Recommendation Systems: Applications and Examples in 2024

The recent global pandemic not only raised the demand for online shopping but also changed consumers’ behavior, as they required more personalized services from brands (Figure 1).
As the e-commerce industry grows , the demand for recommendation systems will grow with it. If you are planning to leverage recommendation systems to enhance your online store, keep reading.
In this article, we cover the following:
- What is a recommendation system, and how it works?
- Its benefits
- Top 6 industry applications/use cases
- Some case studies/examples
- Potential vendors
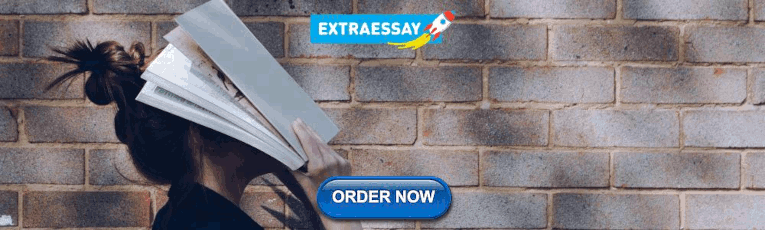
Figure 1. The importance of personalization in the post-pandemic market
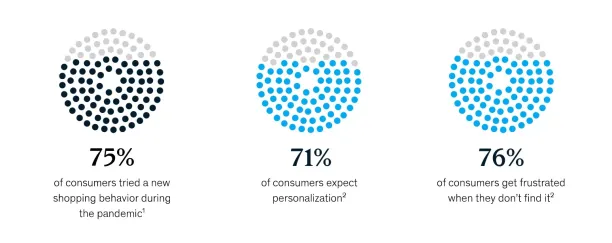
What is a recommendation system?
It is easy to get confused about recommendation systems as they are also sometimes called recommender systems or recommendation engines. All of these perform the same actions; they are systems that predict what your customers want by analyzing their behavior which contains information on past preferences.
How does it work?
Recommendation systems collect customer data and auto-analyze it to generate customized recommendations for your customers. These systems rely on both:
- Implicit data, such as browsing history and past purchases
- Explicit data, such as ratings provided by the user.
Content-based filtering and collaborative filtering are two approaches commonly used to generate recommendations. For more, please read the approaches section of our list of recommendation system vendors .
Benefits of recommendation systems
1. increased sales/conversion.
There are very few ways to achieve increased sales without increased marketing effort, and a recommendation system is one of them. Once you set up an automated recommendation system, you get recurring additional sales without any effort since it connects the shoppers with their desired products much faster.
2. Increased user satisfaction
The shortest path to a sale is great since it reduces the effort for both you and your customer. Recommendation systems allow you to reduce your customers’ path to a sale by recommending them a suitable option, sometimes even before they search for it.
3. Increased loyalty and share of mind
By getting customers to spend more on your website, you can increase their familiarity with your brand and user interface, increasing their probability of making future purchases from you.
4. Reduced churn
Recommendation system-powered emails are one of the best ways to re-engage customers. Discounts or coupons are other effective yet costly ways of re-engaging customers, and they can be coupled with recommendations to increase customers’ probability of conversion.
Applicable areas
Almost any business can benefit from a recommendation system. There are two important aspects that determine the level of benefit a business can gain from the technology.
- The breadth of data: A business serving only a handful of customers that behave in different ways will not receive many benefits from an automated recommendation system. Humans are still much better than machines in the area of learning from a few examples. In such cases, your employees will use their logic and qualitative and quantitative understanding of customers to make accurate recommendations.
- The depth of data : Having a single data point on each customer is also not helpful to recommendation systems. Deep data about customers’ online activities and, if possible, offline purchases can guide accurate recommendations
With this framework, we can identify industries that stand to gain from recommendation systems:
1. E-Commerce
Is an industry where recommendation systems were first widely used. With millions of customers and data on their online behavior, e-commerce companies are best suited to generate accurate recommendations.
Target scared shoppers back in the 2000s when Target systems were able to predict pregnancies even before mothers realized their own pregnancies . Shopping data is the most valuable data as it is the most direct data point on a customer’s intent. Retailers with troves of shopping data are at the forefront of companies making accurate recommendations.
Similar to e-commerce, media businesses are one of the first to jump into recommendations. It is difficult to see a news site without a recommendation system.
A mass-market product that is consumed digitally by millions. Banking for the masses and SMEs are prime for recommendations. Knowing a customer’s detailed financial situation, along with their past preferences, coupled with data of thousands of similar users, is quite powerful.
It Shares similar dynamics with banking. Telcos have access to millions of customers whose every interaction is recorded. Their product range is also rather limited compared to other industries, making recommendations in telecom an easier problem.
6. Utilities
Similar dynamics with telecom, but utilities have an even narrower range of products, making recommendations rather simple.
Examples from companies that use a recommendation engine
1. amazon.com.
Amazon.com uses item-to-item collaborative filtering recommendations on most pages of their website and e-mail campaigns. According to McKinsey, 35% of Amazon purchases are thanks to recommendation systems. Some examples of where Amazon uses recommendation systems are
Netflix is another data-driven company that leverages recommendation systems to boost customer satisfaction. The same Mckinsey study we mentioned above highlights that 75% of Netflix viewing is driven by recommendations. In fact, Netflix is so obsessed with providing the best results for users that they held data science competitions called Netflix Prize where one with the most accurate movie recommendation algorithm wins a prize worth $1,000,000.
Every week, Spotify generates a new customized playlist for each subscriber called “Discover Weekly” which is a personalized list of 30 songs based on users’ unique music tastes. Their acquisition of Echo Nest, a music intelligence and data-analytics startup, enable them to create a music recommendation engine that uses three different types of recommendation models:
- Collaborative filtering: Filtering songs by comparing users’ historical listening data with other users’ listening history.
- Natural language processing: Scraping the internet for information about specific artists and songs. Each artist or song is then assigned a dynamic list of top terms that changes daily and is weighted by relevance. The engine then determines whether two pieces of music or artists are similar.
- Audio file analysis: The algorithm each individual audio file’s characteristics, including tempo, loudness, key, and time signature, and makes recommendations accordingly.
4. Linkedin
Just like any other social media channel, LinkedIn also uses “You may also know” or “You may also like” types of recommendations.
Setting up a recommendation system
While most companies would benefit from adopting an existing solution, companies in niche categories or very high scale could experiment with building their own recommendation engine.
1. Using an out-of-the-box solution
Recommendation systems are one of the earliest and most mature AI use cases. As of Jan/2022, we have identified 10+ products in this domain. Visit our guide on recommendation systems to see all the vendors and learn more about specific recommendation engines.
The advantages of this approach include fast implementation and highly accurate results for most cases:
- Including a code snippet of the vendor can be enough to get started.
- Solutions tend to be accurate since vendors use data from thousands of transactions of their customers in an anonymized manner to improve their models.
To pick the right system, you can use historical or, even better, live data to test the effectiveness of different systems quickly.
2. Building your own solution
This can make sense if
- you are in a niche domain where recommendation engines were not used before or
- you own one of the world’s largest marketplaces where slightly better recommendations can make an important difference in your business outcomes.
Recommendation systems in the market today use logic like: customers with the similar purchase and browsing histories will purchase similar products in the future. To make such a system work, you either need a large number of historical transactions or detailed data on your user’s behavior on other websites. If you need such data, you could search for it in data marketplaces .
More data and better algorithms improve recommendations. You need to make use of all relevant data in your company, and you could expand your customer data with 3rd party data. If a regular customer of yours has been looking for red sneakers on other websites, why shouldn’t you show them a great pair when they visit your website?
3. Working with a consultant to build your own solutions
A slightly better recommendation engine could boost a company’s sales by a few percentage points, which could make a dramatic change in the profitability of a company with low-profit margins. Therefore, it can make sense to invest in building better recommendation engines if the company is not having satisfactory results from existing solution providers in the market .
AI consultants can help build specific models. We can help you identify partners in building custom recommendation engines:
If you want to learn more about custom AI solutions, feel free to read our whitepaper on the topic:
4. Running a data science competition to build your own solution
One possible approach is to use the wisdom of the crowd to build such systems. Companies can use encrypted historical data, launch data science competitions or work with consultants and get models providing highly effective recommendations.
Further reading
AI is not only applied to recommendation personalization. You can check out AI applications in marketing , sales , customer service , IT , data or analytics .
If you have any questions about recommendation systems, let us know:

Cem has been the principal analyst at AIMultiple since 2017. AIMultiple informs hundreds of thousands of businesses (as per similarWeb) including 60% of Fortune 500 every month. Cem's work has been cited by leading global publications including Business Insider , Forbes, Washington Post , global firms like Deloitte , HPE, NGOs like World Economic Forum and supranational organizations like European Commission . You can see more reputable companies and media that referenced AIMultiple. Throughout his career, Cem served as a tech consultant, tech buyer and tech entrepreneur. He advised businesses on their enterprise software, automation, cloud, AI / ML and other technology related decisions at McKinsey & Company and Altman Solon for more than a decade. He also published a McKinsey report on digitalization. He led technology strategy and procurement of a telco while reporting to the CEO. He has also led commercial growth of deep tech company Hypatos that reached a 7 digit annual recurring revenue and a 9 digit valuation from 0 within 2 years. Cem's work in Hypatos was covered by leading technology publications like TechCrunch and Business Insider . Cem regularly speaks at international technology conferences. He graduated from Bogazici University as a computer engineer and holds an MBA from Columbia Business School.
To stay up-to-date on B2B tech & accelerate your enterprise:
Next to Read
Business to business (b2b) marketing agency categorization in '24, b2b marketing survey: an in-depth guide in 2024, the ultimate 4 step guide to b2b marketing in 2024.
Your email address will not be published. All fields are required.
Related research
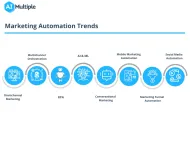
Top 8 Marketing Automation Trends for 2024 (with case studies)
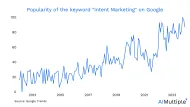
Intent Marketing: Overview, How-to, 3 Challenges & Best Practices
- Survey paper
- Open access
- Published: 03 May 2022
A systematic review and research perspective on recommender systems
- Deepjyoti Roy ORCID: orcid.org/0000-0002-8020-7145 1 &
- Mala Dutta 1
Journal of Big Data volume 9 , Article number: 59 ( 2022 ) Cite this article
59k Accesses
92 Citations
6 Altmetric
Metrics details
Recommender systems are efficient tools for filtering online information, which is widespread owing to the changing habits of computer users, personalization trends, and emerging access to the internet. Even though the recent recommender systems are eminent in giving precise recommendations, they suffer from various limitations and challenges like scalability, cold-start, sparsity, etc. Due to the existence of various techniques, the selection of techniques becomes a complex work while building application-focused recommender systems. In addition, each technique comes with its own set of features, advantages and disadvantages which raises even more questions, which should be addressed. This paper aims to undergo a systematic review on various recent contributions in the domain of recommender systems, focusing on diverse applications like books, movies, products, etc. Initially, the various applications of each recommender system are analysed. Then, the algorithmic analysis on various recommender systems is performed and a taxonomy is framed that accounts for various components required for developing an effective recommender system. In addition, the datasets gathered, simulation platform, and performance metrics focused on each contribution are evaluated and noted. Finally, this review provides a much-needed overview of the current state of research in this field and points out the existing gaps and challenges to help posterity in developing an efficient recommender system.
Introduction
The recent advancements in technology along with the prevalence of online services has offered more abilities for accessing a huge amount of online information in a faster manner. Users can post reviews, comments, and ratings for various types of services and products available online. However, the recent advancements in pervasive computing have resulted in an online data overload problem. This data overload complicates the process of finding relevant and useful content over the internet. The recent establishment of several procedures having lower computational requirements can however guide users to the relevant content in a much easy and fast manner. Because of this, the development of recommender systems has recently gained significant attention. In general, recommender systems act as information filtering tools, offering users suitable and personalized content or information. Recommender systems primarily aim to reduce the user’s effort and time required for searching relevant information over the internet.
Nowadays, recommender systems are being increasingly used for a large number of applications such as web [ 1 , 67 , 70 ], books [ 2 ], e-learning [ 4 , 16 , 61 ], tourism [ 5 , 8 , 78 ], movies [ 66 ], music [ 79 ], e-commerce, news, specialized research resources [ 65 ], television programs [ 72 , 81 ], etc. It is therefore important to build high-quality and exclusive recommender systems for providing personalized recommendations to the users in various applications. Despite the various advances in recommender systems, the present generation of recommender systems requires further improvements to provide more efficient recommendations applicable to a broader range of applications. More investigation of the existing latest works on recommender systems is required which focus on diverse applications.
There is hardly any review paper that has categorically synthesized and reviewed the literature of all the classification fields and application domains of recommender systems. The few existing literature reviews in the field cover just a fraction of the articles or focus only on selected aspects such as system evaluation. Thus, they do not provide an overview of the application field, algorithmic categorization, or identify the most promising approaches. Also, review papers often neglect to analyze the dataset description and the simulation platforms used. This paper aims to fulfil this significant gap by reviewing and comparing existing articles on recommender systems based on a defined classification framework, their algorithmic categorization, simulation platforms used, applications focused, their features and challenges, dataset description and system performance. Finally, we provide researchers and practitioners with insight into the most promising directions for further investigation in the field of recommender systems under various applications.
In essence, recommender systems deal with two entities—users and items, where each user gives a rating (or preference value) to an item (or product). User ratings are generally collected by using implicit or explicit methods. Implicit ratings are collected indirectly from the user through the user’s interaction with the items. Explicit ratings, on the other hand, are given directly by the user by picking a value on some finite scale of points or labelled interval values. For example, a website may obtain implicit ratings for different items based on clickstream data or from the amount of time a user spends on a webpage and so on. Most recommender systems gather user ratings through both explicit and implicit methods. These feedbacks or ratings provided by the user are arranged in a user-item matrix called the utility matrix as presented in Table 1 .
The utility matrix often contains many missing values. The problem of recommender systems is mainly focused on finding the values which are missing in the utility matrix. This task is often difficult as the initial matrix is usually very sparse because users generally tend to rate only a small number of items. It may also be noted that we are interested in only the high user ratings because only such items would be suggested back to the users. The efficiency of a recommender system greatly depends on the type of algorithm used and the nature of the data source—which may be contextual, textual, visual etc.
Types of recommender systems
Recommender systems are broadly categorized into three different types viz. content-based recommender systems, collaborative recommender systems and hybrid recommender systems. A diagrammatic representation of the different types of recommender systems is given in Fig. 1 .
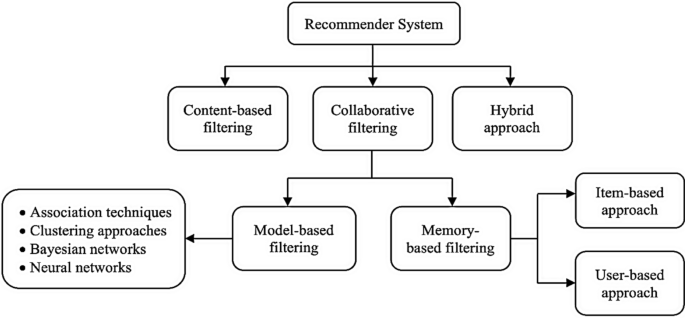
Content-based recommender system
In content-based recommender systems, all the data items are collected into different item profiles based on their description or features. For example, in the case of a book, the features will be author, publisher, etc. In the case of a movie, the features will be the movie director, actor, etc. When a user gives a positive rating to an item, then the other items present in that item profile are aggregated together to build a user profile. This user profile combines all the item profiles, whose items are rated positively by the user. Items present in this user profile are then recommended to the user, as shown in Fig. 2 .
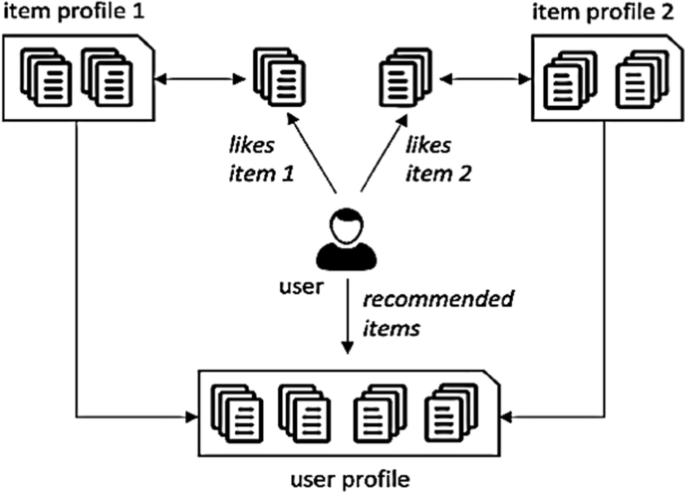
One drawback of this approach is that it demands in-depth knowledge of the item features for an accurate recommendation. This knowledge or information may not be always available for all items. Also, this approach has limited capacity to expand on the users' existing choices or interests. However, this approach has many advantages. As user preferences tend to change with time, this approach has the quick capability of dynamically adapting itself to the changing user preferences. Since one user profile is specific only to that user, this algorithm does not require the profile details of any other users because they provide no influence in the recommendation process. This ensures the security and privacy of user data. If new items have sufficient description, content-based techniques can overcome the cold-start problem i.e., this technique can recommend an item even when that item has not been previously rated by any user. Content-based filtering approaches are more common in systems like personalized news recommender systems, publications, web pages recommender systems, etc.
Collaborative filtering-based recommender system
Collaborative approaches make use of the measure of similarity between users. This technique starts with finding a group or collection of user X whose preferences, likes, and dislikes are similar to that of user A. X is called the neighbourhood of A. The new items which are liked by most of the users in X are then recommended to user A. The efficiency of a collaborative algorithm depends on how accurately the algorithm can find the neighbourhood of the target user. Traditionally collaborative filtering-based systems suffer from the cold-start problem and privacy concerns as there is a need to share user data. However, collaborative filtering approaches do not require any knowledge of item features for generating a recommendation. Also, this approach can help to expand on the user’s existing interests by discovering new items. Collaborative approaches are again divided into two types: memory-based approaches and model-based approaches.
Memory-based collaborative approaches recommend new items by taking into consideration the preferences of its neighbourhood. They make use of the utility matrix directly for prediction. In this approach, the first step is to build a model. The model is equal to a function that takes the utility matrix as input.
Model = f (utility matrix)
Then recommendations are made based on a function that takes the model and user profile as input. Here we can make recommendations only to users whose user profile belongs to the utility matrix. Therefore, to make recommendations for a new user, the user profile must be added to the utility matrix, and the similarity matrix should be recomputed, which makes this technique computation heavy.
Recommendation = f (defined model, user profile) where user profile ∈ utility matrix
Memory-based collaborative approaches are again sub-divided into two types: user-based collaborative filtering and item-based collaborative filtering. In the user-based approach, the user rating of a new item is calculated by finding other users from the user neighbourhood who has previously rated that same item. If a new item receives positive ratings from the user neighbourhood, the new item is recommended to the user. Figure 3 depicts the user-based filtering approach.
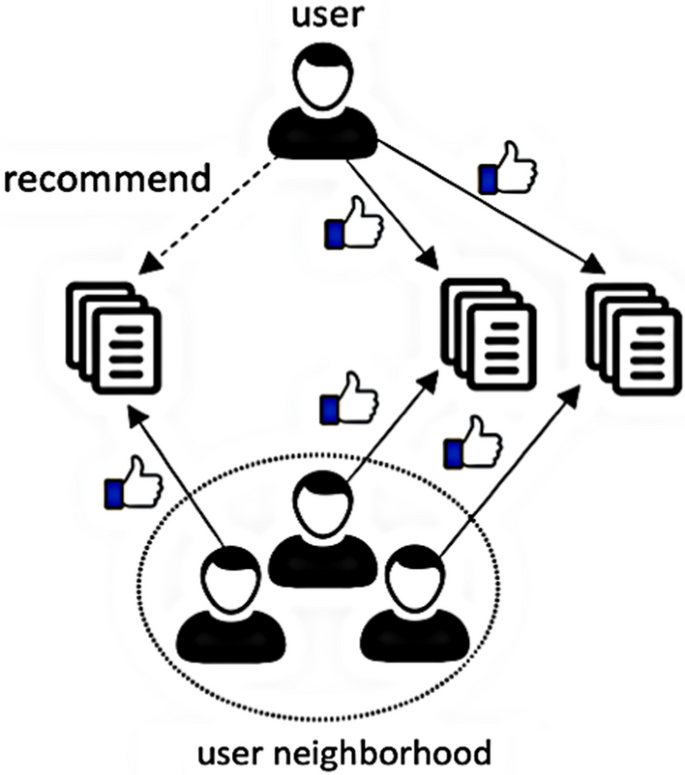
User-based collaborative filtering
In the item-based approach, an item-neighbourhood is built consisting of all similar items which the user has rated previously. Then that user’s rating for a different new item is predicted by calculating the weighted average of all ratings present in a similar item-neighbourhood as shown in Fig. 4 .
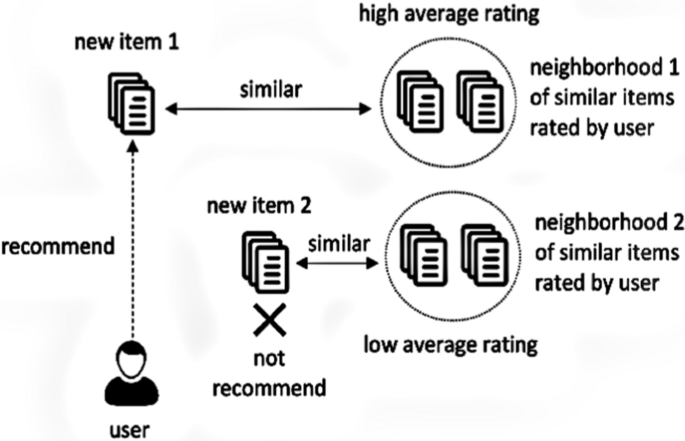
Item-based collaborative filtering
Model-based systems use various data mining and machine learning algorithms to develop a model for predicting the user’s rating for an unrated item. They do not rely on the complete dataset when recommendations are computed but extract features from the dataset to compute a model. Hence the name, model-based technique. These techniques also need two steps for prediction—the first step is to build the model, and the second step is to predict ratings using a function (f) which takes the model defined in the first step and the user profile as input.
Recommendation = f (defined model, user profile) where user profile ∉ utility matrix
Model-based techniques do not require adding the user profile of a new user into the utility matrix before making predictions. We can make recommendations even to users that are not present in the model. Model-based systems are more efficient for group recommendations. They can quickly recommend a group of items by using the pre-trained model. The accuracy of this technique largely relies on the efficiency of the underlying learning algorithm used to create the model. Model-based techniques are capable of solving some traditional problems of recommender systems such as sparsity and scalability by employing dimensionality reduction techniques [ 86 ] and model learning techniques.
Hybrid filtering
A hybrid technique is an aggregation of two or more techniques employed together for addressing the limitations of individual recommender techniques. The incorporation of different techniques can be performed in various ways. A hybrid algorithm may incorporate the results achieved from separate techniques, or it can use content-based filtering in a collaborative method or use a collaborative filtering technique in a content-based method. This hybrid incorporation of different techniques generally results in increased performance and increased accuracy in many recommender applications. Some of the hybridization approaches are meta-level, feature-augmentation, feature-combination, mixed hybridization, cascade hybridization, switching hybridization and weighted hybridization [ 86 ]. Table 2 describes these approaches.
Recommender system challenges
This section briefly describes the various challenges present in current recommender systems and offers different solutions to overcome these challenges.
Cold start problem
The cold start problem appears when the recommender system cannot draw any inference from the existing data, which is insufficient. Cold start refers to a condition when the system cannot produce efficient recommendations for the cold (or new) users who have not rated any item or have rated a very few items. It generally arises when a new user enters the system or new items (or products) are inserted into the database. Some solutions to this problem are as follows: (a) Ask new users to explicitly mention their item preference. (b) Ask a new user to rate some items at the beginning. (c) Collect demographic information (or meta-data) from the user and recommend items accordingly.
Shilling attack problem
This problem arises when a malicious user fakes his identity and enters the system to give false item ratings [ 87 ]. Such a situation occurs when the malicious user wants to either increase or decrease some item’s popularity by causing a bias on selected target items. Shilling attacks greatly reduce the reliability of the system. One solution to this problem is to detect the attackers quickly and remove the fake ratings and fake user profiles from the system.
Synonymy problem
This problem arises when similar or related items have different entries or names, or when the same item is represented by two or more names in the system [ 78 ]. For example, babywear and baby cloth. Many recommender systems fail to distinguish these differences, hence reducing their recommendation accuracy. To alleviate this problem many methods are used such as demographic filtering, automatic term expansion and Singular Value Decomposition [ 76 ].
Latency problem
The latency problem is specific to collaborative filtering approaches and occurs when new items are frequently inserted into the database. This problem is characterized by the system’s failure to recommend new items. This happens because new items must be reviewed before they can be recommended in a collaborative filtering environment. Using content-based filtering may resolve this issue, but it may introduce overspecialization and decrease the computing time and system performance. To increase performance, the calculations can be done in an offline environment and clustering-based techniques can be used [ 76 ].
Sparsity problem
Data sparsity is a common problem in large scale data analysis, which arises when certain expected values are missing in the dataset. In the case of recommender systems, this situation occurs when the active users rate very few items. This reduces the recommendation accuracy. To alleviate this problem several techniques can be used such as demographic filtering, singular value decomposition and using model-based collaborative techniques.
Grey sheep problem
The grey sheep problem is specific to pure collaborative filtering approaches where the feedback given by one user do not match any user neighbourhood. In this situation, the system fails to accurately predict relevant items for that user. This problem can be resolved by using pure content-based approaches where predictions are made based on the user’s profile and item properties.
Scalability problem
Recommender systems, especially those employing collaborative filtering techniques, require large amounts of training data, which cause scalability problems. The scalability problem arises when the amount of data used as input to a recommender system increases quickly. In this era of big data, more and more items and users are rapidly getting added to the system and this problem is becoming common in recommender systems. Two common approaches used to solve the scalability problem is dimensionality reduction and using clustering-based techniques to find users in tiny clusters instead of the complete database.
Methodology
The purpose of this study is to understand the research trends in the field of recommender systems. The nature of research in recommender systems is such that it is difficult to confine each paper to a specific discipline. This can be further understood by the fact that research papers on recommender systems are scattered across various journals such as computer science, management, marketing, information technology and information science. Hence, this literature review is conducted over a wide range of electronic journals and research databases such as ACM Portal, IEEE/IEE Library, Google Scholars and Science Direct [ 88 ].
The search process of online research articles was performed based on 6 descriptors: “Recommender systems”, “Recommendation systems”, “Movie Recommend*”, “Music Recommend*”, “Personalized Recommend*”, “Hybrid Recommend*”. The following research papers described below were excluded from our research:
News articles.
Master’s dissertations.
Non-English papers.
Unpublished papers.
Research papers published before 2011.
We have screened a total of 350 articles based on their abstracts and content. However, only research papers that described how recommender systems can be applied were chosen. Finally, 60 papers were selected from top international journals indexed in Scopus or E-SCI in 2021. We now present the PRISMA flowchart of the inclusion and exclusion process in Fig. 5 .
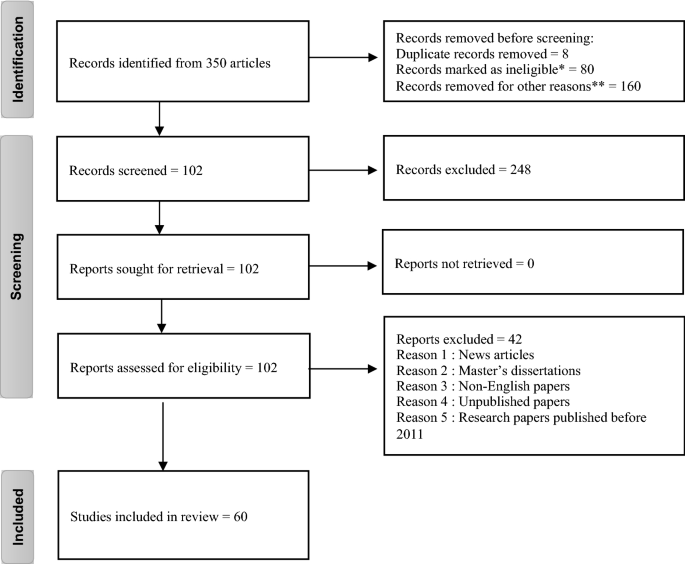
PRISMA flowchart of the inclusion and exclusion process. Abstract and content not suitable to the study: * The use or application of the recommender system is not specified: **
Each paper was carefully reviewed and classified into 6 categories in the application fields and 3 categories in the techniques used to develop the system. The classification framework is presented in Fig. 6 .
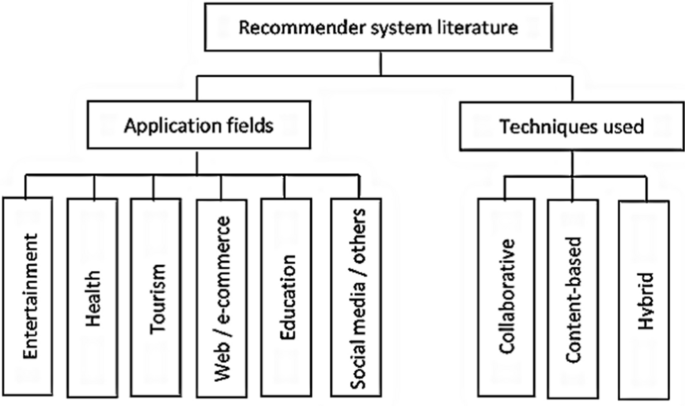
Classification framework
The number of relevant articles come from Expert Systems with Applications (23%), followed by IEEE (17%), Knowledge-Based System (17%) and Others (43%). Table 3 depicts the article distribution by journal title and Table 4 depicts the sector-wise article distribution.
Both forward and backward searching techniques were implemented to establish that the review of 60 chosen articles can represent the domain literature. Hence, this paper can demonstrate its validity and reliability as a literature review.
Review on state-of-the-art recommender systems
This section presents a state-of-art literature review followed by a chronological review of the various existing recommender systems.
Literature review
In 2011, Castellano et al. [ 1 ] developed a “NEuro-fuzzy WEb Recommendation (NEWER)” system for exploiting the possibility of combining computational intelligence and user preference for suggesting interesting web pages to the user in a dynamic environment. It considered a set of fuzzy rules to express the correlations between user relevance and categories of pages. Crespo et al. [ 2 ] presented a recommender system for distance education over internet. It aims to recommend e-books to students using data from user interaction. The system was developed using a collaborative approach and focused on solving the data overload problem in big digital content. Lin et al. [ 3 ] have put forward a recommender system for automatic vending machines using Genetic algorithm (GA), k-means, Decision Tree (DT) and Bayesian Network (BN). It aimed at recommending localized products by developing a hybrid model combining statistical methods, classification methods, clustering methods, and meta-heuristic methods. Wang and Wu [ 4 ] have implemented a ubiquitous learning system for providing personalized learning assistance to the learners by combining the recommendation algorithm with a context-aware technique. It employed the Association Rule Mining (ARM) technique and aimed to increase the effectiveness of the learner’s learning. García-Crespo et al. [ 5 ] presented a “semantic hotel” recommender system by considering the experiences of consumers using a fuzzy logic approach. The system considered both hotel and customer characteristics. Dong et al. [ 6 ] proposed a structure for a service-concept recommender system using a semantic similarity model by integrating the techniques from the view of an ontology structure-oriented metric and a concept content-oriented metric. The system was able to deliver optimal performance when compared with similar recommender systems. Li et al. [ 7 ] developed a Fuzzy linguistic modelling-based recommender system for assisting users to find experts in knowledge management systems. The developed system was applied to the aircraft industry where it demonstrated efficient and feasible performance. Lorenzi et al. [ 8 ] presented an “assumption-based multiagent” system to make travel package recommendations using user preferences in the tourism industry. It performed different tasks like discovering, filtering, and integrating specific information for building a travel package following the user requirement. Huang et al. [ 9 ] proposed a context-aware recommender system through the extraction, evaluation and incorporation of contextual information gathered using the collaborative filtering and rough set model.
In 2012, Chen et al. [ 10 ] presented a diabetes medication recommender model by using “Semantic Web Rule Language (SWRL) and Java Expert System Shell (JESS)” for aggregating suitable prescriptions for the patients. It aimed at selecting the most suitable drugs from the list of specific drugs. Mohanraj et al. [ 11 ] developed the “Ontology-driven bee’s foraging approach (ODBFA)” to accurately predict the online navigations most likely to be visited by a user. The self-adaptive system is intended to capture the various requirements of the online user by using a scoring technique and by performing a similarity comparison. Hsu et al. [ 12 ] proposed a “personalized auxiliary material” recommender system by considering the specific course topics, individual learning styles, complexity of the auxiliary materials using an artificial bee colony algorithm. Gemmell et al. [ 13 ] demonstrated a solution for the problem of resource recommendation in social annotation systems. The model was developed using a linear-weighted hybrid method which was capable of providing recommendations under different constraints. Choi et al. [ 14 ] proposed one “Hybrid Online-Product rEcommendation (HOPE) system” by the integration of collaborative filtering through sequential pattern analysis-based recommendations and implicit ratings. Garibaldi et al. [ 15 ] put forward a technique for incorporating the variability in a fuzzy inference model by using non-stationary fuzzy sets for replicating the variabilities of a human. This model was applied to a decision problem for treatment recommendations of post-operative breast cancer.
In 2013, Salehi and Kmalabadi [ 16 ] proposed an e-learning material recommender system by “modelling of materials in a multidimensional space of material’s attribute”. It employed both content and collaborative filtering. Aher and Lobo [ 17 ] introduced a course recommender system using data mining techniques such as simple K-means clustering and Association Rule Mining (ARM) algorithm. The proposed e-learning system was successfully demonstrated for “MOOC (Massively Open Online Courses)”. Kardan and Ebrahimi [ 18 ] developed a hybrid recommender system for recommending posts in asynchronous discussion groups. The system was built combining both collaborative filtering and content-based filtering. It considered implicit user data to compute the user similarity with various groups, for recommending suitable posts and contents to its users. Chang et al. [ 19 ] adopted a cloud computing technology for building a TV program recommender system. The system designed for digital TV programs was implemented using Hadoop Fair Scheduler (HFC), K-means clustering and k-nearest neighbour (KNN) algorithms. It was successful in processing huge amounts of real-time user data. Lucas et al. [ 20 ] implemented a recommender model for assisting a tourism application by using associative classification and fuzzy logic to predict the context. Niu et al. [ 21 ] introduced “Affivir: An Affect-based Internet Video Recommendation System” which was developed by calculating user preferences and by using spectral clustering. This model recommended videos with similar effects, which was processed to get optimal results with dynamic adjustments of recommendation constraints.
In 2014, Liu et al. [ 22 ] implemented a new route recommendation model for offering personalized and real-time route recommendations for self-driven tourists to minimize the queuing time and traffic jams infamous tourist places. Recommendations were carried out by considering the preferences of users. Bakshi et al. [ 23 ] proposed an unsupervised learning-based recommender model for solving the scalability problem of recommender systems. The algorithm used transitive similarities along with Particle Swarm Optimization (PSO) technique for discovering the global neighbours. Kim and Shim [ 24 ] proposed a recommender system based on “latent Dirichlet allocation using probabilistic modelling for Twitter” that could recommend the top-K tweets for a user to read, and the top-K users to follow. The model parameters were learned from an inference technique by using the differential Expectation–Maximization (EM) algorithm. Wang et al. [ 25 ] developed a hybrid-movie recommender model by aggregating a genetic algorithm (GA) with improved K-means and Principal Component Analysis (PCA) technique. It was able to offer intelligent movie recommendations with personalized suggestions. Kolomvatsos et al. [ 26 ] proposed a recommender system by considering an optimal stopping theory for delivering books or music recommendations to the users. Gottschlich et al. [ 27 ] proposed a decision support system for stock investment recommendations. It computed the output by considering the overall crowd’s recommendations. Torshizi et al. [ 28 ] have introduced a hybrid recommender system to determine the severity level of a medical condition. It could recommend suitable therapies for patients suffering from Benign Prostatic Hyperplasia.
In 2015, Zahálka et al. [ 29 ] proposed a venue recommender: “City Melange”. It was an interactive content-based model which used the convolutional deep-net features of the visual domain and the linear Support Vector Machine (SVM) model to capture the semantic information and extract latent topics. Sankar et al. [ 30 ] have proposed a stock recommender system based on the stock holding portfolio of trusted mutual funds. The system employed the collaborative filtering approach along with social network analysis for offering a decision support system to build a trust-based recommendation model. Chen et al. [ 31 ] have put forward a novel movie recommender system by applying the “artificial immune network to collaborative filtering” technique. It computed the affinity of an antigen and the affinity between an antibody and antigen. Based on this computation a similarity estimation formula was introduced which was used for the movie recommendation process. Wu et al. [ 32 ] have examined the technique of data fusion for increasing the efficiency of item recommender systems. It employed a hybrid linear combination model and used a collaborative tagging system. Yeh and Cheng [ 33 ] have proposed a recommender system for tourist attractions by constructing the “elicitation mechanism using the Delphi panel method and matrix construction mechanism using the repertory grids”, which was developed by considering the user preference and expert knowledge.
In 2016, Liao et al. [ 34 ] proposed a recommender model for online customers using a rough set association rule. The model computed the probable behavioural variations of online consumers and provided product category recommendations for e-commerce platforms. Li et al. [ 35 ] have suggested a movie recommender system based on user feedback collected from microblogs and social networks. It employed the sentiment-aware association rule mining algorithm for recommendations using the prior information of frequent program patterns, program metadata similarity and program view logs. Wu et al. [ 36 ] have developed a recommender system for social media platforms by aggregating the technique of Social Matrix Factorization (SMF) and Collaborative Topic Regression (CTR). The model was able to compute the ratings of users to items for making recommendations. For improving the recommendation quality, it gathered information from multiple sources such as item properties, social networks, feedback, etc. Adeniyi et al. [ 37 ] put forward a study of automated web-usage data mining and developed a recommender system that was tested in both real-time and online for identifying the visitor’s or client’s clickstream data.
In 2017, Rawat and Kankanhalli [ 38 ] have proposed a viewpoint recommender system called “ClickSmart” for assisting mobile users to capture high-quality photographs at famous tourist places. Yang et al. [ 39 ] proposed a gradient boosting-based job recommendation system for satisfying the cost-sensitive requirements of the users. The hybrid algorithm aimed to reduce the rate of unnecessary job recommendations. Lee et al. [ 40 ] proposed a music streaming recommender system based on smartphone activity usage. The proposed system benefitted by using feature selection approaches with machine learning techniques such as Naive Bayes (NB), Support Vector Machine (SVM), Multi-layer Perception (MLP), Instance-based k -Nearest Neighbour (IBK), and Random Forest (RF) for performing the activity detection from the mobile signals. Wei et al. [ 41 ] have proposed a new stacked denoising autoencoder (SDAE) based recommender system for cold items. The algorithm employed deep learning and collaborative filtering method to predict the unknown ratings.
In 2018, Li et al. [ 42 ] have developed a recommendation algorithm using Weighted Linear Regression Models (WLRRS). The proposed system was put to experiment using the MovieLens dataset and it presented better classification and predictive accuracy. Mezei and Nikou [ 43 ] presented a mobile health and wellness recommender system based on fuzzy optimization. It could recommend a collection of actions to be taken by the user to improve the user’s health condition. Recommendations were made considering the user’s physical activities and preferences. Ayata et al. [ 44 ] proposed a music recommendation model based on the user emotions captured through wearable physiological sensors. The emotion detection algorithm employed different machine learning algorithms like SVM, RF, KNN and decision tree (DT) algorithms to predict the emotions from the changing electrical signals gathered from the wearable sensors. Zhao et al. [ 45 ] developed a multimodal learning-based, social-aware movie recommender system. The model was able to successfully resolve the sparsity problem of recommender systems. The algorithm developed a heterogeneous network by exploiting the movie-poster image and textual description of each movie based on the social relationships and user ratings.
In 2019, Hammou et al. [ 46 ] proposed a Big Data recommendation algorithm capable of handling large scale data. The system employed random forest and matrix factorization through a data partitioning scheme. It was then used for generating recommendations based on user rating and preference for each item. The proposed system outperformed existing systems in terms of accuracy and speed. Zhao et al. [ 47 ] have put forward a hybrid initialization method for social network recommender systems. The algorithm employed denoising autoencoder (DAE) neural network-based initialization method (ANNInit) and attribute mapping. Bhaskaran and Santhi [ 48 ] have developed a hybrid, trust-based e-learning recommender system using cloud computing. The proposed algorithm was capable of learning online user activities by using the Firefly Algorithm (FA) and K-means clustering. Afolabi and Toivanen [ 59 ] have suggested an integrated recommender model based on collaborative filtering. The proposed model “Connected Health for Effective Management of Chronic Diseases”, aimed for integrating recommender systems for better decision-making in the process of disease management. He et al. [ 60 ] proposed a movie recommender system called “HI2Rec” which explored the usage of collaborative filtering and heterogeneous information for making movie recommendations. The model used the knowledge representation learning approach to embed movie-related information gathered from different sources.
In 2020, Han et al. [ 49 ] have proposed one Internet of Things (IoT)-based cancer rehabilitation recommendation system using the Beetle Antennae Search (BAS) algorithm. It presented the patients with a solution for the problem of optimal nutrition program by considering the objective function as the recurrence time. Kang et al. [ 50 ] have presented a recommender system for personalized advertisements in Online Broadcasting based on a tree model. Recommendations were generated in real-time by considering the user preferences to minimize the overhead of preference prediction and using a HashMap along with the tree characteristics. Ullah et al. [ 51 ] have implemented an image-based service recommendation model for online shopping based random forest and Convolutional Neural Networks (CNN). The model used JPEG coefficients to achieve an accurate prediction rate. Cai et al. [ 52 ] proposed a new hybrid recommender model using a many-objective evolutionary algorithm (MaOEA). The proposed algorithm was successful in optimizing the novelty, diversity, and accuracy of recommendations. Esteban et al. [ 53 ] have implemented a hybrid multi-criteria recommendation system concerned with students’ academic performance, personal interests, and course selection. The system was developed using a Genetic Algorithm (GA) and aimed at helping university students. It combined both course information and student information for increasing system performance and the reliability of the recommendations. Mondal et al. [ 54 ] have built a multilayer, graph data model-based doctor recommendation system by exploiting the trust concept between a patient-doctor relationship. The proposed system showed good results in practical applications.
In 2021, Dhelim et al. [ 55 ] have developed a personality-based product recommending model using the techniques of meta path discovery and user interest mining. This model showed better results when compared to session-based and deep learning models. Bhalse et al. [ 56 ] proposed a web-based movie recommendation system based on collaborative filtering using Singular Value Decomposition (SVD), collaborative filtering and cosine similarity (CS) for addressing the sparsity problem of recommender systems. It suggested a recommendation list by considering the content information of movies. Similarly, to solve both sparsity and cold-start problems Ke et al. [ 57 ] proposed a dynamic goods recommendation system based on reinforcement learning. The proposed system was capable of learning from the reduced entropy loss error on real-time applications. Chen et al. [ 58 ] have presented a movie recommender model combining various techniques like user interest with category-level representation, neighbour-assisted representation, user interest with latent representation and item-level representation using Feed-forward Neural Network (FNN).
Comparative chronological review
A comparative chronological review to compare the total contributions on various recommender systems in the past 10 years is given in Fig. 7 .
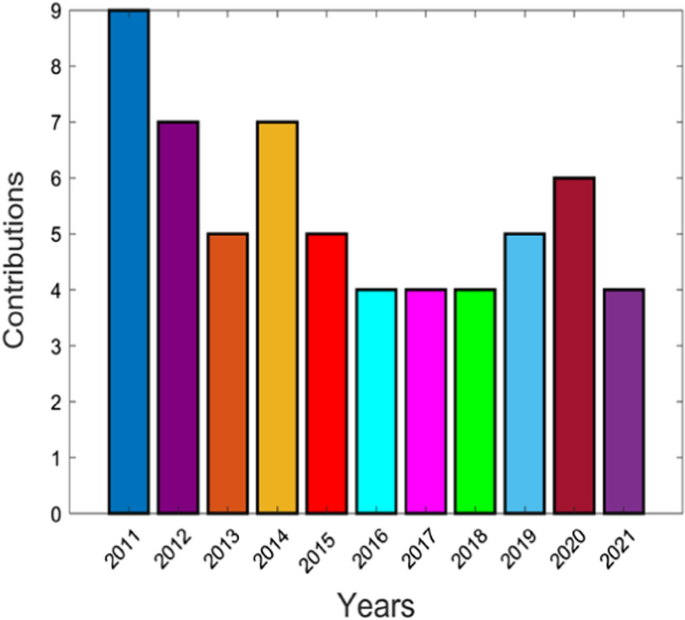
Comparative chronological review of recommender systems under diverse applications
This review puts forward a comparison of the number of research works proposed in the domain of recommender systems from the year 2011 to 2021 using various deep learning and machine learning-based approaches. Research articles are categorized based on the recommender system classification framework as shown in Table 5 . The articles are ordered according to their year of publication. There are two key concepts: Application fields and techniques used. The application fields of recommender systems are divided into six different fields, viz. entertainment, health, tourism, web/e-commerce, education and social media/others.
Algorithmic categorization, simulation platforms and applications considered for various recommender systems
This section analyses different methods like deep learning, machine learning, clustering and meta-heuristic-based-approaches used in the development of recommender systems. The algorithmic categorization of different recommender systems is given in Fig. 8 .
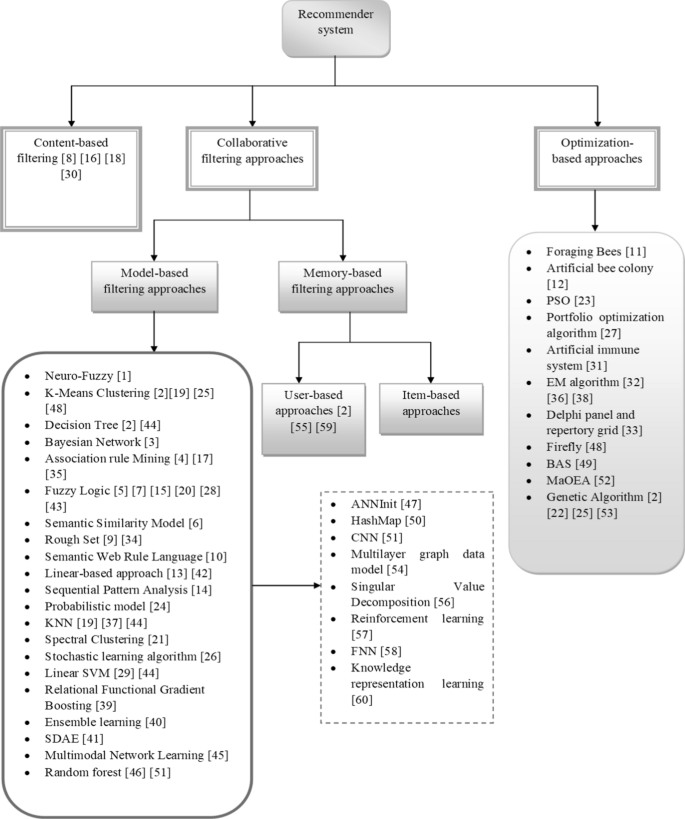
Algorithmic categorization of different recommender systems
Categorization is done based on content-based, collaborative filtering-based, and optimization-based approaches. In [ 8 ], a content-based filtering technique was employed for increasing the ability to trust other agents and for improving the exchange of information by trust degree. In [ 16 ], it was applied to enhance the quality of recommendations using the account attributes of the material. It achieved better performance concerning with F1-score, recall and precision. In [ 18 ], this technique was able to capture the implicit user feedback, increasing the overall accuracy of the proposed model. The content-based filtering in [ 30 ] was able to increase the accuracy and performance of a stock recommender system by using the “trust factor” for making decisions.
Different collaborative filtering approaches are utilized in recent studies, which are categorized as follows:
Model-based techniques
Neuro-Fuzzy [ 1 ] based technique helps in discovering the association between user categories and item relevance. It is also simple to understand. K-Means Clustering [ 2 , 19 , 25 , 48 ] is efficient for large scale datasets. It is simple to implement and gives a fast convergence rate. It also offers automatic recovery from failures. The decision tree [ 2 , 44 ] technique is easy to interpret. It can be used for solving the classic regression and classification problems in recommender systems. Bayesian Network [ 3 ] is a probabilistic technique used to solve classification challenges. It is based on the theory of Bayes theorem and conditional probability. Association Rule Mining (ARM) techniques [ 4 , 17 , 35 ] extract rules for projecting the occurrence of an item by considering the existence of other items in a transaction. This method uses the association rules to create a more suitable representation of data and helps in increasing the model performance and storage efficiency. Fuzzy Logic [ 5 , 7 , 15 , 20 , 28 , 43 ] techniques use a set of flexible rules. It focuses on solving complex real-time problems having an inaccurate spectrum of data. This technique provides scalability and helps in increasing the overall model performance for recommender systems. The semantic similarity [ 6 ] technique is used for describing a topological similarity to define the distance among the concepts and terms through ontologies. It measures the similarity information for increasing the efficiency of recommender systems. Rough set [ 9 , 34 ] techniques use probability distributions for solving the challenges of existing recommender models. Semantic web rule language [ 10 ] can efficiently extract the dataset features and increase the model efficiency. Linear programming-based approaches [ 13 , 42 ] are employed for achieving quality decision making in recommender models. Sequential pattern analysis [ 14 ] is applied to find suitable patterns among data items. This helps in increasing model efficiency. The probabilistic model [ 24 ] is a famous tool to handle uncertainty in risk computations and performance assessment. It offers better decision-making capabilities. K-nearest neighbours (KNN) [ 19 , 37 , 44 ] technique provides faster computation time, simplicity and ease of interpretation. They are good for classification and regression-based problems and offers more accuracy. Spectral clustering [ 21 ] is also called graph clustering or similarity-based clustering, which mainly focuses on reducing the space dimensionality in identifying the dataset items. Stochastic learning algorithm [ 26 ] solves the real-time challenges of recommender systems. Linear SVM [ 29 , 44 ] efficiently solves the high dimensional problems related to recommender systems. It is a memory-efficient method and works well with a large number of samples having relative separation among the classes. This method has been shown to perform well even when new or unfamiliar data is added. Relational Functional Gradient Boosting [ 39 ] technique efficiently works on the relational dependency of data, which is useful for statical relational learning for collaborative-based recommender systems. Ensemble learning [ 40 ] combines the forecast of two or more models and aims to achieve better performance than any of the single contributing models. It also helps in reducing overfitting problems, which are common in recommender systems.
SDAE [ 41 ] is used for learning the non-linear transformations with different filters for finding suitable data. This aids in increasing the performance of recommender models. Multimodal network learning [ 45 ] is efficient for multi-modal data, representing a combined representation of diverse modalities. Random forest [ 46 , 51 ] is a commonly used approach in comparison with other classifiers. It has been shown to increase accuracy when handling big data. This technique is a collection of decision trees to minimize variance through training on diverse data samples. ANNInit [ 47 ] is a type of artificial neural network-based technique that has the capability of self-learning and generating efficient results. It is independent of the data type and can learn data patterns automatically. HashMap [ 50 ] gives faster access to elements owing to the hashing methodology, which decreases the data processing time and increases the performance of the system. CNN [ 51 ] technique can automatically fetch the significant features of a dataset without any supervision. It is a computationally efficient method and provides accurate recommendations. This technique is also simple and fast for implementation. Multilayer graph data model [ 54 ] is efficient for real-time applications and minimizes the access time through mapping the correlation as edges among nodes and provides superior performance. Singular Value Decomposition [ 56 ] can simplify the input data and increase the efficiency of recommendations by eliminating the noise present in data. Reinforcement learning [ 57 ] is efficient for practical scenarios of recommender systems having large data sizes. It is capable of boosting the model performance by increasing the model accuracy even for large scale datasets. FNN [ 58 ] is one of the artificial neural network techniques which can learn non-linear and complex relationships between items. It has demonstrated a good performance increase when employed in different recommender systems. Knowledge representation learning [ 60 ] systems aim to simplify the model development process by increasing the acquisition efficiency, inferential efficiency, inferential adequacy and representation adequacy. User-based approaches [ 2 , 55 , 59 ] specialize in detecting user-related meta-data which is employed to increase the overall model performance. This technique is more suitable for real-time applications where it can capture user feedback and use it to increase the user experience.
Optimization-based techniques
The Foraging Bees [ 11 ] technique enables both functional and combinational optimization for random searching in recommender models. Artificial bee colony [ 12 ] is a swarm-based meta-heuristic technique that provides features like faster convergence rate, the ability to handle the objective with stochastic nature, ease for incorporating with other algorithms, usage of fewer control parameters, strong robustness, high flexibility and simplicity. Particle Swarm Optimization [ 23 ] is a computation optimization technique that offers better computational efficiency, robustness in control parameters, and is easy and simple to implement in recommender systems. Portfolio optimization algorithm [ 27 ] is a subclass of optimization algorithms that find its application in stock investment recommender systems. It works well in real-time and helps in the diversification of the portfolio for maximum profit. The artificial immune system [ 31 ]a is computationally intelligent machine learning technique. This technique can learn new patterns in the data and optimize the overall system parameters. Expectation maximization (EM) [ 32 , 36 , 38 ] is an iterative algorithm that guarantees the likelihood of finding the maximum parameters when the input variables are unknown. Delphi panel and repertory grid [ 33 ] offers efficient decision making by solving the dimensionality problem and data sparsity issues of recommender systems. The Firefly algorithm (FA) [ 48 ] provides fast results and increases recommendation efficiency. It is capable of reducing the number of iterations required to solve specific recommender problems. It also provides both local and global sets of solutions. Beetle Antennae Search (BAS) [ 49 ] offers superior search accuracy and maintains less time complexity that promotes the performance of recommendations. Many-objective evolutionary algorithm (MaOEA) [ 52 ] is applicable for real-time, multi-objective, search-related recommender systems. The introduction of a local search operator increases the convergence rate and gets suitable results. Genetic Algorithm (GA) [ 2 , 22 , 25 , 53 ] based techniques are used to solve the multi-objective optimization problems of recommender systems. They employ probabilistic transition rules and have a simpler operation that provides better recommender performance.
Features and challenges
The features and challenges of the existing recommender models are given in Table 6 .
Simulation platforms
The various simulation platforms used for developing different recommender systems with different applications are given in Fig. 9 .
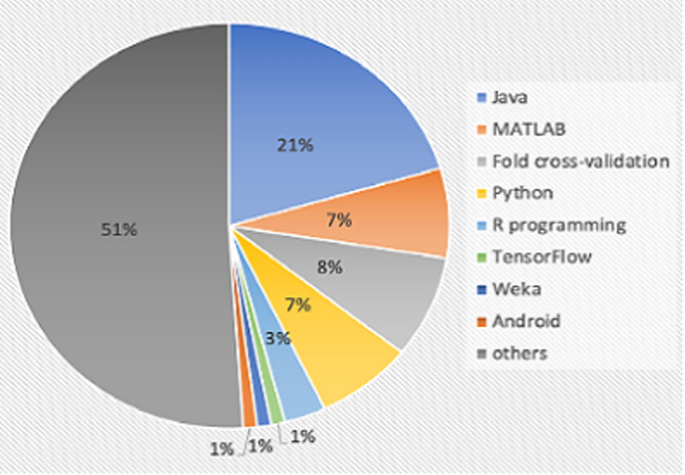
Simulation platforms used for developing different recommender systems
Here, the Java platform is used in 20% of the contributions, MATLAB is implemented in 7% of the contributions, different fold cross-validation are used in 8% of the contributions, 7% of the contributions are utilized by the python platform, 3% of the contributions employ R-programming and 1% of the contributions are developed by Tensorflow, Weka and Android environments respectively. Other simulation platforms like Facebook, web UI (User Interface), real-time environments, etc. are used in 50% of the contributions. Table 7 describes some simulation platforms commonly used for developing recommender systems.
Application focused and dataset description
This section provides an analysis of the different applications focused on a set of recent recommender systems and their dataset details.
Recent recommender systems were analysed and found that 11% of the contributions are focused on the domain of healthcare, 10% of the contributions are on movie recommender systems, 5% of the contributions come from music recommender systems, 6% of the contributions are focused on e-learning recommender systems, 8% of the contributions are used for online product recommender systems, 3% of the contributions are focused on book recommendations and 1% of the contributions are focused on Job and knowledge management recommender systems. 5% of the contributions concentrated on social network recommender systems, 10% of the contributions are focused on tourist and hotels recommender systems, 6% of the contributions are employed for stock recommender systems, and 3% of the contributions contributed for video recommender systems. The remaining 12% of contributions are miscellaneous recommender systems like Twitter, venue-based recommender systems, etc. Similarly, different datasets are gathered for recommender systems based on their application types. A detailed description is provided in Table 8 .
Performance analysis of state-of-art recommender systems
The performance evaluation metrics used for the analysis of different recommender systems is depicted in Table 9 . From the set of research works, 35% of the works use recall measure, 16% of the works employ Mean Absolute Error (MAE), 11% of the works take Root Mean Square Error (RMSE), 41% of the papers consider precision, 30% of the contributions analyse F1-measure, 31% of the works apply accuracy and 6% of the works employ coverage measure to validate the performance of the recommender systems. Moreover, some additional measures are also considered for validating the performance in a few applications.
Research gaps and challenges
In the recent decade, recommender systems have performed well in solving the problem of information overload and has become the more appropriate tool for multiple areas such as psychology, mathematics, computer science, etc. [ 80 ]. However, current recommender systems face a variety of challenges which are stated as follows, and discussed below:
Deployment challenges such as cold start, scalability, sparsity, etc. are already discussed in Sect. 3.
Challenges faced when employing different recommender algorithms for different applications.
Challenges in collecting implicit user data
Challenges in handling real-time user feedback.
Challenges faced in choosing the correct implementation techniques.
Challenges faced in measuring system performance.
Challenges in implementing recommender system for diverse applications.
Numerous recommender algorithms have been proposed on novel emerging dimensions which focus on addressing the existing limitations of recommender systems. A good recommender system must increase the recommendation quality based on user preferences. However, a specific recommender algorithm is not always guaranteed to perform equally for different applications. This encourages the possibility of employing different recommender algorithms for different applications, which brings along a lot of challenges. There is a need for more research to alleviate these challenges. Also, there is a large scope of research in recommender applications that incorporate information from different interactive online sites like Facebook, Twitter, shopping sites, etc. Some other areas for emerging research may be in the fields of knowledge-based recommender systems, methods for seamlessly processing implicit user data and handling real-time user feedback to recommend items in a dynamic environment.
Some of the other research areas like deep learning-based recommender systems, demographic filtering, group recommenders, cross-domain techniques for recommender systems, and dimensionality reduction techniques are also further required to be studied [ 83 ]. Deep learning-based recommender systems have recently gained much popularity. Future research areas in this field can integrate the well-performing deep learning models with new variants of hybrid meta-heuristic approaches.
During this review, it was observed that even though recent recommender systems have demonstrated good performance, there is no single standardized criteria or method which could be used to evaluate the performance of all recommender systems. System performance is generally measured by different evaluation matrices which makes it difficult to compare. The application of recommender systems in real-time applications is growing. User satisfaction and personalization play a very important role in the success of such recommender systems. There is a need for some new evaluation criteria which can evaluate the level of user satisfaction in real-time. New research should focus on capturing real-time user feedback and use the information to change the recommendation process accordingly. This will aid in increasing the quality of recommendations.
Conclusion and future scope
Recommender systems have attracted the attention of researchers and academicians. In this paper, we have identified and prudently reviewed research papers on recommender systems focusing on diverse applications, which were published between 2011 and 2021. This review has gathered diverse details like different application fields, techniques used, simulation tools used, diverse applications focused, performance metrics, datasets used, system features, and challenges of different recommender systems. Further, the research gaps and challenges were put forward to explore the future research perspective on recommender systems. Overall, this paper provides a comprehensive understanding of the trend of recommender systems-related research and to provides researchers with insight and future direction on recommender systems. The results of this study have several practical and significant implications:
Based on the recent-past publication rates, we feel that the research of recommender systems will significantly grow in the future.
A large number of research papers were identified in movie recommendations, whereas health, tourism and education-related recommender systems were identified in very few numbers. This is due to the availability of movie datasets in the public domain. Therefore, it is necessary to develop datasets in other fields also.
There is no standard measure to compute the performance of recommender systems. Among 60 papers, 21 used recall, 10 used MAE, 25 used precision, 18 used F1-measure, 19 used accuracy and only 7 used RMSE to calculate system performance. Very few systems were found to excel in two or more matrices.
Java and Python (with a combined contribution of 27%) are the most common programming languages used to develop recommender systems. This is due to the availability of a large number of standard java and python libraries which aid in the development process.
Recently a large number of hybrid and optimizations techniques are being proposed for recommender systems. The performance of a recommender system can be greatly improved by applying optimization techniques.
There is a large scope of research in using neural networks and deep learning-based methods for developing recommender systems. Systems developed using these methods are found to achieve high-performance accuracy.
This research will provide a guideline for future research in the domain of recommender systems. However, this research has some limitations. Firstly, due to the limited amount of manpower and time, we have only reviewed papers published in journals focusing on computer science, management and medicine. Secondly, we have reviewed only English papers. New research may extend this study to cover other journals and non-English papers. Finally, this review was conducted based on a search on only six descriptors: “Recommender systems”, “Recommendation systems”, “Movie Recommend*”, “Music Recommend*”, “Personalized Recommend*” and “Hybrid Recommend*”. Research papers that did not include these keywords were not considered. Future research can include adding some additional descriptors and keywords for searching. This will allow extending the research to cover more diverse articles on recommender systems.
Availability of data and materials
Not applicable.
Castellano G, Fanelli AM, Torsello MA. NEWER: A system for neuro-fuzzy web recommendation. Appl Soft Comput. 2011;11:793–806.
Article Google Scholar
Crespo RG, Martínez OS, Lovelle JMC, García-Bustelo BCP, Gayo JEL, Pablos PO. Recommendation system based on user interaction data applied to intelligent electronic books. Computers Hum Behavior. 2011;27:1445–9.
Lin FC, Yu HW, Hsu CH, Weng TC. Recommendation system for localized products in vending machines. Expert Syst Appl. 2011;38:9129–38.
Wang SL, Wu CY. Application of context-aware and personalized recommendation to implement an adaptive ubiquitous learning system. Expert Syst Appl. 2011;38:10831–8.
García-Crespo Á, López-Cuadrado JL, Colomo-Palacios R, González-Carrasco I, Ruiz-Mezcua B. Sem-Fit: A semantic based expert system to provide recommendations in the tourism domain. Expert Syst Appl. 2011;38:13310–9.
Dong H, Hussain FK, Chang E. A service concept recommendation system for enhancing the dependability of semantic service matchmakers in the service ecosystem environment. J Netw Comput Appl. 2011;34:619–31.
Li M, Liu L, Li CB. An approach to expert recommendation based on fuzzy linguistic method and fuzzy text classification in knowledge management systems. Expert Syst Appl. 2011;38:8586–96.
Lorenzi F, Bazzan ALC, Abel M, Ricci F. Improving recommendations through an assumption-based multiagent approach: An application in the tourism domain. Expert Syst Appl. 2011;38:14703–14.
Huang Z, Lu X, Duan H. Context-aware recommendation using rough set model and collaborative filtering. Artif Intell Rev. 2011;35:85–99.
Chen RC, Huang YH, Bau CT, Chen SM. A recommendation system based on domain ontology and SWRL for anti-diabetic drugs selection. Expert Syst Appl. 2012;39:3995–4006.
Mohanraj V, Chandrasekaran M, Senthilkumar J, Arumugam S, Suresh Y. Ontology driven bee’s foraging approach based self-adaptive online recommendation system. J Syst Softw. 2012;85:2439–50.
Hsu CC, Chen HC, Huang KK, Huang YM. A personalized auxiliary material recommendation system based on learning style on facebook applying an artificial bee colony algorithm. Comput Math Appl. 2012;64:1506–13.
Gemmell J, Schimoler T, Mobasher B, Burke R. Resource recommendation in social annotation systems: A linear-weighted hybrid approach. J Comput Syst Sci. 2012;78:1160–74.
Article MathSciNet Google Scholar
Choi K, Yoo D, Kim G, Suh Y. A hybrid online-product recommendation system: Combining implicit rating-based collaborative filtering and sequential pattern analysis. Electron Commer Res Appl. 2012;11:309–17.
Garibaldi JM, Zhou SM, Wang XY, John RI, Ellis IO. Incorporation of expert variability into breast cancer treatment recommendation in designing clinical protocol guided fuzzy rule system models. J Biomed Inform. 2012;45:447–59.
Salehi M, Kmalabadi IN. A hybrid attribute–based recommender system for e–learning material recommendation. IERI Procedia. 2012;2:565–70.
Aher SB, Lobo LMRJ. Combination of machine learning algorithms for recommendation of courses in e-learning System based on historical data. Knowl-Based Syst. 2013;51:1–14.
Kardan AA, Ebrahimi M. A novel approach to hybrid recommendation systems based on association rules mining for content recommendation in asynchronous discussion groups. Inf Sci. 2013;219:93–110.
Chang JH, Lai CF, Wang MS, Wu TY. A cloud-based intelligent TV program recommendation system. Comput Electr Eng. 2013;39:2379–99.
Lucas JP, Luz N, Moreno MN, Anacleto R, Figueiredo AA, Martins C. A hybrid recommendation approach for a tourism system. Expert Syst Appl. 2013;40:3532–50.
Niu J, Zhu L, Zhao X, Li H. Affivir: An affect-based Internet video recommendation system. Neurocomputing. 2013;120:422–33.
Liu L, Xu J, Liao SS, Chen H. A real-time personalized route recommendation system for self-drive tourists based on vehicle to vehicle communication. Expert Syst Appl. 2014;41:3409–17.
Bakshi S, Jagadev AK, Dehuri S, Wang GN. Enhancing scalability and accuracy of recommendation systems using unsupervised learning and particle swarm optimization. Appl Soft Comput. 2014;15:21–9.
Kim Y, Shim K. TWILITE: A recommendation system for twitter using a probabilistic model based on latent Dirichlet allocation. Inf Syst. 2014;42:59–77.
Wang Z, Yu X, Feng N, Wang Z. An improved collaborative movie recommendation system using computational intelligence. J Vis Lang Comput. 2014;25:667–75.
Kolomvatsos K, Anagnostopoulos C, Hadjiefthymiades S. An efficient recommendation system based on the optimal stopping theory. Expert Syst Appl. 2014;41:6796–806.
Gottschlich J, Hinz O. A decision support system for stock investment recommendations using collective wisdom. Decis Support Syst. 2014;59:52–62.
Torshizi AD, Zarandi MHF, Torshizi GD, Eghbali K. A hybrid fuzzy-ontology based intelligent system to determine level of severity and treatment recommendation for benign prostatic hyperplasia. Comput Methods Programs Biomed. 2014;113:301–13.
Zahálka J, Rudinac S, Worring M. Interactive multimodal learning for venue recommendation. IEEE Trans Multimedia. 2015;17:2235–44.
Sankar CP, Vidyaraj R, Kumar KS. Trust based stock recommendation system – a social network analysis approach. Procedia Computer Sci. 2015;46:299–305.
Chen MH, Teng CH, Chang PC. Applying artificial immune systems to collaborative filtering for movie recommendation. Adv Eng Inform. 2015;29:830–9.
Wu H, Pei Y, Li B, Kang Z, Liu X, Li H. Item recommendation in collaborative tagging systems via heuristic data fusion. Knowl-Based Syst. 2015;75:124–40.
Yeh DY, Cheng CH. Recommendation system for popular tourist attractions in Taiwan using delphi panel and repertory grid techniques. Tour Manage. 2015;46:164–76.
Liao SH, Chang HK. A rough set-based association rule approach for a recommendation system for online consumers. Inf Process Manage. 2016;52:1142–60.
Li H, Cui J, Shen B, Ma J. An intelligent movie recommendation system through group-level sentiment analysis in microblogs. Neurocomputing. 2016;210:164–73.
Wu H, Yue K, Pei Y, Li B, Zhao Y, Dong F. Collaborative topic regression with social trust ensemble for recommendation in social media systems. Knowl-Based Syst. 2016;97:111–22.
Adeniyi DA, Wei Z, Yongquan Y. Automated web usage data mining and recommendation system using K-Nearest Neighbor (KNN) classification method. Appl Computing Inform. 2016;12:90–108.
Rawat YS, Kankanhalli MS. ClickSmart: A context-aware viewpoint recommendation system for mobile photography. IEEE Trans Circuits Syst Video Technol. 2017;27:149–58.
Yang S, Korayem M, Aljadda K, Grainger T, Natarajan S. Combining content-based and collaborative filtering for job recommendation system: A cost-sensitive Statistical Relational Learning approach. Knowl-Based Syst. 2017;136:37–45.
Lee WP, Chen CT, Huang JY, Liang JY. A smartphone-based activity-aware system for music streaming recommendation. Knowl-Based Syst. 2017;131:70–82.
Wei J, He J, Chen K, Zhou Y, Tang Z. Collaborative filtering and deep learning based recommendation system for cold start items. Expert Syst Appl. 2017;69:29–39.
Li C, Wang Z, Cao S, He L. WLRRS: A new recommendation system based on weighted linear regression models. Comput Electr Eng. 2018;66:40–7.
Mezei J, Nikou S. Fuzzy optimization to improve mobile health and wellness recommendation systems. Knowl-Based Syst. 2018;142:108–16.
Ayata D, Yaslan Y, Kamasak ME. Emotion based music recommendation system using wearable physiological sensors. IEEE Trans Consum Electron. 2018;64:196–203.
Zhao Z, Yang Q, Lu H, Weninger T. Social-aware movie recommendation via multimodal network learning. IEEE Trans Multimedia. 2018;20:430–40.
Hammou BA, Lahcen AA, Mouline S. An effective distributed predictive model with matrix factorization and random forest for big data recommendation systems. Expert Syst Appl. 2019;137:253–65.
Zhao J, Geng X, Zhou J, Sun Q, Xiao Y, Zhang Z, Fu Z. Attribute mapping and autoencoder neural network based matrix factorization initialization for recommendation systems. Knowl-Based Syst. 2019;166:132–9.
Bhaskaran S, Santhi B. An efficient personalized trust based hybrid recommendation (TBHR) strategy for e-learning system in cloud computing. Clust Comput. 2019;22:1137–49.
Han Y, Han Z, Wu J, Yu Y, Gao S, Hua D, Yang A. Artificial intelligence recommendation system of cancer rehabilitation scheme based on IoT technology. IEEE Access. 2020;8:44924–35.
Kang S, Jeong C, Chung K. Tree-based real-time advertisement recommendation system in online broadcasting. IEEE Access. 2020;8:192693–702.
Ullah F, Zhang B, Khan RU. Image-based service recommendation system: A JPEG-coefficient RFs approach. IEEE Access. 2020;8:3308–18.
Cai X, Hu Z, Zhao P, Zhang W, Chen J. A hybrid recommendation system with many-objective evolutionary algorithm. Expert Syst Appl. 2020. https://doi.org/10.1016/j.eswa.2020.113648 .
Esteban A, Zafra A, Romero C. Helping university students to choose elective courses by using a hybrid multi-criteria recommendation system with genetic optimization. Knowledge-Based Syst. 2020;194:105385.
Mondal S, Basu A, Mukherjee N. Building a trust-based doctor recommendation system on top of multilayer graph database. J Biomed Inform. 2020;110:103549.
Dhelim S, Ning H, Aung N, Huang R, Ma J. Personality-aware product recommendation system based on user interests mining and metapath discovery. IEEE Trans Comput Soc Syst. 2021;8:86–98.
Bhalse N, Thakur R. Algorithm for movie recommendation system using collaborative filtering. Materials Today: Proceedings. 2021. https://doi.org/10.1016/j.matpr.2021.01.235 .
Ke G, Du HL, Chen YC. Cross-platform dynamic goods recommendation system based on reinforcement learning and social networks. Appl Soft Computing. 2021;104:107213.
Chen X, Liu D, Xiong Z, Zha ZJ. Learning and fusing multiple user interest representations for micro-video and movie recommendations. IEEE Trans Multimedia. 2021;23:484–96.
Afolabi AO, Toivanen P. Integration of recommendation systems into connected health for effective management of chronic diseases. IEEE Access. 2019;7:49201–11.
He M, Wang B, Du X. HI2Rec: Exploring knowledge in heterogeneous information for movie recommendation. IEEE Access. 2019;7:30276–84.
Bobadilla J, Serradilla F, Hernando A. Collaborative filtering adapted to recommender systems of e-learning. Knowl-Based Syst. 2009;22:261–5.
Russell S, Yoon V. Applications of wavelet data reduction in a recommender system. Expert Syst Appl. 2008;34:2316–25.
Campos LM, Fernández-Luna JM, Huete JF. A collaborative recommender system based on probabilistic inference from fuzzy observations. Fuzzy Sets Syst. 2008;159:1554–76.
Funk M, Rozinat A, Karapanos E, Medeiros AKA, Koca A. In situ evaluation of recommender systems: Framework and instrumentation. Int J Hum Comput Stud. 2010;68:525–47.
Porcel C, Moreno JM, Herrera-Viedma E. A multi-disciplinar recommender system to advice research resources in University Digital Libraries. Expert Syst Appl. 2009;36:12520–8.
Bobadilla J, Serradilla F, Bernal J. A new collaborative filtering metric that improves the behavior of recommender systems. Knowl-Based Syst. 2010;23:520–8.
Ochi P, Rao S, Takayama L, Nass C. Predictors of user perceptions of web recommender systems: How the basis for generating experience and search product recommendations affects user responses. Int J Hum Comput Stud. 2010;68:472–82.
Olmo FH, Gaudioso E. Evaluation of recommender systems: A new approach. Expert Syst Appl. 2008;35:790–804.
Zhen L, Huang GQ, Jiang Z. An inner-enterprise knowledge recommender system. Expert Syst Appl. 2010;37:1703–12.
Göksedef M, Gündüz-Öğüdücü S. Combination of web page recommender systems. Expert Syst Appl. 2010;37(4):2911–22.
Shao B, Wang D, Li T, Ogihara M. Music recommendation based on acoustic features and user access patterns. IEEE Trans Audio Speech Lang Process. 2009;17:1602–11.
Shin C, Woo W. Socially aware tv program recommender for multiple viewers. IEEE Trans Consum Electron. 2009;55:927–32.
Lopez-Carmona MA, Marsa-Maestre I, Perez JRV, Alcazar BA. Anegsys: An automated negotiation based recommender system for local e-marketplaces. IEEE Lat Am Trans. 2007;5:409–16.
Yap G, Tan A, Pang H. Discovering and exploiting causal dependencies for robust mobile context-aware recommenders. IEEE Trans Knowl Data Eng. 2007;19:977–92.
Meo PD, Quattrone G, Terracina G, Ursino D. An XML-based multiagent system for supporting online recruitment services. IEEE Trans Syst Man Cybern. 2007;37:464–80.
Khusro S, Ali Z, Ullah I. Recommender systems: Issues, challenges, and research opportunities. Inform Sci Appl. 2016. https://doi.org/10.1007/978-981-10-0557-2_112 .
Blanco-Fernandez Y, Pazos-Arias JJ, Gil-Solla A, Ramos-Cabrer M, Lopez-Nores M. Providing entertainment by content-based filtering and semantic reasoning in intelligent recommender systems. IEEE Trans Consum Electron. 2008;54:727–35.
Isinkaye FO, Folajimi YO, Ojokoh BA. Recommendation systems: Principles, methods and evaluation. Egyptian Inform J. 2015;16:261–73.
Yoshii K, Goto M, Komatani K, Ogata T, Okuno HG. An efficient hybrid music recommender system using an incrementally trainable probabilistic generative model. IEEE Trans Audio Speech Lang Process. 2008;16:435–47.
Wei YZ, Moreau L, Jennings NR. Learning users’ interests by quality classification in market-based recommender systems. IEEE Trans Knowl Data Eng. 2005;17:1678–88.
Bjelica M. Towards TV recommender system: experiments with user modeling. IEEE Trans Consum Electron. 2010;56:1763–9.
Setten MV, Veenstra M, Nijholt A, Dijk BV. Goal-based structuring in recommender systems. Interact Comput. 2006;18:432–56.
Adomavicius G, Tuzhilin A. Toward the next generation of recommender systems: a survey of the state-of-the-art and possible extensions. IEEE Trans Knowl Data Eng. 2005;17:734–49.
Symeonidis P, Nanopoulos A, Manolopoulos Y. Providing justifications in recommender systems. IEEE Transactions on Systems, Man, and Cybernetics - Part A: Systems and Humans. 2009;38:1262–72.
Zhan J, Hsieh C, Wang I, Hsu T, Liau C, Wang D. Privacy preserving collaborative recommender systems. IEEE Trans Syst Man Cybernet. 2010;40:472–6.
Burke R. Hybrid recommender systems: survey and experiments. User Model User-Adap Inter. 2002;12:331–70.
Article MATH Google Scholar
Gunes I, Kaleli C, Bilge A, Polat H. Shilling attacks against recommender systems: a comprehensive survey. Artif Intell Rev. 2012;42:767–99.
Park DH, Kim HK, Choi IY, Kim JK. A literature review and classification of recommender systems research. Expert Syst Appl. 2012;39:10059–72.
Download references
Acknowledgements
We thank our colleagues from Assam Down Town University who provided insight and expertise that greatly assisted this research, although they may not agree with all the interpretations and conclusions of this paper.
No funding was received to assist with the preparation of this manuscript.
Author information
Authors and affiliations.
Department of Computer Science & Engineering, Assam Down Town University, Panikhaiti, Guwahati, 781026, Assam, India
Deepjyoti Roy & Mala Dutta
You can also search for this author in PubMed Google Scholar
Contributions
DR carried out the review study and analysis of the existing algorithms in the literature. MD has been involved in drafting the manuscript or revising it critically for important intellectual content. Both authors read and approved the final manuscript.
Corresponding author
Correspondence to Deepjyoti Roy .
Ethics declarations
Ethics approval and consent to participate, consent for publication, competing interests.
On behalf of all authors, the corresponding author states that there is no conflict of interest.
Additional information
Publisher's note.
Springer Nature remains neutral with regard to jurisdictional claims in published maps and institutional affiliations.
Rights and permissions
Open Access This article is licensed under a Creative Commons Attribution 4.0 International License, which permits use, sharing, adaptation, distribution and reproduction in any medium or format, as long as you give appropriate credit to the original author(s) and the source, provide a link to the Creative Commons licence, and indicate if changes were made. The images or other third party material in this article are included in the article's Creative Commons licence, unless indicated otherwise in a credit line to the material. If material is not included in the article's Creative Commons licence and your intended use is not permitted by statutory regulation or exceeds the permitted use, you will need to obtain permission directly from the copyright holder. To view a copy of this licence, visit http://creativecommons.org/licenses/by/4.0/ .
Reprints and permissions
About this article
Cite this article.
Roy, D., Dutta, M. A systematic review and research perspective on recommender systems. J Big Data 9 , 59 (2022). https://doi.org/10.1186/s40537-022-00592-5
Download citation
Received : 04 October 2021
Accepted : 28 March 2022
Published : 03 May 2022
DOI : https://doi.org/10.1186/s40537-022-00592-5
Share this article
Anyone you share the following link with will be able to read this content:
Sorry, a shareable link is not currently available for this article.
Provided by the Springer Nature SharedIt content-sharing initiative
- Recommender system
- Machine learning
- Content-based filtering
- Collaborative filtering
- Deep learning
Deep Learning for Recommender Systems: A Netflix Case Study
- Harald Steck Netflix
- Linas Baltrunas Netflix
- Ehtsham Elahi Netflix
- Dawen Liang Netflix
- Yves Raimond Netflix
- Justin Basilico Netflix
Deep learning has profoundly impacted many areas of machine learning. However, it took a while for its impact to be felt in the field of recommender systems. In this article, we outline some of the challenges encountered and lessons learned in using deep learning for recommender systems at Netflix. We first provide an overview of the various recommendation tasks on the Netflix service. We found that different model architectures excel at different tasks. Even though many deep-learning models can be understood as extensions of existing (simple) recommendation algorithms, we initially did not observe significant improvements in performance over well-tuned non-deep-learning approaches. Only when we added numerous features of heterogeneous types to the input data, deep-learning models did start to shine in our setting. We also observed that deep-learning methods can exacerbate the problem of offline–online metric (mis-)alignment. After addressing these challenges, deep learning has ultimately resulted in large improvements to our recommendations as measured by both offline and online metrics. On the practical side, integrating deep-learning toolboxes in our system has made it faster and easier to implement and experiment with both deep-learning and non-deep-learning approaches for various recommendation tasks. We conclude this article by summarizing our take-aways that may generalize to other applications beyond Netflix.
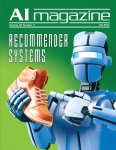
How to Cite
- Endnote/Zotero/Mendeley (RIS)
- The author(s) warrants that they are the sole author and owner of the copyright in the above article/paper, except for those portions shown to be in quotations; that the article/paper is original throughout; and that the undersigned right to make the grants set forth above is complete and unencumbered.
- The author(s) agree that if anyone brings any claim or action alleging facts that, if true, constitute a breach of any of the foregoing warranties, the author(s) will hold harmless and indemnify AAAI, their grantees, their licensees, and their distributors against any liability, whether under judgment, decree, or compromise, and any legal fees and expenses arising out of that claim or actions, and the undersigned will cooperate fully in any defense AAAI may make to such claim or action. Moreover, the undersigned agrees to cooperate in any claim or other action seeking to protect or enforce any right the undersigned has granted to AAAI in the article/paper. If any such claim or action fails because of facts that constitute a breach of any of the foregoing warranties, the undersigned agrees to reimburse whomever brings such claim or action for expenses and attorneys’ fees incurred therein.
- Author(s) retain all proprietary rights other than copyright (such as patent rights).
- Author(s) may make personal reuse of all or portions of the above article/paper in other works of their own authorship.
- Author(s) may reproduce, or have reproduced, their article/paper for the author’s personal use, or for company use provided that original work is property cited, and that the copies are not used in a way that implies AAAI endorsement of a product or service of an employer, and that the copies per se are not offered for sale. The foregoing right shall not permit the posting of the article/paper in electronic or digital form on any computer network, except by the author or the author’s employer, and then only on the author’s or the employer’s own web page or ftp site. Such web page or ftp site, in addition to the aforementioned requirements of this Paragraph, must provide an electronic reference or link back to the AAAI electronic server, and shall not post other AAAI copyrighted materials not of the author’s or the employer’s creation (including tables of contents with links to other papers) without AAAI’s written permission.
- Author(s) may make limited distribution of all or portions of their article/paper prior to publication.
- In the case of work performed under U.S. Government contract, AAAI grants the U.S. Government royalty-free permission to reproduce all or portions of the above article/paper, and to authorize others to do so, for U.S. Government purposes.
- In the event the above article/paper is not accepted and published by AAAI, or is withdrawn by the author(s) before acceptance by AAAI, this agreement becomes null and void.
Information
- For Readers
- For Authors
Developed By
Part of the PKP Publishing Services Network
Copyright © 2021, Association for the Advancement of Artificial Intelligence. All rights reserved.

- Search Menu
- Volume 2024, 2024 (In Progress)
- Volume 2023, 2023
- Author Guidelines
- Submission Site
- Open Access
- About Database
- About the International Society for Biocuration
- Editorial Board
- Advertising and Corporate Services
- Journals Career Network
- Self-Archiving Policy
- Journals on Oxford Academic
- Books on Oxford Academic
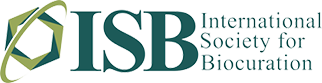
Article Contents
Introduction, methods and evaluation, conclusion and future work, acknowledgement, conflicts of interest..
- < Previous
A content-based dataset recommendation system for researchers—a case study on Gene Expression Omnibus (GEO) repository

- Article contents
- Figures & tables
- Supplementary Data
Braja Gopal Patra, Kirk Roberts, Hulin Wu, A content-based dataset recommendation system for researchers—a case study on Gene Expression Omnibus (GEO) repository, Database , Volume 2020, 2020, baaa064, https://doi.org/10.1093/database/baaa064
- Permissions Icon Permissions
It is a growing trend among researchers to make their data publicly available for experimental reproducibility and data reusability. Sharing data with fellow researchers helps in increasing the visibility of the work. On the other hand, there are researchers who are inhibited by the lack of data resources. To overcome this challenge, many repositories and knowledge bases have been established to date to ease data sharing. Further, in the past two decades, there has been an exponential increase in the number of datasets added to these dataset repositories. However, most of these repositories are domain-specific, and none of them can recommend datasets to researchers/users. Naturally, it is challenging for a researcher to keep track of all the relevant repositories for potential use. Thus, a dataset recommender system that recommends datasets to a researcher based on previous publications can enhance their productivity and expedite further research. This work adopts an information retrieval (IR) paradigm for dataset recommendation. We hypothesize that two fundamental differences exist between dataset recommendation and PubMed-style biomedical IR beyond the corpus. First, instead of keywords, the query is the researcher, embodied by his or her publications. Second, to filter the relevant datasets from non-relevant ones, researchers are better represented by a set of interests, as opposed to the entire body of their research. This second approach is implemented using a non-parametric clustering technique. These clusters are used to recommend datasets for each researcher using the cosine similarity between the vector representations of publication clusters and datasets. The maximum normalized discounted cumulative gain at 10 (NDCG@10), precision at 10 (p@10) partial and p@10 strict of 0.89, 0.78 and 0.61, respectively, were obtained using the proposed method after manual evaluation by five researchers. As per the best of our knowledge, this is the first study of its kind on content-based dataset recommendation. We hope that this system will further promote data sharing, offset the researchers’ workload in identifying the right dataset and increase the reusability of biomedical datasets.
Database URL : http://genestudy.org/recommends/#/
In the Big Data era, extensive amounts of data have been generated for scientific discoveries. However, storing, accessing, analyzing and sharing a vast amount of data are becoming major bottlenecks for scientific research. Furthermore, making a large number of public scientific data findable, accessible, interoperable and reusable is a challenging task.
The research community has devoted substantial effort to enable data sharing. Promoting existing datasets for reuse is a major initiative that gained momentum in the past decade ( 1 ). Many repositories and knowledge bases have been established for specific types of data and domains. Gene Expression Omnibus (GEO) (https://www.ncbi.nlm.nih.gov/geo/), UKBioBank (https://www.ukbiobank.ac.uk/), ImmPort (https://www.immport.org/shared/home) and TCGA (https://portal.gdc.cancer.gov/) are some examples of repositories for biomedical datasets. DATA.GOV archives the U.S. Government’s open data related to agriculture, climate, education, etc. for research use. However, a researcher looking for previous datasets on a topic still has to painstakingly visit all the individual repositories to find relevant datasets. This is a tedious and time-consuming process.
An initiative was taken by the developers of DataMed (https://datamed.org) to solve the aforementioned issues for the biomedical community by combining biomedical repositories together and enhancing the query searching based on advanced natural language processing (NLP) techniques ( 1 , 2 ). DataMed indexes provides the functionality to search diverse categories of biomedical datasets ( 1 ). The research focus of this last work was retrieving datasets using a focused query. In addition to that biomedical and healthCAre Data Discovery Index Ecosystem (bioCADDIE) dataset retrieval challenge was organized in 2016 to evaluate the effectiveness of information retrieval (IR) techniques in identifying relevant biomedical datasets in DataMed ( 3 ). Among the teams participated in this shared task, use of probabilistic or machine learning based IR ( 4 ), medical subject headings (MeSH) term based query expansion ( 5 ), word embeddings and identifying named entity ( 6 ), and re-ranking ( 7 ) for searching datasets using a query were the prevalent approaches. Similarly, a specialized search engine named Omicseq was developed for retrieving omics data ( 8 ).
Google Dataset Search (https://toolbox.google.com/datasetsearch) provides the facility to search datasets on the web, similar to DataMed. While DataMed indexes only biomedical domain data, indexing in Google Dataset Search covers data across several domains. Datasets are created and added to repositories frequently, which makes it difficult for a researcher to know and keep track of all datasets. Further, search engines such as DataMed or Google Dataset Search are helpful when the user knows what type of dataset to search for, but determining the user intent in web searches is a difficult problem due to the sparse data available concerning the searcher ( 9 ). To overcome the aforementioned problems and make dataset search more user-friendly, a dataset recommendation system based on a researcher’s profile is proposed here. The publications of researchers indicate their academic interest, and this information can be used to recommend datasets. Recommending a dataset to an appropriate researcher is a new field of research. There are many datasets available that may be useful to certain researchers for further exploration, and this important aspect of dataset recommendation has not been explored earlier.
Recommendation systems, or recommenders, are an information filtering system that deploys data mining and analytics of users’ behaviors, including preferences and activities, for predictions of users’ interests on information, products or services. Research publications in recommendation systems can be broadly grouped as content-based or collaborative filtering recommendation systems ( 10 ). This article describes the development of a recommendation system for scholarly use. In general, developing a scholarly recommendation system is both challenging and unique because semantic information plays an important role in this context, as inputs such as title, abstract and keywords need to be considered ( 11 ). The usefulness of similar research article recommendation systems has been established by the acceptance of applications such as Google Scholar (https://scholar.google.com/), Academia.edu (https://www.academia.edu/), ResearchGate (https://www.researchgate.net/), Semantic Scholar (https://www.semanticscholar.org/) and PubMed (https://www.ncbi.nlm.nih.gov/pubmed/) by the research community.
Dataset recommendation is a challenging task due to the following reasons. First, while standardized formats for dataset metadata exist ( 12 ), no such standard has achieved universal adoption, and researchers use their own convention to describe their datasets. Further, many datasets do not have proper metadata, which makes the prepared dataset difficult to reuse/recommend. Second, there are many dataset repositories with the same dataset in different formats, making recommendation a challenging task. Additionally, the dataset recommendation system should be scalable to the increasing number of online datasets. We cast the problem of recommending datasets to researchers as a ranking problem of datasets matched against the researcher’s individual publication(s). The recommendation system can be viewed as an IR system where the most similar datasets can be retrieved for a researcher using his/her publications.
Data linking or identifying/clustering similar datasets have received relatively less attention in research on recommendation systems. Previous work on this topic includes ( 13–15 ). Reference ( 13 ) defined dataset recommendation as to the problem of computing a rank score for each of a set of target datasets ( D T ) so that the rank score indicates the relatedness of D T to a given source dataset ( D S ). The rank scores provide information on the likelihood of a D T to contain linking candidates for D S . Reference ( 15 ) proposed a dataset recommendation system by first creating similarity-based dataset networks, and then recommending connected datasets to users for each dataset searched. Despite the promising result this approach suffers from the cold start problem. Here cold start problem refers to the user’s initial dataset selection, where the user has no idea what dataset to select/search. If a user chooses a wrong dataset initially, then the system will always recommend wrong datasets to the user.
Some experiments were performed to identify datasets shared in the biomedical literature ( 16–18 ). Reference ( 17 ) identified data shared in biomedical literature articles using regular expression patterns and machine learning algorithms. Reference ( 16 ) identified datasets in social sciences papers using a semi-automatic method. The last system reportedly performed well (F-measure of 0.83) in finding datasets in the da|ra dataset registry. Different deep learning methods were used to extract the dataset mentions in publication and detect mention text fragment to a particular dataset in the knowledge base ( 18 ). Further, a content-based recommendation system was developed for recommending literature for datasets in ( 11 ), which was the first step toward developing a literature recommendation tool by recommending relevant literature for datasets.
This article proposes a dataset recommender that recommends datasets to researchers based on their publications. We collected dataset metadata (title and summary) from GEO and researcher’s publications (title, abstract and year of publication) from PubMed using name and curriculum vitae (CV) for developing a dataset recommendation system. A vector space model (VSM) is used to compare publications and datasets. We propose two novel ideas:
A method for representing researchers with multiple vectors reflecting each researcher’s diverse interests.
A system for recommending datasets to researchers based on their research vectors.
For the datasets, we focus on GEO (https://www.ncbi.nlm.nih.gov/geo/). GEO is a public repository for high-throughput microarray and next-generation sequence functional genomics data. It was found that an average of 21 datasets was added daily in the last 6 years (i.e. 2014–19). This gives a glimpse of the increasing number of datasets being made available online, considering that there are many other online data repositories as well. Many of these datasets were collected at significant expense, and most of these datasets were used only once. We believe that reusability of these datasets can be improved by recommending these to appropriate researchers.
Efforts on restructuring GEO have been performed by curating available metadata. In reference ( 19 ), the authors identified the important keywords present in the datasets descriptions and searched other similar datasets. Another task on restructuring the GEO database, ReGEO (http://regeo.org/) was developed by ( 20 ), who identified important metadata such as time points and cell lines for datasets using automated NLP techniques.
We developed this dataset recommendation system for researchers as a part of the dataset reusability platform (GETc Research Platform(http://genestudy.org/)) for GEO developed at the University Texas Health Science Center at Houston. This website recommends datasets to users using their publications.
The rest of the article is organized in the following manner. Section 2 provides an overview of GEO datasets and researcher publications. Methods used for developing the recommendation system and evaluation techniques used in this experiment are described in Section 3 . Section 4 describes results. Section 5 provides a discussion. Finally, conclusion and future directions are discussed in Section 6 .
The proposed dataset recommendation system requires both dataset metadata and the user profile for which datasets will be recommended. We collected metadata of datasets from the GEO repository, and researcher publications from PubMed using their names and CVs. The data collection methods and summaries of data are discussed next.
GEO Datasets
GEO is one of the most popular public repositories for functional genomics data. As of December 18, 2019, there were 122 222 series of datasets available in GEO. Histograms of datasets submitted to GEO per day and per year as presented in Figure 1 showed an increasing trend of submitting datasets to GEO, which justified our selection of this repository for developing the recommendation system.

Histogram of datasets submitted to GEO based on datasets collected on December 18, 2019

Overview of dataset indexing pipeline
Statistics of datasets collected from GEO
For the present experiment, metadata such as title, summary, submission date and name of dataset creator(s) were collected from GEO and indexed in a database, as shown in Figure 2 . We also collected the PMIDs of articles associated with each dataset. However, many datasets did not have articles associated with them. The detailed information of collected datasets is presented in Table 1 . Out of a total of 122 222 GEO datasets, 89 533 had 92 884 associated articles, out of which 61 228 were unique. The maximum number of articles associated with the datasets (‘GSE15907’ and ‘GSE31312’) was 10. These articles were used to remove the publications that were not related to GEO. Further, we used the GEO-related publications for building word embeddings to be used for subsequent text normalization as outlined in Section 3 .
Researcher publications
A researcher’s academic interest can be extracted from publications, grants, talks, seminars and much more. All this information is typically available in the CV, but it is presented in the form of titles/short texts. Here, short texts imply limited information. Further, lack of standardization in CV formats poses challenges to parse the CVs. In this work, an alternative approach was undertaken, which is outlined next.
Title and year of the researcher’s publications were present in the CV. However, we required title, abstract and year of publication for our experiment. A researcher’s list of publications (titles and abstracts) are easier to get from web sources such as Google Scholar, PubMed, Semantic Scholar and others. Unfortunately, the full texts of most scientific articles are not publicly available. Thus, for the present experiment, we used only the title and abstract of publications in identifying the researcher’s areas of research.

Overview of researcher’s publication extraction system to remove the author disambiguation
Given a researcher, we searched the researcher’s name in PubMed using Entrez API (https://www.ncbi.nlm.nih.gov/books/NBK25 501/) and collected all the publications. Multiple researchers with exact same name might exist, thus, querying the name in PubMed might sometime result in publications from other researchers as well. This is a typical challenge of author disambiguation. However, there are a few attempts that have been undertaken to resolve the issue of author disambiguation, and one of them is ORCID (https://orcid.org). A researcher needs to provide ORCID id to access his/her ORCID details. However, to the best of our knowledge, many researchers in the biomedical domain did not have an associated ORCID account. Thus we used a simple method to disambiguate the authors by using their CVs. Initially, the recommendation system prompts a researcher to provide his/her name and a CV (or list of publications). Next, we collected the publications (titles, names, MeSH terms and year of publication) for a researcher from PubMed by searching his/her name. For removing the publications of other authors with the same name, titles of all collected publications from PubMed were matched against the titles present in the CV. In the case of a match, publications were kept for further processing. An overview of the technique used for the researcher’s publication collection is provided in Figure 3 .
One of the limitations of the above publication collection method is that the publications could not be collected if they were not listed in PubMed. Further, the datasets used in the present experiments were from the biomedical domain, and the publications not listed in PubMed were less pertinent to biomedical datasets. For example, someone’s biomedical interests (in PubMed) may be more reliable markers for biomedical datasets than a theoretical computer science or statistics paper. Another downside is if the researcher’s CV may not be fully up-to-date.
This section describes how the two main objects of interest (datasets and publications of researchers) were embedded in a vector space and then how these vectors were compared in order to make recommendations. First, both datasets and papers were treated as text objects: the text of a dataset includes its title and summary, while the text of a paper includes its title and abstract. Pre-processing was performed on both a researcher’s publications and datasets by removing the low-value stopwords, links, punctuation and junk words. Further, the nltk WordNet lemmatizer (https://www.nltk.org/_modules/nltk/stem/wordnet.html) was used to get the root forms of the words. Next, we describe the methods used for converting datasets and researchers into vectors.
Dataset vector generation
VSMs can be built from text in a variety of ways, each of which has its distinct advantages and thus merit experimentation. For the present experiment, we used TF-IDF because it achieved better results for related literature recommendation for datasets in ( 11 ).
TF-IDF : For vocabulary W , each unique word w ∈ W is assigned a score proportional to its frequency in the text (term frequency, TF) and its inverse frequency in the full collection (inverse document frequency, IDF). We tuned parameters such as minimum document frequency (min-df) and maximum n-gram size. For the present study, we kept maximum n-gram size = 2 (i.e. unigrams and bigrams) as including the higher n-gram increases the sparsity as well as computational complexity.
We converted each dataset into a vector using TF-IDF. For each dataset, the title and summary were preprocessed and normalized and then converted into a single vector. Finally, each publication vector (or publication cluster vector) is compared with dataset vectors to generate the recommendation score. Different methods for representing a researcher’s papers as vectors are discussed next.
Researcher vector generation
Baseline method.
For the baseline method, we combined multiple text-derived paper vectors into a single researcher vector ( v r ) in the same vector space using Equation ( 1 ):
where P r is the set of papers of a researcher r ; N r is the total number of papers of that researcher, and it acts as a normalization term; v p is the vector for a single paper p using TF-IDF; λ p is a recency penalty to favor more recent papers (thus better reflecting the researcher’s current interest).
It is evident that a researcher will be interested in datasets recommended for his/her current work rather than the work performed a few years back. Thus, we penalized each of the paper vectors from a different year, as stated in Equation ( 2 ):
where t is the difference between the current year and year of publication. k is the decaying function to decrease the rate proportional to its current value, and for the present study, we kept k =0.05.
. Multi-interest dataset recommendation (MIDR)
The baseline method for creating a researcher vector may be helpful for new researchers without many publications, whereas an established researcher may have multiple areas of expertise with multiple papers in each. Also, if the number of papers is imbalanced in multiple areas, then the above baseline method may not work. With a highly imbalanced set of publications this would obviously bias dataset recommendation to the dominant interest. For a more balanced set of interests that are highly dispersed, this mixture would result in the ‘centroid’ of these interests, which could be quite distinct from the individual interests. Both these cases are undesirable. The centroid of a researcher’s interests may not be of much interest to them (e.g. a researcher interested in mouse genomics and HIV vaccines may not be interested in mouse vaccines ).
For example, initial experiments were performed on Researcher 1 (mentioned later in Section 4), and it was observed that the datasets recommended for a researcher were biased toward a single research area with the largest number of publications. For example, Researcher 1 has a dominant number of publications on HIV and the baseline system recommends only HIV datasets, even if Researcher 1 has multiple research areas.
A critical limitation of the above baseline approach is that researchers can have multiple areas of expertise. We can easily build multiple vectors, each corresponding to a different expertise if we know how to properly group/cluster a researcher’s papers according to expertise or topic. However, parametric methods such as k-means clustering and latent Dirichlet allocation require specifying a priori how many clusters/topics to utilize. Generalizing the number of clusters is not possible due to a varying number of publications of researchers. Instead, our insight is that the more publications a researcher has, the more interests or areas of expertise he/she likely has as well, but this should be modeled as a ‘soft’ constraint rather than a ‘hard’ constraint. We propose to employ the non-parametric Dirichlet Process Mixture Model (DPMM) ( 21 ) to cluster papers into several groups of expertise.

High level architecture of proposed dataset recommendation
DPMM : We employed a Gibbs Sampling-based Dirichlet Process Mixture Modeling for text clustering. DPMM offers the following advantages over its traditional counterparts. First, the number of text clusters need not be specified; second, it is relatively scalable and third, it is robust to outliers ( 22 ). The technique employs a collapsed Gibbs Sampling mechanism for Dirichlet process models wherein the clusters are added or removed based on the probability of a cluster associating with a given document. The scalability of the technique stems from the fact that word frequencies are used for text clustering. This reduces the computational burden significantly, considering the large number of samples associated with text processing problems. Further, the optimal number of clusters is likely to be chosen, as clusters with low association probability with documents are eliminated, and new clusters are created for documents that do not belong to selected clusters with high probability. For example, if a cluster c 1 contains five documents, each with low association probability, then the cluster c 1 is eliminated, and new clusters are initialized. In DPMM, the decision to create a new cluster is based on the number of papers to be clustered and the similarity of a given paper to previously clustered papers. Thus, researchers with many papers but few interests can still result in fewer clusters than a researcher with fewer papers but more interests. For example, our evaluation includes two researchers, one with 53 papers and one with 32; however, the DPMM resulted in five and six clusters, respectively. After clustering, we created a pseudo-researcher for each cluster using Equation ( 1 ), though one that can be tied back to the original researcher. The recommendation system uses these pseudo-researchers in its similarity calculations along the same lines as described above. Further, the α parameter was tuned to control the number of clusters ( 22 ). We describe tuning of the α parameter in Section 3.4.
Text normalization : Text normalization plays an important role in improving the performance of any NLP system. We also implemented text normalization techniques to improve the efficiency of the proposed clustering algorithm. We normalized similar words by grouping them together and replacing them with the most frequent words in the same word group. For example, HIV, HIV-1, HIV/AIDS and AIDS were replaced with the most frequent word HIV . For identifying similar words, we trained a word2vec model on the articles from PubMed using Gensim (https://radimrehurek.com/gensim/). The datasets are related to gene expressions, while the articles collected from PubMed contain a variety of topics related to biomedicine and life sciences which may not be suitable for building a word embedding in the current study (since some of these articles are highly unrelated to the type of information in GEO). The articles before 1998 were removed as the research on micro-array data started during that year ( 23 ). The publications related to GEO are filtered using the MeSH terms. We also developed a MeSH term classification system for those publications without MeSH terms. More details on GEO related publications filtering can be found in ( 11 ).
The similar words were identified using the most_similar function of word2vec . We only considered the top five similar words for each word using most_similar function. The normalized text was used for clustering. It was observed from the initial experiments that the text normalization improved clustering and resulted in the reduced number of clusters using DPMM.
Dataset recommendation
The most similar datasets can be recommended to researchers simply by comparing the cosine similarity of the researcher and dataset vectors using Equation ( 3 ):
where D is all the datasets that can be recommended to researcher r ; |$\cos(v_r, v_d)$| is the cosine similarity between researcher vector ( v r ) and dataset vector ( v d ).
The high-level system architecture of the dataset recommendation system is shown in Figure 4 . This dataset recommendation system is initiated by a researcher (user) by submitting his/her name and CV (or list of publications). The name is searched in PubMed for publication details, and then titles of publications from PubMed were matched with publication titles in CV. The matched publications are then clustered using DPMM to identify research fields of the researcher. Finally, the top similar datasets are recommended using the calculated cosine similarity between the researcher vector (or researcher’s cluster vector) and dataset vectors. The researcher vector (or researcher’s cluster vector) is calculated using Equation ( 1 ).
Three dataset recommendation systems are evaluated in this article: a baseline method using the researcher’s vector generation method and two proposed methods using the proposed researcher’s vector generation method.
Baseline system
The baseline system uses the researcher’s vector using Equation ( 1 ) of the baseline method in Section 3.2.1 . The top datasets are recommended after calculating the cosine similarity between the researcher’s vector and dataset vectors. This system reflects only one research field for each researcher.
MIDR System
The cluster vectors are generated using the modified Equation ( 1 ). Here, cluster-specific research area vectors are created for each researcher, instead of a single vector for each researcher as in baseline system. Papers in a single cluster are multiplied with their recency factors and summed. Then, the summation was divided the number of papers in that cluster.
This system uses multiple pseudo vectors for multiple clusters of a researcher ( |$v_{c_i}$| for i th cluster), indicating different research fields that a researcher might have, as mentioned in Section 3.2.2 .
This system compares each cluster vector with the dataset vectors and recommends the top datasets by computing the cosine similarity among them. Finally, it merges all the recommended datasets in a round-robin fashion for all the clusters, so that the researcher is able to see various datasets related to different research fields together.
MIDR System (Separate)
This system is an extension of our proposed MIDR system. Some researchers liked the way recommended datasets were merged. However, other researchers wanted dataset recommendations for each cluster separately. For this reason, another system was developed where the recommended datasets were shown separately for each research cluster, allowing researchers to obtain different recommended datasets for different research interests.
Number of clusters with varying α values for proposed α based on our initial evaluation. Abbreviations: P: Proposed, a: total number of clusters, b: number of clusters which contains more than one paper, c: number of clusters which contains only one paper
Tuning the α parameter
A researcher with a higher number of publications is more likely to have more research interests. In this paper, research interests are represented as clusters, expressed as vectors. A Dirichlet process is non-parametric because, in theory, there can be an infinite number of clusters. By changing the α parameter, DPMM can vary the number of clusters. The α value is inversely related to the number of clusters, i.e. decreasing the α parameter in DPMM may increase the number of output clusters. Therefore, we propose an α value, which is also inversely related to the number of research publications. Further, the α value must stabilize after a certain threshold to avoid the formation of too many clusters, and it must be generalized to the number of publications. To this end, α is calculated as follows:
where N is the total number of papers for a researcher. The α value is proposed based on manually observing the clusters and collecting feedback from different researchers. Apart from inherent requirements for setting α , Equation ( 4 ) maintains a reasonable number of clusters, which was found useful by most of the evaluators.
Different α values and their corresponding number of clusters are provided in Table 2 . The number of clusters are divided into three categories: (a) total number of clusters, (b) number of clusters which contains more than one paper, (c) number of clusters which contains only one paper. We removed the clusters with one paper and used the clusters with two or more papers for recommending datasets. We observed that the number of clusters did not entirely depend upon the number of papers, a researcher had. Moreover, it largely reflected the number of research fields that the researcher participated in. For example, Researcher 2 had fewer publications than Researcher 1 and Researcher 3, but the number of clusters was more than the others. This shows that non-parametric clustering is a good technique for segmenting research areas.
There is no existing labeled clustered publication datasets available for automatic evaluation. Again, manually evaluating the clusters was a time and resource-consuming task. It might be biased as the evaluation depends upon different judgments for different researchers. Thus, we implemented K-Means for comparing to the proposed DPMM. The automatic cluster comparison was performed using inter- and intra-cluster cosine similarity (IACCS) of words and MeSH terms in the publications, separately. IACCS was the mean cosine similarity of words or MeSH terms for each pair of papers in a given cluster. Considering a cluster of size n ( |$X=\{x_1, x_2, \dots x_n\}$| ), the IACCS can be formulated using Equation ( 5 ):
where, x i and x j are the list of MeSH terms or words of the i th and j th paper, respectively, and |$\cos(x_i, x_j)$| is the cosine similarity between them. Finally, the mean of IACCS was calculated using the IACCS of individual clusters.
We computed the mean cosine similarity between words or MeSH terms of papers within clusters to calculate the inter-cluster cosine similarity (ICCS). Considering n clusters ( |$c_1, c_2, \dots c_n$| ), ICSS can be formulated using Equation ( 6 ):
where, c i and c j are the list of MeSH terms or words of all the papers in the i th and j th clusters, respectively, and |$\cos(c_i, c_j)$| is the cosine similarity between them.
For the baseline comparison, publication vectors are created using TF-IDF, then K-Means is used to compute the publication clusters. K-Means is a parametric unsupervised clustering. We implemented K-Means with two and five clusters separately for comparison purposes. On the other hand, the tuning parameter proposed for DPMM resulted in a variable number of clusters for different researchers, and these clusters were used for comparison.
Recommendation system
Mean IACCS and ICCS for K-Means and DPMM (with different cluster sizes as mentioned in Table 2 ).
Being a novel task, no prior ground truth annotations exist for publication-driven dataset recommendation. Thus, we performed a manual evaluation for each developed dataset recommendation system. We asked researchers to rate each retrieved dataset based on their publications or publication clusters. The researchers included in this study have already worked on the datasets from GEO and published papers on these datasets. The rating criterion was how likely they want to work on the retrieved datasets. We asked them to rate using one to three ‘stars’, with three stars being the highest score. Later, normalized discounted cumulative gain (NDCG) at 10 and Precision at 10 (P@10) were calculated to evaluate different systems. The ratings are:
1 star [not relevant] : This dataset is not useful at all.
2 star [partially relevant] : This dataset is partially relevant to the publication cluster. The researcher has already used this dataset or maybe work on it in the future.
3 star [most relevant] : This dataset is most relevant to the publication cluster, and the researcher wants to work on this dataset as soon as possible.
The primary evaluation metric used in this work is NDCG, which is a family of ranking measures widely used in IR applications. It has advantages compared to many other measures. First, NDCG allows each retrieved document to have a graded relevance, while most traditional ranking measures only allow binary relevance (i.e. each document is viewed as either relevant or not relevant). This enables the three-point scale to be directly incorporated into the evaluation metric. Second, NDCG involves a discount function over the rank while many other measures uniformly weight all positions. This feature is particularly important for search engines as users care about top-ranked documents much more than others ( 24 ). NDCG is calculated as follows:
where rating ( i ) is the i th dataset rating provided by users. For the present study, we set p = 10 for the simplicity of manual annotation.
The NDCG@10 for the baseline and MIDR systems is calculated using the ratings of only the top ten retrieved datasets. For the MIDR system (separate), there were multiple publication clusters for a single user, and for each publication cluster we recommended datasets separately. NDCG@10 was calculated for each publication cluster using the top ten datasets and later averaged to get a final NDCG@10 for a single researcher. For NDCG@10 calculation, the 1-star, 2-star and 3-star are converted to 0, 1 and 2, respectively. We also calculated P@10 (strict and partial) for the baseline and proposed systems. Strict considers only 3-star, while partial considers both 2- and 3-star results. The results presented in this study were evaluated using a total of five researchers (with an average of 32 publications) who already worked on GEO datasets. This is admittedly a small sample size, but is large enough to draw coarse comparisons on this novel task.
We compared DPMM clustering with K-Means as mentioned in Section 3.5 . ICCS and mean IACCS values for different clustering methods are presented in Table 3 . In general, higher mean IACSS and lower ICCS generally indicate better clustering. However, this is not always the case, especially when the number of clusters are small, and each cluster contains multiple publications for a single researcher. In this situation, the IACSS for individual cluster decreases after being divided by the number of publication pairs in each cluster. Furthermore, DPMM and K-Means were comparable when the number of clusters produced by both were close to each other. For all the cases, DPMM had higher mean IACSS and lower ICCS than K-Means using words. This suggests that DPMM was well-suited for clustering a researcher’s publications into multiple research fields.
Researcher-specific results of the dataset recommendation system are shown in Table 4 . The results for individual researchers are listed for all the systems. Metric-specific average results for all the systems are also shown in Table 4 . The baseline system did not have any publication clusters and all publications were vectorized using Equation ( 1 ). Next, the top ten similar datasets were used to evaluate the results of the baseline system and it obtained the average NDGC@10, P@10 (P) and P@10 (S) of 0.80, 0.69 and 0.45, respectively.
The proposed MIDR system obtained the average NDCG@10, P@10 (P) and P@10 (S) of 0.89, 0.78 and 0.61, respectively. The proposed MIDR (separate) system obtained the average NDGC@10, P@10 (P) and P@10 (S) of 0.62, 0.45 and 0.31, respectively. For calculating NDCG@10 and P@10 in the proposed MIDR (separate) system, individual cluster scores were calculated first, and then divided by the total number of clusters.
NDCG@10, partial and strict P@10 values of the different dataset recommendation systems based on three evaluators. Abbreviations: Partial: P; Strict: S
The proposed MIDR system performed better than the baseline system. The MIDR system recommended a variety of datasets involving multiple clusters/research fields as opposed to the baseline system recommended datasets from a single research field with the maximum number of publications.
Performances of the baseline and proposed MIDR (separate) systems could not be directly compared. Evaluation of the MIDR (separate) system was performed over multiple clusters with ten datasets recommended for each cluster. In contrast, evaluation of the baseline system was performed only on 10 datasets, for example, for Researcher 1 in Table 4 , evaluations of baseline system and MIDR (separate) system were performed on 10 and 50 datasets, respectively. There were other advantages of the MIDR (separate) system over the baseline system, irrespective of higher NDCG@10 for the latter. The baseline system had a bias toward a specific research field which was eliminated in the MIDR (separate) system. For Researchers 1 and 2 in Table 4 , the datasets recommended by the baseline system were found in the results of two clusters/research fields (which had the maximum number of publications) in the proposed MIDR (separate) system. However, for Researcher 3 in Table 4 , recommended datasets of the baseline system were found in the results of only one research field (with the maximum number of publications) in the proposed MIDR (separate) system.
For Researcher 1 in Table 4 , there were 31 papers with HIV keywords and those papers were not published recently. We penalized the papers according to the year of publication for all methods. However, the top datasets contained ‘HIV’ or related keywords for the baseline method. We manually checked the top 100 results and found that those were relevant to HIV . Whereas, the proposed MIDR system clustered the publications into different groups (such as HIV, Flu/Influenza , and others), which resulted in recommendations for different research fields. Therefore, Researcher 1 had the flexibility to choose the datasets after looking at the preferred clusters in the proposed MIDR or MIDR (separate) system.
Similarly, the results of the MIDR and MIDR (separate) systems could not be directly compared. Evaluation of the MIDR system was performed based on 10 datasets recommended for each researcher, whereas evaluation of MIDR (separate) system was performed based on 10 recommended datasets for each research field (cluster), which could be more than 10 datasets if a researcher had more than one research fields (clusters). Hence, the NDCG@10 and P@10 scores of MIDR (separate) system were less than the MIDR system.
For researchers looking to find specific types of datasets, a keyword-based IR system might be more useful. For researcher who generally wanted to find datasets related to their interests, but did not have a particular interest in mind, could benefit from our system. For instance, if a researcher wanted a regular update of datasets relevant to their interest, our method would be better suited. However, this proposed system may not be useful to early-stage researchers due to fewer publications. They may take advantage of the available dataset retrieval systems such as DataMed, Omicseq and Google Dataset Search; or the text-based dataset searching that we provided on the website.
Error analysis
For some clusters, evaluators rated all recommended datasets as one star. In most of these cases, we observed that the research field of that cluster was out of the scope of GEO. In this case, the NDCG@10 score was close to 1, but the P@10 score was 0. This may be one of the reasons why NDCG@10 scores were much higher compared to P@10 scores.

Screenshots of dataset recommendation system Researcher 1 (up) and Researcher 2 (down).
Initially, we had not identified whether the clusters were related to GEO or not. We recommended datasets for these unrelated clusters. For example, Researcher 2 in Table 4 had a paper cluster which was related to statistical image analysis. For this specific cluster, Researcher 2 rated all the recommended datasets as one star, which reduced the scores of the systems.
Later, we identified a threshold by averaging the similarity scores of publications and datasets for each cluster, and were able to remove the clusters which were not related to GEO. The threshold was set to 0.05 for the present study, i.e. a cluster was not considered for evaluation or showing recommendation if the average similarity score of the top 10 datasets for that cluster was less than or equals to 0.05. This threshold technique improved the results of proposed systems by 3% for Researcher 2. However, a thorough investigation on threshold involving datasets from different biomedical domains is needed for future work.
Further, a dislike button for each cluster may be provided, and users may press the dislike button if that cluster is not related to GEO datasets. Later, this information can be used to build a machine learning-based system to identify and remove such clusters from further processing. This will improve the usefulness and reduce time complexity of the proposed recommendation system.
. Limitations
The researchers’ names are searched in PubMed to collect their publications. Many recent conference/journal publications are not updated in PubMed. Further, if the researcher has most of his/her publications that did not belong to the biomedical domain, then there is a low chance of getting those papers in PubMed. This makes the dataset recommendation task harder. Authors might later be able to include a subset of their non-PubMed articles for consideration in dataset recommendation (e.g. bioRxiv preprints), but this work is currently limited to PubMed publications only.
We used PubMed name search to find the titles of a researcher’s papers. Finally, the titles were matched with the text in the CV to get publications. If there is any typo in the CV, then that publication would be rejected from being processed in further steps. As we do not fully parse the CV, instead just performing string matching to find publications, there is a high chance of rejecting publications with small typos.
The manual evaluation was performed by five researchers only. For each cluster, 10 datasets were recommended, and each researcher has to evaluate an average of 40 datasets. It was a time-consuming task for evaluators to check each of the recommended datasets. For manual evaluation, we required the human judges with expertise on the GEO datasets, which was challenging to find. Further research will entail the scaling of this evaluation process.
GETc Platform
We developed the GETc research platform that recommends datasets to researchers using the proposed methods. A researcher needs to provide his/her name (as in PubMed) and CV (or list of publications) in the website. After processing his/her publications collected from PubMed, the recommendation system recommends datasets from GEO. Researchers can provide feedback for the datasets recommended by our system based on the evaluation criteria mentioned in Section 3.5 . A screenshot of the dataset recommendation system is shown in Figure 5 . This platform also recommends datasets using texts/documents, where cosine similarity of text and datasets are calculated, and datasets with a high score are recommended to users. Apart from dataset recommendation, it can also recommend literature and collaborators for each dataset. The platform analyzes time-course datasets using a specialized analysis pipeline (http://genestudy.org/pipeline) ( 25 ). We believe that these functions implemented in the GETc platform will significantly improve the reusability of datasets.
This work is the first step toward developing a dataset recommendation tool to connect researchers to relevant datasets they may not otherwise be aware of. The maximum NDGC@10, P@10 (P) and P@10 (S) of 0.89, 0.78 and 0.61 were achieved based on the proposed method (MIDR) using five evaluators. This recommendation system will hopefully lead to greater biomedical data reuse and improved scientific productivity. Similar dataset recommendation can be developed for different datasets from both biomedical and other domains.
The next goal is to identify the clusters which are not related to datasets and used for recommendations in the present article. These clusters can be removed from further experiments. Later, we plan to implement other embedding methods and test the dataset recommendation system on a vast number of users. A user-specific feedback-based system can be developed to remove datasets from the recommendations. Several additional dataset repositories can be added in the future. Other APIs can also be added to retrieve more complete representation of researcher’s publication history.
Availability: http://genestudy.org/recommends/#/
We thank Drs. H.M, J.T.C, A.G and W.J.Z. for their help in evaluating the results and comments on designing that greatly improved the GETc research platform.
This project is mainly supported by the Center for Big Data in Health Sciences (CBD-HS) at School of Public Health, University of Texas Health Science Center at Houston (UTHealth) and partially supported by the Cancer Research and Prevention Institute of Texas (CPRIT) project RP170 668 (K.R., H.W.) as well as the National Institute of Health (NIH) (grant R00LM012104) (K.R.).
None declared.
Chen X. et al. . ( 2018 ) Datamed–an open source discovery index for finding biomedical datasets . Journal of the American Medical Informatics Association , 25 , 300 – 308 . doi: 10.1093/jamia/ocx121
Google Scholar
Roberts K. et al. . ( 2017 ) Information retrieval for biomedical datasets: the 2016 biocaddie dataset retrieval challenge . Database , 2017 , 1 – 9 . doi: 10.1093/database/bax068
Cohen T. et al. . ( 2017 ) A publicly available benchmark for biomedical dataset retrieval: the reference standard for the 2016 biocaddie dataset retrieval challenge . Database , 2017 , 1 – 10 . doi: 10.1093/database/bax061
Karisani P. , Qin Z.S. and Agichtein E. et al. ( 2018 ) Probabilistic and machine learning-based retrieval approaches for biomedical dataset retrieval . Database , 2018 , 1 – 12 . doi: 10.1093/database/bax104
Wright T.B. , Ball D. and Hersh W. et al. ( 2017 ) Query expansion using mesh terms for dataset retrieval: Ohsu at the biocaddie 2016 dataset retrieval challenge . Database , 2017 , 1 – 9 . doi: 10.1093/database/bax065
Scerri A. et al. . ( 2017 ) Elsevier’s approach to the biocaddie 2016 dataset retrieval challenge . Database , 2017 , 1 – 12 . doi: 10.1093/database/bax056
Wei W. et al. . ( 2018 ) Finding relevant biomedical datasets: the UC San Diego solution for the biocaddie retrieval challenge . Database , 2018 , 1 – 10 . doi: 10.1093/database/bay017
Sun X. et al. . ( 2017 ) Omicseq: a web-based search engine for exploring omics datasets . Nucleic acids research , 45 , W445 – W452 . doi: 10.1093/nar/gkx258
Jansen B.J. et al. . ( 2007 ) Determining the user intent of web search engine queries . In Proceedings of the 16th international conference on World Wide Web . ACM , Banff, Alberta, Canada pp. 1149 – 1150 .
Achakulvisut T. et al. . ( 2016 ) Science concierge: A fast content-based recommendation system for scientific publications . PloS one , 11 , e0158423. doi: 10.1371/journal.pone.0158423
Patra B.G. et al. . ( 2020 ) A content-based literature recommendation system for datasets to improve data reusability. A case study on Gene Expression Omnibus (GEO) datasets . Journal of Biomedical Informatics , 104 , 103399. doi: 10.1016/j.jbi.2020.103399
Sansone S.-A. et al. . ( 2017 ) Dats, the data tag suite to enable discoverability of datasets . Scientific data , 4 , 170059. doi: 10.1038/sdata.2017.59
Ellefi M.B. et al. ( 2016 ) Dataset recommendation for data linking: An intensional approach . In European Semantic Web Conference . Springer , Heraklion, Crete, Greece pp. 36 – 51 .
Nunes B.P. et al. ( 2013 ). Combining a co-occurrence-based and a semantic measure for entity linking . In Extended Semantic Web Conference , Springer , Montpellier, France 548 – 562 .
Srivastava K.S. ( 2018 ). Predicting and recommending relevant datasets in complex environments . US Patent App. 15/721,122.
Ghavimi B. et al. ( 2016 ) Identifying and improving dataset references in social sciences full texts . arXiv preprint arXiv:1603.01774 Positioning and Power in Academic Players, Agents and Agendas IOS Press 105 – 114 . doi: 10.3233/978-1-61499-649-1-105
Piwowar H.A. and Chapman W.W. ( 2008 ) Identifying data sharing in biomedical literature . In AMIA Annual Symposium Proceedings . American Medical Informatics Association , Washington, D.C., USA Vol. 2008 , p 596.
Prasad A. et al. . ( 2019 ) Dataset mention extraction and classification . In Proceedings of the Workshop on Extracting Structured Knowledge from Scientific Publications . Association for Computational Linguistics , Minneapolis, Minnesota, USA pp 31 – 36 .
Li Z. , Li J. and Yu P. et al. . ( 2018 ) Geometacuration: a web-based application for accurate manual curation of gene expression omnibus metadata . Database , 2018 , 1 – 8 . doi: 10.1093/database/bay019
Chen G. et al. ( 2019 ) Restructured geo: restructuring gene expression omnibus metadata for genome dynamics analysis . Database , 2019 , 1 – 8 . doi: 10.1093/database/bay145
Neal R.M. ( 2000 ) Markov chain sampling methods for dirichlet process mixture models . Journal of computational and graphical statistics , 9 , 249 – 265 .
Yin J. and Wang J. ( 2016 ) A model-based approach for text clustering with outlier detection . In Proceedings of the 2016 IEEE 32nd International Conference on Data Engineering (ICDE) . IEEE , Helsinki, Finland , pp 625 – 636 .
Lenoir T. and Giannella E. ( 2006 ) The emergence and diffusion of dna microarray technology . Journal of biomedical discovery and collaboration , 1 , 11. doi: 10.1186/1747-5333-1-11
Wang Y. et al. . ( 2013 ) A theoretical analysis of ndcg ranking measures . In 26th Annual Conference on Learning Theory (COLT 2013) PMLR Princeton, NJ, USA . Vol. 8 .
Carey M. et al. . ( 2018 ) A big data pipeline: Identifying dynamic gene regulatory networks from time-course gene expression omnibus data with applications to influenza infection . Statistical methods in medical research , 27 , 1930 – 1955 . doi: 10.1177/0962280217746719
Author notes
Citation details: Patra,B.G., Roberts,K., Wu,H., A content-based dataset recommendation system for researchers—a case study on Gene Expression Omnibus (GEO) repository. Database (2020) Vol. 00: article ID baaa064; doi:10.1093/database/baaa064
Email alerts
Citing articles via.
- Recommend to your Library
Affiliations
- Online ISSN 1758-0463
- Copyright © 2024 Oxford University Press
- About Oxford Academic
- Publish journals with us
- University press partners
- What we publish
- New features
- Open access
- Institutional account management
- Rights and permissions
- Get help with access
- Accessibility
- Advertising
- Media enquiries
- Oxford University Press
- Oxford Languages
- University of Oxford
Oxford University Press is a department of the University of Oxford. It furthers the University's objective of excellence in research, scholarship, and education by publishing worldwide
- Copyright © 2024 Oxford University Press
- Cookie settings
- Cookie policy
- Privacy policy
- Legal notice
This Feature Is Available To Subscribers Only
Sign In or Create an Account
This PDF is available to Subscribers Only
For full access to this pdf, sign in to an existing account, or purchase an annual subscription.
Book a Demo
Multiply your Shopify Store's Revenue with Personalization
30 day free-trial
Try Argoid for your business now!
Get product recommendation ribbons like 'Trending', 'Similar Products' and more, to improve conversion and sales Try Argoid, risk-free
5 Use Case Scenarios for Recommendation Systems and How They Help
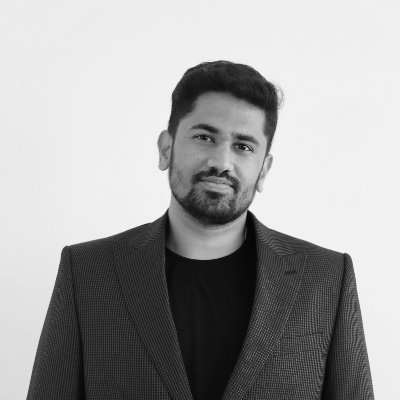
SHARE THIS BLOG
The market for recommendation engines is projected to grow from USD 1.14B in 2018 to 12.03B by 2025 with a CAGR of 32.39%, for the forecasted period. These figures are an indication of the growing emphasis on customer experience while also being a byproduct of the widespread proliferation of data.
On that note, here are five practical use cases of recommendation systems across different industry verticals. Use these to understand the multiple ways in which recommendation systems can add value to your business.
Five Practical Use Cases of Recommendation Systems
1. ecommerce recommendations.
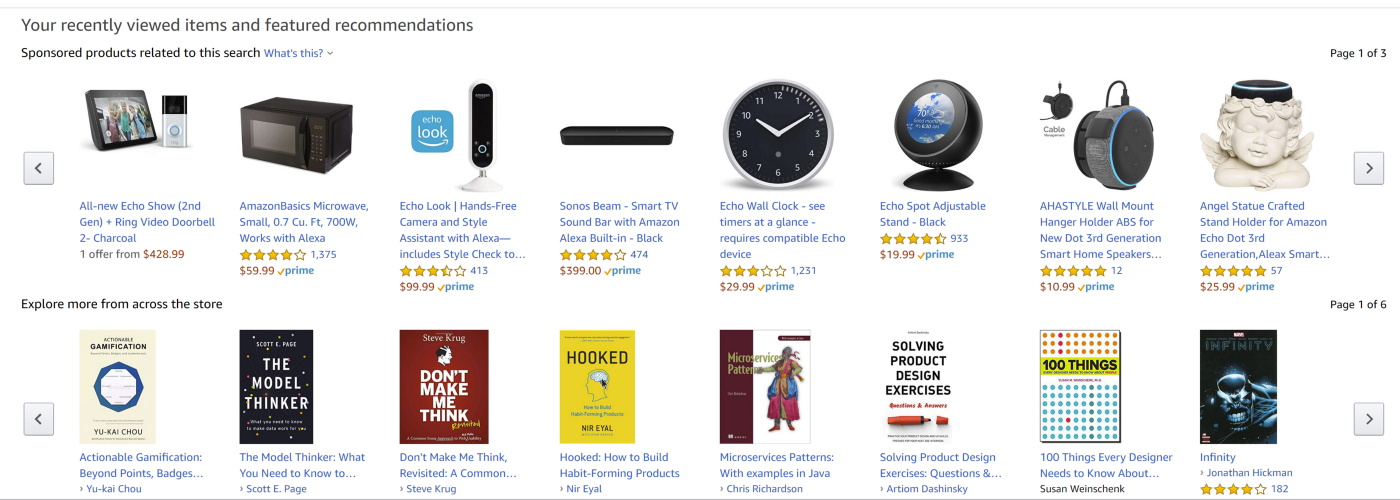
eCommerce is by far the commonest and most frequently encountered use case of recommendation systems in action.
Amazon was a pioneer in introducing this change back in 2012 by making use of item-item collaborative filtering to recommend products to the buyers. The result? A resounding 29% uplift in sales in comparison to the performance in the previous quarter! Soon enough, the recommendation engine contributed to 35% of purchases made on the platform, which was bound to impact the bottom line of the eCommerce giant.
To this date, Amazon continues to remain a market leader by virtue of its helpful and user-friendly recommender engine that now also extends to the streaming platform - Amazon Prime (more on this later). The recommendation system is designed to intuitively understand and predict account interest and behaviors to drive purchases, boost engagement, increase cart volume, up-sell and cross-sell, and prevent cart abandonment.
Other retailers like ASOS, Pandora, and H&M utilize recommender systems to achieve a gamut of favorable results.
2. Media Recommendations
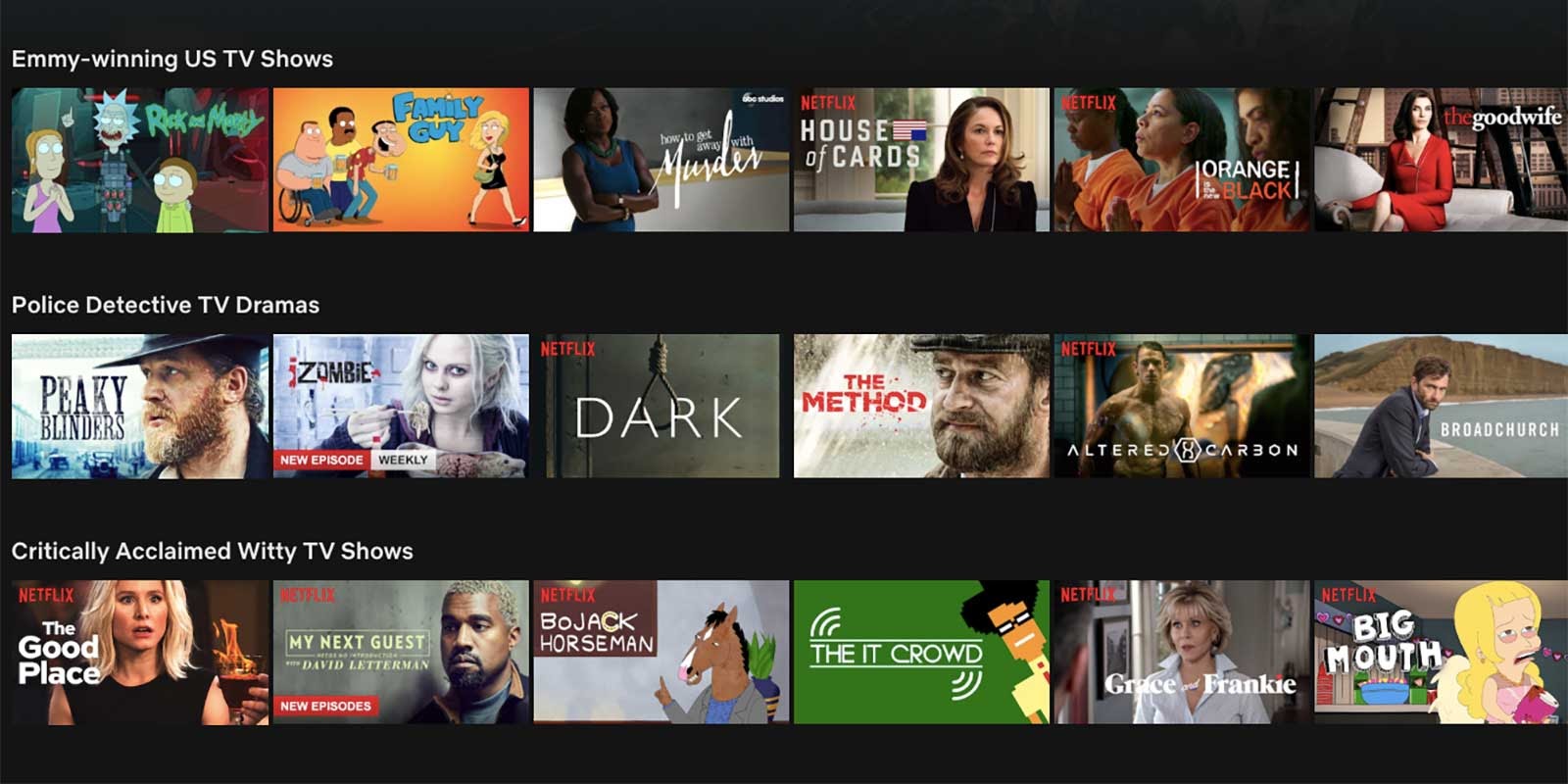
If Amazon is a frontrunner in the recommendation engine race, platforms like Netflix, Spotify, Prime Video, YouTube, and Disney+ are consolidating the role of recommendations in the field of media, entertainment, publishing, etc. Such channels have successfully normalized recommendation systems in the real world.
Typically, most media streaming service providers employ a relational understanding of the type of content consumed by the user to suggest fresh content accordingly. Additionally, the self-learning and self-training aspect of AI in recommendation engines improves relevancy to maintain high levels of engagement while preventing customer churn.
Consider Netflix, for example. About 75% of what users watch on Netflix is a result of its product recommendation algorithm. As a result, it is unsurprising that the platform has pegged its personalized recommendation engine at a whopping USD 1B per year as it maintains sustained subscription rates and delivers an impressive ROI that the company can redirect in fresh content creation.
3. Video Games and Stores
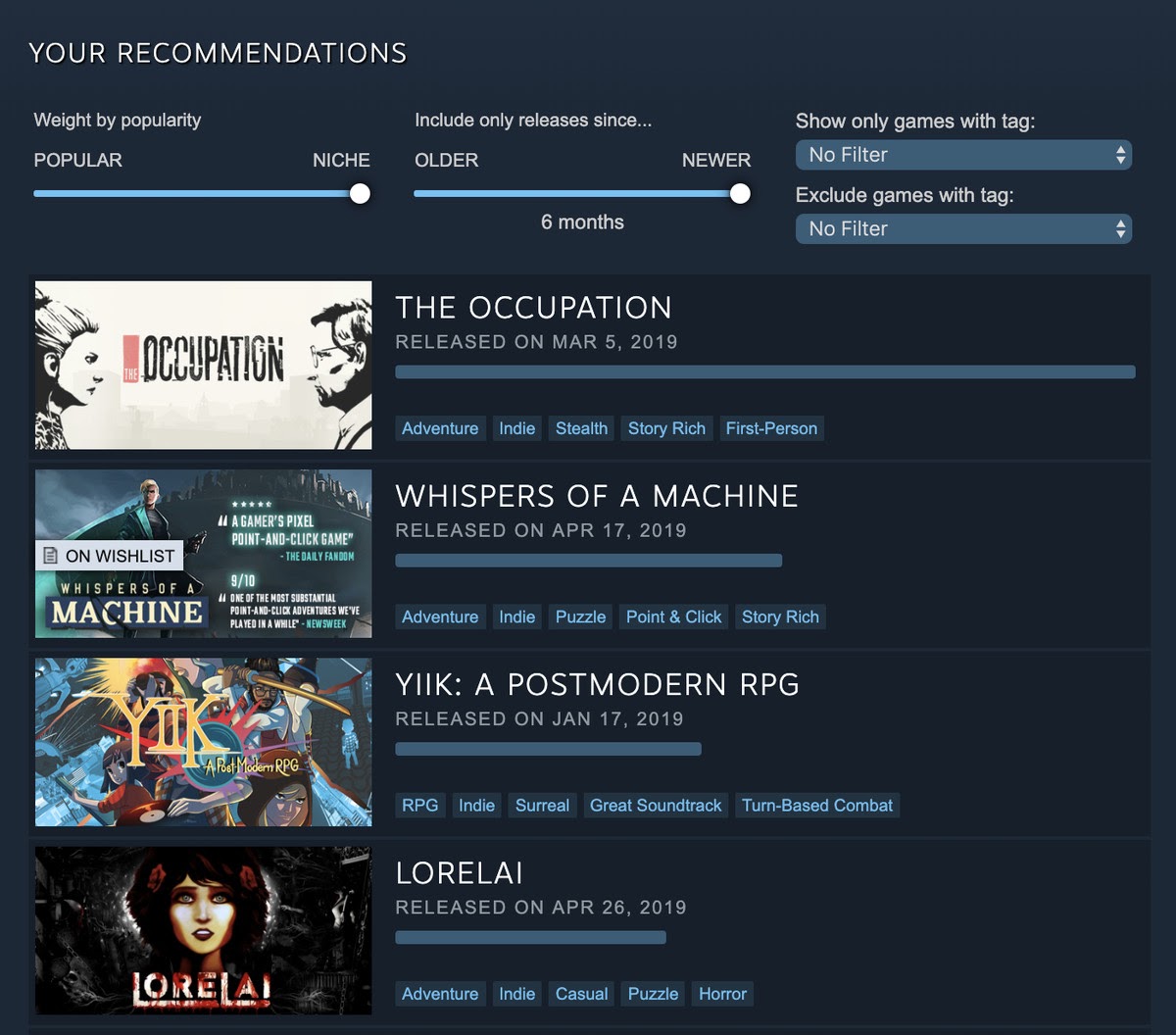
Video games are a treasure trove of user-generated data as it contains everything - from the games they play to the choices they make. This stored repository of action, reaction, and behavior translates into usable data that allows developers to curate the experience to maximize revenue without coming off as pushy or annoying.
Gaming platforms like Steam, Xbox Games Store, PlayStation Store are already well-known for their excellent recommender engines that suggest games based on the player’s gaming history, browsing history, and purchase history. As such, someone who has an interest in battle royale games like Fortnite will recommend games like PUBG, Apex Legends, and CoD rather than MMORPGs like WoW.
Similarly, video games can also deploy recommender systems to nudge players towards the top of the micro-transactions funnel to make the gaming experience easier or more rewarding. Apart from boosting engagements and in-game purchases, AI-based recommender algorithms can unlock cross-selling and up-selling opportunities.
4. Location-Based Recommendations
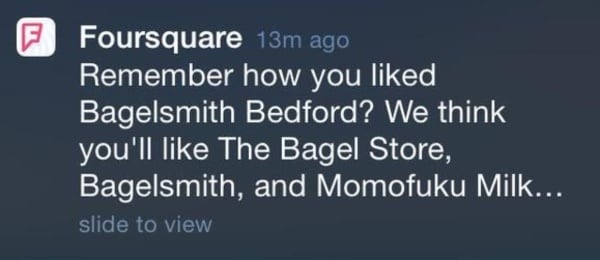
Geographic location can be a demographic factor that acts as a glue between the online and offline customer experience. It can augment marketing, advertising, and sales efforts to improve overall profitability. As a result, businesses have been in the works of developing a reliable location-based recommender system (LBRS) or a location-aware recommender system (LARS) for quite a while now, and have registered successful results.
Sephora, for instance, issues geo-triggered app notifications alerting customers on existing promos and offers when they are in the vicinity of a physical store. The Starbucks app also follows a similar system for recommending happy hours and store locations. An extension of this feature is seen in Foursquare, a local search-and-discovery mobile app, which matches users with establishments like local eateries, breweries, or activity centers, based on customer location and preferences. In the process, it maintains high engagement levels and promotes businesses in the same breath.
5. Health and Fitness

Health and fitness is one of the newest entrants in the recommender system but enjoys immense potential because of this lag. Applications can capture user inputs, such as their dietary preferences, activity levels, fitness goals, height and weight, BMI, etc., to suggest customized diet plans, recipes, or workout routines to match their fitness goals.
In addition to logging data on such platforms, its integration with wearable devices can streamline its ability to make more accurate and valuable suggestions - such as suggesting meditation or mindfulness exercises to high-risk groups upon registering elevated vitals.
Most importantly, it can capture user feedback on their fitness journey and experiences in the form of ratings to fine tune the plan and make the recommendation smarter and more personalized. Say, someone is dissatisfied with the level of difficulty of the exercise regimen, then the app can recalibrate it to suit their abilities.
Final Words
Recommendations have become an implicit customer expectation as they no longer wish to sift through stores and websites or find things that they do not like. As a result, AI-based content recommendation engines, such as the one developed by Argoid , are no longer a “nice-to-have” feature but the life blood for your business. Talk to us to know more!
FAQs on Recommendation System Use Cases
What is a recommendation system.
A recommendation system uses the process of information filtering to predict the products a user will like and accordingly rate the products based on users' preferences. A recommender system easily highlights the most relevant products to the users and ensures faster conversion.
Why recommendation systems are essential for eCommerce stores?
A recommendation system makes the shopping journey simpler and enjoyable for users. With a recommendation system, you can get product recommendations with minimum search efforts and create long-term engagement.
Which recommendation system should you use?
A recommendation system that you must use is - Argoid. It helps with 1:1 personalized product recommendations for your eCommerce store and ensures faster conversion and retention.
Similar blogs recommended for you
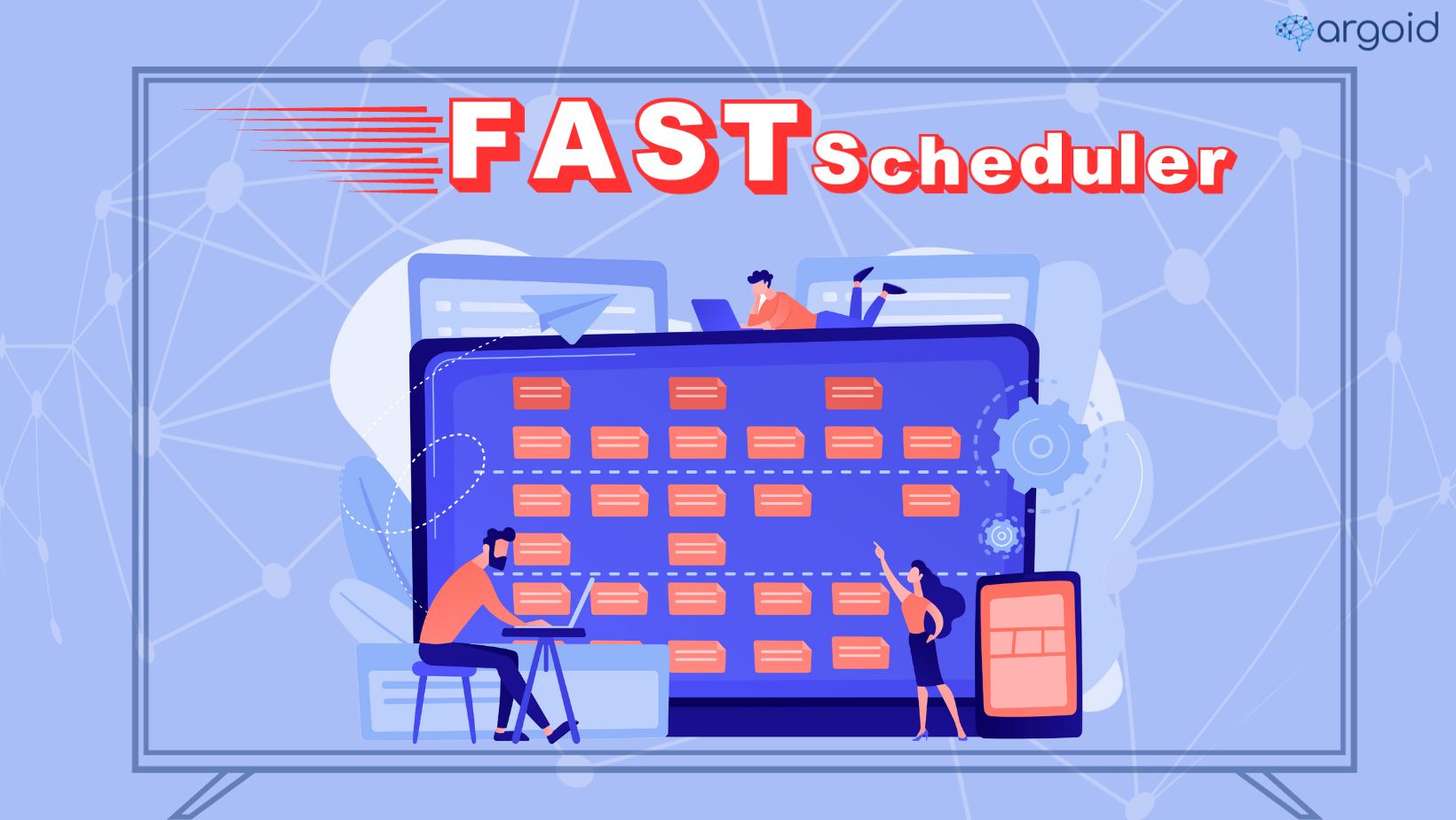
Revolutionizing TV Content Scheduling with AI: Introducing Argoid’s FAST Channel AI Co-Planner
Learn about Argoid's FAST channel AI Co-planner
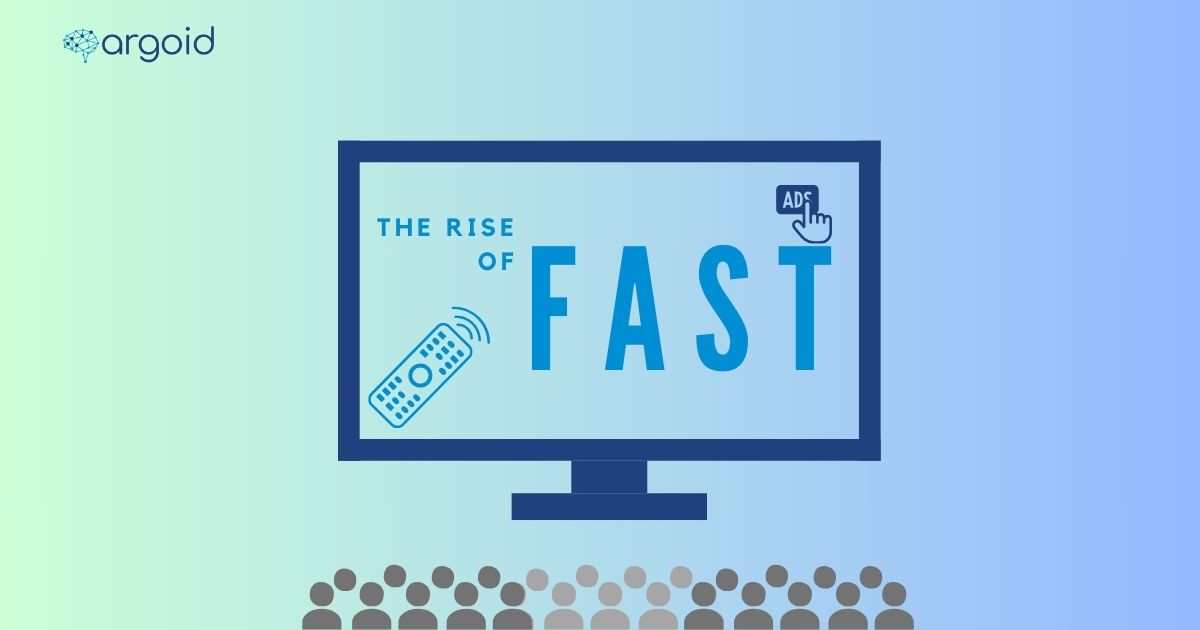
The Rise of FAST: How Ad-Supported Streaming is Changing TV
A brief on what is FAST and it is changing the television
.png)
Framing the Future: How Streaming Media Recommendation Engines Can Transform Your Content Strategy
Learn how you can leverage streaming streaming media recommendation engines to transform your content strategy
Try Argoid for your business
Zero setup fee . comprehensive product . packages that suit your business..
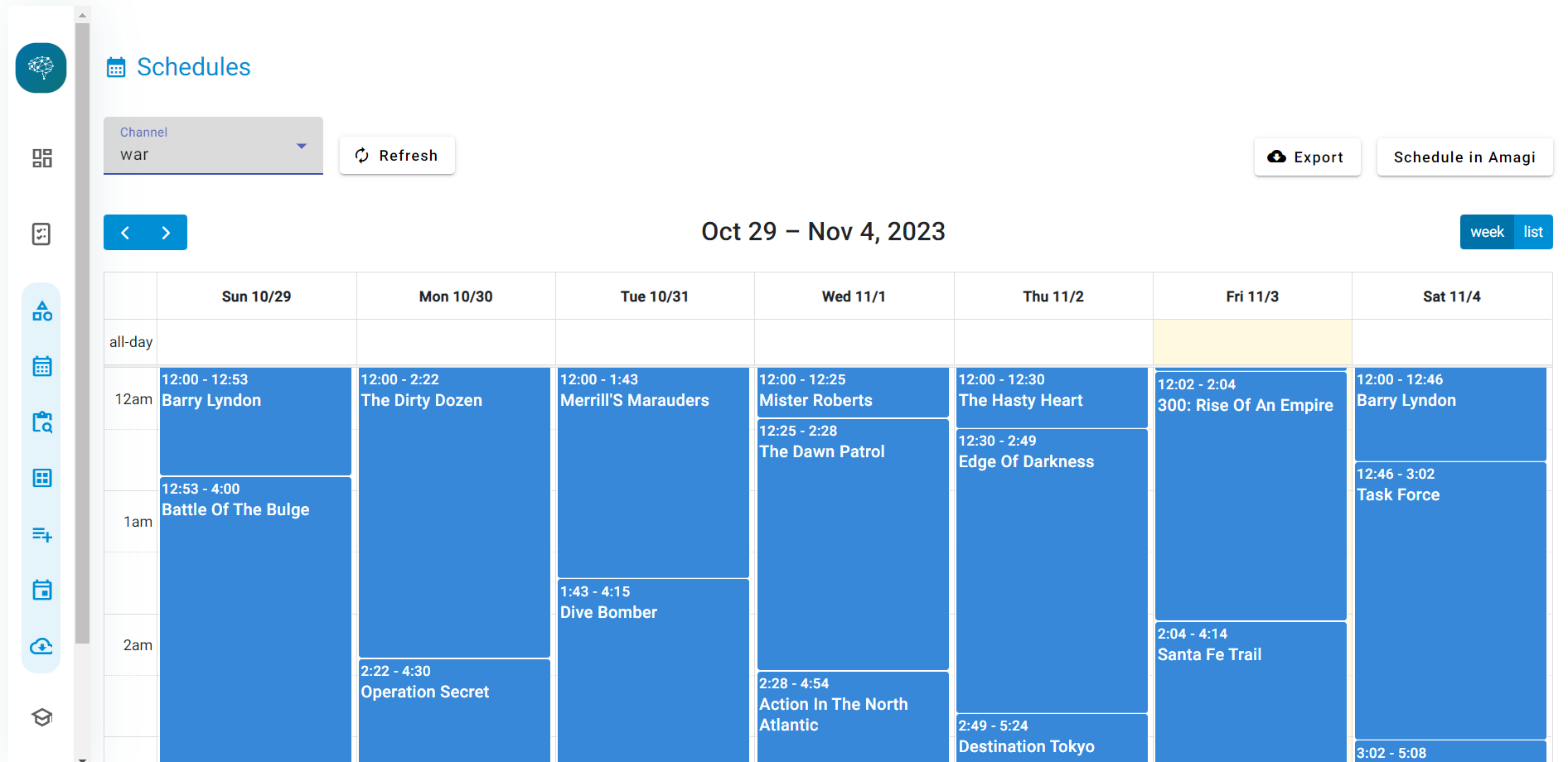
AI-powered real-time relevance for your viewers!

Subscribe to our newsletter
Get the latest on AI in eCommerce and Streaming/OTT, AI-based recommendation systems and hyper-personalization.
Advances, Systems and Applications
- Open access
- Published: 07 October 2020
A hybrid recommendation model in social media based on deep emotion analysis and multi-source view fusion
- Liang Jiang 1 , 2 ,
- Jingjing Yao 4 &
- Leilei Shi 1 , 2
Journal of Cloud Computing volume 9 , Article number: 57 ( 2020 ) Cite this article
7847 Accesses
11 Citations
4 Altmetric
Metrics details
The recommendation system is an effective means to solve the information overload problem that exists in social networks, which is also one of the most common applications of big data technology. Thus, the matrix decomposition recommendation model based on scoring data has been extensively studied and applied in recent years, but the data sparsity problem affects the recommendation quality of the model. To this end, this paper proposes a hybrid recommendation model based on deep emotion analysis and multi-source view fusion which makes a personalized recommendation with user-post interaction ratings, implicit feedback and auxiliary information in a hybrid recommendation system. Specifically, the HITS algorithm is used to process the data set, which can filter out the users and posts with high influence and eliminate most of the low-quality users and posts. Secondly, the calculation method of measuring the similarity of candidate posts and the method of calculating K nearest neighbors are designed, which solves the problem that the text description information of post content in the recommendation system is difficult to mine and utilize. Then, the cooperative training strategy is used to achieve the fusion of two recommended views, which eliminates the data distribution deviation added to the training data pool in the iterative training. Finally, the performance of the DMHR algorithm proposed in this paper is compared with other state-of-art algorithms based on the Twitter dataset. The experimental results show that the DMHR algorithm has significant improvements in score prediction and recommendation performance.
Introduction
With the development of information technology, the data on the Internet has grown exponentially, and how to effectively provide relevant information to users in need is facing great challenges [ 1 , 2 , 3 , 4 ] in recent years. To this end, various information sharing systems have been spawned, and online social networks are undoubtedly one of the most popular Internet products in the last decade [ 5 , 6 , 7 ], which provides the basic conditions for maintaining social relationships, such as discovering users with similar interests and hobbies, and acquiring information and knowledge shared by other users. These features have made online social networks attract a large number of users who generate a large amount of user generated contents since the day they were born. Therefore, how to use these user generated contents to recommend the information users are interested in and how to continuously optimize the recommendation model for improving the recommendation quality have become one of the hot issues of research [ 8 , 9 , 10 ].
At present, a large number of recommended algorithms emerge in which collaborative filtering and content-based semantic model are the more popular algorithms in the early development of recommendation systems, which have been greatly developed in the past decade [ 11 , 12 ]. The recommendation model based on deep learning has gradually become the hot spot of researchers in the face of the remarkable achievements of deep learning technology in many applications of artificial intelligence. Besides, the user rating matrix is still the main data source used by most recommendation systems, but the recommendation based on user reviews, user implicit feedback, and project content information is getting more and more attention [ 13 ]. However, the progress made in these aspects of research is not very satisfactory due to the constraints of text mining and user behavior analysis, but they have important potential in solving the recommendation accuracy, cold start and interpretability of the recommendation system. Meanwhile, there are usually more serious data sparse and cold start problems in social networks compared with the traditional recommendation algorithm, which brings great challenges to the research of social recommendation algorithms.
Aiming at the above-mentioned issues, the collaborative filtering algorithm is highly praised by researchers [ 14 , 15 ]. Its goal is to transform the binary relationship between users and posts into a score prediction problem, and then collaborative filtering or sorting based on users’ scores of posts to generate a recommendation list. Furthermore, subsequent research work has found that the recommendation results based on user ratings do not accurately reflect the user’s interest preferences due to the constraints of user ratings and the sparseness of the scoring matrix.
In the content-based recommendation, the description text information of the post content is an important recommendation basis [ 16 ]. Content-based recommendation can effectively solve the cold start problem, and is not constrained by the score sparsity, which can discover hidden information and has a good user experience. Hence, it receives wide attention in these days. However, the short text natural language description (usually short and fragmented) for the content of the post does not have enough information for the machine to make statistical inferences, which brings great difficulties to the semantic understanding of the post content.
At present, the research of deep learning technology of integrating multi-source heterogeneous data, fusion scoring matrix and review text, and multi-featured collaborative recommendation has become a hot topic [ 17 ] [ 18 , 19 , 20 ]. Based on the above research, this paper proposes a hybrid recommendation model based on deep emotion analysis and multi-source view fusion (DMHR algorithm), which aims at the balance of user score distribution and the difficulty of multi-recommendation in recommendation system. The multi-source view here is the multidimensional recommendation factor in the recommendation system. And the hybrid recommendation method of this paper combines three recommended views, such as user rating matrix, user review text, and content description information of posts, which is different from the traditional hybrid methods such as weighted fusion and cascading, this paper designs a recommendation algorithm based on collaborative training to achieve the fusion of the behavioral view of user ratings and post content.
The main contribution of this paper is to propose a scoring prediction method based on multi-recommended view fusion of collaborative training, and to explore the integration of auxiliary language information such as user review text in recommendation system by using natural language processing technology based on deep learning. The tasks of this paper are mainly reflected in the following aspects:
(1) A data preprocessing method based on HITS algorithm is introduced, which filters out the users and posts with high influence, so as to eliminate most of the low-quality users and posts and ensure better efficiency in subsequent processing. At the same time, the authority value of the post is obtained and used as the user’s initial rating for the post. Besides, a method based on a comprehensive measure of the user’s emotional tendency and the original rating level is proposed. The deviation of the user’s original score is corrected from the user’s real interest preference by mining the emotional tendency of user’s reviews. And the perspective pre-filtering method is used to achieve a comprehensive measure of the user’s emotional tendency and the original rating level, and provides a more accurate comprehensive scoring data reflecting the user’s real interest preference for the post-based collaborative filtering recommendation model.
(2) A method for text information mining based on post content description is proposed. The text information of the content description of the post is mined, the neural network method is used to represent it as a distributed paragraph vector, and the similarity calculation of the content of the post is realized, thereby constructing a recommendation model based on the content of the post. The calculation method of measuring the similarity of candidate posts and the method of calculating K nearest neighbors are designed, which solves the problem that the text description information of post content in the recommendation system is difficult to mine and utilize.
(3) A hybrid recommendation algorithm based on collaborative training is proposed. The cooperative training strategy is used to achieve the fusion of two recommended views, adds a data selection strategy based on confidence estimation and cluster analysis in collaborative training, eliminating the data distribution deviation added to the training data pool in the iterative training. On this basis, the initial recommendation results are filtered and sorted by using the scoring matrix and the similarity of posts output from the collaborative training model, and the final recommendation results are obtained.
The rest of the paper is organized as follows. In section II related work on recommendation algorithms based on collaborative filtering and content description has been discussed. In Section III and IV, a hybrid recommendation model based on deep emotion analysis and multi-source view fusion is presented. Our experiments have been analyzed and discussed in Section V. The conclusions have been given and our future work has been outlined in the last section.
Related work
The recommendation system is an effective means to solve the information overload problem and is one of the most common applications of big data technology. It utilizes knowledge discovery technology to filter information and products that users are interested in according to their historical information, hobbies and other characteristics, thereby achieving personalized recommendation. In addition, recommendation algorithms based on collaborative filtering and content description are the two most common Recommendation algorithms [ 21 ].
Recommendation algorithms based on collaborative filtering
Collaborative filtering recommendation algorithms can often be divided into user rating-based methods and implicit semantic model-based methods. User rating-based methods which use historical scoring data to discover similar users or similar projects, can generate recommendation lists based on similarity. Implicit semantic model-based methods which map the user and the project to a feature vector with some real meaning, can calculate the user’s preference for the project by calculating the inner product of the vector. For example, Guo et al. [ 22 ] proposed a neural variational collaborative filtering framework for top- k recommendation. The actual effect of the algorithm is improved by incorporating the side information of user and project, and employing a Stochastic Gradient Variational Bayes approach. Yan et al. [ 23 ] proposed a stage-wise matrix factorization algorithm by exploiting manifold optimization techniques. Applying this algorithm to the collaborative filtering recommendation model can greatly improve performance and efficiency on large-scale real data. Koren [ 24 ] proposed a matrix factorization-based model for recommendation in social rating networks, named SVD++ algorithm, which introduces the trust delivery mechanism into the social recommendation, and better reflects the influence of the social network trust relationship on the recommendation. Although the collaborative filtering algorithm is widely recommended and easy to implement, it has many problems, such as high computing cost, poor scalability and sparse data.
The social network-based recommendation is the extension of Collaborative Filtering Recommendation Algorithm in social networks, which has the characteristics of data diversity, real-time data update and high interaction. Guo et al. [ 25 ] proposed a collaborative filtering recommendation algorithm named TDSRec algorithm that integrates the characteristics of social networks. It obtains the trust and trusted characteristic matrix, and recommends accordingly. It solves the problem of data sparsity in traditional collaborative filtering algorithm to some extent. Forsati et al. [ 26 ] proposed a matrix factorization-based model for recommendation in social rating networks, named SocialMF algorithm, which introduces the trust delivery mechanism into the social recommendation, and better reflects the influence of the social network trust relationship on the recommendation. To a certain extent, the social recommendation algorithm has a wide range of applications, such as the huge amount of data, complex data content, complex algorithm implementation, high time complexity, and weak personalized recommendation results.
Recommendation algorithms based on content description
In the content-based recommendation, the content information of the project is an important recommendation basis, and it is also an important way to solve the cold start problem, but this recommendation method is subject to the information acquisition technology [ 27 ]. Content-based recommendation is based on the user’s favorite project content information to find similar projects for recommendation. The current popular practice is to use the relevant theories, methods and techniques in information retrieval to model the project content information. Zhao et al. [ 28 ] proposed a review-based recommendation model by fusing users’ internal influence into a matrix factorization to improve the accuracy of rating predictions. User sentimental deviations and the review’s reliability are explored to measure their impact on Social Recommendation. McAuley et al. [ 29 ] proposed an HFT algorithm which fuses the scoring matrix and the review text during the parameter learning and fitting phases. It models user ratings and user reviews by establishing a link between the topic distribution of the user’s reviews and the potential factors of the user or post. Bao et al. [ 30 ] proposed TopicMF algorithm which uses non-negative matrix factorization to mine the topic distribution of a single comment. It is considered that the topic distribution reflects user preferences and project characteristics, and maps with user potential factors and project potential factors. Ding et al. [ 31 ] proposed a learning algorithm based on the element-wise Alternating Least Squares learner which integrates view data into a recommendation system based on implicit feedback to mine hidden preference information other than primary feedback data such as purchases. However, text content is usually short and fragmented. If historical information is not referred to, it is easy to cause insufficient information for the machine to make statistical inferences, which brings great difficulty to the semantic understanding of the content of the item. The recommended information is also single and the user’s interest is limited.
Human emotion expressed in social media plays an increasingly important role in shaping policies and decisions. Emotion analysis on social networks has attracted increasing research attention. In order to improve the accuracy of recommendation, emotion analysis is combined with other factors. Chouchani et al. [ 18 ] used information about social influence processes to improve emotion analysis. Phan et al. [ 19 ] proposed a new approach based on a feature ensemble model related to tweets containing fuzzy emotion by taking into account elements such as lexical, word-type, semantic, position, and emotion polarity of words. Chung et al. [ 20 ] developed a novel framework for dissecting emotion and examining user influence in social media which comprehensively considered emotions, social positions, influence and other factors. However, human emotion is fluctuating, more user history data is needed and multiple recommendation factors are not easy to integrate. Recommended model based on emotion analysis easily restricted by data sparsity and cold start, so it is not easy to obtain good recommendation effect.
Data preprocessing
The data obtained from social networks is disorganized and faces the problem of sparse data and cold start, which requires pre-processing the data to improve the recommendation model. To this end, this paper introduces the HITS algorithm [ 32 ] to the recommendation model.
The HITS algorithm is one of the classic algorithms for web search. It finds the authority page and the hub page in the page collection by analyzing the hyperlinks between the pages. These characteristics of the HITS algorithm have attracted many researchers’ attention and have been introduced into online social networks. Similarly, the authority value and the hub value are used to represent the influence of users and posts respectively. HITS algorithm is used to process the data set and filter out the users and posts with high influence, so as to eliminate most of the low-quality users and posts, which ensures better efficiency in subsequent processing. At the same time, the authority value of the post is obtained and used as the user’s initial rating for the post. The authority value of the post can be represented by the sum of the hub scores of all users who have forward the particular posts:
The authority value of the post a ( p ) is standardized:
The authority value of the post a ( p ) is obtained by iterating repeatedly until a ( p ) converges. However, the initial score obtained in this way does not take into account the time attribute and the actual interest of the user, and the recommended model proposed in this paper overcomes these shortcomings.
Hybrid recommendation model based on deep emotion analysis and multi-source view fusion
In view of the above discussion on the status quo of recommendation model research, this paper proposes a hybrid recommendation model based on deep emotion analysis of user reviews and cooperative fusion of multi-source recommendation views, named DMHR. The process of DMHR hybrid recommendation model is as follow: firstly, The perspective pre-filtering method [ 33 ] is used to achieve a comprehensive measure of the user’s emotional tendency and the original rating level, and provides a more accurate comprehensive scoring data reflecting the user’s real interest preference for the post-based collaborative filtering recommendation model. Simultaneously, the text information of the content description of the post is mined, and the neural network method is used to represent it as a distributed paragraph vector, realizing the similarity calculation of the content of the post, and then a recommendation model based on the content of the post is constructed. Secondly, the cooperative training strategy is used to achieve the fusion of two recommended views, adding a data selection strategy based on confidence estimation and cluster analysis in collaborative training, and eliminating the data distribution deviation added to the training data pool in the iterative training. Finally, On this basis, the initial recommendation results are filtered and sorted by using the scoring matrix and the similarity of posts output from the collaborative training model, and the final recommendation results are obtained. The deviation of the user’s original score from the user’s real interest preference is corrected by mining the emotional tendency of user’s reviews for the next recommendation. The hybrid recommendation model system framework is shown in Fig. 1 .
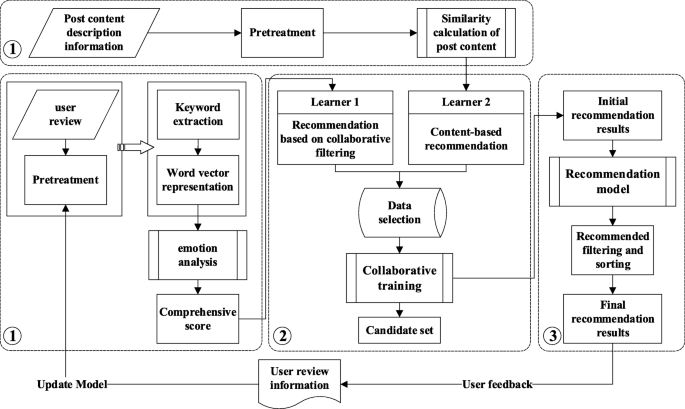
Hybrid recommendation model system framework
Emotional analysis of user reviews
Distributed vector representation of user review text.
Through statistical analysis of the user review text in the recommendation model, it is found that the presentation form is usually a keyword and a short text. Research shows that these short text messages are usually processed differently from long text. The short text has the characteristics of short length and irregular grammar, which makes traditional natural language processing technology powerless in short text analysis. Early analysis and application of short text mainly rely on enumeration or keyword matching, avoiding the semantic understanding of text, while automatic short text understanding usually relies on additional knowledge. In this paper, we use the keyword representation method based on word vector to solve the dimension disaster of traditional sparse representation and the problem of unable to express semantic information. At the same time, the association attributes between words are also mined, which improves the accuracy of the semantic representation of keywords.
Word2vec is a predictive model for high-efficiency word nesting learning, including two variants of CBOW model and Skip-Gram model [ 34 ]. CBOW predicts the probability of occurrence of a central word through words within the window, while Skip-Gram is based on the probability that the word appears within the window of the central word prediction. Its training goal is to find the vector representation of the words useful for predicting the surrounding words in sentences or documents. If for a given sentence, ω 1 , ω 2 , …, ω T means the words in the sentence, the objective function g(ω) of Skip-Gram model is to maximize the average logarithmic probability.
In the above formula, c denotes the number of training texts, the larger c is, and the higher the accuracy of the model may be. The Skip-Gram model uses the hierarchical Softmax function to define p(ω t + j | ω t ). Hierarchy Softmax uses W words as the binary tree representation of the leaf’s output layer. For each node, the relative probability of its sub-nodes is clearly expressed. Random walk algorithm is used to assign the probability of each word.
Word2vec automatically learns syntactic and semantic information from large-scale unlabeled user reviews, enabling the characterization of keywords in user reviews. The use of Word2vec to vectorize the short text information of user reviews is mainly divided into the following two steps:
(i) According to the collected user review text data, using the Skip-Gram or CBOW training word vector model, each word is expressed as a K-dimensional vector real value;
(ii) For the short text of user reviews, Top-N words are extracted to express the emotion of the text based on word segmentation using TF-IDF and other algorithms, and then K-dimensional vector representation of the extracted Top-N words is found from the word vector model.
After obtaining the K-dimension real vector representation of each key word, a common method is to use weighted average method to process the vector of the key word, which is equivalent to the vector representation of the user review text, in order to realize the emotional analysis of the review information. This weighted averaging method ignores the influence of word order on the affective prediction model. Because word vector representation based on Word2vec is only based on the dimension of words to carry out “semantic analysis”, while weighted average processing of word vectors does not have the ability of “semantic analysis” of context. Therefore, this paper constructs an emotional computing model based on word vector and long short-term memory network to realize the emotional analysis of user reviews.
Emotional calculation based on word vector and long short-term memory network
In text information processing, the commonly used method is the Recurrent Neural Network (RNN) [ 35 ]. However, RNN can lead to the disappearance of gradient in optimization when dealing with long sequences. To solve this problem, the researchers proposed a threshold (Gated RNN), the most famous of which is the Long Short-Term Memory Network (LSTM) [ 36 ]. The research also shows that the neural network with LSTM structure performs better than that with the standard RNN network in many tasks.
LSTM uses a “gate” structure to remove or add information to the cell state. It achieves the purpose of enhancing or forgetting information by adding three “gate” structures of input gate, forgetting gate and output gate in the neuron, so that the weight of the self-loop is changed. The model based on LSTM can effectively avoid the gradient expansion and even disappearance of the RNN network structure by dynamically changing the accumulation at different times when the parameters are fixed. In the LSTM network structure, the calculation formula of each LSTM unit is as shown in formulas ( 4 ) to ( 9 ).
In formulas ( 4 ) ~ ( 9 ), f t denotes the forgetting gate, i t denotes the input gate, O t denotes the output gate; \( \overset{\sim }{C_t} \) denotes the state of the cell at the previous moment, C t denotes the state of the current cell, and h t − 1 and h t respectively represent the previous moment unit output and current unit output.
In this paper, an emotional analysis method based on Word2vec and LSTM is presented as Fig. 2 . Firstly, the input of matrix form is coded into the one-dimensional vector by Word2vec to save most useful information; Then, LSTM algorithm is used to train the emotional classification model of user review text, and the grading prediction of user review is realized. At the same time, in order to take account of the interaction of user ratings and review information on real emotions, this paper uses the pre-filtering method based on viewpoints and the embedding method based on user ratings to fuse user ratings and emotional prediction ratings respectively. The former uses the LSTM network to get the prediction score, and then weights the sum with the original user score. The method based on user score embedding combines the LSTM network vector with the user rating information, and uses the result as the input of the last layer to directly output the final comprehensive score.
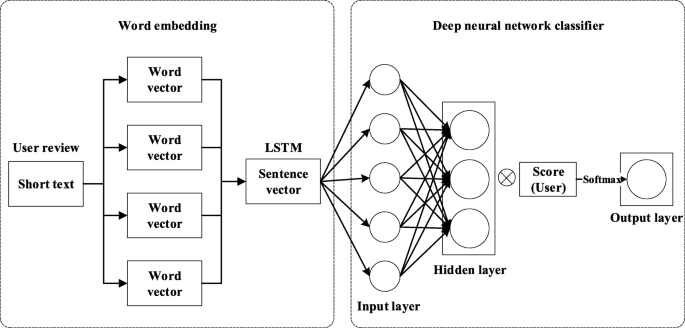
emotional analysis method based on Word2vec and LSTM
Based on the method of perspective pre-filtering, the emotion analysis of user review text modeling is performed by Word2vec and LSTM, and the emotional tendency score score r of each user’s review on the post is predicted, and the user’s original score is weighted and summed to obtain a comprehensive score score c .
In the above formula, score r represents the user’s emotional prediction score for the post review, score H represents post’s authority value in HITS algorithm, due to the limit of the number of data taken, the post’s authority value is small. In order to increase its impact on the results, it is expanded by 100 times. α is the balance factor between the two scores.
The method based on user rating embedding is based on the emotional analysis of the user review information, combining the obtained LSTM output vector with the user rating information, then the above result is used as the input to the last layer (fully connected layer) and the final comprehensive emotional score is directly output via the SoftMax activation function.
Calculation of similarity based on post content
In the recommendation model, since the natural language description of the post content is short and mostly incomplete, and usually does not follow the grammatical rules. Thus this paper uses the paragraph vector [ 37 ] to distribute the short text of the post content description. Paragraph vector is a neural network-based implicit short text comprehension model, which uses a short text vector as “context” to assist in reasoning. In maximal likelihood estimation, text vector is also updated as model parameters. It also adds encoding to the paragraph during the model training process compared with the text vector representation method based on Word2vec. Like ordinary words, paragraph coding is also mapped to a vector (i.e. paragraph coding vector). In the calculation, paragraph coding vectors and word vectors are accumulated or connected as input of SoftMax in the output layer. The paragraph code remains unchanged during the training of the text description of the post, and semantic information of the entire sentence is integrated every time the word probability is predicted. In the prediction phase, a new paragraph code is assigned to the description text of the post content while keeping the parameters of the word vector and the input layer SoftMax unchanged. Finally, the gradient descent method is used to train the new post description text until it converges, resulting in a low-dimensional vector representation of the post content. The distributed representation of the paragraph vector of the post content is shown below (Fig. 3 ).
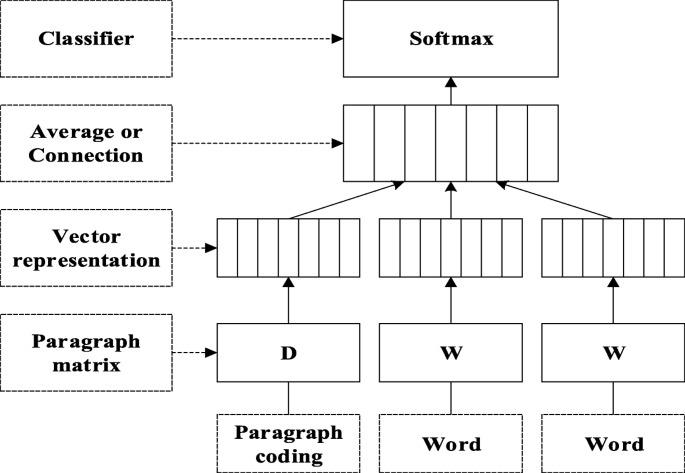
The distributed representation of the paragraph vector of the post content
After obtaining the unique d -dimensional distributed vector representation of the post content, the similarity and distance between each two post contents can be obtained by the similarity calculation. This paper uses the cosine formula to measure the similarity between two posts, and uses the Mahala Nobis distance to calculate the distance between the natural language descriptions of the two posts. Assume that the paragraph vectors of the natural language description of the two post contents are represented as PV a = ( x 11 , x 12 , …, x 1 d ) and PV b = ( x 21 , x 22 , …, x 2 d ), where d denotes the dimensions of two paragraph vectors. Then the similarity and distance between them are defined as follows:
where S is the covariance matrix of eigenvectors PV a and PV b .
Multi-source view fusion based on collaborative training
In the construction of the hybrid recommendation model, this paper uses the user comprehensive scoring view to build a post-based collaborative filtering recommendation model; at the same time, a recommendation model based on post content is constructed by using the natural language description view of post content; Finally, the fusion of two recommendation views is realized based on cooperative training strategy. In data selection, data selection algorithm based on confidence estimation and clustering analysis is used to filter the data, and then added to the training data pool of another classifier for the next round of training, so as to iterate.
Hybrid recommendation algorithm based on collaborative training
The hybrid recommendation algorithm based on collaborative training is used to construct the initial scoring matrix based on the user’s scoring of the posts. Then the perspective pre-filtering method is used to measure the composite score to update the scoring matrix. Finally, a hybrid recommendation algorithm based on collaborative training is designed in which the scoring matrix is cyclically filled and optimized according to the vector similarity of the comprehensive scoring matrix and the post content description, so as to achieve recommendation and sorting. Besides the hybrid recommendation algorithm based on collaborative training is shown in the following Fig. 4 .
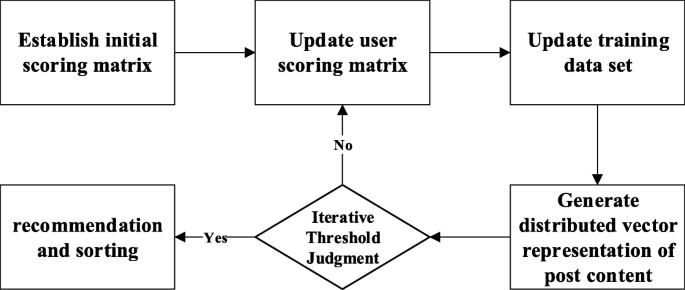
The hybrid recommendation algorithm based on collaborative training
In the recommendation model, the score of user u on post p is recorded as R u ( p ) which takes from post’s authority value in HITS algorithm; The corresponding scoring matrix is R m × n ( U , P ), where the row vector m represents the number of users, and the column vector n represents the number of posts. In the object-based collaborative filtering recommendation model, input the user’s original scoring matrix R m × n ( U , P ), where R u ( p ) ∈ [0, 1], and the virtual scoring matrix \( {\overrightarrow{R}}_{m\times n}\left(U,P\right) \) predicted by the emotion analysis model, where \( {\overrightarrow{R}}_u(p)\in \left\{0,1\right\} \) , 0 means that the user’s emotion is negative, and 1 means that the user’s emotion is positive, output as data set D train . The description of the post-based collaborative filtering recommendation algorithm is as shown in Algorithm 1.
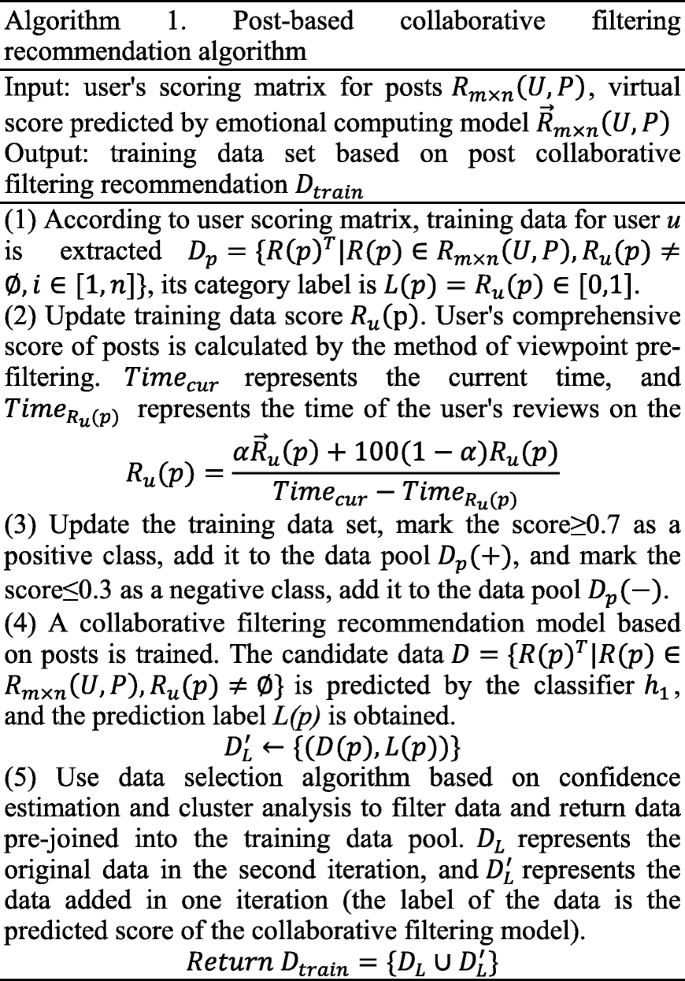
In Algorithm 1, the post-based collaborative filtering recommendation method is used to populate the default value of the user’s scoring matrix and update the training data set of user u at the same time. In the emotional classification model, it is generally divided into fine-grained (5-level classification) and coarse-grained (2-level classification). Considering that the accuracy of the 2-level emotional classification model is much higher than that of the 5-level emotional classification model, this paper adopts 2-level emotional classification in the recommendation algorithm. The user’s emotions were set to 1 point and 0 point, respectively. Then, the user’s emotional scores and original scores were comprehensively measured by means of viewpoint pre-filtering. Finally, the post-based collaborative filtering model is used to predict and fill the scoring matrix, and the data selection algorithm based on confidence estimation and cluster analysis is used to filter the data, and add the incremental data to the training data set of user u .
In the content-based description model, K -nearest neighbor algorithm is used to calculate the distance of content description, and the cosine similarity of posts and the Mahala Nobis distance of K nearest neighbor posts are used to update or fill in the user’s score and default value, which is then used in the content-based recommendation model for the next iteration. The description of the recommendation algorithm based on the content of the post is as shown in Algorithm 2.
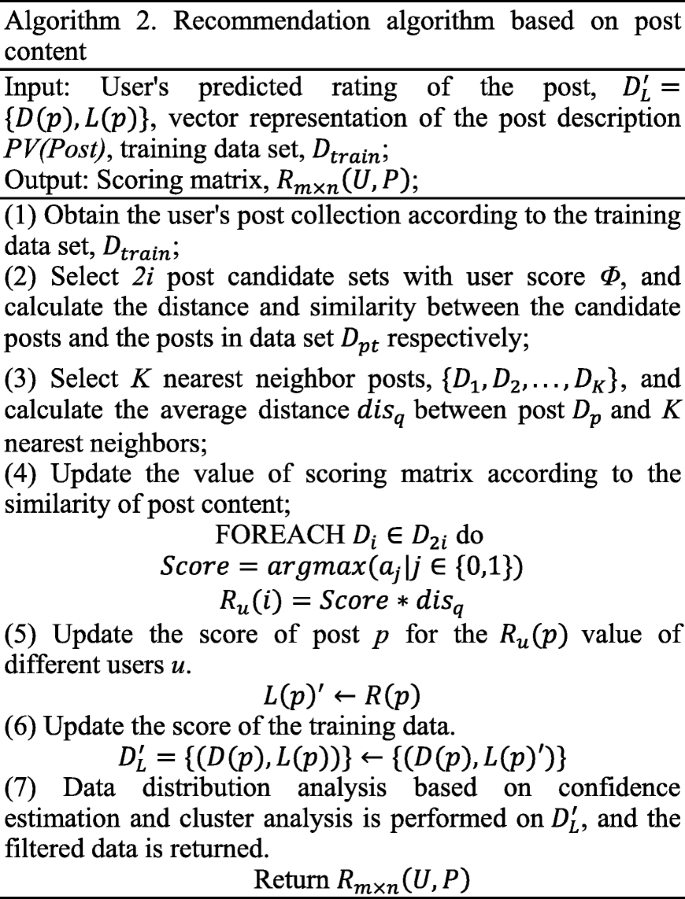
The multiple recommended techniques are mixed within the hybrid recommendation method to compensate for the shortcomings and achieve better recommendations. Different from traditional hybrid recommendation technologies, such as weighted fusion, hybrid recommendation and cascade recommendation, the collaborative training strategy is used in this paper to construct a hybrid model of collaborative filtering recommendation based on posts (Algorithm 1) and content-based recommendation (Algorithm 2). In each iterative training process of the collaborative training model, the calculated comprehensive scoring data is used to train the scoring prediction model to achieve the filling and updating of the scoring matrix. Then, the training model based on the content of the post is trained to be scored according to the updated scoring matrix and the content description information of the post (the posts with the score ≥ 0.7 and the score ≤ 0.3 are respectively placed in the training pool of the post that the user likes and dislikes). The matrix is filled and updated, and it is used as the input of the post-based collaborative filtering recommendation model for the next iteration training. This paper proposes a hybrid recommendation method based on collaborative training compared with weighted fusion hybrid recommendation, which needs to adjust the weight of each recommendation result, the difficulty of ranking hybrid recommendation, and the staged process of cascaded recommendation, which makes full use of user’s scoring information of the post (Post Profile view) and the content description information of the post (metadata view of the post) in each iteration training to achieve the fusion of the two kinds of recommendation views and a better mixed recommendation effect.
Data selection in collaborative training
In this paper, a data selection strategy is added to construct the collaborative training model to filter the data to join the training pool. Each grade of the user is specified as a category in the data. The training data in the data pool is tagged data, and the data to be predicted is unlabeled data. In the data selection strategy, not only the confidence score of the sample belongs to a certain category, but also the selected samples are evenly distributed in each cluster, which can avoid the large estimation bias of the selected training data on the Gaussian distribution. A data selection algorithm based on confidence estimation and cluster analysis is described as Algorithm 3.
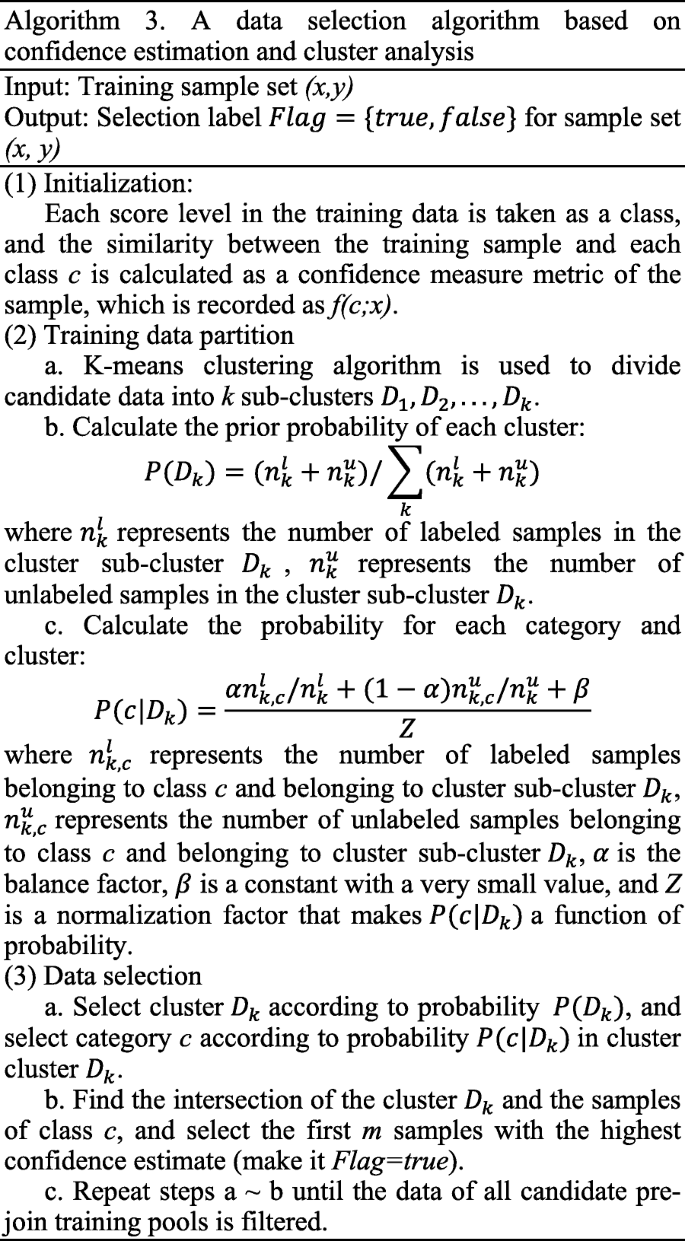
Experiments
Experiment settings and datasets.
The experiment is carried out on a computer with Intel I7 processor and 16GB memory. The datasets selected in this paper cover about 25 million reviews from Twitter from April 2015 to October 2019. The datasets contain the following contents: user information, post information and plain text review information. The specific descriptions of the datasets are shown in the following table (Table 1 ).
In preprocessing, the HITS algorithm is used to process the data set and filter out the users and posts with high influence, which lays the foundation for subsequent recommendation. The specific results of the HITS algorithm are shown in the following table (Tables 2 and 3 ).
As can be seen from the above table, the HITS algorithm is used to screen out the top ten most influential users and posts, and the authorities of the posts is used as the user’s initial rating for the post to participate in subsequent calculations.
Evaluation measures
In order to evaluate the performance of the proposed algorithm, we choose the classical accuracy index in the recommendation model: mean absolute error (MAE). For a user u and post p in our datasets, r up is the actual score of user u on post p , \( \tilde{r}_{up} \) is the predicted score obtained by the algorithm proposed in this paper. T is the number of scores of user u on post p in our datasets. Then the evaluation index MAE in the recommendation model is calculated as follows:
The lower the MAE value is, the higher the accuracy of the algorithm prediction is.
Comparative methods
In the experiment of this paper, four more classical recommendation algorithms are selected as the comparison algorithm of the proposed DMHR algorithm. The performance of each algorithm is evaluated by performance indicator MAE. In the case study, the performance of DMHR algorithm in this paper is evaluated by the Top N recommendation of some specific instances. The four comparison recommendation algorithms are described in detail as follows:
TDSRec algorithm [ 25 ]: It is a collaborative filtering recommendation algorithm that integrates the characteristics of social networks. It obtains the trust and trusted characteristic matrix, and recommends accordingly. It solves the problem of data sparsity in traditional collaborative filtering algorithm to some extent.
SocialMF algorithm [ 26 ]: It is a matrix factorization-based model for recommendation in social rating networks, which introduces the trust delivery mechanism into the social recommendation, and better reflects the influence of the social network trust relationship on the recommendation.
SVD++ algorithm [ 24 ]: It is an improved singular value decomposition (SVD) technique that introduces implicit feedback based on SVD. User’s historical browsing data and user’s historical rating data are all used as new parameters.
HFT algorithm [ 29 ]: It models user ratings and user reviews by establishing a link between the topic distribution of the user’s reviews and the potential factors of the user or post.
The influence of parameters
Effect of balance factor α
In the DMHR algorithm proposed in this paper, there is an important parameter α , which reflects the weighting of the original user scores and the emotion analysis virtual scores of the user reviews based on the perspective pre-filtering method. The formula is used to evaluate the emotional tendency of the post:
The larger the value of α is, the greater the weight of the virtual score predicted by the emotion classification model in the comprehensive score. In this experiment, the value of α is set from 0 to 1.0 , and the step size is 0.1 . The experimental results obtained by our datasets are shown in Fig. 5 below.
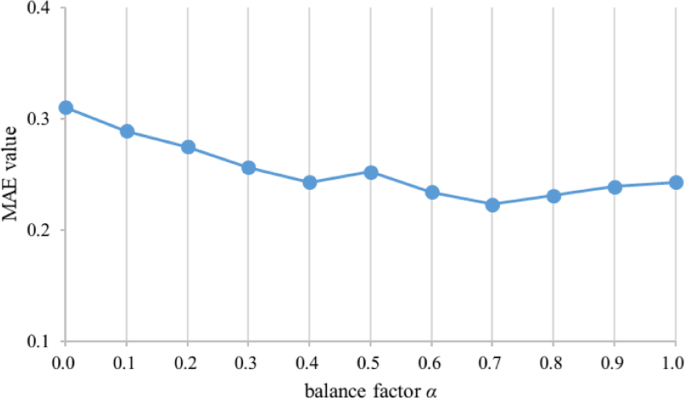
As can be seen from the data in the above Fig. 5 , when α = 0.7, the MAE value of the dataset reaches the minimum value. In the perspective pre-filtering method, the value of α represents the weight of the virtual score in the comprehensive score. This shows that the virtual score calculated by the emotion classification model has an important influence on the accuracy of the recommended prediction scoring model. To a certain extent, it also verifies the assumption proposed in this paper that the user’s review information can better reflect the user’s real interest preferences. In order to reduce the estimation bias of noise data to the score prediction, the weighted synthesis of the original user score and the user’s commentary emotional score based on the perspective pre-filtering method can be used to solve the problem of large deviation between the user’s original score and the real interest preference.
The influence of the number of neighboring posts K
In this paper, the collaborative training strategy is used to fuse user score data and post content description information to construct a hybrid recommendation system. In the post content recommendation model, the KNN algorithm is used to calculate the distance of the post content description, and the cosine similarity is used to measure the similarity of the post content description, so as to update or fill in user’s score and default value by using the score of K nearest neighbor posts. Finally, experiments have shown that choosing the appropriate K value has an important impact on the final recommendation. In this experiment, the value of K is set from 10 to 100 , and the step size is 10 . The experimental results obtained by our datasets are shown in Fig. 6 below.
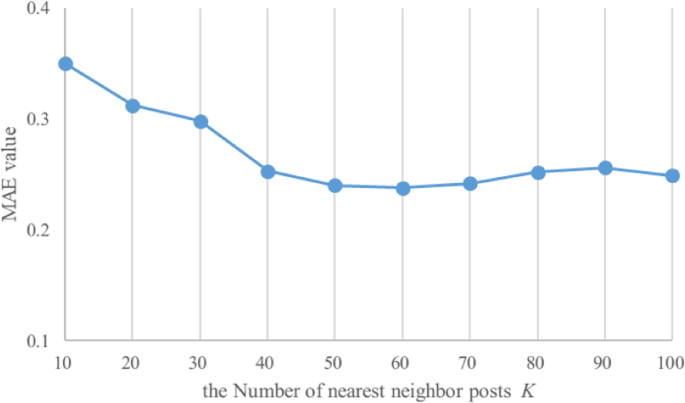
As can be seen from the data in the above Fig. 6 , the MAE value of the dataset reaches the minimum value when K = 60. Subsequently, as the K value continues to increase, the MAE value of the model also increases, indicating that the effect of the recommended model is worse. It is concluded that the recommended effect of the model on the dataset has a greater relationship with the value of the nearest neighbor number K . However, the MAE accuracy of the recommended model is not particularly sensitive to the K value, and the relatively ideal MAE accuracy can be obtained within a certain range when the value of K is large. In this experiment, it is better to choose K in the range of [50, 70]. Therefore, K = 60 is selected as the parameter of DMHR algorithm when using KNN algorithm to calculate the content description of similar posts.
Iterations of emotion classification model N
In order to take account of the interaction of user ratings and review information on real emotions, this paper uses the pre-filtering method based on viewpoints and the embedding method based on user ratings to fuse user ratings and emotional prediction ratings respectively. The former uses the LSTM network to get the prediction score, and then weights the sum with the original user score. The LSTM network vector with the user rating information is combined with the method based on user score embedding, and the result is used as the input of the last layer to directly output the final comprehensive score. In order to show the performance of the emotion classification model trained by LSTM algorithm more clearly, we compared the accuracy of the model in different iterations. Set N = {1,10,20,30, …, 100} respectively, and the 10-fold cross-validation method is used to evaluate the dataset. The detailed emotional classification model performance indicators are shown in Fig. 7 below.
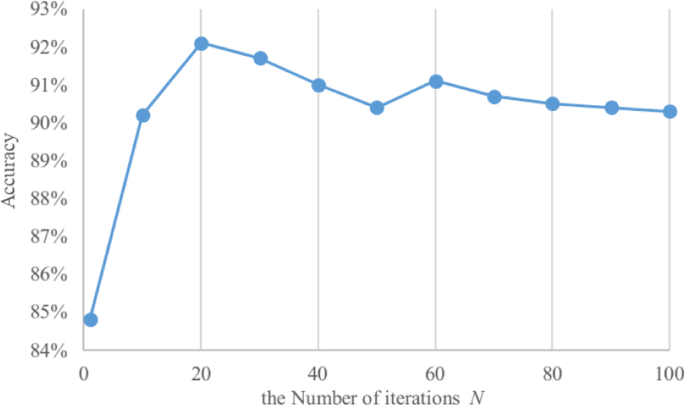
As can be seen from the data in the above Fig. 7 , in the case of the same parameter settings, the accuracy of the method based on user score embedding reaches the maximum value 92.1% when N = 20. With the further increase of the number of iterations, the accuracy of the model fluctuates above 90%, which indicates that the performance of the emotion classification model trained by LSTM algorithm is relatively stable. And when N = 20, the emotion classification model can achieve the best results to ensure the effect of subsequent experiments.
Result analysis
In this experiment, MAE is used as the evaluation index to measure the experimental effect of various recommended algorithms. On the same data set, the TDSRec algorithm, the SocialMF algorithm, the SVD++ algorithm, the HFT algorithm and the DMHR algorithm proposed in this paper are used for comparison experiments. In the DMHR algorithm, the comprehensive scoring result of the perspective pre-filtering method is adopted, and the parameters α = 0.7, K = 60 when the best result is obtained are set. For each of the other recommended algorithms, the parameters are also set to the parameters at which the best results are obtained. The specific recommendation results are shown below (Fig. 8 ).
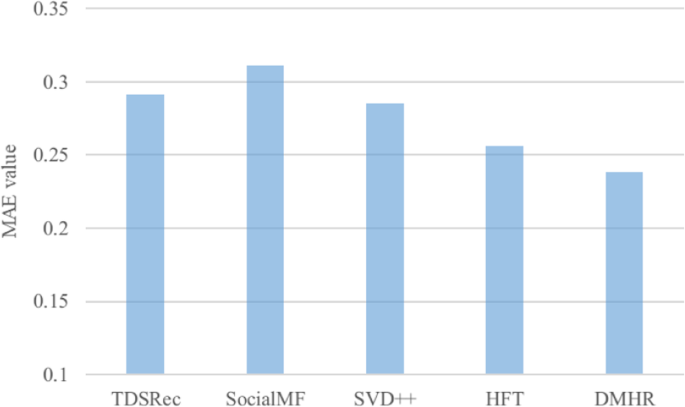
The MAE value comparison
As can be seen from the data in the above figure, overall, the DMHR algorithm proposed in this paper is superior to the other four classic recommendation algorithms in MAE evaluation indicators. The SocialMF algorithm has the worst overall performance, because the algorithm only introduces the trust delivery mechanism into the recommendation model, and cannot achieve good results on the dataset of the social network. The overall performance of the TDSRec algorithm is similar to that of the SVD++ algorithm, and it is significantly improved compared with the SocialMF algorithm. This is mainly because the two algorithms add trust and trust characteristic matrix and user history data to the model respectively, which can improve the performance of the recommended model. It shows that it is feasible to use the auxiliary information such as user reviews to improve the recommendation effect. However, the review information and interest preference are not always positively correlated. The fusion of multiple recommendation views does not always improve the performance of the model. If some unreliable recommendation factors are introduced into the model, it will have a negative effect on the performance of the system.
Based on the above analysis data, the DMHR algorithm proposed in this paper has a significant improvement on the MAE evaluation index compared with the traditional algorithm, which indicates that the prediction accuracy of the recommendation model is related to the real user score and using the perspective pre-filter based method to fuse the virtual score and get the user’s comprehensive score can effectively improve the user’s scoring accuracy, ultimately affects the recommendation model’s scoring prediction accuracy. In addition, the cold start issue is one of the most interesting issues in the recommended scenario, however few records (including ratings and reviews) are considered relevant to the “cold start”. The DMHR algorithm proposed in this paper combines post-based collaborative filtering recommendation and post content-based recommendation. The recommendation factor incorporates the emotional tendency of user reviews and the semantic information of natural language description of post content. Moreover, the data preprocessing method is used to process the messy data obtained from social networks and eliminated most of the low-quality users and posts, which ensures better efficiency in subsequent processing. Theoretically, this auxiliary information will help to solve the cold start and sparse data problem to a certain extent.
In order to evaluate the performance of the proposed model, this paper uses the leave-one-out method which has been widely used in most literatures [ 38 ]. In this section of case study, the way of Top- N recommendation is used to verify the effectiveness of the algorithm. The experiment selects 100 posts that have not been rated by the user and are most similar to the posts that the user likes, as the candidate posts. The 100 posts have been manually sorted based on the user’s attention and timeliness of the post. We take this sorting result as a real result and compare it with the recommended results obtained by the various algorithms mentioned above. The hit rate HR (Hit radio) is used to evaluate the performance of the model.
where T @ N represents the number of candidate test sets, Num @ N represents the number of Top N posts obtained by the algorithm mentioned above among the Top N recommended posts of the real result, and ranking is not considered here. The final result is shown in the figure below (Fig. 9 ).
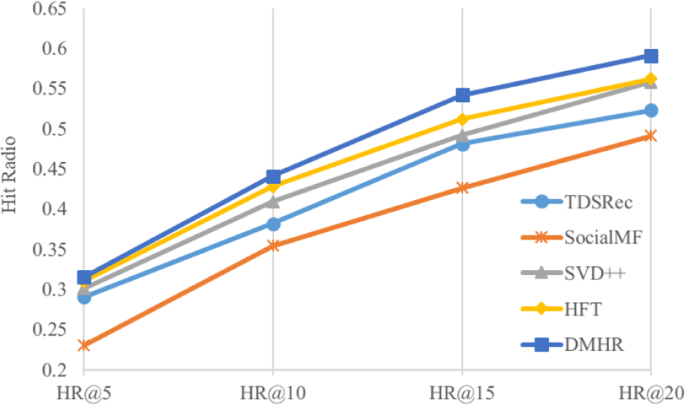
Hit radio comparison in the Top- N recommendation
It can be seen from the experimental results that the proposed DMHR algorithm and the HFT algorithm achieve better performance than other algorithms in the HR @ N the evaluation indexes. Since these two algorithms mine user comment information and use the description text information of the post content in the collaborative training model, they can better overcome the cold start problem of the recommendation system. Therefore, they have achieved good results in reflecting the recall rate performance of the recommendation system ( HR @ N ). In contrast, other algorithms such as the TDSRec algorithm, the SocialMF algorithm and the SVD++ algorithm only use the traditional collaborative filtering algorithm based on posts and use the topic distribution information to build the model, so they can’t get good recommendations. This also proves the feasibility of the idea of building a hybrid recommendation system by integrating multiple recommendation views proposed in this paper. Compared with the HFT algorithm, the DMHR algorithm uses the method of perspective pre-filtering to calculate the user’s comprehensive rating of the post, and adds the time dimension to the user’s review. The closer the time is, the larger the weighting factor is, the longer the time is, and the smaller the weighting factor is. The time factor of the recommended post is also considered when the Top- N recommendation ranking is performed. Experiments show that considering the time span in the recommendation process has a more important impact on the final recommendation results.
Conclusion and future work
The recommendation system is the most effective tool for solving information overload, and it has received much attention in the current academic and industrial circles. This paper proposes a hybrid recommendation model based on deep emotion analysis and multi-source view fusion. Based on the analysis of user behavior preferences, which focuses on the emotional mining and deep semantic analysis of text information; and the natural language description information of the post content is mined, and combined with the collaborative training strategy in semi-supervised learning, the post-based collaborative filtering recommendation view and the content-based recommendation view are combined to build a hybrid recommendation system. Because the method adopts the collaborative filtering model, it can effectively solve the problem that the user’s original score and the real interest preference are deviated in the recommendation system, and the score distribution is extremely uneven. Since the DMHR recommendation algorithm proposed in this paper takes into account the content information of the post, which effectively solves the cold start problem of the recommendation system and improves the recommended recall rate of the recommendation system. Furthermore, in terms of the recommended effect, the experimental results show that the accuracy of the DMHR algorithm proposed in this paper has been significantly improved compared with existing methods, and the problem of cold start has also been solved to some extent.
In the next step, future research can consider the impact of user preferences over time, reviews on text emotions, weights of potential features, and social relationships on recommendations. In addition, the DMHR model can be applied to group recommendation, friend relationship recommendation and other issues in the future work.
Availability of data and materials
The datasets used or analysed during the current study are available from the corresponding author on reasonable request.
Daud NN, Ab Hamid SH, Saadoon M, Sahran F, Anuar NB (2020) Applications of link prediction in social networks: a review. J Netw Comput Appl 166:102716
Article Google Scholar
Yi B et al (2019) Deep matrix factorization with implicit feedback embedding for recommendation system. IEEE Transactions Industrial Informatics 15(8):4591–4601
Kant S, Mahara T (2018) Nearest biclusters collaborative filtering framework with fusion. J Comput Sci 25:204–212
Salawu S, He Y, Lumsden J (2020) Approaches to automated detection of Cyberbullying: a survey. IEEE Trans Affect Comput 11(1):3–24
Wei J, He J, Chen K, Zhou Y, Tang Z (2017) Collaborative filtering and deep learning based recommendation system for cold start items. Expert Syst Appl 69:29–39
Nguyen V-D, Sriboonchitta S, Huynh V-N (2017) Using community preference for overcoming sparsity and cold-start problems in collaborative filtering system offering soft ratings. Electron Commer Res Appl 26:101–108
Shi L, Liu L, Wu Y, Jiang L, Hardy J (2017) Event detection and user interest discovering in social media data streams. IEEE Access 5:20953–20964
Gu K, Fan Y, Di Z (2020) How to predict recommendation lists that users do not like. Physica A: Statistical Mechanics and its Applications 537:122684
Yuan W, Wang H, Yu X, Liu N, Li Z (2020) Attention-based context-aware sequential recommendation model. Inf Sci 510:122–134
Shi L, Liu L, Wu Y, Jiang L, Panneerselvam J, Crole R (2019) A social sensing model for event detection and user influence discovering in social media data streams. IEEE Transactions on Computational Social Systems:1–10
Yu S, Yang M, Qu Q, Shen Y (2019) Contextual-boosted deep neural collaborative filtering model for interpretable recommendation. Expert Syst Appl 136:365–375
Liu H et al (2020) Hybrid neural recommendation with joint deep representation learning of ratings and reviews. Neurocomputing 374:77–85
Xiao H, Chen Y, Shi X, Xu G (2019) Multi-perspective neural architecture for recommendation system. Neural Netw 118:280–288
Rosa RL, Schwartz GM, Ruggiero WV, Rodríguez DZ (2019) A knowledge-based recommendation system that includes sentiment analysis and deep learning. IEEE Transactions on Industrial Informatics 15(4):2124–2135
Shi L et al (2019) Human-centric cyber social computing model for hot-event detection and propagation. IEEE Transactions on Computational Social Systems 6(5):1042–1050
Sanz-Cruzado J, Castells P, Macdonald C, Ounis I (2020) Effective contact recommendation in social networks by adaptation of information retrieval models. Information Processing & Management 57(5):102285
Almaghrabi M, Chetty G (2018) "A Deep Learning Based Collaborative Neural Network Framework for Recommender System," in 2018. International Conference on Machine Learning and Data Engineering (iCMLDE), Los Alamitos, pp 121–127
Google Scholar
Chouchani N, Abed M (2020) Enhance sentiment analysis on social networks with social influence analytics. J Ambient Intell Humaniz Comput 11(1):139–149
Phan HT, Tran VC, Nguyen NT, Hwang D (2020) Improving the performance of sentiment analysis of tweets containing fuzzy sentiment using the feature ensemble model. IEEE Access 8:14630–14641
W. Chung and D. Zeng, "Dissecting emotion and user influence in social media communities: An interaction modeling approach" Information Management, 57, 1, 103108, 2020
Shi C, Hu B, Zhao WX, Yu PS (2019) Heterogeneous information network embedding for recommendation. IEEE Trans Knowl Data Eng 31(2):357–370
Deng X, Zhuang F, Zhu Z (2019) Neural variational collaborative filtering with side information for top-K recommendation. Int J Machine Learning Cybernetics 10(11):3273–3284
Yan Y, Tan M, Tsang I, Yang Y, Shi Q, Zhang C (2020) Fast and low memory cost matrix factorization: algorithm, analysis and case study. IEEE Trans Knowl Data Eng 32(2):288–301
Koren Y (2008) “Factorization meets the neighborhood: A multifaceted collaborative filtering model,” Proceedings of the 14th ACM SIGKDD international conference on Knowledge discovery and data mining, Las Vegas, Nevada, pp 426–434
Guo N, Wang B, Hou Y (2018) Collaborative filtering recommendation algorithm based on characteristics of social network. J Front Computer Science and Technology 12(2):208–217
Forsati R, Mahdavi M, Shamsfard M, Sarwat M (2014) Matrix factorization with explicit trust and distrust side information for improved social recommendation. ACM Trans Inf Syst 32(4):1–38
Feng Y, Zhou P, Wu D, Hu Y (2018) Accurate content push for content-centric social networks: a big data support online learning approach. IEEE Transactions on Emerging Topics in Computational Intelligence 2(6):426–438
Zhao G, Lei X, Qian X, Mei T (2019) Exploring Users' internal influence from reviews for social recommendation. IEEE Transactions on Multimedia 21(3):771–781
McAuley J, Leskovec J (2013) “Hidden factors and hidden topics: Understanding rating dimensions with review text,” Proceedings of the 7th ACM Conference on Recommender Systems, Hong Kong, China, pp 165–172
Bao Y, Fang H, Zhang J (2014) TopicMF: simultaneously exploiting ratings and reviews for recommendation. Proceedings of the Twenty-Eighth AAAI Conference on Artificial Intelligence, Québec City, Québec, Canada:2–8
Ding J, Yu G, He X et al (2018) Improving Implicit Recommender Systems with View Data. Proceedings of the 27th International Joint Conference on Artificial Intelligence, Stockholm, pp 3343–3349
Jiang L, Shi L, Liu L, Yao J, Yuan B, Zheng Y (2019) An efficient evolutionary user interest community discovery model in dynamic social networks for internet of people. IEEE Internet Things J 6(6):9226–9236
Pero S, Horvath T (2013) “Opinion-Driven Matrix Factorization for Rating Prediction,” Proceedings of the 21st International Conference on User Modeling, Adaptation, and Personalization Rome, Italy, pp 1–13
T. Mikolov, I. Sutskever, K. Chen, G. Corrado, and J. Dean, "Distributed representations of words and phrases and their compositionality," Proceedings of the 26th International Conference on Neural Information Processing Systems - Volume 2, Lake Tahoe, Nevada, pp. 3111–3119, 2013
T. Yu, H. Hui, W. Z. Zhang, and Y. Jia, "Automatic Generation of Review Content in Specific Domain of Social Network Based on RNN," in 2018 IEEE Third International Conference on Data Science in Cyberspace (DSC), Los Alamitos, CA, USA, pp. 601–608, 2018
Shi M, Y T, Liu J (2019) Functional and contextual attention-based LSTM for service recommendation in Mashup creation. IEEE Transactions on Parallel and Distributed Systems 30(5):1077–1090
Q. Le and T. Mikolov, "Distributed Representations of Sentences and Documents," Proceedings of the 31st International Conference on International Conference on Machine Learning - Volume 32, Beijing, China, pp. 1188–1196, 2014
Gantner Z, Rendle s, Freudenthaler C, et al. “MyMedialite A free recommender system library,” Proceedings of the 5th ACM Conference on Recommender Systems. Chicago, USA, pp. 305–308, 2011
Download references
Acknowledgements
Not applicable.
This work was supported in part by the National Natural Science Foundation of China under Grant 71701082, in part by the Natural Science Foundation of Jiangsu Province under Grant BK20170069, in part by the U.K.–Jiangsu 20–20 World Class University Initiative Programme, in part by the U.K.–China Knowledge Economy Education Partnership, in part by the Postgraduate Research and Practice Innovation Program of Jiangsu Province under Grant KYCX17_1808, and in part by Natural Science Research Projects of Jiangsu Higher Education Institutions under Grant 19KJB520027.
Author information
Authors and affiliations.
School of Computer Science and Telecommunication Engineering, Jiangsu University, Zhenjiang, China
Liang Jiang & Leilei Shi
Jiangsu Key Laboratory of Security Technology for Industrial Cyberspace, Jiangsu University, Zhenjiang, China
School of Informatics, University of Leicester, Leicester, UK
School of Economy and Finance, Jiangsu University, Zhenjiang, China
Jingjing Yao
You can also search for this author in PubMed Google Scholar
Contributions
Liang Jiang, Lu Liu, Jingjing Yao, and Leilei Shi developed the idea of the study, participated in its design and coordination and helped to draft the manuscript. Liang Jiang and Leilei Shi contributed to the acquisition and interpretation of data. Lu Liu provided critical review and substantially revised the manuscript. All authors read and approved the final manuscript.
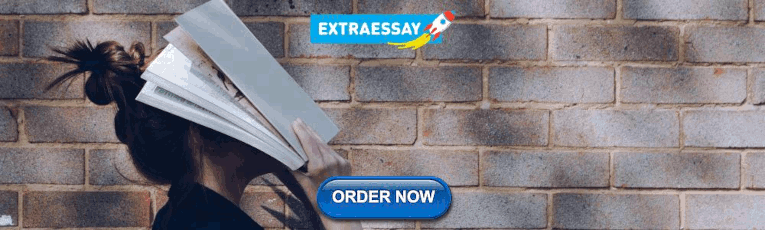
Authors’ information
LIANG JIANG received the B.S. degree from the Nanjing University of Posts and Telecommunications, China, in 2007, and the M.S. degree from Jiangsu University, Zhenjiang, China, in 2011, where he is currently pursuing the Ph.D. degree with the School of Computer Science and Telecommunication Engineering. His research interests include OSNs, computer networks, and network security. LU LIU received the M.S. degree from Brunel University and the Ph.D. degree from the University of Surrey. He is currently a Professor of Distributed Computing with the University of Leicester, U.K. His research interests are in areas of cloud computing, social computing, service-oriented computing, and peer-to-peer computing. Prof. Liu is a fellow of the British Computer Society. JINGJING YAO received the B.E. degree from Jiangsu University, Zhenjiang, China, in 2011, and the D.M. degree from Jiangsu University, Zhenjiang, China, in 2016. Her research interests include complex network, information dissemination. LEILEI SHI received the B.S. degree from Nantong University, Nantong, China, in 2012, and the M.S. degree from Jiangsu University, Zhenjiang, China, in 2015, where he is currently pursuing the Ph.D. degree with the School of Computer Science and Telecommunication Engineering. His research interests include event detection, data mining, social computing, and cloud computing.
Corresponding author
Correspondence to Lu Liu .
Ethics declarations
Competing interests.
The authors declare no conflict of interest.
Additional information
Publisher’s note.
Springer Nature remains neutral with regard to jurisdictional claims in published maps and institutional affiliations.
Rights and permissions
Open Access This article is licensed under a Creative Commons Attribution 4.0 International License, which permits use, sharing, adaptation, distribution and reproduction in any medium or format, as long as you give appropriate credit to the original author(s) and the source, provide a link to the Creative Commons licence, and indicate if changes were made. The images or other third party material in this article are included in the article's Creative Commons licence, unless indicated otherwise in a credit line to the material. If material is not included in the article's Creative Commons licence and your intended use is not permitted by statutory regulation or exceeds the permitted use, you will need to obtain permission directly from the copyright holder. To view a copy of this licence, visit http://creativecommons.org/licenses/by/4.0/ .
Reprints and permissions
About this article
Cite this article.
Jiang, L., Liu, L., Yao, J. et al. A hybrid recommendation model in social media based on deep emotion analysis and multi-source view fusion. J Cloud Comp 9 , 57 (2020). https://doi.org/10.1186/s13677-020-00199-2
Download citation
Received : 15 January 2020
Accepted : 08 September 2020
Published : 07 October 2020
DOI : https://doi.org/10.1186/s13677-020-00199-2
Share this article
Anyone you share the following link with will be able to read this content:
Sorry, a shareable link is not currently available for this article.
Provided by the Springer Nature SharedIt content-sharing initiative
- Hybrid recommendation system
- Emotion analysis
- Multi-source view
Advertisement
Artificial intelligence in recommender systems
- Position Paper
- Open access
- Published: 01 November 2020
- Volume 7 , pages 439–457, ( 2021 )
Cite this article
You have full access to this open access article
- Qian Zhang 1 ,
- Jie Lu ORCID: orcid.org/0000-0003-0690-4732 1 &
- Yaochu Jin 2
63k Accesses
138 Citations
249 Altmetric
40 Mentions
Explore all metrics
Recommender systems provide personalized service support to users by learning their previous behaviors and predicting their current preferences for particular products. Artificial intelligence (AI), particularly computational intelligence and machine learning methods and algorithms, has been naturally applied in the development of recommender systems to improve prediction accuracy and solve data sparsity and cold start problems. This position paper systematically discusses the basic methodologies and prevailing techniques in recommender systems and how AI can effectively improve the technological development and application of recommender systems. The paper not only reviews cutting-edge theoretical and practical contributions, but also identifies current research issues and indicates new research directions. It carefully surveys various issues related to recommender systems that use AI, and also reviews the improvements made to these systems through the use of such AI approaches as fuzzy techniques, transfer learning, genetic algorithms, evolutionary algorithms, neural networks and deep learning, and active learning. The observations in this paper will directly support researchers and professionals to better understand current developments and new directions in the field of recommender systems using AI.
Similar content being viewed by others
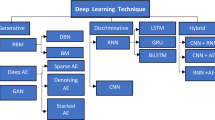
Recommendation system based on deep learning methods: a systematic review and new directions
Aminu Da’u & Naomie Salim
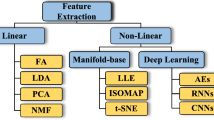
Autoencoders and their applications in machine learning: a survey
Kamal Berahmand, Fatemeh Daneshfar, … Yue Xu
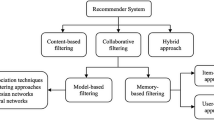
A systematic review and research perspective on recommender systems
Deepjyoti Roy & Mala Dutta
Avoid common mistakes on your manuscript.
Introduction
It is challenging for businesses in a competitive marketplace to offer products and services that appeal directly to an individual customer’s needs. Personalized e-services help to solve a major problem—that of information overload—thereby making the decision process easier for customers and enhancing user experience. The recommender systems used in these personalized e-services were first established twenty years ago and were developed by employing techniques and theories drawn from other artificial intelligence (AI) fields for user profiling and preference discovery. The past few years have seen a huge increase in successful AI-driven applications. Successes include Deepmind’s AlphaGo, the AI-driven program that famously won the game ‘Go’ against a professional human player, and the self-driving car, as well as others in the areas of computer vision and speech recognition. These continuing advances in AI, data analytics and big data present a great opportunity for recommender systems to embrace the impressive achievements of AI.
Various AI techniques have more recently been applied to recommender systems, helping to enhance the user experience and increase user satisfaction. AI enables a higher quality of recommendation than conventional recommendation methods can achieve. This has propelled a new era for recommender systems, creating advanced insights into the relationships between users and items, presenting more complex data representations, and discovering comprehensive knowledge in demographical, textural, virtual and contextual data.
The aim of this paper is to review the most recent and cutting-edge theoretical and practical contributions to the field, to identify limitations, and to indicate new research directions in the development and application of AI in recommender systems. It will attempt to survey the issues related to recommender systems using AI, and the capacity of AI to aid the understanding of large data sets and convert data into knowledge. In this paper, we have reviewed the improvements AI has made to recommender systems, such as the inclusion of fuzzy techniques, transfer learning, neural networks and deep learning, active learning, natural language processing, computer vision and evolutionary computing. The main contributions of this paper are as follows:
A systematic review of eight fields of AI methods and their applications in recommender systems;
An overview of state-of-the-art AI in recommender systems including models, methods and applications;
A discussion of open research issues, revealing the directions of new trends and future development, expanding the scope of how AI techniques can be applied in recommender systems.
The remainder of this paper is as follows. Section 2 provides an introduction to the basics of recommender system models and methods; Section 3 examines the AI techniques currently used in recommender systems; Section 4 reviews how AI techniques are used in recommender systems and their areas of application; Section 5 considers the challenges and future directions of research on AI driven recommender systems. Finally, Section 6 concludes this paper.
Recommender systems: main models and methods
The explosive growth in information on the World Wide Web and the rapid increase in e-services has presented users with a huge number of choices, which often lead to more complex decision-making. Recommender systems are primarily devised to assist individuals who are short on experience or knowledge to deal with the vast array of choices they are presented with [ 1 ]. Recommender systems take advantage of several sources of information to predict the preferences of users for items of interest [ 2 ]. This area of research has been the focus of great concern for the past twenty years in both academia and industry, and research in this field is often motivated by the potential profit that recommender systems can generate for businesses such as Amazon [ 3 ]. Recommender systems were first applied in e-commerce to solve the information overload problem caused by Web 2.0, and they were quickly expanded to the personalization of e-government, e-business, e-learning, and e-tourism [ 4 ]. Nowadays, recommender systems are an indispensable feature of Internet websites such as Amazon.com, YouTube, Netflix, Yahoo, Facebook, Last.fm, and Meetup. In brief, recommender systems are designed to estimate the utility of an item and predict whether it is worth recommending. The core element of a recommender system is [ 5 ]:
This is a function to define the utility of a specific item \(i \in I\) to a user \(u \in U\) . \(D\) is the final recommendation list containing a set of items ranked according to the utility of all the items the user has not consumed. The utility of an item is presented in terms of user ratings. Recommender systems find an item for the user by maximizing the utility function, formulated as follows [ 5 ]:
Predicting the utility of items for a particular user varies according to the recommendation algorithm selected. Referencing the classical taxonomies of previous research [ 4 , 5 , 6 ], recommendation techniques fall into three categories: content-based, collaborative filtering (CF)-based and knowledge-based approaches. These three categories will be reviewed in the following subsections.
Content-based recommender systems
As the name suggests, content-based recommender systems make use of the content of an item’s description to predict its utility based on a user’s profile [ 7 ]. Content-based recommender systems aim to recommend items that are similar to items that have previously interested in a specific user. First, different item properties are extracted from documents/descriptions. For instance, a movie can be represented by attributes such as genre, the director, writer, actors, storyline, etc. These properties can be obtained directly from structured data, such as a table, or from unstructured data, such as an article or news. One of the most commonly used retrieval techniques in content-based recommender systems is a keyword-based model known as the vector space model with term frequency-inverse document frequency weighting [ 8 ]. Content-based recommender systems profile a user’s preferences from items in that user’s consumption records. The profile usually comprises information about what the user has liked or disliked in the past. Thus, the profiling process can be seen as a typical binary classification problem, which has been well studied in machine learning and data mining fields. Classic methods such as Naïve Bayes, nearest neighbor algorithms and decision trees are used in this step [ 9 ]. Once the user’s profile has been established, the system compares the item’s attributes with the user’s profile and finds the most relevant items from which to form a recommendation list. Recommendation in a content-based recommender system is a filtering and matching process between the item representation and the user profile, based on the features acquired in the first two steps. The final result is to forward the matched items and remove those items the user tends to dislike, so the relevance evaluation of the recommendation is clearly dependent on the accuracy of the item’s representation and the user’s profile [ 10 ].
The content-based recommender system has several advantages [ 11 , 12 ]. First, content-based recommendation is based on item representation and is thus user independent. As a result, this kind of system does not suffer from the data sparsity problem. Second, content-based recommender systems are able to recommend new items to users, which solves the new item cold-start problem. Finally, content-based recommender systems can provide a clear explanation of the recommendation result. The transparency of this kind of system is a great advantage compared to other techniques in real-world applications. There are nevertheless several limitations to content-based recommender systems [ 5 , 13 ]. Although such systems overcome the new item problem, they still suffer from the new user problem because the lack of user profile information seriously affects the accuracy of the recommendation result. Furthermore, content-based systems always choose similar items for users, leading to overspecialization in the recommendation. Users tend to become bored with these types of recommendation lists because most users want to learn about new and fashionable items rather than being limited to items similar to those they have previously used. Another issue is that items cannot always be easily represented in the specific form required by content-based recommender systems. This kind of system is, therefore, more suitable for recommending articles or news items rather than images or music.
Collaborative filtering-based recommender systems
In contrast to content-based recommender systems, which are independent of other users but dependent on a user’s personal historical records, CF-based recommender systems infer the utility of an item according to other users’ ratings [ 13 ]. This technique has been widely researched in academia [ 14 ] and was quickly applied in the industry more than 20 years ago [ 15 ]. Today, CF is still the most popular technique applied in recommender systems [ 16 ]. The basic assumption underpinning the CF technique is that users who share similar interests will consume similar items, so a system using the CF technique relies on information provided by users who have similar preferences to the given user. A classic scenario in CF is to predict a user’s ratings on unconsumed items from a user-item rating matrix, which is related to the matrix completion problem [ 17 ]. CF-based techniques are classified into two categories [ 18 ]: memory-based CF and model-based CF.
Memory-based CF is an early generation CF that uses heuristic algorithms to calculate similarity values between users or items, and can therefore be subdivided into two types: user-based CF and item-based CF [ 19 ]. The core algorithm used in the memory-CF technique is the nearest neighbor algorithm. The recommendation calculates and ranks the rating of a target user on different items based on the neighbor ratings of a user or item. This algorithm is well accepted because of its simplicity, efficiency and ability to produce accurate results. Although memory-based CF is well known for its easy implementation and relatively effective and practical application, the technique still has some non-negligible drawbacks [ 5 ]. First, it is not able to deal with the cold-start problem. When a new user/item enters the system, there are no ratings for the system to use to make predictions. Second, if an item is not new but is unpopular with users, it will receive very few ratings from consumers. Memory-based CF is unlikely to recommend unpopular items to users; therefore, the recommendation coverage is limited. Third, it cannot provide a real-time recommendation. The heuristic process takes a long time to provide a recommendation result, especially when the dimension of the user-item rating matrix is high. This problem can be partially solved by a pre-calculated and pre-stored weighting matrix in item-based CF [ 19 ], but the scalability is still unable to meet practical needs.
Model-based CF builds a model to predict a user’s rating on items using machine learning or data mining methods rather than heuristic methods, as discussed in the previous section. This technique was originally designed to remedy the defects in memory-based CF, but it has been widely studied for solving problems in other domains. In addition to the user-item rating matrix, side information is used, such as location, tags and reviews [ 20 ]. The model-based CF technique is a good choice if this ancillary information is combined with the rating matrix. Matrix factorization was a product of the Netflix Prize competition of 2009 [ 21 ], and it is still one of the most popular algorithms in this field. It projects both user space and item space onto the same latent factor space so that they are comparable. Three advantages of matrix factorization contribute to its popularity. First, the dimension of the user-item rating matrix can be reduced significantly, so the scalability of the system employing matrix factorization is secured. Second, the factorization process makes a dense rating matrix, so that the sparsity problem can be alleviated [ 22 ]. Users who only have a few ratings can acquire relatively more accurate recommendation through matrix factorization, which is a significant improvement over memory-based methods. Third, matrix factorization is highly suitable for integrating a variety of side information [ 23 ]. This helps to profile user preferences and improves the performance of recommender systems.
Knowledge-based recommender systems
In knowledge-based recommender systems, recommendations are based on existing knowledge or rules about user needs and item functions [ 6 ]. Unlike content-based and CF-based techniques, knowledge-based recommender systems retain a knowledge base that is constructed with knowledge extracted from a user’s previous records. This knowledge-base contains previous problems, constraints, and corresponding solutions. Knowledge in the knowledge base is referenced when the system encounters a new recommendation problem [ 24 ]. Case-based reasoning uses previous cases to solve the current problem [ 25 ] and is a commonly used technique for knowledge-based systems. In contrast to content-based recommender systems, finding the similarities between products requires more structured representations. In this process, a comparison of a previous case and the current case is made, along with solution adaptation.
The application of the knowledge-based recommendation technique is of particular value in house sales, financial services, and health decision support [ 26 ]. These services are characterized by highly specific domain knowledge, and each case presents a unique situation. One advantage of this technique is that the new item/user problem does not exist, since prior knowledge is acquired and stored in the knowledge base. Another advantage is that users can impose constraints on the recommendation results [ 27 ]. However, no advantage comes without a corresponding disadvantage, and in this case, the cost of system setup and management in building and maintaining the knowledge base is usually high.
Artificial intelligence: main models and methods
Artificial intelligence is a fast-developing field in which applications range from playing chess to learning systems or diagnosing disease [ 28 ]. The goal of developing AI techniques is to achieve automation of intelligent behaviors which mainly cover six areas: knowledge engineering, reasoning, planning, communication, perception, and motion [ 29 ]. Specifically, knowledge engineering refers to techniques that are used for knowledge representation and modelling to enable machines to understand and process knowledge; Techniques for reasoning are developed for problem solving and logical deduction; Planning is to help machines to set and achieve a goal; Communication aims to understand natural language and communicate with human; Perception plays the role of analyzing and processing inputs such as images or speech; and finally motion is about movement and manipulation. Except for the motion, techniques in the first five areas can be applied to enhance and boost the development of recommender systems due to the huge information processing demands.
In this section, we will introduce eight main models and methodologies as shown in Fig. 1 . Deep neural networks, transfer learning, active learning, and fuzzy techniques are representatives for knowledge and reasoning and are interconnected with each other. Evolutionary algorithms and reinforcement learning are related to reasoning and planning, while natural language processing is the main technique for communication and perception, and computer vision is for the perception of images. Among the eight methods, natural language processing and computer vision are two application areas of AI techniques in recommender systems.
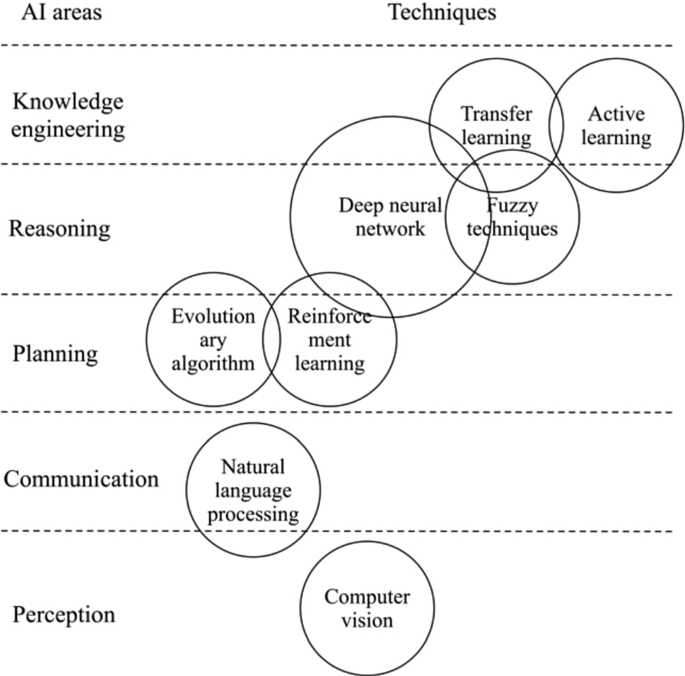
AI areas and techniques
Deep neural network
Neural network is inspired by the network of neurons in the human brain. A neural net consists of a set of neurons (or nodes) that receive and process signals from connected neurons/nodes. Each neuron can change its internal state (activation) according to the signal received so that activation weights and functions can be learned and modified in the learning process. In 1980s, neural nets were largely forsaken and ignored by the machine learning community. By the late of 1990, however, a particular type of deep feedforward network called convolutional neural network (CNN) was developed which is much easier to train [ 30 ]. CNN can also be much better generalized than traditional neural networks; they were thus quickly adopted in the areas of speech recognition and computer vision [ 31 ]. Deep learning includes the following diverse types [ 32 ]:
Multilayer perceptions (MLP) [ 33 ] are feed-forward neural networks consisting of three or more layers with a non-linear activation. It allows approximate solutions to be found for both regression and classification problems.
Autoencoders (AE) [ 34 ] are unsupervised neural networks for learning feature representations where the purpose is dimensionality reduction, data compression, or data denoising. It usually consists of two parts, the encoder and the decoder, which reconstruct the input in the output.
Convolutional neural networks (CNN) [ 35 ] are capable of processing images and visual information. It consists of an input layer, an output layer and multiple hidden layers, in which convolutional layers, pooling layers, fully connected layers or normalization layers are usually contained.
Recurrent neural networks (RNN) [ 36 ] are designed to deal with sequence data since its node connections form a directed graph. It uses internal states as memory so that sequence processes can be remembered. Representative RNN is a long short-term memory (LSTM) network [ 37 ] which is suitable for time series prediction.
Generative adversarial networks (GAN) [ 38 ] are used for unsupervised learning tasks and is implemented by two sets of models. One is a generative model and the other is a discriminative model. These two models compete to generate samples that look like the original samples.
Graph neural networks (GNNs) [ 39 ] are motivated by CNN and graph embedding to model the graph structure between nodes with neighborhood information included. GNNs have advantages in graph structured data for representation learning, link prediction and node classification, due to their high performance and good interpretability.
Transfer learning
Machine learning has attracted great attention because of the assumption that trained models can solve problems of prediction or classification, given that the training data and test data are under the same distribution. In practice, however, test data is usually dynamic and diverges from the training data. This results in the inapplicability of the current model and requires it to be rebuilt, which takes great effort. It is not always possible to retrain and build a new learning-based model since the newly collected data may be insufficient, and there are usually not enough labels accompanying the new data. This problem is extremely serious in many real-world scenarios.
Unlike traditional machine learning, transfer learning has developed as a means of transferring knowledge from a domain with relatively rich data (source domain) to a domain with scarce data (target domain) [ 40 ]. In this definition, transfer learning aims to extract knowledge from one or more source data to assist a learning task with target data. Transfer learning techniques can be divided into three main categories [ 41 ]. (1) Inductive transfer learning. The target task is different from the source task. When labeled data are available in the target domain, inductive transfer learning is similar to multi-task learning [ 42 ]. On the other hand, if there are no labeled data in the target domain, it is known as self-taught learning. (2) Transductive transfer learning. The source and target tasks are the same, but the source and target domains are different. Transductive transfer learning is also used interchangeably with domain adaptation [ 43 ]. For this type of transfer learning technique, the discrepancy between the source domain and the target domain can be caused by the existence of different feature spaces, or the different marginal distribution of feature spaces [ 44 ]. (3) Unsupervised transfer learning. The setting is similar to inductive transfer learning, but the target tasks are unsupervised learning tasks. Unsupervised transfer learning is similar to semi-supervised learning [ 45 ], except that there are no labeled data for either the source domain or the target domain. In the literature, domain adaptation, covariate shift, sample selection bias, multi-task learning, robust learning, and concept drift are all terms which have been used to describe the related scenarios.
Active learning
The basic idea of active learning is to selectively choose from training data to enable machine learning to perform better with less information. A system with an active learning strategy may query users to provide labels for unlabeled instances [ 46 ]. As the labeling process may be expensive, time-consuming and sometimes impossible, active learning can usefully be applied to many areas in AI and is especially suitable for online systems. Many AI areas related to classification or regression problems, such as speech recognition, information retrieval and computational biology, benefit from active learning [ 47 ].
Active learning strategies can be roughly divided into several groups according to their evaluation criteria on unlabeled instances. They include uncertainty sampling, query-by-committee, expected model change, expected error reduction, variance reduction, and density-weighted methods [ 48 ]. Uncertainty sampling queries instances that are least confident to be labeled. Query-by-committee is a framework that aims to minimize the inconsistency of the query to current labeled training data. Expected model change selects those instances that maintain the least change to the established model. Expected error reduction measures global error and reduces potential risk to include the queried instance. Variance reduction follows a similar direction as expected error reduction but cuts down on variance to increase the stability of the established model. Density-weighted methods search for representative instances which are important for boundary decisions or representing controversial situations.
Reinforcement learning
Reinforcement learning aims to maximize reward in a sequence of actions of a learning agent to achieve a goal, while the next situation (input) will be affected by the actions in an interactive way [ 49 ]. Different from supervised learning which relies on a labeled training set, reinforcement learning is to train an agent that can act in a situation that is not shown in the training set. It is also different from unsupervised learning, which mine patterns from unlabeled data whereas reinforcement learning is to achieve the long-term goal by interaction with the environment. The generality of reinforcement learning makes it widely applied in various aspects such as game theory [ 50 ], optimal control [ 51 ], swarm intelligence [ 52 ] and other areas such as healthcare [ 53 ] and psychology [ 54 ].
Usually, reinforcement learning follows the definition of Markov decision process [ 55 ] to describe how the agent interacts with the environment: at a step, the agent receives a state, selects an action according to a policy and receives a reward for this step, then transit to the next step. A value function will define the long-term reward accumulated during the whole process containing a series of steps. A unique challenge that exists in reinforcement learning is the dilemma between exploration and exploitation [ 56 ]. The learning agent is facing a choice to take actions that it has experienced in the past or try new actions that may bring more rewards. The balance of the dilemma lies in whether to exploit actions that in the historical records or explore new actions that finally come to a reward maximization. The methods of reinforcement learning can be divided according to value function, policy, and model in value-based or policy-based, off-policy or on-policy, model-based or model-free and hybrids of the above [ 57 ]. Recently, the combination of deep neural networks and reinforcement learning becomes popular with two well-known and successful works: deep Q-network [ 58 ] and AlphaGo [ 59 ]. Deep neural networks significantly boosted reinforcement learning in dealing with high dimensional states or/and actions and make it as an indispensable component in future AI systems.
Fuzzy techniques
Fuzzy techniques can be used to model real-world concepts that cannot be represented in a precise way; thus, it is widely used in the AI area. Fuzzy techniques have attracted considerable attention in the literature; for example, researchers have applied fuzzy sets to represent linguistic variables when feature values cannot be precisely described in numerical values, and to describe fuzzy distance for the retrieval of similar cases [ 60 ]. Knowledge extracted from data is hidden and uncertain by nature, so using fuzzy logic and fuzzy rule theory to handle the associated vagueness and uncertainty is apt and can improve the accuracy of both classification and regression [ 61 ]. Fuzzy techniques facilitate data and knowledge sharing between businesses where knowledge can be used to build data analytics models efficiently [ 62 ]. This has the advantage of significantly reducing the computational expense incurred by businesses, particularly in data-shortage and rapidly-changing environments, and provides outstanding benefit to their business intelligence systems.
Evolutionary algorithms
Evolutionary algorithms (EAs) are a sub-area of AI research that form a class of nature-inspired, population-based search algorithms for global optimization. An evolutionary algorithm starts with an initial population, known as the parent population, which is a set of candidate solutions to a problem to be solved. New solutions, called offspring, are generated by applying genetic operators such as crossover and mutation to parent individuals. Offspring individuals are selected according to their fitness to become the parents of the next generation. This process continues until certain termination conditions are met.
There are three independently developed streams of evolutionary algorithms: the genetic algorithm [ 63 ], evolution strategies [ 64 ], and genetic programming [ 65 ]. Other popular EAs include estimation of distribution algorithms [ 66 ] and differential evolution [ 67 ]. Several other nature-inspired meta-heuristic algorithms have also been developed, such as particle swarm optimization [ 68 ] and ant colony optimization [ 69 ], which are sometimes categorized as EAs in a very loose sense. Although they were designed to solve a wide range of problems, EAs have been shown to be very powerful in solving complex optimization problems that are difficult for traditional mathematical programming techniques to solve. Evolutionary algorithms (EAs) are divided into single-objective and multi-objective EAs [ 70 ] according to the number of objectives to be optimized. Multi-objective EAs that have more than three objectives are also termed many-objective EAs [ 71 ].
Natural language processing
Natural language processing is a traditional research area in AI that dates back to the 1950s. Its origins lie in the recognition of hand-written image analysis, and it entered a new era with the development of machine learning [ 72 ]. Text data are different from other kinds of structured data; their most important characteristics are sparsity and high dimensionality. They can be analyzed at different levels of representation, such as bag-of-words, topics or embedded vectors. Many machine learning algorithms, such as support vector machine and Bayesian network [ 73 ], can be applied to a wide range of natural language processing areas, as detailed below.
To illustrate the broad reach of natural language processing, the various tasks are clustered but not limited to the following aspects. Information extraction aims to extract structured information from unstructured text and includes entity extraction and relationship extraction [ 74 ]. Text summarization analyzes the importance of sentences, then scores and selects the set of best sentences to compose a summary. Text classification is widely used in data mining research to label text and relate it to multiple applications, such as customer segmentation, document organization, and CF [ 75 ]. Sentiment analysis extracts hidden opinion, sentiment and subjective information from the text to assist with classification or prediction [ 76 ]. Dimensionality reduction techniques such as latent semantic indexing, topic modeling, and latent Dirichlet allocation are widely used in natural language processing to reduce the number of variables and obtain a set of principal variables [ 77 ]. The evolution of text corpus and its interactions with other context data or heterogeneous data have also been well researched in AI.
Computer vision
Humans can directly recognize an object by discerning its shape, color, motion and related characteristics. As increasing amounts of data with images and video accumulate, it is desirable for machines to obtain high-level understanding from vision through such techniques as object capture, recognition or tracking [ 78 ]. A number of models have been established that describe and process images or videos to effectively contribute to classification, detection, and segmentation problems. Recent developments in deep learning have revolutionized the computer vision research area, given the ability of deep learning methods to extract features [ 79 ]. This has prompted their use in computer vision tasks for analyzing, processing and describing digital images and videos. In particular, CNN has been widely adopted for recognition and detection tasks [ 80 ], which has resulted in huge changes being made in image processing, not only in academia but also in industry.
Recommender systems with artificial intelligence
Multiple artificial intelligent techniques have been introduced and applied to recommender systems to meet the increased recommendation demands of the big data information explosion. In this section, we highlight six AI techniques that have enhanced recommender systems.
Deep neural networks in recommender systems
Neural network is rarely used in recommender systems since the task of recommendation concerns the ranking of items rather than classification. In an early work, Salakhutdinov et al. proposed a two-layer restricted Boltzmann machine (RBM) to explore the ordinal property of ratings. This method attracted great attention in the 2009 Netflix Prize competition [ 81 ], but there has been little follow-up work apart from research by Truyen et al., who extended this work by studying the parameterization options of RBM in recommendation [ 82 ]. In contrast, deep learning has achieved great success in the fields of natural language processing, speech recognition and computer vision [ 31 ]. With the availability of more data (e.g., user-generated comments or visual photos of items), the need to integrate all the information and provide recommendation for multi-media items, such as images or videos, prompted the development of deep learning-based recommender systems [ 83 ]. In this sub-section, we divide deep learning-based recommender systems according to the different types of deep neural networks applied in recommender systems.
Multi-layer perceptron-based recommender systems
Multi-layer perceptron is used in factorization machines to help with feature engineering. It combines the advantages of linear and non-linear modeling in one recommendation framework [ 84 ]. Guo et al. improved the wide and deep model in [ 84 ] as the proposed factorization machines can be trained without feature engineering [ 85 ]. He et al. proposed neural collaborative filtering (NCF) to model the non-linear relationship between users and items in conjunction with matrix factorization to model the linear relationship [ 86 ]. NCF, which is based on multi-layer perceptrons, is widely used in recommender systems as a general model for user-item interactions.
Autoencoder-based recommender systems
AutoRec integrates an autoencoder with matrix factorization with the aim of learning non-linear latent representations of users or items [ 87 ]. AutoSVD++ is a hybrid method that fuses a contractive autoencoder and matrix factorization to generate item feature representations from item content [ 88 ]. Strub et al. improved AutoRec by boosting its robustness through the use of denoising techniques and integrating such side information as item content or user-contributed tags [ 89 ]. Autoencoder serves as a basic building block for representation learning which is well suited for user profiling and item representation learning in recommender systems.
Convolutional neural network-based recommender systems
By integrating two parallel neural networks, DeepCoNN jointly models users and items through reviews [ 90 ]. The two CNNs are connected by a shared layer facilitated by factorization machines. To exploit the information in user-contributed reviews and address the data sparsity problem, ConvMF integrates CNN into matrix factorization to improve rating prediction accuracy [ 91 ]. CNN has also been used for the hashtag recommendation task in microblogging by introducing the attention mechanism in the process of selecting the hashtags [ 92 ].
Recurrent neural network-based recommender systems
Since RNN is suitable for sequential data, it is mainly used to model and analyze the evolution of user interests or item features. Dai et al. applied RNN and proposed a co-evolutionary latent feature process for modeling the temporal dynamics of user-item interactions [ 93 ]. Wu et al. used an LSTM-based model to capture the dynamics of user behavior to predict whether or not to inherit existing user behavior in the future [ 94 ]. LSTM is also used in recommender systems to make in-time music recommendations, to predict when users will return to a music system and what their interest will be at that time [ 95 ].
RNNs have emerged as a new direction known as session-based recommender systems or sequential recommender systems where the real-time recommendation is refined according to the historical sequential data [ 96 , 97 ]. In [ 98 ], the most recent states are modelled by an RNN to predict the next item that may attract the interests of users. The early works did not take into consideration of the short-term and long-term user interests in the sequence. Later, the current state is modelled as a short-term user preference and the session state is modelled by RNNs with an attention mechanism as the long-term preference. They are equally integrated and matched with an item through a bi-linear scheme [ 99 ]. The short-term user preference is enhanced in [ 100 ] and user preference drift is also taken into consideration. Further, the two kinds of preferences are fine-tuned by a hierarchical attention network [ 101 ]. Sequential recommender systems are gaining more attention in research dealing with the relationship between short-term and long-term interests as well as integrating contextual information and preference dynamics.
Generative adversarial network-based recommender systems
Wang et al. integrated GAN to a unified information retrieval framework. It contains a generative retrieval model that learns the distribution over documents and try to generate relevant documents that look like the ground truth to fool the discriminative model, and a discriminative model that aims to classify the ground-truth documents from the generated ones as an opponent to the generative model [ 102 ]. This approach shows that GAN-based information retrieval systems offer promise, and further effort is needed specifically in the recommender system area. He et al. introduced perturbations on the user and item embedding as an adversarial regularizer under the framework of Bayesian personalized ranking [ 103 ]. A GAN is used to learn robust user/item representations not only from user-item interactions but also from knowledge graph [ 104 ], tags and images [ 105 ].
Graph neural network-based recommender systems
The ability of GNNs to learn feature for nodes from the information of neighborhoods in the graph is highly desired for recommender systems, as the user-item relationships are usually represented as a bipartite graph. The feature embedding by a GNN and random walk are incorporated in [ 106 ] and a highly scalable and efficient recommendation method is proposed and deployed in Pinterest. This work shows the great potential of GNNs to improve the productivity of recommender systems. A generalized graph neural network-based CF framework is proposed in [ 107 ] with attention-based massage-passing method for information propagation. GNN is also suited for sequential recommender systems to model the item sequences as a graph [ 108 ]. It is superior as user-item interactions are considered in the sequence while an RNN can only model one-side item information. GNN-based recommender systems are just emerging and more studies in social recommendation, sequential recommendation and cross-domain recommendation are expected.
Current trends of application of deep neural networks in recommender systems are towards addressing more complex situations such as dynamic environments, multiple data sources and heterogeneous data representations. They aim to develop methods and build models with hybrids of different types of deep neural networks to comprehensively model the user preferences.
Transfer learning in recommender systems
Transfer learning has demonstrated great success and a promising future in the machine learning field. In the field of recommender systems, transfer learning extends recommendation requests from a single domain to multiple domains. By exploiting the correlation of several domains, all domains can benefit from mining user preferences that cannot be found with single domain data. For example, an active user in a movie domain is likely to be interested in books and music related to movies they like. Another reason to exploit multiple domains is to solve the data sparsity or cold-start problem, as there may be insufficient data in one domain but relatively rich data in another domain. For example, a user may have few records in a book category in an online review and rating system but may have a large number of movie ratings, thus an abundance of data in a secondary domain can assist recommendation in the target domain. This demand for a rich and diverse recommendation, together with the ability to alleviate the data sparsity problem, has driven the development of cross-domain recommender systems (CDRS).
The biggest difference between CDRS and other transfer learning methods is that there is no explicit feature space in CDRS. This means that CDRS cannot be classified as a single type of transfer learning method, because they involve the practical application of multiple transfer learning techniques. From the practical perspective, CDRS provide multi-domain recommendation for online shopping retailers selling a variety of goods while at the same time offering a solution to the data sparsity problem. Some methods connect two domains through auxiliary information other than preference data [ 20 ], while CDRS based on preference data can be strategically designed according to the overlap of users and items, the form the data takes, or the tasks the system needs to handle [ 109 ]. We classify CDRS according to these three different scenarios and review them below.
CDRS with side information
For this type of recommender system, it is assumed that some side information on entities is available, such as user-generated information, social information or item attributes. Collective matrix factorization (CMF) is designed for scenarios in which a user-item rating matrix and an item-attribute matrix for the same group of items are available [ 110 ]. CMF collectively factorizes these two matrixes by sharing item parameters, since the items are the same. Other methods have since been developed that exploit social network information to assist cross-domain recommender systems. Yang et al. used a bipartite graph to represent the relationships between entities across heterogeneous domains and exploit hidden similarity to help recommendations in two domains [ 111 ]. Excluding social network information, many user-generated tags in online systems provide auxiliary data for CDRS. Abel et al. used both a form-based user profile and a tag-based profile to investigate how the social web can be connected with recommender systems to assist with cross-system user modeling [ 112 ]. Tag-informed collaborative filtering (TagiCoFi) is a proposed method in which a user-item rating matrix and a user-tag matrix for the same group of users are used [ 113 ]. User similarities extracted from shared tags are used to assist the matrix factorization of the original rating matrix. Tag cross-domain CF (TagCDCF) extends TagiCoFi to two domain scenarios each containing data from these two matrixes [ 114 ]. By simultaneously integrating intra-domain and inter-domain correlations to matrix factorization, TagCDCF improves recommender system performance in the target domain.
CDRS with non-overlapping entities
Methods that handle two domains with non-overlapping entities transfer knowledge at group-level. Users and items are clustered into groups and knowledge is shared through group-level rating patterns; for example, codebook transfer (CBT) clusters users and items into groups and extracts group-level knowledge as a “codebook” [ 115 ]. A probabilistic model named rating matrix generated model (RMGM) was extended from CBT which relaxes the hard group membership to soft membership [ 116 ]. However, these two methods are unable to ensure that the information in the two groups from two different domains is consistent, and the effectiveness of the knowledge transfer is not guaranteed. Zhang et al. [ 117 ] used a domain adaptation technique to extract consistent knowledge from the source domain, which proved to be a more superior method, especially when the statistics between the source domain data and the target domain data are divergent. Zhang et al. [ 118 ] extended RMGM with an active learning strategy in a multi-domain scenario, which enables queries to be made across several domains by considering both domain-specific and domain-independent knowledge and benefits recommendation in each of these domains.
CDRS with partially or fully overlapping entities
Given the assumption that entities between two domains overlap, the source domain and target domain are bridged by constraints on the overlapping entities. Methods to handle data where the user and/or item in both domains partially or fully corresponds usually collectively factorize two matrixes in each domain by sharing some part of the factorization parameters. Transfer collective factorization (TCF) [ 119 ] has been developed to use implicit data in the source domain to help the prediction of explicit feedback, i.e., ratings in the target domain. Cross-domain triadic factorization (CDTF) models a user-item-domain tensor to integrate both explicit and implicit user feedback [ 120 ]. Users are fully overlapped, and the user factor matrix is the same, thus bridging all the domains. Cluster-based matrix factorization (CBMF) tries to boost CDTF to partially-overlapping entities [ 121 ]. Since entity correspondence is not always fully available, some strategies have been developed that match users or items in two domains. Unknown user/item mappings are identified in [ 122 ] using latent space matching. The identification of the mapping is time-consuming, so an active-learning framework is sometimes developed to identify the most valuable entity correspondences in the source domain [ 123 ]. Zhang et.al proposed a kernel-induced knowledge transfer method for cross-domain recommender systems with partially overlapped entities where alignment on heterogeneous latent feature spaces between two domains is taken into consideration [ 124 ].
The above mentioned CDRSs are mainly based on shallow learning methods. The recent developments of deep neural networks are also applied in knowledge transfer and cross-domain recommendation. A framework for CDRS on partially overlapping entities with a deep neural network is proposed in [ 125 ]. Knowledge transfer between two domains in this framework is achieved by mapping the user/item features in the target domain with the combined features obtained from both domains. Hu et al. also propose a cross-domain recommendation method by sharing the hidden layers between two domains [ 126 ]. GAN is applied with an additional objective function to discriminate user/item embedding features into different domains [ 127 ]. A general CDRS framework with a GAN is proposed in [ 128 ] to deal with all the three scenarios above. The application of deep neural networks in CDRS is well received due to their power of robust feature extraction and their capability of sharing knowledge in different levels of granularity. Knowledge is transferred through the overlapped entities as a bridge with both rating and content information and benefits both the source and the target domains in [ 129 ]. As the data are accumulated from multiple sources, further studies of CDRS that is able to deal with multi-domain knowledge transfer are needed.
Active learning in recommender systems
Each user-item correlation in a recommender system—especially one based on explicit ratings or implicit interactions between users and items—is crucial for profiling user preferences and substantially affects system performance. The challenge of data sparsity in recommendation reveals that the greater the number of ratings acquired from users, the better a system will perform in providing a recommendation. However, it is time-consuming, labour-intensive, and therefore almost impossible to query users to rate all, or most, items. Active learning has been introduced to help recommender systems select the most representative items and deliver them to users to rate [ 130 ]. As user experience is valued and user interactions with systems are desirable in the information era, active learning techniques have been adopted that improve both the efficiency and the accuracy of recommender systems.
Active strategies that used pre-computed bounds on the value of information were employed in early works to reduce the online computation time in recommender systems [ 131 ], but academics soon found that the item selection greatly influences rating prediction. There are many different active learning strategies, such as rating impact analysis [ 132 ] and bootstrapping [ 133 ], and such active learning strategies have been integrated with common recommendation models such as the aspect model [ 134 ], decision trees [ 135 ], and matrix factorization [ 136 ]. Complex factors such as naturally acquired ratings by users [ 137 ], the probability of a user being able to provide a rating for the system query [ 138 ], the influence of items [ 139 ] and the item attributes [ 140 ] have been added to the active learning strategy. The active learning strategies are also brought to a multi-domain recommendation scenario in rating selection [ 141 ] and entity correspondence selection [ 123 ].
Active learning is mostly used in the early work for item selection in recommender systems. Its combination with more advanced model-based recommendation methods may lead to novel directions. Although many factors have been considered as we reviewed above, still active learning for contextual information selection is rare. The combination of active learning and reinforcement learning is another direction that worth more attention, as its application in recommender systems will further enhance their performance.
Reinforcement learning in recommender systems
The nature of using recommender system is an interactive process between the user and the system with a series of states and action, which is in accordance with reinforcement learning. Different from traditional recommender systems, which usually focus on predicting interests of users at a specific time point, the reinforcement learning-based recommender systems aim to maximize the engagement and satisfaction of users in a long term. Under the framework of reinforcement learning, the recommender system is treated as a learning agent, the user behaviours correspond to the states and the actions are recommendations generated by the system. The reward is the feedback of the users on the recommendation results, such as the click through the rate or the time duration on the webpage. The target is to find a policy or a value function for the users to maximize the long-term rewards. The challenge of reinforcement learning lies in the large number of items that are available to users, which creates a large action space for learning agents and increases the complexity of the system.
The early work studies mainly the balance of exploration and exploitation, which is also known as bandit problems [ 142 ]. A direct implementation of MDP to recommender systems without considering the balance is proposed in [ 143 ] to recommend the next item with the previous k consumed items. Later, the trade-off between exploration and exploitation is addressed with linear reinforcement learning with theoretical guarantee [ 144 ]. There is also some work which treats the interactive process between the user and the recommender system as a multi-arm bandit problem [ 145 ] and later extended with contextual information [ 146 , 147 ].
Researches reviewed above mostly focus on the immediate rewards and ignores the long-term rewards. Recently, deep reinforcement learning has gained more attention with the breakthrough of deep Q-network and deep deterministic policy gradient, which have advantages in addressing the immediate and long-term rewards simultaneously [ 148 ]. The challenge of large and dynamic actions is tackled in [ 149 ] with Actor-Critic architecture to reduce the computational complexity. Negative feedback of the user is taken into consideration to boost deep reinforcement learning-based recommendation with a pair-wise regularization [ 150 ]. The current trend in this direction is to take into account complex user behaviours and knowledge graph information to achieve high efficiency with a large amount of data and large number of items [ 151 ]. The application of reinforcement learning techniques in industrial recommender systems is also prevalent, such as in YouTube [ 152 ] and Alibaba [ 153 ]. The development of deep reinforcement learning-based recommender systems will continue to be a hot area and will be more heavily driven by real-world industrial applications.
Fuzzy techniques in recommender systems
Item features and user behaviors in real-world recommender systems are usually subjective, incomplete and vague. Fuzzy set and fuzzy relation theories offer an effective way to deal with information uncertainty problems, and can also be adopted in recommender systems [ 154 ]. In this section, three groups of fuzzy recommendation approaches are discussed based on the classification of recommender system methods: (1) Content-based recommender systems with fuzzy techniques, (2) memory-based CF recommender systems with fuzzy techniques, and (3) model-based CF recommender systems with fuzzy techniques.
In content-based recommender systems, fuzzy techniques are applied to two phases of the process: profiling and the matching of appropriate items. Fuzzy sets are used to express the uncertainty in item features, especially vague and incomplete item descriptions, as well as the subjective user feedback on those items. Recommendation approaches are developed using fuzzy set theories to discover user preferences and create item representations [ 155 , 156 ]. As product information often takes the form of tree-structured content information, and because user preferences are vague and fuzzy, a number of fuzzy tree-based recommender systems have been developed for e-commerce [ 157 ], business-to-business e-services [ 158 ] and e-learning systems [ 158 ].
In memory-based CF recommender systems, fuzzy set theories are used to profile the uncertainty in customer preferences [ 159 ]. By matching customer interests with the service provided and managing the natural noise of uncertainty, these methods can improve accuracy in certain areas [ 160 ]. Cornelis et al. [ 161 ] extended the CF framework to make one-and-only item recommendation for personalized e-government by modeling user preferences and similarities with fuzzy relationships. Son et al. [ 162 ] used intuitionistic fuzzy recommender systems to enhance diagnoses in clinical medicine. Zhang et al. [ 163 ] built a fuzzy user-interest drift detection approach to deal with dynamic user preferences in rapidly changing big data, using fuzzy relationships to measure user-interest consistency.
Several different techniques have been applied in model-based CF recommender systems, including fuzzy network, fuzzy clustering, and fuzzy Bayesian. In fuzzy network techniques, fuzzy rules are extracted using the adaptive neuro-fuzzy inference system (ANFIS) to alleviate the data sparsity issue in CF and predict user preferences, especially for multi-criteria CF [ 164 ]. Nilashi et al. [ 165 ] used ANFIS for recommender systems with a hybrid of self-organizing map (SOM), based on several fuzzy-based distance measures and similarities. In fuzzy clustering, compared with CF methods with singular value decomposition (SVD) which only allows hard membership clustering, fuzzy C-means is a soft clustering and allows users/items to belong to several groups [ 166 ]. Xu et al. transformed user profiles by fuzzifying rating records and clustering them to exclude the noise of uncertainty to improve the accuracy and scalability of item-based CF recommender systems [ 167 ]. With regard to fuzzy Bayesian technique, Kant et al. proposed a fuzzy naïve Bayesian classifier which was extended with CF-based, reclusive-based and hybrid recommendation methods [ 168 ]. Campos et al. modeled uncertainty in the probability of related users and the description of ratings, combining Bayesian network, soft computing and CF techniques [ 169 ]. Fuzzy-based recommendation methods have also been developed for new applications. For example, a recommender system for digital libraries has been developed that suggests useful resources for researchers by using Google Wave technology and integrating fuzzy linguistic modeling [ 170 ]. In addition, Bedi et al. used fuzzy logic to measure the agreement of arguments and enhance recommendation with trust, as well as adding an explanation of the recommendation results [ 171 ].
Fuzzy techniques are well suited for handling imprecise user preference descriptions (e.g. linguistic terms), knowledge description, and the gradual accumulation of user preference profiles. A future trend is to integrate fuzzy profiling and fuzzy relationship into advanced recommendation methods, including the development of fuzzy neural networks to enhance the performance of recommender systems.
Evolutionary algorithms in recommender systems
Evolutionary algorithms (EAs) are used to combine the outputs of multiple recommendation algorithms when the recommendation is treated as a multi-objective optimization problem. They are also used to generate user/item profiles and are employed to handle ratings in the recommendation. The application of EAs in recommender systems can be broadly divided into the following three categories.
Multi-objective recommender systems
Evolutionary algorithms (EAs) are used to optimize these recommender systems by considering multiple performance indicators, e.g., accuracy, novelty and diversity [ 172 , 173 , 174 ]. To achieve accurate and diverse recommendations, Karabadji et al. [ 175 ] improved a memory-based CF method by using multi-objective optimization to find neighbors. A new probabilistic multi-objective evolutionary algorithm was proposed in [ 118 ] that strikes a good balance between accuracy and diversity, in which a new crossover operator called multi-parent probability genetic operator and a new topic diversity indicator were introduced.
Evolutionary optimization of user/item profiles
To achieve accurate personalized recommendation, Mu et al. [ 176 ] proposed a novel EA with elite population to find the information core, i.e., core users. In the proposed algorithm, an elite population with a new crossover, termed “ordered crossover”, is adopted to accelerate the evolution. To address changing user profiles in recommender systems, Rana and Jain [ 177 ] developed a dynamic recommender system that uses an evolutionary clustering algorithm to identify similar users. Chen et al. [ 178 ] proposed an interactive estimation of distribution algorithm to offer users recommendations in an interactive manner. The algorithm quantitatively expresses user preference based on human–computer interactions and trains an RBF neural network as the preference surrogate.
Evolutionary optimization of ratings
Adomavicius et al. [ 5 , 179 ] discussed how to integrate multi-criteria ratings into recommender systems. This category of algorithms engages multi-criteria ratings in recommendations, which leverages more sophisticated user preferences. Like evolutionary optimization, multi-criteria approach supports decision-making by aggregating a multi-objective optimization problem into a single-objective problem, by searching for Pareto optimal recommendations, or by taking the multiple criteria as the constraints. To handle the data sparsity problem, Hu et al. [ 65 ] utilized a genetic algorithm to optimize the weights of the domains to weight their influences within the framework called generalized cross-domain triadic factorization model over the triadic relation user-item-domain.
One future trend of EA applications will be to develop secure federated recommender systems and interactive recommender systems. Federated learning [ 180 ] is able to preserve privacy by sending model parameters to a server instead of storing data in a central server. To reduce communication overheads, it is important to reduce the number of parameters in a model, thus EAs can be used to optimize models in federated learning. Additionally, they can play an important role in creating secure recommender systems in which the model is less vulnerable to adversarial attacks, e.g., malicious manipulation of the data [ 181 ], because they can be used to generate models that are less sensitive to malicious data manipulation. Due to its capability of handling multiple objectives, new requirements can be taken into account in designing recommender systems, in addition to accuracy and diversity [ 182 ]. These requirements can also be produced from an interactive process, where EAs can be used to fulfill user requirements in each state.
Natural language processing in recommender systems
Recent developments in deep neural networks exploit the structure of natural language and vision, especially in the RNN, CNN and GNN-based methods. In addition to the reviews, we did in Sect. 4.1, the following two sections will introduce how recommender systems can benefit from natural language processing and computer vision with the integration of free text (e.g. reviews) and visual images (e.g. photo of items).
Recommender systems in the movie and star rating domains are well developed, but a huge amount of text information such as item metadata, item description text, user-generated tags or reviews is not taken into account. Many fine-grained opinion mining and topic modeling methods have already been established in natural language processing, and efforts are increasingly being made to connect these two areas to extract information from the text and incorporate it into the recommendation process. Most recommender systems benefit from review information extracted by natural language processing to complement the rating matrix and alleviate the data sparsity problem. In extreme conditions when ratings are not available, virtual ratings are generated by sentiment polarity gained from review classification [ 183 ]. Item metadata in “bag-of-words” representation are analyzed by topic models, which are integrated with matrix factorization methods to manage both cold-start and warm-start scenarios [ 184 ]. By mining feature-based product descriptions from reviews, Dong et al. enhanced recommendation with feature sentiment and product experience to provide superior products according to user query [ 185 ]. In a similar case, user expertise was evaluated and the evolution of user experience was tracked through online reviews, suggesting that similar users with an equivalent level of experience are likely to respond similarly to the same product [ 186 ].
Free-text information is still of great value even when data are not sparse. User reviews are required to discover and interpret latent user features and improve the quality of recommendation in both accuracy and transparency [ 187 ]. Ling et al. extended this method to make the learnt latent topic interpretable, thus enabling the recommendation of completely “cold” items [ 188 ]. Review text has been incorporated in cross-domain recommendation methods where user vectors are mapped through non-linear functions [ 189 ]. The neural embedding algorithm, which has recently become popular in natural language processing, has also been linked with a CF framework to infer item similarity correlations [ 190 ], and multi-level item organization has been learnt and applied to personalized ranking [ 191 ].
Previous works mostly focus on static data of reviews, text content or item descriptions. As the digital voice systems such as Siri, Google home are becoming more and more mature [ 192 ], an interactive recommender system with voice feedback is a new direction where natural language processing techniques will play an important role.
Computer vision in recommender systems
Recommender systems have benefited from the development of computer vision technologies, especially in the areas of fashion analysis and products that are highly related to visual appearance, such as clothes, jewellery, and images. The combination of image recognition and deep learning neural networks in recommender systems produces outstanding results.
One direct application is used in image recommendation. A duel-net deep network was proposed in [ 193 ] that directly applies computer vision to image recommendation to map images and user preferences. Early works in other e-commerce recommendation areas take advantage of the features extracted from images using deep neural networks and integrate them with existing methods for clothing recommendation [ 194 ]. Extended research in this area has added low-level features that mimic aspects of the human vision system, such as color characteristics, into this framework [ 195 ]. Zhao et al. integrated the visual features extracted from movie posters and still frames with a matrix factorization model to understand user preferences in movie recommendation from a new aspect [ 196 ]. Visual content has also been used in point of interest recommendations since photos and user-posted images contain large numbers of landmarks [ 197 ]. To reveal evolving fashion trends among users, He et al. modeled non-visual and visual dimensions with temporal dynamics and deep convolutional networks [ 198 ]. Jaradat proposed the transfer of knowledge between domains using two convolutional neural networks, one each for image and text, thus exploiting user preferences hidden in social media platforms such as Instagram [ 199 ].
Recommender system is required to be capable of profiling users from multimedia data, where visual information will be a significant component. Applications of multi-model fusion and multi-task learning in recommender systems are needed to comprehensively model user preferences. New functions such as cloth design and collocation are highly demanded in future fashion recommender systems.
Future directions
Current developments in recommender systems focus on providing decision support with a wide range of information related to the metadata of items, images, social networks, and user-contributed reviews. In this paper, we have reviewed the various areas of AI that relate to such systems and chronicled their development. Given that the anticipated recommendation should always meet user requirements while also gaining a better understanding of what interests a broad range of users, we identify several emerging research aspects that will benefit from future research on recommender systems.
Concept drift detection and reaction in recommender systems
Although recommender systems have achieved great success in the past, the complex and dynamic characteristics that are a feature of big data are not handled well in these systems [ 200 ]. Traditional recommender systems assume that user preference is relatively static over a period of time, so users' history records are weighted equally. However, user preferences change because of the gradual evolution of individual tastes, personal experiences or popularity-driven influences. This is a phenomenon commonly seen in Big Data streams and widely known as concept drift [ 201 ]. As a user’s history records accumulate, older records may be inconsistent with the user's new requests. Using all the available data indiscriminately jeopardizes prediction accuracy, and recommender systems that fail to take this into consideration run the risk of performance degradation.
Time-aware recommender systems were developed to address this issue [ 202 ]. Most of the methods used in time-aware recommender systems tried to accommodate user-preference drift in their models without detecting the drift. Time-window and instance decay approach determine the weights of data instances along the timeline according to the principle that old data weighs less [ 203 ]. Besides penalizing the old data, some methods used dynamic matrix factorization, in which time is considered to be one more dimension of the data [ 204 ]. However, since these methods fail to detect the change, they cannot determine the direction of the change either, resulting in bias in the proposed adaptation and weighting decay. In the big data era, methods that can manage temporal dynamics and can describe changes are required.
Long tail in recommender systems (imbalanced data)
Long-tail items are items that are unpopular and seldom noticed by users. More attention should be paid by recommender systems to long-tail items, to help users discover them. Long-tail items are noticed less by users precisely because fewer data about them are collected, which results in these items being forgotten by users and e-commerce companies. When exploited, however, long-tail items can bring huge benefits to both customers and companies [ 205 ]. Cross-domain recommender systems offer a potential means to solve the long tail item problem because of their ability to transfer knowledge from related but different data from one domain to another domain even when the data are scarce. Therefore, recommender systems for long-tail items present great opportunities for future study.
Privacy-preserving and secure recommender systems
The use of recommender systems grows widely into various application areas, which lead users to more concerns about their privacy. As a result, users are reluctant to provide authentic information and preferences when using the system, which on the other hand, impairs the performance of the recommender systems. The capability of evolutionary algorithms of covering multiple objectives enables its application in developing privacy-preserving recommender systems. One way to implement privacy by encryptions on the user profile, such as a distributed CF model with encrypted data [ 206 ]. The main concern of this method is its high computational cost. Another way is to transform user profiles and prevent the possible inference of user data. In [ 207 ] randomness is added to user data by perturbation so that privacy is preserved while keeping the accuracy of recommendation. How to preserve privacy is also studied on the CF method where similar users are clustered by data-independent hashing [ 208 ]. With more cross-platform systems developed, the development of privacy-preserving and secure recommender systems is intensively needed. The application of recommender systems in domains with high privacy risks such as healthcare or banking will prompt the development of privacy-preserving techniques.
Recommender system visualization
Many recommender systems focus on methods and accuracy but lack adequate explanation. Although the performance of recommender systems is very good, users find them difficult to trust due to opacity and privacy concerns. This is a challenging limitation in many recommender systems, especially those that are combined with complex artificial intelligence techniques such as deep learning or natural language processing.
Visualization is incorporated into recommender systems to provide a means for users to quickly and easily understand and interact with the system. Interactive and non-interactive strategies are compared in [ 209 ], illustrating how a visual interface can improve user satisfaction by providing explanatory notes. Several works have discussed possible options for visualizing and explaining the recommendation entity or process to users in traditional recommendation methods [ 210 , 211 ], but the interpretation of how a system works for hybrid methods in which AI techniques are integrated is still lacking. It is necessary for systems to include a deeper illustration of the process and enhanced user interaction so that more works on recommender system visualization can be developed in the future.
In this position paper, we review eight fields of AI, introduce their applications in recommender systems, discuss the open research issues, and give directions of possible future research on how AI techniques will be applied in recommender systems. This paper highlights how the recommender system can be enhanced by AI techniques and aims to provide guidance for researchers and practitioners in the area of recommender systems.
Shapira B, Ricci F, Kantor PB, Rokach L (2011) Recommender systems handbook. Springer, New York
MATH Google Scholar
Bobadilla J, Ortega F, Hernando A, Gutiérrez A (2013) Recommender systems survey. Knowl Based Syst 46:109–132
Google Scholar
Ben Schafer J, Konstan J, Riedl J (1999) Recommender systems in e-commerce. In: Proceedings of the 1st ACM Conference on Electronic Commerce, 1999, pp 158–166
Lu J, Wu D, Mao M, Wang W, Zhang G (2015) Recommender system application developments: a survey. Decis Support Syst 74:12–32
Adomavicius G, Tuzhilin A (2005) Toward the next generation of recommender systems: a survey of the state-of-the-art and possible extensions. IEEE Trans Knowl Data Eng 17(6):734–749
Burke R (2002) Hybrid recommender systems: survey and experiments. User Model User-adapt Interact 12(4):331–370
Shardanand U, Maes P (1995) Social information filtering: algorithms for automating ‘word of mouth’. In: Proceedings of the SIGCHI Conference on Human Factors in Computing Systems, 1995, pp 210–217
Salton G, Wong A, Yang C-S (1975) A vector space model for automatic indexing. Commun ACM 18(11):613–620
Sebastiani F (2002) Machine learning in automated text categorization. ACM Comput Surv 34(1):1–47
Herlocker JL, Konstan JA, Terveen LG, Riedl JT (2004) Evaluating collaborative filtering recommender systems. ACM Trans Inf Syst 22(1):5–53
Lops P, De Gemmis M, Semeraro G (2011) Content-based recommender systems: state of the art and trends. Recommender systems handbook. Springer, Berlin, pp 73–105
Shambour Q, Lu J (2012) A trust-semantic fusion-based recommendation approach for e-business applications. Decis Support Syst 54(1):768–780
Balabanović M, Shoham Y (1997) Fab: content-based, collaborative recommendation. Commun ACM 40(3):66–72
Resnick P, Iacovou N, Suchak M, Bergstrom P, Riedl J (1994) GroupLens: an open architecture for collaborative filtering of netnews. In: Proceedings of the 1994 ACM Conference on Computer Supported Cooperative Work, 1994, pp 175–186
Linden G, Smith B, York J (2003) Amazon.com recommendations: item-to-item collaborative filtering. IEEE Internet Comput 7(1):76–80
Liu H, Hu Z, Mian A, Tian H, Zhu X (2014) A new user similarity model to improve the accuracy of collaborative filtering. Knowl Based Syst 56:156–166
Hu Y, Zhang D, Ye J, Li X, He X (2013) Fast and accurate matrix completion via truncated nuclear norm regularization. IEEE Trans Pattern Anal Mach Intell 35(9):2117–2130
Su X, Khoshgoftaar TM (2009) A survey of collaborative filtering techniques. Adv Artif Intell 2009:1–19
Deshpande M, Karypis G (2004) Item-based top-n recommendation algorithms. ACM Trans Inf Syst 22(1):143–177
Shi Y, Larson M, Hanjalic A (2014) Collaborative filtering beyond the user-item matrix: a survey of the state of the art and future challenges. ACM Comput Surv 47(1):3
Koren Y, Bell R, Volinsky C (2009) Matrix factorization techniques for recommender systems. Computer 42(8):30–37
Luo X, Zhou M, Li S, You Z, Xia Y, Zhu Q (2016) A nonnegative latent factor model for large-scale sparse matrices in recommender systems via alternating direction method. IEEE Trans Neural Netw Learn Syst 27(3):579–592
MathSciNet Google Scholar
Liu B, Xiong H, Papadimitriou S, Fu Y, Yao Z (2015) A general geographical probabilistic factor model for point of interest recommendation. IEEE Trans Knowl Data Eng 27(5):1167–1179
Smyth B (2007) Case-based recommendation. The adaptive web. Springer, Berlin, pp 342–376
Aamodt A, Plaza E (1994) Case-based reasoning: foundational issues, methodological variations, and system approaches. AI Commun 7(1):39–59
Felfernig A, Friedrich G, Jannach D, Zanker M (2011) Developing constraint-based recommenders. Recommender systems handbook. Springer, Berlin, pp 187–215
Felfernig A, Burke R (2008) Constraint-based recommender systems: technologies and research issues. In: Proceedings of the 10th International Conference on Electronic Commerce, 2008, p 3
Luger GF (2005) Artificial intelligence: structures and strategies for complex problem solving. Pearson Education, London
Russell SJ, Norvig P (2016) Artificial intelligence: a modern approach. Pearson Education Limited, Malaysia
LeCun Y, Bengio Y (1995) Convolutional networks for images, speech, and time series. Handb Brain Theor Neural Netw 3361(10):1995
Lecun Y, Bengio Y, Hinton G (2015) Deep learning. Nature 521(7553):436–444
Goodfellow I, Bengio Y, Courville A (2016) Deep learning. MIT press, Cambridge
Murtagh F (1991) Multilayer perceptrons for classification and regression. Neurocomputing 2(5–6):183–197
Wang Y, Yao H, Zhao S (2016) Auto-encoder based dimensionality reduction. Neurocomputing 184:232–242
Lawrence S, Giles CL, Tsoi AC, Back AD (1997) Face recognition: a convolutional neural-network approach. IEEE Trans Neural Netw 8(1):98–113
Rumelhart DE, Hinton GE, Williams RJ (1986) Learning representations by back-propagating errors. Nature 323(6088):533–536
Hochreiter S, Schmidhuber J (1997) LSTM can solve hard long time lag problems. Advances in neural information processing systems. MIT Press, Cambridge, pp 473–479
Goodfellow I et al (2014) Generative adversarial nets. Advances in neural information processing systems. MIT Press, Cambridge, pp 2672–2680
Zhou J et al (2018) Graph neural networks: a review of methods and applications. arXiv Prepr. arXiv1812.08434
Lu J, Zuo H, Zhang G (2019) Fuzzy multiple-source transfer learning. IEEE Trans. Fuzzy Syst
Lu J, Behbood V, Hao P, Zuo H, Xue S, Zhang G (2015) Transfer learning using computational intelligence: a survey. Knowl Based Syst 80:14–23
Kang Z, Grauman K, Sha F (2011) Learning with whom to share in multi-task feature learning. In: The 28th International Conference on Machine Learning, pp 521–528
Arnold A, Nallapati R, Cohen WW (2007) A comparative study of methods for transductive transfer learning. In: The 7th IEEE International Conference on Data Mining Workshops, 2007, pp 77–82
Lu J, Xuan J, Zhang G, Luo X (2018) Structural property-aware multilayer network embedding for latent factor analysis. Pattern Recogn 76:228–241
Zhu X, Lafferty J, Rosenfeld R (2005) Semi-supervised learning with graphs. Carnegie Mellon University, Language Technologies Institute, School of Computer Science, Pittsburgh
Aghdam HH, Gonzalez-Garcia A, van de Weijer J, López AM (2019) Active learning for deep detection neural networks. In: Proceedings of the IEEE International Conference on Computer Vision, 2019, pp 3672–3680
Settles B (2011) From theories to queries: active learning in practice. In: Active Learning and Experimental Design workshop in conjunction with AISTATS 2010, 2011, pp 1–18
Settles B (2010) Active learning literature survey. University of California, Santa Cruz
Sutton RS, Barto AG (2011) Reinforcement learning: An introduction. MIT Press, Cambridge
Peng et al P (2017) Multiagent bidirectionally-coordinated nets: emergence of human-level coordination in learning to play starcraft combat games. arXiv Prepr. arXiv1703.10069
Bai W, Li T, Tong S (2020) NN reinforcement learning adaptive control for a class of nonstrict-feedback discrete-time systems. IEEE Trans Cybern
Hüttenrauch M, Adrian S, Neumann G (2019) Deep reinforcement learning for swarm systems. J Mach Learn Res 20(54):1–31
MathSciNet MATH Google Scholar
Neftci EO, Averbeck BB (2019) Reinforcement learning in artificial and biological systems. Nat Mach Intell 1(3):133–143
Botvinick M, Ritter S, Wang JX, Kurth-Nelson Z, Blundell C, Hassabis D (2019) Reinforcement learning, fast and slow. Trends Cogn Sci 23(5):408–422
Bellman R (1957) A Markovian decision process. J Math Mech 679–684
Henderson P, Islam R, Bachman P, Pineau J, Precup D, Meger D (2017) Deep reinforcement learning that matters. arXiv Prepr. arXiv1709.06560
Kaelbling LP, Littman ML, Moore AW (1996) Reinforcement learning: a survey. J Artif Intell Res 4:237–285
Mnih V et al (2015) Human-level control through deep reinforcement learning. Nature 518(7540):529–533
Silver D et al (2016) Mastering the game of Go with deep neural networks and tree search. Nature 529(7587):484–489
Tran L, Duckstein L (2002) Comparison of fuzzy numbers using a fuzzy distance measure. Fuzzy Sets Syst 130(3):331–341
Roubos JA, Setnes M, Abonyi J (2003) Learning fuzzy classification rules from labeled data. Inf Sci (Ny) 150(1–2):77–93
Chen S-M, Wang C-Y (2013) Fuzzy decision making systems based on interval type-2 fuzzy sets. Inf Sci (Ny) 242:1–21
Holland JH (1975) Adaption in natural and artificial systems
Beyer H-G, Beyer H-G, Schwefel H-P, Schwefel H-P (2002) Evolution strategies: a comprehensive introduction. Nat Comput 1(1):3–52
Koza JR (1992) Genetic programming: on the programming of computers by means of natural selection. The MIT Press, Cambridge
Larrañaga P, Lozano JA (2001) Estimation of distribution algorithms: a new tool for evolutionary computation, vol 2. Springer Science & Business Media, Berlin
Storn R, Price K (1997) Differential evolution: a simple and efficient heuristic for global optimization over continuous spaces. J Glob Optim 11(4):341–359
Eberhart R, Kennedy J (1995) A new optimizer using particle swarm theory. In: Proceedings of the 6th International Symposium on Micro Machine and Human Science, 1995, pp 39–43
Dorigo M, Gambardella LM (1997) Ant colony system: a cooperative learning approach to the traveling salesman problem. IEEE Trans Evol Comput 1(1):53–66
Miettinen K (1999) Nonlinear multiobjective optimization. Kluwer Academic Publishers, Dordrecht
Li B, Li J, Tang K, Yao X (2015) Many-objective evolutionary algorithms: a survey. ACM Comput Surv 48(1):1–35
Chowdhary KR (2020) Natural language processing. Fundamentals of artificial intelligence. Springer, Berlin, pp 603–649
Chien J-T (2019) Deep Bayesian natural language processing. In: Proceedings of the 57th Annual Meeting of the Association for Computational Linguistics: Tutorial Abstracts, 2019, pp 25–30
Collobert R, Weston J, Bottou L, Karlen M, Kavukcuoglu K, Kuksa P (2011) Natural language processing (almost) from scratch. J Mach Learn Res 12:2493–2537
Zhang W, Yoshida T, Tang X (2008) Text classification based on multi-word with support vector machine. Knowl Based Syst 21(8):879–886
Yi J, Nasukawa T, Bunescu R, Niblack W (2003) Sentiment analyzer: Extracting sentiments about a given topic using natural language processing techniques. In: Third IEEE international conference on data mining, 2003, pp 427–434
Blei DM, Ng AY, Jordan MI (2003) Latent dirichlet allocation. J. Mach. Learn. Res. 3:993–1022
Forsyth DA, Ponce J (2002) Computer vision: a modern approach. Prentice Hall Professional Technical Reference, Upper Saddle River
Voulodimos A, Doulamis N, Doulamis A, Protopapadakis E (2018) Deep learning for computer vision: a brief review. Comput Intell Neurosci 2018:7068349
Khan S, Rahmani H, Shah SAA, Bennamoun M (2018) A guide to convolutional neural networks for computer vision. Synth Lect Comput Vis 8(1):1–207
Salakhutdinov R, Mnih A, Hinton G (2007) Restricted Boltzmann machines for collaborative filtering. In: Proceedings of the 24th International Conference on Machine Learning, 2007, pp 791–798
Truyen TT, Phung DQ, Venkatesh S (2009) Ordinal Boltzmann machines for collaborative filtering. In: Proceedings of the 25th Conference on Uncertainty in Artificial Intelligence, 2009, pp 548–556
Zhang S, Yao L (2017) Deep learning based recommender system: a survey and new perspectives. ACM J Comput Cult Herit Artic 1(35):1–35
Cheng et al. HT (2016) Wide and deep learning for recommender systems. arXiv Prepr. pp 1–4
Guo H, Tang R, Ye Y, Li Z, He X (2017) DeepFM: a factorization-machine based neural network for CTR prediction. In: International Joint Conference on Artificial Intelligence, 2017, pp 1725–1731
He X, Liao L, Zhang H, Nie L, Hu X, Chua TS (2017) Neural collaborative filtering. In: Proceedings of the 26th International Conference on World Wide Web, 2017, pp 173–182
Sedhain S, Menon AK, Sanner S, Xie L (2015) AutoRec: autoencoders meet collaborative filtering. In: Proceedings of the 24th International Conference on World Wide Web, 2015, pp 111–112
Zhang S, Yao L, Xu X (2017) AutoSVD++: an efficient hybrid collaborative filtering model via contractive auto-encoders. In: Proceedings of the 40th International ACM SIGIR Conference on Research and Development in Information Retrieval, 2017, pp 2–5
Strub F, Gaudel R, Mary J (2016) Hybrid recommender system based on autoencoders. In: Proceedings of the 1st Workshop on Deep Learning for Recommender Systems, 2016, pp 1–5
Diao Q, Qiu M, Wu CY, Smola AJ, Jiang J, Wang C (2014) Jointly modeling aspects, ratings and sentiments for movie recommendation. In: Proceedings of the 20th ACM SIGKDD International Conference on Knowledge Discovery and Data Mining, 2014, pp 193–202
Kim D, Park C, Oh J, Lee S, Yu H (2016) Convolutional matrix factorization for document context-aware recommendation. In: RecSys 2016—Proceedings of the 10th ACM Conference on Recommender Systems, 2016, pp 233–240
Yuyun G, Qi Z (2016) Hashtag recommendation using attention-based convolutional neural network. In: International Joint Conference on Artificial Intelligence, 2016, pp 2782–2788
Dai H, Wang Y, Trivedi R, Song L (2016) Recurrent coevolutionary feature embedding processes for recommendation. In: Proceedings of the 1st Workshop on Deep Learning for Recommender Systems, 2016, pp 1–11
Wu CY, Ahmed A, Beutel A, Smola AJ, Jing H (2017) Recurrent recommender networks. In: Proceedings of the 10th ACM International Conference on Web Search and Data Mining, 2017, pp 495–503
Jing H, Smola AJ (2017) Neural survival recommender. In: Proceedings of the 10th ACM International Conference on Web Search and Data Mining, 2017, pp 515–524
Wang S, Hu L, Wang Y, Cao L, Sheng QZ, Orgun M (2019) Sequential recommender systems: challenges, progress and prospects. arXiv Prepr. arXiv2001.04830
Hidasi B, Karatzoglou A, Baltrunas L, Tikk D (2016) Session-based recommendations with recurrent neural networks. In: 4th Int. Conf. Learn. Represent, pp 1–10, 2016
Wu S, Ren W, Yu C, Chen G, Zhang D, Zhu J (2016) Personal recommendation using deep recurrent neural networks in NetEase. In: Proceeding of the 32nd International Conference on Data Engineering, 2016, pp 1218–1229
Li J, Ren R, Chen Z, Ren Z, Lian T, Ma J (2017) Neural attentive session-based recommendation. In: Int. Conf. Inf. Knowl. Manag. Proc., vol. Part F1318, pp 1419–1428, 2017
Liu Q, Zeng Y, Mokhosi R, Zhang H (2018) STAMP: Short-term attention/memory priority model for session-based recommendation. In: Proceedings of the 24th ACM SIGKDD International Conference on Knowledge Discovery and Data Mining, 2018, pp. 1831–1839
Ying H et al. (2018) Sequential recommender system based on hierarchical attention network. In: International Joint Conference on Artificial Intelligence, 2018.
Wang J et al. (2017) IRGAN: a minimax game for unifying generative and discriminative information retrieval models. In: Proceedings of the 40th International ACM SIGIR Conference on Research and Development in Information Retrieval, 2017, pp 515–524
He X, He Z, Du X, Chua TS (2018) Adversarial personalized ranking for recommendation. In: The 41st International ACM SIGIR Conference on Research & Development in Information Retrieval, 2018, pp 355–364
Yang D, Guo Z, Wang Z, Jiang J, Xiao Y, Wang W (2018) A knowledge-enhanced deep recommendation framework incorporating GAN-based models. In: 2018 IEEE International Conference on Data Mining, 2018, pp 1368–1373
Tang J, Du X, He X, Yuan F, Tian Q, Chua T-S (2019) Adversarial training towards robust multimedia recommender system. IEEE Trans Knowl Data Eng 32(5):855–867
Ying R, He R, Chen K, Eksombatchai P, Hamilton WL, Leskovec J (2018) Graph convolutional neural networks for web-scale recommender systems. In: Proceedings of the 24th ACM SIGKDD International Conference on Knowledge Discovery and Data Mining, 2018, pp 974–983
Yin R, Li K, Zhang G, Lu J (2019) A deeper graph neural network for recommender systems. Knowl Based Syst 185:105020
Wu S, Tang Y, Zhu Y, Wang L, Xie X, Tan T (2019) Session-based recommendation with graph neural Networks. In: Proceedings of the AAAI Conference on Artificial Intelligence, 2019, vol. 33, pp 346–353
Cantador I, Fernández-Tobías I, Berkovsky S, Cremonesi P (2015) Cross-domain recommender systems. Recommender systems handbook. Springer, Berlin, pp 919–959
Singh AP, Gordon GJ (2008) Relational learning via collective matrix factorization. In: Proceedings of the 14th ACM SIGKDD International Conference on Knowledge Discovery and Data Mining, 2008, pp 650–658
Yang D, He J, Qin H, Xiao Y, Wang W (2015) A graph-based recommendation across heterogeneous domains, In; Proceedings of the 24th ACM International on Conference on Information and Knowledge Management, 2015, pp 463–472
Abel F, Herder E, Houben G-J, Henze N, Krause D (2013) Cross-system user modeling and personalization on the social web. User Model. User-adapt. Interact, pp 1–41
Zhen Y, Li WJ, Yeung DY (2009) TagiCoFi: Tag informed collaborative filtering. In: RecSys’09—Proceedings of the 3rd ACM Conference on Recommender Systems, 2009, pp 69–76
Hao P, Zhang G, Martinez L, Lu J (2017) Regularizing knowledge transfer in recommendation with tag-inferred correlation. IEEE Trans Cybern
Li B, Yang Q, Xue X (2009) Can movies and books collaborate? Cross-domain collaborative filtering for sparsity reduction. In: Proceedings of the 21st International Joint Conference on Artificial Intelligence, 2009, vol 9, pp 2052–2057
Li B, Yang Q, Xue X (2009) Transfer learning for collaborative filtering via a rating-matrix generative model. In: Proceedings of the 26th International Conference on Machine Learning, ICML 2009, 2009, pp 617–624
Zhang Q, Wu D, Lu J, Liu F, Zhang G (2017) A cross-domain recommender system with consistent information transfer. Decis Support Syst 104:49–63
Zhang Y, Cao B, Yeung DY (2010) Multi-domain collaborative filtering. In: Proceedings of the 26th Conference on Uncertainty in Artificial Intelligence, 2010, pp 725–732
Pan W, Yang Q (2013) Transfer learning in heterogeneous collaborative filtering domains. Artif Intell 197:39–55
Hu L, Cao J, Xu G, Cao L, Gu Z, Zhu C (2013) Personalized recommendation via cross-domain triadic factorization. In: Proceedings of the 22nd International Conference on World Wide Web, 2013, pp 595–606
Mirbakhsh N, Ling CX (2015) Improving top-n recommendation for cold-start users via cross-domain information. ACM Trans Knowl Discov Data 9(4):33
Li CY, Lin SD (2014) Matching users and items across domains to improve the recommendation quality. In: Proceedings of the 20th ACM SIGKDD International Conference on Knowledge Discovery and Data Mining, 2014, pp 801–810
Zhao L, Pan SJ, Yang Q (2017) A unified framework of active transfer learning for cross-system recommendation. Artif Intell 245:38–55
Zhang Q, Lu J, Wu D, Zhang G (2019) A cross-domain recommender system with kernel-induced knowledge transfer for overlapping entities. IEEE Trans Neural Netw Learn Syst 30(7):1998–2012
Zhu F, Wang Y, Chen, Liu G, Orgun M, Wu (2018) A deep framework for cross-domain and cross-system recommendations. In: IJCAI International Joint Conference on Artificial Intelligence, 2018
Hu G, Zhang Y, Yang Q (2018) Conet: collaborative cross networks for cross-domain recommendation. In: Proceedings of the 27th ACM International Conference on Information and Knowledge Management, 2018, pp 667–676
Wang C, Niepert M, Li H (2019) Recsys-dan: discriminative adversarial networks for cross-domain recommender systems. IEEE Trans Neural Networks Learn Syst
Yuan F, Yao L, Benatallah B (2019) DARec: Deep domain adaptation for cross-domain recommendation via transferring rating patterns. In: Proceedings of the Twenty-Eighth International Joint Conference on Artificial Intelligence, 2019
Zhu F, Chen C, Wang Y, Liu G, Zheng X (2019) Dtcdr: a framework for dual-target cross-domain recommendation. In: Proceedings of the 28th ACM International Conference on Information and Knowledge Management, 2019, pp 1533–1542
Elahi M, Ricci F, Rubens N (2016) A survey of active learning in collaborative filtering recommender systems. Comput Sci Rev 20:29–50
Boutilier C, Zemel RS, Marlin B (2003) Active collaborative filtering. In: Proceedings of the 19th Conference on Uncertainty in Artificial Intelligence, 2003, pp 98–106
Mello CE, Aufaure MA, Zimbrao G (2010) Active learning driven by rating impact analysis. In: Proceedings of the 4th ACM Conference on Recommender Systems, 2010, pp 341–344
Golbandi N, Koren Y, Lempel R (2010) On bootstrapping recommender systems. In: Proceedings of the 19th ACM International Conference on Information and Knowledge Management, 2010, pp 1805–1808
Karimi R, Freudenthaler C, Nanopoulos A, Schmidt-Thieme L (2011) Active learning for aspect model in recommender systems. In: IEEE Symposium on Computational Intelligence and Data Mining, 2011, pp 162–167
Golbandi N, Koren Y, Lempel R (2011) Adaptive bootstrapping of recommender systems using decision trees. In: Proceedings of the 4th ACM International Conference on Web Search and Data Mining, 2011, pp 595–604
Karimi R, Freudenthaler C, Nanopoulos A, Schmidt-Thieme L (2011) Non-myopic active learning for recommender systems based on matrix factorization. In: IEEE International Conference on Information Reuse & Integration, 2011, pp 299–303
Wiesner M, Pfeifer D (2010) Adapting recommender systems to the requirements of personal health record systems. In: Proceedings of the 1st ACM International Health Informatics Symposium, 2010, pp 410–414
Elahi M, Ricci F, Rubens N (2012) Adapting to natural rating acquisition with combined active learning strategies. In: International Symposium on Methodologies for Intelligent Systems, 2012, pp 254–263
Rubens N, Sugiyama M (2007) Influence-based collaborative active learning. In: Proceedings of the 1st ACM Conference on Recommender Systems, 2007, pp 145–148
He L, Liu NN, Yang Q (2011) Active dual collaborative filtering with both item and attribute feedback. In: Proceedings of the National Conference on Artificial Intelligence, 2011, vol. 2, pp 1186–1191
Zhang Z, Jin X, Li L, Ding G, Yang Q (2016) Multi-domain active learning for recommendation. In: AAAI, 2016, pp 2358–2364
Berry DA, Fristedt B (1985) Bandit problems: sequential allocation of experiments (Monographs on statistics and applied probability). London Chapman Hall 5(71–87):7
Shani G, Heckerman D, Brafman RI (2005) An MDP-based recommender system. J Mach Learn Res 6:1265–1295
Warlop R et al (2018) Fighting boredom in recommender systems with linear reinforcement learning. No. NeurIPS, 2018
Wang H, Wu Q, Wang H (2017) Factorization bandits for interactive recommendation. AAAI 17:2695–2702
Li L, Chu W, Langford J, Schapire RE (2010) A contextual-bandit approach to personalized news article recommendation. In: Proc. 19th Int. Conf. World Wide Web, pp. 661–670, 2010
Zeng C, Wang Q, Mokhtari S, Li T (2016) Online context-aware recommendation with time varying multi-armed bandit. In: Proceedings of the 22nd ACM SIGKDD International Conference on Knowledge Discovery and Data Mining, 2016, pp 2025–2034
Zheng G et al (2018) DRN: a deep reinforcement learning framework for news recommendation. Proc World Wide Web Conf 2:167–176
Zhao X, Xia L, Zhang L, Ding Z, Yin D, Tang J (2018) Deep reinforcement learning for page-wise recommendations. In: 12th ACM Conf Recomm Syst, pp 95–103, 2018
Zhao X, Xia L, Zhang L, Tang J, Ding Z, Yin D (2018) Recommendations with negative feedback via pairwise deep reinforcement learning. In: Proc. ACM SIGKDD Int. Conf. Knowl. Discov. Data Min., pp. 1040–1048, 2018
Zhou S et al (2020) Interactive recommender system via knowledge graph-enhanced reinforcement learning. pp 179–188
Ie E et al (2019) SLateq: a tractable decomposition for reinforcement learning with recommendation sets. In: Int Jt Conf Artif Intell, vol. 2019-Augus, pp 2592–2599, 2019
Hu Y, Da Q, Zeng A, Yu Y, Xu Y (2018) Reinforcement learning to rank in E-commerce search engine: Formalization, analysis, and application. In: Proc. ACM SIGKDD Int. Conf. Knowl. Discov. Data Min., pp 368–377, 2018
Chung F, Rhee H (2007) “Uncertain fuzzy clustering: insights and recommendations. IEEE Comput Intell Mag 2(1):44–56
Yager RR (2003) Fuzzy logic methods in recommender systems. Fuzzy Sets Syst 136(2):133–149
Zenebe A, Zhou L, Norcio AF (2010) User preferences discovery using fuzzy models. Fuzzy Sets Syst 161(23):3044–3063
Mao M, Lu J, Zhang G, Zhang J (2015) A fuzzy content matching-based e-commerce recommendation approach. In: IEEE International Conference on Fuzzy Systems, 2015
Wu D, Zhang G, Lu J (2015) A fuzzy preference tree-based recommender system for personalized business-to-business e-services. IEEE Trans Fuzzy Syst 23(1):29–43
Zhang Z, Lin H, Liu K, Wu D, Zhang G, Lu J (2013) A hybrid fuzzy-based personalized recommender system for telecom products/services. Inf Sci (Ny) 235:117–129
Yera R, Castro J, Martínez L (2016) A fuzzy model for managing natural noise in recommender systems. Appl Soft Comput J 40:187–198
Cornelis C, Lu J, Guo X, Zhang G (2007) One-and-only item recommendation with fuzzy logic techniques. Inf Sci (Ny) 177(22):4906–4921
Son LH, Thong NT (2015) Intuitionistic fuzzy recommender systems: an effective tool for medical diagnosis. Knowl Based Syst 74:133–150
Zhang Q, Wu D, Zhang G, Lu J (2016) Fuzzy user-interest drift detection based recommender systems. In: International Conference on Fuzzy Systems, 2016, pp 1274–1281
Nilashi M, Bin-Ibrahim O, Ithnin N (2014) “Multi-criteria collaborative filtering with high accuracy using higher order singular value decomposition and neuro-fuzzy system. Knowl Based Syst 60:82–101
Nilashi M, Bin-Ibrahim O, Ithnin N (2014) Hybrid recommendation approaches for multi-criteria collaborative filtering. Expert Syst Appl 41(8):3879–3900
Treerattanapitak K, Jaruskulchai C (2012) Exponential fuzzy C-means for collaborative filtering. J Comput Sci Technol 27(3):567–576
Xu S, Watada J (2014) A method for hybrid personalized recommender based on clustering of fuzzy user profiles. In: IEEE International Conference on Fuzzy Systems, 2014, pp 2171–2177
Kant V, Bharadwaj KK (2013) Integrating collaborative and reclusive methods for effective recommendations: a fuzzy Bayesian approach. Int J Intell Syst 28(11):1099–1123
de Campos LM, Fernández-Luna JM, Huete JF (2008) A collaborative recommender system based on probabilistic inference from fuzzy observations. Fuzzy Sets Syst 159(12):1554–1576
Serrano-Guerrero J, Herrera-Viedma E, Olivas JA, Cerezo A, Romero FP (2011) A Google wave-based fuzzy recommender system to disseminate information in University Digital Libraries 2.0. Inf Sci (Ny) 181(9):1503–1516
Bedi P, Vashisth P (2014) Empowering recommender systems using trust and argumentation. Inf Sci (Ny) 279(22):569–586
Zhang X, Duan F, Zhang L, Cheng F, Jin Y, Tang K (2017) Pattern recommendation in task-oriented applications: a multi-objective perspective. IEEE Computational Intelligence Magazine, vol. 12, no. 3, IEEE, pp 43–53, 2017
Ribeiro MT, Lacerda A, Veloso A, Ziviani N (2012) Pareto-efficient hybridization for multi-objective recommender systems. In: Proceedings of the 6th ACM Conference on Recommender Systems, 2012, pp 19–26
Rodriguez M, Posse C, Zhang E (2012) Multiple objective optimization in recommender systems. In: Proceedings of the 6th ACM Conference on Recommender Systems, 2012, pp 11–18
Karabadji NEI, Beldjoudi S, Seridi H, Aridhi S, Dhifli W (2018) Improving memory-based user collaborative filtering with evolutionary multi-objective optimization. Expert Syst Appl 98:153–165
Mu C, Jiao L, Liu Y, Li Y (2015) Multiobjective nondominated neighbor coevolutionary algorithm with elite population. Soft Comput 19(5):1329–1349
Rana C, Jain SK (2015) A study of the dynamic features of recommender systems. Artif Intell Rev 43(1):141–153
Chen Y, Sun X, Gong D, Zhang Y, Choi J, Klasky S (2017) Personalized search inspired fast interactive estimation of distribution algorithm and its application. IEEE Trans Evol Comput 21(4):588–600
Adomavicius G, Kwon Y (2015) Multi-criteria recommender systems. Recommender systems handbook. Springer, Berlin, pp 847–880
Konečný J, McMahan HB, Yu FX, Richtárik P, Suresh AT, Bacon D (2016) Federated learning: strategies for improving communication efficiency. arXiv Prepr. arXiv1610.05492
Huang L, Joseph AD, Nelson B, Rubinstein BIP, Tygar JD (2011) Adversarial machine learning. In: Proceedings of the 4th ACM Workshop on Security and Artificial Intelligence, 2011, pp 43–58
Zhu H, Jin Y (2020) Multi-objective evolutionary federated learning. IEEE Trans Neural Netw Learn Syst 31(4):1310–1322
Zhang W, Ding G, Chen L, Li C, Zhang C (2013) Generating virtual ratings from chinese reviews to augment online recommendations. ACM Trans Intell Syst Technol 4(1):1–17
Agarwal D, Chen BC (2010) fLDA: matrix factorization through latent Dirichlet allocation. In: Proceedings of the 3rd ACM International Conference on Web Search and Data Mining, 2010, pp 91–100
Dong R, Schaal M, O’Mahony MP, McCarthy K, Smyth B (2013) Sentimental product recommendation. In: Proceedings of the 7th ACM Conference on Recommender Systems, 2013, pp 44–58
McAuley J, Leskovec J (2013) From amateurs to connoisseurs: modeling the evolution of user expertise through online reviews. In: Proc. 22nd Int. Conf. World Wide Web, pp 897–908
McAuley J, Leskovec J (2013) Hidden factors and hidden topics: understanding rating dimensions with review text. In: Proceedings of the 7th ACM Conference on Recommender Systems, 2013, pp 165–172
Ling G, Lyu MR, King I (2014) Ratings meet reviews, a combined approach to recommend. In: Proceedings of the 8th ACM Conference on Recommender Systems, 2014, pp 105–112
Xin X, Liu Z, Lin CY, Huang H, Wei X, Guo P (2015) Cross-domain collaborative filtering with review text. In: International Joint Conference on Artificial Intelligence, 2015, pp 1827–1834
Barkan O, Noam K (2016) Item2vec: neural item embedding for CF. In: IEEE 26th International Workshop on Machine Learning for Signal Processing, 2016, pp 1–6
Sun Z, Yang J, Zhang J, Bozzon A, Chen Y, Xu C (2017) MRLR: multi-level representation learning for personalized ranking in recommendation. In: International Joint Conference on Artificial Intelligence, 2017, pp 2807–2813
Iovine A, Narducci F, Semeraro G (2020) Conversational recommender systems and natural language: a study through the ConveRSE framework. Decis Support Syst 131:113250
Lei C, Liu D, Li W, Zha ZJ, Li H (2016) Comparative deep learning of hybrid representations for image recommendations. In: Proceedings of the IEEE Conference on Computer Vision and Pattern Recognition, 2016, pp 2545–2553
He R, McAuley J (2015) VBPR: visual bayesian personalized ranking from implicit feedback. In: AAAI, 2015, pp 144–150
Gaspar P (2017) User preferences analysis using visual stimuli. In: Proceedings of the 11th ACM Conference on Recommender Systems, 2017, pp 436–440
Zhao L, Lu Z, Pan SJ, Yang Q (2016) Matrix factorization+ for movie recommendation. In: International Joint Conference on Artificial Intelligence, 2016, pp 3945–3951
Wang S, Wang Y, Tang J, Shu K, Ranganath S, Liu H (2017) What your images reveal: exploiting visual contents for point-of-interest recommendation. In: Proceedings of the 26th International Conference on World Wide Web, 2017, pp 391–400
He R, McAuley J (2016) Ups and downs: modeling the visual evolution of fashion trends with one-class collaborative filtering. In: Proceedings of the 25th International Conference on World Wide Web, 2016, pp 507–517
Jaradat S (2017) Deep cross-domain fashion recommendation. In: Proceedings of the 11th ACM Conference on Recommender Systems, 2017, pp 407–410
Lu J, Liu A, Song Y, Zhang G (2020) Data-driven decision support under concept drift in streamed big data. Complex Intell Syst 6(1):157–163
Harries M, Horn K (1995) Detecting concept drift in financial time series prediction using symbolic machine learning. In: Proceedings of the 8th Australian Joint Conference on Artificial Intelligence, 1995, pp 91–98
Campos PG, Díez F, Cantador I (2014) Time-aware recommender systems: a comprehensive survey and analysis of existing evaluation protocols. User Model User-Adapt Interact 24(1–2):67–119
Yin H, Cui B, Chen L, Hu Z, Zhou X (2015) Dynamic user modeling in social media systems. ACM Trans Inf Syst 33(3):10
Chua FCT, Oentaryo RJ, Lim EP (2013) Modeling temporal adoptions using dynamic matrix factorization. In: Proceedings of IEEE International Conference on Data Mining, 2013, pp 91–100
Yin H, Cui B, Li J, Yao J, Chen C (2012) Challenging the long tail recommendation. In: Proceedings of the VLDB Endowment, 2012, vol 5, no 9, pp 896–907
Canny J (2002) Collaborative filtering with privacy. In: Proc. IEEE Symp. Secur. Priv., vol. 2002-Jan, pp 45–57, 2002
Kikuchi H, Mochizuki A (2013) Privacy-preserving collaborative filtering using randomized response. J Inf Process 21(4):617–623
Chow R, Pathak MA, Wang C (2012) A practical system for privacy-preserving collaborative filtering. In: Proc. 12th IEEE Int. Conf. Data Min. Work. ICDMW 2012, pp 547–554, 2012
Bostandjiev S, O’Donovan J, Höllerer T (2012) TasteWeights: a visual interactive hybrid recommender system. In: Proceedings of the sixth ACM conference on Recommender systems, 2012, pp 35–42
Wang W, Zhang G, Lu J (2017) Hierarchy visualization for group recommender systems. In: IEEE Trans Syst Man Cybern Syst, pp 1–12, 2017
Hernando A, Moya R, Ortega F, Bobadilla J (2014) Hierarchical graph maps for visualization of collaborative recommender systems. J Inf Sci 40(1):97–106
Download references
Acknowledgements
The work presented in this paper was supported by the Australian Research Council (ARC) under the Australian Laureate Fellowship [FL190100149] and the UTS Distinguished Visiting Scholars (DVS) Scheme.
Author information
Authors and affiliations.
Decision Systems and e-Service Intelligence Laboratory, Australian Artificial Intelligence Institute, University of Technology Sydney, Sydney, NSW, 2007, Australia
Qian Zhang & Jie Lu
Department of Computer Science, University of Surrey, Guildford, Surrey, GU27XH, UK
You can also search for this author in PubMed Google Scholar
Corresponding author
Correspondence to Jie Lu .
Ethics declarations
Conflict of interest.
On behalf of all authors, the corresponding author states that there is no conflict of interest.
Additional information
Publisher's note.
Springer Nature remains neutral with regard to jurisdictional claims in published maps and institutional affiliations.
Rights and permissions
Open Access This article is licensed under a Creative Commons Attribution 4.0 International License, which permits use, sharing, adaptation, distribution and reproduction in any medium or format, as long as you give appropriate credit to the original author(s) and the source, provide a link to the Creative Commons licence, and indicate if changes were made. The images or other third party material in this article are included in the article's Creative Commons licence, unless indicated otherwise in a credit line to the material. If material is not included in the article's Creative Commons licence and your intended use is not permitted by statutory regulation or exceeds the permitted use, you will need to obtain permission directly from the copyright holder. To view a copy of this licence, visit http://creativecommons.org/licenses/by/4.0/ .
Reprints and permissions
About this article
Zhang, Q., Lu, J. & Jin, Y. Artificial intelligence in recommender systems. Complex Intell. Syst. 7 , 439–457 (2021). https://doi.org/10.1007/s40747-020-00212-w
Download citation
Received : 26 June 2020
Accepted : 28 September 2020
Published : 01 November 2020
Issue Date : February 2021
DOI : https://doi.org/10.1007/s40747-020-00212-w
Share this article
Anyone you share the following link with will be able to read this content:
Sorry, a shareable link is not currently available for this article.
Provided by the Springer Nature SharedIt content-sharing initiative
- Recommender systems
- Artificial intelligence
- Computational intelligence
- Find a journal
- Publish with us
- Track your research

An official website of the United States government
The .gov means it’s official. Federal government websites often end in .gov or .mil. Before sharing sensitive information, make sure you’re on a federal government site.
The site is secure. The https:// ensures that you are connecting to the official website and that any information you provide is encrypted and transmitted securely.
- Publications
- Account settings
Preview improvements coming to the PMC website in October 2024. Learn More or Try it out now .
- Advanced Search
- Journal List
- J Med Internet Res
- v.23(6); 2021 Jun

Health Recommender Systems: Systematic Review
Robin de croon.
1 Department of Computer Science, KU Leuven, Leuven, Belgium
Leen Van Houdt
Nyi nyi htun, gregor Štiglic.
2 Faculty of Health Sciences, University of Maribor, Maribor, Slovenia
Vero Vanden Abeele
Katrien verbert, associated data.
Coded data set of all included papers.
Overview of recommended items by 73 studies.
Overview of evaluation approaches.
Health recommender systems (HRSs) offer the potential to motivate and engage users to change their behavior by sharing better choices and actionable knowledge based on observed user behavior.
We aim to review HRSs targeting nonmedical professionals (laypersons) to better understand the current state of the art and identify both the main trends and the gaps with respect to current implementations.
We conducted a systematic literature review according to the PRISMA (Preferred Reporting Items for Systematic Reviews and Meta-Analyses) guidelines and synthesized the results. A total of 73 published studies that reported both an implementation and evaluation of an HRS targeted to laypersons were included and analyzed in this review.
Recommended items were classified into four major categories: lifestyle, nutrition, general health care information, and specific health conditions. The majority of HRSs use hybrid recommendation algorithms. Evaluations of HRSs vary greatly; half of the studies only evaluated the algorithm with various metrics, whereas others performed full-scale randomized controlled trials or conducted in-the-wild studies to evaluate the impact of HRSs, thereby showing that the field is slowly maturing. On the basis of our review, we derived five reporting guidelines that can serve as a reference frame for future HRS studies. HRS studies should clarify who the target user is and to whom the recommendations apply, what is recommended and how the recommendations are presented to the user, where the data set can be found, what algorithms were used to calculate the recommendations, and what evaluation protocol was used.
Conclusions
There is significant opportunity for an HRS to inform and guide health actions. Through this review, we promote the discussion of ways to augment HRS research by recommending a reference frame with five design guidelines.
Introduction
Research goals.
Current health challenges are often related to our modern way of living. High blood pressure, high glucose levels, and physical inactivity are all linked to a modern lifestyle characterized by sedentary living, chronic stress, or a high intake of energy-dense foods and recreational drugs [ 1 ]. Moreover, people usually make poor decisions related to their health for distinct reasons, for example, busy lifestyles, abundant options, and a lack of knowledge [ 2 ]. Practically, all modern lifestyle health risks are directly affected by people’s health decisions [ 3 ], such as an unhealthy diet or physical inactivity, which can contribute up to three-fourth of all health care costs in the United States [ 4 ]. Most risks can be minimized, prevented, or sometimes even reversed with small lifestyle changes. Eating healthily, increasing daily activities, and knowing where to find validated health information could lead to improved health status [ 5 ].
Health recommender systems (HRSs) offer the potential to motivate and engage users to change their behavior [ 6 ] and provide people with better choices and actionable knowledge based on observed behavior [ 7 - 9 ]. The overall objective of the HRS is to empower people to monitor and improve their health through technology-assisted, personalized recommendations. As one approach of modern health care is to involve patients in the cocreation of their own health, rather than just leaving it in the hands of medical experts [ 10 ], we limit the scope of this paper to HRSs that focus on laypersons, for example, nonhealth care professionals. These HRSs are different from clinical decision support systems that provide recommendations for health care professionals. However, laypersons also need to understand the rationale of recommendations, as echoed by many researchers and practitioners [ 11 ]. This paper also studies the role of a graphical user interface. To guide this study, we define our research questions (RQs) as follows:
RQ1: What are the main applications of the recent HRS, and what do these HRSs recommend?
RQ2: Which recommender techniques are being used across different HRSs?
RQ3: How are the HRSs evaluated, and are end users involved in their evaluation?
RQ4: Is a graphical user interface designed, and how is it used to communicate the recommended items to the user?
Recommender Systems and Techniques
Recommender techniques are traditionally divided into different categories [ 12 , 13 ] and are discussed in several state-of-the-art surveys [ 14 ]. Collaborative filtering is the most used and mature technique that compares the actions of multiple users to generate personalized suggestions. An example of this technique can typically be found on e-commerce sites, such as “Customers who bought this item also bought...” Content-based filtering is another technique that recommends items that are similar to other items preferred by the specific user. They rely on the characteristics of the objects themselves and are likely to be highly relevant to a user’s interests. This makes content-based filtering especially valuable for application domains with large libraries of a single type of content, such as MedlinePlus’ curated consumer health information [ 15 ]. Knowledge-based filtering is another technique that incorporates knowledge by logic inferences. This type of filtering uses explicit knowledge about an item, user preferences, and other recommendation criteria. However, knowledge acquisition can also be dynamic and relies on user feedback. For example, a camera recommender system might inquire users about their preferences, fixed or changeable lenses, and budget and then suggest a relevant camera. Hybrid recommender systems combine multiple filtering techniques to increase the accuracy of recommendation systems. For example, the companies you may want to follow feature in LinkedIn uses both content and collaborative filtering information [ 16 ]: collaborative filtering information is included to determine whether a company is similar to the ones a user already followed, whereas content information ensures whether the industry or location matches the interests of the user. Finally, recommender techniques are often augmented with additional methods to incorporate contextual information in the recommendation process [ 17 ], including recommendations via contextual prefiltering, contextual postfiltering, and contextual modeling [ 18 ].
HRSs for Laypersons
Ricci et al [ 12 ] define recommender systems as:
Recommender Systems (RSs) are software tools and techniques providing suggestions for items to be of use to a user [ 13 , 19 , 20 ]. The suggestions relate to various decision-making processes, such as what items to buy, what music to listen to, or what online news to read.
In this paper, we analyze how recommender systems have been used in health applications, with a focus on laypersons. Wiesner and Pfeifer [ 21 ] broadly define an HRS as:
a specialization of an RS [recommender system] as defined by Ricci et al [ 12 ]. In the context of an HRS, a recommendable item of interest is a piece of nonconfidential, scientifically proven or at least generally accepted medical information.
Researchers have sought to consolidate the vast body of literature on HRSs by publishing several surveys, literature reviews, and state-of-the-art overviews. Table 1 provides an overview of existing summative studies on HRSs that identify existing research and shows the number of studies included, the method used to analyze the studies, the scope of the paper, and their contribution.
An overview of the existing health recommender system overview papers.
a HCI: human-computer interaction.
b HRS: health recommender system.
c CTHC: computer-tailored health communication.
As can be seen in Table 1 , the scope of the existing literature varies greatly. For example, Ferretto et al [ 26 ] focused solely on HRSs in mobile apps. A total of 3 review studies focused specifically on the patient side of the HRS: (1) Calero Valdez et al [ 23 ] analyzed the existing literature from a human-computer interaction perspective and stressed the importance of a good HRS graphical user interface; (2) Schäfer et al [ 28 ] focused on tailoring recommendations to end users based on health context, history, and goals; and (3) Hors-Fraile et al [ 27 ] focused on the individual user by analyzing how HRSs can target behavior change strategies. The most extensive study was conducted by Sadasivam et al [ 29 ]. In their study, most HRSs used knowledge-based recommender techniques, which might limit individual relevance and the ability to adapt in real time. However, they also reported that the HRS has the opportunity to use a near-infinite number of variables, which enables tailoring beyond designer-written rules based on data. The most important challenges reported were the cold start [ 31 ] where limited data are available at the start of the intervention, limited sample size, adherence, and potential unintended consequences [ 29 ]. Finally, we observed that these existing summative studies were often restrictive in their final set of papers.
Our contributions to the community are four-fold. First, we analyze a broader set of research studies to gain insights into the current state of the art. We do not limit the included studies to specific devices or patients in a clinical setting but focus on laypersons in general. Second, through a comprehensive analysis, we aim to identify the applications of recent HRS apps and gain insights into actionable knowledge that HRSs can provide to users (RQ1), to identify which recommender techniques have been used successfully in the domain (RQ2), how HRSs have been evaluated (RQ3), and the role of the user interface in communicating recommendations to users (RQ4). Third, based on our extensive literature review, we derive a reference frame with five reporting guidelines for future layperson HRS research. Finally, we collected and coded a unique data set of 73 papers, which is publicly available in Multimedia Appendix 1 [ 7 - 9 , 15 , 32 - 100 ] for other researchers.
Search Strategy
This study was conducted according to the key steps required for systematic reviews according to PRISMA (Preferred Reporting Items for Systematic Reviews and Meta-Analyses) guidelines [ 101 ]. A literature search was conducted using the ACM Digital Library (n=2023), IEEExplore (n=277), and PubMed (n=93) databases. As mentioned earlier, in this systematic review we focused solely on HRSs with a focus on laypersons. However, many types of systems, algorithms, and devices can be considered as a HRS. For example, push notifications in a mobile health app or health tips prompted by web services can also be considered as health-related recommendations. To outline the scope, we limited the search terms to include a recommender or recommendation, as reported by the authors. The search keywords were as follows, using an inclusive OR: ( recommender OR recommendation systems OR recommendation system ) AND (health OR healthcare OR patient OR patients ).
In addition, a backward search was performed by examining the bibliographies of the survey and review papers discussed in the Introduction section and the reference list of included studies to identify any additional studies. A forward search was performed to search for articles that cited the work summarized in Table 1 .
Study Inclusion and Exclusion Criteria
As existing work did not include many studies ( Table 1 ) and focused on a specific medical domain or device, such as mobile phones, this literature review used nonrestrictive inclusion criteria. Studies that met all the following criteria were included in the review: described an HRS whose primary focus was to improve health (eg, food recommenders solely based on user preferences [ 102 ] were not included); targeted laypersons (eg, activity recommendations targeted on a proxy user such as a coach [ 103 ] were not included); implemented the HRS (eg, papers describing an HRS concept are not included); reported an evaluation, either web-based or offline evaluation; peer-reviewed and published papers; published in English.
Papers were excluded when one of the following was true: the recommendations of HRSs were unclear; the full text was unavailable; or a newer version was already included.
Finally, when multiple papers described the same HRS, only the latest, relevant full paper was included.
Classification
To address our RQs, all included studies were coded for five distinct coding categories.
Study Details
To contextualize new insights, the publication year and publication venue were analyzed.
Recommended Items
HRSs are used across different health domains. To provide details on what is recommended, all papers were coded according to their respective health domains. To not limit the scope of potential items, no predefined coding table was used. Instead, all papers were initially coded by the first author. These resulting recommendations were then clustered together in collaboration with the coauthors into four categories, as shown in Multimedia Appendix 2 .
Recommender Techniques
This category encodes the recommender techniques that were used: collaborative filtering [ 104 ], content-based filtering [ 105 ], knowledge-based filtering [ 106 ], and their hybridizations [ 107 ]. Some studies did not specify any algorithmic details or compared multiple techniques. Finally, when an HRS used contextual information, it was coded whether they used pre- or postfiltering or contextual modeling.
Evaluation Approach
This category encodes which evaluation protocols were used to measure the effect of HRSs. We coded whether the HRSs were evaluated through offline evaluations (no users involved), surveys, heuristic feedback from expert users, controlled user studies, deployments in the wild , and randomized controlled trials (RCTs). We also coded sample size and study duration and whether ethical approval was gathered and needed.
Interface and Transparency
Recommender systems are often perceived as a black box , as the rationale for recommendations is often not explained to end users. Recent research increasingly focuses on providing transparency to the inner logic of the system [ 11 ]. We encoded whether explanations are provided and, in this case, how such transparency is supported in the user interface. Furthermore, we also classified whether the user interface was designed for a specific platform, categorized as mobile , web , or other.
Data Extraction, Intercoder Reliability, and Quality Assessment
The required information for all included technologies and studies was coded by the first author using a data extraction form. Owing to the large variety of study designs, the included studies were assessed for quality (detailed scores given in Multimedia Appendix 1 ) using the tool by Hawker et al [ 108 ]. Using this tool, the abstract and title , introduction and aims , method and data , sample size (if applicable), data analysis , ethics and bias , results , transferability or generalizability , and implications and usefulness were allocated a score between 1 and 4, with higher scoring studies indicating higher quality. A random selection with 14% (10/73) of the papers was listed in a spreadsheet and coded by a second researcher following the defined coding categories and subcategories. The decisions made by the second researcher were compared with the first. With the recommended items ( Multimedia Appendix 2 ), there was only one small disagreement between physical activity and leisure activity [ 32 ], but all other recommended items were rated exactly the same; the recommender techniques had a Cohen κ value of 0.71 ( P <.001) and the evaluation approach scored a Cohen κ value of 0.81 ( P <.001). There was moderate agreement (Cohen κ=0.568; P <.001) between the researchers concerning the quality of the papers. The interfaces used were in perfect agreement. Finally, the coding data are available in Multimedia Appendix 1 .
The literature in three databases yielded 2340 studies, of which only 23 were duplicates and 53 were full proceedings, leaving 2324 studies to be screened for eligibility. A total of 2161 studies were excluded upon title or abstract screening because they were unrelated to health or targeted at medical professionals or because the papers did not report an evaluation. Thus, the remaining 163 full-text studies were assessed for eligibility. After the removal of 90 studies that failed the inclusion criteria or met the exclusion criteria, 73 published studies remained. The search process is illustrated in Figure 1 .

Flow diagram according to the PRISMA (Preferred Reporting Items for Systematic Reviews and Meta-Analyses) guidelines. EC: exclusion criteria; IC: inclusion criteria.
All included papers were published in 2009 or later, following an upward trend of increased popularity. The publication venues of HRSs are diverse. Only the PervasiveHealth [ 33 - 35 ], RecSys [ 36 , 37 , 109 ], and WI-IAT [ 38 - 40 ] conferences published 3 papers each that were included in this study. The Journal of Medical Internet Research was the only journal that occurred more frequently in our data set; 5 papers were published by Journal of Medical Internet Research [ 41 - 45 ]. The papers were first rated using Hawker tool [ 108 ]. Owing to a large number of offline evaluations, we did not include the sample score to enable a comparison between all included studies. The papers received an average score of 24.32 (SD 4.55, max 32; data set presented in Multimedia Appendix 1 ). Most studies scored very poor on reporting ethics and potential biases, as illustrated in Figure 2 . However, there is an upward trend over the years in more adequate reporting of ethical issues and potential biases. The authors also limited themselves to their specific case studies and did not make any recommendations for policy (last box plot is presented in Figure 2 ). All 73 studies reported the use of different data sets. Although all recommended items were health related, only Asthana et al [ 46 ] explicitly mentioned using electronic health record data. Only 14% (10/73) [ 7 , 47 - 55 ] explicitly reported that they addressed the cold-start problem.

Distribution of the quality assessment using Hawker tool.
Most HRSs operated in different domains and thus recommended different items. In this study, four nonmutually exclusive categories of recommended items were identified: lifestyle 33% (24/73), nutrition 36% (26/73), general health information 32% (23/73), and specific health condition–related recommendations 12% (9/73). The only significant trend we found is the increasing popularity of nutrition advice . Multimedia Appendix 2 shows the distribution of these recommended items.
Many HRSs, 33% (24/73) of the included studies, suggest lifestyle-related items, but they differ greatly in their exact recommendations. Physical activity is often recommended. Physical activities are often personalized according to personal interests [ 56 ] or the context of the user [ 35 ]. In addition to physical activities, Kumar et al [ 32 ] recommend eating, shopping, and socializing activities. One study analyzes the data and measurements to be tracked for an individual and then recommends the appropriate wearable technologies to stimulate proactive health [ 46 ]. A total of 7 studies [ 7 , 9 , 42 , 53 , 57 - 59 ] more directly try to convince users to alter their behavior by recommending them to change, or alter their behavior: for example, Rabbi et al [ 7 ] learn “a user’s physical activity and dietary behavior and strategically suggests changes to those behaviors for a healthier lifestyle . ” In another example, both Marlin et al [ 59 ] and Sadasivam et al [ 42 ] motivate users to stop smoking by providing them with tailored messages, such as “Keep in mind that cravings are temporary and will pass.” Messages could reflect the theoretical determinants of quitting, such as positive outcome expectations and self-efficacy enhancing small goals [ 42 ].
The influence of food on health is also clear from the large subset of HRSs dealing with nutrition recommendations. A mere 36% (26/73) of the studies recommend nutrition-related information, such as recipes [ 50 ], meal plans [ 36 ], restaurants [ 60 ], or even help with choosing healthy items from a restaurant menu [ 61 ]. Wayman and Madhvanath [ 37 ] provide automated, personalized, and goal-driven dietary guidance to users based on grocery receipt data. Trattner and Elsweiler [ 62 ] use postfiltering to focus on healthy recipes only and extended them with nutrition advice, whereas Ge et al [ 48 ] require users to first enter their preferences for better recommendations. Moreover, Gutiérrez et al [ 63 ] propose healthier alternatives through augmented reality when the users are shopping. A total of 7 studies specifically recommend healthy recipes [ 47 , 48 , 50 , 62 , 64 - 66 ]. Most HRSs consider the health condition of the user, such as the DIETOS system [ 67 ]. Other systems recommend recipes that are synthesized based on existing recipes and recommend new recipes [ 64 ], assist parents in making appropriate food for their toddlers [ 47 ], or help users to choose allergy-safe recipes [ 65 ].
General Health Information
According to 32% (23/73) of the included studies, providing access to trustworthy health care information is another common objective. A total of 5 studies focused on personalized, trustworthy information per se [ 15 , 55 , 68 - 70 ], whereas 5 others focused on guiding users through health care forums [ 52 , 71 - 74 ]. In total, 3 studies [ 55 , 68 , 69 ] provided personalized access to general health information. For example, Sanchez Bocanegra et al [ 15 ] targeted health-related videos and augmented them with trustworthy information from the United States National Library of Medicine (MedlinePlus) [ 110 ]. A total of 3 studies [ 52 , 72 , 74 ] related to health care forums focused on finding relevant threads. Cho et al [ 72 ] built “an autonomous agent that automatically responds to an unresolved user query by posting an automated response containing links to threads discussing similar medical problems.” However, 2 studies [ 71 , 73 ] helped patients to find similar patients. Jiang and Yang [ 71 ] investigated approaches for measuring user similarity in web-based health social websites, and Lima-Medina et al [ 73 ] built a virtual environment that facilitates contact among patients with cardiovascular problems. Both studies aim to help users seek informational and emotional support in a more efficient way. A total of 4 studies [ 41 , 75 - 77 ] helped patients to find appropriate doctors for a specific health problem, and 4 other studies [ 51 , 78 - 80 ] focused on finding nearby hospitals. A total of 2 studies [ 78 , 79 ] simply focused on the clinical preferences of the patients, whereas Krishnan et al [ 111 ] “provide health care recommendations that include Blood Donor recommendations and Hospital Specialization.” Finally, Tabrizi et al [ 80 ] considered patient satisfaction as the primary feature of recommending hospitals to the user.
Specific Health Conditions
The last group of studies (9/73, 12%) focused on specific health conditions. However, the recommended items vary significantly. Torrent-Fontbona and Lopez Ibanez [ 81 ] have built a knowledge-based recommender system to assist diabetes patients in numerous cases, such as the estimated carbohydrate intake and past and future physical activity. Pustozerov et al [ 43 ] try to “reduce the carbohydrate content of the desired meal by reducing the amount of carbohydrate-rich products or by suggesting variants of products for replacement.” Li and Kong [ 82 ] provided diabetes-related information, such as the need for a low-sodium lunch, targeted on American Indians through a mobile app. Other health conditions supported by recommender systems include depression and anxiety [ 83 ], mental disorders [ 45 ], and stress [ 34 , 54 , 84 , 85 ]. Both the mental disorder [ 45 ] and the depression and anxiety [ 83 ] HRSs recommend mobile apps. For example, the app MoveMe suggests exercises tailored to the user’s mood. The HRS to alleviate stress includes recommending books to read [ 54 ] and meditative audios [ 85 ].
The recommender techniques used varied greatly. Table 2 shows the distributions of these recommender techniques.
Overview of the different recommender techniques used in the studies.
a The papers are classified based on how the authors reported their techniques.
Recommender Techniques in Practice
The majority of HRSs (49/73, 67%) rely on knowledge-based techniques, either directly (17/49, 35%) or in a hybrid approach (32/49, 65%). Knowledge-based techniques are often used to incorporate additional information of patients into the recommendation process [ 112 ] and have been shown to improve the quality of recommendations while alleviating other drawbacks such as cold-start and sparsity issues [ 14 ]. Some studies use straightforward approaches, such as if-else reasoning based on domain knowledge [ 9 , 79 , 81 , 82 , 88 , 90 , 100 ]. Other studies use more complex algorithms such as particle swarm optimization [ 57 ], fuzzy logic [ 68 ], or reinforcement algorithms [ 44 , 84 ].
In total, 32 studies reported using a combination of recommender techniques and are classified as hybrid recommender systems . Different knowledge-based techniques are often combined. For example, Ali et al [ 56 ] used a combination of rule-based reasoning, case-based reasoning, and preference-based reasoning to recommend personalized physical activities according to the user’s specific needs and personal interests. Asthana et al [ 46 ] combined the knowledge of a decision tree and demographic information to identify the health conditions. When health conditions are known, the system knows which measurements need to be monitored. A total of 7 studies used a content-based technique to recommend educational content [ 15 , 72 , 87 ], activities [ 32 , 86 ], reading materials [ 54 ], or nutritional advice [ 63 ].
Although collaborative filtering is a popular technique [ 113 ], it is not used frequently in the HRS domain. Marlin et al [ 59 ] used collaborative filtering to personalize future smoking cessation messages based on explicit feedback on past messages. This approach is used more often in combination with other techniques. A total of 2 studies [ 38 , 92 ] combined content-based techniques with collaborative filtering. Esteban et al [ 92 ], for instance, switched between content-based and collaborative approaches. The former approach is used for new physiotherapy exercises and the latter, when a new patient is registered or when previous recommendations to a patient are updated.
Context-Based Recommender Techniques
From an HRS perspective, context is described as an aggregate of various information that describes the setting in which an HRS is deployed, such as the location, the current activity, and the available time of the user. A total of 5 studies use contextual information to improve their recommendations but use a different technique; a prefilter uses contextual information to select or construct the most relevant data for generating recommendations. For example, in Narducci et al [ 75 ], the set of potentially similar patients was restricted to consultation requests in a specific medical area. Rist et al [ 33 ] applied a rule-based contextual prefiltering approach [ 114 ] to filter out inadequate recommendations, for example, “if it is dark outside, all outdoor activities, such as ‘take a walk,’ are filtered out” [ 33 ] before they are fed to the recommendation algorithm. However, a postfilter removes the recommended items after they are generated, such as filtering outdoor activities while it is raining. Casino et al [ 97 ] used a postfiltering technique by running the recommended items through a real-time constraint checker . Finally, contextual modeling, which was used by 2 studies [ 35 , 58 ], uses contextual information directly in the recommendation function as an explicit predictor of a user’s rating for an item [ 114 ].
Location, agenda, and weather are examples of contextual information used by Lin et al [ 35 ] to promote the adoption of a healthy and active lifestyle. Cerón-Rios et al [ 58 ] used a decision tree to analyze user needs, health information, interests, time, location, and lifestyle to promote healthy habits. Casino et al [ 97 ] gathered contextual information through smart city sensor data to recommend healthier routes. Similarly, contextual information was acquired by Rist et al [ 33 ] using sensors embedded in the user’s environment.
Comparisons
A total of 8 papers compared different recommender techniques to find the most optimal algorithm for a specific data set, end users, domain, and goal. Halder et al [ 52 ] used two well-known health forum data sets (PatientsLikeMe [ 115 ] and HealthBoards [ 116 ]) to compare 7 recommender techniques (among collaborative filtering and content-based filtering) and found that a hybrid approach scored best [ 52 ]. Another example is the study by Narducci et al [ 75 ], who compared four recommendation algorithms: cosine similarity as a baseline, collaborative filtering, their own HealthNet algorithm, and a hybrid of HealthNet and cosine similarity. They concluded that a prefiltering technique for similar patients in a specific medical area can drastically improve the recommendation accuracy [ 75 ]. The average and SD of the resulting ratings of the two collaborative techniques are compared with random recommendations by Li et al [ 60 ]. They show that a hybrid approach of a collaborative filter augmented with the calculated health level of the user performs better. In their nutrition-based meal recommender system, Yang et al [ 49 ] used item-wise and pairwise image comparisons in a two-step process. In conclusion, the 8 studies showed that recommendations can be improved when the benefits of multiple recommender techniques are combined in a hybrid solution [ 60 ] or contextual filters are applied [ 75 ].
HRSs can be evaluated in multiple ways. In this study, we found two categories of HRS evaluations: (1) offline evaluations that use computational approaches to evaluate the HRS and (2) evaluations in which an end user is involved. Some studies used both, as shown in Multimedia Appendix 3 .
Offline Evaluations
Of the total studies, 47% (34/73) do not involve users directly in their method of evaluation. The evaluation metrics also vary greatly, as many distinct metrics are reported in the included papers ( Multimedia Appendix 3 ). Precision 53% (18/34), accuracy 38% (13/34), performance 35% (12/34), and recall 32% (11/34) were the most commonly used offline evaluation metrics. Recall has been used significantly more in recent papers, whereas accuracy also follows an upward trend. Moreover, performance was defined differently across studies. Torrent-Fontbona and Lopez Ibanez [ 81 ] compared the “amount of time in the glycaemic target range by reducing the time below the target” as performance. Cho et al [ 72 ] compared the precision and recall to report the performance. Clarke et al [ 84 ] calculated their own reward function to compare different approaches, and Lin et al [ 35 ] measured system performance as the number of messages sent in their in the wild study. Finally, Marlin et al [ 59 ] tested the predictive performance using a triple cross-validation procedure.
Other popular offline evaluation metrics are accuracy-related measurements, such as mean absolute (percentage) error, 18% (6/34); normalized discounted cumulative gain (nDCG), 18% (6/34); F 1 score, 15% (5/34); and root mean square error, 15% (5/34). The other metrics were measured inconsistently. For example, Casino et al [ 97 ] reported that they measure robustness but do not outline what they measure as robustness. However, they measured the mean absolute error. Torrent-Fontbona and Lopez Ibanez [ 81 ] defined robustness as the capability of the system to handle missing values. Effectiveness is also measured with different parameters, such as its ability to take the right classification decisions [ 75 ] or in terms of key opinion leaders’ identification [ 41 ]. Finally, Li and Zaman [ 68 ] measured trust with a proxy: “evaluate the trustworthiness of a particular user in a health care social network based on factors such as role and reputation of the user in the social community” [ 68 ].
User Evaluations
Of the total papers, 53% (39/73) included participants in their HRS evaluation, with an average sample size of 59 (SD 84) participants (excluding the outlier of 8057 participants, as recruited in the study by Cheung et al [ 83 ]). On average, studies ran for more than 2 months (68, SD 56 days) and included all age ranges. There is a trend of increasing sample size and study duration over the years. However, only 17 studies reported the study duration; therefore, these trends were not significant. Surveys (12/39, 31%), user studies (10/39, 26%), and deployments in the wild (10/39, 26%) were the most used user evaluations. Only 6 studies used an RCT to evaluate their HRS. Finally, although all the included studies focused on HRSs and were dealing with sensitive data, only 12% (9/73) [ 9 , 34 , 42 - 45 , 73 , 83 , 95 ] reported ethical approval by a review board.
No universal survey was found, as all the studies deployed a distinct survey. Ge et al [ 48 ] used the system usability scale and the framework of Knijnenburg et al [ 117 ] to explain the user experience of recommender systems. Esteban et al [ 95 ] designed their own survey with 10 questions to inquire about user experience. Cerón-Rios [ 58 ] relied on the ISO/IEC (International Organization of Standardization/International Electrotechnical Commission) 25000 standard to select 7 usability metrics to evaluate usability. Although most studies did not explicitly report the surveys used, user experience was a popular evaluation metric, as in the study by Wang et al [ 69 ]. Other metrics range from measuring user satisfaction [ 69 , 99 ] and perceived prediction accuracy [ 59 ] (with 4 self-composed questions). Nurbakova et al [ 98 ] combined data analytics with surveys to map their participants’ psychological background, including orientations to happiness measured using the Peterson scale [ 118 ], personality traits using the Mini-International Personality Item Pool [ 119 ], and Fear of Missing Out based on the Przybylski scale [ 120 ].
Single-Session Evaluations (User Studies)
A total of 10 studies recruited users and asked them to perform certain tasks in a single session. Yang et al [ 49 ] performed a 60-person user study to assess its feasibility and effectiveness. Each participant was asked to rate meal recommendations relative to those made using a traditional survey-based approach. In a study by Gutiérrez et al [ 63 ], 15 users were asked to use the health augmented reality assistant and measure the qualities of the recommender system, users’ behavioral intentions, perceived usefulness, and perceived ease of use. Jiang and Xu [ 77 ] performed 30 consultations and invited 10 evaluators majoring in medicine and information systems to obtain an average rating score and nDCG. Radha et al [ 8 ] used comparative questions to evaluate the feasibility. Moreover, Cheng et al [ 89 ] used 2 user studies to rank two degrees of compromise (DOC). A low DOC assigns more weight to the algorithm, and a high DOC assigns more weight to the user’s health perspective. Recommendations with a lower DOC are more efficient for the user’s health, but recommendations with a high DOC could convince users to believe that the recommended action is worth doing. Other approaches used are structured interviews [ 58 ], ranking [ 86 , 89 ], asking for unstructured feedback [ 40 , 88 ], and focus group discussions [ 87 ]. Finally, 3 studies [ 15 , 75 , 90 ] evaluated their system through a heuristic evaluation with expert users.
In the Wild
Only 2 studies tested their HRS into the wild recruited patients (people with a diagnosed health condition) in their evaluation. Yom-Tov et al [ 44 ] provided 27 sedentary patients with type 2 diabetes with a smartphone-based pedometer and a personal plan for physical activity. They assessed the effectiveness by calculating the amount of activity that the patient performed after the last message was sent. Lima-Medina et al [ 73 ] interviewed 45 patients with cardiovascular problems after a 6-month study period to measure (1) social management results, (2) health care plan results, and (3) recommendation results. Rist et al [ 33 ] performed an in-situ evaluation in an apartment of an older couple and used the data logs to describe the usage but augmented the data with a structured interview.
Yang et al [ 49 ] conducted a field study of 227 anonymous users that consisted of a training phase and a testing phase to assess the prediction accuracy. Buhl et al [ 99 ] created three user groups according to the recommender technique used and analyzed log data to compare the response rate, open email rate, and consecutive log-in rate. Similarly, Huang et al [ 76 ] compared the ratio of recommended doctors chosen and reserved by patients with the recommended doctors. Lin et al [ 35 ] asked 6 participants to use their HRSs for 5 weeks, measured system performance, studied user feedback to the recommendations, and concluded with an open-user interview. Finally, Ali et al [ 56 ] asked 10 volunteers to use their weight management systems for a couple of weeks. However, they do not focus on user-centric evaluation, as “only a prototype of the [...] platform is implemented.”
Rabbi et al [ 7 ] followed a single case with multiple baseline designs [ 121 ]. Single-case experiments achieve sufficient statistical power with a large number of repeated samples from a single individual. Moreover, Rabbi et al [ 7 ] argued that HRSs suit this requirement “since enough repeated samples can be collected with automated sensing or daily manual logging [ 121 ].” Participants were exposed to 2, 3, or 4 weeks of the control condition. The study ran for 7-9 weeks to compensate for the novelty effects. Food and exercise log data were used to measure changes in food calorie intake and calorie loss during exercise.
Randomized Controlled Trials
Only 6 studies followed an RCT approach. In the RCT by Bidargaddi et al [ 45 ], a large group of patients (n=192) and control group (n=195) were asked to use a web-based recommendation service for 4 weeks that recommended mental health and well-being mobile apps. Changes in well-being were measured using the Mental Health Continuum-Short Form [ 122 ]. The RCT by Sadasivam et al [ 42 ] enrolled 120 current smokers (n=74) and control group (n=46) as a follow-up to a previous RCT [ 123 ] that evaluated their portal to specifically evaluate the HRS algorithm. Message ratings were compared between the intervention and control groups.
Cheung et al [ 83 ] measured app loyalty through the number of weekly app sessions over a period of 16 weeks with 8057 users. In the study by Paredes et al [ 34 ], 120 participants had to use the HRS for at least 26 days. Self-reported stress assessment was performed before and after the intervention. Agapito et al [ 67 ] used an RCT with 40 participants to validate the sensitivity (true positive rate/[true positive rate+false negative rate]) and specificity (true negative rate/[true negative rate+false positive rate]) of the DIETOS HRS. Finally, Luo et al [ 93 ] performed a small clinical trial for more than 3 months (but did not report the number of participants). Their primary outcome measures included two standard clinical blood tests: fasting blood glucose and laboratory-measured glycated hemoglobin, before and after the intervention.
Only 47% (34/73) of the studies reported implementing a graphical user interface to communicate the recommended health items to the user. As illustrated in Table 3 , 53% (18/34) use a mobile interface, usually through a mobile (web) app, whereas 36% (14/34) use a web interface to show the recommended items. Rist et al [ 33 ] built a kiosk into older adults’ homes, as illustrated in Figure 3 . Gutiérrez et al [ 63 ] used Microsoft HoloLens to project healthy food alternatives in augmented reality surrounding a physical object that the user holds, as shown in Figure 4 .
Distribution of the interfaces used among the different health recommender systems (n=34).

Rist et al installed a kiosk in the home of older adults as a direct interface to their health recommender system.

An example of the recommended healthy alternatives by Gutiérrez et al.
Visualization
A total of 7 studies [ 33 , 34 , 37 , 63 , 79 , 88 , 97 ] or approximately one-fourth of the studies with an interface included visualizations. However, the approach used was different for all studies, as shown in Table 4 . Showing stars to show the relevance of a recommended item are only used by Casino et al [ 97 ] and Gutiérrez et al [ 63 ]. Wayman and Madhvanath [ 37 ] also used bar charts to visualize the progress toward a health goal. They visualize the healthy proportions, that is, what the user should eat. Somewhat more complex visualizations are used by Ho and Chen [ 88 ] who visualized the user’s ECG zones. Paredes et al [ 34 ] presented an emotion graph as an input screen. Rist et al [ 33 ] visualized an example of how to perform the recommended activity.
Distribution of the visualizations used among the different health recommender systems (n=7).
Transparency
In the study by Lage et al [ 87 ], participants expressed that:
they would like to have more control over recommendations received. In that sense, they suggested more information regarding the reasons why the recommendations are generated and more options to assess them.
A total of 7 studies [ 7 , 37 , 41 , 45 , 63 , 66 , 82 ] explained the reasoning behind recommendations to end users at the user interface. Gutiérrez et al [ 63 ] provided recommendations for healthier food products and mentioned that the items ( Figure 4 ) are based on the users’ profile. Ueta et al [ 66 ] explained the relationship between the recommended dishes and a person’s health conditions. For example, a person with acne can see the following text: “15 dishes that contained Pantothenic acid thought to be effective in acne a lot became a hit” [ 66 ]. Li and Kong [ 82 ] showed personalized recommended health actions in a message center. Color codes are used to differentiate between reminders, missed warnings, and recommendations. Rabbi et al [ 7 ] showed tailored motivational messages to explain why activities are recommended. For example, when the activity walk near East Ave is recommended, the app shows the additional message:
1082 walks in 240 days, 20 mins of walk everyday. Each walk nearly 4 min. Let us get 20 mins or more walk here today 7
Wayman and Madhvanath [ 37 ] first visualized the user’s personal nutrition profile and used the lower part of the interface to explain why the item was recommended. They provided an illustrative example of spaghetti squash. The explanation shows that:
This product is high in Dietary_fiber, which you could consume more of. Try to get 3 servings a week 37
Guo et al [ 41 ] recommended doctors and showed a horizontal bar chart to visualize the user’s values compared with the average values. Finally, Bidargaddi et al [ 45 ] visualized how the recommended app overlaps with the goal set by the users, as illustrated in Figure 5 .

A screenshot from the health recommender system of Bidargaddi et al. Note the blue tags illustrating how each recommended app matches the users’ goals.
Principal Findings
HRSs cover a multitude of subdomains, recommended items, implementation techniques, evaluation designs, and means of communicating the recommended items to the target user. In this systematic review, we clustered the recommended items into four groups: lifestyle, nutrition, general health care information, and specific health conditions. There is a clear trend toward HRSs that provide well-being recommendations but do not directly intervene in the user’s medical status. For example, almost 70% (50/73; lifestyle and nutrition) focused on no strict medical recommendations. In the lifestyle group, physical activities (10/24, 42%) and advice on how to potentially change behavior (7/24, 29%) were recommended most often. In the nutrition group, these recommendations focused on nutritional advice (8/26, 31%), diets (7/26, 27%), and recipes (7/26, 27%). A similar trend was observed in the health care information group, where HRSs focused on guiding users to the appropriate environments such as hospitals (5/23, 22%) and medical professionals (4/23, 17%) or on helping users find qualitative information (5/23, 22%) on validated sources or from experiences by similar users and patients on health care forums (3/23, 13%). Thus, they only provide general information and do not intervene by recommending, for example, changing medication. Finally, when HRSs targeted specific health conditions, they recommended nonintervening actions, such as meditation sessions [ 84 ] or books to read [ 54 ].
Although collaborative filtering is commonly the most used technique in other domains [ 124 ], here only 3 included studies reported the use of a collaborative filtering approach. Moreover, 43% (32/73) of the studies applied a hybrid approach, showing that HRS data sets might need special attention, which might also be the reason why all 73 studies used distinct data sets. In addition, the HRS evaluations varied greatly and were divided over evaluations where the end user was involved and evaluations that did not evolve users (offline evaluations). Only 47% (34/73) of the studies reported implementing a user interface to communicate recommendations to the user, despite the need to show the rationale of recommendations, as echoed by many researchers and practitioners [ 11 ]. Moreover, only 15% (7/47) included a (basic) visualization.
Unfortunately, this general lack of agreement on how to report HRSs might introduce researcher bias, as a researcher is currently completely unconstrained in defining what and how to measure the added value of an HRS. Therefore, further debate in the health recommender community is needed on how to define and measure the impact of HRSs. On the basis of our review and contribution to this discussion, we put forward a set of essential information that researchers should report in their studies.
Considerations for Practice
The previously discussed results have direct implications in practice and provide suggestions for future research. Figure 6 shows a reference frame of these requirements that can be used in future studies as a quality assessment tool.

A reference frame to report health recommender system studies. On the basis of the results of this study, we suggest that it should be clear what and how items are recommended (A), who the target user is (B), which data are used (C), and which recommender techniques are applied (D). Finally, the evaluation design should be reported in detail (E).
Define the Target User
As shown in this review, HRSs are used in a plethora of subdomains and each domain has its own experts. For example, in nutrition, the expert is most likely a dietician. However, the user of an HRS is usually a layperson without the knowledge of these domain experts, who often have different viewing preferences [ 125 ]. Furthermore, each user is unique. All individuals have idiosyncratic reasons for why they act, think, behave, and feel in a certain way at a specific stage of their life [ 126 ]. Not everybody is motivated by the same elements. Therefore, it is important to know the target user of the HRS. What is their previous knowledge, what are their goals, and what motivates them to act on a recommended item?
Show What Is Recommended (and How)
Researchers have become aware that accuracy is not sufficient to increase the effectiveness of a recommender system [ 127 ]. In recent years, research on human factors has gained attention. For example, He et al [ 11 ] surveyed 24 existing interactive recommender systems and compared their transparency, justification, controllability, and diversity. However, none of these 24 papers discussed HRSs. This indicates the gap between HRSs and recommender systems in other fields. Human factors have gained interest in the recommender community by “combining interactive visualization techniques with recommendation techniques to support transparency and controllability of the recommendation process” [ 11 ]. However, in this study, only 10% (7/73) explained the rationale of recommendations and only 10% (7/73) included a visualization to communicate the recommendations to the user. We do not argue that all HRSs should include a visualization or an explanation. However, researchers should pay attention to the delivery of these recommendations. Users need to understand, believe, and trust the recommended items before they can act on it.
To compare and assess HRSs, researchers should unambiguously report what the HRS is recommending. After all, typical recommender systems act like a black box , that is, they show suggestions without explaining the provenance of these recommendations [ 11 ]. Although this approach is suitable for typical e-commerce applications that involve little risk, transparency is a core requirement in higher risk application domains such as health [ 128 ]. Users need to understand why a recommendation is made, to assess its value and importance [ 12 ]. Moreover, health information can be cumbersome and not always easy to understand or situate within a specific health condition [ 129 ]. Users need to know whether the recommended item or action is based on a trusted source, tailored to their needs, and actionable [ 130 ].
Report the Data Set Used
All 73 studies used a distinct data set. Furthermore, some studies combine data from multiple databases, making it even more difficult to judge the quality of the data [ 35 ]. Nonetheless, most studies use self-generated data sets. This makes it difficult to compare and externally validate HRSs. Therefore, we argued that researchers should clarify the data used and potentially share whether these data are publicly available. However, in health data are often highly privacy sensitive and cannot be shared among researchers.
Outline the Recommender Techniques
The results show that there is no panacea for which recommender technique to use. The included studies differ from logic filters to traditional recommender techniques, such as collaborative filtering and content-based filtering to hybrid solutions and self-developed algorithms. However, with 44% (32/73), there is a strong trend toward the use of hybrid recommender techniques. The low number of collaborative filter techniques might be related to the fact that the evaluation sample sizes were also relatively low. Unfortunately, some studies have not fully disclosed the techniques used and only reported on the main algorithm used. It is remarkable that studies published in high-impact journals, such as studies by Bidargaddi et al [ 45 ] and Cheung et al [ 83 ], did not provide information on the recommender technique used. Nonetheless, disclosing the recommender technique allows other researchers not only to build on empirically tested technologies but also to verify whether key variables are included [ 29 ]. User data and behavior data can be identified to augment theory-based studies [ 29 ]. Researchers should prove that the algorithm is capable of recommending valid and trustworthy recommendations to the user based on their available data set.
Elaborate on the Evaluation Protocols
HRSs can be evaluated using different evaluation protocols. However, the protocol should be outlined mainly by the research goals of the authors. On the basis of the papers included in this study, we differentiate between the two approaches. In the first approach, the authors aim to influence their users’ health, for example, by providing personalized diabetes guidelines [ 81 ] or prevention exercises for users with low back pain [ 95 ]. Therefore, the end user should always be involved in both the design and evaluation processes. However, only 8% (6/73) performed an RCT and 14% (10/73) deployed their HRS in the wild. This lack of user involvement has been noted previously by researchers and has been identified as a major challenge in the field [ 27 , 28 ]. Nonetheless, in other domains, such as job recommenders [ 131 ] or agriculture [ 132 ], user-centered design has been proposed as an important methodology in the design and development of tools used by end users, with the purpose of gaining trust and promoting technology acceptance, thereby increasing adoption with end users. Therefore, we recommend that researchers evaluate their HRSs with actual users. A potential model for a user-centric approach to recommender system evaluation is the user-centric framework proposed by Knijnenburg et al [ 117 ].
Research protocols need to be elaborated and approved by an ethical review board to prevent any impact on users. Authors should report how they informed their users and how they safeguarded the privacy of the users. This is in line with the modern journal and conference guidelines. For example, editorial policies of the Journal of Medical Internet Research state that “when reporting experiments on human subjects, authors should indicate IRB (Institutional Rese[a]rch Board, also known as REB) approval/exemption and whether the procedures followed were in accordance with the ethical standards of the responsible committee on human experimentation” [ 133 ]. However, only 12% (9/73) reported their approval by an ethical review board. Acquiring review board approval will help the field mature and transition from small incremental studies to larger studies with representative users to make more reliable and valid findings.
In the second approach, the authors aim to design a better algorithm, where better is again defined by the authors. For example, the algorithm might perform faster, be more accurate, and be more efficient in computing power. Although the F 1 score, the mean absolute error, and nDCG are well defined and known within the recommender domain, other parameters are more ambiguous. For example, the performance or effectiveness can be assessed using different measurements. However, a health parameter can be monitored, such as the duration that a user remains within healthy ranges [ 81 ]. Furthermore, it could be a predictive parameter, such as an improved precision and recall as a proxy for performance [ 72 ]. Unfortunately, this difference makes it difficult to compare health recommendation algorithms. Furthermore, this inconsistency in measurement variables makes it infeasible to report in this systematic review which recommender techniques to use. Therefore, we argue that HRS algorithms should always be evaluated for other researchers to validate the results, if needed.
Limitations
This study has some limitations that affect its contribution. Although an extensive scope search was conducted in scientific databases and most relevant health care informatic journals, some relevant literature in other domains might have been excluded. The keywords used in the search string could have impacted the results. First, we did not include domain-specific constructs of health, such as asthma, pregnancy, and iron deficiency. Many studies may implicitly report healthy computer-generated recommendations when they research the impact of a new intervention. In these studies, however, building an HRS is often not their goal and, therefore, was excluded from this study. Second, we searched for papers that reported studying an HRS; nonincluded studies might have built an HRS but did not report it as such. Considering our RQs, we deemed it important that authors explicitly reported their work as a recommender system. To conclude, in this study, we provide a large cross-domain overview of health recommender techniques targeted to laypersons and deliver a set of recommendations that could help the field of HRS mature.
This study presents a comprehensive report on the use of HRS across domains. We have discussed the different subdomains HRS applied in, the different recommender techniques used, the different manners in which they are evaluated, and finally, how they present the recommendations to the user. On the basis of this analysis, we have provided research guidelines toward a consistent reporting of HRSs. We found that although most applications are intended to improve users’ well-being, there is a significant opportunity for HRSs to inform and guide users’ health actions. Although many of the studies present a lack of a user-centered evaluation approach, some studies performed full-scale RCT evaluations or elaborated in the wild studies to validate their HRS, showing the field of HRS is slowly maturing. On the basis of this study, we argue that it should always be clear what the HRS is recommending and to whom these recommendations are for. Graphical assets should be added to show how recommendations are presented to users. Authors should also report which data sets and algorithms were used to calculate the recommendations. Finally, detailed evaluation protocols should be reported.
We conclude that the results motivate the creation of richer applications in future design and development of HRSs. The field is maturing, and interesting opportunities are being created to inform and guide health actions.
Acknowledgments
This work was part of the research project PANACEA Gaming Platform with project HBC.2016.0177, which was financed by Flanders Innovation & Entrepreneurship and research project IMPERIUM with research grant G0A3319N from the Research Foundation-Flanders (FWO) and the Slovenian Research Agency grant ARRS-N2-0101. Project partners were BeWell Innovations and the University Hospital of Antwerp.
Abbreviations
Multimedia appendix 1, multimedia appendix 2, multimedia appendix 3.
Conflicts of Interest: None declared.
- Explore AI by Industry PLUS
- Consumer goods
- Heavy industry
- Natural resources
- Professional services
- Transportation
- AI Best Practice Guides PLUS
- AI White Paper Library PLUS
- AI Business Process Explorer PLUS
- Enterprise AI Newsletter
- Emerj Plus Research
- AI in Business Podcast
- The AI Consulting Podcast
- AI in Financial Services Podcast
- Precisely – Building Trust in Data
- Shift Technology – How Insurers are Using AI
- Uniphore – The Future of Banking CX in APAC
- Uniphore – The Economic Impact of Conversational AI and Automation
- Uniphore – The Future of Complaints Management
- Uniphore – Conversational AI in Banking
Use Cases of Recommendation Systems in Business – Current Applications and Methods
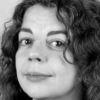
Corinna Underwood has been a published author for more than a decade. Her non-fiction has been published in many outlets including Fox News, CrimeDesk24, Life Extension, Chronogram, After Dark and Alive.
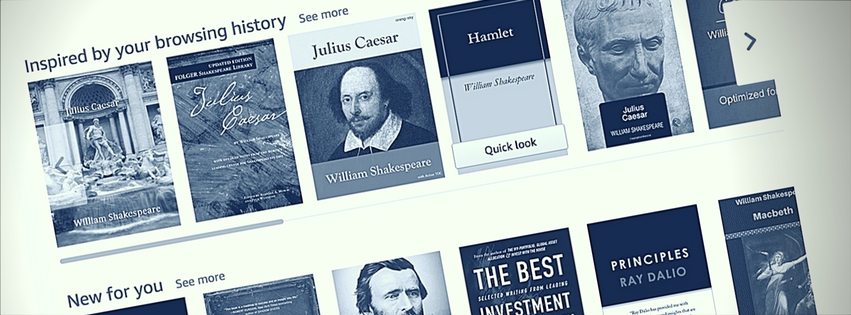
An increasing number of online companies are utilizing recommendation systems to increase user interaction and enrich shopping potential. Use cases of recommendation systems have been expanding rapidly across many aspects of eCommerce and online media over the last 4-5 years, and we expect this trend to continue.
Recommendation systems (often called “recommendation engines”) have the potential to change the way websites communicate with users and to allow companies to maximize their ROI based on the information they can gather on each customer’s preferences and purchases.
This article breaks down the insights that non-technical managers and execs should understand about the business applications of recommendation systems, including:
- Common benefits of recommendation systems (with examples)
- Basic terminology / approaches / algorithms of recommendation engines
- Current recommendation engine use-cases at Amazon, Netflex, BestBuy, and others
- Potential future trends and improvements to today’s recommendation engines
We’ll start off with some of the major benefits that recommendation systems offer businesses:

Potential Benefits of Recommendation Engines
Below are some of the various potential benefits of recommendation systems in business, and the companies that use them:
- You’re much less likely to switch to a Netflix competitor when Netflix has such a wonderful sense of which movies and shows you might want to watch next (i.e. they “know you so well”). Because most of Netflix’s revenues come from a fixed-rate recurring billing model subscription, the company’s biggest ROI “win” with recommendation systems is retention.
- Amazon’s quick delivery and emphasis on customer service has earned them millions of customers. Recommendation engines play a role not only in helping customers find more of what they need (and see Amazon as an authority), but these systems also improve cart value. If Amazon doesn’t have to pay much more for shipping to send you two or three times as many products, their profit margins improve.
- YouTube has subscription options, but the majority of the firm’s revenues are driven through advertisements placed across its wide array of video properties. The company makes more money when users come back time and time again. YouTube doesn’t optimize for short-term view length, as this might encourage pushy or flashy tactics that wouldn’t genuinely delight users. Instead, the service aims to encourage long-term use, because advertising views is the ROI that these systems serve at YouTube. Facebook is another obvious example of a similar application of recommendation engines.
It’s also important to note that recommendation systems (a) are likely only to be a fit for companies with enough data and in-house AI talent to use them well, and that (b) many businesses and business models may be better off not using recommendation systems as they are not guaranteed to be a higher yield approach than the alternatives.
That being said, there are some sectors (most notably digital media, eCommerce) where such systems seem to be borderline inevitable.
Recommendation Engine / Recommendation System Fundamental Terms
Recommendation systems are important and valuable tools for companies like Amazon and Netflix, who are both known for their personalized customer experiences. Each of these companies collects and analyzes demographic data from customers and adds it to information from previous purchases, product ratings, and user behavior.
These details are then used to predict how customers will rate sets of related products, or how likely a customer is to buy an additional product.
Before diving into specific recommendation engine applications from well-known retailers and online services, I think it’s important for us to explain the different types of recommendation systems . We’ve linked to further reading in case some of you would like to dive further into the science behind each of these approaches:
- Collaborative filtering : This type of recommendation system makes predictions of what might interest a person based on the taste of many other users. It assumes that if person X likes Snickers, and person Y likes Snickers and Milky Way, then person X might like Milky Way as well.
- Content-based filtering : This type of recommendation system focuses on the products themselves and recommends other products that have similar attributes. Content-based filtering relies on the characteristics of the products themselves, so it doesn’t rely on other users to interact with the products before making a recommendation.
- Demographic based recommender system : This type of recommendation system categorizes users based on a set of demographic classes. This algorithm requires market research data to fully implement. The main benefit is that it doesn’t need history of user ratings.
- Utility-based recommender system: This type of system makes recommendations based on a computation of its usefulness for each individual user. This relies on each industry’s ability to decide on a user-specific utility function. The main advantage of this system is it can make recommendation that are unrelated product’s attributes, such as availability and vendor reliability.
- Knowledge based Recommender System : This type of system makes suggestions based on information relating to each user’s preferences and needs. Using function knowledge it can draw connections between a customer’s need and a suitable product.
- Hybrid filtering : This type of recommendation system can implement a combination fo any two of the above systems.
(Readers with a deeper interest in understanding the applications of recommendation engines may want to listen to our full recommendation engine interview with AI researcher Raefer Gabriel .)
Return on investment (ROI) ultimately has to boil down to (a) saving more money, or (b) making more money. Businesses don’t invest time and resources into recommendation engines to be “hip” (we’ve warned companies against creating “test applications” of AI that aren’t directly tied to value). Smart companies who leverage these systems do so to improve their bottom line.
However, “improving the bottom line” can be accomplished through a variety of means, and each company will approach the problem differently. Recommendation engines don’t serve the same purpose across companies, and it’s important for business leaders to get a sense of what different kinds of goals a recommendation engine can aim to accomplish.
Real World Applications Today
Now that we’ve covered some of the basic benefits and terminology, we’ll explore applications of recommendation engines across large and well-known companies, beginning with Amazon.
1 – Amazon
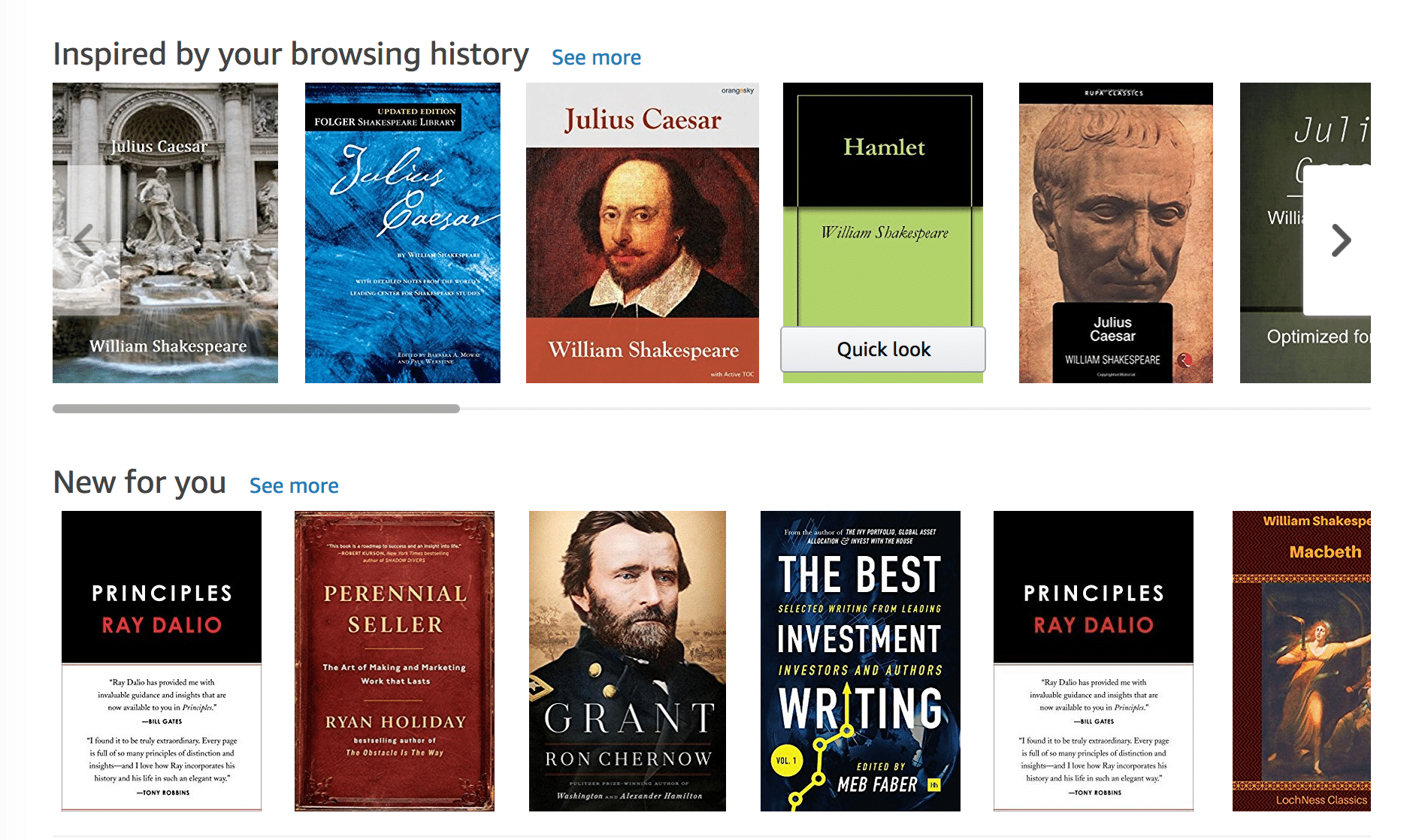
Amazon has single-handedly put a spotlight on the retail value of AI , and recommendations are part of what put the company on the map (in addition to their robotics initiatives, and AWS).
Amazon.com uses recommendations as a targeted marketing tool throughout its website. When a customer clicks on the “your recommendations” the link leads to another page where recommendations may be filtered even further by subject area, product types, and ratings of previous products and purchases. The customer can even see why a particular product has been recommended.
“ At Amazon.com, we use recommendation algorithms to personalize the online store for each customer. The store radically changes based on customer interests, showing programming titles to a software engineer and baby toys to a new mother,” explain Greg Linden, Brent Smith, and Jeremy York in their paper Amazon.com Recommendations: Item-to-Item Collaborative Filtering .
In this instance, collaborative filtering doesn’t just match each use to similar customers. The item-to-item connects each user’s purchase to similar items and compiles a recommendation list from them. For example, if you’re enthusiastic about the latest technology, you may find your Amazon web page suggests the latest device and gadgets, if cooking is your thing, you’re sure to find plenty of recommendations for recipe books and cookware.
According to McKinsey & Company , 35% of Amazon.com’s revenue is generated by its recommendation engine.
2 – Netflix
According to a paper written by Netflix executives Carlos A. Gomez-Uribe and Neil Hunt, the video streaming service’s AI recommendation system saves the company around $1 billion each year. This allows them to invest more money on new content which viewers will continue to view, giving them a good ROI.
“We have discovered through the years that there is tremendous value to our subscribers in incorporating recommendations to personalize as much of Netflix as possible,” say by Xavier Amatriain and Justin Basilico (Personalization Science and Engineering) in their Netflix Tech Blog.
Here’s how Netflix explains their recommendation engine on their own YouTube channel:
Netflix uses RS personalized diversity to generate Top Ten recommendations for user households, so that it can offer videos that each member of the household may be interested in. The company also focuses on awareness and promoting trust to help develop its personalized approach. Netflix implements these strategies by explaining why it makes video recommendation and encouraging members to give feedback, so no opportunities to personalize are missed.
According to McKinsey, 75 percent of what users watch on Netflix come from product recommendations. We spoke openly about the general web trend of “personalization” and recommendation in our AI podcast with Adam Spector of LiftIgniter , which might be worth a listen for executives considering how recommendations might be used in their own business.
3 – Spotify
In the presentation below, Spotify’s Christopher Johnson (who was previously working on a PhD in machine learning at UT Austin) explains the basics of Spotify’s music recommendation approach:
Possibly one of Spotify’s most innovative uses of AI and recommendation systems is their popular Discover Weekly playlist. Known as Release Radar, this algorithmically powered tool updates personal playlists on a weekly basis so that users won’t miss newly released music by artists they like.
“With the huge amount of new music released every week, it can be difficult to keep up with the latest tracks,” Spotify’s Matt Ogle, who leads the development of Discover Weekly, said in a statement . “With Release Radar, we wanted to create the simplest way for you to find all the newly released music that matters the most to you, in one playlist.”
Discover Weekly works by looking at the 2 billion plus playlist created by users, each based on music fans’ individual tastes. Spotify then collates this information with the company’s own playlists and fills in the blanks by comparing a user’s listening habits to those of users with similar tastes. The approach also uses collaborative filtering in combination with deep learning to detect patterns within huge amount of data to improve weekly selections.
The new recommendation system has helped Spotify increase its number of monthly users from 75 million to 100 million at a time, in spite of competition from rival streaming service Apple Music.
4 – Best Buy
Another company utilizing RS to increase revenues and improve customer experience is Best Buy . The company’s strategy is based on query search and click data. Since 2015, Best Buy has used the information in an attempt to predict what customers are interested in. The query-based and item-to-item system creates cluster models that allow the company to make customer recommendations.
“We’ve had a very material transformation of our marketing efforts in the last three years, with the headline being, of course, more personalized. We went from analog and mass communication to much more targeted, relevant, personalized and digital communications,” said CEO Hubert Joly in an interview with Diginomica .
Best Buy has been using its recommendation system for eCommerce since 2015. The system works by predicting what a customer is interested in based on their individual browsing and purchase data. President of Global E-Commerce Scott Durchslag said that he wants Best Buy to become “the Netflix of consumer electronics.”
In 2016, CNBC reported a 23.7 percent growth in online sales for Best Buy, which marked a second straight quarter of almost 24 percent increase. The growth was attributes in part to the company’s improved recommendation system.
5 – YouTube
Fortunately for us, YouTube has a series of “Help” videos that answer basic user questions about YouTube and the technology that supports it. Below is a three minute explanation of how YouTube provides its video recommendations:
The YouTube online video community uses RS to create personalized recommendations so users can quickly and easily find videos that are relevant to their interests. Because of the value of keeping users engages, YouTube strives to keep the recommendations updated on a regular basis, to reflect each user’s activity on the site and to simultaneously highlight the wide range of available content.
“We believe that for every human being on earth, there’s 100 hours of YouTube that they would love to watch,” Christos Goodrow, YouTube’s director of engineering for search and discovery told Business Insider . “And the content is already there. We have billions of videos. So we start with that premise and then it’s our job to help viewers to find the videos that they would enjoy watching.”
The RS is driven by the Google Brain deep learning artificial intelligence project and is comprised of two neural networks. The first collects and collates information on users’ watch history and uses collaborative filtering to select hundreds of videos. This process, known as candidate generation, uses feedback from users to train the model. The second neural network ranks the selected videos in order to make recommendations to users.
According to YouTube after implementation of the RS for more than a year, it has been successful in terms of their stated goals, with recommendations accounting for around 60 percent of video clicks from the homepage.
Concluding Thoughts on Recommendation / Personalization in Business
Companies across many different areas of enterprise are beginning to implement recommendation systems in an attempt to enhance their customer’s online purchasing experience, increase sales and retain customers. Business owners are recognizing potential in the fact that recommendation systems allow the collection of a huge amount of information relating to user’s behavior and their transactions within an enterprise. This information can then be systematically stored within user profiles to be used for future interactions.
As well as improving customer experience, the information gathered from a recommendation system can also be used as an ad targeting tool. By integrating a recommendation system with ad exchanges, a business may have the ability to target other website users with products they have liked on the company’s site.
Revenues can be increased using simple strategies such as:
- Adding matching product recommendations to your purchase confirmation
- Collecting information about abandoned shopping carts
- Sharing “what customers are buying now”
- Sharing other customer’s views and purchases
- Making personalized recommendations
Another way to make good use of the wealth of information garnered from recommendation systems is to trigger emails based on online interactions. For example, a business could send an email to a user who viewed five pages of laptops with a discount coupon or code for a selection of those products. Businesses can also use reverse triggers to send emails targeted at products that the user has not yet viewed.
As more and more products become available online, recommendation engines are crucial to the future of e-commerce. Not only because they help increase customer sales and interactions, but also because they will continue to help companies weed out their inventory so they can supply customers with products they really like.
The next generation of recommendation systems may include the following improvements:
- More relevant recommendations : By digging deeper into customers’ interests and preferences, recommendation systems will be able to present users with more-relevant, predictive recommendations.
- Incorporate item profitability : Instead of having recommendation based solely on a customer’s browsing history and past purchases, this would allow businesses to control how much a profit-based recommendation differs from the traditional recommendation and to set a balance so that customer trust would not be compromised.
- Increase product reach : Each retailer has an individual catalogue of products, improved recommendation systems would be able to access a broader range of merchandise in order to include new or niche items in shoppers’ recommendations.
- Reach shoppers through multiple channels : Next generation recommendation systems should be able to reach customers across a range of channels including email, social media, on an off-site shopping widgets, mobile apps, and the retail customer service centers.
According to a press release from IDC, worldwide spending on cognitive and AI systems is predicted to reach $12.5 billion in 2017 (we cover additional projections in our “AI Market Size” article ). The largest areas of spending ($4.5 billion) will be cognitive applications; which include systems which are able to make recommendations or predictions.
Stay in touch with our marketing category (or our newsletter, in the footer of this page) to read more of our updates about cutting-edge AI marketing applications.
Image credit: Amazon
Related Posts
Reuters referenced a Stratistics MRC figure estimating the size of the business intelligence industry around $15.64…
Decision-makers in the banking sector have a unique set of business intelligence needs, and artificial…
In 2017, Emerj conducted research into the applications of machine learning in marketing with 51…
In their recent Worldwide Spending on Cognitive and Artificial Intelligence Systems report, IDC estimated that…
The retail industry collects massive amounts of data every day, and this makes its key…
Related posts (5)
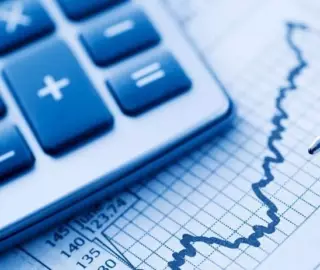
Business Intelligence in Finance – Current Applications
Reuters referenced a Stratistics MRC figure estimating the size of the business intelligence industry around $15.64 billion in 2016. It follows that AI would find its way into the business intelligence world. In our previous report, we covered 6 use-cases for AI in business intelligence. As of now, numerous companies claim to assist business leaders in the finance domain, specifically, in aspects of their roles using AI.
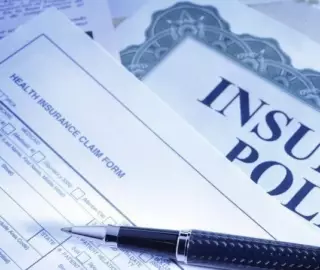
Business Intelligence in Insurance – Current Applications
In the past few decades, insurance companies have collected vast amounts of data relevant to their business processes, customers, claims, and so on. This data can be unstructured in the form of PDFs, text documents, images, and videos, or structured, organized and curated for big data analytics.
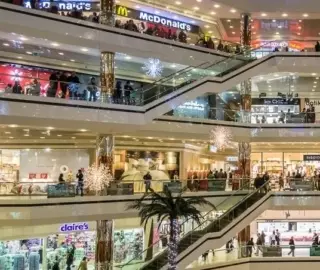
Business Intelligence in Retail – Current Applications
In 2017, Emerj conducted research into the applications of machine learning in marketing with 51 different AI-focused marketing executives. The AI marketing vendors we spoke to named retail and eCommerce as the top sectors ripe for applying marketing AI software. Below is a graphic from our research showing the sectors that AI marketing vendors sell into most:
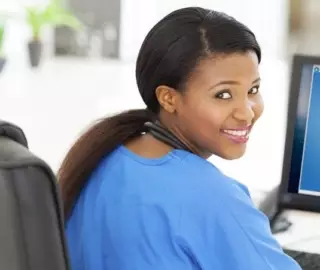
Business Intelligence in Healthcare – Current Applications
According to Deloitte, global healthcare spending is expected to grow annually by 4.1% from 2017-2021, up from just 1.3% in 2012-2016. The report suggests this growth will be fuelled by aging, rising populations, the growth of developing markets, advances in medical treatments, and rising labor costs.
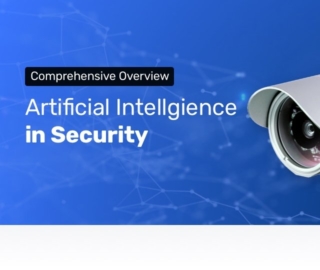
AI in Biometrics and Security – Current Business Applications
Biometric solutions are typically used for security and access control across businesses and government organizations. The U.S. government has taken keen interest in biometric applications and has been aggressively funding advanced research programs in businesses that offer biometrics.
- Market Reasearch and Advisory
- AI Presentations and Keynotes
- Emerj Plus Membership
- AI In Business Podcast
- AI In Finance Services Podcast
- Subscribe to our AI Newsletter
- Advertise with us
- Terms and Conditions
- Refund and Cancellation Policy
- Privacy Policy
How To Make Recommendation in Case Study (With Examples)
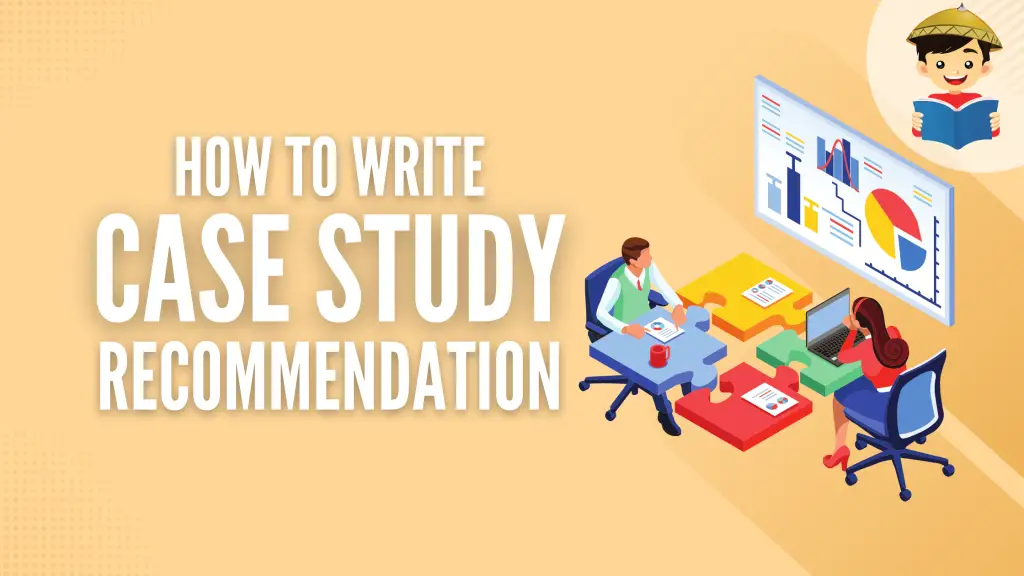
After analyzing your case study’s problem and suggesting possible courses of action , you’re now ready to conclude it on a high note.
But first, you need to write your recommendation to address the problem. In this article, we will guide you on how to make a recommendation in a case study.
Table of Contents
What is recommendation in case study, what is the purpose of recommendation in the case study, 1. review your case study’s problem, 2. assess your case study’s alternative courses of action, 3. pick your case study’s best alternative course of action, 4. explain in detail why you recommend your preferred course of action, examples of recommendations in case study, tips and warnings.
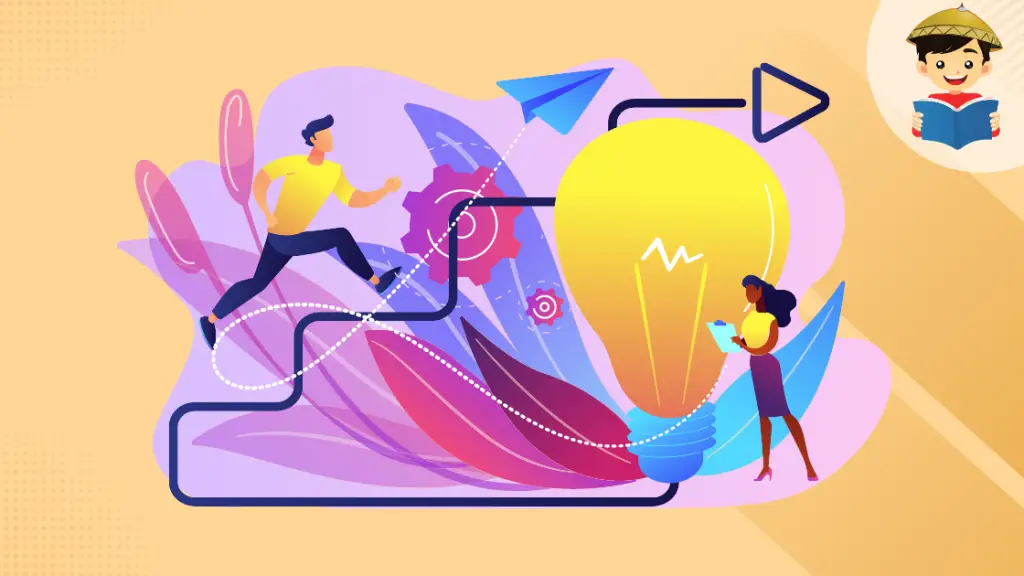
The Recommendation details your most preferred solution for your case study’s problem.
After identifying and analyzing the problem, your next step is to suggest potential solutions. You did this in the Alternative Courses of Action (ACA) section. Once you’re done writing your ACAs, you need to pick which among these ACAs is the best. The chosen course of action will be the one you’re writing in the recommendation section.
The Recommendation portion also provides a thorough justification for selecting your most preferred solution.
Notice how a recommendation in a case study differs from a recommendation in a research paper . In the latter, the recommendation tells your reader some potential studies that can be performed in the future to support your findings or to explore factors that you’re unable to cover.
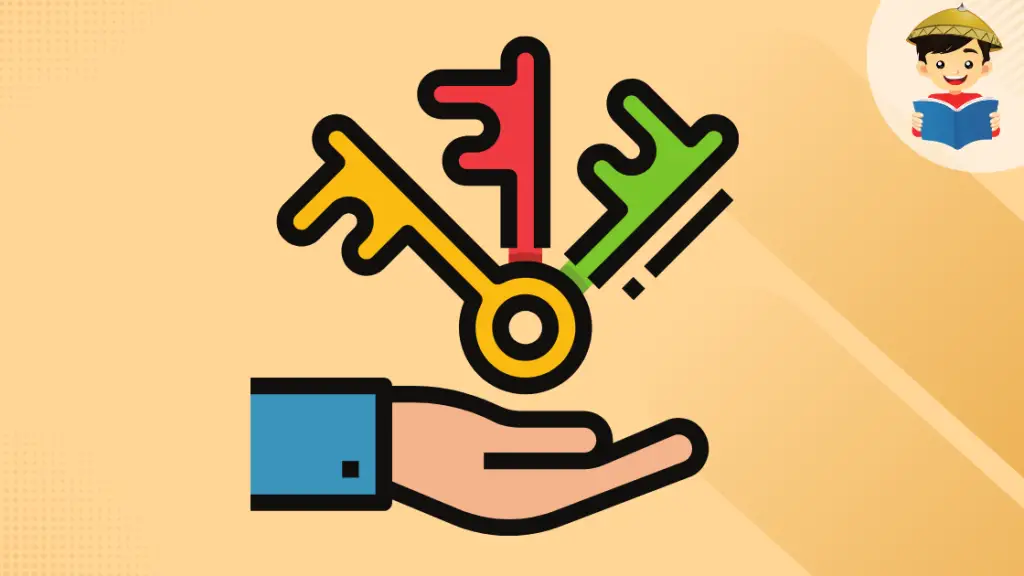
Your main goal in writing a case study is not only to understand the case at hand but also to think of a feasible solution. However, there are multiple ways to approach an issue. Since it’s impossible to implement all these solutions at once, you only need to pick the best one.
The Recommendation portion tells the readers which among the potential solutions is best to implement given the constraints of an organization or business. This section allows you to introduce, defend, and explain this optimal solution.
How To Write Recommendation in Case Study
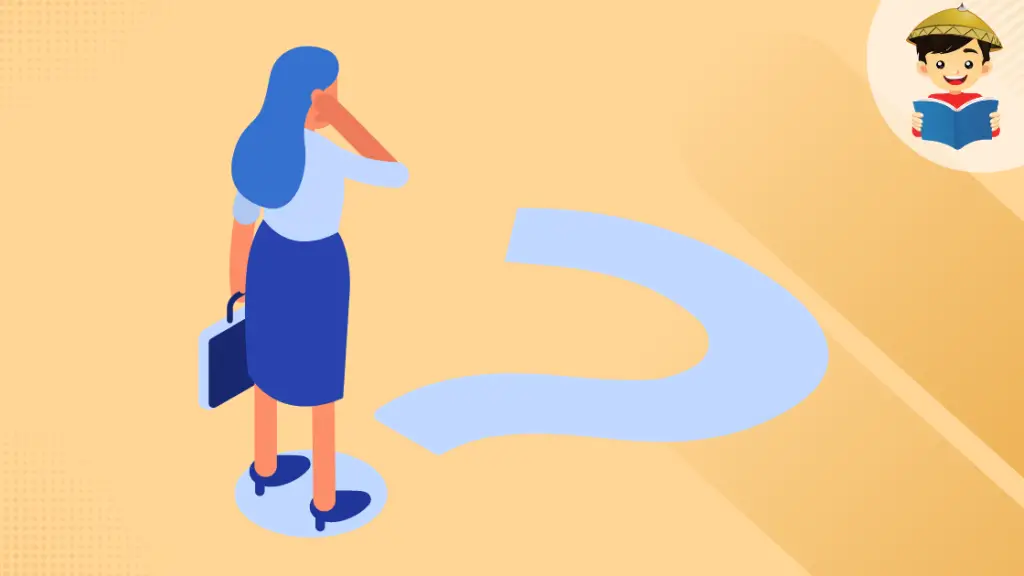
You cannot recommend a solution if you are unable to grasp your case study’s issue. Make sure that you’re aware of the problem as well as the viewpoint from which you want to analyze it .
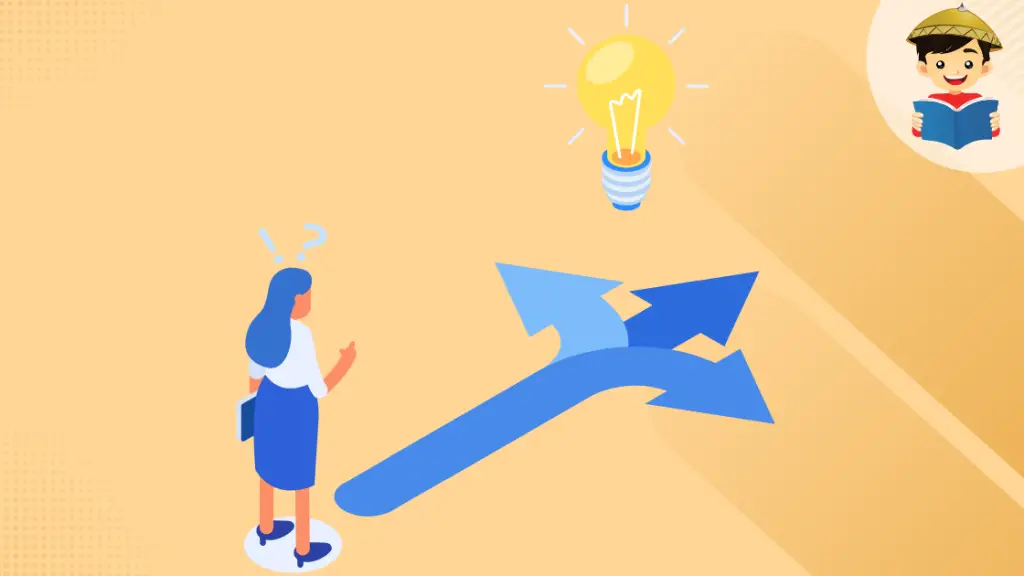
Once you’ve fully grasped your case study’s problem, it’s time to suggest some feasible solutions to address it. A separate section of your manuscript called the Alternative Courses of Action (ACA) is dedicated to discussing these potential solutions.
Afterward, you need to evaluate each ACA by identifying its respective advantages and disadvantages.
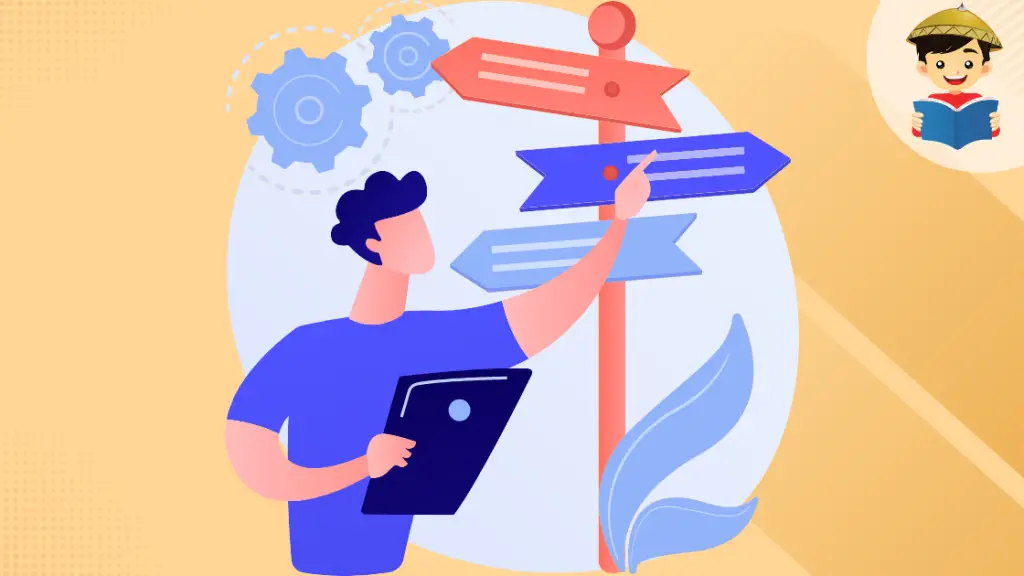
After evaluating each proposed ACA, pick the one you’ll recommend to address the problem. All alternatives have their pros and cons so you must use your discretion in picking the best among these ACAs.
To help you decide which ACA to pick, here are some factors to consider:
- Realistic : The organization must have sufficient knowledge, expertise, resources, and manpower to execute the recommended solution.
- Economical: The recommended solution must be cost-effective.
- Legal: The recommended solution must adhere to applicable laws.
- Ethical: The recommended solution must not have moral repercussions.
- Timely: The recommended solution can be executed within the expected timeframe.
You may also use a decision matrix to assist you in picking the best ACA 1 . This matrix allows you to rank the ACAs based on your criteria. Please refer to our examples in the next section for an example of a Recommendation formed using a decision matrix.
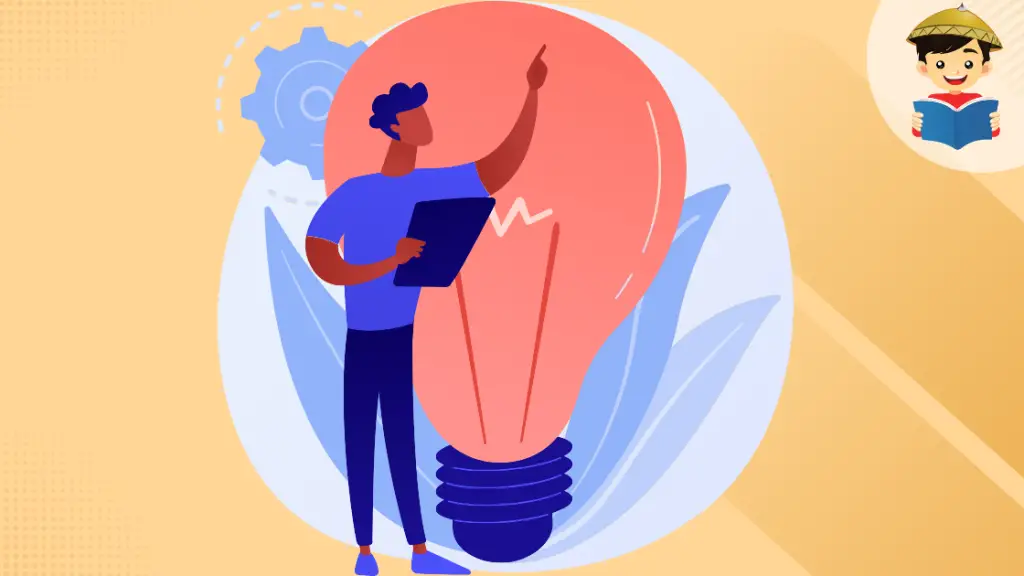
Provide your justifications for why you recommend your preferred solution. You can also explain why other alternatives are not chosen 2 .
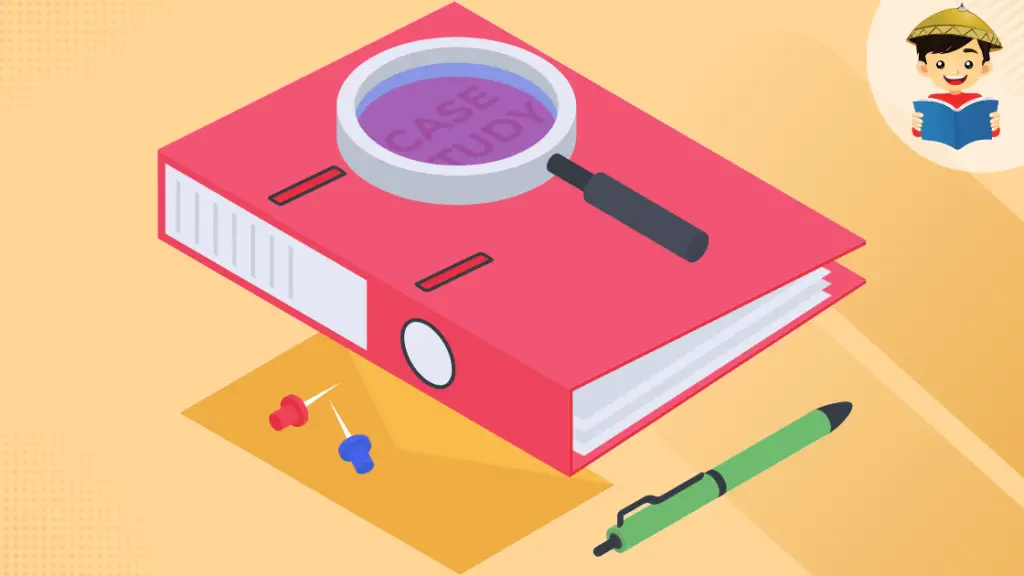
To help you understand how to make recommendations in a case study, let’s take a look at some examples below.
Case Study Problem : Lemongate Hotel is facing an overwhelming increase in the number of reservations due to a sudden implementation of a Local Government policy that boosts the city’s tourism. Although Lemongate Hotel has a sufficient area to accommodate the influx of tourists, the management is wary of the potential decline in the hotel’s quality of service while striving to meet the sudden increase in reservations.
Alternative Courses of Action:
- ACA 1: Relax hiring qualifications to employ more hotel employees to ensure that sufficient human resources can provide quality hotel service
- ACA 2: Increase hotel reservation fees and other costs as a response to the influx of tourists demanding hotel accommodation
- ACA 3: Reduce privileges and hotel services enjoyed by each customer so that hotel employees will not be overwhelmed by the increase in accommodations.
Recommendation:
Upon analysis of the problem, it is recommended to implement ACA 1. Among all suggested ACAs, this option is the easiest to execute with the minimal cost required. It will not also impact potential profits and customers’ satisfaction with hotel service.
Meanwhile, implementing ACA 2 might discourage customers from making reservations due to higher fees and look for other hotels as substitutes. It is also not recommended to do ACA 3 because reducing hotel services and privileges offered to customers might harm the hotel’s public reputation in the long run.
The first paragraph of our sample recommendation specifies what ACA is best to implement and why.
Meanwhile, the succeeding paragraphs explain that ACA 2 and ACA 3 are not optimal solutions due to some of their limitations and potential negative impacts on the organization.
Example 2 (with Decision Matrix)
Case Study: Last week, Pristine Footwear released its newest sneakers model for women – “Flightless.” However, the management noticed that “Flightless” had a mediocre sales performance in the previous week. For this reason, “Flightless” might be pulled out in the next few months. The management must decide on the fate of “Flightless” with Pristine Footwear’s financial performance in mind.
- ACA 1: Revamp “Flightless” marketing by hiring celebrities/social media influencers to promote the product
- ACA 2: Improve the “Flightless” current model by tweaking some features to fit current style trends
- ACA 3: Sell “Flightless” at a lower price to encourage more customers
- ACA 4: Stop production of “Flightless” after a couple of weeks to cut losses
Decision Matrix
Recommendation
Based on the decision matrix above 3 , the best course of action that Pristine Wear, Inc. must employ is ACA 3 or selling “Flightless” shoes at lower prices to encourage more customers. This solution can be implemented immediately without the need for an excessive amount of financial resources. Since lower prices entice customers to purchase more, “Flightless” sales might perform better given a reduction in its price.
In this example, the recommendation was formed with the help of a decision matrix. Each ACA was given a score of between 1 – 4 for each criterion. Note that the criterion used depends on the priorities of an organization, so there’s no standardized way to make this matrix.
Meanwhile, the recommendation we’ve made here consists of only one paragraph. Although the matrix already revealed that ACA 3 tops the selection, we still provided a clear explanation of why it is the best.
- Recommend with persuasion 4 . You may use data and statistics to back up your claim. Another option is to show that your preferred solution fits your theoretical knowledge about the case. For instance, if your recommendation involves reducing prices to entice customers to buy higher quantities of your products, you may invoke the “law of demand” 5 as a theoretical foundation of your recommendation.
- Be prepared to make an implementation plan. Some case study formats require an implementation plan integrated with your recommendation. Basically, the implementation plan provides a thorough guide on how to execute your chosen solution (e.g., a step-by-step plan with a schedule).
- Manalili, K. (2021 – 2022). Selection of Best Applicant (Unpublished master’s thesis). Bulacan Agricultural State College. Retrieved September 23, 2022, from https://www.studocu.com/ph/document/bulacan-agricultural-state-college/business-administration/case-study-human-rights/19062233.
- How to Analyze a Case Study. (n.d.). Retrieved September 23, 2022, from https://wps.prenhall.com/bp_laudon_essbus_7/48/12303/3149605.cw/content/index.html
- Nguyen, C. (2022, April 13). How to Use a Decision Matrix to Assist Business Decision Making. Retrieved September 23, 2022, from https://venngage.com/blog/decision-matrix/
- Case Study Analysis: Examples + How-to Guide & Writing Tips. (n.d.). Retrieved September 23, 2022, from https://custom-writing.org/blog/great-case-study-analysis
- Hayes, A. (2022, January O8). Law of demand. Retrieved September 23, 2022, from https://www.investopedia.com/terms/l/lawofdemand.asp
Written by Jewel Kyle Fabula
in Career and Education , Juander How
Last Updated September 23, 2022 07:23 PM
Jewel Kyle Fabula
Jewel Kyle Fabula is a Bachelor of Science in Economics student at the University of the Philippines Diliman. His passion for learning mathematics developed as he competed in some mathematics competitions during his Junior High School years. He loves cats, playing video games, and listening to music.
Browse all articles written by Jewel Kyle Fabula
Copyright Notice
All materials contained on this site are protected by the Republic of the Philippines copyright law and may not be reproduced, distributed, transmitted, displayed, published, or broadcast without the prior written permission of filipiknow.net or in the case of third party materials, the owner of that content. You may not alter or remove any trademark, copyright, or other notice from copies of the content. Be warned that we have already reported and helped terminate several websites and YouTube channels for blatantly stealing our content. If you wish to use filipiknow.net content for commercial purposes, such as for content syndication, etc., please contact us at legal(at)filipiknow(dot)net

An official website of the United States government
Here’s how you know
Official websites use .gov A .gov website belongs to an official government organization in the United States.
Secure .gov websites use HTTPS A lock ( Lock A locked padlock ) or https:// means you’ve safely connected to the .gov website. Share sensitive information only on official, secure websites.
- Press Releases
Cyber Safety Review Board Releases Report on Microsoft Online Exchange Incident from Summer 2023
WASHINGTON – Today, the U.S. Department of Homeland Security (DHS) released the Cyber Safety Review Board’s (CSRB) findings and recommendations following its independent review of the Summer 2023 Microsoft Exchange Online intrusion . The review detailed operational and strategic decisions that led to the intrusion and recommended specific practices for industry and government to implement to ensure an intrusion of this magnitude does not happen again. Secretary of Homeland Security Alejandro N. Mayorkas received the CSRB report from the Board and delivered it to President Biden. This is the third review completed by the CSRB since the Board was announced in February 2022.
“Individuals and organizations across the country rely on cloud services every day, and the security of this technology has never been more important,” said Secretary Mayorkas . “Nation-state actors continue to grow more sophisticated in their ability to compromise cloud service systems. Public-private partnerships like the CSRB are critical in our efforts to mitigate the serious cyber threat these nation-state actors pose. The Department of Homeland Security appreciates the Board’s comprehensive review and report of the Storm-0558 incident. Implementation of the Board’s recommendations will enhance our cybersecurity for years to come.”
The CSRB provides a unique forum for leading government and industry experts to review significant cybersecurity events and provide independent, strategic, and actionable recommendations to the President, the Secretary, and the Director of the Cybersecurity and Infrastructure Security Agency (CISA) to better protect our nation. The Board is made up of cybersecurity leaders from the private sector and senior officials from DHS, CISA, the Defense Department, the National Security Agency, the Department of Justice, the Federal Bureau of Investigation, the Office of the National Cyber Director, and the Federal Chief Information Officer.
In August 2023, DHS announced that the CSRB would assess the recent Microsoft Exchange Online intrusion, initially reported in July 2023, and conduct a broader review of issues relating to cloud-based identity and authentication infrastructure affecting applicable cloud service providers (CSP) and their customers. The CSRB obtained data from and conducted interviews with 20 organizations and experts including cybersecurity companies, technology companies, law enforcement organizations, security researchers, academics, as well as several impacted organizations.
The inclusive review process developed actionable findings and recommendations. As a result of the CSRB’s recommendations, CISA plans to convene major CSPs to develop cloud security practices aligned with the CSRB recommendations and a process for CSPs to regularly attest and demonstrate alignment.
“DHS is committed to efforts that meaningfully improve cybersecurity resilience and preparedness for our nation, and the work of the CSRB is reflective of our determination and dedication to this cause,” said CISA Director Jen Easterly . “I am confident that the findings and recommendations from the Board’s report will catalyze action to reduce risk to the critical infrastructure Americans rely on every day.”
The CSRB’s review found that the intrusion by Storm-0558, a hacking group assessed to be affiliated with the People’s Republic of China, was preventable. It identified a series of Microsoft operational and strategic decisions that collectively pointed to a corporate culture that deprioritized enterprise security investments and rigorous risk management, at odds with the company’s centrality in the technology ecosystem and the level of trust customers place in the company to protect their data and operations. The Board recommends that Microsoft develop and publicly share a plan with specific timelines to make fundamental, security-focused reforms across the company and its suite of products. Microsoft fully cooperated with the Board’s review.
“Cloud computing is some of the most critical infrastructure we have, as it hosts sensitive data and powers business operations across our economy,” said DHS Under Secretary of Policy and CSRB Chair Robert Silvers . “It is imperative that cloud service providers prioritize security and build it in by design. The Board has become the authoritative organization for conducting fact-finding and issuing recommendations in the wake of major cyber incidents, receiving extensive industry and expert input in each of its three reviews to date. We appreciate Microsoft’s full cooperation in the course of the Board’s seven-month, independent review. We also appreciate the input received from 19 additional companies, government agencies, and individual experts.”
“The threat actor responsible for this brazen intrusion has been tracked by industry for over two decades and has been linked to 2009 Operation Aurora and 2011 RSA SecureID compromises,” said CSRB Acting Deputy Chair Dmitri Alperovitch . “This People’s Republic of China affiliated group of hackers has the capability and intent to compromise identity systems to access sensitive data, including emails of individuals of interest to the Chinese government. Cloud service providers must urgently implement these recommendations to protect their customers against this and other persistent and pernicious threats from nation-state actors.”
The CSRB recommends specific actions to all cloud service providers and government partners to improve security and build resilience against the types of attacks conducted by Storm-0558 and associated groups. Select recommendations include:
- Cloud Service Provider Cybersecurity Practices: Cloud service providers should implement modern control mechanisms and baseline practices, informed by a rigorous threat model, across their digital identity and credential systems to substantially reduce the risk of system-level compromise.
- Audit Logging Norms: Cloud service providers should adopt a minimum standard for default audit logging in cloud services to enable the detection, prevention, and investigation of intrusions as a baseline and routine service offering without additional charge.
- Digital Identity Standards and Guidance: Cloud service providers should implement emerging digital identity standards to secure cloud services against prevailing threat vectors. Relevant standards bodies should refine, update, and incorporate these standards to address digital identity risks commonly exploited in the modern threat landscape.
- Cloud Service Provider Transparency: Cloud service providers should adopt incident and vulnerability disclosure practices to maximize transparency across and between their customers, stakeholders, and the United States government.
- Victim Notification Processes: Cloud service providers should develop more effective victim notification and support mechanisms to drive information-sharing efforts and amplify pertinent information for investigating, remediating, and recovering from cybersecurity incidents.
- Security Standards and Compliance Frameworks: The United States government should update the Federal Risk Authorization Management Program and supporting frameworks and establish a process for conducting discretionary special reviews of the program’s authorized Cloud Service Offerings following especially high-impact situations. The National Institute of Standards and Technology should also incorporate feedback about observed threats and incidents related to cloud provider security.
As directed by President Biden through Executive Order 14028 Improving the Nation’s Cybersecurity, Secretary Mayorkas established the CSRB in February 2022. The Board’s investigations are conducted independently, and its conclusions are independently reached. DHS and the CSRB are committed to transparency and will, whenever possible, release public versions of CSRB reports, consistent with applicable law and the need to protect sensitive information from disclosure.
To read the full report, visit the Report on the Microsoft Online Exchange Incident from Summer 2023 .
- Cybersecurity
- Secretary of Homeland Security
- Cyber Incident
- Cybersecurity and Infrastructure Security Agency (CISA)
- Secretary Alejandro Mayorkas
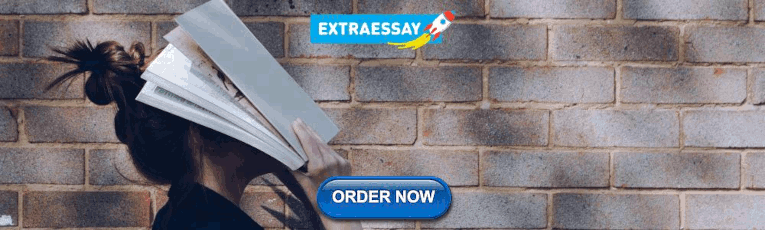
COMMENTS
The study of the recommendation system is a branch of information filtering systems (Recommender system, 2020). Information filtering systems deal with removing unnecessary information from the data stream before it reaches a human. Recommendation systems deal with recommending a product or assigning a rating to item.
A Case Study on Recommendation Systems Based on Big Data 415. 8 Challenges of Recommendation System. 8.1 Sparsity. Most of the user will not rate the items and resulted in rating matrix turns into ...
In a real-world recommender system, the various biases in the user-item interaction-data, like presentation or position biases, can possibly be amplified due to a feedback loop, where the recommender system is trained on the observed user-actions from a previous time-step, which may be biased due to the recommendations shown to the users at ...
Abstract. Recommender systems mainly utilize for finding and recover contents from large datasets; it has been determining and analysis based on the scenario—Big Data. In this paper, we describe the process of recommendation system using big data with a clear explanation in representing the operation of mapreduce.
With this framework, we can identify industries that stand to gain from recommendation systems: 1. E-Commerce. Is an industry where recommendation systems were first widely used. With millions of customers and data on their online behavior, e-commerce companies are best suited to generate accurate recommendations. 2.
Recommender systems are efficient tools for filtering online information, which is widespread owing to the changing habits of computer users, personalization trends, and emerging access to the internet. Even though the recent recommender systems are eminent in giving precise recommendations, they suffer from various limitations and challenges like scalability, cold-start, sparsity, etc. Due to ...
In this article, we outline some of the challenges encountered and lessons learned in using deep learning for recommender systems at Netflix. We first provide an overview of the various recommendation tasks on the Netflix service. We found that different model architectures excel at different tasks. Even though many deep-learning models can be ...
We observe a number of studies in recommendation systems that utilize a variety of approaches, such as rule-based recommendation systems, case-based reasoning-based recommendation systems, and hybrid recommendations systems using collaborative filtering-based algorithms [18,19,20,21,22,23,24]. However, in this particular study, we propose a ...
The goal of this chapter is to give an up-to-date overview of recommender systems techniques used in an industrial setting. We will give a high-level description the practical use of recommendation and personalization techniques. We will highlight some of the main lessons learned from the Netflix Prize. We will then use Netflix personalization ...
Abstract. Recommender systems (RSs), as used by Netflix, YouTube, or Amazon, are one of the most compelling success stories of AI. Enduring research activity in this area has led to a continuous improvement of recommendation techniques over the years, and today's RSs are indeed often capable to make astonishingly good suggestions.
Amazon is the largest e-commerce brand in the world in terms of revenue and market share. ( Statista) In 2021, Amazon's net revenue from e-commerce sales was US$470 billion, and about 35 percent of all sales on Amazon happen via recommendations. This clearly elucidates the power of recommendations. In this case study, we look at how Amazon is ...
A content-based dataset recommendation system for researchers—a case study on Gene Expression Omnibus (GEO) repository ... Recommendation systems, or recommenders, are an information filtering system that deploys data mining and analytics of users' behaviors, including preferences and activities, for predictions of users' interests on ...
We examined case studies conducted over the previous six years (2017-2022), with a focus on 35 key studies selected from 1938 academic papers found using the CADIMA tool. ... The study addresses recommendation systems in the Education sector. The study must be primary. In this stage, we excluded 1199 research papers; thus, the number of ...
A detailed review of various recommendation systems is presented and typically recommender systems are based on the keyword search which allows the efficient scanning of very large document collections. The goal of a recommender system is to generate relevant recommendations for users. It is an information filtering technique that assists users by filtering the redundant and unwanted data from ...
5 Use Case Scenarios for Recommendation Systems and How They Help. The market for recommendation engines is projected to grow from USD 1.14B in 2018 to 12.03B by 2025 with a CAGR of 32.39%, for the forecasted period. These figures are an indication of the growing emphasis on customer experience while also being a byproduct of the widespread ...
The recommendation system is an effective means to solve the information overload problem that exists in social networks, which is also one of the most common applications of big data technology. ... In this section of case study, the way of Top-N recommendation is used to verify the effectiveness of the algorithm. The experiment selects 100 ...
Authors: Zehao Zhao. University of California, Berkeley. In this study, we develop a Dynamic Recommender System for movie recommendations using a framework with integrated Actor and Critic ...
This knowledge-base contains previous problems, constraints, and corresponding solutions. Knowledge in the knowledge base is referenced when the system encounters a new recommendation problem . Case-based reasoning uses previous cases to solve the current problem and is a commonly used technique for knowledge-based systems. In contrast to ...
Random Forests is a powerful machine-learning algorithm that has immense potential in various applications, including movie recommendation systems. It combines the concepts of Bagging and Random ...
The search keywords were as follows, using an inclusive OR: (recommender OR recommendation systems OR recommendation system) AND ... The authors also limited themselves to their specific case studies and did not make any recommendations for policy (last box plot is presented in Figure 2). All 73 studies reported the use of different data sets.
The goal of a recommender system is to generate relevant recom-mendations for users. It is an information filtering technique that assists users by filtering the redundant and unwanted data from a data chunk and delivers relevant information to the users. An in-formation system is known as recommendation engine when the delivered information ...
An increasing number of online companies are utilizing recommendation systems to increase user interaction and enrich shopping potential. Use cases of recommendation systems have been expanding rapidly across many aspects of eCommerce and online media over the last 4-5 years, and we expect this trend to continue.
How To Write Recommendation in Case Study. 1. Review Your Case Study's Problem. 2. Assess Your Case Study's Alternative Courses of Action. 3. Pick Your Case Study's Best Alternative Course of Action. 4. Explain in Detail Why You Recommend Your Preferred Course of Action.
A cross-sectional study exploring community perspectives on the impacts of COVID-19 in Nunavut and recommendations for a Holistic Inuit Qaujimajatuqangit approach to emergency response. ... The first case was confirmed in Sanikiluaq, ... Participants in the study highlighted longstanding and system-wide challenges with Nunavut's healthcare ...
WASHINGTON - Today, the U.S. Department of Homeland Security (DHS) released the Cyber Safety Review Board's (CSRB) findings and recommendations following its independent review of the Summer 2023 Microsoft Exchange Online intrusion.The review detailed operational and strategic decisions that led to the intrusion and recommended specific practices for industry and government to implement to ...