- Bipolar Disorder
- Therapy Center
- When To See a Therapist
- Types of Therapy
- Best Online Therapy
- Best Couples Therapy
- Best Family Therapy
- Managing Stress
- Sleep and Dreaming
- Understanding Emotions
- Self-Improvement
- Healthy Relationships
- Student Resources
- Personality Types
- Guided Meditations
- Verywell Mind Insights
- 2023 Verywell Mind 25
- Mental Health in the Classroom
- Editorial Process
- Meet Our Review Board
- Crisis Support
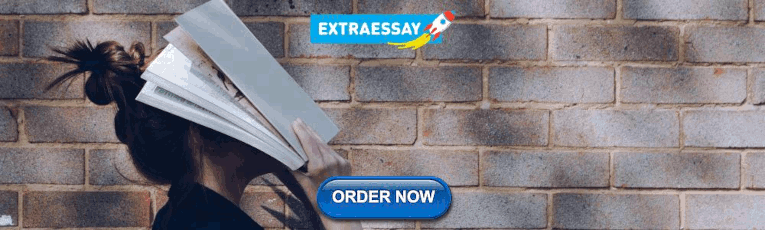
How to Write a Great Hypothesis
Hypothesis Definition, Format, Examples, and Tips
Kendra Cherry, MS, is a psychosocial rehabilitation specialist, psychology educator, and author of the "Everything Psychology Book."
:max_bytes(150000):strip_icc():format(webp)/IMG_9791-89504ab694d54b66bbd72cb84ffb860e.jpg)
Amy Morin, LCSW, is a psychotherapist and international bestselling author. Her books, including "13 Things Mentally Strong People Don't Do," have been translated into more than 40 languages. Her TEDx talk, "The Secret of Becoming Mentally Strong," is one of the most viewed talks of all time.
:max_bytes(150000):strip_icc():format(webp)/VW-MIND-Amy-2b338105f1ee493f94d7e333e410fa76.jpg)
Verywell / Alex Dos Diaz
- The Scientific Method
Hypothesis Format
Falsifiability of a hypothesis.
- Operationalization
Hypothesis Types
Hypotheses examples.
- Collecting Data
A hypothesis is a tentative statement about the relationship between two or more variables. It is a specific, testable prediction about what you expect to happen in a study. It is a preliminary answer to your question that helps guide the research process.
Consider a study designed to examine the relationship between sleep deprivation and test performance. The hypothesis might be: "This study is designed to assess the hypothesis that sleep-deprived people will perform worse on a test than individuals who are not sleep-deprived."
At a Glance
A hypothesis is crucial to scientific research because it offers a clear direction for what the researchers are looking to find. This allows them to design experiments to test their predictions and add to our scientific knowledge about the world. This article explores how a hypothesis is used in psychology research, how to write a good hypothesis, and the different types of hypotheses you might use.
The Hypothesis in the Scientific Method
In the scientific method , whether it involves research in psychology, biology, or some other area, a hypothesis represents what the researchers think will happen in an experiment. The scientific method involves the following steps:
- Forming a question
- Performing background research
- Creating a hypothesis
- Designing an experiment
- Collecting data
- Analyzing the results
- Drawing conclusions
- Communicating the results
The hypothesis is a prediction, but it involves more than a guess. Most of the time, the hypothesis begins with a question which is then explored through background research. At this point, researchers then begin to develop a testable hypothesis.
Unless you are creating an exploratory study, your hypothesis should always explain what you expect to happen.
In a study exploring the effects of a particular drug, the hypothesis might be that researchers expect the drug to have some type of effect on the symptoms of a specific illness. In psychology, the hypothesis might focus on how a certain aspect of the environment might influence a particular behavior.
Remember, a hypothesis does not have to be correct. While the hypothesis predicts what the researchers expect to see, the goal of the research is to determine whether this guess is right or wrong. When conducting an experiment, researchers might explore numerous factors to determine which ones might contribute to the ultimate outcome.
In many cases, researchers may find that the results of an experiment do not support the original hypothesis. When writing up these results, the researchers might suggest other options that should be explored in future studies.
In many cases, researchers might draw a hypothesis from a specific theory or build on previous research. For example, prior research has shown that stress can impact the immune system. So a researcher might hypothesize: "People with high-stress levels will be more likely to contract a common cold after being exposed to the virus than people who have low-stress levels."
In other instances, researchers might look at commonly held beliefs or folk wisdom. "Birds of a feather flock together" is one example of folk adage that a psychologist might try to investigate. The researcher might pose a specific hypothesis that "People tend to select romantic partners who are similar to them in interests and educational level."
Elements of a Good Hypothesis
So how do you write a good hypothesis? When trying to come up with a hypothesis for your research or experiments, ask yourself the following questions:
- Is your hypothesis based on your research on a topic?
- Can your hypothesis be tested?
- Does your hypothesis include independent and dependent variables?
Before you come up with a specific hypothesis, spend some time doing background research. Once you have completed a literature review, start thinking about potential questions you still have. Pay attention to the discussion section in the journal articles you read . Many authors will suggest questions that still need to be explored.
How to Formulate a Good Hypothesis
To form a hypothesis, you should take these steps:
- Collect as many observations about a topic or problem as you can.
- Evaluate these observations and look for possible causes of the problem.
- Create a list of possible explanations that you might want to explore.
- After you have developed some possible hypotheses, think of ways that you could confirm or disprove each hypothesis through experimentation. This is known as falsifiability.
In the scientific method , falsifiability is an important part of any valid hypothesis. In order to test a claim scientifically, it must be possible that the claim could be proven false.
Students sometimes confuse the idea of falsifiability with the idea that it means that something is false, which is not the case. What falsifiability means is that if something was false, then it is possible to demonstrate that it is false.
One of the hallmarks of pseudoscience is that it makes claims that cannot be refuted or proven false.
The Importance of Operational Definitions
A variable is a factor or element that can be changed and manipulated in ways that are observable and measurable. However, the researcher must also define how the variable will be manipulated and measured in the study.
Operational definitions are specific definitions for all relevant factors in a study. This process helps make vague or ambiguous concepts detailed and measurable.
For example, a researcher might operationally define the variable " test anxiety " as the results of a self-report measure of anxiety experienced during an exam. A "study habits" variable might be defined by the amount of studying that actually occurs as measured by time.
These precise descriptions are important because many things can be measured in various ways. Clearly defining these variables and how they are measured helps ensure that other researchers can replicate your results.
Replicability
One of the basic principles of any type of scientific research is that the results must be replicable.
Replication means repeating an experiment in the same way to produce the same results. By clearly detailing the specifics of how the variables were measured and manipulated, other researchers can better understand the results and repeat the study if needed.
Some variables are more difficult than others to define. For example, how would you operationally define a variable such as aggression ? For obvious ethical reasons, researchers cannot create a situation in which a person behaves aggressively toward others.
To measure this variable, the researcher must devise a measurement that assesses aggressive behavior without harming others. The researcher might utilize a simulated task to measure aggressiveness in this situation.
Hypothesis Checklist
- Does your hypothesis focus on something that you can actually test?
- Does your hypothesis include both an independent and dependent variable?
- Can you manipulate the variables?
- Can your hypothesis be tested without violating ethical standards?
The hypothesis you use will depend on what you are investigating and hoping to find. Some of the main types of hypotheses that you might use include:
- Simple hypothesis : This type of hypothesis suggests there is a relationship between one independent variable and one dependent variable.
- Complex hypothesis : This type suggests a relationship between three or more variables, such as two independent and dependent variables.
- Null hypothesis : This hypothesis suggests no relationship exists between two or more variables.
- Alternative hypothesis : This hypothesis states the opposite of the null hypothesis.
- Statistical hypothesis : This hypothesis uses statistical analysis to evaluate a representative population sample and then generalizes the findings to the larger group.
- Logical hypothesis : This hypothesis assumes a relationship between variables without collecting data or evidence.
A hypothesis often follows a basic format of "If {this happens} then {this will happen}." One way to structure your hypothesis is to describe what will happen to the dependent variable if you change the independent variable .
The basic format might be: "If {these changes are made to a certain independent variable}, then we will observe {a change in a specific dependent variable}."
A few examples of simple hypotheses:
- "Students who eat breakfast will perform better on a math exam than students who do not eat breakfast."
- "Students who experience test anxiety before an English exam will get lower scores than students who do not experience test anxiety."
- "Motorists who talk on the phone while driving will be more likely to make errors on a driving course than those who do not talk on the phone."
- "Children who receive a new reading intervention will have higher reading scores than students who do not receive the intervention."
Examples of a complex hypothesis include:
- "People with high-sugar diets and sedentary activity levels are more likely to develop depression."
- "Younger people who are regularly exposed to green, outdoor areas have better subjective well-being than older adults who have limited exposure to green spaces."
Examples of a null hypothesis include:
- "There is no difference in anxiety levels between people who take St. John's wort supplements and those who do not."
- "There is no difference in scores on a memory recall task between children and adults."
- "There is no difference in aggression levels between children who play first-person shooter games and those who do not."
Examples of an alternative hypothesis:
- "People who take St. John's wort supplements will have less anxiety than those who do not."
- "Adults will perform better on a memory task than children."
- "Children who play first-person shooter games will show higher levels of aggression than children who do not."
Collecting Data on Your Hypothesis
Once a researcher has formed a testable hypothesis, the next step is to select a research design and start collecting data. The research method depends largely on exactly what they are studying. There are two basic types of research methods: descriptive research and experimental research.
Descriptive Research Methods
Descriptive research such as case studies , naturalistic observations , and surveys are often used when conducting an experiment is difficult or impossible. These methods are best used to describe different aspects of a behavior or psychological phenomenon.
Once a researcher has collected data using descriptive methods, a correlational study can examine how the variables are related. This research method might be used to investigate a hypothesis that is difficult to test experimentally.
Experimental Research Methods
Experimental methods are used to demonstrate causal relationships between variables. In an experiment, the researcher systematically manipulates a variable of interest (known as the independent variable) and measures the effect on another variable (known as the dependent variable).
Unlike correlational studies, which can only be used to determine if there is a relationship between two variables, experimental methods can be used to determine the actual nature of the relationship—whether changes in one variable actually cause another to change.
The hypothesis is a critical part of any scientific exploration. It represents what researchers expect to find in a study or experiment. In situations where the hypothesis is unsupported by the research, the research still has value. Such research helps us better understand how different aspects of the natural world relate to one another. It also helps us develop new hypotheses that can then be tested in the future.
Thompson WH, Skau S. On the scope of scientific hypotheses . R Soc Open Sci . 2023;10(8):230607. doi:10.1098/rsos.230607
Taran S, Adhikari NKJ, Fan E. Falsifiability in medicine: what clinicians can learn from Karl Popper [published correction appears in Intensive Care Med. 2021 Jun 17;:]. Intensive Care Med . 2021;47(9):1054-1056. doi:10.1007/s00134-021-06432-z
Eyler AA. Research Methods for Public Health . 1st ed. Springer Publishing Company; 2020. doi:10.1891/9780826182067.0004
Nosek BA, Errington TM. What is replication ? PLoS Biol . 2020;18(3):e3000691. doi:10.1371/journal.pbio.3000691
Aggarwal R, Ranganathan P. Study designs: Part 2 - Descriptive studies . Perspect Clin Res . 2019;10(1):34-36. doi:10.4103/picr.PICR_154_18
Nevid J. Psychology: Concepts and Applications. Wadworth, 2013.
By Kendra Cherry, MSEd Kendra Cherry, MS, is a psychosocial rehabilitation specialist, psychology educator, and author of the "Everything Psychology Book."

What Is A Research (Scientific) Hypothesis? A plain-language explainer + examples
By: Derek Jansen (MBA) | Reviewed By: Dr Eunice Rautenbach | June 2020
If you’re new to the world of research, or it’s your first time writing a dissertation or thesis, you’re probably noticing that the words “research hypothesis” and “scientific hypothesis” are used quite a bit, and you’re wondering what they mean in a research context .
“Hypothesis” is one of those words that people use loosely, thinking they understand what it means. However, it has a very specific meaning within academic research. So, it’s important to understand the exact meaning before you start hypothesizing.
Research Hypothesis 101
- What is a hypothesis ?
- What is a research hypothesis (scientific hypothesis)?
- Requirements for a research hypothesis
- Definition of a research hypothesis
- The null hypothesis
What is a hypothesis?
Let’s start with the general definition of a hypothesis (not a research hypothesis or scientific hypothesis), according to the Cambridge Dictionary:
Hypothesis: an idea or explanation for something that is based on known facts but has not yet been proved.
In other words, it’s a statement that provides an explanation for why or how something works, based on facts (or some reasonable assumptions), but that has not yet been specifically tested . For example, a hypothesis might look something like this:
Hypothesis: sleep impacts academic performance.
This statement predicts that academic performance will be influenced by the amount and/or quality of sleep a student engages in – sounds reasonable, right? It’s based on reasonable assumptions , underpinned by what we currently know about sleep and health (from the existing literature). So, loosely speaking, we could call it a hypothesis, at least by the dictionary definition.
But that’s not good enough…
Unfortunately, that’s not quite sophisticated enough to describe a research hypothesis (also sometimes called a scientific hypothesis), and it wouldn’t be acceptable in a dissertation, thesis or research paper . In the world of academic research, a statement needs a few more criteria to constitute a true research hypothesis .
What is a research hypothesis?
A research hypothesis (also called a scientific hypothesis) is a statement about the expected outcome of a study (for example, a dissertation or thesis). To constitute a quality hypothesis, the statement needs to have three attributes – specificity , clarity and testability .
Let’s take a look at these more closely.
Need a helping hand?
Hypothesis Essential #1: Specificity & Clarity
A good research hypothesis needs to be extremely clear and articulate about both what’ s being assessed (who or what variables are involved ) and the expected outcome (for example, a difference between groups, a relationship between variables, etc.).
Let’s stick with our sleepy students example and look at how this statement could be more specific and clear.
Hypothesis: Students who sleep at least 8 hours per night will, on average, achieve higher grades in standardised tests than students who sleep less than 8 hours a night.
As you can see, the statement is very specific as it identifies the variables involved (sleep hours and test grades), the parties involved (two groups of students), as well as the predicted relationship type (a positive relationship). There’s no ambiguity or uncertainty about who or what is involved in the statement, and the expected outcome is clear.
Contrast that to the original hypothesis we looked at – “Sleep impacts academic performance” – and you can see the difference. “Sleep” and “academic performance” are both comparatively vague , and there’s no indication of what the expected relationship direction is (more sleep or less sleep). As you can see, specificity and clarity are key.
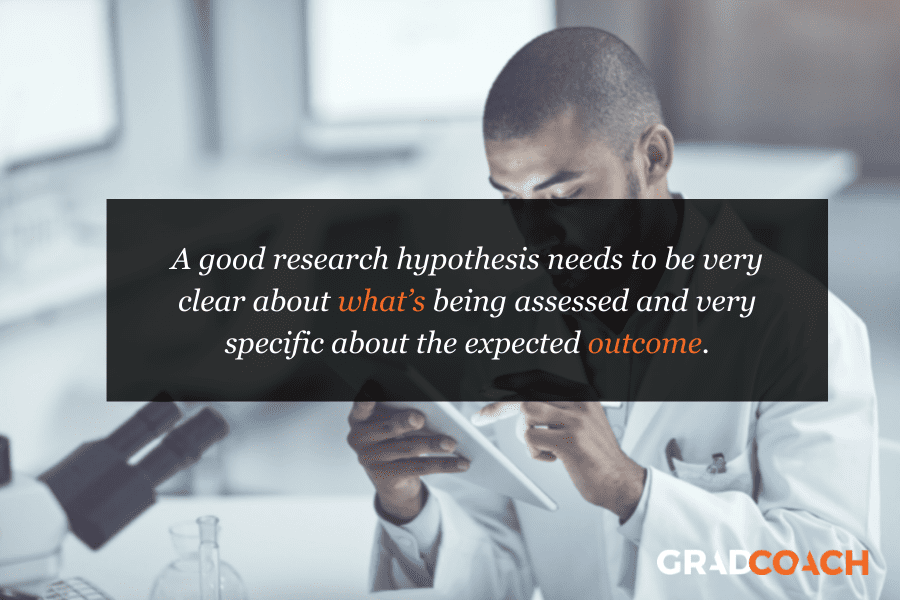
Hypothesis Essential #2: Testability (Provability)
A statement must be testable to qualify as a research hypothesis. In other words, there needs to be a way to prove (or disprove) the statement. If it’s not testable, it’s not a hypothesis – simple as that.
For example, consider the hypothesis we mentioned earlier:
Hypothesis: Students who sleep at least 8 hours per night will, on average, achieve higher grades in standardised tests than students who sleep less than 8 hours a night.
We could test this statement by undertaking a quantitative study involving two groups of students, one that gets 8 or more hours of sleep per night for a fixed period, and one that gets less. We could then compare the standardised test results for both groups to see if there’s a statistically significant difference.
Again, if you compare this to the original hypothesis we looked at – “Sleep impacts academic performance” – you can see that it would be quite difficult to test that statement, primarily because it isn’t specific enough. How much sleep? By who? What type of academic performance?
So, remember the mantra – if you can’t test it, it’s not a hypothesis 🙂
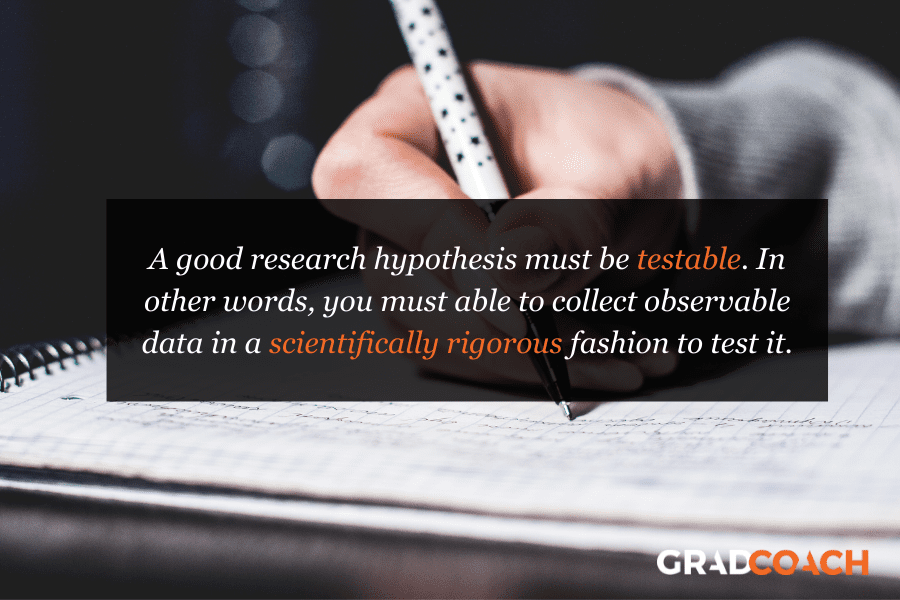
Defining A Research Hypothesis
You’re still with us? Great! Let’s recap and pin down a clear definition of a hypothesis.
A research hypothesis (or scientific hypothesis) is a statement about an expected relationship between variables, or explanation of an occurrence, that is clear, specific and testable.
So, when you write up hypotheses for your dissertation or thesis, make sure that they meet all these criteria. If you do, you’ll not only have rock-solid hypotheses but you’ll also ensure a clear focus for your entire research project.
What about the null hypothesis?
You may have also heard the terms null hypothesis , alternative hypothesis, or H-zero thrown around. At a simple level, the null hypothesis is the counter-proposal to the original hypothesis.
For example, if the hypothesis predicts that there is a relationship between two variables (for example, sleep and academic performance), the null hypothesis would predict that there is no relationship between those variables.
At a more technical level, the null hypothesis proposes that no statistical significance exists in a set of given observations and that any differences are due to chance alone.
And there you have it – hypotheses in a nutshell.
If you have any questions, be sure to leave a comment below and we’ll do our best to help you. If you need hands-on help developing and testing your hypotheses, consider our private coaching service , where we hold your hand through the research journey.
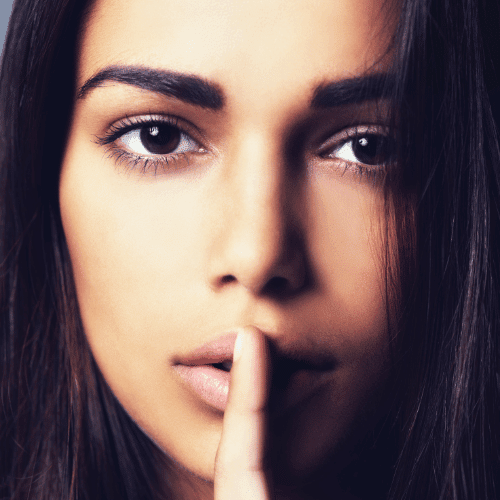
Psst... there’s more!
This post was based on one of our popular Research Bootcamps . If you're working on a research project, you'll definitely want to check this out ...
You Might Also Like:
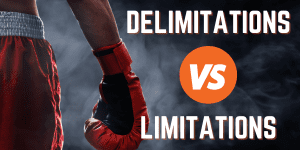
16 Comments
Very useful information. I benefit more from getting more information in this regard.
Very great insight,educative and informative. Please give meet deep critics on many research data of public international Law like human rights, environment, natural resources, law of the sea etc
In a book I read a distinction is made between null, research, and alternative hypothesis. As far as I understand, alternative and research hypotheses are the same. Can you please elaborate? Best Afshin
This is a self explanatory, easy going site. I will recommend this to my friends and colleagues.
Very good definition. How can I cite your definition in my thesis? Thank you. Is nul hypothesis compulsory in a research?
It’s a counter-proposal to be proven as a rejection
Please what is the difference between alternate hypothesis and research hypothesis?
It is a very good explanation. However, it limits hypotheses to statistically tasteable ideas. What about for qualitative researches or other researches that involve quantitative data that don’t need statistical tests?
In qualitative research, one typically uses propositions, not hypotheses.
could you please elaborate it more
I’ve benefited greatly from these notes, thank you.
This is very helpful
well articulated ideas are presented here, thank you for being reliable sources of information
Excellent. Thanks for being clear and sound about the research methodology and hypothesis (quantitative research)
I have only a simple question regarding the null hypothesis. – Is the null hypothesis (Ho) known as the reversible hypothesis of the alternative hypothesis (H1? – How to test it in academic research?
this is very important note help me much more
Trackbacks/Pingbacks
- What Is Research Methodology? Simple Definition (With Examples) - Grad Coach - […] Contrasted to this, a quantitative methodology is typically used when the research aims and objectives are confirmatory in nature. For example,…
Submit a Comment Cancel reply
Your email address will not be published. Required fields are marked *
Save my name, email, and website in this browser for the next time I comment.
- Print Friendly
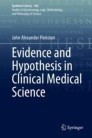
Evidence and Hypothesis in Clinical Medical Science pp 39–66 Cite as
Confirmation of Hypotheses in Clinical Medical Science
- John Alexander Pinkston 7
- First Online: 09 May 2020
166 Accesses
Part of the book series: Synthese Library ((SYLI,volume 426))
In this chapter I discuss the ways in which information is gathered and used to confirm typical hypotheses encountered in clinical medical science. I discuss three kinds of hypotheses, namely, therapeutic , etiologic , and diagnostic . Therapeutic hypotheses are those concerned with treatments or other interventions, and etiologic hypotheses are those concerned with disease causation. Diagnostic hypotheses are those considered by clinicians when making a diagnosis . Examples from the medical scientific literature are extensively used. Included are an example of a randomized clinical trial and an N of 1 study for therapeutic hypotheses, and cohort, case – control, and cross – sectional studies are used for etiologic hypotheses. Approaches to the confirmation of diagnostic hypotheses are illustrated using actual published cases with discussions of the various strategies that are employed. Some possible pitfalls that may occur in the confirmation process are briefly discussed.
This is a preview of subscription content, log in via an institution .
Buying options
- Available as PDF
- Read on any device
- Instant download
- Own it forever
- Available as EPUB and PDF
- Compact, lightweight edition
- Dispatched in 3 to 5 business days
- Free shipping worldwide - see info
- Durable hardcover edition
Tax calculation will be finalised at checkout
Purchases are for personal use only
The stratification referred to is a common method for attempting to control bias such as confounding. For example, by choosing controls with the same age structure (strata) as cases, any influence due to age imbalance would be minimized or eliminated.
For example, a person who had smoked 20 cigarettes daily for 10 years was classed as having smoked 10 cigarettes daily for 20 years.
Both equations contain a random error term that has been omitted.
For example, laboratory tests may be in error, or signs or symptoms may be evolving or misinterpreted.
A “pathognomonic” finding is one that can occur only with a single condition.
Additional references include Gill et al. ( 2005 ), Willis et al. ( 2013 ) , and Jain ( 2017 ).
Anderson, Kristin E., Thomas M. Mack, and Debra T. Silverman. 2006. Cancer of the pancreas. In Cancer epidemiology and prevention , ed. David Schottenfeld and Joseph F. Fraumeni Jr., 3rd ed., 721–762. Oxford: Oxford University Press.
Google Scholar
Berghmans, Ron, and Harry C. Schouten. 2011. Sir Karl Popper, swans, and the general practitioner. British Medical Journal 343: d5469.
Casey, Jonathan D., Daniel H. Solomon, Thomas A. Gaziano, Amy Leigh Miller, and Joseph Loscalzo. 2013. A patient with migrating polyarthralgias. New England Journal of Medicine 369: 75–80.
Checkoway, Harvey, Neil E. Pearce, and Douglas J. Crawford-Brown. 1989. Research methods in occupational epidemiology . Oxford: Oxford University Press.
Clavel, Françoise, Ellen Benhamou, Ariane Auquier, Michèle Tarayre, and Robert Flamant. 1989. Coffee, alcohol, smoking and cancer of the pancreas: A case-control study. International Journal of Cancer 43: 17–21.
Cox, D.R. 1972. Regression models and life-tables. Journal of the Royal Statistical Society, Series B 34: 187–220.
Dajani, Adnan S., Elia Ayoub, Fredrick Z. Bierman, Alan L. Bisno, Floyd W. Denny, David T. Durak, Patricia Ferrieri, et al. 1993. Guidelines for the diagnosis of rheumatic fever: Jones criteria, updated 1992. Circulation 87: 302–307.
Daling, Janet R., and Karen J. Sherman. 1996. Cancers of the vulva and vagina. In Cancer epidemiology and prevention , ed. David Schottenfeld and Joseph F. Fraumeni Jr., 2nd ed., 1117–1129. Oxford: Oxford University Press.
Doll, Richard, and A. Bradford Hill. 1950. Smoking and carcinoma of the lung. Preliminary report. British Medical Journal 221: 739–748.
Doll, Richard, Richard Peto, Jillian Boreham, and Isabelle Sutherland. 2004. Mortality in relation to smoking: 50 years’ observations on male British doctors. British Medical Journal 328: 1519–1528.
Eddy, David M., and Charles H. Clanton. 1982. The art of diagnosis. Solving the clinicopathological exercise. New England Journal of Medicine 306: 1263–1268.
Elstein, Arthur S., and Alan Schwarz. 2002. Clinical problem solving and diagnostic decision making: Selective review of the cognitive literature. British Medical Journal 324: 729–732.
Gill, Christopher J., Lora Sabin, and Christopher H. Schmid. 2005. Why clinicians are natural bayesians. British Medical Journal 330: 1080–1083.
Greenhalgh, Trisha. 2010. How to read a paper: The basics of evidence-based medicine . 4th ed. Chichester: Wiley.
Gutensohn, Nancy, Frederick P. Li, Ralph E. Johnson, and Philip Cole. 1975. Hodgkin’s disease, tonsillectomy, and family size. New England Journal of Medicine 292: 22–25.
Guyatt, Gordon, Sackett David, D. Wayne Taylor, John Chong, Robin Roberts, and Stewart Pugsley. 1986. Determining optimal therapy – Randomized trials in individual patients. New England Journal of Medicine 314: 889–892.
Harvey, A. McGehee. 1994. Foreword. In Differential diagnosis , ed. Jeremiah A. Barondess, Charles C.J. Carpenter, and A. McGehee Harvey. Philadelphia: Lea and Febiger.
Herbst, Arthur L., Howard Ulfelder, and David Poskanzer. 1971. Adenocarcinoma of the vagina. Association of maternal stilbestrol therapy with tumor appearance in young women. New England Journal of Medicine 284: 878–881.
Hoen, Bruno, Isabelle Béguinot, Christian Rabaud, Roland Jaussaud, Christine Selton-Suty, Thierry May, and Philippe Canton. 1996. The Duke criteria for diagnosing infective endocarditis are specific: Analysis of 100 patients with acute fever or fever of unknown origin. Clinical Infectious Diseases 23: 298–302.
Hosmer, David W., and Stanley Lemeshow. 1989. Applied logistic regression . New York: Wiley.
International Agency for Research on Cancer. 2004. Tobacco smoke and involuntary smoking , IARC monographs on the evaluation of carcinogenic risks to humans. Vol. 83. Lyon: IARC.
Jain, Bimal. 2017. The scientific nature of diagnosis. Diagnosis 4: 17–19.
Jones, T. Duckett. 1944. The diagnosis of rheumatic fever. Journal of the American Medical Association 126: 481–484.
Kaplan, E.L., and Paul Meier. 1958. Nonparametric estimation from incomplete observations. Journal of the American Statistical Association 53: 457–481.
Kassirer, Jerome P., John B. Wong, and Richard I. Kopelman. 2010. Learning clinical reasoning . 2nd ed. Baltimore: Lippincott Williams and Wilkins.
Kelsey, Jennifer L., W. Douglas Thompson, and Alfred S. Evans. 1986. Methods in observational epidemiology. Volume 10 of monographs in epidemiology and biostatistics , General ed. Abraham M. Lilienfeld. Oxford: Oxford University Press.
Li, Jennifer S., Daniel J. Sexton, Nathan Mick, Richard Nettles, Vance G. Fowler Jr., Thomas Ryan, Thomas Bashore, and G. Ralph Corey. 2000. Proposed modifications to the Duke criteria for the diagnosis of infective endocarditis. Clinical Infectious Diseases 30: 633–638.
MacMahon, Brian, Stella Yen, Dimitrios Trichopoulos, Kenneth Warren, and George Nardi. 1981. Coffee and cancer of the pancreas. New England Journal of Medicine 304: 630–633.
Mantel, Nathan, and William Haenszel. 1959. Statistical aspects of the analysis of data from retrospective studies of disease. Journal of the National Cancer Institute 22: 719–748.
Mayo, Deborah G. 1996. Error and the growth of experimental knowledge . Chicago: University of Chicago Press.
Miettinen, Olli S. 2001a. The modern scientific physician: 2. Medical science versus scientific medicine. Canadian Medical Association Journal 165: 591–592.
———. 2001b. The modern scientific physician: 3. Scientific diagnosis. Canadian Medical Association Journal 165: 781–782.
Morris, J.N., J.A. Heady, P.A.B. Raffle, C.G. Roberts, and J.W. Parks. 1953. Coronary heart-disease and physical activity of work. Lancet 2: 1053–1057.
Mueller, Nancy E. 1996. Hodgkin’s disease. In Cancer epidemiology and prevention , ed. David Schottenfeld and Joseph F. Fraumeni Jr., 2nd ed., 893–919. Oxford: Oxford University Press.
Mueller, Nancy, G. Marie Swanson, Chung-cheng Hsieh, and Philip Cole. 1987. Tonsillectomy and Hodgkin’s disease: Results from companion population-based studies. Journal of the National Cancer Institute 78: 1–5.
Rothman, Kenneth J., Sander Greenland, and Timothy L. Lash. 2008. Case-control studies. In Modern epidemiology , ed. Kenneth J. Rothman, Sander Greenland, and Timothy L. Lash, 3rd ed., 111–127. Philadelphia: Lippincott Williams and Wilkins.
Slone, Dennis, Samuel Shapiro, Lynn Rosenberg, David W. Kaufman, Stuart C. Hartz, Allen C. Rossi, Paul D. Stolley, and Olli S. Miettinen. 1978. Relation of cigarette smoking to myocardial infarction in young women. New England Journal of Medicine 298: 1273–1276.
Sox, Harold C., Marshall A. Blott, Michael C. Higgins, and Keith I. Martin. 2007. Medical decision making . Philadelphia: American College of Physicians.
Straus, Sharon E., Glasziou Paul, W. Scott Richardson, and R. Brian Haynes. 2011. Evidence-based medicine. How to practice and teach it . 4th ed. Edinburgh: Churchill Livingstone.
Thomas, Patrick R.M., and Anne S. Lindblad. 1988. Adjuvant postoperative radiotherapy and chemotherapy in rectal carcinoma: A review of the gastrointestinal tumor study group experience. Radiotherapy and Oncology 13: 245–252.
Ueshima, Hirotsugu, Takashi Shimamoto, Minoru Iida, Masamitsu Konishi, Masato Tanigaki, Mitsunori Doi, Katsuhiko Tsujioka, et al. 1984. Alcohol intake and hypertension among urban and rural Japanese populations. Journal of Chronic Diseases 37: 585–592.
Willis, Brian H., Helen Beebee, and Daniel S. Lasserson. 2013. Philosophy of science and the diagnostic process. Family Practice 30: 501–505.
Wynder, Ernest L., and Evarts A. Graham. 1950. Tobacco smoking as a possible etiologic factor in bronchiogenic carcinoma. Journal of the American Medical Association 143: 329–336.
Download references
Author information
Authors and affiliations.
Birmingham Radiological Group, Birmingham, AL, USA
John Alexander Pinkston
You can also search for this author in PubMed Google Scholar
Rights and permissions
Reprints and permissions
Copyright information
© 2020 Springer Nature Switzerland AG
About this chapter
Cite this chapter.
Pinkston, J.A. (2020). Confirmation of Hypotheses in Clinical Medical Science. In: Evidence and Hypothesis in Clinical Medical Science. Synthese Library, vol 426. Springer, Cham. https://doi.org/10.1007/978-3-030-44270-5_4
Download citation
DOI : https://doi.org/10.1007/978-3-030-44270-5_4
Published : 09 May 2020
Publisher Name : Springer, Cham
Print ISBN : 978-3-030-44269-9
Online ISBN : 978-3-030-44270-5
eBook Packages : Religion and Philosophy Philosophy and Religion (R0)
Share this chapter
Anyone you share the following link with will be able to read this content:
Sorry, a shareable link is not currently available for this article.
Provided by the Springer Nature SharedIt content-sharing initiative
- Publish with us
Policies and ethics
- Find a journal
- Track your research
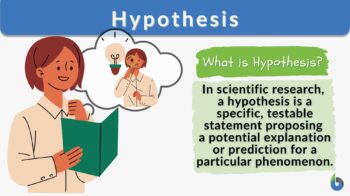
Hypothesis n., plural: hypotheses [/haɪˈpɑːθəsɪs/] Definition: Testable scientific prediction
Table of Contents
What Is Hypothesis?
A scientific hypothesis is a foundational element of the scientific method . It’s a testable statement proposing a potential explanation for natural phenomena. The term hypothesis means “little theory” . A hypothesis is a short statement that can be tested and gives a possible reason for a phenomenon or a possible link between two variables . In the setting of scientific research, a hypothesis is a tentative explanation or statement that can be proven wrong and is used to guide experiments and empirical research.
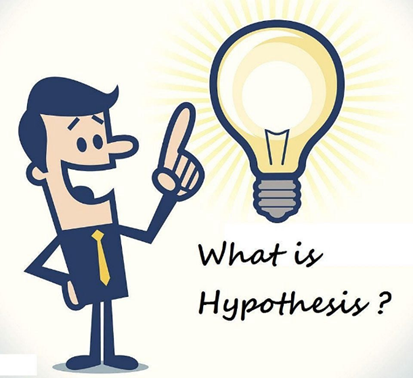
It is an important part of the scientific method because it gives a basis for planning tests, gathering data, and judging evidence to see if it is true and could help us understand how natural things work. Several hypotheses can be tested in the real world, and the results of careful and systematic observation and analysis can be used to support, reject, or improve them.
Researchers and scientists often use the word hypothesis to refer to this educated guess . These hypotheses are firmly established based on scientific principles and the rigorous testing of new technology and experiments .
For example, in astrophysics, the Big Bang Theory is a working hypothesis that explains the origins of the universe and considers it as a natural phenomenon. It is among the most prominent scientific hypotheses in the field.
“The scientific method: steps, terms, and examples” by Scishow:
Biology definition: A hypothesis is a supposition or tentative explanation for (a group of) phenomena, (a set of) facts, or a scientific inquiry that may be tested, verified or answered by further investigation or methodological experiment. It is like a scientific guess . It’s an idea or prediction that scientists make before they do experiments. They use it to guess what might happen and then test it to see if they were right. It’s like a smart guess that helps them learn new things. A scientific hypothesis that has been verified through scientific experiment and research may well be considered a scientific theory .
Etymology: The word “hypothesis” comes from the Greek word “hupothesis,” which means “a basis” or “a supposition.” It combines “hupo” (under) and “thesis” (placing). Synonym: proposition; assumption; conjecture; postulate Compare: theory See also: null hypothesis
Characteristics Of Hypothesis
A useful hypothesis must have the following qualities:
- It should never be written as a question.
- You should be able to test it in the real world to see if it’s right or wrong.
- It needs to be clear and exact.
- It should list the factors that will be used to figure out the relationship.
- It should only talk about one thing. You can make a theory in either a descriptive or form of relationship.
- It shouldn’t go against any natural rule that everyone knows is true. Verification will be done well with the tools and methods that are available.
- It should be written in as simple a way as possible so that everyone can understand it.
- It must explain what happened to make an answer necessary.
- It should be testable in a fair amount of time.
- It shouldn’t say different things.
Sources Of Hypothesis
Sources of hypothesis are:
- Patterns of similarity between the phenomenon under investigation and existing hypotheses.
- Insights derived from prior research, concurrent observations, and insights from opposing perspectives.
- The formulations are derived from accepted scientific theories and proposed by researchers.
- In research, it’s essential to consider hypothesis as different subject areas may require various hypotheses (plural form of hypothesis). Researchers also establish a significance level to determine the strength of evidence supporting a hypothesis.
- Individual cognitive processes also contribute to the formation of hypotheses.
One hypothesis is a tentative explanation for an observation or phenomenon. It is based on prior knowledge and understanding of the world, and it can be tested by gathering and analyzing data. Observed facts are the data that are collected to test a hypothesis. They can support or refute the hypothesis.
For example, the hypothesis that “eating more fruits and vegetables will improve your health” can be tested by gathering data on the health of people who eat different amounts of fruits and vegetables. If the people who eat more fruits and vegetables are healthier than those who eat less fruits and vegetables, then the hypothesis is supported.
Hypotheses are essential for scientific inquiry. They help scientists to focus their research, to design experiments, and to interpret their results. They are also essential for the development of scientific theories.
Types Of Hypothesis
In research, you typically encounter two types of hypothesis: the alternative hypothesis (which proposes a relationship between variables) and the null hypothesis (which suggests no relationship).
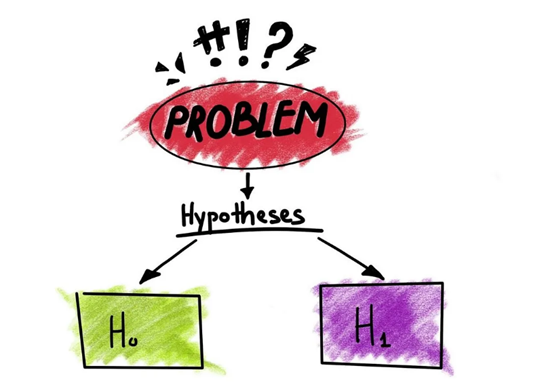
Simple Hypothesis
It illustrates the association between one dependent variable and one independent variable. For instance, if you consume more vegetables, you will lose weight more quickly. Here, increasing vegetable consumption is the independent variable, while weight loss is the dependent variable.
Complex Hypothesis
It exhibits the relationship between at least two dependent variables and at least two independent variables. Eating more vegetables and fruits results in weight loss, radiant skin, and a decreased risk of numerous diseases, including heart disease.
Directional Hypothesis
It shows that a researcher wants to reach a certain goal. The way the factors are related can also tell us about their nature. For example, four-year-old children who eat well over a time of five years have a higher IQ than children who don’t eat well. This shows what happened and how it happened.
Non-directional Hypothesis
When there is no theory involved, it is used. It is a statement that there is a connection between two variables, but it doesn’t say what that relationship is or which way it goes.
Null Hypothesis
It says something that goes against the theory. It’s a statement that says something is not true, and there is no link between the independent and dependent factors. “H 0 ” represents the null hypothesis.
Associative and Causal Hypothesis
When a change in one variable causes a change in the other variable, this is called the associative hypothesis . The causal hypothesis, on the other hand, says that there is a cause-and-effect relationship between two or more factors.
Examples Of Hypothesis
Examples of simple hypotheses:
- Students who consume breakfast before taking a math test will have a better overall performance than students who do not consume breakfast.
- Students who experience test anxiety before an English examination will get lower scores than students who do not experience test anxiety.
- Motorists who talk on the phone while driving will be more likely to make errors on a driving course than those who do not talk on the phone, is a statement that suggests that drivers who talk on the phone while driving are more likely to make mistakes.
Examples of a complex hypothesis:
- Individuals who consume a lot of sugar and don’t get much exercise are at an increased risk of developing depression.
- Younger people who are routinely exposed to green, outdoor areas have better subjective well-being than older adults who have limited exposure to green spaces, according to a new study.
- Increased levels of air pollution led to higher rates of respiratory illnesses, which in turn resulted in increased costs for healthcare for the affected communities.
Examples of Directional Hypothesis:
- The crop yield will go up a lot if the amount of fertilizer is increased.
- Patients who have surgery and are exposed to more stress will need more time to get better.
- Increasing the frequency of brand advertising on social media will lead to a significant increase in brand awareness among the target audience.
Examples of Non-Directional Hypothesis (or Two-Tailed Hypothesis):
- The test scores of two groups of students are very different from each other.
- There is a link between gender and being happy at work.
- There is a correlation between the amount of caffeine an individual consumes and the speed with which they react.
Examples of a null hypothesis:
- Children who receive a new reading intervention will have scores that are different than students who do not receive the intervention.
- The results of a memory recall test will not reveal any significant gap in performance between children and adults.
- There is not a significant relationship between the number of hours spent playing video games and academic performance.
Examples of Associative Hypothesis:
- There is a link between how many hours you spend studying and how well you do in school.
- Drinking sugary drinks is bad for your health as a whole.
- There is an association between socioeconomic status and access to quality healthcare services in urban neighborhoods.
Functions Of Hypothesis
The research issue can be understood better with the help of a hypothesis, which is why developing one is crucial. The following are some of the specific roles that a hypothesis plays: (Rashid, Apr 20, 2022)
- A hypothesis gives a study a point of concentration. It enlightens us as to the specific characteristics of a study subject we need to look into.
- It instructs us on what data to acquire as well as what data we should not collect, giving the study a focal point .
- The development of a hypothesis improves objectivity since it enables the establishment of a focal point.
- A hypothesis makes it possible for us to contribute to the development of the theory. Because of this, we are in a position to definitively determine what is true and what is untrue .
How will Hypothesis help in the Scientific Method?
- The scientific method begins with observation and inquiry about the natural world when formulating research questions. Researchers can refine their observations and queries into specific, testable research questions with the aid of hypothesis. They provide an investigation with a focused starting point.
- Hypothesis generate specific predictions regarding the expected outcomes of experiments or observations. These forecasts are founded on the researcher’s current knowledge of the subject. They elucidate what researchers anticipate observing if the hypothesis is true.
- Hypothesis direct the design of experiments and data collection techniques. Researchers can use them to determine which variables to measure or manipulate, which data to obtain, and how to conduct systematic and controlled research.
- Following the formulation of a hypothesis and the design of an experiment, researchers collect data through observation, measurement, or experimentation. The collected data is used to verify the hypothesis’s predictions.
- Hypothesis establish the criteria for evaluating experiment results. The observed data are compared to the predictions generated by the hypothesis. This analysis helps determine whether empirical evidence supports or refutes the hypothesis.
- The results of experiments or observations are used to derive conclusions regarding the hypothesis. If the data support the predictions, then the hypothesis is supported. If this is not the case, the hypothesis may be revised or rejected, leading to the formulation of new queries and hypothesis.
- The scientific approach is iterative, resulting in new hypothesis and research issues from previous trials. This cycle of hypothesis generation, testing, and refining drives scientific progress.
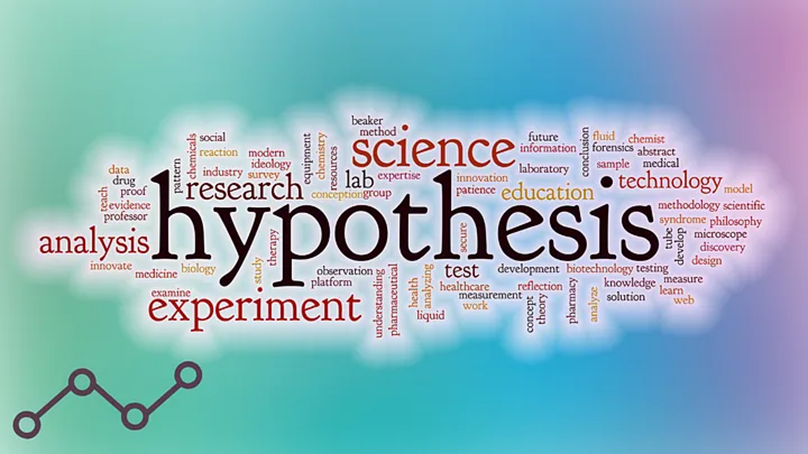
Importance Of Hypothesis
- Hypothesis are testable statements that enable scientists to determine if their predictions are accurate. This assessment is essential to the scientific method, which is based on empirical evidence.
- Hypothesis serve as the foundation for designing experiments or data collection techniques. They can be used by researchers to develop protocols and procedures that will produce meaningful results.
- Hypothesis hold scientists accountable for their assertions. They establish expectations for what the research should reveal and enable others to assess the validity of the findings.
- Hypothesis aid in identifying the most important variables of a study. The variables can then be measured, manipulated, or analyzed to determine their relationships.
- Hypothesis assist researchers in allocating their resources efficiently. They ensure that time, money, and effort are spent investigating specific concerns, as opposed to exploring random concepts.
- Testing hypothesis contribute to the scientific body of knowledge. Whether or not a hypothesis is supported, the results contribute to our understanding of a phenomenon.
- Hypothesis can result in the creation of theories. When supported by substantive evidence, hypothesis can serve as the foundation for larger theoretical frameworks that explain complex phenomena.
- Beyond scientific research, hypothesis play a role in the solution of problems in a variety of domains. They enable professionals to make educated assumptions about the causes of problems and to devise solutions.
Research Hypotheses: Did you know that a hypothesis refers to an educated guess or prediction about the outcome of a research study?
It’s like a roadmap guiding researchers towards their destination of knowledge. Just like a compass points north, a well-crafted hypothesis points the way to valuable discoveries in the world of science and inquiry.
Choose the best answer.
Send Your Results (Optional)
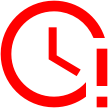
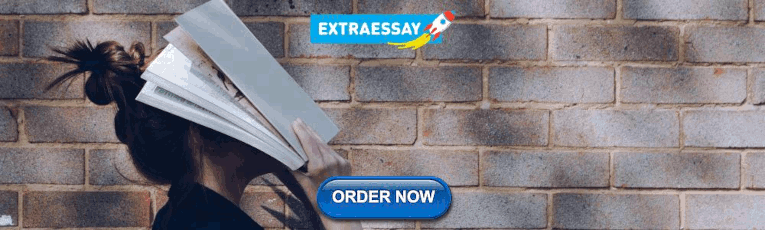
Further Reading
- RNA-DNA World Hypothesis
- BYJU’S. (2023). Hypothesis. Retrieved 01 Septermber 2023, from https://byjus.com/physics/hypothesis/#sources-of-hypothesis
- Collegedunia. (2023). Hypothesis. Retrieved 1 September 2023, from https://collegedunia.com/exams/hypothesis-science-articleid-7026#d
- Hussain, D. J. (2022). Hypothesis. Retrieved 01 September 2023, from https://mmhapu.ac.in/doc/eContent/Management/JamesHusain/Research%20Hypothesis%20-Meaning,%20Nature%20&%20Importance-Characteristics%20of%20Good%20%20Hypothesis%20Sem2.pdf
- Media, D. (2023). Hypothesis in the Scientific Method. Retrieved 01 September 2023, from https://www.verywellmind.com/what-is-a-hypothesis-2795239#toc-hypotheses-examples
- Rashid, M. H. A. (Apr 20, 2022). Research Methodology. Retrieved 01 September 2023, from https://limbd.org/hypothesis-definitions-functions-characteristics-types-errors-the-process-of-testing-a-hypothesis-hypotheses-in-qualitative-research/#:~:text=Functions%20of%20a%20Hypothesis%3A&text=Specifically%2C%20a%20hypothesis%20serves%20the,providing%20focus%20to%20the%20study.
©BiologyOnline.com. Content provided and moderated by Biology Online Editors.
Last updated on September 8th, 2023
You will also like...
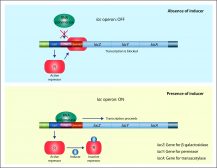
Gene Action – Operon Hypothesis
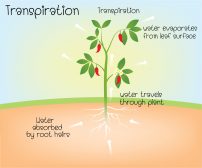
Water in Plants
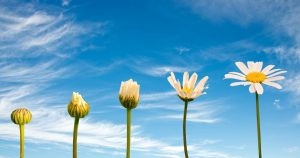
Growth and Plant Hormones
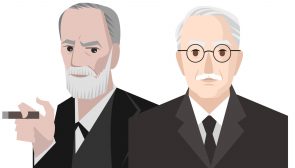
Sigmund Freud and Carl Gustav Jung
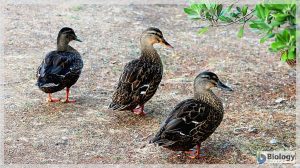
Population Growth and Survivorship
Related articles....
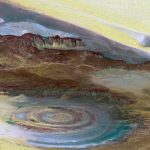
RNA-DNA World Hypothesis?
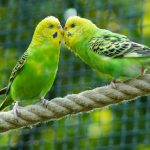
On Mate Selection Evolution: Are intelligent males more attractive?
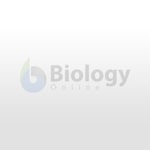
Actions of Caffeine in the Brain with Special Reference to Factors That Contribute to Its Widespread Use
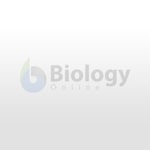
Dead Man Walking
Click through the PLOS taxonomy to find articles in your field.
For more information about PLOS Subject Areas, click here .
Loading metrics
Open Access
Peer-reviewed
Research Article
How Doctors Generate Diagnostic Hypotheses: A Study of Radiological Diagnosis with Functional Magnetic Resonance Imaging
* E-mail: [email protected]
Affiliation Laboratory of Medical Informatics (LIM 01), Faculty of Medicine of the University of São Paulo, São Paulo, Brazil
Affiliation Department and Institute of Radiology (LIM 44), Faculty of Medicine of the University of São Paulo, São Paulo, Brazil
Affiliations Department and Institute of Radiology (LIM 44), Faculty of Medicine of the University of São Paulo, São Paulo, Brazil, Center for Mathematics, Computation and Cognition, Federal University of ABC, Santo André, Brazil
Affiliation Wellcome Trust Centre for Neuroimaging, University College London, London, United Kingdom
- Marcio Melo,
- Daniel J. Scarpin,
- Edson Amaro Jr,
- Rodrigo B. D. Passos,
- João R. Sato,
- Karl J. Friston,
- Cathy J. Price
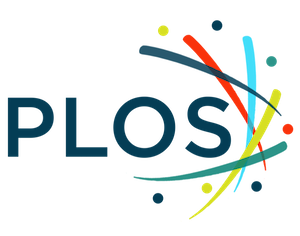
- Published: December 14, 2011
- https://doi.org/10.1371/journal.pone.0028752
- Reader Comments
In medical practice, diagnostic hypotheses are often made by physicians in the first moments of contact with patients; sometimes even before they report their symptoms. We propose that generation of diagnostic hypotheses in this context is the result of cognitive processes subserved by brain mechanisms that are similar to those involved in naming objects or concepts in everyday life.
Methodology and Principal Findings
To test this proposal we developed an experimental paradigm with functional magnetic resonance imaging (fMRI) using radiological diagnosis as a model. Twenty-five radiologists diagnosed lesions in chest X-ray images and named non-medical targets (animals) embedded in chest X-ray images while being scanned in a fMRI session. Images were presented for 1.5 seconds; response times (RTs) and the ensuing cortical activations were assessed. The mean response time for diagnosing lesions was 1.33 (SD ±0.14) seconds and 1.23 (SD ±0.13) seconds for naming animals. 72% of the radiologists reported cogitating differential diagnoses during trials (3.5 seconds). The overall pattern of cortical activations was remarkably similar for both types of targets. However, within the neural systems shared by both stimuli, activation was significantly greater in left inferior frontal sulcus and posterior cingulate cortex for lesions relative to animals.
Conclusions
Generation of diagnostic hypotheses and differential diagnoses made through the immediate visual recognition of clinical signs can be a fast and automatic process. The co-localization of significant brain activation for lesions and animals suggests that generating diagnostic hypotheses for lesions and naming animals are served by the same neuronal systems. Nevertheless, diagnosing lesions was cognitively more demanding and associated with more activation in higher order cortical areas. These results support the hypothesis that medical diagnoses based on prompt visual recognition of clinical signs and naming in everyday life are supported by similar brain systems.
Citation: Melo M, Scarpin DJ, Amaro E Jr, Passos RBD, Sato JR, Friston KJ, et al. (2011) How Doctors Generate Diagnostic Hypotheses: A Study of Radiological Diagnosis with Functional Magnetic Resonance Imaging. PLoS ONE 6(12): e28752. https://doi.org/10.1371/journal.pone.0028752
Editor: André Aleman, University of Groningen, Netherlands
Received: August 15, 2011; Accepted: November 14, 2011; Published: December 14, 2011
Copyright: © 2011 Melo et al. This is an open-access article distributed under the terms of the Creative Commons Attribution License, which permits unrestricted use, distribution, and reproduction in any medium, provided the original author and source are credited.
Funding: This study is part of the Cooperação Interinstitucional de Apoio à Pesquisa sobre o Cerebro (CINAPCE) funded by Fundação de Amparo à Pesquisa do Estado de São Paulo (FAPESP), Brazil. Cathy J. Price and Karl J. Friston are funded by the Wellcome Trust, UK. The funders had no role in study design, data collection and analysis, decision to publish, or preparation of the manuscript.
Competing interests: The authors have declared that no competing interests exist.
Introduction
There is substantial and converging evidence that a significant part of the understanding of the environment that we have in our everyday lives is carried out by brain mechanisms that are fast, automatic, and effortless [1] , [2] , [3] . Possibly as a consequence of these processes, diagnostic hypotheses in medical practice are often made by physicians in the first moments of contact with patients; sometimes even before the report of symptoms [4] , [5] , [6] , [7] , [8] . To exemplify, when a doctor encounters a patient with pronounced jaundice diagnostic hypotheses related to liver diseases immediately and automatically come to her/his awareness. This type of diagnosis has been ascribed to pattern recognition or non-analytical reasoning [9] , [10] .
We propose that the generation of diagnostic hypotheses in such circumstances is the result of neurocognitive processes that are similar to those involved in naming objects or concepts in everyday life. Conversely, recognition of objects in everyday life can be conceptualized as a diagnostic process [11] . A critical test of this proposal would be to compare the brain systems involved in diagnosing lesions with those involved in naming. To explore this hypothesis, radiological diagnosis was used as a model in the visual domain. We developed an experimental paradigm in which radiologists diagnosed lesions in chest X-ray images and named non-medical targets (animals) embedded in chest X-ray images, during functional magnetic resonance imaging (fMRI). We expected to show that diagnosing lesions and naming animals, presented in the same context, would produce a similar pattern of brain activations. Naming letters was introduced as a control task (see Figure 1 ).
- PPT PowerPoint slide
- PNG larger image
- TIFF original image
*Arrows pointing to targets in the image; not present in the original images.
https://doi.org/10.1371/journal.pone.0028752.g001
Mean response times (RTs), error and hesitation rates are shown in Table 1 .
https://doi.org/10.1371/journal.pone.0028752.t001
When naming lesions, subjects reported becoming aware of a greater number of potential names in comparison to animals and letters (as evidenced by the lexical semantic association indices in Table 1 ). The associated words were usually different names, i.e. synonyms, for the same target, e.g. ‘enlarged heart’ while diagnosing cardiomegaly, or the two words name for the lesion, e.g. ‘mediastinal enlargement’. However, 18 (72%) participants reported that while diagnosing some lesions the name of alternative diagnoses came to mind, e.g. ‘bulla’ while diagnosing a cavitation; 15.8% of the lexical semantic associations for lesions were differential diagnoses. Twenty-two (88%) participants reported becoming aware of the name of other animals as alternatives to some of the animals they were naming; e.g. ‘dromedary’ while naming a camel; 64.0% of the lexical semantic associations in this category were the names of other animals.
In 8.00% of the correct responses for lesions, subjects responded with a one-word name other than the name learned during training (e.g. ‘condensation’ in response to pneumonia). Also in 5.13% of lesion trials, the correct responses had more than one word (e.g. ‘aortic elongation’ or ‘pleural effusion’).
The patterns of cortical activations observed when naming each category of stimulus (relative to a control baseline of null events), were strikingly similar in their anatomical deployment ( Figure 2 ). When lexical semantic associations were not controlled, activation was higher for naming lesions than naming animals and letters in the left inferior frontal sulcus and posterior cingulate cortex ( Table 2 and Figure 3 ). Activation in the same areas was also higher for naming animals than naming letters (p<0.001 uncorrected). This decreasing order of activation: lesions>animals>letters ( Figure 3 ) is parallel to a similar order of diminishing lexical semantic association indices ( Table 1 ). Indeed, when lexical semantic associations were co-varied out in the second statistical analysis, there were no areas where activation was significantly higher for lesions than animals and letters. This contrasts to the activation in more posterior regions, posterior fusiform gyrus and posterolateral occipital cortex, that was higher for animals than lesions ( Table 2 and Figure 2 ).
*family wise error rate corrected p<0.05. Statistical parametric maps rendered on an International Consortium of Brain Mapping individual brain.
https://doi.org/10.1371/journal.pone.0028752.g002
* 90% confidence interval. # contrast [lesions>(animals and letters)] inclusively masked with lesions>baseline, lesions>animals, and lesions>letters at p = 0.001.
https://doi.org/10.1371/journal.pone.0028752.g003
https://doi.org/10.1371/journal.pone.0028752.t002
In summary, naming lesions, animals and letters activated the same set of distributed brain regions but to significantly different degrees. In high-order cortical regions (prefrontal and cingulate cortices), activation was proportional to the number of lexical semantic associations (lesions>animals>letters), while in visual cortices activation was higher for animal naming.
This investigation was conducted to test the proposition that generation of diagnostic hypotheses evoked by the immediate visual recognition of clinical signs engages neural systems that are recruited when naming objects in everyday life. The results support this hypothesis by showing very significant and similar activations in a circumscribed set of distributed cortical regions when naming radiological lesions and animals in the same context. However, diagnosing lesions was cognitively more demanding and associated with more activation in higher order cortical areas.
Higher mean RTs, error, and hesitation rates suggest that, on average, diagnosing lesions was more difficult than naming animals in our experimental setting. This could be related to the visual characteristics of lesions and/or the fact that low-frequency (in everyday language) lesion names are more difficult to recall, compared to high-frequency animal and letter names [12] . Lexical semantic associations, i.e. being aware of words/concepts other than that vocalized, were more frequent while diagnosing lesions ( Table 1 ), as compared to the other two categories, also indicating greater cognitive demand related to the selection of appropriate names in this particular task.
A relevant aspect of our results was that generation of diagnostic hypotheses can be very fast; the mean RT to diagnose lesions was 1.33 seconds. It is possible that the training before the fMRI experiment contributed to this performance. But very rapid identification of lesions (<1 second) has already been reported in radiology studies [13] , [14] , [15] .
An important finding was that radiologists were able to cogitate differential diagnoses during the 3.5 seconds of a trial. A similar awareness of alternative names of other animals was reported while naming animals. In a few cases even letters evoked words to subjects ( Table 1 ). Participants were not instructed to make differential diagnoses or to think about alternative names for animals during the task. These results are compatible with a fast and automatic semantic association process, in which the recall of a diagnosis or a name of an animal occurs with the concomitant activation of semantically related concepts [16] , [17] , [18] . Clearly, a formal and definitive diagnosis can not be made in seconds but its core cognitive process, the generation of diagnostic hypotheses which were the names of lesions in our study, is crucial for a final correct diagnosis [4] , [5] , [6] , [7] , [8] .
Diagnosing lesions in radiological images can be conceptualized as a process that is similar to localizing and naming objects in a scene [19] . There are several fMRI studies of location and recognition [20] or naming objects [21] but we are unaware of studies in which all of these tasks are combined. We found very significant brain activations associated with the localization, recognition and naming of targets in radiological images, be they lesions, animals, or letters ( Figure 2 ). Activations were greater in the left inferior frontal sulcus and posterior cingulate cortex for naming lesions than animals and also greater for naming animals than letters ( Table 2 and Figure 3 ). When lexical semantic associations, more frequent for lesions ( Table1 ), were taken into account in an analysis of covariance the difference of activations between those regions was no longer significant (at a corrected level). All the regions activated in the present study have also been reported in object naming in other studies [21] , therefore there was no indication that the participating radiogists were naming animals differently from laypeople.
In agreement with our findings, activation in the left inferior frontal sulcus was reported in two fMRI studies of semantic verbal fluency - generating and vocalizing associated words in response to a word - in which there was greater lexical semantic demand in contrast to the comparison task, reading aloud [22] , [23] . This is consistent with increased cognitive control when it is necessary to make a choice between synonymous or competing concepts, e.g. synonym words or differential diagnoses in our study, respectively [24] .
There were regions more activated by naming animals relative to diagnosing lesions. These regions are usually associated with visual processing and recognition of stimuli; namely, posterior fusiform cortex and posterolateral occipital cortex ( Table 2 and Figure 2 ) [25] . We believe that differences in the visual characteristics of the stimulus categories we used are responsible for the observed differences in activations in these cortical areas.
The cognitive mechanisms underlying medical diagnosis have been studied with different conceptual strategies [10] , [26] , [27] . One important approach relevant to the present study considers it a classification process similar to categorization in everyday life and several authors investigated diagnostic processes with different categorization models [28] , [29] , [30] , [31] , [32] , [33] , [34] . These studies explored several aspects of the cognitive psychology of diagnostic reasoning but did not include a comparison of medical diagnosis tasks with, e.g., categorization of objects.
Interestingly, from a historical point of view, similarities between the classification of diseases and living creatures have been suggested in the past. In the XVII th century Thomas Sydenham in his influential definition of diseases proposed to conceptualize them as specific (ontological) entities similar to plant species [35] . Influenced by Sydenham ideas, Boissier des Sauvages created a classification of diseases, nosology, based on taxonomic principles used in botany and his approach was followed by other phyisicians in the XVIII th century, including Carl Linnaeus [36] .
Instead of using categorization, a concept with different meanings and diverse and conflicting models [37] , [38] , [39] , we chose naming as a conceptually more prudent and descriptive approach. Picture naming has been a model extensively used in cognitive psychology [40] , [41] , [42] , [43] , and functional neuroimaging [21] to investigate how objects are recognized and named.
Some cognitive processes underlying medical skills have been studied with fMRI: one study investigated the neural substrate of visuo-spatial skills in surgery residents [44] and the other compared brain activations in radiologists versus lay participants while viewing X-ray images [45] . However, the neural basis of the medical diagnostic processes per se has not been investigated before.
Our experimental design was not planned to assess the generation of differential diagnostic hypotheses. Taking into account the limitations of the cued retrospective recall employed in the study, the conclusions resulting from the lexical semantic associations data need to be replicated using other methodological approaches.
In contrast to naturalistic and observational studies, planned experiments are by definition artificial and reductionistic due to the need to limit and control independent variables. Radiologists in their usual practice do not usually vocalize their diagnostic hypotheses as they come to their awareness. Conversely, we do not habitually vocalize the names of objects as we recognize them in our everyday life.
Radiologists customarily verbalize their diagnosis using more than one word, e.g. pleural effusion in right hemithorax, in contrast to one-word names for animals. This difference in length of responses could introduce an important confound variable [46] , [47] . To circumvent it, we trained the participants to preferentially use one-word names to diagnose lesions (see Methods and Appendix S1 for details). Probably as a consequence of this experimental stratagem the complete names of the lesions were reported to came to the awareness of participants and were considered competing lexical-semantic associations (see Results ).
Under the blanket rubric ‘medical diagnosis’ there are different cognitive tasks and processes. Considering the case in point of radiological diagnosis: The immediate recognition and diagnosis of an obvious lesion probably recruits different neurocognitive processes as compared to the diagnosis of a subtle and ambiguous alteration with complex differential diagnoses requiring a detailed examination of the radiological image. To create our experimental design we had to limit the scope of the investigation and the conclusions of our study are restricted to the diagnosis of lesions that are immediately identified and diagnosed. It will be important to replicate these results with other approaches, e.g. electrophysiological methods such as electroencephalography or magnetoencephalography. The conceptual hypothesis also needs to be tested in other medical specialties in which diagnosis is strongly based on visual clinical data, e.g. dermatology.
This study is an attempt to investigate the brain mechanisms subserving medical diagnosis. We have demonstrated that differential diagnoses can be automatically elicited in a time frame of seconds in response to clinical signs. Our results support the hypothesis that a process similar to naming things in everyday life occurs when a physician promptly recognizes a characteristic and previously known lesion. In our experimental model, the diagnostic task was cognitively more taxing; more activation in higher order cortical areas was plausibly associated with demands related to the selection of appropriate names as compared to the control task.
The importance of non-analytical reasoning in medical diagnosis has been increasingly stressed [9] , [10] , [27] , [48] . Our study is a contribution to the understanding of its mechanisms. There are recent reviews proposing the application of the knowledge acquired in neuroscience to improve medical education methods [49] , [50] , [51] . An implication of our results is that information obtained from cognitive neuroscience studies on the recognition and naming of objects can be brought to bear on the improvement of diagnostic expertise in the visual domain. In addition, the conceptual hypothesis and the methodological approach described in the present investigation may open new ways to develop studies in medical diagnosis.
Materials and Methods
Participants.
Twenty-six radiologists participated in the investigation. One subject was excluded because the responses were not recorded due to technical problems. Inclusion criteria were completion of radiology residency, right-handedness (as assessed by a modified version of the Edinburgh Handedness Inventory [52] ), and Portuguese as the native language; exclusion criteria were neurological and psychiatric disorders. Sixteen participants were male. The mean age of subjects was 35.9 years (range: 27–55), with a mean of 11.6 years of radiological practice (range: 4–30).
Ethics statement
The protocol was approved by the research ethics committee of the Clinics Hospital, Faculty of Medicine of the University of São Paulo, Brazil. All participants gave written informed consent. They did not receive monetary compensation for their participation.
Radiological images
Our experimental design required the radiological images to have just one circumscribed visual target that could be named. Since many thoracic radiological lesions co-occur, e.g. cardiomegaly is commonly associated with radiological signs of pulmonary venous congestion, we embedded lesions in normal X-ray images using image editing software.
Twenty different types of thoracic radiological lesions, with six different exemplars of each, were created. We used clearly identifiable and easily diagnosable lesions to minimize expertise confounds at the between-subject level and to ensure ceiling performance (to preclude performance confounds). The face validity [53] of radiological images with lesions was assessed by two senior thoracic radiologists. To create non-medical targets line drawings of animals were superimposed on the radiological images. These targets were selected from the database of the International Picture Naming Project [54] . Each type of animal, with six different exemplars, was paired with one type of lesion. Finally, 20 different consonant letters, each with six exemplars from different fonts, were paired with each type of lesion. The resulting radiological images comprised six sets of 60 different stimuli: 20 with lesions, 20 with animals, and 20 with letters.
Longer words or naming targets with more than one word might be associated with longer response times and different patterns of brain activations in regions involved in language processing [46] , [47] . To control for this confounder, we created a list of one-word names to diagnose lesions, e.g. ‘effusion’ for pleural effusion, and asked subjects to use these terms. In additon the duration of vocalization of the radiological lesion names was paired to that of the animal names. Searching for lesions, animals, and letters with the accompanying eye movements was an important variable. We controlled for it matching the locations of the three types of alterations in the chest X-ray images. The methodology used to create the radiological images is detailed in Appendix S1 .
There are many subtleties in a veridical radiological lesion that can not be reproduced with image editing software. For this reason the lesions we created can be considered caricatures of true lesions in the same way that line drawings are an iconic representation of animals.
The key differences between the three categories of stimuli comprised: 1- the visual attributes of lesions where most lesions had simpler and more heterogeneous forms compared to animals and letters which had more defined and homogeneous contours (see figure 1 ); 2- despite the absence of quantitative data on word frequency in medical domain, probably medical terms have in general a lower frequency in daily language and an older age of acquisition in comparison to animals and letters.
The experiment
The creation of images with just one target and the short viewing time of the stimulus images were critical points of our experimental strategy; they were intended to block a more careful scanning of the radiological images, which normally occurs in radiological practice. The neurocognitive processes involved in detailed scanning of the image and images with different numbers of targets to name would be important experimental confounders.
Radiological stimuli were projected through a magnetic shielded glass window to a screen inside the scanner room using a Dell 2400MP digital slide projector. The stimuli subtended 12.5° horizontal and 9.4° vertical visual angles. Each image was presented for 1.5 seconds. Radiologists were oriented just to name the target (lesion, animal or letter) as soon as it was recognized. The task implicitly involved localizing the target, recognizing it, retrieving its name and articulating the response [21] , [55] , [56] . Every image presentation was followed by a black screen with a white (central) fixation cross for 2.0 seconds (i.e., 3.5 seconds per trial). This design was optimized during pilot testing to minimize trial duration, while preserving near-ceiling performance. Participants were trained immediately before the scanning session with three different sets of images.
We used an event-related design: In each session, there were 60 trials (20 lesions, 20 animals, and 20 letters) and 20 null events (with just a fixation cross). There were three sessions per participant with three different sets of images to preclude perceptual learning, repetition suppression and other adaptation effects confounding the naming related responses. Three different sequences of stimuli presentation were optimized in terms of the efficiency to disclose fMRI responses using a genetic algorithm [57] . Each of the three sets of images was presented with one of the three optimal sequences. The order of the sequences was counterbalanced over subjects. The image sets and the order of their presentation for training and scanning were also counterbalanced between participants.
There were two control conditions: 1- naming letters, a high-level baseline, with all cognitive components of the task in diagnosing lesions and naming animals except word retrieval; 2- null events intermixed with stimuli, with a fixation cross during 3.5 seconds, during which participants had no task to execute.
Response time was defined as the elapsed time between the stimulus onset (image presentation) to the beginning of vocalization of the response. Error was defined as error proper or no response. Hesitations were considered as: 1- beginning to vocalize a word and changing to another or 2- stammering on the beginning of the vocalization.
Words and concepts may have different numbers of other words and concepts semantically associated to them [18] . There are indications that the number of potential associates to a word may influence the pattern of brain activations in tasks involving word production [58] . To control for this variable subjects were debriefed immediately after the experiment to assess lexical semantic associations - words other than that vocalized that came to their awareness while naming the different types of stimuli - in each type of stimulus following a standardized protocol (see Appendix S1 ). Participants were not informed beforehand of the debriefing protocol.
Retrospective recall has limitations [59] but we could not monitor those associations during fMRI data acquisition. To instruct subjects to report them after each stimulus could induce participants to actively search for associated words and concepts and create an important confounder in the experimental design. However, classical memory studies investigating cued recall found very high recall rates in tasks more demanding than in our investigation in particular when there was semantic processing while encoding the stimuli [60] , [61] , [62] . Also, retrospective recall methods have been used as reliable assessments in the context of fMRI experiments [63] , [64] .
Data acquisition
MR images were acquired in a 3 Tesla Philips Achieva system with an 8-channel head coil. Blood oxygenation level-dependent (BOLD) sensitive T2*-weighted images were obtained using an SENSE gradient-echo echo-planar imaging pulse sequence with the following parameters: repetition time: 2500 ms, echo time: 30 ms, flip angle: 90°, field of view: 240 mm 2 , in-plane voxel resolution: 3 mm 2 . Fifty 3 mm axial slices were acquired, with a slice gap of 0.3 mm and a +30° image plan tilt to reduce artifacts in inferior temporal lobe [65] . Functional sessions were preceded by 10.0 s of dummy scans to ensure steady-state magnetization. A T1-weighted structural image (voxel size: 1 mm 3 ) was acquired after the functional sessions for coregistration with the fMRI data.
Stimulus presentation and response recording were performed with E-Prime 2.0 software (Psychology Software Tools). A plastic mouthpiece was anatomically adjusted to the mouth of the participants enabling to isolate the sound of the vocalization of responses from the scanner's noise. The voice sound was conducted through a pneumatic system to a high-sensitivity microphone outside the scanner room, pre-amplified, and recorded. Barch et al. described a similar approach to record overt verbal responses [66] . Response times were measured following a standardized protocol using Praat 5.1, a software for phonetic analysis [67] , after filtration of the background noise.
Statistical and data analyses
Data processing and statistical analyses were conducted using SPM8 software (Wellcome Trust Centre for Neuroimaging) [68] . Functional volumes were realigned, un-warped, coregistered to the structural image, normalized to the MNI space, and smoothed with an isotropic Gaussian kernel with 6 mm FWHM.
After preprocessing, a first-level analysis was conducted at the individual level to estimate category-specific activations at each voxel. Time-series from each voxel were high-pass filtered with a cut-off period of 1/128 Hz to remove signal drift and low-frequency noise. A gray-matter image resulting from the segmentation of the structural image was used as a mask in the analysis of functional activations. All category-specific (lesion, animal or letter) trials were modeled as a stick-function and convolved with a canonical hemodynamic response function. Trials with errors, hesitations, and outlying RT's (>2 standard deviations of the mean RT for the respective target type) were modeled as events of no interest. RT's were also included as nuisance variables to remove response time effects within condition.
Category-specific activations were estimated in the usual way using the appropriate t-contrast. The resulting subject-specific contrast images were then entered into a second-level analysis of covariance (ANCOVA). This (random effects) between-subject analysis was conducted without and with lexical semantic associations (the mean number of lexical semantic associations for each category made by every participant) as a nuisance variable. The differences in category-specific activation were then assessed using statistical parametric maps (SPMs) with a criterion of p <0.05 (corrected for multiple comparisons using random field theory).
To limit head movements during the experiment participants were trained before the scanning session. The un-warping of the functional images during preprocessing with SPM8 was an additional measure to compensate for movements during the vocalization of responses [69] . Head movements during fMRI sessions were generally small, with intra-session translation and rotation movements of less than 1.5 mm and 1.5° respectively.
Supporting information.
The methodology for the creation of radiological images with embedded targets, training and debriefing protocols are detailed in Appendix S1 .
Supporting Information
Appendix s1..
https://doi.org/10.1371/journal.pone.0028752.s001
Acknowledgments
We are grateful for the cooperation of the radiologists that participated in all stages of the study and to the staff of the Magnetic Resonance Service of the Institute of Radiology, Clinics Hospital of the Faculty of Medicine, University of São Paulo. We are also thankful for the collaboration of the following people: Maria da Graça Morais Martin contributed to the experimental design suggesting the insertion of objects in the radiological images; Claudio Lucarelli and Marcelo Buarque de Gusmão Funari were responsible for the validation of the lesion images. José Jamelão Macedo de Medeiros, Fernando Araujo Del Lama, Claudia da Costa Leite, and Eduardo Massad assisted the project in several ways.
Data sharing . The complete set of radiological images used in the study are available on request from the corresponding author.
Author Contributions
Conceived and designed the experiments: MM DJS EA RBDP KJF CJP. Performed the experiments: MM. Analyzed the data: MM CJP JRS EA KJF. Contributed reagents/materials/analysis tools: KJF. Wrote the paper: MM KJF CJP. Development of the conceptual model: MM. Creation of the stimuli (radiological images): DJS RBDP MM.
- View Article
- Google Scholar
- 4. Elstein AS, Shulman LS, Sprafka SA (1978) Medical Problem Solving: An Analysis of Clinical Reasoning. Cambridge, MA: Harvard University Press.
- 17. Anderson JR (1983) The Architecture of Cognition. Cambridge, MA: Harvard University Press.
- 25. Grill-Spector K (2009) What has fMRI taught us about object recognition? In: Dickinson SJ, Leonardis A, Schiele B, Tarr MJ, editors. Object Categorization: Computer and Human Vision Perspectives. Cambridge, U.K.: Cambridge University Press. pp. 102–128.
- 27. Kassirer JP, Wong J, Kopelman RI (2010) Learning Clinical Reasoning. Baltimore, MD: Wolters Kluwer.
- 35. Faber K (1930) Nosography: The Evolution of Clinical Medicine in Modern Times. New York: Paul B. Hoeber Inc.
- 36. Bynum WF (1997) Nosology. In: Bynum WF, Porter R, editors. Companion Encyclopedia of the History of Medicine. London: Routledge. pp. 335–356.
- 37. Smith EE, Medin DL (1981) Categories and Concepts. Cambridge, MA: Harvard University Press.
- 39. Murphy GL (2004) The Big Book of Concepts. Cambridge, MA: MIT Press.
- 53. Kerlinger FN, Lee HB (2000) Validity. In: Kerlinger FN, Lee HB, editors. Foundations of Behavioral Research. 4th ed. Belmont, CA: Cengage. pp. 665–688.
- 59. Lockhart RS (2000) Methods of memory research. In: Tulving E, Craik FIM, editors. The Oxford Handbook of Memory. Oxford, U.K.: Oxford University Press. pp. 45–57.
- 68. Friston KJ, Ashburner JT, Kiebel SJ, Nichols TE, Penny WD (2007) Statistical Parametric Mapping: the Analysis of Functional Brain Images. London: Academic Press.
Frequently asked questions
What is the definition of a hypothesis.
A hypothesis states your predictions about what your research will find. It is a tentative answer to your research question that has not yet been tested. For some research projects, you might have to write several hypotheses that address different aspects of your research question.
A hypothesis is not just a guess. It should be based on existing theories and knowledge. It also has to be testable, which means you can support or refute it through scientific research methods (such as experiments, observations, and statistical analysis of data).
Frequently asked questions: Methodology
Quantitative observations involve measuring or counting something and expressing the result in numerical form, while qualitative observations involve describing something in non-numerical terms, such as its appearance, texture, or color.
To make quantitative observations , you need to use instruments that are capable of measuring the quantity you want to observe. For example, you might use a ruler to measure the length of an object or a thermometer to measure its temperature.
Scope of research is determined at the beginning of your research process , prior to the data collection stage. Sometimes called “scope of study,” your scope delineates what will and will not be covered in your project. It helps you focus your work and your time, ensuring that you’ll be able to achieve your goals and outcomes.
Defining a scope can be very useful in any research project, from a research proposal to a thesis or dissertation . A scope is needed for all types of research: quantitative , qualitative , and mixed methods .
To define your scope of research, consider the following:
- Budget constraints or any specifics of grant funding
- Your proposed timeline and duration
- Specifics about your population of study, your proposed sample size , and the research methodology you’ll pursue
- Any inclusion and exclusion criteria
- Any anticipated control , extraneous , or confounding variables that could bias your research if not accounted for properly.
Inclusion and exclusion criteria are predominantly used in non-probability sampling . In purposive sampling and snowball sampling , restrictions apply as to who can be included in the sample .
Inclusion and exclusion criteria are typically presented and discussed in the methodology section of your thesis or dissertation .
The purpose of theory-testing mode is to find evidence in order to disprove, refine, or support a theory. As such, generalisability is not the aim of theory-testing mode.
Due to this, the priority of researchers in theory-testing mode is to eliminate alternative causes for relationships between variables . In other words, they prioritise internal validity over external validity , including ecological validity .
Convergent validity shows how much a measure of one construct aligns with other measures of the same or related constructs .
On the other hand, concurrent validity is about how a measure matches up to some known criterion or gold standard, which can be another measure.
Although both types of validity are established by calculating the association or correlation between a test score and another variable , they represent distinct validation methods.
Validity tells you how accurately a method measures what it was designed to measure. There are 4 main types of validity :
- Construct validity : Does the test measure the construct it was designed to measure?
- Face validity : Does the test appear to be suitable for its objectives ?
- Content validity : Does the test cover all relevant parts of the construct it aims to measure.
- Criterion validity : Do the results accurately measure the concrete outcome they are designed to measure?
Criterion validity evaluates how well a test measures the outcome it was designed to measure. An outcome can be, for example, the onset of a disease.
Criterion validity consists of two subtypes depending on the time at which the two measures (the criterion and your test) are obtained:
- Concurrent validity is a validation strategy where the the scores of a test and the criterion are obtained at the same time
- Predictive validity is a validation strategy where the criterion variables are measured after the scores of the test
Attrition refers to participants leaving a study. It always happens to some extent – for example, in randomised control trials for medical research.
Differential attrition occurs when attrition or dropout rates differ systematically between the intervention and the control group . As a result, the characteristics of the participants who drop out differ from the characteristics of those who stay in the study. Because of this, study results may be biased .
Criterion validity and construct validity are both types of measurement validity . In other words, they both show you how accurately a method measures something.
While construct validity is the degree to which a test or other measurement method measures what it claims to measure, criterion validity is the degree to which a test can predictively (in the future) or concurrently (in the present) measure something.
Construct validity is often considered the overarching type of measurement validity . You need to have face validity , content validity , and criterion validity in order to achieve construct validity.
Convergent validity and discriminant validity are both subtypes of construct validity . Together, they help you evaluate whether a test measures the concept it was designed to measure.
- Convergent validity indicates whether a test that is designed to measure a particular construct correlates with other tests that assess the same or similar construct.
- Discriminant validity indicates whether two tests that should not be highly related to each other are indeed not related. This type of validity is also called divergent validity .
You need to assess both in order to demonstrate construct validity. Neither one alone is sufficient for establishing construct validity.
Face validity and content validity are similar in that they both evaluate how suitable the content of a test is. The difference is that face validity is subjective, and assesses content at surface level.
When a test has strong face validity, anyone would agree that the test’s questions appear to measure what they are intended to measure.
For example, looking at a 4th grade math test consisting of problems in which students have to add and multiply, most people would agree that it has strong face validity (i.e., it looks like a math test).
On the other hand, content validity evaluates how well a test represents all the aspects of a topic. Assessing content validity is more systematic and relies on expert evaluation. of each question, analysing whether each one covers the aspects that the test was designed to cover.
A 4th grade math test would have high content validity if it covered all the skills taught in that grade. Experts(in this case, math teachers), would have to evaluate the content validity by comparing the test to the learning objectives.
Content validity shows you how accurately a test or other measurement method taps into the various aspects of the specific construct you are researching.
In other words, it helps you answer the question: “does the test measure all aspects of the construct I want to measure?” If it does, then the test has high content validity.
The higher the content validity, the more accurate the measurement of the construct.
If the test fails to include parts of the construct, or irrelevant parts are included, the validity of the instrument is threatened, which brings your results into question.
Construct validity refers to how well a test measures the concept (or construct) it was designed to measure. Assessing construct validity is especially important when you’re researching concepts that can’t be quantified and/or are intangible, like introversion. To ensure construct validity your test should be based on known indicators of introversion ( operationalisation ).
On the other hand, content validity assesses how well the test represents all aspects of the construct. If some aspects are missing or irrelevant parts are included, the test has low content validity.
- Discriminant validity indicates whether two tests that should not be highly related to each other are indeed not related
Construct validity has convergent and discriminant subtypes. They assist determine if a test measures the intended notion.
The reproducibility and replicability of a study can be ensured by writing a transparent, detailed method section and using clear, unambiguous language.
Reproducibility and replicability are related terms.
- A successful reproduction shows that the data analyses were conducted in a fair and honest manner.
- A successful replication shows that the reliability of the results is high.
- Reproducing research entails reanalysing the existing data in the same manner.
- Replicating (or repeating ) the research entails reconducting the entire analysis, including the collection of new data .
Snowball sampling is a non-probability sampling method . Unlike probability sampling (which involves some form of random selection ), the initial individuals selected to be studied are the ones who recruit new participants.
Because not every member of the target population has an equal chance of being recruited into the sample, selection in snowball sampling is non-random.
Snowball sampling is a non-probability sampling method , where there is not an equal chance for every member of the population to be included in the sample .
This means that you cannot use inferential statistics and make generalisations – often the goal of quantitative research . As such, a snowball sample is not representative of the target population, and is usually a better fit for qualitative research .
Snowball sampling relies on the use of referrals. Here, the researcher recruits one or more initial participants, who then recruit the next ones.
Participants share similar characteristics and/or know each other. Because of this, not every member of the population has an equal chance of being included in the sample, giving rise to sampling bias .
Snowball sampling is best used in the following cases:
- If there is no sampling frame available (e.g., people with a rare disease)
- If the population of interest is hard to access or locate (e.g., people experiencing homelessness)
- If the research focuses on a sensitive topic (e.g., extra-marital affairs)
Stratified sampling and quota sampling both involve dividing the population into subgroups and selecting units from each subgroup. The purpose in both cases is to select a representative sample and/or to allow comparisons between subgroups.
The main difference is that in stratified sampling, you draw a random sample from each subgroup ( probability sampling ). In quota sampling you select a predetermined number or proportion of units, in a non-random manner ( non-probability sampling ).
Random sampling or probability sampling is based on random selection. This means that each unit has an equal chance (i.e., equal probability) of being included in the sample.
On the other hand, convenience sampling involves stopping people at random, which means that not everyone has an equal chance of being selected depending on the place, time, or day you are collecting your data.
Convenience sampling and quota sampling are both non-probability sampling methods. They both use non-random criteria like availability, geographical proximity, or expert knowledge to recruit study participants.
However, in convenience sampling, you continue to sample units or cases until you reach the required sample size.
In quota sampling, you first need to divide your population of interest into subgroups (strata) and estimate their proportions (quota) in the population. Then you can start your data collection , using convenience sampling to recruit participants, until the proportions in each subgroup coincide with the estimated proportions in the population.
A sampling frame is a list of every member in the entire population . It is important that the sampling frame is as complete as possible, so that your sample accurately reflects your population.
Stratified and cluster sampling may look similar, but bear in mind that groups created in cluster sampling are heterogeneous , so the individual characteristics in the cluster vary. In contrast, groups created in stratified sampling are homogeneous , as units share characteristics.
Relatedly, in cluster sampling you randomly select entire groups and include all units of each group in your sample. However, in stratified sampling, you select some units of all groups and include them in your sample. In this way, both methods can ensure that your sample is representative of the target population .
When your population is large in size, geographically dispersed, or difficult to contact, it’s necessary to use a sampling method .
This allows you to gather information from a smaller part of the population, i.e. the sample, and make accurate statements by using statistical analysis. A few sampling methods include simple random sampling , convenience sampling , and snowball sampling .
The two main types of social desirability bias are:
- Self-deceptive enhancement (self-deception): The tendency to see oneself in a favorable light without realizing it.
- Impression managemen t (other-deception): The tendency to inflate one’s abilities or achievement in order to make a good impression on other people.
Response bias refers to conditions or factors that take place during the process of responding to surveys, affecting the responses. One type of response bias is social desirability bias .
Demand characteristics are aspects of experiments that may give away the research objective to participants. Social desirability bias occurs when participants automatically try to respond in ways that make them seem likeable in a study, even if it means misrepresenting how they truly feel.
Participants may use demand characteristics to infer social norms or experimenter expectancies and act in socially desirable ways, so you should try to control for demand characteristics wherever possible.
A systematic review is secondary research because it uses existing research. You don’t collect new data yourself.
Ethical considerations in research are a set of principles that guide your research designs and practices. These principles include voluntary participation, informed consent, anonymity, confidentiality, potential for harm, and results communication.
Scientists and researchers must always adhere to a certain code of conduct when collecting data from others .
These considerations protect the rights of research participants, enhance research validity , and maintain scientific integrity.
Research ethics matter for scientific integrity, human rights and dignity, and collaboration between science and society. These principles make sure that participation in studies is voluntary, informed, and safe.
Research misconduct means making up or falsifying data, manipulating data analyses, or misrepresenting results in research reports. It’s a form of academic fraud.
These actions are committed intentionally and can have serious consequences; research misconduct is not a simple mistake or a point of disagreement but a serious ethical failure.
Anonymity means you don’t know who the participants are, while confidentiality means you know who they are but remove identifying information from your research report. Both are important ethical considerations .
You can only guarantee anonymity by not collecting any personally identifying information – for example, names, phone numbers, email addresses, IP addresses, physical characteristics, photos, or videos.
You can keep data confidential by using aggregate information in your research report, so that you only refer to groups of participants rather than individuals.
Peer review is a process of evaluating submissions to an academic journal. Utilising rigorous criteria, a panel of reviewers in the same subject area decide whether to accept each submission for publication.
For this reason, academic journals are often considered among the most credible sources you can use in a research project – provided that the journal itself is trustworthy and well regarded.
In general, the peer review process follows the following steps:
- First, the author submits the manuscript to the editor.
- Reject the manuscript and send it back to author, or
- Send it onward to the selected peer reviewer(s)
- Next, the peer review process occurs. The reviewer provides feedback, addressing any major or minor issues with the manuscript, and gives their advice regarding what edits should be made.
- Lastly, the edited manuscript is sent back to the author. They input the edits, and resubmit it to the editor for publication.
Peer review can stop obviously problematic, falsified, or otherwise untrustworthy research from being published. It also represents an excellent opportunity to get feedback from renowned experts in your field.
It acts as a first defence, helping you ensure your argument is clear and that there are no gaps, vague terms, or unanswered questions for readers who weren’t involved in the research process.
Peer-reviewed articles are considered a highly credible source due to this stringent process they go through before publication.
Many academic fields use peer review , largely to determine whether a manuscript is suitable for publication. Peer review enhances the credibility of the published manuscript.
However, peer review is also common in non-academic settings. The United Nations, the European Union, and many individual nations use peer review to evaluate grant applications. It is also widely used in medical and health-related fields as a teaching or quality-of-care measure.
Peer assessment is often used in the classroom as a pedagogical tool. Both receiving feedback and providing it are thought to enhance the learning process, helping students think critically and collaboratively.
- In a single-blind study , only the participants are blinded.
- In a double-blind study , both participants and experimenters are blinded.
- In a triple-blind study , the assignment is hidden not only from participants and experimenters, but also from the researchers analysing the data.
Blinding is important to reduce bias (e.g., observer bias , demand characteristics ) and ensure a study’s internal validity .
If participants know whether they are in a control or treatment group , they may adjust their behaviour in ways that affect the outcome that researchers are trying to measure. If the people administering the treatment are aware of group assignment, they may treat participants differently and thus directly or indirectly influence the final results.
Blinding means hiding who is assigned to the treatment group and who is assigned to the control group in an experiment .
Explanatory research is a research method used to investigate how or why something occurs when only a small amount of information is available pertaining to that topic. It can help you increase your understanding of a given topic.
Explanatory research is used to investigate how or why a phenomenon occurs. Therefore, this type of research is often one of the first stages in the research process , serving as a jumping-off point for future research.
Exploratory research is a methodology approach that explores research questions that have not previously been studied in depth. It is often used when the issue you’re studying is new, or the data collection process is challenging in some way.
Exploratory research is often used when the issue you’re studying is new or when the data collection process is challenging for some reason.
You can use exploratory research if you have a general idea or a specific question that you want to study but there is no preexisting knowledge or paradigm with which to study it.
To implement random assignment , assign a unique number to every member of your study’s sample .
Then, you can use a random number generator or a lottery method to randomly assign each number to a control or experimental group. You can also do so manually, by flipping a coin or rolling a die to randomly assign participants to groups.
Random selection, or random sampling , is a way of selecting members of a population for your study’s sample.
In contrast, random assignment is a way of sorting the sample into control and experimental groups.
Random sampling enhances the external validity or generalisability of your results, while random assignment improves the internal validity of your study.
Random assignment is used in experiments with a between-groups or independent measures design. In this research design, there’s usually a control group and one or more experimental groups. Random assignment helps ensure that the groups are comparable.
In general, you should always use random assignment in this type of experimental design when it is ethically possible and makes sense for your study topic.
Clean data are valid, accurate, complete, consistent, unique, and uniform. Dirty data include inconsistencies and errors.
Dirty data can come from any part of the research process, including poor research design , inappropriate measurement materials, or flawed data entry.
Data cleaning takes place between data collection and data analyses. But you can use some methods even before collecting data.
For clean data, you should start by designing measures that collect valid data. Data validation at the time of data entry or collection helps you minimize the amount of data cleaning you’ll need to do.
After data collection, you can use data standardisation and data transformation to clean your data. You’ll also deal with any missing values, outliers, and duplicate values.
Data cleaning involves spotting and resolving potential data inconsistencies or errors to improve your data quality. An error is any value (e.g., recorded weight) that doesn’t reflect the true value (e.g., actual weight) of something that’s being measured.
In this process, you review, analyse, detect, modify, or remove ‘dirty’ data to make your dataset ‘clean’. Data cleaning is also called data cleansing or data scrubbing.
Data cleaning is necessary for valid and appropriate analyses. Dirty data contain inconsistencies or errors , but cleaning your data helps you minimise or resolve these.
Without data cleaning, you could end up with a Type I or II error in your conclusion. These types of erroneous conclusions can be practically significant with important consequences, because they lead to misplaced investments or missed opportunities.
Observer bias occurs when a researcher’s expectations, opinions, or prejudices influence what they perceive or record in a study. It usually affects studies when observers are aware of the research aims or hypotheses. This type of research bias is also called detection bias or ascertainment bias .
The observer-expectancy effect occurs when researchers influence the results of their own study through interactions with participants.
Researchers’ own beliefs and expectations about the study results may unintentionally influence participants through demand characteristics .
You can use several tactics to minimise observer bias .
- Use masking (blinding) to hide the purpose of your study from all observers.
- Triangulate your data with different data collection methods or sources.
- Use multiple observers and ensure inter-rater reliability.
- Train your observers to make sure data is consistently recorded between them.
- Standardise your observation procedures to make sure they are structured and clear.
Naturalistic observation is a valuable tool because of its flexibility, external validity , and suitability for topics that can’t be studied in a lab setting.
The downsides of naturalistic observation include its lack of scientific control , ethical considerations , and potential for bias from observers and subjects.
Naturalistic observation is a qualitative research method where you record the behaviours of your research subjects in real-world settings. You avoid interfering or influencing anything in a naturalistic observation.
You can think of naturalistic observation as ‘people watching’ with a purpose.
Closed-ended, or restricted-choice, questions offer respondents a fixed set of choices to select from. These questions are easier to answer quickly.
Open-ended or long-form questions allow respondents to answer in their own words. Because there are no restrictions on their choices, respondents can answer in ways that researchers may not have otherwise considered.
You can organise the questions logically, with a clear progression from simple to complex, or randomly between respondents. A logical flow helps respondents process the questionnaire easier and quicker, but it may lead to bias. Randomisation can minimise the bias from order effects.
Questionnaires can be self-administered or researcher-administered.
Self-administered questionnaires can be delivered online or in paper-and-pen formats, in person or by post. All questions are standardised so that all respondents receive the same questions with identical wording.
Researcher-administered questionnaires are interviews that take place by phone, in person, or online between researchers and respondents. You can gain deeper insights by clarifying questions for respondents or asking follow-up questions.
In a controlled experiment , all extraneous variables are held constant so that they can’t influence the results. Controlled experiments require:
- A control group that receives a standard treatment, a fake treatment, or no treatment
- Random assignment of participants to ensure the groups are equivalent
Depending on your study topic, there are various other methods of controlling variables .
An experimental group, also known as a treatment group, receives the treatment whose effect researchers wish to study, whereas a control group does not. They should be identical in all other ways.
A true experiment (aka a controlled experiment) always includes at least one control group that doesn’t receive the experimental treatment.
However, some experiments use a within-subjects design to test treatments without a control group. In these designs, you usually compare one group’s outcomes before and after a treatment (instead of comparing outcomes between different groups).
For strong internal validity , it’s usually best to include a control group if possible. Without a control group, it’s harder to be certain that the outcome was caused by the experimental treatment and not by other variables.
A questionnaire is a data collection tool or instrument, while a survey is an overarching research method that involves collecting and analysing data from people using questionnaires.
A Likert scale is a rating scale that quantitatively assesses opinions, attitudes, or behaviours. It is made up of four or more questions that measure a single attitude or trait when response scores are combined.
To use a Likert scale in a survey , you present participants with Likert-type questions or statements, and a continuum of items, usually with five or seven possible responses, to capture their degree of agreement.
Individual Likert-type questions are generally considered ordinal data , because the items have clear rank order, but don’t have an even distribution.
Overall Likert scale scores are sometimes treated as interval data. These scores are considered to have directionality and even spacing between them.
The type of data determines what statistical tests you should use to analyse your data.
A research hypothesis is your proposed answer to your research question. The research hypothesis usually includes an explanation (‘ x affects y because …’).
A statistical hypothesis, on the other hand, is a mathematical statement about a population parameter. Statistical hypotheses always come in pairs: the null and alternative hypotheses. In a well-designed study , the statistical hypotheses correspond logically to the research hypothesis.
Cross-sectional studies are less expensive and time-consuming than many other types of study. They can provide useful insights into a population’s characteristics and identify correlations for further research.
Sometimes only cross-sectional data are available for analysis; other times your research question may only require a cross-sectional study to answer it.
Cross-sectional studies cannot establish a cause-and-effect relationship or analyse behaviour over a period of time. To investigate cause and effect, you need to do a longitudinal study or an experimental study .
Longitudinal studies and cross-sectional studies are two different types of research design . In a cross-sectional study you collect data from a population at a specific point in time; in a longitudinal study you repeatedly collect data from the same sample over an extended period of time.
Longitudinal studies are better to establish the correct sequence of events, identify changes over time, and provide insight into cause-and-effect relationships, but they also tend to be more expensive and time-consuming than other types of studies.
The 1970 British Cohort Study , which has collected data on the lives of 17,000 Brits since their births in 1970, is one well-known example of a longitudinal study .
Longitudinal studies can last anywhere from weeks to decades, although they tend to be at least a year long.
A correlation reflects the strength and/or direction of the association between two or more variables.
- A positive correlation means that both variables change in the same direction.
- A negative correlation means that the variables change in opposite directions.
- A zero correlation means there’s no relationship between the variables.
A correlational research design investigates relationships between two variables (or more) without the researcher controlling or manipulating any of them. It’s a non-experimental type of quantitative research .
A correlation coefficient is a single number that describes the strength and direction of the relationship between your variables.
Different types of correlation coefficients might be appropriate for your data based on their levels of measurement and distributions . The Pearson product-moment correlation coefficient (Pearson’s r ) is commonly used to assess a linear relationship between two quantitative variables.
Controlled experiments establish causality, whereas correlational studies only show associations between variables.
- In an experimental design , you manipulate an independent variable and measure its effect on a dependent variable. Other variables are controlled so they can’t impact the results.
- In a correlational design , you measure variables without manipulating any of them. You can test whether your variables change together, but you can’t be sure that one variable caused a change in another.
In general, correlational research is high in external validity while experimental research is high in internal validity .
The third variable and directionality problems are two main reasons why correlation isn’t causation .
The third variable problem means that a confounding variable affects both variables to make them seem causally related when they are not.
The directionality problem is when two variables correlate and might actually have a causal relationship, but it’s impossible to conclude which variable causes changes in the other.
As a rule of thumb, questions related to thoughts, beliefs, and feelings work well in focus groups . Take your time formulating strong questions, paying special attention to phrasing. Be careful to avoid leading questions , which can bias your responses.
Overall, your focus group questions should be:
- Open-ended and flexible
- Impossible to answer with ‘yes’ or ‘no’ (questions that start with ‘why’ or ‘how’ are often best)
- Unambiguous, getting straight to the point while still stimulating discussion
- Unbiased and neutral
Social desirability bias is the tendency for interview participants to give responses that will be viewed favourably by the interviewer or other participants. It occurs in all types of interviews and surveys , but is most common in semi-structured interviews , unstructured interviews , and focus groups .
Social desirability bias can be mitigated by ensuring participants feel at ease and comfortable sharing their views. Make sure to pay attention to your own body language and any physical or verbal cues, such as nodding or widening your eyes.
This type of bias in research can also occur in observations if the participants know they’re being observed. They might alter their behaviour accordingly.
A focus group is a research method that brings together a small group of people to answer questions in a moderated setting. The group is chosen due to predefined demographic traits, and the questions are designed to shed light on a topic of interest. It is one of four types of interviews .
The four most common types of interviews are:
- Structured interviews : The questions are predetermined in both topic and order.
- Semi-structured interviews : A few questions are predetermined, but other questions aren’t planned.
- Unstructured interviews : None of the questions are predetermined.
- Focus group interviews : The questions are presented to a group instead of one individual.
An unstructured interview is the most flexible type of interview, but it is not always the best fit for your research topic.
Unstructured interviews are best used when:
- You are an experienced interviewer and have a very strong background in your research topic, since it is challenging to ask spontaneous, colloquial questions
- Your research question is exploratory in nature. While you may have developed hypotheses, you are open to discovering new or shifting viewpoints through the interview process.
- You are seeking descriptive data, and are ready to ask questions that will deepen and contextualise your initial thoughts and hypotheses
- Your research depends on forming connections with your participants and making them feel comfortable revealing deeper emotions, lived experiences, or thoughts
A semi-structured interview is a blend of structured and unstructured types of interviews. Semi-structured interviews are best used when:
- You have prior interview experience. Spontaneous questions are deceptively challenging, and it’s easy to accidentally ask a leading question or make a participant uncomfortable.
- Your research question is exploratory in nature. Participant answers can guide future research questions and help you develop a more robust knowledge base for future research.
The interviewer effect is a type of bias that emerges when a characteristic of an interviewer (race, age, gender identity, etc.) influences the responses given by the interviewee.
There is a risk of an interviewer effect in all types of interviews , but it can be mitigated by writing really high-quality interview questions.
A structured interview is a data collection method that relies on asking questions in a set order to collect data on a topic. They are often quantitative in nature. Structured interviews are best used when:
- You already have a very clear understanding of your topic. Perhaps significant research has already been conducted, or you have done some prior research yourself, but you already possess a baseline for designing strong structured questions.
- You are constrained in terms of time or resources and need to analyse your data quickly and efficiently
- Your research question depends on strong parity between participants, with environmental conditions held constant
More flexible interview options include semi-structured interviews , unstructured interviews , and focus groups .
When conducting research, collecting original data has significant advantages:
- You can tailor data collection to your specific research aims (e.g., understanding the needs of your consumers or user testing your website).
- You can control and standardise the process for high reliability and validity (e.g., choosing appropriate measurements and sampling methods ).
However, there are also some drawbacks: data collection can be time-consuming, labour-intensive, and expensive. In some cases, it’s more efficient to use secondary data that has already been collected by someone else, but the data might be less reliable.
Data collection is the systematic process by which observations or measurements are gathered in research. It is used in many different contexts by academics, governments, businesses, and other organisations.
A mediator variable explains the process through which two variables are related, while a moderator variable affects the strength and direction of that relationship.
A confounder is a third variable that affects variables of interest and makes them seem related when they are not. In contrast, a mediator is the mechanism of a relationship between two variables: it explains the process by which they are related.
If something is a mediating variable :
- It’s caused by the independent variable
- It influences the dependent variable
- When it’s taken into account, the statistical correlation between the independent and dependent variables is higher than when it isn’t considered
Including mediators and moderators in your research helps you go beyond studying a simple relationship between two variables for a fuller picture of the real world. They are important to consider when studying complex correlational or causal relationships.
Mediators are part of the causal pathway of an effect, and they tell you how or why an effect takes place. Moderators usually help you judge the external validity of your study by identifying the limitations of when the relationship between variables holds.
You can think of independent and dependent variables in terms of cause and effect: an independent variable is the variable you think is the cause , while a dependent variable is the effect .
In an experiment, you manipulate the independent variable and measure the outcome in the dependent variable. For example, in an experiment about the effect of nutrients on crop growth:
- The independent variable is the amount of nutrients added to the crop field.
- The dependent variable is the biomass of the crops at harvest time.
Defining your variables, and deciding how you will manipulate and measure them, is an important part of experimental design .
Discrete and continuous variables are two types of quantitative variables :
- Discrete variables represent counts (e.g., the number of objects in a collection).
- Continuous variables represent measurable amounts (e.g., water volume or weight).
Quantitative variables are any variables where the data represent amounts (e.g. height, weight, or age).
Categorical variables are any variables where the data represent groups. This includes rankings (e.g. finishing places in a race), classifications (e.g. brands of cereal), and binary outcomes (e.g. coin flips).
You need to know what type of variables you are working with to choose the right statistical test for your data and interpret your results .
Determining cause and effect is one of the most important parts of scientific research. It’s essential to know which is the cause – the independent variable – and which is the effect – the dependent variable.
You want to find out how blood sugar levels are affected by drinking diet cola and regular cola, so you conduct an experiment .
- The type of cola – diet or regular – is the independent variable .
- The level of blood sugar that you measure is the dependent variable – it changes depending on the type of cola.
No. The value of a dependent variable depends on an independent variable, so a variable cannot be both independent and dependent at the same time. It must be either the cause or the effect, not both.
Yes, but including more than one of either type requires multiple research questions .
For example, if you are interested in the effect of a diet on health, you can use multiple measures of health: blood sugar, blood pressure, weight, pulse, and many more. Each of these is its own dependent variable with its own research question.
You could also choose to look at the effect of exercise levels as well as diet, or even the additional effect of the two combined. Each of these is a separate independent variable .
To ensure the internal validity of an experiment , you should only change one independent variable at a time.
To ensure the internal validity of your research, you must consider the impact of confounding variables. If you fail to account for them, you might over- or underestimate the causal relationship between your independent and dependent variables , or even find a causal relationship where none exists.
A confounding variable is closely related to both the independent and dependent variables in a study. An independent variable represents the supposed cause , while the dependent variable is the supposed effect . A confounding variable is a third variable that influences both the independent and dependent variables.
Failing to account for confounding variables can cause you to wrongly estimate the relationship between your independent and dependent variables.
There are several methods you can use to decrease the impact of confounding variables on your research: restriction, matching, statistical control, and randomisation.
In restriction , you restrict your sample by only including certain subjects that have the same values of potential confounding variables.
In matching , you match each of the subjects in your treatment group with a counterpart in the comparison group. The matched subjects have the same values on any potential confounding variables, and only differ in the independent variable .
In statistical control , you include potential confounders as variables in your regression .
In randomisation , you randomly assign the treatment (or independent variable) in your study to a sufficiently large number of subjects, which allows you to control for all potential confounding variables.
In scientific research, concepts are the abstract ideas or phenomena that are being studied (e.g., educational achievement). Variables are properties or characteristics of the concept (e.g., performance at school), while indicators are ways of measuring or quantifying variables (e.g., yearly grade reports).
The process of turning abstract concepts into measurable variables and indicators is called operationalisation .
In statistics, ordinal and nominal variables are both considered categorical variables .
Even though ordinal data can sometimes be numerical, not all mathematical operations can be performed on them.
A control variable is any variable that’s held constant in a research study. It’s not a variable of interest in the study, but it’s controlled because it could influence the outcomes.
Control variables help you establish a correlational or causal relationship between variables by enhancing internal validity .
If you don’t control relevant extraneous variables , they may influence the outcomes of your study, and you may not be able to demonstrate that your results are really an effect of your independent variable .
‘Controlling for a variable’ means measuring extraneous variables and accounting for them statistically to remove their effects on other variables.
Researchers often model control variable data along with independent and dependent variable data in regression analyses and ANCOVAs . That way, you can isolate the control variable’s effects from the relationship between the variables of interest.
An extraneous variable is any variable that you’re not investigating that can potentially affect the dependent variable of your research study.
A confounding variable is a type of extraneous variable that not only affects the dependent variable, but is also related to the independent variable.
There are 4 main types of extraneous variables :
- Demand characteristics : Environmental cues that encourage participants to conform to researchers’ expectations
- Experimenter effects : Unintentional actions by researchers that influence study outcomes
- Situational variables : Eenvironmental variables that alter participants’ behaviours
- Participant variables : Any characteristic or aspect of a participant’s background that could affect study results
The difference between explanatory and response variables is simple:
- An explanatory variable is the expected cause, and it explains the results.
- A response variable is the expected effect, and it responds to other variables.
The term ‘ explanatory variable ‘ is sometimes preferred over ‘ independent variable ‘ because, in real-world contexts, independent variables are often influenced by other variables. This means they aren’t totally independent.
Multiple independent variables may also be correlated with each other, so ‘explanatory variables’ is a more appropriate term.
On graphs, the explanatory variable is conventionally placed on the x -axis, while the response variable is placed on the y -axis.
- If you have quantitative variables , use a scatterplot or a line graph.
- If your response variable is categorical, use a scatterplot or a line graph.
- If your explanatory variable is categorical, use a bar graph.
A correlation is usually tested for two variables at a time, but you can test correlations between three or more variables.
An independent variable is the variable you manipulate, control, or vary in an experimental study to explore its effects. It’s called ‘independent’ because it’s not influenced by any other variables in the study.
Independent variables are also called:
- Explanatory variables (they explain an event or outcome)
- Predictor variables (they can be used to predict the value of a dependent variable)
- Right-hand-side variables (they appear on the right-hand side of a regression equation)
A dependent variable is what changes as a result of the independent variable manipulation in experiments . It’s what you’re interested in measuring, and it ‘depends’ on your independent variable.
In statistics, dependent variables are also called:
- Response variables (they respond to a change in another variable)
- Outcome variables (they represent the outcome you want to measure)
- Left-hand-side variables (they appear on the left-hand side of a regression equation)
Deductive reasoning is commonly used in scientific research, and it’s especially associated with quantitative research .
In research, you might have come across something called the hypothetico-deductive method . It’s the scientific method of testing hypotheses to check whether your predictions are substantiated by real-world data.
Deductive reasoning is a logical approach where you progress from general ideas to specific conclusions. It’s often contrasted with inductive reasoning , where you start with specific observations and form general conclusions.
Deductive reasoning is also called deductive logic.
Inductive reasoning is a method of drawing conclusions by going from the specific to the general. It’s usually contrasted with deductive reasoning, where you proceed from general information to specific conclusions.
Inductive reasoning is also called inductive logic or bottom-up reasoning.
In inductive research , you start by making observations or gathering data. Then, you take a broad scan of your data and search for patterns. Finally, you make general conclusions that you might incorporate into theories.
Inductive reasoning is a bottom-up approach, while deductive reasoning is top-down.
Inductive reasoning takes you from the specific to the general, while in deductive reasoning, you make inferences by going from general premises to specific conclusions.
There are many different types of inductive reasoning that people use formally or informally.
Here are a few common types:
- Inductive generalisation : You use observations about a sample to come to a conclusion about the population it came from.
- Statistical generalisation: You use specific numbers about samples to make statements about populations.
- Causal reasoning: You make cause-and-effect links between different things.
- Sign reasoning: You make a conclusion about a correlational relationship between different things.
- Analogical reasoning: You make a conclusion about something based on its similarities to something else.
It’s often best to ask a variety of people to review your measurements. You can ask experts, such as other researchers, or laypeople, such as potential participants, to judge the face validity of tests.
While experts have a deep understanding of research methods , the people you’re studying can provide you with valuable insights you may have missed otherwise.
Face validity is important because it’s a simple first step to measuring the overall validity of a test or technique. It’s a relatively intuitive, quick, and easy way to start checking whether a new measure seems useful at first glance.
Good face validity means that anyone who reviews your measure says that it seems to be measuring what it’s supposed to. With poor face validity, someone reviewing your measure may be left confused about what you’re measuring and why you’re using this method.
Face validity is about whether a test appears to measure what it’s supposed to measure. This type of validity is concerned with whether a measure seems relevant and appropriate for what it’s assessing only on the surface.
Statistical analyses are often applied to test validity with data from your measures. You test convergent validity and discriminant validity with correlations to see if results from your test are positively or negatively related to those of other established tests.
You can also use regression analyses to assess whether your measure is actually predictive of outcomes that you expect it to predict theoretically. A regression analysis that supports your expectations strengthens your claim of construct validity .
When designing or evaluating a measure, construct validity helps you ensure you’re actually measuring the construct you’re interested in. If you don’t have construct validity, you may inadvertently measure unrelated or distinct constructs and lose precision in your research.
Construct validity is often considered the overarching type of measurement validity , because it covers all of the other types. You need to have face validity , content validity, and criterion validity to achieve construct validity.
Construct validity is about how well a test measures the concept it was designed to evaluate. It’s one of four types of measurement validity , which includes construct validity, face validity , and criterion validity.
There are two subtypes of construct validity.
- Convergent validity : The extent to which your measure corresponds to measures of related constructs
- Discriminant validity: The extent to which your measure is unrelated or negatively related to measures of distinct constructs
Attrition bias can skew your sample so that your final sample differs significantly from your original sample. Your sample is biased because some groups from your population are underrepresented.
With a biased final sample, you may not be able to generalise your findings to the original population that you sampled from, so your external validity is compromised.
There are seven threats to external validity : selection bias , history, experimenter effect, Hawthorne effect , testing effect, aptitude-treatment, and situation effect.
The two types of external validity are population validity (whether you can generalise to other groups of people) and ecological validity (whether you can generalise to other situations and settings).
The external validity of a study is the extent to which you can generalise your findings to different groups of people, situations, and measures.
Attrition bias is a threat to internal validity . In experiments, differential rates of attrition between treatment and control groups can skew results.
This bias can affect the relationship between your independent and dependent variables . It can make variables appear to be correlated when they are not, or vice versa.
Internal validity is the extent to which you can be confident that a cause-and-effect relationship established in a study cannot be explained by other factors.
There are eight threats to internal validity : history, maturation, instrumentation, testing, selection bias , regression to the mean, social interaction, and attrition .
A sampling error is the difference between a population parameter and a sample statistic .
A statistic refers to measures about the sample , while a parameter refers to measures about the population .
Populations are used when a research question requires data from every member of the population. This is usually only feasible when the population is small and easily accessible.
Systematic sampling is a probability sampling method where researchers select members of the population at a regular interval – for example, by selecting every 15th person on a list of the population. If the population is in a random order, this can imitate the benefits of simple random sampling .
There are three key steps in systematic sampling :
- Define and list your population , ensuring that it is not ordered in a cyclical or periodic order.
- Decide on your sample size and calculate your interval, k , by dividing your population by your target sample size.
- Choose every k th member of the population as your sample.
Yes, you can create a stratified sample using multiple characteristics, but you must ensure that every participant in your study belongs to one and only one subgroup. In this case, you multiply the numbers of subgroups for each characteristic to get the total number of groups.
For example, if you were stratifying by location with three subgroups (urban, rural, or suburban) and marital status with five subgroups (single, divorced, widowed, married, or partnered), you would have 3 × 5 = 15 subgroups.
You should use stratified sampling when your sample can be divided into mutually exclusive and exhaustive subgroups that you believe will take on different mean values for the variable that you’re studying.
Using stratified sampling will allow you to obtain more precise (with lower variance ) statistical estimates of whatever you are trying to measure.
For example, say you want to investigate how income differs based on educational attainment, but you know that this relationship can vary based on race. Using stratified sampling, you can ensure you obtain a large enough sample from each racial group, allowing you to draw more precise conclusions.
In stratified sampling , researchers divide subjects into subgroups called strata based on characteristics that they share (e.g., race, gender, educational attainment).
Once divided, each subgroup is randomly sampled using another probability sampling method .
Multistage sampling can simplify data collection when you have large, geographically spread samples, and you can obtain a probability sample without a complete sampling frame.
But multistage sampling may not lead to a representative sample, and larger samples are needed for multistage samples to achieve the statistical properties of simple random samples .
In multistage sampling , you can use probability or non-probability sampling methods.
For a probability sample, you have to probability sampling at every stage. You can mix it up by using simple random sampling , systematic sampling , or stratified sampling to select units at different stages, depending on what is applicable and relevant to your study.
Cluster sampling is a probability sampling method in which you divide a population into clusters, such as districts or schools, and then randomly select some of these clusters as your sample.
The clusters should ideally each be mini-representations of the population as a whole.
There are three types of cluster sampling : single-stage, double-stage and multi-stage clustering. In all three types, you first divide the population into clusters, then randomly select clusters for use in your sample.
- In single-stage sampling , you collect data from every unit within the selected clusters.
- In double-stage sampling , you select a random sample of units from within the clusters.
- In multi-stage sampling , you repeat the procedure of randomly sampling elements from within the clusters until you have reached a manageable sample.
Cluster sampling is more time- and cost-efficient than other probability sampling methods , particularly when it comes to large samples spread across a wide geographical area.
However, it provides less statistical certainty than other methods, such as simple random sampling , because it is difficult to ensure that your clusters properly represent the population as a whole.
If properly implemented, simple random sampling is usually the best sampling method for ensuring both internal and external validity . However, it can sometimes be impractical and expensive to implement, depending on the size of the population to be studied,
If you have a list of every member of the population and the ability to reach whichever members are selected, you can use simple random sampling.
The American Community Survey is an example of simple random sampling . In order to collect detailed data on the population of the US, the Census Bureau officials randomly select 3.5 million households per year and use a variety of methods to convince them to fill out the survey.
Simple random sampling is a type of probability sampling in which the researcher randomly selects a subset of participants from a population . Each member of the population has an equal chance of being selected. Data are then collected from as large a percentage as possible of this random subset.
Sampling bias occurs when some members of a population are systematically more likely to be selected in a sample than others.
In multistage sampling , or multistage cluster sampling, you draw a sample from a population using smaller and smaller groups at each stage.
This method is often used to collect data from a large, geographically spread group of people in national surveys, for example. You take advantage of hierarchical groupings (e.g., from county to city to neighbourhood) to create a sample that’s less expensive and time-consuming to collect data from.
In non-probability sampling , the sample is selected based on non-random criteria, and not every member of the population has a chance of being included.
Common non-probability sampling methods include convenience sampling , voluntary response sampling, purposive sampling , snowball sampling , and quota sampling .
Probability sampling means that every member of the target population has a known chance of being included in the sample.
Probability sampling methods include simple random sampling , systematic sampling , stratified sampling , and cluster sampling .
Samples are used to make inferences about populations . Samples are easier to collect data from because they are practical, cost-effective, convenient, and manageable.
While a between-subjects design has fewer threats to internal validity , it also requires more participants for high statistical power than a within-subjects design .
Advantages:
- Prevents carryover effects of learning and fatigue.
- Shorter study duration.
Disadvantages:
- Needs larger samples for high power.
- Uses more resources to recruit participants, administer sessions, cover costs, etc.
- Individual differences may be an alternative explanation for results.
In a factorial design, multiple independent variables are tested.
If you test two variables, each level of one independent variable is combined with each level of the other independent variable to create different conditions.
Yes. Between-subjects and within-subjects designs can be combined in a single study when you have two or more independent variables (a factorial design). In a mixed factorial design, one variable is altered between subjects and another is altered within subjects.
Within-subjects designs have many potential threats to internal validity , but they are also very statistically powerful .
- Only requires small samples
- Statistically powerful
- Removes the effects of individual differences on the outcomes
- Internal validity threats reduce the likelihood of establishing a direct relationship between variables
- Time-related effects, such as growth, can influence the outcomes
- Carryover effects mean that the specific order of different treatments affect the outcomes
Quasi-experimental design is most useful in situations where it would be unethical or impractical to run a true experiment .
Quasi-experiments have lower internal validity than true experiments, but they often have higher external validity as they can use real-world interventions instead of artificial laboratory settings.
In experimental research, random assignment is a way of placing participants from your sample into different groups using randomisation. With this method, every member of the sample has a known or equal chance of being placed in a control group or an experimental group.
A quasi-experiment is a type of research design that attempts to establish a cause-and-effect relationship. The main difference between this and a true experiment is that the groups are not randomly assigned.
In a between-subjects design , every participant experiences only one condition, and researchers assess group differences between participants in various conditions.
In a within-subjects design , each participant experiences all conditions, and researchers test the same participants repeatedly for differences between conditions.
The word ‘between’ means that you’re comparing different conditions between groups, while the word ‘within’ means you’re comparing different conditions within the same group.
A confounding variable , also called a confounder or confounding factor, is a third variable in a study examining a potential cause-and-effect relationship.
A confounding variable is related to both the supposed cause and the supposed effect of the study. It can be difficult to separate the true effect of the independent variable from the effect of the confounding variable.
In your research design , it’s important to identify potential confounding variables and plan how you will reduce their impact.
Triangulation can help:
- Reduce bias that comes from using a single method, theory, or investigator
- Enhance validity by approaching the same topic with different tools
- Establish credibility by giving you a complete picture of the research problem
But triangulation can also pose problems:
- It’s time-consuming and labour-intensive, often involving an interdisciplinary team.
- Your results may be inconsistent or even contradictory.
There are four main types of triangulation :
- Data triangulation : Using data from different times, spaces, and people
- Investigator triangulation : Involving multiple researchers in collecting or analysing data
- Theory triangulation : Using varying theoretical perspectives in your research
- Methodological triangulation : Using different methodologies to approach the same topic
Experimental designs are a set of procedures that you plan in order to examine the relationship between variables that interest you.
To design a successful experiment, first identify:
- A testable hypothesis
- One or more independent variables that you will manipulate
- One or more dependent variables that you will measure
When designing the experiment, first decide:
- How your variable(s) will be manipulated
- How you will control for any potential confounding or lurking variables
- How many subjects you will include
- How you will assign treatments to your subjects
Exploratory research explores the main aspects of a new or barely researched question.
Explanatory research explains the causes and effects of an already widely researched question.
The key difference between observational studies and experiments is that, done correctly, an observational study will never influence the responses or behaviours of participants. Experimental designs will have a treatment condition applied to at least a portion of participants.
An observational study could be a good fit for your research if your research question is based on things you observe. If you have ethical, logistical, or practical concerns that make an experimental design challenging, consider an observational study. Remember that in an observational study, it is critical that there be no interference or manipulation of the research subjects. Since it’s not an experiment, there are no control or treatment groups either.
These are four of the most common mixed methods designs :
- Convergent parallel: Quantitative and qualitative data are collected at the same time and analysed separately. After both analyses are complete, compare your results to draw overall conclusions.
- Embedded: Quantitative and qualitative data are collected at the same time, but within a larger quantitative or qualitative design. One type of data is secondary to the other.
- Explanatory sequential: Quantitative data is collected and analysed first, followed by qualitative data. You can use this design if you think your qualitative data will explain and contextualise your quantitative findings.
- Exploratory sequential: Qualitative data is collected and analysed first, followed by quantitative data. You can use this design if you think the quantitative data will confirm or validate your qualitative findings.
Triangulation in research means using multiple datasets, methods, theories and/or investigators to address a research question. It’s a research strategy that can help you enhance the validity and credibility of your findings.
Triangulation is mainly used in qualitative research , but it’s also commonly applied in quantitative research . Mixed methods research always uses triangulation.
Operationalisation means turning abstract conceptual ideas into measurable observations.
For example, the concept of social anxiety isn’t directly observable, but it can be operationally defined in terms of self-rating scores, behavioural avoidance of crowded places, or physical anxiety symptoms in social situations.
Before collecting data , it’s important to consider how you will operationalise the variables that you want to measure.
Hypothesis testing is a formal procedure for investigating our ideas about the world using statistics. It is used by scientists to test specific predictions, called hypotheses , by calculating how likely it is that a pattern or relationship between variables could have arisen by chance.
There are five common approaches to qualitative research :
- Grounded theory involves collecting data in order to develop new theories.
- Ethnography involves immersing yourself in a group or organisation to understand its culture.
- Narrative research involves interpreting stories to understand how people make sense of their experiences and perceptions.
- Phenomenological research involves investigating phenomena through people’s lived experiences.
- Action research links theory and practice in several cycles to drive innovative changes.
There are various approaches to qualitative data analysis , but they all share five steps in common:
- Prepare and organise your data.
- Review and explore your data.
- Develop a data coding system.
- Assign codes to the data.
- Identify recurring themes.
The specifics of each step depend on the focus of the analysis. Some common approaches include textual analysis , thematic analysis , and discourse analysis .
In mixed methods research , you use both qualitative and quantitative data collection and analysis methods to answer your research question .
Methodology refers to the overarching strategy and rationale of your research project . It involves studying the methods used in your field and the theories or principles behind them, in order to develop an approach that matches your objectives.
Methods are the specific tools and procedures you use to collect and analyse data (e.g. experiments, surveys , and statistical tests ).
In shorter scientific papers, where the aim is to report the findings of a specific study, you might simply describe what you did in a methods section .
In a longer or more complex research project, such as a thesis or dissertation , you will probably include a methodology section , where you explain your approach to answering the research questions and cite relevant sources to support your choice of methods.
The research methods you use depend on the type of data you need to answer your research question .
- If you want to measure something or test a hypothesis , use quantitative methods . If you want to explore ideas, thoughts, and meanings, use qualitative methods .
- If you want to analyse a large amount of readily available data, use secondary data. If you want data specific to your purposes with control over how they are generated, collect primary data.
- If you want to establish cause-and-effect relationships between variables , use experimental methods. If you want to understand the characteristics of a research subject, use descriptive methods.
Ask our team
Want to contact us directly? No problem. We are always here for you.
- Chat with us
- Email [email protected]
- Call +44 (0)20 3917 4242
- WhatsApp +31 20 261 6040
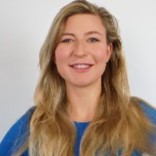
Our support team is here to help you daily via chat, WhatsApp, email, or phone between 9:00 a.m. to 11:00 p.m. CET.
Our APA experts default to APA 7 for editing and formatting. For the Citation Editing Service you are able to choose between APA 6 and 7.
Yes, if your document is longer than 20,000 words, you will get a sample of approximately 2,000 words. This sample edit gives you a first impression of the editor’s editing style and a chance to ask questions and give feedback.
How does the sample edit work?
You will receive the sample edit within 24 hours after placing your order. You then have 24 hours to let us know if you’re happy with the sample or if there’s something you would like the editor to do differently.
Read more about how the sample edit works
Yes, you can upload your document in sections.
We try our best to ensure that the same editor checks all the different sections of your document. When you upload a new file, our system recognizes you as a returning customer, and we immediately contact the editor who helped you before.
However, we cannot guarantee that the same editor will be available. Your chances are higher if
- You send us your text as soon as possible and
- You can be flexible about the deadline.
Please note that the shorter your deadline is, the lower the chance that your previous editor is not available.
If your previous editor isn’t available, then we will inform you immediately and look for another qualified editor. Fear not! Every Scribbr editor follows the Scribbr Improvement Model and will deliver high-quality work.
Yes, our editors also work during the weekends and holidays.
Because we have many editors available, we can check your document 24 hours per day and 7 days per week, all year round.
If you choose a 72 hour deadline and upload your document on a Thursday evening, you’ll have your thesis back by Sunday evening!
Yes! Our editors are all native speakers, and they have lots of experience editing texts written by ESL students. They will make sure your grammar is perfect and point out any sentences that are difficult to understand. They’ll also notice your most common mistakes, and give you personal feedback to improve your writing in English.
Every Scribbr order comes with our award-winning Proofreading & Editing service , which combines two important stages of the revision process.
For a more comprehensive edit, you can add a Structure Check or Clarity Check to your order. With these building blocks, you can customize the kind of feedback you receive.
You might be familiar with a different set of editing terms. To help you understand what you can expect at Scribbr, we created this table:
View an example
When you place an order, you can specify your field of study and we’ll match you with an editor who has familiarity with this area.
However, our editors are language specialists, not academic experts in your field. Your editor’s job is not to comment on the content of your dissertation, but to improve your language and help you express your ideas as clearly and fluently as possible.
This means that your editor will understand your text well enough to give feedback on its clarity, logic and structure, but not on the accuracy or originality of its content.
Good academic writing should be understandable to a non-expert reader, and we believe that academic editing is a discipline in itself. The research, ideas and arguments are all yours – we’re here to make sure they shine!
After your document has been edited, you will receive an email with a link to download the document.
The editor has made changes to your document using ‘Track Changes’ in Word. This means that you only have to accept or ignore the changes that are made in the text one by one.
It is also possible to accept all changes at once. However, we strongly advise you not to do so for the following reasons:
- You can learn a lot by looking at the mistakes you made.
- The editors don’t only change the text – they also place comments when sentences or sometimes even entire paragraphs are unclear. You should read through these comments and take into account your editor’s tips and suggestions.
- With a final read-through, you can make sure you’re 100% happy with your text before you submit!
You choose the turnaround time when ordering. We can return your dissertation within 24 hours , 3 days or 1 week . These timescales include weekends and holidays. As soon as you’ve paid, the deadline is set, and we guarantee to meet it! We’ll notify you by text and email when your editor has completed the job.
Very large orders might not be possible to complete in 24 hours. On average, our editors can complete around 13,000 words in a day while maintaining our high quality standards. If your order is longer than this and urgent, contact us to discuss possibilities.
Always leave yourself enough time to check through the document and accept the changes before your submission deadline.
Scribbr is specialised in editing study related documents. We check:
- Graduation projects
- Dissertations
- Admissions essays
- College essays
- Application essays
- Personal statements
- Process reports
- Reflections
- Internship reports
- Academic papers
- Research proposals
- Prospectuses
Calculate the costs
The fastest turnaround time is 24 hours.
You can upload your document at any time and choose between three deadlines:
At Scribbr, we promise to make every customer 100% happy with the service we offer. Our philosophy: Your complaint is always justified – no denial, no doubts.
Our customer support team is here to find the solution that helps you the most, whether that’s a free new edit or a refund for the service.
Yes, in the order process you can indicate your preference for American, British, or Australian English .
If you don’t choose one, your editor will follow the style of English you currently use. If your editor has any questions about this, we will contact you.
What is Medical Terminology? [Explanations + Helpful Resources]
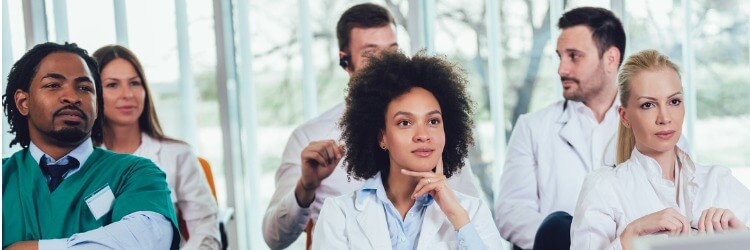
Medical Terminology 101: Definitions, Applications & Helpful Resources
Understanding medical terminology can make all the difference in critical health situations. And while understanding medical language is serious business for care providers, learning medical terminology can actually be a thought-provoking, interesting crash course in the history and development of language and parts of speech.
For those who are new to, or are looking to enter the medical field, having a firm grasp on medical verbiage, roots and meanings can give you a leg up as you continue your studies and progress in your career. To get you started, here is our introductory guide to medical terminology, which will serve as a solid foundation as you work to diversify and enhance your medical language skills.
What is Medical Terminology
Medical terminology refers to the words and language used specifically in the medical and health fields. The proper definition describes medical terminology as language used to describe anatomical structures, procedures, conditions, processes and treatments in the medical field.
While memorizing terms across that many disciplines might seem impossible, medical language can be simplified when you understand that nearly all medical terms can be broken down into three parts: the prefix, the root and the suffix.
- Prefix — appears at the beginning of a term and indicates a location, direction, type, quality or quantity.
- Root — can appear at the beginning of the word if prefix is absent, but the root indicates the primary meaning of the medical term.
- Suffix — appears at the end of the word and indicates a specialty, test, procedure, function, disorder or status.
Why is Medical Terminology Important in the Medical Field
Medical terminology is the linguistic foundation on which the entire healthcare system is built. Because physicians are trained at varying universities and complete their training across different hospitals and disciplines and geographies, having a singular medical terminology system ensures that effective care is delivered while reducing the potential for miscommunication.
- More specifically, medical terminology is important for a few reasons, both for medical professionals and the field itself. Medical terminology courses or certificates are usually a prerequisite for most graduate degree or certificate programs in the healthcare field.
- For those already working in the medical field — such as medical records specialists, radiology techs and even physicians — a refresher on traditional and new medical terminology can be helpful and may even be required for license renewal.
Why is Standardization of Medical Terminology Important?
Studies show that a standardized medical terminology system can improve overall hospital operations — both in terms of data and clinical applications of such data. A second report found that medical terminology and the usage of structured reporting can improve the usage of medical information in secondary activities, such as research, public health and case studies. This not only will help make research efforts more efficient, it will likely result in better outcomes for patients and medical innovation.
Additional benefits of standardized medical terminology include:
- Ensures consistent documentation of clinical information
- Creates a streamlined system for data entry and retrieval across electronic medical records for billing and insurance purposes
- Ensures a consistent understanding of language across the continuum of care
- Ensures that all relevant parties are educated across a universal standard Improves patient care and safety, research and evidence-based medicine
- Helps promote efficient communication
- Helps create a seamless patient experience
A MEDICAL TERMINOLOGY COURSE IS VALUABLE TO YOUR CAREER
Taking a medical terminology course is a great first step to equip you with the skills and knowledge you’ll need to be successful.
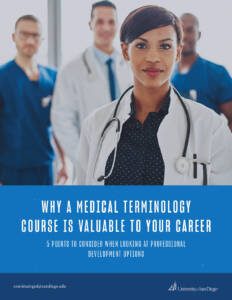
Medical Terminology: Examples of Prefixes
Medical terminology: examples of root words.
A lot of medical terms get their roots from Latin and ancient Greek language, much like many words in the English and romance languages. Here are some of the most common medical root words healthcare providers must know.
Medical Terminology: Examples of Suffixes
The last ingredient in a medical term is likely the suffix, which provides more specifics about the term’s application. Here are just a few of the most important medical suffixes to know.
Medical Terminology Resources List
Just as you would with any new skill, expanding your vocabulary and learning medical terminology takes practice, practice, practice. To assist you during your studies, we’ve rounded up some helpful resources and study tools focused on medical terminology.
- University of San Diego Medical Terminology online course
- Merck Medical Terminology Manual
- Merriam-Webster Medical Dictionary
- OpenMD.com Medical Terminology Dictionary
- Understanding Medical Words: A Tutorial from the National Library of Medicine
- CMA (AAMA) Practice Exam: Medical Terminology
- Free Online Medical Terminology Course
- Medical Terminology Flashcard Sets and Study Tools
No matter what resource you turn to, we recommend starting with the Medical Terminology course from the University of San Diego . This online, self-paced course allows you to immerse yourself in the world of medical language in a way that works for you and your schedule. Participants in this course gain a deep understanding of important medical, diagnostic and laboratory abbreviations and terms they will need throughout their medical careers. To learn more about this course or any of our healthcare programs, please contact us.
Common Questions About the USD Medical Terminology Course
How long will this course take me to complete.
This course has a maximum of six (6) months from the date of enrollment to complete the course. Students are recommended to allocate a minimum of two weeks (14 calendar days) per semester unit to complete a course; this time frame allows for instructor interaction and feedback between assignments.
How is this course accessed?
This course is taught in an online Learning Management System. Students will be provided access information upon registration.
When can I order transcripts?
Once all course work is submitted, a final grade is submitted by the instructor. The grade is then approved by the office and posted. Once a final grade is posted, official transcripts can be requested — learn more about transcript requests here .
What materials do I need to purchase?
The textbook set for this course should be purchased directly from the publisher.
Curriculum covered in this article
Be sure to share this article.
- Share on Twitter
- Share on Facebook
- Share on LinkedIn
Your health care career
Improve your medical terminology, mental health, and more with 100% online courses.

- Cambridge Dictionary +Plus
Meaning of hypothesis in English
Your browser doesn't support HTML5 audio
- abstraction
- afterthought
- anthropocentrism
- anti-Darwinian
- exceptionalism
- foundation stone
- great minds think alike idiom
- non-dogmatic
- non-empirical
- non-material
- non-practical
- social Darwinism
- supersensible
- the domino theory
hypothesis | American Dictionary
Hypothesis | business english, examples of hypothesis, translations of hypothesis.
Get a quick, free translation!
Word of the Day
rescue centre
a place where animals who are ill, injured, not cared for, or badly treated can be taken and given treatment and care

Binding, nailing, and gluing: talking about fastening things together

Learn more with +Plus
- Recent and Recommended {{#preferredDictionaries}} {{name}} {{/preferredDictionaries}}
- Definitions Clear explanations of natural written and spoken English English Learner’s Dictionary Essential British English Essential American English
- Grammar and thesaurus Usage explanations of natural written and spoken English Grammar Thesaurus
- Pronunciation British and American pronunciations with audio English Pronunciation
- English–Chinese (Simplified) Chinese (Simplified)–English
- English–Chinese (Traditional) Chinese (Traditional)–English
- English–Dutch Dutch–English
- English–French French–English
- English–German German–English
- English–Indonesian Indonesian–English
- English–Italian Italian–English
- English–Japanese Japanese–English
- English–Norwegian Norwegian–English
- English–Polish Polish–English
- English–Portuguese Portuguese–English
- English–Spanish Spanish–English
- English–Swedish Swedish–English
- Dictionary +Plus Word Lists
- English Noun
- American Noun
- Business Noun
- Translations
- All translations
Add hypothesis to one of your lists below, or create a new one.
{{message}}
Something went wrong.
There was a problem sending your report.
- Dictionaries home
- American English
- Collocations
- German-English
- Grammar home
- Practical English Usage
- Learn & Practise Grammar (Beta)
- Word Lists home
- My Word Lists
- Recent additions
- Resources home
- Text Checker
Definition of hypothesis noun from the Oxford Advanced Learner's Dictionary
- to formulate/confirm a hypothesis
- a hypothesis about the function of dreams
- There is little evidence to support these hypotheses.
- formulate/advance a theory/hypothesis
- build/construct/create/develop a simple/theoretical/mathematical model
- develop/establish/provide/use a theoretical/conceptual framework
- advance/argue/develop the thesis that…
- explore an idea/a concept/a hypothesis
- make a prediction/an inference
- base a prediction/your calculations on something
- investigate/evaluate/accept/challenge/reject a theory/hypothesis/model
- design an experiment/a questionnaire/a study/a test
- do research/an experiment/an analysis
- make observations/measurements/calculations
- carry out/conduct/perform an experiment/a test/a longitudinal study/observations/clinical trials
- run an experiment/a simulation/clinical trials
- repeat an experiment/a test/an analysis
- replicate a study/the results/the findings
- observe/study/examine/investigate/assess a pattern/a process/a behaviour
- fund/support the research/project/study
- seek/provide/get/secure funding for research
- collect/gather/extract data/information
- yield data/evidence/similar findings/the same results
- analyse/examine the data/soil samples/a specimen
- consider/compare/interpret the results/findings
- fit the data/model
- confirm/support/verify a prediction/a hypothesis/the results/the findings
- prove a conjecture/hypothesis/theorem
- draw/make/reach the same conclusions
- read/review the records/literature
- describe/report an experiment/a study
- present/publish/summarize the results/findings
- present/publish/read/review/cite a paper in a scientific journal
- Her hypothesis concerns the role of electromagnetic radiation.
- Her study is based on the hypothesis that language simplification is possible.
- It is possible to make a hypothesis on the basis of this graph.
- None of the hypotheses can be rejected at this stage.
- Scientists have proposed a bold hypothesis.
- She used this data to test her hypothesis
- The hypothesis predicts that children will perform better on task A than on task B.
- The results confirmed his hypothesis on the use of modal verbs.
- These observations appear to support our working hypothesis.
- a speculative hypothesis concerning the nature of matter
- an interesting hypothesis about the development of language
- Advances in genetics seem to confirm these hypotheses.
- His hypothesis about what dreams mean provoked a lot of debate.
- Research supports the hypothesis that language skills are centred in the left side of the brain.
- The survey will be used to test the hypothesis that people who work outside the home are fitter and happier.
- This economic model is really a working hypothesis.
- speculative
- concern something
- be based on something
- predict something
- on a/the hypothesis
- hypothesis about
- hypothesis concerning
Join our community to access the latest language learning and assessment tips from Oxford University Press!
- It would be pointless to engage in hypothesis before we have the facts.
Other results
Nearby words.
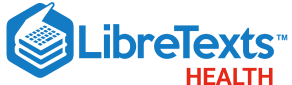
- school Campus Bookshelves
- menu_book Bookshelves
- perm_media Learning Objects
- login Login
- how_to_reg Request Instructor Account
- hub Instructor Commons
- Download Page (PDF)
- Download Full Book (PDF)
- Periodic Table
- Physics Constants
- Scientific Calculator
- Reference & Cite
- Tools expand_more
- Readability
selected template will load here
This action is not available.
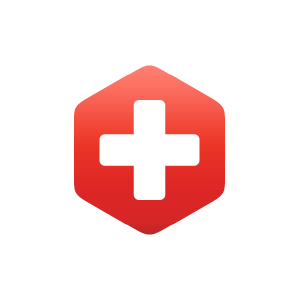
1.2: Prefixes and Suffixes
- Last updated
- Save as PDF
- Page ID 65807
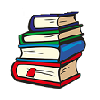
- Andrea Nelson and Katherine Greene
- University of West Florida
Learning Objectives
- Understand the difference between a prefix and a suffix.
- Differentiate prefixes that deal with body parts, color, and direction.
- Distinguish suffixes that deal with procedures.
Prefixes are located at the beginning of a medical term. The prefix alters the meaning of the medical term. It is important to spell and pronounce prefixes correctly.
Many prefixes that you find in medical terms are common to English language prefixes. A good technique to help with memorization is the following:
- Start by reviewing the most common prefixes.
- Consider common English language words that begin with the same prefixes.
- Compare them to the examples of use in medical terms.
Common Prefixes
Body part prefixes, color prefixes, physical property and shape prefixes, direction and position prefixes, quantity prefixes, concept check.
- Do you know the difference between the prefixes inter- , infra- , and intra- ?
- What color is an erythrocyte? A leukocyte?
- around something else?
- within something else?
- below something else?
Suffixes are word parts that are located at the end of words. Suffixes can alter the meaning of medical terms. It is important to spell and pronounce suffixes correctly.
Suffixes in medical terms are common to English language suffixes. Suffixes are not always explicitly stated in the definition of a word. It is common that suffixes will not be explicitly stated when defining a medical term in the workplace. However, when transcribing or reading medical reports the suffix is always clearly written. In order to properly spell and pronounce medical terms, it is helpful to learn the suffixes.
Common Suffixes
Procedure suffixes.
- Do you know the difference between the suffixes -gram , -graph , and -graphy?
- Which suffixes denote a condition or disease?
Word parts and definitions from “Appendix A: Word Parts and What They Mean” by MedlinePlus and is under public domain.
Definitions of medical term examples from:
- Anatomy and Physiology (on OpenStax ), by Betts et al. and is used under a CC BY 4.0 international license . Download and access this book for free at https://openstax.org/books/anatomy-and-physiology/pages/1-introduction
- Concepts of Biology (on OpenStax ), by Fowler et al. and is used under a CC BY 4.0 international license . Download and access this book for free at https://openstax.org/books/concepts-biology/pages/1-introduction
- NCI Dictionary of Cancer Terms by the National Cancer Institute and is used under public domain.

An official website of the United States government
The .gov means it's official. Federal government websites often end in .gov or .mil. Before sharing sensitive information, make sure you're on a federal government site.
The site is secure. The https:// ensures that you are connecting to the official website and that any information you provide is encrypted and transmitted securely.
- Publications
- Account settings
- Browse Titles
NCBI Bookshelf. A service of the National Library of Medicine, National Institutes of Health.
StatPearls [Internet]. Treasure Island (FL): StatPearls Publishing; 2024 Jan-.

StatPearls [Internet].
Beenish S. Bhutta ; Faysal Alghoula ; Ilya Berim .
Affiliations
Last Update: August 9, 2022 .
- Continuing Education Activity
Hypoxia is a state in which oxygen is not available in sufficient amounts at the tissue level to maintain adequate homeostasis; this can result from inadequate oxygen delivery to the tissues either due to low blood supply or low oxygen content in the blood (hypoxemia). Hypoxia can vary in intensity from mild to severe and can present in acute, chronic, or acute and chronic forms. This activity reviews the etiology, pathophysiology, and presentation of hypoxia and highlights the role of the interprofessional team in the management of affected patients.
- Review the various etiologies of hypoxia.
- Describe the presentation of a patient with hypoxia.
- Outline the management options available for hypoxia.
- Identify interprofessional team strategies for improving care coordination and outcomes in patients with hypoxia.
- Introduction
Hypoxia is a state in which oxygen is not available in sufficient amounts at the tissue level to maintain adequate homeostasis; this can result from inadequate oxygen delivery to the tissues either due to low blood supply or low oxygen content in the blood (hypoxemia).
Hypoxia can vary in intensity from mild to severe and can present in acute, chronic, or acute and chronic forms. The response to hypoxia is variable; while some tissues can tolerate some forms of hypoxia/ischemia for a longer duration, other tissues are severely damaged by low oxygen levels. [1] [2] [3]
There are two major causes of hypoxia at the tissue level, low blood flow to the tissue, or low oxygen content in the blood (hypoxemia). [4] [5] [6]
In order to understand the mechanism of hypoxia, we have to know that in order to have the oxygen carried by hemoglobin, direct interaction between red blood cells in pulmonary capillaries and the air in the alveoli is needed. This process can be compromised at any one of the following three points: blood flow to the lung (perfusion), airflow to the alveoli (ventilation), and the gas exchange through the interstitial tissue (diffusion).
Reduced Oxygen Tension
As in cases of high altitude.
Hypoventilation
- Airway obstruction can be proximal as in laryngeal edema or foreign body inhalation, or distal as in bronchial asthma or chronic obstructive pulmonary disease (COPD).
- Impaired respiratory drive as in cases of deep sedation or coma.
- Restricted movement of the chest wall as in obesity hypoventilation syndrome, circumferential burns, massive ascites, or ankylosing spondylitis.
- Neuromuscular diseases, such as myasthenia gravis, muscular dystrophy, amyotrophic lateral sclerosis, or phrenic nerve injuries.
Ventilation-Perfusion Mismatch (V/Q Mismatch)
- Decreased V/Q Ratio (impaired ventilation or high perfusion) such as chronic bronchitis, obstructive airway disease, mucus plugs, pulmonary edema impair the ventilation and therefore decrease the ratio of ventilation to perfusion.
- Increased V/Q Ratio (impaired perfusion): such as in cases of pulmonary embolism or increased ventilation as in emphysema (large bullae in the lungs) the surface area available for gas exchange is decreased, which causes higher ventilation in comparison to perfusion leading to a high V/Q ratio.
Right to Left Shunt
The blood crosses from the right to the left side of the heart without being oxygenated. Causes include:
- Anatomic Shunts: Blood bypasses the alveoli, e.g., intracardiac shunts (ASD, VSD, PDA), pulmonary arteriovenous malformations, fistulas, and hepato-pulmonary syndrome.
- Physiologic Shunting: Blood passes through non-ventilated alveoli, for example, pneumonia, atelectasis, and ARDS.
Impaired Diffusion of Oxygen
Oxygen diffusion is impaired between the alveolus and the pulmonary capillaries. Causes are usually interstitial edema, interstitial inflammation, or fibrosis. Clinical examples include pulmonary edema and interstitial lung disease.
- Epidemiology
Hypoxia is a common disorder that we encounter every day in the hospital. However, the causes of hypoxia are multiple, and its prevalence is variable. Some of these causes are very common like pneumonia or chronic obstructive pulmonary disease (COPD); others are quite rare like hypoxia due to reduced oxygen tension as in high altitude or due to cyanide poisoning.
- Pathophysiology
This includes the factors that decrease the percentage of oxygen in the alveoli, either due to obstruction of the airways or an increase in partial pressure of alveolar gases other than oxygen. Carbon dioxide is one example. Hypoventilation can also occur due to impaired respiratory drive as in cases of deep sedation or because of restricted movement of the chest wall as in obesity hypoventilation syndrome or ankylosing spondylitis. In this setting, the A-a gradient will be normal as the oxygen is deficient in both alveoli and the bloodstream.
In alveoli, an increase in partial pressure of one gas will be on the cost of the other gases composing the air, e.g., an increase in carbon dioxide partial pressure results in a decrease of partial pressure of oxygen, both at alveolar as well as the arterial level. This type of hypoxemia is easily corrected with supplemental oxygen.
This occurs when there is an imbalance between lung ventilation and blood flow. Even in the normal lung, there is a V/Q mismatch. In an upright individual, the V/Q ratio is higher in the apices than at the lung base. This difference is responsible for the normal A-a gradient. V/Q mismatch increases in pulmonary vascular disease, thromboembolic disease, or atelectasis to name a few. Such a process ultimately results in hypoxemia which is more difficult to correct with supplemental oxygen.
Occurs when blood passes from the right to the left side of the heart without being oxygenated. Anatomic abnormalities, such as atrial or ventricular septal defects as well as pulmonary arteriovenous malformations can cause hypoxemia that is notoriously difficult to correct with supplemental oxygen. Similar physiology is observed in hepato-pulmonary syndrome. Physiologic right-to-left shunt exists when the blood passes through non-ventilated alveoli in cases of atelectasis, pneumonia, and acute respiratory distress syndrome (ARDS).
Impaired Diffusion of Oxygen Across the Alveoli into Blood
The usual causes are interstitial edema, lung tissue inflammation, or fibrosis. Depending on the disease's extent, a moderate to a large amount of supplemental oxygen may be required to correct this type of hypoxemia. Exercise can worsen hypoxemia resulting from impaired diffusion. An increase in cardiac output with exercise results in accelerated blood flow through alveoli, reducing the time available for gas exchange. In the case of the abnormal pulmonary interstitium, gas exchange time becomes insufficient, and hypoxemia ensues.
- History and Physical
The presentation of hypoxia can be acute or chronic; acutely the hypoxia may present with dyspnea and tachypnea. Symptom severity usually depends on the severity of hypoxia. Sufficiently severe hypoxia can result in tachycardia to provide sufficient oxygen to the tissues. Some of the signs are very evident on physical exam; stridor can be heard once the patient arrives in cases of upper airway obstruction. Skin can be cyanotic, which might indicate severe hypoxia.
When oxygen delivery is severely compromised, organ function will start to deteriorate. Neurologic manifestations include restlessness, headache, and confusion with moderate hypoxia. In severe cases, altered mentation and coma can occur, and if not corrected quickly may lead to death.
The chronic presentation is usually less dramatic, with dyspnea on exertion as the most common complaint. Symptoms of the underlying condition that induced the hypoxia can help in narrowing the differential diagnosis. For instance, productive cough and fever will be seen in cases of lung infection, leg edema, and orthopnea in cases of heart failure, and chest pain and unilateral leg swelling may point to pulmonary embolism as a cause of hypoxia.
The physical exam may show tachycardia, tachypnea, and low oxygen saturation. Fever may point to infection as the cause of hypoxia.
Lung auscultation can yield a lot of useful information. Bilateral basilar crackles may indicate pulmonary edema or volume overload, other signs of that includes jugular venous distention and lower limb edema. Wheezing and rhonchi can be found in obstructive lung disease. Absent unilateral air entry can be caused by either massive pleural effusion or pneumothorax. Chest percussion can help differentiate the two and will reveal dullness in cases of pleural effusion and hyper-resonance in cases of pneumothorax. Clear lung fields in a setting of hypoxia should raise suspicion of pulmonary embolism, especially if the patient is tachycardic and has evidence of deep vein thrombosis (DVT).
Evaluation of Acute Hypoxia
Pulse Oximetry to Evaluate Arterial Oxygen Saturation (SaO2)
The arterial oxygen saturation (SaO2) refers to the amount of oxygen bound to hemoglobin in arterial blood. The measurement is given as a percentage. Resting SaO2 less than or equal to 95% or exercise desaturation greater than or equal to 5% is considered abnormal. However, clinical correlation is always necessary as the exact cutoff below which tissue hypoxia ensues has not been defined. [7] [8] [9]
Arterial Blood Gas
It is a useful tool to evaluate hypoxemia. Aside from the diagnosis of hypoxemia, additional information obtained, such as PCO2, can shed light on the etiology of the process.
- Arterial oxygen tension (PaO2): Partial pressure of oxygen is the amount of oxygen dissolved in the plasma. A PaO2 less than 80 mmHg is considered abnormal. However, this should be in line with the clinical situation.
- The partial pressure of CO2: It is an indirect measure of exchange of CO2 with the air via the alveoli, its level is related to minute ventilation. PCO2 is elevated in hypoventilation like in obesity hypoventilation, deep sedation, or maybe in the setting of acute hypoxia secondary to tachypnea and washout of CO2.
PaO2:FiO2 ratio (Normal ratio is 300 to 500), if this ratio drops this may indicate a deterioration in gas exchange, this is particularly important in defining ARDS.
Imaging studies of the chest, such as chest x-rays or CT help in identifying the cause of the hypoxia, e.g., pneumonia, pulmonary edema, hyperinflated lungs in COPD, and other conditions. CT chest can give more detailed images that outline the exact pathology, CT angiogram of the chest is of particular importance in detecting the pulmonary embolism. Another modality is the VQ scan which can detect the ventilation-perfusion mismatch, which is helpful in diagnostics of acute or chronic pulmonary embolism. VQ scan can be particularly useful when renal failure or allergy to iodinated contrast increases the risks of CT angiography.
The first step in evaluating the hypoxia is to calculate the A-a gradient of oxygen. This is the difference in the amount of oxygen between the Alveoli “A” and the amount of oxygen in the blood “a." In other terms, the A-a oxygen gradient = PAO2 - PaO2.
PaO2 can be obtained from the arterial blood gas; however, PAO2 is calculated using the alveolar gas equation:
- PAO2 = (FiO2 x [760-47]) - PaCO2/0.8)
760 is the atmospheric pressure at the sea level in mmHg, 47 is the partial pressure of water at a temperature of 37 C, and 0.8 is the steady-state respiratory quotient.
The A-a gradient changes with age, and thus it is corrected for age using this equation; A-a gradient = (age/4+4).
If the A-a gradient is normal, then the cause of hypoxia is low oxygen content in the alveoli, either due to low O2 content in the air (low FiO2, as in the high altitude) or more commonly due to hypoventilation like the central nervous system (CNS) depression, OHS, or obstructed airways as in COPD exacerbation.
If the gradient is height then the cause of hypoxia is either due to a diffusion defect or perfusion defect (VQ mismatch), an alternative explanation is shunting of blood flow around the alveolar circulation, administering 1.0 FiO2 may help differentiate the two, as the oxygenation will improve in VQ mismatch in contrast to cases where shunt physiology is present.
PaO2:FiO2 Ratio
This ratio is another way to measure the degree of hypoxia. A normal PaO2/FiO2 ratio is about 300 to 500 mmHg. The ratio of less than 300 indicates abnormal gas exchange, and values less than 200 mmHg indicate severe hypoxemia. The PaO2/FiO2 ratio is used mostly as a definition of acute respiratory distress syndrome severity.
Evaluation of Chronic Hypoxia
Pulmonary Function Test (PFT)
PFT provides a direct measure of the lung volumes, bronchodilator response, and diffusion capacity, which can help in establishing the diagnosis and guiding the treatment of lung disorders. Aiding the history and physical exam, PFTs can be used to differentiate between the obstructive (bronchial asthma, COPD, upper airway obstruction) versus restrictive lung diseases (interstitial lung diseases, chest wall abnormalities). PFTs play a role in the assessment of airway obstruction severity as well as a response to therapy. One has to keep in mind that PFTs are effort-dependent and require the patient's ability to cooperate and understand instructions.
Nocturnal (overnight) Trend Oximetry
It provides information about oxyhemoglobin saturation over a period (usually overnight). This test is primarily used to assess adequacy or need for oxygen supplementation at night. Use of overnight trend oximetry as a surrogate for a diagnostic sleep study is possible, however, is discouraged. A formal sleep study should be used whenever possible.
Six-Minute Walk Test
This test provides information on oxyhemoglobin saturation response to exercise as well as the total distance a patient can walk in 6 minutes on a ground level. This information can be used to titrate oxygen supplementation as well as evaluate the response to therapy. The 6-minutes walk test is frequently used in the preoperative pulmonary evaluation, pulmonary hypertension treatment and assessment of supplemental oxygen need with exercise.
Secondary polycythemia can be an indicator of chronic hypoxia.
- Treatment / Management
Management of hypoxia falls under 3 categories: maintaining patent airways, increasing the oxygen content of the inspired air, and improving the diffusion capacity. [10] [11] [12]
Maintaining Patent Airways
Ensure patency of the upper airways with good suctioning, maneuvers that prevent occlusion of the throat (head tilt and jaw thrust if necessary), sometimes the placement of an endotracheal tube or tracheostomy is necessary.
In chronic conditions like obesity hyperventilation syndrome, maintaining patent airways can be achieved with positive pressure ventilation like CPAP or BiPAP.
Bronchodilators and aggressive pulmonary hygiene, such as chest physiotherapy, the flutter valve, and incentive spirometry can be used to maintain the patency of the lower airways.
Increase Fraction of the Inspired O2 (FiO2)
This is indicated for low PaO2 less than 60 or SaO2 less than 90, and this can be achieved by increasing the percentage of oxygen in the inspired air that reaches the alveoli.
Low-Flow Devices
- Nasal Cannula
- Use: mild hypoxia (with FiO2 approximately 92%)
- Flow rate: up to 6 L per minute
- FiO2 delivered: up to 45% (0.45)
- Advantage: Easy to use and more convenient to the patient (can be used during eating, drinking, talking)
- Disadvantage: Dry nasal mucosa (humidify if the flow is greater than or equal to 4 L per minute), FiO2 being delivered varies greatly. Mouth breathers derive less benefit from using a nasal cannula.
- The following formula can be used to approximate the percentage of FiO2; FiO2 = 20% + (4 times oxygen flow liters) For example, oxygen flow 2L/min would deliver approximately FiO2 of 0.3, 6 L per minute would deliver approximately FiO2 of 0.45 (more commonly known as 45%).
- Simple Face Mask
- Use: Moderate to severe hypoxia, initial treatment
- Flow rate: up to 10 L per minute
- FiO2 delivered: 35% to 50%
- Advantage: provides higher FiO2, no pressures involved, well tolerated by patients
- Disadvantage: Dry oral mucosa (needs humidification), the flow must be at least 5 L per minute to flush CO2, not high flow. Also, the mask itself can interfere with activities of daily living.
- Reservoir Cannulas (Oxymizer)
- The device uses a reservoir space, which stores O2 during expiration, making it available as a bolus during the next inspiration. This way the patient gets a higher oxygen delivery without increasing flow.
- Flow rate: up to 16 L per minute.
- FiO2 = up to 90% (0.9)
- Reservoir cannulas are available as mustache configuration (Oxymizer), where the reservoir is located directly beneath the nose, pendant configuration (Oxymizer Pendant) which is connected to a plastic reservoir on the anterior chest
- Partial-rebreather Mask
- Has a 300 to 500 mL reservoir bag and 2 one-way valves to prevent exhaling into the reservoir
- Flow rate: 6 to 10 L per minute (flow must be sufficient to keep reservoir bag from collapse during inspiration)
- FiO2 delivered: 50% to 70%
- Advantage: Higher FiO2 can be delivered
- Disadvantage: Interferes with activities of daily living
- Non-rebreather Mask
- Has a 300 to 500 mL reservoir bag and 2 one-way valves
- Use: Moderate to severe acute hypoxia, initial treatment
- Flow rate: 10 to 15 (at least 10 L per minute to avoid bag collapse during inspiration)
- FiO2 delivered: 85% to 90%
- Advantage: even higher FiO2 can be achieved
- Disadvantage: Interferes with activities of daily living
High-Flow Devices
Usually, this requires an oxygen blender, humidifier, and heated tubing.
- Venturi Mask
- Mask attached an air entrainment valve
- The flow rate and FiO2: (depends on the color). (Blue = 2 to 4 L per minute = 24% O2, White = 4 to 6 L per minute = 28% O2, Yellow = 8 to 10 L per minute = 35% O2, Red = 10 to 12 L per minute = 40% O2, Green = 12 to 15 L per minute = 60% O2)
- Advantage: provides the most accurate O2 delivery, high flow
- Disadvantage: need to be removed for eating. Less accurate at high flow rates
- Does not guarantee the total flow with O2 percentages above 35% in patients with high inspiratory flow demands; the problem with air entrainment systems is that as this is increased, the air to oxygen ratio decreases
- High-flow Nasal Cannula
- High-flow oxygen (HFO) consists of a heated, humidified O2
- Flow rate: 10 to 60 L per minute
- FiO2 delivered: Up to 100%
- Advantages: More convenient, Can deliver up to 100% heated and humidified oxygen at a maximum flow of 60 L
- Disadvantages: Fairly large cannula, can be a source of (although usually rather minimal) discomfort
- Air/oxygen Blender
- Provides accurate oxygen delivery independent of the patient’s inspiratory flow demands
- Positive end-expiratory pressure may be generated
- For approximately every 10 liters of flow delivered, about 1 cm/HO of positive pressure is obtained
Positive Pressure Ventilation
It allows for accurate delivery of any necessary FiO2 and includes the following:
Non-Invasive Ventilation
It is usually used as the last resort to avoid the intubation
- Continuous Positive Airways Pressure Mask (CPAP)
- Mainly used in patients with obstructive sleep apnea or in acute pulmonary edema.
- Delivers oxygen (or air) under pre-determined high pressure via a tightly fitting face mask.
- Positive pressure is continuous, to ensure that the airways are open (split them).
- Bilevel Positive Airways Pressure (BiPAP)
- Mainly used in patients with acute Hypercarbia as in patients with COPD exacerbation and ARDS patients.
- High positive pressure on inspiration and lower positive pressure on expiration.
- Pressure delivery is variable throughout the respiratory cycle, with high positive pressure on inspiration and lower positive pressure on expiration.
Invasive Ventilation
- Positive pressure ventilator attached to (usually) endotracheal tube.
- Allows for accurate delivery of predetermined minute ventilation as well as accurate FiO2 and positive end-expiratory pressure.
- Can be used electively during surgery.
Improve the Diffusion of Oxygen through the Alveolar Interstitial Tissue
The overall idea s to treat the underlying cause of respiratory failure:
- Diuretics can be used in cases of pulmonary edema.
- Steroids in certain cases of interstitial lung disease.
- Extracorporeal membrane oxygenation (ECMO) can be used as an ultimate method of increasing oxygenation.
- Differential Diagnosis
Hypoxemic Hypoxia
Low oxygen tension in the arterial blood (PaO2) is due to the inability of the lungs to properly oxygenate the blood. Causes include hypoventilation, impaired alveolar diffusion, and pulmonary shunting .
Circulatory Hypoxia
It is due to pump failure (heart is unable to pump enough blood, and therefore oxygen delivery is impaired).
Anemic Hypoxia
It is because of a decrease in oxygen-carrying capacity due to low hemoglobin leading to inadequate oxygen delivery.
Histotoxic Hypoxia (Dysoxia)
Cells are unable to utilize oxygen effectively, the best example of this is Cyanide poisoning which inhibits the enzyme cytochrome C oxidase in the mitochondria, blocking the use of oxygen to make ATP.
- Pearls and Other Issues
- The characteristics of each category of hypoxemia are as follows:
- Hypoventilation presents with an elevated PaCO2 with a normal A-a gradient.
- Low-inspired oxygen presents with a normal PaC02 plus normal A-a gradient.
- Shunting presents with a normal PaC02 and elevated A-a gradient that does not correct with the administration of 100% oxygen.
- V/Q mismatch presents with a normal PaC02 and elevated A-a gradient that does correctly with 100% oxygen.
- Oxygen supplementation varies between FiO2 of 0.21 and 1.00. A variety of low and high flow devices exist to facilitate this process, each with unique advantages and disadvantages.
- The delivery of oxygen depends on two variables:
- There are several devices designed to deliver oxygen at different rates and concentrations as described above.
- Oxygen toxicity may result if oxygen is delivered at a higher concentration for a long duration of time.
- Decreased body temperature decreases metabolic rate, which lowers oxygen consumption and minimizes the adverse effects of tissue hypoxia (especially brain) Therapeutic hypothermia is based on this principle.
- Long-term oxygen therapy can reduce mortality, and it is indicated in these patient populations:
- Group 1 (Absolute): PaO2 55 mm Hg or SaO2 88%
- Group 2 (In the presence of cor pulmonale): PaO2 55 to 59 mm Hg or SaO2 89%, ECG evidence of right atrial enlargement, hematocrit greater than 55%, congestive heart failure
- Enhancing Healthcare Team Outcomes
Hypoxia is low oxygen content at the tissue level to meet the metabolic needs of the cells. The condition can occur in a patient for a variety of reasons and the interprofessional team must be aware of the workup of such a patient.
Classic causes of hypoxia include hypoventilation, ventilation-perfusion mismatch, the low oxygen content in the air, right to left shunting, or impaired diffusion. By analyzing the arterial blood gas (ABG), calculating the alveolar-arterial oxygen gradient (A-a gradient), and determining whether administration of 100% oxygen leads to improvement, one can determine the specific type of hypoxemia plaguing a particular patient. Leaving hypoxia untreated for prolonged periods leads to permanent organ injury including death.
- Review Questions
- Access free multiple choice questions on this topic.
- Comment on this article.
Disclosure: Beenish Bhutta declares no relevant financial relationships with ineligible companies.
Disclosure: Faysal Alghoula declares no relevant financial relationships with ineligible companies.
Disclosure: Ilya Berim declares no relevant financial relationships with ineligible companies.
This book is distributed under the terms of the Creative Commons Attribution-NonCommercial-NoDerivatives 4.0 International (CC BY-NC-ND 4.0) ( http://creativecommons.org/licenses/by-nc-nd/4.0/ ), which permits others to distribute the work, provided that the article is not altered or used commercially. You are not required to obtain permission to distribute this article, provided that you credit the author and journal.
- Cite this Page Bhutta BS, Alghoula F, Berim I. Hypoxia. [Updated 2022 Aug 9]. In: StatPearls [Internet]. Treasure Island (FL): StatPearls Publishing; 2024 Jan-.
In this Page
Bulk download.
- Bulk download StatPearls data from FTP
Related information
- PMC PubMed Central citations
- PubMed Links to PubMed
Similar articles in PubMed
- Review Tissue oxygenation in low flow states and during hypoxemia. [Crit Care Med. 1993] Review Tissue oxygenation in low flow states and during hypoxemia. Grum CM. Crit Care Med. 1993 Feb; 21(2 Suppl):S44-9.
- [The impact of early hypoxemia on the prognosis of patients undergone general anesthesia patients in intensive care unit]. [Zhonghua Wei Zhong Bing Ji Jiu...] [The impact of early hypoxemia on the prognosis of patients undergone general anesthesia patients in intensive care unit]. Wang SP, Li M, Yi L, Huang X, Duan J, Song HM, Li G. Zhonghua Wei Zhong Bing Ji Jiu Yi Xue. 2013 Oct; 25(10):600-3.
- Review [What degree of hypoxemia is tolerable for human beings?]. [Dtsch Med Wochenschr. 2010] Review [What degree of hypoxemia is tolerable for human beings?]. Köhler D. Dtsch Med Wochenschr. 2010 Mar; 135(10):474-7. Epub 2010 Mar 2.
- Analysis of oxygen delivery and uptake relationships in the Krogh tissue model. [J Appl Physiol (1985). 1989] Analysis of oxygen delivery and uptake relationships in the Krogh tissue model. Schumacker PT, Samsel RW. J Appl Physiol (1985). 1989 Sep; 67(3):1234-44.
- Factors that render the kidney susceptible to tissue hypoxia in hypoxemia. [Am J Physiol Regul Integr Comp...] Factors that render the kidney susceptible to tissue hypoxia in hypoxemia. Evans RG, Goddard D, Eppel GA, O'Connor PM. Am J Physiol Regul Integr Comp Physiol. 2011 Apr; 300(4):R931-40. Epub 2011 Jan 19.
Recent Activity
- Hypoxia - StatPearls Hypoxia - StatPearls
Your browsing activity is empty.
Activity recording is turned off.
Turn recording back on
Connect with NLM
National Library of Medicine 8600 Rockville Pike Bethesda, MD 20894
Web Policies FOIA HHS Vulnerability Disclosure
Help Accessibility Careers
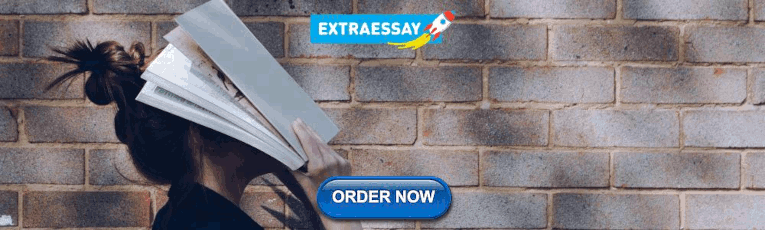
IMAGES
VIDEO
COMMENTS
The present paper attempts to put the P value in proper perspective by explaining different types of probabilities, their role in clinical decision making, medical research and hypothesis testing. Keywords: Hypothesis testing, P value, Probability. The clinician who wishes to remain abreast with the results of medical research needs to develop ...
HYPOTHESIS TESTING. A clinical trial begins with an assumption or belief, and then proceeds to either prove or disprove this assumption. In statistical terms, this belief or assumption is known as a hypothesis. Counterintuitively, what the researcher believes in (or is trying to prove) is called the "alternate" hypothesis, and the opposite ...
A hypothesis is a tentative statement about the relationship between two or more variables. It is a specific, testable prediction about what you expect to happen in a study. It is a preliminary answer to your question that helps guide the research process. Consider a study designed to examine the relationship between sleep deprivation and test ...
A research hypothesis (also called a scientific hypothesis) is a statement about the expected outcome of a study (for example, a dissertation or thesis). To constitute a quality hypothesis, the statement needs to have three attributes - specificity, clarity and testability. Let's take a look at these more closely.
The term differential diagnosis refers to evaluating more than one diagnostic hypothesis that may be entertained in the process of making a diagnosis . This process may involve taking a medical history, performing a physical examination, collecting laboratory and imaging data, and performing other tests like electrocardiograms, among other things.
2. The scientific hypothesis. In this section, we will describe a functional and descriptive role regarding how scientists use hypotheses. Jeong & Kwon [] investigated and summarized the different uses the concept of 'hypothesis' had in philosophical and scientific texts.They identified five meanings: assumption, tentative explanation, tentative cause, tentative law, and prediction.
The term hypothesis means "little theory". A hypothesis is a short statement that can be tested and gives a possible reason for a phenomenon or a possible link between two variables . In the setting of scientific research, a hypothesis is a tentative explanation or statement that can be proven wrong and is used to guide experiments and ...
Background In medical practice, diagnostic hypotheses are often made by physicians in the first moments of contact with patients; sometimes even before they report their symptoms. We propose that generation of diagnostic hypotheses in this context is the result of cognitive processes subserved by brain mechanisms that are similar to those involved in naming objects or concepts in everyday life.
A hypothesis states your predictions about what your research will find. It is a tentative answer to your research question that has not yet been tested. For some research projects, you might have to write several hypotheses that address different aspects of your research question. A hypothesis is not just a guess. It should be based on ...
Medical Hypotheses is a forum for ideas in medicine and related biomedical sciences. It will publish interesting and important theoretical papers that foster the diversity and debate upon which the scientific process thrives. The Aims and Scope of Medical Hypotheses are no different now from what was proposed by the founder of the journal, the ...
Medical Terminology 101: Definitions, Applications & Helpful Resources. Understanding medical terminology can make all the difference in critical health situations. And while understanding medical language is serious business for care providers, learning medical terminology can actually be a thought-provoking, interesting crash course in the ...
hypothesis. (hī-pŏth′ĭ-sĭs) n. pl. hypothe·ses (-sēz′) 1. A tentative explanation for an observation, phenomenon, or scientific problem that can be tested by further investigation. 2. Something taken to be true for the purpose of argument or investigation; an assumption. 3. The antecedent of a conditional statement.
Glossary of Common Research Terms Term Definition Abstract This is a brief summary of a research study and its results. It should tell you why the study was done, how the researchers went about it and what they found. Action Research Action research is used to bring about improvement or practical change. A group of people who know about a
A hypothesis test is a procedure used in statistics to assess whether a particular viewpoint is likely to be true. They follow a strict protocol, and they generate a 'p-value', on the basis of which a decision is made about the truth of the hypothesis under investigation.All of the routine statistical 'tests' used in research—t-tests, χ 2 tests, Mann-Whitney tests, etc.—are all ...
hypothesis definition: 1. an idea or explanation for something that is based on known facts but has not yet been proved…. Learn more.
A well-defined group of people who have had a common experience or exposure and are then followed up, as in a cohort study or prospective study, to determine the incidence of new diseases or health conditions. Cohort, birth. A group of people born during a particular period or year. Cohort study. See Study, cohort.
1 [countable] an idea or explanation of something that is based on a few known facts but that has not yet been proved to be true or correct synonym theory to formulate/confirm a hypothesis a hypothesis about the function of dreams There is little evidence to support these hypotheses. Topic Collocations Scientific Research theory. formulate/advance a theory/hypothesis
The hypothesis predicts that children will perform better on task A than on task B. The results confirmed his hypothesis on the use of modal verbs. These observations appear to support our working hypothesis. a speculative hypothesis concerning the nature of matter; an interesting hypothesis about the development of language
Suffixes. Suffixes are word parts that are located at the end of words. Suffixes can alter the meaning of medical terms. It is important to spell and pronounce suffixes correctly. Suffixes in medical terms are common to English language suffixes. Suffixes are not always explicitly stated in the definition of a word.
hypothesis. (hī-pŏth′ĭ-sĭs) n. pl. hypothe·ses (-sēz′) 1. A tentative explanation for an observation, phenomenon, or scientific problem that can be tested by further investigation. 2. Something taken to be true for the purpose of argument or investigation; an assumption. 3. The antecedent of a conditional statement.
This chapter provides an overview of diagnosis in health care, including the committee's conceptual model of the diagnostic process and a review of clinical reasoning. Diagnosis has important implications for patient care, research, and policy. Diagnosis has been described as both a process and a classification scheme, or a "pre-existing set of categories agreed upon by the medical ...
hypothesis. (hī-pŏth′ĭ-sĭs) n. pl. hypothe·ses (-sēz′) 1. A tentative explanation for an observation, phenomenon, or scientific problem that can be tested by further investigation. 2. Something taken to be true for the purpose of argument or investigation; an assumption. 3. The antecedent of a conditional statement.
Hypoxia is a state in which oxygen is not available in sufficient amounts at the tissue level to maintain adequate homeostasis; this can result from inadequate oxygen delivery to the tissues either due to low blood supply or low oxygen content in the blood (hypoxemia). Hypoxia can vary in intensity from mild to severe and can present in acute ...