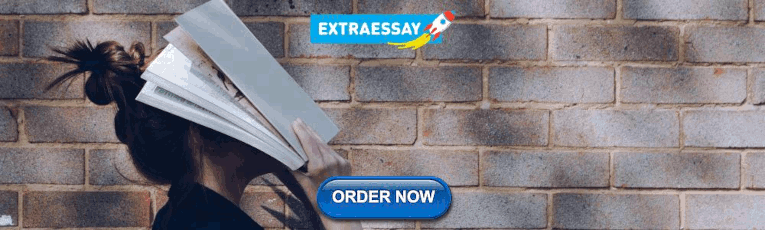
Cross-sectional studies
Descriptive research is a cross-sectional study because it examines several areas of the same group. It involves obtaining data on multiple variables at the personal level during a certain period. It’s helpful when trying to understand a larger community’s habits or preferences.
Carried out in a natural environment
Descriptive studies are usually carried out in the participants’ everyday environment, which allows researchers to avoid influencing responders by collecting data in a natural setting. You can use online surveys or survey questions to collect data or observe.
Basis for further research
You can further dissect descriptive research’s outcomes and use them for different types of investigation. The outcomes also serve as a foundation for subsequent investigations and can guide future studies. For example, you can use the data obtained in descriptive research to help determine future research designs.
- Descriptive research methods
There are three basic approaches for gathering data in descriptive research: observational, case study, and survey.
You can use surveys to gather data in descriptive research. This involves gathering information from many people using a questionnaire and interview .
Surveys remain the dominant research tool for descriptive research design. Researchers can conduct various investigations and collect multiple types of data (quantitative and qualitative) using surveys with diverse designs.
You can conduct surveys over the phone, online, or in person. Your survey might be a brief interview or conversation with a set of prepared questions intended to obtain quick information from the primary source.
Observation
This descriptive research method involves observing and gathering data on a population or phenomena without manipulating variables. It is employed in psychology, market research , and other social science studies to track and understand human behavior.
Observation is an essential component of descriptive research. It entails gathering data and analyzing it to see whether there is a relationship between the two variables in the study. This strategy usually allows for both qualitative and quantitative data analysis.
Case studies
A case study can outline a specific topic’s traits. The topic might be a person, group, event, or organization.
It involves using a subset of a larger group as a sample to characterize the features of that larger group.
You can generalize knowledge gained from studying a case study to benefit a broader audience.
This approach entails carefully examining a particular group, person, or event over time. You can learn something new about the study topic by using a small group to better understand the dynamics of the entire group.
- Types of descriptive research
There are several types of descriptive study. The most well-known include cross-sectional studies, census surveys, sample surveys, case reports, and comparison studies.
Case reports and case series
In the healthcare and medical fields, a case report is used to explain a patient’s circumstances when suffering from an uncommon illness or displaying certain symptoms. Case reports and case series are both collections of related cases. They have aided the advancement of medical knowledge on countless occasions.
The normative component is an addition to the descriptive survey. In the descriptive–normative survey, you compare the study’s results to the norm.
Descriptive survey
This descriptive type of research employs surveys to collect information on various topics. This data aims to determine the degree to which certain conditions may be attained.
You can extrapolate or generalize the information you obtain from sample surveys to the larger group being researched.
Correlative survey
Correlative surveys help establish if there is a positive, negative, or neutral connection between two variables.
Performing census surveys involves gathering relevant data on several aspects of a given population. These units include individuals, families, organizations, objects, characteristics, and properties.
During descriptive research, you gather different degrees of interest over time from a specific population. Cross-sectional studies provide a glimpse of a phenomenon’s prevalence and features in a population. There are no ethical challenges with them and they are quite simple and inexpensive to carry out.
Comparative studies
These surveys compare the two subjects’ conditions or characteristics. The subjects may include research variables, organizations, plans, and people.
Comparison points, assumption of similarities, and criteria of comparison are three important variables that affect how well and accurately comparative studies are conducted.
For instance, descriptive research can help determine how many CEOs hold a bachelor’s degree and what proportion of low-income households receive government help.
- Pros and cons
The primary advantage of descriptive research designs is that researchers can create a reliable and beneficial database for additional study. To conduct any inquiry, you need access to reliable information sources that can give you a firm understanding of a situation.
Quantitative studies are time- and resource-intensive, so knowing the hypotheses viable for testing is crucial. The basic overview of descriptive research provides helpful hints as to which variables are worth quantitatively examining. This is why it’s employed as a precursor to quantitative research designs.
Some experts view this research as untrustworthy and unscientific. However, there is no way to assess the findings because you don’t manipulate any variables statistically.
Cause-and-effect correlations also can’t be established through descriptive investigations. Additionally, observational study findings cannot be replicated, which prevents a review of the findings and their replication.
The absence of statistical and in-depth analysis and the rather superficial character of the investigative procedure are drawbacks of this research approach.
- Descriptive research examples and applications
Several descriptive research examples are emphasized based on their types, purposes, and applications. Research questions often begin with “What is …” These studies help find solutions to practical issues in social science, physical science, and education.
Here are some examples and applications of descriptive research:
Determining consumer perception and behavior
Organizations use descriptive research designs to determine how various demographic groups react to a certain product or service.
For example, a business looking to sell to its target market should research the market’s behavior first. When researching human behavior in response to a cause or event, the researcher pays attention to the traits, actions, and responses before drawing a conclusion.
Scientific classification
Scientific descriptive research enables the classification of organisms and their traits and constituents.
Measuring data trends
A descriptive study design’s statistical capabilities allow researchers to track data trends over time. It’s frequently used to determine the study target’s current circumstances and underlying patterns.
Conduct comparison
Organizations can use a descriptive research approach to learn how various demographics react to a certain product or service. For example, you can study how the target market responds to a competitor’s product and use that information to infer their behavior.
- Bottom line
A descriptive research design is suitable for exploring certain topics and serving as a prelude to larger quantitative investigations. It provides a comprehensive understanding of the “what” of the group or thing you’re investigating.
This research type acts as the cornerstone of other research methodologies . It is distinctive because it can use quantitative and qualitative research approaches at the same time.
What is descriptive research design?
Descriptive research design aims to systematically obtain information to describe a phenomenon, situation, or population. More specifically, it helps answer the what, when, where, and how questions regarding the research problem rather than the why.
How does descriptive research compare to qualitative research?
Despite certain parallels, descriptive research concentrates on describing phenomena, while qualitative research aims to understand people better.
How do you analyze descriptive research data?
Data analysis involves using various methodologies, enabling the researcher to evaluate and provide results regarding validity and reliability.
Get started today
Go from raw data to valuable insights with a flexible research platform
Editor’s picks
Last updated: 21 December 2023
Last updated: 16 December 2023
Last updated: 6 October 2023
Last updated: 25 November 2023
Last updated: 12 May 2023
Last updated: 15 February 2024
Last updated: 11 March 2024
Last updated: 12 December 2023
Last updated: 18 May 2023
Last updated: 6 March 2024
Last updated: 10 April 2023
Last updated: 20 December 2023
Latest articles
Related topics, log in or sign up.
Get started for free
Module 1: Lifespan Development
Developmental research designs, learning outcomes.
- Compare advantages and disadvantages of developmental research designs (cross-sectional, longitudinal, and sequential)
Now you know about some tools used to conduct research about human development. Remember, research methods are tools that are used to collect information. But it is easy to confuse research methods and research design. Research design is the strategy or blueprint for deciding how to collect and analyze information. Research design dictates which methods are used and how. Developmental research designs are techniques used particularly in lifespan development research. When we are trying to describe development and change, the research designs become especially important because we are interested in what changes and what stays the same with age. These techniques try to examine how age, cohort, gender, and social class impact development.
Cross-sectional designs
The majority of developmental studies use cross-sectional designs because they are less time-consuming and less expensive than other developmental designs. Cross-sectional research designs are used to examine behavior in participants of different ages who are tested at the same point in time. Let’s suppose that researchers are interested in the relationship between intelligence and aging. They might have a hypothesis (an educated guess, based on theory or observations) that intelligence declines as people get older. The researchers might choose to give a certain intelligence test to individuals who are 20 years old, individuals who are 50 years old, and individuals who are 80 years old at the same time and compare the data from each age group. This research is cross-sectional in design because the researchers plan to examine the intelligence scores of individuals of different ages within the same study at the same time; they are taking a “cross-section” of people at one point in time. Let’s say that the comparisons find that the 80-year-old adults score lower on the intelligence test than the 50-year-old adults, and the 50-year-old adults score lower on the intelligence test than the 20-year-old adults. Based on these data, the researchers might conclude that individuals become less intelligent as they get older. Would that be a valid (accurate) interpretation of the results?
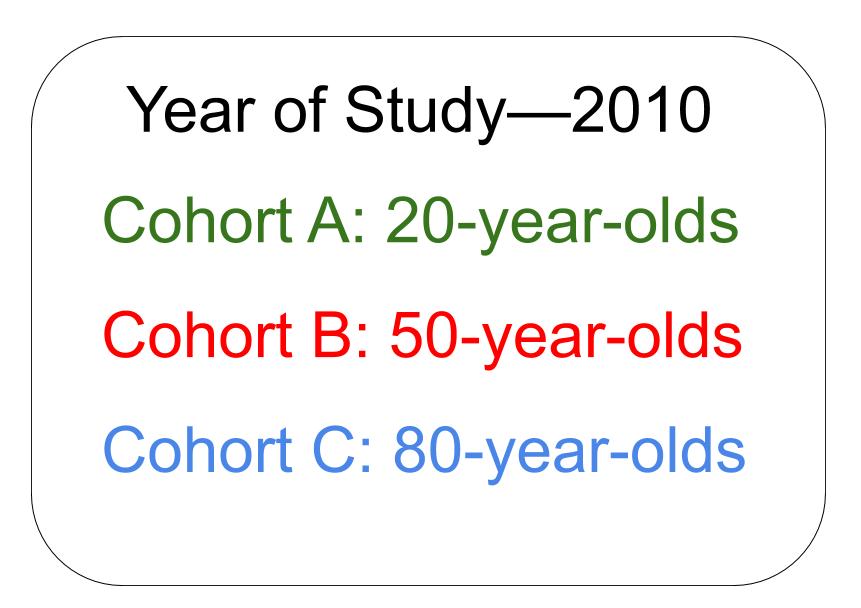
Figure 1 . Example of cross-sectional research design
No, that would not be a valid conclusion because the researchers did not follow individuals as they aged from 20 to 50 to 80 years old. One of the primary limitations of cross-sectional research is that the results yield information about age differences not necessarily changes with age or over time. That is, although the study described above can show that in 2010, the 80-year-olds scored lower on the intelligence test than the 50-year-olds, and the 50-year-olds scored lower on the intelligence test than the 20-year-olds, the data used to come up with this conclusion were collected from different individuals (or groups of individuals). It could be, for instance, that when these 20-year-olds get older (50 and eventually 80), they will still score just as high on the intelligence test as they did at age 20. In a similar way, maybe the 80-year-olds would have scored relatively low on the intelligence test even at ages 50 and 20; the researchers don’t know for certain because they did not follow the same individuals as they got older.
It is also possible that the differences found between the age groups are not due to age, per se, but due to cohort effects. The 80-year-olds in this 2010 research grew up during a particular time and experienced certain events as a group. They were born in 1930 and are part of the Traditional or Silent Generation. The 50-year-olds were born in 1960 and are members of the Baby Boomer cohort. The 20-year-olds were born in 1990 and are part of the Millennial or Gen Y Generation. What kinds of things did each of these cohorts experience that the others did not experience or at least not in the same ways?
You may have come up with many differences between these cohorts’ experiences, such as living through certain wars, political and social movements, economic conditions, advances in technology, changes in health and nutrition standards, etc. There may be particular cohort differences that could especially influence their performance on intelligence tests, such as education level and use of computers. That is, many of those born in 1930 probably did not complete high school; those born in 1960 may have high school degrees, on average, but the majority did not attain college degrees; the young adults are probably current college students. And this is not even considering additional factors such as gender, race, or socioeconomic status. The young adults are used to taking tests on computers, but the members of the other two cohorts did not grow up with computers and may not be as comfortable if the intelligence test is administered on computers. These factors could have been a factor in the research results.
Another disadvantage of cross-sectional research is that it is limited to one time of measurement. Data are collected at one point in time and it’s possible that something could have happened in that year in history that affected all of the participants, although possibly each cohort may have been affected differently. Just think about the mindsets of participants in research that was conducted in the United States right after the terrorist attacks on September 11, 2001.
Longitudinal research designs
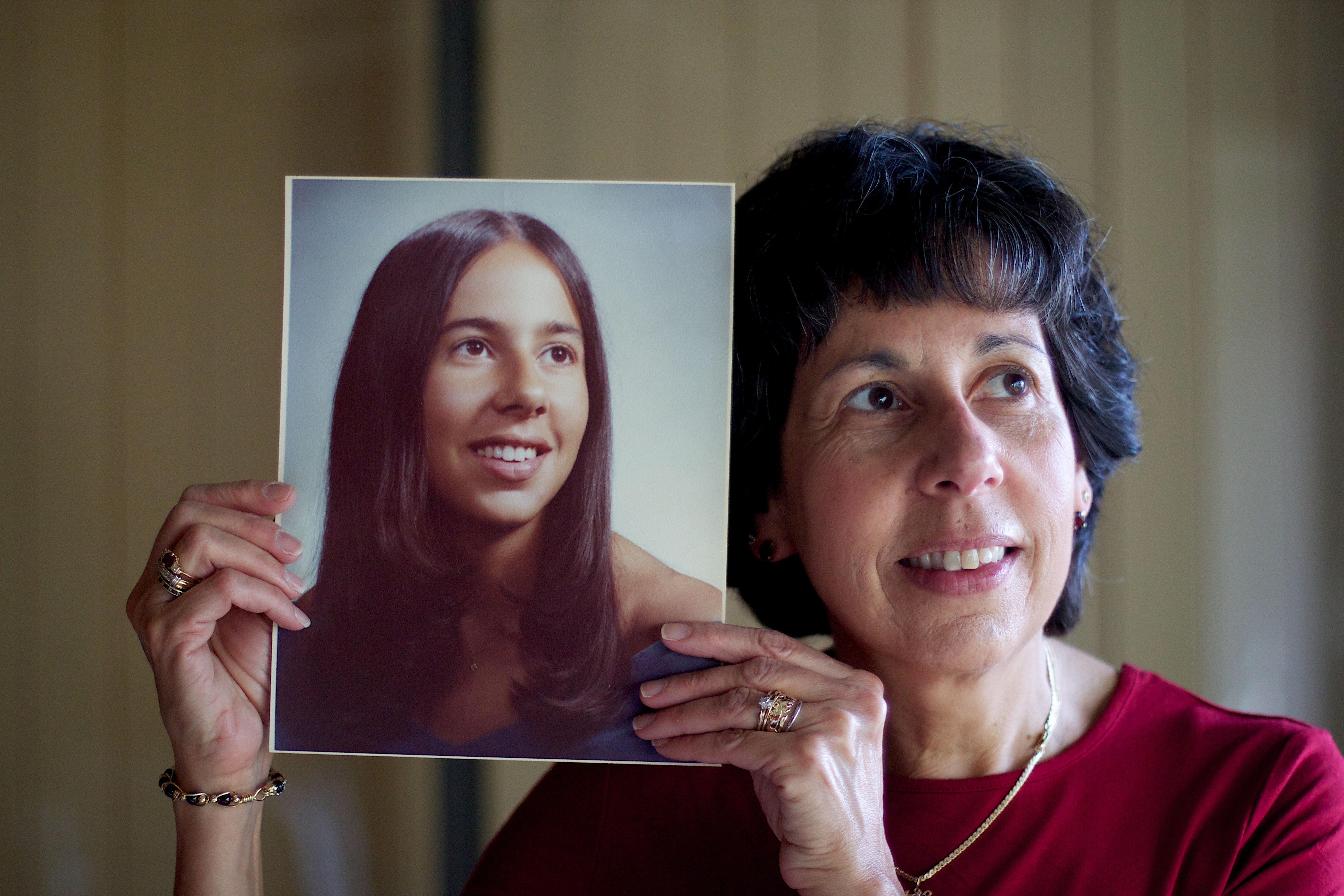
Figure 2 . Longitudinal research studies the same person or group of people over an extended period of time.
Longitudinal research involves beginning with a group of people who may be of the same age and background (cohort) and measuring them repeatedly over a long period of time. One of the benefits of this type of research is that people can be followed through time and be compared with themselves when they were younger; therefore changes with age over time are measured. What would be the advantages and disadvantages of longitudinal research? Problems with this type of research include being expensive, taking a long time, and participants dropping out over time. Think about the film, 63 Up , part of the Up Series mentioned earlier, which is an example of following individuals over time. In the videos, filmed every seven years, you see how people change physically, emotionally, and socially through time; and some remain the same in certain ways, too. But many of the participants really disliked being part of the project and repeatedly threatened to quit; one disappeared for several years; another died before her 63rd year. Would you want to be interviewed every seven years? Would you want to have it made public for all to watch?
Longitudinal research designs are used to examine behavior in the same individuals over time. For instance, with our example of studying intelligence and aging, a researcher might conduct a longitudinal study to examine whether 20-year-olds become less intelligent with age over time. To this end, a researcher might give an intelligence test to individuals when they are 20 years old, again when they are 50 years old, and then again when they are 80 years old. This study is longitudinal in nature because the researcher plans to study the same individuals as they age. Based on these data, the pattern of intelligence and age might look different than from the cross-sectional research; it might be found that participants’ intelligence scores are higher at age 50 than at age 20 and then remain stable or decline a little by age 80. How can that be when cross-sectional research revealed declines in intelligence with age?

Figure 3 . Example of a longitudinal research design
Since longitudinal research happens over a period of time (which could be short term, as in months, but is often longer, as in years), there is a risk of attrition. Attrition occurs when participants fail to complete all portions of a study. Participants may move, change their phone numbers, die, or simply become disinterested in participating over time. Researchers should account for the possibility of attrition by enrolling a larger sample into their study initially, as some participants will likely drop out over time. There is also something known as selective attrition— this means that certain groups of individuals may tend to drop out. It is often the least healthy, least educated, and lower socioeconomic participants who tend to drop out over time. That means that the remaining participants may no longer be representative of the whole population, as they are, in general, healthier, better educated, and have more money. This could be a factor in why our hypothetical research found a more optimistic picture of intelligence and aging as the years went by. What can researchers do about selective attrition? At each time of testing, they could randomly recruit more participants from the same cohort as the original members, to replace those who have dropped out.
The results from longitudinal studies may also be impacted by repeated assessments. Consider how well you would do on a math test if you were given the exact same exam every day for a week. Your performance would likely improve over time, not necessarily because you developed better math abilities, but because you were continuously practicing the same math problems. This phenomenon is known as a practice effect. Practice effects occur when participants become better at a task over time because they have done it again and again (not due to natural psychological development). So our participants may have become familiar with the intelligence test each time (and with the computerized testing administration).
Another limitation of longitudinal research is that the data are limited to only one cohort. As an example, think about how comfortable the participants in the 2010 cohort of 20-year-olds are with computers. Since only one cohort is being studied, there is no way to know if findings would be different from other cohorts. In addition, changes that are found as individuals age over time could be due to age or to time of measurement effects. That is, the participants are tested at different periods in history, so the variables of age and time of measurement could be confounded (mixed up). For example, what if there is a major shift in workplace training and education between 2020 and 2040 and many of the participants experience a lot more formal education in adulthood, which positively impacts their intelligence scores in 2040? Researchers wouldn’t know if the intelligence scores increased due to growing older or due to a more educated workforce over time between measurements.
Sequential research designs
Sequential research designs include elements of both longitudinal and cross-sectional research designs. Similar to longitudinal designs, sequential research features participants who are followed over time; similar to cross-sectional designs, sequential research includes participants of different ages. This research design is also distinct from those that have been discussed previously in that individuals of different ages are enrolled into a study at various points in time to examine age-related changes, development within the same individuals as they age, and to account for the possibility of cohort and/or time of measurement effects. In 1965, K. Warner Schaie [1] (a leading theorist and researcher on intelligence and aging), described particular sequential designs: cross-sequential, cohort sequential, and time-sequential. The differences between them depended on which variables were focused on for analyses of the data (data could be viewed in terms of multiple cross-sectional designs or multiple longitudinal designs or multiple cohort designs). Ideally, by comparing results from the different types of analyses, the effects of age, cohort, and time in history could be separated out.
Consider, once again, our example of intelligence and aging. In a study with a sequential design, a researcher might recruit three separate groups of participants (Groups A, B, and C). Group A would be recruited when they are 20 years old in 2010 and would be tested again when they are 50 and 80 years old in 2040 and 2070, respectively (similar in design to the longitudinal study described previously). Group B would be recruited when they are 20 years old in 2040 and would be tested again when they are 50 years old in 2070. Group C would be recruited when they are 20 years old in 2070 and so on.
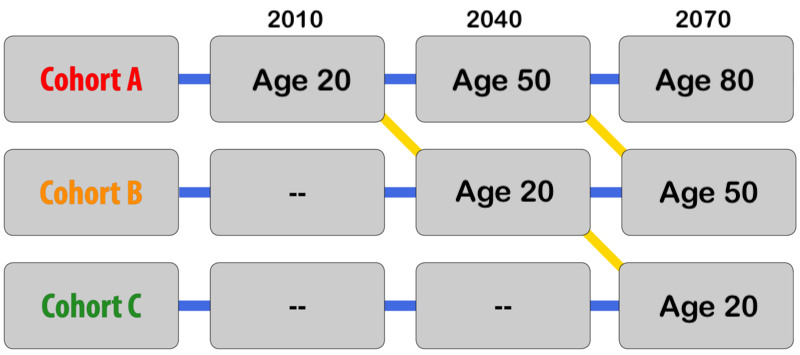
Figure 4. Example of sequential research design
Studies with sequential designs are powerful because they allow for both longitudinal and cross-sectional comparisons—changes and/or stability with age over time can be measured and compared with differences between age and cohort groups. This research design also allows for the examination of cohort and time of measurement effects. For example, the researcher could examine the intelligence scores of 20-year-olds in different times in history and different cohorts (follow the yellow diagonal lines in figure 3). This might be examined by researchers who are interested in sociocultural and historical changes (because we know that lifespan development is multidisciplinary). One way of looking at the usefulness of the various developmental research designs was described by Schaie and Baltes (1975) [2] : cross-sectional and longitudinal designs might reveal change patterns while sequential designs might identify developmental origins for the observed change patterns.
Since they include elements of longitudinal and cross-sectional designs, sequential research has many of the same strengths and limitations as these other approaches. For example, sequential work may require less time and effort than longitudinal research (if data are collected more frequently than over the 30-year spans in our example) but more time and effort than cross-sectional research. Although practice effects may be an issue if participants are asked to complete the same tasks or assessments over time, attrition may be less problematic than what is commonly experienced in longitudinal research since participants may not have to remain involved in the study for such a long period of time.
When considering the best research design to use in their research, scientists think about their main research question and the best way to come up with an answer. A table of advantages and disadvantages for each of the described research designs is provided here to help you as you consider what sorts of studies would be best conducted using each of these different approaches.
Contribute!
Improve this page Learn More
- Schaie, K.W. (1965). A general model for the study of developmental problems. Psychological Bulletin, 64(2), 92-107. ↵
- Schaie, K.W. & Baltes, B.P. (1975). On sequential strategies in developmental research: Description or Explanation. Human Development, 18: 384-390. ↵
- Modification, adaptation, and original content. Authored by : Margaret Clark-Plaskie for Lumen Learning. Provided by : Lumen Learning. License : CC BY-NC-SA: Attribution-NonCommercial-ShareAlike
- Research Methods in Developmental Psychology. Authored by : Angela Lukowski and Helen Milojevich. Provided by : University of Calfornia, Irvine. Located at : https://nobaproject.com/modules/research-methods-in-developmental-psychology?r=LDcyNTg0 . Project : The Noba Project. License : CC BY-NC-SA: Attribution-NonCommercial-ShareAlike
- Woman holding own photograph. Provided by : Pxhere. Located at : https://pxhere.com/en/photo/221167 . License : CC0: No Rights Reserved

Bridging the Gap: Overcome these 7 flaws in descriptive research design
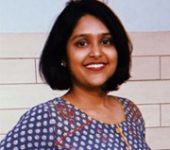
Descriptive research design is a powerful tool used by scientists and researchers to gather information about a particular group or phenomenon. This type of research provides a detailed and accurate picture of the characteristics and behaviors of a particular population or subject. By observing and collecting data on a given topic, descriptive research helps researchers gain a deeper understanding of a specific issue and provides valuable insights that can inform future studies.
In this blog, we will explore the definition, characteristics, and common flaws in descriptive research design, and provide tips on how to avoid these pitfalls to produce high-quality results. Whether you are a seasoned researcher or a student just starting, understanding the fundamentals of descriptive research design is essential to conducting successful scientific studies.
Table of Contents
What Is Descriptive Research Design?
The descriptive research design involves observing and collecting data on a given topic without attempting to infer cause-and-effect relationships. The goal of descriptive research is to provide a comprehensive and accurate picture of the population or phenomenon being studied and to describe the relationships, patterns, and trends that exist within the data.
Descriptive research methods can include surveys, observational studies , and case studies, and the data collected can be qualitative or quantitative . The findings from descriptive research provide valuable insights and inform future research, but do not establish cause-and-effect relationships.
Importance of Descriptive Research in Scientific Studies
1. understanding of a population or phenomenon.
Descriptive research provides a comprehensive picture of the characteristics and behaviors of a particular population or phenomenon, allowing researchers to gain a deeper understanding of the topic.
2. Baseline Information
The information gathered through descriptive research can serve as a baseline for future research and provide a foundation for further studies.
3. Informative Data
Descriptive research can provide valuable information and insights into a particular topic, which can inform future research, policy decisions, and programs.
4. Sampling Validation
Descriptive research can be used to validate sampling methods and to help researchers determine the best approach for their study.
5. Cost Effective
Descriptive research is often less expensive and less time-consuming than other research methods , making it a cost-effective way to gather information about a particular population or phenomenon.
6. Easy to Replicate
Descriptive research is straightforward to replicate, making it a reliable way to gather and compare information from multiple sources.
Key Characteristics of Descriptive Research Design
The primary purpose of descriptive research is to describe the characteristics, behaviors, and attributes of a particular population or phenomenon.
2. Participants and Sampling
Descriptive research studies a particular population or sample that is representative of the larger population being studied. Furthermore, sampling methods can include convenience, stratified, or random sampling.
3. Data Collection Techniques
Descriptive research typically involves the collection of both qualitative and quantitative data through methods such as surveys, observational studies, case studies, or focus groups.
4. Data Analysis
Descriptive research data is analyzed to identify patterns, relationships, and trends within the data. Statistical techniques , such as frequency distributions and descriptive statistics, are commonly used to summarize and describe the data.
5. Focus on Description
Descriptive research is focused on describing and summarizing the characteristics of a particular population or phenomenon. It does not make causal inferences.
6. Non-Experimental
Descriptive research is non-experimental, meaning that the researcher does not manipulate variables or control conditions. The researcher simply observes and collects data on the population or phenomenon being studied.
When Can a Researcher Conduct Descriptive Research?
A researcher can conduct descriptive research in the following situations:
- To better understand a particular population or phenomenon
- To describe the relationships between variables
- To describe patterns and trends
- To validate sampling methods and determine the best approach for a study
- To compare data from multiple sources.
Types of Descriptive Research Design
1. survey research.
Surveys are a type of descriptive research that involves collecting data through self-administered or interviewer-administered questionnaires. Additionally, they can be administered in-person, by mail, or online, and can collect both qualitative and quantitative data.
2. Observational Research
Observational research involves observing and collecting data on a particular population or phenomenon without manipulating variables or controlling conditions. It can be conducted in naturalistic settings or controlled laboratory settings.
3. Case Study Research
Case study research is a type of descriptive research that focuses on a single individual, group, or event. It involves collecting detailed information on the subject through a variety of methods, including interviews, observations, and examination of documents.
4. Focus Group Research
Focus group research involves bringing together a small group of people to discuss a particular topic or product. Furthermore, the group is usually moderated by a researcher and the discussion is recorded for later analysis.
5. Ethnographic Research
Ethnographic research involves conducting detailed observations of a particular culture or community. It is often used to gain a deep understanding of the beliefs, behaviors, and practices of a particular group.
Advantages of Descriptive Research Design
1. provides a comprehensive understanding.
Descriptive research provides a comprehensive picture of the characteristics, behaviors, and attributes of a particular population or phenomenon, which can be useful in informing future research and policy decisions.
2. Non-invasive
Descriptive research is non-invasive and does not manipulate variables or control conditions, making it a suitable method for sensitive or ethical concerns.
3. Flexibility
Descriptive research allows for a wide range of data collection methods , including surveys, observational studies, case studies, and focus groups, making it a flexible and versatile research method.
4. Cost-effective
Descriptive research is often less expensive and less time-consuming than other research methods. Moreover, it gives a cost-effective option to many researchers.
5. Easy to Replicate
Descriptive research is easy to replicate, making it a reliable way to gather and compare information from multiple sources.
6. Informs Future Research
The insights gained from a descriptive research can inform future research and inform policy decisions and programs.
Disadvantages of Descriptive Research Design
1. limited scope.
Descriptive research only provides a snapshot of the current situation and cannot establish cause-and-effect relationships.
2. Dependence on Existing Data
Descriptive research relies on existing data, which may not always be comprehensive or accurate.
3. Lack of Control
Researchers have no control over the variables in descriptive research, which can limit the conclusions that can be drawn.
The researcher’s own biases and preconceptions can influence the interpretation of the data.
5. Lack of Generalizability
Descriptive research findings may not be applicable to other populations or situations.
6. Lack of Depth
Descriptive research provides a surface-level understanding of a phenomenon, rather than a deep understanding.
7. Time-consuming
Descriptive research often requires a large amount of data collection and analysis, which can be time-consuming and resource-intensive.
7 Ways to Avoid Common Flaws While Designing Descriptive Research
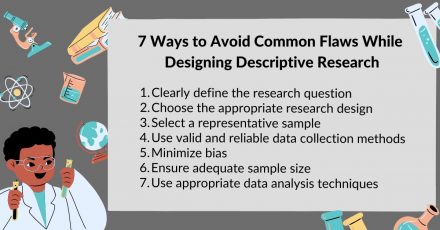
1. Clearly define the research question
A clearly defined research question is the foundation of any research study, and it is important to ensure that the question is both specific and relevant to the topic being studied.
2. Choose the appropriate research design
Choosing the appropriate research design for a study is crucial to the success of the study. Moreover, researchers should choose a design that best fits the research question and the type of data needed to answer it.
3. Select a representative sample
Selecting a representative sample is important to ensure that the findings of the study are generalizable to the population being studied. Researchers should use a sampling method that provides a random and representative sample of the population.
4. Use valid and reliable data collection methods
Using valid and reliable data collection methods is important to ensure that the data collected is accurate and can be used to answer the research question. Researchers should choose methods that are appropriate for the study and that can be administered consistently and systematically.
5. Minimize bias
Bias can significantly impact the validity and reliability of research findings. Furthermore, it is important to minimize bias in all aspects of the study, from the selection of participants to the analysis of data.
6. Ensure adequate sample size
An adequate sample size is important to ensure that the results of the study are statistically significant and can be generalized to the population being studied.
7. Use appropriate data analysis techniques
The appropriate data analysis technique depends on the type of data collected and the research question being asked. Researchers should choose techniques that are appropriate for the data and the question being asked.
Have you worked on descriptive research designs? How was your experience creating a descriptive design? What challenges did you face? Do write to us or leave a comment below and share your insights on descriptive research designs!

extremely very educative
Indeed very educative and useful. Well explained. Thank you
Simple,easy to understand
Rate this article Cancel Reply
Your email address will not be published.
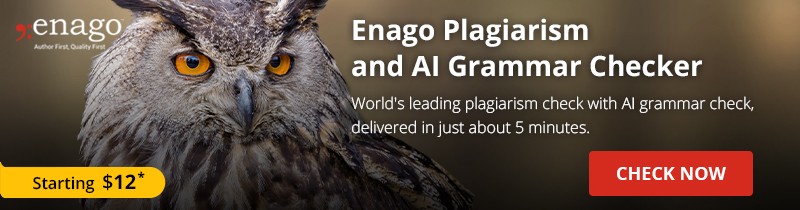
Enago Academy's Most Popular Articles
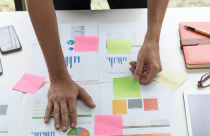
- Publishing Research
- Reporting Research
How to Optimize Your Research Process: A step-by-step guide
For researchers across disciplines, the path to uncovering novel findings and insights is often filled…
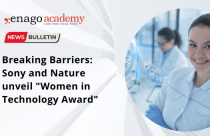
- Industry News
- Trending Now
Breaking Barriers: Sony and Nature unveil “Women in Technology Award”
Sony Group Corporation and the prestigious scientific journal Nature have collaborated to launch the inaugural…
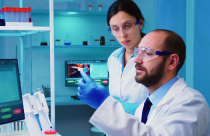
Achieving Research Excellence: Checklist for good research practices
Academia is built on the foundation of trustworthy and high-quality research, supported by the pillars…
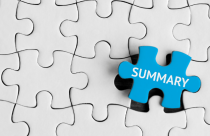
- Promoting Research
Plain Language Summary — Communicating your research to bridge the academic-lay gap
Science can be complex, but does that mean it should not be accessible to the…
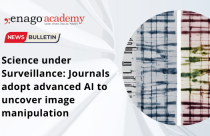
Science under Surveillance: Journals adopt advanced AI to uncover image manipulation
Journals are increasingly turning to cutting-edge AI tools to uncover deceitful images published in manuscripts.…
Choosing the Right Analytical Approach: Thematic analysis vs. content analysis for…
Comparing Cross Sectional and Longitudinal Studies: 5 steps for choosing the right…
Research Recommendations – Guiding policy-makers for evidence-based decision making

Sign-up to read more
Subscribe for free to get unrestricted access to all our resources on research writing and academic publishing including:
- 2000+ blog articles
- 50+ Webinars
- 10+ Expert podcasts
- 50+ Infographics
- 10+ Checklists
- Research Guides
We hate spam too. We promise to protect your privacy and never spam you.
I am looking for Editing/ Proofreading services for my manuscript Tentative date of next journal submission:
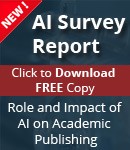
What should universities' stance be on AI tools in research and academic writing?
Descriptive Research and Qualitative Research
Cite this chapter.
- Eunsook T. Koh 2 &
- Willis L. Owen 2
906 Accesses
18 Citations
Descriptive research is a study of status and is widely used in education, nutrition, epidemiology, and the behavioral sciences. Its value is based on the premise that problems can be solved and practices improved through observation, analysis, and description. The most common descriptive research method is the survey, which includes questionnaires, personal interviews, phone surveys, and normative surveys. Developmental research is also descriptive. Through cross-sectional and longitudinal studies, researchers investigate the interaction of diet (e.g., fat and its sources, fiber and its sources, etc.) and life styles (e.g., smoking, alcohol drinking, etc.) and of disease (e.g., cancer, coronary heart disease) development. Observational research and correlational studies constitute other forms of descriptive research. Correlational studies determine and analyze relationships between variables as well as generate predictions. Descriptive research generates data, both qualitative and quantitative, that define the state of nature at a point in time. This chapter discusses some characteristics and basic procedures of the various types of descriptive research.
This is a preview of subscription content, log in via an institution to check access.
Access this chapter
- Available as PDF
- Read on any device
- Instant download
- Own it forever
- Compact, lightweight edition
- Dispatched in 3 to 5 business days
- Free shipping worldwide - see info
- Durable hardcover edition
Tax calculation will be finalised at checkout
Purchases are for personal use only
Institutional subscriptions
Unable to display preview. Download preview PDF.
Achterberg C. Qualitative methods in nutritional education evaluation research. J Nutr Educ 1988; 20: 244
Article Google Scholar
Agar, M.H. The Professional Stranger: an Informal Introduction to Ethnography. Academic Press, New York: Academic Press 1980.
Google Scholar
Amstrong B., Doll R. Environmental factors and cancer incidence and mortality in different countries, with special reference to dietary practices. Int J Cancer, 1975; 15:617–631
Bang H.O., Dyerberg J., Hjorne, N. The composition of food consumed by Greenland Eskimos. Acta Med Scand 1976; 200: 69–75
Article CAS Google Scholar
Bailey, K.D. Methods of Social Research. New York: Free Press, Macmillan Publishing Co, Inc, 1978.
Beal V.A. The nutritional history in longitudinal research. J Am Dietet A 1967; 51: 526–531
Berdie D.R. Questionnaire length and response rate. J Appl Psychol 1973; 58:278–280
Berdie, D.R., Anderson J.F., Niebuhr, M.A. Questionnaires: Design and Use. Metuchen, N.J.: Scarescrow Press, 1986.
Buell P. Changing incidence of breast cancer in Japanese-American women. JNCI 1973; 51:1479–1483
CAS Google Scholar
Dyberg J., Bang H.O., Hjorne N. Fatty acid composition of the plasma lipids in Greenland Eskimos. Am J Clin Nutr 1975; 28: 958–961
Frank G. Life history model of adaptation to disability: the case of a congenital amputee. Soc Sci Med 1984; 19: 639–645
Fetterman, D.L. “A Walk Through the Wilderness: Learning to Find Your Way.” In Experiencing Fieldwork: An Inside View of Qualitative Research. Shaffir, W., Stebbins, R. eds. Newbury Park, CA: Sage, 1991.
Fieldhouse, P. Food & Nutrition: Customs & Culture. New York: Croom Helm, 1986
Fielding, N.G., Fielding, J.L. Linking Data. Beverly Hills, CA: Sage, 1986.
Firestone W.A. Meaning in method: The rhetoric of quantitative and qualitative research. Educational Researcher 1987; 16:16–21
Geertz, C. The interpretation of Cultures. New York: Basic Books, 1973.
Glaser B.G., Strauss, A.L. The Discovery of Grounded Theory: Strategies for Qualitative Research. New York: Aldine Publishing Co, 1967.
Goldberger, J.E. Goldberger on Pellagra. Baton Rouge, LA: Louisiana State University Press, 1964.
Greer J.G. What do open-ended questions measure? Public Opinion Quart 1988; 52:365–371
Gordis, L. Epidemiology. Philadelphia PA: Saunders, 1996.
Hammersley, M., Atkinson, P. Ethnography: Principles in Practice. London: Tavistock, 1983.
Headland, T.N., Pike, K.L., Harris, M. Emics and Etics: The Insider/Outsider Debate. Newbury Park, CA: Sage, 1990.
Hodge, R., Kress, G. Social Semiotics. Ithaca, NY: Cornell University Press, 1988.
Holbrook J.T., Patterson K.Y., Bodner J.E., Douglas L.W., Veillon C., Kelsey J.L. Mertz W, Smith J.C. Sodium and potassium intake and balance in adults consuming self selected diets. Am J Clin Nutr 1984; 40: 786–793
Human Nutrition Information Service, US Department of Agriculture, Food Consumption: Households in the United States, Spring 1977, Washington, D.E.: Government Printing Office, Publication H-1, 1982.
ICNND (International Committee on Nutrition for National Defense) Manual for Nutrition Surveys. Second edition. Superintendent of Documents. Washington, D.C.: U.S. Government Printing Office, 1963
Jacob E. Qualitative research traditions: A review. Rev Educ Res 1987; 57: 1–4
Jelliffe, D.B. The Assessment of the Nutritional Status of the Community. WHO Monograph 53. Geneva: World Health Organization, 1966.
Keys, A. Seven Countries: A Multivariate Analysis of Death and Corornary Heart Disease. Cambridge, MA: Harvard University Press, 1980.
Kittler, P.G., Sucher, K. Food and Culture in America. New York: Reinhold, 1989.
Kirk, J., Miller, M.L. Reliability and Validity in Qualitative Research. Beverly Hills, CA: Sage, 1986.
Koh E.T., Caples V. Nutrient intake of low-income, black families in Southwest Mississippi. J Am Dietet A 1979; 75:665–670
Kolonel, L.N., Hinds, M.W., Hankin, J.H. “Cancer Patterns Among Migrant and Native-Born Japanese in Hawaii in Relation to Smoking, Drinking, and Dietary Habits.” In Genetic and Environmental Factors in Experimental and Human Cancer. Gelboin, H.V. et al. eds. Tokyo, Japan: Science Press, 1980.
Kromann N., Green A. Epidemiological studies in the Upernavik district, Greenland: Incidence of some chronic disease 1950–1974. Acta Med Scand 1980; 401–405
Krueger, R. Focus Groups: A Practical Guide for Applied Rese arch. Newbury Park, CA: Sage, 1988.
Langness, L.L. Frank, G. Lives: An Anthropological Approach to Biography. Novato, CA: Chandler & Sharp, 1981.
Lind, J. (1753) A Treatise on the Scurvy. Reprinted Edinburgh: Edinburgh University Press, 1953.
Lock, L.F. “The Question of Quality in Qualitative Research.” In Proceedings of the 5th Measurement and Evaluation Symposium. Nelson, J.K. ed. Baton Rouge, LA: Louisiana State University Press, 1987.
Lofland, J., Lofland, L. Analyzing Social Settings: A Guide to Qualitative Research. Belmont, CA: Wadsworth, 1984.
Marshall, C; Rossman, G.B. Designing Qualitative Research. Newbury Park, CA: Sage, 1989.
Mauser, J.S., Kramer, S. Epidemiology — An Introductory Text. Philadelphia PA: Saunders, 1985.
McClendon M.J., O’Brien D.J. Question-order effects on the determinants of subjective well-being. Public Opinion Quart 1988; 52:351–364
McCraken, G. The Long Interview. Newbury Park, CA: Sage, 1988.
McMichael A.J., McCall M.G., Hartshorne J.M., Woodings T.L. Patterns of gastro-intestinal cancer in European migrants to Australia: The role of dietary changes. In J Cancer 1980; 25:431–437
Miles, M.B., Huberman, A.M. Qualitative Data Analysis: A Sourcebook of New Methods. (2nd ed.) Thousand Oaks, CA: Sage, 1994.
Montgomery A.C., Crittenden K.S. Improving coding reliability for open-ended questions. Public Opinion Quart 1977; 41: 235–243
National Center for Health Statistics: Plan and Operation of the HANES. Vital and Health Statistics, Series 1, Nos 10a and 10b, DHEW Pub No (HSM) 73–130. Washington, D.C.: U.S. Government Printing Office, 1973.
O’Brien T., Dugdale V. Questionnaire administration by computer. J Market Res Soc, 1978; 20:228–237
Okolo, E.N. Health Research Design and Methodology. Boca Raton, FL: CRC Press 1990.
Parkin, D.M., Muir, C.S., Whelan, S.L., Gao, Y.T., Ferlay, J., Powell, J. Cancer Incidence in Five Continents VI IARC Sci Publ 120, Lyon: International Agency for Research on Cancer, 1992.
Peterkin B.B., Rizek R.L., Tippett K.S. Nationwide food consumption survey, 1987. Nutr Today 1988; 23:18–24
Poe G.S., Seeman I., McLaughlin J., Mehl E., Dietz M. Don’t know boxes in factual questions in a mail questionnaire: Effects on level and quality of response. Public Opinion Quart 1988; 52: 212–222
Poikolainen K., Karkkainen P. Nature of questionnaire options affects estimates of alcohol intake. J Stud Alcohol 1985; 46: 219–222
Potter, J.D. Food, Nutrition and the Prevention of Cancer: A Global Perspective. Washington, D.C.: American Institute for Cancer Research, 1997.
Rose, G.A., Blackburn, H. Cardiovascular Survey Methods. Geneva, Switzerland: World Health Organization 1968.
Runcie, J.F. Experiencing Social Sresearch. Homewood, IL.: Dorsey Press, 1976.
Sandelowski, M. The Problem of Rigor in Qualitative Research. Adv Nurs Sci April 27–37, 1986.
Schutz R.W. Qualitative research: comments and controversies. Research Quarterly Exercise Sport, 1989; 60:30–35
Sheatsley, P.B. “Questionnaire Construction and Item Writing.” In Handbook of Survey Research. Rossi, P.H., Wright, J.D., Anderson, A.B. eds. Orlando, FL: Academic Press, 1983.
Sherry, B. “Epidemiologic Analytical Research.” In Research. Monsen, E.R. Chicago, IL: American Dietetic Association, 1992
Spradley, J. The Ethnographic Interview. Chicago, IL: Holtz, Rinehart & Winston, 1979.
Spradley, J.P. Participant Observation. New York: Holt, Rinehart & Winston, 1980.
Sudman, S., Bradburn, N.M. Asking Questions: A Practical Guide to Questionnaire Design. (2nd ed.) Washington, D.C.: Jossey-Bass Publisher, 1983.
Taylor, S.J., Bogdan, R. Introduction to Qualitative Research Methods. (2nd ed.) New York: Wiley, 1984.
Thomas, J.R. Nelson, J.K. Research Methods in Physical Activity. (3rd ed.), Champaign, IL: Human Kinetics, 1996.
Van Maanen, J. ed. Qualitative Methodology. Newbury Park, CA: Sage, 1983.
Willett, W. Nutritional Epidemiology. (2nd ed.) New York: Oxford University Press, 1998.
Book Google Scholar
Download references
Author information
Authors and affiliations.
University of Oklahoma Health Sciences Center, USA
Eunsook T. Koh & Willis L. Owen
You can also search for this author in PubMed Google Scholar
Rights and permissions
Reprints and permissions
Copyright information
© 2000 Springer Science+Business Media New York
About this chapter
Koh, E.T., Owen, W.L. (2000). Descriptive Research and Qualitative Research. In: Introduction to Nutrition and Health Research. Springer, Boston, MA. https://doi.org/10.1007/978-1-4615-1401-5_12
Download citation
DOI : https://doi.org/10.1007/978-1-4615-1401-5_12
Publisher Name : Springer, Boston, MA
Print ISBN : 978-1-4613-5535-9
Online ISBN : 978-1-4615-1401-5
eBook Packages : Springer Book Archive
Share this chapter
Anyone you share the following link with will be able to read this content:
Sorry, a shareable link is not currently available for this article.
Provided by the Springer Nature SharedIt content-sharing initiative
- Publish with us
Policies and ethics
- Find a journal
- Track your research
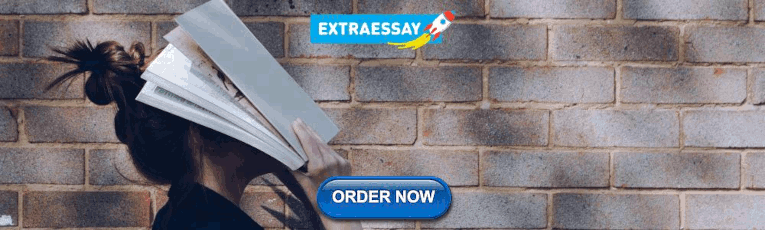
IMAGES
VIDEO
COMMENTS
Definition: Descriptive research design is a type of research methodology that aims to describe or document the characteristics, behaviors, attitudes, opinions, or perceptions of a group or population being studied. ... Limited utility for theory development: Descriptive research design may not be useful for developing theories about the ...
Research design is the strategy or blueprint for deciding how to collect and analyze information. Research design dictates which methods are used and how. Developmental research designs are techniques used particularly in lifespan development research. When we are trying to describe development and change, the research designs become especially ...
Remember, research methods are tools that are used to collect information, while r esearch design is the strategy or blueprint for deciding how to collect and analyze information. Research design dictates which methods are used and how. There are three types of developmental research designs: cross-sectional, longitudinal, and sequential.
The term design refers to "when," "where," and "how" data are collected, so the term can be used in three different ways. Descriptive developmental designs refer to when data are collected. These included cross-sectional, longitudinal, and sequential designs. Explanatory designs refer to how and where data are collected, so they ...
Descriptive research aims to accurately and systematically describe a population, situation or phenomenon. It can answer what, where, when and how questions, but not why questions. A descriptive research design can use a wide variety of research methods to investigate one or more variables. Unlike in experimental research, the researcher does ...
Developmental research designs are techniques used particularly in lifespan development research. When we are trying to describe development and change, the research designs become especially important because we are interested in what changes and what stays the same with age. These techniques try to examine how age, cohort, gender, and social ...
Descriptive research aims to accurately and systematically describe a population, situation or phenomenon. It can answer what, where, when, and how questions, but not why questions. A descriptive research design can use a wide variety of research methods to investigate one or more variables. Unlike in experimental research, the researcher does ...
Descriptive developmental research seeks to document, describe and analyze the conditions under which infants and children live and learn. Here, we articulate how open-science practices can be incorporated into descriptive research to increase its transparency, reliability and replicability. To date, most open-science practices have been ...
Developmental research is a particular way of addressing the basic questions of why and how to teach what to whom. It involves a cyclical process of small-scale in-depth development and evaluation, at a content-specific level, of exemplary teaching-learning sequences. It aims to produce an empirically supported justification of the inner ...
The Developmental Research Design was utilized by the researchers in the creation and evaluation of the Reading Assessment Manager as it is a "systematic study of designing, developing, and ...
The primary variable in developmental research is the subject's age. Developmental research may use research methods or research designs. Research designs are the plans developed to answer the ...
6 Developmental Research Designs . Anne Baird. These designs examine what changes and what stays the same in a human life. Chronological age, cohort membership, and time of measurement are the basic elements of research designs looking at development. The frustrating thing about doing this kind of research is that you only can vary two of these three elements at a time.
Descriptive research is an exploratory research method.It enables researchers to precisely and methodically describe a population, circumstance, or phenomenon.. As the name suggests, descriptive research describes the characteristics of the group, situation, or phenomenon being studied without manipulating variables or testing hypotheses.This can be reported using surveys, observational ...
Developmental research designs are techniques used particularly in lifespan development research. When we are trying to describe development and change, the research designs become especially important because we are interested in what changes and what stays the same with age. These techniques try to examine how age, cohort, gender, and social ...
The descriptive research design involves observing and collecting data on a given topic without attempting to infer cause-and-effect relationships. The goal of descriptive research is to provide a comprehensive and accurate picture of the population or phenomenon being studied and to describe the relationships, patterns, and trends that exist ...
The research design used was development research with the stages of the procedure, namely design, development, and evaluation [16]. The Design step begins with conducting a literature review of ...
The study of human development involves the investigation of changes that occur throughout the entire life span. Developmental research is often equated with research on children because the bulk of the literature focuses on child and adolescent development. Although this chapter primarily draws on research examples from school-aged children, it recognizes that the research investigating other ...
Descriptive research is a study of status and is widely used in education, nutrition, epidemiology, and the behavioral sciences. Its value is based on the premise that problems can be solved and practices improved through observation, analysis, and description. The most common descriptive research method is the survey, which includes ...
Descriptive Developmental Methodology. Research Design The researcher used the descriptive developmental method. Gillaco (2014), discussed that descriptive method seeks the real facts in relation to a current situation. Furthermore, this method works primarily on the description, comparison, analysis and interpretation of data that exists.
Richey, Rita C. Developmental research, as opposed to simple instructional development, has been defined as the systematic study of designing, developing, and evaluating instructional programs, processes, and products that must meet criteria of internal consistency and effectiveness. Developmental research is particularly important in the field ...
A descriptive survey research design was used in this study. A descriptive survey is a systematic method of gathering data from a sample of individuals through questionnaires or interviews to ...
Developmental Research Methods: Creating Knowledge from Instructional Design and Development Practice Rita C. Richey Wayne State University James D. Klein Arizona State University ABSTRACT T HE PURPOSE OF THIS ARTICLE is to provide direction to those entertaining a developmental research project. There are
The field of instructional technology has traditionally involved a unique blend of theory and practice. This blend is most obvious in developmental research, those studies that involve the production of knowledge with the ultimate aim of improving the processes of instructional design, development, and evaluation. Such research is based on either situation-specific problem solving or ...