Root out friction in every digital experience, super-charge conversion rates, and optimize digital self-service
Uncover insights from any interaction, deliver AI-powered agent coaching, and reduce cost to serve
Increase revenue and loyalty with real-time insights and recommendations delivered to teams on the ground
Know how your people feel and empower managers to improve employee engagement, productivity, and retention
Take action in the moments that matter most along the employee journey and drive bottom line growth
Whatever they’re are saying, wherever they’re saying it, know exactly what’s going on with your people
Get faster, richer insights with qual and quant tools that make powerful market research available to everyone
Run concept tests, pricing studies, prototyping + more with fast, powerful studies designed by UX research experts
Track your brand performance 24/7 and act quickly to respond to opportunities and challenges in your market
Explore the platform powering Experience Management
- Free Account
- For Digital
- For Customer Care
- For Human Resources
- For Researchers
- Financial Services
- All Industries
Popular Use Cases
- Customer Experience
- Employee Experience
- Employee Exit Interviews
- Net Promoter Score
- Voice of Customer
- Customer Success Hub
- Product Documentation
- Training & Certification
- XM Institute
- Popular Resources
- Customer Stories
Market Research
- Artificial Intelligence
- Partnerships
- Marketplace
The annual gathering of the experience leaders at the world’s iconic brands building breakthrough business results, live in Salt Lake City.
- English/AU & NZ
- Español/Europa
- Español/América Latina
- Português Brasileiro
- REQUEST DEMO
- Experience Management
- Descriptive Statistics
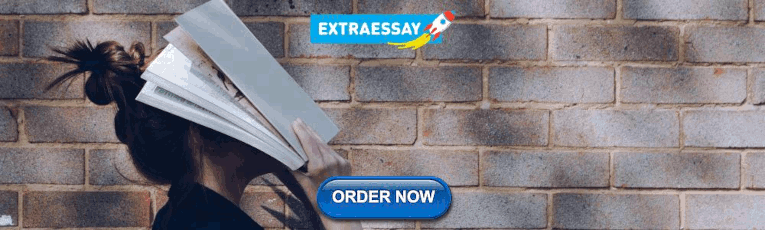
Try Qualtrics for free
Descriptive statistics in research: a critical component of data analysis.
15 min read With any data, the object is to describe the population at large, but what does that mean and what processes, methods and measures are used to uncover insights from that data? In this short guide, we explore descriptive statistics and how it’s applied to research.
What do we mean by descriptive statistics?
With any kind of data, the main objective is to describe a population at large — and using descriptive statistics, researchers can quantify and describe the basic characteristics of a given data set.
For example, researchers can condense large data sets, which may contain thousands of individual data points or observations, into a series of statistics that provide useful information on the population of interest. We call this process “describing data”.
In the process of producing summaries of the sample, we use measures like mean, median, variance, graphs, charts, frequencies, histograms, box and whisker plots, and percentages. For datasets with just one variable, we use univariate descriptive statistics. For datasets with multiple variables, we use bivariate correlation and multivariate descriptive statistics.
Want to find out the definitions?
Univariate descriptive statistics: this is when you want to describe data with only one characteristic or attribute
Bivariate correlation: this is when you simultaneously analyze (compare) two variables to see if there is a relationship between them
Multivariate descriptive statistics: this is a subdivision of statistics encompassing the simultaneous observation and analysis of more than one outcome variable
Then, after describing and summarizing the data, as well as using simple graphical analyses, we can start to draw meaningful insights from it to help guide specific strategies. It’s also important to note that descriptive statistics can employ and use both quantitative and qualitative research .
Describing data is undoubtedly the most critical first step in research as it enables the subsequent organization, simplification and summarization of information — and every survey question and population has summary statistics. Let’s take a look at a few examples.
Examples of descriptive statistics
Consider for a moment a number used to summarize how well a striker is performing in football — goals scored per game. This number is simply the number of shots taken against how many of those shots hit the back of the net (reported to three significant digits). If a striker is scoring 0.333, that’s one goal for every three shots. If they’re scoring one in four, that’s 0.250.
A classic example is a student’s grade point average (GPA). This single number describes the general performance of a student across a range of course experiences and classes. It doesn’t tell us anything about the difficulty of the courses the student is taking, or what those courses are, but it does provide a summary that enables a degree of comparison with people or other units of data.
Ultimately, descriptive statistics make it incredibly easy for people to understand complex (or data intensive) quantitative or qualitative insights across large data sets.
Take your research to the next level with XM for Strategy & Research
Types of descriptive statistics
To quantitatively summarize the characteristics of raw, ungrouped data, we use the following types of descriptive statistics:
- Measures of Central Tendency ,
- Measures of Dispersion and
- Measures of Frequency Distribution.
Following the application of any of these approaches, the raw data then becomes ‘grouped’ data that’s logically organized and easy to understand. To visually represent the data, we then use graphs, charts, tables etc.
Let’s look at the different types of measurement and the statistical methods that belong to each:
Measures of Central Tendency are used to describe data by determining a single representative of central value. For example, the mean, median or mode.
Measures of Dispersion are used to determine how spread out a data distribution is with respect to the central value, e.g. the mean, median or mode. For example, while central tendency gives the person the average or central value, it doesn’t describe how the data is distributed within the set.
Measures of Frequency Distribution are used to describe the occurrence of data within the data set (count).
The methods of each measure are summarized in the table below:
Mean: The most popular and well-known measure of central tendency. The mean is equal to the sum of all the values in the data set divided by the number of values in the data set.
Median: The median is the middle score for a set of data that has been arranged in order of magnitude. If you have an even number of data, e.g. 10 data points, take the two middle scores and average the result.
Mode: The mode is the most frequently occurring observation in the data set.
Range: The difference between the highest and lowest value.
Standard deviation: Standard deviation measures the dispersion of a data set relative to its mean and is calculated as the square root of the variance.
Quartile deviation : Quartile deviation measures the deviation in the middle of the data.
Variance: Variance measures the variability from the average of mean.
Absolute deviation: The absolute deviation of a dataset is the average distance between each data point and the mean.
Count: How often each value occurs.
Scope of descriptive statistics in research
Descriptive statistics (or analysis) is considered more vast than other quantitative and qualitative methods as it provides a much broader picture of an event, phenomenon or population.
But that’s not all: it can use any number of variables, and as it collects data and describes it as it is, it’s also far more representative of the world as it exists.
However, it’s also important to consider that descriptive analyses lay the foundation for further methods of study. By summarizing and condensing the data into easily understandable segments, researchers can further analyze the data to uncover new variables or hypotheses.
Mostly, this practice is all about the ease of data visualization. With data presented in a meaningful way, researchers have a simplified interpretation of the data set in question. That said, while descriptive statistics helps to summarize information, it only provides a general view of the variables in question.
It is, therefore, up to the researchers to probe further and use other methods of analysis to discover deeper insights.
Things you can do with descriptive statistics
Define subject characteristics
If a marketing team wanted to build out accurate buyer personas for specific products and industry verticals, they could use descriptive analyses on customer datasets (procured via a survey) to identify consistent traits and behaviors.
They could then ‘describe’ the data to build a clear picture and understanding of who their buyers are, including things like preferences, business challenges, income and so on.
Measure data trends
Let’s say you wanted to assess propensity to buy over several months or years for a specific target market and product. With descriptive statistics, you could quickly summarize the data and extract the precise data points you need to understand the trends in product purchase behavior.
Compare events, populations or phenomena
How do different demographics respond to certain variables? For example, you might want to run a customer study to see how buyers in different job functions respond to new product features or price changes. Are all groups as enthusiastic about the new features and likely to buy? Or do they have reservations? This kind of data will help inform your overall product strategy and potentially how you tier solutions.
Validate existing conditions
When you have a belief or hypothesis but need to prove it, you can use descriptive techniques to ascertain underlying patterns or assumptions.
Form new hypotheses
With the data presented and surmised in a way that everyone can understand (and infer connections from), you can delve deeper into specific data points to uncover deeper and more meaningful insights — or run more comprehensive research.
Guiding your survey design to improve the data collected
To use your surveys as an effective tool for customer engagement and understanding, every survey goal and item should answer one simple, yet highly important question:
What am I really asking?
It might seem trivial, but by having this question frame survey research, it becomes significantly easier for researchers to develop the right questions that uncover useful, meaningful and actionable insights.
Planning becomes easier, questions clearer and perspective far wider and yet nuanced.
Hypothesize – what’s the problem that you’re trying to solve? Far too often, organizations collect data without understanding what they’re asking, and why they’re asking it.
Finally, focus on the end result. What kind of data do you need to answer your question? Also, are you asking a quantitative or qualitative question? Here are a few things to consider:
- Clear questions are clear for everyone. It takes time to make a concept clear
- Ask about measurable, evident and noticeable activities or behaviors.
- Make rating scales easy. Avoid long lists, confusing scales or “don’t know” or “not applicable” options.
- Ensure your survey makes sense and flows well. Reduce the cognitive load on respondents by making it easy for them to complete the survey.
- Read your questions aloud to see how they sound.
- Pretest by asking a few uninvolved individuals to answer.
Furthermore…
As well as understanding what you’re really asking, there are several other considerations for your data:
Keep it random
How you select your sample is what makes your research replicable and meaningful. Having a truly random sample helps prevent bias, increasingly the quality of evidence you find.
Plan for and avoid sample error
Before starting your research project, have a clear plan for avoiding sample error. Use larger sample sizes, and apply random sampling to minimize the potential for bias.
Don’t over sample
Remember, you can sample 500 respondents selected randomly from a population and they will closely reflect the actual population 95% of the time.
Think about the mode
Match your survey methods to the sample you select. For example, how do your current customers prefer communicating? Do they have any shared characteristics or preferences? A mixed-method approach is critical if you want to drive action across different customer segments.
Use a survey tool that supports you with the whole process
Surveys created using a survey research software can support researchers in a number of ways:
- Employee satisfaction survey template
- Employee exit survey template
- Customer satisfaction (CSAT) survey template
- Ad testing survey template
- Brand awareness survey template
- Product pricing survey template
- Product research survey template
- Employee engagement survey template
- Customer service survey template
- NPS survey template
- Product package testing survey template
- Product features prioritization survey template
These considerations have been included in Qualtrics’ survey software , which summarizes and creates visualizations of data, making it easy to access insights, measure trends, and examine results without complexity or jumping between systems.
Uncover your next breakthrough idea with Stats iQ™
What makes Qualtrics so different from other survey providers is that it is built in consultation with trained research professionals and includes high-tech statistical software like Qualtrics Stats iQ .
With just a click, the software can run specific analyses or automate statistical testing and data visualization. Testing parameters are automatically chosen based on how your data is structured (e.g. categorical data will run a statistical test like Chi-squared), and the results are translated into plain language that anyone can understand and put into action.
Get more meaningful insights from your data
Stats iQ includes a variety of statistical analyses, including: describe, relate, regression, cluster, factor, TURF, and pivot tables — all in one place!
Confidently analyze complex data
Built-in artificial intelligence and advanced algorithms automatically choose and apply the right statistical analyses and return the insights in plain english so everyone can take action.
Integrate existing statistical workflows
For more experienced stats users, built-in R code templates allow you to run even more sophisticated analyses by adding R code snippets directly in your survey analysis.
Advanced statistical analysis methods available in Stats iQ
Regression analysis – Measures the degree of influence of independent variables on a dependent variable (the relationship between two or multiple variables).
Analysis of Variance (ANOVA) test – Commonly used with a regression study to find out what effect independent variables have on the dependent variable. It can compare multiple groups simultaneously to see if there is a relationship between them.
Conjoint analysis – Asks people to make trade-offs when making decisions, then analyses the results to give the most popular outcome. Helps you understand why people make the complex choices they do.
T-Test – Helps you compare whether two data groups have different mean values and allows the user to interpret whether differences are meaningful or merely coincidental.
Crosstab analysis – Used in quantitative market research to analyze categorical data – that is, variables that are different and mutually exclusive, and allows you to compare the relationship between two variables in contingency tables.
Go from insights to action
Now that you have a better understanding of descriptive statistics in research and how you can leverage statistical analysis methods correctly, now’s the time to utilize a tool that can take your research and subsequent analysis to the next level.
Try out a Qualtrics survey software demo so you can see how it can take you through descriptive research and further research projects from start to finish.
Take your research to the next level with XM for Strategy & Research
Related resources
Market intelligence 10 min read, marketing insights 11 min read, ethnographic research 11 min read, qualitative vs quantitative research 13 min read, qualitative research questions 11 min read, qualitative research design 12 min read, primary vs secondary research 14 min read, request demo.
Ready to learn more about Qualtrics?
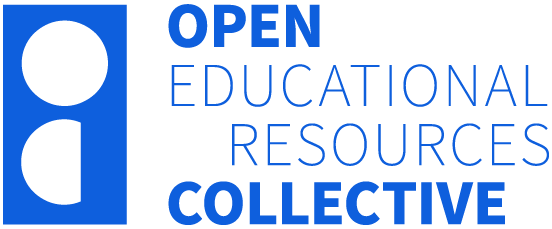
Want to create or adapt books like this? Learn more about how Pressbooks supports open publishing practices.
Chapter 5: Qualitative descriptive research
Darshini Ayton
Learning outcomes
Upon completion of this chapter, you should be able to:
- Identify the key terms and concepts used in qualitative descriptive research.
- Discuss the advantages and disadvantages of qualitative descriptive research.
What is a qualitative descriptive study?
The key concept of the qualitative descriptive study is description.
Qualitative descriptive studies (also known as ‘exploratory studies’ and ‘qualitative description approaches’) are relatively new in the qualitative research landscape. They emerged predominantly in the field of nursing and midwifery over the past two decades. 1 The design of qualitative descriptive studies evolved as a means to define aspects of qualitative research that did not resemble qualitative research designs to date, despite including elements of those other study designs. 2
Qualitative descriptive studies describe phenomena rather than explain them. Phenomenological studies, ethnographic studies and those using grounded theory seek to explain a phenomenon. Qualitative descriptive studies aim to provide a comprehensive summary of events. The approach to this study design is journalistic, with the aim being to answer the questions who, what, where and how. 3
A qualitative descriptive study is an important and appropriate design for research questions that are focused on gaining insights about a poorly understood research area, rather than on a specific phenomenon. Since qualitative descriptive study design seeks to describe rather than explain, explanatory frameworks and theories are not required to explain or ‘ground’ a study and its results. 4 The researcher may decide that a framework or theory adds value to their interpretations, and in that case, it is perfectly acceptable to use them. However, the hallmark of genuine curiosity (naturalistic enquiry) is that the researcher does not know in advance what they will be observing or describing. 4 Because a phenomenon is being described, the qualitative descriptive analysis is more categorical and less conceptual than other methods. Qualitative content analysis is usually the main approach to data analysis in qualitative descriptive studies. 4 This has led to criticism of descriptive research being less sophisticated because less interpretation is required than with other qualitative study designs in which interpretation and explanation are key characteristics (e.g. phenomenology, grounded theory, case studies).
Diverse approaches to data collection can be utilised in qualitative description studies. However, most qualitative descriptive studies use semi-structured interviews (see Chapter 13) because they provide a reliable way to collect data. 3 The technique applied to data analysis is generally categorical and less conceptual when compared to other qualitative research designs (see Section 4). 2,3 Hence, this study design is well suited to research by practitioners, student researchers and policymakers. Its straightforward approach enables these studies to be conducted in shorter timeframes than other study designs. 3 Descriptive studies are common as the qualitative component in mixed-methods research ( see Chapter 11 ) and evaluations ( see Chapter 12 ), 1 because qualitative descriptive studies can provide information to help develop and refine questionnaires or interventions.
For example, in our research to develop a patient-reported outcome measure for people who had undergone a percutaneous coronary intervention (PCI), which is a common cardiac procedure to treat heart disease, we started by conducting a qualitative descriptive study. 5 This project was a large, mixed-methods study funded by a private health insurer. The entire research process needed to be straightforward and achievable within a year, as we had engaged an undergraduate student to undertake the research tasks. The aim of the qualitative component of the mixed-methods study was to identify and explore patients’ perceptions following PCI. We used inductive approaches to collect and analyse the data. The study was guided by the following domains for the development of patient-reported outcomes, according to US Food and Drug Administration (FDA) guidelines, which included:
- Feeling: How the patient feels physically and psychologically after medical intervention
- Function: The patient’s mobility and ability to maintain their regular routine
- Evaluation: The patient’s overall perception of the success or failure of their procedure and their perception of what contributed to it. 5(p458)
We conducted focus groups and interviews, and asked participants three questions related to the FDA outcome domains:
- From your perspective, what would be considered a successful outcome of the procedure?
Probing questions: Did the procedure meet your expectations? How do you define whether the procedure was successful?
- How did you feel after the procedure?
Probing question: How did you feel one week after and how does that compare with how you feel now?
- After your procedure, tell me about your ability to do your daily activities?
Prompt for activities including gardening, housework, personal care, work-related and family-related tasks.
Probing questions: Did you attend cardiac rehabilitation? Can you tell us about your experience of cardiac rehabilitation? What impact has medication had on your recovery?
- What, if any, lifestyle changes have you made since your procedure? 5(p459)
Data collection was conducted with 32 participants. The themes were mapped to the FDA patient-reported outcome domains, with the results confirming previous research and also highlighting new areas for exploration in the development of a new patient-reported outcome measure. For example, participants reported a lack of confidence following PCI and the importance of patient and doctor communication. Women, in particular, reported that they wanted doctors to recognise how their experiences of cardiac symptoms were different to those of men.
The study described phenomena and resulted in the development of a patient-reported outcome measure that was tested and refined using a discrete-choice experiment survey, 6 a pilot of the measure in the Victorian Cardiac Outcomes Registry and a Rasch analysis to validate the measurement’s properties. 7
Advantages and disadvantages of qualitative descriptive studies
A qualitative descriptive study is an effective design for research by practitioners, policymakers and students, due to their relatively short timeframes and low costs. The researchers can remain close to the data and the events described, and this can enable the process of analysis to be relatively simple. Qualitative descriptive studies are also useful in mixed-methods research studies. Some of the advantages of qualitative descriptive studies have led to criticism of the design approach, due to a lack of engagement with theory and the lack of interpretation and explanation of the data. 2
Table 5.1. Examples of qualitative descriptive studies
Qualitative descriptive studies are gaining popularity in health and social care due to their utility, from a resource and time perspective, for research by practitioners, policymakers and researchers. Descriptive studies can be conducted as stand-alone studies or as part of larger, mixed-methods studies.
- Bradshaw C, Atkinson S, Doody O. Employing a qualitative description approach in health care research. Glob Qual Nurs Res. 2017;4. doi:10.1177/2333393617742282
- Lambert VA, Lambert CE. Qualitative descriptive research: an acceptable design. Pac Rim Int J Nurs Res Thail. 2012;16(4):255-256. Accessed June 6, 2023. https://he02.tci-thaijo.org/index.php/PRIJNR/article/download/5805/5064
- Doyle L et al. An overview of the qualitative descriptive design within nursing research. J Res Nurs. 2020;25(5):443-455. doi:10.1177/174498711988023
- Kim H, Sefcik JS, Bradway C. Characteristics of qualitative descriptive studies: a systematic review. Res Nurs Health. 2017;40(1):23-42. doi:10.1002/nur.21768
- Ayton DR et al. Exploring patient-reported outcomes following percutaneous coronary intervention: a qualitative study. Health Expect. 2018;21(2):457-465. doi:10.1111/hex.1263
- Barker AL et al. Symptoms and feelings valued by patients after a percutaneous coronary intervention: a discrete-choice experiment to inform development of a new patient-reported outcome. BMJ Open. 2018;8:e023141. doi:10.1136/bmjopen-2018-023141
- Soh SE et al. What matters most to patients following percutaneous coronary interventions? a new patient-reported outcome measure developed using Rasch analysis. PLoS One. 2019;14(9):e0222185. doi:10.1371/journal.pone.0222185
- Hiller RM et al. Coping and support-seeking in out-of-home care: a qualitative study of the views of young people in care in England. BMJ Open. 2021;11:e038461. doi:10.1136/bmjopen-2020-038461
- Backman C, Cho-Young D. Engaging patients and informal caregivers to improve safety and facilitate person- and family-centered care during transitions from hospital to home – a qualitative descriptive study. Patient Prefer Adherence. 2019;13:617-626. doi:10.2147/PPA.S201054
Qualitative Research – a practical guide for health and social care researchers and practitioners Copyright © 2023 by Darshini Ayton is licensed under a Creative Commons Attribution-NonCommercial 4.0 International License , except where otherwise noted.
Share This Book
Open Access is an initiative that aims to make scientific research freely available to all. To date our community has made over 100 million downloads. It’s based on principles of collaboration, unobstructed discovery, and, most importantly, scientific progression. As PhD students, we found it difficult to access the research we needed, so we decided to create a new Open Access publisher that levels the playing field for scientists across the world. How? By making research easy to access, and puts the academic needs of the researchers before the business interests of publishers.
We are a community of more than 103,000 authors and editors from 3,291 institutions spanning 160 countries, including Nobel Prize winners and some of the world’s most-cited researchers. Publishing on IntechOpen allows authors to earn citations and find new collaborators, meaning more people see your work not only from your own field of study, but from other related fields too.
Brief introduction to this section that descibes Open Access especially from an IntechOpen perspective
Want to get in touch? Contact our London head office or media team here
Our team is growing all the time, so we’re always on the lookout for smart people who want to help us reshape the world of scientific publishing.
Home > Books > Recent Advances in Biostatistics [Working Title]
Introduction to Descriptive Statistics
Submitted: 04 July 2023 Reviewed: 20 July 2023 Published: 07 September 2023
DOI: 10.5772/intechopen.1002475
Cite this chapter
There are two ways to cite this chapter:
From the Edited Volume
Recent Advances in Biostatistics [Working Title]
B. Santhosh Kumar
Chapter metrics overview
146 Chapter Downloads
Impact of this chapter
Total Chapter Downloads on intechopen.com
This chapter offers a comprehensive exploration of descriptive statistics, tracing its historical development from Condorcet’s “average” concept to Galton and Pearson’s contributions. Emphasizing its pivotal role in academia, descriptive statistics serve as a fundamental tool for summarizing and analyzing data across disciplines. The chapter underscores how descriptive statistics drive research inspiration and guide analysis, and provide a foundation for advanced statistical techniques. It delves into their historical context, highlighting their organizational and presentational significance. Furthermore, the chapter accentuates the advantages of descriptive statistics in academia, including their ability to succinctly represent complex data, aid decision-making, and enhance research communication. It highlights the potency of visualization in discerning data patterns and explores emerging trends like large dataset analysis, Bayesian statistics, and nonparametric methods. Sources of variance intrinsic to descriptive statistics, such as sampling fluctuations, measurement errors, and outliers, are discussed, stressing the importance of considering these factors in data interpretation.
- academic research
- data analysis
- data visualization
- decision-making
- research methodology
- data summarization
Author Information
Olubunmi alabi *.
- African University of Science and Technology, Abuja, Nigeria
Tosin Bukola
- University of Greenwich, London, United Kingdom
*Address all correspondence to: [email protected]
1. Introduction
The French mathematician and philosopher Condorcet established the idea of the “average” as a means to summarize data, which is when descriptive statistics got their start. Yet, the widespread use of descriptive statistics in academic study did not start until the 19th century. Francis Galton, who was concerned in the examination of human features and attributes, was one of the major forerunners of descriptive statistics. Galton created various statistical methods that are still frequently applied in academic research today, such as the correlation and regression analysis concepts. The American statistician and mathematician in the early 20th century Karl Pearson created the “normal distribution,” which is a bell-shaped curve that characterizes the distribution of many natural occurrences. Moreover, Pearson created a number of correlational measures and popularized the chi-square test, which evaluates the significance of variations between observed and predicted frequencies. With the advent of new methods like multivariate analysis and factor analysis in the middle of the 20th century, the development of electronic computers sparked a revolution in statistical analysis. Descriptive statistics is the analysis and summarization of data to gain insights into its characteristics and distribution [ 1 ].
Descriptive statistics help researchers generate study ideas and guide further analysis by allowing them to explore data patterns and trends [ 2 ]. Descriptive statistics were used more often in academic research because they helped researchers better comprehend their datasets and served as a basis for more sophisticated statistical techniques. Similarly, Descriptive statistics are used to summarize and analyze data in a variety of academic areas, including psychology, sociology, economics, education, and epidemiology [ 3 ]. Descriptive statistics continue to be a crucial research tool in academia today, giving researchers a method to compile and analyze data from many fields. It is now simpler than ever to analyze and understand data, enabling researchers to make better informed judgments based on their results. This is due to the development of new statistical techniques and computer tools. Descriptive statistics can benefit researchers in hypothesis creation and exploratory analysis by identifying trends, patterns, and correlations between variables in huge datasets [ 4 ]. Descriptive statistics are important in data-driven decision-making processes because they allow stakeholders to make educated decisions based on reliable data [ 5 ].
2. Background
The history of descriptive statistics may be traced back to the 17th century, when early pioneers like John Graunt and William Petty laid the groundwork for statistical analysis [ 6 ]. Descriptive statistics is a fundamental concept in academia that is widely used across many disciplines, including social sciences, economics, medicine, engineering, and business. Descriptive statistics provides a comprehensive background for understanding data by organizing, summarizing, and presenting information effectively [ 7 ]. In academia, descriptive statistics is used to summarize and analyze data, providing insights into the patterns, trends, and characteristics of a dataset. Similarly, in academic research, descriptive statistics are often used as a preliminary analysis technique to gain a better understanding of the dataset before applying more complex statistical methods. Descriptive statistics lay the groundwork for inferential statistics by assisting researchers in drawing inferences about a population based on observed sample data [ 8 ]. Descriptive statistics aid in the identification and analysis of outliers, which can give useful insights into unusual observations or data collecting problems [ 9 ].
Descriptive statistics enable researchers to synthesize both quantitative and qualitative data, allowing for a thorough examination of factors [ 10 ]. Descriptive statistics can provide valuable information about the central tendency, variability, and distribution of the data, allowing researchers to make informed decisions about the appropriate statistical techniques to use. Descriptive statistics are an essential component of survey research technique, allowing researchers to efficiently summarize and display survey results [ 11 ]. Descriptive statistics may be used to summarize data as well as spot outliers, or observations that dramatically depart from the trend of the data as a whole. Finding outliers can help researchers spot any issues or abnormalities in the data so they can make the necessary modifications or repairs. In academic research, descriptive statistics are frequently employed to address research issues and evaluate hypotheses. Descriptive statistics, for instance, can be used to compare the average scores of two groups to see if there is a significant difference between them. In order to create new hypotheses or validate preexisting ideas, descriptive statistics may also be used to find patterns and correlations in the data.
There are several sources of variation that can affect the descriptive statistics of a data set, some of which include: Sampling Variation, descriptive statistics are often calculated using a sample of data rather than the entire population. Therefore, the descriptive statistics can vary depending on the particular sample that is selected. This is known as sampling variation. Measurement Variation, different measurement methods can produce different results, leading to variation in descriptive statistics. For example, if a scale is used to measure the weight of objects, slight differences in how the scale is used can produce slightly different measurements.
Data entry errors are mistakes made during the data entry process which can lead to variation in descriptive statistics. Even small errors, such as transposing two digits, can significantly impact the results. Outliers, Outliers are extreme values that fall outside of the expected range of values. These values can skew the descriptive statistics, making them appear more or less extreme than they actually are. Natural Variation, Natural variation refers to the inherent variability in the data itself. For example, if a data set contains measurements of the heights of trees, there will naturally be variation in the heights of the trees. It is important to understand these sources of variation when interpreting and using descriptive statistics in academia. Properly accounting for these sources of variation can help ensure that the descriptive statistics accurately reflect the underlying data.
Some emerging patterns in descriptive statistics in academia include: Big data analysis, with the increasing availability of large data sets, researchers are using descriptive statistics to identify patterns and trends in the data. The use of big data analysis techniques, such as machine learning and data mining, is becoming more common in academic research. Visualization techniques, advances in data visualization techniques are enabling researchers to more easily identify patterns in data sets. For example, heat maps and scatter plots can be used to visualize the relationship between different variables. Bayesian statistics is an emerging area of research in academia, which involves using probability theory to make inferences about data. Bayesian statistics can provide more accurate estimates of descriptive statistics, particularly when dealing with complex data sets.
Non-parametric statistics are becoming increasingly popular in academia, particularly when dealing with data sets that do not meet the assumptions of traditional parametric statistical tests. Non-parametric tests do not require the data to be normally distributed, and can be more robust to outliers. Open science practices, such as pre-registration and data sharing, are becoming more common in academia. This is enabling researchers to more easily replicate and verify the results of descriptive statistical analyses, which can improve the quality and reliability of research findings. Overall, the emerging patterns in descriptive statistics in academia reflect the increasing availability of data, the need for more accurate and robust statistical techniques, and a growing emphasis on transparency and openness in research practices.
3. Benefits of descriptive statistics
The advantages of descriptive statistics extend beyond research and academia, with applications in commercial decision-making, public policy, and strategic planning [ 12 ]. The benefits of descriptive statistics include providing a clear and concise summary of data, aiding in decision-making processes, and facilitating effective communication of findings [ 13 ]. Descriptive statistics provide numerous benefits to academia, some of which include: Summarization of Data: descriptive statistics allow researchers to quickly and efficiently summarize large data sets, providing a snapshot of the key characteristics of the data. This can help researchers identify patterns and trends in the data, and can also help to simplify complex data sets. Better decision making: descriptive statistics can help researchers make data-driven decisions. For example, if a researcher is comparing the effectiveness of two different treatments, descriptive statistics can be used to identify which treatment is more effective based on the data. Visualization of data: descriptive statistics can be used to create visualizations of data, which can make it easier to communicate research findings to others.
Histograms, bar charts, and scatterplots are examples of data visualization techniques that may be used to graphically depict data in order to detect trends, outliers, and correlations [ 14 ]. Visualizations can also help to identify patterns and trends in the data that might not be immediately apparent from raw data. Hypothesis Testing: descriptive statistics are often used in hypothesis testing, which allows researchers to determine whether a particular hypothesis about a data set is supported by the data. This can help to validate research findings and increase confidence in the conclusions drawn from the data. Improved data quality: Descriptive statistics can help to identify errors or inconsistencies in the data, which can help researchers improve the quality of the data. This can lead to more accurate research findings and a better understanding of the underlying phenomena. Overall, the benefits of descriptive statistics in academia are many and varied. They help researchers summarize large data sets, make data-driven decisions, visualize data, validate research findings, and improve the quality of the data. By using descriptive statistics, researchers can gain valuable insights into complex data sets and make more informed decisions based on the data.
4. Practical applications of descriptive statistics
Descriptive statistics has practical applications in disciplines such as business, social sciences, healthcare, finance, and market research [ 15 ]. Descriptive statistics have a wide range of practical applications in academia, some of which include: Data Summarization: Descriptive statistics can be used to summarize large data sets, making it easier for researchers to understand the key characteristics of the data. This is particularly useful when dealing with complex data sets that contain many variables. Hypothesis Testing: Descriptive statistics can be used to test hypotheses about a data set. For example, researchers can use descriptive statistics to test whether the mean value of a particular variable is significantly different from a hypothesized value. Data visualization: descriptive statistics can be used to create visualizations of data, which can make it easier to identify patterns and trends in the data. For example, a histogram or boxplot can be used to visualize the distribution of a variable. Comparing Groups: Descriptive statistics can be used to compare different groups within a data set. For example, researchers may compare the mean values of a particular variable between different demographic groups, such as age or gender. Predictive modeling: Descriptive statistics can be used to build predictive models, which can be used to forecast future trends or outcomes. For example, a researcher might use descriptive statistics to identify the key variables that predict student performance in a particular course. The practical applications of descriptive statistics in academia are wide-ranging and varied. They can be used in many different fields, including psychology, economics, sociology, and biology, among others, to provide insights into complex data sets and help researchers make data-driven decisions ( Figure 1 ).
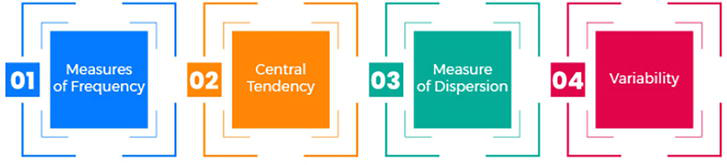
Types of descriptive statistics. Ref: https://www.analyticssteps.com/blogs/types-descriptive-analysis-examples-steps .
Descriptive statistics is a useful tool for researchers in a variety of sectors since it allows them express the major characteristics of a dataset, such as its frequency, central tendency, variability, and distribution.
4.1 Central tendency measurements
Central tendency metrics, such as mean, median, and mode, are essential descriptive statistics that offer information about the average or typical value in a collection [ 16 ]. One of the primary purposes of descriptive statistics is to summarize data in a succinct and useful manner. Measures of central tendency, such as the median, are resistant to outliers and offer a more representative assessment of the average value in a skewed distribution [ 17 ]. The mean, median, and mode are measures of central tendency that are used to characterize the usual or center value of a dataset. The mean of a dataset is the arithmetic average, but the median is the midway number when the data is ordered in order of magnitude. The mode is the most often occurring value in the collection. Central tendency measurements are one of the most important aspects of descriptive statistics, as they provide a summary of the “typical” value of a data set.
The three most commonly used measures of central tendency are: Mean: the mean is calculated by adding up all the values in a data set and dividing by the total number of values. The mean is sensitive to outliers, as even one extreme value can greatly affect the mean. Median: the median is the middle value in a data set when the values are ordered from smallest to largest. If the data set has an odd number of values, the median is the middle value. If the data set has an even number of values, the median is the average of the two middle values. The median is more robust to outliers than the mean. Mode: the mode is the most common value in a data set. In some cases, there may be multiple modes (i.e. bimodal or multimodal distributions). The mode is useful for identifying the most frequently occurring value in a data set. Each of these measures of central tendency provides a different perspective on the “typical” value of a data set, and which measure is most appropriate to use depends on the nature of the data and the research question being addressed. For example, if the data set contains extreme outliers, the median may be a better measure of central tendency than the mean. Conversely, if the data set is symmetrical and normally distributed, the mean may provide the best measure of central tendency.
4.2 Variability indices
It is another key part of descriptive statistics is determining data variability. The spread or dispersion of data points about the central tendency readings is quantified by variability indices such as range, variance, and standard deviation [ 18 ]. Variability measures, such as range, variance, and standard deviation, reveal information about the spread or dispersion of the data. Variability indices, such as the coefficient of variation, allow you to compare variability across various datasets with different scales or units of measurement [ 19 ]. The range is the distance between the dataset’s greatest and lowest values, and the variance and standard deviation are measures of how much the data values depart from the mean. Variability indices are measures used in descriptive statistics to provide information about how much the data varies or how spread out it is. Variability indices, such as the interquartile range, give insights into data distribution while being less impacted by extreme values than the standard deviation [ 20 ]. Some commonly used variability indices include:
Range: The range is the difference between the largest and smallest values in a data set. It provides a simple measure of the spread of the data, but is sensitive to outliers. Interquartile Range (IQR): The IQR is the range of the middle 50% of the data. It is calculated by subtracting the 25th percentile (lower quartile) from the 75th percentile (upper quartile). The IQR is more robust to outliers than the range. Variance: The variance is a measure of how spread out the data is around the mean. It is calculated by taking the average of the squared differences between each data point and the mean. The variance is sensitive to outliers. Standard Deviation: The standard deviation is the square root of the variance. It provides a measure of how much the data varies from the mean, and is more commonly used than the variance because it has the same units as the original data.
Coefficient of Variation (CV): The CV is a measure of relative variability, expressed as a percentage. It is calculated by dividing the standard deviation by the mean and multiplying by 100. The CV is useful for comparing variability across different data sets that have different units or scales. These variability indices provide important information about the spread and variability of the data, which can help researchers better understand the characteristics of the data and draw meaningful conclusions from it.
4.3 Data visualization
Data may be visually represented using graphical approaches in addition to numerical metrics. Graphs and charts, such as histograms, box plots, and scatterplots, allow researchers investigate data patterns and correlations. Box plots and violin plots are efficient data visualization approaches for showing data distribution and spotting potential outliers [ 21 ]. They may also be used to detect outliers, or data points that deviate dramatically from the rest of the data. Data visualization is an important aspect of descriptive statistics, as it allows researchers to communicate complex data in a visual and easily understandable format. Some common types of data visualization used in descriptive statistics include: Histograms: Histograms are used to display the distribution of a continuous variable. The data is divided into intervals (or “bins”), and the number of observations falling into each bin is displayed on the vertical axis. Histograms provide a visual representation of the shape of the distribution, and can help to identify outliers or skewness. Box plots: Box plots provide a graphical representation of the distribution of a continuous variable. The application of graphical approaches, such as scatterplots and heat maps, improves comprehension of correlations and patterns in large datasets [ 22 ].
The box represents the middle 50% of the data, with the median displayed as a horizontal line inside the box. The whiskers extend to the minimum and maximum values in the data set, and any outliers are displayed as points outside the whiskers. Box plots are useful for comparing distributions across different groups or for identifying outliers. Scatter plots: Scatter plots are used to display the relationship between two continuous variables. Each data point is represented as a point on the graph, with one variable displayed on the horizontal axis and the other variable displayed on the vertical axis. Scatter plots can help to identify patterns or relationships in the data, such as a positive or negative correlation. Bar charts: Bar charts are used to display the distribution of a categorical variable.
The categories are displayed on the horizontal axis, and the frequency or percentage of observations falling into each category is displayed on the vertical axis. Bar charts can help to compare the frequency of different categories or to display the results of a survey or questionnaire. Heat maps: Heat maps are used to display the relationship between two categorical variables. The categories are displayed on both the horizontal and vertical axes, and the frequency or percentage of observations falling into each combination of categories is displayed using a color scale. Heat maps can help to identify patterns or relationships in the data, such as a higher frequency of observations in certain combinations of categories. These types of data visualizations can help researchers to communicate complex data in a clear and understandable format, and can also provide insights into the characteristics of the data that may not be immediately apparent from the raw data.
4.4 Data cleaning and preprocessing
Data cleaning and preprocessing procedures, such as imputation methods for missing data, aid in the preservation of data integrity and the reduction of bias in descriptive analysis [ 23 ]. Before beginning any statistical analysis, be certain that the data is clean and well arranged. The process of discovering and fixing flaws or inconsistencies in data, such as missing numbers or outliers, is known as data cleaning. Data preparation is the process of putting data into an appropriate format for analysis, such as scaling or normalizing the data. Data cleaning and preprocessing are essential steps in descriptive analysis, as they help to ensure that the data is accurate, complete, and ready for analysis. Some common data cleaning and preprocessing steps include: Handling missing data: Missing data can be a common problem in datasets and can impact the accuracy of the analysis. Depending on the amount of missing data, researchers may choose to remove incomplete cases or impute missing values using techniques such as mean imputation, regression imputation, or multiple imputation. Handling outliers: Outliers are extreme values that are different from the majority of the data points and can distort the analysis. Outlier identification and removal procedures, for example, assist increase the accuracy and reliability of descriptive statistics [ 24 ].
To assure the correctness and dependability of descriptive statistics, data cleaning and preprocessing require finding and dealing with missing values, outliers, and data inconsistencies [ 25 ]. Researchers may choose to remove or transform outliers to better reflect the characteristics of the data. Data transformation: Data transformation is used to normalize the data or to make it easier to analyze. Common transformations include logarithmic, square root, or Box-Cox transformations. Handling categorical data: Categorical data, such as nominal or ordinal data, may need to be recoded into numerical data before analysis. Researchers may also need to handle missing or inconsistent categories within the data. Standardizing data: Standardizing data involves scaling the data to have a mean of zero and a standard deviation of one. This can be useful for comparing variables with different units or scales. Data integration: Data integration involves merging or linking multiple datasets to create a single, comprehensive dataset for analysis. This may involve matching or merging datasets based on common variables or identifiers. By performing these data cleaning and preprocessing steps, researchers can ensure that the data is accurate and ready for analysis, which can lead to more reliable and meaningful insights from the data.
5. Descriptive statistics in academic methodology
Descriptive statistics are important in academic technique because they enable researchers to synthesize and describe data collected for research objectives [ 26 ]. Descriptive statistics is often used in combination with other statistical techniques, such as inferential statistics, to draw conclusions and make predictions from the data. In academic research, descriptive statistics is used in a variety of ways, such as describing sample characteristics. Descriptive statistics is used to describe the characteristics of a sample, such as the mean, median, and standard deviation of a variable. This information can be used to identify patterns, trends, or differences within the sample. Identifying data outliers: Descriptive statistics can help researchers identify potential outliers or anomalies in the data, which can affect the validity of the results. For example, identifying extreme values in a dataset can help researchers to investigate whether these values are due to measurement error or a true characteristic of the population.
Communicating research findings: Descriptive statistics is used to summarize and communicate research findings in a clear and concise manner. Graphs, charts, and tables can be used to display descriptive statistics in a way that is easy to understand and interpret. Testing assumptions: Descriptive statistics can be used to test assumptions about the data, such as normality or homogeneity of variance, which are important for selecting appropriate statistical tests and interpreting the results. Overall, descriptive statistics is a critical methodology in academic research that helps researchers to describe and understand the characteristics of their data. By using descriptive statistics, researchers can draw meaningful insights and conclusions from their data, and communicate these findings to others in a clear and concise manner.
6. Pitfalls of descriptive statistics
The possibility for misunderstanding, reliance on summary measures alone, and susceptibility to high values or outliers are all disadvantages of descriptive statistics [ 27 ]. While descriptive statistics is an essential tool in academic statistics, there are several potential pitfalls that researchers should be aware of: Limited scope: Descriptive statistics can provide a useful summary of the characteristics of a dataset, but it is limited in its ability to provide insights into the underlying causes or mechanisms that drive the data. Descriptive statistics alone cannot establish causal relationships or test hypotheses. Misleading interpretations: Descriptive statistics can be misleading if not interpreted correctly. For example, a small sample size may not accurately represent the population, and summary statistics such as the mean may not be meaningful if the data is not normally distributed.
Incomplete analysis: Descriptive statistics can only provide a limited view of the data, and researchers may need to use additional statistical techniques to fully analyze the data. For example, hypothesis testing and regression analysis may be needed to establish relationships between variables and make predictions. Biased data: Descriptive statistics can be biased if the data is not representative of the population of interest. Sampling bias, measurement bias, or non-response bias can all impact the validity of descriptive statistics. Over-reliance on summary statistics: Descriptive statistics can be over-reliant on summary statistics such as the mean or median, which may not provide a complete picture of the data. Visualizations and other descriptive statistics, such as measures of variability, can provide additional insight into the data. To avoid these pitfalls, researchers should carefully consider the scope and limitations of descriptive statistics and use additional statistical techniques as needed. They should also ensure that their data is representative of the population of interest and interpret their descriptive statistics in a thoughtful and nuanced manner.
7. Conclusion
Researchers can test the normalcy assumptions of their data by using relevant descriptive statistics techniques such as measures of skewness and kurtosis [ 28 ]. Descriptive statistics has become a fundamental methodology in academic research that is used to summarize and describe the characteristics of a dataset, such as the central tendency, variability, and distribution of the data. It is used in a wide range of disciplines, including social sciences, natural sciences, engineering, and business. Descriptive statistics can be used to describe sample characteristics, identify data outliers, communicate research findings, and test assumptions. The kind of data, research topic, and particular aims of the study all influence the right choice and implementation of descriptive statistical approaches [ 29 ].
However, there are several potential pitfalls of descriptive statistics, including limited scope, misleading interpretations, incomplete analysis, biased data, and over-reliance on summary statistics. The use of descriptive statistics in data presentation can improve the interpretability of study findings, making complicated material more accessible to a larger audience [ 30 ]. To use descriptive statistics effectively in academic research, researchers should carefully consider the limitations and scope of the methodology, use additional statistical techniques as needed, ensure that their data is representative of the population of interest, and interpret their descriptive statistics in a thoughtful and nuanced manner.
Conflict of interest
The authors declare no conflict of interest.
- 1. Agresti A, Franklin C. Statistics: The Art and Science of Learning from Data. Upper Saddle River, NJ: Pearson; 2009
- 2. Norman GR, Streiner DL. Biostatistics: The Bare Essentials. 4th ed. Shelton (CT): PMPH-USA; 2014
- 3. Cohen J, Cohen P, West SG, Aiken LS. Applied Multiple Regression/Correlation Analysis for the Behavioral Sciences. New York: Routledge; 2013
- 4. Osborne J. Best practices in exploratory factor analysis: Four recommendations for getting the most from your analysis. Practical Assessment. 2019; 10 (7):1-9
- 5. Field A, Hole G. How To Design and Report Experiments Sage. The Tyranny of Evaluation Human Factors in Computing Systems CHI Fringe; 2003
- 6. Anders H. A History of Mathematical Statistics from 1750 to 1930. New York: Wiley; 1998. p. xvii+795. ISBN 0-471-17912-4
- 7. Rebecca M. Warner’s Applied Statistics: From Bivariate Through Multivariate Techniques. Second Edition. Thousand Oaks, California: SAGE Publications; 2012
- 8. Sullivan LM, Artino AR Jr. Analyzing and interpreting continuous data using ordinal regression. Journal of Graduate Medical Education. 2013; 5 (4):542-543
- 9. Hoaglin DC, Mosteller F, Tukey JW. Understanding Robust and Exploratory Data Analysis. John Wiley & Sons; 2011
- 10. Maxwell SE, Delaney HD, Kelley K. Designing Experiments and Analyzing Data: A Model Comparison Perspective. Routledge; 2017
- 11. De Leeuw ED, Hox JJ. International Handbook of Survey Methodology. Routledge; 2008
- 12. Chatfield C. The Analysis of Time Series: An Introduction. CRC Press; 2016
- 13. Tabachnick BG, Fidell LS. Using Multivariate Statistics. Pearson; 2013
- 14. Wickham H. ggplot2: Elegant Graphics for Data Analysis. Springer; 2016
- 15. Field A, Miles J, Field Z. Discovering Statistics Using R. Sage; 2012
- 16. Howell DC. Statistical Methods for Psychology. Cengage Learning; 2013
- 17. Wilcox RR. Modern Statistics for the Social and Behavioral Sciences: A Practical Introduction. CRC Press; 2017
- 18. Hair JF, Black WC, Babin BJ, Anderson RE. Multivariate Data Analysis. Pearson; 2019
- 19. Beasley TM, Schumacker RE. Multiple regression approach to analyzing contingency tables: Post hoc and planned comparison procedures. Journal of Experimental Education. 2013; 81 (3):310-312
- 20. Dodge Y. The Concise Encyclopedia of Statistics. Springer Science & Business Media; 2008
- 21. Krzywinski M, Altman N. Points of significance: Visualizing samples with box plots. Nature Methods. 2014; 11 (2):119-120
- 22. Cleveland WS. Visualizing data. Hobart Press; 1993
- 23. Little RJ, Rubin DB. Statistical Analysis with Missing Data. John Wiley & Sons; 2019
- 24. Filzmoser P, Maronna R, Werner M. Outlier identification in high dimensions. Computational Statistics & Data Analysis. 2008; 52 (3):1694-1711
- 25. Shmueli G, Bruce PC, Yahav I, Patel NR, Lichtendahl KC Jr, Desarbo WS. Data Mining for Business Analytics: Concepts, Techniques, and Applications in R. John Wiley & Sons; 2017
- 26. Aguinis H, Gottfredson RK. Statistical power analysis in HRM research. Organizational Research Methods. 2013; 16 (2):289-324
- 27. Stevens JP. Applied Multivariate Statistics for the Social Sciences. Routledge; 2012
- 28. Byrne BM. Structural Equation Modeling with AMOS: Basic Concepts, Applications, and Programming. Routledge; 2016
- 29. Everitt BS, Hothorn T. An Introduction to Applied Multivariate Analysis with R. Springer; 2011
- 30. Kosslyn SM. Graph Design for the Eye and Mind. Oxford University Press; 2006
© The Author(s). Licensee IntechOpen. This chapter is distributed under the terms of the Creative Commons Attribution 3.0 License , which permits unrestricted use, distribution, and reproduction in any medium, provided the original work is properly cited.
Have a thesis expert improve your writing
Check your thesis for plagiarism in 10 minutes, generate your apa citations for free.
- Knowledge Base
Descriptive Statistics | Definitions, Types, Examples
Published on 4 November 2022 by Pritha Bhandari . Revised on 9 January 2023.
Descriptive statistics summarise and organise characteristics of a data set. A data set is a collection of responses or observations from a sample or entire population .
In quantitative research , after collecting data, the first step of statistical analysis is to describe characteristics of the responses, such as the average of one variable (e.g., age), or the relation between two variables (e.g., age and creativity).
The next step is inferential statistics , which help you decide whether your data confirms or refutes your hypothesis and whether it is generalisable to a larger population.
Table of contents
Types of descriptive statistics, frequency distribution, measures of central tendency, measures of variability, univariate descriptive statistics, bivariate descriptive statistics, frequently asked questions.
There are 3 main types of descriptive statistics:
- The distribution concerns the frequency of each value.
- The central tendency concerns the averages of the values.
- The variability or dispersion concerns how spread out the values are.
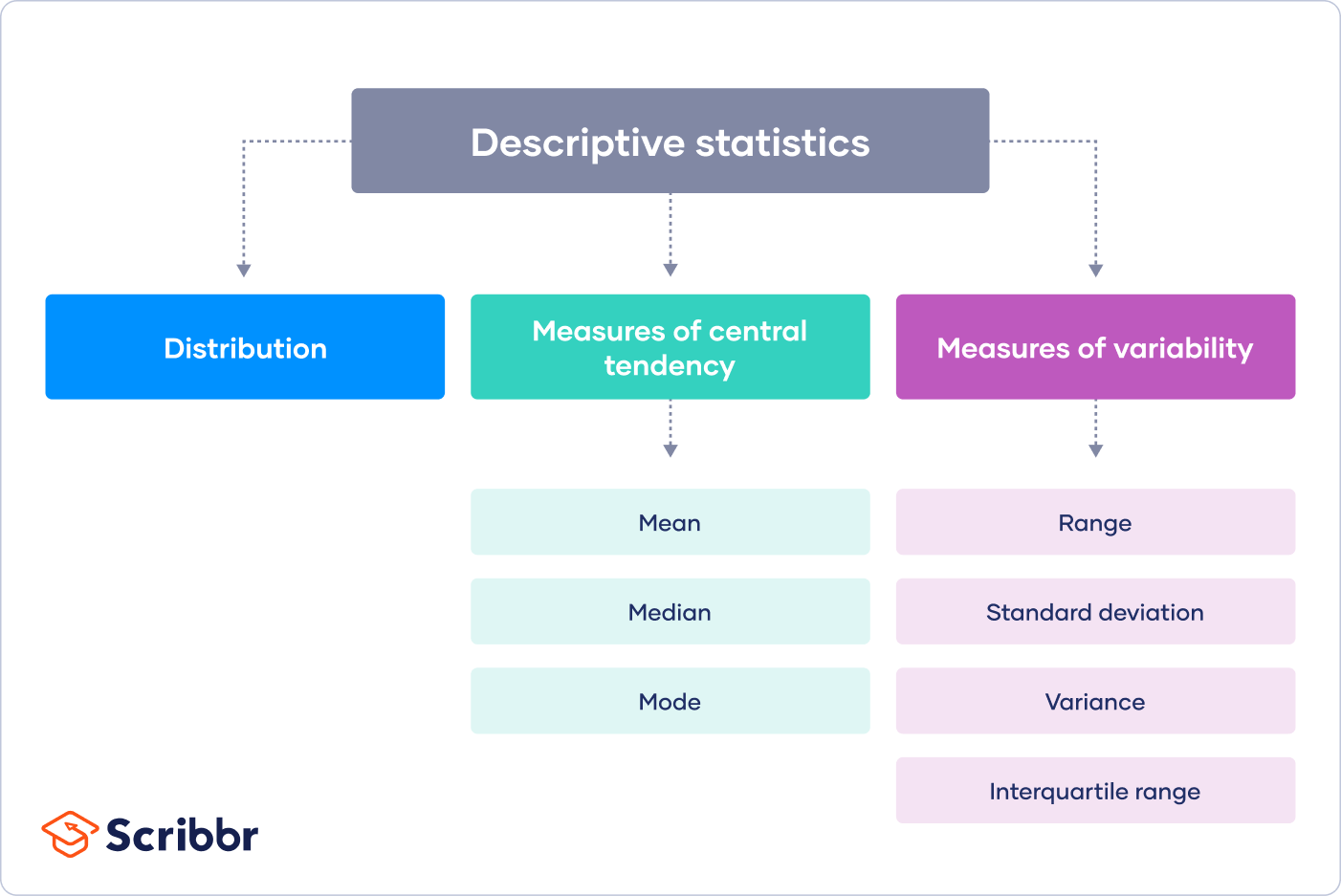
You can apply these to assess only one variable at a time, in univariate analysis, or to compare two or more, in bivariate and multivariate analysis.
- Go to a library
- Watch a movie at a theater
- Visit a national park
A data set is made up of a distribution of values, or scores. In tables or graphs, you can summarise the frequency of every possible value of a variable in numbers or percentages.
- Simple frequency distribution table
- Grouped frequency distribution table
From this table, you can see that more women than men or people with another gender identity took part in the study. In a grouped frequency distribution, you can group numerical response values and add up the number of responses for each group. You can also convert each of these numbers to percentages.
Measures of central tendency estimate the center, or average, of a data set. The mean , median and mode are 3 ways of finding the average.
Here we will demonstrate how to calculate the mean, median, and mode using the first 6 responses of our survey.
The mean , or M , is the most commonly used method for finding the average.
To find the mean, simply add up all response values and divide the sum by the total number of responses. The total number of responses or observations is called N .
The median is the value that’s exactly in the middle of a data set.
To find the median, order each response value from the smallest to the biggest. Then, the median is the number in the middle. If there are two numbers in the middle, find their mean.
The mode is the simply the most popular or most frequent response value. A data set can have no mode, one mode, or more than one mode.
To find the mode, order your data set from lowest to highest and find the response that occurs most frequently.
Measures of variability give you a sense of how spread out the response values are. The range, standard deviation and variance each reflect different aspects of spread.
The range gives you an idea of how far apart the most extreme response scores are. To find the range , simply subtract the lowest value from the highest value.
Standard deviation
The standard deviation ( s ) is the average amount of variability in your dataset. It tells you, on average, how far each score lies from the mean. The larger the standard deviation, the more variable the data set is.
There are six steps for finding the standard deviation:
- List each score and find their mean.
- Subtract the mean from each score to get the deviation from the mean.
- Square each of these deviations.
- Add up all of the squared deviations.
- Divide the sum of the squared deviations by N – 1.
- Find the square root of the number you found.
Step 5: 421.5/5 = 84.3
Step 6: √84.3 = 9.18
The variance is the average of squared deviations from the mean. Variance reflects the degree of spread in the data set. The more spread the data, the larger the variance is in relation to the mean.
To find the variance, simply square the standard deviation. The symbol for variance is s 2 .
Univariate descriptive statistics focus on only one variable at a time. It’s important to examine data from each variable separately using multiple measures of distribution, central tendency and spread. Programs like SPSS and Excel can be used to easily calculate these.
If you were to only consider the mean as a measure of central tendency, your impression of the ‘middle’ of the data set can be skewed by outliers, unlike the median or mode.
Likewise, while the range is sensitive to extreme values, you should also consider the standard deviation and variance to get easily comparable measures of spread.
If you’ve collected data on more than one variable, you can use bivariate or multivariate descriptive statistics to explore whether there are relationships between them.
In bivariate analysis, you simultaneously study the frequency and variability of two variables to see if they vary together. You can also compare the central tendency of the two variables before performing further statistical tests .
Multivariate analysis is the same as bivariate analysis but with more than two variables.
Contingency table
In a contingency table, each cell represents the intersection of two variables. Usually, an independent variable (e.g., gender) appears along the vertical axis and a dependent one appears along the horizontal axis (e.g., activities). You read ‘across’ the table to see how the independent and dependent variables relate to each other.
Interpreting a contingency table is easier when the raw data is converted to percentages. Percentages make each row comparable to the other by making it seem as if each group had only 100 observations or participants. When creating a percentage-based contingency table, you add the N for each independent variable on the end.
From this table, it is more clear that similar proportions of children and adults go to the library over 17 times a year. Additionally, children most commonly went to the library between 5 and 8 times, while for adults, this number was between 13 and 16.
Scatter plots
A scatter plot is a chart that shows you the relationship between two or three variables. It’s a visual representation of the strength of a relationship.
In a scatter plot, you plot one variable along the x-axis and another one along the y-axis. Each data point is represented by a point in the chart.
From your scatter plot, you see that as the number of movies seen at movie theaters increases, the number of visits to the library decreases. Based on your visual assessment of a possible linear relationship, you perform further tests of correlation and regression.
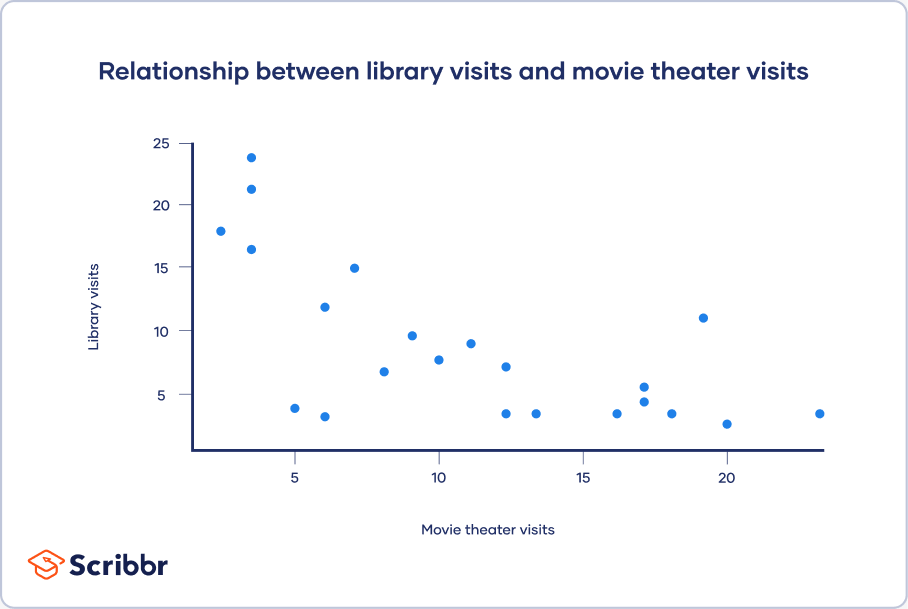
Descriptive statistics summarise the characteristics of a data set. Inferential statistics allow you to test a hypothesis or assess whether your data is generalisable to the broader population.
The 3 main types of descriptive statistics concern the frequency distribution, central tendency, and variability of a dataset.
- Distribution refers to the frequencies of different responses.
- Measures of central tendency give you the average for each response.
- Measures of variability show you the spread or dispersion of your dataset.
- Univariate statistics summarise only one variable at a time.
- Bivariate statistics compare two variables .
- Multivariate statistics compare more than two variables .
Cite this Scribbr article
If you want to cite this source, you can copy and paste the citation or click the ‘Cite this Scribbr article’ button to automatically add the citation to our free Reference Generator.
Bhandari, P. (2023, January 09). Descriptive Statistics | Definitions, Types, Examples. Scribbr. Retrieved 15 April 2024, from https://www.scribbr.co.uk/stats/descriptive-statistics-explained/
Is this article helpful?
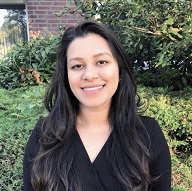
Pritha Bhandari
Other students also liked, data collection methods | step-by-step guide & examples, variability | calculating range, iqr, variance, standard deviation, normal distribution | examples, formulas, & uses.
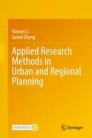
Applied Research Methods in Urban and Regional Planning pp 149–165 Cite as
Qualitative Data Analysis
- Yanmei Li 3 &
- Sumei Zhang 4
- First Online: 13 April 2022
1543 Accesses
3 Citations
After introducing basic statistical methods analyzing quantitative data this chapter turns to analyzing qualitative data, such as open-ended survey questions, planning documents, and narrative data collected from storytelling, planning workshops, public meetings, public hearings, planning forums, or focus groups. Practicing planners collect these types of data regularly and they are often the foundation of community needs analysis. Analyzing these data requires specialized methods. This chapter introduces methods to analyze qualitative data and conduct content analysis. Identifying trends and patterns of the data is the key to analyzing qualitative data. Related software, such as Atlas.ti, will be briefly explored to help researchers analyze complex qualitative data with complicated content or a large number of observations.
This is a preview of subscription content, log in via an institution .
Buying options
- Available as PDF
- Read on any device
- Instant download
- Own it forever
- Available as EPUB and PDF
- Compact, lightweight edition
- Dispatched in 3 to 5 business days
- Free shipping worldwide - see info
- Durable hardcover edition
Tax calculation will be finalised at checkout
Purchases are for personal use only
Fielding, N. G., & Lee, R. M. (1998). Computer analysis and qualitative research . Sage.
Google Scholar
Frechtling, J., & Sharp, L. (1997). User-friendly handbook for mixed method evaluations . National Science Foundation. https://www.nsf.gov/pubs/1997/nsf97153/start.htm
Hesse-Biber, S. (2010). Analyzing qualitative data: With or without software . (Lecture notes). https://www.bumc.bu.edu/crro/files/2010/07/Hesse-Bieber-4-10.pdf .
Kelle, U. (Ed.). (1996). Computer-aided qualitative data analysis . Sage.
MATH Google Scholar
Leech, N. L., & Onsuegbuzie, A. J. (2007). An array of qualitative data analysis tools: A call for data analysis triangulation. School Psychology Quarterly, 22 (4), 557–584. https://doi.org/10.1037/1045-3830.22.4.557
Article Google Scholar
Mahoney, C. (1997). Common qualitative methods. In J. Frechtling & L. Sharp (Eds.), User-friendly handbook for mixed method evaluations . National Science Foundation (NSF).
Neuman, W. L. (2011). Social research methods: Qualitative and quantitative approaches (7th ed.). Pearson Education.
Onsuegbuzie, A. J., & Teddlie, C. (2003). A framework for analyzing data in mixed methods research. In A. Tashakkori & C. Teddlie (Eds.), Handbook for mixed methods in social and behavioral research (pp. 351–383). Sage.
Patton, M. Q. (1990). Qualitative evaluation and research methods (2nd ed.). Sage.
Spadley, J. P. (1979). The ethnographic interview . Holt, Rinehart and Winston.
St. Johnson, W., & Johnson, P. (2004). The pros and cons of data analysis software for qualitative research. Journal of Nursing Scholarship, 32 (4), 393–397. https://doi.org/10.1111/j.1547-5069.2000.00393.x
Wong, L. P. (2008). Data analysis in qualitative research: A brief guide to using Nvivo. Malaysia Family Physician, 3 (1), 14–20. https://www.ncbi.nlm.nih.gov/pmc/articles/PMC4267019/
Zamawe, F. C. (2015). The implication of using NVivo software in qualitative data analysis: Evidence-based reflections. Malawi Medical Journal, 27 (1). https://doi.org/10.4314/mmj.v27i1.4
Download references
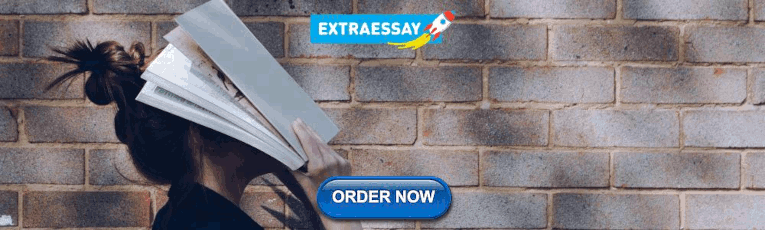
Author information
Authors and affiliations.
Florida Atlantic University, Boca Raton, FL, USA
University of Louisville, Louisville, KY, USA
Sumei Zhang
You can also search for this author in PubMed Google Scholar
Electronic Supplementary Material
Caption (docx 15 kb), rights and permissions.
Reprints and permissions
Copyright information
© 2022 Springer Nature Switzerland AG
About this chapter
Cite this chapter.
Li, Y., Zhang, S. (2022). Qualitative Data Analysis. In: Applied Research Methods in Urban and Regional Planning. Springer, Cham. https://doi.org/10.1007/978-3-030-93574-0_8
Download citation
DOI : https://doi.org/10.1007/978-3-030-93574-0_8
Published : 13 April 2022
Publisher Name : Springer, Cham
Print ISBN : 978-3-030-93573-3
Online ISBN : 978-3-030-93574-0
eBook Packages : Mathematics and Statistics Mathematics and Statistics (R0)
Share this chapter
Anyone you share the following link with will be able to read this content:
Sorry, a shareable link is not currently available for this article.
Provided by the Springer Nature SharedIt content-sharing initiative
- Publish with us
Policies and ethics
- Find a journal
- Track your research
Child Care and Early Education Research Connections
Descriptive Statistics
This page describes graphical and pictorial methods of descriptive statistics and the three most common measures of descriptive statistics (central tendency, dispersion, and association).
Descriptive statistics can be useful for two purposes: 1) to provide basic information about variables in a dataset and 2) to highlight potential relationships between variables. The three most common descriptive statistics can be displayed graphically or pictorially and are measures of:
Graphical/Pictorial Methods
Measures of central tendency, measures of dispersion, measures of association.
There are several graphical and pictorial methods that enhance researchers' understanding of individual variables and the relationships between variables. Graphical and pictorial methods provide a visual representation of the data. Some of these methods include:
Scatter plots
Geographical Information Systems (GIS)
Visually represent the frequencies with which values of variables occur
Each value of a variable is displayed along the bottom of a histogram, and a bar is drawn for each value
The height of the bar corresponds to the frequency with which that value occurs
Display the relationship between two quantitative or numeric variables by plotting one variable against the value of another variable
For example, one axis of a scatter plot could represent height and the other could represent weight. Each person in the data would receive one data point on the scatter plot that corresponds to his or her height and weight
Geographic Information Systems (GIS)
A GIS is a computer system capable of capturing, storing, analyzing, and displaying geographically referenced information; that is, data identified according to location
Using a GIS program, a researcher can create a map to represent data relationships visually
Display networks of relationships among variables, enabling researchers to identify the nature of relationships that would otherwise be too complex to conceptualize
Visit the following websites for more information:
Graphical Analytic Techniques
Geographic Information Systems
Glossary terms related to graphical and pictorial methods:
GIS Histogram Scatter Plot Sociogram
Measures of central tendency are the most basic and, often, the most informative description of a population's characteristics. They describe the "average" member of the population of interest. There are three measures of central tendency:
Mean -- the sum of a variable's values divided by the total number of values Median -- the middle value of a variable Mode -- the value that occurs most often
Example: The incomes of five randomly selected people in the United States are $10,000, $10,000, $45,000, $60,000, and $1,000,000.
Mean Income = (10,000 + 10,000 + 45,000 + 60,000 + 1,000,000) / 5 = $225,000 Median Income = $45,000 Modal Income = $10,000
The mean is the most commonly used measure of central tendency. Medians are generally used when a few values are extremely different from the rest of the values (this is called a skewed distribution). For example, the median income is often the best measure of the average income because, while most individuals earn between $0 and $200,000, a handful of individuals earn millions.
Basic Statistics
Measures of Position
Glossary terms related to measures of central tendency:
Average Central Tendency Confidence Interval Mean Median Mode Moving Average Point Estimate Univariate Analysis
Measures of dispersion provide information about the spread of a variable's values. There are four key measures of dispersion:
Standard Deviation
Range is simply the difference between the smallest and largest values in the data. The interquartile range is the difference between the values at the 75th percentile and the 25th percentile of the data.
Variance is the most commonly used measure of dispersion. It is calculated by taking the average of the squared differences between each value and the mean.
Standard deviation , another commonly used statistic, is the square root of the variance.
Skew is a measure of whether some values of a variable are extremely different from the majority of the values. For example, income is skewed because most people make between $0 and $200,000, but a handful of people earn millions. A variable is positively skewed if the extreme values are higher than the majority of values. A variable is negatively skewed if the extreme values are lower than the majority of values.
Example: The incomes of five randomly selected people in the United States are $10,000, $10,000, $45,000, $60,000, and $1,000,000:
Range = 1,000,000 - 10,000 = 990,000 Variance = [(10,000 - 225,000)2 + (10,000 - 225,000)2 + (45,000 - 225,000)2 + (60,000 - 225,000)2 + (1,000,000 - 225,000)2] / 5 = 150,540,000,000 Standard Deviation = Square Root (150,540,000,000) = 387,995 Skew = Income is positively skewed
Survey Research Tools
Variance and Standard Deviation
Summarizing and Presenting Data
Skewness Simulation
Glossary terms related to measures of dispersion:
Confidence Interval Distribution Kurtosis Point Estimate Quartiles Range Skewness Standard Deviation Univariate Analysis Variance
Measures of association indicate whether two variables are related. Two measures are commonly used:
Correlation
As a measure of association between variables, chi-square tests are used on nominal data (i.e., data that are put into classes: e.g., gender [male, female] and type of job [unskilled, semi-skilled, skilled]) to determine whether they are associated*
A chi-square is called significant if there is an association between two variables, and nonsignificant if there is not an association
To test for associations, a chi-square is calculated in the following way: Suppose a researcher wants to know whether there is a relationship between gender and two types of jobs, construction worker and administrative assistant. To perform a chi-square test, the researcher counts up the number of female administrative assistants, the number of female construction workers, the number of male administrative assistants, and the number of male construction workers in the data. These counts are compared with the number that would be expected in each category if there were no association between job type and gender (this expected count is based on statistical calculations). If there is a large difference between the observed values and the expected values, the chi-square test is significant, which indicates there is an association between the two variables.
*The chi-square test can also be used as a measure of goodness of fit, to test if data from a sample come from a population with a specific distribution, as an alternative to Anderson-Darling and Kolmogorov-Smirnov goodness-of-fit tests. As such, the chi square test is not restricted to nominal data; with non-binned data, however, the results depend on how the bins or classes are created and the size of the sample
A correlation coefficient is used to measure the strength of the relationship between numeric variables (e.g., weight and height)
The most common correlation coefficient is Pearson's r , which can range from -1 to +1.
If the coefficient is between 0 and 1, as one variable increases, the other also increases. This is called a positive correlation. For example, height and weight are positively correlated because taller people usually weigh more
If the correlation coefficient is between -1 and 0, as one variable increases the other decreases. This is called a negative correlation. For example, age and hours slept per night are negatively correlated because older people usually sleep fewer hours per night
Chi-Square Procedures for the Analysis of Categorical Frequency Data
Chi-square Analysis
Glossary terms related to measures of association:
Association Chi Square Correlation Correlation Coefficient Measures of Association Pearson's Correlational Coefficient Product Moment Correlation Coefficient
- Open access
- Published: 12 April 2024
Healthcare team resilience during COVID-19: a qualitative study
- John W. Ambrose 1 ,
- Ken Catchpole 2 ,
- Heather L. Evans 3 ,
- Lynne S. Nemeth 1 ,
- Diana M. Layne 1 &
- Michelle Nichols 1
BMC Health Services Research volume 24 , Article number: 459 ( 2024 ) Cite this article
186 Accesses
Metrics details
Resilience, in the field of Resilience Engineering, has been identified as the ability to maintain the safety and the performance of healthcare systems and is aligned with the resilience potentials of anticipation, monitoring, adaptation, and learning. In early 2020, the COVID-19 pandemic challenged the resilience of US healthcare systems due to the lack of equipment, supply interruptions, and a shortage of personnel. The purpose of this qualitative research was to describe resilience in the healthcare team during the COVID-19 pandemic with the healthcare team situated as a cognizant, singular source of knowledge and defined by its collective identity, purpose, competence, and actions, versus the resilience of an individual or an organization.
We developed a descriptive model which considered the healthcare team as a unified cognizant entity within a system designed for safe patient care. This model combined elements from the Patient Systems Engineering Initiative for Patient Safety (SEIPS) and the Advanced Team Decision Making (ADTM) models. Using a qualitative descriptive design and guided by our adapted model, we conducted individual interviews with healthcare team members across the United States. Data were analyzed using thematic analysis and extracted codes were organized within the adapted model framework.
Five themes were identified from the interviews with acute care professionals across the US ( N = 22): teamwork in a pressure cooker , consistent with working in a high stress environment; healthcare team cohesion , applying past lessons to present challenges , congruent with transferring past skills to current situations; knowledge gaps , and altruistic behaviors , aligned with sense of duty and personal responsibility to the team. Participants’ described how their ability to adapt to their environment was negatively impacted by uncertainty, inconsistent communication of information, and emotions of anxiety, fear, frustration, and stress. Cohesion with co-workers, transferability of skills, and altruistic behavior enhanced healthcare team performance.
Working within the extreme unprecedented circumstances of COVID-19 affected the ability of the healthcare team to anticipate and adapt to the rapidly changing environment. Both team cohesion and altruistic behavior promoted resilience. Our research contributes to a growing understanding of the importance of resilience in the healthcare team. And provides a bridge between individual and organizational resilience.
Peer Review reports
Introduction
The COVID-19 pandemic highlighted the complexity and dynamic nature of healthcare systems. It also created a unique opportunity to look at the concept of resilience through the lens of the healthcare team versus the more common approach of situating the concept within the individual or the organization. The early phase of the pandemic was marked by challenges, such as limited access to personal protective equipment, personnel shortages, drug shortages, and increased risks of infection [ 1 , 2 ]. Ensuring patient safety and proper functioning requires coordination and adaptation of the healthcare team and various processes across the health system infrastructure [ 3 , 4 ]. Resilience results from adaptive coordination which enables healthcare systems to maintain routine function in the face of all conditions [ 5 , 6 ].
Resilience in healthcare has been operationalized through resilience engineering, an interdisciplinary aspect of systems engineering focused on promotingpatient safety through the design, implementation, and management of healthcare systems [ 7 , 8 , 9 ] (e.g., how healthcare systems adapt and adjust to maneuver through the daily complexities and challenges to identify effective practices, prevent errors and maintain resilient performance) [ 6 , 8 , 9 , 10 , 11 ]. Resilient performance in healthcare is proposed to be the net result of reaching the threshold of four resilience capabilities within the system: anticipation, the ability to expect and prepare for the unexpected; monitoring, the ability to observe threats to daily system performance; responding, the ability to adapt how the performance is enacted; and learning, the ability to learn from present and past accomplishments within the system [ 12 ]. At present, there is a paucity of research on the resilience of the healthcare team as a cohesive, singular conscious source of knowledge in a highly complex healthcare system. While the resilience of both healthcare systems [ 11 , 13 ] and healthcare workers [ 14 ] has been investigated, there is a gap in knowledge specific to the resilience of the healthcare team as a unified singular consciousness. The circumstances surrounding the COVID-19 pandemic presented a unique opportunity to understand the resilience of the healthcare team in a highly complex system as a singular aware entity within the system; how it acknowledges itself, defines its purpose, and performs under extenuating circumstances. This shifts the emphasis of individual and organization resilience to the resilience in the interconnected healthcare team that extends beyond the boundary of any single person.
The adapted model situates the healthcare team as a cohesive singlular conscious source of knowledge within an intricate and highly complex system [ 15 ]. This model was designed as a bridge between resilience found in individuals within the healthcare system and the organization to emphasize the healthcare team as an aware, unified whole. Our model [ 15 ] combines the existing Systems Engineering Initiative for Patient Safety (SEIPS) model [ 16 ] (version 1), which is based on five domains (organization, person, tasks, technologies, and tools), and environment and the Advanced Team Decision Making Model [ 17 ], which includes components for team performance [ 17 , 18 , 19 ]. Team performance is comprised of team identity, team cognition, team competency, and team metacognition [ 17 , 18 , 19 ]. Team identity describes how the team identifies their purpose to help one another [ 17 ]. Team cognition describes the state of mind of the team, their focus, and common goals [ 17 ]. Team competency describes how well the team accomplishes tasks, and team metacognition describes problem solving and responsibility [ 17 , 19 ], Fig. 1 .
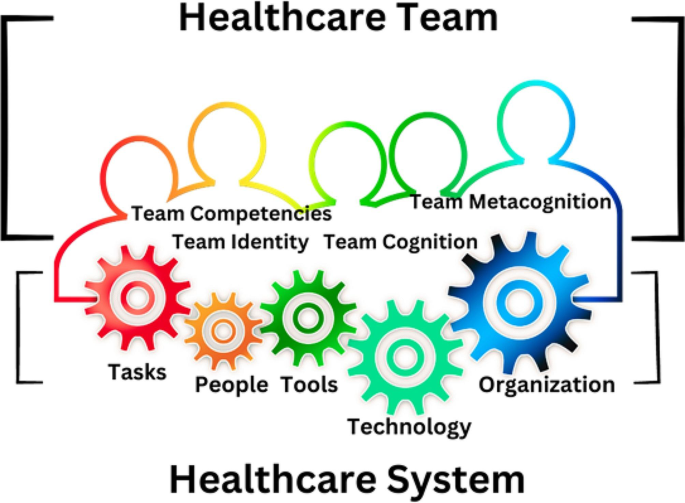
Healthcare Team as a cohesive, singular conscious source of knowledge in a highly complex system. The continuous variegated border represents the singularity and connectedness of the healthcare team within the system. The gears represent the processes, people, technology, and tasks within this highly dynamic healthcare system
The purpose of this qualitative research was to describe resilience in the healthcare team during the COVID-19 pandemic with the healthcare team situated as a singular conscious source of knowledge defined by its collective identity, purpose, competence, and actions. Additionally, we sought to identify factors that may facilitate or hinder the healthcare team from achieving the necessary capabilities to monitor, anticipate, adapt, and learn to meet the standard for resilient performance.
Methodology
A qualitative descriptive design [ 20 , 21 ] was employed. The interview guide was framed using the adapted model to explore various aspects of healthcare team performance (identity, purpose, competence, and cognition). These questions were pilot tested on the first 3 participants and no further changes were needed. Specifically, we aimed to investigate resilience capabilities, decision-making processes, and overall healthcare team performance.
Sampling strategy
A purposive snowball sample was used to identify healthcare team members who worked in U.S. acute care settings between January 2020–December 2020. This sampling method was used to ensure recruitment of participants most likely to have insight into the phenomenon of resilience in the acute care setting.
Inclusion criteria
To explore a wide range of interprofessional experience, participants were recruited across geographic regions and professional roles through personal contacts and social media [ 22 , 23 , 24 , 25 ]. Eligible participants included English-speaking individuals ages 20 and older with a valid personal email address, internet access, and the ability to participate in an online video interview. Potential participants had to be employed full or part-time for any period from January 2020–December 2020 in any of the following acute healthcare environments: emergency room (ER), intensive care unit (ICU), COVID- 19 ICU, COVID-19 floor, gastroenterology inpatient unit, endoscopy suite, operating room (OR), post anesthesia recovery room (PACU), pre-operative holding area, hospital administration, or inpatient medical and/or surgical patient care unit.
Exclusion criteria
Healthcare team members who did not complete the pre-screening survey or failed to schedule an interview were not enrolled.
National recruitment in the U.S
Upon approval by MUSC Institutional Review Board (IRB), registered under Pro00100917, fliers, social media posts on Twitter TM (version 9.34 IOS, San Francisco, California) and Facebook TM (version 390.1 IOS, Menlo Park, CA), and word of mouth were used to initiate recruitment efforts. Interested participants were sent a link to an electronic screening survey explaining the purpose of the study and verifying the respondents’ eligibility to participate. Informed consent was obtained from all subjects.
Data collection
Data were collected via an initial screening questionnaire to determine eligibility. Data were managed using REDCap™ (version 11.2.2) electronic data capture tools hosted at MUSC. Demographic data included age, sex, race, professional role, years of experience, geographic region, patient population served, practice specialty area, and deployment status during the pandemic. Deployment refers to the reassignment of personnel from their primary clinical area to another area to meet the demands of another clinical area without regard for the participant’s clinical expertise. Qualitative data were collected through semi-structured audio video recorded interviews to understand the healthcare team in their natural environment. Recorded interviews were conducted via Microsoft® Teams (version 1.5.00.17261, Microsoft Corporation) from the PIs private office to mitigate the risk of COVID-19 transmission and promote participation across the U.S.
Data monitoring and safety
The quality of the demographic data was monitored to ensure completeness. Potential participants who submitted incomplete responses on the questionnaire were excluded. Interviews were transcribed using software, transcriptions were reviewed and verified for accuracy, and then uploaded to MAXQDA Analytics Pro, Version 2022 (VERBI software) to facilitate data analysis. Transcripts were not returned to the participants. Qualitative codebooks, institutional review board (IRB) logs, and other study records were stored on a secure university server, with access limited to authorized study personnel. Adherence to Consolidated Criteria for Reporting Qualitative Research (COREQ) standards were maintained throughout the study and analysis [ 26 ].
Data analysis
Quantitative analysis.
Demographic data were analyzed using SPSS Statistics for MAC, version 28 (IBM). Both descriptive statistics for the continuous variables of age and years of experience (mean, standard deviation) and frequency tables (age, sex, race, role, geographic region, population served, deployment status) were analyzed.
Qualitative analysis
The Principal Investigator (PI) (JA) and senior mentor (MN) independently coded the interview transcripts. Open coding method was used to identify the categories of data [ 22 , 27 ]. Both a reflexive journal and audit trail were maintained. Codes were identified through induction from participant experiences and verified through weekly consensus meetings, while theoretical deductive analysis was guided by the adapted model and the four resilience capabilities (anticipation, monitoring, responding, learning [ 12 ]. Reflexive thematic analysis (TA) [ 28 , 29 , 30 , 31 ] was used to analyze the coded data and generate themes. Data were collected and categorized into the codebook until no further codes were identified by the PI and research mentor [ 22 , 27 ]. Participant checking was not employed.
Demographics
The eligibility pool was established based on survey completion. Eighty-nine healthcare team members opened the online screening survey; 21 were incomplete and eliminated from the dataset, which left a pool of 68 potential eligible participants. Eligible participants (100%) were contacted by email and phone to determine their interest in completing the study interview. Twenty-two participants completed screening surveys and study interviews between May–September 2021, equating to a 32.5% enrollment rate. Participant interviews lasted between 21 and 91 min with an average of 43 min. None of the interviews were repeated. Participant demographics, including descriptive statistic and role key, are noted in Tables 1 and 2 , respectively.
Five themes were identified: team work in a pressure cooker , healthcare team cohesion , applying past lessons to present challenges , knowledge gaps , and altruistic behaviors .
Teamwork in a pressure cooker
The theme teamwork in a pressure cooker describes the relentless pressures and emotional stressors (e.g., fear, anxiety, frustration, and stress) experienced by the healthcare team from the risks and potential threats associated with COVID-19 contamination and infection. Factors associated with these pressures included risk of COVID-19 exposure, lack of COVID-19 testing, rapid changes to policies and procedures from the standard, personnel shortages, limited physical space, and limited supplies. Exemplary quotes highlighting participant descriptions of these pressures or subthemes are noted in Table 3 .
The healthcare team described an unprecedented level of stress in the workplace as the healthcare team had to adjust to rapidly changing protocols. The lack of protective equipment, shortage of providers to perform patient care and a lack of a familiar clinical routine saturated them in overwhelming pressure and emotions that stuck to them as they navigated uncharted territory. Exemplary quotes highlighting the healthcare team’s descriptions of these emotions are noted in Table 4 .
“It was…uncharted territory for me.” (P1, DIR) “You were stuck in a situation you never— you didn’t know when it was going to end.” (P4, RN PACU) “They have not enough staff—they can’t do it—they—I don’t know what we’re going to do.” (P6, DIR). “When we deployed—trying to get re-accustomed to the changes—with the needs that had to be met was very difficult.” (P10, RN ENDO) “I wasn’t about to sign up for extra time working in under those stressful conditions.” (P17, RN PACU)
The fear of the unknown, combined with the constant need to adapt to rapidly changing circumstances, led to widespread stress, frustration, anxiety, and exhaustion within the healthcare team. This theme was characterized by the constant pressure both inside and outside of work experienced by the healthcare team.
“Driving to the hospital, crying, driving back from the hospital, crying, still doesn’t sum it up— surrounded by people who were just dying. And what could you do?” (P6, DIR) “It was constant. It was terrible. I couldn’t sleep at night. I’d wake up worried.” (P8, ER MD) “It was kind of like just keep sending the Calvary forward—and when one drops, you just walk over them.” (P17, RN PACU) “It was always there—COVID here, COVID there—you never could just completely get away from it. It was basically the center of everybody’s conversation everywhere you went or if you were on the phone with somebody.” (P18, RN COVID ICU) “I was having to call my parents before I’d leave my apartment to go into work— to vent to them and cry— to let out my frustration and my anxiety—and have them essentially convince me to go into work.” (P19, RN ICU). “Working so much— COVID was all that was on my brain—and it was a lot of pressure.” (P22, MGR)
Working during COVID-19 challenged the resilience of the healthcare team in the face of constant fear and uncertainty. The pressure to maintain team performance, while dealing with constant fear associated with the pandemic effected the healthcare team’s resilience.
“I have to tell you that after being in hospital—I don’t feel resilient right now— doing all the things I’ve done—I just want to be out of the hospital— [crying] I can tell you that it will stay with me the rest of my life— It will always stay with me.” (P6, DIR) “I feel like my team has used up all of their resilience. I don’t think there’s much left.” (P8, ER MD)
However, one team member stood out as an exception. They reported the pressures from the environment helped them to make decisions. This demonstrates that environmental pressures affect members of the healthcare team differently. They reported that the pressure and intensity of the situation sharpened their focus and allowed them to make choices more quickly and effectively.
“I make better decisions when I’m under pressure.” (P22, MGR)
Healthcare team cohesion
The theme healthcare team cohesion describes the unique experience of working together during the pandemic that created a means among the healthcare team to form close relationships and unite. This bond was characterized by the emergence of strong interpersonal connections among healthcare professionals during the COVID-19 pandemic. These connections shaped healthcare team relationships and were a factor in the collaborative decision-making processes within healthcare team for their day-to day functions. This cohesive bonding was fueled by the stress and uncertainty of the situation, which brought the healthcare team together illustrated by their solidarity, camaraderie, trust, and empowerment.
“All those decisions, important decisions were made together.” (P7, CRNA) “Everyone felt like they were they were, you know, in a in a battle zone and on the same side—and so that kind of brought people together.” (P8, ER MD) “I think our team worked as one.” (P11, CEO)
Solidarity described the sense of unity evident among the members of the healthcare team. This was characterized by connectedness and a sense of reliance on one another that promoted teamwork and resilience within the team from support both given and received. The sub-theme camaraderie described the close personal connection and support between the healthcare team that went beyond normal social interactions prior to the pandemic. These connections were filled with trust and respect for other healthcare team members.
“I think we were all trying to do the best we could do and help each other do the best they could do—I think early on just camaraderie helped a lot within the department and, you know, just relying on each other for support.” (P8, ER MD) “We knew that we can depend on each other and we all had different skill sets— I think that that was very important—that made us feel secure— rather than going alone.” (P10, RN ENDO) “We [The ICU Nurses] developed a sense of camaraderie that I mean, it’s nothing I’ve ever felt before, like we had to trust each other with our licenses, with our own health—my resiliency came from my coworkers.” (P14, CHG RN) “One of the things that I think the pandemic did in a positive—was—I believe that the teams that I worked for really started to solidify. We leaned on each other. I felt more of a team environment than I had had pre-pandemic—I felt that people were a bit better together. We all needed each other, and we all leaned on each other, and we gave each other support—more so than before COVID- 19.” (P15, CRNA) ”The nurses on the unit were always there for me—they became my friends— my family.” (P19, RN ICU)
The sub theme of empowerment referred to the ability of the healthcare team to confidently make decisions and assume responsibility for their actions within the healthcare setting. This process involved a sense of authority and the ability to exercise agency in decision-making together to respond and adapt to the demands the healthcare team experienced. The combination of solidarity, camaraderie, trust, and empowerment resulted in a strong sense of cohesion within the healthcare team which led to improved relationships and enhanced resilience in their performance.
“I felt that I felt that the team—we all needed each other and we all leaned on each other and we gave each other support—more so than before COVID.” (P15, CRNA) “How do you want to handle this? What’s the plan?—and we collaborated in the true sense of collaboration.” (P15, CRNA) “We just knew that we could count on each other—we knew that we could count on each other at any time if we had questions, because we all worked so closely together during this. We really became a really tight knit group, and it was great.” (P22, MGR)
The benefits of the cohesion found in the healthcare team were significant and apparent during the COVID-19 pandemic. The strengthened relationships and increased resilience allowed for improved communication and collaboration, leading to better patient care and outcomes. Despite these advantages, it was noted by one participant that the relationships developed were not sustained beyond the peak of the pandemic.
“Now that COVID is kind of at bay in our area, it’s kind of gone back to the same way it was— it has not stuck.” (P15, CRNA)
Applying past lessons to present challenges
The theme applying past lessons to present challenges describes how the knowledge and understanding gained from prior participant experiences was used to adapt to the novel clinical and infrastructural challenges faced during the pandemic. Past experiences facilitated the healthcare team to strategize ways to meet the demands of the healthcare system during this time.
Participants described two strategies the healthcare team used to improve the system’s ability to adapt and function effectively: changing roles and deploying personnel. The process of changing roles involved assigning new responsibilities to individuals based on priority-based initiatives, while deployment involved transferring clinical staff from areas with lower patient care needs to those with higher needs to optimize their utilization. Eleven participants (50%) were affected by these strategies. Of these, 73% were assigned to clinical areas for direct patient care, while the remaining 27% underwent a role change to support the operational needs of the system. The participants’ preexisting work relationships, specialized clinical expertise, and leadership abilities helped them adapt to their new clinical and non-clinical roles, which in turn enhanced the resilience of the healthcare team.
“We wanted to make sure that we were putting people into the right area where their skill set could be used the best.” (P1, DIR) “I’m known for moving people forward—I’m also well known for speaking up when I don’t think it is right and there was a lot of stuff that I didn’t think was right— and not only speaking up, I’m also going to come with the solution.” (P6, DIR)
Participants indicated the lessons learned from prior experience positively impacted team performance and improved patient care outcomes. There were two significant examples in the data: the perspective of a nurse who was redeployed to work in an obstetrics unit (P5, ENDO RN) and the perspective of a nursing director (P6, DIR) whose role was changed to develop a program to ensure adequate staffing.
“Because we [the team of interprofessionals] were all very familiar with what we had to do at the task, at handit [the experience of the provision of care] was very fluid—I think it’s because of our years of experience and working with each other for so long that it just worked out very well ”. (P5, ENDO RN) “Staff believed in me when I said I would do something— I could galvanize people because of my reputation of caring for staff, so I was chosen specifically because of my ability to move people forward in spite of things.” (P6, DIR)
Participants identified being assigned to unfamiliar clinical areas or working with unfamiliar patient populations as a barrier that hindered their ability to adapt to clinical situations. The lack of clinical competence among some personnel led to an increase in workload for other healthcare team members, who had to provide additional instruction and guidance on how to complete the task. Decision-makers who deployed nursing staff to a clinical area with higher staffing needs may have believed that the individual nurse had specific clinical skills that would be helpful in that area, and this was not the case.
“She [the patient] felt like it was that he [the new nurse]—really didn’t know what he was doing—not only were we kind of reintroduced to that role of caring for patients where we haven’t been recently, but we’re also in a teaching mode, too, for the new nurses—we had to prioritize how sick the patients were, from basic vital signs to wound dressings to respiratory, and help those new nurses know which to attend to first.” (P10, RN ENDO) “Nurses weren’t really put in a place with enough support and enough resources to be able to do a job, and to do a job that maybe they haven’t done for a few years.” (P10, RN ENDO)
The participants indicated that clinical competencies of a healthcare provider in one patient population may not necessarily be applicable to another patient group. For instance, a neonatal intensive care unit (NICU) nurse who has experience in managing Extra Corporeal Membranous Oxygen (ECMO) in newborns may not have the necessary skills to care for adult ECMO patients in an adult COVID-19 intensive care unit.
“The ECMO nurse was a NICU nurse, so she really could not help me.” (P14, CHG RN)
Knowledge gaps
The theme knowledge gaps refers to the disparity between the existing knowledge of the healthcare team and the knowledge required for the team to effectively respond and adapt to the needs of the healthcare system. The lack of COVID-19 specific knowledge led to gaps in the healthcare team’s understanding, while the lack of communication made it difficult for necessary information to be effectively conveyed and received (e.g., medical logistics, human resources, and other operational policies and procedures). This knowledge gap created a barrier to healthcare team resilience as their capacities to surveil, anticipate, and respond were diminished from the lack of knowledge.
“That [information] is pretty fundamental to how you [the healthcare team] function.” (P17, RN PACU) “I don’t think any amount of preparation could have actually prepared us for how bad COVID was—but we were very, very, very unprepared.” (P18, RN COVID ICU) “It was confusing, it was disruptive to the patients that we had there. They sensed that. And that’s— OK—screw with me, screw with my colleagues, but don’t screw with the patient.” (P21, RN ENDO)
All the participants in leadership roles during the COVID-19 pandemic emphasized the importance of having a thorough understanding of the information and effectively communicating it to the frontline healthcare team members most involved in providing patient care.
“There’s nothing worse than having to learn something in the moment and not being prepared for it.” (P1, DIR) “That made us communicate in multiple ways throughout a day because we all know people learn and adapt it could be in print. It could be in person; it could be a video. We tried to have multiple ways of getting messages out and knowing we needed to repeat messages because this was so unknown, and people were so stressed.” (P11, CEO)
One team member (P13, CRNA), highlighted areas where there were gaps in knowledge in greater detail.
“It was as if the unit was being run by all these sort of substitute teachers that were called in at the last minute. Nobody knew where stuff was—nobody knew what the protocol was—the communication was terrible.” (P13, CRNA)
The cumulative effect from the knowledge gaps contributed to the lack of a practical working knowledge for the healthcare team and affected the healthcare team’s ability to anticipate what needed to be done and adapt their performance to accomplish it. Despite knowledge gaps, healthcare team members reported their capability to learn was facilitated by incremental gains in practical knowledge through their experience over time.
“—people got to be experts at protecting patients and keeping themselves safe.” (P8, ER MD) “I think it kind of was like on the job training at that point, I felt like we were all just trying to survive—learning was like—you went out —then you came back, and you would share how things went.” (P15, CRNA) “You tried to educate yourself so you could be safe.” (P17, RN PACU)
The participant responses received from the leadership (CNO, Directors, and Manager) and front-line personnel (administrative staff, nurses, and physicians) regarding the importance of communication highlighted a difference in perspective. Leadership exhibited a strong commitment toward effective communication and made efforts to ensure all healthcare team members were well informed. On the other hand, the frontline participants indicated instances where communication strategies were not perceived as effective.
“I wasn’t contacted by a manager from the unit or anything to be able to reassure, reassure me that things were being followed through and it should be okay, so that was tough.” (P10, RN ENDO) “It really seemed like there was no communication between—like staffing and the floor—we would get up to the floor and they would say, who are you? What are you doing here? What are we supposed to do with you?” (P20, RN OR)
Altruistic behaviors
The theme altruistic behaviors , encompasses the participants’ perception of their obligation and accountability to their patients and healthcare team, and their steadfastness in supporting the healthcare team even if it meant facing personal or professional repercussions. This readiness to aid the healthcare team and accept consequences showcased their altruism and commitment to the healthcare team. The team’s dedication to both their patients and each other was a primary focus driven by a strong sense of responsibility and obligation.
“I want to be able to look myself in the mirror and feel like I did the right thing—.” (P6, DIR) “My resiliency came from my coworkers. I wanted to come back to work to help them.” (P14, RN COVID ICU) “People really looked out for each other—and people were really kind and compassionate to each other—we all were in this together.” (P15, CRNA) “I’m grateful for the experience that I had and all of the different patients that I was able to help in my time there definitely solidified that being a nurse is what I needed to do—and why I chose the profession is exactly what I should have been doing.” (P19, RN ICU) “You just have to go with what seems right—.” (P22, MGR)
A defining characteristic of this theme was a willingness to endure consequences for the benefit of the healthcare team. These consequences varied from contracting the virus, facing criticism from the healthcare team, to foregoing financial incentives, and even job loss.
“I felt like I was punished for speaking up and I was punished for doing the right thing for patients.” (P6, DIR) “I mean, I literally broke the law so many times. Do you know how many times I started pressors [vasoactive drugs to increase blood pressure] on patients that I had no orders for [because a physician would not enter the ICU]?” (P14, CHG RN)
We identified five key themes based on the coded data; namely teamwork in a pressure cooker , healthcare team cohesion , applying past lessons to present challenges , knowledge gaps , and altruistic behaviors . The researchers propose that stressors arising from the COVID-19 pandemic had an impact on the healthcare team’s resilience. In addition, strong healthcare team cohesion, selfless behaviors among the healthcare team, shared knowledge, and job competence within the healthcare team, enhanced resilient performance.
The healthcare team experienced significant stress and uncertainty, due to the COVID-19 pandemic. This is consistent with previous research that has shown that the unprecedented nature of the pandemic led to challenging working conditions, limited resources, lack of information, and concerns about infecting loved ones [ 32 , 33 , 34 , 35 , 36 , 37 , 38 , 39 , 40 , 41 , 42 , 43 , 44 ]. The collective global impact of COVID-19 on healthcare systems is likely a contributing factor to these stressors [ 45 , 46 , 47 , 48 ].
Our study, along with those conducted by Anjara et al. (2021)[ 49 ] and Kaye-Kauderer et al. (2022) [ 50 ], found that solidarity and camaraderie among healthcare professionals improve resilience. Specifically, Anjara et al. observed increased collaboration among the healthcare professionals they studied in Ireland during the COVID-19 pandemic, while Kaye-Kauderer et al. identified team camaraderie among their sample of front-line healthcare workers from New York. Kinsella et al. (2023) [ 51 ] reported that COVID − 19 offered frontline workers in the UK the opportunity to work together toward a common goal. Potential explanations for these findings align with the concepts of social capital proposed by Coleman [ 52 ] and social identification with other as proposed by Drury [ 54 ]. Coleman suggests an individual’s skills and capabilities are enhanced through their interdependent relationships with others [ 52 ]. Drury found in communities affected by disasters, mutual aid and support emerged from a shared social identity, which serves to strengthen the community [ 53 ]. Brooks et al. (2021) [ 54 ] conducted a study with healthcare, police, and commercial sectors in England. They found it was important for these individuals to receive support from and provide support to their colleagues to mitigate the psychological impact of disaster exposure [ 54 ]. In addition, like our findings, Aufegger and colleague’s 2019 systematic review [ 55 ] found that social support in acute care healthcare teams creates a supportive atmosphere where team members help each other communicate problems, fulfill needs, and deal with stress.
Our results are consistent with those of Liu et al. (2020) [ 32 ] and Banerjee et al. (2021) [ 44 ] who each found that healthcare professionals frequently feel a sense of personal responsibility to overcome challenges. One potential explanation for this may be the influence of collectivism in their cultures. Similarly, our study suggests the sense of camaraderie among healthcare professionals may also contribute to a sense of responsibility and increased altruistic behavior. However, other studies have highlighted different perspectives on healthcare professionals’ sense of responsibility and duty. Godkin and Markwell’s (2003) [ 56 ] revealed that healthcare professionals’ sense of responsibility during the Severe Acute Respiratory Syndrome (SARS) outbreak was dependent on the protective measures and support offered by the healthcare system where most SARS infected patients were hospitalized. More recently, Gray et al. (2021) reported that nurses’ sense of responsibility stems from their ethical obligations, regardless of potential personal or familial risks [ 57 ].
The altruistic behaviors described by our participants helped maintain the performance of the healthcare team. It is too soon to see the long-term impact from working in this high-pressure environment; however, past research by Liu et al. (2012) [ 58 ] and Wu (2009) [ 59 ] demonstrated that “altruistic-risk acceptance” during the SARS outbreak was shown to decrease depressive symptoms among hospital employees in China.
Our research on resilience has important implications for healthcare organizations and professionals. In order to ready themselves for forthcoming events, healthcare systems must emphasize the significance of shared knowledge and its influence on the healthcare team’s ability to foresee and monitor effectively. This knowledge can help the healthcare organization function as a unified entity, rather than as individuals in separate roles or clusters within the organization to improve healthcare team preparedness. Establishing a cohesive, clinically competent healthcare team benefits the organization and the patients served. Measures to enhance social support, improve communication and ensure clinical competence maintain healthcare team resilience.
There are several limitations to consider when interpreting the results of this study. First, the sample was obtained using purposive snowball sampling, which may have introduced sampling bias and may not accurately represent the larger population of healthcare team members who worked during the COVID-19, as 95% of the sample were white. Second, our study did not have equal representation of all interprofessional team members. It is possible that a more heterogenous sample regarding role, race and gender may have introduced additional codes. Additionally, the PI (JA) worked as a Certified Registered Nurse Anesthesiologist (CRNA) in acute care during the pandemic and personal experience may have introduced confirmation bias. Also, the focus of our research was to fill a gap in the existing knowledge of what is known about healthcare team resilience in pandemic disasters, and help to answer if and how it intersects with individual and organizational resilience. It is possible this novel conceptualization of healthcare team as a cohesive singular conscious source of knowledge did not adequately address this.
Steps to ensure rigor and mitigate any potential shortcomings of qualitative data analysis were the maintenance of a reflexive journal, a willingness of the PI to let go of unsupported ideas and constant verification of codes and themes with the research mentor (MN) for coherence and consistency within the coded data, selected methodology and research questions.
Overall, the extracted themes of teamwork in a pressure cooker; healthcare team cohesion; applying past lessons to present challenges; knowledge gaps; and altruistic behaviors illustrate comparable experiences within the healthcare team. As healthcare professionals and organizations continue to navigate the challenges of the COVID-19 pandemic and other crises, our findings provide valuable insights into how team cohesion, along with altruistic behaviors, may enhance resilience capabilities to create and maintain a unified resilient healthcare team.
Data availability
The data for this study are confidential as required by the IRB approval. To protect the anonymity of the participants, the data are not publicly available. Additional information about the research method, Interview questions, informant data, and the study in general can be requested from the corresponding author, J.A.
Berlin G, Singhal S, Lapointe M, Schulz J. Challenges emerge for the US healthcare system as COVID-19 cases rise. 2020;9.
Stevens JP, O’Donoghue A, Horng S, Tabb K. Healthcare’s earthquake: Lessons from complex adaptive systems to develop Covid-19-responsive measures and models. 2020.
Kopach-Konrad R, Lawley M, Criswell M, Hasan I, Chakraborty S, Pekny J, et al. Applying systems Engineering principles in improving Health Care Delivery. J Gen Intern Med. 2007;22(S3):431–7.
Article PubMed PubMed Central Google Scholar
Compton WD, Fanjiang G, Grossman JH, Reid PP. Institute of Medicine (U.S.), National Academy of Engineering. Building a better delivery system: a new engineering/health care partnership [Internet]. Washington, D.C.: National Academies Press; 2005 [cited 2021 Feb 12]. http://public.ebookcentral.proquest.com/choice/publicfullrecord.aspx?p=3378176 .
Hollnagel E, Woods DD. Resilience Engineering concepts and precepts. 1st ed. Boca Raton, FL: CRC Press/Routledge/Taylor & Francis Group; 2006. p. 416.
Google Scholar
Wiig S, O’Hara JK. Resilient and responsive healthcare services and systems: challenges and opportunities in a changing world. BMC Health Serv Res. 2021;21(1):1037.
Nemeth C, Wears RL, Patel S, Rosen G, Cook R. Resilience is not control: healthcare, crisis management, and ICT. Cogn Tech Work. 2011;13(3):189–202.
Article Google Scholar
Hollnagel E. Safety-II in Practice: Developing the Resilience Potentials [Internet]. 1st ed. Routledge; 2017 [cited 2022 May 7]. https://www.taylorfrancis.com/books/9781351780766 .
Braithwaite J, Wears RL, Hollnagel E. Resilient health care: turning patient safety on its head. Int J Qual Health Care. 2015;27(5):418–20.
Article PubMed Google Scholar
Madni AM, Jackson S. Towards a conceptual Framework for Resilience Engineering. IEEE Syst J. 2009;3(2):181–91.
Carthey J. Institutional resilience in healthcare systems. Qual Health Care. 2001;10(1):29–32.
Article CAS PubMed PubMed Central Google Scholar
Hollnagel E. The Four cornerstones of Resilience Engineering. In: Dekker, editor. Resilience engineering perspcetives. E. Hollnagel&S. Ashgate: Farnham, UK; 2009. pp. 117–33.
Fridell M, Edwin S, von Schreeb J, Saulnier DD. Health System Resilience: what are we talking about? A scoping review mapping characteristics and keywords. Int J Health Policy Manag. 2019;9(1):6–16.
Article PubMed Central Google Scholar
Curtin M, Richards HL, Fortune DG. Resilience among health care workers while working during a pandemic: a systematic review and meta synthesis of qualitative studies. Clin Psychol Rev. 2022;95:102173.
Ambrose JW, Layne DM, Catchpole K, Evans H, Nemeth LS. A qualitative protocol to examine Resilience Culture in Healthcare teams during COVID-19. Healthcare. 2021;9(9):1168.
Carayon P, Hundt AS, Karsh B, Gurses AP, Alvarado CJ, Smith M, et al. Work system design for patient safety: the SEIPS model. Qual Saf Health Care. 2006;15(Suppl 1):i50–8.
Thordsen ML, Kyne MM, Klein G, A Model of Advanced Team Decision Making and Performance.: Summary Report: [Internet]. Fort Belvoir, VA: Defense Technical Information Center; 1994 Sep [cited 2021 Feb 13]. http://www.dtic.mil/docs/citations/ADA400497 .
Zsambok CE. Advanced Team Decision Making: A Model and Training Implications.
Klein GA. Sources of power: how people make decisions. MIT Press; 1988.
Doyle L, McCabe C, Keogh B, Brady A, McCann M. An overview of the qualitative descriptive design within nursing research. J Res Nurs. 2020;25(5):443–55.
Siedlecki SL. Understanding descriptive research designs and methods. Clin Nurse Spec. 2020;34(1):8–12.
Crabtree BF, Miller WL. Doing qualitative research. Second. Thousand Oaks, CA: Sage; 1999. p. 406.
Bradley EH, Curry LA, Devers KJ. Qualitative Data Analysis for Health Services Research: developing taxonomy, themes, and theory. Health Serv Res. 2007;42(4):1758–72.
Gale NK, Heath G, Cameron E, Rashid S, Redwood S. Using the framework method for the analysis of qualitative data in multi-disciplinary health research. BMC Med Res Methodol. 2013;13(1):117.
Lincoln Y, Guba E. Naturalistic Inquiry. California: Sage; 1985.
Book Google Scholar
Tong A, Sainsbury P, Craig J. Consolidated criteria for reporting qualitative research (COREQ): a 32-item checklist for interviews and focus groups. Int J Qual Health Care. 2007;19(6):349–57.
Saldaña J. The coding manual for qualitative rearchers. Los Angeles, USA: Sage; 2021.
Boyatzis RE. Transforming qualitative information: thematic analysis and code development. Thousand Oaks, CA: SAGE Publications Ltd; 1998.
Braun V, Clarke V. What can thematic analysis offer health and wellbeing researchers? Int J Qualitative Stud Health Well-being. 2014;9(1):26152.
Braun V, Clarke V. Thematic analysis. In: Cooper H, Camic PM, Long DL, Panter AT, Rindskopf D, Sher KJ, editors. APA handbook of research methods in psychology, Vol 2: Research designs: Quantitative, qualitative, neuropsychological, and biological [Internet]. Washington: American Psychological Association; 2012 [cited 2022 May 15]. pp. 57–71. http://content.apa.org/books/13620-004 .
Braun V, Clarke V. Conceptual and design thinking for thematic analysis. Qualitative Psychol. 2022;9(1):3–26.
Liu Y, Zhai Z, Han Y, Liu Y, Liu F, Hu D. Experiences of front-line nurses combating coronavirus disease‐2019 in China: a qualitative analysis. Public Health Nurs. 2020;37(5):757–63.
Catania G, Zanini M, Hayter M, Timmins F, Dasso N, Ottonello G, et al. Lessons from Italian front-line nurses’ experiences during the COVID‐19 pandemic: a qualitative descriptive study. J Nurs Manag. 2021;29(3):404–11.
Croghan IT, Chesak SS, Adusumalli J, Fischer KM, Beck EW, Patel SR et al. Stress, Resilience, and Coping of Healthcare Workers during the COVID-19 Pandemic. Journal of Primary Care and Community Health [Internet]. 2021;12. https://www.scopus.com/inward/record.uri?eid=2-s2.0-85104122303&doi=10.1177%2f21501327211008448&partnerID=40&md5=96ad0164880c9725ce14d534e3c3117
Arnetz JE, Goetz CM, Arnetz BB, Arble E. Nurse reports of stressful situations during the COVID-19 pandemic: qualitative analysis of survey responses. IJERPH. 2020;17(21):8126.
Dagyaran I, Risom SS, Berg SK, Højskov IE, Heiden M, Bernild C, et al. Like soldiers on the front– a qualitative study understanding the frontline healthcare professionals’ experience of treating and caring for patients with COVID-19. BMC Health Serv Res. 2021;21(1):666.
Goh Y, Ow Yong QYJ, Chen TH, Ho SHC, Chee YIC, Chee TT. The impact of COVID-19 on nurses working in a University Health System in Singapore: a qualitative descriptive study. Int J Mental Health Nurs. 2021;30(3):643–52.
LoGiudice JA, Bartos S. Experiences of nurses during the COVID-19 pandemic: a mixed-methods study. AACN Adv Crit Care. 2021;32(1):14–26.
O’Brien JM, Bae FA, Kawchuk J, Reimche E, Abramyk CA, Kitts C et al. We were treading water. Experiences of healthcare providers in Canadian ICUs during COVID-19 visitor restrictions: a qualitative descriptive study.
Perraud F, Ecarnot F, Loiseau M, Laurent A, Fournier A, Lheureux F, et al. A qualitative study of reinforcement workers’ perceptions and experiences of working in intensive care during the COVID-19 pandemic: a PsyCOVID-ICU substudy. Sharma GA, editor. PLoS ONE. 2022;17(3):e0264287.
Shanafelt T, Ripp J, Trockel M. Understanding and addressing sources of anxiety among Health Care professionals during the COVID-19 pandemic. JAMA. 2020;323(21):2133.
Article CAS PubMed Google Scholar
Speroni KG, Seibert DJ, Mallinson RK. Nurses’ perceptions on Ebola Care in the United States, Part 2: a qualitative analysis. JONA: J Nurs Adm. 2015;45(11):544–50.
Sonis J, Pathman DE, Read S, Gaynes BN, Canter C, Curran P, et al. Effects of Healthcare Organization Actions and policies related to COVID-19 on Perceived Organizational Support among U.S. internists: a National Study. J Healthc Manag. 2022;67(3):192–205.
PubMed Google Scholar
Banerjee D, Sathyanarayana Rao TS, Kallivayalil RA, Javed A. Psychosocial Framework of Resilience: navigating needs and adversities during the pandemic, a qualitative exploration in the Indian Frontline Physicians. Front Psychol. 2021;12:622132.
Freudenberg LS, Paez D, Giammarile F, Cerci J, Modiselle M, Pascual TNB, et al. Global impact of COVID-19 on Nuclear Medicine departments: an International Survey in April 2020. J Nucl Med. 2020;61(9):1278–83.
Haldane V, Morgan GT. From resilient to transilient health systems: the deep transformation of health systems in response to the COVID-19 pandemic. Health Policy Plann. 2021;36(1):134–5.
Shrestha N, Shad MY, Ulvi O, Khan MH, Karamehic-Muratovic A, Nguyen USDT, et al. The impact of COVID-19 on globalization. One Health. 2020;11:100180.
Jean WC, Ironside NT, Sack KD, Felbaum DR, Syed HR. The impact of COVID-19 on neurosurgeons and the strategy for triaging non-emergent operations: a global neurosurgery study. Acta Neurochir. 2020;162(6):1229–40.
Anjara S, Fox R, Rogers L, De Brún A, McAuliffe E. Teamworking in Healthcare during the COVID-19 pandemic: a mixed-method study. IJERPH. 2021;18(19):10371.
Kaye-Kauderer H, Loo G, Murrough JW, Feingold JH, Feder A, Peccoralo L, et al. Effects of Sleep, Exercise, and Leadership Support on Resilience in Frontline Healthcare workers during the COVID-19 pandemic. J Occup Environ Med. 2022;64(5):416–20.
Kinsella EL, Muldoon OT, Lemon S, Stonebridge N, Hughes S, Sumner RC. In it together? Exploring solidarity with frontline workers in the United Kingdom and Ireland during COVID-19. Br J Social Psychol. 2023;62(1):241–63.
Coleman JS. Social Capital in the creation of Human Capital. Am J Sociol. 1988;94:S95–120.
Drury J, Carter H, Cocking C, Ntontis E, Tekin Guven S, Amlôt R. Facilitating collective psychosocial resilience in the Public in emergencies: twelve recommendations based on the Social Identity Approach. Front Public Health. 2019;7:141.
Brooks SK, Dunn R, Amlôt R, Rubin GJ, Greenberg N. Protecting the psychological wellbeing of staff exposed to disaster or emergency at work: a qualitative study. BMC Psychol. 2019;7(1):78.
Aufegger L, Shariq O, Bicknell C, Ashrafian H, Darzi A. Can shared leadership enhance clinical team management? A systematic review. LHS. 2019;32(2):309–35.
Godkin D, Markwell H. The Duty to Care of Healthcare Professionals: Ethical Issues and Guidelines for Policy Development. Submitted to SARS Expert Panel Secretariat.:23.
Gray K, Dorney P, Hoffman L, Crawford A. Nurses’ pandemic lives: a mixed-methods study of experiences during COVID-19. Appl Nurs Res. 2021;60:151437.
Liu X, Kakade M, Fuller CJ, Fan B, Fang Y, Kong J, et al. Depression after exposure to stressful events: lessons learned from the severe acute respiratory syndrome epidemic. Compr Psychiatr. 2012;53(1):15–23.
Wu P, Fang Y, Guan Z, Fan B, Kong J, Yao Z, et al. The psychological impact of the SARS Epidemic on Hospital employees in China: exposure, risk perception, and Altruistic Acceptance of Risk. Can J Psychiatry. 2009;54(5):302–11.
Download references
Acknowledgements
The authors want to thank all the interviewed healthcare team participants for their time and sharing their personal stories and for their continued service during the COVID-19 pandemic. We would also like to acknowledge Ayaba Logan, the Research and Education Informationist, Mohan Madisetti, the MUSC College of Nursing Director of Research, the staff of the MUSC Center for Academic Excellence and the reviewers of this journal for their constructive criticism.
This research (software, transcription services, etc.) was solely funded by the Principal Investigator, J.A.
Author information
Authors and affiliations.
College of Nursing, Medical University of South Carolina, Charleston, SC, USA
John W. Ambrose, Lynne S. Nemeth, Diana M. Layne & Michelle Nichols
Department of Anesthesia and Perioperative Medicine, College of Medicine, Medical University of South Carolina, Charleston, SC, USA
Ken Catchpole
Department of Surgery, College of Medicine, Medical University of South Carolina, Charleston, SC, USA
Heather L. Evans
You can also search for this author in PubMed Google Scholar
Contributions
Conceptualization J.A., K.C., L.N., D.L., H.E., and M.N.; methodology J.A. and M.N.; J.A. led the study, recruited the interviewees, conducted interviews, led the data analysis, and drafted the manuscript. J.A., and M.N. conducted the data analyses; review and editing K.C., H.E., D.L., and M.N.; supervision M.N.; research project administration J.A. and M.N.; funding acquisition J.A. All authors reviewed the manuscript.
Corresponding author
Correspondence to John W. Ambrose .
Ethics declarations
Ethics approval and consent to participate.
This study presented no greater than minimal risk to participants and met exempt status per regulatory criteria established by 45 CFR 46.104 and 21 CFR 56.104. The study protocol and all materials were approved by the MUSC Institutional Review Board (IRB), [Pro00100917 ]. All study procedures were followed in accordance with these standards.
Consent for publication
Not applicable.
Competing interests
The authors declare that they have no competing interests.
Additional information
Publisher’s note.
Springer Nature remains neutral with regard to jurisdictional claims in published maps and institutional affiliations.
Electronic supplementary material
Below is the link to the electronic supplementary material.
Supplementary Material 1
Rights and permissions.
Open Access This article is licensed under a Creative Commons Attribution 4.0 International License, which permits use, sharing, adaptation, distribution and reproduction in any medium or format, as long as you give appropriate credit to the original author(s) and the source, provide a link to the Creative Commons licence, and indicate if changes were made. The images or other third party material in this article are included in the article’s Creative Commons licence, unless indicated otherwise in a credit line to the material. If material is not included in the article’s Creative Commons licence and your intended use is not permitted by statutory regulation or exceeds the permitted use, you will need to obtain permission directly from the copyright holder. To view a copy of this licence, visit http://creativecommons.org/licenses/by/4.0/ . The Creative Commons Public Domain Dedication waiver ( http://creativecommons.org/publicdomain/zero/1.0/ ) applies to the data made available in this article, unless otherwise stated in a credit line to the data.
Reprints and permissions
About this article
Cite this article.
Ambrose, J.W., Catchpole, K., Evans, H.L. et al. Healthcare team resilience during COVID-19: a qualitative study. BMC Health Serv Res 24 , 459 (2024). https://doi.org/10.1186/s12913-024-10895-3
Download citation
Received : 25 February 2023
Accepted : 25 March 2024
Published : 12 April 2024
DOI : https://doi.org/10.1186/s12913-024-10895-3
Share this article
Anyone you share the following link with will be able to read this content:
Sorry, a shareable link is not currently available for this article.
Provided by the Springer Nature SharedIt content-sharing initiative
- Resilience Engineering
- Healthcare System
- Healthcare Administration
- Healthcare Team
- Thematic Analysis
- Qualitative Research
BMC Health Services Research
ISSN: 1472-6963
- General enquiries: [email protected]

An official website of the United States government
The .gov means it's official. Federal government websites often end in .gov or .mil. Before sharing sensitive information, make sure you're on a federal government site.
The site is secure. The https:// ensures that you are connecting to the official website and that any information you provide is encrypted and transmitted securely.
- Publications
- Account settings
- Browse Titles
NCBI Bookshelf. A service of the National Library of Medicine, National Institutes of Health.
StatPearls [Internet]. Treasure Island (FL): StatPearls Publishing; 2024 Jan-.

StatPearls [Internet].
Qualitative study.
Steven Tenny ; Janelle M. Brannan ; Grace D. Brannan .
Affiliations
Last Update: September 18, 2022 .
- Introduction
Qualitative research is a type of research that explores and provides deeper insights into real-world problems. [1] Instead of collecting numerical data points or intervene or introduce treatments just like in quantitative research, qualitative research helps generate hypotheses as well as further investigate and understand quantitative data. Qualitative research gathers participants' experiences, perceptions, and behavior. It answers the hows and whys instead of how many or how much. It could be structured as a stand-alone study, purely relying on qualitative data or it could be part of mixed-methods research that combines qualitative and quantitative data. This review introduces the readers to some basic concepts, definitions, terminology, and application of qualitative research.
Qualitative research at its core, ask open-ended questions whose answers are not easily put into numbers such as ‘how’ and ‘why’. [2] Due to the open-ended nature of the research questions at hand, qualitative research design is often not linear in the same way quantitative design is. [2] One of the strengths of qualitative research is its ability to explain processes and patterns of human behavior that can be difficult to quantify. [3] Phenomena such as experiences, attitudes, and behaviors can be difficult to accurately capture quantitatively, whereas a qualitative approach allows participants themselves to explain how, why, or what they were thinking, feeling, and experiencing at a certain time or during an event of interest. Quantifying qualitative data certainly is possible, but at its core, qualitative data is looking for themes and patterns that can be difficult to quantify and it is important to ensure that the context and narrative of qualitative work are not lost by trying to quantify something that is not meant to be quantified.
However, while qualitative research is sometimes placed in opposition to quantitative research, where they are necessarily opposites and therefore ‘compete’ against each other and the philosophical paradigms associated with each, qualitative and quantitative work are not necessarily opposites nor are they incompatible. [4] While qualitative and quantitative approaches are different, they are not necessarily opposites, and they are certainly not mutually exclusive. For instance, qualitative research can help expand and deepen understanding of data or results obtained from quantitative analysis. For example, say a quantitative analysis has determined that there is a correlation between length of stay and level of patient satisfaction, but why does this correlation exist? This dual-focus scenario shows one way in which qualitative and quantitative research could be integrated together.
Examples of Qualitative Research Approaches
Ethnography
Ethnography as a research design has its origins in social and cultural anthropology, and involves the researcher being directly immersed in the participant’s environment. [2] Through this immersion, the ethnographer can use a variety of data collection techniques with the aim of being able to produce a comprehensive account of the social phenomena that occurred during the research period. [2] That is to say, the researcher’s aim with ethnography is to immerse themselves into the research population and come out of it with accounts of actions, behaviors, events, etc. through the eyes of someone involved in the population. Direct involvement of the researcher with the target population is one benefit of ethnographic research because it can then be possible to find data that is otherwise very difficult to extract and record.
Grounded Theory
Grounded Theory is the “generation of a theoretical model through the experience of observing a study population and developing a comparative analysis of their speech and behavior.” [5] As opposed to quantitative research which is deductive and tests or verifies an existing theory, grounded theory research is inductive and therefore lends itself to research that is aiming to study social interactions or experiences. [3] [2] In essence, Grounded Theory’s goal is to explain for example how and why an event occurs or how and why people might behave a certain way. Through observing the population, a researcher using the Grounded Theory approach can then develop a theory to explain the phenomena of interest.
Phenomenology
Phenomenology is defined as the “study of the meaning of phenomena or the study of the particular”. [5] At first glance, it might seem that Grounded Theory and Phenomenology are quite similar, but upon careful examination, the differences can be seen. At its core, phenomenology looks to investigate experiences from the perspective of the individual. [2] Phenomenology is essentially looking into the ‘lived experiences’ of the participants and aims to examine how and why participants behaved a certain way, from their perspective . Herein lies one of the main differences between Grounded Theory and Phenomenology. Grounded Theory aims to develop a theory for social phenomena through an examination of various data sources whereas Phenomenology focuses on describing and explaining an event or phenomena from the perspective of those who have experienced it.
Narrative Research
One of qualitative research’s strengths lies in its ability to tell a story, often from the perspective of those directly involved in it. Reporting on qualitative research involves including details and descriptions of the setting involved and quotes from participants. This detail is called ‘thick’ or ‘rich’ description and is a strength of qualitative research. Narrative research is rife with the possibilities of ‘thick’ description as this approach weaves together a sequence of events, usually from just one or two individuals, in the hopes of creating a cohesive story, or narrative. [2] While it might seem like a waste of time to focus on such a specific, individual level, understanding one or two people’s narratives for an event or phenomenon can help to inform researchers about the influences that helped shape that narrative. The tension or conflict of differing narratives can be “opportunities for innovation”. [2]
Research Paradigm
Research paradigms are the assumptions, norms, and standards that underpin different approaches to research. Essentially, research paradigms are the ‘worldview’ that inform research. [4] It is valuable for researchers, both qualitative and quantitative, to understand what paradigm they are working within because understanding the theoretical basis of research paradigms allows researchers to understand the strengths and weaknesses of the approach being used and adjust accordingly. Different paradigms have different ontology and epistemologies . Ontology is defined as the "assumptions about the nature of reality” whereas epistemology is defined as the “assumptions about the nature of knowledge” that inform the work researchers do. [2] It is important to understand the ontological and epistemological foundations of the research paradigm researchers are working within to allow for a full understanding of the approach being used and the assumptions that underpin the approach as a whole. Further, it is crucial that researchers understand their own ontological and epistemological assumptions about the world in general because their assumptions about the world will necessarily impact how they interact with research. A discussion of the research paradigm is not complete without describing positivist, postpositivist, and constructivist philosophies.
Positivist vs Postpositivist
To further understand qualitative research, we need to discuss positivist and postpositivist frameworks. Positivism is a philosophy that the scientific method can and should be applied to social as well as natural sciences. [4] Essentially, positivist thinking insists that the social sciences should use natural science methods in its research which stems from positivist ontology that there is an objective reality that exists that is fully independent of our perception of the world as individuals. Quantitative research is rooted in positivist philosophy, which can be seen in the value it places on concepts such as causality, generalizability, and replicability.
Conversely, postpositivists argue that social reality can never be one hundred percent explained but it could be approximated. [4] Indeed, qualitative researchers have been insisting that there are “fundamental limits to the extent to which the methods and procedures of the natural sciences could be applied to the social world” and therefore postpositivist philosophy is often associated with qualitative research. [4] An example of positivist versus postpositivist values in research might be that positivist philosophies value hypothesis-testing, whereas postpositivist philosophies value the ability to formulate a substantive theory.
Constructivist
Constructivism is a subcategory of postpositivism. Most researchers invested in postpositivist research are constructivist as well, meaning they think there is no objective external reality that exists but rather that reality is constructed. Constructivism is a theoretical lens that emphasizes the dynamic nature of our world. “Constructivism contends that individuals’ views are directly influenced by their experiences, and it is these individual experiences and views that shape their perspective of reality”. [6] Essentially, Constructivist thought focuses on how ‘reality’ is not a fixed certainty and experiences, interactions, and backgrounds give people a unique view of the world. Constructivism contends, unlike in positivist views, that there is not necessarily an ‘objective’ reality we all experience. This is the ‘relativist’ ontological view that reality and the world we live in are dynamic and socially constructed. Therefore, qualitative scientific knowledge can be inductive as well as deductive.” [4]
So why is it important to understand the differences in assumptions that different philosophies and approaches to research have? Fundamentally, the assumptions underpinning the research tools a researcher selects provide an overall base for the assumptions the rest of the research will have and can even change the role of the researcher themselves. [2] For example, is the researcher an ‘objective’ observer such as in positivist quantitative work? Or is the researcher an active participant in the research itself, as in postpositivist qualitative work? Understanding the philosophical base of the research undertaken allows researchers to fully understand the implications of their work and their role within the research, as well as reflect on their own positionality and bias as it pertains to the research they are conducting.
Data Sampling
The better the sample represents the intended study population, the more likely the researcher is to encompass the varying factors at play. The following are examples of participant sampling and selection: [7]
- Purposive sampling- selection based on the researcher’s rationale in terms of being the most informative.
- Criterion sampling-selection based on pre-identified factors.
- Convenience sampling- selection based on availability.
- Snowball sampling- the selection is by referral from other participants or people who know potential participants.
- Extreme case sampling- targeted selection of rare cases.
- Typical case sampling-selection based on regular or average participants.
Data Collection and Analysis
Qualitative research uses several techniques including interviews, focus groups, and observation. [1] [2] [3] Interviews may be unstructured, with open-ended questions on a topic and the interviewer adapts to the responses. Structured interviews have a predetermined number of questions that every participant is asked. It is usually one on one and is appropriate for sensitive topics or topics needing an in-depth exploration. Focus groups are often held with 8-12 target participants and are used when group dynamics and collective views on a topic are desired. Researchers can be a participant-observer to share the experiences of the subject or a non-participant or detached observer.
While quantitative research design prescribes a controlled environment for data collection, qualitative data collection may be in a central location or in the environment of the participants, depending on the study goals and design. Qualitative research could amount to a large amount of data. Data is transcribed which may then be coded manually or with the use of Computer Assisted Qualitative Data Analysis Software or CAQDAS such as ATLAS.ti or NVivo. [8] [9] [10]
After the coding process, qualitative research results could be in various formats. It could be a synthesis and interpretation presented with excerpts from the data. [11] Results also could be in the form of themes and theory or model development.
Dissemination
To standardize and facilitate the dissemination of qualitative research outcomes, the healthcare team can use two reporting standards. The Consolidated Criteria for Reporting Qualitative Research or COREQ is a 32-item checklist for interviews and focus groups. [12] The Standards for Reporting Qualitative Research (SRQR) is a checklist covering a wider range of qualitative research. [13]
Examples of Application
Many times a research question will start with qualitative research. The qualitative research will help generate the research hypothesis which can be tested with quantitative methods. After the data is collected and analyzed with quantitative methods, a set of qualitative methods can be used to dive deeper into the data for a better understanding of what the numbers truly mean and their implications. The qualitative methods can then help clarify the quantitative data and also help refine the hypothesis for future research. Furthermore, with qualitative research researchers can explore subjects that are poorly studied with quantitative methods. These include opinions, individual's actions, and social science research.
A good qualitative study design starts with a goal or objective. This should be clearly defined or stated. The target population needs to be specified. A method for obtaining information from the study population must be carefully detailed to ensure there are no omissions of part of the target population. A proper collection method should be selected which will help obtain the desired information without overly limiting the collected data because many times, the information sought is not well compartmentalized or obtained. Finally, the design should ensure adequate methods for analyzing the data. An example may help better clarify some of the various aspects of qualitative research.
A researcher wants to decrease the number of teenagers who smoke in their community. The researcher could begin by asking current teen smokers why they started smoking through structured or unstructured interviews (qualitative research). The researcher can also get together a group of current teenage smokers and conduct a focus group to help brainstorm factors that may have prevented them from starting to smoke (qualitative research).
In this example, the researcher has used qualitative research methods (interviews and focus groups) to generate a list of ideas of both why teens start to smoke as well as factors that may have prevented them from starting to smoke. Next, the researcher compiles this data. The research found that, hypothetically, peer pressure, health issues, cost, being considered “cool,” and rebellious behavior all might increase or decrease the likelihood of teens starting to smoke.
The researcher creates a survey asking teen participants to rank how important each of the above factors is in either starting smoking (for current smokers) or not smoking (for current non-smokers). This survey provides specific numbers (ranked importance of each factor) and is thus a quantitative research tool.
The researcher can use the results of the survey to focus efforts on the one or two highest-ranked factors. Let us say the researcher found that health was the major factor that keeps teens from starting to smoke, and peer pressure was the major factor that contributed to teens to start smoking. The researcher can go back to qualitative research methods to dive deeper into each of these for more information. The researcher wants to focus on how to keep teens from starting to smoke, so they focus on the peer pressure aspect.
The researcher can conduct interviews and/or focus groups (qualitative research) about what types and forms of peer pressure are commonly encountered, where the peer pressure comes from, and where smoking first starts. The researcher hypothetically finds that peer pressure often occurs after school at the local teen hangouts, mostly the local park. The researcher also hypothetically finds that peer pressure comes from older, current smokers who provide the cigarettes.
The researcher could further explore this observation made at the local teen hangouts (qualitative research) and take notes regarding who is smoking, who is not, and what observable factors are at play for peer pressure of smoking. The researcher finds a local park where many local teenagers hang out and see that a shady, overgrown area of the park is where the smokers tend to hang out. The researcher notes the smoking teenagers buy their cigarettes from a local convenience store adjacent to the park where the clerk does not check identification before selling cigarettes. These observations fall under qualitative research.
If the researcher returns to the park and counts how many individuals smoke in each region of the park, this numerical data would be quantitative research. Based on the researcher's efforts thus far, they conclude that local teen smoking and teenagers who start to smoke may decrease if there are fewer overgrown areas of the park and the local convenience store does not sell cigarettes to underage individuals.
The researcher could try to have the parks department reassess the shady areas to make them less conducive to the smokers or identify how to limit the sales of cigarettes to underage individuals by the convenience store. The researcher would then cycle back to qualitative methods of asking at-risk population their perceptions of the changes, what factors are still at play, as well as quantitative research that includes teen smoking rates in the community, the incidence of new teen smokers, among others. [14] [15]
Qualitative research functions as a standalone research design or in combination with quantitative research to enhance our understanding of the world. Qualitative research uses techniques including structured and unstructured interviews, focus groups, and participant observation to not only help generate hypotheses which can be more rigorously tested with quantitative research but also to help researchers delve deeper into the quantitative research numbers, understand what they mean, and understand what the implications are. Qualitative research provides researchers with a way to understand what is going on, especially when things are not easily categorized. [16]
- Issues of Concern
As discussed in the sections above, quantitative and qualitative work differ in many different ways, including the criteria for evaluating them. There are four well-established criteria for evaluating quantitative data: internal validity, external validity, reliability, and objectivity. The correlating concepts in qualitative research are credibility, transferability, dependability, and confirmability. [4] [11] The corresponding quantitative and qualitative concepts can be seen below, with the quantitative concept is on the left, and the qualitative concept is on the right:
- Internal validity--- Credibility
- External validity---Transferability
- Reliability---Dependability
- Objectivity---Confirmability
In conducting qualitative research, ensuring these concepts are satisfied and well thought out can mitigate potential issues from arising. For example, just as a researcher will ensure that their quantitative study is internally valid so should qualitative researchers ensure that their work has credibility.
Indicators such as triangulation and peer examination can help evaluate the credibility of qualitative work.
- Triangulation: Triangulation involves using multiple methods of data collection to increase the likelihood of getting a reliable and accurate result. In our above magic example, the result would be more reliable by also interviewing the magician, back-stage hand, and the person who "vanished." In qualitative research, triangulation can include using telephone surveys, in-person surveys, focus groups, and interviews as well as surveying an adequate cross-section of the target demographic.
- Peer examination: Results can be reviewed by a peer to ensure the data is consistent with the findings.
‘Thick’ or ‘rich’ description can be used to evaluate the transferability of qualitative research whereas using an indicator such as an audit trail might help with evaluating the dependability and confirmability.
- Thick or rich description is a detailed and thorough description of details, the setting, and quotes from participants in the research. [5] Thick descriptions will include a detailed explanation of how the study was carried out. Thick descriptions are detailed enough to allow readers to draw conclusions and interpret the data themselves, which can help with transferability and replicability.
- Audit trail: An audit trail provides a documented set of steps of how the participants were selected and the data was collected. The original records of information should also be kept (e.g., surveys, notes, recordings).
One issue of concern that qualitative researchers should take into consideration is observation bias. Here are a few examples:
- Hawthorne effect: The Hawthorne effect is the change in participant behavior when they know they are being observed. If a researcher was wanting to identify factors that contribute to employee theft and tells the employees they are going to watch them to see what factors affect employee theft, one would suspect employee behavior would change when they know they are being watched.
- Observer-expectancy effect: Some participants change their behavior or responses to satisfy the researcher's desired effect. This happens in an unconscious manner for the participant so it is important to eliminate or limit transmitting the researcher's views.
- Artificial scenario effect: Some qualitative research occurs in artificial scenarios and/or with preset goals. In such situations, the information may not be accurate because of the artificial nature of the scenario. The preset goals may limit the qualitative information obtained.
- Clinical Significance
Qualitative research by itself or combined with quantitative research helps healthcare providers understand patients and the impact and challenges of the care they deliver. Qualitative research provides an opportunity to generate and refine hypotheses and delve deeper into the data generated by quantitative research. Qualitative research does not exist as an island apart from quantitative research, but as an integral part of research methods to be used for the understanding of the world around us. [17]
- Enhancing Healthcare Team Outcomes
Qualitative research is important for all members of the health care team as all are affected by qualitative research. Qualitative research may help develop a theory or a model for health research that can be further explored by quantitative research. Much of the qualitative research data acquisition is completed by numerous team members including social works, scientists, nurses, etc. Within each area of the medical field, there is copious ongoing qualitative research including physician-patient interactions, nursing-patient interactions, patient-environment interactions, health care team function, patient information delivery, etc.
- Review Questions
- Access free multiple choice questions on this topic.
- Comment on this article.
Disclosure: Steven Tenny declares no relevant financial relationships with ineligible companies.
Disclosure: Janelle Brannan declares no relevant financial relationships with ineligible companies.
Disclosure: Grace Brannan declares no relevant financial relationships with ineligible companies.
This book is distributed under the terms of the Creative Commons Attribution-NonCommercial-NoDerivatives 4.0 International (CC BY-NC-ND 4.0) ( http://creativecommons.org/licenses/by-nc-nd/4.0/ ), which permits others to distribute the work, provided that the article is not altered or used commercially. You are not required to obtain permission to distribute this article, provided that you credit the author and journal.
- Cite this Page Tenny S, Brannan JM, Brannan GD. Qualitative Study. [Updated 2022 Sep 18]. In: StatPearls [Internet]. Treasure Island (FL): StatPearls Publishing; 2024 Jan-.
In this Page
Bulk download.
- Bulk download StatPearls data from FTP
Related information
- PMC PubMed Central citations
- PubMed Links to PubMed
Similar articles in PubMed
- Suicidal Ideation. [StatPearls. 2024] Suicidal Ideation. Harmer B, Lee S, Duong TVH, Saadabadi A. StatPearls. 2024 Jan
- Folic acid supplementation and malaria susceptibility and severity among people taking antifolate antimalarial drugs in endemic areas. [Cochrane Database Syst Rev. 2022] Folic acid supplementation and malaria susceptibility and severity among people taking antifolate antimalarial drugs in endemic areas. Crider K, Williams J, Qi YP, Gutman J, Yeung L, Mai C, Finkelstain J, Mehta S, Pons-Duran C, Menéndez C, et al. Cochrane Database Syst Rev. 2022 Feb 1; 2(2022). Epub 2022 Feb 1.
- Macromolecular crowding: chemistry and physics meet biology (Ascona, Switzerland, 10-14 June 2012). [Phys Biol. 2013] Macromolecular crowding: chemistry and physics meet biology (Ascona, Switzerland, 10-14 June 2012). Foffi G, Pastore A, Piazza F, Temussi PA. Phys Biol. 2013 Aug; 10(4):040301. Epub 2013 Aug 2.
- Review Evidence Brief: The Effectiveness Of Mandatory Computer-Based Trainings On Government Ethics, Workplace Harassment, Or Privacy And Information Security-Related Topics [ 2014] Review Evidence Brief: The Effectiveness Of Mandatory Computer-Based Trainings On Government Ethics, Workplace Harassment, Or Privacy And Information Security-Related Topics Peterson K, McCleery E. 2014 May
- Review Public sector reforms and their impact on the level of corruption: A systematic review. [Campbell Syst Rev. 2021] Review Public sector reforms and their impact on the level of corruption: A systematic review. Mugellini G, Della Bella S, Colagrossi M, Isenring GL, Killias M. Campbell Syst Rev. 2021 Jun; 17(2):e1173. Epub 2021 May 24.
Recent Activity
- Qualitative Study - StatPearls Qualitative Study - StatPearls
Your browsing activity is empty.
Activity recording is turned off.
Turn recording back on
Connect with NLM
National Library of Medicine 8600 Rockville Pike Bethesda, MD 20894
Web Policies FOIA HHS Vulnerability Disclosure
Help Accessibility Careers
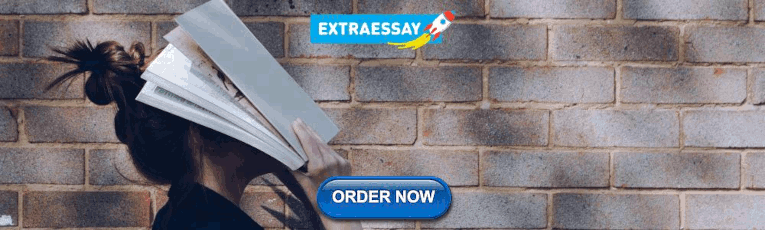
IMAGES
VIDEO
COMMENTS
Qualitative and descriptive research methods have been very common procedures for conducting research in many disciplines, including education, psychology, and social sciences. These types of research have also begun to be increasingly used in the field of second language teaching and learning. ... Chi-square statistics were used to examine the ...
Qualitative description (QD) is a label used in qualitative research for studies which are descriptive in nature, particularly for examining health care and nursing-related phenomena (Polit & Beck, 2009, 2014).QD is a widely cited research tradition and has been identified as important and appropriate for research questions focused on discovering the who, what, and where of events or ...
Using the data from these three rows, we can draw the following descriptive picture. Mentabil scores spanned a range of 50 (from a minimum score of 85 to a maximum score of 135). Speed scores had a range of 16.05 s (from 1.05 s - the fastest quality decision to 17.10 - the slowest quality decision).
Descriptive statistics summarize and organize characteristics of a data set. A data set is a collection of responses or observations from a sample or entire population. ... In quantitative research, after collecting data, the first step of statistical analysis is to describe characteristics of the responses, such as the average of one variable ...
Descriptive statistics in research: a critical component of data analysis ... It's also important to note that descriptive statistics can employ and use both quantitative and qualitative research. Describing data is undoubtedly the most critical first step in research as it enables the subsequent organization, simplification and summarization ...
Qualitative descriptive designs are common in nursing and healthcare research due to their inherent simplicity, flexibility and utility in diverse healthcare contexts. However, the application of descriptive research is sometimes critiqued in terms of scientific rigor. Inconsistency in decision making within the research process coupled with a ...
However, most qualitative descriptive studies use semi-structured interviews (see Chapter 13) because they provide a reliable way to collect data. 3 The technique applied to data analysis is generally categorical and less conceptual when compared to other qualitative research designs (see Section 4). 2,3 Hence, this study design is well suited ...
Descriptive research methods. Descriptive research is usually defined as a type of quantitative research, though qualitative research can also be used for descriptive purposes. The research design should be carefully developed to ensure that the results are valid and reliable.. Surveys. Survey research allows you to gather large volumes of data that can be analyzed for frequencies, averages ...
Descriptive statistics enable researchers to synthesize both quantitative and qualitative data, ... In academic research, descriptive statistics are frequently employed to address research issues and evaluate hypotheses. Descriptive statistics, for instance, can be used to compare the average scores of two groups to see if there is a ...
Therefore, we talk about "generic" or "descriptive-interpretive" approaches to qualitative research that share in common an effort to describe, summarize, and classify what is present in the data, which always, as we explain in Chapter 4, involves a degree of interpretation. 3.
For many researchers unfamiliar with qualitative research, determining how to conduct qualitative analyses is often quite challenging. Part of this challenge is due to the seemingly limitless approaches that a qualitative researcher might leverage, as well as simply learning to think like a qualitative researcher when analyzing data. From framework analysis (Ritchie & Spencer, 1994) to content ...
Characteristics of Qualitative Descriptive Studies: A Systematic Review. Hyejin Kim, Corresponding Author. Hyejin Kim ... In this systematic review, we identified characteristics of methods and findings reported in research articles published in 2014 whose authors identified the work as QD. After searching and screening, data were extracted ...
Abstract. Descriptive research is a study of status and is widely used in education, nutrition, epidemiology, and the behavioral sciences. Its value is based on the premise that problems can be solved and practices improved through observation, analysis, and description. The most common descriptive research method is the survey, which includes ...
Box 5. Common Uses of Descriptive Accounts in Education Research and Practice 7 Box 6. Steps in a Descriptive Analysis—An Iterative Process 8 Box 7. Data Summaries Are Not Descriptive Analysis 10 Box 8. An Example of Using Descriptive Analysis to Support or Rule Out Explanations 13 Box 9. An example of the Complexity of Describing Constructs 20
Descriptive statistics summarise and organise characteristics of a data set. A data set is a collection of responses or observations from a sample or entire population . In quantitative research , after collecting data, the first step of statistical analysis is to describe characteristics of the responses, such as the average of one variable (e ...
Qualitative description (QD) is a term that is widely used to describe qualitative studies of health care and nursing‐related phenomena. However, limited discussions regarding QD are found in the existing literature. In this systematic review, we identified characteristics of methods and findings reported in research articles published in 2014 whose authors identified the work as QD. After ...
Researchers must utilize exploratory data techniques to present findings to a target audience and create appropriate graphs and figures. Researchers can determine if outliers exist, data are missing, and statistical assumptions will be upheld by understanding data. Additionally, it is essential to comprehend these data when describing them in conclusions of a paper, in a meeting with ...
Qualitative research involves collecting and analyzing non-numerical data (e.g., text, video, or audio) to understand concepts, opinions, or experiences. It can be used to gather in-depth insights into a problem or generate new ideas for research. Qualitative research is the opposite of quantitative research, which involves collecting and ...
Chapter 4 introduces the typology of data, where quantitative data indicate numeric data and qualitative data are those non-numeric. In general, data can be measured at four levels: nominal, ordinal, interval, and ratio, where ratio data are those that can be calculated and manipulated in any mathematical formulas and equations, and nominal data have the most restriction in terms of ...
Descriptive statistics can be useful for two purposes: 1) to provide basic information about variables in a dataset and 2) to highlight potential relationships between variables. The three most common descriptive statistics can be displayed graphically or pictorially and are measures of: Graphical/Pictorial Methods. Measures of Central Tendency.
Abstract. This short "snippet" covers three important aspects related to statistics - the concept of variables, the importance, and practical aspects related to descriptive statistics and issues related to sampling - types of sampling and sample size estimation. Keywords: Biostatistics, descriptive statistics, sample size, variables.
Doyle L, McCabe C, Keogh B, Brady A, McCann M. An overview of the qualitative descriptive design within nursing research. J Res Nurs. 2020;25(5):443-55. Article PubMed Google Scholar Siedlecki SL. Understanding descriptive research designs and methods. Clin Nurse Spec. 2020;34(1):8-12.
Qualitative research is a type of research that explores and provides deeper insights into real-world problems.[1] Instead of collecting numerical data points or intervene or introduce treatments just like in quantitative research, qualitative research helps generate hypotheses as well as further investigate and understand quantitative data. Qualitative research gathers participants ...