
- SUGGESTED TOPICS
- The Magazine
- Newsletters
- Managing Yourself
- Managing Teams
- Work-life Balance
- The Big Idea
- Data & Visuals
- Reading Lists
- Case Selections
- HBR Learning
- Topic Feeds
- Account Settings
- Email Preferences
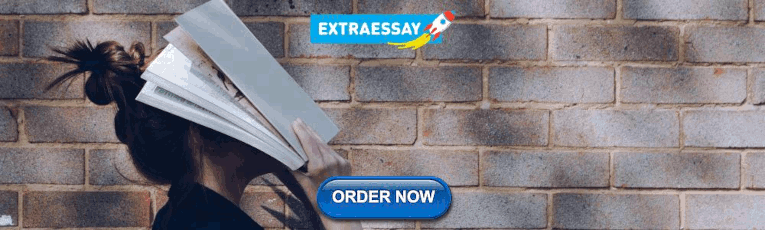
Don’t Underestimate the Power of Your Voice
- Dan Bullock
- Raúl Sánchez

It’s not just what you say, it’s how you say it.
Our voices matter as much as our words matter. They have the power to awaken the senses and lead others to act, close deals, or land us successful job interviews. Through our voices, we create nuances of meaning, convey our emotions, and find the secret to communicating our executive presence. So, how do we train our voices to be more visceral, effective, and command attention?
- The key lies in harnessing our voices using the principles of vocalics. Vocalics primarily consists of three linguistic elements: stress (volume) , intonation (rising and falling tone), and rhythm (pacing). By combining vocalics with public speaking skills, we can colors our words with the meaning and emotion that motivates others to act.
- Crank up your volume: No, we don’t mean shout. The effective use of volume goes beyond trying to be the loudest person in the room. To direct the flow of any conversation, you must overtly stress what linguists call focus words. When you intentionally place volume on certain words, you emphasize parts of a message and shift the direction of a conversation toward your preferred outcome.
- Use a powerful speech style: The key to achieving a powerful speech style, particularly during job interviews and hiring decisions, is to first concentrate on the “melody” of your voice, also called intonation. This rise or fall of our voice conveys grammatical meaning (questions or statements) or even attitude (surprise, joy, sarcasm).
- Calibrate your vocal rhythm with the right melody: Our messages are perceived differently depending on the way we use rhythm in our voices. Deliberately varying our pacing with compelling pauses creates “voiced” punctuation, a powerful way to hold the pulse of the moment.
[derivative id="274848" style="inline"]
- Dan Bullock is a language and communications specialist/trainer at the United Nations Secretariat, training diplomats and global UN staff. Dan is the co-author of How to Communicate Effectively with Anyone, Anywhere (Career Press, 2021). He also serves as faculty teaching business communication, linguistics, and public relations within the Division of Programs in Business at New York University’s School of Professional Studies. Dan was the director of corporate communications at a leading NYC public relations firm, and his corporate clients have included TD Bank and Pfizer.
- Raúl Sánchez is an award-winning clinical assistant professor and the corporate program coordinator at New York University’s School of Professional Studies. Raúl is the co-author of How to Communicate Effectively with Anyone, Anywhere (Career Press, 2021). He has designed and delivered corporate trainings for Deloitte and the United Nations, as well as been a writing consultant for Barnes & Noble Press and PBS. Raúl was awarded the NYU School of Professional Studies Teaching Excellence Award and specializes in linguistics and business communication.
Partner Center
The power of ‘voice,’ and empowering the voiceless
- Search Search
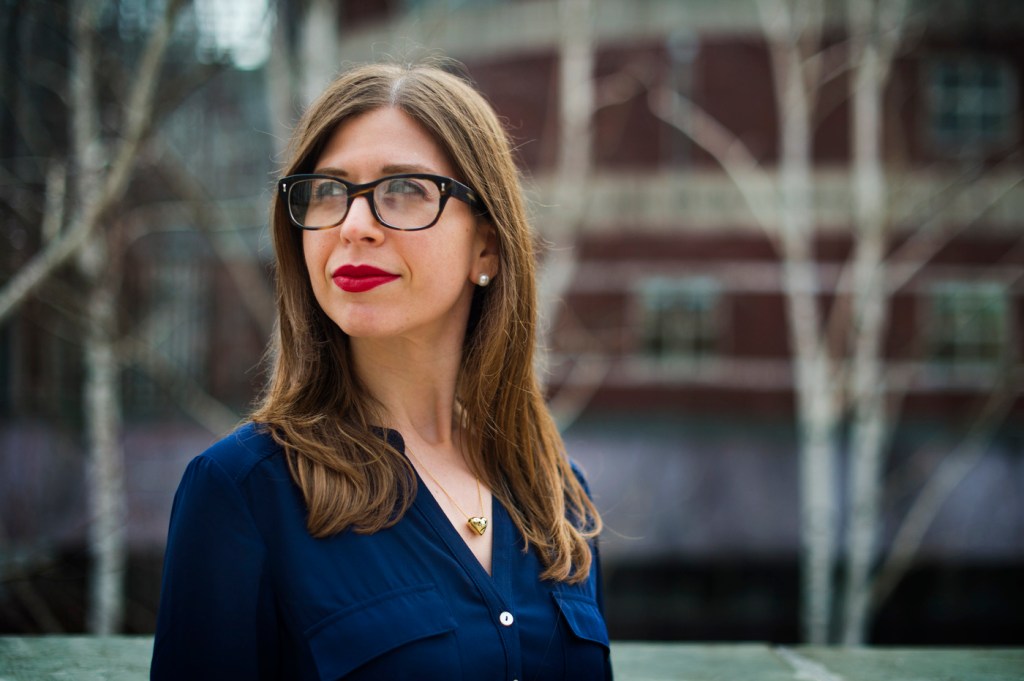
Many people use their voices everyday—to talk to people, to communicate their needs and wants—but the idea of ‘voice’ goes much deeper. Having a voice gives an individual agency and power, and a way to express his or her beliefs. But what happens when that voice is expressed differently from the norm? What happens when that voice is in some way silenced?

Meryl Alper, assistant professor of communication studies at Northeastern, explored this idea of “voice” in children and young teenagers who used an iPad app that converted symbols to audible words to help them communicate.
While it may seem like the app helped to return voice to those who used it, Alper found that the technology was subject to economic structures and defined through the lens of ableism.
“People with disabilities are not passively given voices by the able-bodied; disabled individuals, rather, are actively taking and making them,” she said.
Her book on the subject, Giving Voice: Mobile Communication, Disability, and Inequality , was recently recognized by the Association of American Publishers’ PROSE Awards, which honor “the very best in professional and scholarly publishing.”
We often hear about technology giving voice to the voiceless. What does ‘voice’ represent in your research? And what sorts of ‘voices’ are left out of technological advances?
“Giving voice to the voiceless” regularly signifies that the historically underrepresented, disadvantaged, or vulnerable gain opportunities to organize, increase visibility, and express themselves by leveraging the strengths of information, media, and communication technologies. A long list of tools and platforms—including the internet, Facebook, Twitter, community radio, and free and open software—have all been said to “give voice.”
In the book, I critically reflect on how “giving voice to the voiceless” becomes a powerful, and potentially harmful, trope in our society that masks structural inequalities. I do this by considering the separate meanings of “giving,” “voice,” and “the voiceless.” The notion of “the voiceless” suggests a static and clearly defined group. Discussions about “giving” them voice can reinforce and naturalize not “having” a voice, without also questioning the complex dynamics between having and giving, as well as speaking and listening. Additionally, “giving voice” does not challenge the means and methods by which voice may have been obtained, taken, or even stolen in the first place, and how technology and technological infrastructure can and does uphold the status quo.
What were the biggest takeaways from your research?
I studied how non- and minimally-speaking youth with developmental disabilities impacting their speech used voice output communication technologies that take the form of mobile tablets and apps—think of the technology used by the late Stephen Hawking, but simplified on an iPad. The impact of these technologies on the lives of these children and their families was at once positive, negative, and sometimes of little impact at all. We are collectively responsible for how overly simplistic narratives about technology metaphorically and materially “giving voice” to those with disabilities circulate, particularly as social media platforms monetize and incentivize clicks and retweets of stories. These kinds of news and media portrayals are derided among many in the disability community as “inspiration porn.” In economically, politically, and socially uncertain times, certainty in technology as a fix, certainty in disability as something in need of fixing, and the relationship between these certain fixations is something to think very critically about.
We also need to stay vigilant about protecting disability rights and improving disability policy, as well as the policies that acutely impact people with disabilities, such as education, healthcare, and internet access. Having a voice in general, and the role of technology in exploiting that voice, must be understood in relation to other forms of exploitation. People with disabilities are not passively given voices by the able-bodied; individuals with disabilities, rather, are actively taking and making them. Considering all the ways in which our media ecology and political environment are rapidly changing, at stake in these matters is not only which voices get to speak, but who is thought to have agency to speak in the first place.
Giving Voice received an honorable mention from the PROSE Awards. What does this honor mean to you and for your work?
It is a great privilege for my book to be counted among the 2018 honorees and as one of two winners in the Media and Cultural Studies category, as hundreds of exceptional books were published in the discipline in 2017. Media, communication, and cultural studies is a wide and vibrant field, encompassing two different departments at Northeastern alone (communication studies, and media and screen studies). As an assistant professor, it is immensely rewarding and affirming for my work to be considered of a similar caliber to past category winners, including acclaimed senior scholars in my field.
The award also makes a clear statement about the future of the discipline. Giving Voice is broadly about what it means to have a voice in a technologized world and is based on qualitative research among children, families, and people with disabilities. Those populations, and their concerns, are more often than not treated as niche or specialty within the academy. Qualitative research is also regularly undervalued compared to quantitative research. The honor motivates me to keep following my instincts, centering marginalized groups in empirical and theoretical work on technology and society, and posing research questions that excite me.
Editor's Picks
What’s in a name a linguistics expert explains why some baby names dominate the charts year after year, more researchers are need to rid the internet of harmful material, uk communications boss says at northeastern conference, northeastern’s barnett institute of chemical and biological analysis celebrates 50th anniversary by looking to the future, northeastern’s vancouver campus celebrates lifelong learning and opportunity to thrive during convocation, ticks carry more than lyme disease. here’s what you need to know about babesiosis and powassan, featured stories, our brains trick us into thinking consciousness can reside outside the body, new northeastern research proves, raising awareness about global health issues and developing therapeutics is the mission of knight-hennessy scholarship recipient, what is net neutrality and why it’s vital to the future of the internet, northeastern computer scientist explains, how the man behind the uk’s answer to silicon valley found a home at northeastern’s london startup hub, science & technology.
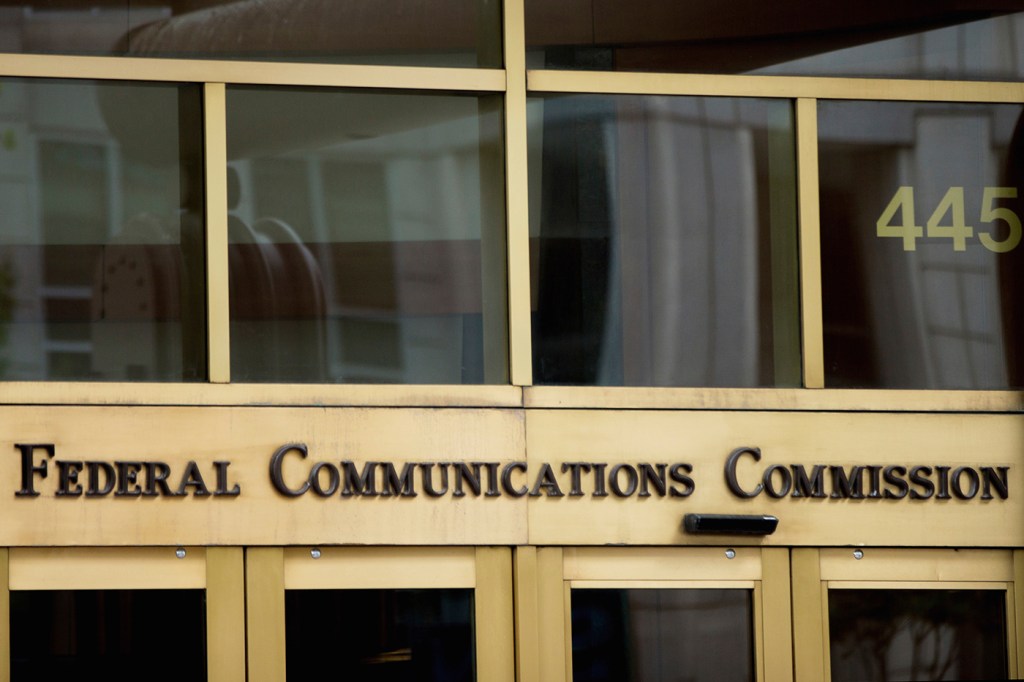
Recent Stories
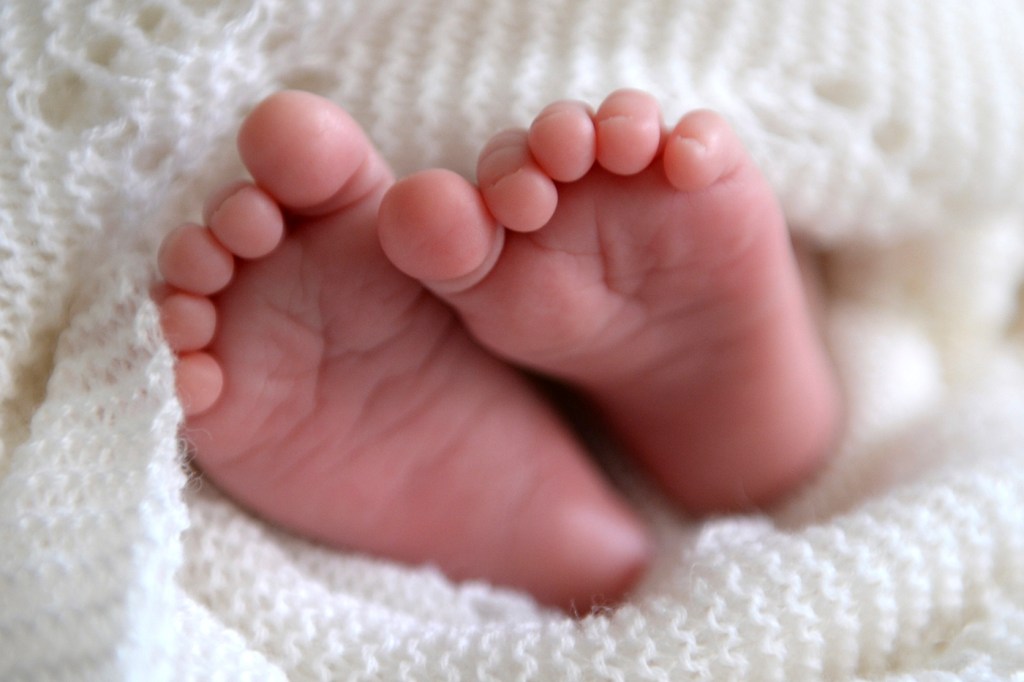
The Power of Using your voice
A voice is a tool that transports us into the future. A future that has more possibilities and more solutions. A voice is a tool that can be used for standing up for what is right, rather than what is easy. A voice gives your opinions a platform, and gifts you with the opportunity to have perspective and knowledge on things that matter. No two voices are the same, each voice has something different to say. And in a world that needs to represent freedom and democracy, a voice is a powerful symbol of this. It is what has allowed people to protest injustice, to sing for freedom, or simply speak the truth. A voice can be a source of hope in difficult times.
Using your voice for the truth is important to create a better world. Everyone’s voice matters. It is important to not let yourself become silenced, because when a voice is not used it prevents the opportunity for a true democracy where each voice is valued in a peaceful manner. Voices convey passion and excitement; voices can convey anything, whether it’s a feeling, a place, or an idea. In a way, voices are a superpower if you know how to use it.
Voices can be used to create change. People can take anything material from you, but your voice is one of the things that cannot be taken away. Voices are meant to encourage other voices too, to unite and support each other. One of the most powerful things someone can do is to use their voice.
View the discussion thread.
Related Stories

Why voices need to be heard

You(th) are the future.
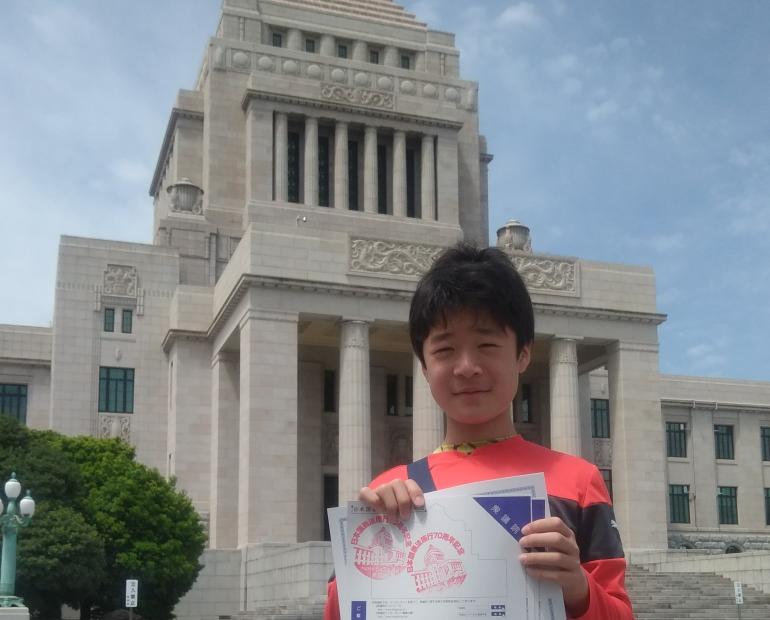
The Future of Youth Activism: How Our Votes can Uplift our Society for Generations
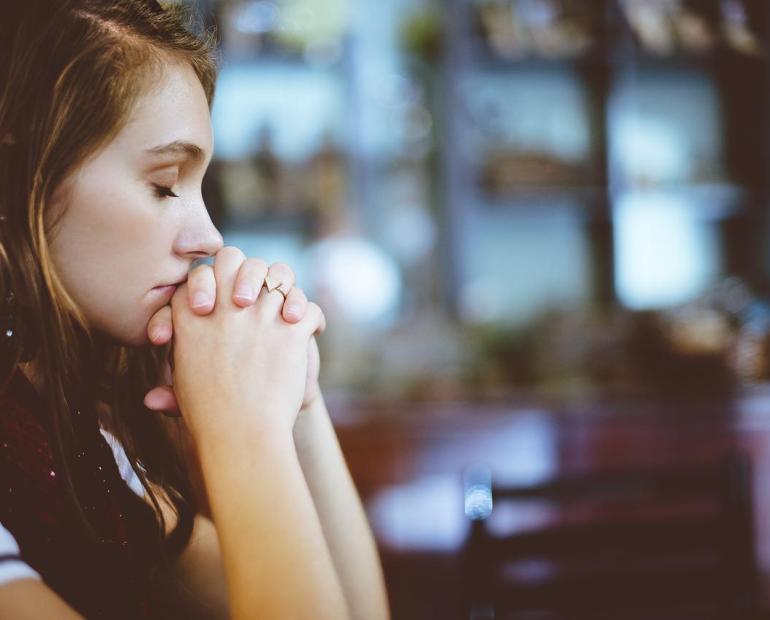
Mental Health during an Economic Crisis
C 2019 Voices of Youth. All Rights Reserved.
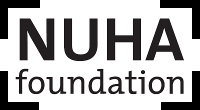
- Mission Statement
- Our History
- A few of our favourite quotes
- Young Writers
- Matched Prizes
- Frequently Asked Questions
- Blogging Entries
- Other Blog Posts
- Country Resource Pages on Education
- Alliance for Development and Population Services
- Alternatives Durables pour le Development
- Health and Education NOW!
- Green Village Children Centre
- Future Foundations
- Educate A Child International
- ComplitKenya
- Club des Amis du Cameroun (CAMIC)
- Canada-Mathare Education Trust (CMETrust)
- Busoga Volunteers for Community Development
- Bamburi Great News School
- Angelic Army School
- Akili Girls’ Preparatory School
- Wamulu International
- World Action Fund
- English Conversation Programme (ECP)
- Trivia about NUHA
The Power of the Human Voice
Posted on August 8, 2014 by the Editor
It takes the human voice to infuse words with shades of deeper meaning. The role of the human voice in giving deeper meaning to words is crucial when one looks at the significance of denotative and connotative meanings of expressions. For example, one person can utter the following words: l am thirsty . The surface or general meaning is that the person needs some water. However, depending on the context of the utterance, in terms of the reason for such expression, the role and position of the speaker-on a deeper or connotative basis the same words could mean: Give me some water now! In which case: I am thirsty would galvanise the person receiving the order to fetch water as quickly as humanly possible.
The human voice is able to infuse words with shades of deeper meaning because that power of speech can unearth the real intentions, mood, character, identity and culture of the speaker in question. It is easy for a person to write down something and mislead his or her audience or the entire world. However, once one has an opportunity to physically interact with and listen to the person`s voice- the real emotional, physical and cultural elements of the speaker can be easily picked up and placed in their right perspective. By the same token, actors, educators, editors, politicians, religious leaders, advertisers, insurance agents, singers, writers, inspirational speakers suffuse their voices with certain words to successfully appeal to their audiences.
Verbal communication is unique to humans. Human beings are emotional creatures. The human voice is thought to convey emotional valence, arousal and intensity. Music is a powerful medium capable of eliciting a broad range of emotions. The ability to detect emotion in speech and music is an important task in our daily lives. Studies have been conducted to determine why and how music is able to influence its listeners’ moods and emotions. Results showed that melodies with the voice were better recognised than all other instrumental melodies. The authors suggest that the biological significance of the human voice provides a greater depth of processing and enhanced memory.
Think about a normal day in one’s life. How many words does a person speak? How many words do you hear? According to Caleb Lott in the article titled: The Power of the Human Voice , while there are several different numbers floating around, an average human speaks a minimum of 7000 words every day. The same writer goes on to say that the human voice is a tremendous asset which can be used to make the ordinary extraordinary. For example, the games Thomas Was Alone and Bastion use the human voice in a unique way that dynamically affects the players’ experiences of the games. This is so because a narrative-focused game is not only a powerful and amazing way to tell the story but also does so in a way that the visuals cannot convey. The writing is amazing, but without the awe-inspiring narration, the impact of the writing would be lessened.
The human voice is an amazing tool that can have a profound effect on video games. Using a narrator affects the gameplay and the experience the player remembers after walking away from the game. Think of being held in awe, listening to the radio where the mellifluous voices of one`s favourite program’s hosts awaken, mesmerise, excite or sooth one. This boils down to the fact that our visceral reactions to the ways people play form an integral part of our interactions and communication. Annie Tucker Morgan in Talk to Me: The Powerful Effects of the Human Voice says there is a reason why many people’s first instinct when they are upset is to call their mother. Mother’s love is not only enduring but it is something strong that a person finds echoing instinctively and emotionally. She goes on to explain how a University of Wisconsin -Madison study has identified a concrete link between the sound of Mom’s voice and the soothing of jangled nerves through the release of stress-relieving oxytocin -also known as the “love hormone” in the brain. Researchers say that women prefer deep male voices on the condition that those voices are saying complementary things, but also that a woman’s particular preference for the pitch of a male voice depends on the pitch of her own. Jeffrey Jacob, founder and president of Persuasive Speaking highlighted the correlation between people’s voices and their professional and personal successes. A study conducted showed that if the other person does not like the sound of one’s voice, one might have a hard time securing his or her approval.
If we do not verbalise we write down things. Is writing not something of great magnificence? If so, why can we not make a difference?
The world has never been static, so has writing. It is dynamic. It makes the world revel and reveal itself. Out went the traditional writing feather or pen, and in surged the typewriter, then the “wise” computer. Kudos, the world crooned in celebration of probably one of civilization’s amazing conquest and result.
However, this does not mean that the pen is down and out. Not at all. Neither does it mean that the pen has ceased to be mightier than the sword. Writing is writing whether by virtue of the might of the pen or the wizardry of the computer. In verbal communication one can detect the power of the human voice and the mood of the speaker through such elements of speech as intonation, speed, pause, pitch and emphasis. In the written text, register and paragraphing (for example through the use exclamations) can help detect the speaker’s intentions and emotions.
Different words mean different things to different people. How do writers hold the attention of readers? Through the beauty of words, story-telling helps us derive entertainment from reading, escape from an onerous or anxious life, and of course, understand more about of the world. Through words writers create plots that are not devoid of suspense and mystery. Watts in Writing A Novel says, “A plot is like a knitted sweater-only as good as the stitches. Without the links we have a tangle of wool, chaotic and uninteresting. We get immersed in reading because of the power of causality, the power of words. Words play a crucial role in creating a work of art like a novel. Watts in Writing A Novel says a good answer to a narrative question is as satisfying as scratching an itch.
Through writing we find courage, ammunition and inspiration to go on, in spite of all the odds, we find vision to define and refine our identities and destinies. Yes, through writing we find ourselves, our voice and verve.
J.D. Salinger came up with an interesting observation. He said “What really knocks me out is a book that, when you’re all done reading it, you wish the author that wrote it was a terrific friend of yours and you could call him up on the phone whenever you felt like it. That doesn’t happen much, though.” Are you not ready to knock many a reader out? Are you not ready to unleash your greatness? How many writers are sitting on their works of art?
Writers and words are good bedfellows. Pass that word. Maya Angelou, the famous author of I Know Why the Caged Bird Sings says “Words mean more than what is set down on paper. It takes the human voice to infuse them with shades of deeper meaning.” A word is a unit of expression which is intertwined with sight, sound, smell, touch, and body movement. I think it is memorable (and obviously powerful) because it appeals to our physical, emotional and intellectual processes. As language practitioners, this knowledge (of the mental schema) is crucial.
What is in a word? For me, words illuminate, revel and reveal the world. Literature is literature because of words that constitute it. Patrick Rothfuss says, “Words are pale shadows of forgotten names. As names have power, words have power. Words can light fires in the minds of men. Words can wring tears from the hardest hearts.” Yet, Rudyard Kipling claims, “Words are, of course, the most powerful drug used by mankind” I think this is a very interesting observation.
Patrick Rothfuss illustrates this by declaring, “Words are pale shadows of forgotten names. As names have power, words have power. Words can light fires in the minds of men. Words can wring tears from the hardest hearts.”
The beauty of literature is in seeking and gaining an insight into the complexity and diversity of life through the analysis of how the human voice infuses words with shades of deeper meaning. For indeed the dynamic human voice can roar, soar and breathe life into different pregnant clouds of words and meanings.
14 comments on “ The Power of the Human Voice ”
Powerful essay, indeed the human voice has power to articulate emotions, ideas, perception, convictions and so much more and by so doing, breathing life into words.
Henry, thank you for your great words of encouragement.
Wonderful! Spoken words externalise how the speaker perceive the world, how the speaker feels inside…..
Francisco, thank you for stopping by!
Indeed what a wonderful piece of literature,It reminds me of my secondary education days back in the early 1980s when I did “ANIMAL FARM ” by Charles Dickens.
Mr. Mlotshwa, thank you for stopping by. Much appreciated.
Speechless! the language in this piece is just amazing.Well done Mr Ndaba
Khalaz, thank you!
this is a very nice and awesome essay. Great job! 😀
Musa, many thanks!
Ndaba is a compelling writer. An informative piec
Claire, thank you. Humbled.
Wow. This is very excellent, well-written,powerful and informative. You are a great writer. Keep writing.
Tshego, thank you for your kind words!
Leave a Reply Cancel reply
Your email address will not be published. Required fields are marked *
Save my name, email, and website in this browser for the next time I comment.
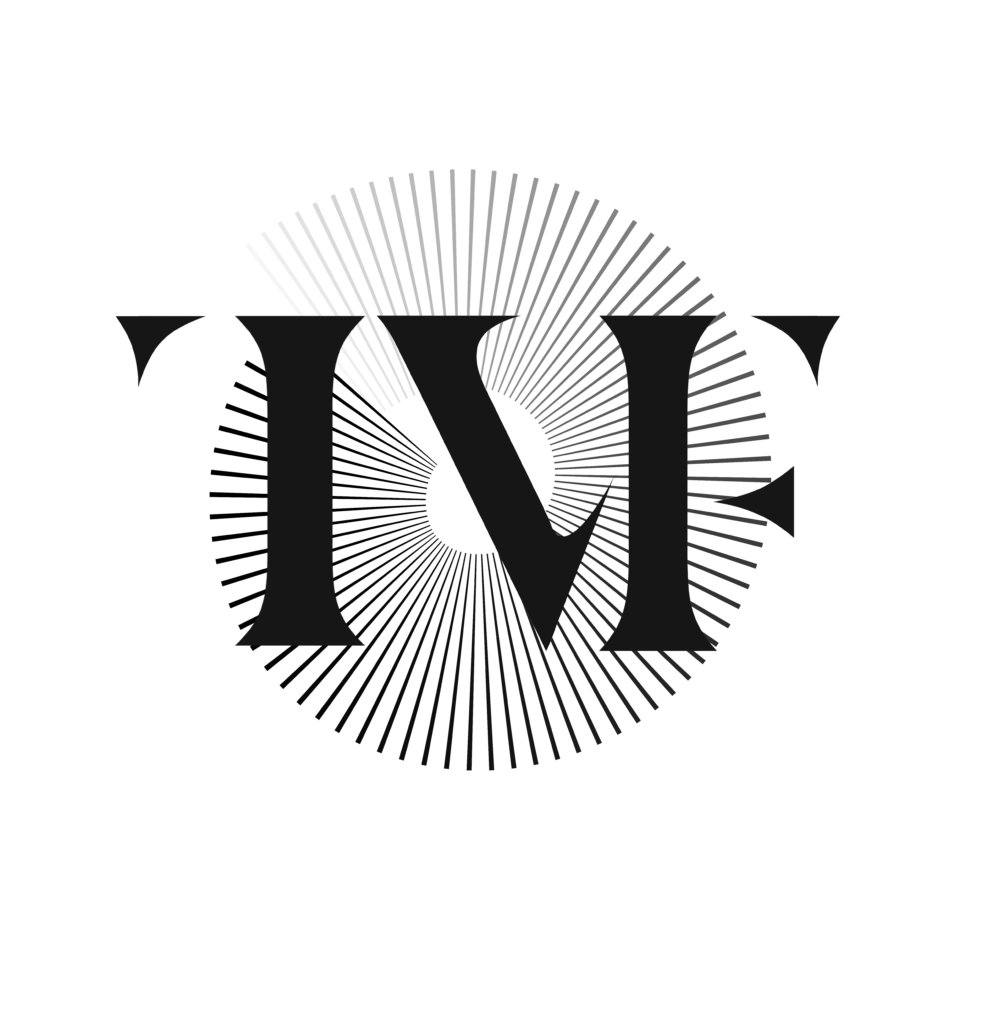
The Voice Foundation
Advancing understanding of the voice through interdisciplinary research & education , philadelphia, new york, los angeles, cleveland, boston, paris, lebanon, brazil, china, japan, india , mexico.
Anatomy and Physiology of Voice Production | Understanding How Voice is Produced | Learning About the Voice Mechanism | How Breakdowns Result in Voice Disorders
Larynx Highly specialized structure atop the windpipe responsible for sound production, air passage during breathing and protecting the airway during swallowing
Vocal Folds (also called Vocal Cords) “Fold-like” soft tissue that is the main vibratory component of the voice box; comprised of a cover (epithelium and superficial lamina propria), vocal ligament (intermediate and deep laminae propria), and body (thyroarytenoid muscle)
Glottis (also called Rima Glottides) Opening between the two vocal folds; the glottis opens during breathing and closes during swallowing and sound production
Voice as We Know It = Voiced Sound + Resonance + Articulation
The “spoken word” results from three components of voice production: voiced sound, resonance, and articulation.
Voiced sound: The basic sound produced by vocal fold vibration is called “voiced sound.” This is frequently described as a “buzzy” sound. Voiced sound for singing differs significantly from voiced sound for speech.
Resonance: Voiced sound is amplified and modified by the vocal tract resonators (the throat, mouth cavity, and nasal passages). The resonators produce a person’s recognizable voice.
Articulation: The vocal tract articulators (the tongue, soft palate, and lips) modify the voiced sound. The articulators produce recognizable words.
Voice Depends on Vocal Fold Vibration and Resonance
Sound is produced when aerodynamic phenomena cause vocal folds to vibrate rapidly in a sequence of vibratory cycles with a speed of about:
- 110 cycles per second or Hz (men) = lower pitch
- 180 to 220 cycles per second (women) = medium pitch
- 300 cycles per second (children) = higher pitchhigher voice: increase in frequency of vocal fold vibrationlouder voice: increase in amplitude of vocal fold vibration
Vibratory Cycle = Open + Close Phase
The vocal fold vibratory cycle has phases that include an orderly sequence of opening and closing the top and bottom of the vocal folds, letting short puffs of air through at high speed. Air pressure is converted into sound waves.
Not Like a Guitar String
Vocal folds vibrate when excited by aerodynamic phenomena; they are not plucked like a guitar string. Air pressure from the lungs controls the open phase. The passing air column creates a trailing “Bernoulli effect,” which controls the close phase.
Voice production involves a three-step process.
- A column of air pressure is moved towards the vocal folds
- Air is moved out of the lungs and towards the vocal folds by coordinated action of the diaphragm, abdominal muscles, chest muscles, and rib cage
- Vocal folds are moved to midline by voice box muscles, nerves, and cartilages
- Column of air pressure opens bottom of vocal folds
- Column of air continues to move upwards, now towards the top of vocal folds, and opens the top
- The low pressure created behind the fast-moving air column produces a “Bernoulli effect” which causes the bottom to close, followed by the top
- Closure of the vocal folds cuts off the air column and releases a pulse of air
- New cycle repeats
- Loudness: Increase in air flow “blows” vocal folds wider apart, which stay apart longer during a vibratory cycle – thus increasing amplitude of the sound pressure wave
- Pitch: Increase in frequency of vocal fold vibration raises pitch

– repeat 1-10 In the closed position (—) maintained by muscle, opens and closes in a cyclical, ordered and even manner (1 – 10) as a column of air pressure from the lungs below flows through. This very rapid ordered closing and opening produced by the column of air is referred to as the mucosal wave. The lower edge opens first (2-3) followed by the upper edge thus letting air flow through (4-6). The air column that flows through creates a “Bernouli effect” which causes the lower edge to close (7-9) as it escapes upwards. The escaping “puffs of air” (10) are converted to sound which is then transformed into voice by vocal tract resonators. Any change that affects this mucosal wave – stiffness of vocal fold layers, weakness or failure of closure, imbalance between R and L vocal folds from a lesion on one vocal fold – causes voice problems. (For more information, see Anatomy: How Breakdowns Result in Voice Disorders .)
- Vocal tract – resonators and articulators: The nose, pharynx, and mouth amplify and modify sound, allowing it to take on the distinctive qualities of voiceThe way that voice is produced is analogous to the way that sound is produced by a trombone. The trombone player produces sound at the mouthpiece of the instrument with his lips vibrating from air that passes from the mouth. The vibration within the mouthpiece produces sound, which is then altered or “shaped” as it passes throughout the instrument. As the slide of the trombone is changed, the sound of the musical instrument is similarly changed.
Amazing Outcomes of Human Voice
The human voice can be modified in many ways. Consider the spectrum of sounds – whispering, speaking, orating, shouting – as well as the different sounds that are possible in different forms of vocal music, such as rock singing, gospel singing, and opera singing.
Key Factors for Normal Vocal Fold Vibration
To vibrate efficiently vocal folds need to be:
At the midline or “closed”: Failure to move vocal folds to the midline, or any lesion which prevents the vocal fold edges from meeting, allows air to escape and results in breathy voice.Key players: muscles, cartilages, nerves
Pliable: The natural “built-in” elasticity of vocal folds makes them pliable. The top, edge, and bottom of the vocal folds that meet in the midline and vibrate need to be pliable. Changes in vocal fold pliability, even if limited to just one region or “spot,” can cause voice disorders, as seen in vocal fold scarring.Key players: epithelium, superficial lamina propria
“Just right” tension: Inability to adjust tension during singing can cause a failure to reach high notes or breaks in voice.Key players: muscle, nerve, cartilages
“Just right” mass: Changes in the soft tissue bulk of the vocal folds – such as decrease or thinning as in scarring or increase or swelling, as in Reinke’s edema, produce many voice symptoms – hoarseness, altered voice pitch, effortful phonation, etc. (For more information, see Vocal Fold Scarring and Reinke’s Edema .)Key players: muscles, nerves, epithelium, superficial lamina propria
Learning About the Voice Mechanism
Greater Good Science Center • Magazine • In Action • In Education
The Human Voice Can Communicate 24 Emotions
Ooh, surprise! Those spontaneous sounds we make to express everything from elation (woohoo) to embarrassment (oops) say a lot more about what we’re feeling than previously understood, according to new UC Berkeley research.
Proving that a sigh is not just a sigh, scientists conducted a statistical analysis of listener responses to more than 2,000 nonverbal exclamations known as “vocal bursts” and found they convey at least 24 kinds of emotion. Previous studies of vocal bursts set the number of recognizable emotions closer to 13.
The results, recently published online in the American Psychologist journal, are demonstrated in vivid sound and color on the first-ever interactive audio map of nonverbal vocal communication.
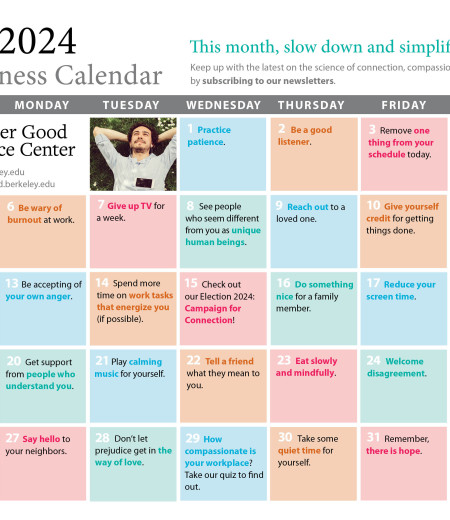
“This study is the most extensive demonstration of our rich emotional vocal repertoire, involving brief signals of upwards of two dozen emotions as intriguing as awe, adoration, interest, sympathy, and embarrassment,” said study senior author Dacher Keltner, a psychology professor at UC Berkeley and faculty director of the Greater Good Science Center, which helped support the research.
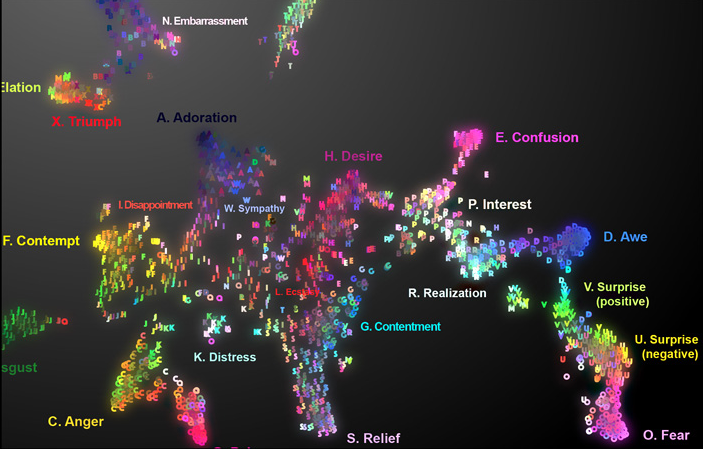
For millions of years, humans have used wordless vocalizations to communicate feelings that can be decoded in a matter of seconds, as this latest study demonstrates.
“Our findings show that the voice is a much more powerful tool for expressing emotion than previously assumed,” said study lead author Alan Cowen, a Ph.D. student in psychology at UC Berkeley.
On Cowen’s audio map, one can slide one’s cursor across the emotional topography and hover over fear (scream), then surprise (gasp), then awe (woah), realization (ohhh), interest (ah?), and finally confusion (huh?).
Among other applications, the map can be used to help teach voice-controlled digital assistants and other robotic devices to better recognize human emotions based on the sounds we make, he said.
As for clinical uses, the map could theoretically guide medical professionals and researchers working with people with dementia, autism, and other emotional processing disorders to zero in on specific emotion-related deficits.
“It lays out the different vocal emotions that someone with a disorder might have difficulty understanding,” Cowen said. “For example, you might want to sample the sounds to see if the patient is recognizing nuanced differences between, say, awe and confusion.”
Though limited to U.S. responses, the study suggests humans are so keenly attuned to nonverbal signals—such as the bonding “coos” between parents and infants—that we can pick up on the subtle differences between surprise and alarm, or an amused laugh versus an embarrassed laugh.
For example, by placing the cursor in the embarrassment region of the map, you might find a vocalization that is recognized as a mix of amusement, embarrassment, and positive surprise.
“A tour through amusement reveals the rich vocabulary of laughter, and a spin through the sounds of adoration, sympathy, ecstasy, and desire may tell you more about romantic life than you might expect,” said Keltner.
How they conducted the study
Researchers recorded more than 2,000 vocal bursts from 56 male and female professional actors and non-actors from the United States, India, Kenya, and Singapore by asking them to respond to emotionally evocative scenarios.
Next, more than 1,000 adults recruited online listened to the vocal bursts and evaluated them based on the emotions and meaning they conveyed and whether the tone was positive or negative, among several other characteristics.
A statistical analysis of their responses found that the vocal bursts fit into at least two dozen distinct categories, including amusement, anger, awe, confusion, contempt, contentment, desire, disappointment, disgust, distress, ecstasy, elation, embarrassment, fear, interest, pain, realization, relief, sadness, surprise (positive), surprise (negative), sympathy, and triumph.
For the second part of the study, researchers sought to present real-world contexts for the vocal bursts. They did this by sampling YouTube video clips that would evoke the 24 emotions established in the first part of the study, such as babies falling, puppies being hugged, and spellbinding magic tricks.
This time, 88 adults of all ages judged the vocal bursts extracted from YouTube videos. Again, the researchers were able to categorize their responses into 24 shades of emotion. The full set of data were then organized into a semantic space on an interactive map.
“These results show that emotional expressions color our social interactions with spirited declarations of our inner feelings that are difficult to fake, and that our friends, coworkers, and loved ones rely on to decipher our true commitments,” Cowen said.
This article was originally published on Berkeley News . Read the original article .
About the Author
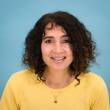
Yasmin Anwar
Yasmin Anwar is a Media Relations Representative at UC Berkeley.
You May Also Enjoy
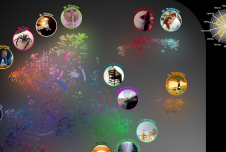
How Many Different Human Emotions Are There?
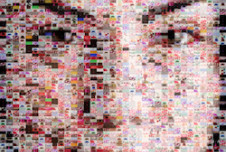
Do Feelings Look the Same in Every Human Face?
Are facial expressions universal.
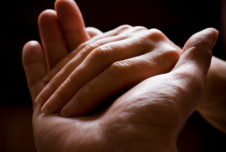
Hands On Research: The Science of Touch
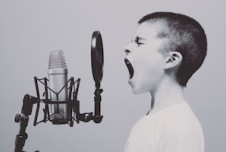
Does Your Voice Reveal More Emotion Than Your Face?
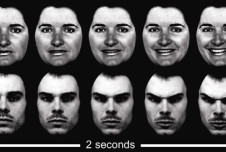
Are Emotions Born or Made?

The Human Voice in Speech and Singing
- Reference work entry
- Cite this reference work entry
- Björn Lindblom Prof. 2 &
- Johan Sundberg 3
Part of the book series: Springer Handbooks ((SHB))
10k Accesses
9 Citations
3 Altmetric
This chapter describes various aspects of the human voice as a means of communication in speech and singing. From the point of view of function, vocal sounds can be regarded as the end result of a three stage process: (1) the compression of air in the respiratory system, which produces an exhalatory airstream, (2) the vibrating vocal foldsʼ transformation of this air stream to an intermittent or pulsating air stream, which is a complex tone, referred to as the voice source, and (3) the filtering of this complex tone in the vocal tract resonator. The main function of the respiratory system is to generate an overpressure of air under the glottis, or a subglottal pressure. Section 16.1 describes different aspects of the respiratory system of significance to speech and singing, including lung volume ranges, subglottal pressures, and how this pressure is affected by the ever-varying recoil forces. The complex tone generated when the air stream from the lungs passes the vibrating vocal folds can be varied in at least three dimensions: fundamental frequency, amplitude and spectrum. Section 16.2 describes how these properties of the voice source are affected by the subglottal pressure, the length and stiffness of the vocal folds and how firmly the vocal folds are adducted. Section 16.3 gives an account of the vocal tract filter, how its form determines the frequencies of its resonances, and Sect. 16.4 gives an account for how these resonance frequencies or formants shape the vocal sounds by imposing spectrum peaks separated by spectrum valleys, and how the frequencies of these peaks determine vowel and voice qualities. The remaining sections of the chapter describe various aspects of the acoustic signals used for vocal communication in speech and singing. The syllable structure is discussed in Sect. 16.5 , the closely related aspects of rhythmicity and timing in speech and singing is described in Sect. 16.6 , and pitch and rhythm aspects in Sect. 16.7 . The impressive control of all these acoustic characteristics of vocal signals is discussed in Sect. 16.8 , while Sect. 16.9 considers expressive aspects of vocal communication.
This is a preview of subscription content, log in via an institution to check access.
Access this chapter
- Available as PDF
- Read on any device
- Instant download
- Own it forever
- Available as EPUB and PDF
Tax calculation will be finalised at checkout
Purchases are for personal use only
Institutional subscriptions
Abbreviations
articulation class
long-term-average spectra
maximum flow declination rate
magnetic resonance imaging
resting expiratory level
sound pressure level
speech transmission index
total lung capacity
vital capacity
T.J. Hixon: Respiratory Function in Speech and Song (Singular, San Diego 1991) pp. 1–54
Google Scholar
A. L. Winkworth, P. J. Davis, E. Ellis, R. D. Adams: Variability and consistency in speech breathing during reading: Lung volumes, speech intensity, and linguistic factors, JSHR 37 , 535–556 (1994)
M. Thomasson: From Air to Aria , Ph.D. Thesis (Music Acoustics, KTH 2003)
B. Conrad, P. Schönle: Speech and respiration, Arch. Psychiat. Nervenkr. 226 , 251–268 (1979)
M.H. Draper, P. Ladefoged, D. Whitteridge: Respiratory muscles in speech, J. Speech Hear. Disord. 2 , 16–27 (1959)
R. Netsell: Speech physiology. In: Normal aspects of speech, hearing, and language , ed. by P.D. Minifie, T.J. Hixon, P. Hixon, P. Williams (Prentice-Hall, Englewood Cliffs 1973) pp. 211–234
P. Ladefoged, M.H. Draper, D. Whitteridge: Syllables and stress, Misc. Phonetica 3 , 1–14 (1958)
P. Ladefoged: Speculations on the control of speech. In: A Figure of Speech: A Festschrift for John Laver , ed. by W.J. Hardcastle, J. Mackenzie Beck (Lawrence Erlbaum, Mahwah 2005) pp. 3–21
T.J. Hixon, G. Weismer: Perspectives on the Edinburgh study of speech breathing, J. Speech Hear. Disord. 38 , 42–60 (1995)
S. Nooteboom: The prosody of speech: melody and rhythm. In: The Handbook of Phonetic Sciences , ed. by W.J. Hardcastle, J. Laver (Blackwell, Oxford 1997) pp. 640–673
M. Rothenberg: The breath-stream dynamics of simple-released-plosive production Bibliotheca Phonetica 6 (Karger, Basel 1968)
D.H. Klatt, K.N. Stevens, J. Mead: Studies of articulatory activity and airflow during speech, Ann. NY Acad. Sci. 155 , 42–55 (1968)
ADS Google Scholar
J.J. Ohala: Respiratory activity in speech. In: Speech production and speech modeling , ed. by W.J. Hardcastle, A. Marchal (Dordrecht, Kluwer 1990) pp. 23–53
R.H. Stetson: Motor Phonetics: A Study of Movements in Action (North Holland, Amsterdam 1951)
P. Ladefoged: Linguistic aspects of respiratory phenomena, Ann. NY Acad. Sci. 155 , 141–151 (1968)
L.H. Kunze: Evaluation of methods of estimating sub-glottal air pressure muscles, J. Speech Hear. Disord. 7 , 151–164 (1964)
R. Leanderson, J. Sundberg, C. von Euler: Role of the diaphragmatic activity during singing: a study of transdiaphragmatic pressures, J. Appl. Physiol. 62 , 259–270 (1987)
J. Sundberg, N. Elliot, P. Gramming, L. Nord: Short-term variation of subglottal pressure for expressive purposes in singing and stage speech. A preliminary investigation, J. Voice 7 , 227–234 (1993)
J. Sundberg: Synthesis of singing, in Musica e Technologia: Industria e Cultura per lo Sviluppo del Mezzagiorno. In: Proceedings of a symposium in Venice , ed. by R. Favaro (Unicopli, Milan 1987) pp. 145–162
J. Sundberg: Synthesis of singing by rule. In: Current Directions in Computer Music Research, System Development Foundation Benchmark Series , ed. by M. Mathews, J. Pierce (MIT, Cambridge 1989), 45-55 & 401-403
J. Molinder: Akustiska och perceptuella skillnader mellan röstfacken lyrisk och dramatisk sopran, unpublished thesis work (Lund Univ. Hospital, Dept of Logopedics, Lund 1997)
T. Baer: Reflex activation of laryngeal muscles by sudden induced subglottal pressure changes, J. Acoust. Soc. Am. 65 , 1271–1275 (1979)
T. Cleveland, J. Sundberg: Acoustic analyses of three male voices of different quality. In: SMAC 83. Proceedings of the Stockholm Internat Music Acoustics Conf , Vol. 1, ed. by A. Askenfelt, S. Felicetti, E. Jansson, J. Sundberg (Roy. Sw. Acad. Music, Stockholm 1985) pp. 143–156, No. 46:1
J. Sundberg, C. Johansson, H. Willbrand, C. Ytterbergh: From sagittal distance to area, Phonetica 44 , 76–90 (1987)
I.R. Titze: Phonation threshold pressure: A missing link in glottal aerodynamics, J. Acoust. Soc. Am. 91 , 2926–2935 (1992)
I.R. Titze: Principles of Voice Production (Prentice-Hall, Englewood Cliffs 1994)
G. Fant: Acoustic theory of speech production (Mouton, The Hague 1960)
K.N. Stevens: Acoustic Phonetics (MIT, Cambridge 1998)
M. Hirano: Clinical Examination of Voice (Springer, New York 1981)
M. Rothenberg: A new inversefiltering technique for deriving the glottal air flow waveform during voicing, J. Acoust. Soc. Am. 53 , 1632–1645 (1973)
G. Fant: The voice source – Acoustic modeling. In: STL/Quart. Prog. Status Rep. 4 (Royal Inst. of Technology, Stockholm 1982) pp. 28–48
C. Gobl: The voice source in speech communication production and perception experiments involving inverse filtering and synthesis. D.Sc. thesis (Royal Inst. of Technology (KTH), Stockholm 2003)
G. Fant, J. Liljencrants, Q. Lin: A four-parameter model of glottal flow. In: STL/Quart. Prog. Status Rep. 4, Speech, Music and Hearing (Royal Inst. of Technology, Stockholm 1985) pp. 1–13
D.H. Klatt, L.C. Klatt: Analysis, synthesis and pereception of voice quality variations among female and male talkers, J. Acoust. Soc. Am. 87 (2), 820–857 (1990)
M. Ljungqvist, H. Fujisaki: A comparative study of glottal waveform models. In: Technical Report of the Institute of Electronics and Communications Engineers , Vol. EA85-58 (Institute of Electronics and Communications Engineers, Tokyo 1985) pp. 23–29
A.E. Rosenberg: Effect of glottal pulse shape on the quality of natural vowels, J. Acoust. Soc. Am. 49 , 583–598 (1971)
M. Rothenberg, R. Carlson, B. Granström, J. Lindqvist-Gauffin: A three- parameter voice source for speech synthesis. In: Proceedings of the Speech Communication Seminar 2 , ed. by G. Fant (Almqvist & Wiksell, Stockholm 1975) pp. 235–243
K. Ishizaka, J.L. Flanagan: Synthesis of voiced sounds from a two-mass model of the vocal cords, The Bell Syst. Tech. J. 52 , 1233–1268 (1972)
Liljencrants: Chapter A translating and rotating mass model of the vocal folds. In: STL/Quart. Prog. Status Rep. 1, Speech, Music and Hearing (Royal Inst. of Technology, Stockholm 1991) pp. 1–18
A. Ní Chasaide, C. Gobl: Voice source variation. In: The Handbook of Phonetic Sciences , ed. by W.J. Hardcastle, J. Laver (Blackwell, Oxford 1997) pp. 427–462
E.B. Holmberg, R.E. Hillman, J.S. Perkell: Glottal air flow and pressure measurements for loudness variation by male and female speakers, J. Acoust. Soc. Am. 84 , 511–529 (1988)
J.S. Perkell, R.E. Hillman, E.B. Holmberg: Group differences in measures of voice production and revised values of maximum airflow declination rate, J. Acoust. Soc. Am. 96 , 695–698 (1994)
J. Gauffin, J. Sundberg: Spectral correlates of glottal voice source waveform characteristics, J. Speech Hear. Res. 32 , 556–565 (1989)
J. Svec, H. Schutte, D. Miller: On pitch jumps between chest and falsetto registers in voice: Data on living and excised human larynges, J. Acoust. Soc. Am. 106 , 1523–1531 (1999)
J. Sundberg, M. Andersson, C. Hultqvist: Effects of subglottal pressure variation on professional baritone singers voice sources, J. Acoust. Soc. Am. 105 , 1965–1971 (1999)
J. Sundberg, E. Fahlstedt, A. Morell: Effects on the glottal voice source of vocal loudness variation in untrained female and male subjects, J. Acoust. Soc. Am. 117 , 879–885 (2005)
P. Sjölander, J. Sundberg: Spectrum effects of subglottal pressure variation in professional baritone singers, J. Acoust. Soc. Am. 115 , 1270–1273 (2004)
P. Branderud, H. Lundberg, J. Lander, H. Djamshidpey, I. Wäneland, D. Krull, B. Lindblom: X-ray analyses of speech: Methodological aspects, Proc. of 11th Swedish Phonetics Conference (Stockholm Univ., Stockholm 1996) pp. 168–171
B. Lindblom: A numerical model of coarticulation based on a Principal Components analysis of tongue shapes. In: Proc. 15th Int. Congress of the Phonetic Sciences , ed. by D. Recasens, M. Josep Solé, J. Romero (Universitat Autònoma de Barcelona, Barcelona 2003), CD-ROM
G.E. Peterson, H. Barney: Control methods used in a study of the vowels, J. Acoust. Soc. Am. 24 , 175–184 (1952)
Hillenbrand et al.: Acoustic characteristics of American English vowels, J. Acoust. Soc. Am. 97 (5), 3099–3111 (1995)
G. Fant: Analysis and synthesis of speech processes. In: Manual of Phonetics , ed. by B. Malmberg (North-Holland, Amsterdam 1968) pp. 173–277
G. Fant: Formant bandwidth data. In: STL/Quart. Prog. Status Rep. 7 (Royal Inst. of Technology, Stockholm 1962) pp. 1–3
G. Fant: Vocal tract wall effects, losses, and resonance bandwidths. In: STL/Quart. Prog. Status Rep. 2-3 (Royal Inst. of Technology, Stockholm 1972) pp. 173–277
A.S. House, K.N. Stevens: Estimation of formant bandwidths from measurements of transient response of the vocal tract, J. Speech Hear. Disord. 1 , 309–315 (1958)
O. Fujimura, J. Lindqvist: Sweep-tone measurements of vocal-tract characteristics, J. Acoust. Soc. Am. 49 , 541–558 (1971)
I. Lehiste, G.E. Peterson: Vowel amplitude and phonemic stress in American English, J. Acoust. Soc. Am. 3 , 428–435 (1959)
I. Lehiste: Suprasegmentals (MIT Press, Cambridge 1970)
O. Jespersen: Lehrbuch der Phonetik (Teubner, Leipzig 1926)
T. Bartholomew: A physical definition of good voice quality in the male voice, J. Acoust. Soc. Am. 6 , 25–33 (1934)
J. Sundberg: Production and function of the singing formant. In: Report of the eleventh congress Copenhagen 1972 (Proceedings of the 11th international congress of musicology) , ed. by H. Glahn, S. Sörensen, P. Ryom (Wilhelm Hansen, Copenhagen 1972) pp. 679–686
J. Sundberg: Articulatory interpretation of the ʼsinging formantʼ, J. Acoust. Soc. Am. 55 , 838–844 (1974)
J. Sundberg: Level and center frequency of the singer´s formant, J. Voice. 15 , 176–186 (2001)
MathSciNet Google Scholar
G. Berndtsson, J. Sundberg: Perceptual significance of the center frequency of the singers formant, Scand. J. Logopedics Phoniatrics 20 , 35–41 (1995)
L. Dmitriev, A. Kiselev: Relationship between the formant structure of different types of singing voices and the dimension of supraglottal cavities, Fol. Phoniat. 31 , 238–41 (1979)
P. Ladefoged: Three areas of experimental phonetics (Oxford Univ. Press, London 1967)
J. Barnes, P. Davis, J. Oates, J. Chapman: The relationship between professional operatic soprano voice and high range spectral energy, J. Acoust. Soc. Am. 116 , 530–538 (2004)
M. Nordenberg, J. Sundberg: Effect on LTAS on vocal loudness variation, Logopedics Phoniatrics Vocology 29 , 183–191 (2004)
R. Weiss, W.S. Brown, J. Morris: Singerʼs formant in sopranos: Fact or fiction, J. Voice 15 , 457–468 (2001)
J.M. Heinz, K.N. Stevens: On the relations between lateral cineradiographs, area functions, and acoustics of speech. In: Proc. Fourth Int. Congress on Acoustics , Vol. 1a (1965), paper A44
C. Johansson, J. Sundberg, H. Willbrand: X-ray study of articulation and formant frequencies in two female singers. In: Proc. of Stockholm Music Acoustics Conference 1983 (SMAC 83) , Vol. 46(1), ed. by A. Askenfelt, S. Felicetti, E. Jansson, J Sundberg (Kgl. Musikaliska Akad., Stockholm 1985) pp. 203–218
T. Baer, J.C. Gore, L.C. Gracco, P. Nye: Analysis of vocal tract shape and dimensions using magnetic resonance imaging: Vowels, J. Acoust. Soc. Am. 90 (2), 799–828 (1991)
D. Demolin, M. George, V. Lecuit, T. Metens, A. Soquet: Détermination par IRM de lʼouverture du velum des voyelles nasales du français. In: Actes des XXièmes Journées dʼÉtudes sur la Parole (1996)
A. Foldvik, K. Kristiansen, J. Kvaerness, A. Torp, H. Torp: Three-dimensional ultrasound and magnetic resonance imaging: a new dimension in phonetic research (Proc. Fut. Congress Phonetic Science Stockholm Univ., Stockholm 1995), Vol. 4, 46-49
B.H. Story, I.R. Titze, E.A. Hoffman: Vocal tract area functions from magnetic resonance imaging, J. Acoust. Soc. Am. 100 , 537–554 (1996)
O. Engwall: Talking tongues, D.Sc. thesis (Royal Institute of Technology (KTH), Stockholm 2002)
B. Lindblom, J. Sundberg: Acoustical consequences of lip, tongue, jaw and larynx movement, J. Acoust. Soc. Am. 50 , 1166–1179 (1971), also in Papers in Speech Communication: Speech Production, ed. by R.D. Kent, B.S. Atal, J.L. Miller (Acoust. Soc. Am., New York 1991) pp.329-342
J. Stark, B. Lindblom, J. Sundberg: APEX - an articulatory synthesis model for experimental and computational studies of speech production. In: Fonetik 96: Papers presented at the Swedish Phonetics Conference TMH-QPSR 2/1996 (Royal Institute of Technology, Stockholm 1996) pp. 45–48
J. Stark, C. Ericsdotter, B. Lindblom, J. Sundberg: Using X-ray data to calibrate the APEX the synthesis. In: Fonetik 98: Papers presented at the Swedish Phonetics Conference (Stockholm Univ., Stockholm 1998)
J. Stark, C. Ericsdotter, P. Branderud, J. Sundberg, H.-J. Lundberg, J. Lander: The APEX model as a tool in the specification of speaker-specific articulatory behavior. In: Proc. 14th Int. Congress of the Phonetic Sciences , ed. by J.J. Ohala (1999)
C. Ericsdotter: Articulatory copy synthesis: Acoustic performane of an MRI and X-ray-based framework. In: Proc. 15th Int. Congress of the Phonetic Sciences , ed. by D. Recasens, M. Josep Solé, J. Romero (Universitat Autònoma de Barcelona, Barcelona 2003), CD-ROM
C. Ericsdotter: Articulatory-acoustic relationships in Swedish vowel sounds, PhD thesis (Stockholm University, Stockholm 2005)
K.N. Stevens, A.S. House: Development of a quantitative description of vowel articulation, J. Acoust. Soc. Am. 27 , 484–493 (1955)
S. Maeda: Compensatory articulation during speech: Evidence from the analysis and synthesis of vocal-tract shapes using an articulatory model. In: Speech Production and Speech Modeling , ed. by W.J. Hardcastle, A. Marchal (Dordrecht, Kluwer 1990) pp. 131–150
P. Branderud, H. Lundberg, J. Lander, H. Djamshidpey, I. Wäneland, D. Krull, B. Lindblom: X-ray analyses of speech: methodological aspects. In: Proc. XIIIth Swedish Phonetics Conf. (FONETIK 1998) (KTH, Stockholm 1998)
C.Y. Espy-Wilson: Articulatory strategies, speech acoustics and variability. In: From sound to Sense: 50+ Years of Discoveries in Speech Communication , ed. by J. Slifka, S. Manuel, M. Mathies (MIT, Cambridge 2004)
J. Sundberg: Formant technique in a professional female singer, Acustica 32 , 89–96 (1975)
J. Sundberg, J. Skoog: Dependence of jaw opening on pitch and vowel in singers, J. Voice 11 , 301–306 (1997)
G. Fant: Glottal flow, models and interaction, J. Phon. 14 , 393–399 (1986)
E. Joliveau, J. Smith, J. Wolfe: Vocal tract resonances in singing: The soprano voice, J. Acoust. Soc. Am. 116 , 2434–2439 (2004)
R.K. Potter, A.G. Kopp, H.C. Green: Visible Speech (Van Norstrand, New York 1947)
M. Joosg: Acoustic phonetics, Language 24 , 447–460 (2003), supplement 2
C.F. Hockett: A Manual of Phonology (Indiana Univ. Publ., Bloomington 1955)
F.H. Guenther: Speech sound acquisition, coarticulation, and rate effects in a neural network model of speech production, Psychol. Rev. 102 , 594–621 (1995)
R.D. Kent, B.S. Atal, J.L. Miller: Papers in Speech Communication: Speech Perception (Acoust. Soc. Am., New York 1991)
S.D. Goldinger, D.B. Pisoni, P. Luce: Speech perception and spoken word recognition. In: Principles of experimental phonetics , ed. by N.J. Lass (Mosby, St Louis 1996) pp. 277–327
H.M. Sussman, D. Fruchter, J. Hilbert, J. Sirosh: Linear correlates in the speech signal: The orderly output constraint, Behav. Brain Sci. 21 , 241–299 (1998)
B. Lindblom: Economy of speech gestures. In: The Production of Speech , ed. by P.F. MacNeilage (Springer, New York 1983) pp. 217–245
P.A. Keating, B. Lindblom, J. Lubker, J. Kreiman: Variability in jaw height for segments in English and Swedish VCVs, J. Phonetics 22 , 407–422 (1994)
K. Rapp: A study of syllable timing. In: STL/Quart. Prog. Status Rep. 1 (Royal Inst. of Technology, Stockholm( 1971) pp. 14–19
F. Koopmans-van Beinum, J. Van der Stelt (Eds.): Early stages in the development of speech movements (Stockton, New York 1986)
K. Oller: Metaphonology and infant vocalizations. In: Precursors of early speech , ed. by B. Lindblom, R. Zetterström (Stockton, New York 1986) pp. 21–36
L. Roug, L. Landberg, L. Lundberg: Phonetic development in early infancy, J. Child Language 16 , 19–40 (1989)
R. Stark: Stages of speech development in the first year of life. In: Child Phonology: Volume 1: Production , ed. by G. Yeni-Komshian, J. Kavanagh, C. Ferguson (Academic, New York 1980) pp. 73–90
R. Stark: Prespeech segmental feature development. In: Language Acquisition , ed. by P. Fletcher, M. Garman (Cambridge UP, New York 1986) pp. 149–173
D.K. Oller, R.E. Eilers: The role of audition in infant babbling, Child Devel. 59 (2), 441–449 (1988)
C. Stoel-Gammon, D. Otomo: Babbling development of hearing-impaired and normally hearing subjects, J. Speech Hear. Dis. 51 , 33–41 (1986)
R.E. Eilers, D.K. Oller: Infant vocalizations and the early diagnosis of severe hearing impairment, J. Pediatr. 124 (2), 99–203 (1994)
D. Ertmer, J. Mellon: Beginning to talk at 20 months: Early vocal development in a young cochlear implant recipient, J. Speech Lang. Hear. Res. 44 , 192–206 (2001)
R.D. Kent, M.J. Osberger, R. Netsell, C.G. Hustedde: Phonetic development in identical twins who differ in auditory function, J. Speech Hear. Dis. 52 , 64–75 (1991)
M. Lynch, D. Oller, M. Steffens: Development of speech-like vocalizations in a child with congenital absence of cochleas: The case of total deafness, Appl. Psychol. 10 , 315–333 (1989)
C. Stoel-Gammon: Prelinguistic vocalizations of hearing-impaired and normally hearing subjects: A comparison of consonantal inventories, J. Speech Hear. Dis. 53 , 302–315 (1988)
P.F. MacNeilage, B.L. Davis: Acquisition of speech production: The achievement of segmental independence. In: Speech production and speech modeling , ed. by W.J. Hardcastle, A. Marchal (Dordrecht, Kluwer 1990) pp. 55–68
T. Houtgast, H.J.M. Steeneken: A review of the MTF concept in room acoustics and its use for estimating speech intelligibility in auditoria, J. Acoust. Soc. Am. 77 , 1069–1077 (1985)
T. Houtgast, H.J.M. Steeneken: Past, Present and Future of the Speech Transmission Index , ed. by S.J. van Wijngaarden (NTO Human Factors, Soesterberg 2002)
R. Drullman, J.M. Festen, R. Plomp: Effect of temporal envelope smearing on speech reception, J. Acoust. Soc. Am. 95 , 1053–1064 (1994)
R. Drullman, J.M. Festen, R. Plomp: Effect of reducing slow temporal modulations on speech reception, J. Acoust. Soc. Am. 95 , 2670–2680 (1994)
J. Morton, S. Marcus, C. Frankish: Perceptual centers (P-centers), Psych. Rev. 83 , 405–408 (1976)
S. Marcus: Acoustic determinants of perceptual center (P-center) location, Perception & Psychophysics 30 , 247–256 (1981)
G. Allen: The place of rhythm in a theory of language, UCLA Working Papers 10 , 60–84 (1968)
G. Allen: The location of rhythmic stress beats in English: An experimental study, UCLA Working Papers 14 , 80–132 (1970)
J. Eggermont: Location of the syllable beat in routine scansion recitations of a Dutch poem, IPO Annu. Prog. Rep. 4 , 60–69 (1969)
V.A. Kozhevnikov, L.A. Chistovich: Speech Articulation and Perception, JPRS 30 , 543 (1965)
C.E. Hoequist: The perceptual center and rhythm categories, Lang. Speech 26 , 367–376 (1983)
K.J. deJong: The correlation of P-center adjustments with articulatory and acoustic events, Perception Psychophys. 56 , 447–460 (1994)
A.D. Patel, A. Löfqvist, W. Naito: The acoustics and kinematics of regularly timed speech: a database and method for the study of the P-center problem. In: Proc. 14th Int. Congress of the Phonetic Sciences , ed. by J.J. Ohala (1999)
P. Howell: Prediction of P-centre location from the distribution of energy in the amplitude envelope: I & II, Perception Psychophys. 43 , 90–93, 99 (1988)
B. Pompino-Marschall: On the psychoacoustic nature of the Pcenter phenomenon, J. Phonetics 17 , 175–192 (1989)
C.A. Harsin: Perceptual-center modeling is affected by including acoustic rate-of-change modulations, Perception Psychophys. 59 , 243–251 (1997)
C.A. Fowler: Converging sources of evidence on spoken and perceived rhythms of speech: Cyclic production of vowels in monosyllabic stress feet, J. Exp. Psychol. Gen. 112 , 386–412 (1983)
H. Fujisaki: Dynamic characteristics of voice fundamental frequency in speech and singing. In: The Production of Speech , ed. by P.F. MacNeilage (Springer, New York 1983) pp. 39–55
J. Frid: Lexical and acoustic modelling of Swedish prosody, Dissertation (Lund University, Lund 2003)
S. Öhman: Numerical model of coarticulation, J. Acoust. Soc. Am. 41 , 310–320 (1967)
J. tʼHart: F0 stylization in speech: Straight lines versus parabolas, J. Acoust. Soc. Am. 90 (6), 3368–3370 (1991)
D. Abercrombie: Elements of General Phonetics (Edinburgh Univ. Press, Edinburgh 1967)
K.L. Pike: The intonation of America English (Univ. of Michigan Press, Ann Arbor 1945)
G. Fant, A. Kruckenberg: Notes on stress and word accent in Swedish. In: STL/Quart. Prog. Status Rep. 2-3 (Royal Inst. of Technology, Stockholm 1994) pp. 125–144
R. Dauer: Stress timing and syllable-timing reanalyzed, J. Phonetics 11 , 51–62 (1983)
A. Eriksson: Aspects of Swedish rhythm, PhD thesis, Gothenburg Monographs in Linguistics (Gothenburg University, Gothenburg 1991)
O. Engstrand, D. Krull: Duration of syllable-sized units in casual and elaborated speech: cross-language observations on Swedish and Spanish, TMH-QPSR 44 , 69–72 (2002)
A.D. Patel, J.R. Daniele: An empirical comparison of rhythm in language and music, Cognition 87 , B35–B45 (2003)
D. Huron, J. Ollen: Agogic contrast in French and English themes: Further support for Patel and Daniele (2003), Music Perception 21 , 267–271 (2003)
D.H. Klatt: Synthesis by rule of segmental durations in English sentences. In: Frontiers of speech communication research , ed. by B. Lindblom, S. Öhman (Academic, London 1979) pp. 287–299
B. Lindblom: Final lengthening in speech and music. In: Nordic Prosody , ed. by E. Gårding, R. Bannert (Department of Linguistics Lund University, Lund 1978) pp. 85–102
A. Friberg, U Battel: Structural communication. In: The Science and Psychology of Music Performance , ed. by R. Parncutt, GE McPherson (Oxford Univ., Oxford 2001) pp. 199–218
J. Sundberg: Emotive transforms, Phonetica 57 , 95–112 (2000)
Brownlee: The role of sentence stress in vowel reduction and formant undershoot: A study of lab speech and informal spontaneous speech, PhD thesis (University of Texas, Austin 1996)
S.-J. Moon: An acoustic and perceptual study of undershoot in clear and citation- form speech, PhD dissertation (Univ. of Texas, Austin 1991)
K.N. Stevens, A.S. House: Perturbation of vowel articulations by consonantal context. An acoustical study, JSHR 6 , 111–128 (1963)
B. Lindblom: Spectrographic study of vowel reduction, J. Acoust. Soc. Am. 35 , 1773–1781 (1963)
P. Delattre: An acoustic and articulatory study of vowel reduction in four languages, IRAL-Int. Ref. Appl. VII/ 4 , 295–325 (1969)
D.P. Kuehn, K.L. Moll: A cineradiographic study of VC and CV articulatory velocities, J. Phonetics 4 , 303–320 (1976)
J.E. Flege: Effects of speaking rate on tongue position and velocity of movement in vowel production, J. Acoust. Soc. Am. 84 (3), 901–916 (1988)
R.J.J.H. van Son, L.C.W. Pols: "Formant movements of Dutch vowels in a text, read at normal and fast rate, J. Acoust. Soc. Am. 92 (1), 121–127 (1992)
D. van Bergem: Acoustic and Lexical Vowel Reduction, Doctoral Dissertation (University of Amsterdam, Amsterdam 1995)
W.L. Nelson, J.S. Perkell, J.R. Westbury: Mandible movements during increasingly rapid articulations of single syllables: Preliminary observations, J. Acoust. Soc. Am. 75 (3), 945–951 (1984)
S.-J. Moon, B. Lindblom: Interaction between duration, context and speaking style in English stressed vowels, J. Acoust. Soc. Am. 96 (1), 40–55 (1994)
C.S. Sherrington: Man on his nature (MacMillan, London 1986)
R. Granit: The Purposive Brain (MIT, Cambridge 1979)
N. Bernstein: The coordination and regulation of movements (Pergamon, Oxford 1967)
P.F. MacNeilage: Motor control of serial ordering of speech, Psychol. Rev. 77 , 182–196 (1970)
A. Löfqvist: Theories and Models of Speech Production. In: The Handbook of Phonetic Sciences , ed. by W.J. Hardcastle, J. Laver (Blackwell, Oxford 1997) pp. 405–426
J.S. Perkell: Articulatory processes. In: The Handbook of Phonetic Sciences. 5 , ed. by W.J. Hardcastle, J. Laver. (Blackwell, Oxford 1997) pp. 333–370
J. Sundberg, R. Leandersson, C. von Euler, E. Knutsson: Influence of body posture and lung volume on subglottal pressure control during singing, J. Voice 5 , 283–291 (1991)
T. Sears, J. Newsom Davis: The control of respiratory muscles during voluntary breathing. In: Sound production in man , ed. by A. Bouhuys et al. (Annals of the New York Academy of Science, New York 1968) pp. 183–190
B. Lindblom, J. Lubker, T. Gay: Formant frequencies of some fixed-mandible vowels and a model of motor programming by predictive simulation, J. Phonetics 7 , 147–161 (1979)
T. Gay, B. Lindblom, J. Lubker: Production of bite-block vowels: Acoustic equivalence by selective compensation, J. Acoust. Soc. Am. 69 (3), 802–810 (1981)
W.J. Hardcastle, J. Laver (Eds.): The Handbook of Phonetic Sciences (Blackwell, Oxford 1997)
J. S. Perkell, D. H. Klatt: Invariance and variability in speech processes (LEA, Hillsdale 1986)
A. Liberman, I. Mattingly: The motor theory of speech perception revised, Cognition 21 , 1–36 (1985)
C.A. Fowler: An event approach to the study of speech perception from a direct- realist perspective, J. Phon. 14 (1), 3–28 (1986)
E.L. Saltzman, K.G. Munhall: A dynamical approach to gestural patterning in speech production, Ecol. Psychol. 1 , 91–163 (1989)
M. Studdert-Kennedy: How did language go discrete?. In: Evolutionary Prerequisites of Language , ed. by M. Tallerman (Oxford Univ., Oxford 2005) pp. 47–68
R. Jakobson, G. Fant, M. Halle: Preliminaries to Speech Analysis, Acoustics Laboratory, MIT Tech. Rep. No. 13 (MIT, Cambridge 1952)
B. Lindblom: Explaining phonetic variation: A sketch of the H&H theory. In: Speech Production and Speech Modeling , ed. by W.J. Hardcastle, A. Marchal (Dordrecht, Kluwer 1990) pp. 403–439
B. Lindblom: Role of articulation in speech perception: Clues from production, J. Acoust. Soc. Am. 99 (3), 1683–1692 (1996)
E. Rapoport: Emotional expression code in opera and lied singing, J. New Music Res. 25 , 109–149 (1996)
J. Sundberg, E. Prame, J. Iwarsson: Replicability and accuracy of pitch patterns in professional singers. In: Vocal Fold Physiology, Controlling Complexity and Chaos , ed. by P. Davis, N. Fletcher (Singular, San Diego 1996) pp. 291–306, Chap. 20
J.J. Ohala: An ethological perspective on common cross-language utilization of F0 of voice, Phonetica 41 , 1–16 (1984)
I. Fónagy: Hörbare Mimik, Phonetica 1 , 25–35 (1967)
K. Scherer: Expression of emotion in voice and music, J. Voice 9 , 235–248 (1995)
P. Juslin, P. Laukka: Communication of emotions in vocal expression and music performance: Different channels, same code?, Psychol. Rev. 129 , 770–814 (2003)
J. Sundberg, J. Iwarsson, H. Hagegård: A singers expression of emotions in sung performance,. In: Vocal Fold Physiology: Voice Quality Control , ed. by O. Fujimura, M. Hirano (Singular, San Diego 1995) pp. 217–229
Download references
Author information
Authors and affiliations.
Department of Linguistics, Stockholm University, 10691, Stockholm, Sweden
Björn Lindblom Prof.
Department of Speech, Music, and Hearing, KTH–Royal Institute of Technology, SE-10044, Stockholm, Sweden
Johan Sundberg
You can also search for this author in PubMed Google Scholar
Corresponding authors
Correspondence to Björn Lindblom Prof. or Johan Sundberg .
Editor information
Editors and affiliations.
Center for Computer Research in Music and Acoustics, Stanford University, 94305, Stanford, CA, USA
Thomas D. Rossing Prof.
Rights and permissions
Reprints and permissions
Copyright information
© 2007 Springer Science+Business Media, LLC New York
About this entry
Cite this entry.
Lindblom, B., Sundberg, J. (2007). The Human Voice in Speech and Singing. In: Rossing, T. (eds) Springer Handbook of Acoustics. Springer Handbooks. Springer, New York, NY. https://doi.org/10.1007/978-0-387-30425-0_16
Download citation
DOI : https://doi.org/10.1007/978-0-387-30425-0_16
Publisher Name : Springer, New York, NY
Print ISBN : 978-0-387-30446-5
Online ISBN : 978-0-387-30425-0
eBook Packages : Physics and Astronomy Reference Module Physical and Materials Science Reference Module Chemistry, Materials and Physics
Share this entry
Anyone you share the following link with will be able to read this content:
Sorry, a shareable link is not currently available for this article.
Provided by the Springer Nature SharedIt content-sharing initiative
- Publish with us
Policies and ethics
- Find a journal
- Track your research
- Search Menu
- Browse content in Arts and Humanities
- Browse content in Architecture
- History of Architecture
- Browse content in Art
- History of Art
- Browse content in History
- Colonialism and Imperialism
- Historical Geography
- History by Period
- Industrial History
- Intellectual History
- Political History
- Regional and National History
- Social and Cultural History
- Urban History
- Language Teaching and Learning
- Browse content in Linguistics
- Cognitive Linguistics
- Grammar, Syntax and Morphology
- Historical and Diachronic Linguistics
- Language Evolution
- Language Acquisition
- Language Variation
- Language Families
- Lexicography
- Linguistic Theories
- Phonetics and Phonology
- Psycholinguistics
- Sociolinguistics
- Browse content in Literature
- Literary Studies (19th Century)
- Media Studies
- Browse content in Music
- Music Theory and Analysis
- Musicology and Music History
- Browse content in Philosophy
- Aesthetics and Philosophy of Art
- Epistemology
- History of Western Philosophy
- Metaphysics
- Moral Philosophy
- Philosophy of Language
- Philosophy of Mind
- Philosophy of Perception
- Philosophy of Science
- Philosophy of Mathematics and Logic
- Practical Ethics
- Social and Political Philosophy
- Browse content in Society and Culture
- Cultural Studies
- Technology and Society
- Visual Culture
- Browse content in Medicine and Health
- History of Medicine
- Browse content in Public Health and Epidemiology
- Public Health
- Browse content in Science and Mathematics
- Browse content in Biological Sciences
- Biochemistry
- Developmental Biology
- Ecology and Conservation
- Evolutionary Biology
- Genetics and Genomics
- Microbiology
- Molecular and Cell Biology
- Research Methods in Life Sciences
- Zoology and Animal Sciences
- Browse content in Computer Science
- Artificial Intelligence
- Programming Languages
- Browse content in Computing
- Computer Games
- Browse content in Earth Sciences and Geography
- Maps and Map-making
- Environmental Science
- History of Science and Technology
- Browse content in Mathematics
- Biomathematics and Statistics
- Probability and Statistics
- Browse content in Neuroscience
- Cognition and Behavioural Neuroscience
- Development of the Nervous System
- Disorders of the Nervous System
- History of Neuroscience
- Neuroscientific Techniques
- Sensory and Motor Systems
- Browse content in Physics
- History of Physics
- Browse content in Psychology
- Clinical Psychology
- Cognitive Psychology
- Cognitive Neuroscience
- Developmental Psychology
- Health Psychology
- Neuropsychology
- Social Psychology
- Browse content in Social Sciences
- Browse content in Business and Management
- Business Strategy
- Business History
- Business and Government
- Corporate Governance
- Industry Studies
- Information and Communication Technologies
- International Business
- Knowledge Management
- Organizational Theory and Behaviour
- Public and Nonprofit Management
- Browse content in Economics
- Agricultural, Environmental, and Natural Resource Economics
- Asian Economics
- Econometrics and Mathematical Economics
- Economic History
- Economic Systems
- Economic Development and Growth
- Financial Markets
- Financial Institutions and Services
- Health, Education, and Welfare
- History of Economic Thought
- International Economics
- Labour and Demographic Economics
- Macroeconomics and Monetary Economics
- Microeconomics
- Public Economics
- Welfare Economics
- Browse content in Education
- Educational Strategies and Policy
- Browse content in Environment
- Climate Change
- Social Impact of Environmental Issues (Social Science)
- Browse content in Interdisciplinary Studies
- Communication Studies
- Museums, Libraries, and Information Sciences
- Browse content in Politics
- Comparative Politics
- Conflict Politics
- Environmental Politics
- Human Rights and Politics
- International Relations
- Latin American Politics
- Political Economy
- Political Institutions
- Political Theory
- Public Policy
- Russian Politics
- Security Studies
- US Politics
- Browse content in Sociology
- Economic Sociology
- Health, Illness, and Medicine
- Social Movements and Social Change
- Sport and Leisure
- Reviews and Awards
- Journals on Oxford Academic
- Books on Oxford Academic

- < Previous
- Next chapter >

1 Vox Humana: The Instrumental Representation of the Human Voice
- Published: August 2010
- Cite Icon Cite
- Permissions Icon Permissions
The question of authenticity is relevant to writing about instrumental representations of the human voice. This chapter examines “modality theory,” an approach that asks how “authentic” voices have been represented and not whether they actually are “authentic.” It demonstrates that musical representations of the human voice have always been relatively abstract, perhaps seeking to provide a kind of discourse about the human voice, rather than seeking to be heard as realistic representations of human voices. The chapter describes how musical instruments have evolved over time, from mechanical contraptions to modern digital instruments that sound like the human voice. The goal of instrument makers was not to deceive the ear, but to create a discourse of “humanness,” and a sound that would musically express subjectivity, individuality, and emotionality. The chapter also explores how the modern Roland piano imitates the human voice by briefly recapitulating the theory of “modality.”
Signed in as
Institutional accounts.
- GoogleCrawler [DO NOT DELETE]
- Google Scholar Indexing
Personal account
- Sign in with email/username & password
- Get email alerts
- Save searches
- Purchase content
- Activate your purchase/trial code
- Add your ORCID iD
Institutional access
Sign in with a library card.
- Sign in with username/password
- Recommend to your librarian
- Institutional account management
- Get help with access
Access to content on Oxford Academic is often provided through institutional subscriptions and purchases. If you are a member of an institution with an active account, you may be able to access content in one of the following ways:
IP based access
Typically, access is provided across an institutional network to a range of IP addresses. This authentication occurs automatically, and it is not possible to sign out of an IP authenticated account.
Sign in through your institution
Choose this option to get remote access when outside your institution. Shibboleth/Open Athens technology is used to provide single sign-on between your institution’s website and Oxford Academic.
- Click Sign in through your institution.
- Select your institution from the list provided, which will take you to your institution's website to sign in.
- When on the institution site, please use the credentials provided by your institution. Do not use an Oxford Academic personal account.
- Following successful sign in, you will be returned to Oxford Academic.
If your institution is not listed or you cannot sign in to your institution’s website, please contact your librarian or administrator.
Enter your library card number to sign in. If you cannot sign in, please contact your librarian.
Society Members
Society member access to a journal is achieved in one of the following ways:
Sign in through society site
Many societies offer single sign-on between the society website and Oxford Academic. If you see ‘Sign in through society site’ in the sign in pane within a journal:
- Click Sign in through society site.
- When on the society site, please use the credentials provided by that society. Do not use an Oxford Academic personal account.
If you do not have a society account or have forgotten your username or password, please contact your society.
Sign in using a personal account
Some societies use Oxford Academic personal accounts to provide access to their members. See below.
A personal account can be used to get email alerts, save searches, purchase content, and activate subscriptions.
Some societies use Oxford Academic personal accounts to provide access to their members.
Viewing your signed in accounts
Click the account icon in the top right to:
- View your signed in personal account and access account management features.
- View the institutional accounts that are providing access.
Signed in but can't access content
Oxford Academic is home to a wide variety of products. The institutional subscription may not cover the content that you are trying to access. If you believe you should have access to that content, please contact your librarian.
For librarians and administrators, your personal account also provides access to institutional account management. Here you will find options to view and activate subscriptions, manage institutional settings and access options, access usage statistics, and more.
Our books are available by subscription or purchase to libraries and institutions.
- About Oxford Academic
- Publish journals with us
- University press partners
- What we publish
- New features
- Open access
- Rights and permissions
- Accessibility
- Advertising
- Media enquiries
- Oxford University Press
- Oxford Languages
- University of Oxford
Oxford University Press is a department of the University of Oxford. It furthers the University's objective of excellence in research, scholarship, and education by publishing worldwide
- Copyright © 2024 Oxford University Press
- Cookie settings
- Cookie policy
- Privacy policy
- Legal notice
Realistic Text-to-Speech AI converter
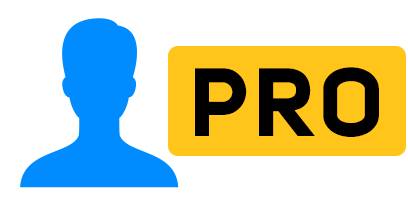
Create realistic Voiceovers online! Insert any text to generate speech and download audio mp3 or wav for any purpose. Speak a text with AI-powered voices.You can convert text to voice for free for reference only. For all features, purchase the paid plans
How to convert text into speech?
- Just type some text or import your written content
- Press "generate" button
- Download MP3 / WAV
Full list of benefits of neural voices
Downloadable tts.
You can download converted audio files in MP3, WAV, OGG for free.
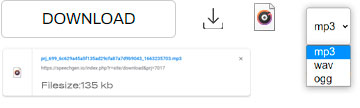
If your Limit balance is sufficient, you can use a single query to convert a text of up to 2,000,000 characters into speech.
Commercial Use
You can use the generated audio for commercial purposes. Examples: YouTube, Tik Tok, Instagram, Facebook, Twitch, Twitter, Podcasts, Video Ads, Advertising, E-book, Presentation and other.
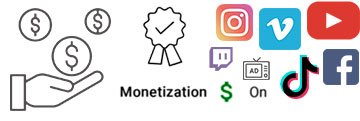
Multi-voice editor
Dialogue with AI Voices. You can use several voices at once in one text.

Custom voice settings
Change Speed, Pitch, Stress, Pronunciation, Intonation , Emphasis , Pauses and more. SSML support .
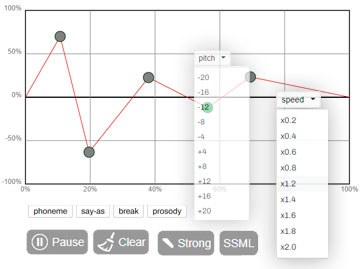
You spend little on re-dubbing the text. Limits are spent only for changed sentences in the text.
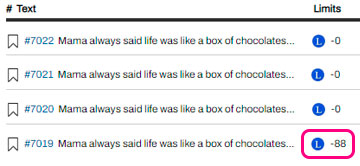
Over 1000 Natural Sounding Voices
Crystal-clear voice over like a Human. Males, females, children's, elderly voices.
Powerful support
We will help you with any questions about text-to-speech. Ask any questions, even the simplest ones. We are happy to help.
Compatible with editing programs
Works with any video creation software: Adobe Premier, After effects, Audition, DaVinci Resolve, Apple Motion, Camtasia, iMovie, Audacity, etc.
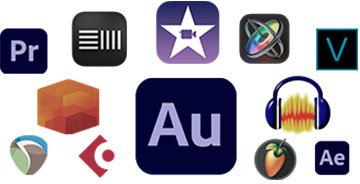
You can share the link to the audio. Send audio links to your friends and colleagues.
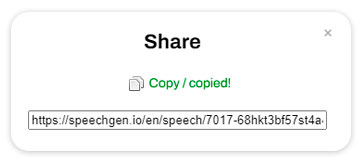
Cloud save your history
All your files and texts are automatically saved in your profile on our cloud server. Add tracks to your favorites in one click.
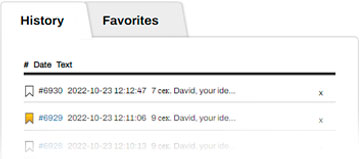
Use our text to voice converter to make videos with natural sounding speech!
Say goodbye to expensive traditional audio creation
Cheap price. Create a professional voiceover in real time for pennies. it is 100 times cheaper than a live speaker.
Traditional audio creation
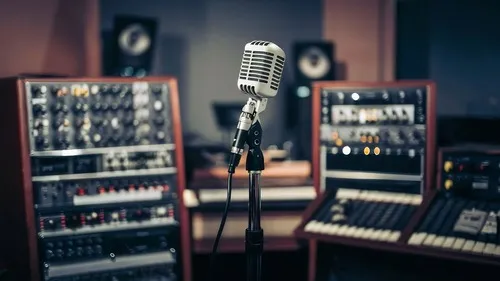
- Expensive live speakers, high prices
- A long search for freelancers and studios
- Editing requires complex tools and knowledge
- The announcer in the studio voices a long time. It takes time to give him a task and accept it.
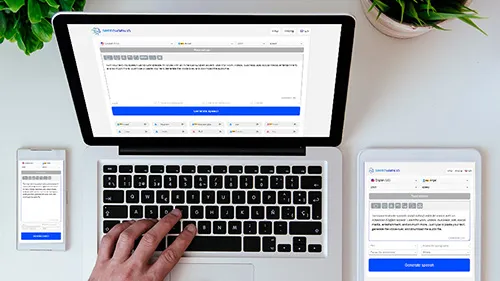
- Affordable tts generation starting at $0.08 per 1000 characters
- Website accessible in your browser right now
- Intuitive interface, suitable for beginners
- SpeechGen generates text from speech very quickly. A few clicks and the audio is ready.
Create AI-generated realistic voice-overs.
Ways to use. Cases.
See how other people are already using our realistic speech synthesis. There are hundreds of variations in applications. Here are some of them.
- Voice over for videos. Commercial, YouTube, Tik Tok, Instagram, Facebook, and other social media. Add voice to any videos!
- E-learning material. Ex: learning foreign languages, listening to lectures, instructional videos.
- Advertising. Increase installations and sales! Create AI-generated realistic voice-overs for video ads, promo, and creatives.
- Public places. Synthesizing speech from text is needed for airports, bus stations, parks, supermarkets, stadiums, and other public areas.
- Podcasts. Turn text into podcasts to increase content reach. Publish your audio files on iTunes, Spotify, and other podcast services.
- Mobile apps and desktop software. The synthesized ai voices make the app friendly.
- Essay reader. Read your essay out loud to write a better paper.
- Presentations. Use text-to-speech for impressive PowerPoint presentations and slideshow.
- Reading documents. Save your time reading documents aloud with a speech synthesizer.
- Book reader. Use our text-to-speech web app for ebook reading aloud with natural voices.
- Welcome audio messages for websites. It is a perfect way to re-engage with your audience.
- Online article reader. Internet users translate texts of interesting articles into audio and listen to them to save time.
- Voicemail greeting generator. Record voice-over for telephone systems phone greetings.
- Online narrator to read fairy tales aloud to children.
- For fun. Use the robot voiceover to create memes, creativity, and gags.
Maximize your content’s potential with an audio-version. Increase audience engagement and drive business growth.
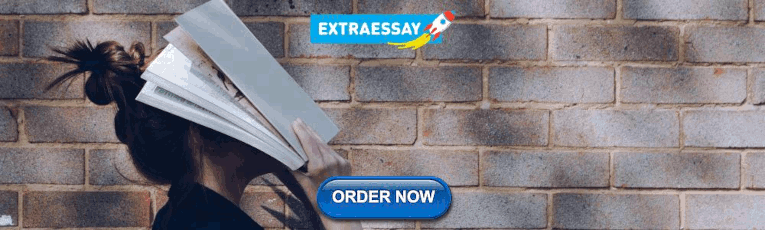
Who uses Text to Speech?
SpeechGen.io is a service with artificial intelligence used by about 1,000 people daily for different purposes. Here are examples.
Video makers create voiceovers for videos. They generate audio content without expensive studio production.
Newsmakers convert text to speech with computerized voices for news reporting and sports announcing.
Students and busy professionals to quickly explore content
Foreigners. Second-language students who want to improve their pronunciation or listen to the text comprehension
Software developers add synthesized speech to programs to improve the user experience.
Marketers. Easy-to-produce audio content for any startups
IVR voice recordings. Generate prompts for interactive voice response systems.
Educators. Foreign language teachers generate voice from the text for audio examples.
Booklovers use Speechgen as an out loud book reader. The TTS voiceover is downloadable. Listen on any device.
HR departments and e-learning professionals can make learning modules and employee training with ai text to speech online software.
Webmasters convert articles to audio with lifelike robotic voices. TTS audio increases the time on the webpage and the depth of views.
Animators use ai voices for dialogue and character speech.
Text to Speech enables brands, companies, and organizations to deliver enhanced end-user experience, while minimizing costs.
Frequently Asked Questions
Convert any text to super realistic human voices. See all tariff plans .
Enhance Your Content Accessibility
Boost your experience with our additional features. Easily convert PDFs, DOCx files, and video subtitles into natural-sounding audio.
📄🔊 PDF to Audio
Transform your PDF documents into audible content for easier consumption and enhanced accessibility.
📝🎧 DOCx to mp3
Easily convert Word documents into speech for listening on the go or for those who prefer audio format
📺💬 Subtitles to Speech
Make your video content more accessible by converting subtitles into natural-sounding audio.
Supported languages
- Amharic (Ethiopia)
- Arabic (Algeria)
- Arabic (Egypt)
- Arabic (Saudi Arabia)
- Bengali (India)
- Catalan (Spain)
- English (Australia)
- English (Canada)
- English (GB)
- English (Hong Kong)
- English (India)
- English (Philippines)
- German (Austria)
- Hindi India
- Spanish (Argentina)
- Spanish (Mexico)
- Spanish (United States)
- Tamil (India)
- All languages: +76
We use cookies to ensure you get the best experience on our website. Learn more: Privacy Policy
The Relationship Between Acoustics and Human Voice Essay
Introduction, the method used to determine sound absorption, type of sound absorbers, porous absorbers, membrane absorbers, resonator absorber, practical use of sound absorbers in room acoustics, works cited.
The term ‘acoustic’ is synonymous with the study of sound waves and their effects. In a synopsis, this study basically centers on the consequences of wave motions across the three states of matter including solids, liquids and gasses. As such, the scope of acoustics cut across array of disciplines, and to this effect, the terms, for instance, psychoacoustics and bioacoustics are popular among acousticians. Moreover, acoustics find application in technical fields including noise control, transducer technology, design of theatre halls, and “sound recording and production” (Finn et al. 103). With regards to the scope of this paper, our main interest is centered on sound absorbers and how they are applied in room designs.
The reverberation time (T 60 = 0.16V/A) as derived by Sabine is the most vital formula when it comes to room acoustics. As such, on predicting T 60 of materials one is in a better position to determine the surface, acoustic characteristics of a room and hence; clad a room appropriately. Principally, in order for one to achieve an ideal room design meant for a specific application, an acoustician need to have knowledge on “absorption coefficient per octave band” (Finn et al. 103) of a diversity of materials. In a nutshell, in a room, materials including wooden doors and windows are known to absorbing sound of low frequency.
In the contrary, fabrics and clothes are known to absorbing middle and high frequency sound waves. Consequently, one can strike a balance between these materials so as to achieve appropriate T 60 versus frequency combinations in a room. In a synopsis, the specific objective of this paper is to introduce and hence appreciate the physical mechanisms vital in sound absorption cum reverberation control. To this effect, the knowledge of sound absorption coefficient would come in handy when describing the properties of these materials. In some places, for example, in churches, there is a general demand for control of reverberations. As such, this paper will try to demonstrate how an effective room design dampens these effects.
Generally, the inner surfaces of a room receive sound from a wide diffuse field typified by the figure below:
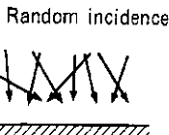
Consequently, this chapter describes a relevant sound absorption method that would be used to determine the sound absorption coefficient emanating from the above incidence. This method is also reffered to as the reverbaration room method. Ideally, the experiment is performed in a “reverbaration room with highly irregular or non parallel surfaces and/or suspended, sound defusing elements” (Finn et al. 103). The assumption in this experiment is that the varied sound field would concur with the requirements stipulated in Subine’s reverberation formula. To derive Subines formula we assume that a vacant reverberation room has the following parameters; an absorption coefficient, α empty , inner surface area, S, and has a volume, V, then the below equation is derived:
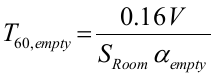
In this room an introduction of a foreign material, S sample , changes the entire equation to:

On combining the two equations and eliminating S yields the equation below:
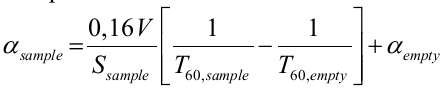
The above equation is fundamental in determining the coefficient, α sample , of a foreign material. Importantly, the measurement is “normally carried out in 1/1 or 1/3 octave bands from 100 to 5000 MHz” (Finn et al. 104). The results obtained in this method can only be credible if the size of the material sample relative to the area of the room is logical. Basically, if a very small material is introduced to an abnormally large room then the results obtained would be faulty (α>1) (see the graph below). This phenomenon is accounted for by the effects of diffraction of sound waves which dwarfs the absorption properties of the sample.
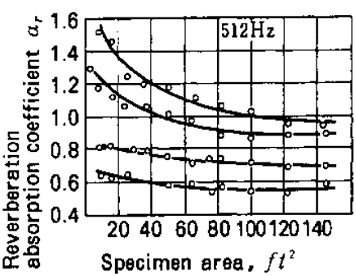
This chapter describes the most common types of materials used for sound absorption. These materials include the membrane, resonate and porous absorbers. This section tries to relate the absorption coefficient of these materials to the frequency of the same as typified by the graph below:
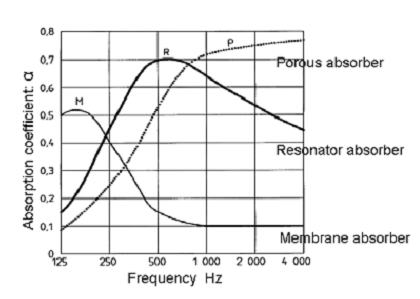
Porous absorbers are common in our houses and they are presented in numerous forms including garments like curtains, furniture, carpets, and the ceiling material. Porous materials are basically characterized by the presence of air pockets which can be pressed more or less thanks to the resistivity of the material. Chiefly, the absorption property of a material is a function of the friction drag force present in air molecules under motion, and the nature of the material in contact with the air where heat transfer happens (from kinetic in sound to heat energy on the material).
Taking into account a scenario where a sound wave is incident but normally on a porous material mounted on a rigid surface, the trend exhibited by the graph below (left) of a standing wave together with its pressure amplitude on the left would result. The sound pressure coincides with the particle velocity.
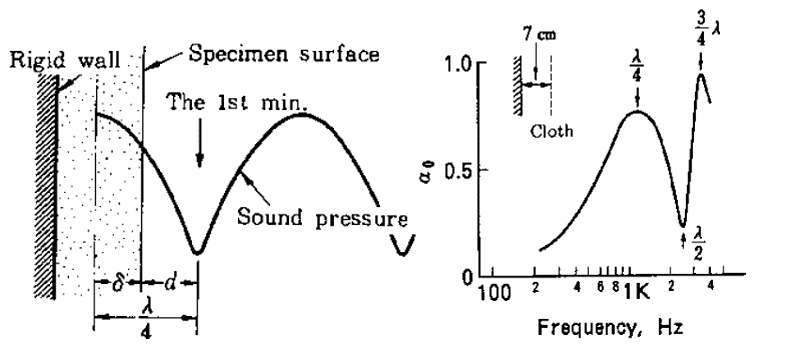
This is in contrast with when the same wave bombards a rigid termination (see figure below). Basically, the pressure and the particle velocity cancel out. The rational behind this analysis is that it aids in determining sound absorption efficacy of a material. Ideally, to achieve the best sound absorption efficacy then the thickness of a material should be at least greater than a quarter the wavelenght of the sound wave.
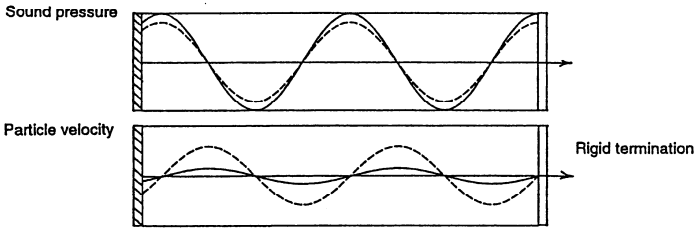
In a nutshell, sound absorbance efficacy of a given thickness of a material will be deemed to have failed if a certain threshold frequency of the sound wave fails to attain. The graph below portrays how absorption coefficient versus frequency differs with various thicknesses of a wool mat.
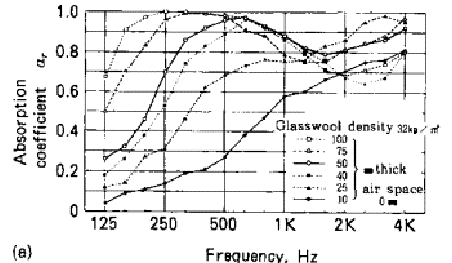
A membrane absorber is a “kind of double walled sound absorber with an air-filled cavity sandwiched between the walls” (Finn et al. 106). The frequency of resonance (f o ) of the entire setup is a function of the mass, m, of a unit area of a plate, and the spring ability of the enclosed air which depends on the deepness, L, of the enclosed air. This can be represented by the equation below:
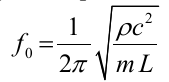
Nevertheless, this is limited to when the plate is absolutely limp. Other plate parameters e.g. stiffness as well as the mode of pulsation are vital in determining the resonance frequency. To this end, this value can be obtained using the expression below:
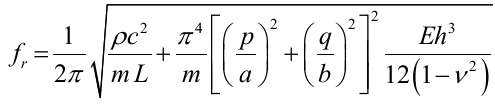
As such, the variables “a and b represent the dimensions of the material, h is the thickness while E and v are the Young’s Modulus and poisons ratio respectively” (Finn et al. 106).
The graph below shows a plot of absorbance coefficient against sound frequency for two sample materials (plywood) having different thickness. Furthermore, one of the plates had been mounted with glass wool while the other one was without. The trends as attested by the graphs below confirm the above two equations. They reveal “that the thickness is inversely proportional to resonance frequency” (Finn et al. 106). Also, it has been established that the presence of a glass wool enhances the absorption efficacy. This also decimates the resonance frequency.
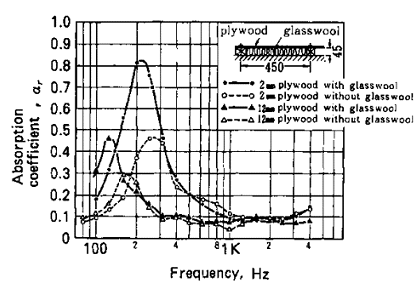
These absorbers are found in our houses typically as wooden floor surfaces, and they result in a controlled low, resonance frequency value contrary to concrete built rooms. The later, is synonymous with blurred sounds at low frequencies.
A more advanced system of a membrane absorber is a resonator which utilizes an oscillating air sandwiched in a double walled cavity. This has an opening on the surface to the outer atmosphere. To this effect the enclosed air act as a spring function (see the figure below).
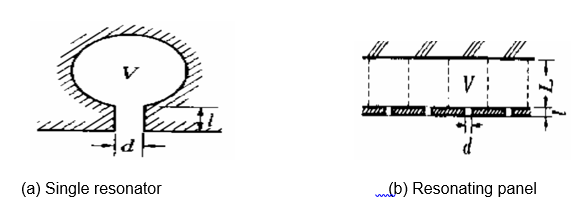
For type (a) the resonance frequency is obtained through the expression below:
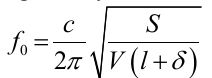
The variables S, V, l and δ represent the crossection area of the openning, volume of enclosed air, neck length and a correction factor respectively. However, this type of absober is not common since it has a very short frequency range. As such, a resonating panel overcomes the shortcomings of the former model by providing for a relatively wider frequency range. Thus, its resonance frequency is given by:
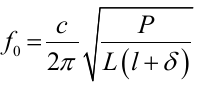
The variables P and L represent the extent of perforation and depth of the air pockets respectively.
As compared to a membrane absorber, a resonator is an efficient sound absorber. The damping efficacy and hence the sound absorbance can be optimized by mounting a monolayer of mineral wool in the perforations. Also, this can significantly be enhanced by the reduction of the pore diameters. Most common form of this type of an absorber is a perforated gypsum board.
The essence of understanding the physics behind sound absorbers is to equip oneself with the knowledge of how reverberation can be contained in room designs. This will in turn reduce noise and enhance intelligibility. Some states’ building laws, for example Denmark Building Law, clearly stipulates for an optimum T 60 value for diverse working environs including schools and day-care institutions among others. Furthermore, this law recommends for specific designs for buildings including theatre and concert halls among others.
Room acoustic designers usually target the ceiling because it provides for manipulation since most of its surface is always unoccupied. Consequently, acousticians have come up with designs including suspended ceilings and mineral wool baffles that aid in damping reverberations. These designs are portrayed by the pictures below:
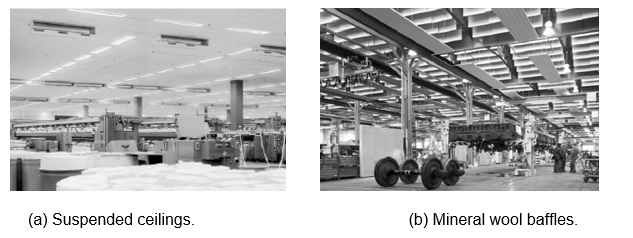
Principally, in room acoustics where a majority of sound absorbers are biased towards the ceiling, the T 60 becomes a function of the room height. Therefore;
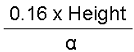
Many a public places demand for a noise free environ thanks to acousticians’ efforts in trying to dampen reverberation. However, an ideal situation requires for an intelligible speech too. To this effect, the figures below show how a delayed sound decay in a room can facade weak phonemes typified schematically as perpendicular bars. Basically, in speeches, a consonant element that is usually overlooked as a weaker sound can serve to jeopardize the intelligibility of a speech if the decay time is not checked. As illustrated by the schematic diagrams below, a long reverberation has a potential to extremely deteriorate the entire speech.
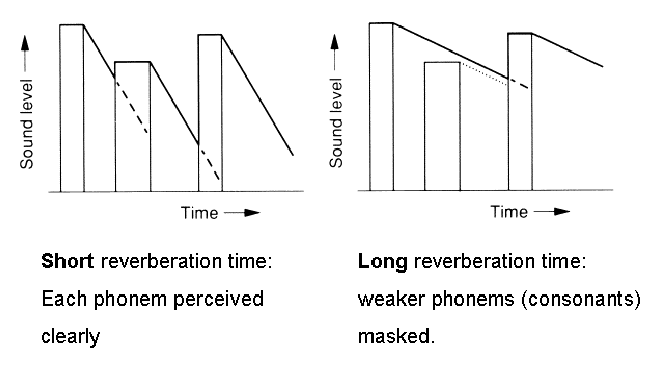
Finn, Jacobsen, Torben Poulsen, Jens Rindel, Anders Gade and Mogens Ohlrich. FUNDERMENTALS OF ACOUSTICS AND NOISE CONTROL . Odense: Department of Electric Enginearing, 2011. Print.
- Chicago (A-D)
- Chicago (N-B)
IvyPanda. (2022, July 27). The Relationship Between Acoustics and Human Voice. https://ivypanda.com/essays/the-relationship-between-acoustics-and-human-voice/
"The Relationship Between Acoustics and Human Voice." IvyPanda , 27 July 2022, ivypanda.com/essays/the-relationship-between-acoustics-and-human-voice/.
IvyPanda . (2022) 'The Relationship Between Acoustics and Human Voice'. 27 July.
IvyPanda . 2022. "The Relationship Between Acoustics and Human Voice." July 27, 2022. https://ivypanda.com/essays/the-relationship-between-acoustics-and-human-voice/.
1. IvyPanda . "The Relationship Between Acoustics and Human Voice." July 27, 2022. https://ivypanda.com/essays/the-relationship-between-acoustics-and-human-voice/.
Bibliography
IvyPanda . "The Relationship Between Acoustics and Human Voice." July 27, 2022. https://ivypanda.com/essays/the-relationship-between-acoustics-and-human-voice/.
- "The Acoustics of Loneliness" by Mary Shine Literary Analysis
- Glassware Packing Company Analysis
- Diplomacy: Two Level Games and Bargaining Outcomes
- The Use of Physics in my Daily Activities
- GE Taps into Coolest Energy Storage Technology around
- Physics: Sliding Bubble Dynamics
- The Sun’s Light and Heat: Solar Energy Issue
- Rotational Response and Slip Prediction of Serpentine Belt Drive Systems
Thank you for visiting nature.com. You are using a browser version with limited support for CSS. To obtain the best experience, we recommend you use a more up to date browser (or turn off compatibility mode in Internet Explorer). In the meantime, to ensure continued support, we are displaying the site without styles and JavaScript.
- View all journals
- My Account Login
- Explore content
- About the journal
- Publish with us
- Sign up for alerts
- Open access
- Published: 30 October 2023
A large-scale comparison of human-written versus ChatGPT-generated essays
- Steffen Herbold 1 ,
- Annette Hautli-Janisz 1 ,
- Ute Heuer 1 ,
- Zlata Kikteva 1 &
- Alexander Trautsch 1
Scientific Reports volume 13 , Article number: 18617 ( 2023 ) Cite this article
19k Accesses
14 Citations
94 Altmetric
Metrics details
- Computer science
- Information technology
ChatGPT and similar generative AI models have attracted hundreds of millions of users and have become part of the public discourse. Many believe that such models will disrupt society and lead to significant changes in the education system and information generation. So far, this belief is based on either colloquial evidence or benchmarks from the owners of the models—both lack scientific rigor. We systematically assess the quality of AI-generated content through a large-scale study comparing human-written versus ChatGPT-generated argumentative student essays. We use essays that were rated by a large number of human experts (teachers). We augment the analysis by considering a set of linguistic characteristics of the generated essays. Our results demonstrate that ChatGPT generates essays that are rated higher regarding quality than human-written essays. The writing style of the AI models exhibits linguistic characteristics that are different from those of the human-written essays. Since the technology is readily available, we believe that educators must act immediately. We must re-invent homework and develop teaching concepts that utilize these AI models in the same way as math utilizes the calculator: teach the general concepts first and then use AI tools to free up time for other learning objectives.
Similar content being viewed by others
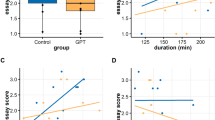
ChatGPT-3.5 as writing assistance in students’ essays
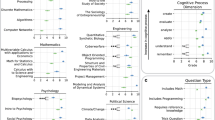
Perception, performance, and detectability of conversational artificial intelligence across 32 university courses
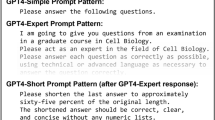
The model student: GPT-4 performance on graduate biomedical science exams
Introduction.
The massive uptake in the development and deployment of large-scale Natural Language Generation (NLG) systems in recent months has yielded an almost unprecedented worldwide discussion of the future of society. The ChatGPT service which serves as Web front-end to GPT-3.5 1 and GPT-4 was the fastest-growing service in history to break the 100 million user milestone in January and had 1 billion visits by February 2023 2 .
Driven by the upheaval that is particularly anticipated for education 3 and knowledge transfer for future generations, we conduct the first independent, systematic study of AI-generated language content that is typically dealt with in high-school education: argumentative essays, i.e. essays in which students discuss a position on a controversial topic by collecting and reflecting on evidence (e.g. ‘Should students be taught to cooperate or compete?’). Learning to write such essays is a crucial aspect of education, as students learn to systematically assess and reflect on a problem from different perspectives. Understanding the capability of generative AI to perform this task increases our understanding of the skills of the models, as well as of the challenges educators face when it comes to teaching this crucial skill. While there is a multitude of individual examples and anecdotal evidence for the quality of AI-generated content in this genre (e.g. 4 ) this paper is the first to systematically assess the quality of human-written and AI-generated argumentative texts across different versions of ChatGPT 5 . We use a fine-grained essay quality scoring rubric based on content and language mastery and employ a significant pool of domain experts, i.e. high school teachers across disciplines, to perform the evaluation. Using computational linguistic methods and rigorous statistical analysis, we arrive at several key findings:
AI models generate significantly higher-quality argumentative essays than the users of an essay-writing online forum frequented by German high-school students across all criteria in our scoring rubric.
ChatGPT-4 (ChatGPT web interface with the GPT-4 model) significantly outperforms ChatGPT-3 (ChatGPT web interface with the GPT-3.5 default model) with respect to logical structure, language complexity, vocabulary richness and text linking.
Writing styles between humans and generative AI models differ significantly: for instance, the GPT models use more nominalizations and have higher sentence complexity (signaling more complex, ‘scientific’, language), whereas the students make more use of modal and epistemic constructions (which tend to convey speaker attitude).
The linguistic diversity of the NLG models seems to be improving over time: while ChatGPT-3 still has a significantly lower linguistic diversity than humans, ChatGPT-4 has a significantly higher diversity than the students.
Our work goes significantly beyond existing benchmarks. While OpenAI’s technical report on GPT-4 6 presents some benchmarks, their evaluation lacks scientific rigor: it fails to provide vital information like the agreement between raters, does not report on details regarding the criteria for assessment or to what extent and how a statistical analysis was conducted for a larger sample of essays. In contrast, our benchmark provides the first (statistically) rigorous and systematic study of essay quality, paired with a computational linguistic analysis of the language employed by humans and two different versions of ChatGPT, offering a glance at how these NLG models develop over time. While our work is focused on argumentative essays in education, the genre is also relevant beyond education. In general, studying argumentative essays is one important aspect to understand how good generative AI models are at conveying arguments and, consequently, persuasive writing in general.
Related work
Natural language generation.
The recent interest in generative AI models can be largely attributed to the public release of ChatGPT, a public interface in the form of an interactive chat based on the InstructGPT 1 model, more commonly referred to as GPT-3.5. In comparison to the original GPT-3 7 and other similar generative large language models based on the transformer architecture like GPT-J 8 , this model was not trained in a purely self-supervised manner (e.g. through masked language modeling). Instead, a pipeline that involved human-written content was used to fine-tune the model and improve the quality of the outputs to both mitigate biases and safety issues, as well as make the generated text more similar to text written by humans. Such models are referred to as Fine-tuned LAnguage Nets (FLANs). For details on their training, we refer to the literature 9 . Notably, this process was recently reproduced with publicly available models such as Alpaca 10 and Dolly (i.e. the complete models can be downloaded and not just accessed through an API). However, we can only assume that a similar process was used for the training of GPT-4 since the paper by OpenAI does not include any details on model training.
Testing of the language competency of large-scale NLG systems has only recently started. Cai et al. 11 show that ChatGPT reuses sentence structure, accesses the intended meaning of an ambiguous word, and identifies the thematic structure of a verb and its arguments, replicating human language use. Mahowald 12 compares ChatGPT’s acceptability judgments to human judgments on the Article + Adjective + Numeral + Noun construction in English. Dentella et al. 13 show that ChatGPT-3 fails to understand low-frequent grammatical constructions like complex nested hierarchies and self-embeddings. In another recent line of research, the structure of automatically generated language is evaluated. Guo et al. 14 show that in question-answer scenarios, ChatGPT-3 uses different linguistic devices than humans. Zhao et al. 15 show that ChatGPT generates longer and more diverse responses when the user is in an apparently negative emotional state.
Given that we aim to identify certain linguistic characteristics of human-written versus AI-generated content, we also draw on related work in the field of linguistic fingerprinting, which assumes that each human has a unique way of using language to express themselves, i.e. the linguistic means that are employed to communicate thoughts, opinions and ideas differ between humans. That these properties can be identified with computational linguistic means has been showcased across different tasks: the computation of a linguistic fingerprint allows to distinguish authors of literary works 16 , the identification of speaker profiles in large public debates 17 , 18 , 19 , 20 and the provision of data for forensic voice comparison in broadcast debates 21 , 22 . For educational purposes, linguistic features are used to measure essay readability 23 , essay cohesion 24 and language performance scores for essay grading 25 . Integrating linguistic fingerprints also yields performance advantages for classification tasks, for instance in predicting user opinion 26 , 27 and identifying individual users 28 .
Limitations of OpenAIs ChatGPT evaluations
OpenAI published a discussion of the model’s performance of several tasks, including Advanced Placement (AP) classes within the US educational system 6 . The subjects used in performance evaluation are diverse and include arts, history, English literature, calculus, statistics, physics, chemistry, economics, and US politics. While the models achieved good or very good marks in most subjects, they did not perform well in English literature. GPT-3.5 also experienced problems with chemistry, macroeconomics, physics, and statistics. While the overall results are impressive, there are several significant issues: firstly, the conflict of interest of the model’s owners poses a problem for the performance interpretation. Secondly, there are issues with the soundness of the assessment beyond the conflict of interest, which make the generalizability of the results hard to assess with respect to the models’ capability to write essays. Notably, the AP exams combine multiple-choice questions with free-text answers. Only the aggregated scores are publicly available. To the best of our knowledge, neither the generated free-text answers, their overall assessment, nor their assessment given specific criteria from the used judgment rubric are published. Thirdly, while the paper states that 1–2 qualified third-party contractors participated in the rating of the free-text answers, it is unclear how often multiple ratings were generated for the same answer and what was the agreement between them. This lack of information hinders a scientifically sound judgement regarding the capabilities of these models in general, but also specifically for essays. Lastly, the owners of the model conducted their study in a few-shot prompt setting, where they gave the models a very structured template as well as an example of a human-written high-quality essay to guide the generation of the answers. This further fine-tuning of what the models generate could have also influenced the output. The results published by the owners go beyond the AP courses which are directly comparable to our work and also consider other student assessments like Graduate Record Examinations (GREs). However, these evaluations suffer from the same problems with the scientific rigor as the AP classes.
Scientific assessment of ChatGPT
Researchers across the globe are currently assessing the individual capabilities of these models with greater scientific rigor. We note that due to the recency and speed of these developments, the hereafter discussed literature has mostly only been published as pre-prints and has not yet been peer-reviewed. In addition to the above issues concretely related to the assessment of the capabilities to generate student essays, it is also worth noting that there are likely large problems with the trustworthiness of evaluations, because of data contamination, i.e. because the benchmark tasks are part of the training of the model, which enables memorization. For example, Aiyappa et al. 29 find evidence that this is likely the case for benchmark results regarding NLP tasks. This complicates the effort by researchers to assess the capabilities of the models beyond memorization.
Nevertheless, the first assessment results are already available – though mostly focused on ChatGPT-3 and not yet ChatGPT-4. Closest to our work is a study by Yeadon et al. 30 , who also investigate ChatGPT-3 performance when writing essays. They grade essays generated by ChatGPT-3 for five physics questions based on criteria that cover academic content, appreciation of the underlying physics, grasp of subject material, addressing the topic, and writing style. For each question, ten essays were generated and rated independently by five researchers. While the sample size precludes a statistical assessment, the results demonstrate that the AI model is capable of writing high-quality physics essays, but that the quality varies in a manner similar to human-written essays.
Guo et al. 14 create a set of free-text question answering tasks based on data they collected from the internet, e.g. question answering from Reddit. The authors then sample thirty triplets of a question, a human answer, and a ChatGPT-3 generated answer and ask human raters to assess if they can detect which was written by a human, and which was written by an AI. While this approach does not directly assess the quality of the output, it serves as a Turing test 31 designed to evaluate whether humans can distinguish between human- and AI-produced output. The results indicate that humans are in fact able to distinguish between the outputs when presented with a pair of answers. Humans familiar with ChatGPT are also able to identify over 80% of AI-generated answers without seeing a human answer in comparison. However, humans who are not yet familiar with ChatGPT-3 are not capable of identifying AI-written answers about 50% of the time. Moreover, the authors also find that the AI-generated outputs are deemed to be more helpful than the human answers in slightly more than half of the cases. This suggests that the strong results from OpenAI’s own benchmarks regarding the capabilities to generate free-text answers generalize beyond the benchmarks.
There are, however, some indicators that the benchmarks may be overly optimistic in their assessment of the model’s capabilities. For example, Kortemeyer 32 conducts a case study to assess how well ChatGPT-3 would perform in a physics class, simulating the tasks that students need to complete as part of the course: answer multiple-choice questions, do homework assignments, ask questions during a lesson, complete programming exercises, and write exams with free-text questions. Notably, ChatGPT-3 was allowed to interact with the instructor for many of the tasks, allowing for multiple attempts as well as feedback on preliminary solutions. The experiment shows that ChatGPT-3’s performance is in many aspects similar to that of the beginning learners and that the model makes similar mistakes, such as omitting units or simply plugging in results from equations. Overall, the AI would have passed the course with a low score of 1.5 out of 4.0. Similarly, Kung et al. 33 study the performance of ChatGPT-3 in the United States Medical Licensing Exam (USMLE) and find that the model performs at or near the passing threshold. Their assessment is a bit more optimistic than Kortemeyer’s as they state that this level of performance, comprehensible reasoning and valid clinical insights suggest that models such as ChatGPT may potentially assist human learning in clinical decision making.
Frieder et al. 34 evaluate the capabilities of ChatGPT-3 in solving graduate-level mathematical tasks. They find that while ChatGPT-3 seems to have some mathematical understanding, its level is well below that of an average student and in most cases is not sufficient to pass exams. Yuan et al. 35 consider the arithmetic abilities of language models, including ChatGPT-3 and ChatGPT-4. They find that they exhibit the best performance among other currently available language models (incl. Llama 36 , FLAN-T5 37 , and Bloom 38 ). However, the accuracy of basic arithmetic tasks is still only at 83% when considering correctness to the degree of \(10^{-3}\) , i.e. such models are still not capable of functioning reliably as calculators. In a slightly satiric, yet insightful take, Spencer et al. 39 assess how a scientific paper on gamma-ray astrophysics would look like, if it were written largely with the assistance of ChatGPT-3. They find that while the language capabilities are good and the model is capable of generating equations, the arguments are often flawed and the references to scientific literature are full of hallucinations.
The general reasoning skills of the models may also not be at the level expected from the benchmarks. For example, Cherian et al. 40 evaluate how well ChatGPT-3 performs on eleven puzzles that second graders should be able to solve and find that ChatGPT is only able to solve them on average in 36.4% of attempts, whereas the second graders achieve a mean of 60.4%. However, their sample size is very small and the problem was posed as a multiple-choice question answering problem, which cannot be directly compared to the NLG we consider.
Research gap
Within this article, we address an important part of the current research gap regarding the capabilities of ChatGPT (and similar technologies), guided by the following research questions:
RQ1: How good is ChatGPT based on GPT-3 and GPT-4 at writing argumentative student essays?
RQ2: How do AI-generated essays compare to essays written by students?
RQ3: What are linguistic devices that are characteristic of student versus AI-generated content?
We study these aspects with the help of a large group of teaching professionals who systematically assess a large corpus of student essays. To the best of our knowledge, this is the first large-scale, independent scientific assessment of ChatGPT (or similar models) of this kind. Answering these questions is crucial to understanding the impact of ChatGPT on the future of education.
Materials and methods
The essay topics originate from a corpus of argumentative essays in the field of argument mining 41 . Argumentative essays require students to think critically about a topic and use evidence to establish a position on the topic in a concise manner. The corpus features essays for 90 topics from Essay Forum 42 , an active community for providing writing feedback on different kinds of text and is frequented by high-school students to get feedback from native speakers on their essay-writing capabilities. Information about the age of the writers is not available, but the topics indicate that the essays were written in grades 11–13, indicating that the authors were likely at least 16. Topics range from ‘Should students be taught to cooperate or to compete?’ to ‘Will newspapers become a thing of the past?’. In the corpus, each topic features one human-written essay uploaded and discussed in the forum. The students who wrote the essays are not native speakers. The average length of these essays is 19 sentences with 388 tokens (an average of 2.089 characters) and will be termed ‘student essays’ in the remainder of the paper.
For the present study, we use the topics from Stab and Gurevych 41 and prompt ChatGPT with ‘Write an essay with about 200 words on “[ topic ]”’ to receive automatically-generated essays from the ChatGPT-3 and ChatGPT-4 versions from 22 March 2023 (‘ChatGPT-3 essays’, ‘ChatGPT-4 essays’). No additional prompts for getting the responses were used, i.e. the data was created with a basic prompt in a zero-shot scenario. This is in contrast to the benchmarks by OpenAI, who used an engineered prompt in a few-shot scenario to guide the generation of essays. We note that we decided to ask for 200 words because we noticed a tendency to generate essays that are longer than the desired length by ChatGPT. A prompt asking for 300 words typically yielded essays with more than 400 words. Thus, using the shorter length of 200, we prevent a potential advantage for ChatGPT through longer essays, and instead err on the side of brevity. Similar to the evaluations of free-text answers by OpenAI, we did not consider multiple configurations of the model due to the effort required to obtain human judgments. For the same reason, our data is restricted to ChatGPT and does not include other models available at that time, e.g. Alpaca. We use the browser versions of the tools because we consider this to be a more realistic scenario than using the API. Table 1 below shows the core statistics of the resulting dataset. Supplemental material S1 shows examples for essays from the data set.
Annotation study
Study participants.
The participants had registered for a two-hour online training entitled ‘ChatGPT – Challenges and Opportunities’ conducted by the authors of this paper as a means to provide teachers with some of the technological background of NLG systems in general and ChatGPT in particular. Only teachers permanently employed at secondary schools were allowed to register for this training. Focusing on these experts alone allows us to receive meaningful results as those participants have a wide range of experience in assessing students’ writing. A total of 139 teachers registered for the training, 129 of them teach at grammar schools, and only 10 teachers hold a position at other secondary schools. About half of the registered teachers (68 teachers) have been in service for many years and have successfully applied for promotion. For data protection reasons, we do not know the subject combinations of the registered teachers. We only know that a variety of subjects are represented, including languages (English, French and German), religion/ethics, and science. Supplemental material S5 provides some general information regarding German teacher qualifications.
The training began with an online lecture followed by a discussion phase. Teachers were given an overview of language models and basic information on how ChatGPT was developed. After about 45 minutes, the teachers received a both written and oral explanation of the questionnaire at the core of our study (see Supplementary material S3 ) and were informed that they had 30 minutes to finish the study tasks. The explanation included information on how the data was obtained, why we collect the self-assessment, and how we chose the criteria for the rating of the essays, the overall goal of our research, and a walk-through of the questionnaire. Participation in the questionnaire was voluntary and did not affect the awarding of a training certificate. We further informed participants that all data was collected anonymously and that we would have no way of identifying who participated in the questionnaire. We orally informed participants that they consent to the use of the provided ratings for our research by participating in the survey.
Once these instructions were provided orally and in writing, the link to the online form was given to the participants. The online form was running on a local server that did not log any information that could identify the participants (e.g. IP address) to ensure anonymity. As per instructions, consent for participation was given by using the online form. Due to the full anonymity, we could by definition not document who exactly provided the consent. This was implemented as further insurance that non-participation could not possibly affect being awarded the training certificate.
About 20% of the training participants did not take part in the questionnaire study, the remaining participants consented based on the information provided and participated in the rating of essays. After the questionnaire, we continued with an online lecture on the opportunities of using ChatGPT for teaching as well as AI beyond chatbots. The study protocol was reviewed and approved by the Research Ethics Committee of the University of Passau. We further confirm that our study protocol is in accordance with all relevant guidelines.
Questionnaire
The questionnaire consists of three parts: first, a brief self-assessment regarding the English skills of the participants which is based on the Common European Framework of Reference for Languages (CEFR) 43 . We have six levels ranging from ‘comparable to a native speaker’ to ‘some basic skills’ (see supplementary material S3 ). Then each participant was shown six essays. The participants were only shown the generated text and were not provided with information on whether the text was human-written or AI-generated.
The questionnaire covers the seven categories relevant for essay assessment shown below (for details see supplementary material S3 ):
Topic and completeness
Logic and composition
Expressiveness and comprehensiveness
Language mastery
Vocabulary and text linking
Language constructs
These categories are used as guidelines for essay assessment 44 established by the Ministry for Education of Lower Saxony, Germany. For each criterion, a seven-point Likert scale with scores from zero to six is defined, where zero is the worst score (e.g. no relation to the topic) and six is the best score (e.g. addressed the topic to a special degree). The questionnaire included a written description as guidance for the scoring.
After rating each essay, the participants were also asked to self-assess their confidence in the ratings. We used a five-point Likert scale based on the criteria for the self-assessment of peer-review scores from the Association for Computational Linguistics (ACL). Once a participant finished rating the six essays, they were shown a summary of their ratings, as well as the individual ratings for each of their essays and the information on how the essay was generated.
Computational linguistic analysis
In order to further explore and compare the quality of the essays written by students and ChatGPT, we consider the six following linguistic characteristics: lexical diversity, sentence complexity, nominalization, presence of modals, epistemic and discourse markers. Those are motivated by previous work: Weiss et al. 25 observe the correlation between measures of lexical, syntactic and discourse complexities to the essay gradings of German high-school examinations while McNamara et al. 45 explore cohesion (indicated, among other things, by connectives), syntactic complexity and lexical diversity in relation to the essay scoring.
Lexical diversity
We identify vocabulary richness by using a well-established measure of textual, lexical diversity (MTLD) 46 which is often used in the field of automated essay grading 25 , 45 , 47 . It takes into account the number of unique words but unlike the best-known measure of lexical diversity, the type-token ratio (TTR), it is not as sensitive to the difference in the length of the texts. In fact, Koizumi and In’nami 48 find it to be least affected by the differences in the length of the texts compared to some other measures of lexical diversity. This is relevant to us due to the difference in average length between the human-written and ChatGPT-generated essays.
Syntactic complexity
We use two measures in order to evaluate the syntactic complexity of the essays. One is based on the maximum depth of the sentence dependency tree which is produced using the spaCy 3.4.2 dependency parser 49 (‘Syntactic complexity (depth)’). For the second measure, we adopt an approach similar in nature to the one by Weiss et al. 25 who use clause structure to evaluate syntactic complexity. In our case, we count the number of conjuncts, clausal modifiers of nouns, adverbial clause modifiers, clausal complements, clausal subjects, and parataxes (‘Syntactic complexity (clauses)’). The supplementary material in S2 shows the difference between sentence complexity based on two examples from the data.
Nominalization is a common feature of a more scientific style of writing 50 and is used as an additional measure for syntactic complexity. In order to explore this feature, we count occurrences of nouns with suffixes such as ‘-ion’, ‘-ment’, ‘-ance’ and a few others which are known to transform verbs into nouns.
Semantic properties
Both modals and epistemic markers signal the commitment of the writer to their statement. We identify modals using the POS-tagging module provided by spaCy as well as a list of epistemic expressions of modality, such as ‘definitely’ and ‘potentially’, also used in other approaches to identifying semantic properties 51 . For epistemic markers we adopt an empirically-driven approach and utilize the epistemic markers identified in a corpus of dialogical argumentation by Hautli-Janisz et al. 52 . We consider expressions such as ‘I think’, ‘it is believed’ and ‘in my opinion’ to be epistemic.
Discourse properties
Discourse markers can be used to measure the coherence quality of a text. This has been explored by Somasundaran et al. 53 who use discourse markers to evaluate the story-telling aspect of student writing while Nadeem et al. 54 incorporated them in their deep learning-based approach to automated essay scoring. In the present paper, we employ the PDTB list of discourse markers 55 which we adjust to exclude words that are often used for purposes other than indicating discourse relations, such as ‘like’, ‘for’, ‘in’ etc.
Statistical methods
We use a within-subjects design for our study. Each participant was shown six randomly selected essays. Results were submitted to the survey system after each essay was completed, in case participants ran out of time and did not finish scoring all six essays. Cronbach’s \(\alpha\) 56 allows us to determine the inter-rater reliability for the rating criterion and data source (human, ChatGPT-3, ChatGPT-4) in order to understand the reliability of our data not only overall, but also for each data source and rating criterion. We use two-sided Wilcoxon-rank-sum tests 57 to confirm the significance of the differences between the data sources for each criterion. We use the same tests to determine the significance of the linguistic characteristics. This results in three comparisons (human vs. ChatGPT-3, human vs. ChatGPT-4, ChatGPT-3 vs. ChatGPT-4) for each of the seven rating criteria and each of the seven linguistic characteristics, i.e. 42 tests. We use the Holm-Bonferroni method 58 for the correction for multiple tests to achieve a family-wise error rate of 0.05. We report the effect size using Cohen’s d 59 . While our data is not perfectly normal, it also does not have severe outliers, so we prefer the clear interpretation of Cohen’s d over the slightly more appropriate, but less accessible non-parametric effect size measures. We report point plots with estimates of the mean scores for each data source and criterion, incl. the 95% confidence interval of these mean values. The confidence intervals are estimated in a non-parametric manner based on bootstrap sampling. We further visualize the distribution for each criterion using violin plots to provide a visual indicator of the spread of the data (see Supplementary material S4 ).
Further, we use the self-assessment of the English skills and confidence in the essay ratings as confounding variables. Through this, we determine if ratings are affected by the language skills or confidence, instead of the actual quality of the essays. We control for the impact of these by measuring Pearson’s correlation coefficient r 60 between the self-assessments and the ratings. We also determine whether the linguistic features are correlated with the ratings as expected. The sentence complexity (both tree depth and dependency clauses), as well as the nominalization, are indicators of the complexity of the language. Similarly, the use of discourse markers should signal a proper logical structure. Finally, a large lexical diversity should be correlated with the ratings for the vocabulary. Same as above, we measure Pearson’s r . We use a two-sided test for the significance based on a \(\beta\) -distribution that models the expected correlations as implemented by scipy 61 . Same as above, we use the Holm-Bonferroni method to account for multiple tests. However, we note that it is likely that all—even tiny—correlations are significant given our amount of data. Consequently, our interpretation of these results focuses on the strength of the correlations.
Our statistical analysis of the data is implemented in Python. We use pandas 1.5.3 and numpy 1.24.2 for the processing of data, pingouin 0.5.3 for the calculation of Cronbach’s \(\alpha\) , scipy 1.10.1 for the Wilcoxon-rank-sum tests Pearson’s r , and seaborn 0.12.2 for the generation of plots, incl. the calculation of error bars that visualize the confidence intervals.
Out of the 111 teachers who completed the questionnaire, 108 rated all six essays, one rated five essays, one rated two essays, and one rated only one essay. This results in 658 ratings for 270 essays (90 topics for each essay type: human-, ChatGPT-3-, ChatGPT-4-generated), with three ratings for 121 essays, two ratings for 144 essays, and one rating for five essays. The inter-rater agreement is consistently excellent ( \(\alpha >0.9\) ), with the exception of language mastery where we have good agreement ( \(\alpha =0.89\) , see Table 2 ). Further, the correlation analysis depicted in supplementary material S4 shows weak positive correlations ( \(r \in 0.11, 0.28]\) ) between the self-assessment for the English skills, respectively the self-assessment for the confidence in ratings and the actual ratings. Overall, this indicates that our ratings are reliable estimates of the actual quality of the essays with a potential small tendency that confidence in ratings and language skills yields better ratings, independent of the data source.
Table 2 and supplementary material S4 characterize the distribution of the ratings for the essays, grouped by the data source. We observe that for all criteria, we have a clear order of the mean values, with students having the worst ratings, ChatGPT-3 in the middle rank, and ChatGPT-4 with the best performance. We further observe that the standard deviations are fairly consistent and slightly larger than one, i.e. the spread is similar for all ratings and essays. This is further supported by the visual analysis of the violin plots.
The statistical analysis of the ratings reported in Table 4 shows that differences between the human-written essays and the ones generated by both ChatGPT models are significant. The effect sizes for human versus ChatGPT-3 essays are between 0.52 and 1.15, i.e. a medium ( \(d \in [0.5,0.8)\) ) to large ( \(d \in [0.8, 1.2)\) ) effect. On the one hand, the smallest effects are observed for the expressiveness and complexity, i.e. when it comes to the overall comprehensiveness and complexity of the sentence structures, the differences between the humans and the ChatGPT-3 model are smallest. On the other hand, the difference in language mastery is larger than all other differences, which indicates that humans are more prone to making mistakes when writing than the NLG models. The magnitude of differences between humans and ChatGPT-4 is larger with effect sizes between 0.88 and 1.43, i.e., a large to very large ( \(d \in [1.2, 2)\) ) effect. Same as for ChatGPT-3, the differences are smallest for expressiveness and complexity and largest for language mastery. Please note that the difference in language mastery between humans and both GPT models does not mean that the humans have low scores for language mastery (M=3.90), but rather that the NLG models have exceptionally high scores (M=5.03 for ChatGPT-3, M=5.25 for ChatGPT-4).
When we consider the differences between the two GPT models, we observe that while ChatGPT-4 has consistently higher mean values for all criteria, only the differences for logic and composition, vocabulary and text linking, and complexity are significant. The effect sizes are between 0.45 and 0.5, i.e. small ( \(d \in [0.2, 0.5)\) ) and medium. Thus, while GPT-4 seems to be an improvement over GPT-3.5 in general, the only clear indicator of this is a better and clearer logical composition and more complex writing with a more diverse vocabulary.
We also observe significant differences in the distribution of linguistic characteristics between all three groups (see Table 3 ). Sentence complexity (depth) is the only category without a significant difference between humans and ChatGPT-3, as well as ChatGPT-3 and ChatGPT-4. There is also no significant difference in the category of discourse markers between humans and ChatGPT-3. The magnitude of the effects varies a lot and is between 0.39 and 1.93, i.e., between small ( \(d \in [0.2, 0.5)\) ) and very large. However, in comparison to the ratings, there is no clear tendency regarding the direction of the differences. For instance, while the ChatGPT models write more complex sentences and use more nominalizations, humans tend to use more modals and epistemic markers instead. The lexical diversity of humans is higher than that of ChatGPT-3 but lower than that of ChatGPT-4. While there is no difference in the use of discourse markers between humans and ChatGPT-3, ChatGPT-4 uses significantly fewer discourse markers.
We detect the expected positive correlations between the complexity ratings and the linguistic markers for sentence complexity ( \(r=0.16\) for depth, \(r=0.19\) for clauses) and nominalizations ( \(r=0.22\) ). However, we observe a negative correlation between the logic ratings and the discourse markers ( \(r=-0.14\) ), which counters our intuition that more frequent use of discourse indicators makes a text more logically coherent. However, this is in line with previous work: McNamara et al. 45 also find no indication that the use of cohesion indices such as discourse connectives correlates with high- and low-proficiency essays. Finally, we observe the expected positive correlation between the ratings for the vocabulary and the lexical diversity ( \(r=0.12\) ). All observed correlations are significant. However, we note that the strength of all these correlations is weak and that the significance itself should not be over-interpreted due to the large sample size.
Our results provide clear answers to the first two research questions that consider the quality of the generated essays: ChatGPT performs well at writing argumentative student essays and outperforms the quality of the human-written essays significantly. The ChatGPT-4 model has (at least) a large effect and is on average about one point better than humans on a seven-point Likert scale.
Regarding the third research question, we find that there are significant linguistic differences between humans and AI-generated content. The AI-generated essays are highly structured, which for instance is reflected by the identical beginnings of the concluding sections of all ChatGPT essays (‘In conclusion, [...]’). The initial sentences of each essay are also very similar starting with a general statement using the main concepts of the essay topics. Although this corresponds to the general structure that is sought after for argumentative essays, it is striking to see that the ChatGPT models are so rigid in realizing this, whereas the human-written essays are looser in representing the guideline on the linguistic surface. Moreover, the linguistic fingerprint has the counter-intuitive property that the use of discourse markers is negatively correlated with logical coherence. We believe that this might be due to the rigid structure of the generated essays: instead of using discourse markers, the AI models provide a clear logical structure by separating the different arguments into paragraphs, thereby reducing the need for discourse markers.
Our data also shows that hallucinations are not a problem in the setting of argumentative essay writing: the essay topics are not really about factual correctness, but rather about argumentation and critical reflection on general concepts which seem to be contained within the knowledge of the AI model. The stochastic nature of the language generation is well-suited for this kind of task, as different plausible arguments can be seen as a sampling from all available arguments for a topic. Nevertheless, we need to perform a more systematic study of the argumentative structures in order to better understand the difference in argumentation between human-written and ChatGPT-generated essay content. Moreover, we also cannot rule out that subtle hallucinations may have been overlooked during the ratings. There are also essays with a low rating for the criteria related to factual correctness, indicating that there might be cases where the AI models still have problems, even if they are, on average, better than the students.
One of the issues with evaluations of the recent large-language models is not accounting for the impact of tainted data when benchmarking such models. While it is certainly possible that the essays that were sourced by Stab and Gurevych 41 from the internet were part of the training data of the GPT models, the proprietary nature of the model training means that we cannot confirm this. However, we note that the generated essays did not resemble the corpus of human essays at all. Moreover, the topics of the essays are general in the sense that any human should be able to reason and write about these topics, just by understanding concepts like ‘cooperation’. Consequently, a taint on these general topics, i.e. the fact that they might be present in the data, is not only possible but is actually expected and unproblematic, as it relates to the capability of the models to learn about concepts, rather than the memorization of specific task solutions.
While we did everything to ensure a sound construct and a high validity of our study, there are still certain issues that may affect our conclusions. Most importantly, neither the writers of the essays, nor their raters, were English native speakers. However, the students purposefully used a forum for English writing frequented by native speakers to ensure the language and content quality of their essays. This indicates that the resulting essays are likely above average for non-native speakers, as they went through at least one round of revisions with the help of native speakers. The teachers were informed that part of the training would be in English to prevent registrations from people without English language skills. Moreover, the self-assessment of the language skills was only weakly correlated with the ratings, indicating that the threat to the soundness of our results is low. While we cannot definitively rule out that our results would not be reproducible with other human raters, the high inter-rater agreement indicates that this is unlikely.
However, our reliance on essays written by non-native speakers affects the external validity and the generalizability of our results. It is certainly possible that native speaking students would perform better in the criteria related to language skills, though it is unclear by how much. However, the language skills were particular strengths of the AI models, meaning that while the difference might be smaller, it is still reasonable to conclude that the AI models would have at least comparable performance to humans, but possibly still better performance, just with a smaller gap. While we cannot rule out a difference for the content-related criteria, we also see no strong argument why native speakers should have better arguments than non-native speakers. Thus, while our results might not fully translate to native speakers, we see no reason why aspects regarding the content should not be similar. Further, our results were obtained based on high-school-level essays. Native and non-native speakers with higher education degrees or experts in fields would likely also achieve a better performance, such that the difference in performance between the AI models and humans would likely also be smaller in such a setting.
We further note that the essay topics may not be an unbiased sample. While Stab and Gurevych 41 randomly sampled the essays from the writing feedback section of an essay forum, it is unclear whether the essays posted there are representative of the general population of essay topics. Nevertheless, we believe that the threat is fairly low because our results are consistent and do not seem to be influenced by certain topics. Further, we cannot with certainty conclude how our results generalize beyond ChatGPT-3 and ChatGPT-4 to similar models like Bard ( https://bard.google.com/?hl=en ) Alpaca, and Dolly. Especially the results for linguistic characteristics are hard to predict. However, since—to the best of our knowledge and given the proprietary nature of some of these models—the general approach to how these models work is similar and the trends for essay quality should hold for models with comparable size and training procedures.
Finally, we want to note that the current speed of progress with generative AI is extremely fast and we are studying moving targets: ChatGPT 3.5 and 4 today are already not the same as the models we studied. Due to a lack of transparency regarding the specific incremental changes, we cannot know or predict how this might affect our results.
Our results provide a strong indication that the fear many teaching professionals have is warranted: the way students do homework and teachers assess it needs to change in a world of generative AI models. For non-native speakers, our results show that when students want to maximize their essay grades, they could easily do so by relying on results from AI models like ChatGPT. The very strong performance of the AI models indicates that this might also be the case for native speakers, though the difference in language skills is probably smaller. However, this is not and cannot be the goal of education. Consequently, educators need to change how they approach homework. Instead of just assigning and grading essays, we need to reflect more on the output of AI tools regarding their reasoning and correctness. AI models need to be seen as an integral part of education, but one which requires careful reflection and training of critical thinking skills.
Furthermore, teachers need to adapt strategies for teaching writing skills: as with the use of calculators, it is necessary to critically reflect with the students on when and how to use those tools. For instance, constructivists 62 argue that learning is enhanced by the active design and creation of unique artifacts by students themselves. In the present case this means that, in the long term, educational objectives may need to be adjusted. This is analogous to teaching good arithmetic skills to younger students and then allowing and encouraging students to use calculators freely in later stages of education. Similarly, once a sound level of literacy has been achieved, strongly integrating AI models in lesson plans may no longer run counter to reasonable learning goals.
In terms of shedding light on the quality and structure of AI-generated essays, this paper makes an important contribution by offering an independent, large-scale and statistically sound account of essay quality, comparing human-written and AI-generated texts. By comparing different versions of ChatGPT, we also offer a glance into the development of these models over time in terms of their linguistic properties and the quality they exhibit. Our results show that while the language generated by ChatGPT is considered very good by humans, there are also notable structural differences, e.g. in the use of discourse markers. This demonstrates that an in-depth consideration not only of the capabilities of generative AI models is required (i.e. which tasks can they be used for), but also of the language they generate. For example, if we read many AI-generated texts that use fewer discourse markers, it raises the question if and how this would affect our human use of discourse markers. Understanding how AI-generated texts differ from human-written enables us to look for these differences, to reason about their potential impact, and to study and possibly mitigate this impact.
Data availability
The datasets generated during and/or analysed during the current study are available in the Zenodo repository, https://doi.org/10.5281/zenodo.8343644
Code availability
All materials are available online in form of a replication package that contains the data and the analysis code, https://doi.org/10.5281/zenodo.8343644 .
Ouyang, L. et al. Training language models to follow instructions with human feedback (2022). arXiv:2203.02155 .
Ruby, D. 30+ detailed chatgpt statistics–users & facts (sep 2023). https://www.demandsage.com/chatgpt-statistics/ (2023). Accessed 09 June 2023.
Leahy, S. & Mishra, P. TPACK and the Cambrian explosion of AI. In Society for Information Technology & Teacher Education International Conference , (ed. Langran, E.) 2465–2469 (Association for the Advancement of Computing in Education (AACE), 2023).
Ortiz, S. Need an ai essay writer? here’s how chatgpt (and other chatbots) can help. https://www.zdnet.com/article/how-to-use-chatgpt-to-write-an-essay/ (2023). Accessed 09 June 2023.
Openai chat interface. https://chat.openai.com/ . Accessed 09 June 2023.
OpenAI. Gpt-4 technical report (2023). arXiv:2303.08774 .
Brown, T. B. et al. Language models are few-shot learners (2020). arXiv:2005.14165 .
Wang, B. Mesh-Transformer-JAX: Model-Parallel Implementation of Transformer Language Model with JAX. https://github.com/kingoflolz/mesh-transformer-jax (2021).
Wei, J. et al. Finetuned language models are zero-shot learners. In International Conference on Learning Representations (2022).
Taori, R. et al. Stanford alpaca: An instruction-following llama model. https://github.com/tatsu-lab/stanford_alpaca (2023).
Cai, Z. G., Haslett, D. A., Duan, X., Wang, S. & Pickering, M. J. Does chatgpt resemble humans in language use? (2023). arXiv:2303.08014 .
Mahowald, K. A discerning several thousand judgments: Gpt-3 rates the article + adjective + numeral + noun construction (2023). arXiv:2301.12564 .
Dentella, V., Murphy, E., Marcus, G. & Leivada, E. Testing ai performance on less frequent aspects of language reveals insensitivity to underlying meaning (2023). arXiv:2302.12313 .
Guo, B. et al. How close is chatgpt to human experts? comparison corpus, evaluation, and detection (2023). arXiv:2301.07597 .
Zhao, W. et al. Is chatgpt equipped with emotional dialogue capabilities? (2023). arXiv:2304.09582 .
Keim, D. A. & Oelke, D. Literature fingerprinting : A new method for visual literary analysis. In 2007 IEEE Symposium on Visual Analytics Science and Technology , 115–122, https://doi.org/10.1109/VAST.2007.4389004 (IEEE, 2007).
El-Assady, M. et al. Interactive visual analysis of transcribed multi-party discourse. In Proceedings of ACL 2017, System Demonstrations , 49–54 (Association for Computational Linguistics, Vancouver, Canada, 2017).
Mennatallah El-Assady, A. H.-J. & Butt, M. Discourse maps - feature encoding for the analysis of verbatim conversation transcripts. In Visual Analytics for Linguistics , vol. CSLI Lecture Notes, Number 220, 115–147 (Stanford: CSLI Publications, 2020).
Matt Foulis, J. V. & Reed, C. Dialogical fingerprinting of debaters. In Proceedings of COMMA 2020 , 465–466, https://doi.org/10.3233/FAIA200536 (Amsterdam: IOS Press, 2020).
Matt Foulis, J. V. & Reed, C. Interactive visualisation of debater identification and characteristics. In Proceedings of the COMMA workshop on Argument Visualisation, COMMA , 1–7 (2020).
Chatzipanagiotidis, S., Giagkou, M. & Meurers, D. Broad linguistic complexity analysis for Greek readability classification. In Proceedings of the 16th Workshop on Innovative Use of NLP for Building Educational Applications , 48–58 (Association for Computational Linguistics, Online, 2021).
Ajili, M., Bonastre, J.-F., Kahn, J., Rossato, S. & Bernard, G. FABIOLE, a speech database for forensic speaker comparison. In Proceedings of the Tenth International Conference on Language Resources and Evaluation (LREC’16) , 726–733 (European Language Resources Association (ELRA), Portorož, Slovenia, 2016).
Deutsch, T., Jasbi, M. & Shieber, S. Linguistic features for readability assessment. In Proceedings of the Fifteenth Workshop on Innovative Use of NLP for Building Educational Applications , 1–17, https://doi.org/10.18653/v1/2020.bea-1.1 (Association for Computational Linguistics, Seattle, WA, USA \(\rightarrow\) Online, 2020).
Fiacco, J., Jiang, S., Adamson, D. & Rosé, C. Toward automatic discourse parsing of student writing motivated by neural interpretation. In Proceedings of the 17th Workshop on Innovative Use of NLP for Building Educational Applications (BEA 2022) , 204–215, https://doi.org/10.18653/v1/2022.bea-1.25 (Association for Computational Linguistics, Seattle, Washington, 2022).
Weiss, Z., Riemenschneider, A., Schröter, P. & Meurers, D. Computationally modeling the impact of task-appropriate language complexity and accuracy on human grading of German essays. In Proceedings of the Fourteenth Workshop on Innovative Use of NLP for Building Educational Applications , 30–45, https://doi.org/10.18653/v1/W19-4404 (Association for Computational Linguistics, Florence, Italy, 2019).
Yang, F., Dragut, E. & Mukherjee, A. Predicting personal opinion on future events with fingerprints. In Proceedings of the 28th International Conference on Computational Linguistics , 1802–1807, https://doi.org/10.18653/v1/2020.coling-main.162 (International Committee on Computational Linguistics, Barcelona, Spain (Online), 2020).
Tumarada, K. et al. Opinion prediction with user fingerprinting. In Proceedings of the International Conference on Recent Advances in Natural Language Processing (RANLP 2021) , 1423–1431 (INCOMA Ltd., Held Online, 2021).
Rocca, R. & Yarkoni, T. Language as a fingerprint: Self-supervised learning of user encodings using transformers. In Findings of the Association for Computational Linguistics: EMNLP . 1701–1714 (Association for Computational Linguistics, Abu Dhabi, United Arab Emirates, 2022).
Aiyappa, R., An, J., Kwak, H. & Ahn, Y.-Y. Can we trust the evaluation on chatgpt? (2023). arXiv:2303.12767 .
Yeadon, W., Inyang, O.-O., Mizouri, A., Peach, A. & Testrow, C. The death of the short-form physics essay in the coming ai revolution (2022). arXiv:2212.11661 .
TURING, A. M. I.-COMPUTING MACHINERY AND INTELLIGENCE. Mind LIX , 433–460, https://doi.org/10.1093/mind/LIX.236.433 (1950). https://academic.oup.com/mind/article-pdf/LIX/236/433/30123314/lix-236-433.pdf .
Kortemeyer, G. Could an artificial-intelligence agent pass an introductory physics course? (2023). arXiv:2301.12127 .
Kung, T. H. et al. Performance of chatgpt on usmle: Potential for ai-assisted medical education using large language models. PLOS Digital Health 2 , 1–12. https://doi.org/10.1371/journal.pdig.0000198 (2023).
Article Google Scholar
Frieder, S. et al. Mathematical capabilities of chatgpt (2023). arXiv:2301.13867 .
Yuan, Z., Yuan, H., Tan, C., Wang, W. & Huang, S. How well do large language models perform in arithmetic tasks? (2023). arXiv:2304.02015 .
Touvron, H. et al. Llama: Open and efficient foundation language models (2023). arXiv:2302.13971 .
Chung, H. W. et al. Scaling instruction-finetuned language models (2022). arXiv:2210.11416 .
Workshop, B. et al. Bloom: A 176b-parameter open-access multilingual language model (2023). arXiv:2211.05100 .
Spencer, S. T., Joshi, V. & Mitchell, A. M. W. Can ai put gamma-ray astrophysicists out of a job? (2023). arXiv:2303.17853 .
Cherian, A., Peng, K.-C., Lohit, S., Smith, K. & Tenenbaum, J. B. Are deep neural networks smarter than second graders? (2023). arXiv:2212.09993 .
Stab, C. & Gurevych, I. Annotating argument components and relations in persuasive essays. In Proceedings of COLING 2014, the 25th International Conference on Computational Linguistics: Technical Papers , 1501–1510 (Dublin City University and Association for Computational Linguistics, Dublin, Ireland, 2014).
Essay forum. https://essayforum.com/ . Last-accessed: 2023-09-07.
Common european framework of reference for languages (cefr). https://www.coe.int/en/web/common-european-framework-reference-languages . Accessed 09 July 2023.
Kmk guidelines for essay assessment. http://www.kmk-format.de/material/Fremdsprachen/5-3-2_Bewertungsskalen_Schreiben.pdf . Accessed 09 July 2023.
McNamara, D. S., Crossley, S. A. & McCarthy, P. M. Linguistic features of writing quality. Writ. Commun. 27 , 57–86 (2010).
McCarthy, P. M. & Jarvis, S. Mtld, vocd-d, and hd-d: A validation study of sophisticated approaches to lexical diversity assessment. Behav. Res. Methods 42 , 381–392 (2010).
Article PubMed Google Scholar
Dasgupta, T., Naskar, A., Dey, L. & Saha, R. Augmenting textual qualitative features in deep convolution recurrent neural network for automatic essay scoring. In Proceedings of the 5th Workshop on Natural Language Processing Techniques for Educational Applications , 93–102 (2018).
Koizumi, R. & In’nami, Y. Effects of text length on lexical diversity measures: Using short texts with less than 200 tokens. System 40 , 554–564 (2012).
spacy industrial-strength natural language processing in python. https://spacy.io/ .
Siskou, W., Friedrich, L., Eckhard, S., Espinoza, I. & Hautli-Janisz, A. Measuring plain language in public service encounters. In Proceedings of the 2nd Workshop on Computational Linguistics for Political Text Analysis (CPSS-2022) (Potsdam, Germany, 2022).
El-Assady, M. & Hautli-Janisz, A. Discourse Maps - Feature Encoding for the Analysis of Verbatim Conversation Transcripts (CSLI lecture notes (CSLI Publications, Center for the Study of Language and Information, 2019).
Hautli-Janisz, A. et al. QT30: A corpus of argument and conflict in broadcast debate. In Proceedings of the Thirteenth Language Resources and Evaluation Conference , 3291–3300 (European Language Resources Association, Marseille, France, 2022).
Somasundaran, S. et al. Towards evaluating narrative quality in student writing. Trans. Assoc. Comput. Linguist. 6 , 91–106 (2018).
Nadeem, F., Nguyen, H., Liu, Y. & Ostendorf, M. Automated essay scoring with discourse-aware neural models. In Proceedings of the Fourteenth Workshop on Innovative Use of NLP for Building Educational Applications , 484–493, https://doi.org/10.18653/v1/W19-4450 (Association for Computational Linguistics, Florence, Italy, 2019).
Prasad, R. et al. The Penn Discourse TreeBank 2.0. In Proceedings of the Sixth International Conference on Language Resources and Evaluation (LREC’08) (European Language Resources Association (ELRA), Marrakech, Morocco, 2008).
Cronbach, L. J. Coefficient alpha and the internal structure of tests. Psychometrika 16 , 297–334. https://doi.org/10.1007/bf02310555 (1951).
Article MATH Google Scholar
Wilcoxon, F. Individual comparisons by ranking methods. Biom. Bull. 1 , 80–83 (1945).
Holm, S. A simple sequentially rejective multiple test procedure. Scand. J. Stat. 6 , 65–70 (1979).
MathSciNet MATH Google Scholar
Cohen, J. Statistical power analysis for the behavioral sciences (Academic press, 2013).
Freedman, D., Pisani, R. & Purves, R. Statistics (international student edition). Pisani, R. Purves, 4th edn. WW Norton & Company, New York (2007).
Scipy documentation. https://docs.scipy.org/doc/scipy/reference/generated/scipy.stats.pearsonr.html . Accessed 09 June 2023.
Windschitl, M. Framing constructivism in practice as the negotiation of dilemmas: An analysis of the conceptual, pedagogical, cultural, and political challenges facing teachers. Rev. Educ. Res. 72 , 131–175 (2002).
Download references
Open Access funding enabled and organized by Projekt DEAL.
Author information
Authors and affiliations.
Faculty of Computer Science and Mathematics, University of Passau, Passau, Germany
Steffen Herbold, Annette Hautli-Janisz, Ute Heuer, Zlata Kikteva & Alexander Trautsch
You can also search for this author in PubMed Google Scholar
Contributions
S.H., A.HJ., and U.H. conceived the experiment; S.H., A.HJ, and Z.K. collected the essays from ChatGPT; U.H. recruited the study participants; S.H., A.HJ., U.H. and A.T. conducted the training session and questionnaire; all authors contributed to the analysis of the results, the writing of the manuscript, and review of the manuscript.
Corresponding author
Correspondence to Steffen Herbold .
Ethics declarations
Competing interests.
The authors declare no competing interests.
Additional information
Publisher's note.
Springer Nature remains neutral with regard to jurisdictional claims in published maps and institutional affiliations.
Supplementary Information
Supplementary information 1., supplementary information 2., supplementary information 3., supplementary tables., supplementary figures., rights and permissions.
Open Access This article is licensed under a Creative Commons Attribution 4.0 International License, which permits use, sharing, adaptation, distribution and reproduction in any medium or format, as long as you give appropriate credit to the original author(s) and the source, provide a link to the Creative Commons licence, and indicate if changes were made. The images or other third party material in this article are included in the article's Creative Commons licence, unless indicated otherwise in a credit line to the material. If material is not included in the article's Creative Commons licence and your intended use is not permitted by statutory regulation or exceeds the permitted use, you will need to obtain permission directly from the copyright holder. To view a copy of this licence, visit http://creativecommons.org/licenses/by/4.0/ .
Reprints and permissions
About this article
Cite this article.
Herbold, S., Hautli-Janisz, A., Heuer, U. et al. A large-scale comparison of human-written versus ChatGPT-generated essays. Sci Rep 13 , 18617 (2023). https://doi.org/10.1038/s41598-023-45644-9
Download citation
Received : 01 June 2023
Accepted : 22 October 2023
Published : 30 October 2023
DOI : https://doi.org/10.1038/s41598-023-45644-9
Share this article
Anyone you share the following link with will be able to read this content:
Sorry, a shareable link is not currently available for this article.
Provided by the Springer Nature SharedIt content-sharing initiative
This article is cited by
Defense against adversarial attacks: robust and efficient compressed optimized neural networks.
- Insaf Kraidia
- Afifa Ghenai
- Samir Brahim Belhaouari
Scientific Reports (2024)
AI-driven translations for kidney transplant equity in Hispanic populations
- Oscar A. Garcia Valencia
- Charat Thongprayoon
- Wisit Cheungpasitporn
How will the state think with ChatGPT? The challenges of generative artificial intelligence for public administrations
- Thomas Cantens
AI & SOCIETY (2024)
By submitting a comment you agree to abide by our Terms and Community Guidelines . If you find something abusive or that does not comply with our terms or guidelines please flag it as inappropriate.
Quick links
- Explore articles by subject
- Guide to authors
- Editorial policies
Sign up for the Nature Briefing: AI and Robotics newsletter — what matters in AI and robotics research, free to your inbox weekly.

Home — Essay Samples — Entertainment — Television — Voice

Essays on Voice
My brothers voice characteristics, a study of the voice used in the poisonwood bible, made-to-order essay as fast as you need it.
Each essay is customized to cater to your unique preferences
+ experts online
The Power of Voice in "My Last Duchess"
Acceptance of artificial larynx among total laryngectomy patient: case studies , janie’s response to desertion in their eyes were watching god, vision through voice: the poetry of basho in the english language, let us write you an essay from scratch.
- 450+ experts on 30 subjects ready to help
- Custom essay delivered in as few as 3 hours
Narrative Voice in William Faulkner’s Absalom, Absalom
The effectiveness of the opening of the rise and fall of little voice, complexity in second person: how the narrator, or narrative voice, of aura is deceptively straightforward, mari’s character and use of language in scene seven of "the rise and fall of little voice", get a personalized essay in under 3 hours.
Expert-written essays crafted with your exact needs in mind
Analysis of The Film Beasts of No Nation
The mirror of simple souls: marguerite porete voice and use of gender, examining the voice of negation in a close reading of "goblin market", singing as a natural anti-depressant, the voices of the voiceless: comparing the poetry of langston hughes and countee cullen, our voice should be heard to stop child labor, extra-narrative voices and character agency in dead souls, the gorgeous voice of nina simone, the importance of several voices in extremely loud and incredibly close, how to improve your vocal skills, woman voice to challenge immorlarity, multiplicity of voices in purple hibiscus by chimamanda ngozi adichie, both poor and rich have an opportunity to voice feelings, doyle’s manipulation of language to create paddy’s voice: the grand national race, voice pollution: unheard consequences of noise pollution, relevant topics.
- A Class Divided
- Oprah Winfrey
- Breaking Bad
- Game of Thrones
- Grey's Anatomy
- Reality Television
- 13 Reasons Why
By clicking “Check Writers’ Offers”, you agree to our terms of service and privacy policy . We’ll occasionally send you promo and account related email
No need to pay just yet!
We use cookies to personalyze your web-site experience. By continuing we’ll assume you board with our cookie policy .
- Instructions Followed To The Letter
- Deadlines Met At Every Stage
- Unique And Plagiarism Free

- Your Voice 3
- Vocal Coach Approach
- Musical Theater Voice Pedagogy/a>
- Basics of Voice Science & Pedagogy
- Kinesthetic Voice Ped 2
- Great Teachers on Great Singing
- Singing: The Timeless Muse
- Songs & Arias (Lit for Teaching)
- Literature for Teaching
- Resonance in Singing
- Fundamentals of Great Vocal Technique
- Sing into your Sixties
- Most Rewarding Independent Music Studio
- Special Offers
- Combination Offers at 25% discount
- Contact info
- Tech Support
- Corrections
Now Available
Singing, the timeless muse, essays on the human voice, singing, and spirituality, compiled by darlene c. wiley.
ISBN: 978-1-7335060-0-7 Soft cover, 229 pages
We are currently closed for a brief holiday. Books and e-books will resume shipping on May 15, 2024
Proceeds from the sale of this book are contributed to New Music USA , a non-profit organization
Praise for Singing: The Timeless Muse
Singing: The Timeless Muse is an extraordinary collection of essays, bringing together writings about the act of singing, the mind, and spirit, and in fact what it means to be human. That our discipline is at a significant time of expansiveness and evolution is not to be argued. “Once we understand the complexity of singing, we can certainly broaden our scope of what it means to sing.” Indeed, Richard Miller’s quotation from a personal conversation in 1970 with Darlene Wiley foreshadowed continuing fascinations for his remaining days. How fortunate that his words were catalyst for this insightful book. Professor Wiley has assembled a significant body of essays and interviews by compelling, thought-provoking individuals.This is a book that will remain on my shelf, whether to ponder a single essay or to read again as a whole. It will inspire lovers of singing to move forward seeking solutions that not only enhance the traditional canon but also are inclusive of the breadth of vocal expression, learning, style, and divergent techniques. - Lorraine Manz , Professor of Voice, Oberlin College Conservatory of Music
As a composer of opera, I have often wished for a broader stylistic and expressive range from the singers who perform my works, and it has often felt that the narrowness of conservatory training—of what constitutes acceptable vocal practice—precludes such variety. Darlene Wiley's wonderful new book addresses this issue and much more with great insight and nuance. -Kevin Puts , Pulitzer Prize winning composer of the opera Silent Night
Introduction (Darlene C. Wiley) Singing, Speaking, and the Difference (Jeanette Bicknell) Singing and Signification (John M. Carvalho) The Etic Voice: An Ethnomusicological Perspective on Voice Research in Turkish Secular and Sacred Practices (Eve McPherson) To Become Human: A Comparative Investigation into Lena McLin´s Vocal Pedagogy and the Italian School of Vocologia Artistica (Gianpaolo Chiriacò) Play it and they Will Come: Re-Approching Gesture in Classical Music (Jesús A. Ramos-Kittrell) What do you believe is the future of singing? (Margo Garrett) Singing and the Multicultural Platform (Carolyn Sebron) The Voice in My Life (Robert S. Hatten) Teaching Voice in a 21st Century World (Graham Reynolds) The Imagined Voice: How Singing and Vocal Music Affect Me and My Work (Dan Welcher) Gary Powell in the Studio (Discussion between Mr. Powell and Darlene C. Wiley) An Interview with Andrea Clearfield (Andrea Clearfield and Darlene C. Wiley) Personal Notes on Coaching and Singing (Robert Spillman) Texting from the Stage: Singers as Communicators (Dan Kurland) We Care If You Listen (Kathleen Kelly) For the Love of the Voice: Toward a New Generation of Coaches (Richard Masters) The Nature of Singing (George Shirley) I Sing the Body Eclectic: Empowering the Process-Oriented Vocal Artis t (Estelí Gomez) An Essay on Singers (Lesley F. Childs, MD) Voice, the Music of the Soul (Lynn Helding)
Darlene Wiley , lyric coloratura soprano, began her career at the Staatstheater Darmstadt, performing over 65 roles in such operas as I Pagliacci, Die Zauberflöte, Don Pasquale, Tales of Hoffmann, La Traviata , and Le Nozze di Figaro . A veteran of over 1,500 opera performances, Ms. Wiley has sung at over 25 houses, including Mannheim, Wiesbaden, Kassel, Mainz, Ulm, and Kiel. She has been heralded as “a thrilling Nedda…. amazingly sung… passionately acted” ( Opern Welt ). “With sassy charm and convincing Chaplinesque style, this graceful soprano is the ideal Rossini coloratura” ( Frankfurter Allgemeine Zeitung ). She currently is Professor of Voice at the University of Texas at Austin, director of the Vocal Arts Lab, supervisor of the DMA Vocal Pedagogy Program, and founder/director of the Butler International Opera Competition. Several of her current and former students are emerging as artists in their own right, with appearances at the Staatsoper Berlin, Salzburg Festspiele, Washington Opera, Los Angeles Opera, St. Louis Opera, City Opera of New York, Austin Opera, Houston Grand Opera, Tulsa Opera, Amarillo Opera, Miami Opera, Staatstheater Braunschweig, Theater Hagen, Theater Regensburg, Orlando Opera, Seattle Opera, Palm Beach Opera, Miami Opera, Paris Opera, and Florida Grand Opera. Prof. Wiley holds a BME (Instrumental Emphasis) from the College of Wooster and MM in Voice from the University of Illinois.
- Boredom Makes Us Human

I n a recent article in the Financial Times, Markham Heid shares with us a peculiar life crisis. At 41, he has built what many would regard as the good life: he has a family; he is healthy, productive, and creative; he has time to travel, read, exercise, and see friends. Yet, he feels that “something is off.” He gives this state a variety of names, including mid-life melancholy, ennui, and despair. He also diagnoses it in others all around him. To fight against it, some of his friends have turned to ayahuasca retreats, others to fitness. What renders Heid’s malaise somewhat strange is that it does not seem to arise from anything specific. If Heid had lost his job, had no time for himself, or was struggling in his marriage, some of these feelings would seem less puzzling.
In the history of philosophy, there have been many attempts to understand such powerful but objectless feelings. Boredom , anxiety , and despair are some of the descriptions these moods have received. In the novel Nausea , the French existentialist philosopher Jean-Paul Sartre describes someone who mysteriously experiences that feeling whenever they are confronted with ordinary objects, like a pebble on the beach. The German philosopher Martin Heidegger describes an uncanny unease we may feel when we are bored and searching desperately for distractions. The Danish philosopher Søren Kierkegaard speaks of a silent despair in the background of our lives, a sense of discord or dread of an unknown something that can grab us momentarily.
Sadly, the philosophical descriptions of such moods have often been misunderstood as sombre or romantic moments of existential reflection where we recognize our mortality or the meaninglessness of life. Pictured in this way, these moments are bound to stay isolated from the anxiety, despair, and melancholy that we face in our ordinary life and seek help for. But if we look beyond the existentialist clichés, the philosophical ideas on such moods can offer a new way forward. What could Heid have learnt from the philosophers?
Moods of nothing
Despite Heid’s references to Heidegger, we do not read anything about the philosopher’s own ruminations of a very similar experience of flatness: a feeling that all things (and we ourselves) sink into indifference; a sense that things around us slip away or we slip away from ourselves; a malaise related to a vacant stillness. What is remarkable, for Heidegger, is that such intense affects arise despite the fact that nothing may have changed in our lives: one is still surrounded by the same people, events, and activities, but these do not engage us as they used to. It is this feature that makes him describe what he calls “anxiety” as a mood generated by nothing in particular.
This makes such feelings doubly unwelcome. Most of us can tolerate negative emotions if we see them as instrumental to something desirable—we do not run to a therapist to treat a fear if we think that it holds us back from doing something obviously risky. But unlike fear, what Heidegger calls anxiety and what Heid’s article describes do not protect us from anything specific. No wonder why Sigmund Freud called anxiety a “ riddle .”
But this view is too simplistic for Heidegger. It risks concealing both the value and meaning of the feelings he describes. First, the human emotional life is much more complex than a simple battle between positive and negative feelings, or useful and useless emotions. Second, objectless moods can teach us something significant not about specific risks or problems in our lives but about the fact that we have a life to live at all. Learning from them can allow us to find what Heidegger describes as a sense of peace and joy within the malaise.
What’s missing?
Heid says that “some essential aspect of life is missing or not sufficiently represented.” He ends up attributing his melancholy to the lack of new experiences. Kierkegaard calls this the illusion of “crop rotation,” the idea that changing the soil frequently can save us from boredom and despair.
But what really drives such moods is not the need for new experiences. It is not even the particulars of our individual lives or the culture we belong to, but that we have been given a life to live in the first place, the taste of possibility that comes with being alive. The kinds of questions that arise are not questions like “have I married the right person?” “will parenthood enrich my life?” or “do I have enough hobbies?” It is the more fundamental questions like “what does it mean to be human?” “what am I supposed to do with the fact that I was given a life?” and “what kind of life is possible for me?” that best explain our human tendency for anxiety, despair, or boredom .
This is why such moods are likely to appear as a mid-life crisis. With many of our life goals fulfilled, we start to wonder what life is for, what is possible for human existence, and what we are doing for it. Humans are inherently ambivalent toward possibility, attracted but also repelled by it. On one hand, we can experience it as a radical openness, an appreciation of our life as a gift. On the other, the open-endedness of possibility, the sense that one could always be doing more with their life, can create a great sense of agony about who we are and how we should go on.
Throwing us out of our everyday lives, such moods make us ponder existence itself. They are cases where who we are and what we are for becomes an issue for each one of us. These questions never assume a final answer. Hovering over our lives, they can always leave us with a sense of unease. Recognizing that these questions are there, and that they matter, can at least allow us to know what may be missing, even when all is good.
More Must-Reads From TIME
- Putin’s Enemies Are Struggling to Unite
- Women Say They Were Pressured Into Long-Term Birth Control
- What Student Photojournalists Saw at the Campus Protests
- Scientists Are Finding Out Just How Toxic Your Stuff Is
- John Mulaney Has What Late Night Needs
- The 100 Most Influential People of 2024
- Want Weekly Recs on What to Watch, Read, and More? Sign Up for Worth Your Time
Contact us at [email protected]

People without an inner voice have poorer verbal memory
Between 5-10 per cent of the population do not experience an inner voice.
The vast majority of people have an ongoing conversation with themselves, an inner voice, that plays an important role in their daily lives. But between 5-10 per cent of the population do not have the same experience of an inner voice, and they find it more difficult to perform certain verbal memory tasks, new research shows.
Previously, it was commonly assumed that having an inner voice had to be a human universal. But in recent years, researchers have become aware that not all people share this experience.
According to postdoc and linguist Johanne Nedergård from the University of Copenhagen, people describe the condition of living without an inner voice as time-consuming and difficult because they must spend time and effort translating their thoughts into words:
"Some say that they think in pictures and then translate the pictures into words when they need to say something. Others describe their brain as a well-functioning computer that just does not process thoughts verbally, and that the connection to loudspeaker and microphone is different from other people's. And those who say that there is something verbal going on inside their heads will typically describe it as words without sound."
Harder to remember words and rhymes
Johanne Nedergård and her colleague Gary Lupyan from the University of Wisconsin-Madison are the first researchers in the world to investigate whether the lack of an inner voice, or anendophasia as they have coined the condition, has any consequences for how these people solve problems, for example how they perform verbal memory tasks.
People who reported that they either experienced a high degree of inner voice or very little inner voice in everyday life were subjected to one experiment that aimed to determine whether there was a difference in their ability to remember language input and one about their ability to find rhyme words. The first experiment involved the participants remembering words in order -- words that were similar, either phonetically or in spelling, e.g. "bought," "caught," "taut" and "wart."
"It is a task that will be difficult for everyone, but our hypothesis was that it might be even more difficult if you did not have an inner voice because you have to repeat the words to yourself inside your head in order to remember them," Johanne Nedergård explains and continues:
"And this hypothesis turned out to be true: The participants without an inner voice were significantly worse at remembering the words. The same applied to an assignment in which the participants had to determine whether a pair of pictures contained words that rhyme, e.g. pictures of a sock and a clock. Here, too, it is crucial to be able to repeat the words in order to compare their sounds and thus determine whether they rhyme."
In two other experiments, in which Johanne Nedergård and Gary Lupyan tested the role of the inner voice in switching quickly between different tasks and distinguishing between figures that are very similar, they did not find any differences between the two groups. Despite the fact that previous studies indicate that language and the inner voice play a role in these types of experiments.
"Maybe people who don't have an inner voice have just learned to use other strategies. For example, some said that they tapped with their index finger when performing one type of task and with their middle finger when it was another type of task," Johanne Nedergård says.
The results of the two researchers' study have just been published in the article "Not everybody has an inner voice: Behavioural consequences of anendophasia" in the scientific journal Psychological Science .
Does it make a difference?
According to Johanne Nedergård, the differences in verbal memory that they have identified in their experiments will not be noticed in ordinary everyday conversations. And the question is, does not having an inner voice hold any practical or behavioural significance?
"The short answer is that we just don't know because we have only just begun to study it. But there is one field where we suspect that having an inner voice plays a role, and that is therapy; in the widely used cognitive behavioural therapy, for example, you need to identify and change adverse thought patterns, and having an inner voice may be very important in such a process. However, it is still uncertain whether differences in the experience of an inner voice are related to how people respond to different types of therapy," says Johanne Nedergård, who would like to continue her research to find out whether other language areas are affected if you do not have an inner voice.
"The experiments in which we found differences between the groups were about sound and being able to hear the words for themselves. I would like to study whether it is because they just do not experience the sound aspect of language, or whether they do not think at all in a linguistic format like most other people," she concludes.
About the study
Johanne Nedergård's and Gary Lupyan's study comprised almost a hundred participants, half of whom experienced having very little inner voice and the other half very much inner voice.
The participants were subjected to four experiments, e.g. remembering words in sequence and switching between different tasks. The study has been published in the scientific journal Psychological Science.
Johanne Nedergård and Gary Lupyan have dubbed the condition of having no inner voice anendophasia, which means without an inner voice.
- Language Acquisition
- Child Development
- Infant and Preschool Learning
- Intelligence
- Limbic system
- Left-handed
- Consumerism
- Memory-prediction framework
- Obsessive-compulsive personality disorder
- Double blind
Story Source:
Materials provided by University of Copenhagen - Faculty of Humanities . Note: Content may be edited for style and length.
Journal Reference :
- Johanne S. K. Nedergaard, Gary Lupyan. Not Everybody Has an Inner Voice: Behavioral Consequences of Anendophasia . Psychological Science , 2024; DOI: 10.1177/09567976241243004
Cite This Page :
Explore More
- Nature's 3D Printer: Bristle Worms
- Giant ' Cotton Candy' Planet
- A Young Whale's Journey
- No Inner Voice Linked to Poorer Verbal Memory
- Bird Flu A(H5N1) Transmitted from Cow to Human
- Universe's Oldest Stars in Our Galactic Backyard
- Polygenic Embryo Screening for IVF: Opinions
- VR With Cinematoghraphics More Engaging
- 2023 Was the Hottest Summer in 2000 Years
- Fastest Rate of CO2 Rise Over Last 50,000 Years
Trending Topics
Strange & offbeat.
Advertisement
Supported by
OpenAI Unveils New ChatGPT That Listens, Looks and Talks
Chatbots, image generators and voice assistants are gradually merging into a single technology with a conversational voice.
- Share full article

By Cade Metz
Reporting from San Francisco
As Apple and Google transform their voice assistants into chatbots, OpenAI is transforming its chatbot into a voice assistant.
On Monday, the San Francisco artificial intelligence start-up unveiled a new version of its ChatGPT chatbot that can receive and respond to voice commands, images and videos.
The company said the new app — based on an A.I. system called GPT-4o — juggles audio, images and video significantly faster than previous versions of the technology. The app will be available starting on Monday, free of charge, for both smartphones and desktop computers.
“We are looking at the future of the interaction between ourselves and machines,” said Mira Murati, the company’s chief technology officer.
The new app is part of a wider effort to combine conversational chatbots like ChatGPT with voice assistants like the Google Assistant and Apple’s Siri. As Google merges its Gemini chatbot with the Google Assistant, Apple is preparing a new version of Siri that is more conversational.
OpenAI said it would gradually share the technology with users “over the coming weeks.” This is the first time it has offered ChatGPT as a desktop application.
The company previously offered similar technologies from inside various free and paid products. Now, it has rolled them into a single system that is available across all its products.
During an event streamed on the internet, Ms. Murati and her colleagues showed off the new app as it responded to conversational voice commands, used a live video feed to analyze math problems written on a sheet of paper and read aloud playful stories that it had written on the fly.
The new app cannot generate video. But it can generate still images that represent frames of a video.
With the debut of ChatGPT in late 2022 , OpenAI showed that machines can handle requests more like people. In response to conversational text prompts, it could answer questions, write term papers and even generate computer code.
ChatGPT was not driven by a set of rules. It learned its skills by analyzing enormous amounts of text culled from across the internet, including Wikipedia articles, books and chat logs. Experts hailed the technology as a possible alterative to search engines like Google and voice assistants like Siri.
Newer versions of the technology have also learned from sounds, images and video. Researchers call this “multimodal A.I.” Essentially, companies like OpenAI began to combine chatbots with A.I. image , audio and video generators.
(The New York Times sued OpenAI and its partner, Microsoft, in December, claiming copyright infringement of news content related to A.I. systems.)
As companies combine chatbots with voice assistants, many hurdles remain. Because chatbots learn their skills from internet data, they are prone to mistakes. Sometimes, they make up information entirely — a phenomenon that A.I. researchers call “ hallucination .” Those flaws are migrating into voice assistants.
While chatbots can generate convincing language, they are less adept at taking actions like scheduling a meeting or booking a plane flight. But companies like OpenAI are working to transform them into “ A.I. agents ” that can reliably handle such tasks.
OpenAI previously offered a version of ChatGPT that could accept voice commands and respond with voice. But it was a patchwork of three different A.I. technologies: one that converted voice to text, one that generated a text response and one that converted this text into a synthetic voice.
The new app is based on a single A.I. technology — GPT-4o — that can accept and generate text, sounds and images. This means that the technology is more efficient, and the company can afford to offer it to users for free, Ms. Murati said.
“Before, you had all this latency that was the result of three models working together,” Ms. Murati said in an interview with The Times. “You want to have the experience we’re having — where we can have this very natural dialogue.”
An earlier version of this article misstated the day when OpenAI introduced its new version of ChatGPT. It was Monday, not Tuesday.
How we handle corrections
Cade Metz writes about artificial intelligence, driverless cars, robotics, virtual reality and other emerging areas of technology. More about Cade Metz
Explore Our Coverage of Artificial Intelligence
News and Analysis
As experts warn that A.I.-generated images, audio and video could influence the 2024 elections, OpenAI is releasing a tool designed to detect content created by DALL-E , its popular image generator.
American and Chinese diplomats plan to meet in Geneva to begin what amounts to the first, tentative arms control talks over the use of A.I.
Wayve, a London maker of A.I. systems for autonomous vehicles, said that it had raised $1 billion , an illustration of investor optimism about A.I.’s ability to reshape industries.
The Age of A.I.
A new category of apps promises to relieve parents of drudgery, with an assist from A.I. But a family’s grunt work is more human, and valuable, than it seems.
Despite Mark Zuckerberg’s hope for Meta’s A.I. assistant to be the smartest , it struggles with facts, numbers and web search.
Much as ChatGPT generates poetry, a new A.I. system devises blueprints for microscopic mechanisms that can edit your DNA.
Which A.I. system writes the best computer code or generates the most realistic image? Right now, there’s no easy way to answer those questions, our technology columnist writes .
MIT Technology Review
- Newsletters
Google helped make an exquisitely detailed map of a tiny piece of the human brain
A small brain sample was sliced into 5,000 pieces, and machine learning helped stitch it back together.
- Cassandra Willyard archive page
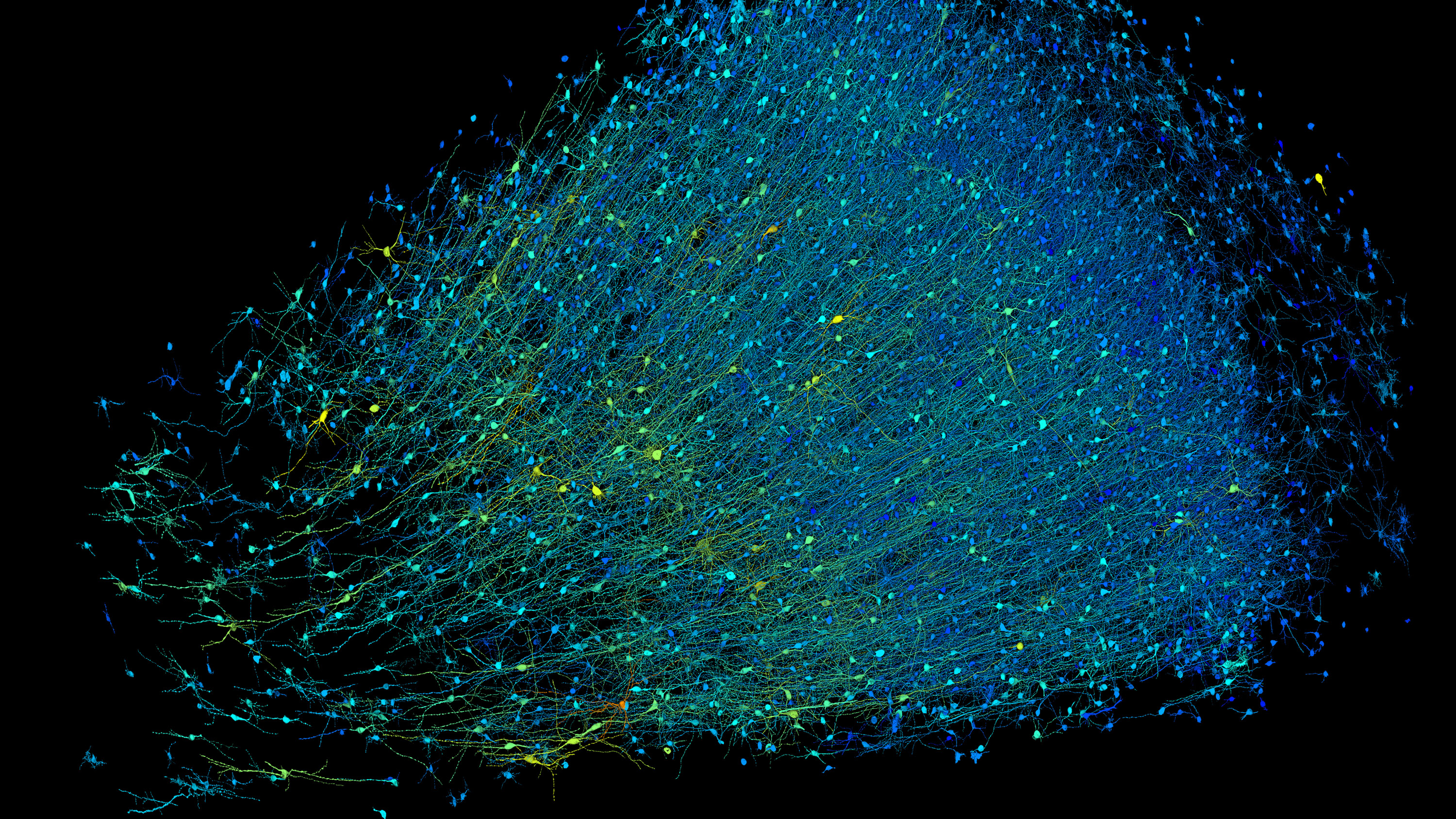
A team led by scientists from Harvard and Google has created a 3D, nanoscale-resolution map of a single cubic millimeter of the human brain. Although the map covers just a fraction of the organ—a whole brain is a million times larger—that piece contains roughly 57,000 cells, about 230 millimeters of blood vessels, and nearly 150 million synapses. It is currently the highest-resolution picture of the human brain ever created.
To make a map this finely detailed, the team had to cut the tissue sample into 5,000 slices and scan them with a high-speed electron microscope. Then they used a machine-learning model to help electronically stitch the slices back together and label the features. The raw data set alone took up 1.4 petabytes. “It’s probably the most computer-intensive work in all of neuroscience,” says Michael Hawrylycz, a computational neuroscientist at the Allen Institute for Brain Science, who was not involved in the research. “There is a Herculean amount of work involved.”
Many other brain atlases exist, but most provide much lower-resolution data. At the nanoscale, researchers can trace the brain’s wiring one neuron at a time to the synapses, the places where they connect. “To really understand how the human brain works, how it processes information, how it stores memories, we will ultimately need a map that’s at that resolution,” says Viren Jain, a senior research scientist at Google and coauthor on the paper, published in Science on May 9 . The data set itself and a preprint version of this paper were released in 2021 .
Brain atlases come in many forms. Some reveal how the cells are organized. Others cover gene expression. This one focuses on connections between cells, a field called “connectomics.” The outermost layer of the brain contains roughly 16 billion neurons that link up with each other to form trillions of connections. A single neuron might receive information from hundreds or even thousands of other neurons and send information to a similar number. That makes tracing these connections an exceedingly complex task, even in just a small piece of the brain..
To create this map, the team faced a number of hurdles. The first problem was finding a sample of brain tissue. The brain deteriorates quickly after death, so cadaver tissue doesn’t work. Instead, the team used a piece of tissue removed from a woman with epilepsy during brain surgery that was meant to help control her seizures.
Once the researchers had the sample, they had to carefully preserve it in resin so that it could be cut into slices, each about a thousandth the thickness of a human hair. Then they imaged the sections using a high-speed electron microscope designed specifically for this project.
Next came the computational challenge. “You have all of these wires traversing everywhere in three dimensions, making all kinds of different connections,” Jain says. The team at Google used a machine-learning model to stitch the slices back together, align each one with the next, color-code the wiring, and find the connections. This is harder than it might seem. “If you make a single mistake, then all of the connections attached to that wire are now incorrect,” Jain says.
“The ability to get this deep a reconstruction of any human brain sample is an important advance,” says Seth Ament, a neuroscientist at the University of Maryland. The map is “the closest to the ground truth that we can get right now.” But he also cautions that it’s a single brain specimen taken from a single individual.
The map, which is freely available at a web platform called Neuroglancer , is meant to be a resource other researchers can use to make their own discoveries. “Now anybody who’s interested in studying the human cortex in this level of detail can go into the data themselves. They can proofread certain structures to make sure everything is correct, and then publish their own findings,” Jain says. (The preprint has already been cited at least 136 times .)
The team has already identified some surprises. For example, some of the long tendrils that carry signals from one neuron to the next formed “whorls,” spots where they twirled around themselves. Axons typically form a single synapse to transmit information to the next cell. The team identified single axons that formed repeated connections—in some cases, 50 separate synapses. Why that might be isn’t yet clear, but the strong bonds could help facilitate very quick or strong reactions to certain stimuli, Jain says. “It’s a very simple finding about the organization of the human cortex,” he says. But “we didn’t know this before because we didn’t have maps at this resolution.”
The data set was full of surprises, says Jeff Lichtman, a neuroscientist at Harvard University who helped lead the research. “There were just so many things in it that were incompatible with what you would read in a textbook.” The researchers may not have explanations for what they’re seeing, but they have plenty of new questions: “That’s the way science moves forward.”
Biotechnology and health
How scientists traced a mysterious covid case back to six toilets.
When wastewater surveillance turns into a hunt for a single infected individual, the ethics get tricky.
An AI-driven “factory of drugs” claims to have hit a big milestone
Insilico is part of a wave of companies betting on AI as the "next amazing revolution" in biology
- Antonio Regalado archive page
The quest to legitimize longevity medicine
Longevity clinics offer a mix of services that largely cater to the wealthy. Now there’s a push to establish their work as a credible medical field.
- Jessica Hamzelou archive page
There is a new most expensive drug in the world. Price tag: $4.25 million
But will the latest gene therapy suffer the curse of the costliest drug?
Stay connected
Get the latest updates from mit technology review.
Discover special offers, top stories, upcoming events, and more.
Thank you for submitting your email!
It looks like something went wrong.
We’re having trouble saving your preferences. Try refreshing this page and updating them one more time. If you continue to get this message, reach out to us at [email protected] with a list of newsletters you’d like to receive.
OpenAI unveils huge upgrade to ChatGPT that makes it more eerily human than ever
ChatGPT's latest upgrade means the voice assistant can now respond to audio, text and visual inputs in real time. The new chatbot, named ChatGPT-4o, will be rolled out to alpha testers in the coming weeks.
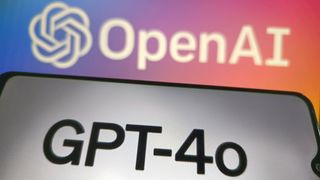
A new version of ChatGPT can read facial expressions, mimic human voice patterns and have near real-time conversations, its creators have revealed.
OpenAI demonstrated the upcoming version of the artificial intelligence (AI) chatbot, called GPT-4o, in an apparently real-time presentation on Monday (May 13). The chatbot, which spoke out loud with presenters through a phone, appeared to have an eerie command of human conversation and its subtle emotional cues — switching between robotic and singing voices upon command, adapting to interruptions and visually processing the facial expressions and surroundings of its conversational partners.
During the demonstration, the AI voice assistant showcased its skills by completing tasks such as real-time language translation, solving a math equation written on a piece of paper and guiding a blind person around London's streets.
"her," Sam Altman, OpenAI's CEO, wrote in a one-word post on the social media platform X after the presentation had ended. The post is a reference to the 2013 film of the same name, in which a lonely man falls in love with an AI assistant.
To show off its ability to read visual cues, the chatbot used the phone’s camera lens to read one OpenAI engineer’s facial expressions and describe their emotions.
Related: MIT gives AI the power to 'reason like humans' by creating hybrid architecture
"Ahh, there we go, it looks like you're feeling pretty happy and cheerful with a big smile and a touch of excitement," said the bot, which answered to the name ChatGPT. "Whatever is going on, it looks like you're in a good mood. Care to share the source of those good vibes?"
Sign up for the Live Science daily newsletter now
Get the world’s most fascinating discoveries delivered straight to your inbox.
If the demonstration is an accurate representation of the bot's abilities, the new capabilities are a massive improvement on the limited voice features in the company's previous models — which were incapable of handling interruptions or responding to visual information.
— Scientists create 'toxic AI' that is rewarded for thinking up the worst possible questions we could imagine
— Researchers gave AI an 'inner monologue' and it massively improved its performance
— AI models can talk to each other and pass on skills with limited human input, scientists say
"We're looking at the future of interaction between ourselves and the machines," Mira Murati , OpenAI's chief technology officer, said at the news conference. "We think GPT-4o is really shifting that paradigm."
The new voice assistant is set to be released in a limited form to alpha testers in the coming weeks, followed by a wider rollout that will begin with paying ChatGPT Plus subscribers. The announcement also follows a Bloomberg report that the company is nearing a deal with Apple to integrate ChatGPT on the iPhone — opening a possibility that GPT-4o could be used to upgrade Siri, the iPhone's voice assistant.
But the new technology comes with significant safety concerns. The bot's ability to process real-time text, audio and visual input means that it could be used for spying. And its convincing emotional mimicry might also make it adept at conducting scam phone calls or presenting dangerous misinformation in a convincing manner.
In response to these issues, Murati said that OpenAI was working to build "mitigations against misuse" of the new technology.
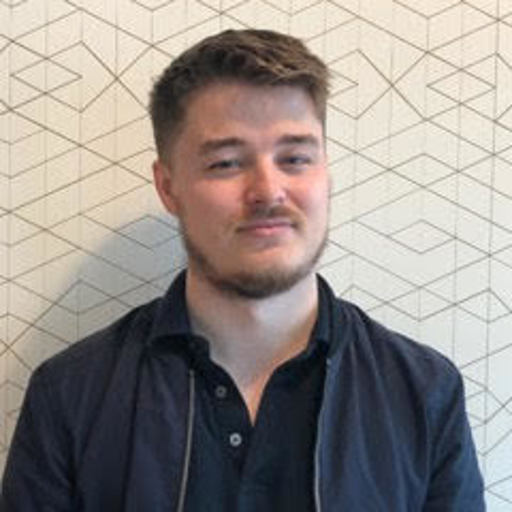
Ben Turner is a U.K. based staff writer at Live Science. He covers physics and astronomy, among other topics like tech and climate change. He graduated from University College London with a degree in particle physics before training as a journalist. When he's not writing, Ben enjoys reading literature, playing the guitar and embarrassing himself with chess.
MIT gives AI the power to 'reason like humans' by creating hybrid architecture
'It would be within its natural right to harm us to protect itself': How humans could be mistreating AI right now without even knowing it
Heavy metals in Beethoven's hair may explain his deafness, study finds
Most Popular
- 2 EV batteries could last much longer thanks to new capacitor with 19-times higher energy density that scientists created by mistake
- 3 Can mirrors facing each other create infinite reflections?
- 4 'The most critically harmful fungi to humans': How the rise of C. auris was inevitable
- 5 2,500-year-old Illyrian helmet found in burial mound likely caused 'awe in the enemy'
- 2 Papua New Guineans, genetically isolated for 50,000 years, carry Denisovan genes that help their immune system, study suggests
- 3 Massive study of 8,000 cats reveals which breeds live longest
- 4 Why can't we see the far side of the moon?
- 5 'The most critically harmful fungi to humans': How the rise of C. auris was inevitable
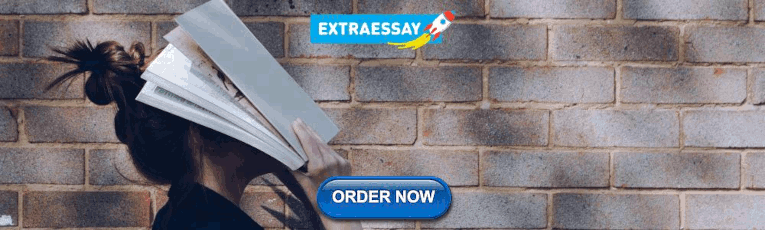
IMAGES
VIDEO
COMMENTS
Don't Underestimate the Power of Your Voice. Summary. Our voices matter as much as our words matter. They have the power to awaken the senses and lead others to act, close deals, or land us ...
Many people use their voices everyday—to talk to people, to communicate their needs and wants—but the idea of 'voice' goes much deeper. Having a voice gives an individual agency and power, and a way to express his or her beliefs. But what happens when that voice is in some way silenced? Meryl Alper, assistant professor of communication studies, found out.
Human Rights. The Power of Using your voice . February 17, 2021. By Wafiya. Like 855. Comments. Like 855. Comments. Post Submit a post. Image. A voice is a tool that transports us into the future. A future that has more possibilities and more solutions. A voice is a tool that can be used for standing up for what is right, rather than what is easy.
The human voice is an amazing tool that can have a profound effect on video games. Using a narrator affects the gameplay and the experience the player remembers after walking away from the game. Think of being held in awe, listening to the radio where the mellifluous voices of one`s favourite program's hosts awaken, mesmerise, excite or sooth ...
Amazing Outcomes of Human Voice. The human voice can be modified in many ways. Consider the spectrum of sounds - whispering, speaking, orating, shouting - as well as the different sounds that are possible in different forms of vocal music, such as rock singing, gospel singing, and opera singing. Key Factors for Normal Vocal Fold Vibration
The following essay is reprinted with permission from The Conversation, ... Each human being has a voice that is distinct and different from everyone else's. So it seems intuitive that we'd be ...
The Human Voice Can Communicate 24 Emotions. Researchers created an interactive map of all the emotional sounds that humans make. Ooh, surprise! Those spontaneous sounds we make to express everything from elation (woohoo) to embarrassment (oops) say a lot more about what we're feeling than previously understood, according to new UC Berkeley ...
A. Vocal fold anatomy and biomechanics. The human vocal system includes the lungs and the lower airway that function to supply air pressure and airflow (a review of the mechanics of the subglottal system can be found in Hixon, 1987), the vocal folds whose vibration modulates the airflow and produces voice source, and the vocal tract that modifies the voice source and thus creates specific ...
The human voice is an extremely expressive instrument both when used for speech and for singing. By means of subtle variations of timing and pitch contour speakers and singers add a substantial amount of expressiveness to the linguistic or musical content and we are quite skilled in deciphering this information. Indeed a good deal of vocal ...
Request PDF | AI vs. Human Voices: How Delivery Source and Narrative Format Influence the Effectiveness of Persuasion Messages | AI communicators (e.g., AI voice assistants) play an increasingly ...
The Human Voice Essay. Our voice is our primary mean of communication, and most of us can't go for more than a couple of minutes without using it. We don't use your voice for just talking though, our voice can be used to do a variation of things. The most obvious example would be singing. So it is obvious the human voice is a means of ...
Writing about instrumental representations of the human voice, it is impossible not to touch on the question of authenticity. To do so, I will use "modality theory," an approach that asks not whether voices actually are "authentic," but as how "authentic" they have been represented. As it turns out, musical representations of the human voice have always been relatively abstract ...
Essays on the Human Voice, Singing, and Sprirtuality. About the author (2018) Darlene Wiley has been critically acclaimed on three continents for her work in lieder, opera and oratorio. She began her career as lyric coloratura at the Staatstheater Darmstadt performing over 50 roles in such operas as I Pagliacci, Die Zauberflöte, Don Pasquale ...
Abstract. This paper examines the resonance of Barthes' essays on the human voice in works such as 'The Grain of the Voice' in the context of Barthes' own writings on mélodie and the body, as well as in the more recent and broader sphere of media and 'sound studies', a field devoted to investigating the production, circulation, and socio-cultural significance of sounds and ...
The individual view of voice closely ties voice in writing to the spoken human voice in a sense that everyone's voice in both speech and writing is unique, distinct and identifiable. ... Voice in student essays. Stance and voice in written academic genres, Palgrave Macmillan, London (2012), pp. 151-165. CrossRef View in Scopus Google Scholar.
Just type or paste your text, generate the voice-over, and download the audio file. Create realistic Voiceovers online! Insert any text to generate speech and download audio mp3 or wav for any purpose. Speak a text with AI-powered voices.You can convert text to voice for free for reference only. For all features, purchase the paid plans.
These are some essentially human questions that Darlene C. Wiley purports to answer in her work, Singing: The Timeless Muse, Essays on the Human Voice, Singing, and Spirituality. It is a compilation of twenty reflections in the form of essays and interviews by participants from different professional backgrounds related to the singing voice.
FUNDERMENTALS OF ACOUSTICS AND NOISE CONTROL. Odense: Department of Electric Enginearing, 2011. Print. This essay, "The Relationship Between Acoustics and Human Voice" is published exclusively on IvyPanda's free essay examples database. You can use it for research and reference purposes to write your own paper.
The statistical analysis of the ratings reported in Table 4 shows that differences between the human-written essays and the ones generated by both ChatGPT models are significant. The effect sizes ...
Essays on Voice . Essay examples. Essay topics. 25 essay samples found. Sort & filter. 1 My Brothers Voice Characteristics . 1 page / 402 words . ... Introduction The human voice is remarkable instrument as voice is work as the unique identification for each individual. A speaker can evoke a wide range of emotion and mental images by slight ...
Essays on the Human Voice, Singing, and Spirituality compiled by Darlene C. Wiley. ISBN: 978-1-7335060-0-7 Soft cover, 229 pages. Please Select We are currently closed for a brief holiday. Books and e-books will resume shipping on May 15, 2024 . Proceeds from the sale of this book are contributed to New Music USA ...
The human voice has the power to create beautiful, unique music that has the ability move one to tears. These tears are often due to the emotional impact a song has on a person, but in some cases, these tears are the body's response to a terrible, uncomfortable sound- the sound of somebody singing terribly. ... The word 'resonance' has been ...
Text to speech (TTS) is a technology that converts text into spoken audio. It can read aloud PDFs, websites, and books using natural AI voices. Text-to-speech (TTS) technology can be helpful for anyone who needs to access written content in an auditory format, and it can provide a more inclusive and accessible way of communication for many ...
Boredom, anxiety, and despair are some of the descriptions these moods have received. In the novel Nausea, the French existentialist philosopher Jean-Paul Sartre describes someone who mysteriously ...
Summary: The vast majority of people have an ongoing conversation with themselves, an inner voice, that plays an important role in their daily lives. But between 5-10 per cent of the population do ...
But unlike its existing voice mode, Murati said, GPT-4o's voice feature reacts in real time, getting rid of the two- or three-second lag to emulate human response times.
On Monday, the San Francisco artificial intelligence start-up unveiled a new version of its ChatGPT chatbot that can receive and respond to voice commands, images and videos. The company said the ...
Prior to GPT-4o, you could use Voice Mode to talk to ChatGPT with latencies of 2.8 seconds (GPT-3.5) and 5.4 seconds (GPT-4) on average. To achieve this, Voice Mode is a pipeline of three separate models: one simple model transcribes audio to text, GPT-3.5 or GPT-4 takes in text and outputs text, and a third simple model converts that text back to audio.
A massive suite of papers offers a high-res view of the human and non-human primate brain. Many other brain atlases exist, but most provide much lower-resolution data.
By Ben Turner. published 14 May 2024. ChatGPT's latest upgrade means the voice assistant can now respond to audio, text and visual inputs in real time. The new chatbot, named ChatGPT-4o, will be ...