Research support
See a list of all subjects a-z.
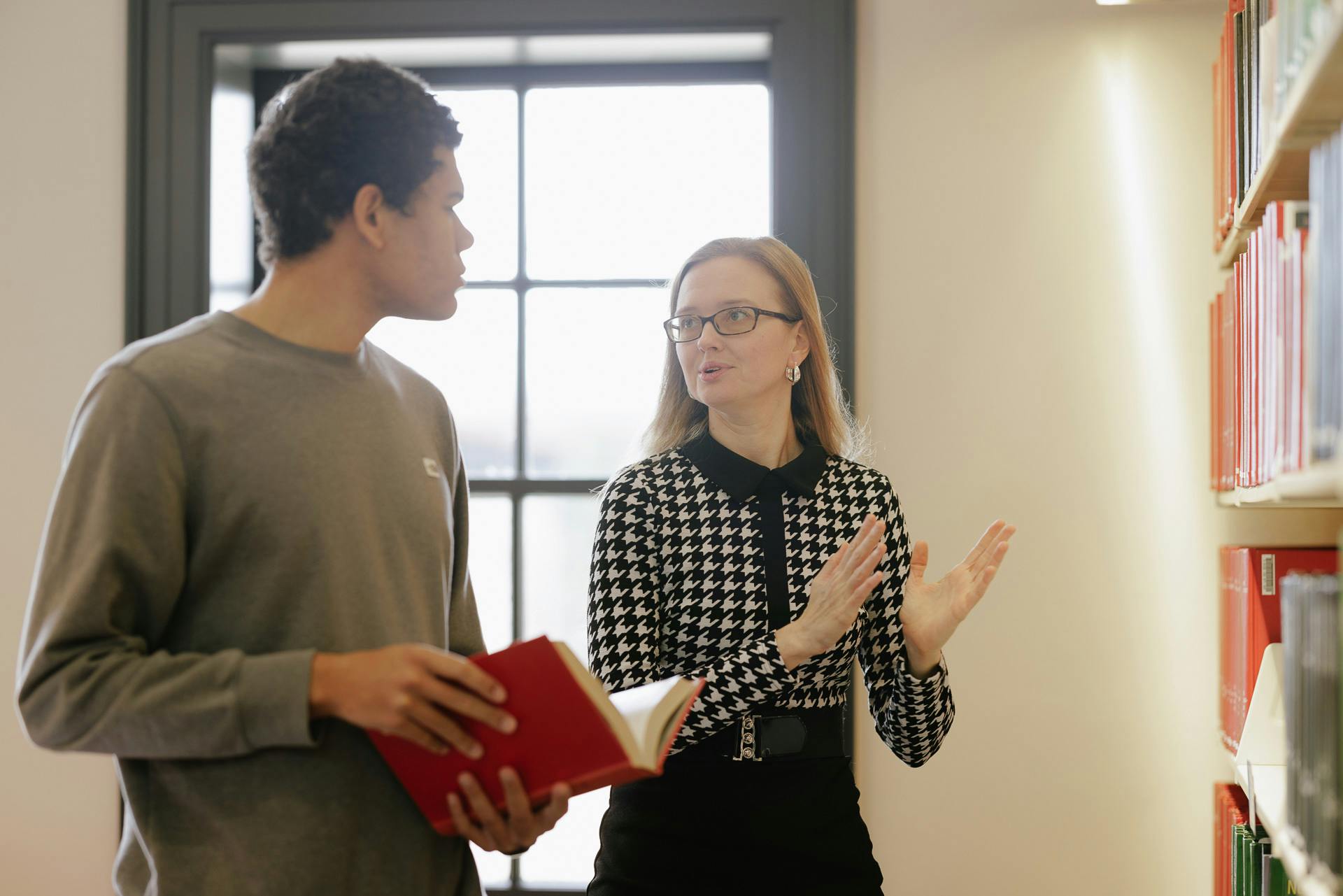
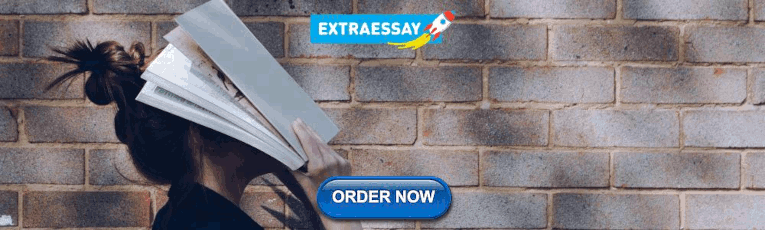
Find a subject specialist
We have over fifty subject specialists that can help you with your research. Whether you are new to Stanford or a seasoned researcher, we are here to help.

Guides are created by subject librarians to help you navigate the library.
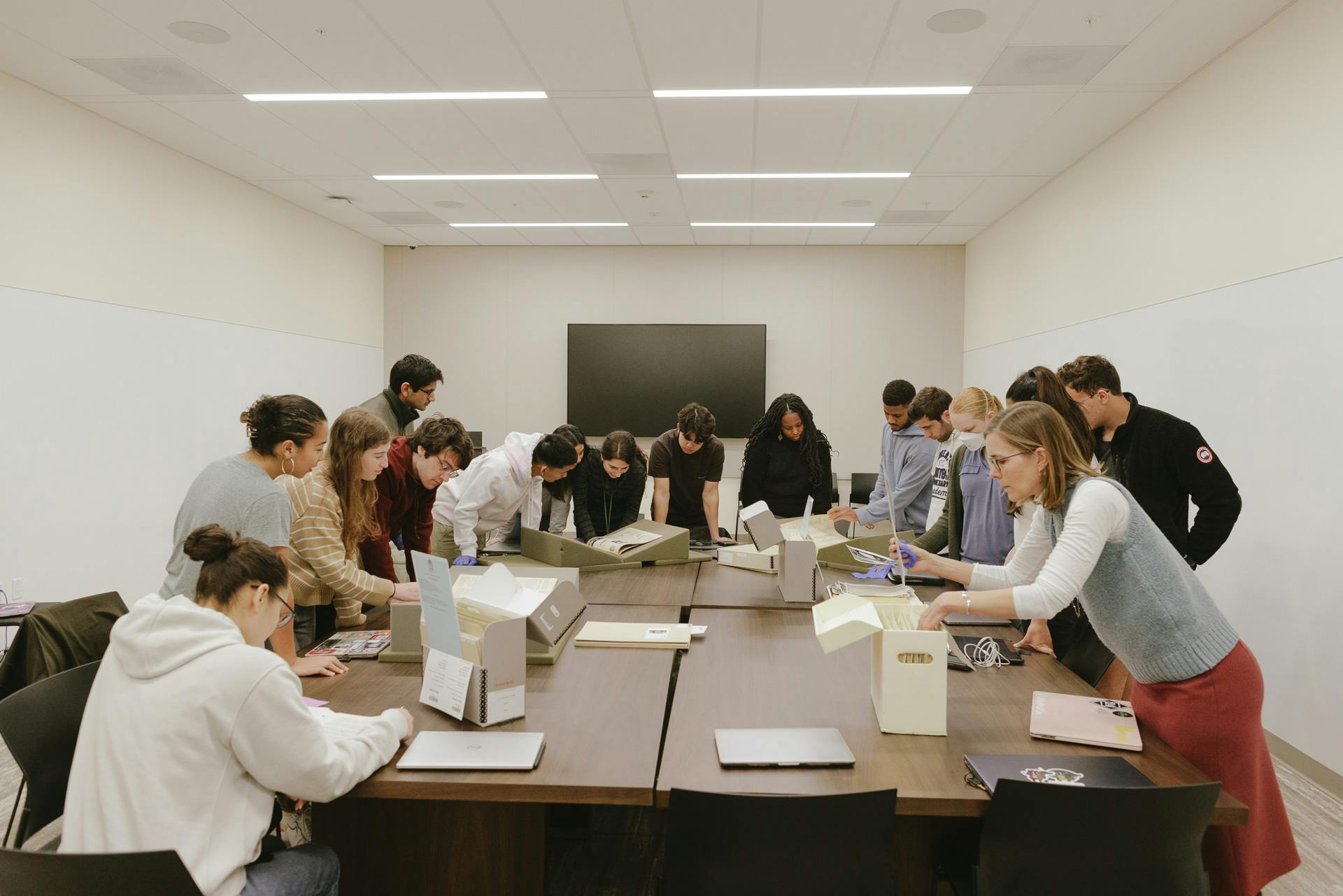
We offer hour-long workshops to get you up and running in software essential to research.
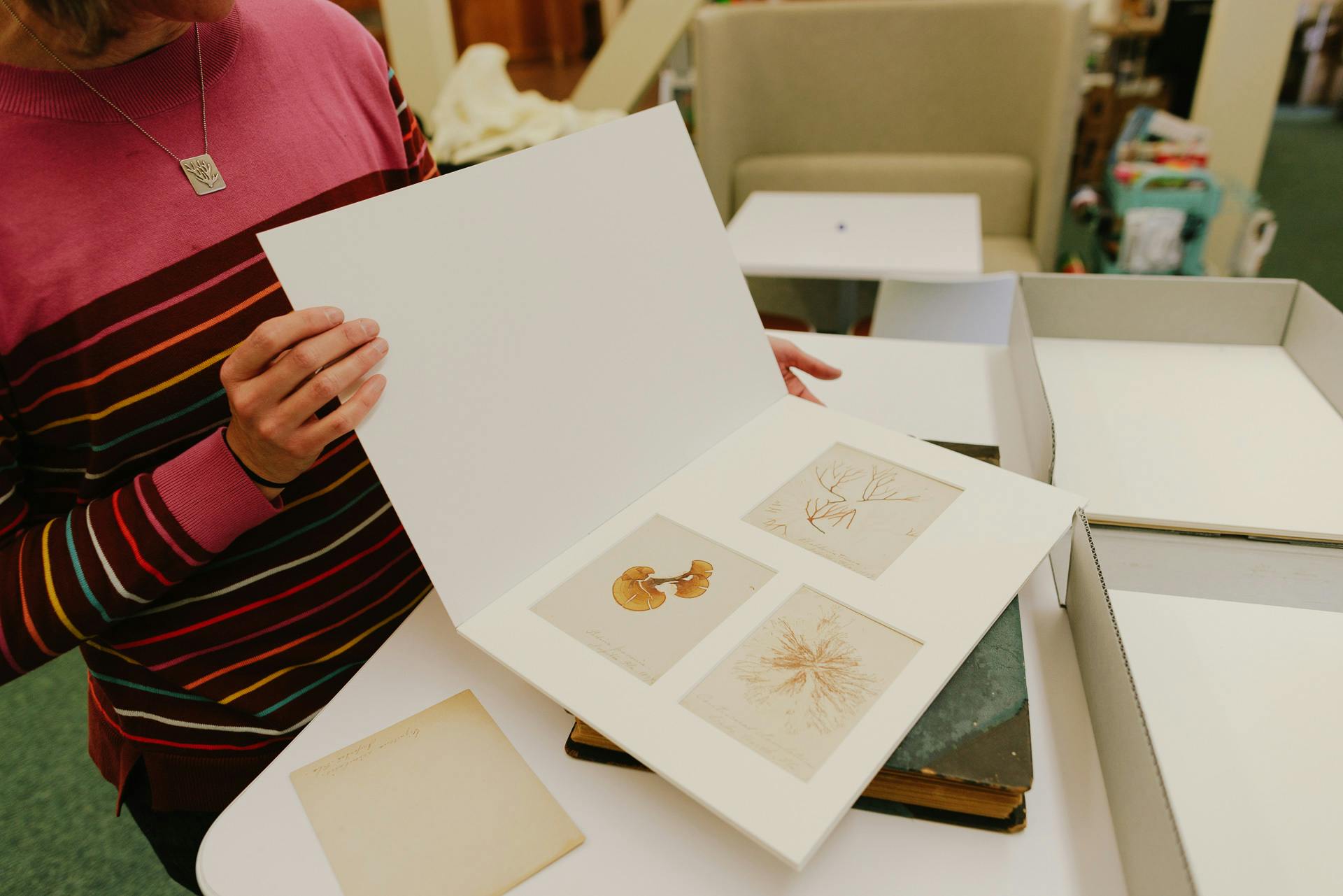
Increase your research impact
Make your research more discoverable and get help navigating the publishing landscape
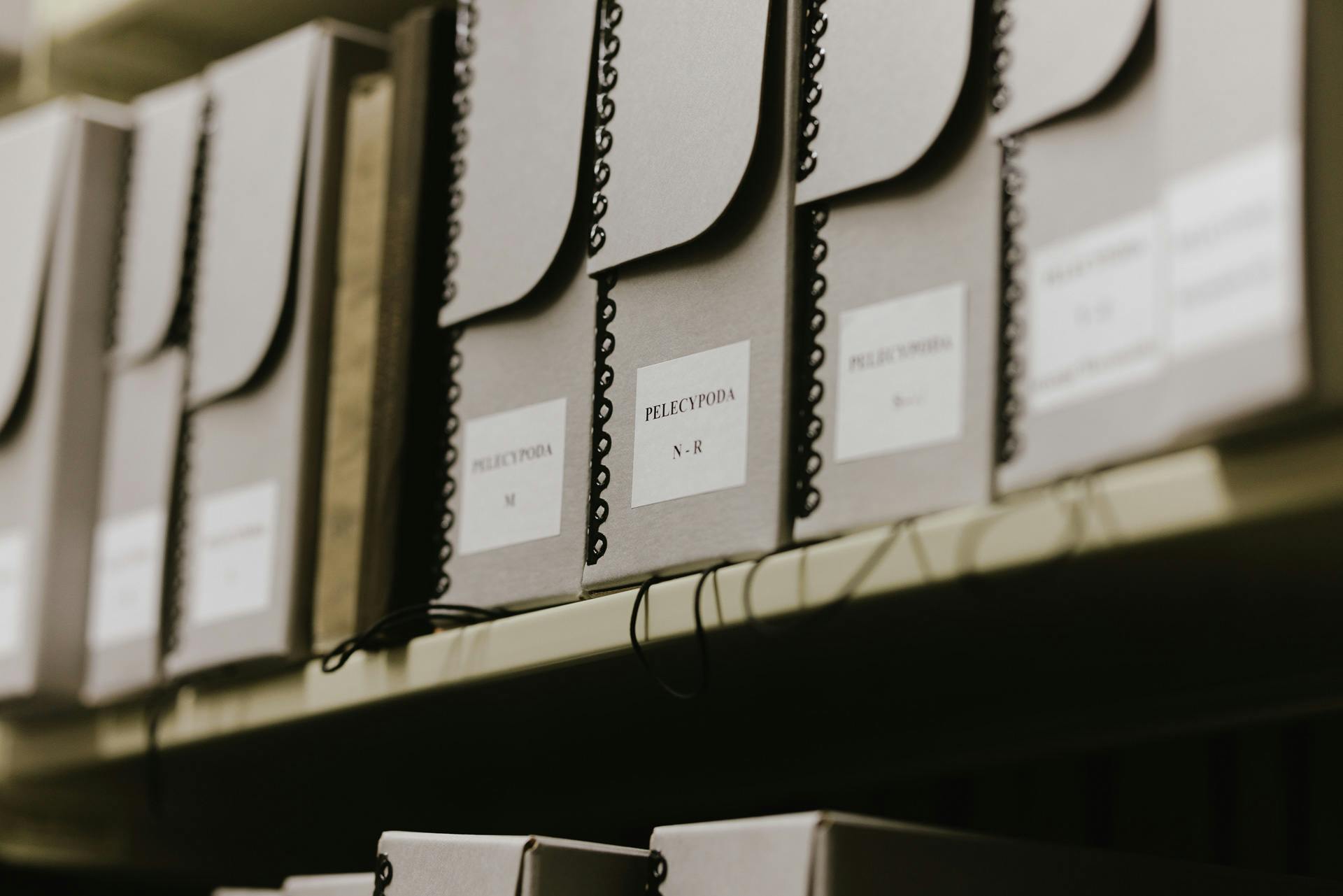
Data support and services
We offer comprehensive data support from research conception through publication.
General Research Support
We provide a wide range of resources and support for researchers as they work to advance scientific and medical discoveries.

Creating a safe working environment for our people and minimizing detrimental impact on our planet.
Laboratory Animal Care (APLAC)
Caring responsibly for animals involved in research studies to help advance science and fight diseases.
Data and Informatics
Working with research teams to provide advice on getting approvals, data abstraction, reporting, and storage.
Biostatistics, Epidemiology, and Research Design (BERD)
Giving investigators support for research design, data analysis, and interpretation.
Data Science Resources (SDSR)
Providing access to tools, datasets, data platforms, and methodologies for conducting innovative research.
Research Computing Center (SRCC)
Supporting programs that advance computational and data-intensive research across Stanford, including traditional high-performance computing (HPC) and resources for high throughput and data-intensive computing.
Stanford Program on Research Rigor & Reproducibility (SPORR)
Maximizing the scientific value of Stanford biomedical research through education, monitoring, and more.
Data Management & Sharing Plans
Contact John Borghi
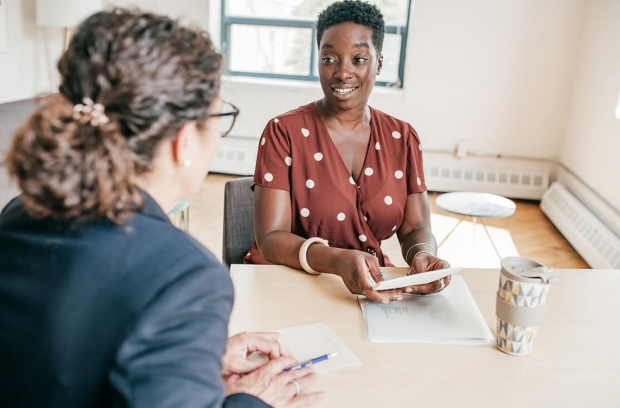
Conflicts of Interest (COI) Office
Supporting the Stanford community with disclosure and management of conflicts of commitment and interest.
Intellectual Property (OTL)
Promoting the transfer of Stanford technology for society’s benefit and generating income to support research and education.
School of Medicine Research Office
The Research Lifecycle
Inspired by an idea? We are your partners in curiosity.
Harvard Library drives progress. As much as we specialize in finding information that is known, we remain in awe of all that is unknown, and it is our privilege to join you in exploring the ideas that inspire new and renewed research efforts.
Together we can build a dream team of experts and share a variety of library discovery systems and tools for building on your curiosity.
Research Consultations
Let our collections inspire you.
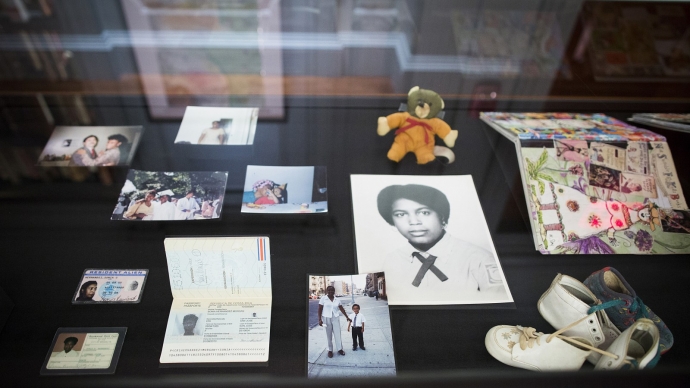
Explore Collections
Browse through materials Harvard curators have been collecting for nearly 300 years - physical, digital and beyond!
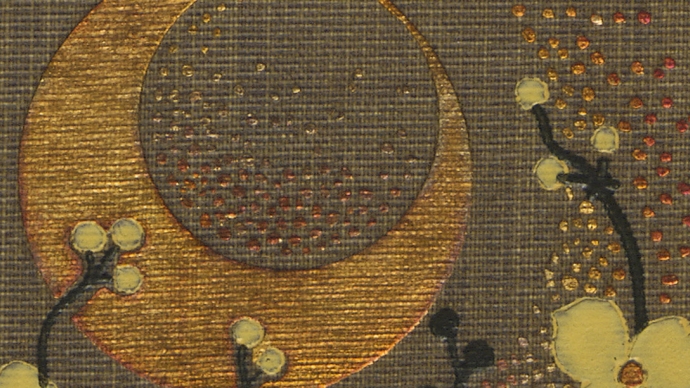
Harvard Digital Collections
Search and discover more than six million publicly available, digitized items. Make new discoveries and uncover hidden treasures.
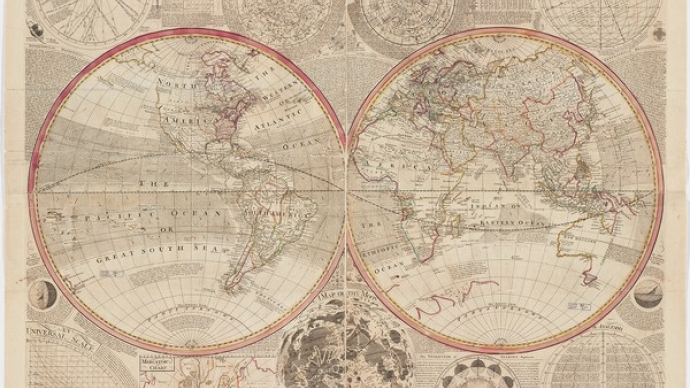
Take a closer look at Harvard's digital collections with CURIOSity's curated views and specialized search options.
We can collaborate to plan, scope, and organize your exploration.
Your research does not exist in isolation. Neither does Harvard Library. We bring people and ideas together to get you what you need. Whether you are planning original data collection, seeking out existing source material, identifying funding streams and support, or looking for opportunities to partner with other researchers, Harvard Library is here to help.
Bringing your research into focus, in whatever form it takes.
Partnership in research creates more interesting results. From the humanities to hard sciences, data visualization or qualitative analysis, Harvard Library staff can help you execute your research agenda and create the artifacts of your exploration.
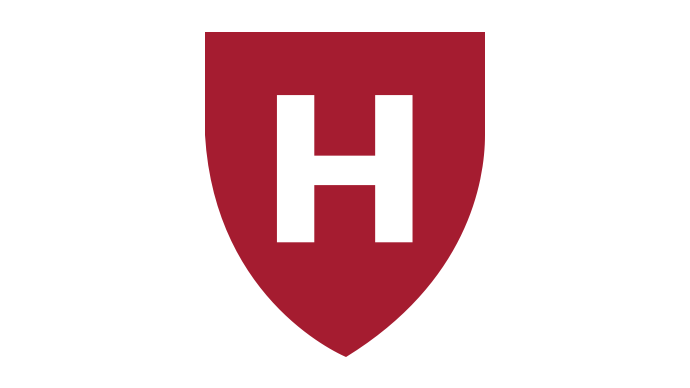
HOLLIS is the Harvard Library catalog. It includes books, articles, media and more. You can log in to renew items, check due dates and more.
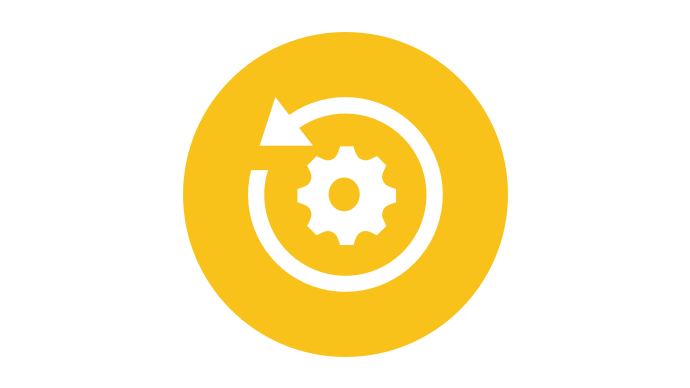
ILL & Scanning Services
Can't find the materials you need at Harvard? Need a book chapter or article scanned? Submit a request and we'll get you what you need.
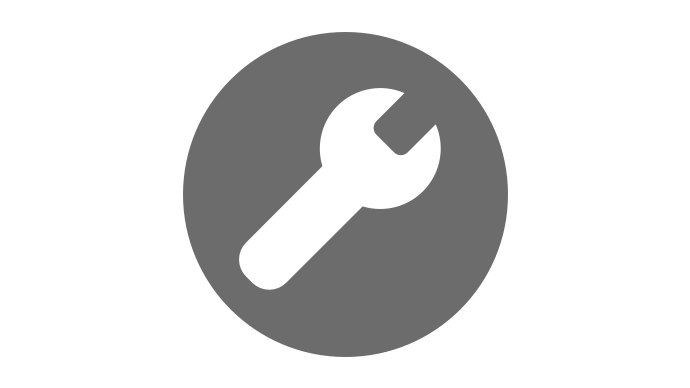
Lean Library
Use this browser extension to seamlessly access Harvard Library subscriptions from anywhere on the web.
Services for Research with Media and Technology
The hive: augmented and virtual reality studio, cabot media studios, lamont multimedia lab, tools for text and image research, hollis for archival discovery, hollis images, tools for geospatial and data research, harvard geospatial library, digital mapping and gis support.
A Master Class in Library Research for GSAS and DCE Students, offered in January.
Transform and translate your findings to create something new.
Harvard Library is a creative space. We build on inspiration, supporting your effort and springboarding your creative outputs to contribute to a diverse and robust global knowledge commons.
Visualization Support
Teaching and learning with 3d content.
Speed up the process of writing, editing and producing research papers, and collaborating with others. Free Overleaf Pro+ accounts available for all Harvard University students, faculty and staff.
Extending the reach of your data and the products of your research.
Harvard Library is committed to opening and broadening your scholarly reach to help share, promote, publish, and preserve your workspace and your work product.
Harvard Dataverse
Harvard Library is committed to prioritizing open access across disciplines - advancing equitable, open models of scholarly communication.
Harvard-Styles Open Access Policy
The basic idea of OA is simple: Make research literature available online without price barriers and without most permission barriers.
Open Science Publishing
One example of the Open Science movement is Pubfair - a modular, flexible, open-source framework for sustainable, distributed, open science publishing services.
Interenational Image Interoperability Framework (IIIF)
Harvard Library’s standard for providing access to more than 53,000,000 images from our libraries, archives, and museums.
Your research matters. We build pathways to measure the impact.
Harvard researchers change the world, and the world continues to change Harvard. In completing the research lifecycle, we want to ensure that you can track and measure your impact. How your work influences others and contributes to building a more diverse body of scholarship and furthering our collective intellectual curiosity.
The Library wishes you a nice holiday break. Buildings will be closed from 12/23/22 to 12/31/22. For a full list of closing and opening times, please visit the library hours page.
- Undergraduate Students
- Graduate & Medical Students
- Medical & Clinical Faculty
- Visiting Scholars
- Special Collections Researchers
- Library Staff
Brown University Library
Research support services.
Consultations
Library Experts
Request classes and workshops
Brown Digital Repository
Health and Biomedical Library Services
Center for Digital Scholarship
John Hay Library
Researchers@Brown
The Brown University Library provides support to researchers across all stages of the research cycle.
This site provides access to information and tools to plan, conduct, manage, disseminate and preserve research. Please contact [email protected] with any questions or requests for support.
Request a workshop for your course, lab, or department.
Citation Management Copyright and Fair Use Digital Scholarship Funding and Grant Support Finding, Analyzing, and Using Data Library Collections Literature Review Services
Publishing and Scholarly Communication Repository Services Research Consultations and Training Research Data Management Research Impact and Metrics Special Collections and Archival Research
- Citation Management
Citation Management tools to help you cite and manage your sources.
- Guidance for Formatting Citations for Publication
- Copyright and Fair Use
Help interpreting copyright, fair use, and how to obtain permission for use.
- Assistance With Obtaining Permissions
- Consultations to Determine Copyright Status and Application of Fair Use
- Guidance on Author’s Rights
Digital Scholarship
Support for the full lifecycle of digital scholarship projects, including working with data, creating and disseminating digital publications, and applying for grants.
- Brown University Digital Publications
- Creating and Processing Data Sets
- Data Visualization
- Digital Research Project Consulting, Design, and Implementation
- Digital Scholarship Methodologies
- Grant and Funding Support
- Locating and Accessing Data Sets
- Network Analysis
- Text Analysis and Data Mining
Funding and Grant Support
Guidance and resources for the grant application process and compliance.
- Guidance on Engaged Scholarship and Broader Impacts
- Research Data Management and Sharing Plans
- Scanning and Digitization
- Support for Public Access Compliance Mandates
Finding, Analyzing, and Using Data
How to find, create, process, visualize, and analyze data.
- Census and Demographic Data
- Financial and Business Data
- GIS and Geospatial Data
Library Collections
Access to Library collections to support research.
- Archival and Special Collections
- Interlibrary Loan and Borrow Direct
- Request Library Materials to Support Research
Literature Review Services
Library expert staff offer overviews and evaluations of existing literature on a research topic.
- Health Sciences Literature Review Service
- Literature Review Consultations
Publishing and Scholarly Communication
Resources for preserving and communicating your scholarly output, including Open Access.
- Acquire a Domain and Web Space
- Deposit Materials in the Brown Digital Repository
- Digital Object Identifier (DOI) Services
- Licensing, Publisher Agreements, and Publication Outlets
- Open Access Publishing and Public Access Compliance
- Open Educational Resources (OER)
Repository Services
Library infrastructure for storing and maintaining research materials and data.
- Deposit Research Data
Research Consultations and Training
Library experts are here to help you with your research projects in any discipline.
- Assistance with Research Strategy, and Finding and Locating Information
- Consultations for Digital Projects
- Current Awareness and Research Alerts
- Entrepreneurial Research
- Guides and Tutorials for Self-Guided Training
- Training for Researchers and Assistants
Research Data Management
Expertise on data curation and management as well as resources for storing and publishing data.
- Data Curation
- Data Management and Sharing Plans
- Data Rights and Permissions
- Electronic Lab Notebooks
- Publishing and Citing Research Data
Research Impact and Metrics
Resources for assessing and demonstrating the research impact.
- Journal Impact and Citation Metrics
- Research Impact for Individuals and Departments/Centers
- Researcher Identity
Special Collections and Archival Research
Access and use Brown’s special collections and archives.
- Access to Archival and Special Collections
- Permissions and Publications
- Records Management
- Reproduction Services
- Research Support and Consultations for Brown Community and Greater Public
- Utility Menu

Harvard researchers have access to a wide range of service offerings across the University. From planning a project or study, to sharing and archiving methods or findings, our services span the entire research lifecycle. This website brings together Harvard’s offerings across central units and schools, including support for research administration and compliance, data management and scholarship, and research computing.
Browse by Service Type
Research administration and compliance →.
Harvard offers a full spectrum of resources to support and facilitate research and researcher compliance with internal and external regulations and policies. Services available include administrative reviews, consultations, guidance, and tools. Resources span the research lifecycle, including pre- and post-award services, human subject and animal research resources, data use agreement support, and research data safety resources.
Research Computing →
Research Computing at Harvard facilitates the advancement of research by providing leading-edge computing services including cluster computing, storage, software licenses, virtual instances, and software engineering. Services range from consultation and guidance to fully managed support. Guidance about working with data in compliance with Harvard's data security levels is also available from Research Computing specialists.
Research Data and Scholarship →
Researchers at Harvard generate data and scholarship that changes the world. Services across the University are available to support data creation, curation, and transformation, as well as research publishing and dissemination. Research support partners are available for consultation, providing access to existing information and tools, and exposing research through robust repositories for data and scholarly communication.
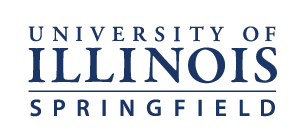
Research Support for Online Students
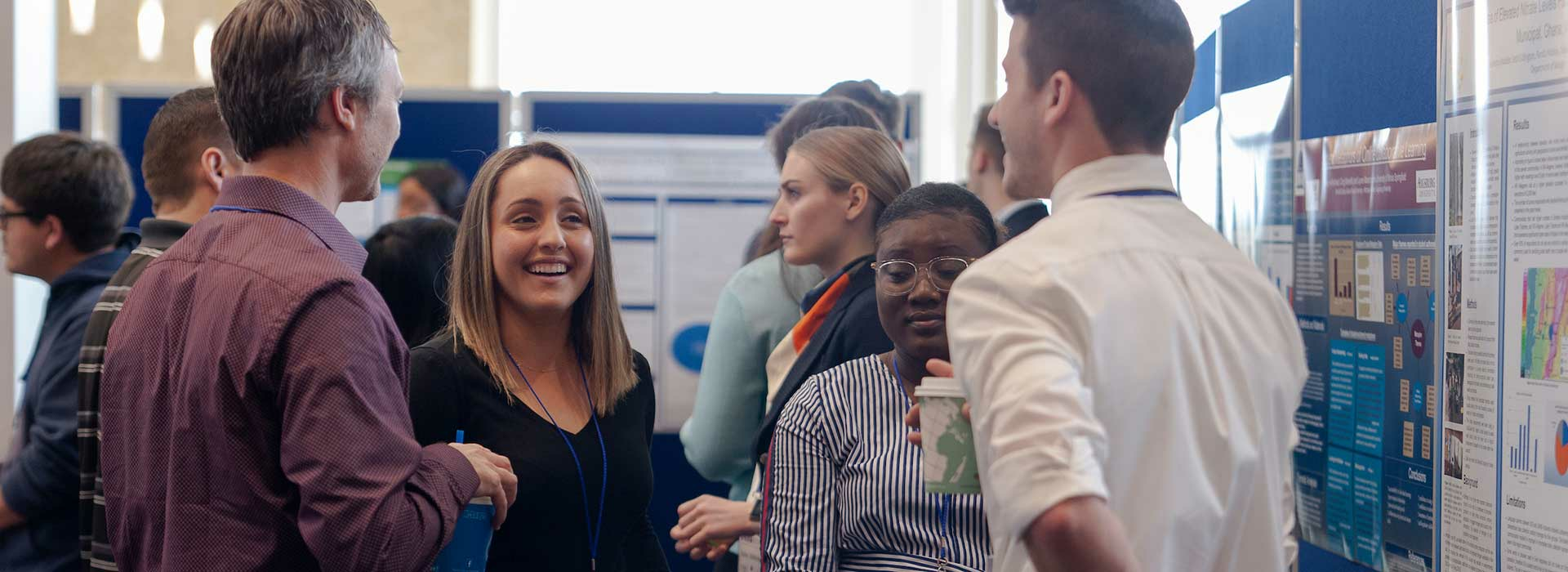
- Quick links Applicants & Students Important Apps & Links Alumni Faculty and Staff Community Admissions How to Apply Cost & Aid Tuition Calculator Registrar Orientation Visit Campus Academics Register for Class Programs of Study Online Degrees & Programs Graduate Education International Student Services Study Away Student Support Bookstore UIS Life Dining Diversity & Inclusion Get Involved Health & Wellness COVID-19 United in Safety Residence Life Student Life Programs UIS Connection Important Apps UIS Mobile App Advise U Canvas myUIS i-card Balance Pay My Bill - UIS Bursar Self-Service Email Resources Bookstore Box Information Technology Services Library Orbit Policies Webtools Get Connected Area Information Calendar Campus Recreation Departments & Programs (A-Z) Parking UIS Newsroom Connect & Get Involved Update your Info Alumni Events Alumni Networks & Groups Volunteer Opportunities Alumni Board News & Publications Featured Alumni Alumni News UIS Alumni Magazine Resources Order your Transcripts Give Back Alumni Programs Career Development Services & Support Accessibility Services Campus Services Campus Police Facilities & Services Registrar Faculty & Staff Resources Website Project Request Web Services Training & Tools Academic Impressions Career Connect CSA Reporting Cybersecurity Training Faculty Research FERPA Training Website Login Campus Resources Newsroom Campus Calendar Campus Maps i-Card Human Resources Public Relations Webtools Arts & Events UIS Performing Arts Center Visual Arts Gallery Event Calendar Sangamon Experience Center for Lincoln Studies ECCE Speaker Series Community Engagement Center for State Policy and Leadership Illinois Innocence Project Innovate Springfield Central IL Nonprofit Resource Center NPR Illinois Community Resources Child Protection Training Academy Office of Electronic Media University Archives/IRAD Institute for Illinois Public Finance
- Request Info Request info for.... Undergraduate/Graduate Online Study Away Continuing & Professional Education International Student Services General Inquiries
Online Student Support Programs at UIS
UIS supports online students with lots of resources to enable them thrive, regardless of their location at UIS and beyond.
- Brookens Library Research Assistance for Students and Faculty
- Conferences and Publications
- Internship Office
- Other Useful Resources
Get Involved!
Office of Internships and Prior Learning : Learn about recent and available project internships.
Faculty Fellows : Learn about current research fellows and how you can apply to participate. Some UIS faculty are willing to distance mentor undergraduate research student, so communicate with your online instructors.
Faculty Publications : View the repository of faculty research organized by the Center for Online Learning, Research, and Service
Follow us on Facebook , Twitter , Instagram , and at our blog .
Share your research or creative activities by completing the Student Research Highlights form .
- President’s Research in Diversity Travel Award Program (application deadlines twice a year) Check with your college for other funding opportunities (eg. CLAS Student-Faculty Creative Activities program)
- Brookens Library
- Center for Online Learning, Research, and Service [COLRS]
Online student support: a framework for embedding support interventions into the online learning cycle
- O. Rotar ORCID: orcid.org/0000-0001-7298-6444 nAff1
Research and Practice in Technology Enhanced Learning volume 17 , Article number: 2 ( 2022 ) Cite this article
20k Accesses
25 Citations
9 Altmetric
Metrics details
Support is one of the crucial elements of online students’ success. Although many support strategies have been documented in the past, less is known at what stages of the learning cycle suggested interventions can be embedded into the online learning curriculum. This paper offers a systematic review of the 28 empirical studies on effective support strategies and interventions that are indexed by the SCOPUS database between 2010 and 2020. Following an Inclusive Student Services Process Model framework, identified strategies are allocated across different phases of student learning to indicate where and when they can be delivered to online students. The analysis suggests that the effectiveness of the support provision depends on the time when support is offered. Furthermore, it was found that two areas support delivery, namely support at transitions and measurement of support interventions, remain under-researched. Finally, the analysis showed two emerging trends in online students support: an increasing role of technology and social network sites to design support interventions and a shift to a more personalised yet holistic approach to student support.
Introduction
Successful learning at a distance requires students to possess specific skills and attributes. As Brindley ( 2014 ) emphasised, “studying at a distance requires maturity, a high level of motivation, the capacity to multi-task, goal-directedness, and the ability to work independently and cooperatively” (p. 278). Furthermore, she stated that,
Distance learners are expected to plan their academic programs, set their study schedules, balance their studies with other responsibilities (work/family), communicate proficiently in writing, find and use learning resources well, and read and synthesise efficiently. Those distance learners studying in cohorts are expected to collaborate effectively with their peers in virtual groups, and those studying in self-paced courses are often expected to create their own learning networks (Brindley, 2014 , p. 278).
However, mentioned above prerequisites may not be present in all students, and many online learners face challenges and barriers in their learning (Martin & Bolliger, 2018 ; Stone, 2017 ; Woodley & Simpson, 2014 ). It is widely recognised that student support is critical for overcoming barriers to learning and ensuring learner engagement, motivation and success in online higher education (see Rotar, 2020 ; Muljana & Luo, 2019 ). Although many support strategies are available for review, there were no attempts to systematically analyse them in relation to different stages of student learning. As a result, there is a lack of understanding of where and when student support can be embedded into the online learning curriculum (Rumble, 2000 ). To address limitations of the past research, this study poses the following research question: What support strategies and interventions can be offered to online students at different phases of the learning cycle?
By bringing together research on reported support strategies and interventions, this paper aims to generate insights into the development of a framework for embedding support interventions into the online learning cycle. In doing so, this paper offers a unique contribution to the research on student support within the field of online education.
Literature review
A provision of student support is a necessary element of online student success (Rumble, 2000 ) that has been recognised in the past (Woodley & Simpson, 2014 ) and continues to be relevant within the research on student’s attrition, retention, and dropout (Rotar, 2020 ). Different support models offer valuable conceptual frameworks for thinking about the approach to online student support (see Floyd & Casey-Powell, 2004 ; Ryan, 2004 ; Simpson, 2008 ). Ryan ( 2004 ) advocated a “centrality of student–student communication for retention and study success” (p. 131). He proposed a logical framework for maintaining support for online learners with a student as a centre of the model and argued that his framework “is best situated within the knowledge of the distance student’s lifecycle, from initial interest in distance education as a possible avenue for study, to inquiry at a particular institution, through academic counselling, to study and eventual graduation” (p. 127).
Floyd and Casey-Powell ( 2004 ) offered an Inclusive Student Services Process Model (ISSPM) where they summarised characteristics of successful support service and distinguished five phases of the learning cycle where support can be provided. Another model developed by Simpson ( 2008 ) advocates the need for proactive rather than reactive support interventions. Simpson’s Proactive Motivational Support model (Simpson, 2008 ) contains motivation and psychology elements and determines a proactive student outreach as an effective support intervention.
The models of online student support evolved since the invention of the Internet. The Internet facilitated the emergence of customised and automated services that can be delivered by computers rather than humans (Brindley, 2014 ; Dollingeret al., 2020 ; Walsh et al., 2020 ). It also enabled an opportunity to include social elements, e.g., online interactions and engagement, into support interventions. As Zawacki-Richter and Anderson ( 2014 ) emphasised, “the online world itself affords new tools for communication, knowledge and skill acquisition, and peer and group support that was not available to earlier generations of distance students” (p. 23).
Within the discourse on the affordances of the Internet, Moore and Kearsley ( 2012 ) introduced a theory of transactional distance that placed a significant emphasis on the development of an understanding of the very concept of online learner support. A new approach presented online student support as an intervention that intends to decrease a transactional distance between learners, a tutor, and an educational institution and helps the learner develop autonomy.
Whilst offering multiple benefits for providing new forms of student support via advanced technological and pedagogical tools, there has been a criticism of the potentially oppressive nature of online learning environments (Öztok, 2019 ; Rice et al., 2020 ). Such criticism resulted in the turn to the humanistic view on online student support, which placed a greater emphasis on personalised learner support. Research on online students’ experiences and perceptions also confirmed that embodied humanistic (as opposed to mechanistic) approaches for online learning support are critical for transformative learning experiences (Brown & Wilson, 2016 ; Sewart, 1993 ; Stone, 2019 ; Thorpe, 2002 ).
The reviewed literature highlights the evolution of support strategies from depersonalised and additional services into the more targeted and tailored to diverse student bodies’ needs, emphasises the increasing utilisation of the affordance of the Internet in offering support interventions. As Brindley ( 2014 ) states, “support systems for distance learners have become more proactive, more purposeful, and more effective in helping learners succeed in their studies”. (p. 305). Furthermore, the literature suggests that the consideration of the stage where support is offered is essential for the support strategy to be effective (Floyd & Casey-Powell, 2004 ; Ryan, 2004 ). However, little research has been done to systematically analyse specifically designed for online students support strategies or interventions in relation to the stages where they can be best implemented. By bringing together research on online student support strategies and interventions, this paper aims to generate insights into the development of the embedded support system that incorporates different phases of the learning cycle. In doing so, this paper offers a unique contribution to the research on student support within the field of online education.
Analytical frame
To analyse the identified support strategies and interventions, I employ an Inclusive Student Services Process Model (ISSPM) developed by Floyd and Casey-Powell ( 2004 ). The ISSPM is based loosely on Miller and Prince’s ( 1976 ) student development process model, which was intended to “meet the needs of all students, to plan for change rather than react to it, and to engage the full academic community in this collaborative effort” (p. 21).
In the ISSPM, Floyd and Casey-Powell ( 2004 ) summarised characteristics of successful support services and distinguished five phases of the learning cycle where support can be provided namely the student intake phase, student intervention phase, student support phase, the student transition phase, and a measurement phase. The focus during each phase is summarised in Table 1 .
The ISSPM framework is useful for the analysis as it identifies essential tasks for each stage of student learning and provides examples of support services that can be offered. The five stages of this multidimensional model offer a systemic and targeted approach to student support, placing the needs of students upfront. Furthermore, it suggests that the successfully support service holds the potential to benefit both learners and an educational institution. Thus, the application of the model as an analytical frame provides a wide angle for the analysis of the existing online student support strategies and interventions and assists in addressing the research question.
Methodology
This paper offers a systematic review of the online student support strategies and interventions reported over the last ten years. Furthermore, it provides a thematic analysis of recommendations for student support offered in the empirical studies in relation to the five stages of the learning cycle where identified strategies fit the best.
To examine online students’ support strategies and interventions, I considered studies published from 2010 to 2020 in peer-reviewed journals. To identify relevant studies, a set of inclusion and exclusion criteria has been set:
Due to the application of the ISSPM (Floyd & Casey-Powell, 2004 ) as an analytical frame, only research focused on the conception of online student support within the scope of five stages of the learning cycle: (1) intake stage, (2) orientation stage, (3) support stage, (4) transition stage and (5) measurement stage has been included into the analysis.
Only empirical studies have been considered. Non-empirical and opinion papers were excluded.
Only research that reported a concrete support strategy or support intervention within the scope of five stages of the learning cycle had been included in the further analysis.
Only peer-reviewed papers published in the English language between 2010 and 2020 have been considered. The ten-year period has been set to examine the most up-to-date literature on online students’ support.
Identification of the relevant studies
I adopted the identification process that involved three phases: searching, screening and analysis (Karabulut-Ilgu et al., 2018 ; Muljana & Luo, 2019 ).
Relevant studies were retrieved through a series of search efforts. Eligible research that meets the selection criteria were then identified. The search was carried out in two stages. First, an initial search was performed in the Scopus database. Keyword searches were conducted using the combinations of terms “support strateg*”, “support intervention*”, “student support” AND “online education”, “online higher education”, “online learning”, “distance learning”, “distance education”. Keywords have been selected to reflect the scope of this research and the research question. As a result, I identified 180 peer reviewed articles from this phase of searching.
The screening process aimed to identify relevant studies by reading the abstract of the paper and by application of the selected criteria. During this process, articles that did not meet the inclusion criteria (e.g., non-empirical articles, opinion papers, not peer-reviewed papers) were excluded from the further analysis. Additionally, duplicate reports of the same study were eliminated. The abstract screening yielded 38 peer-reviewed articles on the topic of interest for this paper, published between 2010 and 2020.
The analysis process consisted of full-text reading. At this stage, several non-empirical studies and studies that deviate from the focus of this paper (e.g., articles that reported support interventions that do not fall into any phase of the learning cycle) have been identified and excluded from the analysis. The process of analysis resulted in a pool of 28 articles. All articles were arranged in tabular form. Five phases of the learning cycle have been analysed applying the ISSPM framework (Floyd & Casey-Powell, 2004 ). During the analysis phase, I identified additional literature that did not meet inclusion criteria but was relevant for enhancing the analysis and discussion.
In the following part of the paper, I discuss the results of the systematic literature review of the studies that reported online student support strategies and interventions. The summary of the identified relevant studies is presented in Table 1 . The Table contains the details of selected studies, including author (s), publication date, considered strategy, and its effectiveness.
Following the ISSPM framework, the identified support strategies, recommendations and interventions are allocated across the five phases of the educational life cycle to indicate the areas where they can be embedded into the online learning curriculum.
Student Intake Phase
Advising prior to enrolment.
Past research advocates the provision of advising services on various aspects of learning prior to the enrolment (Cain & Lockee, 2002 ; Clay et al., 2008 ). It was found that the lack of comprehensive information about the chosen online programme was a main cause of misconceptions about requirements and a difficulty of the course (Clay et al., 2008 ). The review of the considered studies also supported the importance of the pre-enrolment advising. Gaytan ( 2015 ) believes that academic advisors must articulate study agreements regarding the credit transfer more clearly and provide advising strategies to ensure that students receive credit for previous coursework. Another example is a mandatory pre-enrolment initiative is an online readiness survey (Nichols, 2010 ). The author proposed that such a survey provide students with essential information needed for the decision-making process and decrease the mismatch in students’ expectations.
Orientation
Orientation programmes are proven to support student transition to the higher education (HE) and ensure the “scaffolded entry” (Stone, 2019 , p. 5) to the online learning environment (Horvath et al., 2019 ; Nichols, 2010 ; Walters-Archie, 2018 ). This support strategy implies a “greater emphasis at the front end” (Stone, 2019 , p. 5) and an establishment of an early connection with students (Gaytan, 2015 ). Through the orientation programme, students can also access “online tester experiences” (Kear et al., 2016 , p. 141) that allow them to evaluate their online learning skills and readiness to study in the online learning environment. As emphasised in the E-excellence framework, “students should be informed prior to registration about the skills they will need to develop, and the study skills support available to them” (Kear et al., 2016 , p. 141).
Orientation programmes also prepare students for their learning online after the enrolment. One example is a holistic pre-course orientation programme (see Walters-Archie, 2018 ) that consisted of four phases: an introduction to the online learning environment with the focus on navigation skills, an introduction to the structure and requirements of the programme, an introduction to the learning environment with the focus on practical activities (e.g. group discussions, quizzes), and an introduction to course facilitators. Walters-Archie ( 2018 ) reported that 94% of students found the orientation programme beneficial. Another example is orientation programme designed by Horvath et al. ( 2019 ) that include three modules, namely plan, prepare, and connect. Each module provides students with learning resources, video presentations, study guides, “Meet the experts” interactive Zoom seminars and “Getting Prepared for Study” quizzes that aim to clarify student expectations, present available support services and enhance students’ engagement.
Intervention phase
Identification of students at- risk and early interventions.
Netanda et al. ( 2019 ) found that novice online learners are also at a greater risk to face challenges when adjusting to the online learning environment than more experienced learners (p. 405). According to Purnell et al. ( 2010 ) “early intervention with weaker achieving student” enhance student retention (p. 78).
Identification of students at risk has been also emphasized in the past research (Gibbs et al., 2006 ; McKavanagh & Purnell, 2007 ). McKavanagh and Purnell ( 2007 ) pointed at distinctive features of those students such as lack of motivation, unrealistic time management expectations, and hesitation to reach for help (p. 79). In the United Kingdom Open University (UK OU) vulnerable or at-risk students have been identified based on the analysis institutional data (e.g. students’ sex, age, educational and professional experience) (Gibbs et al., 2006 ). A proposed support strategy involved contacting those students who have been identified as needing advice or support in order to offer them an appropriate help (Gibbs et al., 2006 ). Similarly, Simpson ( 2008 ) argued that in the situation of scarce recourses the most effective way to improve student retention is to focus on those students who require support and are most likely benefit from it. What makes a difference for at risk student retentions is the time between the student identification and a time of support intervention (Gibbs et al., 2006 ; Purnell et al., 2010 ).
Proactive support and student outreach
Empirical studies confirm the importance of proactive rather than reactive support for online distance learners. Robb and Sutton ( 2014 ) showed that motivational emails initiated by the educational institution significantly enhanced final course grade and course interest survey scores. Students reported that such emails encouraged them to put more effort into learning and eliminated discomfort in communication with their tutors. Core strategies for proactive tutor support in the UK OU included consideration of the students’ workload and pacing students’ learning against milestones, monitoring students’ learning outcomes in order to identify those who are at risk of falling behind and dropping out and getting in touch with students prior to the submission of the first assignment in order to identify those who struggle academically (Whitelock et al., 2015 ). Proactively contact students using learning analytics have been also advocated by Walsh et al. ( 2020 ).
Past research also emphasised the importance of prolonged proactive interventions at the early stage of the learning cycle (Anderson, 2003 ). Similarly, Simpson ( 2003 ) emphasised a positive impact of the motivational calls and postcards on UK OU students’ retention, speculating that motivational emails can have the same effect. Another example of proactive institutional support is an introduction of the possibility to re-submit an unsatisfactory assessment to individual students (Pinchbeck & Heaney, 2017 ). It is notable that online tutors play an invaluable part in the provision of the proactive support. According to Rendon ( 1994 ), a validation of the tutor in the form of encouragement or an interest in the students’ activities positively impacted students’ learning. Simpson ( 2004 ) found that online distance learners who have been approached by a tutor via phone call with an encouraging conversation had higher retention at the end of the programme than the students who did not received this support.
Addressing external factors
Online students require support not only with their learning, but with balancing external factors and commitments (Sorensen & Donovan, 2017 ; Stone, 2019 ; Whitelock et al., 2015 ). Whitelock et al. ( 2015 ) reported that the importance of the workload for online learners who have pressures with work and family responsibilities should be recognised and taken into consideration. Emerged from the overlap or clash of assignments with particularly busy periods of online students’ life may result in the heavy overload and a student may fall behind. Thus, it should not be assumed that students are always on track of their study schedules. Instead, an introduction of catch up or review weeks can enhance students’ motivation and contribute to their learning progress (Whitelock et al., 2015 ).
Support phase
Mentoring and peer support.
Mentoring and peer support proved to improve students’ adaptation to online learning environment (Kumar & Coe, 2017 ), contribute to the development of communication skills, and result in the better academic performance (Ashwin, 2003 ) and a higher persistence (Congos & Stout, 2003 ; McLean, 2004 ; Muldoon, 2008 ). Brindley ( 2014 ) argues that for educational institutions that have a constant enrolment in self passed learning an establishment of strong peer support networks in crucial for student success. Peer mentoring was the main mechanism in the designed by Horvath et al. ( 2019 ) orientation programme within which mentors helped new students to develop realistic expectations about their online learning, clarified the programme requirements, and overall served as learning models. Kumar and Coe ( 2017 ) too explained that through mentoring, new students can receive not only academic but also “socio-emotional support” (p. 15) since mentors play a mediating role for the knowledge and experience development. The participants of their study referred to the peer support as a paramount element for the development of community and persistence during the dissertation writing process.
Boyle et al. ( 2010 ) found that mentoring has a clear impact on student retention, offering a cost-efficient support strategy for the educational institution which is often underused in distance education. They proposed a “study dating” initiative designed to match students according to their characteristics, interests and other provided information (Boyle et al., 2010 , p. 129). Such use of technology and social network sites is a new turn in establishing student support networks. Indeed, Internet can offer additional affordances for online peer support and mentoring (Dollinger et al., 2020 ; Hsiao & Huang, 2019 ; Marineo & Shi, 2019 ).
The relative simplicity of peer mentoring, a cost-effective strategy considered earlier, as a support strategy embedded into the learning curriculum is also neglected (Boyle et al., 2010 ). Although it involves an establishment of initial connections between students and assistance in developing a peer network from the side of educational institution, this strategy takes less effort from academic and administrative staff than any other intervention. As, Brindley ( 2014 ) pointed out, “as institutions grapple with how to continue to provide quality support to greater numbers of students, it is likely that peer support will become much more important” (p. 297).
More attention has been paid to the indirect student support and caring. Robb and Sutton ( 2014 ) found that the student perception of a “caring instructor” (p. 6) or caring professor (Tippens, 2012 ) added a personal touch to the online class. Brown and Wilson ( 2016 ) proposed two initiatives, namely online caring groups and Caring connection website to facilitate students’ habits to care for themselves and care for others in an online learning environment. Prior research also recognised the value of indirect support. Jones ( 2010 ) argued that academic caring is an important factor for online students’ success. Similarly, Chen and Jang ( 2010 ) explain that students need to be surrounded by the atmosphere that allows a free expression of “feelings, thoughts, and concerns” (Chen & Jang, 2010 , p. 750), whereas the traditional form of depersonalised support can create barriers for expressing students concerns. Overall, an emphasis on care can facilitate genuine student connection with the educational institution and foster the development of the community of learners.
A provision of structure
Kumar and Johnson ( 2017 ) found that, from mentors’ perspective, the structure and scaffolding in online learning environment are the necessary strategies for students’ progress and elimination of the feeling of isolation. They found that organised group meetings and a provision of the peer feedback in a structured way assist students in being on top of their learning (p. 68). Smailes and Gannon-Leary ( 2011 ) also identified that a provision of scaffolding positively influenced students’ motivation. Educational scaffolding items mentioned by the students in their study are the well-organized structure of the courses, weekly email prompts and active learning tasks (Smailes & Gannon-Leary, 2011 ).
Fostering a strong sense of community
A community of learners is a “powerful motivator and a powerful mechanism” for supporting online students and their learning experience (Collins et al., 1987, in Boling et al., 2012 , p. 121). Hew ( 2015 ) argued that online learning experience can be enhanced by the reinforcement of the “social nature of learning” through the community where learners can socialise and support each other’s learning (p. 2).
Kumar and Coe ( 2017 ) supported a cohort model of learning that allows students to form meaningful interpersonal connections and be better supported in their learning challenges. In the past, “fostering sense of belonging” (p. 59) has been emphasised by Floyd and Casey-Powell ( 2004 ). Boyle et al. ( 2010 ) argued that feeling of belonging can be increased with the implementation of the peer support networks. Yet, individual institutions are recommended to identify communicative activities that work best for encouraging a greater sense of community among their students.
Interactions
Past research showed that well designed interactions improve students’ satisfaction, retention (Rienties & Toetenel, 2016 ) and learning outcomes (Kuo & Belland, 2016 ; Richardson et al., 2017 ). Boling et al. ( 2012 ) argue that online students’ connection with their tutors remains the most significant success factor. Among strategies to facilitate learner – content interactions Kuo and Belland ( 2016 ) suggested the use of technology enhanced tools (e.g., audio and video materials, multimedia, software that facilitates students’ learning) and a structured and easy to access online learning content. Interactions with the tutor can be enhanced by the provision of the encouragement and personalized guidance whereas the effectiveness of student communication can be facilitated through the guidance for interactions and collaborative work, with explanation of requirements, expectations, and online etiquette (Kuo & Belland, 2016 ).
Development of meaningful relationships
In online student support literature, there is a noticeable emphasis on the development of meaningful relationships. Scholars found that a teacher engagement and connection with online students has a positive effect on retention numbers (Glazier, 2016 ; Stone & O’Shea, 2019 ). Glazier ( 2016 ) identified that high-rapport relationship with the instructor is a key factor in student success and retention. To facilitate meaningful relationships, Glazier ( 2016 ) suggested implementing rapport-building teaching strategies, such as video updates, personal e-mails, and personalized electronic comments on assignments into online course.
Past research also indicate that the absence of the personal contact may result in the development of the feeling of loneliness (Sorensen & Donovan, 2017 ) and create communicative barriers especially for the less proactive learners (Brown et al., 2020 ; Paechter et al., 2010 ). To support the development of meaningful relationships, the teacher is expected to take onboard additional responsibilities (Russo-Gleicher, 2013 ).
Support with the development of necessary skills
Support with the development of skills necessary for online study may enhance students’ learning experience. Kuo and Belland ( 2016 ) found that pre-class training on the Internet-based technology facilitated students’ online interaction and overall learning experience and progress. In the study conducted by McLoughlin and Alam ( 2014 ), students were assisted in developing skills to work with social media and reported benefits of collaboration and peer networking of such support. Hsiao and Huang ( 2019 ) too suggested the use of wiki site as a strategy to support the development of the personal knowledge skills. Students found this support useful for a better personal knowledge management but not so for the purposes of socialisation. The concrete strategies for the development of student skills that the authors proposed are a provision of training, guidance, and examples of peer feedback and a peer reviewing process that enhances the development of the personal knowledge skills. In other works, despite the potential for enhancing students’ online learning experience the use of the Web.2.0. tools require guidance in how they are used by students and an ongoing evaluation of the effectiveness of their use.
Among interventions that support the development of online study skills reported in the past are time management and study management training for students with multiple priorities. Grant et al. ( 2011 ) designed an online study skills workshop aimed to develop students’ self-directedness and online learning skills. McLoughlin and Alam ( 2014 ) advocated scaffolded teaching as a way to increase collaborative learning interactions and to develop social media skills. In their study, they used Twitter as a tool for group interactions and found that twitter facilitated the development of a unique culture of peer communication and engagement.
For a diverse online student population, there is a need to re-think a meaningless and depersonalised provision of feedback. As Whitelock ( 2010 ) emphasised, timely and meaningful feedback influence online student progress as it is perceived as an “advice for action” (p. 323). Gaytan ( 2015 ) found that comprehensive feedback positively influences online students’ academic performance, resulting in an increase of student knowledge and decrease of the feeling of frustration. Uribe and Vaughan ( 2017 ) proposed a feedback cycle, suggesting two phases of potential frustration and difficulties: an encountering of the formative assessment feedback and a situation when a student does not seek a feedback clarification. The authors found that in these phases students may experience misunderstanding and confusion. Thus, as Whitelock et al. ( 2015 ) argued, affective and cognitive domains of the tutor feedback should be balanced in different ways for different learners, providing them with an adequate combination of “socio-emotive and cognitive support” (p. 171).
Personal advising and counselling
Although in the reviewed studies personal advising and counselling has not been differentiated as distinctive strategies, they were advocated as a part of the wholistic approach to supporting online students (Kelly et al., 2020 ; Zuhairi et al., 2019 ; Britto & Rush, 2013 ).
Research conducted in the past emphasises that the availability of advising services has direct impact on online students’ satisfaction and course retention (Cain & Lockee, 2002 ). Although such support is easily accessible for campus-based students, there is need for its provision for distance learners using multiple technological means, such as phone calls, emails, online conference tools. Furthermore, sufficient information about the personal support and counselling should be visible and available through the educational institution.
Transition phase
In the selected for analysis studies, there was no reference on concrete strategies or interventions at student transition points, apart from suggestions offered by Gibbs et al.( 2006 ) and Gibau, ( 2015 ). Yet, past research emphasises that those timely interventions at transition points, between different parts of the study and during the induction period, make a positive difference in students learning progress (Baxter, 2012 ). Following types of transitions have been identified in the past: transition to higher education, transition between different stages of learning, and transition to the labour market.
Transition to the higher education
Although the literature on the strategies for supporting students at transition points is scarce, past research focused on the transition to higher education, and especially the literature on socialisation, suggests that novice students benefit from guided transition (Gibau, 2015 ; Ward & Commander, 2011 ). Gibau ( 2015 ) explains that student transition often involves both social and physical adjustment (p. 6), which is in line with the models on students’ retention and progress (Tinto, 1975 ; Rovai, 2003 ). Pedagogical models presume that if a student cannot make a social and academic adaptation, then the result may be dropping out from the course of study.
To prepare students for the initial transition to the HE, such an early outreach and “intentional peer mentoring”- also discussed in other phases- have been suggested (Gibau, 2015 , p. 10) as strategies to support new students in their adjustment and transition to the higher education. Such activities contribute to the development of the kinship and can be arranged through matching different cohorts of students (Gibau, 2015 ).
Transitions between different stages of learning
The UK OU advocates focused support interventions in the periods of transition from one course to another to support students’ decision-making process (Gibbs et al., 2006 ). For some students, support in transition is a motivating factor, particularly if interventions from both tutors and support staff can address the lack of confidence- a common feeling reported by students in a new situation or environment. Baxter ( 2012 ) explained that if students are not supported at these ambiguous stages, they may experience exclusion and fail to progress.
Transition to the labour market
Another form of transition is the transitions from the university to the labour market (Dahlgren et al., 2006 ; Merrill, 2020 ). In the study conducted by Sisselman-Borgia and Torino ( 2017 ), students reported that “it was difficult to make a transition into a new field of work without ever having an experience in the new field” (p.). Learning experiences that aim to provide students with authentic experience in the field and evaluate their fitness (but also being flexible enough to fit into their current schedules) are among previously employed support strategies (see Sisselman-Borgia & Torino, 2017 ).
Measurement phase
Use of data analytics.
Learning analytics offered the potential to identify at-risk students based on predictor variables (Simpson, 2004 ). Moreover, institutional data allows matching the most appropriate strategies with students who may benefit from such support (Gibbs et al., 2006 ; Walsh et al., 2020 ). In the past, there were attempts to measure the effectiveness of student support strategies and interventions. For instance, Simpson ( 2004 ) proposed a “maximum possible increase in retention” indicator to measure the effectiveness of support strategies (p. 82). Using student data to conduct predictive models, educational institutions can develop targeted interventions and help learners make more informed decisions about available support services (Brindley, 2014 ).
Cost–benefit analysis
Although there was no data on the analysis of costs involved in the design of support interventions in the revised studies, prior research showed that employing the cost analysis can justify the concreate support strategy or intervention. In the UK OU, Gibbs et al. ( 2006 ) predicted a profit of $2,087,302 a year by implementing a new proactive support system for 35.000 online students that cost $1,085,000 per year (p. 371). Such evaluation allows implementing support strategy that is “backed by cost-effectiveness data based on evaluations of controlled experiments and driven by management information systems” (Gibbs et al., 2006 , p. 259). Furthermore, the cost–benefit may assist educational institutions in designing personalised, yet cost-effective support interventions based on the analysis of institutional data to identify vulnerable and at-risk students. However, the analysis of the research on support strategies and interventions showed that there are no embedded mechanisms to evaluate student support interventions in terms of their economic costs and benefits.
Surveys and interviews
Surveys of satisfaction with support services are most often used to measure quality and identify any unmet needs (Brindley, 2014 ). Nicholas et al. ( 2012 ) conducted pre-and post-intervention interviews with two groups of students, those who received support and those who did not. Qualitative interviews with intervention group participants proved to be helpful in identifying the beneficial impact of support. Specifically, the researchers were able to collect data on students’ decreased isolation, gain in knowledge, and normalisation of experience (Nicholas et al., 2012 ).
Self-evaluation tools
Higher educational institutions can use a variety of tools to assess the support services they offer. One assessment tool reported in the past is the Online Student Services Self-Assessment Tool, which helps educators review links to the support services and evaluate which areas of support are not addressed (Floyd & Casey-Powell, 2004 , p. 56). Among the considered studies, Boyle et al. ( 2010 ) also employed self-reports from online students to measure the effectiveness of the implemented peer support strategy. The data showed that mentored students had a higher persistence rate compare to the unsupported students. Analysis of such data helped to plan the further implementation of the peer-support strategy in the institution.
The analysis of support strategies, initiative and interventions using the MIPS framework aimed to answer the research question What support strategies and interventions can be offered to online students at different phases of the learning cycle ? Using Floyd and Casey-Powell ( 2004 )’ IMSS framework, I allocated identified support strategies for online students across different phases of the learning cycle. Figure 1 shows that various forms of support can be offered to students during the different stages of their learning.
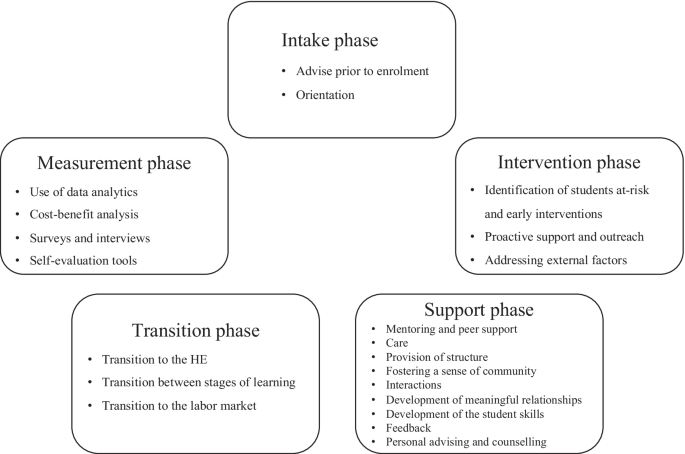
Allocation of online student support strategies and intervention across different phases of the learning cycle
The results of this study support prior research on the positive effect of initial student support during the student intake phase (see studies on orientation programmes House & Kuchynka, 1997 ; Williford et al., 2001 ; Wilson, 2008 ).
At the student intervention phase, the analysis results appear to be in line with studies that advocate early and proactive interventions. Specifically, the findings support research on early measurement for identifying students' prior knowledge and behavioural characteristics that provide a clue for deciding on proactive intervention (Muljana & Luo, 2019 ; Nistor & Neubauer, 2010 ). The approaches to student outreach tend to utilise technologies and data analytics rather than the old form of proactive support such as telephone calls. Specifically, the use of learning analytics to identify at-risk students offers unlimited opportunities for targeted interventions (Simpson, 2004 ). This line of research holds the promise that predictor variables become more accurate in targeting students and the learner analytics approach more sophisticated.
In regard to the student support phase, there are multiple ways in which support can be offered (Tait, 2004 ). This study confirmed the importance of advising and counselling, the development of the sense of community and support with academic skills- essential elements of support emphasised by Floyd and Casey-Powell ( 2004 ). The analysis also found that there is a recognition of the positive impact of peer mentoring and support for online student progress and retention. Moreover, this strategy is suggested to be relatively cost-effective. However, in an online learning environment, peer support and mentoring remain under-researched domains, and there is "little evidence of the existence of UK programmes of peer mentoring in virtual formats" (Smailes & Gannon-Leary, 2011 ). Boyle et al. ( 2010 ) point out that "the key success factors consistently identified include appropriate mentor training and the inclusion of peer mentoring as part of the formal curriculum. (p.120). Thus, it is crucial to bear in mind that such conventional forms of student support may still have much to offer in enhancing student engagement and retention (Boyle et al., 2010 ).
The identified support interventions are in line with research that differentiates supports into academic, technological, and personal (Anderson, 2008 ; LaPadula, 2003 ). The study also recognises the importance to maintain a sense of belongingness to the community- key component that can combat student attrition and drop out (Lee & Choi, 2011 ).
Systematic literature review revealed that two phases of the learning cycle- transition and measurement- are not well supported by the existing research on online student support strategies and interventions. However, this does not minimise their importance but rather reveals the lack of research in those areas. The transition phase showed the aspect of students' transitions, such as a transition to the HE, transitions between different parts of study and during the induction period, and any other forms of transition that require student adjustment to the new environment or routine. This is in line with scholars who emphasised the multifaceted nature of the process of transition (Hussey & Smith, 2010 ; Maunder et al., 2013 ), which may involve not only educational adjustment but also personal adaptation and adjustment of the lifestyle. Past research suggests that there are different types of transition to which students require to adapt (Maunder et al., 2013 ). Maunder et al. ( 2013 ) emphasise that transitions not always associated with educational adaptation but may involve personal, lifestyle and identity adjustments (Cocquyt et al., 2017 ). Yet, most of the research on students' transitions have been predominantly focused on student transitions during the first year (Reay, 2003 ; Tett, 2000 ). Educators are invited to recognise the multifaceted nature of online students' transitions that can occur at different stages of their learning.
In regard to the measurement phase, the main finding of this study is that although attrition studies often conclude with recommendations for specific kinds of support interventions, relatively little evaluative research has been done to find out about their impact. Although, according to Rumble ( 2000 ), distance educational institutions are "more driven by concern for planning customer care and support than the traditional universities" (p. 219), mechanisms for measuring the suggested interventions are less developed. Tait (1995) emphasises that the rationale for online student support remains relatively weak and, therefore, continue to be "subject to wild fluctuations in terms of financial support" (Tait, 1995, in Rumble, 2000 , p. 216). Gibbs et al. ( 2006 ) agree that in the situation of scarce resources, evaluations of cost-effective cases should be done to gather evidence on the impact, effectiveness, and value of the support interventions. The issue that the authors emphasise is that the recommendations have been developed predominantly within a controlled environment rather than tested in real learning situations. Moreover, they argue, there is no comprehensive system that evaluates the effectiveness of the developed interventions (Gibbs et al., 2006 , p. 375). Therefore, more effective use of institutional data and data analytics is encouraged as they have proven its potential to evaluate the effect of support services. The impact of digital technologies on student support in distance and e-learning allows taking into accounts both internal and external factors and not only integrating student support with teaching and learning at different phases but also go gather data on their effectiveness automatically (Zuhairi et al., 2019 , p. 15). In other words, this research emphasised the need to not only understand the value of offered support for students but also to develop instruments for measuring the result of each intervention for educational institutions in order to effectively allocate scarce economic resources.
Finally, the analysis showed two emerging trends in online students support. The first one is the increase in the use of technology and social network sites to design support interventions (Dollinger et al., 2020 ; Hsiao & Huang, 2019 ; Marineo & Shi, 2019 ). Specifically, online tools and resources are used to increase communication and decrease transactional distance (Moore & Kearsley, 2012 ). Another interesting development is a shift to a more holistic approach to student support (Kelly et al., 2020 ; Zuhairi et al., 2019 ; Britto & Rush, 2013 ; Johns & Oestreich, 2019 ). Support strategies and services designed under a holistic approach and embrace all the aspects of the university experience, such as administrative, educational, pastoral, and personal, hold great potential for ensuring student success (Kear et al., 2016 ). As Brindley ( 2014 ) point out, a holistic approach to student support that is based on previous analyses of students' needs and demands, considering different learner groups and the specific characteristics of lifelong learners, will ensure that support is embedded at an institutional level. To embrace a holistic approach means to integrate different types of resources, coordinating staff to give support in academic, technical, administrative, and other relevant areas.
Limitations
Although this paper gathered valuable information on the effective support strategies and interventions, it has several limitations. First, this study did not intend to test employed IMMS model but rather used it as a frame for the analysis. As a result, some of the phases of the proposed framework are not supported by empirical evidence and discussed within the knowledge offered in the past research. This, however, allowed me to emphasise potential directions for the further research. Secondly, the study provides an analysis of the literature using a selected theoretical model which itself may need an empirical testing.
The reviewed literature provides a rather comprehensive set of online student support interventions and their allocation across the different phases of the learning cycle. It is suggested that the effectiveness of the implementation of these strategies and interventions depend on the time of student support provision, advocating the embedment of the support provision into the learning curriculum. Finally, this review indicates two under-investigated areas of student support, namely support at transition and a measurement of the effect from support interventions.
A widening participation agenda caused an emergence of the diverse online student body. Due to both the development of online learning technologies and diversification of the online student body, the provision of support services evolved, with significant variations among educational institutions. As Thorpe ( 2002 ) stated, “learner support” is the arena within which transformations in nature and the scale of activities made feasible by online teaching are generating widespread change in pedagogies and learning communities, and across institutions as a whole in ODL [online distance learning]” (p. 117).
A wide range of support interventions designed to support students at different stages suggests a need for an embedded, holistic approach to student support. Such a systemic approach may involve the development of specific policies and programmes and the integration of specialised support units and expertise across the institution. Furthermore, as the analysis showed, the time and relevancy of the provided support should not be neglected as in many cases, “support activity simply occurred too late in the course to be effective or after students had already decided to withdraw” (Gibbs et al., 2006 , p. 375).
Availability of data and materials
Data for the analysis was extracted from publications identified through the Scopus and Google Scholar databases and can be accessed online.
Analysed articles are marked with *.
Anderson, T. (2003). Getting the mix right again: An updated and theoretical rationale for interaction. International Review of Research in Open and Distance Learning, 4 (2), 1–14.
Article Google Scholar
Anderson, T. (Ed.). (2008). The theory and practice of online learning . Alberta, Canada: Athabasca University Press.
Google Scholar
Ashwin, P. (2003). Peer support: Relations between the context, process and outcomes for the students who are supported. Instructional Science, 31 (3), 159–173.
Baxter, J. (2012). Who am I and what keeps me going? Profiling the distance learning student in higher education. International Review of Research in Open and Distributed Learning , 13 (4), 107–129.
Boling, E. C., Hough, M., Krinsky, H., Saleem, H., & Stevens, M. (2012). Cutting the distance in distance education: Perspectives on what promotes positive, online learning experiences. The Internet and Higher Education, 15 (2), 118–126.
*Boyle, F., Kwon, J., Ross, C., & Simpson, O. (2010). Student–student mentoring for retention and engagement in distance education. Open Learning: THe Journal of Open, Distance and e-Learning, 25 (2), 115–130.
Brindley, J. E. (2014). Learner support in online distance education: Essential and evolving. In O. Zawacki-Richter & A. Terry (Eds.), Online distance education. Towards a research agenda (pp. 287–310). AU Press.
*Britto, M., & Rush, S. (2013). Developing and implementing comprehensive student support services for online students. Journal of Asynchronous Learning Networks, 17 (1), 29–42.
*Brown, C. J., & Wilson, C. B. (2016). One university making a difference in graduate education: Caring in the online learning environment. Journal of Holistic Nursing, 34 (4), 402–407.
Brown, A., Lawrence, J., Basson, M., & Redmond, P. (2020). A conceptual framework to enhance student online learning and engagement in higher education. Higher Education Research & Development , 1-16.
Cain, D., & Lockee, B. (2002). Student support services at a distance: Are institutions meeting the needs of distance learners? ERIC Documentation Reproduction Service N° ED 468 729.
Chen, K. C., & Jang, S. J. (2010). Motivation in online learning: Testing a model of self-determination theory. Computers in Human Behavior, 26 (4), 741–752.
Clay, M. N., Rowland, S., & Packard, A. (2008). Improving undergraduate online retention through gated advisement and redundant communication. Journal of College Student Retention: Research, Theory & Practice, 10 (1), 93–102.
Cocquyt, C., Diep, N. A., Zhu, C., De Greef, M., & Vanwing, T. (2017). Examining social inclusion and social capital among adult learners in blended and online learning environments. European Journal for Research on the Education and Learning of Adults, 8 (1), 77–101.
Congos, D., & Stout, B. (2003). The benefits of SI leadership after graduation. Research and Teaching in Developmental Education , 29-41.
Dahlgren, A., Hult, H., Dahlgren, L., Segerstad, H., & Johansson, K. (2006). From senior student to novice worker: learning trajectories in political science, psychology, and mechanical engineering. Studies in Higher Education , 31 , 569–86.
*Dollinger, M., Cox, S., Eaton, R., Vanderlelie, J., & Ridsdale, S. (2020). Investigating the usage and perceptions of third-party online learning support services for diverse students. Journal of Interactive Media in Education, 1 , 1–9.
Floyd, D. L., & Casey-Powell, D. (2004). New roles for student support services in distance learning. New Directions for Community Colleges, 128 , 55–64.
*Gaytan, J. (2015). Comparing faculty and student perceptions regarding factors that affect student retention in online education. American Journal of Distance Education, 29 (1), 56–66.
*Gibau, G. S. (2015). Considering student voices: Examining the experiences of underrepresented students in intervention programs. CBE-Life Sciences Education, 14 (3), 1–12.
Gibbs, G., Regan, P., & Simpson, O. (2006). Improving student retention through evidence based proactive systems at the Open University (UK). Journal of College Student Retention: Research, Theory & Practice, 8 (3), 359–376.
*Glazier, R. A. (2016). Building rapport to improve retention and success in online classes. Journal of Political Science Education, 12 (4), 437–456.
Grant, R., Olivier, G., Rawlings, C., & Ross, C. (2011). Enhancing the engagement and success of distance students through targeted support programmes. The Open Polytechnic of New Zealand Working paper, 1-19.
Hew, K. F. (2015). Towards a model of engaging online students: Lessons from MOOCs and four policy documents. International Journal of Information and Education Technology, 5 (6), 425–431.
*Horvath, D., Stirling, E., Bevacqua, J., Coldrey, M., Buultjens, P., Buultjens, M., & Larsen, A. (2019). Plan, prepare and connect: How investing in understanding and tracking the evolving needs of online students informs the development of targeted programs for transition and success. Journal of University Teaching & Learning Practice, 16 (1), 1–14.
House, J. D., & Kuchynka, S. J. (1997). The effects of a freshmen orientation course on the achievement of health science students. Journal of College Student Development , 38 (5), 540–542.
*Hsiao, E., & Huang, X. (2019). Strategies to support personal knowledge management using a wiki site in online courses. Journal of Educators Online, 16 (1), 1–12.
Hussey, T., & Smith, P. (2010). Transitions in higher education. Innovations in Education and Teaching International, 47 (2), 155–164.
Johns, E. M., & Oestreich, S. (2019). On the edge: How to provide course-and program-integrated library support without being embedded. Journal of Library & Information Services in Distance Learning, 13 (1–2), 1–20.
Jones, B. D. (2010). An examination of motivation model components in face-to-face and online instruction. Electronic Journal of Research in Educational Psychology, 8 (3), 915–944.
Karabulut-Ilgu, A., Jaramillo Cherrez, N., & Jahren, C. T. (2018). A systematic review of research on the flipped learning method in engineering education. British Journal of Educational Technology , 49 (3), 398–411.
*Kear, K., Rosewell, J., Williams, K., Ossiannilsson, E., Rodrigo, C., Sánchez-Elvira Paniagua, A., Santamaría Lancho, M., Vyt, A., & Mellar, H. (2016). Quality assessment for e-learning: A benchmarking approach (third edition) [online]. European Association of Distance Teaching Universities (EADTU). Retrieved from http://e-xcellencelabel.eadtu.eu/images/E-xcellence_manual_2016_third_edition.pdf .
*Kelly, A., Johnston, N., & Matthews, S. (2020). Online self-access learning support during the COVID-19 pandemic: An Australian University case study. Studies in Self-Access Learning Journal, 11 (3), 187–198.
*Kumar, S., & Coe, C. (2017). Mentoring and student support in online doctoral programs. American Journal of Distance Education, 31 (2), 128–142.
*Kumar, S., & Johnson, M. (2017). Mentoring doctoral students online: Mentor strategies and challenges. Mentoring & Tutoring: Partnership in Learning, 25 (2), 202–222.
*Kuo, Y. C., & Belland, B. R. (2016). An exploratory study of adult learners’ perceptions of online learning: Minority students in continuing education. Educational Technology Research and Development, 64 (4), 661–680.
LaPadula, M. (2003). A comprehensive look at online student support services for distance learners. The American Journal of Distance Education, 17 (2), 119–128.
Lee, Y., & Choi, J. (2011). A review of online course dropout research: Implications for practice and future research. Educational Technology Research and Development, 59 (5), 593–618.
Maunder, R. E., Cunliffe, M., Galvin, J., Mjali, S., & Rogers, J. (2013). Listening to student voices: Student researchers exploring undergraduate experiences of university transition. Higher Education, 66 (2), 139–152.
*Marineo, F., & Shi, Q. (2019). Supporting student success in the first-year experience: Library instruction in the learning management system. Journal of Library & Information Services in Distance Learning, 13 (1–2), 40–55.
Martin, F., & Bolliger, D. U. (2018). Engagement matters: Student perceptions on the importance of engagement strategies in the online learning environment. Online Learning , 22 (1), 205–222.
*McDougall, J. (2019). ‘I never felt like I was alone’: A holistic approach to supporting students in an online, pre-university programme. Open Learning: THe Journal of Open, Distance and e-Learning, 34 (3), 241–256.
McKavanagh, M., & Purnell, K. (2007). Student learning journey: Supporting student success through the student readiness questionnaire. Studies in Learning, Evaluation, Innovation and Development, 4 (2), 27–38.
McLean, M. (2004). Does the curriculum matter in peer mentoring? From mentee to mentor in problem-based learning: A unique case study. Mentoring & Tutoring: Partnership in Learning , 12 (2), 173–186.
*McLoughlin, C. E., & Alam, S. L. (2014). A case study of instructor scaffolding using Web 2.0 tools to teach social informatics. Journal of Information Systems Education, 25 (2), 125–136.
Merrill, B. (2020). Biographical Inquiry: A Collaborative and Egalitarian Approach to Adult Education Research. In B. Grummell & F. Finnegan (Eds.), Doing Critical and Creative Research in Adult Education (pp. 15–24). Leiden, Netherlands: Brill.
Miller, T., & Prince, J. (1976). The future of student affairs. A guide to student development for tomorrow’s higher education . Jossey-Bass.
Moore, M. G., & Kearsley, G. (2012). Distance education: A systematic view of online learning (3rd ed.). Wadsworth Cengage Learning.
Muldoon, R. (2008). Recognising and rewarding the contribution and personal development of peer supporters at university. Journal of Further and Higher Education , 32 (3), 207–219.
Muljana, P. S., & Luo, T. (2019). Factors contributing to student retention in online learning and recommended strategies for improvement: A systematic literature review. Journal of Information Technology Education Research , 18 , 19–57.
*Netanda, R. S., Mamabolo, J., & Themane, M. (2019). Do or die: Student support interventions for the survival of distance education institutions in a competitive higher education system. Studies in Higher Education, 44 (2), 397–414.
*Nicholas, D. B., Fellner, K. D., Frank, M., Small, M., Hetherington, R., Slater, R., & Daneman, D. (2012). Evaluation of an online education and support intervention for adolescents with diabetes. Social Work in Health Care, 51 (9), 815–827.
*Nichols, M. (2010). Student perceptions of support services and the influence of targeted interventions on retention in distance education. Distance Education, 31 (1), 93–113.
Nistor, N., & Neubauer, K. (2010). From participation to dropout: Quantitative participation patterns in online university courses. Computers & Education , 55 (2), 663–672.
Öztok, M. (2019). The hidden curriculum of online learning: understanding social justice through critical pedagogy . Routledge.
Book Google Scholar
Paechter, M., Maier, B., & Macher, D. (2010). Students’ expectations of, and experiences in e-learning: Their relation to learning achievements and course satisfaction. Computer Education , 54 (1), 222–229.
Pinchbeck, J., & Heaney, C. (2017). Case report: The impact of a resubmission intervention on level 1 distance learning students. Open Learning: THe Journal of Open, Distance and e-Learning, 32 (3), 236–242.
*Purnell, K., McCarthy, R., & McLeod, M. (2010). Student success at university: Using early profiling and interventions to support learning. Studies in Learning, Evaluation, Innovation and Development, 7 (3), 77–86.
Reay, D. (2003). A Risky Business? Mature Working-class Women Students and Access to Higher Education. Gender and Education , 15 (3), 301–17.
Rendon, L. I. (1994). Validating culturally diverse students: Toward a new model of learning and student development. Innovative Higher Education, 19 (1), 33–51.
Rice, M., Lowentha, P., & Woodley, X. (2020). Distance education across critical theoretical landscapes: Touchstones for quality research and teaching. Distance Education, 41 (3), 319–325.
Richardson, J. C., Maeda, Y., Lv, J., & Caskurlu, S. (2017). Social presence in relation to students’ satisfaction and learning in the online environment: A meta-analysis. Computers in Human Behavior, 71 , 402–417.
Rienties, B., & Toetenel, L. (2016). The impact of learning design on student behaviour, satisfaction and performance: A cross-institutional comparison across 151 modules. Computers in Human Behavior, 60 , 333–341.
*Robb, C. A., & Sutton, J. (2014). The importance of social presence and motivation in distance learning. The Journal of Technology, Management, and Applied Engineering, 31 (2), 2–10.
Rotar, O. (2020). A missing element of online HE students’ attrition, retention, and success: an analysis through a systematic literature review (Working Paper No. 3). Retrieved from https://www.lancaster.ac.uk/educational-research/research/centre-for-higher-education-research-and-evaluation/working-papers/
Rovai, A. (2003). In search of higher persistence rates in distance education online programs. The Internet and Higher Education , 6 (1), 1–16.
Rumble, G. (2000). Student support in distance education in the 21st century: Learning from service management. Distance Education, 21 (2), 216–235.
Russo-Gleicher, R. J. (2013). Qualitative insights into faculty use of student support services with online students at risk: Implications for student retention. Journal of Educators Online , 10 (1), 1–32.
Ryan, Y. (2004). Pushing the boundaries with online learner support. In J. Brindley, C. Walti, & O. Zawacki-Richter (Eds.), Learner support in open, distance and online learning environments (pp. 125–134). Bibliotheks und Informations system der Universität Oldenburg.
Sewart, D. (1993). Student support systems in distance education. Open Learning: THe Journal of Open, Distance and e-Learning, 8 (3), 3–12.
Simpson, O. (2003). Student retention in online open and distance learning . Kogan Page.
Simpson, O. (2004). The impact on retention of interventions to support distance learning students. Open Learning: THe Journal of Open, Distance and e-Learning, 19 (1), 79–95.
Simpson, O. (2008). Motivating learners in open and distance learning: Do we need a new theory of learner support? Open Learning: THe Journal of Open, Distance and e-Learning, 23 (3), 159–170.
*Sisselman-Borgia, A. G., & Torino, G. C. (2017). Innovations in experiential learning for adult learners. Journal of Applied Learning in Higher Education, 7 , 3–13.
*Smailes, J., & Gannon-Leary, P. (2011). Peer mentoring—Is a virtual form of support a viable alternative? Research in Learning Technology, 19 (2), 129–142.
Sorensen, C., & Donovan, J. (2017). An examination of factors that impact the retention of online students at a for-profit university. Online Learning, 21 (3), 206–221.
Stone, C. (2017). Opportunity through online learning: Improving student access, participation and success in higher education (NCSEHE 2016 Equity Fellowship Final Report). Perth: Curtin University, National Centre for Higher Education. Retrieved from https://www.ncsehe.edu.au/publications/opportunity-online-learning-improving-student-access - participation-success-higher-education/
Stone, C. (2019). Online learning in Australian higher education: Opportunities, challenges and transformations. Student Success, 10 (2), 1–11.
Stone, C., & O'Shea, S. (2019). Older, online and first: Recommendations for retention and success. Australasian Journal of Educational Technology , 35 (1), 57–69.
Tait, A. (2004). On institutional models and concepts of student support services: The case of the Open University, UK, 3rd EDEN Research Workshop. Retrieved from http://www.c3l.uni-oldenburg.de/cde/support/fa04/Vol.%209%20chapters/KeynoteTait.pdf
Tett, L. (2000). ‘I’m Working Class and Proud of It’ – Gendered Experiences of Non-traditional Participants in Higher Education. Gender and Education , 12 (2), 183–94.
Thorpe, M. (2002). Rethinking learner support: The challenge of collaborative online learning. Open Learning: THe Journal of Open, Distance and e-Learning, 17 (2), 105–119.
Tinto, V. (1975). Dropout from higher education: A theoretical synthesis of recent research. Review of Educational Research , 45 (1), 89–125.
Tippens, D. (2012). Technology has its place: Behind a caring teacher. Chronicle of Higher Education. Retrieved from http://chronicle.com/article/technology-has-itsplace-133328/
*Uribe, S. N., & Vaughan, M. (2017). Facilitating student learning in distance education: A case study on the development and implementation of a multifaceted feedback system. Distance Education, 38 (3), 288–301.
*Walsh, C., Mital, A., Ratcliff, M., Yap, A., & Jamaleddine, Z. (2020). A public-private partnership to transform online education through high levels of academic student support. Australasian Journal of Educational Technology, 36 (5), 30–45.
*Walters-Archie, A. (2018). Academic support for online students in the English-speaking Caribbean at the University of the West Indies Open Campus. Journal of Further and Higher Education, 42 (6), 868–878.
Ward, T., & Commander, N. E. (2011). The power of student voices: An investigation of the enduring qualities of freshmen learning communities. Journal of College Student Retention: Research, Theory & Practice , 13 (1), 63–85.
Whitelock, D. (2010). Activating Assessment for Learning: are we on the way with Web 2.0? In M. J. W. Lee & C. McLoughlin (Eds.), Web 2.0- Based-E-Learning: Applying Social Informatics for Tertiary Teaching (pp. 319–342). Hershey, PA: IGI Global.
*Whitelock, D., Thorpe, M., & Galley, R. (2015). Student workload: A case study of its significance, evaluation and management at the Open University. Distance Education, 36 (2), 161–176.
Williford, A. M., Chapman, L. C., & Kahrig, T. (2001). The university experience course: A longitudinal study of student performance, retention, and graduation. Journal of College Student Retention: Research, Theory & Practice , 2 (4), 327–340.
Wilson, M. (2008). An investigation into the perceptions of first-time online undergraduate learners on orientation events. Journal of Online Teaching and Learning , 4 (1), 73–83.
Woodley, A., & Simpson, O. (2014). The elephant in the room. In Online Distance Education: Towards a Research Agenda (pp. 459–485).
Zawacki-Richter, O., & Anderson, T. (2014). Online distance education: Towards a research agenda . AU Press.
Zuhairi, A., Karthikeyan, N., & Priyadarshana, S. T. (2019). Supporting students to succeed in open and distance learning in the Open University of Sri Lanka and Universitas Terbuka Indonesia. Asian Association of Open Universities Journal , 13–34
Download references
Acknowledgements
I would like to thank Professor Don Passey and two anonymous reviewers for their suggestions for improving this manuscript.
This research was supported by the FfWG of the British Federation of Women Graduates, Ref: GA-00764.
Author information
Present address: Centre for Higher Education Research and Evaluation, Lancaster University, Bailrigg, Lancaster, LA1 4YW, UK
Authors and Affiliations
You can also search for this author in PubMed Google Scholar
Contributions
All authors read and approved the final manuscript.
Corresponding author
Correspondence to O. Rotar .
Ethics declarations
Competing interests.
No competing interests has been identified.
Additional information
Publisher's note.
Springer Nature remains neutral with regard to jurisdictional claims in published maps and institutional affiliations.
Rights and permissions
Open Access This article is licensed under a Creative Commons Attribution 4.0 International License, which permits use, sharing, adaptation, distribution and reproduction in any medium or format, as long as you give appropriate credit to the original author(s) and the source, provide a link to the Creative Commons licence, and indicate if changes were made. The images or other third party material in this article are included in the article's Creative Commons licence, unless indicated otherwise in a credit line to the material. If material is not included in the article's Creative Commons licence and your intended use is not permitted by statutory regulation or exceeds the permitted use, you will need to obtain permission directly from the copyright holder. To view a copy of this licence, visit http://creativecommons.org/licenses/by/4.0/ .
Reprints and permissions
About this article
Cite this article.
Rotar, O. Online student support: a framework for embedding support interventions into the online learning cycle. RPTEL 17 , 2 (2022). https://doi.org/10.1186/s41039-021-00178-4
Download citation
Received : 11 March 2021
Accepted : 25 November 2021
Published : 06 January 2022
DOI : https://doi.org/10.1186/s41039-021-00178-4
Share this article
Anyone you share the following link with will be able to read this content:
Sorry, a shareable link is not currently available for this article.
Provided by the Springer Nature SharedIt content-sharing initiative
- Student support
- Support strategies
- Embedded support
- Online higher education
Support Topics
Learn more about various research support topics that help shape the trajectory and success of your research.
Animal Subject Research
Regulation, compliance, and support for studies and experiments conducted on animals.
Biological Safety
Practices, policies, and procedures aimed at ensuring the safe handling, storage, and disposal of biological materials.
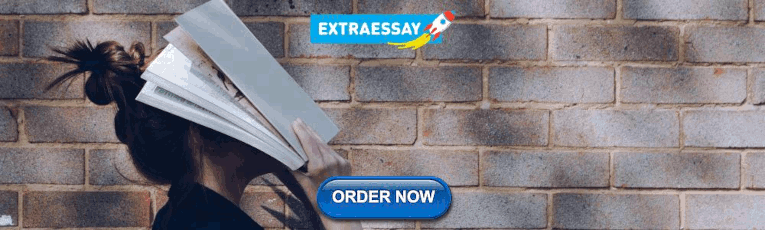
Chemical Safety
Practices, policies, and procedures aimed at ensuring the safe handling, storage, and disposal of chemical materials.
Clinical Trials
Regulation, compliance, and support for scientific studies or research investigations conducted with human participants.
Commercialization
Resources for translating discoveries and inventions from the lab into solutions with economic and societal impact.
Commercial Agreements & Contracts
Regulation, compliance, and support for research collaborations, projects, or partnerships with commercial and financial implications.
Community Outreach
Guidance and resources to engage communities in research endeavors.
Compliance for external consulting arrangements.
Corporate Engagement
Guidance on engaging with outside industry to perform research.
Data Management
Guidelines and related resources related to collecting, organizing, analyzing, and protecting research data.
Diversity, Equity, and Inclusion
Best practices and support for integrating diversity, equity, and inclusion into research topics and research management.
Export Compliance
Regulation and guidance for the international export of goods, technology, and information related to research.
Financial Conflicts of Interest
Regulation and compliance related to financial conflicts of interest (FCOI) and FCOI disclosure.
Funding Support
Resources and opportunities for research funding support.
Human Subject Research
Regulation, compliance, and support for studies and experiments involving human subjects.
Institutional Animal Care and Use
Policies and procedures related to the oversight of ethical treatment of animals used in research.
Institutional Review Board
Policies and procedures related to the oversight of ethical treatment of human participants in research.
Intellectual Property
Policies and procedures related to the legal rights of inventions produced by research.
International Research
Support for research involving international collaboration and partners.
Learning & Professional Development
Resources and support to enhance professional skills related to research.
Occupational Safety
Practices, policies, and regulations aimed at ensuring the health, safety, and well-being of employees and workers in their workplaces
Patent Policy
Policies and guidelines at Penn related to the creation, ownership, management, and use of patents.
Postdoctoral Affairs
Guidance, resources, and support for postdoctoral researchers and faculty mentors.
Post-Award Administration
Guidance and resources to ensure funded research is in compliance with the funding agency or organization.
Pre-Award Administration
Resources to support research activity and projects prior to award.
Proposal Preparation
Support and guidance in developing a research proposal.
Resources to support the publication process.
Radiation Safety
Practices, policies, and procedures aimed at ensuring the safe use of radiation and radiated materials in research.
Research Agreements
Resources and support for developing agreements governing a research project or collaboration.
Research Integrity
Policies and resources at Penn related to the ethical principles, values, and practices of research conduct and the dissemination of research findings.
Research Management
Guidance for planning and coordinating research activities and initiatives.
Research Misconduct
Policies and procedures related to potential cases of research misconduct.
Responsible Conduct of Research
Training and resources regarding ethical and professional standards for the responsible conduct of research.
Technology Licensing
Regulation and compliance related to the legal licensing of technology for use by an outside party.
Venture Formation
Guidance and support for the formation of a business or startup related to research activities.

- Sacred Heart University
- Research Guides
- Homepage Group
Research Support
Research support: home.

Research Support Options
ask a librarian.
Ask us questions by chat, email, text, phone or by appointments.
Appointments
Make an appointment with a librarian to get research help.
Tutorials
Quickly learn how to use many of our services and research tools.
Interlibrary Loan (ILL)
Request articles and books and we will try to get them from other libraries for free.
Research Guides
Quickly find useful resources based on specific subject areas.
Databases By Subject
Find useful databases based on specific subject areas.
Citation Help
Find useful resources for making citations quick and easy.
Zotero
Learn how to use the citation management software Zotero.
Ask a Librarian Video Tutorial
Transcripts
Video Transcript
- Ask a Librarian
In this short video, you will learn how to get in touch with the librarians at Sacred Heart University Library when you need some help.
Librarians are one of the best resources at the SHU Library whether you are trying to find something specific or just need help getting started with an assignment. There are a few ways to get in touch with us.

Remember: no matter how big or small the question, we are here to help! We look forward to hearing from you!
PDF Transcript
- Last Updated: Apr 30, 2021 4:22 PM
- URL: https://library.sacredheart.edu/researchSupport
- QuickSearch
- Library Catalog
- Databases A-Z
- Publication Finder
- Course Reserves
- Citation Linker
- Digital Commons
- Our Website
- Appointments
- Interlibrary Loan (ILL)
- Databases by Subject
- Citation Help
Using the Library
- Reserve a Group Study Room
- Renew Books
- Honors Study Rooms
- Off-Campus Access
- Library Policies
- Library Technology
User Information
- Grad Students
- Online Students
- COVID-19 Updates
- Staff Directory
- News & Announcements
- Library Newsletter
My Accounts
- Interlibrary Loan
- Staff Site Login

FIND US ON
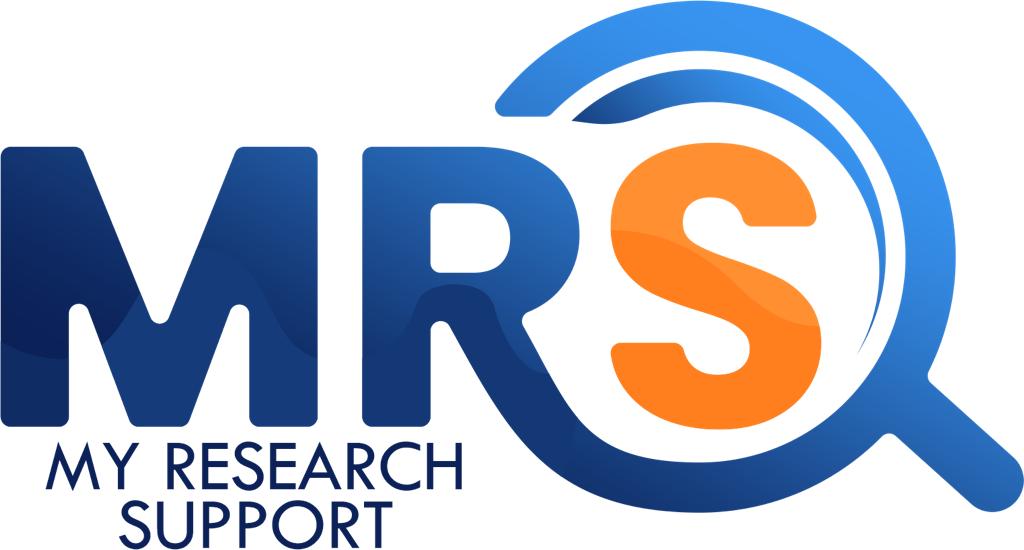
Research is the compass guiding humanity towards a brighter tomorrow.
Follow us on YouTube to never miss any updates from My Research Support
“None of us, including me, ever do great things. But we can all do small things, with great love, and together we can do something wonderful.” – Mother Teresa
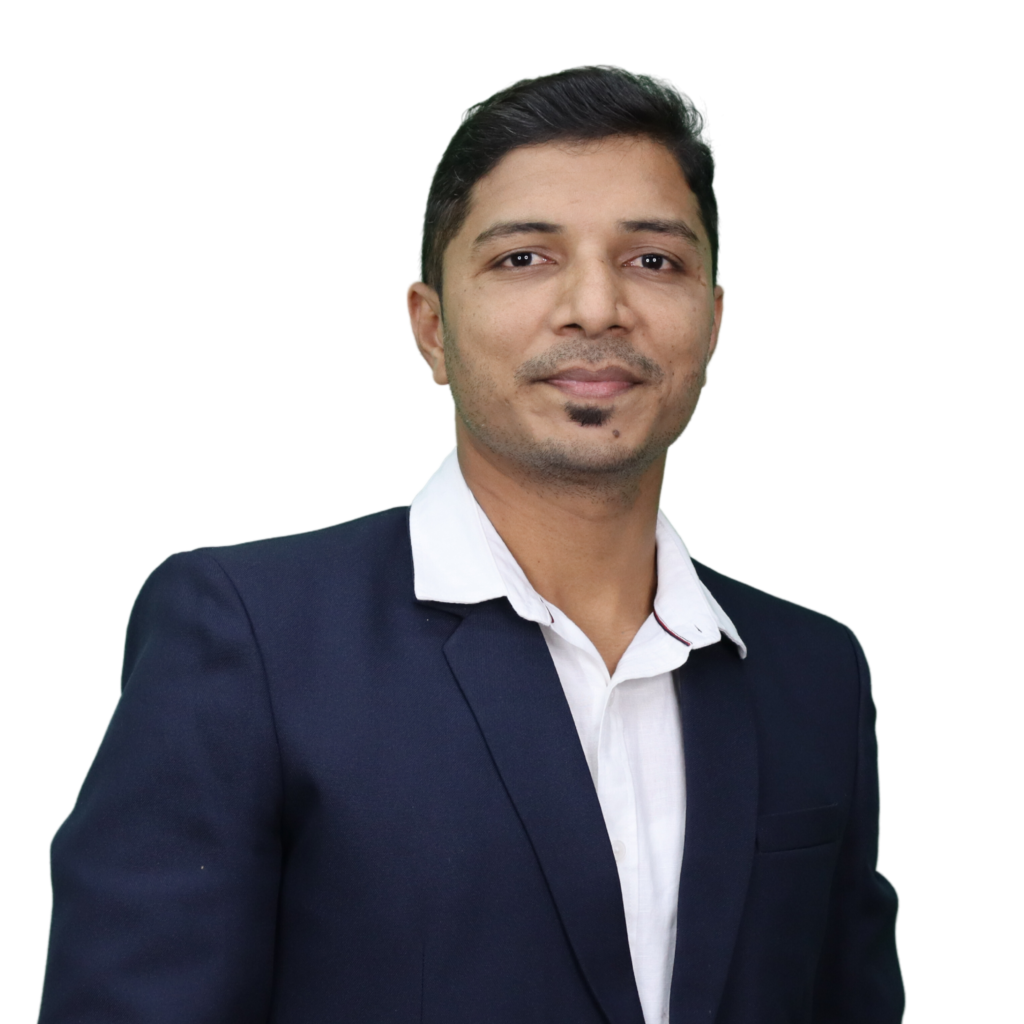
Welcome to My Research Support!
Nilesh Kumbhar is a research scientist, and he is here to support your research journey. The platform is meticulously designed to empower individuals with knowledge, guidance, and resources, making it an invaluable resource for both seasoned researchers and those who are just beginning their academic pursuits. His YouTube channel, “My Research Support,” is a treasure trove of valuable insights, tutorials, and tips spanning a wide range of research topics, from literature reviews to data analysis, ensuring comprehensive coverage of the subject matter.
Join us on this exciting journey of discovery and academic growth. Let’s get started!
Ricky Chandran
I enrolled in the research guidance course, and it exceeded all my expectations. The instructors were incredibly knowledgeable and supportive. Highly recommended
Pradnya Deshmukh
The research publication course not only provided me with valuable submission and publication techniques but also inspired me to pursue more ambitious research goals.
The instructors’ passion for research was contagious, and it motivated me to aim higher in my academic pursuits. Thank You

An official website of the United States government
The .gov means it’s official. Federal government websites often end in .gov or .mil. Before sharing sensitive information, make sure you’re on a federal government site.
The site is secure. The https:// ensures that you are connecting to the official website and that any information you provide is encrypted and transmitted securely.
- Publications
- Account settings
Preview improvements coming to the PMC website in October 2024. Learn More or Try it out now .
- Advanced Search
- Journal List
- HHS Author Manuscripts

The Online Social Support Scale: Measure Development and Validation
David A. Cole, Psychology and Human Development, Vanderbilt University
A new measure, the Online Social Support Scale, was developed based on previous theory, research, and measurement of in-person social support. It includes four subscales: esteem/emotional support, social companionship, informational support, and instrumental support. In college and community samples, factor analytic and item response theory results suggest that subtypes of in-person social support also pertain in the online world. Evidence of reliability, convergent validity, and discriminant validity provide excellent psychometric support for the measure. Construct validity accrues to the measure vis-à-vis support for three hypotheses: (1) Various broad types of Internet platforms for social interactions are differentially associated with online social support and online victimization, (2) Similar to in-person social support, online social support offsets the adverse effect of negative life events on self-esteem and depression-related outcome, and (3) Online social support counteracts the effects of online victimization in much the same way that in-person friends in one social niche counterbalance rejection in other social niches.
For millions of people around the world, the Internet now plays an enormous role in the development and maintenance of social relations. Online social media platforms have given rise to new and pervasive social niches that appear to operate in ways quite similar to in-person social niches ( Gonçalves et al., 2011 ). Many studies have focused on potential dangers and adverse effects of social media, including cybervictimization, online predators, and Internet addiction ( Aboujaoude, Savage, Starcevic, & Salame, 2015 ; Cassidy, Faucher, & Jackson, 2013 ; Wingate, Minney, & Guadagno, 2013). Despite the fact that online social relations (like all social relations) convey benefits as well as risks, research on positive aspects of online social relations is only just beginning. One such benefit is what we refer to as online social support (OSS), defined as the Internet-facilitated receipt of tangible and intangible assistance from friends, family, and others in one’s social circle ( House, 1981 ). The empirical studies of OSS that comprise this incipient literature have tended to rely on ad hoc measures often without strong psychometric support. Furthermore, different measures are based upon different theoretical conceptualizations of social support, thus complicating interpretation. The overarching goal of the current study was to develop and validate a new measure of OSS, consisting of four factors that have consistently emerged throughout the rich history of in-person social support research and theory.
Collectively, online social media platforms represent an increasingly popular vehicle for social interactions between people of all ages. In the United States between 2005 and 2015, the use of social networking sites increased from 55% to 76% among adolescents, from 12% to 90% among 18–29 year olds, from 8% to 77% among 30–49 year olds, from 5% to 51% among 50–64 year olds, and from 2% to 35% among people over 65 years old ( Lenhart, Purcell, Smith, & Zickuhr, 2010 ; Lenhart, 2015 ; Perrin, 2015 ). Similar trends are evident around the world ( Pew Research Center, 2012 ). The numbers are even larger when other online spaces (e.g., texting, email, multiplayer games) that also enable social interactions are included. The sheer number of online communications is staggering, with over 700,000,000 Snapchat and 500,000,000 Twitter posts per day ( Mulshine, 2015 ; Oreskovic, 2015 ). Engagement with online spaces is extremely high; for example, 1.13 billion people are “daily active users” of Facebook, meaning they access the site every day ( Facebook, 2016 ). Moreover, 92% of teens use the Internet daily, and 24% report using it “almost constantly” ( Lenhard, 2015 ).
Researchers have noted a variety of ways that online social interactions are similar to in-person interactions (e.g., Gonçalves et al., 2011 ; Gruzd, Wellman, & Takhteyev, 2011 ). Most research has focused special attention on negative features. For example, among high school students and young adults, cybervictimization has been associated with most of the same adverse outcomes that have been linked to in-person victimization, including depression, anxiety, somatic complaints, and low self-esteem ( Burnett, Yozwiak, & Omar, 2013 ; Gini, & Espelage, 2014 ). Some researchers have even suggested that cybervictimization has more severe or farther reaching consequences than does in-person victimization ( Cole, Zelkowitz e t al., 2016 ; Thomas, Connor, & Scott, 2015 ).
Far less research has focused on positive features of social media, such as its contributions to social support, despite the fact that social support theories (e.g., Cohen & Wills, 1985 ) and social capital theories (e.g., Ahn, 2012 ; Donath & Boyd, 2004 ; Ellison, Steinfield, & Lampe, 2007 ; Putnam, 2000 ; Steinfield, Ellison, & Lampe, 2008 ; cf., Lin, 1999 ) do not distinguish between online and in-person social phenomena. Cohen and Wills (1985) set forth two broad hypotheses about the ways that social support can mitigate the adverse effects of stress on health-related outcomes, a main effects hypothesis and a buffering hypothesis. The main effects hypothesis is supported when social support has a positive main effect on health and offsets the negative main effect of stress. As such, this model implies that “social resources have a beneficial effect irrespective of whether persons are under stress” or not (p. 310). The buffering hypothesis is supported by a support × stress interaction in which social “support ‘buffers’ (protects) persons from the potentially pathogenic influence of stressful events” (p. 310). As such, “support is related to well-being only (or primarily) for persons under stress” (p. 310).
With respect to online social support, preliminary research has provided evidence consistent with both models, especially in the context of specific health- and illness-focused interventions. For example, Turner-McGrievy and Tate (2013) demonstrated that participants in a weight-loss program were more successful when they used Twitter to engage with the counselor and other participants (main effect). Graham, Papandonatos, Kang, Moreno, and Abrams (2011) found that participation in an online smoking-reduction social support forum was associated with longer periods of smoking abstinence (main effect). Longman, O’Connor, and Obst (2009) reported that greater online social support among World of Warcraft players was associated with lower depression, anxiety, and stress (main effect). Indian and Grieve (2014) reported that online social support on Facebook had was associated with higher levels of well-being for people with high levels of social anxiety (buffering effect).
In these studies, extant measures of OSS tend to be study-specific and have highly diverse structures. Some treat OSS as a unitary construct (e.g., Graham et al., 2011 ), whereas others attempt to divide OSS into subtypes based on one theory or another (e.g., Turner-McGrievy & Tate, 2013 ). To ground our own measure of online social support in social support theory, we began by conducting a literature review of theoretical and empirical attempts to characterize the structure of in-person social support. From 22 papers (including eight contained in Barrera & Ainlay’s, 1983 previous literature review), we examined the number and nature of identified social support subtypes. After collapsing across conceptually similar subtypes and subtypes with poor discriminant validity, we identified 21 different subtypes of social support. In Table 1 , we present a modified stem-and-leaf plot of these results, in which social support subtype names are the stems and numeric representations of the publications are the leaves. Four subtypes were especially prevalent in this literature: (1) Esteem/Emotional support (EE) reflects communications from others that convey being held in high esteem, offering help in managing one’s emotional state, or expressing acceptance, intimacy, caring, liking, respect, validation, empathy, or sympathy. (2) Social Companionship (SC) support conveys a sense of belonging, either directly via expressions of inclusivity or indirectly by spending time together in leisure and recreational activities. (3) Informational support (INF) includes help in defining, understanding, and coping with problems; it may take the form of giving advice, offering appraisal support, sharing new information or perspectives, or providing reference to new resources. (4) Instrumental support (INS) includes provision of financial aid, material resources, and needed services; it includes offering help in getting necessary tasks done, providing something of use, performing a task, or taking on a responsibility. These four subtypes closely resemble those that derived from Cohen and Wills’ (1985) review.
Stem-and-leaf Plot of Literature Supporting Different Subtypes of Social Support
Note : Underlined citations denote the authors have split a particular subtype further into two or more types.
01: Barrera & Ainlay, 1983 (theoretical structure informed by literature review); 02: Barrera & Ainlay 1983 (empirical structure informed by factor analysis); 03: Brandt & Weinert, 1981 ; 04: Weinert, 2003 ; 05: Brim, 1974 ; 06: Caplan, 1976 ; 07: Cobb, 1976 ; Cobb, 1978 ; 08: Cohen & Hoberman, 1983 ; 09: Cohen & Wills, 1985 ; 10: Cutrona & Suhr, 1992 ; 11: Foa, 1993 ; 12: Gottlieb, 1978 ; 13: Hirsch, 1980 ; 14: House, 1981 ; 15: Jacobson, 1986 ; 16: Kahn & Antonucci, 1980 ; 17: Kaplan, Cassel, & Gore, 1977 ; 18: Killilea, 1975; 19: McCallister & Fischer, 1978 ; 20: Pinneau, 1975 ; 21: Tolsdorf, 1976 ; 22: Weiss 1973 .
The general purpose of the current study was to develop and validate a new measure of OSS, assessing the online occurrence of these four dimensions of social support: esteem/emotional support, social companionship, informational support, and instrumental support. This overarching goal was accomplished via four specific tasks. First, we identified potential items to represent each of the four subtypes of OSS that have predominated in the in-person social support literature. Second, we used factor analysis and item response theory to affirm the four-factor structure of the new measure and demonstrate that subtypes of social support that pertain in the in-person world also pertain in the online world. Third, we demonstrated discriminant and convergent validity of the resultant measure vis-à-vis other measures of social desirability, dissemblance, and in-person social support. And fourth, we demonstrated construct validity of the measure by examining its anticipated theoretical relations vis-à-vis three hypotheses: (1) Internet use for interacting with others will be positively associated with OSS and negatively associated with cybervictimization; (2) OSS will alleviate the adverse effects of stressful life events on self-esteem and depression-related outcomes; and (3) OSS will offset some of the adverse effects of online victimization, much like having friends in one in-person social niche can counterbalance the negative effects of victimization in a different social niche.
Online Social Support Scale (OSSS) Item Selection and Measure Construction
In order to obtain items representing all four domains of social support, we derived and adapted items from three sources: pre-existent measures of in-person social support, ad hoc measures of online social support, and published content analyses of online posts and Tweets. From in-person measures, we adapted 179 items from Barrera and Ainlay’s (1983) Inventory of Socially Supportive Behaviors; Barrera, Sandler, and Ramsay’s (1981) earlier version of the Inventory of Socially Supportive Behaviors; Barling, MacEwen, and Pratt’s (1988) questions about social support in response to vignettes; Cohen and Hoberman’s (1983) Interpersonal Support Evaluation List; Gottlieb’s (1978) classification scheme for informal helping behaviors; Norbeck, Lindsey, and Carrieri’s (1981) Norbeck Social Support Questionnaire; Brandt and Weinert’s (1981) Personal Resource Questionnaire; and Weinert’s (2003) Personal Resource Questionnaire. We reworded in-person social support items to reflect online social support. From extant measures of online social support, we obtained 37 items from Cole et al.’s Social Network Scales ( Cole, Nick, Zelkowitz, Roeder, & Spinelli, 2017 ; Cole, Nick, Varga, et al., 2016 ); Graham et al.’s (2011) Online Social Support for Smokers Scale; Krämer, Rösner, Eimler, Winter, and Neubaum’s (2014) Internet social capital work; and Eastin and LaRose’s (2005) modification of the Interpersonal Support Evaluation List ( Cohen, Mermelstein, Kamarck, & Hoberman, 1985 ). From published content analyses of social media material, we derived 63 items from Braithwaite, Waldron, and Finn’s (1999) content analysis of message board posts; Gaysynsky, Romansky-Poulin, and Arpadi’s (2015) content analysis of Facebook posts; and Turner-McGrievy and Tate’s (2013) content analysis based on Cutrona and Suhr’s (1992) work on socially supportive Tweets.
From this pool of 279 items, we began to assemble our measure. Some items were included in our measure with minor modifications. Often the content of items, but not wording or structure, inspired new items. Other items were collapsed because of redundancy or culled because of irrelevance. From the remaining items, four of the authors (EN, DC, DS, and GC) independently assembled their own sets of candidate items covering each subtype of social support. After conferring as a group, we converged on a single list of candidate items. In order to refine item phrasing, we consulted with college students and young adults who were knowledgeable about social media. We also piloted the items with 51 local high school students enrolled in an International Baccalaureate psychology class. They provided information about item clarity, redundancy, and acceptability. Culling ambiguous and redundant items resulted in an initial set of 48 items, with 12 items for each OSS subtype.
In order to orient participants to the kinds of online platforms we intended, we began the measure by asking participants to rate how frequently they use various popular social media sites, apps, and games. The instructions and a complete list of sites are the same as for the final version of the measure (see Appendix A ). Use of each site was rated on five-point Likert scales (0 = never , 1 = rarely , 2 = sometimes , 3 = pretty often , 4 = a lot ). Following these items, the instructions for the 48 OSS items read, “Now, think about the online spaces you use above. Rate how often the following things have happened for you while you interacted with others online over the last two months.” These instructions were followed by the Esteem/Emotional Support items (e.g., “People show that they care about me online”), Social Companionship items (e.g., “Online, I connect with people who like the same things I do”), Informational Support items (e.g., “When I’m online, people give me useful advice”), and Instrumental Support items (e.g., “Online, people offer to do things for me”). Each item was put on the same 5-point Likert scale as above. Higher scores reflected greater online social support.
Participants
Institutional Review Board approval was obtained for all research protocols involved in this project. In order to ensure a high degree of generalizability, we obtained data from both a college student sample and a young to middle-age community adult sample. After comparing the two samples, we combined them into a single data set (pooling their correlations). We conducted all analyses on the aggregated data (final N = 404). For cross validation of the OSSS results we obtained OSSS data on a third sample of young to middle-age community adults.
Sample 1: College student participants and procedures
Participants were 98 undergraduates at a southeastern private university, aged 18–23 (mean age = 19.21, SD = 1.08) and 77.6% female. The sample was moderately ethnically diverse: White (77.6%), Asian or Asian-American (14.3%), Hispanic or Mexican-American (9.2%), Black (7.1%), other (4.1%), and American Indian or Native American (0%). Participants could select more than one ethnicity. Most were freshmen (58.3%); fewer were sophomores (27.1%), juniors (7.3%), or seniors (7.3%).
In return for course credit, participants completed all measures online via Qualtrics. We obtained 113 participants, all of whom earned credit for their work. We eliminated 15 participants for invalid patterns of responding (i.e., completing the survey more quickly than we deemed possible, scoring higher than two standard deviations above the mean on a lie scale or a social desirability scale).
Sample 2: Community participants and procedures
Participants were 306 USA adults, aged 18–42 (mean age = 31.98, SD = 5.18), 46.4% female. The sample was 77.5% White, 11.8% Asian or Asian-American, 9.5% Black, 3.3% Hispanic or Mexican-American, 2.6% American Indian or Native American, and 1% other (participants could select more than one ethnicity). Most had some post-secondary education (mean years of education = 15.03, SD = 1.92). Participants reported working outside the home more than working from home (mean number of hours/week = 28.31, SD = 17.55 versus mean = 14.53, SD = 16.12).
Participants accessed the Qualtrics survey via a Human Intelligence Task on Amazon’s Mechanical Turk (mTurk) system. On mTurk, registered workers across the world can complete computerized tasks, such as online surveys, for small reimbursements. Only USA master workers (workers who have consistently demonstrated accuracy in previous work) could access the study, which was described as intended for workers aged 18–40. We paid each worker $4.00 for their participation, plus a $1.80 fee to Amazon. A total of 315 participants completed enough items for compensation. We eliminated nine participants for invalid patterns of responding (i.e., completing the survey too quickly, scoring higher than two standard deviations above the mean on a lie scale or a social desirability scale).
Sample 3: Community participants and procedures
Participants were 686 USA adults, aged 18–40 (mean age = 29.43, SD = 5.94), 50.1% female. The sample was 73.62% White, 12.24% Asian or Asian-American, 5.10% Black, 13.56% Hispanic or Mexican-American, 2.19% American Indian or Native American, and 1.02% other (participants could select more than one ethnicity). Most had some post-secondary education (mean years of education = 14.78, SD = 2.73). Participants reported working outside the home more than working from home (mean number of hours/week = 25.71, SD = 27.65 versus mean = 6.83, SD = 14.05).
Participants accessed the Qualtrics survey via Qualtrics Panels. Research volunteers who have signed up to work with Qualtrics Panels are alerted when they qualify for studies and are compensated for their time with points redeemable as gift cards, skymiles, credit for online games, or similar. We described the survey as intended for adults age 18–40. A total of 812 participants completed enough items for compensation. We eliminated 126 participants for invalid patterns of responding (i.e., completing the survey too quickly, scoring higher than two standard deviations above the mean on a lie scale or social desirability scale).
In addition to the 48-item version of the OSSS (described above), we administered measures of in-person social support, cybervictimization experiences, life stress, self-esteem, depressive thoughts and symptoms, response style, and time spent online.
Our measure of in-person social support was the Perceived Social Support Scale (PSSS; Procidano & Heller, 1983 ), which measures the extent to which individuals perceive that their needs for support, information, and feedback are being fulfilled by friends and by family. Items inquire about perceptions of support from friends and family, 20 items each. In the current study, we only used the friends subscale. Participants respond to each item on a three-point scale: no (0), I don’t know (1), or yes (2). Higher scores reflect greater support. The scale is well validated and has a high degree of internal consistency, coefficient alpha = .88 ( Procidano & Heller, 1983 ). For our analyses, we recoded answers as yes (1) and no (0). The KR-20 was .94 in sample 1 and .86 in sample 2.
The Cyberbullying Experiences Survey (CES; Doane, Kelley, Chiang, & Padilla, 2013 ) assesses cyberbullying victimization and perpetration in emerging adults. Twenty-one victimization items and 20 perpetration items make up two subscales. In the current study, we only used the victimization items, reflecting four correlated factors: malice, public humiliation, unwanted contact, and deception ( r s ranged from .38 to .53; coefficient alphas ranged from .74 to .89). Respondents rate items for frequency of occurrence on seven-point Likert scales from never (0) to every day/almost every day (6). Higher scores reflect greater cyberbullying. The composite CES (summing all four subscales) generated reliable scores with convergent validity, correlating well with other measures of cyberbullying ( Doane et al., 2013 ). In the current study, coefficient alpha was .94 in the sample 1 and .90 in sample 2.
The Life Experiences Survey (LES; Sarason, Johnson, & Siegel, 1978 ) assesses presence, timing, and impact of positive and negative life events in adults. Sixty-three items (outstanding personal achievements, death of a spouse, etc.) are rated for presence within 0–6 months or 7 months to one year. If present, their impact is rated on seven-point Likert scales ranging from extremely negative (−3) to extremely positive (3). The LES correlates with a variety of relevant dependent measures and has demonstrated good test-retest reliability (e.g., five-week retest correlations ranged from .64 to .88; Sarason et al., 1978 ). In the current study, we rescaled and summed the negative ratings, so that larger scores reflected greater impact of negative events.
The Rosenberg Self-Esteem Scale (RSE; Rosenberg, 1965 ) measures individual self-esteem. Ten items assess positive and negative self-cognitions. People rate items on four-point Likert scales from strongly agree (1) to strongly disagree (4). Negative items are reverse scored and added to positive items such that higher scores reflect greater self-esteem. In prior research, coefficient alphas have been high (.88) and exploratory factor analysis has resulted in a unidimensional solution ( Gray-Little, Hancock, & Williams, 1997 ). In the current study, coefficient alphas were .95 in sample 1 and .90 in sample 2.
The Cognitive Triad Inventory (CTI; Beckham, Leber, Watkins, Boyer, & Cook, 1986 ) assesses respondents’ view of self, world, and future via positively and negatively phrased items. These components reflect Beck’s depressive cognitive triad ( Beck, Rush, Shaw, & Emery, 1979 ). Thirty-six items total (10 for each component plus six filler items that are not scored) are rated on seven-point Likert scales ranging from totally agree (1) to totally disagree (7). Items were rescaled and summed so that higher scores represented more depressive cognitions. Previous research found that coefficient alpha was .95 for the total score ( Beckham et al., 1986 ). In the current study, coefficient alpha was .97 in the sample 1, and .95 in sample 2. To correct for skew, we computed a square-root transformation of the original scores.
The Beck Depression Inventory II (BDI; Beck, Steer, & Brown, 1996 ) is a commonly used, well-validated measure designed to assess the severity of depressive symptoms in a variety of populations. Twenty-one items describe different depressive symptoms that respondents may have experienced over the last two weeks. Respondents rate the severity of these symptoms on a 0 to 3 scale, with higher scores reflecting greater depressive symptoms. The BDI has been independently validated in a university population (coefficient alpha = .9; Dozois, Dobson, & Ahnberg, 1998 ). In the current study, as per IRB requirements, the suicidality item was removed. Coefficient alpha was .96 in the sample 1 and .89 in sample 2. To correct for skew, we computed square root transformations of the BDI scores.
The Marlowe-Crowne Short Form C (MCSF-C; Reynolds, 1982 ) is a 13-item short form of the Crowne - Marlowe Social Desirability Scale ( Crowne & Marlowe, 1960 ) that measures culturally approved but infrequent behaviors. Thirteen items on the MCSF-C Form C are answered as true (1) or false (0) with higher scores reflecting a greater tendency to respond in social desirable manner. The measure has adequate reliability (KR-20 = .76) and correlates well with the full Crowne and Marlowe Social Desirability Scale ( r = .93; Reynolds, 1982 ). In the current study, KR-20 was .76 in sample 1, .73 in sample 2, and .73 in sample 3.
The Revised Eysenck Personality Questionnaire Lie Scale - Short Form (EPQR-S; Eysenck, Eysenck, & Barrett, 1985 ) is a short form of the lie scale from the Revised Eysenck Personality Questionnaire ( Eysenck & Eysenck, 1975 ). The EPQR-S includes 12 yes/no items that measure the extent to which participants deliberately attempt to control their responses (e.g., “Are all your habits good and desirable ones?”). Higher scores reflect greater deliberate control. Reliability coefficients range from .73 to.77 ( Eysenck et al., 1985 ) and two-year longitudinal correlations range from .69 to .81 ( Roberts, Duffy, & Martin, 1995 ). In the current study the KR-20 was .78 in sample 1, .67 in sample 2, and .77 in sample 3.
We also measured time spent online (TSO) by asking participants how many hours per week they spent using online spaces such as text/photo/video sharing sites (e.g., Facebook, Instagram, Twitter, Snapchat, Tumblr, Vine, Google+), text communication sites (e.g., Texting, Email, Kik, Groupme, Whatsapp), anonymous discussion apps (e.g., YikYak, Whatsgoodly), forums (e.g., Reddit, 4chan), dating sites (e.g., Match.com, eHarmony), dating/hookup apps (e.g., Tinder, Bumble), sports/fighting/racing games (e.g., FIFA, Call of Duty, Need for Speed, Grand Theft Auto), and role player/battle arena games (e.g., World of Warcraft, League of Legends).
Schedule of assessments
Members of samples 1 and 2 completed the same battery of measures: the 48-item version of the OSSS, CES, PSSS, LES, RSE, CTI, BDI-II, MCSF-C, EPQR-S, and TSO. Members of sample 3 completed a subset of the above measures: a 40-item version of the OSSS (described below), MCSF-C, and EPQR-S. All participants completed the OSSS first; subsequent measures were ordered randomly to minimize the effects of fatigue.
Preliminary Analyses
First, we examined samples 1, 2, and 3 with respect to their use of the Internet (see Table 2 ). On average, all three samples used the Internet extensively, albeit in somewhat different ways. In sample 1 (college students), the most popular platforms were texting apps, email, SnapChat, GroupMe, Facebook, and Instagram. For sample 2, the most popular platforms were texting apps, email, Facebook, Reddit, Twitter, and YouTube. For sample 3, texting apps, email, Facebook, YouTube, Instagram, Twitter, and Pinterest were the most popular.
Hours/week Spent Using Online Sites, Apps, and Games
Before combining samples 1 and 2, we compared their correlation matrices, on which all subsequent analyses were based. Box’s test of homogeneity across the two 48 × 48 item-level OSSS correlation matrices (using full information maximum likelihood estimation) was significant ( χ 1176 2 = 1982.25 , p < 0.001); however, all other indices suggested that a model with all correlations constrained to be equal across groups provided a good fit to the data: TLI = .91, CFI = .96, RMSEA = .041 (90% CI .038 – .044, p close = 1.00). We conducted a similar cross-group comparison of the scale-level correlations among all 16 primary variables. Again, Box’s test was significant, ( χ 120 2 = 170.25 , p < 0.05); however, all other indices indicated that a fully constrained model provided an excellent fit to the data, TLI = .95, CFI = .97, RMSEA = .031 (90% CI .028 – .036, p close = 1.00).
Exploratory Factor Analyses of the OSSS
Exploratory factor analyses of the 48 OSSS items for samples 1 and 2 were based on the pooled correlation matrices. Analyses were conducted with Mplus, version 7.4, utilizing maximum likelihood estimation and oblique Geomin rotation. Examination of scree plots, parallel analysis results ( Horn, 1965 ; Zwick & Velicer, 1986 ), factor correlations, and factor loadings all suggested that a four-factor solution was optimal. Models with fewer factors provided significantly worse fits (e.g., a three-factor solution fit the data worse than a four-factor solution, Δχ 2 45 = 690.98, p < .001). Models with more factors showed signs of over-factoring (e.g., singlets, doublets, large factor correlations). Furthermore, the addition of more factors yielded only very small changes in the RMSEA (e.g., ΔRMSEA for a four- versus five-factor solution was only = .004.) Interpretability of the resultant factors was excellent, as 47 out of 48 of the items loaded strongly on the factor they were originally designed to represent, and all but one of these primary factor loadings were between .45 and .87. Crossloadings were small, with 139 out of 144 being < .30. Consistent with our a priori goals, factors 1 to 4 represented Esteem/Emotional Support, Social Companionship, Informational Support, and Instrumental Support, respectively. Factor intercorrelations ranged from .46 to .69.
To shorten the measure and eliminate problematic items, we dropped two items per factor. Culled items had relatively small primary factor loadings, relatively large crossloadings, or evidence of relatively large residual correlations. Coefficient alphas for the 10-item OSSS subscales were .95 for Esteem/Emotional Support, .94 for Social Companionship, .95 for Informational Support, and .95 for Instrumental Support (and Guttman’s L2 lower-bound estimates of reliability were .95, .94, .95, and .95, respectively).
We then factor analyzed the reduced, 40-item OSSS (see Appendix A ), using the same methods described above. Scree plots, parallel analysis, and goodness-of-fit statistics for models with 1, 2, 3, 4, 5, and 6 factors (see Table 3 ) collectively provide strong support for a 4-factor model. Factor loadings for the four-factor model appear in Table 4 . Factor correlations ranged from .46 to .69, large enough to justify summing the subscales (if researchers want a measure of total OSS) but not so large as to call into question the discriminant validity of the subscales. 1
Goodness-of-fit Information for Exploratory Factor Analyses of 40-item OSSS.
Exploratory Factor Analysis of the 40 Online Social Support Scale Items
Note . Loadings > .30 are in boldface.
Factor correlations for samples 1, 2: r 12 = .69, r 13 = .61, r 14 = .46, r 23 = .65, r 24 = .51, r 34 = .61.
Factor correlations for sample 3: r 12 = .70, r 13 = .74, r 14 = .55, r 23 = .72, r 24 = .56, r 34 = .60.
To cross-validate these results, we repeated all analyses for the 40-item OSSS using sample 3. Scree plots, parallel analysis, and goodness-of-fit statistics ( Table 3 ), and the factor loadings and factor correlations in Table 4 were very similar to those for samples 1 and 2, again providing strong support for the anticipated four factors. Coefficient alphas and Guttman L2 estimates were identical (to two digits) to those reported above.
Item Response Theory (IRT) Analyses
We conducted IRT analyses on samples 1, 2, and 3 using Mplus, version 7.4. Specifically, we used weighted least squares with adjusted means and variance (WLSMV) with oblique Geomin rotation to estimate model parameters of an exploratory 4-dimensional graded response model. Estimates of item discriminations and thresholds appear in Table 5 . The scale of the estimates was standardized in probit values.
IRT Estimates of Item Discriminations and Thresholds for Samples 1, 2, and 3
Note . EE = Esteem/Emotional, SC = Social companionship, INF = Informational, INS = Instrumental social support. Boldface highlights discrimination estimates > .30. Correlations among the dimensions were 0.623 ( SE =0.022) between EE and SC, 0.652 ( SE =0.018) between EE and INF, 0.619 ( SE =0.027) between SC and INF, 0.517 ( SE =0.022) between EE and INS, 0.485 ( SE =0.030) between SC and INS, and 0.616 ( SE =0.019) between INF and INS.
The discrimination parameter estimates the ability of an item to differentiate between people, with higher values reflecting greater differentiation capability. (Standard errors for these estimates were small, ranging from .017 – .047, Mdn = .022.) As shown in boldface in Table 5 , each set of items had high discriminations only for their respective dimensions.
Item thresholds represent item location information for each item on the sum of the four dimensions weighted by item discriminations. Thresholds in Table 5 represent item location information for each item. Threshold 1 reflects the transition point from a score of 0 (never) to scores 1–4 (rarely, sometimes, pretty often, or a lot); Threshold 2 reflects the transition point from scores of 0–1 to scores of 2–4; Threshold 3 reflects the transition point from scores of 0–2 to scores of 3–4; and Threshold 4 reflects the transition point from scores of 0–3 to a score of 4. For all items, thresholds were in order and well separated. (Standard errors were small, ranging from .017 – .047, Mdn = .022.) Thresholds were similar for items designed to represent the same dimension. These results indicate that items having the response anchors never, rarely, sometimes, pretty often , or a lot behaved as designed to assess individual differences in online social support.
We also plotted Test Information Functions (TIFs) for each dimension, holding the other dimensions constant at their means. A high value of test information and a wide curve on TIFs imply good measurement fidelity across a wide range on the underlying dimension. As shown in the figure, coverage of the EE, SC, and INF dimensions was quite good. Coverage of INS was better in the upper 84% of the dimension (i.e., for people with IRT-scores > −1). For INS, these TIF features are commensurate with its higher thresholds shown in Table 5 . (We note, however, that the information in these plots pertain to each construct controlling for the other three constructs. They do not reflect the multidimensional nature of OSS, on which scores are a function of a linear combination of the four subordinate OSS constructs, such that different people can obtain the same observed score in a wide variety of ways.)
Validation of the OSSS and Hypothesis Testing in Samples 1 and 2
Correlations.
We examined correlations of the OSSS and its subscales with the MCSF-C (social desirability) and EPQR-S (lie scale) for evidence of discriminant validity (see Table 6 ). The OSSS and its subscales correlated .12–.15 with the MCSF-C and .06–.12 with the EPQR-S. The OSSS scores shared less than 2% variance with these two problematic response styles, consistent with our expectation of relative freedom from bias due to impression management.
Pooled Sample Correlations and Separate Sample Means and Standard Deviations for All Study Variables for Samples 1 and 2
Note . OSSS = Online Social Support Scale total, EE = OSSS Esteem/Emotional, SC = OSSS Social Companionship, INF = OSSS Informational, INS = OSSS Instrumental, PSSS = Perceived Social Support Scale, CES = Cyberbullying Experiences Survey, CTI = Cognitive Triad Inventory, RSE = Rosenberg Self Esteem Scale, BDI = Beck Depression Inventory, LES = Life Events Scale, Use = OSSS social media use, TSO = time spent online, SD = Social Desirability Scale, LS = Lie Scale. Numbers in boldface indicate that means are significantly different between the two samples at p ≤ 0.05.
We also examined the relations of the OSSS and its subscales to the PSSS (perceived social support) for evidence of convergent validity . The total OSSS correlated .38 ( p < .01) with the PSSS, and correlations of the subscales with the PSSS ranged from .23 to .49 ( p s < .01). These significant correlations were large enough to suggest convergent validity but are not so large as to suggest redundancy of online and in-person social support. 2
Is Internet use associated with OSS as well as cybervictimization?
Part of validating a new measure is the demonstration that it relates to other constructs in anticipated ways. In this process, we tested several hypotheses. Our first hypothesis was that greater use of the Internet to connect with other people would be associated with not just greater risk for cybervictimization but greater OSS as well. Specifically, we anticipated that Internet spaces designed to promote social relations or communication (e.g., Facebook, Reddit, email) would be associated with greater OSS. Conversely, sites and apps that tend to facilitate evaluation, criticism, or competition (e.g., YikYak, dating sites/apps, competitive sports games) would increase negative exchanges.
We estimated participants’ use of seven broad types of online spaces, by standardizing and averaging usage data from the TSO and the initial part of the OSSS: (1) social media sites like Facebook and Twitter; (2) text communication sites like email and Kik; (3) anonymous discussion apps like YikYak and Whatsgoodly; (4) forums like Reddit and 4chan; (5) dating sites and apps like Tinder, Match, and eHarmony; (6) sports/fighting/racing games like FIFA, Call of Duty, and Need for Speed; and (7) role playing/battle arena games like World of Warcraft and League of Legends. We treated these seven measures as concomitant exogenous variables in a path analysis predicting total OSSS scores and total CES scores (see path diagram in Figure 1 ). All predictors were allowed to correlate, as were the disturbances for the two endogenous variables. For the regression of OSSS onto the seven predictors, the multiple R = .44, F 396 = 13.47, p < .001; social media use, text app use, and forum use were all significant predictors. For the regression of the CES onto the same predictors, the multiple R = .33, F 396 = 6.45, p < .001; anonymous discussion apps, and dating sites/apps, sports/fighting/racing games were all significant predictors.

Path analysis of differential effects of online platform use on online social support versus online victimization (samples 1 and 2).
Does online support alleviate effects of stressful life events on depression-related outcomes?
Our second hypothesis derived from Cohen and Wills’ (1985) main effects and buffering models. Construct validity would accrue to our new measure if OSS counteracted the effects of stressful life events by means of either an offsetting main effect or the buffering effect of an interaction. As stressful events have particularly strong effects on depression-related symptoms ( Hammen, 2005 ), we treated the RSE, CTI, and BDI-II as our outcome measures.
We conducted a series of two-step hierarchical regressions, one for each dependent variable. In each, step 1 constituted our test of the main effects for OSSS and LES. In step 2, we added the OSSS × LES interaction (all predictors were centered at 0, as per Aiken & West, 1991 ). All interactions were small and nonsignificant ( p s > .10, see top half of Table 7 ). In every analysis, a significant adverse main effect for LES was counterbalanced by a main effect for OSSS in the opposite direction. (Note that on the RSE, higher scores represent a better outcome, whereas on the CTI and BDI-II positive scores represent less healthy outcomes.) Follow-up analyses revealed that all four OSSS subscales were related to the RSE and CTI, but only the emotional support subscale was related to the BDI-II after controlling for type I error. These results simultaneously extend support for Cohen and Wills’ (1985) main effects theory to the online world and support the construct validity of the OSSS.
Regression of Self-esteem (RSE), Depressive thoughts (CTI), and Depressive Symptoms (BDI-II) onto Negative Life Events (LES) and either Online Social Support (OSSS) or In-person Social Support (PSSS) for Samples 1 and 2
Note . RSE = Rosenberg Self Esteem Scale, CTI = Cognitive Triad Inventory, BDI = Beck Depression Inventory, LES = Life Events Scale, OSSS = Online Social Support Scale, PSSS = Perceived Support Scale. All OSSS × LES and PSSS × LES interactions were nonsignificant ( p s > .20)
To confirm that the effects of OSS truly parallel the effects of in-person social support in the current data, we conducted a second series of hierarchical regressions, using the PSSS instead of the OSSS. As above, all interactions with LES were small and nonsignificant ( p s > .10). All main effects for PSSS and LES were significant and in opposite directions, consistent with Cohen and Wills’ (1985) main-effects model (see bottom half of Table 7 ). The only substantive difference between the online and in-person results was that the main effects of PSSS were stronger than the main effects of OSSS.
Does online support offset the adverse effects of online victimization?
Previous theory and research on in-person relationships suggests that social support can diminish the negative effects of social rejection or peer victimization ( Bilsky, et al., 2013 ; Maurizi, Grogan-Kaylor, Granillo, & Delva, 2013 ; Rothon, Head, Klineberg, & Stasfeld, 2011 ). Consequently, we examined the ability of both online and in-person social support to alleviate the effects of online victimization. As peer victimization has some of its strongest effects on depression-related symptoms ( Reijntjes, Kamphuis, Prinzie, & Telch, 2010 ), we again treated the RSE, CTI, and BDI-II as our outcome measures.
We conducted separate hierarchical regression analyses, one set for each dependent variable. In step 1 of each analysis, we entered cyberbullying scores (i.e., the CES) in order to document the total effect of online victimization. As shown in the top half of Table 8 , the CES significantly predicted all three dependent variables. In step 2, we added the OSSS. In every analysis, the OSSS and the CES were significant, albeit in opposite directions. Examination of the standardized coefficients reveals that the effect of OSSS was as large as or larger than the effect of CES, suggesting that the salubrious main effect of online social support offset the adverse main effect of online victimization in every analysis (see top of Table 8 ). In step 3, we tested the CES × OSSS interaction. Follow-up analyses revealed that all OSSS subscales were related to all outcome measures, except that INS and INF were not related to BDI-II scores after controlling for type-I error. In every analysis the interaction was not significant ( p s > .10) after statistically controlling for the main effects (results not shown in Table 8 ).
Regression of Self-esteem (RSE), Depressive thoughts (CTI), and Depressive Symptoms (BDI-II) onto Online Victimization (CES) and either Online Social Support (OSSS) or In-person Social Support (PSSS) for Samples 1 and 2
Note . RSE = Rosenberg Self Esteem Scale, CTI = Cognitive Triad Inventory, BDI = Beck Depression Inventory, CES = Cyberbullying Experiences Survey, OSSS = Online Social Support Scale, PSSS = Perceived Support Scale. OSSS × CES interactions were nonsignificant.
In order to see if the OSSS results (above) resembled the results for in-person social support, we conducted a parallel set of analyses, replacing the OSSS with the PSSS (see bottom half of Table 8 ). Step 1 again shows the significant adverse effects of CES on all three outcomes. In step 2, the effect of PSSS was large, significant, and in the opposite direction of the CES. In two of the analyses (predicting RSE and BDI-II), the CES was no longer significant when PSSS was in the model. In step 3, the PSSS × CES interaction emerged as a small but significant predictor of all three dependent variables. Both main effects remained significant as well. The nature of these interactions, however, was not consistent with Cohen and Wills’ (1985) hypothesized buffering effect (see Figure 2 ). Instead, the powerful main effect of low PSSS attenuated the effect of CES on the outcomes. Except for these interactions, the results for PSSS were generally similar to but stronger than the effects of OSSS on the three outcome variables.

Main Effect of Online Social Support and Moderating Effect of In-person Social Support in the Relation of Cybervictimization (CES) to Self-esteem (RSE), Depressive thoughts (CTI), and Depressive Symptoms (BDI-II) (samples 1 and 2).
Four key results emerged from the current study about the measurement, validation, and effects of online social support. First, we developed the Online Social Support Scale, the first of its kind that has both deep theoretical roots and strong psychometric properties. The scale has a highly interpretable factor structure, demonstrating that the four main subtypes of in-person social support also exist in the online world. Second, we found that spending more time online not only increases one’s risk for cybervictimization but also increases the extent of social support from one’s online social network. Third, similar to in-person social support, online social support represents an important positive resource that can offset some of the adverse effects of negative life events. Fourth, online social support (like in-person social support) helps to offset some of the adverse effects of online victimization. Each of these results has implications both for the validation of the OSSS and for the extension of in-person social support theory to the online world of social relations.
Our first goal, the construction of the Online Social Support Scale, was informed by substantial previous empirical and theoretical work on in-person social support plus the small but growing research into online social support. We built the OSSS to represent four major types of social support that have pervaded the in-person social support literature for decades: esteem/emotional support, social companionship, informational support, and instrumental support. Factor analysis of the OSSS revealed strong support for these factors. This result not only helps to validate the OSSS but it augments a growing literature suggesting that online communities function similarly to physical communities ( Gruzd et al., 2011 ). Gonçalves et al. (2011) further noted that the pervasive use of online social media platforms by all layers of society make them “an ideal proxy for the study of social interactions” (pp. 1–2). From the current study, we see that the primary subtypes of social support operating in the in-person world also exist in the online world.
The current study also provides sound psychometric support for the OSSS. The correlation of the OSSS with the PSSS was high enough to suggest good convergent validity but not so high as to call the distinctiveness of the OSSS into question. Correlations of the OSSS with measures of dissemblance and social desirability were small, an important feature given the tendency of some respondents for self-enhancement in survey research. The reliabilities of all four OSSS subscales were quite high.
Our second major finding speaks both to the construct validity of the OSSS and to a larger social issue. Since the emergence of social media, people have (understandably) been very concerned about its abuse. A PsycInfo search for “cybervictimization or cyberbullying” revealed over 1100 studies, dating back to 2006. In contrast, a search for “online social support” found less than 100 citations (most of which emerged since 2013), suggesting that the interpersonal benefits of social media have been somewhat neglected until recently. In the current study, we hypothesized that online social relationships, like in-person social relationships, carry rewards as well as risks. Supporting this contention, we found that increased Internet use was significantly related to not just increased rates of online victimization but increased social support as well.
These results complement a growing literature about the similarities between the in-person and online worlds. For example, research based on social capital theory ( Lin, 1999 ) has shown that social media use predicts bridging social capital and that valence of online communications predicts bonding social capital, in ways similar to face-to-face communications ( Ahn, 2012 ; Donath & Boyd, 2004 ; Ellison et al., 2007 ; Putnam, 2000 ; Steinfield et al., 2008 ). Gonçalves et al. (2011) further noted that the pervasive adoption of social media by all layers of society “makes it an ideal proxy for the study of social interactions” (pp. 1–2). Reflecting the downside of social media, cyber-victimization can have consequences at least as severe as in-person forms of victimization ( Bonanno & Hymel, 2013 ; Cole, Zelkowitz, Nick, Martin, et al., 2016 ; Kowalski, Giumetti, Schroeder, & Lattanner, 2014 ; Kowalski & Limber, 2013 ). Reflecting the upside, an incipient literature has begun to document the positive effects of OSS, at least as a tool for coping with specific, health-related problems (e.g., Braithwaite et al., 1999 ; Gaysynsky et al., 2015 ; Turner-McGrievy & Tate, 2013 ). The current study suggests that online social support is at least as strongly associated with Internet use as is online victimization. Our results further suggest that online social support and victimization are related to time spent in different online spaces. Whereas online support appears to accrue for those spending more time on classic social media sites and spaces facilitating communication (e.g., email, texting apps; forums), victimization appears to be more highly correlated with time spent on anonymous discussion apps, dating sites/apps, and sports, fighting, and racing games. An important direction for future research would be to study these same online spaces in younger samples, as children’s choice of online environment may have important implications for their experiences of online social support or victimization.
Our third major finding was that online social support (measured by the OSSS) counterbalanced some of the adverse effects of negative life events at least insofar as depression-related outcomes were concerned. This result has two implications. It extends support for Cohen and Wills’ (1985) main effects model to the online world, while simultaneously providing additional construct validation of the OSSS. Cohen and Wills’ main effects model suggests that stressful life events take their toll on human psychological and physical well-being but that strong social support can have an opposite salubrious effect for people at all levels of stress. Similar to Longman et al.’s (2009) research on the benefits of social support in online gaming, the current research found positive effects for all types of online social support. Although the results for online and in-person support were quite similar, the effects of in-person social support were stronger. Not surprisingly, social support that derives from traditional face-to-face relationships appears to be more powerful than social support that is completely online. The fact that channels of online communications are inherently more constrained has been deemed a possible simplifying advantage for people who have difficulty in face-to-face interactions ( Mesch & Talmudrence, 2006 ); however, the current results suggest that, on average, the advantages of face-to-face social support may be greater. An important direction for future research would be to explore individual difference characteristics that moderate the utility of online spaces as a source of social support (e.g., Longman et al., 2009 ).
Our fourth finding was that both online and in-person social support offset some of the adverse effects of cybervictimization. Social and developmental psychologists have repeatedly shown that interpersonal support from one social niche can make up for ostracism, rejection, and victimization in another social niche ( Hodges, Malone, & Perry, 1997 ; Parker & Asher, 1993 ; Schwartz, Dodge, Pettit, & Bates, 2000 ). The current results suggest that a similar compensatory model pertains in the online world. In our data, online victimization was associated with low self-esteem and depressive symptoms in a manner similar to the effects of in-person victimization (cf. Reijntjes et al., 2010 , for studies of negative effects of in-person PV). The effects of online social support, however, were just as strong and in the opposite direction, suggesting that the advantages of online social relationships offset their disadvantages. The effects of in-person social support were very similar, except for two things: (1) its main effect was larger and (2) for people with low levels of in-person social support, the additional effect of online victimization was negligible.
Limitations of the current study suggest important avenues for future research. First, we only measured in-person social support from friends. Although the Perceived Social Support Scale (PSSS) has both friends and family subscales, we only used the former. Future research should investigate the relative strengths of different sources of social support (e.g., friends, family, school/work, community, interest/affinity groups) in addition to the means of delivery of that support (in person, online).
Second, in the current study, we validated the OSSS only against depression-related outcomes such as depressive thinking, depressive symptoms, and self-esteem. Within the in-person world, social support relates to an enormous number of mental and physical health-related outcomes ( Ganster & Victor, 1988 ; Schwarzer & Leppin, 1989 ; Uchino, Bowen, Carlisle, & Birmingham, 2012 ). A critical direction for future research would be to examine the relation of the OSSS to a wider variety of outcomes, not only to document further the predictive validity of the measure but also to ascertain how broad the beneficial effects of OSS may be ( Wright & Bell, 2003 ).
Third, the current study documents the overarching merits of online social support; however, the mechanisms underlying its benefits are unknown. One possibility is that the benefits of OSS derive from the inclusion of people who are not part of one’s in-person social network. Alternatively, the benefits of OSS may reflect the fact that the same people behave differently online than they do in-person ( Cummings, Butler, & Kraut, 2002 ). Both of these possibilities are important and deserve further research.
Fourth, the current study examined the utility of OSS across a wide variety of people; however, it did not test for differential effects of OSS for various subtypes of people. Previous research suggests that social support via Facebook was especially useful to people with high levels of social anxiety, who may have found it easier to develop friendships online than in person ( Indian & Grieve, 2014 ). Future research should examine individual difference characteristics that could moderate the effects of OSS.
Fifth, we intentionally focused on broadband online social support, pooling across a wide variety of online platforms. The OSSS can easily be adapted to focus on a particular type of online experience (e.g., social media, gaming) or even a particular site or app (e.g., Reddit, YikYak). As suggested in the current study, different platforms may confer very different risks and benefits. Such information would be especially useful in middle or high school technology classes, where entry-level users may just be learning about different social media platforms.
Finally, it may be tempting to infer from many of our findings that OSS has a prospective if not causal relation to depression-related outcomes; however, the current study was cross-sectional. A valuable direction for future research would be to design longitudinal or experimental studies to test the degree to which OSS actually predicts changes in social, educational, or health outcomes over time.
Public Significance Statement
This study introduces a new self-report questionnaire to measure four broad types of online social support, similar to those found for in-person social support: esteem/emotional support, social companionship, informational support, and instrumental support. Different online sites and apps provide opportunities for different types and amounts of social support. Similar to in-person social support, online social support helps people cope with adversity.
Acknowledgments
This work was supported in part by a training grant from the National Institute of Mental Health (T32 MH018921), the Peter and Malina James & Dr. Louis P. James Legacy Scholarship (American Psychological Foundation), and by a Psi Chi Junior Scientist Fellowship (American Psychological Association of Graduate Students).
Online Social Support Scale (OSSS)
Most sites, apps, services, and games on the Internet can be used in lots of different ways and for different purposes. We’re interested in how much you use these online spaces to connect or interact with other people .
This means we are interested in how much you use these online spaces to talk with people, post, comment, like, send messages, game with others, etc.
This means we are not interested in how much you use these online spaces to scroll through other people’s posts, watch or read content, or just look up information.
How much do you use the following sites, apps, services, or games to connect or interact with other people?
Now, think about the online spaces you use above. Rate how often the following things have happened for you while you interacted with others online over the last two months. Use the following scale:
1 To test the uni-dimensionality of the four resultant factors, we repeated the above factor analysis four times, once for each set of scale-specific items. Each of these four analyses provided strong evidence of a single underlying factor. Further, the factor loadings did not change substantially from those reported in Table 4 .
2 Means and standard deviations for the community and college samples are also presented in Table 6 . The means for the community sample were significantly larger than the college sample for the SC, INF, INS, and LS measures and significantly smaller on the EE, CTI, Use, and TSO measures.
Note: References with asterisks (*) are included in Table 1 .
University of South Florida
Lifelong Learning
Office of Community Partnerships
Main Navigation
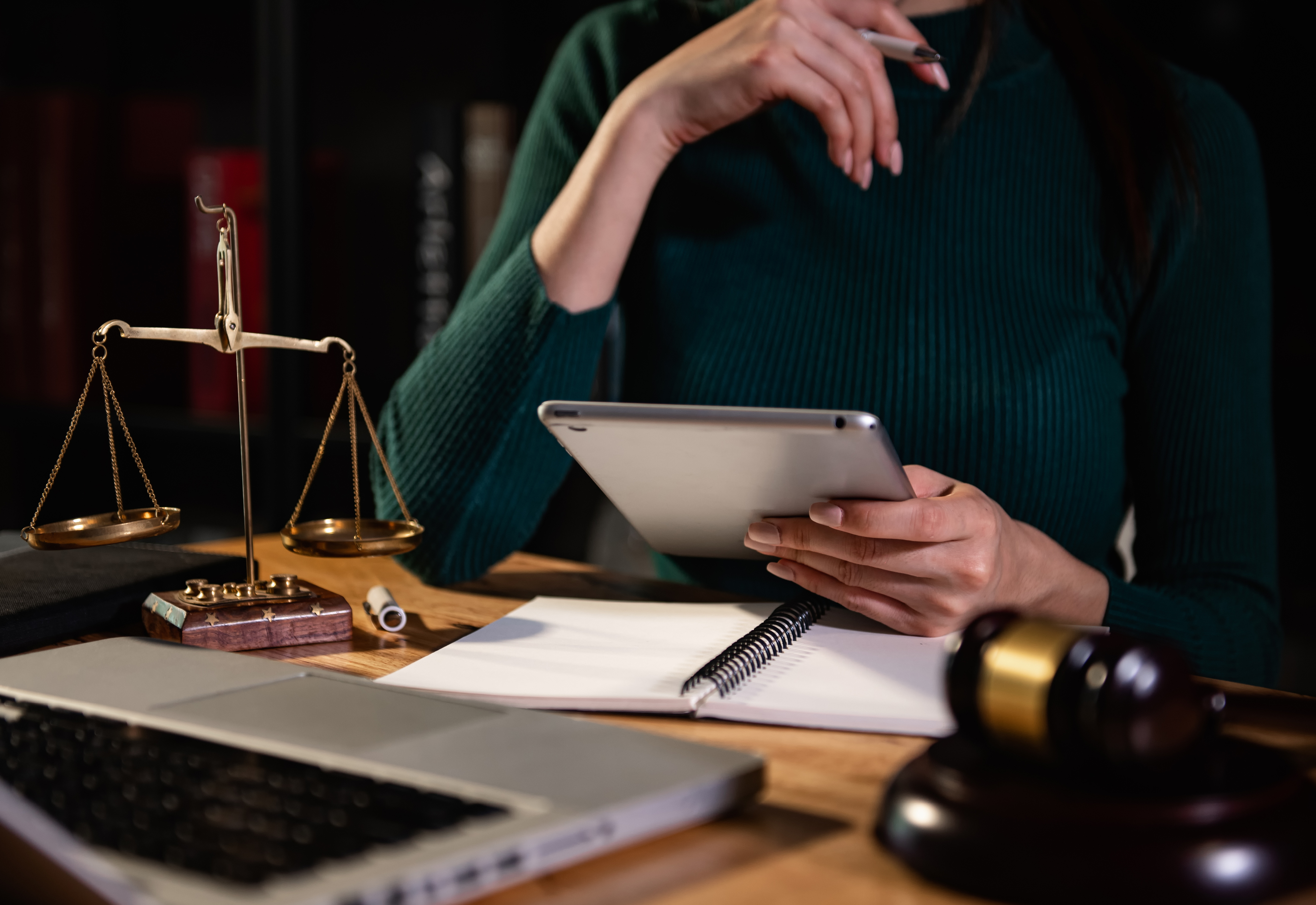
Online Legal Research Resources: Enrich Your Legal Career
- Leigh Perkins
- November 2, 2021
Legal , Paralegal
- Text-based Story
Effective legal research is a process. You need to identify the legal issue, find laws to support your argument, and confirm that your primary and secondary sources are still relevant. To accomplish this as a legal professional of a high standard, you require outstanding research tools. Fortunately, you can choose from a wide range of free and subscription-based online legal research resources to enrich your legal career . Let’s explore some of the most useful ones available.
4 Tips Before You Begin Your Online Legal Research
Stuck in multiyear contracts and paying a premium for add-ons? Legal research services can price themselves out of reach. Start by pinpointing the functions you need to narrow your options . Researching pricing can help you stay within budget – often made easier because many online legal research services are completely free.
1. Know Your Firm’s Needs
Do you need to check primary and secondary sources? Will you use a legal research tool for only a particular practice area?
2. Know the Functions and Tools Available
Does the basic software package fulfill your needs, or would you have to purchase extra features?
3. Know Your Costs
Have you compared the pricing options (some premium online research platforms offer pay-as-you-go plans, others charge by the document)?
4. Know If You Can Give It a Test Run
Have you registered for free trials to see if you like what you’ll get before you sign on?
Free Legal Research Tools
Accurate and efficient legal research does not have to be expensive. In fact, it can be free. Evaluate these highly recommended, no-cost legal research resources.
This free legal research service offers unlimited, easily searchable access to federal and state case law and legal data, such as slip opinions and statutes.
Bound Volumes of the Supreme Court
The Library of Congress provides official reports of decisions from the highest court in the country, which you can search by volume, authoring justice, or major case topic.
Caselaw Access Project (CAP)
CAP is a public-access platform, making all published U.S. court decisions freely available online in a consistent format, digitized from the collection of Harvard Law School Library.
Florida Digitized Legal Collections
As a collaborative effort by the Florida Academic Law Libraries of the law schools in the state of Florida, this searchable collection includes databases such as the Laws of Florida and Florida Supreme Court briefs and opinions.
Google Scholar for Legal Documents
This is a powerful search engine for law-specific resources related to Federal and State legal opinions, case law, and journal articles, which you can filter by jurisdiction.
One of the largest online databases of legal cases, this online legal research service offers case law, codes, opinion summaries, and other basic legal texts, with additional services such as attorney webhosting for a fee.
Get a free answer to your legal question, browse legal articles, download legal forms, and use an online legal dictionary.
Offering one of the web’s largest free libraries of consumer-friendly legal documents and information, this site also provides a lawyer directory, articles, books and software.
Find legal research by area of practice, information on federal judges and courts, law libraries, law schools and law dictionaries, plus a comprehensive breakdown of opinions by city, country, industry, medical treatment, sports league and more.
PublicLegal
In addition to law school rankings, salaries, tuition and state and federal government resources, you will find links to essential law search tools, such as U.S. Supreme Court decisions.
Enter a keyword, case name, judge, or statute and this free online collection delivers results.
Research common U.S. laws, locate a federal inmate, and review topics such as employment law and disability rights.
Premium Legal Research Tools
If you need quick and accurate legal research to support your case, it might be worth it to pay for access to high-quality databases . Their prices and specializations vary, but most are available for a monthly subscription fee.
Pay an all-inclusive monthly fee for access to federal and state cases, statutes, regulations and rules with annotated codified law, advanced citator and an AI-powered analyzer tool that finds relevant cases.
Bloomberg Law
Combining AI-enabled legal technology with workflow tools, comprehensive primary and secondary sources, news, and analysis, this subscription-based resource includes tools such as BCite, a citator’s tool.
Leveraging AI, this cloud-based legal research software company organizes the law with intelligent algorithms, making its data and legal content highly searchable, similar to Google.
Specializing in searchable, image-based PDFs that are an exact facsimile of the print document, this subscription database service has a massive archive of scholarly journals, case law, overseas cases and special collections, such as legal documents related to COVID-19.
With computer-assisted legal research by category or jurisdiction, analytics and practical legal guidance, this paid platform allows you to complete work with practice notes and checklists. It even provides a recommender system for specific issues and citation patterns.
Thomson Reuters WestLaw Edge
Offering accurate and up-to-date primary and secondary sources, litigation materials such as integrated briefs and dockets, the KeyCite tool for citators and document analysis, this paid platform provides news, business, public records and a useful list of sources.
Trellis Law
Powered by AI and paid by monthly subscription, this searchable database of state trial court records allows you to see how legal issues are decided across counties and states, or how judges have ruled on similar motions.
USF Is Committed to Your Success in the Legal Professions
If you’re beginning your career as a paralegal or seeking new skills for stress management as a lawyer, USF’s self-paced online legal courses are here to help you succeed. You can even earn continuing legal education (CLE) credit. Learn more about our program options today .
Return to article listing
Explore More Categories
- Director's Corner
- Hospitality
- Human Resources
- K-12 Education
- Leadership and Management
- Process Improvement
- Professional Development
- Project Management
- Sales and Marketing
About Corporate Training and Professional Education
USF Corporate Training and Professional Education empowers people to craft their future without limits through engaging professional growth learning and certification programs. Its programs focus on an array of topics – human resources, project management, paralegal, process improvement, leadership skills, technology, and much more.
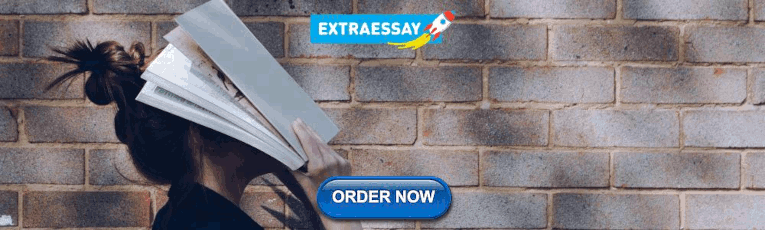
IMAGES
VIDEO
COMMENTS
Connect with your scientific community. Share your research, collaborate with your peers, and get the support you need to advance your career.
Research Computing at Harvard facilitates the advancement of research by providing leading-edge computing services including cluster computing, storage, software licenses, virtual instances, and software engineering. Services range from consultation and guidance to fully managed support. Guidance about working with data in compliance with ...
Qualitative data is gathered from sources like interviews, questionnaires, focus groups, participant observation, open-ended surveys, and recordings. We offer resources, software training, and general support to help you plan a qualitative research project and collect, analyze, and share qualitative data. Qualitative research support is open to ...
Research support. See a list of all subjects A-Z. Find a subject specialist. We have over fifty subject specialists that can help you with your research. Whether you are new to Stanford or a seasoned researcher, we are here to help. Find a subject specialist. Guides.
Make an appointment with a library liaison to get the help you need to get your work done. Many of Harvard's professional schools offer specialized support for data services in certain disciplines. Publishing Data Services at Countway Library Countway Library Review Service. Research Data Program at Baker Library. Show More.
General Research Support. We provide a wide range of resources and support for researchers as they work to advance scientific and medical discoveries. On This Page. Biosafety. Laboratory Animal Care (APLAC) Data and Informatics. Stanford Program on Research Rigor & Reproducibility (SPORR) Conflicts of Interest (COI) Office.
2. JSTOR. For journal articles, books, images, and even primary sources, JSTOR ranks among the best online resources for academic research. JSTOR's collection spans 75 disciplines, with strengths in the humanities and social sciences. The academic research database includes complete runs of over 2,800 journals.
Harvard Library's standard for providing access to more than 53,000,000 images from our libraries, archives, and museums. Your research matters. We build pathways to measure the impact. Harvard researchers change the world, and the world continues to change Harvard. In completing the research lifecycle, we want to ensure that you can track ...
Research Support Services. The Brown University Library provides support to researchers across all stages of the research cycle. This site provides access to information and tools to plan, conduct, manage, disseminate and preserve research. Please contact [email protected] with any questions or requests for support.
Research Computing at Harvard facilitates the advancement of research by providing leading-edge computing services including cluster computing, storage, software licenses, virtual instances, and software engineering. Services range from consultation and guidance to fully managed support. Guidance about working with data in compliance with ...
Resources for researchers. Expert guidance and resources to help you advance your research and authorship. Use our services and products to inspire your work, help find collaborators, improve grant proposals, get published, promote your research, track your influence and more.
Get Involved! Office of Internships and Prior Learning: Learn about recent and available project internships.. Faculty Fellows: Learn about current research fellows and how you can apply to participate.Some UIS faculty are willing to distance mentor undergraduate research student, so communicate with your online instructors.
Universities expand their online graduate programs daily, which challenges academic librarians to maintain research support and information literacy instruction for the growing number of online students. Performance support offers a scalable solution to the problems of limited time and staffing that academic librarians face. When embedded in ...
RESEARCH PERFORMANCE SUPPORT 5 by online doctoral students was attributed to the placement of the tutorials; these resources were not placed directly in the curriculum at "strategic points" (Kumar & Ochoa, 2012, p. 74). Point-of-need resources embedded strategically in the curriculum can be a successful way to
Google Scholar provides a simple way to broadly search for scholarly literature. Search across a wide variety of disciplines and sources: articles, theses, books, abstracts and court opinions.
A quantitative research approach was employed in the study to investigate the use of online research support platforms among undergraduate students at a university in Ghana. The results suggest that, even though there are online library research support platforms available for use, they are underutilized by undergraduates.
It is one of the most used tools for researchers and acts as an online search engine for academics looking for scholarly literature and relevant sources to cite. It is tailored for academics, which means you can use different keywords to look up various research articles, theses, books, and conference papers to support your research study.
File a complaint to Elsevier If you're not happy, we're not happy. We try to get everything right first time, but we know that sometimes things can go wrong. If you would like to contact us use this customer complaint form. Find answers to popular FAQs and contact options for Elsevier products, share feedback, or file a complaint.
A provision of student support is a necessary element of online student success (Rumble, 2000) that has been recognised in the past (Woodley & Simpson, 2014) and continues to be relevant within the research on student's attrition, retention, and dropout (Rotar, 2020).Different support models offer valuable conceptual frameworks for thinking about the approach to online student support (see ...
Venture Formation. Guidance and support for the formation of a business or startup related to research activities. Learn more about various research support topics that help shape the trajectory and success of your research.
©2020 Sacred Heart University Library 5151 Park Ave, Fairfield, CT 06825 tel. (203) 371-7702
Welcome to My Research Support! Nilesh Kumbhar is a research scientist, and he is here to support your research journey. The platform is meticulously designed to empower individuals with knowledge, guidance, and resources, making it an invaluable resource for both seasoned researchers and those who are just beginning their academic pursuits.
A new measure, the Online Social Support Scale, was developed based on previous theory, research, and measurement of in-person social support. It includes four subscales: esteem/emotional support, social companionship, informational support, and instrumental support. In college and community samples, factor analytic and item response theory ...
This study aims to examine how the process of online support obtainment may affect cognitive and behavioral coping during a public crisis. A cross-sectional online survey (N = 555) was conducted during the early stage of the COVID-19 pandemic in the U.S. Our findings revealed that informational support, obtained primarily through passive and ...
Research common U.S. laws, locate a federal inmate, and review topics such as employment law and disability rights. Premium Legal Research Tools. If you need quick and accurate legal research to support your case, it might be worth it to pay for access to high-quality databases. Their prices and specializations vary, but most are available for ...
Although our research identified a broad array of both structured and organic support networks available to U.S. journalists, the journalists we spoke with still felt that they lacked essential mechanisms for managing the negative health effects of on-the-job stressors; this was especially true with respect to online abuse, a finding also ...
H. also thanks Famille Huguenin for their guidance and everyone at the Climate Research Centre for their support. We thank the Consortium for Ocean-Sea Ice Modelling in Australia (COSIMA; https://cosima.org.au/) for their technical help and continued development of ACCESS-OM2. The simulations were conducted with resources and services from the ...
Research also shows that cognitive behavioral therapy (CBT) may be just as effective online as it is in person, but further studies are needed Ruwaard J, Lange A, Schrieken B, Dolan CV, Emmelkamp ...
Shareable Link. Use the link below to share a full-text version of this article with your friends and colleagues. Learn more.
Hainan Provincial Key Laboratory for Tropical Cardiovascular Diseases Research and Key Laboratory of Emergency and Trauma of Ministry of Education, Institute of Cardiovascular Research, Department of Cardiology, The First Affiliated Hospital, Hainan Medical University, Haikou, China ... The data that support the findings of this study are ...