Institute of Urban and Regional Development
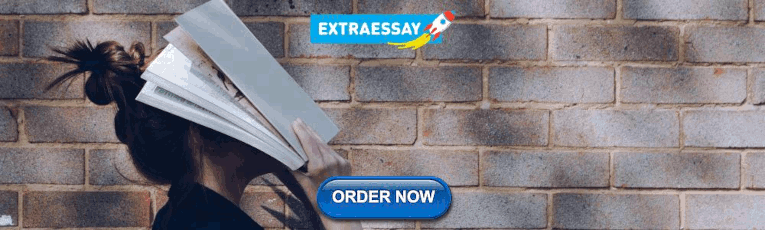
Sustainable Urban Development: A Literature Review and Analysis
- Wheeler, Stephen
This report reviews current literature on sustainable development and proposes a framework for applying this concept to city and regional planning. It begins by exploring interpretations of the concept of sustainability itself, next looks at some urban planning traditions toward an urban planning framework that can incorporate this concept. The following definition of sustainable urban development is proposed:
Sustainable urban development seeks to create cities and towns that improve the long-term health of the planet’s human and ecological systems.
Means to achieve this objective include protecting and restoring natural ecosystems in urban areas, creating community environments that nurture human potential, using land and resources wisely, and facilitating human lifestyles that contribute to global sustainability.
The author argues that sustainable urban development is indeed possible if 1) some degree of consensus is reached on values that can underlie it, 2) methods are developed to evaluate progress towards or away from sustainability, 3) specific policies, designs and programs are developed to implement sustainable urban development based on these values and yardsticks, and 4) the necessary political organizing, leadership development, and public education can be carried out.
Enter the password to open this PDF file:
Services on Demand
Related links.

South African Journal of Industrial Engineering
On-line version issn 2224-7890 print version issn 1012-277x, s. afr. j. ind. eng. vol.30 n.3 pretoria nov. 2019, http://dx.doi.org/10.7166/30-3-2247 .
SPECIAL EDITION
A Systematic Literature Review of Sustainable Urban Planning Challenges Associated with Developing Countries
A.F. Jooste * ; I.H. de Kock; J.K. Musango
Department of Industrial Engineering, Stellenbosch University, South Africa
The purpose of this study is to uncover the most prominent challenges that urban planners face when tackling sustainable practices in developing countries. The time frame was set to after the 2012 Rio +20 global summit. Therefore, only articles that were produced from 1 January 2013 till 31 March 2019 were included, as they would have adhered to the contributions and protocols set forth at the largest UN Earth Summit conference. In this study, a review of the sustainable urban planning challenges was conducted. The challenges are then grouped into topics that align with the theme of the type of challenge. Finally, the challenges are analysed to identify the most well-known and disruptive challenges that restrict urban planners in developing countries.
Die doel van die studie is die identifikasie van die mees prominente uitdagings wat stad- en streeksbeplanners in ontwikkelende lande in die gesig staar, spesifiek met betrekking tot volhoubare praktyke. Die oorwegingstydperk is beperk tot na die 2012 Rio +20 wêreldberaad. Publikasies vanaf 1 Januarie 2013 tot 31 Maart 2019 was oorweeg, omdat hulle sou voldoen aan die bydrae en protokolle soos bepaal deur die grootste VN Wêreldberaad. 'n Hersiening van volhoubare stadsbeplanning uitdagings is van stapel gestuur en die uitdagings dan gegroepeer in belynde onderwerpe. Laastens is die uitdagings ontleed om sodoende die mees bekende en ontwrigtende uitdagings te identifiseer.
1 INTRODUCTION
Urban planning is a very old profession. It arose when people began to gather together for practical reasons, bringing resources, security, and amenities closer to more people [1]. However, in the 21 st century, traditions have changed rapidly, and cities need to be resilient in the face of urbanisation and population growth. Sustainability is the buzz word that is constantly found in master city plans. But do city planners know what this entails? Do they understand how to balance the environment with the economic, social, and political ideals of the city to ensure a stable and resilient future?
There seems to be a lack of consensus among urban planning practitioners about how to implement sustainable practices in urban development [1]; [2]; [3]; [4]. This is a difficult task, and it is imperative to ensure that the negative aspects of unsustainable situations do not prevail into the next generation. With the exponential growth of technology, it is very important to use the potential benefits in the urban development landscape.
This study seeks to identify the urban planning challenges that arise when planners attempt to initiate sustainable principles. The study used a systematic literature review (SLR) - a structured process that gathers relevant research papers on a specific theme. The challenges related to sustainable urban planning were identified in relevant research papers and synthesised in a large table containing all these challenges. Finally, the pertinent challenges that disrupt urban planners from designing and managing cities sustainably were revealed.
The first section of the study explains the process of following through a systematic literature review from review questions to how the information was extracted. Then there is a brief analysis of the challenges that appear most frequently, together with a synthesis of the findings that will be investigated. The study concludes by answering the review questions and discussing further research.
2 SYSTEMATIC LITERATURE REVIEW
An SLR is a procedurally rigorous examination of research results [5]. The beginning of the project starts with a foundation of knowledge. The difference between a typical literature review and an SLR is found in the fundamental approach. An SLR follows a method that is set out before the review begins, and contains several steps, including extraction and synthesis. The SLR begins with a Boolean search, which refers to sifting through a large database of research articles using a group of synonyms for the theme under review (see Table 1 ). Next, the SLR method scrutinises the new group of research papers to ensure their relevance to the intended theme. See Figure 2 for the SLR search flowchart. Thereafter, the sustainable urban planning challenges are extracted from each paper; this is discussed further in Section 3. Lastly, the challenges are synthesised by tallying the number of challenges in each topic and conclude the SLR.

2.1 Literature review questions
The research questions addressed by this study were:
1. What research topics on sustainable urban planning are being addressed?
2. How effective is the systematic literature review?
3. What are the limitations and biases of a systematic literature review?
The literature review questions are discussed in the conclusion.
2.2 Search strategy
The literature search process was conducted using the Scopus database. The sources searched using a search string are presented in Table 1 .
Study selection criteria
If there are too many research records to choose from, then the search can be reduced using the terms (i) Developing countries, (ii) Sub-Saharan Africa, and (iii) South Africa. Articles on these topics were included if they were published between 1 January 2013 and 31 March 2019. This is because Rio +20 was held from 20 to 22 June 2012. Rio +20 was the biggest UN conference ever presented; the previous Earth summit having been held 10 years before. Therefore, articles are only included from the year following this event, with relevance to sustainable urban planning. This choice is further solidified with the number of articles produced thereafter. See Figure 1 , which displays the number of documents published per year from the relevant research papers produced over the last ten years.
The following types of papers were excluded:
• Informal literature surveys (no references, no publication)
• Papers not subject to peer-review
• Older versions of multiple papers found in more than one journal
Figure 2 is the SLR search flowchart that includes the process of the SLR, when and why papers were excluded, and the number of papers at each step of exclusion.
2.4 Data extraction strategy
The data extracted from each paper were:
• The name(s) of the author(s).
• The year when the paper was published. (Note, if the paper was published in several different sources, all dates were recorded, and the earliest date was used in the analysis.)
• To extract every challenge each paper mentions relating to sustainable urban planning.
• These challenges are referred to as a 'topic'. Main topics contain several subtopics that contribute and connect to each challenge.
• The topics are as follows: (i) sustainability, (ii) planning, (iii) urbanisation, (iv) urban sprawl, (v) society, (vi) environmental, (vii) economic, (viii) developing country, (ix) population growth, (x) government, (xi) energy, (xii) food security, and (xiii) climate change. These topics were found to be the most prevalent over the 41 research papers on sustainable urban planning.
2.5 Synthesis of extracted data
The sources were presented in a matrix, following the steps in section 2.4; articles falling into each topic and subtopic are totalled. Thereafter, a synthesis of the most discussed topics was drawn up.
3 SYSTEMATIC LITERATURE REVIEW CHALLENGES
With the use of a matrix containing all the challenges found in the 41 research papers selected in the SLR on sustainable urban planning, the most prevalent challenges that form most of a developing country's context were chosen. These dominant challenges were used to develop the links between current urban planning tools and techniques. This provided insight into the gap between current sustainable planning practice and the proposed future planning technique that was developed in this research project. In this section, the challenges contained in the SLR are discussed.
As mentioned in section 2.4, the challenges fall under 13 important topics. From this list, six topics have more than 50 challenges. Those are summarised in Table 2 .
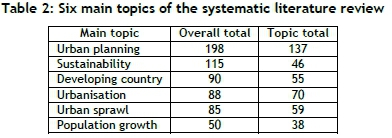
The differences between the 'Overall total' column and the 'Topic total' column are where these challenges appeared. The 'Topic total' was found only under the specific topic in question. However, the 'Overall total' is where all the specific challenges were found throughout the matrix. For example, 'Overall total' contained the challenges found under any topic. If a challenge was also associated with an economic and urban planning issue, it was considered within the 'Overall total'.
There are 581 challenges in the SLR challenges matrix that were extracted from the 41 selected research articles. Furthermore, 34 per cent of the total challenges were about planning, while 20 per cent were linked to sustainability. From Table 2 , it is evident that the total of all the main topic challenges adds up to 626. This is because the matrix is set up with each topic area containing topics to which the challenge is linked. Take, for example, the challenge that states, "Identifying a sustainable form of growth, especially when considering specific local context and conditions is a difficult task. Implementation of plans and the realisation of urban forms are even more challenging" [6]. This challenge is about the combination of sustainability and urban planning. Thus, it was placed under the main topic of sustainability and under the subtopic of urban planning. The reason for the overlaps was to show the links between all the topics and to allow for a greater synthesis of the challenges.
Urban planning challenges
The three prominent subtopics from the SLR that are found in urban planning are sustainability, society, and environment. Out of 137 challenges, 19, 17, and 10 of those subtopics respectively were found only in the topic of urban planning and not overall, as seen in Table 2 .
3.1.1 Sustainability
There is a lack of consensus about how to incorporate sustainable traditions into urban planning [1]; [2]; [3]; [4]. The challenges arise when trying to translate sustainability into city development. Planners have their own definitions and contexts that will disrupt any collaboration that may need to take place between policy coordinators, stakeholders, and government.
3.1.2 Society
Poor urban planning causes unwanted and spatially disjointed urban forms, leading to increased traffic congestion, vulnerability, and risks, and decreasing public health for the inhabitants in vulnerable areas [1]; [7]; [8]; [9]. Research reveals that planners do not realise the larger impact that their inconsistencies have on social dynamics in communities.
3.1.3 Environment
Urban planners seem to have the right intentions to protect the environment through designing cities and buildings. However, this has led to a contradictory outcome in which urban expansion has become unplanned, thus increasing carbon emissions and developing environmentally hazardous zones for residents [10]; [11]; [12]. Urban planners need to take more responsibility in realising their impact on the environment.
Sustainability challenges
The three prominent subtopics from the SLR that are found under 'sustainability' are urban planning, resource management, and environment. Out of 46 challenges, 11, four, and four of those subtopics respectively were found only in the topic of sustainability and not overall, as seen in Table 2 .
3.2.1 Urban planning
Sustainable urban planning is imperative in today's city planning strategies. However, with everyone using the term, one would think that there would be a set definition to which everyone would adhere. However, "there is no agreement of sustainable urban forms" [13]. The confusion has led to the term ' sustainable urban planning' becoming distorted and impractical [8]. Even if one were to agree on a sustainable urban form for a specific context, there would still be little support from policy-makers and government to implement the practices [14]; [6].
3.2.2 Resource management
The essence of sustainability is to provide for future needs. A major concern is the depletion of resources such as energy, water, and food. "Natural resources should be seen as capital and not an income source" [15]. Critical analysis should be done to understand the stock of a city's resources, and venture to become a more self-sufficient system via circulation and limiting human activities [16]; [17]; [2].
3.2.3 Environment
Environmental conservation is rarely implemented into a nation's policy, as it is seen as anti-development, especially in developing countries, whose main goal is to be economic [14]; [8]; [18].
3.3 Developing country challenges
The three prominent subtopics from the SLR that are found in developing countries are economy, society, and urban planning. Out of 55 challenges, six, six, and five of those subtopics respectively were found only in the topic of developing country and not overall, as seen in Table 2 .
3.3.1 Economic
Developing countries face many challenges to implement sustainable urban planning practices due to economic constraints [10]; [14]; [19]; [20]; [18]. Whether aligned with transport, water security, or enabling technological assistance, developing countries are falling further behind in applying sustainable projects.
3.3.2 Society
Developing countries face great challenges in the realm of social sustainability. A general definition of a developing country is one that is plagued with inequalities of income and quality of life, in which residents experience underemployment and inadequate shelter and living environments [16]; [21]; [20]. This is coupled with political systems that believe that all development is good if it provides jobs [18]. This statement does not follow sustainable practices: it is one-sided in following an economic path without taking the public's concerns for their wellbeing into account.
3.3.3 Urban planning
Urban planning in low- to middle-income countries cannot follow the development trends of developed countries due to the deep-rooted influences of uneven development caused by colonialism [22]. Furthermore, even if developing countries want to produce sustainable urban forms, they lack the infrastructure and management capabilities [23]; [10].
3.4 Urbanisation challenges
The three prominent subtopics from the SLR that are found in urbanisation are sustainability, society, and developing country. Out of 70 challenges, 14, 10, and nine of those subtopics respectively were found only in the topic of urbanisation and not overall, as seen in Table 2 .
3.4.1 Sustainability
Rapid urbanisation affects major issues such as resource consumption, environmental damage, economic and political changes, along with social problems; and so it is unsustainable in respect of urban planning [16]; [10]; [24]; [25]; [15]; [1]; [12].
3.4.2 Society
Urbanisation causes large disruptions from the perspective of social sustainability, producing social segregation, higher levels of pollution, unequal distribution of wealth, and poor public health
systems [26]; [15]; [27]; [12]; [7].
3.4.3 Developing country
Urbanisation has a significant impact on developing countries, creating unplanned urban development, changing economy-oriented priorities for governments, threatening resource bases, and dictating fuel consumption patterns [26]; [17]; [25]; [28]; [15].
3.5 Urban sprawl challenges
The three prominent subtopics from the SLR that are found in urban sprawl are transportation, urban planning, and society. Out of 59 challenges, 11, 10, and nine of those subtopics respectively were found only in the topic of urban sprawl and not overall, as seen in Table 2 .
3.5.1 Transporta tion
"Sprawl, as a low-density and dispersed form of urban expansion, is generally considered to be a threat to sustainability: it is characterised by inefficient modes of transit" [6]. Sprawling development patterns cause auto-dependent societies: people are forced to travel long distances, spending up to 40 per cent of their income to get to work in town [21]; [29]; [30]; [31]; [7], further increasing carbon emissions and contributing to climate change.
3.5.2 Urban planning
Urban sprawl is a major problem for urban planners. It exists due to inadequate decision-making and management systems [1], leading to concerns about the availability of arable land for future generations' food security [32]; [2].
3.5.3 Society
Urban sprawl disrupts the social environments of communities on the peripheries of cities, reducing public health, and increasing unemployment and social segregation [4]; [6]; [1]; [7].
3.6 Population growth
The two prominent subtopics from the SLR that are found in population growth from the SLR are developing country and urban planning. Out of 38 challenges, 10 and five of those subtopics respectively were found only in the topic of population growth and not overall, as seen in Table 2 .
3.6.1 Developing country
Worldwide population growth in future decades will be concentrated in developing countries [4]; [14]; [22]; [9]; [7]; [32].
3.6.2 Urban planning
With the unprecedented population growth occurring all over the globe, urban planners are struggling to keep up with all the infrastructural needs [19]; [3]; [24]; [33].
4 SYNTHESIS OF SLR CHALLENGES
Table 2 identifies the six most discussed topics that were found in the SLR. The topics that coincide with the search criteria are urban planning, sustainability, and developing country. Therefore, the topics that are of importance from the SLR are urbanisation, urban sprawl, and population growth. It is evident that these three phenomena are dependent on one another. For example, population growth leads to urbanisation as people need to survive, and cities have all the services and resources. This combination leads to rapid expansion, causing urban sprawl, along with all its negative impacts. To mitigate the problems caused by urban sprawl, urban planners need to resist these anti-development phenomena. Adaption methods and techniques must first be incorporated to manage the current issues. Then the new plans must develop a resilient structure to combat these changes as they arise. It is more important than ever for urban planners to use technology to predict these rapidly changing trends and to enhance the sustainability of cities in developing countries. It is known that developing countries lack data and specialised personnel. But, with this research, the hope is that a foundation can begin to be laid with the latest effective technology.
5 CONCLUSION
The research questions were formulated to ensure that the quality of the SLR was high. First, what were the research topics addressed? The SLR was framed around sustainable urban planning challenges in the context of developing countries. The topics that most clearly challenge urban planners were urbanisation, urban sprawl, and population growth. These topics are dependent on one another, and so need further investigation to identify the crux of the problem for sustainable practices in planning urban development. Second, how effective was the SLR? Forty-one research papers were found to be noteworthy for the SLR. All of the challenges addressed in this study came from 32 research papers. This reveals nine papers that described challenges that the majority were not discussing. This is not to say that the other nine were not considered. However, for the purpose of the SLR, the goal was to identify the most prominent challenges that have faced urban planners in the last seven years. The SLR was 78 per cent effective in identifying most of the challenges. Last, the SLR had limitations and biases. The first limitation was the time frame. Only gathering research papers since 2013 was a risk associated with this study. However, the Rio +20 conference was the defining event that influenced the research that followed. Figure 2 assists this argument, showing a large increase in research produced from 2013 onwards. The bias in the study emanates from the search criteria. The context of developing countries was a necessary focus for the study. Sustainable practices used in developed countries would be either too advanced or too expensive to allow developing nations to address their problems realistically.
The SLR discussed in this paper will be further implemented to uncover the tools and techniques used in current sustainable urban planning practices. Together with the synthesis in section 4, a more structured approach will be identified for how to plan urban settings sustainably to ensure that future generations will not be left sorting out our problems.
[1] Bibri, S.E. 2018. A foundational framework for smart sustainable city development: Theoretical, disciplinary, and discursive dimensions and their synergies. Sustainable Cities and Society, 38(September) pp. 758-794. doi: 10.1016/j.scs.2017.12.032. [ Links ]
[2] Gradinaru, S.R., Triboi, R., Ioja, C. & Artmann, M. 2018. Contribution of agricultural activities to urban sustainability: Insights from pastoral practices in Bucharest and its peri-urban area. Habitat International, 82(September), pp. 62-71. doi: 10.1016/j.habitatint.2018.09.005. [ Links ]
[3] Mohareb, E., Derrible, S. & Peiravian, F. 2016. Intersections of Jane Jacobs' conditions for diversity and low-carbon urban systems: A look at four global cities. Journal of Urban Planning and Development, 142(2), pp. 1-14. doi: 10.1061/(ASCE)UP.1943-5444.0000287. [ Links ]
[4] Shummadtayar, U., Hokao, K. & Iamtrakul, P. 2013. Investigating the low-income settlement in an urbanization and urban form a consequences of Bangkok growing city, Thailand. Lowland Technology International 15(1), pp. 45-54. [ Links ]
[5] Kitchenham, B. 2007. Guidelines for performing systematic literature reviews in software engineering. Software Engineering Group School of Computer Science and Mathematics, p. 65. doi: 10.1145/1134285.1134500. [ Links ]
[6] Slaev, A.D. & Nedovic-Budic, Z. 2017. The challenges of implementing sustainable development: The case of Sofia's master plan. Sustainability (Switzerland), 9(1) pp. 1-19. doi: 10.3390/su9010015. [ Links ]
[7] Moroke, T., Schoeman, C. & Schoeman, I. 2019. Developing a neighbourhood sustainability assessment model: An approach to sustainable urban development. Sustainable Cities and Society, January 2018, p. 101433. doi: 10.1016/j.scs.2019.101433. [ Links ]
[8] Kaagaard, L.M.K. 2016. Planning for sustainability. Management of Creative Business Processes, March 2016, 1. [ Links ]
[9] Endo, T. & Shibuya, M.K. 2017. Urban risk, risk response and well-being in Asian cities: The case of Tokyo, Shanghai, and Bangkok. Procedia Engineering, 198(September), pp. 975-984. doi: 10.1016/j.proeng.2017.07.143. [ Links ]
[10] Wamsler, C., Brink, E. & Rivera, C. 2013. Planning for climate change in urban areas: From theory to practice. Journal of Cleaner Production, 50, pp. 68-81. doi: 10.1016/j.jclepro.2012.12.008. [ Links ]
[11] Laffta, S. & Al-Rawi, A. 2018. Green technologies in sustainable urban planning. MATEC Web of Conferences, 162, 2018, pp. 1 -7. [ Links ]
[12] Shabatura, L., Bauer, N. & Iatsevich, O. 2018. Socio-cultural problems of sustainable urban environment. IOP Conference Series: Materials Science and Engineering, 2018. doi: 10.1088/1757-899X/463/2/022009. [ Links ]
[13] Habibi, S. & Zebardast, E. 2016. How compact are midsize cities in Iran?, Journal of urban planning and development, 142(4), 2016, pp. 1-11. doi: 10.1061/(ASCE)UP.1943-5444.0000333. [ Links ]
[14] Russo, T., Alfredo, K. & Fisher, J. 2014. Sustainable water management in urban, agricultural, and natural systems. Water (Switzerland), 6(12), pp. 3934-3956. doi: 10.3390/w6123934. [ Links ]
[15] Randhawa, A. & Kumar, A. 2017. Exploring sustainability of smart development initiatives in India. International Journal of Sustainable Built Environment, 6(2), pp. 701-710. doi: 10.1016Aj.ijsbe.2017.08.002. [ Links ]
[16] Chang, I.C. & Sheppard, E. 2013. China's eco-cities as variegated urban sustainability: Dongtan eco-city and Chongming. Journal of Urban Technology, 12(2) pp 57-75. doi: 10.1080/10630732.2012.735104. [ Links ]
[17] Currie, P.K. & Musango, J.K. 2016. African urbanization assimilating urban metabolism into sustainability discourse. Journal of Industrial Ecology, 21(5), pp 1262-1276. doi: 10.1111 /jiec. 12517. [ Links ]
[19] Ding, X., Zhong, W., Shearmur, R.G., Zhang, X. & Huisingh, D. 2015. An inclusive model for assessing the sustainability of cities in developing countries - Trinity of Cities ' Sustainability from Spatial , Logical and Time Dimensions (TCS-SLTD). Journal of Cleaner Production, 109, pp. 62-75. doi: 10.1016Aj.jclepro.2015.06.140. [ Links ]
[20] Bai, M., Zhou, S., Zhao, M. & Yu, J. 2017. Water use efficiency improvement against a backdrop of expanding city agglomeration in developing countries: A case study on industrial and agricultural water use in the Bohai Bay Region. Water (Switzerland), 9(2). doi: 10.3390/w9020089. [ Links ]
[21] Simon, D. 2013. Climate and environmental change and the potential for greening African cities. Local Economy, 28(2), pp 203-217. doi: 10.1177/0269094212463674. [ Links ]
[22] Horn, A. 2015. Urban growth management best practices: Towards implications for the developing world. International Planning Studies, 20, pp. 131-145. doi: 10.1080/13563475.2014.942513. [ Links ]
[23] Babalik-Sutcliffe, E. 2013. Urban form and sustainable transport: Lessons from the Ankara case urban form and sustainable transport. International Journal of Sustainable Transportation, 7(5), pp 416-430. doi: 10.1080/15568318.2012.676152. [ Links ]
[24] Zhang, X. 2016. Sustainable urbanization: A bi-dimensional matrix model. Journal of Cleaner Production, 134, pp. 425-433. doi: 10.1016/j.jclepro.2015.08.036. [ Links ]
[25] Brelsford, C., Lobo, J., Hand, J. & Benttencourt, L.M.A. 2017. Heterogeneity and scale of sustainable development in cities. Proceedings of the National Academy of Sciences of the United States of America, 114(34), pp 8963-8968. doi: 10.1073/pnas.1606033114. [ Links ]
[26] Musango, J.K. 2014. Energy for sustainable development: Household electricity access and consumption behaviour in an urban environment: The case of Gauteng in South Africa. Energy for Sustainable Development, 23, pp. 305-316. doi: 10.1016/j.esd.2014.06.003. [ Links ]
[27] Xu, Y., Ren, C., Ma, P., Ho, J., Wang, W., Lau, K.K.L., Lin, H. & Ng, E. 2017. Landscape and urban planning: Urban morphology detection and computation for urban climate research. Landscape and Urban Planning, 167(May), pp. 212-224. doi: 10.1016/j.landurbplan.2017.06.018. [ Links ]
[28] Broto, V.C. 2017. Energy landscapes and urban trajectories towards sustainability. Energy Policy, 108(January), pp. 755-764. doi: 10.1016/j.enpol.2017.01.009. [ Links ]
[29] Dur, F., Yigitcanlar, T. & Bunker, J. 2014. A spatial-indexing model for measuring neighbourhood-level land-use and transport integration. Environment and Planning B: Planning and Design, 41 (5), pp. 792-812. doi: 10.1068/b39028. [ Links ]
[30] Ahmed, K.G. 2017. Designing sustainable urban social housing in the United Arab Emirates. Sustainability (Switzerland), 9(8). doi: 10.3390/su9081413. [ Links ]
[31] Artmann, M., Kohler, M., Meinel, G., Gan, J. & Ioja, I. 2019. How smart growth and green infrastructure can mutually support each other: A conceptual framework for compact and green cities. Ecological Indicators, 96(June), pp. 10-22. doi: 10.1016/j.ecolind.2017.07.001. [ Links ]
[32] Aburas, M.M., Ho, Y.M., Ramli, M.F. & Ash'aari, Z.H. 2018. Monitoring and assessment of urban growth patterns using spatio-temporal built-up area analysis. Environmental Monitoring and Assessment, 190(3). doi: 10.1007/s10661-018-6522-9. [ Links ]
[33] Li, W., Zhou, W., Han, L. & Qian, Y. 2019. Uneven urban-region sprawl of China's megaregions and the spatial relevancy in a multi-scale approach. Ecological Indicators, 97(December), pp. 194-203. doi: 10.1016/j.ecolind.2018.10.004. [ Links ]
* Corresponding author: [email protected] # Author was enrolled for an MEng in the Department of Industrial Engineering, Stellenbosch University, South Africa
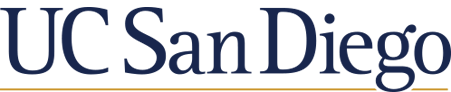
- Research & Collections
- Borrow & Request
- Computing & Technology

Urban Studies & Planning: Intro to Lit Reviews
- Intro to the Library
- Intro to Lit Reviews
- Background Info
- Journal List
- News Sources
- Govt Documents
- Google Tips
- Historical Planning Resources
- San Diego Planning Resources
Literature Review: An Overview
A literature review provides an overview of the scholarly writings published on a topic by scholars and researchers. A good literature review forms the justification for and drives your own research: it is the platform upon which you will build your argument, place your research in context ("They say") , and demonstrate how your research contributes to the broader discussion ("I say").
Specifically, a literature review:
- Focuses on a particular question, area of research, theory or issue.
- Provides an overview of the existing literature and knowledge gaps on a topic and reflects a critical analysis of this research.
- Demonstrates that you've read extensively in your field, have a thorough understanding of that field, and are capable of intelligently critiquing others' work.
Conducting a Literature Review
1. Define a research question.
Your literature review should be guided by a central research question. The research question should be neither too broad nor too narrow; it may help to start with a broad question and narrow it as you read through initial literature.
2. Decide on the scope of your review.
How comprehensive does it need to be? For example, how many years should it cover? What geographic area?
3. Determine your search strategies.
Think of keywords and related terms that are relevant to your topic. It will probably help to start broad and then narrow the keywords based on your search results.
Look at different types of information resources and think of how they might relate and contribute to your search. (Note that this guide is intentionally designed with this idea in mind!)
- The web and subject encyclopedias/handbooks can be useful for getting an initial feel for the topic.
- Books are good for in-depth research, but may not be as timely as journal articles.
- Journal articles are especially good for finding current research on a topic. Peer-reviewed journal articles are written by scholars and professionals in the field.
- Dissertations can be especially helpful for finding related local research and bibliographies.
- Government documents are great for primary source material, especially related to policy issues.
- Newspapers are good for very current and very local information.
Select the databases you will use to conduct your searches. Your database choices will be partially or completely dependent on the subject you're researching. Start with the databases on this guide, then broaden out to others if necessary. Feel free to contact Kelly if you need suggestions.
4. Conduct your searches and find your literature.
Review the abstracts of research studies carefully, rather than reading the complete articles, to determine relevance.
Write down the searches you conduct in each database so that you can duplicate them if necessary.
Be sure to get all the information you will need to cite each source; also keep track of which database each article was found in.
Use the bibliographies and cited references of studies you find to locate additional related studies.
Keep in mind that research outside your primary focus area may be helpful for providing context.
5. Review the literature. Some guiding questions to keep in mind are:
What was the research question of the study you are reviewing? What were the authors trying to discover?
Does the research seem to be complete? Can you identify potential problems in how the research was conducted?
How does the research contribute to your understanding of the issue you are researching?
Do various authors come to the same or different conclusions? How are the articles related?
What ideas have not been covered? What are areas ripe for future research?
6. Write your paper.
You may want to sort the materials you have read based on their different themes, theoretical foundations, or conclusions. Then, for each article, describe the research that was done and the conclusions of the authors. Discuss how that particular work contributes to the understanding of the subject that you are working on .
- << Previous: Intro to the Library
- Next: Background Info >>
- Last Updated: May 15, 2024 8:47 AM
- URL: https://ucsd.libguides.com/usp

- University of Texas Libraries
- UT Libraries
Community and Regional Planning
- Literature Reviews
- Databases and Journals
- Digital Collections
- Statistical Data
- GIS & Geospatial Data
- Codes and Standards
- Local Data Sources
- Visual Resources
- Writing a Research Paper
- Evaluate Sources
- Case Studies
- Citations and Data
- American Planning Association
- Suggested Reading
Literature Review
What is a Literature Review?
It is…. a systematic and critical analysis of the literature on a specific topic. It describes trends, quality, relationships, inconsistencies and gaps in the research; and it details how the works enhance your understanding of the topic at large.
It is NOT…. simply an annotated bibliography that summarizes and/or assesses each article. There is not one, correct way to approach and write a literature review. It can be a stand-alone paper or part of a thesis/dissertation. Format and requirements can vary between disciplines, purpose and intended audience.
A literature review is an overview of existing literature (books, articles, dissertations, conference proceedings, and other sources) in a particular scholarly area. With a lit review, you will:
- Gather information about your topic, including the sources used by others who have previously conducted research
- Find out if your specific research question has already been answered
- Find out what areas or perspectives have not yet been covered by others on your topic
- Analyze and evaluate existing information
The literature review will assist you in considering the validity and scope of your research question so that you can do the necessary revision and fine tuning to it. It provides the foundation to formulate and present strong arguments to justify your chosen research topic.
- How to Write a Literature Review (University of California, Santa Cruz)
Check out these books from the library for further guidance:
- Després, Carole. "The meaning of home: literature review and directions for future research and theoretical development." Journal of architectural and planning research 8, no.2, (Summer 1991): 96-155.
- Steiner, Frederick R. "Philadelphia, the holy experiment: A literature review and analysis." Ekistics , 49, (1982): 298-305.
Reckoning with Authorities
As you are developing your Lit Review, part of your objective is to identify the leading authorities within the field or who address your topic or theme. Some tips for identifying the scholars:
Old Fashioned Method:
- Keep notes on footnotes and names as you read articles, books, blogs, exhibition catalogs, etc. Are there names or works that everyone references? Use the catalog to track these reference down.
- Consider looking for state of the field articles often found either in a discipline's primary journal or in conference proceedings - keynote speakers.
- Look for book reviews.
Publication Metrics:
- These resources include information about the frequency of citations for an article/author.
- These resources are not specifically for Architecture or Planning. Remember therefore to be critical and careful about the assumptions you make with regard to the results!
The Web of Science platform currently also provides temporary access to several databases that are not part of the Core Collection, including Biosis Citation Index, Data Citation Index, and Zoological Record.
Use this link to access Google Scholar, and see our Google Scholar Guide for information on using this resource.
If you encounter a warning about the security certificate when using the FindIt@UT tool in Google Scholar, you can learn more about that using this guide .
- Last Updated: Dec 21, 2023 1:25 PM
- URL: https://guides.lib.utexas.edu/crp
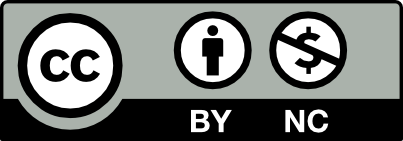
Urban & Regional Planning
- Databases & Journals
- Demographics
- ArcGIS at Georgetown This link opens in a new window
- Local (DC & DMV) Resources
- Organizations and Associations
Writing the Literature Review
Sample literature reviews.
- Chicago 17th Edition
Review the following websites for tips on writing a literature review:
Literature Reviews. The Writing Center, University of North Carolina at Chapel Hill
Write a Literature Review: Virginia Commonwealth University
Chapin, Timothy S. "From Growth Controls, to Comprehensive Planning, to Smart Growth: Planning’s Emerging Fourth Wave." Journal Of The American Planning Association 78, no. 1 (Winter 2012): 5-15. Environment Complete, EBSCOhost.
https://doi.org/10.1080/01944363.2011.645273
- << Previous: Organizations and Associations
- Next: Chicago 17th Edition >>
- Last Updated: Jan 26, 2024 10:55 AM
- URL: https://guides.library.georgetown.edu/urbanplanning

Planning : Urban and Regional
- Key resources
- Journal articles
- Maps and images
- Planning schemes and property information
- Standards and statistics
- Planning sites
- Specialised resources
- Research support
What is a literature review / annotated bibliography?
Finding the gaps and advances in your topic, useful resources on literature or systematic reviews, referencing, finding theses.
A literature review is an assessment and critical analysis of the literature (what has been published) on a particular topic. Literature Review Guide
An annotated bibliography provides:
- A reference list with a summary of the main arguments or idea of each source
- A critique or evaluation of the source’s usefulness, reliability, objectivity or bias
- A reflection on how the source fits into your research
Both provide a critical review of the literature.
For writing help - contact Student Support Assignment types
- Finding review articles Reading review articles is a good way to get an overview of the current research on a topic. Review articles provide a comprehensive summary of the research on a topic, offer a perspective on the status, and often deliver recommendations for future research.
- Set up research alerts Keep up to date with new research on your topic by setting up alerts.
- Cited reference searching Find more articles on your topic.
- Griffith School of Environment - Systematic quantitative literature review Developed by the School of Environment at Griffith University for environmental science students, this resource shows how to systematically search the literature using online databases; save and manage the information and quantify the current status of the literature.
- Systematic searching for environmental evidence using multiple tools and sources Environmental Evidence The official journal of the Collaboration for Environmental Evidence, 2017 6:23. https://doi.org/10.1186/s13750-017-0099-6 © The Author(s) 2017
- Guidelines for Systematic Reviews in Environmental Management Collaboration for Environmental Evidence. 2013. Guidelines for Systematic Review and Evidence Synthesis in Environmental Management. Version 4.2. Environmental Evidence: http://environmentalevidence.org/wp-content/uploads/2014/06/Review-guidelinesversion-4.2-finalPRINT.pdf
- GEOS6001 Qualitative research and Literature reviews
- UQ Student Support - writing a literature review A guide to the process of planning and writing a literature review.
- Literature reviews guide How to search for, store, organise, evaluate and critique information for your literature review. Includes techniques, books, articles and more to help you do your literature review.
Referencing is a crucial part of successful academic writing and is key to your assignments and research.
- Referencing style guides
- Download and use EndNote How to download EndNote and instructions on setting up EndNote, exporting references from databases, getting EndNote references into Word and troubleshooting issues.
Search for theses - UQ, Australian, UK, North American and other sources .
- << Previous: Research support
- Last Updated: May 7, 2024 12:39 PM
- URL: https://guides.library.uq.edu.au/planning
ORIGINAL RESEARCH article
Urban amenity and urban economic resilience: evidence from china.
- 1 School of Economics, Huazhong University of Science and Technology, Wuhan, Hubei, China
- 2 International Business School, Shaanxi Normal University, Xi'an, Shaanxi, China
Under the influence of multiple uncertain factors at home and abroad, urban amenities, as the underlying support for urban renewal activities, are of great significance in enhancing urban economic resilience. The panel data of Chinese cities from 2011 to 2019 is used in this study. Urban amenity is measured from artificial amenities and climate amenities, respectively. By using a two-way fixed effects model, we empirically test the impact of urban amenities on urban economic resilience. The key findings of this study are as follows. (1) Urban amenities can significantly enhance urban economic resilience. (2) Heterogeneity analysis shows that there are regional differences in the role of urban amenities in promoting urban economic resilience, with cities in the eastern region, strong environmental regulations, and high urbanization rates benefiting more. (3) We further find that urban amenities mainly enhance economic resilience by promoting population agglomeration, attracting labor migration, improving the quality of human capital, and stimulating urban innovation. Our conclusions recommend to rationally allocate and optimize urban amenity resources, strengthen urban planning and construction management, and create a more livable urban environment, thereby enhancing urban economic resilience.
1 Introduction
Amid the complex backdrop of markedly increased internal and external environmental risks and the continued instability of the global economic situation, the Chinese economy is facing unprecedented challenges ( 1 , 2 ). Enhancing economic resilience and ensuring stable economic operations have become critical issues that urgently need to be addressed in the process of achieving sustainable economic development ( 3 ). Urban economic resilience refers to the adaptability and resilience of the economic system of a city in the face of external shocks and changes ( 4 ), and is a concentrated expression of whether a country or region could effectively cope with the uncertainty shocks or resist and resolve economic risks. Improving the economic resilience of cities is conducive to the stability of the urban economic system and the high-quality development of the urban economy and society, which is an important focus of urban construction and development, and an inevitable choice for building a new urban development pattern. However, previous studies on the factors affecting urban economic resilience mainly focus on land, labor and capital ( 5 – 7 ). This model of improving urban economic resilience from the perspective of industrial production ignores the “human” factor and the essential needs of human beings, leading to problems such as urban environmental pollution, traffic congestion, too little public space, and shortage of leisure and entertainment facilities. These consequences could constrain the improvement of urban economic resilience. Particularly, China has experienced a rapid urbanization process, with hundreds of thousands of people moving from the countryside to the cities, which has led to a rapid expansion in the size of cities, and cities are facing great challenges in resource allocation and environmental pollution control ( 8 ). Researching urban amenities and economic resilience helps understand how Chinese cities perform in this dynamic change and contributes to the formulation of sustainable urban development strategies.
The Chinese government pointed out that the level of urban planning, construction and governance should be improved, and urban renewal actions should be carried out to build livable cities. In the context of China's new-type urbanization with “people” as the core and people's increasing expectations for a high-quality living environment, urban amenities and livability have become key elements to attract talents, promote innovation and cultivate emerging industries ( 9 ), and are directly related to the quality of life of urban residents and the efficiency of resource utilization in cities ( 10 ). Therefore, the construction of urban amenities not only concerns the quality of life and welfare of individuals but has also become a new driving force for enhancing the overall competitiveness of cities. It could have a positive impact on the long-term economic resilience of cities. Within the overall framework of urban growth theory, research on urban amenity can effectively address the deficiencies of traditional growth theories in explaining urban economic resilience, aiding city policymakers in the rational planning of urban comfort facilities. This approach could enhance local attractiveness and, consequently, improve long-term economic resilience. However, to date, there is scarce literature that delves into the impact of urban amenity on urban economic resilience from both theoretical and empirical perspectives. Therefore, in the context of increasing economic uncertainty, could enhance urban amenity lead to improved economic resilience in cities? What pathways and mechanisms facilitate this impact? Exploring these questions can expedite urban renewal activities, promote the construction of comfortable and livable urban environments, and provide policy direction and practical guidance for exploring ways to strengthen urban economic resilience.
The remaining part of this paper is organized as follows: Section 2 presents the literature review; Section 3 provides theoretical analysis and research hypotheses; Section 4 outlines the setting of the econometric model and variable explanations; Section 5 presents empirical analysis results; Section 6 concludes the paper and provides research insights.
2 Literature review and theoretical analysis
2.1 literature review.
With the development of information technology in the post-industrial era, cities have become not only carriers for economic benefits but also organic entities that meet the growing needs of residents. In this context, the theory of urban amenity has emerged. Foreign research on urban amenities mainly focuses on the connotation of urban amenities, the evaluation of urban amenities and its realistic value. Amenities are categorized into natural amenities, artificial amenities, and social atmosphere amenities based on the connotation of amenities. The study that urban amenities as pleasant living conditions that could promote population growth and economic growth ( 11 ). Some scholars believe that urban amenity also include various amenities that make residents feel physically and mentally pleased and attract them to live and settle in the city ( 12 ). Rogerson ( 13 ) argued that urban amenities not only enhance the happiness and satisfaction of city residents but also attract investors and innovative talents, serving as a novel external marketing strategy ( 13 ). In the evaluation of amenities, foreign studies mainly measure urban amenities through the following three methods. First, economists generally use housing prices and wage levels to reflect urban amenity, and use hedonic price models to quantify it ( 14 ). Second, a questionnaire is used to investigate the perception of comfort at the individual level ( 15 ). Third, conduct a comprehensive evaluation based on the connotation construction index of amenity items ( 16 , 17 ). In addition, previous research has found that urban amenities can not only provide social value such as culture and art, but also promote urban economic development, thereby generating economic value ( 18 ). Currently, the research on urban amenities in China is mainly based on China's rapid urbanization process and the orientation of urban sustainable development policies, which provides an important research background. Research on urban amenities in China focuses mainly on two aspects: first of all, combined with the reality of China's development, the criteria for the construction of China's amenity system and the dynamic evolution process are proposed ( 19 ); secondly, empirically testing the economic effects of urban amenities on urban development, such as influencing land prices, increasing the degree of industrial agglomeration, and attracting labor inflow ( 20 – 22 ).
As the risks of uncertainty increasingly mount, the study of economic resilience has attracted widespread interest from scholars around the world. Davies explains economic resilience from three dimensions: first, the ability of an economy to withstand external risks and challenges, second, the ability of an economy to recover from negative shocks through self-regulation after the impact, third, the ability to innovate new growth pathways, enhancing the capacity for long-term growth ( 23 , 24 ). Currently, there are various methods for measuring economic resilience, ranging from the sensitivity index method to comprehensive indicator evaluation methods ( 25 – 27 ). Urban economic resilience is thought to be determined by the dynamics of four interacting subsystems: the structural and business subsystem, the labor market subsystem, the financial subsystem, and the governance subsystem ( 4 ). Firstly, in terms of industrial structure and business system, existing scholars mainly study the impact of diversified or specialized industrial structure and technological innovation on economic resilience ( 5 , 28 , 29 ). Secondly, in labor market and financial subsystem, some scholars study the impact of human capital ( 30 ) and digital finance ( 23 , 31 ) on economic resilience. Finally, there are also scholars discussing the influence factors of economic resilience from the perspective of governance such as policy support and political systems ( 32 , 33 ).
Existing theoretical and empirical research on urban amenities and urban economic resilience provides a solid foundation for this paper. However, there are still two shortcomings. Firstly, while current studies have examined the impact of various factors on urban economic resilience, there has been less focus on the influence of urban amenities on urban economic resilience. Therefore, the relationship and impact mechanisms between urban amenities and urban economic resilience require further in-depth research. Secondly, the current methods for measuring different urban amenities levels need improvement. Existing studies often measure urban amenities from the perspective of guaranteeing indicators such as transportation, medical care, and the environment, with less consideration for social factors such as education and culture within the city. In the meanwhile, there is also less focus on the impact of climate amenities on urban economic resilience. Therefore, this paper takes 255 prefecture-level cities nationwide as the research objects, constructs artificial amenities and climate amenities, and studies the impact and internal mechanisms of urban amenities on urban economic resilience.
The marginal contributions of this paper may be reflected in three aspects. Firstly, existing research primarily focuses on the impact of industrial structure ( 6 ), technological innovation ( 5 , 28 ), regional integration ( 7 ), infrastructures ( 34 ), digital finance ( 23 , 31 ), and on economic resilience. Unlike previous studies that mainly focused on economic perspectives in studying economic resilience, this paper starts from the basic needs of “people,” using urban amenities as an entry point to explain how to enhance economic resilience. It supplements relevant studies on factors influencing urban economic resilience.
Secondly, previous studies have often selected indicators focusing on the natural environment and infrastructure to measure urban amenities ( 35 , 36 ), with less emphasis on indicators related to healthcare and transportation. We chose a broader range of suitable indicators to assess the state of urban medical services and transportation infrastructure, thereby more comprehensively measuring urban amenities. Additionally, previous studies mainly used temperature and humidity indexes to study climate amenities ( 36 , 37 ). In our study, based on the national standard for Climatic Suitability Evaluating on Human Settlement formulated by the Chinese government, we measure urban climate amenities more comprehensively, considering factors such as city temperature, humidity, wind speed, and sunlight duration. Thus, our construction of indicators more fully reflects both the artificial and climate amenities of cities, enhancing the accuracy of the indicators.
Thirdly, in terms of research content, we will identify external factors that differentiate the impact of urban amenities construction on urban economic resilience. This paper conducts a heterogeneity analysis from the perspectives of local environmental governance and regional development situations, expanding the directions in which urban amenities exert positive effects and the application scenarios of amenity theory, providing a new development approach for urban development models, offering more targeted support to comprehensively enhance urban economic resilience. Furthermore, from the perspective of urban population aggregation effects and innovation effects, we explore the potential impact mechanisms of amenities construction on urban economic resilience. Through comprehensive analysis of various channels, it helps identify the underlying logical impact.
2.2 Theoretical analysis and research hypotheses
Cities with more amenities can attract highly mobile resources such as capital, technology, and manpower, as well as foreign consumers and investors. This improves the city's competitiveness in acquiring these resources, thereby improving the urban quality and overall competitiveness as a comprehensive consumer product ( 38 ). This is beneficial for strengthening urban economic resilience. In addition, cities that are rich in amenities such as cultural institutions, educational facilities, and leisure spaces generally exhibit higher levels of economic diversification and complexity ( 39 ). The diversity of the economic system can help cities reduce their dependence on a single industry, enhance the city's ability to withstand stress, and enable cities to better cope with the risks of economic uncertainty. In an era of rapid urbanization, urban amenity has received attention for its key role in shaping urban development patterns and enhancing economic resilience.
2.2.1 Urban amenity and population agglomeration effects
Firstly, according to the amenity migration theory, amenities in terms of natural environment, service environment, social culture, etc. are the main reasons for attracting population agglomeration and labor mobility. People tend to choose to move to cities with superior natural environments such as warm winters, cool summers, abundant sunshine, abundant green space and vegetation, and less pollution ( 40 , 41 ). They also tend to move to cities with comfortable service environments such as diverse dining facilities, shopping malls, and efficient transportation infrastructure. At the same time, cities with more amenities develop faster and are more able to attract labor to move in, because cities with high amenity levels not only provide higher economic income but also provide a better quality of life ( 18 ). The process of population agglomeration in high-amenity cities will enhance urban economic resilience. On the one hand, the scale effect caused by population agglomeration can reduce transaction costs and create demand, support stable urban economic growth, and thereby enhance economic resilience. Population agglomeration can bring abundant labor resources to cities, provide enterprises with a variety of labor forces with professional skills, and reduce enterprises' labor search costs ( 29 ). It can also improve the allocation level of factor resources and improve economic efficiency, thereby enhancing urban economic resilience ( 30 ). Population agglomeration can also provide enterprises with a higher degree of talent adaptability, which can effectively avoid sharp changes in enterprise labor demand after economic shocks, quickly realize adaptive production structure adjustments, and thereby achieve sustainable economic resilience. In addition, as the population agglomerates in cities, it will expand the city's internal market demand, prompting industrial entities to produce more products. It will also prompt the government to increase infrastructure construction and improve the level of public services such as education and medical care, thereby reducing the impact of sudden changes in the external environment ( 17 , 30 ). On the other hand, population agglomeration can also produce external effects. Population agglomeration will lead to the expansion and sharing of the labor market, which is conducive to increasing the degree of industrial agglomeration, promoting the refinement of the city's industrial division of labor, and forming an industrial structure system with complementary functions ( 42 ). The diversified industrial agglomeration has the function of an automatic stabilizer, which can effectively enhance urban economic toughness ( 43 ). Thus, urban amenities can increase population aggregation, thereby enhancing urban economic resilience.
Secondly, cities that offer a high quality of life, rich cultural experiences, a good social environment and diverse opportunities tend to attract more highly skilled people ( 44 ). Florida refers to the human capital that generates new technologies, knowledge and art as the “creative class,” which plays a decisive role in the innovative development of cities ( 45 ). Compared with low-skilled labor, high-skill “creative classes” are more sensitive to the living environment and working environment. A more comfortable environment can improve the work satisfaction and comfort of high-skilled talents, improve the efficiency of labor work, and reduce the loss of human capital ( 46 ). Multi-level and multi-skill labor can provide high-quality human capital for the professional industrial chain of the enterprise, and human capital is the key element of regional economic resilience construction ( 47 ). High-quality human capital can accelerate the flow and diffusion of innovative elements, release consumer domestic demand, and stimulate income effects, etc., promote the sustainable development of cities and enterprises ( 48 ). It gives cities the ability to adapt and resist external impact and enhance the urban long-term economic resilience ( 49 ). Therefore, cities with higher comfort standards such as culture and public services can attract the inflow of high-tech talents, and increase the local human capital stock, thereby promoting the high-quality development of cities and enhancing urban economic resilience ( 50 ).
2.2.2 Urban amenity and innovation effects
As mentioned above, urban amenity is a key influencing factor of talent migration. Cities with higher levels of amenities are more able to attract innovative talents, and the comfort migration of talents can enhance urban economic resilience by stimulating innovation ( 51 ). Firstly, high-amenity cities can provide more space and facilities for relieving stress for innovative talents facing high work pressure, helping to create an inclusive and diverse social atmosphere, and providing greater social support for high-risk innovation ( 52 ). Secondly, a pleasant and comfortable environment, along with an innovative atmosphere, contributes to lowering the “talent entry barrier” ( 38 ). It encourages employees to showcase their abilities, enhances the enthusiasm for technological innovation among talents, attracts innovative companies and venture capital institutions, prompts cities to increase policy support for innovation resources, reduces innovation costs for relevant enterprises, and improves overall innovation efficiency. Finally, the concentration of innovative talents with diverse knowledge backgrounds in highly comfortable cities may lead to higher levels of technological spillover. Not only does this enhance the diversity and depth of technology, but it also encourages open and diverse innovative thinking, thereby expanding the boundaries of urban innovation ( 53 ). In addition, well-established infrastructures such as communication facilities can also facilitate technical exchange and information sharing among different cities, creating a diversified pool of technologies and a spillover effect of knowledge. The basis of promoting the efficient integration of traditional factors, drives the emergence of innovative schemes and products, providing businesses with more technological choices and higher-quality technological innovation ( 54 ). Furthermore, the technological innovation of cities also contributes to enhancing the city's economic resilience. On the one hand, innovation can improve the efficiency of resource allocation and the output efficiency of production factors. It can eliminate old production models and expand the scope of use of production factors, thereby deepening specialization, exerting the core driving force of market entities, and thus enhancing economic resilience ( 5 , 55 , 56 ). On the other hand, innovation can drive industrial structural transformation and upgrading, promote the transformation of industries toward rationalization, diversification, and sophistication, improve the overall division of labor and cooperation level of the urban economy, and enable cities to have a strong economic foundation to cope with adverse shocks ( 57 ). Therefore, urban amenities affect the spatial mobility of innovative talents, enhancing the level of urban innovation, and thus forming a city development model of “city amenity attracts talents, talents stimulate innovation, and innovation enhances resilience.”
Based on this, this paper proposes the following hypotheses:
Hypothesis 1: The improvement of urban amenities can enhance the city's economic resilience.
Hypothesis 2: Urban amenities can enhance economic resilience by promoting population agglomeration, attracting labor migration, and increasing the quality of human capital.
Hypothesis 3: Urban amenities can enhance the city's economic resilience by enhancing urban innovation.
In conclusion, the research framework is shown in Figure 1 . It includes impact effects and impact mechanisms.
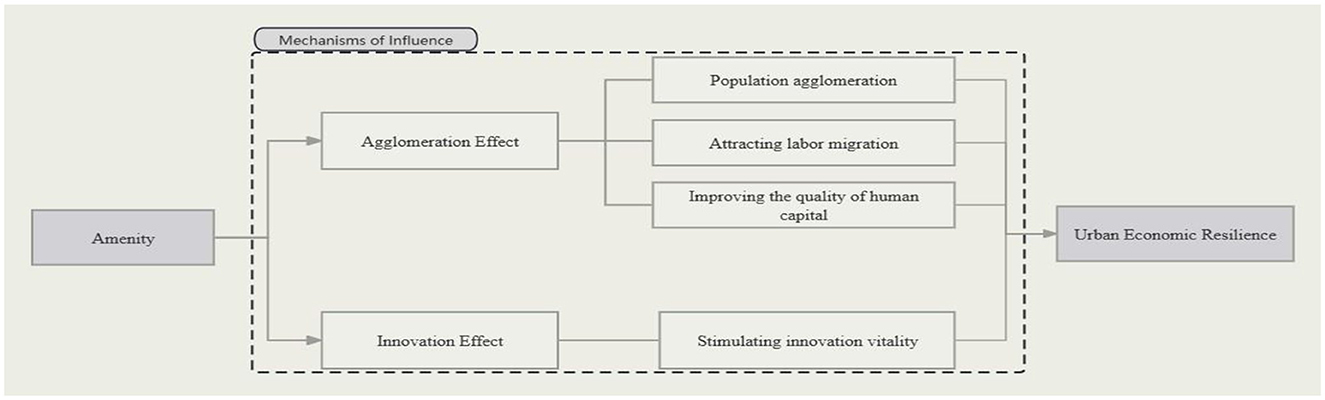
Figure 1 . Influence diagram of the mechanisms by which urban amenity affects urban economic resilience.
3 Methods and data
3.1 empirical model.
We control individual fixed effects and time-fixed effects. Individual fixed effects are used to control unobservable individual characteristic factors that do not change over time at the individual level but affect urban economic resilience. Time-fixed effects are time-characteristic factors that do not change with individuals but affect urban economic resilience. Fixed effects models can reduce endogeneity problems caused by omitted variables ( 58 ). We use Equation 1 to verify the impact of urban amenities on urban economic resilience.
Where i and t represent the city and time, respectively, j represents the j -th control variable; Resilience represents the economic resilience of the city, Amenity represents the level of artificial amenities of the city, X represents control variables, μ i represents city fixed effects, δ t represents time fixed effects, and ε it is the error term.
3.2 Variables
3.2.1 dependent variable.
There are two main methods for measuring urban economic resilience. One is the indicator system method, which uses a series of indicators to measure economic resilience ( 59 , 60 ). However, the indicator method has certain flaws. There is still no recognized reasonable indicator, and it is easy to confuse the causal relationship. The indicators used by researchers may be the reason why cities have economic resilience. Another method is to measure economic sensitivity indicators such as employment and GDP. Considering that the single indicator method has the characteristics of representativeness and continuity, we use this method to measure economic resilience ( 61 ). At the same time, considering that GDP is the core indicator of urban economic development, the sustained growth of urban GDP is the basis for solving a series of problems such as employment and welfare, and can directly reflect the ability of the urban economy to withstand shocks. Therefore, this article draws on Martin's economic sensitivity index and calculates urban economic resilience based on the ratio of the growth rate of urban regional GDP to the growth rate of China's national regional GDP ( 62 ). The city's economic resilience is evaluated by comparing the sensitivity index value with 1. If the sensitivity index is >1, then the city is more resilient and resistant to shocks than the national average, so the city is more resilient.
3.2.2 Independent variable
At present, urban amenity is mainly measured by constructing an index system. Referring to the research of Diamond ( 46 ) and Zhang and Fang ( 20 ), our selects five major categories of indicators: culture, education, medical care, transportation, and environment, and uses the analytic hierarchy process to construct a comprehensive index that reflects urban artificial amenity (Amenity). We first constructed a hierarchical evaluation index system based on the principles of systematicness and availability of indicators. To avoid randomness in indicator selection, we fully draw on previous research and suggestions from experts in related fields. Secondly, use the upper-level indicators as the benchmark, compare each indicator at the same level, construct a judgment matrix, conduct consistency testing, and determine the weight of each indicator. Finally, according to the weighting method, the weight value of all indicators at this level to the previous level is calculated, and the comprehensive weight is obtained layer by layer. Previous literature also used the entropy weight method to calculate index weights, but the entropy weight method will lead to the loss of data information ( 63 ). Therefore, we use the analytic hierarchy process to determine specific weights. The five major categories of indicator data are all from the “China Urban Statistical Yearbook.” Table 1 reports the types and specific descriptions of the five major categories of indicators. We constructed the urban amenity variable based on these indicator systems.
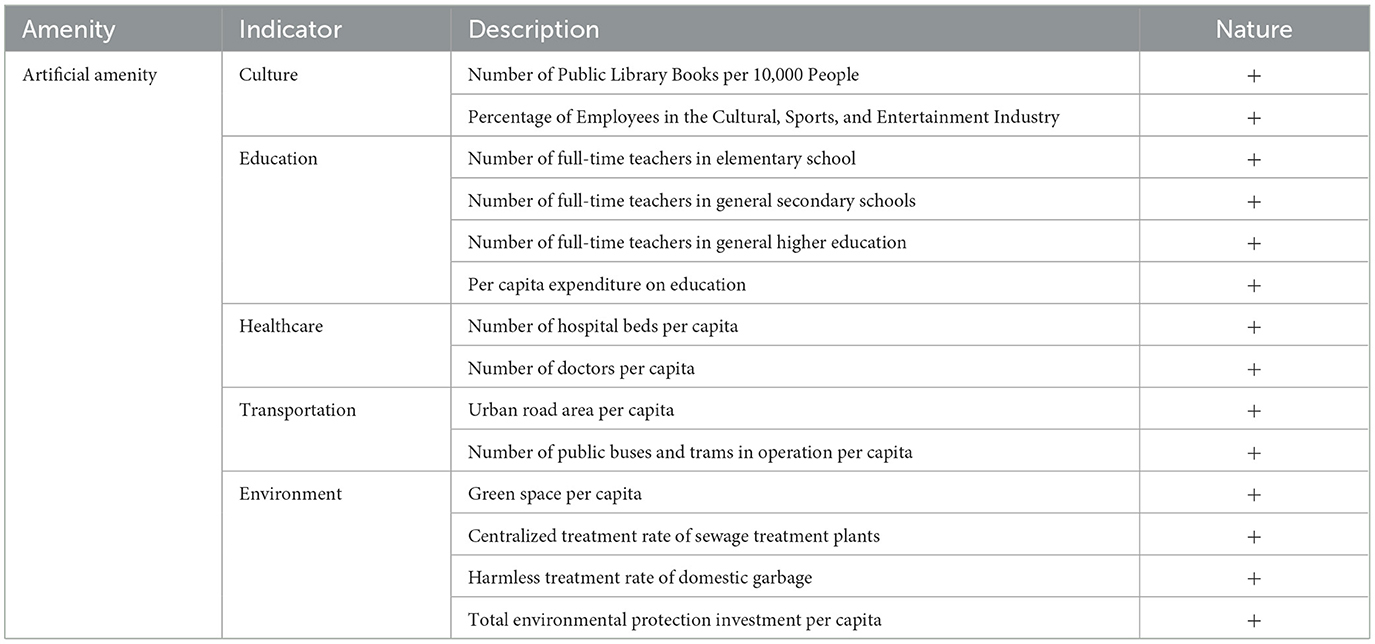
Table 1 . Selection of indicators for principal component analysis of urban artificial amenities.
In the robustness test, we constructed a climate amenity index (Climate) based on the climate amenity evaluation standard for human settlements proposed by the China Meteorological Administration (GB/T 27963-2011) and replaced artificial amenity with climatic amenity as a proxy variable for urban amenity. The steps to construct climate amenities are as follows. The temperature and humidity index I and wind efficiency index K are calculated according to the following formulas (2) and (3), respectively. Based on the availability of data, the wind efficiency index is used in areas where the average wind speed during the evaluation period is >3m/s, otherwise, the temperature and humidity index is used. Finally, to ensure a consistent trend, according to the classification table of human settlement environment amenity levels in Table 2 . Reassign “levels 1 and 5, levels 2 and 4, and level 3” to 1, 2, and 3, that is, the higher the value, the more comfortable the urban climate will be.

Table 2 . Habitat amenities classification table.
We use Equation 2 to calculate the temperature and humidity index. Among them, I represents the temperature and humidity index, T represents the average temperature during the evaluation period, and RH represents the average relative air humidity during the evaluation period.
We use Equation 3 to calculate the wind efficiency index. Among them, K represents the wind efficiency index, T represents the average temperature during the evaluation period, V represents the average wind speed during the evaluation period, and S represents average sunshine hours during the evaluation period. We construct an urban climate amenity index from this standard design.
3.2.3 Control variables
When conducting empirical analysis, we refer to the research of Xu and Deng ( 56 ), Feng et al. ( 7 ), and Zhang et al. ( 64 ), and select economic scale, financial development level, fixed asset scale, industrial structure, fiscal expenditure and urban freight carrying capacity as control variables. 1. Economic scale (GDP). Cities with larger economies have stronger economic bases and resources in multiple dimensions, making them better able to adapt and respond to adverse economic conditions. This article uses the logarithm of GDP to measure the economic size of a city. 2. Financial development level (Finance). Cities with high financing potential are often able to attract more resources and investments, which can help cities build more resilient economies. We measure it by the ratio of deposit balances of financial institutions to GDP at the end of the year. 3. Scale of fixed assets (Investment). The scale of fixed assets is measured by the ratio of the city's total fixed assets to GDP. 4. Industrial structure (Structure). A diversified industrial structure can not only disperse the risks of economic shocks in a short period but also promote regional innovation and new technology innovation, allowing cities to adapt to resource reorganization and structural transformation and adjustment after the crisis. 5. Financial expenditure (Expenditure). Fiscal spending can improve economic resilience by stimulating demand, building infrastructure, and providing social protection. The city's financial expenditure is measured as the logarithm of the city's general fiscal budget expenditure. 6. Urban freight carrying capacity (Freight). The city's transportation infrastructure helps the city resist the impact of economic risks. The city's freight carrying capacity is measured by the logarithm of highway freight volume.
3.2.4 Mechanism variables
We select the following variables as mechanism variables. 1. Population aggregation (Population). We measure the degree of population agglomeration using the logarithm of urban population density. 2. Labor supply and human capital. Labor supply (Labor) is measured by the ratio of the number of urban employees to the total population at the end of the year. Human capital stock (Humancap) is measured by the number of students in school. 3. Urban innovation. This article uses the following two indicators to evaluate urban innovation: the number of urban patent applications (Patent) and the level of R&D investment (R&D). The level of urban R&D investment is measured by the ratio of urban science education expenditure to GDP.
Considering the completeness of the data and the impact of public events, our study selects 255 cities in China from 2011 to 2019 as research samples. All macro-level data of cities are sourced from the “China Urban Statistical Yearbook.” The climate amenities indicators selected in this study include variables such as average temperature, average relative humidity, average wind speed, and average hours of sunshine for each city. The original meteorological data are obtained from the China Surface Climate Data Daily Value dataset (V3.0). We refer to the design method of Deschênes and Greenstone ( 65 ). We use the inverse distance weighted interpolation method (IDW) to interpolate daily meteorological data into grid data, and then obtain annual meteorological data for each district and county. To eliminate the influence of extreme values, all continuous variable data are trimmed by 1% above and below.
3.4 Probability density plot and descriptive statistics
Figures 2 , 3 respectively present the three-dimensional probability distribution diagrams of urban resilience and amenity of Chinese cities over the years. From Figure 2 , it can be observed that the dispersion and right-skewness of the amenity probability distribution in Chinese cities are increasing year by year, the right tail is lengthening year by year, and the range is gradually expanding. The reason is that although the resilience values in the high quantile increase year by year, the values in the middle and low quantiles do not increase significantly, resulting in the gradual widening of the spatial gap in amenity among Chinese cities. From Figure 3 , we can understand that the overall distribution of urban resilience in China fluctuates violently over the years, and the concentration ratio shows an “S”-shaped trend of first declining, then rising, and then falling again. Overall, there has been no significant improvement. Compared with other years, China's urban resilience was generally low in 2011, while in 2017 China's urban resilience was generally high. This may be due to the impact of the global financial crisis in 2008. China's resilience had not yet fully recovered in 2011. After 2015, China's implementation of supply-side structural reforms revitalized urban resilience. Secondly, we can also find that the spatial differences in urban resilience in 2019 are large, which may be due to the severe differences in urban resilience in China due to the Sino-US trade dispute in 2018. The above characteristics show that China's urban resilience still has room for optimization and improvement.
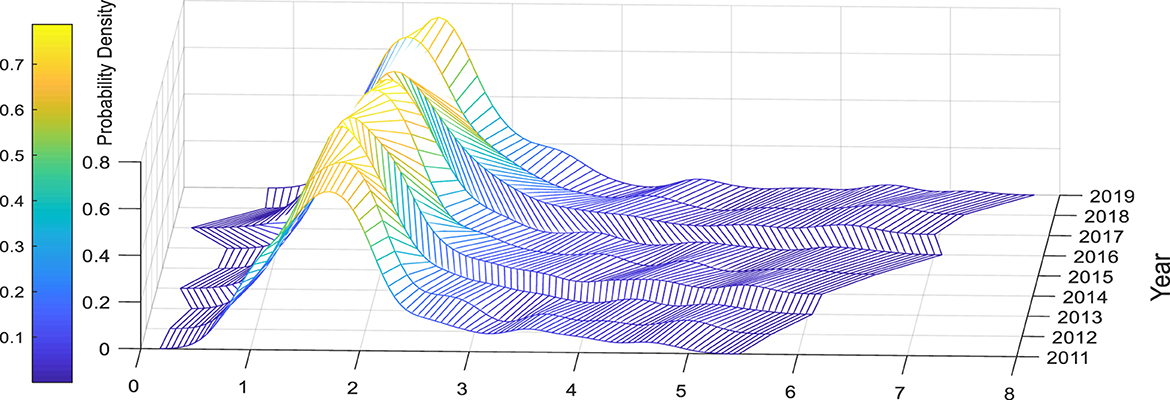
Figure 2 . Probability density plot of amenity.
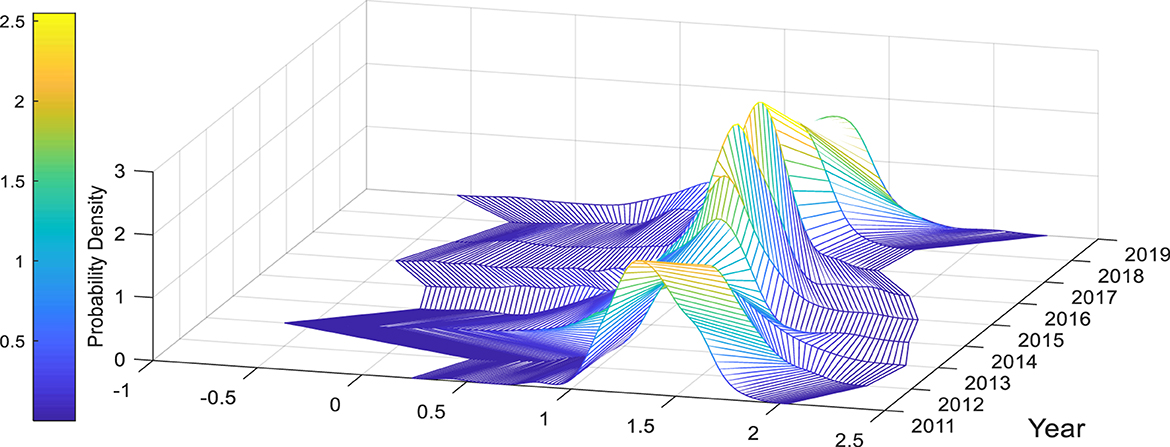
Figure 3 . Probability density plot of resilience.
Descriptive statistics of variables are presented in Table 3 . The mean value of resilience is 1.198, that is, the majority of urban economies demonstrate resilience. In addition, the average value of urban amenity is 2.295, the minimum value is 0.769, and the maximum value is 7.152, indicating that there is a significant difference in amenity levels in various cities.
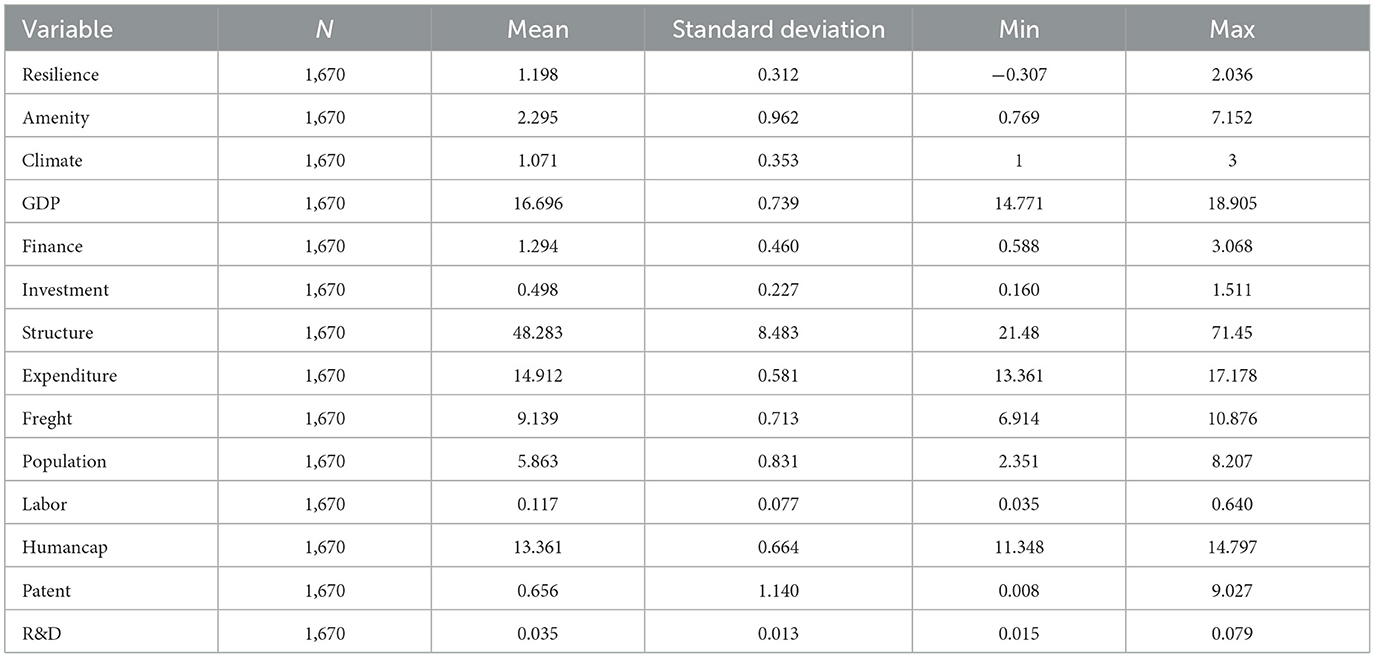
Table 3 . Descriptive statistics.
4 Empirical results analysis
4.1 baseline regression results.
The baseline regression results are shown in Table 4 . Column (1) only includes core explanatory variables, and the results show that the improvement of urban amenities can significantly increase the economic resilience of a region. When control variables, city fixed effects, and year fixed effects are added in sequence from column (2) to column (5), the goodness of fit of the model is significantly improved. At the same time, the test results all show that the impact of urban amenities on urban economic resilience is significantly positive. As can be seen from column (5) in Table 4 , after controlling for other factors, every 1-unit increase in urban amenities will increase urban economic resilience by 0.091 units. This result shows that the improvement of urban amenities can significantly enhance urban economic resilience. Economic resilience. Hypothesis 1 was verified.
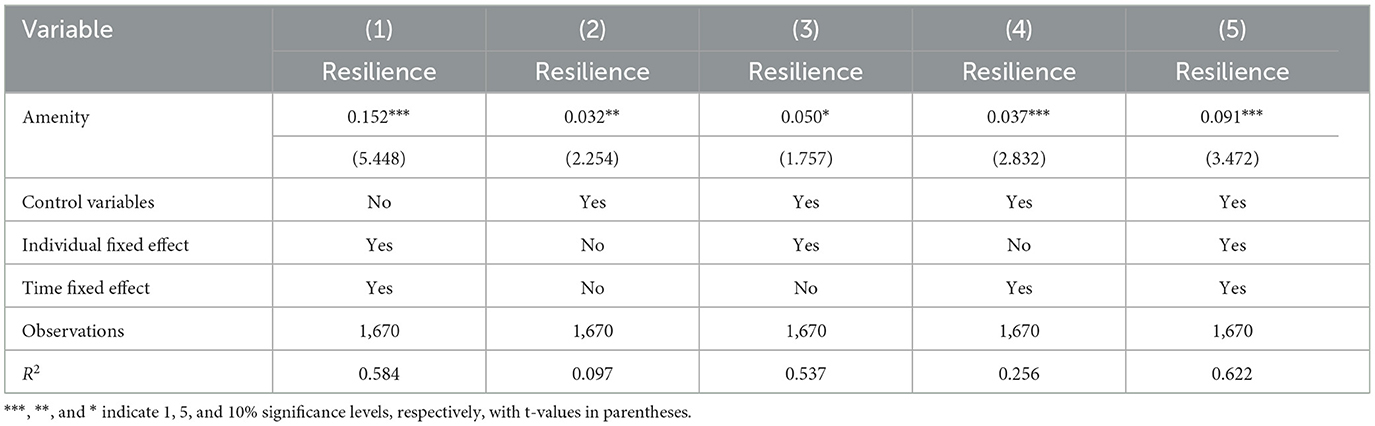
Table 4 . Baseline regression results.
4.2 Robustness test
4.2.1 replacing the dependent variable.
In the robustness test, we use two alternative indicators to measure the economic resilience of cities. The first method follows the research of Tan et al. ( 66 ), using China's annual actual GDP growth rate as the counterfactual basis for urban development, then calculating the difference between each city's annual actual GDP growth rate and this counterfactual basis, and using this difference to reflect the city's economic resilience level. This difference is used to construct a sensitivity index reflecting the level of economic resilience of cities. We put the sensitivity index into the benchmark model for regression, and the regression results are shown in column (1) of Table 5 . The second method draws on the relevant research by Chen ( 67 ), using the regional employment sensitivity index to measure China's economic resilience and perform regression using the ratio between the change rate of urban employment and the change rate of national employment as the proxy explained variable. Regression is conducted with the ratio of the change rate of urban employment to the change rate of national employment as the proxy for the explained variable. The regression results are shown in column (2) of Table 5 . The results all show that urban amenities can improve the economic resilience of the city, validating the baseline regression results.
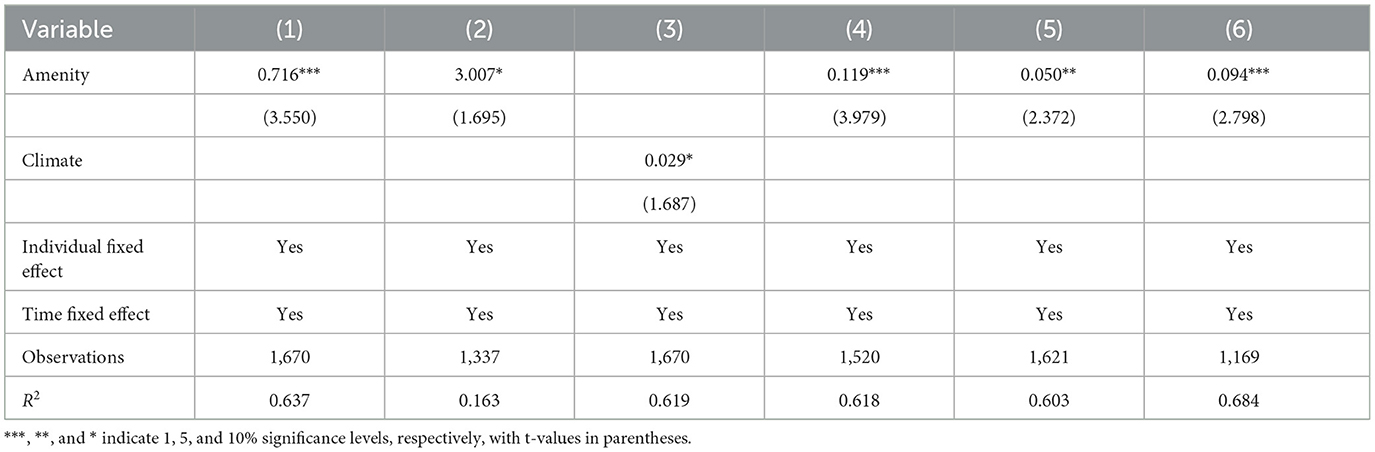
Table 5 . Robustness test.
4.2.2 Replacing the independent variable
This study replaces urban artificial amenity with the climate amenity index. The empirical results are shown in column (3) of Table 5 . The results show that climate amenities can significantly improve a city's economic resilience. The possible reason is that improved climate amenities are more conducive to attracting population agglomeration and business investment. Cities with a pleasant climate are usually rich in natural resources and ecosystem services, which not only provide urban residents with natural places for leisure and entertainment but also help improve their health and quality of life. High-quality ecosystem services can also attract more tourists and businesses, promoting the vigorous development of tourism and cultural industries. In addition, the improvement of investment attractiveness will help promote the city's infrastructure construction and industrial diversity development, thereby improving the city's overall economic level and enhancing the city's economic resilience.
4.2.3 Excluding capital cities samples
As the administrative center of a province, provincial cities usually receive more government support and often have stronger resource attraction. These cities have large populations, high levels of economic development, complete infrastructure, and resource advantages in the construction of urban amenities. The interaction of these factors helps to improve the ability of provincial capital cities to cope with challenges and uncertainties, so the economic resilience of provincial capital cities may be stronger. Therefore, compared with non-provincial capital cities, provincial capital cities have a certain “siphon effect” in terms of resource acquisition and policy support, which may have an impact on the empirical results. To improve the credibility of the research conclusions, the empirical analysis was re-conducted after deleting provincial capital city data from all samples. Column (4) of Table 5 reports the results of regression on all non-provincial capital city samples after excluding provincial capital cities. A one-unit increase in urban amenities is associated with a 0.119-unit increase in economic resilience for non-capital cities. After provincial capital cities are eliminated, non-provincial capital cities without excess resources can also improve urban economic resilience through the construction of amenities. The results show that the impact of urban amenities in non-provincial capital cities on the city's economic resilience is still significantly positive, and the baseline regression is robust.
4.2.4 Different samples and variable selection
We further select different samples and variables for robustness testing. Firstly, to further reduce the impact of extreme values on model estimation, the samples are winsorized at the 5% level, and the results are shown in column (5) of Table 5 . Robustness results show that the core conclusions of this article still hold. Secondly, industrial agglomeration usually leads to the concentration of resources such as technology, talents, and raw materials, thereby forming a diversified industrial chain and economies of scale, which in turn helps the city respond to market changes and shocks and improve the city's economic resilience. In addition, FDI can introduce new technology and management experience and attract large amounts of capital investment, which is also important for the stable growth of the urban economy. Therefore, we add the proxy variables of industrial agglomeration and FDI to the control variables. This article selects the industry Herfindahl index to measure the degree of industrial agglomeration and uses the proportion of the total output value of foreign-invested enterprises in GDP to measure FDI. The results in column (6) of Table 5 show that after controlling for industrial agglomeration and FDI, the results are still significant.
4.2.5 Endogeneity discussion
To reduce the problem of biased estimation results caused by reverse causality and omitted variables between urban amenities and economic resilience, this paper uses the instrumental variable method for empirical analysis. Drawing on the research of Xu and Deng ( 56 ), urban terrain slope was selected as the instrumental variable. First of all, terrain slope will have a significant impact on the construction form and investment costs of urban infrastructure such as roads, bridges, and parks. It also affects China's population distribution and labor concentration. The layout of infrastructure and population distribution are closely related to urban amenities. Therefore, there is a correlation between urban amenity and urban terrain slope, which meets the assumption of correlation between endogenous variables and instrumental variables. Secondly, the terrain slope is a naturally formed geographical information variable in the city. It has relatively natural exogenous characteristics and does not directly affect the current economic development level and resilience level of the city. It satisfies the homogeneity assumption of instrumental variables.
However, terrain slope is cross-sectional data in the data dimension and does not change with time, which will result in the inability to control the individual effects of cities in empirical regression. Secondly, the impact of the urban slope index on urban amenities may also change over time, and appropriate instrumental variables need to take into account this difference in time dimension. In addition, consider that urban wind speed may affect urban amenities and urban wind speed is determined by large-scale weather systems. Therefore, it is an exogenous factor in local economic activity. To this end, we multiplied the urban terrain slope (Slope) and the urban wind speed (Wind) and took the logarithm to construct an instrumental variable with a time effect.
Columns (1) and (2) of Table 6 are the instrumental variable regression results after adding only core explanatory variables, and columns (3) and (4) are the instrumental variable regression results after adding control variables. As can be seen from Table 6 , the F values in the first stage are all >10, indicating that the instrumental variables and endogenous variables are related, eliminating the problem of weak instrumental variables. It can be seen from the regression results of the second stage that the coefficient of urban amenity is still significantly positive. This result is consistent with the baseline regression results and verifies the improvement effect of urban amenities on urban economic resilience.
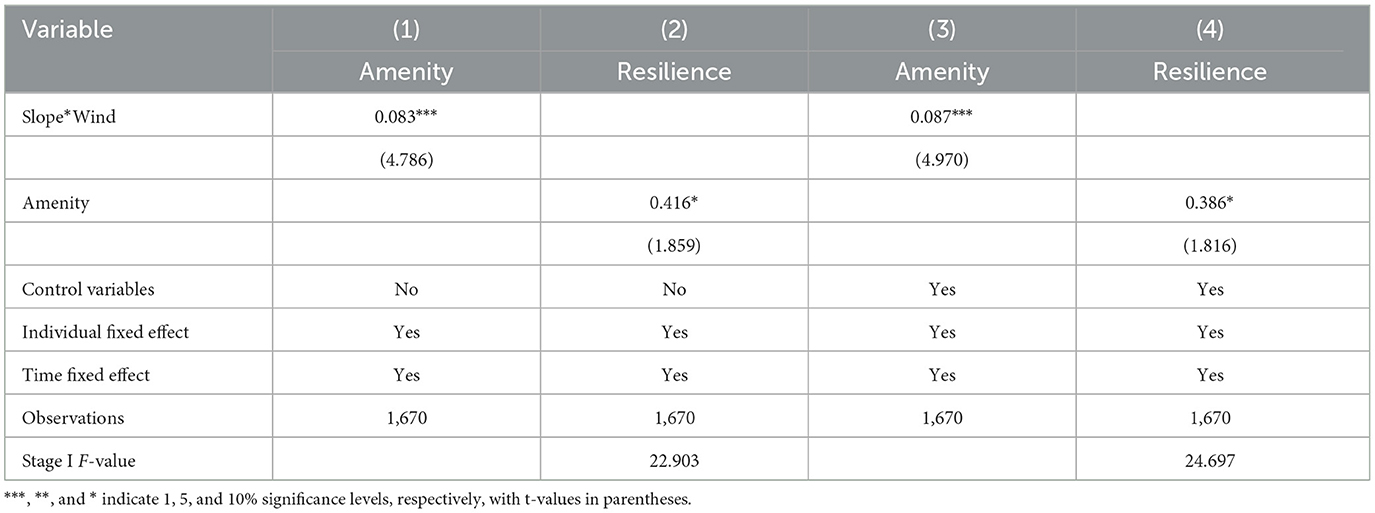
Table 6 . Instrumental variable analysis.
4.3 Heterogeneity tests
4.3.1 regional heterogeneity.
Due to geographical differences, preferential policies, and other reasons, China's urban amenity construction and economic development levels have obvious regional differences. Therefore, there may also be differences in the role of urban amenities in promoting urban economic resilience. We divide the sample into eastern central and western cities for group regression. As shown in columns (1) and (2) of Table 7 , for eastern cities, the improvement of urban amenities can significantly enhance urban economic resilience. The possible reason is that eastern cities usually have high population density, large market size, better infrastructure, and richer development resources. Secondly, eastern cities also have advantages in terms of openness and policy support, which results in the level of technology accumulation and the number of high-quality talents in eastern cities being far superior to that in central and western regions. Therefore, the more eastern cities can create a comfortable living environment through a series of policy measures, thereby enhancing urban economic resilience. The central and western regions are faced with problems such as weak economic foundations, lagging infrastructure construction, and serious population loss, which make them face relatively greater difficulties in improving economic resilience through urban amenity construction.
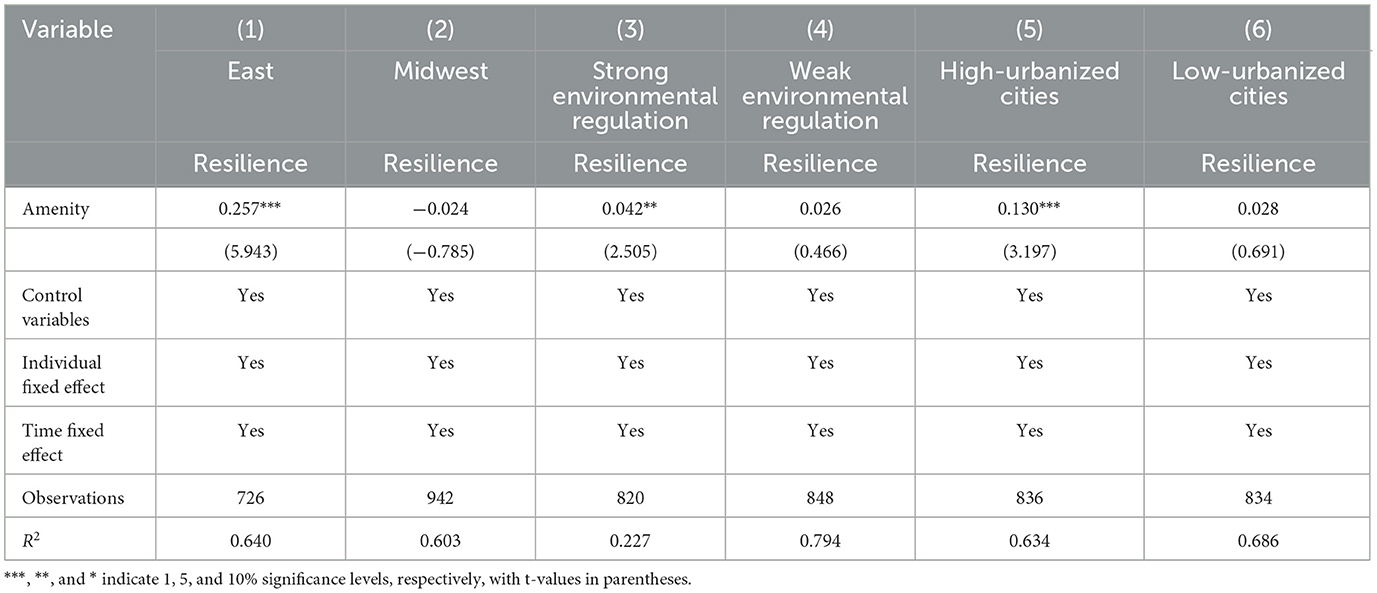
Table 7 . Heterogeneity test.
4.3.2 Environmental regulation
Environmental regulation, as a means of government environmental governance, can effectively reduce environmental pollution problems, improve environmental quality, and enhance urban amenities. In addition, environmental regulatory policies can promote the growth of economic resilience by improving the level of urban technological innovation and promoting urban green transformation. Therefore, areas with strong environmental regulations may enhance the role of urban amenities in promoting urban economic resilience. Referring to the research design method of Chen and Chen ( 68 ), we selected the frequency of words related to environmental regulation in the “Government Work Report” of the prefecture-level city that year to measure the intensity of the city's environmental regulation. We grouped each city according to the median frequency of annual environmental regulation words and obtained the group with high environmental regulation intensity and the group with low environmental regulation intensity. Finally, the group regression analysis results are shown in columns (3) to (4) of Table 7 .
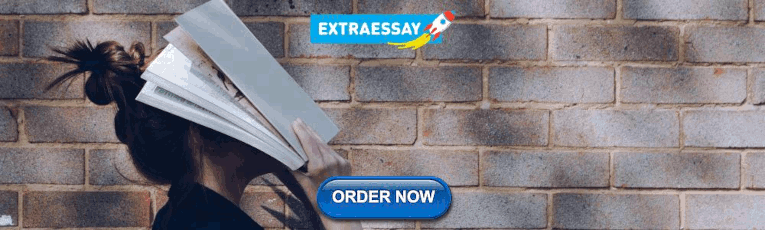
4.3.3 Degree of urbanization
There are significant differences in urbanization rates among different cities, which leads to diversity in the development patterns and speeds of various cities. We divide cities into cities with high urbanization rates and cities with low urbanization rates according to the median urbanization rate of each city. The results are shown in columns (5) and (6) of Table 7 . The results show that when a city's urbanization rate is high, improvements in urban amenities will significantly enhance urban economic resilience. The possible reason is that the urbanization process is usually accompanied by the construction of infrastructure and the optimization of public services, including public transportation, public facilities, education, medical, and community services. High-quality infrastructure and public services can significantly improve the quality of life and satisfaction of urban residents, thereby enhancing the amenities of the city, promoting the development of the urban economy, and improving economic resilience. When the urbanization rate of a city is low, the concentration of production factors is usually relatively low, which may affect the improvement of economic benefits. At the same time, these cities have deficiencies in infrastructure construction and public service provision. Its ability to withstand various risks may also be weaker, affecting the city's economic resilience. Therefore, the urbanization process plays an important supporting role in improving urban amenity and economic resilience.
4.4 Mechanism analysis
According to the aforementioned theoretical analysis, urban amenities may have a significant impact on regional factor supply and resource allocation efficiency. High urban amenity means that the city has good living conditions, employment opportunities, educational resources, medical services, etc. These will increase people's expectations for the quality of life and happiness after migration, thereby increasing their utility expectations of migration. At the same time, areas with high urban amenities can attract high-quality talents in different fields or at different levels, further improving the quality of labor supply and improving the city's human capital level. Therefore, cities with high urban amenities can attract more population inflows and increase the quantity and quality of labor supply. In addition, cities with high urban amenities can also promote technological innovation and increase the supply of intellectual capital. Innovation is an important driving force for economic growth and a key factor in improving production efficiency and competitiveness. Therefore, high urban amenity means that the city has a good innovation atmosphere, scientific research institutions, talent training systems, knowledge exchange channels, etc. These will enhance the effects of population agglomeration and innovation, thereby enhancing urban economic resilience.
To test the channel through which urban amenity affects urban economic resilience, we take urban amenity as the core explanatory variable and replace the explained variables of the model (1) with the degree of population agglomeration, the number of labor forces, human capital, and urban innovation. This model is used to examine the impact of urban amenity on mechanism variables. Columns (1) to (5) of Table 8 respectively verify that the degree of population agglomeration, the number of labor force, human capital, innovation patents, and innovation investment are the paths through which urban amenity affects urban economic resilience. In conclusion, cities with high levels of amenities have a higher quality of life, more labor and high-quality talent inflows, and a stronger innovation atmosphere, which have a positive effect on urban economic resilience. The above analysis has verified to a certain extent the impact mechanism of urban amenities on urban economic resilience.
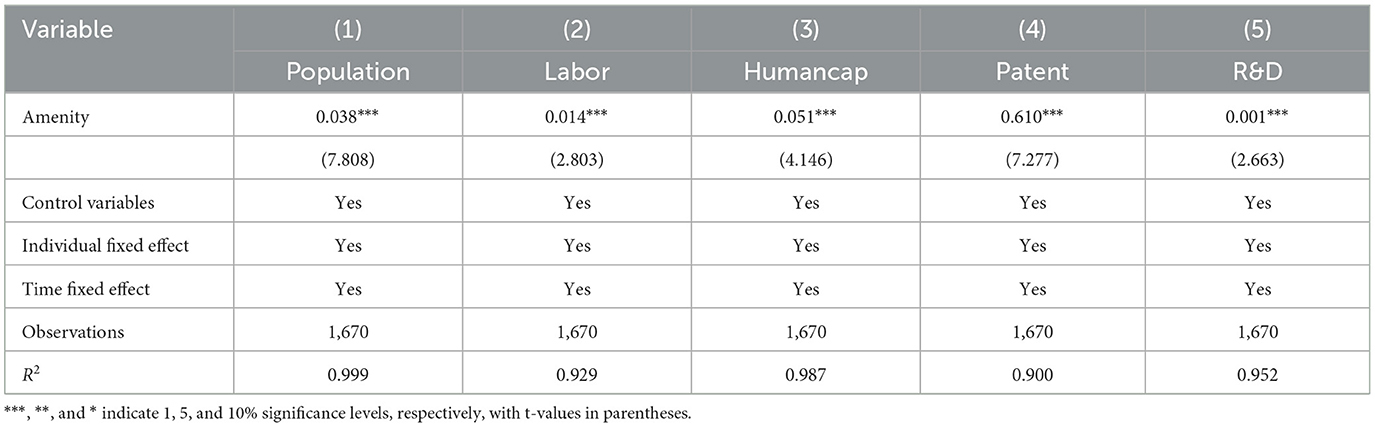
Table 8 . Mechanism analysis.
5 Discussion
We analyze the relationship between urban amenities and urban economic resilience. Our empirical evidence suggests that urban amenities significantly contribute to enhancing urban economic resilience. This aligns with prior research emphasizing the various benefits of amenities in urban sustainable development ( 16 , 21 , 69 ). Urban amenities, encompassing factors such as green spaces, cultural attractions, and public infrastructure, not only enhance the quality of life for residents but also attract investments, stimulate economic activity, and promote sustainable development. The presence of green spaces, for instance, not only enhances environmental sustainability but also fosters community wellbeing and social cohesion, thereby contributing to enhancing urban economic resilience ( 70 ). Similarly, investments in cultural institutions and recreational facilities not only enrich the urban experience but also promote economic development through tourism, cultural events, and creative industries ( 71 ). In particular, we found that the population agglomeration effect and innovation effect are the influencing mechanisms through which urban amenity improves urban economic resilience. Population agglomeration and innovation effects play a very important role in enhancing the economic resilience of urban areas ( 64 , 67 ). Concentration and innovation of population facilitate economies of scale, resource sharing, and enhanced labor market efficiency, all of which contribute to the resilience of local economies. In conclusion, investing in the development and maintenance of urban amenities emerges as a strategic approach to improving economic resilience.
The regional disparities in the impact of urban amenities on economic resilience are noteworthy. Previous studies find that unreasonable investments in amenities in poor regions could exacerbate socioeconomic inequalities, thereby leading to the exclusion of underprivileged residents from benefiting from urban amenities and displacing long-standing communities ( 72 ). This highlights the importance of tailoring urban development strategies to regional contexts. Similar to previous studies, we find that in more economically developed areas such as the East, the construction of infrastructure and other amenities is more conducive to promoting urban economic development ( 73 , 74 ). Economically developed regions tend to have more diversified economies, meaning they are not solely reliant on one industry. Building amenities can contribute to this diversification by attracting different types of businesses and residents. For instance, a city with a vibrant arts scene may appeal to creative professionals, while excellent recreational opportunities may attract outdoor enthusiasts or retirees. This diversity can help protect the city against economic shocks. In addition, we also found that the stronger the urban environmental regulation, the more conducive it is to enhancing economic resilience through the construction of amenities. Environmental regulations targeting climate change mitigation and adaptation measures can enhance urban resilience to cope with extreme weather events and other economic-related challenges. Investments in green infrastructure, such as flood protection systems and sustainable urban planning, can minimize economic disruptions caused by environmental disasters ( 75 ). Therefore, policymakers should consider local conditions, socio-economic dynamics, and environmental factors when planning and allocating resources for urban amenities.
Previous research shows urban growth and economic development have not coincided with urban resilience policies, plans, and practices ( 76 ). Urbanization, especially in developing nations, is often characterized by rapid expansion and resource-intensive development aimed at bolstering economic growth. However, this growth frequently occurs without adequate consideration for the resilience of urban systems to withstand various shocks, ranging from environmental disasters to economic downturns. Consequently, cities may become more susceptible to disruptions, thereby impeding sustained economic progress. Our research underscores the significance of integrating resilience-focused approaches into urban development strategies, particularly through the adoption of suitable amenities. By investing in infrastructural solutions that prioritize both economic growth and economic resilience, cities can better withstand and recover from adverse events while fostering long-term prosperity. In conclusion, our study underscores the importance of aligning urban development efforts with economic resilience principles to promote sustainable economic growth in developing countries. By the construction of rational and moderate amenities, cities can enhance their capacity to withstand and recover from challenges, ultimately fostering more resilient and prosperous urban environments.
6 Conclusion
At this stage, economic uncertainty is becoming normalized. Increased economic uncertainty may increase market risks and trigger economic turmoil, which will have a profound impact on urban economic development. Therefore, how to deal with economic uncertainty and improve the economic resilience of cities has become an important current research topic. This is related to the efficiency of economic operation and the strategic goal of high-quality development of the Chinese economy. At the same time, urban amenity is an important indicator to measure people's happiness. The construction of urban amenities is an important task that conforms to the laws of urban development and the requirements of the times and reflects the comprehensive strength of the city. This is not only an effective way to deal with economic uncertainty, but also an important measure to achieve high-quality urban development. After measuring the city's artificial amenities and climate amenities, we conducted an empirical analysis based on China's urban panel data from 2011 to 2019, tested the impact of urban amenities on urban economic resilience, and came to the following empirical conclusions. Firstly, whether urban amenity is measured in terms of artificial amenities or climate comfort, cities with more amenity show stronger economic resilience when facing external shocks. Secondly, the impact of urban amenity on urban economic resilience shows regional differences. Compared with central and western cities, eastern cities can significantly enhance the city's economic resilience by improving their amenity. At the same time, the greater the intensity of environmental regulation and the higher the degree of urbanization in a city, the stronger the role of urban amenities in promoting economic resilience. Finally, we find that urban amenity affects economic resilience mainly through mechanisms such as population agglomeration, labor migration, improving the quality of human capital, and stimulating innovation vitality.
In light of the above conclusions, we propose the following policy recommendations: First, we suggest strengthening the incentives for local governments to build livable cities and, based on urban development goals and residents' needs, conduct reasonable allocation and optimization of amenity resources. The government should enhance residents' awareness of participation in urban governance, establish surveys on urban residents' satisfaction with livability, and incorporate urban livability satisfaction into the assessment and evaluation of government performance. Additionally, the government should delve deeply into and make good use of urban cultural resources, creating distinctive cultural blocks and cultural industry parks, among others. In the meanwhile, it should improve the allocation of urban land resources, dedicating more land to the construction of parks, green spaces, sports, and fitness facilities, and other public spaces. Lastly, according to the climate characteristics of different cities, the government should actively create green development space, optimize the urban heat island effect, strengthen environmental governance and ecological restoration, in particular, strictly implement the discharge standards of air pollutants and urban sewage and environmental protection policies, improves the quality of air and water resources, and improves the urban climate environment.
Second, considering the differences in natural conditions and the level of economic and social development between different regions, the government should promote the construction of urban amenities in a manner tailored to local conditions. In the eastern regions and cities with higher urbanization rates, it's crucial to fully leverage the advantages of factor agglomeration and location. The focus should be on improving urban environmental quality and public service levels. By intensifying environmental governance, advancing green and low-carbon development, optimizing urban spatial structure, and enhancing urban management efficiency, the goal should be to create ecologically livable, modern, resilient cities. In addition, the government should pay attention to the regional balance of the allocation of amenity resources, improve the financial transfer payment system, and increase the tilt of amenity resources in the central and western regions and cities with low urbanization rates. Governments at all levels should improve the resource scheduling mechanism for amenity construction and increase special support for these areas such as funds. In the meanwhile, the government should promote the central and western regions and cities with low urbanization rates to invest appropriately in infrastructure construction according to the needs of the population, strengthen the development of the value of natural resources, and foster tourism, leisure, and health care industries. The government should narrow the differences in education, medical, and other resources between regions, and strengthen exchanges between cities in school management, curriculum education, and student training. In particular, it should solve the problem of remote medical care and difficult access to medical care, guide the rational flow of the population, and thus enhance the economic resilience of regional cities.
Third, the government should improve the overall environmental quality of the city, attract population and capital inflows, and stimulate the quality of urban innovation. First and foremost, the government should prioritize human-centric improvements in urban public services and infrastructure, enhance urban green spaces, air quality, and other environmental aspects, foster a shared local culture, and judiciously promote unique cultural products in crafts and cuisine to fully accommodate the diverse needs of various demographics and activities. Concurrently, it should establish a tiered housing system to enhance living comfort, expedite the development of affordable housing, and bolster community governance capabilities, all aimed at forging a conducive environment for work, living, leisure, and travel, thereby attracting populations and labor migration. Moreover, the government should ease household registration constraints, diminish labor mobility barriers, and fortify the urban social welfare system, crafting an open, inclusive, and diverse social milieu. Lastly, the government ought to underscore the significance and timing of constructing various amenities, forge an inclusive environment for innovative talents, and high-caliber research platforms, actively recruit and nurture top-tier talent, bolster the growth of diverse innovative bodies, and facilitate the translation and deployment of scientific and technological advancements to fortify urban economic resilience.
The shortcomings and future development ideas of this paper are as follows: First of all, we mainly use objective index data at the city level. Future studies could further consider the use of subjective evaluation index data of urban residents on the amenity level or individual characteristics data at the micro level, and use Hedonic or other models to estimate the implied price of urban comfort attributes and improve the comfort index construction system. Secondly, the measure of economic resilience in this paper has limitations in the selection of variables and the evaluation of effects and lacks the dynamic evaluation of the time dimension. In the future, multiple macroeconomic indicators and time-varying impulse response functions could be selected and used to measure urban economic resilience, to provide ideas for measuring macroeconomic resilience from a dynamic perspective.
Data availability statement
The original contributions presented in the study are included in the article/ supplementary material, further inquiries can be directed to the corresponding author.
Author contributions
RD: Conceptualization, Formal analysis, Funding acquisition, Software, Writing – original draft, Writing – review & editing. KL: Writing – original draft, Writing – review & editing. DZ: Formal analysis, Software, Writing – review & editing. QF: Conceptualization, Writing – review & editing.
The author(s) declare that financial support was received for the research, authorship, and/or publication of this article. This work was supported by “the Fundamental Research Funds for the Central Universities” (Grant No. YCJJ20230686).
Conflict of interest
The authors declare that the research was conducted in the absence of any commercial or financial relationships that could be construed as a potential conflict of interest.
Publisher's note
All claims expressed in this article are solely those of the authors and do not necessarily represent those of their affiliated organizations, or those of the publisher, the editors and the reviewers. Any product that may be evaluated in this article, or claim that may be made by its manufacturer, is not guaranteed or endorsed by the publisher.
1. Fan F, Wen X, Feng Z, Gao Y, Li W. Optimizing urban ecological space based on the scenario of ecological security patterns: the case of central Wuhan, China. Appl Geogr. (2022) 138:102619. doi: 10.1016/j.apgeog.2021.102619
Crossref Full Text | Google Scholar
2. Zhang YY, Zheng Q, Wang H. Challenges and opportunities facing the Chinese economy in the new decade: epidemics, food, labor, E-commerce, and trade. Chin Econ. (2021) 54:373–5. doi: 10.1080/10971475.2021.1890355
3. Hu X, Li L, Dong K. What matters for regional economic resilience amid COVID-19? Evidence from cities in Northeast China. Cities. (2022) 120:103440. doi: 10.1016/j.cities.2021.103440
PubMed Abstract | Crossref Full Text | Google Scholar
4. Martin R, Sunley P. On the notion of regional economic resilience: conceptualization and explanation. J Econ Geogr. (2015) 15:1–42. doi: 10.1093/jeg/lbu015
5. Bristow G, Healy A. Innovation and regional economic resilience: an exploratory analysis. Ann Reg Sci. (2018) 60:265–84. doi: 10.1007/s00168-017-0841-6
6. Duan W, Madasi JD, Khurshid A, Ma D. Industrial structure conditions economic resilience. Technol Forecast Soc Change. (2022) 183:121944. doi: 10.1016/j.techfore.2022.121944
7. Feng Y, Lee CC, Peng D. Does regional integration improve economic resilience? Evidence from urban agglomerations in China. Sustain Cities Soc. (2023) 88:104273. doi: 10.1016/j.scs.2022.104273
8. Zhang Y, Chen X, Mao Y, Shuai C, Jiao L, Wu Y. Analysis of resource allocation and PM25 pollution control efficiency: evidence from 112 Chinese cities. Ecol Indic. (2021) 127:107705. doi: 10.1016/j.ecolind.2021.107705
9. Florek M, Herezniak M, Augustyn A. Measuring the effectiveness of city brand strategy. In search for a universal evaluative framework. Cities. (2021) 110:103079. doi: 10.1016/j.cities.2020.103079
10. Shi T, Zhu W, Fu S. Quality of life in Chinese cities. China Econ Rev. (2021) 69:101682. doi: 10.1016/j.chieco.2021.101682
11. Li H, Wei YD, Wu Y. Urban amenity, human capital and employment distribution in Shanghai. Habitat Int. (2019) 91:102025. doi: 10.1016/j.habitatint.2019.102025
12. Glaeser EL, Kolko J, Saiz A. Consumer city. J Econ Geogr. (2001) 1:27–50. doi: 10.1093/jeg/1.1.27
13. Rogerson R. Quality of life and city competitiveness. Urban Stud. (1999) 36:42098993303. doi: 10.1080/0042098993303
14. Rosen S. Wage-based indexes of urban, quality of life. Curr Iss Urban Econ. (1979) 1979:74–104.
Google Scholar
15. Fabbri K. Indoor Thermal Comfort Perception: A Questionnaire Approach Focusing on Children. New York City, NY: Springer (2015). doi: 10.1007/978-3-319-18651-1
16. Cai Z, Kwak Y, Cvetkovic V, Deal B, Mörtberg U. Urban spatial dynamic modeling based on urban amenity data to inform smart city planning. Anthropocene. (2023) 42:100387. doi: 10.1016/j.ancene.2023.100387
17. Kaufmann T, Radaelli L, Bettencourt LMA, Shmueli E. Scaling of urban amenities: generative statistics and implications for urban planning. EPJ Data Sci. (2022) 11:1–19. doi: 10.1140/epjds/s13688-022-00362-6
18. Garretsen H, Marlet G. Amenities and the attraction of Dutch cities. Reg Stud. (2017) 51:724–36. doi: 10.1080/00343404.2015.1135239
19. Ma L, Li L, Zhu H. The construction of urban amenities index in China: an empirical research based on a statistical analysis of 26 Chinese major cities. Dili Xuebao Acta Geogr Sin. (2018) 73:755–70. doi: 10.11821/dlxb201804012
20. Zhang Y, Fang Q. The impact of city amenity on labor migration [in Chinese]. China Popul Resour Environ. (2019) 29:118–25.
21. Zhang S, Zheng H, Zhou H, Shao Q, Wu Q. Sustainable land urbanization, urban amenities, and population urbanization: evidence from city-level data in China. Soc Sci Q. (2021) 102:1686–98. doi: 10.1111/ssqu.13003
22. He J, Zeng G. Dynamic mechanism of creative industry agglomeration under the driving of urban amenities: a case study of brand design industry in Nanjing. Econ Geogr. (2019) 39:134–42, 161. doi: 10.15957/j.cnki.jjdl.2019.03.016
23. Hou S, Zhang Y, Song L. Digital finance and regional economic resilience: evidence from 283 cities in China. Heliyon. (2023) 9:e21086. doi: 10.1016/j.heliyon.2023.e21086
24. Davies S. Regional resilience in the 2008–2010 downturn: comparative evidence from European countries. Camb J Reg Econ Soc. (2011) 4:369–82. doi: 10.1093/cjres/rsr019
25. Doran J, Fingleton B. US Metropolitan Area Resilience: insights from dynamic spatial panel estimation. Environ Plan A. (2017) 50:0308518X1773606. doi: 10.1177/0308518X17736067
26. Hudec O, Reggiani A, Šiserová M. Resilience capacity and vulnerability: a joint analysis with reference to Slovak urban districts. Cities. (2018) 73:24–35. doi: 10.1016/j.cities.2017.10.004
27. Ringwood L, Watson P, Lewin P. A quantitative method for measuring regional economic resilience to the great recession. Growth Change. (2019) 50:381–402. doi: 10.1111/grow.12265
28. Cainelli G, Ganau R, Modica M. Industrial relatedness and regional resilience in the European Union. Pap Reg Sci. (2019) 98:755–79. doi: 10.1111/pirs.12377
29. Xu Y, Zhang L. The economic resilience of Chinese cities and its origin: from the perspective of diversification of industrial structure [in Chinese]. Fin Trade Econ. (2019) 40:110–26.
30. Zhou Q, Qi Z. Urban economic resilience and human capital: an exploration of heterogeneity and mechanism in the context of spatial population mobility. Sustain Cities Soc. (2023) 99:104983. doi: 10.1016/j.scs.2023.104983
31. Yu Z, Li Y, Dai L. Digital finance and regional economic resilience: theoretical framework and empirical test. Finance Res Lett. (2023) 55:103920. doi: 10.1016/j.frl.2023.103920
32. Ding J, Wang Z, Liu Y, Yu F. Measurement of economic resilience of contiguous poverty-stricken areas in China and influencing factor analysis. Prog Geogr. (2020) 39:924–37. doi: 10.18306/dlkxjz.2020.06.004
33. Rios V, Gianmoena L. The link between quality of government and regional resilience in Europe. J Policy Model. (2020) 42:1064–84. doi: 10.1016/j.jpolmod.2020.02.005
34. Li R, Xu M, Zhou H. Impact of high-speed rail operation on urban economic resilience: evidence from local and spillover perspectives in China. Cities. (2023) 141:104498. doi: 10.1016/j.cities.2023.104498
35. Gu H, Meng X, Shen T, Wen L. China's highly educated talents in 2015: patterns, determinants and spatial spillover effects. Appl Spat Anal Policy. (2020) 13:631–48. doi: 10.1007/s12061-019-09322-6
36. Yu Z, Zhang H, Tao Z, Liang J. Amenities, economic opportunities and patterns of migration at the city level in China. Asian Pac Migr J. (2019) 28:3–27. doi: 10.1177/0117196819832054
37. Zhang Y, Yu Z, Hu Z, Miao C. The influence of urban amenities, economic opportunities and city size on the spatial distribution of highly educated workers in China [in Chinese]. Trop Geogr. (2021) 41:243–55.
38. Zhang M, Partridge MD, Song H. Amenities and the geography of innovation: evidence from Chinese cities. Ann Reg Sci. (2020) 65:105–45. doi: 10.1007/s00168-020-00977-5
39. Juhász S, Pintér G, Kovács ÁJ, Borza E, Mónus G, Lőrincz L, et al. Amenity complexity and urban locations of socio-economic mixing. EPJ Data Sci. (2023) 12:1–18. doi: 10.1140/epjds/s13688-023-00413-6
40. Chen S, Oliva P, Zhang P. The effect of air pollution on migration: evidence from China. J Dev Econ. (2022) 156:102833. doi: 10.1016/j.jdeveco.2022.102833
41. Conroy T, Watson P. Overeducation, natural amenities, and entrepreneurship. Small Bus Econ. (2023) 61:1111–31. doi: 10.1007/s11187-023-00734-w
42. Cai Q, Wang S, Liu H, Shu S. Does the population agglomeration of urban agglomerations promote local firms' innovation [in Chinese]. China Ind Econ . (2023) 2023:152–70.
43. Zheng Z, Zhu Y, Pei Y, Wang L. Spatial-temporal heterogeneity and influencing factors of the coupling between industrial agglomeration and regional economic resilience in China. Environ Dev Sustain. (2023) 25:12735–59. doi: 10.1007/s10668-022-02588-w
44. Buch T, Hamann S, Rossen A, Niebuhr A. What makes cities attractive? The determinants of urban labour migration in Germany. Urban Stud. (2013) 51:96. doi: 10.1177/0042098013499796
45. Merkel J. Richard Florida: the rise of the creative class. In:Eckardt F, , editor. Schlüsselwerke Stadtforsch . Wiesbaden: Springer Fachmedien (2017). p. 69–90.
46. Diamond R. The determinants and welfare implications of US workers' diverging location choices by skill: 1980–2000. Am Econ Rev. (2016) 106:479–524. doi: 10.1257/aer.20131706
47. Di Caro P. Testing and explaining economic resilience with an application to Italian regions. Pap Reg Sci. (2017) 96:93–113. doi: 10.1111/pirs.12168
48. Widarni EL, Bawono S. Human capital, technology, and economic growth: a case study of Indonesia [in Chinese]. J Asian Finance Econ Bus. (2021) 8:29–35.
PubMed Abstract | Google Scholar
49. Glaeser EL. Urban resilience. Urban Stud. (2022) 59:3–35. doi: 10.1177/00420980211052230
50. Zheng L. What city amenities matter in attracting smart people? Pap Reg Sci. (2016) 95:309–27. doi: 10.1111/pirs.12131
51. He J, Huang X, Xi G. Urban amenities for creativity: an analysis of location drivers for photography studios in Nanjing, China. Cities. (2018) 74:310–9. doi: 10.1016/j.cities.2017.12.020
52. Ye X, Chen W. Research on the city attraction for science and technology innovation talents: construction of evaluation indicator system and empirical analysis based on amenities theory [in Chinese]. Stud Sci Sci. (2019) 37:1375–84.
53. Florida R, Mellander C, Stolarick K. Inside the black box of regional development-human capital, the creative class and tolerance. J Econ Geogr. (2008) 8:615–49. doi: 10.1093/jeg/lbn023
54. Rocchetta S, Mina A. Technological coherence and the adaptive resilience of regional economies. Reg Stud. (2019) 53:1421–34. doi: 10.1080/00343404.2019.1577552
55. Huggins R, Thompson P. Local entrepreneurial resilience and culture: the role of social values in fostering economic recovery. Camb J Reg Econ Soc. (2015) 8:rsu035. doi: 10.1093/cjres/rsu035
56. Xu Y, Deng H. Diversification, innovation capability and urban economic resilience [in Chinese]. Econ Perspect . (2020) 2020:88–104.
57. Cheng G, Jin Y. Can improving innovation capabilities enhance urban economic resilience? Mod Econ Inf . (2022) 32:1–11. doi: 10.13891/j.cnki.mer.2022.02.015
58. Li J, Ding H, Hu Y, Wan G. Dealing with dynamic endogeneity in international business research. J Int Bus Stud. (2021) 52:339–62. doi: 10.1057/s41267-020-00398-8
59. Wang H, Liu Z, Zhou Y. Assessing urban resilience in China from the perspective of socioeconomic and ecological sustainability. Environ Impact Assess Rev. (2023) 102:107163. doi: 10.1016/j.eiar.2023.107163
60. Tan J, Zhang P, Lo K, Li J, Liu S. Conceptualizing and measuring economic resilience of resource-based cities: case study of Northeast China. Chin Geogr Sci. (2017) 27:471–81. doi: 10.1007/s11769-017-0878-6
61. Su R, Zhao X, Fang Y. The development of innovation and entrepreneurship, government empowerment and urban economic resilience [in Chinese]. Sci Sci Manag ST. (2023) 44:93–113.
62. Martin R. Regional economic resilience, hysteresis and recessionary shocks. J Econ Geogr. (2012) 12:1–32. doi: 10.1093/jeg/lbr019
63. Huang T, Wei M, Xi J. Evolution and spillover effect of urban ecotourism amenity spatial pattern in Suzhou. Econ Geogr. (2023) 43:210–9. doi: 10.15957/j.cnki.jjdl.2023.08.021
64. Zhang Y, Zhang Y, Deng S. Technology, industrial structure and urban economic resilience: experience investigation from 278 prefecture-level cities in China. Nankai Econ Stud . (2022) 2022:150–68. doi: 10.14116/j.nkes.2022.12.009
65. Deschênes O, Greenstone M. Climate change, mortality, and adaptation: evidence from annual fluctuations in weather in the US. Am Econ J Appl Econ. (2011) 3:152–85. doi: 10.1257/app.3.4.152
66. Tan J, Hu X, Hassink R, Ni J. Industrial structure or agency: what affects regional economic resilience? Evidence from resource-based cities in China. Cities. (2020) 106:102906. doi: 10.1016/j.cities.2020.102906
67. Chen A. Agglomeration and urban economic resilience in China [in Chinese]. J World Econ. (2022) 45:158–81.
68. Chen S, Chen D. Air pollution, government regulations and high-quality economic development [in Chinese]. Econ Res J. (2018) 53:20–34.
69. Wang M. Polycentric urban development and urban amenities: evidence from Chinese cities. Environ Plan B Urban Anal City Sci. (2021) 48:400–16. doi: 10.1177/2399808320951205
70. Kumar A, Ekka P, Upreti M, Shilky A, Saikia P. Urban green spaces for environmental sustainability and climate resilience. In:Nautiyal S, Gupta AK, Goswami M, Imran Khan YD, , editors, Palgrave Handb. Socio-Ecol. Resil. Face Clim. Change Contexts Dev. Ctry . Singapore: Springer Nature (2023). p. 389–409.
71. Liang S, Wang Q. Cultural and creative industries and urban (re)development in China. J Plan Lit. (2020) 35:54–70. doi: 10.1177/0885412219898290
72. Cole HVS, Mehdipanah R, Gullón P, Triguero-Mas M. Breaking down and building up: gentrification, its drivers, and urban health inequality. Curr Environ Health Rep. (2021) 8:157–66. doi: 10.1007/s40572-021-00309-5
73. Wang H, Peng G, Du H. Digital economy development boosts urban resilience-evidence from China. Sci Rep. (2024) 14:2925. doi: 10.1038/s41598-024-52191-4
74. Banerjee A, Duflo E, Qian N. On the road: access to transportation infrastructure and economic growth in China. J Dev Econ. (2020) 145:102442. doi: 10.1016/j.jdeveco.2020.102442
75. Mohanty M. Sustainable urban planning and making sustainable cities. In:Leal Filho W, Azul AM, Brandli L, Özuyar PG, Wall T, , editors, Sustain. Cities Communities . Cham: Springer International Publishing (2020). p. 1–12.
76. Kapucu N, 'Gurt' Ge Y, Martín Y, Williamson Z. Urban resilience for building a sustainable and safe environment. Urban Gov. (2021) 1:10–6. doi: 10.1016/j.ugj.2021.09.001
Keywords: urban amenity, economic resilience, livable city, population agglomeration, sustainable development
Citation: Du R, Liu K, Zhao D and Fang Q (2024) Urban amenity and urban economic resilience: evidence from China. Front. Public Health 12:1392908. doi: 10.3389/fpubh.2024.1392908
Received: 28 February 2024; Accepted: 15 April 2024; Published: 09 May 2024.
Reviewed by:
Copyright © 2024 Du, Liu, Zhao and Fang. This is an open-access article distributed under the terms of the Creative Commons Attribution License (CC BY) . The use, distribution or reproduction in other forums is permitted, provided the original author(s) and the copyright owner(s) are credited and that the original publication in this journal is cited, in accordance with accepted academic practice. No use, distribution or reproduction is permitted which does not comply with these terms.
*Correspondence: Ke Liu, liuke7936@snnu.edu.cn
Disclaimer: All claims expressed in this article are solely those of the authors and do not necessarily represent those of their affiliated organizations, or those of the publisher, the editors and the reviewers. Any product that may be evaluated in this article or claim that may be made by its manufacturer is not guaranteed or endorsed by the publisher.
Big data in transportation: a systematic literature analysis and topic classification
- Brief Report
- Open access
- Published: 08 May 2024
Cite this article
You have full access to this open access article
- Danai Tzika-Kostopoulou 1 ,
- Eftihia Nathanail 1 &
- Konstantinos Kokkinos 2
216 Accesses
Explore all metrics
This paper identifies trends in the application of big data in the transport sector and categorizes research work across scientific subfields. The systematic analysis considered literature published between 2012 and 2022. A total of 2671 studies were evaluated from a dataset of 3532 collected papers, and bibliometric techniques were applied to capture the evolution of research interest over the years and identify the most influential studies. The proposed unsupervised classification model defined categories and classified the relevant articles based on their particular scientific interest using representative keywords from the title, abstract, and keywords (referred to as top words). The model’s performance was verified with an accuracy of 91% using Naïve Bayesian and Convolutional Neural Networks approach. The analysis identified eight research topics, with urban transport planning and smart city applications being the dominant categories. This paper contributes to the literature by proposing a methodology for literature analysis, identifying emerging scientific areas, and highlighting potential directions for future research.
Avoid common mistakes on your manuscript.
1 Introduction
Urbanization, ongoing changes in mobility patterns, and rapid growth in freight transportation pose significant challenges to stakeholders and researchers within the transportation sector. New policies have focused on promoting sustainability and reducing emissions through smart and multimodal mobility [ 1 ]. To address these challenges, authorities are developing strategies that employ technological advances to gain a deeper understanding of travel behavior, produce more accurate travel demand estimates, and enhance transport system performance.
Undoubtedly, the development of Intelligent Transport Systems (ITS) and recent advances in Information and Communication Technology (ICT) have enabled the continuous generation, collection, and processing of data and the observation of mobility behavior with unprecedented precision [ 2 ]. Such data can be obtained from various sources, including ITS, cell phone call records, smart cards, geocoded social media, GPS, sensors, and video detectors.
Over the past decade, there has been increasing research interest in the application of big data in various transportation sectors, such as supply chain and logistics [ 3 ], traffic management [ 4 ], travel demand estimation [ 5 ], travel behavior [ 6 ], and real-time traffic operations [ 7 ]. Additionally, numerous studies in the field of transport planning and modeling have applied big data to extract vital attributes including trip identification [ 8 ] and activity inference [ 9 ]. Despite research efforts and existing applications of big data in transportation, many aspects remain unknown, and the prospects of big data to gain better insights into transport infrastructure and travel patterns have not yet been explored.
To maximize the benefits of big data in traffic operations, infrastructure maintenance, and predictive modeling, several challenges remain to be addressed. These include handling the high velocity and volume of streaming data for real-time applications, integrating multiple data sets with traditional information sources, ensuring that the data is representative of the entire population, and accessing big data without compromising privacy and ethical concerns. In terms of transport modeling and management, the research focuses on achieving short-term stability by incorporating more comprehensive data sets that cover hourly, daily, seasonal, or event-based variations, and on enhancing mobility on demand through real-time data processing and analysis. Additionally, it has become necessary to further investigate the methodology for processing large amounts of spatial and temporal information that has primarily been intended for non-transport purposes and to reconsider the existing analytical approaches to adapt to the changing data landscape.
There is an intention among policymakers, transport stakeholders, and researchers to better understand the relationship between big data and transport. The first step in this direction is to identify the key areas of big data utilization in the transport sector. Therefore, this study attempts to map big data applications within the transport domain. It provides a broad overview of big data applications in transport and contributes to the literature by introducing a methodology for identifying emerging areas where big data can be successfully applied and subfields that can be further developed.
The scope of the current study is twofold. First, a holistic literature analysis based on bibliometric techniques complemented by a topic classification model covering the complete domain of big data applications in the transportation sector was implemented. Despite numerous studies attempting to review the relevant literature, such as big data in public transport planning or transport management [ 10 , 11 ] to the best of our knowledge, no such investigation has produced a comprehensive and systematic cluster of multiple big data applications across the entire transportation domain based on a significant number of literature records. Therefore, the primary objective of this study is to classify the literature according to its particular interest and to pinpoint evolving scientific subfields and current research trends.
Second, as multiple studies have been conducted in this domain, the need to identify and assess them prior to running one’s own research through a thorough literature review is always necessary. However, the analysis and selection of appropriate studies can be challenging, particularly when the database is large. Therefore, this study aims to provide a comprehensive approach for evaluating and selecting appropriate literature that could be a methodological tool in any research field. Bibliometric methods have been widely applied in several scientific fields. Most of these studies use simple statistical methods to determine the evolution of the number of publications over the years, authors’ influence, or geographical distribution. There are also research works that attempt to categorize the literature mainly by manual content analysis [ 12 , 13 ] or by co-citation analysis, applying software for network and graphical analyses [ 14 , 15 ]. In this study, the review process also included an unsupervised topic model to classify literature into categories.
This paper presents a comprehensive evaluation of up-to-date published studies on big data and their applications in the transportation domain. A total of 2671 articles from Elsevier's Scopus database, published between 2012 and 2022 were analyzed. Bibliometric techniques were applied to capture the evolution of research over time and uncover emerging areas of interest. In addition, the focus of this study is to define categories and classify relevant papers based on their scientific interests. To achieve this, unsupervised classification was applied using the topic model proposed by Okafor [ 16 ] to identify clusters, extract the most representative topics, and group the documents accordingly.
The current study attempts to answer the following questions:
Which studies contribute the most to the field of big data in transportation?
What is the evolution of research over time in this field of interest?
What are the main research areas that have potential for further exploration?
What are the directions of future research?
This paper consists of six sections. Following the introduction, Sect. 2 provides a summary of previous research in this subject area. Section 3 outlines the methodology applied in this research. This includes the process of defining the eligible studies, bibliometric techniques utilized, and the topic model employed for paper classification. Section 4 presents the initial statistical results and the classification outcomes derived from the topic model. In Sect. 5 , the findings are summarized, and the results associated with the research questions are discussed. The final Section presents the general conclusions and research perspectives of the study.
2 Literature review
Due to the significant benefits of big data, several studies have been conducted in recent years to review and examine the existing applications of different big data sources in transportation. Most of these focus on a specific transport domain, such as transport planning, transport management and operations, logistics and supply chain and Intelligent Transportation Systems.
In the context of transport planning and modeling, Anda et al. [ 2 ] reviewed the current application of historical big data sources, derived from call data records, smart card data, and geocoded social media records, to understand travel behavior and to examine the methodologies applied to travel demand models. Iliashenko et al. [ 17 ] explored the potential of big data and Internet of Things technologies for transport planning and modeling needs, pointing out possible applications. Wang et al. [ 18 ] analyzed existing studies on travel behavior utilizing mobile phone data. They also identified the main opportunities in terms of data collection, travel pattern identification, modeling and simulation. Huang et al. [ 19 ] conducted a more specialized literature review focusing on the existing mode detection methods based on mobile phone network data. In the public transportation sector, Pelletier et al. [ 20 ] focused on the application of smart card data, showing that in addition to fare collection, these data can also be used for strategic purposes (long-term planning), tactical purposes (service adjustment and network development), and operational purposes (public transport performance indicators and payment management). Zannat et al. [ 10 ] provided an overview of big data applications focusing on public transport planning and categorized the reviewed literature into three categories: travel pattern analysis, public transport modeling, and public transport performance assessment.
In traffic forecasting, Lana et al. [ 21 ] conducted a survey to evaluate the challenges and technical advancements of traffic prediction models using big traffic data, whereas Miglani et al. [ 22 ] investigated different deep learning models for traffic flow prediction in autonomous vehicles. Regarding transport management, Pender et al. [ 23 ] examined social media use during transport network disruption events. Choi et al. [ 11 ] reviewed operational management studies associated with big data and identified key areas including forecasting, inventory management, revenue management, transportation management, supply chain management, and risk analysis.
There is also a range of surveys investigating the use of big data in other transport subfields. Ghofrani et al. [ 24 ] analyzed big data applications in railway engineering and transportation with a focus on three areas: operations, maintenance, and safety. In addition, Borgi et al. [ 25 ] reviewed big data in transport and logistics and highlighted the possibilities of enhancing operational efficiency, customer experience, and business models.
However, there is a lack of studies that have explored big data applications attempting to cover a wider range of transportation aspects. In this regard, Zhu et al. [ 26 ] examined the features of big data in intelligent transportation systems, the methods applied, and their applications in six subfields, namely road traffic accident analysis, road traffic flow prediction, public transportation service planning, personal travel route planning, rail transportation management and control, and asset management. Neilson et al. [ 27 ] conducted a review of big data usage obtained from traffic monitoring systems crowdsourcing, connected vehicles, and social media within the transportation domain and examined the storage, processing, and analytical techniques.
The study by Katrakazas et al. [ 28 ], conducted under the NOESIS project funded by the European Union's (EU) Horizon 2020 (H2020) program, is the only one we located that comprehensively covers the transportation field. Based on the reviewed literature, the study identified ten areas of focus that could further benefit from big data methods. The findings were validated by discussing with experts on big data in transportation. However, the disadvantage of this study lies in its dependence on a limited scope of the reviewed literature.
The majority of current review-based studies concentrate on one aspect of transportation, often analyzing a single big data source. Many of these studies rely on a limited literature dataset, and only a few have demonstrated a methodology for selecting the reviewed literature. Our review differs from existing surveys in the following ways: first, a methodology for defining the selected literature was developed, and the analysis was based on a large literature dataset. Second, this study is the only one to employ an unsupervised topic classification model to extract areas of interest and open challenges in the domain. Finally, it attempts to give an overview of the applications of big data across the entire field of transportation.
3 Research methodology
This study followed a three-stage literature analysis approach. The first stage includes defining the literature source and the papers' search procedures, as well as the “screening” to select the reviewed literature. The second stage involves statistics, which are widely employed in bibliometric analysis, to capture trends and primary insights. In the third stage, a topic classification model is applied to identify developing subfields and their applications. Eventually, the results are presented, and the findings are summarized.
3.1 Literature selection
The first step in this study was to define the reviewed literature. A bibliographic search was conducted using the Elsevier's Scopus database. Scopus and Web of Science (WOS) are the most extensive databases covering multiple scientific fields. However, Scopus offers wider overall coverage than WoS CC and provides a better representation of particular subject fields such as Computer Sciences [ 29 ] which is of interest in this study. Additionally, Scopus comprises 26591 peer-reviewed journals [ 30 ] including publications by Elsevier, Emerald, Informs, Taylor and Francis, Springer, and Interscience [ 15 ], covering the most representative journals in the transportation sector.
The relevant literature was identified and collected using the Scopus search API, which supports Boolean syntax. Four combinations of keywords were used in the “title, abstract, keywords” document search of the Scopus database including: “Big data” and “Transportation”, “Big data” and “Travel”, “Big data” and “Transport”, “Big data” and “Traffic”. The search was conducted in English as it offers a wider range of bibliographic sources. Only the last decade’s peer-reviewed research papers published in scientific journals and conference proceedings have been collected, written exclusively in English. Review papers and document types such as books and book chapters were excluded. As big data in transport is an interdisciplinary field addressed by different research areas, in order to cover the whole field of interest, the following subject areas were predefined in the Scopus search: computer sciences; engineering; social sciences; environmental sciences; energy; business, management and accounting. Fields considered irrelevant to the current research interest were filtered out.
The initial search resulted in a total of 5234 articles published between the period 2012–2022. The data was collected in December 2021 and last updated on the 5th of September 2023. The results were stored in a csv format, including all essential paper information such as paper title, authors’ names, source title, citations, abstracts, year of publication, and keywords. After removing duplications, a final dataset of 3532 papers remained.
The paper dataset went through a subject relevance review, at the first stage, by checking in the papers' title or keywords the presence of at least one combination of the search terms. If this condition was not met, a further review of the paper abstracts was conducted. From both stages, a filtered set of papers was selected, based on their relevance to the current study's areas of interest, associated with the search items. A total of 2671 selected papers formed the dataset which was further analyzed, evaluated, and categorized, based on clustering techniques.
3.2 Initial statistics
Once the dataset was defined, statistical analysis was performed to identify influential journals and articles within the study field. The first task was to understand the role of the different journals and conference proceedings. Those with the most publications were listed and further analyzed according to their publication rate and research area of interest. Second, the number of citations generated by the articles was analyzed as a measure of the quality of the published studies, and the content of the most cited articles was further discussed. The above provided essential insights into research trends and emerging topics.
3.3 Topic classification
A crucial step in our analysis was to extract the most representative sub-topics and classify the articles into categories by applying an unsupervised topic model [ 16 ]. Initially, the Excel file with the selected papers’ data (authors, year, title, abstract, and keywords) was imported into the model. Abstracts, titles, and keywords were analyzed and text-cleaning techniques were applied. This step includes normalizing text, removing punctuations, stop-words, and words of length less than three letters, as well as the lemmatization of the words. The most popular software tools/libraries used for text mining and cleaning, as well as natural language processing (NLP) in the topic model process, are implemented in Python programming and include NLTK ( https://www.nltk.org/ ), spaCy ( https://spacy.io/ ), Gensim ( https://radimrehurek.com/gensim/ ), scikit-learn ( https://scikit-learn.org/stable/ ), and Beautiful Soup ( https://www.crummy.com/software/BeautifulSoup/ ). NLTK is a powerful NLP library with various text preprocessing functions, while spaCy handles tokenization, stop word removal, stemming, lemmatization, and part-of-speech tagging. Gensim is a popular library for topic labeling and document similarity analysis. To process textual data, scikit-learn is a machine learning library with text preprocessing functions. Finally, Beautiful Soup is a web-based library for parsing HTML and XML documents. For the approach explained in the following sections, NLTK and Beautiful Soup were used to parse web metadata for the research papers. Moreover, bigrams and trigrams of two or three words, frequently occurring together in a document, were created.
The basic aim was to generate clusters (topics) using a topic model. The proposed model extracts representative words from the title, abstract, and keyword section of each paper, aiming to cluster research articles into neighborhoods of topics of interest without requiring any prior annotations or labeling of the documents. The method initially constructs a word graph model by counting the Term Frequency – Inverse Document Frequency (TF-IDF) [ 31 ].
The resulting topics are visualized through a diagram that shows the topics as circular areas. For the aforementioned mechanism, the Jensen-Shannon Divergence & Principal Components dimension reduction methodology was used [ 32 ]. The model is implemented in the LDAvis (Latent Dirichlet Allocation) Python library, resulting in two principal components (PC1 and PC2) that visualize the distance between the topics on a two-dimensional plane. The topic circles are created using a computationally greedy approach (the first in-order topic gets the largest area, and the rest of the topics get a proportional area according to their calculated significance). The method picks the circle centroids randomly for the first one (around the intersection of the two axes), and then the distance of the circle centroids is relevant to the overlapping of the top words according to the Jensen-Shannon Divergence model. The former model was applied for numerous numbers of topics, while its performance was experimentally verified using a combination of Naïve Bayesian Networks and a Convolutional Neural Network approach.
In the sequence of the two machine learning methodologies, supervised (Bayesian Neural Networks) and unsupervised (Deep Learning) research article classification were considered via sentiment analysis. For the supervised case, the relations among words were identified, offering interesting qualitative information from the outcome of the TF-IDF approach. The results show that the Bayesian Networks perform in accuracies near 90% of the corresponding statistical approach [ 33 ] and the same happens (somewhat inferior performance) for the unsupervised case [ 34 ]. The two methods validated the results of the TF-IDF, reaching accuracies within the acceptable limits of the aforementioned proven performances.
3.3.1 TF-IDF methodology
The TF-IDF (Term Frequency–Inverse Document Frequency) method is a weighted statistical approach primarily used for mining and textual analysis in large document collections. The method focuses on the statistical importance of a word value emanating by its existence in a document and the frequency of occurrences. In this context, the statistical significance of words grows in proportion to their frequency in the text, but also in inverse proportion to their frequency in the entire corpus of documents. Therefore, if a word or phrase appears with high frequency in an article (TF value is high), but simultaneously seldom appears in other documents (IDF value is low), it is considered a highly candidate word that may represent the article and can be used for classification [ 35 ]. In the calculation of TF-IDF, the TF word value is given as:
In Eq. 1 , \({n}_{ij}\) denotes the frequency of the word occurrence of the term \({t}_{i}\) in the document \({d}_{j}\) , and the denominator of the above fraction is the sum of the occurrence frequency of all terms in the document \({d}_{j}.\) At the same time, the calculation of the IDF value of a term \({t}_{i}\) , is found by dividing the total number of documents in the corpus by the number of documents containing the term \({t}_{i}\) , and then obtains the quotient logarithm:
In Eq . 2 , | D | denotes the total number of documents, and the denominator represents the number of documents j which contain the term \({t}_{i}\) in that specific document \({d}_{j}\) . In other words, we consider only the documents where \({n}_{ij}\ne 0\) in Eq. ( 1 ). In the case scenario in which the term \({t}_{i}\) does not appear in the corpus, it will cause the dividend to be zero in the above equation, causing the denominator to have the values of + 1. Using Eqs. ( 1 ) and ( 2 ), TF-IDF is given by:
From Eq. 3 , it can be assumed that high values of TF-IDF can be produced by high term frequencies in a document, while at the same time, low term frequencies occur in the entire document corpus. For this reason, TF-IDF succeeds in filtering out all high-frequency “common” words, while at the same time, retaining statistically significant words that can be the topic representatives [ 36 ]
3.3.2 Naïve bayes methodology
This methodology evaluates the performance of TF-IDF by following a purely “machine-learning” oriented approach, which is based on the Bayesian likelihood, i.e., reverse reasoning to discover arbitrary factor occurrences that impact a particular result. These arbitrary factors correspond to the corpus terms and their frequencies within each document and corpus. The model is a multinomial naive Bayes classifier that utilizes the Scikit-learn, which is a free programming Artificial Intelligence (AI) library for the Python programming language to support: a. training text, b. feature vectors, c. the predictive model, and d. the grouping mechanism.
The results of the TF-IDF method were imported into the Bayesian classifier. More specifically, the entire dataset was first prepared to be inserted by applying noise, stop-words, and punctuation removal. The text was then tokenized into words and phrases. For topic modeling, TF-IDF was used for feature extraction, creating the corresponding vectors as features for classification. In the next step, the Naive Bayes classifier is trained on the pre-processed and feature-extracted data. During training, it learns the likelihood of observing specific words or features given each topic and the prior probabilities of each topic occurring in the training data. Not all data was used for training. The model split the data into a 70% portion used for the unsupervised training, with the remaining 30% to be used for validation. This split ratio is a rule of thumb and not a strict requirement. However, this popular split ratio is to strike a balance between having enough data for training the machine learning model effectively and having enough data for validation or testing to evaluate the model's performance. The split was used within the k-fold cross-validation to assess the performance of the model while mitigating the risk of overfitting. While the dataset is divided into k roughly equal-sized "folds" or subsets, a fixed train-test split is used within each fold. The results from each fold were then averaged to obtain an overall estimate of model performance. This approach has the advantage of assessing the model's performance in a more granular way within each fold, similar to how it is assessed in a traditional train-test split, but at the same time, it provides additional information about how the model generalizes to different subsets of the data within each fold.
In the process of text examination, a cycle of weighting is dynamically updated for cases of term recurrences in the examined documents. The documents still contain only the title, abstract, and keywords for each article. For these cases, the Bayes theorem is used:
where \(P(c|x)\) is the posterior probability, \(P(x|c)\) is the likelihood, \(P(c)\) is the class prior probability, and \(P(x)\) is the predictor prior probability with \(P(c|x)\) resulting from the individual likelihoods of all documents \(P\left({x}_{i}|c\right),\) as depicted in Eq. ( 5 ):
This model was used as a validation method for the TF-IDF methodology, producing accuracy results reaching values of up to 91%. This value is widely acceptable for most cases of Bayesian classification and is expected to occur since prior classification has been applied [ 37 ].
3.3.3 Deep learning classification methodology
This is a secondary method of TF-IDF validation, which is based on the bibliometric coupling technique. However, this technique does not use the likelihood probability of the initial classification performed by TF-IDF but rather, this classifier deploys a character-based (i.e., the alphabetical letters composing the articles’ text) convolutional deep neural network. Using the letters as basic structural units, a Convolutional Neural Network [ 38 ] learns words and discovers features and word occurrences in various documents. The model has been primarily applied for computer vision and image machine learning techniques [ 39 ], but it is easily adapted for textual analysis.
All features previously used features (title, abstract, keywords) were kept and concatenated into a single string of text, which was itself truncated to a maximum length of 4000 characters. The size of the string can be dynamically adapted for each TensorFlow-model [ 40 ] according to the GPU performance of the graphics adapter of the hardware used, and basically represents the maximum allowable length for each feature in the analysis. The encoding involved all 26 English characters, 10 digits, all punctuation signs, and the space character. Other extraneous characters were eliminated. Furthermore, keyword information was considered primary in topic classification and encoded into a vector of the proportion of each subfield mentioned in the reference list of all documents/articles used in data. The system was rectified to behave as a linear unit, producing the activation function of the deep neural network between each layer. Only the last layer of the network utilized the SoftMax activation function for the final classification. The model was trained with a stochastic gradient descent as the optimizer and categorical cross-entropy as the loss function producing inferior results when compared with the corresponding Bayesian case as expected.
4.1 Source analysis
To understand the role of diverse academic sources, the leading eleven journals or conference proceedings were identified (Table 1 ), which have published a minimum of twenty papers between 2012 and 2022 in the field of interest. According to the preliminary data, 995 journals and conference proceedings have contributed to the publication of 2671 papers. Eleven sources have published 553 articles, representing the 2100% of all published papers.
Three sources in the field of computer science have published 239 articles. Five transportation journals and conference proceedings have published 208 papers, while there are journals on urban planning and policies (e.g., Cities) that have also significantly contributed to the research field (106 papers).
The research findings indicate the interdisciplinarity of the application of big data in transportation, encompassing not only computer science but also transport and urban planning journals, showing that transport specialists acknowledge the advantages of examining the applications of big data in the transport domain.
4.2 Citation analysis
Citation analysis classifies the papers by their citation frequency, aiming to point out their scientific research impact [ 14 ] and to identify influential articles within a research area. Table 2 demonstrates the top fifteen studies published between 2012 and 2022 (based on citation count on Scopus). Lv et al. [ 41 ] published the most influential paper in this period and received 2284 citations. This study applied a novel deep learning method to predict traffic flow. In this direction, four other articles focused on traffic flow prediction, real-time traffic operation, and transportation management [ 7 , 11 , 42 , 43 ].
Other important contributions highlighted the significance of big data generated in cities and analyzed challenges and possible applications in many aspects of the city, such as urban planning, transportation, and the environment [ 44 , 45 , 46 , 47 ]. Xu et al. [ 48 ] focused on the Internet of Vehicles and the generated big data. Finally, among the most influential works, there are papers that investigated big data usage in various subfields of spatial analysis, as well as urban transport planning and modeling, focusing on travel demand prediction, travel behavior analysis, and activity pattern identification [ 5 , 6 , 49 , 50 , 51 ].
4.3 Topic model
As previously mentioned, a topic model based on the TF-IDF methodology was used to categorize the papers under different topics. The basic goal was to identify subscientific areas of transportation where big data were implemented. A critical task was to define the appropriate parameters of the model, particularly the number of topics that could provide the most accurate and meaningful results, which would be further processed. To achieve this, a qualitative analysis was conducted taking into account the accuracy results obtained from the validation methodology. Therefore, to obtain a more precise view of the project, the model was implemented under various scenarios, increasing each time the number of topics by one, starting from four topics to fifteen.
The mapping of the tokenized terms and phrases to each other was used to understand the relationships between words in the context of topics and documents. The technique applied is the Multidimensional Scaling (MDS), which helps to visualize and analyze these relationships in a lower-dimensional space [ 52 , 53 ]. To highlight the relationships between terms, a matrix of tokens in the corpus was created based on their co-occurrence patterns. Each cell in the matrix represents how often two terms appear together in the same document. The MDS methodology provides the ability to view high-dimensional data in a lower-dimensional space while retaining as much of the pairwise differences or similarities between the terms as possible. More specifically, MDS translates the high-dimensional space of term co-occurrence relationships into a lower-dimensional space, where words that frequently co-occur are located closer to one another, and terms that infrequently co-occur are situated farther apart. When terms are displayed as points on a map or scatterplot via MDS, points that are close together in the scatterplot are used in the documents in a more connected manner.
As a consequence, the scatterplot that MDS produces can shed light on the structure of the vocabulary in relation to the topic model. It can assist in identifying groups of related terms that may indicate subjects or themes in the corpus. The pyLDAvis library of the Python programming language was used in conjunction with the sklearn library to incorporate the MDS into the clustering visualization process. This was done by superimposing the scatterplot with details about the subjects assigned to documents or the probability distributions over different topics.
Initially, the papers were divided into four topics. Figure 1 a displays four distinct clusters, each representing a scientific sub-area of interest. Clusters appear as circular areas, while the two principal components (PC1 and PC2) are used to visualize the distance between topics on a two-dimensional plane. Figure 1 b illustrates the most relevant terms for Topic 1 in abstracts, titles, and keywords. Table 3 contains the most essential words associated with each sub-area based on the TF-IDF methodology, representing the nature of the four topics. The results of the model, considering, also, the content of the most influential papers in each group, reveal that the biggest cluster is associated with “transport planning and travel behavior analysis”. In this topic, most papers have focused on long-term planning, utilizing big data mostly from smart cards, mobile phones, social media, and GPS trajectories to reveal travel and activity patterns or estimate crucial characteristics for transport planning, such as travel demand, number of trips, and trip purposes. The second topic refers to “smart cities and applications”, containing papers about how heterogeneous data generated in cities (streamed from sensors, devices, vehicles, or humans) can be used to improve the quality of human life, city operation systems, and the urban environment. Most studies have concentrated on short-term thinking about how innovative services and big data applications can support function and management of cities. In terms of transportation, several studies have managed to integrate Internet of Things (IoT) and big data analytics with intelligent transportation systems, including public transportation service plan, route optimization, parking, rail transportation, and engineering and supply chain management. Two smaller clusters were observed. One cluster is dedicated to “traffic forecasting and management” and includes papers related to traffic flow prediction or accident prediction, while many of them focus on real-time traffic management, city traffic monitoring, and control, mainly using real-time traffic data generated by sensors, cameras, and vehicles. The other is associated with “intelligent transportation systems and new technologies”, focusing on topics such as the connected vehicle-infrastructure environment and connected vehicle technologies as a promising direction to enhance the overall performance of the transportation system. Considerable attention has also been given to Internet of Vehicles (IoV) technology, autonomous vehicles, self-driving vehicle technology, and automatic traffic, while many contributions in this topic are related to green transportation and suggest solutions for optimizing emissions in urban areas with a focus on vehicle electrification. The four topics are represented as circular areas. The two principal components (PC1 and PC2) are used to visualize the distance between topics on a two-dimensional plane.
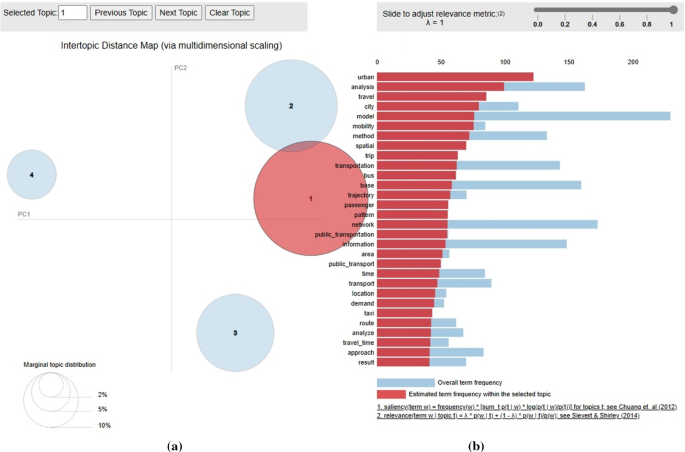
Four topics distance map, b Top 30 most relevant terms for Topic 1
By increasing the number of topics, various categories are becoming more specific, and it can be observed that there is a stronger co-relationship among clusters. The results of the eight topics’ categorization are presented in Fig. 2 a and b and Table 4 . The eight topics are represented as circular areas. The two principal components (PC1 and PC2) are used to visualize the distance between topics on a two-dimensional plane.
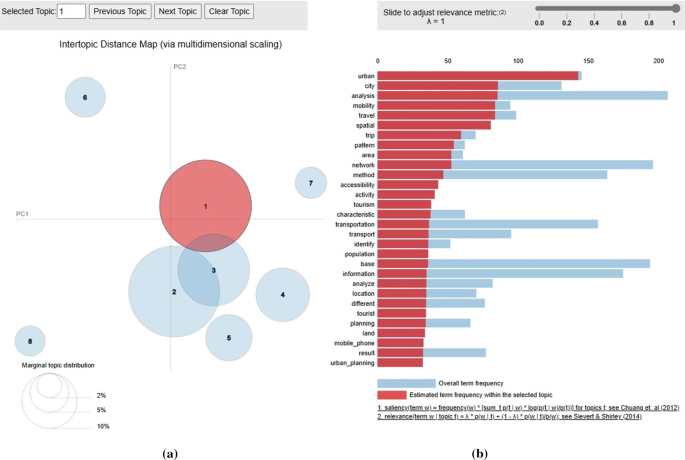
a Eight topics distance map, b Top 30 most relevant terms for Topic 1
According to the top words in each cluster and taking into consideration the content of top papers abstracts, the eight topics were specified as follows:
Topic 1 (742 papers), “Urban transport planning”: This topic concerns long-term transportation planning within cities, utilizing big and mainly historical data, such as call detail records from mobile phones and large-scale geo-location data from social media in conjunction with geospatial data, census records, and surveys. The emphasis is on analyzing patterns and trends in the urban environment. Most studies aim to investigate the spatial–temporal characteristics of urban movements, detect land use patterns, reveal urban activity patterns, and analyze travel behavior. Moreover, many papers focus on travel demand and origin–destination flow estimation or extract travel attributes, such as trip purpose and activity location.
Topic 2 (723 papers), “Smart cities and applications”: This topic remains largely consistent with the previous categorization. As above, the papers aim to take advantage of the various and diverse data generated in cities, analyze new challenges, and propose real-time applications to enhance the daily lives of individuals and city operation systems.
Topic 3 (438 papers), “Traffic flow forecasting and modeling”: This area of research involves the use of machine and deep learning techniques to analyze mainly historical data aiming to improve traffic prediction accuracy. The majority of these papers concentrate on short-term traffic flow forecasting, while a significant number of them address passenger flow and traffic accident prediction.
Topic 4 (231 papers), “Traffic management”: this topic concentrates on traffic management and real-time traffic control. City traffic monitoring, real-time video, and image processing techniques are gaining significant attention. Numerous studies utilize real-time data to evaluate traffic conditions by image-processing algorithms and provide real-time route selection guidance to users. Most of them manage to identify and resolve traffic congestion problems, as well as to detect anomalies or road traffic events, aiming to improve traffic operation and safety.
Topic 5 (194 papers), “Intelligent transportation systems and new technologies”: this topic remains nearly identical to the prior (4-clusters) classification, containing articles on emerging technologies implemented in an intelligent and eco-friendly transport system. Most studies focus on the connected vehicle-infrastructure environment and connected vehicle technologies as a promising direction for improving transportation system performance. Great attention is also given to Internet of Vehicles (IoV) technology and the efficient management of the generated and collected data. Autonomous and self-driving vehicle technologies are also crucial topics. Many papers, also, discuss green transportation and suggest ways to optimize emissions in urban areas, with a particular emphasis on vehicle electrification.
Topic 6 (144 papers), “Public transportation”: since public transportation gained special scientific interest in our database, a separate topic was created regarding public transport policy making, service, and management. Most publications focus on urban means of transport, such as buses, metro, and taxis, while a significant proportion refers to airlines. This topic covers studies related to public transportation network planning and optimization, performance evaluation, bus operation scheduling, analysis of passenger transit patterns, maximization of passenger satisfaction levels, and real-time transport applications. Moreover, smart cards and GPS data are extensively used to estimate origin–destination matrices.
Topic 7 (104 papers), “Railway”: this topic presents research papers that apply big data to railway transportation systems and engineering, encompassing three areas of interest: railway operations, maintenance, and safety. A significant proportion of studies focus on railway operations, including train delay prediction, timetabling improvement, and demand forecasting. Additionally, numerous researchers employ big data to support maintenance decisions and conduct risk analysis of railway networks, such as train derailments and failure prediction. These papers rely on diverse datasets, including GPS data, passenger travel information, as well as inspection, detectors, and failure data.
Topic 8 (95 papers), “GPS Trajectories”: This topic contains papers that take advantage of trajectory data primarily obtained from GPS devices installed in taxis. Most studies forecast the trip purpose of taxi passengers, trip destination, and travel time by analyzing historical GPS data. Additionally, a significant number of these studies focus on real-time analysis to provide passengers with useful applications and enhance the quality of taxi services. Finally, there is research interest in maritime routing and ship trajectory analysis to facilitate maritime traffic planning and service optimization.
In the eight-topic classification, the initial four clusters either remained almost unchanged or were divided into subcategories. For example, the previous cluster “transport planning and travel behavior analysis” is now divided into “urban transport planning” and “public transportation”, with “transport management” constituting a separate category. Moreover, several distinct smaller clusters have been identified (e.g., “railway” and “trajectories”). These, along with “public transportation”, are highly specialized categories with no correlation to the other clusters. Nevertheless, they constitute a significant proportion of the literature and merit separate analysis.
As the number of topics increased, so did the overlaps among the clusters. Thus, based on this observation and the accuracy results of the validation method, it was assumed that eight clusters were the most appropriate for further analysis.
Based on the results of eight-topic classification, Fig. 3 demonstrates the evolution of the number of published articles per topic and per year. As shown three topics have gained researchers’ interest: (1) urban transport planning, (2) smart cities and applications, and (3) traffic forecasting and modeling. Initially, the primary topic was “smart cities”, largely based on the computer science sector. Despite a slight decline in publications in 2019, there is an overall upward trend. “Urban transport planning” experienced a steady and notable increase until 2019. A sudden drop was recorded in 2022, but it is not clear whether this is a coincidence or a trend. However, it remains the dominant topic, with most publications over the years. The observed decrease could indicate further specialization and research evolution in the field, given it is also the topic with the most subcategories during the classification process.
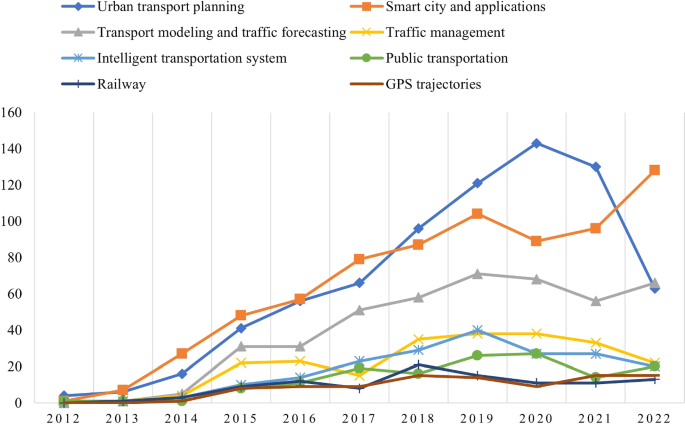
Number of papers per topic and year
5 Discussion
5.1 statistical analysis.
As shown in the analysis, there is an increasing research interest in big data usage in the transportation sector. It is remarkable that besides computer science journals and conferences, transportation journals have also published relevant articles representing a notable proportion of the research and indicating that transportation researchers acknowledge the significance of big data and its contribution to many aspects of transportation. According to the citation analysis, three research areas emerged among the most influential studies: (1) traffic flow prediction and management (2) new challenges of the cities (smart cities) and new technologies (3) urban transport planning and spatial analysis.
5.2 Topic classification
Following the topic model results, eight paper groups are proposed. Most articles (742) fall into the topic of “urban transport planning”. Several representative papers in this area attempted to estimate travel demand [ 5 ], analyze travel behavior [ 6 ], or investigate activity patterns [ 51 ] by utilizing big data sourced primarily from mobile phone records, social media, and smart card fare collection systems.
Big data also has significant impacts on “smart cities and applications”. Topic 2 is a substantial part of the dataset, which includes 723 papers. They mainly refer to new challenges arising from big data analytics to support various aspects of the city, such as transportation or energy [ 44 ] and investigate big data applications in intelligent transportation systems [ 26 ] or in the supply chain and logistics [ 54 ].
A total of 438 papers were categorized in Topic 3 labeled as “traffic flow forecasting and modeling”. The majority applied big data and machine learning techniques to predict traffic flow [ 41 , 42 , 43 ]. In risk assessment, Chen et al. [ 55 ] proposed a logit model to analyze hourly crash likelihood, considering temporal driving environmental data, whereas Yuan et al. [ 56 ] applied a Convolutional Long Short-Term Memory (ConvLSTM) neural network model to forecast traffic accidents.
Among the papers, a special focus is given to different aspects of “traffic management” (231 papers), largely utilizing real-time data. Shi and Abdel-Aty [ 7 ] employed random forest and Bayesian inference techniques in real-time crash prediction models to reduce traffic congestion and crash risk. Riswan et al. [ 57 ] developed a real-time traffic management system based on IoT devices and sensors to capture real-time traffic information. Meanwhile, He Z. et al. [ 58 ] suggested a low-frequency probe vehicle data (PVD)-based method to identify traffic congestion at intersections to solve traffic congestion problems.
Topic 5 includes 194 records on “intelligent transportation systems and new technologies”. It covers topics such as Internet of Vehicles [ 48 , 59 , 60 ], connected vehicle-infrastructure environment [ 61 ], electric vehicles [ 62 ], and the optimization of charging stations location [ 63 ], as well as autonomous vehicles (AV) and self-driving vehicle technology [ 64 ].
In recent years, three smaller and more specialized topics have gained interest. Within Topic 6, there are 144 papers discussing public transport. Tu et al. [ 65 ] examined the use of smart card data and GPS trajectories to explore multi-modal public ridership. Wang et al. [ 66 ] proposed a three-layer management system to support urban mobility with a focus on bus transportation. Tsai et al. [ 67 ] applied simulated annealing (SA) along with a deep neural network (DNN) to forecast the number of bus passengers. Liu and Yen [ 68 ] applied big data analytics to optimize customer complaint services and enhance management process in the public transportation system.
Topic 7 contains 104 papers on how big data is applied in “railway network”, focusing on three sectors of railway transportation and engineering. As mentioned in Ghofrani et al. [ 24 ], these sectors are maintenance [ 69 , 70 , 71 ], operation [ 72 , 73 ] and safety [ 74 ].
Topic 8 (95 papers) refers mainly to data deriving from “GPS trajectories”. Most researchers utilized GPS data from taxis to infer the trip purposes of taxi passengers [ 75 ], explore mobility patterns [ 76 ], estimate travel time [ 77 ], and provide real-time applications for taxi service improvement [ 78 , 79 ]. Additionally, there are papers included in this topic that investigate ship routes. Zhang et al. [ 80 ] utilized ship trajectory data to infer their behavior patterns and applied the Ant Colony Algorithm to deduce an optimal route to the destination, given a starting location, while Gan et al. [ 81 ] predicted ship travel trajectories using historical trajectory data and other factors, such as ship speed, with the Artificial Neural Network (ANN) model.
6 Conclusions
An extensive overview of the literature on big data and transportation from 2012 to 2022 was conducted using bibliometric techniques and topic model classification. This paper presents a comprehensive methodology for evaluating and selecting the appropriate literature. It identifies eight sub-areas of research and highlights current trends. The limitations of the study are as follows: (1) The dataset came up by using a single bibliographic database (Scopus). (2) Research sources, such as book chapters, were excluded. (3) Expanding the keyword combinations could result in a more comprehensive review. Despite these limitations, it is claimed that the reviewed dataset is representative, leading to accurate findings.
In the process of selecting the suitable literature, various criteria were defined in the Scopus database search, including the language, subject area, and document type. Subsequently, duplicate and non-scientific records were removed. However, the last screening of the titles and abstracts to determine the relevance of the studies to the paper’s research interests was conducted manually. This could not be possible for a larger dataset. Additionally, as previously stated, the dataset was divided into eight distinct topics due to multiple overlaps caused by an increase in the number of topics. Nevertheless, the topic of “smart cities and applications” remains broad, even with this division. This makes it challenging to gain in-depth insights into the field and identify specific applications, unlike in “transport planning”, where two additional topics were generated by the further classification. Applying the classification model to each topic separately could potentially overcome these constraints by revealing more precise applications and filtering out irrelevant studies.
Despite the above limitations and constraints, the current study provides an effective methodology for mapping the field of interest as a necessary step to define the areas of successful applications and identify open challenges and sub-problems that should be further investigated. It is worth mentioning that there is an intense demand from public authorities for a better understanding of the potential of big data applications in the transport domain towards more sustainable mobility [ 82 ]. In this direction, our methodology, along with the necessary literature review and discussion with relevant experts, can assist policymakers and transport stakeholders in identifying the specific domains in which big data can be applied effectively and planning future transport investments accordingly.
Having defined the critical areas of big data implementation within transportation, trends, and effective applications, the aim is to conduct a thorough literature review in a subarea of interest. This will focus on transport planning and modeling, and public transportation, which appears to be highly promising, based on our findings. A more extensive literature review and content analysis of key studies are crucial to further examine open challenges and subproblems as well as to investigate the applied methodologies for possible revision or further development.
The current study provides a broad overview of the applications of big data in transport areas, which is the initial step in understanding the characteristics and limitations of present challenges and opportunities for further research in the field.
European Commission (2021) Sustainable and Smart Mobility Strategy. https://ec.europa.eu/transport/sites/default/files/2021-mobility-strategy-and-action-plan.pdf . Accessed 10 Jul 2021
Anda C, Erath A, Fourie PJ (2017) Transport modelling in the age of big data. Int J Urban Sci 21:19–42. https://doi.org/10.1080/12265934.2017.1281150
Article Google Scholar
Zhong RY, Huang GQ, Lan S, Dai QY, Chen X, Zhang T (2015) A big data approach for logistics trajectory discovery from RFID-enabled production data. Int J Prod Econ 165:260–272. https://doi.org/10.1016/j.ijpe.2015.02.014
Nallaperuma D, Nawaratne R, Bandaragoda T, Adikari A, Nguyen S, Kempitiya T, de Silva D, Alahakoon D, Pothuhera D (2019) Online incremental machine learning platform for big data-driven smart traffic management. IEEE Trans Intell Transp Syst 20:4679–4690. https://doi.org/10.1109/TITS.2019.2924883
Toole JL, Colak S, Sturt B, Alexander LP, Evsukoff A, González MC (2015) The path most traveled: travel demand estimation using big data resources. Transp Res Part C Emerg Technol 58:162–177. https://doi.org/10.1016/j.trc.2015.04.022
Chen C, Ma J, Susilo Y, Liu Y, Wang M (2016) The promises of big data and small data for travel behavior (aka human mobility) analysis. Transp Res Part C Emerg Technol 68:285–299
Shi Q, Abdel-Aty M (2015) Big Data applications in real-time traffic operation and safety monitoring and improvement on urban expressways. Transp Res Part C Emerg Technol 58:380–394. https://doi.org/10.1016/j.trc.2015.02.022
Iqbal MS, Choudhury CF, Wang P, González MC (2014) Development of origin-destination matrices using mobile phone call data. Transp Res Part C Emerg Technol 40:63–74. https://doi.org/10.1016/j.trc.2014.01.002
Alexander L, Jiang S, Murga M, González MC (2015) Origin-destination trips by purpose and time of day inferred from mobile phone data. Transp Res Part C Emerg Technol 58:240–250. https://doi.org/10.1016/j.trc.2015.02.018
Zannat KE, Choudhury CF (2019) Emerging big data sources for public transport planning: a systematic review on current state of art and future research directions. J Indian Inst Sci 99:601–619
Choi TM, Wallace SW, Wang Y (2018) Big data analytics in operations management. Prod Oper Manag 27:1868–1883. https://doi.org/10.1111/poms.12838
Chalmeta R, Santos-deLeón NJ (2020) Sustainable supply chain in the era of industry 4.0 and big data: a systematic analysis of literature and research. Sustainability 12:4108. https://doi.org/10.3390/su12104108
De Bakker FGA, Groenewegen P, Den Hond F (2005) A bibliometric analysis of 30 years of research and theory on corporate social responsibility and corporate social performance. Bus Soc 44:283–317. https://doi.org/10.1177/0007650305278086
Mishra D, Gunasekaran A, Papadopoulos T, Childe SJ (2018) Big data and supply chain management: a review and bibliometric analysis. Ann Oper Res 270:313–336. https://doi.org/10.1007/s10479-016-2236-y
Fahimnia B, Sarkis J, Davarzani H (2015) Green supply chain management: a review and bibliometric analysis. Int J Prod Econ 162:101–114. https://doi.org/10.1016/j.ijpe.2015.01.003
Okafor O (2020) Automatic Topic classification of research papers using the NLP topic model NMF. https://obianuju-c-okafor.medium.com/automatic-topic-classification-of-research-papers-using-the-nlp-topic-model-nmf-d4365987ec82f . Accessed 10 Jul 2021
Iliashenko O, Iliashenko V, Lukyanchenko E (2021) Big data in transport modelling and planning. Transp Res Proced 54:900–908
Wang Z, He SY, Leung Y (2018) Applying mobile phone data to travel behaviour research: a literature review. Travel Behav Soc 11:141–155. https://doi.org/10.1016/j.tbs.2017.02.005
Huang H, Cheng Y, Weibel R (2019) Transport mode detection based on mobile phone network data: a systematic review. Transp Res Part C Emerg Technol 101:297–312
Pelletier MP, Trépanier M, Morency C (2011) Smart card data use in public transit: a literature review. Transp Res Part C Emerg Technol 19:557–568. https://doi.org/10.1016/j.trc.2010.12.003
Lana I, Del Ser J, Velez M, Vlahogianni EI (2018) Road traffic forecasting: recent advances and new challenges. IEEE Intell Transp Syst Mag 10:93–109
Miglani A, Kumar N (2019) Deep learning models for traffic flow prediction in autonomous vehicles: a review, solutions, and challenges. Veh Commun 20:100184. https://doi.org/10.1016/j.vehcom.2019.100184
Pender B, Currie G, Delbosc A, Shiwakoti N (2014) Social media use during unplanned transit network disruptions: a review of literature. Transp Rev 34:501–521. https://doi.org/10.1080/01441647.2014.915442
Ghofrani F, He Q, Goverde RMP, Liu X (2018) Recent applications of big data analytics in railway transportation systems: a survey. Transp Res Part C Emerg Technol 90:226–246. https://doi.org/10.1016/j.trc.2018.03.010
Borgi T, Zoghlami N, Abed M (2017). Big data for transport and logistics: a review. In: International conference on advanced systems and electric technologies (IC_ASET), pp 44–49
Zhu L, Yu FR, Wang Y, Ning B, Tang T (2019) Big data analytics in intelligent transportation systems: a survey. IEEE Trans Intell Transp Syst 20:383–398
Neilson A, Indratmo DB, Tjandra S (2019) Systematic review of the literature on big data in the transportation domain: concepts and applications. Big Data Res 17:35–44
Katrakazas C, Antoniou C, Sobrino N, Trochidis I, Arampatzis S (2019). Big data and emerging transportation challenges: findings from the NOESIS project. In: 6th IEEE International conference on models and technologies for intelligent transportation systems (MT-ITS), pp 1–9
Pranckutė R (2021) Web of science (WoS) and scopus: the titans of bibliographic information in today’s academic world. Publications 9(1):12. https://doi.org/10.3390/publications9010012
Elsevier Scopus (2023) Content coverage guide. https://www.elsevier.com/?a=69451 . Accessed 27 Sept 2023
Jiang Z, Gao B, He Y, Han Y, Doyle P, Zhu Q (2021) Text classification using novel term weighting scheme-based improved TF-IDF for internet media reports. Math Probl Eng. https://doi.org/10.1155/2021/6619088
Zhang X, Delpha C, Diallo D (2019) Performance of Jensen Shannon divergence in incipient fault detection and estimation. In: 2019 IEEE international conference on acoustics, speech and signal processing (ICASSP), pp 2742–2746
Ruz GA, Henríquez PA, Mascareño A (2020) Sentiment analysis of twitter data during critical events through Bayesian networks classifiers. Futur Gener Comput Syst 106:92–104. https://doi.org/10.1016/j.future.2020.01.005
Kumar A, Srinivasan K, Cheng WH, Zomaya AY (2020) Hybrid context enriched deep learning model for fine-grained sentiment analysis in textual and visual semiotic modality social data. Inf Process Manag 57:102–141. https://doi.org/10.1016/j.ipm.2019.102141
Pimpalkar AP, Retna Raj RJ (2020) Influence of pre-processing strategies on the performance of ML classifiers exploiting TF-IDF and BOW features. ADCAIJ Adv Distrib Comput Artif Intell J 9:49–68. https://doi.org/10.14201/adcaij2020924968
YueTing H, YiJia X, ZiHe C, Xin T (2019) Short text clustering algorithm based on synonyms and k-means. Computer knowledge and technology 15(1).
Bracewell DB, Yan J, Ren F, Kuroiwa S (2009) Category classification and topic discovery of japanese and english news articles. Electron Notes Theor Comput Sci 225:51–65. https://doi.org/10.1016/j.entcs.2008.12.066
Kalchbrenner N, Grefenstette E, Blunsom P (2014) A convolutional neural network for modelling sentences. In: 52nd Annual meeting of the association for computational linguistics, pp 655–665
Albawi S, Mohammed TA, Al-Zawi S (2017) Understanding of a convolutional neural network. In: International conference on engineering and technology (ICET), pp 1–6
Ertam F, Aydn G (2017) Data classification with deep learning using tensorflow. In: International conference on computer science and engineering (UBMK), pp 755–758
Lv Y, Duan Y, Kang W, Li Z, Wang FY (2015) Traffic flow prediction with big data: a deep learning approach. IEEE Trans Intell Transp Syst 16:865–873. https://doi.org/10.1109/TITS.2014.2345663
Wu Y, Tan H, Qin L, Ran B, Jiang Z (2018) A hybrid deep learning based traffic flow prediction method and its understanding. Transp Res Part C Emerg Technol 90:166–180. https://doi.org/10.1016/j.trc.2018.03.001
Tian Y, Pan L (2015) Predicting short-term traffic flow by long short-term memory recurrent neural network. In: IEEE International conference on smart city/socialcom/sustaincom (SmartCity). IEEE, pp 153–158
Al Nuaimi E, Al Neyadi H, Mohamed N, Al-Jaroodi J (2015) Applications of big data to smart cities. J Internet Serv Appl 6:1–15. https://doi.org/10.1186/s13174-015-0041-5
Batty M (2013) Big data, smart cities and city planning. Dialog Hum Geogr 3:274–279. https://doi.org/10.1177/2043820613513390
Zheng Y, Capra L, Wolfson O, Yang H (2014) Urban computing: concepts, methodologies, and applications. ACM Trans Intell Syst Technol 5(3):1–55. https://doi.org/10.1145/2629592
Mehmood Y, Ahmad F, Yaqoob I, Adnane A, Imran M, Guizani S (2017) Internet-of-things-based smart cities: recent advances and challenges. IEEE Commun Mag 55:16–24. https://doi.org/10.1109/MCOM.2017.1600514
Xu W, Zhou H, Cheng N, Lyu F, Shi W, Chen J, Shen X (2018) Internet of vehicles in big data era. IEEE/CAA J Autom Sin 5:19–35. https://doi.org/10.1109/JAS.2017.7510736
Zhong C, Arisona SM, Huang X, Batty M, Schmitt G (2014) Detecting the dynamics of urban structure through spatial network analysis. Int J Geogr Inf Sci 28:2178–2199. https://doi.org/10.1080/13658816.2014.914521
Yao H, Wu F, Ke J, Tang X, Jia Y, Lu S, Gong P, Ye J, Chuxing D, Li Z (2018) Deep multi-view spatial-temporal network for taxi demand prediction. In: AAAI Conference on artificial intelligence. pp 2588–2595
Hasan S, Ukkusuri SV (2014) Urban activity pattern classification using topic models from online geo-location data. Transp Res Part C Emerg Technol 44:363–381. https://doi.org/10.1016/j.trc.2014.04.003
Saeed N, Nam H, Haq MIU, Saqibm DBM (2018) A survey on multidimensional scaling. ACM Comput Surv (CSUR) 51:1–25
Hout MC, Papesh MH, Goldinger SD (2012) Multidimensional scaling. Wiley Interdiscip Rev Cogn Sci 4:93–103
Kaur H, Singh SP (2018) Heuristic modeling for sustainable procurement and logistics in a supply chain using big data. Comput Oper Res 98:301–321. https://doi.org/10.1016/j.cor.2017.05.008
Article MathSciNet Google Scholar
Chen F, Chen S, Ma X (2018) Analysis of hourly crash likelihood using unbalanced panel data mixed logit model and real-time driving environmental big data. J Saf Res 65:153–159. https://doi.org/10.1016/j.jsr.2018.02.010
Yuan Z, Zhou X, Yang T (2018) Hetero-ConvLSTM: a deep learning approach to traffic accident prediction on heterogeneous spatio-temporal data. In: Proceedings of the ACM SIGKDD international conference on knowledge discovery and data mining. Association for computing machinery, pp 984–992
Riswan P, Suresh K, Babu MR (2016) Real-time smart traffic management system for smart cities by using internet of things and big data. In: ICETT - 2016 : international conference on emerging technological trends in computing, communications and electrical engineering. IEEE, pp 1–7
He Z, Qi G, Lu L, Chen Y (2019) Network-wide identification of turn-level intersection congestion using only low-frequency probe vehicle data. Transp Res Part C Emerg Technol 108:320–339. https://doi.org/10.1016/j.trc.2019.10.001
Zhou Z, Gao C, Xu C, Zhang Y, Mumtaz S, Rodriguez J (2018) Social big-data-based content dissemination in internet of vehicles. IEEE Trans Ind Inf 14:768–777. https://doi.org/10.1109/TII.2017.2733001
Guo L, Dong M, Ota K, Li Q, Ye T, Wu J, Li J (2017) A secure mechanism for big data collection in large scale internet of vehicle. IEEE Internet Things J 4:601–610
Sumalee A, Ho HW (2018) Smarter and more connected: future intelligent transportation system. IATSS Res 42:67–71
Fetene GM, Kaplan S, Mabit SL, Jensen AF, Prato CG (2017) Harnessing big data for estimating the energy consumption and driving range of electric vehicles. Transp Res D Transp Environ 54:1–11. https://doi.org/10.1016/j.trd.2017.04.013
Tu W, Li Q, Fang Z, Shaw S, lung, Zhou B, Chang X, (2016) Optimizing the locations of electric taxi charging stations: a spatial–temporal demand coverage approach. Transp Res Part C Emerg Technol 65:172–189. https://doi.org/10.1016/j.trc.2015.10.004
Najada HA, Mahgoub I (2016) Autonomous vehicles safe-optimal trajectory selection based on big data analysis and predefined user preferences. In: EEE 7th annual ubiquitous computing, electronics mobile communication conference (UEMCON). IEEE, pp 1–6
Tu W, Cao R, Yue Y, Zhou B, Li Q, Li Q (2018) Spatial variations in urban public ridership derived from GPS trajectories and smart card data. J Transp Geogr 69:45–57. https://doi.org/10.1016/j.jtrangeo.2018.04.013
Wang Y, Ram S, Currim F, Dantas E, Sabóia L (2016) A big data approach for smart transportation management on bus network. In: IEEE international smart cities conference (ISC2), pp 1–6
Tsai CW, Hsia CH, Yang SJ, Liu SJ, Fang ZY (2020) Optimizing hyperparameters of deep learning in predicting bus passengers based on simulated annealing. Appl Soft Comput J. https://doi.org/10.1016/j.asoc.2020.106068
Liu WK, Yen CC (2016) Optimizing bus passenger complaint service through big data analysis: systematized analysis for improved public sector management. Sustainability 8:1319. https://doi.org/10.3390/su8121319
Li H, Parikh D, He Q, Qian B, Li Z, Fang D, Hampapur A (2014) Improving rail network velocity: a machine learning approach to predictive maintenance. Transp Res Part C Emerg Technol 45:17–26. https://doi.org/10.1016/j.trc.2014.04.013
Sharma S, Cui Y, He Q, Mohammadi R, Li Z (2018) Data-driven optimization of railway maintenance for track geometry. Transp Res Part C Emerg Technol 90:34–58. https://doi.org/10.1016/j.trc.2018.02.019
Jamshidi A, Hajizadeh S, Su Z, Naeimi M, Núñez A, Dollevoet R, de Schutter B, Li Z (2018) A decision support approach for condition-based maintenance of rails based on big data analysis. Transp Res Part C Emerg Technol 95:185–206. https://doi.org/10.1016/j.trc.2018.07.007
Thaduri A, Galar D, Kumar U (2015) Railway assets: a potential domain for big data analytics. Proced Comput Sci 53:457–467. https://doi.org/10.1016/j.procs.2015.07.323
Oneto L, Fumeo E, Clerico G, Canepa R, Papa F, Dambra C, Mazzino N, Anguita D (2017) Dynamic delay predictions for large-scale railway networks: deep and shallow extreme learning machines tuned via thresholdout. IEEE Trans Syst Man Cybern Syst 47:2754–2767. https://doi.org/10.1109/TSMC.2017.2693209
Sadler J, Griffin D, Gilchrist A, Austin J, Kit O, Heavisides J (2016) GeoSRM: online geospatial safety risk model for the GB rail network. IET Intell Transp Syst 10(1):17–24. https://doi.org/10.1049/iet-its.2015.0038
Gong L, Liu X, Wu L, Liu Y (2016) Inferring trip purposes and uncovering travel patterns from taxi trajectory data. Cartogr Geogr Inf Sci 43:103–114. https://doi.org/10.1080/15230406.2015.1014424
Xia F, Wang J, Kong X, Wang Z, Li J, Liu C (2018) Exploring human mobility patterns in urban scenarios: a trajectory data perspective. IEEE Commun Mag 56:142–149. https://doi.org/10.1109/MCOM.2018.1700242
Qiu J, Du L, Zhang D, Su S, Tian Z (2020) Nei-TTE: intelligent traffic time estimation based on fine-grained time derivation of road segments for smart city. IEEE Trans Ind Inf 16:2659–2666. https://doi.org/10.1109/TII.2019.2943906
Zhou Z, Dou W, Jia G, Hu C, Xu X, Wu X, Pan J (2016) A method for real-time trajectory monitoring to improve taxi service using GPS big data. Inf Manag 53:964–977. https://doi.org/10.1016/j.im.2016.04.004
Xu X, Zhou JY, Liu Y, Xu ZZ, Zha XW (2015) Taxi-RS: taxi-hunting recommendation system based on taxi GPS data. IEEE Trans Intell Transp Syst 16:1716–1727. https://doi.org/10.1109/TITS.2014.2371815
Zhang SK, Shi GY, Liu ZJ, Zhao ZW, Wu ZL (2018) Data-driven based automatic maritime routing from massive AIS trajectories in the face of disparity. Ocean Eng 155:240–250. https://doi.org/10.1016/j.oceaneng.2018.02.060
Gan S, Liang S, Li K, Deng J, Cheng T (2016) Ship trajectory prediction for intelligent traffic management using clustering and ANN. In: 2016 UKACC 11th international conference on control (CONTROL), pp 1–6
European Union (EU) Horizon 2020 (H2020) (2017) NOESIS: novel decision support tool for evaluating strategic big data investments in transport and intelligent mobility services. https://cordis.europa.eu/programme/id/H2020_MG-8-2-2017/en . Accessed 29 Sep 2023
Download references
Acknowledgements
This research is financed by the Research, Innovation and Excellence Program of the University of Thessaly.
Open access funding provided by HEAL-Link Greece. This research is supported by the Research, Innovation and Excellence Program of the University of Thessaly.
Author information
Authors and affiliations.
Department of Civil Engineering, University of Thessaly, Volos, Greece
Danai Tzika-Kostopoulou & Eftihia Nathanail
Department of Digital Systems, University of Thessaly, Larissa, Greece
Konstantinos Kokkinos
You can also search for this author in PubMed Google Scholar
Contributions
The authors confirm their contribution to the paper as follows: study conception and design done by DT and EN; DT helped in data collection; DT, EN, and KK done analysis and interpretation of results, draft manuscript preparation. All authors reviewed the results and approved the final version of the manuscript.
Corresponding author
Correspondence to Danai Tzika-Kostopoulou .
Ethics declarations
Conflict of interest.
The authors have no competing interests to declare that are relevant to the content of this article.
Additional information
Publisher's note.
Springer Nature remains neutral with regard to jurisdictional claims in published maps and institutional affiliations.
Rights and permissions
Open Access This article is licensed under a Creative Commons Attribution 4.0 International License, which permits use, sharing, adaptation, distribution and reproduction in any medium or format, as long as you give appropriate credit to the original author(s) and the source, provide a link to the Creative Commons licence, and indicate if changes were made. The images or other third party material in this article are included in the article's Creative Commons licence, unless indicated otherwise in a credit line to the material. If material is not included in the article's Creative Commons licence and your intended use is not permitted by statutory regulation or exceeds the permitted use, you will need to obtain permission directly from the copyright holder. To view a copy of this licence, visit http://creativecommons.org/licenses/by/4.0/ .
Reprints and permissions
About this article
Tzika-Kostopoulou, D., Nathanail, E. & Kokkinos, K. Big data in transportation: a systematic literature analysis and topic classification. Knowl Inf Syst (2024). https://doi.org/10.1007/s10115-024-02112-8
Download citation
Received : 06 August 2023
Revised : 26 January 2024
Accepted : 21 March 2024
Published : 08 May 2024
DOI : https://doi.org/10.1007/s10115-024-02112-8
Share this article
Anyone you share the following link with will be able to read this content:
Sorry, a shareable link is not currently available for this article.
Provided by the Springer Nature SharedIt content-sharing initiative
- Transportation
- Topic model
- Classification
- Term frequency–inverse document frequency method
- Find a journal
- Publish with us
- Track your research
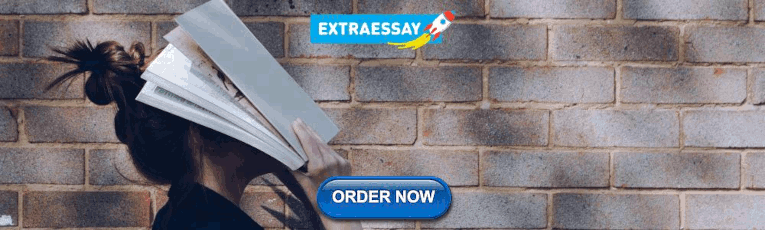
IMAGES
VIDEO
COMMENTS
First, articles relevant to Swedish spatial planning were identified through a search in Web of Science, performed in June 2020. Different urban greening concepts including 'green infrastructure', 'green wedge', 'green belt' and 'nature-based solution' and as well other relevant related concepts such as 'ecosystem services' and 'biodiversity' were used as search keywords.
1996. This report reviews current literature on sustainable development and proposes a framework for applying this concept to city and regional planning. It begins by exploring interpretations of the concept of sustainability itself, next looks at some urban planning traditions toward an urban planning framework that can incorporate this concept.
Journal of Planning Literature (JPL) published quarterly, includes review articles, perspective pieces, and abstracts of recent literature in city and regional planning and design. A typical issue contains refereed literature reviews; a Council of Planning … | View full journal description. This journal is a member of the Committee on ...
A growing body of literature (Freire, 2006; Jooste et al., 2019) report on the challenges surrounding urban planning in the global south, irrespective of the substantive efforts made to control ...
Urbanization is a defining feature of the modern age, yet the current model of urban development profoundly alters the natural environment, often reducing biodiversity and ultimately threatening human wellbeing. An ecologically based urban planning and design paradigm should consider a more harmonious relationship. Through a systematic literature review of 57 papers, this research identified ...
Historically urban design and urban planning both branched off from architecture theories, while planning has been defined as independent discipline for more than a century, urban design emerged only in 1960′s as planning focus more on land-use, social aspect and infrastructure apart from physical qualities, which was the focus of urban ...
Against this background, this study aims to provide a review of the literature published on Urban Studies and Regional & Urban Planning over the past seven decades. More specific objectives are identifying influential authors, sources, and references that have contributed to the field's evolution, mapping the dominant areas of research foci ...
Urbanization has rapidly transformed the global landscape, and its impact on ecology and biodiversity has a critical concern for sustainable development. Integrating urban planning and ecology can help to address these impacts by promoting sustainable urban development and protecting the ecosystem. In this research, a systematic literature review is conducted to explore the integration of ...
A key component of evidence-based approaches is to gather all the relevant findings on a topic by, say, completing a literature review (Krizek et al., 2009 ). Indeed, K. Stevens ( 2001) called systematic reviews in health research "the heart of evidence-based practice" (p. 529). Although both urban planning and public health conduct ...
Keywords: urban planning; systematic literature review; ecosystem services; urban consonance 1. Introduction The impact of human activity on the Earth's environmental systems is now so dominant that it is recognised as a new geological age: the Anthropocene, or human-dominated geological epoch [1].
With regard to urban management, the concept "smart cities" leaves new urban challenges out of reach. These challenges not only require improved efficiency, but also demand approaches based on sustainability and resilience [].To better understand how the problems related to Cognitive Urban Planning have been addressed, this section describes a systematic literature review (SLR) of the ...
Systematic literature review helps synthesize scattered knowledge, findings, and reporting from the previous research to offer a holistic perspective on a particular area of interest. ... To obtain relevant and accurate results, the following keywords were used 'smart city', 'urban planning', 'environment', and 'sustainable ...
In this study, a review of the sustainable urban planning challenges was conducted. The challenges are then grouped into topics that align with the theme of the type of challenge. Finally, the challenges are analysed to identify the most well-known and disruptive challenges that restrict urban planners in developing countries.
An ecologically based urban planning and design paradigm should consider a more harmonious relationship. Through a systematic literature review of 57 papers, this research identified relevant ...
Conducting a Literature Review. 1. Define a research question. Your literature review should be guided by a central research question. The research question should be neither too broad nor too narrow; it may help to start with a broad question and narrow it as you read through initial literature. 2.
A comprehensive exploration of the definitions of UBD in urban planning and related fields is timely and vital. This study is a systematic review of recent literature, consolidating 49 UBD definitions from 48 published articles in 39 journals, and classifying them into four themes: characteristics, sources, analytics, and impact.
The Literature Review by Lawrence A. Machi; Brenda T. McEvoy A clear, understandable six-step method for streamlining the literature review process! Written in user-friendly language, this resource offers master's and doctoral level students in education and the social sciences a road map to developing and writing an effective literature review for a research project, thesis, or dissertation.
Strategies for improving subjective well-being through urban planning. The review of the seven pathways above sheds light on potential strategies to improve SWB by applying urban policies and changing the built environment. In addition to policies and changes in the built environment, it is also urban planning as a process that can influence ...
Review the following websites for tips on writing a literature review: Literature Reviews. The Writing Center, University of North Carolina at Chapel Hill. Write a Literature Review: Virginia Commonwealth University
Find resources for urban and regional planning or design, including books, journal articles, maps and images, planning schemes and property information. ... A literature review is an assessment and critical analysis of the literature (what has been published) on a particular topic.
The concept of public interest legitimises the planning profession, provides a foundational principle, and serves as an ethical norm for planners. However, critical discourses highlight the problems of the assumptions underlying the notion of public interest in spatial planning. Using an explorative literature review approach, the article aims to analyse various interpretations and ...
The Chinese government pointed out that the level of urban planning, construction and governance should be improved, and urban renewal actions should be carried out to build livable cities. ... Section 2 presents the literature review; Section 3 provides theoretical analysis and research hypotheses; Section 4 outlines the setting of the ...
This paper unpacks a case of peri-urban planning that intends to integrate farming at a considerable scale in peri-urban development, i.e. the case of Oosterwold in Almere, the Netherlands. ... The case study departs with a literature review to comprehend peri-urban agriculture in the urban regions of Northwest Europe and comparable regions ...
In ecological research and urban planning, both software tools are frequently used to analyze scientific literature and visualize keyword co-occurrence maps, thematic clusters, and collaboration networks. In the followings, we demonstrate some recent studies that use VOSviewer and CiteSpace to review relevant literature:
To review the literature on urban food systems resilience. *Future studies on alternative resilient food networks should be done. (Wang et al., 2018) ... Exploring urban resilience thinking for its application in urban planning: A review of literature. International Journal of Environmental Science and Technology, 16 (2019), pp. 567-582.
ph.d. in urban and regional planning fall year 1 year 2 years 3-4 winter fall winter may urp 701 epistemology & reasoning for planning research ... comprehensive exam dissertation research and writing advance to candidacy informal full draft review dissertation defense submit final dissertation urp 700 elective towards primary or secondary ...
This paper identifies trends in the application of big data in the transport sector and categorizes research work across scientific subfields. The systematic analysis considered literature published between 2012 and 2022. A total of 2671 studies were evaluated from a dataset of 3532 collected papers, and bibliometric techniques were applied to capture the evolution of research interest over ...
The Systematic Literature Review identified five areas of importance: 1) efficiency, 2) road safety, 3) accessibility, 4) liveability and 5) new technologies. The statements composing this set refer to these and the key concepts of each area. In that sense our Q-method and Systematic Literature Review function as a unified and sequential approach.