Complexity in manufacturing systems: a literature review
- Production Management
- Published: 17 January 2021
- Volume 15 , pages 321–333, ( 2021 )
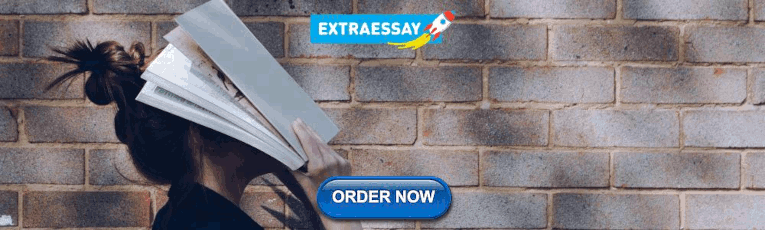
Cite this article
- Germán Herrera Vidal ORCID: orcid.org/0000-0002-0152-6712 1 , 2 &
- Jairo Rafael Coronado Hernández 3
1575 Accesses
11 Citations
Explore all metrics
The business environment is increasingly complex and competitive so organizations must respond to different market conditions by reconfiguring their processes, products and services. The objective of this article is based on a literature review under a scientometric and bibliometric approach, referring to the complexity in manufacturing systems, allowing to find answers to relevant questions with respect to the subject matter and to show literally the scientific fields of application, factors of complexity and methods of solution. Methodologically, a first stage is developed on the problems and formulation of the research questions, the second stage establishes the information search strategy and finally a statistical analysis is carried out. The findings show a positive trend and interest on the part of the scientific community in the number of publications related to the subject in the different databases, highlighting the exercise of the theory of complexity in different disciplines but on a smaller scale in scientific fields such as manufacturing and supply chain management.
This is a preview of subscription content, log in via an institution to check access.
Access this article
Price includes VAT (Russian Federation)
Instant access to the full article PDF.
Rent this article via DeepDyve
Institutional subscriptions
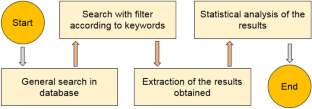
Similar content being viewed by others
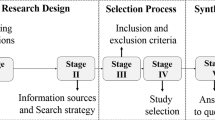
Measuring manufacturing system complexity: a literature review
Complexity drivers in manufacturing companies: a literature review.
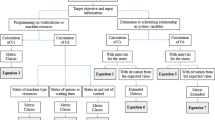
A new perspective on measuring entropic complexity in manufacturing systems
Manuj I, Sahin F (2011). A model of supply chain and supply chain decision-making complexity. Int J Phys Distribut Logist Manag
Perona M, Miragliotta G (2004) Complexity management and supply chain performance assessment. A field study and a conceptual framework. Int J Prod Econ 90(1):103–115
Article Google Scholar
Wu Y, Frizelle G, Ayral L, Marsein J, Van de Merwe E, Zhou D (2002) A simulation study on supply chain complexity in manufacturing industry. In: Proceedings of the conference of the manufacturing complexity network. University of Cambridge
Jacobs MA (2007) Product complexity: a definition and impacts on operations. Decision Line 38(5)
Efthymiou K, Mourtzis D, Pagoropoulos A, Papakostas N, Chryssolouris G (2016) Manufacturing systems complexity analysis methods review. Int J Comput Integr Manuf 29(9):1025–1044
Coronado Hernández JR (2016) Análisis del efecto de algunos factores de complejidad e incertidumbre en el rendimiento de las Cadenas de Suministro. Propuesta de una herramienta de valoración basada en simulación (Doctoral dissertation)
Garbie IH, Shikdar A (2011) Analysis and estimation of complexity level in industrial firms. Int J Ind Syst Eng 8(2):175–197
Google Scholar
Chedid JA, Vidal GH (2012) Análisis del Problema de Planificación de la Producción en Cadenas de Suministro Colaborativas: Una Revisión de la Literatura en el Enfoque de Teoría de Juegos
Bozarth CC, Warsing DP, Flynn BB, Flynn EJ (2009) The impact of supply chain complexity on manufacturing plant performance. J Oper Manage 27(1):78–93
MacDuffie JP, Sethuraman K, Fisher ML (1996) Product variety and manufacturing performance: evidence from the international automotive assembly plant study. Manage Sci 42(3):350–369
Article MATH Google Scholar
Wu Y, Frizelle G, Efstathiou J (2007) A study on the cost of operational complexity in customer–supplier systems. Int J Prod Econ 106(1):217–229
Sivadasan S, Efstathiou J, Calinescu A, Huatuco LH (2006) Advances on measuring the operational complexity of supplier–customer systems. Eur J Oper Res 171(1):208–226
Article MathSciNet MATH Google Scholar
Quirk M (1999) Manufacturing, teams, and improvement: the human art of manufacturing. Prentice-Hall, Upper Saddle River
Tanner JP (1990) Manufacturing engineering: an introduction to the basic functions, revised and expanded, vol 36. CRC Press, Boca Raton
Wilson B (1984) Systems: concepts, methodologies, and applications. Wiley, New York
Chryssolouris G (2006) Overview of manufacturing processes. Manufact Syst Theory Pract 55-124
Martini U (2011) Loris Gaio, Francesca Gino, Enrico Zaninotto, “I sistemi di produzione. Manuale per la gestione operativa dell’impresa”. Sinergie Italian J Manage 59:351–352
Deshmukh AV, Talavage JJ, Barash MM (1998) Complexity in manufacturing systems, Part 1: analysis of static complexity. IIE Trans 30(7):645–655
Companys R (2003) Teoría de la decisión. Cpda-ETSEIB. Intangible Capital. Barcelona, España, 3, 369
Boisot M, Child J (1999) Organizations as adaptive systems in complex environments: The case of China. Organ Sci 10(3):237–252
Isik F (2010) An entropy-based approach for measuring complexity in supply chains. Int J Prod Res 48(12):3681–3696
Abad RC (2002) Introducción a la simulación ya la teoría de colas. Netbiblo
Frizelle G, Woodcock E (1995) Measuring complexity as an aid to developing operational strategy. Int J Oper Prod Manage
Suh NP (2005) A theory of complexity and applications. Massachusetts Institute of Technology Cambridge
Papakostas N, Efthymiou K, Mourtzis D, Chryssolouris G (2009) Modelling the complexity of manufacturing systems using nonlinear dynamics approaches. CIRP Ann 58(1):437–440
Calinescu A, Efstathiou J, Bermejo J, Schirn J (1997) Modelling and simulation of a real complex process-based manufacturing system. In: Proceedings of the Thirty-Second International Matador Conference, pp 137–142. Palgrave, London
Calinescu A, Efstathiou J, Bermejo J, Schirn J (1997) Assessing decision-making and process complexity in a manufacturer through simulation. IFAC Proc Volumes 30(24):149–152
Hu SJ, Zhu X, Wang H, Koren Y (2008) Product variety and manufacturing complexity in assembly systems and supply chains. CIRP Ann 57(1):45–48
Datar SM, Kekre S, Mukhopadhyay T, Srinivasan K (1993) Simultaneous estimation of cost drivers. Account Review, 602–614
Han P, Ma LL, Wu WJ, Liu C (2011) Research on influence factors and levels of automobile manufacturing system complexity. In: 2011 IEEE 18th international conference on industrial engineering and engineering management, pp 186–190. IEEE
Bick W, Drexl-Wittbecker S (2008) Komplexität reduzieren: Konzept. Methoden. Praxis. LOG_X, Stuttgart
Serdarasan S, Tanyas M (2012) Dealing with complexity in the supply chain: The effect of supply chain management initiatives. Available at SSRN 2056331
Serdarasan S (2013) A review of supply chain complexity drivers. Comput Ind Eng 66(3):533–540
Rose-Anderssen C, Baldwin J, Ridgway K (2017) Manufacturing systematics and cladistics: state of the art and generic classification. J Manufact Technol Manage
Arredondo Soto KC, Carrillo Gutiérrez T, Salazar Ruiz E, Solís Quinteros M (2014) Factores relevantes de la complejidad en el proceso de remanufactura: caso de la industria metalmecánica. Epistemus. Ciencia, tecnología y salud 16:88–94
Sinha K, de Weck OL (2016) Empirical validation of structural complexity metric and complexity management for engineering systems. Syst Eng 19(3):193–206
Tamaskar S, Neema K, DeLaurentis D (2014) Framework for measuring complexity of aerospace systems. Res Eng Design 25(2):125–137
Schuh G (2014) Produktkomplexität managen: Strategien-Methoden-Tools. Carl Hanser Verlag GmbH Co KG
Chryssolouris G, Efthymiou K, Papakostas N, Mourtzis D, Pagoropoulos A (2013) Flexibility and complexity: is it a trade-off? Int J Prod Res 51(23–24):6788–6802
Byrne D, Callaghan G (2013) Complexity theory and the social sciences: the state of the art. Routledge, New York
Book Google Scholar
Schuh G (2013) Lean innovation. Springer, Berlin
Singh G, Balaji S, Shah JJ, Corman D, Howard R, Mattikalli R, Stuart D (2012). Evaluation of network measures as complexity metrics. In: International design engineering technical conferences and computers and information in engineering conference, vol 45011, pp 1065–1076. American Society of Mechanical Engineers
Stacey RD, Griffin D, Shaw P (2000) Complexity and management: fad or radical challenge to systems thinking?. Psychology Press, New York
Gare A (2000) Systems theory and complexity: introduction
Simon HA (1991) The architecture of complexity. In: Facets of systems science. Springer, Boston, pp 457–476
Ulrich H, Probst GJ (1988) Anleitung zum ganzheitlichen Denken. Ein Brevier für Führungskräfte. Haupt, Bern
Klir GJ (1985) Complexity: some general observations. Syst Res 2(2):131–140
Yates FE (1978) Complexity and the limits to knowledge. Am J Physiol-Regul Integrat Comparat Physiol 235(5):R201–R204
de Rosnay J (1977) Il macroscopio: verso una visione globale, vol 60. Edızıonı Dedalo
Ashby W (1963) Ross: an introduction to cybernetics. New YorN
Remus W (1987) A study of graphical and tabular displays and their interaction with environmental complexity. Manage Sci 33(9):1200–1204
Rivkin JW (2000) Imitation of complex strategies. Manage Sci 46(6):824–844
Pich MT, Loch CH, Meyer AD (2002) On uncertainty, ambiguity, and complexity in project management. Manage Sci 48(8):1008–1023
Mihm J, Loch C, Huchzermeier A (2003) Problem–solving oscillations in complex engineering projects. Manage Sci 49(6):733–750
Sommer SC, Loch CH (2004) Selectionism and learning in projects with complexity and unforeseeable uncertainty. Manage Sci 50(10):1334–1347
Linn SC, Tay NS (2007) Complexity and the character of stock returns: empirical evidence and a model of asset prices based on complex investor learning. Manage Sci 53(7):1165–1180
Rahmandad H (2008) Effect of delays on complexity of organizational learning. Manage Sci 54(7):1297–1312
Mihm J, Loch CH, Wilkinson D, Huberman BA (2010) Hierarchical structure and search in complex organizations. Manage Sci 56(5):831–848
Kalkanci B, Chen KY, Erhun F (2011) Contract complexity and performance under asymmetric demand information: an experimental evaluation. Manage Sci 57(4):689–704
Briscoe F, Rogan M (2016) Coordinating complex work: knowledge networks, partner departures, and client relationship performance in a law firm. Manage Sci 62(8):2392–2411
Dost HU, Terno J, Scheithauer G (1989) On the stochastic complexity of the asymmetric traveling salesman problem. Eur J Oper Res 43(3):313–316
Chen CL, Bulfin RL (1993) Complexity of single machine, multi-criteria scheduling problems. Eur J Oper Res 70(1):115–125
Cooper WW, Sinha KK, Sullivan RS (1995) Accounting for complexity in costing high technology manufacturing. Eur J Oper Res 85(2):316–326
Rayward-Smith VJ (1995) Introduction to the Theory of Complexity. J Oper Res Soc 46(12):1507–1508
De Reyck B, Herroelen W (1996) On the use of the complexity index as a measure of complexity in activity networks. Eur J Oper Res 91(2):347–366
Soukhal A, Oulamara A, Martineau P (2005) Complexity of flow shop scheduling problems with transportation constraints. Eur J Oper Res 161(1):32–41
Briskorn D, Choi BC, Lee K, Leung J, Pinedo M (2010) Complexity of single machine scheduling subject to nonnegative inventory constraints. Eur J Oper Res 207(2):605–619
Gawiejnowicz S, Kononov A (2010) Complexity and approximability of scheduling resumable proportionally deteriorating jobs. Eur J Oper Res 200(1):305–308
Brucker P, Qu R, Burke E (2011) Personnel scheduling: models and complexity. Eur J Oper Res 210(3):467–473
Espinosa A, Walker J (2013) Complexity management in practice: a Viable System Model intervention in an Irish eco-community. Eur J Oper Res 225(1):118–129
Pinker E, Szmerekovsky J, Tilson V (2014) On the complexity of project scheduling to minimize exposed time. Eur J Oper Res 237(2):448–453
Archetti C, Feillet D, Speranza MG (2015) Complexity of routing problems with release dates. Eur J Oper Res 247(3):797–803
Bruns F, Knust S, Shakhlevich NV (2016) Complexity results for storage loading problems with stacking constraints. Eur J Oper Res 249(3):1074–1081
Kuschel T, Bock S (2016) The weighted uncapacitated planned maintenance problem: complexity and polyhedral properties. Eur J Oper Res 250(3):773–781
Calinescu A, Efstathiou J, Schirn J, Bermejo J (1998) Applying and assessing two methods for measuring complexity in manufacturing. J Oper Res Soc 49(7):723–733
Efstathiou J (2002) The utility of complexity [manufacturing system evolution]. Manufact Eng 81(2):73–76
Huaccho Huatuco L, Efstathiou J, Calinescu A, Sivadasan S, Kariuki S (2009) Comparing the impact of different rescheduling strategies on the entropic-related complexity of manufacturing systems. Int J Prod Res 47(15):4305–4325
Efthymiou K, Pagoropoulos A, Papakostas N, Mourtzis D, Chryssolouris G (2012) Manufacturing systems complexity review: challenges and outlook. Procedia CIRP 3:644–649
ElMaraghy W, ElMaraghy H, Tomiyama T, Monostori L (2012) Complexity in engineering design and manufacturing. CIRP Ann 61(2):793–814
Gresov C, Haveman HA, Oliva TA (1993) Organizational design, inertia and the dynamics of competitive response. Organ Sci 4(2):181–208
Thietart RA, Forgues B (1995) Chaos theory and organization. Organ Sci 6(1):19–31
Anderson P (1999) Perspective: complexity theory and organization science. Organ Sci 10(3):216–232
Lewin AY, Volberda HW (1999) Prolegomena on coevolution: a framework for research on strategy and new organizational forms. Organ Sci 10(5):519–534
Cohen M (1999) Commentary on the Organization Science special issue on complexity. Organ Sci 10(3):373–376
Anderson P, Meyer A, Eisenhardt K, Carley K, Pettigrew A (1999) Introduction to the special issue: Applications of complexity theory to organization science. Organ Sci 10(3):233–236
Morel B, Ramanujam R (1999) Through the looking glass of complexity: the dynamics of organizations as adaptive and evolving systems. Organ Sci 10(3):278–293
McKelvey B (1999) Avoiding complexity catastrophe in coevolutionary pockets: strategies for rugged landscapes. Organ Sci 10(3):294–321
Dooley KJ, Van de Ven AH (1999) Explaining complex organizational dynamics. Organ Sci 10(3):358–372
Siggelkow N, Rivkin JW (2005) Speed and search: designing organizations for turbulence and complexity. Organ Sci 16(2):101–122
Ethiraj SK, Levinthal D (2009) Hoping for A to Z while rewarding only A: complex organizations and multiple goals. Organ Sci 20(1):4–21
Wilding R. (1998). The supply chain complexity triangle: uncertainty generation in the supply chain. Int J Phys Distribut Logist Manage
Novak S, Eppinger SD (2001) Sourcing by design: Product complexity and the supply chain. Manage Sci 47(1):189–204
Milgate M (2001) Supply chain complexity and delivery performance: an international exploratory study. Supply Chain Manage Int J
Vachon S, Klassen RD (2002) An exploratory investigation of the effects of supply chain complexity on delivery performance. IEEE Trans Eng Manage 49(3):218–230
Yang B, Yang Y (2010) Postponement in supply chain risk management: a complexity perspective. Int J Prod Res 48(7):1901–1912
Gimenez C, Van Der Vaart T, Van Donk DP (2012) Supply chain integration and performance: the moderating effect of supply complexity. Int J Oper Prod Manage
Blome C, Schoenherr T, Eckstein D (2014) The impact of knowledge transfer and complexity on supply chain flexibility: a knowledge-based view. Int J Prod Econ 147:307–316
Wildemann H (2000) Komplexitätsmanagement: Vertrieb, Produkte, Beschaffung, F & E, Produktion und Administration.
Meijer BR (2002) Reducing complexity through organizational structuring in manufacturing and engineering. In: Proceedings of Manufacturing Complexity Network Conference, pp 9–10
Seuring S, Goldbach M, Koplin J (2004) Managing time and complexity in supply chains: two cases from the textile industry. Int J Integrated Supply Manage 1(2):180–198
Hoole R (2005) Five ways to simplify your supply chain. Supply Chain Manage Int J
Gabriel A (2007) The effect of internal static manufacturing complexity on manufacturing performance
Haumann M, Westermann HH, Seifert S, Butzer S (2012) Managing comlexity—a methodology, exemplified by the industrial sector of remanufacturing
Park K, Kremer GEO (2015) Assessment of static complexity in design and manufacturing of a product family and its impact on manufacturing performance. Int J Prod Econ 169:215–232
Arredondo K, Ruíz E, Martínez R, Leal J (2017) Análisis de la variabilidad del proceso de remanufactura asociado a la toma de decisiones. Ecodiseño & Sostenibilidad 1:107–126
Modrak V, Soltysova Z (2017) Novel complexity indicator of manufacturing process chains and its relations to indirect complexity indicators. Complexity, 2017
Karp A, Ronen B (1992) Improving shop floor control: an entropy model approach. Int J Prod Res 30(4):923–938
Sivadasan S, Efstathiou J, Frizelle G, Shirazi R, Calinescu A (2002) An information-theoretic methodology for measuring the operational complexity of supplier-customer systems. Int J Oper Prod Manage
Makui A, Aryanezhad MB (2003) A new method for measuring the static complexity in manufacturing. J Oper Res Soc 54(5):555–557
Childerhouse P, Aitken J, Towill DR (2002) Analysis and design of focused demand chains. J Oper Manage 20(6):675–689
Childerhouse P, Towill DR (2003) Simplified material flow holds the key to supply chain integration. Omega 31(1):17–27
Childerhouse P, Towill DR (2004) Reducing uncertainty in European supply chains. J Manufact Technol Manage
Blackhurst J, Wu T, O’grady P (2004) Network-based approach to modelling uncertainty in a supply chain. Int J Prod Res 42(8):1639–1658
Größler A, Grübner A, Milling PM (2006) Organisational adaptation processes to external complexity. Int J Oper Prod Manage
Sivadasan S, Smart J, Huatuco LH, Calinescu A (2010) Operational complexity and supplier–customer integration: case study insights and complexity rebound. J Oper Res Soc 61(12):1709–1718
Gray JV, Roth AV, Leiblein MJ (2011) Quality risk in offshore manufacturing: evidence from the pharmaceutical industry. J Oper Manage 29(7–8):737–752
Isik F (2011) Complexity in supply chains: a new approach to quantitative measurement of the supply-chain-complexity. Supply Chain Manage, 417–432
Vrabic R, Butala P (2012) Assessing operational complexity of manufacturing systems based on statistical complexity. Int J Prod Res 50(14):3673–3685
Efthymiou K, Pagoropoulos A, Papakostas N, Mourtzis D, Chryssolouris G (2014) Manufacturing systems complexity: an assessment of manufacturing performance indicators unpredictability. CIRP J Manufact Sci Technol 7(4):324–334
Hwarng HB, Yuan X (2014) Interpreting supply chain dynamics: a quasi-chaos perspective. Eur J Oper Res 233(3):566–579
Fischi J, Nilchiani R, Wade J (2015) Dynamic complexity measures for use in complexity-based system design. IEEE Syst J 11(4):2018–2027
Huang S, Wang G, Shang X, Yan Y (2018) Reconfiguration point decision method based on dynamic complexity for reconfigurable manufacturing system (RMS). J Intell Manuf 29(5):1031–1043
Towill DR (1999) Simplicity wins: twelve rules for designing effective supply chains. Control, March, 9-13
Calinescu A (2000) Complexity in manufacturing: an information theoretic approach. In: Conference on complexity and complex systems in industry, 19-20 Sept 2000 (pp. 19-20). University of Warwick
Efstathiou J, Calinescu A, Blackburn G (2002) A web-based expert system to assess the complexity of manufacturing organizations. Robot Comput-Integrated Manufact 18(3–4):305–311
Sivadasan S, Efstathiou J, Calinescu A, Huatuco LH (2004) Supply chain complexity In: New, S, Westbrook R (eds), Understanding supply chains
Blecker T, Kersten W, Meyer CM (2005) Development of an approach for analyzing supply chain complexity. In: Mass customization: concepts–tools–realization. proceedings of the international mass customization meeting, pp 47–59
Donner R, Scholz-Reiter B, Hinrichs U (2008) Nonlinear characterization of the performance of production and logistics networks. J Manufact Syst 27(2):84–99
Windt K, Philipp T, Böse F (2008) Complexity cube for the characterization of complex production systems. Int J Comput Integr Manuf 21(2):195–200
Gullander P, Davidsson A, Dencker K, Fasth Å, Fässberg T, Harlin U, Stahre J (2011) Towards a production complexity model that supports operation, re-balancing and man-hour planning. In: Proceedings of the 4th Swedish Production Symposium (SPS): Lund, Sweden
Zhang Z (2011) Modeling complexity of cellular manufacturing systems. Appl Math Model 35(9):4189–4195
Smart J, Calinescu A, Huatuco LH (2013) Extending the information-theoretic measures of the dynamic complexity of manufacturing systems. Int J Prod Res 51(2):362–379
Surana A, Kumara S, Greaves M, Raghavan UN (2005) Supply-chain networks: a complex adaptive systems perspective. Int J Prod Res 43(20):4235–4265
Download references
Acknowledgements
Thank you to the Fundación Universitaria Tecnológico Comfenalco (FUTC), Investigation Group Ciptec, Universidad de la Costa (CUC)—Colombia and to the Universidad Nacional Lomas de Zamora (UNLZ)—Argentina, for the support of their academic and scientific group.
Author information
Authors and affiliations.
Industrial Engineering Department, Fundacion Universitaria Tecnológico Comfenalco, Grupo de Investigación Ciptec, Cartagena, Colombia
Germán Herrera Vidal
Universidad Lomas de Zamora, Lomas De Zamora, Argentina
Industrial Engineering Department, Universidad de la Costa, Barranquilla, Colombia
Jairo Rafael Coronado Hernández
You can also search for this author in PubMed Google Scholar
Corresponding author
Correspondence to Germán Herrera Vidal .
Additional information
Publisher's note.
Springer Nature remains neutral with regard to jurisdictional claims in published maps and institutional affiliations.
Rights and permissions
Reprints and permissions
About this article
Herrera Vidal, G., Coronado Hernández, J.R. Complexity in manufacturing systems: a literature review. Prod. Eng. Res. Devel. 15 , 321–333 (2021). https://doi.org/10.1007/s11740-020-01013-3
Download citation
Received : 24 July 2020
Accepted : 10 December 2020
Published : 17 January 2021
Issue Date : June 2021
DOI : https://doi.org/10.1007/s11740-020-01013-3
Share this article
Anyone you share the following link with will be able to read this content:
Sorry, a shareable link is not currently available for this article.
Provided by the Springer Nature SharedIt content-sharing initiative
- Manufacturing systems
- Decision making
- Find a journal
- Publish with us
- Track your research
To read this content please select one of the options below:
Please note you do not have access to teaching notes, manufacturing strategy: literature review and some issues.
International Journal of Operations & Production Management
ISSN : 0144-3577
Article publication date: 1 July 2001
Manufacturing strategy has attracted serious research attention in the recent past. In this paper, an attempt is made to review the status of literature in manufacturing strategy. A literature classification scheme is suggested. A total of 260 articles from 31 referred journals and international conferences are classified into content (manufacturing capabilities, strategic choices, best practices, trans‐national comparison, literature survey, and performance measurement) and process‐related issues. We have categorized the methodology used in the literature into conceptual, descriptive, empirical, exploratory cross‐sectional and exploratory longitudinal approaches. Based on this, some possible research issues are also identified, viz., resource‐based operations strategy, sector specific manufacturing strategy, relevance of manufacturing strategy to small and medium enterprises, manufacturing strategy in the context of green manufacturing, effect of organizational culture on formulation of manufacturing strategy, and performance measurement.
- Manufacturing strategy
- Performance measurement
- Green issues
- Manufacturing
- Resources management
- Operations strategy
Dangayach, G.S. and Deshmukh, S.G. (2001), "Manufacturing strategy: Literature review and some issues", International Journal of Operations & Production Management , Vol. 21 No. 7, pp. 884-932. https://doi.org/10.1108/01443570110393414
Copyright © 2001, MCB UP Limited
Related articles
We’re listening — tell us what you think, something didn’t work….
Report bugs here
All feedback is valuable
Please share your general feedback
Join us on our journey
Platform update page.
Visit emeraldpublishing.com/platformupdate to discover the latest news and updates
Questions & More Information
Answers to the most commonly asked questions here
Accessibility Links
- Skip to content
- Skip to search IOPscience
- Skip to Journals list
- Accessibility help
- Accessibility Help
Click here to close this panel.
Multi-sensor measurement and data fusion technology for manufacturing process monitoring: a literature review
Lingbao Kong 1 , Xing Peng 1 , Yao Chen 1 , Ping Wang 1 and Min Xu 1
Published 30 March 2020 • © 2020 The Author(s). Published by IOP Publishing Ltd on behalf of the IMMT International Journal of Extreme Manufacturing , Volume 2 , Number 2 Citation Lingbao Kong et al 2020 Int. J. Extrem. Manuf. 2 022001 DOI 10.1088/2631-7990/ab7ae6
Article metrics
21352 Total downloads
Share this article
Author e-mails.
Author affiliations
1 Shanghai Engineering Research Center of Ultra-precision Optical Manufacturing, Department of Optical Science and Engineering, Fudan University, Shanghai 200433, People's Republic of China
Lingbao Kong https://orcid.org/0000-0003-4522-2961
- Received 26 December 2019
- Revised 7 February 2020
- Accepted 27 February 2020
- Published 30 March 2020
Buy this article in print
Due to the rapid development of precision manufacturing technology, much research has been conducted in the field of multisensor measurement and data fusion technology with a goal of enhancing monitoring capabilities in terms of measurement accuracy and information richness, thereby improving the efficiency and precision of manufacturing. In a multisensor system, each sensor independently measures certain parameters. Then, the system uses a relevant signal-processing algorithm to combine all of the independent measurements into a comprehensive set of measurement results. The purpose of this paper is to describe multisensor measurement and data fusion technology and its applications in precision monitoring systems. The architecture of multisensor measurement systems is reviewed, and some implementations in manufacturing systems are presented. In addition to the multisensor measurement system, related data fusion methods and algorithms are summarized. Further perspectives on multisensor monitoring and data fusion technology are included at the end of this paper.
Export citation and abstract BibTeX RIS
Original content from this work may be used under the terms of the Creative Commons Attribution 3.0 licence . Any further distribution of this work must maintain attribution to the author(s) and the title of the work, journal citation and DOI.
1. Introduction
Automated workplaces are widely regarded as important factories of the future because of their flexibility and efficiency. The demand for machined parts with tighter surface finishes and higher machining precision has led to the need for better, more precise control of automated machining processes [ 1 ], mainly due to intensified global competition, shortened product life cycles, and diversified product demand [ 2 – 4 ]. To meet the growing demand, manufacturers are increasingly turning to automated processing systems, which can reduce dependence on operators during production. The realization of automated processing systems depends mainly on reliable and robust monitoring systems, which are used in the online and offline monitoring of critical machining processes.
The sensors are widely used physical devices that respond to a physical stimulus by giving an electrical output. As shown in figure 1 , modern instrumentation systems are equipped with many different sensors, each with its own separate function. Each sensor in the monitoring system can measure a certain parameter independently and use a special signal-processing algorithm to combine all of the independent measurements into a complete measurement value. This kind of system is referred to as a multisensor system [ 6 – 9 ]. For instance, by implementing a multisensor system for monitoring and controlling metal cutting operations and machine tools, a significant reduction in machining errors and better surface finishes can be achieved. This method is increasingly used for lower cost, powerful microprocessors. Meanwhile, sensing instruments make the use of signal-processing systems and digital closed-loop control quite economical [ 10 – 12 ]. Therefore, a multisensor system is an important component for achieving higher machining accuracy.

Figure 1. Framework for intelligent multisensor system. Reproduced with permission from [ 5 ].
Download figure:
In a multisensor system, the key to achieving the system's function is the coordination of all sensors. Figure 1 illustrates the general framework of an intelligent system with multisensor capabilities. In a multisensor system, the sensors provide measurement data to the data fusion layer, which uses advanced signal processing algorithms to combine the information into a whole. Meanwhile, the control application provides sufficient control signals to the actuator [ 5 ]. The process of combining the measurement data of all sensors and utilizing special algorithms to provide a complete overview of the measurement is called multisensor data fusion [ 13 – 20 ]. At present, data fusion technology has become one of the research hot topics worldwide. Data fusion refers to taking full advantage of multiple information sources and combining complementary or redundant information in space or time. Using specific standards, data fusion can obtain consistent interpretation of a tested object, so that the information system performs better compared to a system composed of subsets. Data fusion uses mathematical methods and technical tools to synthesize different sources of information, obtaining high-quality, useful measurement data. Compared with the independent processing of a single source, the advantages of data fusion include improving detectability and reliability, expanding the range of spatial-temporal perception, reducing the degree of ambiguity of inference, improving detection accuracy, increasing the dimension of target features, improving the resolution of spatial questions, and enhancing the fault-tolerant ability of the system [ 21 – 34 ].
This paper describes multisensor measuring and data fusion technology by introducing the architecture of different multisensor process monitoring systems and different, widely applied data fusion algorithms. Some applications of multisensor systems are also described. Section 2 presents a detailed review of multisensor monitoring technology. Section 3 summarizes different sensor fusion algorithms. Section 4 discusses future perspectives of multisensor process monitoring systems and data fusion technology. Finally, section 5 presents conclusions.
2. Multisensor monitoring technology
2.1. additive manufacturing (am) monitoring system.
Compared with the subtractive manufacturing process, AM is a very competitive, low-cost, high manufacturing freedom technology [ 35 – 37 ]. AM technology has many advantages, including the ability to reduce production time, produce complex customizable components, repair various mechanical parts, and fabricate free-form components [ 38 , 39 ]. Despite the benefits described above, AM technology is still considered an exception for two reasons: quality and repeatability. Compared to traditional manufacturing systems, AM's defect rate is still high. To address this issue, many recent thematic studies, projects, and roadmaps have prioritized the development of process monitoring methods [ 40 – 45 ]. Under ASTM standard F2792-12a, AM processes can be divided into seven categories: binder jetting, material extrusion, vat photopolymerization, material jetting, sheet lamination, directed energy deposition (DED), and powder bed fusion (PBF) [ 46 ].
As shown in figure 2 , the PBF system uses a powder deposition method, where a powder layer is spread on the substrate and the powder reservoir. After the powder is evenly distributed, the PBF system uses the heat from a laser or electron beam to track the geometry of the various layers of the slice according to a three-dimensional (3D) model. Once the processing of one layer is finished, the height of the building platform is lowered; and the manufacturing process is executed continuously.
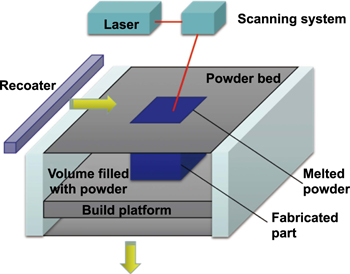
Figure 2. Schematic of PBF system (building chamber). Reproduced from [ 43 ]. © IOP Publishing Ltd. All rights reserved.
In AM process monitoring systems, the mainstream sensors and in situ data measuring equipment can be divided into the following main categories: noncontact temperature measurements, visible imaging, and low-coherence interferometric imaging. In addition, the sensor installation methods can be grouped into 'off-axial' and 'coaxial.' In off-axial configurations, the sensors are installed outside the optical path with a deflection angle relative to the monitored region. However, in coaxial configurations, the sensors can conveniently monitor the optical path of the power source. Figure 3 shows the experimental setup of the AM process monitoring system. The optical monitoring setup consists of a near-infrared complementary metal-oxide-semiconductor (NIR CMOS) camera and photodiode [ 47 – 50 ]. In the process of manufacturing, the laser beam is reflected toward the scanner by a partially semipermeable mirror. Meanwhile, Sensors 1 and 2, which are sensitive to a wavelength between 400 and 1000 nm, capture the radiation emitted by the melting pool with the beam splitter. Then the radiation intensity of the melting pool is integrated into one measurement value. The NIR CMOS camera has a 1280 × 1024 pixel sensor whose rate is 10 kHz, and it is used to determine the shape and temperature distribution of the melting pool during the manufacturing process. However, in order to achieve the 10 kHz sampling rate, the field of view has to be reduced to a limited region.
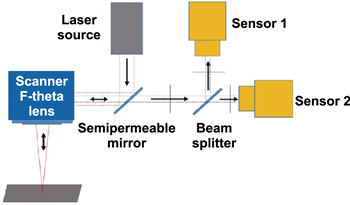
Figure 3. Schematic of the multisensor monitoring system. Reprinted from [ 44 ], Copyright (2010), with permission from Elsevier.
The DED process is a metal-based AM process that creates 3D components by melting materials during deposition. The DED processes can be divided into two categories based on the material delivery mechanism: wire feeding and powder feeding. As shown in figure 4 , unlike the PBF system shown in figure 2 , the DED system does not melt the material previously placed on the powder bed but is used to melt the powder material deposited. In the process of manufacturing, the DED AM system uses a high-powered laser to melt the powder delivered by the cladding nozzle with the inert shield gas [ 51 – 54 ].
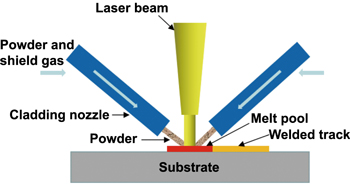
Figure 4. Schematic of the DED process with powder feeding mechanism. Reprinted from [ 51 ] Copyright 2017. With permission of Springer.
The DED process is dedicated to the manufacturing of functional metal parts. The energy input of the DED AM system can be provided by electric arc and high-powered laser, and its deposition material can be in the form of powder and wire. As the DED process is operated in an open-loop environment, it has some drawbacks due to production losses and lack of reproducibility. Therefore, in order to improve the performance of the DED system, the process optical monitoring system is investigated and utilized to control the manufacturing in a timely manner, ensuring high-quality final components. At present, there is some research being conducted that is devoted to designing a single closed-loop control system for the DED process, which can control the material delivery, laser energy, thermal distribution, and so on. As some monitoring systems are limited to controlling only one criterion, multisensor monitoring system methods are proposed; however, the multisensor monitoring systems designed still focus on one phenomenon, mainly the geometric phenomenon in the DED process. In [ 54 ], a new multisensor methodology is proposed for monitoring at least two DED processes. As presented in figures 5 and 6 , the investigation mainly focused on the closed loop of thermal and geometrical control, respectively. An infrared camera was set up in the wire arc additive manufacturing (WAAM) and laser metal deposition (LMD) manufacturing units for global thermal imaging during the manufacturing process. Obviously, the infrared camera was installed far from the melting area to obtain a large enough imaging field of the manufactured parts and to protect the camera from potential damage in the complex processing environment.
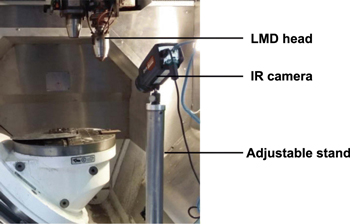
Figure 5. The experimental thermal monitoring setup utilized in LMD. Reprinted from [ 54 ] Copyright 2019. With permission of Springer.
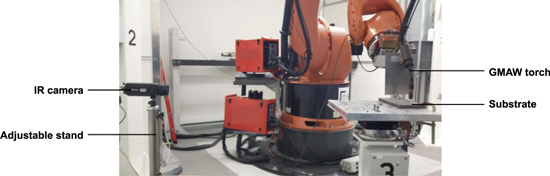
Figure 6. The experimental thermal monitoring setup utilized in WAAM. Reprinted from [ 54 ] Copyright 2019. With permission of Springer.
To calibrate the thermal imaging camera used for thermography measurements, preliminary tests were performed. The stainless steel material's emissivity was set to 0.7. Regarding the conversion from red, green, blue (RGB) to temperature, the camera directly provided a value proportional to the temperature of the objects based on the emissivity value. Meanwhile, the image resolution for the WAAM and LMD applications was approximately 0.5 mm/pixel and 0.2 mm/pixel, respectively.
As presented in figure 7 (a), an infrared camera was used to acquire in situ thermal data. As shown in figure 7 (b), the high temperature zone (HTZ) is highlighted in black. As the liquid phase transition resulted in a local drop of the emissivity properties, the temperature measured at the melt pool location was significantly lower than expected. In figure 7 (b), it can be noted that the HTZ is following the torch path.
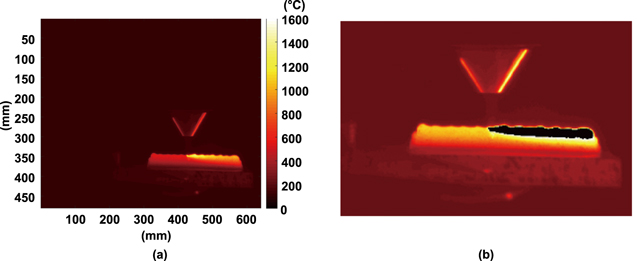
Figure 7. The in situ thermal monitoring in the LMD manufacturing of wall B: (a) thermal field and (b) presentation of the high temperature zone (HTZ). Reprinted from [ 54 ] Copyright 2019. With permission of Springer.
As a promising technology, WAAM has attracted much more attention due to the ability to build fully dense components by continuously depositing layers in the form of wires. Meanwhile, the deposition height is considered to be a key factor for metallic deposition components, which can be detected by the automatic methodology in the robotic gas-tungsten-arc (GTA) based AM processes. For instance, as described in [ 55 ], the optical filters and camera installed monitored the deposition height, defined as the distance from tungsten tip to the top layer. Furthermore, specially designed imaging processing algorithms were used to extract the characteristic parameters of the melting pool. By designing a new tracking algorithm and analyzing continuous images, the location of the solid–liquid separation in the melting pool was determined. Finally, the tracking algorithm was demonstrated to have outstanding effectiveness and anti-interference ability in the GTA-based AM processes. As presented in figure 8 (a), the robotic GTA-based system mainly includes the MagicWave 3000 Job G/F powder equipment, a Motoman MH24 six-axis robot, KD4010 wire feed equipment, and so on. Figure 8 (b) shows the locations of the GTA torch and the optical sensor, respectively.
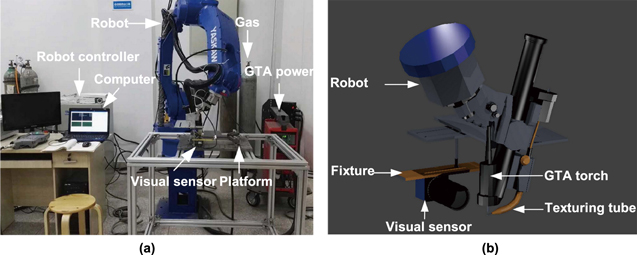
Figure 8. The robotic GTA-based AM systems: (a) experimental system and (b) position relationship between the GTA torch and visual sensor. Reprinted from [ 55 ], Copyright (2019), with permission from Elsevier.
As presented in figure 9 , the visual sensor consists of two filters and a camera; and the sensor is used to observe the current layer and tungsten tip. As a result, the image captured by the sensor in the process of manufacturing is shown in figure 10 . The image is divided into five areas: background, solidified layers, tungsten, arc, and molten pool. The gray values in the area of the arc equaled 255, while the gray value in the area of the background equaled from 0 to 15. As the gray values sharply change along the interface, the molten pool can be conveniently distinguished in the current manufacturing layer.
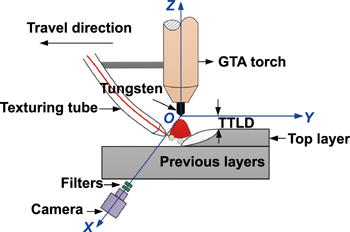
Figure 9. The definition of tungsten to top layer distance (TTLD) and schematic of visual sensing system. Reprinted from [ 55 ], Copyright (2019), with permission from Elsevier.
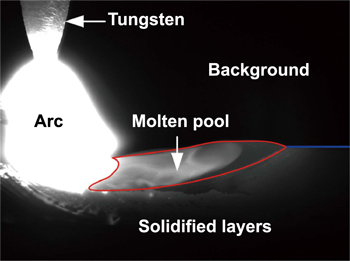
Figure 10. Captured image in the robotic GTA-based AM system. Reprinted from [ 55 ], Copyright (2019), with permission from Elsevier.
In [ 56 ], the MER-125-30UM-L camera sensor used has a sampling rate of 30 fps; and it can capture 1292 × 964 pixel images. Meanwhile, the distance from the tungsten to the lens is 130 mm, and the lens' focal length is 35 mm. In order to protect the camera from strong radiation in the complex manufacturing environment, the dimmer glass was installed in front of the camera, enabling a high-quality image of the molten pool to be obtained with a suitable aperture of the camera. As the deposited region mainly radiates red lights under high-temperature conditions, a filter with a center wavelength of 685 nm was installed in front of the camera, guaranteeing high contrast images of the molten pool and manufactured layers. By utilizing the narrow-band filter, only 685 nm spectra can pass through it; and the images captured by the sensor were composed of an intensity of 685 nm and near this wavelength.
In the images monitored, the position of the tungsten tip is fixed as the camera sensor is installed on the GTA torch. The main challenge is determining the edge of the layer for measuring the tungsten to top layer distance (TTLD). The characteristics of the layer's surface can be extracted from the captured images by the image processing algorithms designed. As shown in figure 11 , image processing in the GTA AM processes consists of several procedures, e.g. gaussian filter process, edge detection, threshold segmentation, Hough transform, and image fusion. As seen in [ 56 ], the original image is handled by some designed algorithms, the gaussian filter is used to remove noises, and the Hough transformation is used for top edge fitting. Once the row coordinate of the surface is obtained, the TTLD value can be calculated by the row deviations.
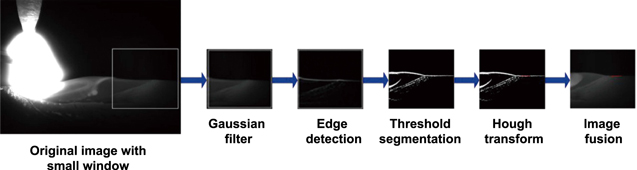
Figure 11. The captured image processing for determining TTLD in GTA-based AM systems. Reprinted from [ 56 ] Copyright 2019. With permission of Springer.
Usually, AM processes involve a variety of complex physical phenomena; and complex process control strategies are required to achieve the advanced mechanical properties of metal parts. Moreover, the complexity of DED technology is even higher when compared with other metal AM technologies. The DED technology has a greater potential for high flexibility and efficiency, but its process control is much more difficult. In [ 57 ], a multisensor method is presented that combines the online signals with data from offline inspection equipment when inspecting the quality of the parts. Furthermore, this data fusion method for process monitoring forms the basis of the process modeling phase; therefore, this method lays the foundation for the realization of intelligent control strategies which would work by adjusting machine process parameters by tracking part size and mechanical quality. The advantages of the proposed method have been evaluated through special experiments on the DED machine.
The major content of this research is represented by the fusion of different kinds of data collected by various sensors during and after deposition. There are some data that can be exploited, such as online machine tracing, which consists of laser location, laser power, and the moving velocity. Meanwhile, the thermal measurements and features of the molten pool are included as the online monitoring categories. Furthermore, there are some points about offline inspection, for instance, porosity from microscopy, 3D reconstruction, and so on.
In the process of manufacturing, the monitored data may be space referenced and time referenced and in both situations using different resolutions. Meanwhile, a comprehensive data fusion method must be used to take full advantage of the information due to its heterogeneity. As presented in figure 12 , the flow of data fusion is quite clear, and this data fusion method takes full consideration of the data acquired through the experimental equipment. The camera captures molten pool images in high velocity, and the machine programmable logic controller records the laser spot location and laser power simultaneously. In addition, the geometry of the resulting samples is 3D scanned after each deposition. Moreover, these data are then recorded and integrated in space or time.
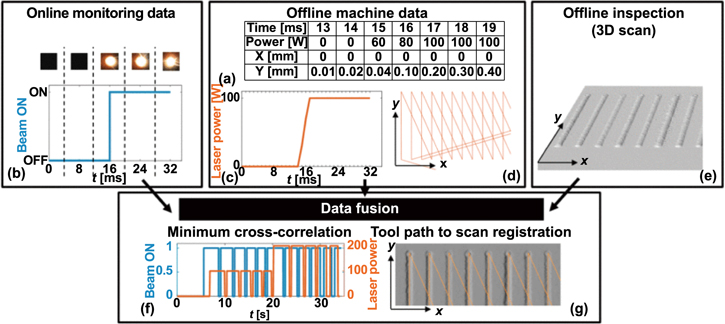
Figure 12. The data fusion methodology. In the machine log file (a), the tool position and laser power are recorded in every stamp. This information for the time-alignment (f) of the molten pool images for the computation of the cross-correlation between the image retrieved beam-ON signal (b) and the laser power signal (c), and for the spatial registration (g) of the tool trace (d) to the 3D deposited geometry (e). Reproduced with permission from [ 57 ].
As presented in figure 13 , the experimental equipment used for the current research is the Prima Power LASERDYNE ® 430 3-axis computer numerically controlled (CNC) machine. The 3-axis CNC machine was originally designed for laser cutting operations, and it has been converted to an AM machine by integrating the four-nozzle Optomec DED head with a forward-looking infrared (FLIR) camera. The FLIR camera is installed onto the deposition head, and it is able to monitor the melting pool by means of the dichroic mirror in the optical channel. For offline measurements, the GOM ATOS Core 200 fringe projection scanner can provide a 3D mesh representing the surface of the part being constructed.
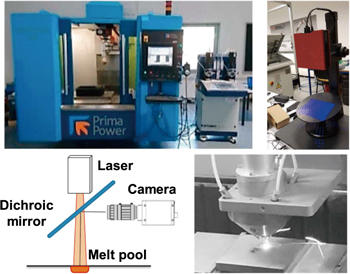
Figure 13. The presentation of equipment: the DED machine (top left), 3D scanner (top right), high-speed camera for monitoring the melting pool (bottom left), and the deposition head (bottom right). Reproduced with permission from [ 57 ].
With the development of multimaterial techniques and component embedding, an AM technique for creating enhanced or multifunctional parts is emerging. In the current state of technology, parts fabrication processes usually require interruption and part removal, which is referred to as stop-and-go fabrication. Position error of the parts can occur during the process of repositioning the parts prior to resumption of manufacturing. In the electron beam melting (EBM) system, the manufacturing environment is an important challenge for stop-and-go fabrication. The ultrahigh vacuum is up to 10 −4 Torr; and the processing temperature is up to 800 °C, which limits access to the EBM chamber. In [ 58 ], part-positioning technology based on infrared images was successfully developed for the EBM manufacturing system to reduce position error for stop-and-go fabrication. In [ 58 ], the infrared imaging technology developed was applied to the stop-and-go fabrication of rectangular prisms and cylindrical components. After manufacturing, the angular and linear displacements of the rectangular prisms and cylindrical components were measured; and as a result, the absolute maximum values were 0.87 mm and 0.17 mm, respectively. Therefore, the infrared image analysis technique of parts positioning errors can be effectively used in multimaterial fabrication, AM fabrication, and so on.
In this system, the expected beam position ( x – y point) relative to the parts in the powder bed has to be considered and determined to accurately build on top of the previous layer. As presented in figure 14 , the infrared (IR) camera was installed outside the manufacturing chamber; and the infrared image analysis algorithms were designed using MATLAB ® software, extracting the rotation and centroid position of a part. Thus, the angular and linear offsets of the parts can be calculated effectively. As shown in figure 15 , the flowchart of the infrared image analysis method is divided into five steps. First, the infrared images are acquired by the IR camera. Then the infrared images are converted into binary images that are used to calculate the centroid location and displacement. Finally, the analysis algorithms are used to detect the part edge and calculate the angular displacement, and the computer-aided design file is updated.
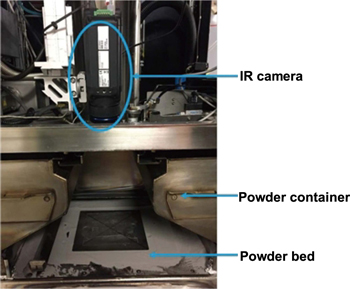
Figure 14. Infrared (IR) camera setup. Reprinted from [ 58 ] Copyright 2018. With permission of Springer.
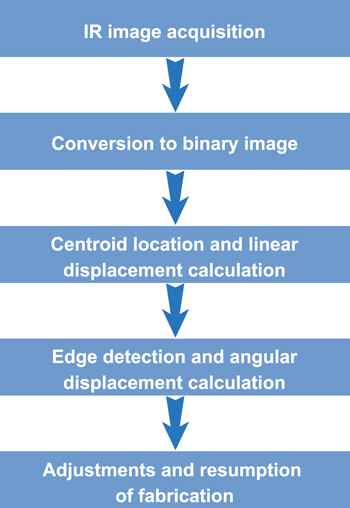
Figure 15. Schematic of the part-positioning technology. Reprinted from [ 58 ] Copyright 2018. With permission of Springer.
An example of an infrared image converted to a binary image is presented in figure 16 . Usually, the two-dimensional image can be considered as a matrix with c columns and r rows; and each position ( r , c ) in the matrix correlates with a pixel in the image. In every pixel, the binary image can be described in black and white, whereas color images can be represented by three 16 bit values, including RGB. In this research, the use of white pixels (pixel value = 1) specifies the area of the part image captured in the process of manufacturing, while the use of the black pixels (pixel value = 0) represents the background. Furthermore, the boundary of the part can be found from the binary image so that the changes in the rotation and centroid position of the part can be measured effectively. As a result, the binary images in this paper were used to detect the part edge with the characteristics of low cost and convenience, whereas more methods were explored by utilizing RGB color images.
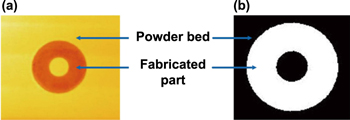
Figure 16. The image captured of the fabricated part. (a) Infrared image and (b) converted binary image. Reprinted from [ 58 ] Copyright 2018. With permission of Springer.
2.2. Laser welding system
During laser welding, the material interacts with the laser beam and gives rise to a range of different process characteristic signals. These can include airborne and structure-borne acoustic emissions, back reflection of the laser beam itself from the interaction zone, and process emissions emitted by the metal vapor in and above the keyhole (present either as a plasma or plume), the molten pool, and the base metal [ 59 ].
The first monitoring approach in the work of [ 59 ] investigated optical-based signals associated with these process emissions. Photodiodes, sensing visible and near-infrared (NIR) emissions, were used to monitor laser-induced, radiative emissions from the laser interaction zone. Correlations of these signals with different types of weld discontinuities were investigated, such as incomplete penetration and porosity. Photodiodes have the advantage of being low cost, capable of detecting process emissions over a wide wavelength range (from ultraviolet (UV) to IR), and are suitable for use in industrial manufacturing environments. In general, a spectral range of photodiodes of 200–850 nm suitable for analyzing emissions from the laser interaction zone has been identified by the research community [ 60 – 63 ]. Bono et al [ 64 ] investigated an established optical-based monitoring method, using photodiodes responsive to either visible or NIR emissions, to correlate the photodiode signals with different types of weld features, imperfections, and/or process anomalies. These included occurrences of internal porosity, changes in penetration depth and beam-to-joint alignment, deliberate changes in laser power, and intentionally poor joint preparation, cleanliness, or fit-up. Also, a new type of commercially available process monitoring approach, based on laser interferometry, was investigated to measure keyhole depth. The results of both approaches confirmed the types of weld features, imperfections, and/or process anomalies most sensitive to these monitoring techniques, with the potential of implementing these findings in a future multisensor solution for real-time process control.
As shown in figure 17 , a continuous wave 5 kW Yb fiber laser beam source (1070 ± 10 nm) was used in the welding trials. The laser beam was delivered through a 0.15 mm core diameter optical fiber to a Precitec welding head comprised of a 100 mm focal length collimating optic and 200 mm focal length focusing optic, providing a 0.3 mm nominal diameter spot at focus. The laser power at the workpiece was measured prior to welding using a water-cooled Ophir power meter. The in-process depth meter (IDM) system was integrated into the welding head. The head itself was fixed onto a mounting plate attached to a z -axis, and an Aerotech ATS50045 linear stage was used to move the workpieces beneath the head in the x – y plane. The head was set up so that the beam impinged vertically on the workpiece surface. Argon shielding gas was used for top bead and under bead shielding. The IDM sensor was able to acquire keyhole depth data at a sampling rate of up to 70 kHz, measuring depths up to 10 mm. A collimation unit on the welding head allowed z -adjustment of the focal position of the measurement beam and an integrated x – y displacement of its alignment to the welding keyhole. An RS422 interface was used for real-time data transmission in combination with a Windows ® -based PC, running the IDM CHRocodile Explorer software.
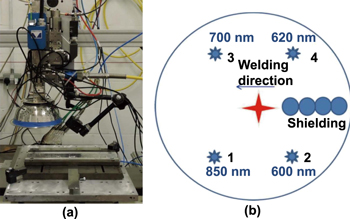
Figure 17. (a) Image of the equipment setup, comprised of the welding head, the in-process depth meter (IDM), and the four photodiodes. (b) Sketch showing the radial positioning of the photodiodes in relation to the welding direction and shielding gas pipes. Reproduced from [ 64 ]. CC BY 4.0 .
A total of four photodiodes were also used. Different narrow bandpass filters were selected, one for each sensor, centered on wavelengths of 600, 620, 700, and 850 nm. A data processing software was used to analyze the photodiode signals. The software included a data processing unit to which a monitor, keyboard, and mouse were connected. A second unit was comprised of digital channels to which the photodiodes could be connected (one per channel) via a Bayonet Neill-Concelman connection. During the trials, data acquisition was performed at 32 768 Hz (2 N , N = 15). The four photodiodes and their related filters were held in position around the laser keyhole in a hemispherical metallic support fixed onto the welding head. Figure 17 (a) shows an image of the laboratory setup, comprised of the welding head, the IDM, and the four photodiodes. Figure 17 (b) shows a sketch of the radial positioning of the photodiodes in relation to the welding direction and the trailing shielding gas pipes used when welding. The photodiodes were aimed at the top of the keyhole at an angle of approximately 45° from the workpiece surface.
Figure 18 shows 700 nm photodiode results from a grease-contaminated butt weld in the same alloy. No background signal correction was applied during the welding trial. In this experiment, the laser power was stepped up halfway along the length of the weld from 1 to 2 kW. Similar results were achieved from the 600, 620, and 850 nm photodiodes. In the case of joint contamination, it was possible to observe an increase in local signal noise corresponding to that length of joint which had been contaminated. Signal noise might, therefore, be used to infer the presence of contaminated joint lengths.
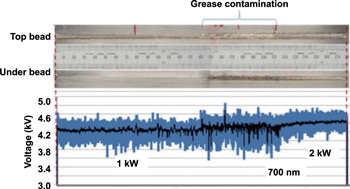
Figure 18. Top bead, under bead, and 700 nm photodiode signal data for a butt weld in nickel (Ni) alloy 718 whose central third length was contaminated with grease. Reproduced from [ 64 ]. CC BY 4.0 .
Figure 19 shows an example of the 850 nm photodiode data from a butt weld in the same alloy, welding over a gap whose width gradually opened from 0 mm (at the weld start) to 1 mm (at the weld end). In this case, a constant beam power of 2 kW was used. Similar results were achieved from the 600, 620, and 700 nm photodiodes. When a joint gap between two abutting workpieces was present, a decrease in the average photodiode signal value was observed, combined with an increase in its noise from the interaction of the beam with the welding jig underneath the joint. Again, this type of change in the signal could be used to infer unacceptably wide joint gaps.
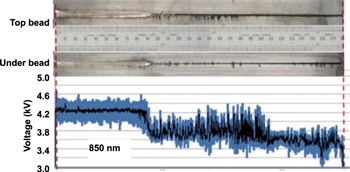
Figure 19. Top bead, under bead, and 850 nm photodiode signal data for a butt weld in Ni alloy 718, when welding over a gradually opening joint gap. Reproduced from [ 64 ]. CC BY 4.0 .
It is essential to install process monitoring equipment in the laser welding system, thereby improving the productivity and ensuring stability. There are some phenomena, such as light emission, stress wave, and optical imaging, that can be observed in the process of welding [ 65 ]. In the process monitoring system, some sensors are integrated as a whole system in order to measure more processing features. For example, in [ 66 ], Zhang et al used the contact probe to measure the voltage of plasma, measuring the charge intensity and analyzing the behavior of the plasma. In [ 67 ], according to the welding conditions, the IR and UV signals were measured and analyzed at the same time. In [ 68 , 69 ], two infrared photodiodes with a wavelength of 950 nm were installed at different angles to measure the plasma phenomenon. In [ 70 ], the P, T, and R sensors were utilized to measure the backscattered laser, the weld pool, and the light of the plasma. In [ 71 , 72 ], Park et al used the photodiodes to measure the experimental parameters, examining the relations between the plasma signals and the welding quality. Furthermore, in [ 73 ], Sibillano et al analyzed the plasma's spectra under aluminum laser welding, while Palanco et al [ 74 ] analyzed the spectra of plasma under aluminum welding. Finally, in order to control the weld penetration, Jauregui [ 75 ] utilized four photodiodes for detection during the laser welding processes.
As described in [ 76 ], a monitoring system was developed for measuring the plasma signals to monitor laser welding processes. The plasma emission characteristics between the aluminum alloy and the laser were analyzed by using the spectral information. Furthermore, by means of experiments, the relationship between the monitoring signals and the process parameters was established; and the prediction algorithms were developed by using the monitoring signals to predict the welding quality. The estimation algorithms predicted the tensile strength by using a neural network model with the pattern recognition algorithm, and the user interface was designed to use this algorithm conveniently.
Figure 20 presents the experimental devices for laser welding which utilize the filler wire provided in front of the laser's moving direction of travel, and the Nd:YAG laser has a maximum output of 4.4 kW. As shown in figure 20 , the laser is tilted approximately 18º from the vertical direction to protect the optical components from damage by the reflected laser. Usually, in order to improve the quality of welded components, it is essential to monitor and control the weld penetration during the welding processes. As the penetration cannot be observed directly from the connectable, movable frontside sensors, monitoring of the weld penetration is a large challenge. Therefore, as frontside sensors can presently measure the signals that inherently correspond to the weld penetration, it has become an attractive project for many researchers.
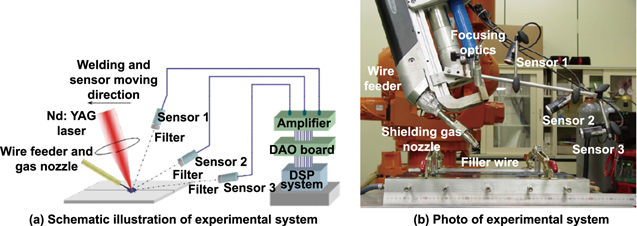
Figure 20. The experimental setup for the laser welding and monitoring system. Reprinted from [ 76 ] Copyright 2016. With permission of Springer.
In [ 77 ], the infrared images were captured and analyzed, illustrating the relationships between the torch position, the weld bead width, and the weld penetration depth. In [ 78 ], Carlson et al estimated the sidewall penetration by using ultrasonic signals. In [ 79 ], the 3D weld pool measurements were reviewed by Wang et al Furthermore, in [ 80 ], the 3D vision sensing system was designed and demonstrated in the gas tungsten arc welding (GTAW) system, which can measure the welding pool surface with weld penetration, to measure the 3D specular welding pool spectrally. This method is considered promising as skilled welders can estimate successfully and control the weld penetration based on observations of the welding pool (mainly 3D surfaces). The authors of [ 80 ] considered some frontside signals as features, such as the welding pool's length, width, etc, aiming to improve the prediction of the bead width. As a result, it demonstrates that the key issue is how to build an accurate model from a bit of training data, as the cost of generating training data is quite high.
In the Welding Lab at the University of Kentucky, an experimental system was designed for the GTAW processes, as shown in figure 21 . In the GTAW processes, the material for welding was stainless steel 304, the wall thickness of the stainless steel pipe was 2.03 mm, and the outer diameter was 113.5 mm. Meanwhile, the 19 by 19 dot matrix laser pattern was generated by the 20 mW illumination laser with a wavelength of 684 nm, which was easily reflected by the melting pool. Under the influence of the plasma generated by the arc, the surface of the welding pool was deformed and depressed, which caused the reflection point matrix to be deformed. As presented in figure 22 (a) [ 81 ], the 3D molten pool was reconstructed by utilizing the image processing effectively. However, in order to improve the ability to predict the bead width more accurately, more nonlinear modeling methodologies were needed which would overcome the limitation of the large amounts of training data needed for experiments. Therefore, a support vector model was proposed in [ 81 ], utilizing kernel functions and reducing the experimental data effectively.
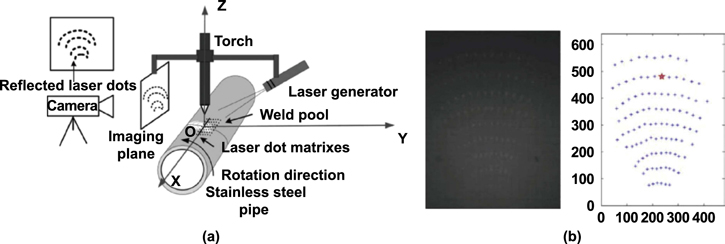
Figure 21. The 3D vision-based monitoring system. (a) The sensing system and (b) the captured image. Reproduced with permission from [ 80 ].
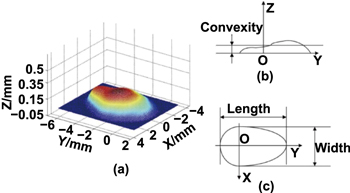
Figure 22. The 3D weld pool surface and its characteristic parameters. (a) The presentation of the 3D reconstruction of the molten pool, (b) weld pool surface convexity, and (c) weld pool width and length. Reprinted from [ 81 ], Copyright (2019), with permission from Elsevier.
Today, multisensor technology is widely used in the field of laser welding. In [ 82 ], x-ray transmission imaging equipment and high-speed camera sensors were used to observe the welding processes, analyzing the welding velocity and laser power density's influence on the welding formation. The welding width and molten pool size can be directly monitored by the radiation intensity received [ 82 , 83 ]. In [ 84 ], the effects of welding velocity on high frequency signals and the relationship with the high frequency were studied using an optical filter and photodiode, acquiring laser reflection and visible light radiation in the process of welding. In [ 85 ], a spectrometer was used to acquire the spectral distribution; and the high-speed camera was utilized to capture the keyhole image. Meanwhile, the weld penetration and weld width were detected by extracting the parameters. You et al used a variety of sensing technologies in the laser welding processes, quantifying various physical and optical characteristics to fully analyze the defects of laser welding. In [ 86 ], as shown in figure 23 , the recognition model was built and the welding state predicted by extracting and analyzing the features from six imaging sensors, resulting in the timely monitoring of the high-power laser welding. In this system, the high-speed camera sensor in the visible wavelength band and ultraviolet band was installed to capture the plume images during the laser welding of stainless steel 304. In addition, the molten images were captured by an x-ray imaging system and NIR wavelength band. The laser-reflected intensity and the visible light intensity were acquired by using a laser sensing photodiode and visible light photodiodes. Furthermore, the correlations between welding conditions and welding features were analyzed by extracting the optical signals from different laser welding conditions.
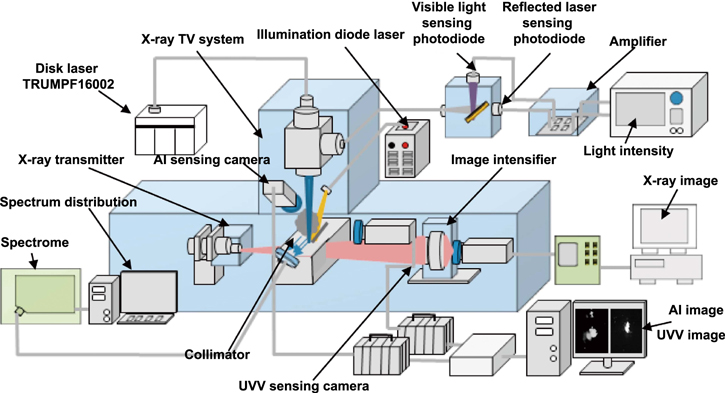
Figure 23. The schematic of a six-sensor monitoring system for laser welding. Reprinted from [ 86 ], Copyright (2015), with permission from Elsevier.
As presented in figure 23 , the multisensor system includes an ultraviolet/visible (UVV) visual sensor, an x-ray visual imaging sensor, a spectral graph, a visible light photoelectric sensor, a laser reflective photoelectric sensor, and an auxiliary illumination visual sensor, which are utilized to achieve timely detection and analysis during laser welding. Meanwhile, the multisensing system and welding system can be synchronized by the trigger sensor, which is installed under the table and connected with the signal box, providing a trigger signal for the sensing system and laser machining system.
Moreover, the metal spatters and plume images can be obtained by means of the high-speed camera sensor with the visual induction filter, analyzing the characteristics of metal spatters and plume. Meanwhile, for analyzing the characteristics, the molten pool images can be obtained by the 40 W diode laser of 976 nm wavelength and the high-speed camera equipped with a narrow band filter. By connecting two fibers to the laser head, the welding areas' light intensity can be transmitted to the optical splitter; and the laser reflection intensity and light intensity can be obtained by the two photoelectric sensors, respectively. The x-rays are emitted from the left side of the weldment and penetrate the entire welding area. Differences in material density cause the different degrees of attenuation of x-rays. In addition, the spectrometer collimator is arranged at the front of the welding direction and focuses on the plasma area. The spectrometer receives spectral information through the optical fiber.
The binarization processing of images is illustrated in figure 24 (a). The median filter is utilized to remove the noise, and thus the color images can be successfully converted to binary images. As presented in figure 24 (b), in the binary images, the ordinate value of keyhole forefront and the top area of keyhole (KS) have been extracted for describing the characteristics more fully. Furthermore, in [ 87 ], the six sensors produced 42 features, and the penetration with weld width were acquired as welding parameters. An association analysis was performed to select the 16 pivotal features most close to the welding situation parameters, and a quantitative analysis was performed on the association rules between the welding parameters and the pivotal features. Under different laser welding conditions, the relationship between the welding features, welding condition, and welding state was revealed by means of a series of experiments. The welds formed were produced in different states, such as blowout, hump, dimple, and stabilization. In order to identify the welding state, the Bayesian network defects detection model was established based on the key features. The tree-augmented naïve (TAN) Bayesian network model is superior to the Markov Bayesian network model, and the prediction accuracy of the TAN Bayesian network model can reach 86.04%, which is 10.92% higher than that of the Markov model.
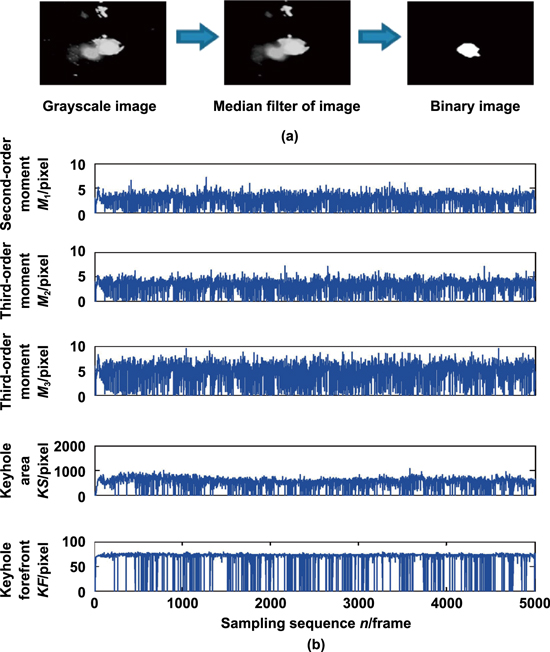
Figure 24. The AI visual sensing signal processing. (a) The keyhole imaging processes and (b) configuration parameters of the keyhole. Reprinted from [ 87 ], Copyright (2019), with permission from Elsevier.
2.3. Milling system
Milling is a common and effective cutting operation, which can intermittently cut components into threads, flat surfaces, and many complex components by using a rotary cutter with one or more teeth. Milling processes are widely used in industrial manufacturing because they are suitable for mass production. Tools are the backbone of the milling process [ 88 ], and tool breakage is the main cause of unplanned downtime in the machining environment. Tool breakage has a negative influence, including indirect and direct effects. Milling machine downtime because tool failure accounts for 7%–20% of total downtime [ 89 , 90 ], while the cost of tools and tool replacement accounts for 3%–12% of the total machining cost [ 91 ]. The timeliness of detecting tool damage is quite important as the condition of tools changes over time, and a suitable tool replacement strategy is needed. In general, tool change strategies are subjective and periodic, and the time period depends on the operator's experience. Early replacement of usable tools wastes tools and increases downtime, while obsolete replacement of worn tools leads to reduced workpiece quality and increased production costs. Therefore, in the milling processes, tool condition monitoring (TCM) has become a critical issue.
In the TCM system, a single sensor cannot achieve good robustness because of the uncertainty and limitations. Processing signals can be obtained by different kinds of measuring sensors, such as current, cutting force, vibration, etc. Therefore, research on multisensors in TCMs has become a trend as the multisensor method can effectively reduce the uncertainty of measurements, enhance the richness of the measuring data, and improve accuracy. Zhou et al [ 92 ] illustrated that in the milling processing system, multisensor methods have been used more frequently. At present, some studies have found that the number of measuring sensors and feature parameters is not directly related to prediction accuracy. The main purpose of feature extraction is to extract feature parameters from the signals and significantly reduce the size of the original information. The monitoring model is greatly affected by the feature extraction, and the model computation would be greatly increased by some characteristic parameters. Zhang et al and Grasso et al [ 93 , 94 ] showed that the performance of the monitoring system can be affected negatively by redundant and irrelevant characteristics' parameters. Presently, feature selection and feature fusion are the two major kinds of multidomain feature extraction methods. Through linear or nonlinear mapping, the new parameters from preset sensor feature parameter sets can be obtained by feature fusion methods, and the new characteristics' features are utilized as the monitoring model's input parameters [ 43 , 44 ]. For example, in [ 95 ], the feature parameter dimension was reduced; and the new fusion parameters were established by using the local preserving projection algorithm. Furthermore, Wang et al [ 96 ] utilized the kernel principal component analysis algorithm to fuse the 54 feature parameters in the frequency domain and time domain. However, although the feature fusion methods have the advantage of providing more comprehensive information and reducing feature dimensionality, all of the sensors must be calculated; and the maintenance cost for the online monitoring may be increased. For instance, Wang et al [ 97 ] selected 13 parameters that significantly correspond to the tool wear values by using Pearson's correlation coefficient from 144 feature parameters. In [ 91 ], the smallest redundant feature, which includes 19 feature parameters, was established by means of the fast correlation filter algorithm, and the total of the frequency domain, wavelet energy, and time domain parameters was 138. Not only did the feature selection methods reduce the number of input parameters, but they also reduced the computation. Nevertheless, the main consideration of these methods is the relationship between the tool situation and the parameters and not the effects on prediction accuracy. Therefore, it is essential to acquire the optimal sensor feature parameter combinations to improve the prediction accuracy effectively.
Introduced in [ 98 ], as presented in figure 25 , is a multisensor fusion method; and the operation of this method consists of three steps. First, the dynamic signals are collected to characterize the milling process. Then the method extracts the parameters from multisensors in the frequency, time, and time-frequency domains. Finally, the tool situation is monitored, and the tool wear values are judged by using the kernel extreme learning machine (KELM), in which the optimal sensor parameter combinations are the inputs.
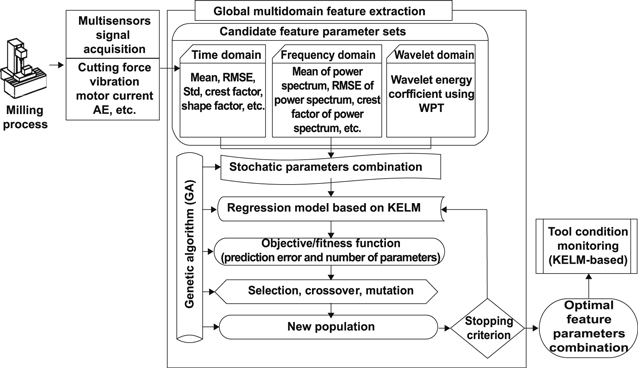
Figure 25. Schematic of the proposed tool condition monitoring methodology. AE: acoustic emission; WPT: wavelet packet transform; KELM: kernel extreme learning machine; RMSE: root mean square error. Reproduced from [ 98 ]. CC BY 4.0 .
In [ 99 ], the research focuses on developing a multisensor measuring system on a rotating tool, which can measure the vibration, temperature, torque, and three components of cutting force simultaneously in a wireless environment. Meanwhile, the cutting force signal is produced by utilizing a beam leg transducer embedded in a standard milling cutter holder. A miniature accelerometer is placed under the force sensor, while a thermocouple is placed near the cutting edge, as shown in figure 26 . Furthermore, by incorporating the inductive wireless transmitter into the standard rotating tool holder, all of the signals are obtained and transferred to the data logger system. As a result, it is suitable and reliable for measuring the machine signals by the multisensor system according to the verification and experimental tests described in this article.
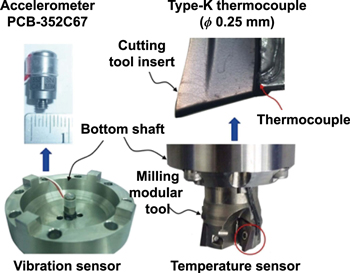
Figure 26. Setup of vibration and temperature sensor. Reprinted from [ 99 ] Copyright 2018. With permission of Springer.
2.4. Condition monitoring and fault detection system
In the process of manufacturing, fault detection and condition monitoring are required to prevent machine failure, extend the life of a machine, reduce operating costs, and increase operating time, which can be achieved by different types of monitoring sensors. Recently, as presented in [ 100 ], infrared thermal imaging methods are beginning to be proposed. Furthermore, infrared thermal imaging can achieve nonintrusive, noncontact, and fine-grained temperature measurements, which is critical for machine condition monitoring and automatic fault detection. Infrared thermal imaging is mainly used with machine learning and image processing to enable the system to detect conditions and faults autonomously [ 101 – 104 ].
It has been proven that the utilization of infrared thermal imaging technology can detect several faults and conditions in rotating machinery, such as loose couplings, misalignments, unbalanced rotors, insufficient lubricants, and damage to the rolling bearings [ 104 – 107 ]. Under certain conditions, it is easy to distinguish why infrared thermography is a benefit in detecting faults/conditions, such as a lack of lubricant. Friction control is one of the main purposes of lubricants. Too little lubricant in the bearing housing can cause excessive friction, which can generate heat that the infrared camera can detect. In addition, if there is too much lubrication in the bearing, agitation can occur; and additional heat can be generated.
Features extracted from established vibration analysis techniques can be used to make up for shortcomings of the infrared thermal-imaging-based methods. Therefore, combining these two methods in a multisensor system can improve accuracy. In [ 108 ], the vibration data and infrared thermal imaging data are used to extract features and fuse features, with the fused features subsequently provided to the classification algorithm.
As shown in figure 27 , during testing, the settings for the rolling element bearings in the right housing were changed, thus this is the housing monitored by the thermal imager. In addition to the infrared camera, a thermocouple was placed indoors to measure the ambient temperature. In addition, two accelerometers were mounted on the bearing block to measure accelerations in the x and y directions. The results showed that several conditions/faults and combinations were detected more accurately by combining these two types of sensor data than when considering sensor flow alone.
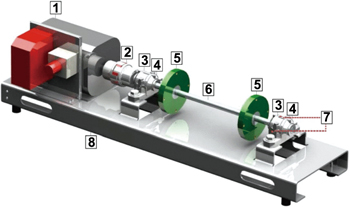
Figure 27. The 3D presentation of the experimental setup. The IR thermal camera is utilized to monitor the red square. (1) Servo-motor with a speed controller, (2) coupling, (3) bearing housing, (4) bearing, (5) disk, (6) shaft, (7) accelerometers, and (8) metal plate. Reproduced with permission from [ 108 ].
Transformers are critical electrical equipment in power systems. Thus, it is essential to find potential transformer faults, study the fault diagnosis methods of transformers, and improve the safety of power systems. Due to the complex structure of transformers, the causes and phenomena of transformer failures are diversity and randomness. Therefore, effective methods must be adopted to comprehensively diagnose transformer failures. Presently, transformer fault diagnosis methods include the improved three ratio, characteristic gas, Rodgers ratio, fuzzy mathematics, neural network, etc [ 109 ]. However, these methods still have a lot of disadvantages in algorithms. For instance, the accuracy is not high enough and they require a large sample. Therefore, it is necessary to find an effective method for diagnosing the fault of the transformer. On the basis of this [ 110 ], uses the photoelectric sensors and discharge circuit acquisition modules to comprehensively diagnose the fault of the transformer, in other words, to diagnose the fault and determine the location of the fault by means of high-precision data fusion, achieving the purpose of unattended operation. The photoelectric multisensor information fusion technique can combine, coordinate, and supplement the information obtained by multiple sensors, thereby overcoming the uncertainty and limitations of a single sensor.
As shown in figure 28 , the platform monitors the transformer failure by using the photoelectric infrared thermal imaging sensor to measure the transformer's internal temperature. A thermal infrared imaging sensor is a photoelectric conversion device, usually made of indium antimony or mercury compounds. It is used to receive infrared radiation energy, further convert it into electrical signals, and, after amplification, plasticity, and modulus conversion into digital signals, the image is finally displayed on a monitor. The grayscale value of each point in the image correlates to the radiation energy of the detected object at that point. The temperature of the infrared thermal sensor can be conveniently determined by receiving the radiation emitted from the surface of the measured object [ 111 , 112 ].
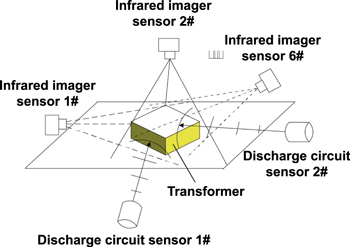
Figure 28. The schematic of the diagnosis setup with multisensor data fusion methods. Reprinted from [ 110 ], Copyright (2019), with permission from Elsevier.
3. Data fusion technology
Data fusion technology has become one of the hot research topics in the world. Data fusion refers to making full use of multiple information sources by combining redundant or complementary information from multiple information sources in space or time according to specific standards to obtain a consistent interpretation or description of the tested object so that the information system performs better compared to the system composed of each subset it contains [ 113 ]. The data fusion process synthesizes different sources of information with mathematical methods and technical tools in order to obtain high quality, useful information. Compared with the independent processing of a single source, the advantages of data fusion include improving detectability and reliability, expanding the range of spatiotemporal perception, reducing the degree of ambiguity of inference, improving detection accuracy, increasing the dimension of target features, improving the resolution of spatial questions, and enhancing the fault-tolerant ability of the system [ 114 ].
In recent years, multisensor data fusion technology has been widely used in both military and civilian fields. It has become a problem of concern to the military, industry, and high-tech developers [ 115 ]. This technology is widely used in command, control, communications, and intelligence (C3I) systems; complex industrial process control; robotics; automatic target recognition; traffic control; inertial navigation; ocean surveillance and management; agriculture; remote sensing; medical diagnosis; image processing; pattern recognition; and other fields [ 116 ]. Practice shows that compared to the single sensor system, using multisensor data fusion technology solves the problem of detection, targets recognition and tracking, enhances the system survival ability, improves the reliability and robustness of the whole system, improves the credibility of the data, improves accuracy, extends the time of the whole system, increases space coverage, increases real-time and information utilization, etc [ 117 ].
Complex industrial process control is an important field in which to apply data fusion. At present, data fusion technology has been applied in the measurement and fault analysis of ultraprecision industrial systems. The purpose of fusion is to identify the defects that cause an abnormal state of the system and trigger an alarm accordingly [ 118 ]. Generally, characteristic data is used for data fusion, and there are different analysis methods to extract characteristic data, such as time series analysis, frequency analysis and wavelet analysis. And then, the characteristic data is input into the neural network pattern recognizer for feature level data fusion. Furthermore, in order to identify the characteristics of the system data, the characteristic data is input into the fuzzy expert system for decision level fusion [ 114 ]. In the fuzzy expert system, domain knowledge rules and parameters are taken from the knowledge base and database to match and fuse with characteristic data. Finally, the operating state, equipment working condition, and fault of the tested system are determined [ 119 ].
3.1. Data fusion hierarchy
Based on the processing information source level, information fusion can be divided into data layer fusion, feature layer fusion, and decision layer fusion [ 120 ]. Data refers to the measurement data collected by sensors in each section. Characteristics are the data results and knowledge after analysis and transformation. Decision making refers to the conclusion of observing the target. The details are as follows.
3.1.1. Data layer fusion
Data layer fusion is the direct fusion of original data. Its input consists of various types of raw data provided by multiple sensors, and its output is the result of feature extraction or local decision-making. The advantages of data layer fusion are that more details can be extracted from the original data that is not found in other fusion layers [ 121 ]. The disadvantages include heavy computing burden, poor real-time performance, and the need for a good fault-tolerant ability to deal with the instability and uncertainty of sensor data itself, which is only applicable to the original data fusion of similar sensors. The methods mainly include the weighted average method, election decision method, Kalman filter method, mathematical statistics method, etc [ 122 ].
3.1.2. Feature layer fusion
Feature layer fusion extracts feature information of data sources for analysis and processing and retains enough important information to provide support for later decision analysis [ 123 ]. The advantages of feature layer fusion are that after extracting the original data information features, the amount of data to be processed is reduced and the real-time performance is improved. The methods mainly include Kalman filter, fuzzy inference, neural network, and production rule.
3.1.3. Decision level integration
As a kind of high-level fusion, decision-level fusion has high flexibility, strong anti-interference, good fault tolerance, and small communication bandwidth requirements [ 124 ]. First, the measurement data of the sensor are preprocessed to obtain a preliminary decision on the research object. Then, all local decision results are combined under certain rules to obtain the final joint decision results. Therefore, decision layer fusion requires compressing sensor measurements, which results not only in high processing costs but also the loss of a lot of detail. Decision-level fusion methods mainly include the Bayesian probabilistic inference method and Dempster–Shafer (DS) evidence inference method.
According to the above analysis, the three different levels of information fusion have their own advantages, disadvantages, and application scope. Typically, features are obtained by fusing the original data; and decisions are made using different fusions. Whatever the fusion strategy, the relevant information needs to be correlated and registered [ 125 ]. The difference is that the data is correlated and matched in a different order. Theoretically, the advantage of data layer fusion is that a large amount of original data can be retained to provide as detailed information as possible for the target and obtain as accurate a fusion effect as possible. Decision layer fusion does not rely on sensors. For a specific purpose, it is a system engineering problem to determine which level of integration to adopt. The comprehensive influence of the environment, computing resources, information source characteristics, and other factors should be considered comprehensively [ 126 ].
3.2. Classification of data fusion algorithms
Data fusion involves various theories and technologies, and there is no completely uniform algorithm that can adapt to all scenarios. Therefore, in application, corresponding algorithms should be selected according to different application backgrounds. The classification of algorithm concept is mainly divided into three categories: physical model algorithm, parameter-based algorithm, and cognition-model-based algorithm [ 127 ].
3.2.1. Physical model algorithm
This kind of algorithm directly calculates the entity characteristics according to the physical model [ 128 ]. The physical model predicting the physical features of an entity must be based on the physical features of the object to be recognized. Although this method is rarely used in practice, it is needed in basic research. The syntactic analysis method and the method of estimation theory belong to a typical physical model algorithm. The syntactic analysis method develops the grammar and syntax of physical objects, the physical objects that are obtained from the sensor data component (or the original information). It is, therefore, possible to aggregate object representations from this assumed structural relationship. Estimation theory includes the Kalman filter, maximum likelihood estimation, and least square method.
3.2.2. Parameter-based algorithm
The parameter classification algorithm is the most widely used and deeply studied algorithm. This algorithm seeks an identification specification and makes it dependent on parameter data, creating a direct image between the parameter data and an identification specification. This kind of algorithm can be further divided into algorithms based on statistics and information theory [ 129 ].
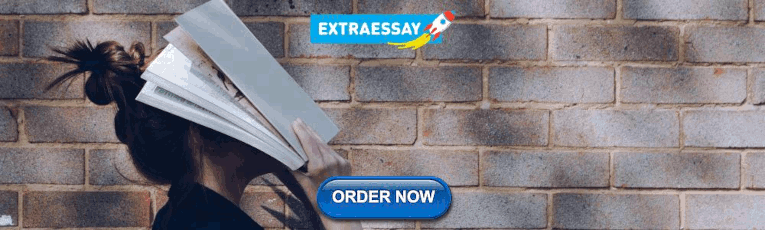
3.2.3. Statistical algorithm
Statistical algorithms mainly include the classical inference algorithm, Bayes inference algorithm, and DS evidence theory algorithm. The classical inference method [ 130 ] describes the probability of the observed data relating to the identification given the assumption that the object exists. For example, it is assumed that each recognized target type is A i and the sensor is j . If n sensors measure m target types, the measurement model can be expressed as normal distribution in formula ( 1 )
where x j is the actual measured observed value, and u ij is the mean value of the target A i to the sensor j , which can be obtained through historical data experience. Its limitation is that it can only estimate two hypotheses: a hypothesis and its alternative hypothesis. When multivariable data is encountered, the complexity of the algorithm increases. The validity of a prior density function is required, otherwise prior estimation cannot be directly used. The Bayes inference algorithm [ 131 ] solves some difficulties of classical inference methods. With the minimum risk cost as the basic model, it can update a hypothetical likelihood function given a prelikelihood estimate and additional evidence (observation).
First, suppose there are n sensors to obtain parameter data of an unknown target. Each sensor provides a description of the target's identity based on sensor observations and a specific sensor classification algorithm. All possible m targets are represented by O 1 , O 2 , O 3 ...O m , D i represents the i th sensor's description of the target's identity, and O 1 , O 2 , O 3 ...O m actually constitutes the incompatible hypothesis of the observation space. It has formula ( 2 ):
where P ( O i ) is the prior probability that O i is true and
where P(O i ∣D i ) is the probability of O i is true and that D i is known; P(D i ∣O i ) is the probability of D i if O i is true.
The disadvantage of Bayes inference is that it is difficult to define a priori likelihood. It is complicated when there are multiple possible hypotheses and multiple conditions. The assumptions that require opposition are incompatible with each other. DS evidence theory [ 132 ] is a generalized extension of Bayes theory, which takes into account the degree of total uncertainty. The DS method uses probability interval and uncertainty interval to determine the likelihood function of a hypothesis under multiple evidence. When all hypotheses are incompatible and complete, the Bayes inference technique and DS method produce the same results.
Assuming that m 1 , m 2 , m 3 ...m n are trust allocation functions and focal elements are A i (i = 1, 2, 3. ..N) , then the DS synthesis rules are as follows in formula ( 4 ):
where K is the conflict coefficient.
Figures 29 (a) and (b) are images of solar cell surface defects taken by 450 and 500 nm multispectral cameras. Image fusion is carried out with the above algorithm. The results are shown in figure 29 (c). The algorithm suppresses the influence of the complex background of polysilicon solar cells on the reliable extraction of surface defects and enhances the defect characteristics. Through the fusion of multispectral images, the contrast of the defect part was improved, and the defect information on a fingerprint and a scratch on the solar cell was enhanced.
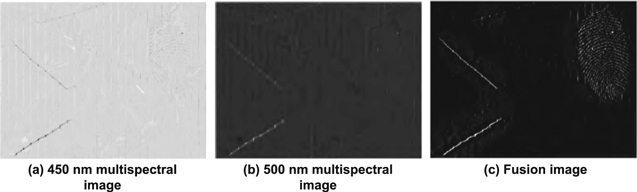
Figure 29. Defect image fusion of solar cells. Reproduced with permission from [ 133 ].
The problem with the DS method is that it cannot deal with contradictory evidence effectively. The amount of computation is increasing exponentially. When the chain of reasoning is long, it is inconvenient to use evidence theory. The DS combination rule has combination sensitivity, but sometimes even a small change in the basic probability assignment may lead to a big change in the result.
3.3. Fusion algorithm based on information theory
In some cases, multisensor data fusion target recognition does not need to directly simulate the random form of observation data by statistical method but relies on the mapping relationship between observation parameters and target identity to identify the target. This kind of method is called fusion recognition algorithm based on information theory, which includes the neural network method [ 133 , 134 ], entropy theory method [ 135 ], and clustering algorithm [ 136 ] and voting method [ 137 ].
An artificial neural network is proposed on the basis of research results of modern neuroscience. The neural network target recognition method is a nonlinear network system composed of interconnection of very simple computational processing units (neurons), with learning, memory, computing power, and various intelligent identification and processing capabilities. All neurons can perform large-scale parallel computation without an external synchronization signal. The most famous neural network target recognition algorithm is the forward multilayer neural network based on adaptive signal processing theory and its back learning algorithm. With the continuous development, Kaiming He et al [ 138 ] proposed a residual neural network (ResNet), which is mainly manifested by taking the characteristic graph obtained by adding the input and output of the network as the final output of the structure on the basis of a shallow network. Adding such a structure into the network cannot only increase the depth of the network but also keep the network performance from degrading. Theoretically, the appearance of ResNet greatly improves the depth of the neural network that can be trained; and optimization of network performance is more free. ResNet is shown in figure 30 .
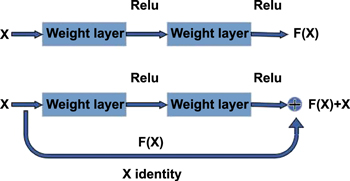
Figure 30. The traditional structure and residual structure of a neural network. Reproduced with permission from [ 138 ].
As can be seen from figure 30 , which compares traditional training methods with a network trained by the residual model, small defect areas on the surface of the magnetic tile can be better identified by the residual model, as shown in the blue circle in figure 31 . In addition, the improved network can also improve the mismarking of the texture of the magnetic tile itself, as shown in the yellow circle in figure 31 .
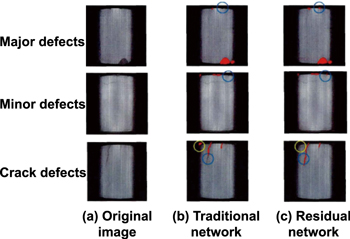
Figure 31. Two kinds of neural network methods of detecting the surface defect segmentation effect in magnetic tile. Reproduced with permission from [ 138 ].
A clustering algorithm is used to gather a large number of multidimensional data samples into categories so that the similarity of samples within a category is the largest, but the similarity of samples in different categories is the smallest. Cluster analysis is a heuristic algorithm that is effective in iconic applications where the number of pattern classes is not precisely known. Clustering analysis can be used as a separate tool to discover some deep, implicit, and useful information about the distribution of data in the database and to summarize the characteristics of each category or to focus on a particular class for further analysis. For instance, k -means clustering aims to partition n objects into k clusters in which each object belongs to the cluster with the nearest mean. This method produces exactly k different clusters of the greatest possible distinction. The best number of clusters k leading to the greatest separation (distance) is not known a priori and must be computed from the data. The objective of k -means clustering is to minimize total intracluster variance or the squared error function, as shown in formula ( 6 ).
Similar to voting in daily life, the voting method is the simplest technique in multisensor data fusion target recognition algorithms. Each sensor provides a judgment on the state of the object under test, which is then searched by a voting algorithm to find a judgment 'agreed' on by more than half of the sensors and the vote is announced. Weighting methods, threshold techniques, and other judgment methods can also be used, which are very useful when there is no accurate prior statistical data available, especially for real-time fusion.
3.4. Fusion algorithm based on cognition model
The algorithms based on the cognition model mainly include the logical template method, fuzzy set theory algorithm, genetic algorithm, and knowledge system method.
3.4.1. Logical template method
The logical template method is essentially a method of matching recognition, which matches a predetermined pattern of the system with the observed data to determine whether the conditions are satisfied and then makes an inference. A predetermined pattern can contain logical conditions, fuzzy concepts, observed data, and uncertainties in the logical relationships used to define a pattern. Therefore, the template is essentially a comprehensive parameter pattern method that represents and matches logical relations [ 140 ]. As a kind of logical template method, the decision template method is a simple and intuitive decision-level fusion target recognition algorithm. The classical decision template method does not make full use of prior information on each sensor's ability to identify different kinds of targets. Zhang et al [ 141 ] proposed to modify the decision template method by using the average measurement entropy of sensors to reasonably measure the ability of multiple sensors to classify and identify different kinds of targets. The simulation results showed that the improved decision template method could improve the correct target recognition rate.
The modified decision template method fusion recognition process diagram is shown in figure 32 . The part in the dotted box is not available in the classic decision template method. The modified matrix is applied to modify the decision distribution diagram of the target, so as to reflect the ability of the sensor to classify and identify specific kinds of targets to obtain the decision distribution diagram of the modified target. The maximum similarity category is selected as the output, and the decision distribution map of the unknown target is classified to realize the fusion target recognition.
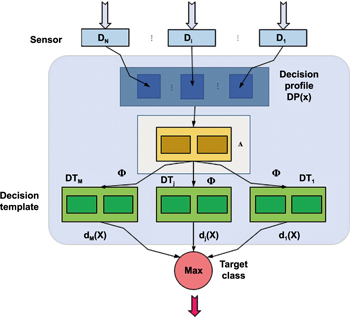
Figure 32. The modified decision template method fuses the identification block diagram. Reproduced with permission from [ 141 ].
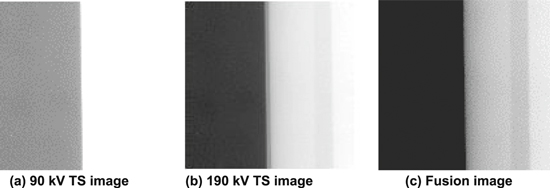
Figure 33. Image fusion results of the titanium alloy edge erosion effect. Reproduced with permission from [ 142 ].
The paper aimed at the defects of the classic decision template method that it cannot reflect the sensor for the classification of the same target identification ability, cannot adapt to the feature vectors to identify the target fluctuation change, and cannot retain all the information of training samples at the same time. It put forward the method based on entropy and the K nearest-neighbor correction decision template method. The decision template for the target of category j , formula ( 7 ), can be obtained:
Figure 33 presents the image fusion results of the titanium alloy edge erosion effects. The multiple sensor fusion target recognition performance is greatly improved.
3.4.2. Fuzzy set theory algorithm
Due to the complexity of the environment, noise interference, the instability of the recognition system, and the influence of different recognition algorithms, the target signal and the extracted characteristic parameter information are inaccurate, incomplete, and unreliable. In addition, there is subjective fuzziness in the judgment method of object recognition. Fuzzy set theory is a powerful mathematical tool to solve such problems. The abundant fusion operators and decision rules in fuzzy set theory provide the necessary means for effective target fusion processing. In recent years, some scholars have begun to apply fuzzy set theory to the problem of object identification. Kewley [ 143 ] used fuzzy logic to fuse multisensor data for target identification based on electronic reconnaissance data. Based on the possibility theory of fuzzy set theory, Roux et al [ 144 ] conducted multispectral satellite image fusion to solve the image classification problem.
Yang et al [ 145 ] established a decision-level fusion model of multisensor target recognition by using the fuzzy synthesis function in fuzzy set theory. According to the probability distribution of accumulated target recognition of each sensor at moment k , the probability distribution of M sensors is fused by the fuzzy synthesis function; and the probability distribution of accumulated target recognition and fusion of the sensor system up to moment k can be obtained as follows:
Liu et al [ 146 ] introduced fuzzy measure and fuzzy integral into multisensor data fusion target recognition. It gave the general technical route of fuzzy integral applied to decision-level data fusion target recognition and provided the measurement method of information source importance.
Among them,
A multiple source, multilevel adaptive variable weight fuzzy algorithm of multisensor target fusion was proposed by [ 146 ] and provides a kind of expression and effective method of dealing with uncertain information, makes full use of the sensor information redundancy and complementarity, and is the source of reliability of the information itself to achieve a good recognition effect.
Figures 34 (a) and (b) are the microscopic image of the stone surface taken by adjusting the focal plane of the microscope lens, while figure 34 (c) is the stone surface image taken by the charge-coupled device camera. Image fusion is carried out with the above algorithm. The results are shown in figure 34 (d). The algorithm is used to achieve microimage fusion, and an approximate full focal image is obtained. The limitation of depth of field of the image sensor can be solved effectively. It can solve the limitation of the field depth of the image sensor and could obtain accurate and complete feature information of the measured object surface.
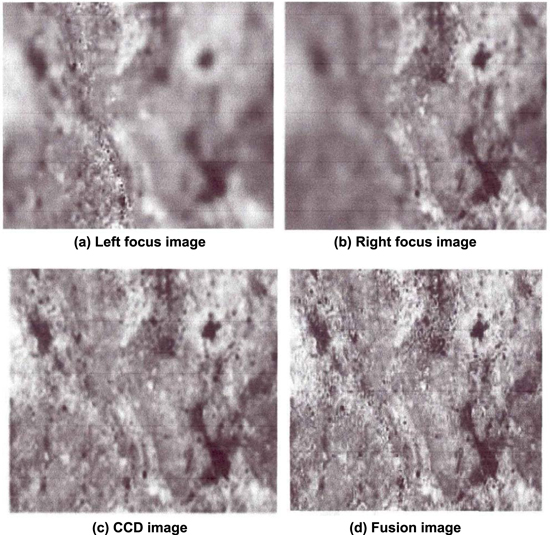
Figure 34. Image fusion results of surface morphology of stone plate. Reproduced with permission from [ 147 ].
Different types of sensors in the same work environment with the ability to identify different algorithms take into account the fuzzy set theory's importance of the information source, reflect objective reality, and result in better fusion recognition than DS evidence theory. Compared with the DS evidence theory, the fuzzy integral domain problem does not involve recognition framework in theory but is beneficial to increasing the recognition framework and improving the practicality of the fusion system.
3.4.3. Genetic algorithm
The genetic algorithm is a population optimization process that starts from a set of initial values for optimization. The optimization process is a process of the population's continuous reproduction, competition, inheritance, and variation [ 148 ]. In [ 149 ], the genetic algorithm and neural network classifier are combined, as shown in figure 35 , by identifying the resulting feedback and control characteristics of the genetic evolution direction of the genetic algorithm to realize optimization and overcome the premature convergence problem of the genetic algorithm and put forward some choice and a combination of adaptive genetic operators to improve the genetic algorithm. The experimental results verify the effectiveness of the method.
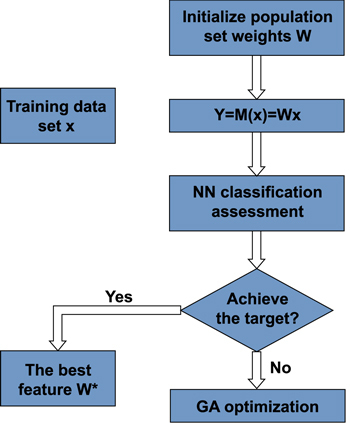
Figure 35. Feature optimization method based on the genetic algorithm. Reproduced with permission from [ 149 ].
Figures 36 (a)–(d) are microscopic images of surface cracks on a steel plate taken by adjusting the focal plane of the microscope lens. The results are shown in figure 36 (e). The algorithm is used to achieve microimage fusion, and an approximately full focal image is obtained. It can reflect the sample surface information clearly and eliminate the effect of depth of field. Then the depth information can be obtained through the focal length of the image, and the three-dimensional reconstruction of the two-dimensional image can be realized. Finally, the length, width, and depth information of the workpiece crack can be measured.
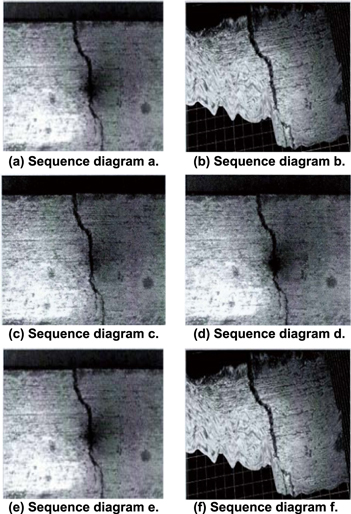
Figure 36. Image fusion result of the surface crack on the steel plate. Reproduced with permission from [ 150 ].
3.4.4. Knowledge system method
The knowledge system method uses the knowledge of some objective objects and characterizes this knowledge through attributes. If the attribute characteristics of a set of objects can be given, the set of objects represents a kind of complete knowledge [ 151 ]. Fisch et al [ 152 ] took process safety management and ontology as the core to build a modeling framework for a knowledge system and divides the structural elements of the knowledge system in the application domain into demand ontology, concept ontology, method ontology, control knowledge, and interpretation knowledge. The method of [ 153 ] also incorporates the concepts of ontology and ontology into multiagent modeling. It discusses the knowledge ontology structure maintenance and management mechanism and system and system modeling for exploring knowledge integration in an effective way. Based on the knowledge system of multisensor data fusion target recognition, algorithm research provides a clear and effective way of thinking.
4. Future trends
At present, the multifunctional sensor has become an emerging research area, and researchers are trying to design a single unit of multifunctional sensor that is able to provide multiple measurements. Furthermore, the utilization of the multifunctional sensor is beneficial as it can occupy less space and reduce the cost, although it has reliability issues. Multifunctional sensors have been increasingly used in a variety of applications ranging from environmental, biomedical, mechanical, electrical fields, etc. Some significant work has been undertaken in multifunctional sensors in material science as well as in electrical engineering.
Data fusion technology, together with multisensory or multifunctional sensor technology, is ascending. Almost all information processing methods can be applied to a data fusion system. With the rapid development of modern technology, such as sensor technology, data processing, computers, network communication, artificial intelligence, parallel computing software and hardware, etc, new data fusion methods will be demanding and emerging and will become the core technology for future industrial complex multisensor data fusion systems for intelligent detection and data processing. The application field will continue to expand. Multisensor data fusion is not a single technology but an interdisciplinary comprehensive theory and method. This is a new research field, still in the process of constant change and development.
However, there is still no unified fusion theory and effective generalized fusion model and algorithm for data fusion. The research on specific methods of data fusion is still in the preliminary stage. The problem of fault tolerance or robustness in a fusion system has not been solved. The ambiguity of association is the main obstacle in data fusion. There are still many practical problems in the design of a data fusion system.
In order to ensure the reliability of a multisensor process monitoring system and the data fusion results, unified fusion theory, data fusion architecture, and a generalized fusion model should be proposed. Related problems should be solved, such as data registration, data preprocessing, database construction, database management, human-machine interface, general software package development, etc, and a data fusion system oriented to specific application requirements with mature auxiliary technology should also be established. Artificial intelligence technologies, such as neural network, genetic algorithm, fuzzy theory, and expert theory, will be introduced into the field of data fusion. Integrated computational intelligence (e.g. fuzzy logic + neural network, genetic algorithm + fuzzy + neural network, etc) will be used to improve the performance of multisensor fusion. The expression and reasoning calculus of uncertain factors will be solved, e.g. introducing the concept of a gray number. Relevant prior data are used to improve the performance of data fusion, and more advanced and complex fusion algorithms (in unknown and dynamic environments, research on multisensor integration and fusion methods with parallel computer structure) should be studied. Under the application background of multiplatform/single platform and heterogeneous/homogeneous multisensors, the data processing model and algorithm with low computational complexity can meet the requirements of the task. A data fusion testing and evaluation platform and multisensor management system need to be established. The existing fusion methods should be standardized, and processing hardware should be developed to provide multiple complex fusion algorithms to complete the fusion in real time while data are acquired.
5. Conclusions
This paper presented an overview of multisensor process monitoring systems and data fusion technology. Generally, single sensors can only obtain part of the information segment of the environment. Integrated and fused multisensor information has the following four characteristics: abundant, complementary, real time, and low cost. Multisensor information fusion technology has prospects for broad application in the fields of industrial robotics, aerospace, military, multitarget tracking, numerical control technology, and intelligent manufacturing. In this investigation on multisensor systems, several applications of multisensor systems in different research fields were identified. Furthermore, with the development of modern technology, such as sensors, big data and cloud processing, computer software and hardware, and artificial intelligence, multisensor data fusion and target recognition will become the focus of future target recognition research. On the premise of introducing the target recognition principle of multisource data fusion and the theoretical basis of its algorithm, the target recognition algorithm of multisensor data fusion was summarized in detail from the perspective of algorithm concept classification, which provides a certain theoretical basis for the next research on target recognition of multisensor data fusion.
Acknowledgments
The authors would like to express their sincere thanks for the financial support from Shanghai Science and Technology Committee Innovation Grand (Grant Nos. 19ZR1404600, 17JC1400601), National Key R&D Program of China (Project Nos. 2017YFA0701200, 2016YFF0102003), and Science Challenging Program of CAEP (Grant No. JCKY2016212A506-0106).
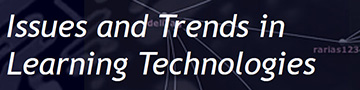
Login | Register
- Editorial Team
An In-Depth Literature Review of E-Portfolio Implementation in Higher Education: Processes, Barriers, and Strategies
Authors: Hongyan Yang (The University of Tennessee, Knoxville) , Rachel Wong (The University of Tennessee, Knoxville)
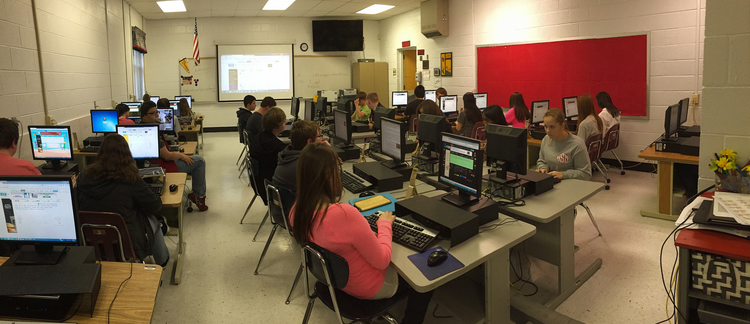
Literature Review
- Harvard Citation Style
- Vancouver Citation Style
- APA Citation Style
- Download RIS
- Download BibTeX
This is an accepted article with a DOI pre-assigned that is not yet published.
This literature review examines the implementation of e-portfolios in higher education, with a focus on the implementation process, potential barriers, and strategies for overcoming challenges. This review seeks to provide instructional designers and higher education instructors with design strategies to effectively implement e-portfolios. Through an analysis of seventeen studies, we identified six common steps in the implementation process, including identifying a purpose, stakeholders, and platform, conducting workshops, creating e-portfolios, and evaluating the project. The implementation process also raised eight concerns, including concerns related to technology, policy, pedagogy, artifact quality, privacy, student motivation, academic integrity, and teacher workload. To address these concerns, existing strategies suggest that successful implementation requires training and policy support, student-centered pedagogy, criteria for assessing artifacts, privacy and data protection, feedback, anti-plagiarism measures, and shared successful models.
Keywords: literature review, e-Portfolio, implementation, higher education
Accepted on 20 Apr 2024
Peer reviewed, creative commons attribution-noncommercial-sharealike 4.0, harvard-style citation.
Yang, H & Wong, R. () 'An In-Depth Literature Review of E-Portfolio Implementation in Higher Education: Processes, Barriers, and Strategies', Issues and Trends in Learning Technologies . doi: 10.2458/itlt.5809
Show: Vancouver Citation Style | APA Citation Style
Vancouver-Style Citation
Yang, H & Wong, R. An In-Depth Literature Review of E-Portfolio Implementation in Higher Education: Processes, Barriers, and Strategies. Issues and Trends in Learning Technologies. ; doi: 10.2458/itlt.5809
Show: Harvard Citation Style | APA Citation Style
APA-Style Citation
Yang, H & Wong, R. (, ). An In-Depth Literature Review of E-Portfolio Implementation in Higher Education: Processes, Barriers, and Strategies. Issues and Trends in Learning Technologies doi: 10.2458/itlt.5809
Show: Harvard Citation Style | {% trans 'Vancouver Citation Style' %}
Non Specialist Summary
This article has no summary
- Open access
- Published: 01 May 2024
Hospital performance evaluation indicators: a scoping review
- Shirin Alsadat Hadian ORCID: orcid.org/0000-0002-1443-1990 1 ,
- Reza Rezayatmand ORCID: orcid.org/0000-0002-9907-3597 2 ,
- Nasrin Shaarbafchizadeh ORCID: orcid.org/0000-0001-7104-2214 3 ,
- Saeedeh Ketabi ORCID: orcid.org/0000-0002-6778-5645 4 &
- Ahmad Reza Pourghaderi ORCID: orcid.org/0000-0003-2682-2160 5
BMC Health Services Research volume 24 , Article number: 561 ( 2024 ) Cite this article
356 Accesses
Metrics details
Hospitals are the biggest consumers of health system budgets and hence measuring hospital performance by quantitative or qualitative accessible and reliable indicators is crucial. This review aimed to categorize and present a set of indicators for evaluating overall hospital performance.
We conducted a literature search across three databases, i.e., PubMed, Scopus, and Web of Science, using possible keyword combinations. We included studies that explored hospital performance evaluation indicators from different dimensions.
We included 91 English language studies published in the past 10 years. In total, 1161 indicators were extracted from the included studies. We classified the extracted indicators into 3 categories, 14 subcategories, 21 performance dimensions, and 110 main indicators. Finally, we presented a comprehensive set of indicators with regard to different performance dimensions and classified them based on what they indicate in the production process, i.e., input, process, output, outcome and impact.
The findings provide a comprehensive set of indicators at different levels that can be used for hospital performance evaluation. Future studies can be conducted to validate and apply these indicators in different contexts. It seems that, depending on the specific conditions of each country, an appropriate set of indicators can be selected from this comprehensive list of indicators for use in the performance evaluation of hospitals in different settings.
Peer Review reports
Healthcare is complex [ 1 ] and a key sector [ 2 ] that is now globally faced with problems of rising costs, lack of service efficiency, competition, and equity as well as responsiveness to users [ 3 ]. One estimate by the WHO has shown a yearly waste of approximately 20–40% of total healthcare resources because of inefficiency [ 4 ]. European countries have spent on average 9.6% of their gross domestic product (GDP) on healthcare in 2017 and 9.92% in 2019. Germany, France, and Sweden reported the highest healthcare expenditures in Europe in 2018 (between 10.9% and 11.5% of GDP) [ 5 ]. In the U.S., healthcare spending consumes 18% of the GDP, which is likely to eclipse $6 trillion by 2027 [ 6 ].
Hospitals, as the biggest consumers of health system budgets [ 7 ], are the major part of the health system [ 8 ]. In many countries 50–80% of the health sector budget is dedicated to hospitals [ 8 , 9 ]. As a result, hospital performance analysis is becoming a routine task for every hospital manager. On the one hand, hospital managers worldwide are faced with difficult decisions regarding cost reduction, increasing service efficiency, and equity [ 10 ]. On the other hand, measuring hospital efficiency is an issue of interest among researchers because patients demand high-quality care at lower expenses [ 11 ].
To address the above mentioned need to measure hospital performance, implementing an appropriate hospital performance evaluation system is crucial in any hospital. In doing so, hospital administrators use various tools to analyse and monitor hospital activities [ 1 ], which need well-defined objectives, standards and quantitative indicators [ 12 ]. The latter are used to evaluate care provided to patients both quantitatively and qualitatively and are often related to input, output, processes, and outcomes. These indicators can be used for continuous quality improvement by monitoring, benchmarking, and prioritizing activities [ 13 ]. These parameters are developed to improve health outcomes and to provide comparative information for monitoring and managing and formulating policy objectives within and across health services [ 12 ]. Studies thus far have used their own set of indicators while evaluating hospital performance, which could be context dependent. In addition, those studies have mostly used a limited set of indicators that focus on few dimensions (2–6 dimensions) of hospital performance [ 14 , 15 , 16 , 17 , 18 ].
Therefore, comprehensive knowledge of potential indicators that can be used for hospital performance evaluation is necessary. It would help choose appropriate indicators when evaluating hospital performance in different contexts. It would also help researchers extend the range of analysis to evaluate performance from a wider perspective by considering more dimensions of performance. Although performance is a very commonly used term, it has several definitions [ 19 , 20 ], yet, it is often misunderstood [ 21 ]. Therefore, some researchers have expressed confusion about the related terms and considered them interchangeable. These terms are effectiveness, efficiency, productivity, quality, flexibility, creativity, sustainability, evaluation, and piloting [ 21 , 22 , 23 ]. Thus, this scoping review aimed to categorize and present a comprehensive set of indicators that can be used as a suitable set for hospital performance evaluation at any needed level of analysis, i.e., clinical, para-clinical, logistical, or departmental, and relate those indicators to the appropriate performance dimensions. The uniqueness of this paper is that it provides its readers with a comprehensive collection of indicators that have been used in different performance analysis studies.
Materials and methods
We conducted a scoping review of a body of literature. The scoping review can be of particular use when the topic has not yet been extensively reviewed or has a complex or heterogeneous nature. This type of review is commonly undertaken to examine the extent, range, and nature of research activity in a topic area; determine the value and potential scope and cost of undertaking a full systematic review; summarize and disseminate research findings; and identify research gaps in the existing literature. As a scoping review provides a rigorous and transparent method for mapping areas of research, it can be used as a standalone project or as a preliminary step to a systematic review [ 24 ]. While a systematic review (qualitative or quantitative) usually addresses a narrow topic/scope and is a method for integrating or comparing findings from previous studies [ 25 ].
In our study, we used the Preferred Reporting Items for Systematic reviews and Meta-Analyses extension for Scoping Reviews (PRISMA-ScR) Checklist following the methods outlined by Arksey and O’Malley [ 26 ] and Tricco [ 27 ]. A systematic search for published and English-language literature on hospital performance evaluation models was conducted, using three databases, i.e., PubMed, Scopus, and Web of Science, from 2013 to January 2023. Initially, the identified keywords were refined and validated by a team of experts. Then, a combination of vocabularies was identified by the authors through a brainstorming process. The search strategy was formulated using Boolean operators. The title and abstract of the formulas were searched in the online databases. The search query for each database is presented in Table 1 .
In the screening process, relevant references related to hospital performance evaluation were screened and abstracted into researcher-developed Microsoft® Excel forms by dual independent reviewers and conflicting information was provided by other reviewers.
The inclusion criteria were as follows: focused only on the hospital setting, available full text and written in English. We excluded studies that focused on health organization indicators, not specifically on hospital indicators; articles without appropriate data (only focused on models and not indicators; or qualitative checklist questionnaires); and articles that focused only on clinical or disease-related indicators, not hospital performance dimensions, and provided very general items as indicators, not the domains of the indicators themselves. Then, a PRISMA-ScR Checklist was used to improve transparency in our review [ 28 ].
To extract the data, researcher-developed Microsoft® Excel forms (data tables) were designed. The following data were subsequently extracted into Microsoft®Excel for synthesis and evaluation: title, author, article year, country, indicator category, study environment (number of hospitals studied), study time frame, indicator name, number of indicators, indicator level (hospital level, department level), evaluation perspective (performance, productivity, efficiency, effectiveness, quality, cost, safety, satisfaction, etc. ) , study type (quantitative or qualitative), indicator subtype (input (structure), process, output (result), outcome and impact), and other explanations. To create a descriptive summary of the results that address the objectives of this scoping review, numerical summarization was also used.
The purpose of creating the main category and the evaluation perspective section was to develop them and create new categories, which focused on the type of indicators related to the performance term. For example, in the “Category” section, the names of the departments or wards of the hospital (such as hospital laboratories, pharmacies, clinical departments, and warehouses) and in the “Evaluation perspective” section, various terms related to the evaluation of hospital performance were extracted. These two types were used after extracting their information under the title “performance dimension”.
The indicators’ levels were collected to determine the level of performance evaluation with the relevant index. Some indicators were used to evaluate the performance of the entire hospital, some were used to evaluate the performance of hospital departments, and some were used to evaluate the performance at the level of a specific project. For example, several indicators (such as bed occupancy ratio, length of stay, and waiting time) were used to evaluate the performance of the entire hospital, and other indicators (such as laboratory department indicators, energy consumption indicators, and neonatal department indicators) were used only to measure the performance of specific departments. This sections were used under the title “category”. The “category” and “indicator’s name” sections were defined according to the results of the “subcategory” section.
The subtypes of indicators (input (structure), process, output(result), outcome and impact) were defined based on the chain model, and each of the selected indicators was linked to it (Appendix 1 ). As a result of the chain model, inputs were used to carry out activities, activities led to the delivery of services or products (outputs). The outputs started to bring about change (outcomes), and eventually, this (hopefully) contributed to the impact [ 29 ]. The classification of the set of input, process, output, outcome and impact indicators was such that readers could access these categories if necessary according to their chosen evaluation models. The term was used under the title “Indicators by types”.
The type of study was considered quantitative or qualitative for determining whether an indicator was able to perform calculations. In this way, readers can choose articles that use quantitative or qualitative indicators to evaluate hospital performance.
We included 91 full-text studies (out of 7475) in English published between 2013 and January 2023 (Fig. 1 ), approximately 40% of which were published between 2020 and 2023. More than 20% of the retrieved studies were conducted in Iran and USA.
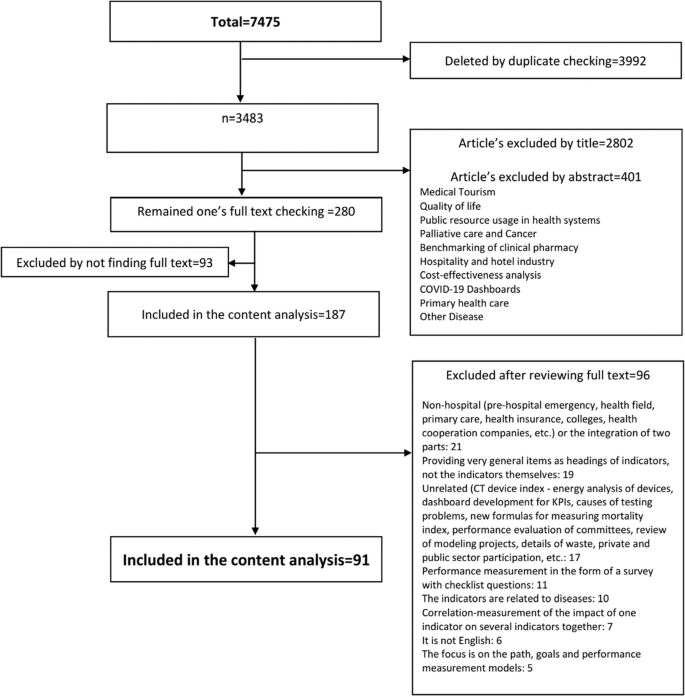
Study selection and data abstraction
Study characteristic
As shown in Table 2 , in 85% of the reviewed studies, a number of hospitals (1 to 3828 hospitals, 13,221 hospitals in total) were evaluated. More than 90% of the studies used a quantitative approach. In more than 70% of the studies, hospital evaluation occurred at the department level, which can also be divided into three levels: administrative, clinical ward, and paramedical department. In addition, the administrative departments consist of 13 departments, including financial management [ 48 , 55 , 61 , 67 , 68 , 80 , 83 , 109 , 113 ], supply chain management and warehouse [ 15 , 43 , 84 ], value-based purchasing [ 33 , 85 ], human resource management [ 97 , 101 ], medical equipment [ 32 , 87 ], health information management department [ 90 ], information systems [ 106 ], nutritional assessment [ 93 ], energy management [ 30 , 45 , 92 ], facility management [ 52 , 53 ], building sustainability and resilience [ 35 ], research activities [ 44 ], and education [ 107 ].
The clinical wards consisted of 8 wards, namely, emergency departments (EDs) [ 16 , 39 , 56 , 57 , 69 , 70 , 89 ], surgery departments [ 58 , 62 , 63 , 91 , 102 ], intensive care units (ICUs) [ 47 , 64 , 65 ], operating rooms (ORs) [ 38 , 88 , 108 ], surgical intensive care units (SICUs) [ 111 ], obstetrics and gynecology department [ 59 ], neonatal intensive care units (NICUs) [ 74 , 103 ] and quality of care [ 18 , 31 , 40 , 50 , 72 , 92 , 95 , 112 ] indicators. The paramedical departments consisted of 3 departments, pharmacy [ 60 , 76 , 98 ], laboratory and blood bank [ 37 , 42 , 43 , 49 ], and outpatient assessment [ 86 ] indicators.
With regard to data categorization, firstly, a total of 1204 indicators in 91 studies were extracted and after detailed examination, 43 indices (such as hospital ownership, level of care, admission process, and personal discipline) were removed due to their generality and impossibility of calculation in the hospital environment. Then, 1161 performance indicators were entered in this research and were categorized based on the performance criteria (more details about the indicators can be found in Appendix 1 ). Secondly, 145 functional dimensions, including divisions based on different departments and units of the hospital, were defined according to several focus group discussions with 5 health experts. Then, re-categorization and functional summarization were performed, after which 21 performance dimensions were finalized.
As shown in Table 4 , the 21 performance dimensions were divided into three parts: category, subcategory, and related indicators. Additionally, according to the hospital levels, there were three categories: ‘organizational management’, ‘clinical management’, and ‘administrative management’. Then, according to the type of indicators, fifteen subcategories were defined for the 110 selected main indicators.
Performance dimensions
The ‘productivity’ dimension focuses on indicators reflecting the macro-performance of the hospital, considering that this index is more effective and efficient. The ‘efficiency’ dimension focuses on general performance indicators for the optimal use of resources to create optimal output in the hospital. The ‘effectiveness’ dimension is a general performance indicator with an outcome view. The ‘speed’ dimension focuses on the indicators that show attention to the service delivery time and the speed of the procedures. The ‘development’ dimension focuses on matters related to employees’ and students’ training and related training courses. In terms of ‘safety’ dimension, there were issues related to patient safety, unwanted and harmful events, and hospital infections.
The “quality of work life” dimension emphasizes matters related to personnel volume and work conditions. The ‘quality’ dimension is related to the quality of service provided in different parts of the hospital and possible complications in improving the quality of services. The ‘satisfaction’ dimension focuses on the satisfaction of patients, employees, and their complaints. The ‘innovation’ dimension relates to the research process and its output. The ‘appropriateness’ dimension involves proper service from clinical departments, pharmaceutical services, and patient treatment. The ‘evaluation’ dimension focuses on the indicators related to the assessment scores of the para-clinical departments of the hospital.
The ‘profitability’ dimension focuses on the overall output indicators for income and profitability. The ‘cost’ dimension focuses on indicators related to general expenditures and the average cost per bed and patient and budgeting. The ‘economy’ dimension is related to financial rates and their indicators. The ‘coherence’ dimension emphasizes the indicators related to the continuity of the service delivery process. The ‘patient-centeredness’ dimension focuses on the indicators related to the patient’s experience of the facility, environment, treatment processes, communications, and relevant support for the patient. The ‘equity’ dimension studies indicators related to social and financial justice and life expectancy. The ‘relationship’ dimension evaluates the process of consultations and discussions required during the patients’ care provided by the treatment team. The ‘sustainability’ dimension focuses on indicators related to energy standards. The ‘flexibility’ dimension focuses on the hospital’s response to the crisis.
According to Table 4 , most studies focused on ‘efficiency’, ‘productivity’, ‘safety’ and ‘effectiveness’ as performance dimensions in 54, 53, 38 and 37 studies, respectively (40–70% of studies). In the ‘efficiency’ subcategory, resource management, supportive unit assessment, and human resource management indicators were the first to third most common indicators used in 26, 23 and 22 studies, respectively (approximately 25% of the studies).
In addition, for the ‘efficiency’ dimension, ‘medical staff numbers’, ‘emergency department bed numbers’, and ‘nonmedical staff numbers’ were reported in 16, 13, and 11 studies, respectively (between 20 and 30% of the studies). For the ‘productivity’ subcategory, ‘bed utilization rate’ and ‘service delivery and treatment’ were reported in 50% and 20% of the studies, respectively (46 and 19 out of 91).
Additionally, for the ‘productivity’ dimension, the ‘length of stay’ indicator was used more than others and reported in approximately 80% of the studies (43 out of 53), followed by the ‘bed occupancy rate’ in approximately 40% of the studies (21 out of 53). The ‘bed turnover ratio’ and ‘hospitalization rate’ were also reported in 12 studies. Furthermore, for ‘safety’ dimensions, all indicators were in the ‘patient safety’ subcategory, which has been reported in 38 studies, and ‘complications’, ‘accidents or adverse events’, and ‘incidents or errors rates’ were the most concentrated indicators by researchers in 13, 12, and 11 studies, respectively. The performance dimension of ‘effectiveness’ was presented in 37 studies (40%), with only two indicators, ‘mortality rate’ in 29 studies and ‘readmission rate’ in 23 studies.
Performance categories
Considering the three categories shown in Table 4 , ‘organizational management’ indicators were more commonly used among the other two categories (‘clinical’ and ‘administrative’) and were present in more than 85% of the studies (78 out of 91). Two categories, ‘clinical management’ and ‘administrative management’, were reported in 62 and 51 studies, respectively.
Performance subcategories
Considering the 14 subcategories shown in Table 4 , both the ‘bed utilization rate’ and ‘patient safety’ indicators were mentioned in 46 studies and were more common among the other subcategories. The second most common indicator of the ‘financial management’ subcategory was reported in 38 studies. At the third level, both the ‘human resource management’ and ‘time management’ indicators were presented in 31 studies. The ‘paramedical’ subcategory indicators were presented in less than 10% of the studies [ 60 , 96 , 97 , 98 , 106 , 113 ].
Performance indicators
According to the indicator columns in Table 3 , the most used indicators in reviewed studies were the length of stay, mortality rate, and readmission rate in 47%, 32%, and 25% of studies, respectively. Bed occupancy rate and non-personnel costs were reported in 23% of studies. Additionally, among the 110 indicators, 16 indicators, namely, the lab cancellation rate, exam-physician ratios, number of coded diagnoses, number of medical records, laboratory sample/report intervals, medical information request time, safety standards in the archives, nutritional risk screening, imaging quality control failures, errors in medical reports, average impact factor, nutritional measures, laboratory scoring, imaging inspection, discharge process and emergency response rate, were reported in less than 1% of the studies.
The classification of the indicators in Table 4 was performed based on the chain model, which included the input, process, output, outcome and impact. The assignment of the indicators to each category was performed according to the experts’ opinions. For instance, the number of publications by academic member of an academic hospital and the average impact factor of those publications were considered outcome indicators. As depicted in the Table 4 , most studies (80%) focused more on output indicators. Additionally, fifteen studies focused on introducing and extracting some of the input, process, output, outcome and impact indicators; among those, only one study [ 96 ] has examined the input, process, output and impact indicators simultaneously.
Additionally, in approximately 42% (36 out of 91) of the studies, the indicators’ definitions, formulas, or descriptions have been illustrated, while less than 10% of the studies have defined measuring units, standard or benchmark units for all studied indicators [ 15 , 43 , 45 , 51 , 52 , 57 , 67 ].
Overall, nine studies related to hospital performance evaluation were conducted using systematic review methodologies (five systematic reviews [ 16 , 29 , 30 , 56 , 113 ], two literature reviews [ 79 , 80 ], one narrative review [ 98 ] and one brief review [ 92 ]). Most of these studies focused on extracting performance indicators from one or more hospital departments (e.g., the emergency department) [ 16 , 56 ], hospital laboratory and radiology information systems [ 106 ], supply chain performance [ 29 ], resources and financial results and activity [ 113 ], hospital water consumption [ 30 ], and the pharmaceutical sector [ 98 ]. Other reviews included a three-step process to review, evaluate and rank these hospital indicators in a systematic approach [ 16 ], or to evaluate performance indicator models to create an interactive network and visualize the causal relationships between performance indicators [ 79 ]; moreover, some have focused on the importance of indicators to ensure adequate coverage of the relevant areas of health care services to be evaluated [ 92 ].
Only one scoping review aimed to identify current assessments of hospital performance and compared quality measures from each method in the context of the six qualitative domains of STEEEP (safety, timeliness, effectiveness, efficiency, equity, and patient-centeredness) of the Institute of Medicine (IOM) in accordance with Donabedian’s framework and formulating policy recommendations [ 115 ].
In addition, 21 studies divided performance indicators into 2 to 6 dimensions of performance. Also, the reviewed studies included 2–40 indicators in zero [ 29 , 30 , 98 ] to 6 domains [ 34 ]. Moreover, none of the studies have tried to comprehensively summarize and categorize the performance indicators in several categories, focusing on all the indicators reflecting the performance of the entire hospital organization, or the indicators of administrative units or clinical departments.
In this scoping review, a unique set of hospital performance evaluation indicators related to the various performance dimensions was categorized from 91 studies over the past ten years.
Similarly, in a study, 19 performance dimensions, 32 sub-dimensions, and 138 indicators were extracted from only six studies. Those dimensions were described by all studies included in the review, but only three studies specified the relevant indicators, and the list provided for all possible indicators was not comprehensive. Also, despite current review, there was no classification of indicators based on the hospital levels: managerial, clinical, or organizational levels [ 116 ]. Another study has similarly investigated the performance evaluation indicators of the hospital in such a way that among 42 studies, 111 indicators were presented in the four categories: input, output, outcome, and impact. But, there was no classification of indicators based on performance dimensions and hospital levels [ 117 ].
In this study, the importance of categorized indicators, for the first time to our knowledge, was determined based on their frequency of use in the published literature (Appendix 2 ). The ‘Organizational management’ indicators were the most common compared with the other two categories (‘clinical’ and ‘administrative’). It could be because of the fact that the indicators such as ‘bed occupancy rate’, ‘average length of stay’, ‘mortality rate’, ‘hospital infection rate’, and ‘patient safety’ are easier to be registered in hospital software compared to other indicators, and also they better reflect the overall performance of hospital. Thus, researchers are more interested in using these indicators.
Considering 14 subcategories, indicators related to three subcategories i.e. bed utilization, patient safety and financial management are the most frequent used indicators for hospital performance evaluation. It reflects the need of hospital managers to increase the profitability of hospital in one hand, and to control cost on the other hand. As a results, researchers have paid special attention to ‘cost income’, ‘profitability’, ‘economic’, etc., as indicators for evaluating hospital performance.
When considering indicators by type, more studies have focused on output indicators, while input indicators were the least common used. This might be because of the fact that at hospital level, it is difficult for managers to change those inputs such as ‘beds’, ‘human resources’, ‘equipment and facilities’. In addition, due to the complexity of interdepartmental relationships in hospitals, process indicators seemed to provide more variety for analysis than input indicators, so they were more often used. As mentioned above, output indicators were the most used indicators for hospital performance evaluation due to their ease of calculation and interpretation.
The main purpose of this paper was to identify a comprehensive set of indicators that can be used to evaluate hospital performance in various hospital settings by being distilled into a smaller and more related set of indicators for every hospital or department setting. future studies could be designed to validate each set of indicators in any specific context. In addition, they could investigate the relationship between the indicators and their outcomes of interest and the performance dimension each could address. This will enable hospital managers to build their own set of indicators for performance evaluation both at organization or at department level. Also it should be mentioned that.
Although some previous studies have provided definitions for each indicator and determined the standard criteria for them, this was not done in this study because the focus of this study was to provide a collection of all the indicators used in hospital performance evaluation, which resulted in the identification of more than a thousand indicators without limiting to specific country or context. So while preparing a smaller set of indicators, specific conditions of each country, such as the type of health system and its policy, the type of financing system, and the structure of services, should be taken into account to select appropriate indicators.
In addition, although it is important to examine the scope of each article to compare the list of indicators and the relationships between the dimensions of the hospital in terms of size and type and between the number and type of selected indicators, this was considered beyond the scope of this review due to the high number of indicators, which made the abovementioned investigations impossible. Future studies could do that while working with a smaller set of indicators.
This review aimed to categorize and present a comprehensive set of indicators for evaluating overall hospital performance in a systematic way. 1161 hospital performance indicators were drawn from 91 studies over the past ten years. They then were summarized into 110 main indicators, and categorized into three categories: 14 subcategories, and 21 performance dimensions This scoping review also highlighted the most frequent used indicators in performance evaluation studies which could reflect their importance for that purpose. The results of this review help hospital managers to build their own set of indicators for performance evaluation both at organization or at department level with regard to various performance dimensions.
As the results of this review was not limited to any specific country or context, specific conditions of each country, such as the type of health system and its policy, the type of financing system, and the structure of services, should be taken into account while selecting appropriate indicators as a smaller set of indicators for hospital performance evaluation in specific context.
Availability of data and materials
The datasets used and/or analyzed during the current study are available from the corresponding author on reasonable request.
Abbreviations
Gross domestic product
Preferred Reporting Items for Systematic reviews and Meta-Analyses extension for Scoping Reviews
Emergency departments
Intensive care unit
Operating room
Surgical intensive care unit
Neonatal intensive care unit
Readmission rate
Quality Control
Medication use evaluation
safety, timeliness, effectiveness, efficiency, equity, and patient-centeredness
Institute of Medicine
Abdullah A, Ahmad S, Athar MA, Rajpoot N, Talib F. Healthcare performance management using integrated FUCOM-MARCOS approach: the case of India. Int J Health Plann Manage. 2022;37(5):2635–68.
Article PubMed Google Scholar
Pestana M, Pereira R, Moro S. Improving health care management in hospitals through a productivity dashboard. J Med Syst. 2020;44(4):87.
Amos D. A practical framework for performance measurement of facilities management services in developing countries’ public hospitals. J Facil Manag. 2022;20(5):713–31.
Article Google Scholar
Ahmed S, Hasan MZ, MacLennan M, Dorin F, Ahmed MW, Hasan MM, et al. Measuring the efficiency of health systems in Asia: a data envelopment analysis. BMJ Open. 2019;9(3):e022155.
Article PubMed PubMed Central Google Scholar
Mitkova Z, Doneva M, Gerasimov N, Tachkov K, Dimitrova M, Kamusheva M, et al. Analysis of healthcare expenditures in Bulgaria. Healthc. 2022;10(2):274.
Patrinely JR, Walker SH, Glassman GE, Davis MJ, Abu-Ghname A, Khan U, et al. The importance of financial metrics in physician funding and performance evaluation. Plast Reconstr Surg. 2021;147:1213–8.
Article CAS PubMed Google Scholar
Buathong S, Bangchokdee S. The use of the performance measures in Thai public hospitals. ASIAN Rev Acc. 2017;25(4):472–85.
Google Scholar
Imani A, Alibabayee R, Golestani M, Dalal K. Key indicators affecting hospital efficiency: a systematic review. Front Public Heal. 2022;10:830102.
Mahdiyan S, Dehghani A, Tafti AD, Pakdaman M, Askari R. Hospitals’ efficiency in Iran: a systematic review and meta-analysis. J Educ Health Promot. 2019;8(1):126.
PubMed PubMed Central Google Scholar
Amos D, Musa ZN, Au-Yong CP. Performance measurement of facilities management services in Ghana’s public hospitals. Build Res Inf. 2020;48(2):218–38.
Feibert DC, Andersen B, Jacobsen P. Benchmarking healthcare logistics processes–a comparative case study of Danish and US hospitals. Total Qual Manag Bus Excell. 2019;30(1–2):108–34.
Gün I, Yilmaz F, Şenel IK. Efficiency analysis of health systems in world bank countries. Arch Heal Sci Res. 2021;8(2):147–52.
Breyer JZ, Giacomazzi J, Kuhmmer R, Lima KM, Hammes LS, Ribeiro RA, et al. Hospital quality indicators: a systematic review. Int J Health Care Qual Assur. 2019;32(2):474–87.
Regragui H, Sefiani N, Azzouzi H. Improving performance through measurement: the application of BSC and AHP in healthcare organization. In: Equipe De Recherche, Ingénierie, Innovation Et Management Des Systèmes Industriels, Université Abdelmalek Saadi. Tanger, Morocco: Institute of Electrical and Electronics Engineers Inc; 2018. p. 51–6.
Ghozali MT, Latifah DN, Darayani A. Analysis of Drug Supply Management of the Pharmacy Warehouse of Prof. Dr. Soerojo Mental Health Hospital, Magelang, Indonesia. Clin Schizophr Relat Psychoses. 2021;15:1–6.
Etu EE, Monplaisir L, Aguwa C, Arslanturk S, Masoud S, Markevych I, et al. Identifying indicators influencing emergency department performance during a medical surge: a consensus-based modified fuzzy Delphi approach. PLoS ONE. 2022;17(4 April):e0265101.
Article CAS PubMed PubMed Central Google Scholar
Lin C-Y, Shih F-C, Ho Y-H. Applying the balanced scorecard to build service performance measurements of medical institutions: An AHP-DEMATEL approach. Int J Environ Res Public Health. 2023;20(2):1022.
Backman C, Vanderloo S, Forster AJ. Measuring and improving quality in university hospitals in Canada: the collaborative for excellence in healthcare quality. Health Policy (New York). 2016;120(9):982–6.
Ghalem Â, Okar C, Chroqui R, Semma E. Performance: A concept to define. In: Performance: A concept to define. LOGISTIQUA 2016; 2016. p. 1–13.
Sonnentag S, Frese M. Performance Concepts and Performance Theory. In 2005. p. 1–25.
Tangen S. Demystifying productivity and performance. Int J Prod Perform Manag. 2005;54:34–46.
Elena-Iuliana I, Maria C. Organizational Performance – A Concept That Self-Seeks To Find Itself. Ann - Econ Ser Constantin Brancusi Univ Fac Econ. 2016;4(4):179–83.
Riratanaphong C, Van der Voordt T, Sarasoja A. Performance Measurement in the context of CREM and FM. In: Per Anker Jensen, Theo Van der Voordt CC, editor. The added value of facilities management: concepts, findings and perspectives. Lyngby Denmark: Polyteknisk Forlag; 2012. p. 1–21.
Pham M, Rajić A, Greig J, Sargeant J, Papadopoulos A, Mcewen S. A scoping review of scoping reviews: advancing the approach and enhancing the consistency. Res Synth Methods. 2014;5:371–85.
Chaney M. So you want to write a narrative review article? J Cardiothorac Vasc Anesth. 2021;35:3045–9.
Arksey H, O’Malley L. Scoping studies: towards a methodological framework. Int J Soc Res Methodol. 2005;8(1):19–32.
Tricco AC, Lillie E, Zarin W, O’Brien KK, Colquhoun H, Levac D, et al. PRISMA extension for scoping reviews (PRISMA-ScR): Checklist and explanation. Ann Intern Med. 2018;169(7):467–73.
Tricco A, Lillie E, Zarin W, O’Brien K, Colquhoun H, Levac D, et al. PRISMA extension for scoping reviews (PRISMA-ScR): Checklist and explanation. Ann Intern Med. 2018;169(7):467–73.
Dolatabad AH, Mahdiraji HA, Babgohari AZ, Garza-Reyes JA, Ai A. Analyzing the key performance indicators of circular supply chains by hybrid fuzzy cognitive mapping and Fuzzy DEMATEL: evidence from healthcare sector. Environ Dev Sustain. 2022;1–27.
Batista KJM, da Silva SR, Rabbani ERK, Zlatar T. Systematic review of indicators for the assessment of water consumption rates at hospitals. Water Supply. 2020;20(2):373–82.
Beta G, Role D, Berloviene D, Balkena Z. PATIENTS’ SATISFICATION AS THE QUALITY INDICATOR OF NURSING. In: Lubkina V, Kaupuzs A, Znotina D, editors. SOCIETY INTEGRATION EDUCATION, VOL VI: PUBLIC HEALTH AND SPORT, RESEARCHES IN ECONOMICS AND MANAGEMENT FOR SUSTAINABLE EDUCATION. 2020. p. 79–88.
Bhardwaj P, Joshi NK, Singh P, Suthar P, Joshi V, Jain YK, et al. Competence-based assessment of biomedical equipment management and maintenance system (e-Upkaran) using benefit evaluation framework. CUREUS J Med Sci. 2022;14(10):e30579.
Cheon O, Song M, Mccrea AM, Meier KJ. Health care in America: the relationship between subjective and objective assessments of hospitals. Int PUBLIC Manag J. 2021;24(5):596–622.
Craig KJT, McKillop MM, Huang HT, George J, Punwani ES, Rhee KB. US hospital performance methodologies: a scoping review to identify opportunities for crossing the quality chasm. BMC Health Serv Res. 2020;20(1):640.
Cristiano S, Ulgiati S, Gonella F. Systemic sustainability and resilience assessment of health systems, addressing global societal priorities: Learnings from a top nonprofit hospital in a bioclimatic building in Africa. Renew Sustain ENERGY Rev. 2021;141:110765.
Dadi D, Introna V, Santolamazza A, Salvio M, Martini C, Pastura T, et al. Private Hospital Energy Performance Benchmarking Using Energy Audit Data: An Italian Case Study. Energies. 2022;15(3):1–16.
Dawande PP, Wankhade RS, Akhtar FI, Noman O. Turnaround time: an efficacy measure for medical laboratories. CUREUS J Med Sci. 2022;14(9):e28824.
De Sousa LR, Mazzo A, De Almeida ACF, Tonello C, Lourençone LFM. Evaluation of quality indicators in the management of an operating room at a tertiary-level hospital. Med. 2022;55(1):1–8.
Drynda S, Schindler W, Slagman A, Pollmanns J, Horenkamp-Sonntag D, Schirrmeister W, et al. Evaluation of outcome relevance of quality indicators in the emergency department (ENQuIRE): study protocol for a prospective multicentre cohort study. BMJ Open. 2020;10(9):e038776.
Fekri O, Manukyan E, Klazinga N. Appropriateness, effectiveness and safety of care delivered in Canadian hospitals: a longitudinal assessment on the utility of publicly reported performance trend data between 2012–2013 and 2016–2017. BMJ Open. 2020;10(6):e035447.
Galloa AJO, Ramírez CA. Evaluating Colombian public hospitals productivity during 2004–2015. A luenberger-indicator approach. Rev Gerenc Y Polit Salud. 2020;19:1–23.
Gebreyes M, Sisay A, Tegen D, Asnake A, Wolde M. Evaluation of laboratory performance, associated factors and staff awareness towards achieving turnaround time in tertiary hospitals, Ethiopia. Ethiop J Health Sci. 2020;30(5):767–76.
Gnanaraj J, Kulkarni RG, Sahoo D, Abhishekh B. Assessment of the Key Performance Indicator Proposed by NABH in the Blood Centre of a Tertiary Health Care Hospital in Southern India. Indian J Hematol Blood Transfus. 2022;39:308–16.
Horenberg F, Lungu DA, Nuti S. Measuring research in the big data era: the evolution of performance measurement systems in the Italian teaching hospitals. Health Policy (New York). 2020;124(12):1387–94.
Hwang DK, Cho J, Moon J. Feasibility study on energy audit and data driven analysis procedure for building energy efficiency: bench-marking in Korean hospital buildings. Energies. 2019;14(15):3006.
Jaskova D. Efficiency of management, processes in a private hospital. Entrep Sustain Issues. 2021;9(1):436–46.
Jebraeily M, Valizadeh MA, Rahimi B, Saeidi S. The development of a clinical dashboard for monitoring of key performance indicators in ICU. J Iran Med Counc. 2022;5(2):308–17.
Kang Y, Kim M, Jung K. The equity of health care spending in South Korea: testing the impact of publicness. Int J Environ Res Public Health. 2020;17(5):1775.
Abou Tarieh RR, Zayyat R, Naoufal RN, Samaha HR. A case study exploring the impact of JCI standards implementation on staff productivity and motivation at the laboratory and blood bank. Heal Sci Rep. 2022;5(1):e497.
Kadoic N, Simic D, Mesaric J, Redep NB. Measuring quality of public hospitals in croatia using a multi-criteria Approach. Int J Environ Res Public Health. 2021;18:19.
Khalilabad T, Amir N, Asl P, Raeissi Shali M, Niknam N. Assessment of clinical and paraclinical departments of military hospitals based on the Pabon Lasso Model. J Educ Health Promot. 2020;9:1–6.
Lai JHK, Hou H, Edwards DJ, Yuen PL. An analytic network process model for hospital facilities management performance evaluation. Facilities. 2022;40(5–6):333–52.
Lai J, Yuen PL. Identification, classification and shortlisting of performance indicators for hospital facilities management. Facilities. 2021;39(1–2):4–18.
Lin CS, Chiu CM, Huang YC, Lang HC, Chen MS. Evaluating the operational efficiency and quality of Tertiary hospitals in Taiwan: the application of the EBITDA Indicator to the DEA Method and TOBIT Regression. Healthcare. 2022;10(1):58.
Matos R, Ferreira D, Pedro MI. Economic analysis of portuguese public hospitals through the construction of quality, efficiency, access, and financial related composite indicators. Soc Indic Res. 2021;157(1):361–92.
Morisod K, Luta X, Marti J, Spycher J, Malebranche M, Bodenmann P. Measuring health equity in emergency care using routinely collected data: a systematic review. Heal Equity. 2021;5(1):801–17.
Nik Hisamuddin R, Tuan Hairulnizam TK. Developing key performance indicators for emergency department of teaching hospitals: a mixed fuzzy Delphi and nominal group technique approach. Malays J Med Sci. 2022;29(2):114–25.
Ramírez Calazans A, Paredes Esteban RM, Grijalva Estrada OB, Ibarra Rodríguez MR. Assessment of quality indicators in pediatric major outpatient surgery. Influence of the COVID-19 pandemic. Cir Pediatr. 2023;36(1):17–21.
PubMed Google Scholar
Shaqura II, Gholami M, Akbari Sari A. Assessment of public hospitals performance in Gaza governorates using the Pabón Lasso Model. Int J Health Plann Manage. 2021;36(4):1223–35.
Al-Jazairi AS, Alnakhli AO. Quantifying clinical pharmacist activities in a tertiary care hospital using key performance indicators. Hosp Pharm. 2021;56(4):321–7.
Aloh HE, Onwujekwe OE, Aloh OG, Nweke CJ. Is bed turnover rate a good metric for hospital scale efficiency? A measure of resource utilization rate for hospitals in Southeast Nigeria. Cost Eff Resour Alloc. 2020;18(1):1–8.
Bari S, Incorvia J, Ahearn O, Dara L, Sharma S, Varallo J, et al. Building safe surgery knowledge and capacity in Cambodia: a mixed-methods evaluation of an innovative training and mentorship intervention. Glob Health Action. 2021;14(1):1998996.
Bari S, Incorvia J, Iverson KR, Bekele A, Garringer K, Ahearn O, et al. Surgical data strengthening in Ethiopia: results of a Kirkpatrick framework evaluation of a data quality intervention. Glob Health Action. 2021;14(1):1–11.
Bastos LSL, Hamacher S, Zampieri FG, Cavalcanti AB, Salluh JIF, Bozza FA. Structure and process associated with the efficiency of intensive care units in low-resource settings: an analysis of the CHECKLIST-ICU trial database. J Crit Care. 2020;59:118–23.
Bastos LSL, Wortel SA, de Keizer NF, Bakhshi-Raiez F, Salluh JIF, Dongelmans DA, et al. Comparing continuous versus categorical measures to assess and benchmark intensive care unit performance. J Crit Care. 2022;70:154063.
Kocisova K, Hass-Symotiuk M, Kludacz-Alessandri M. Use of the dea method to verify the performance model for hospitals. E M Ekon A Manag. 2018;21(4):125–40.
Lee D, Yu S, Yoon SN. Analysis of hospital management based on the characteristics of hospitals: focusing on financial indicators. Glob Bus Financ Rev. 2019;24(3):1–13.
Mirzaei A, Tabibi SJ, Nasiripour AA, Riahi L. Evaluating the feasibility of financial variables of health: A hospital administrator’s viewpoint. Galen Med J. 2016;5(1):25–30.
Middleton S, Gardner G, Gardner A, Considine J, FitzGerald G, Christofis L, et al. Are service and patient indicators different in the presence or absence of nurse practitioners? The EDPRAC cohort study of Australian emergency departments. BMJ Open. 2019;9(7):e024529.
Nobakht S, Jahangiri K, Hajinabi K. Correlation of performance indicators and productivity: A cross sectional study of emergency departments in Tehran, Iran during year 2016. Trauma Mon. 2018;23(5):1–6.
Nuti S, Grillo Ruggieri T, Podetti S. Do university hospitals perform better than general hospitals? A comparative analysis among Italian regions. BMJ Open. 2016;6(8):e011426.
Petrovic GM, Vukovic M, Vranes AJ. The impact of accreditation on health care quality in hospitals. Vojnosanit Pregl. 2018;75(8):803–8.
Pirani N, Zahiri M, Engali KA, Torabipour A. Hospital efficiency measurement before and after health sector evolution plan in Southwest of Iran: a DEA-panel data study. Acta Inf Med. 2018;26(2):106–10.
Profit J, Gould JB, Bennett M, Goldstein BA, Draper D, Phibbs CS, et al. The association of level of care with NICU quality. Pediatrics. 2016;137(3):44–51.
Rahimi H, Bahmaei J, Shojaei P, Kavosi Z, Khavasi M. Developing a strategy map to improve public hospitals performance with balanced scorecard and dematel approach. Shiraz E Med J. 2018;19(7):1–12.
Ahmed S, Hasan MZ, Laokri S, Jannat Z, Ahmed MW, Dorin F, et al. Technical efficiency of public district hospitals in Bangladesh: a data envelopment analysis. COST Eff Resour Alloc. 2019;17:17.
Rahman MH, Tumpa TJ, Ali SM, Paul SK. A grey approach to predicting healthcare performance. Meas J Int Meas Confed. 2019;134:307–25.
Sajadi HS, Sajadi ZS, Sajadi FA, Hadi M, Zahmatkesh M. The comparison of hospitals’ performance indicators before and after the Iran’s hospital care transformations plan. J Educ Health Promot. 2017;6:89.
Si S-L, You X-Y, Liu H-C, Huang J. Identifying key performance indicators for holistic hospital management with a modified DEMATEL approach. Int J Environ Res Public Health. 2017;14(8): 934.
Váchová L, Hajdíková T. Evaluation of Czech hospitals performance using MCDM methods. In: A SI, G WS, C D, editors. Department of exact methods, faculty of management, university of economics, Prague, Jarošovská 1117, Jindřichuv Hradec, vol. 37701. Czech Republic: Newswood Limited; 2017. p. 732–5.
Xenos P, Yfantopoulos J, Nektarios M, Polyzos N, Tinios P, Constantopoulos A. Efficiency and productivity assessment of public hospitals in Greece during the crisis period 2009–2012. Cost Eff Resour Alloc. 2017;15(1):6.
Zhang L, Liu R, Jiang S, Luo G, Liu H-C. Identification of key performance indicators for hospital management using an extended hesitant linguistic DEMATEL Approach. Healthc (Basel Switzerland). 2019;8(1):7.
Aksezer CS. A nonparametric approach for optimal reliability allocation in health services. Int J Qual Reliab Manag. 2016;33(2):284–94.
Cagliano AC, Grimaldi S, Rafele C. Assessing warehouse centralization and outsourcing in the healthcare sector: an Italian case study. In: Department of Management and Production Engineering, Politecnico Di Torino, Corso Duca Degli Abruzzi 24, Torino, 10129. Italy: AIDI - Italian Association of Industrial Operations Professors; 2017. p. 244–50.
Cefalu MS, Elliott MN, Setodji CM, Cleary PD, Hays RD. Hospital quality indicators are not unidimensional: a reanalysis of Lieberthal and Comer. Health Serv Res. 2019;54(2):502–8.
Gao H, Chen H, Feng J, Qin X, Wang X, Liang S, et al. Balanced scorecard-based performance evaluation of Chinese county hospitals in underdeveloped areas. J Int Med Res. 2018;46(5):1947–62.
Gonnelli V, Satta F, Frosini F, Iadanza E. Evidence-based approach to medical equipment maintenance monitoring. In: V HEO, V J, editors. University of Florence, Dept. of Information Engineering. Florence, Italy: Springer; 2017. p. 258–61.
Helkio P, Aantaa R, Virolainen P, Tuominen R. Productivity benchmarks for operative service units. ACTA Anaesthesiol Scand. 2016;60(4):450–6.
Khalifa M, Zabani I. Developing emergency room key performance indicators: What to measure and why should we measure it? J. M, A. H, P. G, A. K, M.S. H, editors. Vol. 226. King Faisal Specialist Hospital and Research Center, Jeddah, Saudi Arabia: IOS Press BV; 2016. p. 179–182.
Ajami S, Ebadsichani A, Tofighi S, Tavakoli N. Medical records department and balanced scorecard approach. J Educ Health Promot. 2013;2:7.
Bosse G, Mtatifikolo F, Abels W, Strosing C, Breuer J-P, Spies C. Immediate outcome indicators in perioperative care: a controlled intervention study on quality improvement in hospitals in Tanzania. PLoS One. 2013;8(6):e65428.
Hung K-Y, Jerng J-S. Time to have a paradigm shift in health care quality measurement. J Formos Med Assoc. 2014;113(10):673–9.
Jeejeebhoy KN, Keller H, Gramlich L, Allard JP, Laporte M, Duerksen DR, et al. Nutritional assessment: comparison of clinical assessment and objective variables for the prediction of length of hospital stay and readmission. Am J Clin Nutr. 2015;101(5):956–65.
Kittelsen SAC, Anthun KS, Goude F, Huitfeldt IMS, Häkkinen U, Kruse M, et al. Costs and quality at the hospital level in the nordic countries. Heal Econ (United Kingdom). 2015;24:140–63.
Koné Péfoyo AJ, Wodchis WP. Organizational performance impacting patient satisfaction in Ontario hospitals: a multilevel analysis. BMC Res Notes. 2013;6: 509.
Li CH, Yu CH. Performance evaluation of public non-profit hospitals using a BP Artificial neural network: the case of Hubei Province in China. Int J Environ Res Public Health. 2013;10(8):3619–33.
Liu K, Jain S, Shi J. Physician performance assessment using a composite quality index. Stat Med. 2013;32(15):2661–80.
Lloyd GF, Bajorek B, Barclay P, Goh S. Narrative review: Status of key performance indicators in contemporary hospital pharmacy practice. J Pharm Pract Res. 2015;45(4):396–403.
Mehrtak M, Yusefzadeh H, Jaafaripooyan E. Pabon Lasso and data envelopment analysis: a complementary approach to hospital performance measurement. Glob J Health Sci. 2014;6(4):107–16.
Mohammadi M, Ziapoor A, Mahboubi M, Faroukhi A, Amani N, Pour FH, et al. Performance evaluation of hospitals under supervision of Kermanshah medical sciences using pabonlasoty diagram of a five-year period (2008–2012). Life Sci J. 2014;11:77–81 ( 1 SPECL. ISSUE) ).
Niaksu O, Zaptorius J. Applying operational research and data mining to performance based medical personnel motivation system. In: Vilnius University, Institute of Mathematics and Informatics. Lithuania: IOS; 2014. p. 63–70.
Córdoba S, Caballero I, Navalón R, Martínez-Sánchez D, Martínez-Morán C, Borbujo J. Analysis of the surgical activity in the dermatology department of Fuenlabrada University Hospital, Madrid, Spain, between 2005 and 2010: determination of the standard operating times. Actas Dermosifiliogr. 2013;104(2):141–7.
Profit J, Kowalkowski MA, Zupancic JAF, Pietz K, Richardson P, Draper D, et al. Baby-MONITOR: a composite indicator of NICU Quality. Pediatrics. 2014;134(1):74–82.
Rabar D, Pap N. Evaluation of crotia’s regional hospital effiency: an application of data envelopment analysis . Bacher U, Barkovic D, Dernoscheg KH, LamzaMaronic M, Matic B, Runzheimer B, editors. Interdisciplinary Management Research IX. 2013;9:649–59.
Ramos MCA, da Cruz LP, Kishima VC, Pollara WM, de Lira ACO, Couttolenc BF. Performance evaluation of hospitals that provide care in the public health system, Brazil. Rev Saude Publica. 2015;49:1–9.
Schuers M, Joulakian MB, Griffon N, Pachéco J, Périgard C, Lepage E, et al. In: S IN, de PM AM, editors. Quality indicators from laboratory and radiology information systems. A. G. Volume 216. France: IOS; 2015. pp. 212–6. Department of Biomedical Informatics, Rouen University Hospital, Rouen Cedex, 76031,.
Tabrizi JS, Saadati M, Sadeghi-Bazargani H, Ebadi A, Golzari SEJ. Developing indicators to improve educational governance in hospitals. Clin Gov. 2014;19(2):117–25.
Costa A Jr, aS., Leão LE, Novais MA, Zucchi P. An assessment of the quality indicators of operative and non-operative times in a public university hospital. Einstein (Sao Paulo). 2015;13(4):594–9.
Coyne JS, Helton J. How prepared are US hospitals for the affordable care act? A financial condition analysis of US hospitals in 2011. J Health Care Finance. 2015;41(3).
Davis P, Milne B, Parker K, Hider P, Lay-Yee R, Cumming J, et al. Efficiency, effectiveness, equity (E-3). Evaluating hospital performance in three dimensions. Health Policy (New York). 2013;112(1–2):19–27.
Flatow VH, Ibragimova N, Divino CM, Eshak DSA, Twohig BC, Bassily-Marcus AM, et al. Quality outcomes in the surgical intensive care unit after electronic health record implementation. Appl Clin Inf. 2015;6(4):611–8.
Article CAS Google Scholar
Fonseca JRS, Ramos RMP, Santos AMP, Fonseca APSS. Policy effects on the quality of public health care: evaluating Portuguese public hospitals’ quality through customers’ views. Cent Eur J Public Policy. 2015;9(2):122–40.
Hadji B, Meyer R, Melikeche S, Escalon S, Degoulet P. Assessing the Relationships Between Hospital Resources and Activities: A Systematic Review. J Med Syst. 2014;38(10):1–21.
Hajduová Z, Herbrik G, Beslerová S. Application of DEA in the environment of Slovak hospitals. Invest Manag Financ Innov. 2015;12(4):148–53.
Thomas Craig KJ, McKillop MM, Huang HT, George J, Punwani ES, Rhee KB. U.S. hospital performance methodologies: a scoping review to identify opportunities for crossing the quality chasm. BMC Health Serv Res. 2020;20(1):640.
Carini E, Gabutti I, Frisicale EM, Di Pilla A, Pezzullo AM, de Waure C, et al. Assessing hospital performance indicators. What dimensions? Evidence from an umbrella review. BMC Health Serv Res. 2020;20(1):1038.
Rasi V, Delgoshaee B, Maleki M. Identification of common indicators of hospital performance evaluation models: a scoping review. J Educ Health Promot. 2020;9(1):63.
Xenos P, Yfantopoulos J, Nektarios M, Polyzos N, Tinios P, Constantopoulos A. Efficiency and productivity assessment of public hospitals in Greece during the crisis period 2009–2012. COST Eff Resour Alloc. 2017;15:15.
Shaqura II, Gholami M, Sari AA. Evaluation of performance at Palestinian public hospitals using Pabon Lasso model. Int J Health Plann Manage. 2021;36(3):896–910.
Li J, Seale H, Ray P, Wang Q, Yang P, Li S, et al. E-Health preparedness assessment in the context of an influenza pandemic: a qualitative study in China. BMJ Open. 2013;3(3):e002293.
Huang C-Y, Lai C-H. Effects of internal branding management in a hospital context. Serv Ind J. 2021;41(15–16):985–1006.
Download references
Acknowledgements
The authors are grateful for the support of the Vice Chancellor for Research of Isfahan University of Medical Sciences.
The present article is part of the result of a doctoral thesis approved by Isfahan University of Medical Sciences with code 55657 (IR.MUI.NUREMA.REC.1401.005), without financial source.
Author information
Authors and affiliations.
Student Research Committee, School of Management and Medical Information Sciences, Isfahan University of Medical Sciences, Isfahan, Iran
Shirin Alsadat Hadian
Health Management and Economics Research Center, Isfahan University of Medical Sciences, Isfahan, Iran
Reza Rezayatmand
Hospital Management Research Center, Health Management Research Institute, Iran University of Medical Sciences, Tehran, Iran
Nasrin Shaarbafchizadeh
Department of Management, Faculty of Administrative Sciences and Economics, University of Isfahan, Isfahan, Iran
Saeedeh Ketabi
School of Public Health and Preventive Medicine, Monash University, Victoria, Australia
Ahmad Reza Pourghaderi
You can also search for this author in PubMed Google Scholar
Contributions
Shirin Alsadat Hadian and Reza Rezayatmans and Saeedeh Ketabi: Study conceptualization and design. Acquisition of data: Shirin Alsadat Hadian, Reza Rezayatmand. Analysis and interpretation of data: Shirin Alsadat Hadian, Reza Rezayatmand, Nasrin Shaarbafchizadeh, Saeedeh Ketabi. Drafting of the manuscript: Shirin Alsadat Hadian, Reza Rezayatmand. Critical revision of the manuscript for important intellectual content: Reza Rezayatmand, Nasrin Shaarbafchizadeh, Saeedeh Ketabi, Ahmad Reza Pourghaderi.
Corresponding author
Correspondence to Reza Rezayatmand .
Ethics declarations
Ethics approval and consent to participate.
Not applicable.
Consent for publication
Competing interests.
The authors declare no competing interests.
Additional information
Publisher’s note.
Springer Nature remains neutral with regard to jurisdictional claims in published maps and institutional affiliations.
Supplementary Information
Supplementary material 1., supplementary material 2., supplementary material 3., rights and permissions.
Open Access This article is licensed under a Creative Commons Attribution 4.0 International License, which permits use, sharing, adaptation, distribution and reproduction in any medium or format, as long as you give appropriate credit to the original author(s) and the source, provide a link to the Creative Commons licence, and indicate if changes were made. The images or other third party material in this article are included in the article's Creative Commons licence, unless indicated otherwise in a credit line to the material. If material is not included in the article's Creative Commons licence and your intended use is not permitted by statutory regulation or exceeds the permitted use, you will need to obtain permission directly from the copyright holder. To view a copy of this licence, visit http://creativecommons.org/licenses/by/4.0/ . The Creative Commons Public Domain Dedication waiver ( http://creativecommons.org/publicdomain/zero/1.0/ ) applies to the data made available in this article, unless otherwise stated in a credit line to the data.
Reprints and permissions
About this article
Cite this article.
Hadian, S.A., Rezayatmand, R., Shaarbafchizadeh, N. et al. Hospital performance evaluation indicators: a scoping review. BMC Health Serv Res 24 , 561 (2024). https://doi.org/10.1186/s12913-024-10940-1
Download citation
Received : 03 January 2024
Accepted : 02 April 2024
Published : 01 May 2024
DOI : https://doi.org/10.1186/s12913-024-10940-1
Share this article
Anyone you share the following link with will be able to read this content:
Sorry, a shareable link is not currently available for this article.
Provided by the Springer Nature SharedIt content-sharing initiative
- Performance evaluation
BMC Health Services Research
ISSN: 1472-6963
- General enquiries: [email protected]
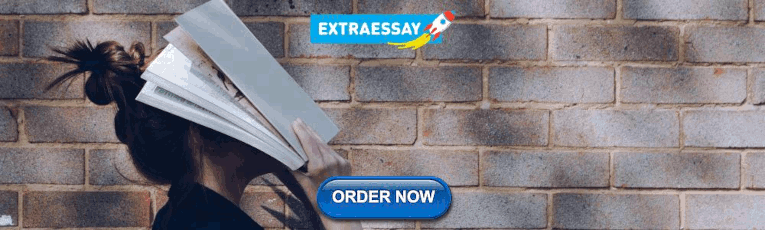
IMAGES
VIDEO
COMMENTS
The business environment is increasingly complex and competitive so organizations must respond to different market conditions by reconfiguring their processes, products and services. The objective of this article is based on a literature review under a scientometric and bibliometric approach, referring to the complexity in manufacturing systems, allowing to find answers to relevant questions ...
A literature review of manufacturing performance measures and measurement in an organizational context: A framework and direction for future research ... "An analytical hierarchy process frame ...
Flow line production is concentrated on multiple equipment or workstations that are arranged in the process sequence (Quadt and Kuhn, 2007). According to Drira et al. (2007), flow line production is characterised by high volume, repetitive and short cycle work. The work piece is physically moved through this sequence in
1. Introduction. The manufacturing industry is currently undergoing numerous transformations including shorter development and innovation times, individualized products, increased flexibility in production and product development, reduction of hierarchies, and resource efficiency [1].To cope with these transformations, industry moves toward a new level of value chain organization and control ...
A literature review is a systematic, ... Hence the multi-agent architecture presented by Zheng et al. [99], simultaneously based on the MPFQ-model (material, production process, production function/future, product quality) and DT technology, could be adopted as the basis of new research. On the other hand, scheduling is only one of the planning ...
The review also uncovers a tendency to devise taxonomies through the lens of experts' domain knowledge, particularly tailored to discrete manufacturing contexts. A critical gap is observed in the lack of a uniform PSF framework, with the current literature reflecting a disparate understanding of PSFs' roles, definitions, and interrelations.
One of the long-standing principles of phenomenological understanding is focused on the role of definition. Definitions are enablers in making something definite, distinct, or clear. This study aims to generate the definition of an emerging domain of Advanced Manufacturing Management using a literature review. First, an examination of Advanced Manufacturing Management (AMM) at an institutional ...
For the methodological process of this systematic literature review, we follow Tranfield et al. (2003) and conduct a sequential approach based on four phases: (1) preparation, (2) quality assurance, (3) data extraction, and (4) data analysis and synthesis. This procedure is widely accepted and frequently used in research (e.g., Delbufalo, 2012; Garza-Reyes, 2015; Licastro and Sergi, 2021).
The systematic literature review is characterised as a replicable, scientific, and transparent method, designed to reduce potential bias through exhaustive literature searches. ... "Smart Manufacturing Execution System (SMES): The Possibilities of Evaluating the Sustainability of a Production Process." 11th Global Conference on Sustainable ...
The first stage of the literature review identified relevant papers for inclusion in the analysis by using the combination of keywords (in title and abstracts) 'sustainable manufacturing' OR 'Industry 4.0' AND 'review' OR 'framework' in order to identify the main theoretical and literature review papers in both fields.
In the literature, this process of recycling and reusing waste powder from AM processes to subsequent ones has been extensively studied, ... Theodoros Nikolaos Maroutas, George Plakas, and Konstantina Dimogiorgi. 2021. "A Systematic Literature Review on Additive Manufacturing in the Context of Circular Economy" Sustainability 13, no. 11: 6007 ...
An extensive literature review was performed and indicated that a large number of process improvement methodologies are available for manufacturing [16]. The study showed that the PDCA and the six ...
The next section provides our literature review and shows the holistic LM-performance framework we used to extract and analyze the data. Research methodology is provided in section 3 where the measurement of the variables and data collection and sampling technique is explained. ... Tiwari AK. Assessment of manufacturing process through lean ...
A literature review of lean manufacturing. Shaman Gupta and Sanjiv Kumar Jain*. Department of Mechanical Engineering, Ambala College of Engineering and Applied Research, Devsthali, Ambala, India ...
Abstract. Manufacturing strategy has attracted serious research attention in the recent past. In this paper, an attempt is made to review the status of literature in manufacturing strategy. A literature classification scheme is suggested. A total of 260 articles from 31 referred journals and international conferences are classified into content ...
Compared with the subtractive manufacturing process, AM is a very competitive, low-cost, high manufacturing freedom technology [35-37].AM technology has many advantages, including the ability to reduce production time, produce complex customizable components, repair various mechanical parts, and fabricate free-form components [38, 39].Despite the benefits described above, AM technology is ...
Re-manufacturing. The process of re-manufacturing has taken an important place in reverse logistics and supply chain management. The remanufactured product maintains the same quality as the new product with updated features. Re-manufacturing also helps to lessen the expenses of production as many parts can be reused.
The definitions of production, process, or manufacturing platform also differs between these publications, or refers to a product platform but to be used in the production domain. 5. Conclusions The purpose of this paper was to review the current product and production platform literature to picture the production platform co-development research.
Based on the results of a literature review, a design structure matrix is constructed to analyze parameter influences. The overall result is a list of relevant parameters that should be recorded in the continuous mixing process in order to characterize the mixing process. ... The electrode manufacturing process is again divided into the process ...
This is an accepted article with a DOI pre-assigned that is not yet published.This literature review examines the implementation of e-portfolios in higher education, with a focus on the implementation process, potential barriers, and strategies for overcoming challenges. This review seeks to provide instructional designers and higher education instructors with design strategies to effectively ...
Hospitals are the biggest consumers of health system budgets and hence measuring hospital performance by quantitative or qualitative accessible and reliable indicators is crucial. This review aimed to categorize and present a set of indicators for evaluating overall hospital performance. We conducted a literature search across three databases, i.e., PubMed, Scopus, and Web of Science, using ...
A Critical Review of Process Parameters in Laminated Object Manufacturing Process. January 2020. DOI: 10.1007/978-981-15-4331-9_3. In book: Advances in Materials Engineering and Manufacturing ...
Process intensification is a manufacturing strategy that combines several unit operations into a single process step in order to increase the productivity of production processes. As a result, the quantity of energy, raw materials, and water needed to make a specific good can be reduced, improving the product's quality and cutting down on waste.
Organophosphonates (OPs) are a unique group of natural and synthetic compounds, characterised by the presence of a stable, hard-to-cleave bond between the carbon and phosphorus atoms. OPs exhibit high resistance to abiotic degradation, excellent chelating properties and high biological activity. Despite the huge and increasing scale of OP production and use worldwide, little is known about ...
Research on interactive alignment encompasses various perspectives, including the recognition of linguistic coordination patterns, mental representation processes between interlocutors, and linguistic and behavioral convergence. In the absence of a literature review that presents the advances in the study of interactive alignment, this study identified 64 theoretical and empirical articles ...
After considering the public's suggestions, panelists work to determine which treatments to recommend. They consider four factors: the overall strength of the evidence, the balance of benefits to potential harms, patients' values and preferences, and the applicability or generalizability of the evidence. They parse their recommendations ...