Applied Topology
Qualitative data analysis
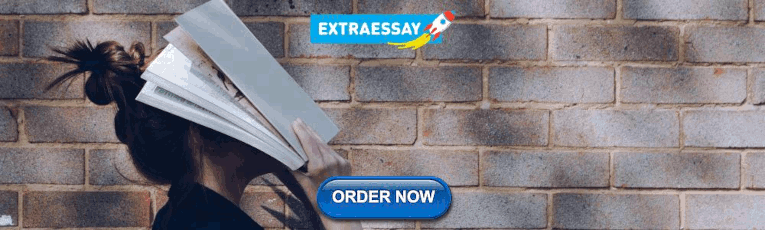
Postdocs and PhD positions in TDA at Swansea
1. Postdoc in Topological Data Analysis (part of the EPSRC-funded Oxford-Livepool-Swansea Centre for Topological Data Analysis) Closing date: 29 February 2020
https://www.swansea.ac.uk/personnel/jobs/details.php?nPostingId=64919&nPostingTargetId=81292&id=QHUFK026203F3VBQB7VLO8NXD&LG=UK&mask=suext
2. Postdoc in computational tropical geometry (Funded by Yue Ren’s UKRI fellowship) Closing date: 1 March 2020
https://www.swansea.ac.uk/personnel/jobs/details.php?nPostingID=65099&nPostingTargetID=81412&option=52&sort=DESC&respnr=1&ID=QHUFK026203F3VBQB7VLO8NXD&JOBADLG=UK&Resultsperpage=20&lg=UK&mask=suext These are both 3-year positions, 100% research focussed with no obligatory teaching load.
3. PhD scholarship in topological data analysis Closing date: 28 February 2020 (open only to EU/UK residents) https://www.swansea.ac.uk/postgraduate/scholarships/research/mathematics-phd-topological-data-biomedical.php
4. Additional PhD funding opportunities at Swansea though CDTs that could cover projects in topological data analysis (both of these are unfortunately open only to EU/UK residents:
• EPSRC multi-disciplinary Centre for Doctoral Training in Human-Centred AI and Data Science. https://www.swansea.ac.uk/science/epsrc-centre-for-doctoral-training/ • UKRI Centre for Doctoral Training in Artificial Intelligence, Machine Learning & Advanced Computing (AIMLAC) http://cdt-aimlac.org/
Leave a Reply Cancel reply
Your email address will not be published. Required fields are marked *
This site uses Akismet to reduce spam. Learn how your comment data is processed .
Global main menu
- School of Mathematical Sciences
- Postgraduate
- Postgraduate Research
- PhD projects
- Current PhD projects
Applications of Universality in Topological Data Analysis
Supervisor: Dr Omer Bobrowski
Project description: Recently discovered, the phenomenon of universality in Topological Data Analysis (TDA) offers a new direction to data analysis. This project will explore its applications and develop new methodologies on top of this phenomenon. There are many possible directions but the initial focus will be on:
- Dimensionality estimation: One of the key challenges in machine learning and data analysis is figuring out how many important features are in a dataset (i.e., its intrinsic dimension). However, t raditional methods, such as principal component analysis (PCA), may not be suitable for high-dimensional or noisy data. The project will develop statistical tools for applying universality for estimating dimension from topological features (including mixed dimensional spaces, relaxing the manifold hypothesis).
- Topological clustering: The connection between clustering and topology is well established with a substantial amount of previous work. This will build on this work to provide a complete framework for proving consistency in different clustering schemes as well as providing a provable approach to estimating the number of clusters from data. This will be driven by applications in a wide range of areas.
- Quantifying disorder: While TDA is often concerned with global structure, there are many cases where the distributions of smaller features in cases such as quasicrystals or other types of materials plays an important role. The goal of this application is to leverage universality to quantify the amount of order (as a form of regularity) in a point set. This will connect to existing work in sampling and discrepancy theory.
Further information: How to apply Entry requirements Fees and funding
An Introduction to Topological Data Analysis: Fundamental and Practical Aspects for Data Scientists
With the recent explosion in the amount, the variety, and the dimensionality of available data, identifying, extracting, and exploiting their underlying structure has become a problem of fundamental importance for data analysis and statistical learning. Topological data analysis (tda) is a recent and fast-growing field providing a set of new topological and geometric tools to infer relevant features for possibly complex data. It proposes new well-founded mathematical theories and computational tools that can be used independently or in combination with other data analysis and statistical learning techniques. This article is a brief introduction, through a few selected topics, to basic fundamental and practical aspects of tda for nonexperts.
- Related Documents
Precision dynamical mapping using topological data analysis reveals a unique hub-like transition state at rest
Even in the absence of external stimuli, neural activity is both highly dynamic and organized across multiple spatiotemporal scales. The continuous evolution of brain activity patterns during rest is believed to help maintain a rich repertoire of possible functional configurations that relate to typical and atypical cognitive phenomena. Whether these transitions or "explorations" follow some underlying arrangement or instead lack a predictable ordered plan remains to be determined. Here, using a precision dynamics approach, we aimed at revealing the rules that govern transitions in brain activity at rest at the single participant level. We hypothesized that by revealing and characterizing the overall landscape of whole brain configurations (or states) we could interpret the rules (if any) that govern transitions in brain activity at rest. To generate the landscape of whole-brain configurations we used Topological Data Analysis based Mapper approach. Across all participants, we consistently observed a rich topographic landscape in which the transition of activity from one state to the next involved a central hub-like "transition state." The hub topography was characterized as a shared attractor-like basin where all canonical resting-state networks were represented equally. The surrounding periphery of the landscape had distinct network configurations. The intermediate transition state and traversal through it via a topographic gradient seemed to provide the underlying structure for the continuous evolution of brain activity patterns at rest. In addition, differences in the landscape architecture were more consistent within than between subjects, providing evidence of idiosyncratic dynamics and potential utility in precision medicine.
Topological data analysis : an overview
A growing area of mathematics topological data analysis (TDA) uses fundamental concepts of topology to analyze complex, high-dimensional data. A topological network represents the data, and the TDA uses the network to analyze the shape of the data and identify features in the network that correspond to patterns in the data. These patterns extract knowledge from the data. TDA provides a framework to advance machine learning’s ability to understand and analyze large, complex data. This paper provides background information about TDA, TDA applications for large data sets, and details related to the investigation and implementation of existing tools and environments.
Options Pricing Via Statistical Learning Techniques: The Support Vector Regression Approach
Topological data analysis beyond genomics, voronoi graph traversal in high dimensions with applications to topological data analysis and piecewise linear interpolation, topological data analysis for evaluating pde-based denoising models, dimensionality reduction of complex reaction networks in heterogeneous catalysis: from l inear‐scaling relationships to statistical learning techniques, topological data analysis for classification of deepsat-4 dataset, scalable topological data analysis for life science applications, topological data analysis approaches to uncovering the timing of ring structure onset in filamentous networks.
AbstractIn developmental biology as well as in other biological systems, emerging structure and organization can be captured using time-series data of protein locations. In analyzing this time-dependent data, it is a common challenge not only to determine whether topological features emerge, but also to identify the timing of their formation. For instance, in most cells, actin filaments interact with myosin motor proteins and organize into polymer networks and higher-order structures. Ring channels are examples of such structures that maintain constant diameters over time and play key roles in processes such as cell division, development, and wound healing. Given the limitations in studying interactions of actin with myosin in vivo, we generate time-series data of protein polymer interactions in cells using complex agent-based models. Since the data has a filamentous structure, we propose sampling along the actin filaments and analyzing the topological structure of the resulting point cloud at each time. Building on existing tools from persistent homology, we develop a topological data analysis (TDA) method that assesses effective ring generation in this dynamic data. This method connects topological features through time in a path that corresponds to emergence of organization in the data. In this work, we also propose methods for assessing whether the topological features of interest are significant and thus whether they contribute to the formation of an emerging hole (ring channel) in the simulated protein interactions. In particular, we use the MEDYAN simulation platform to show that this technique can distinguish between the actin cytoskeleton organization resulting from distinct motor protein binding parameters.
Export Citation Format
Share document.
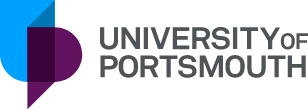
Metric techniques in topological data analysis
Self-funded
Project code
SMAP5370220
Start dates
February and October
Application deadline
Applications accepted all year round
Applications are invited for a 3 year PhD to commence in October 2020 or February 2021.
The PhD will be based in the Faculty of Technology, and will be supervised by Dr Ittay Weiss.
The work on this project could involve:
- Developing new techniques in frontiers of topological data analysis
- Synergising advanced methods of algebraic topology to facilitate new applications and enhance existing ones
- Developing new algorithms based on metric techniques to address problems in data science
Topological Data Analysis (TDA) is an emerging and highly successful approach to Big Data problems. The underlying idea is to employ topological techniques in the analysis of large quantities of data. One of the main difficulties with real-world data is that its collection introduces various types of 'noise'. Distinguishing between true features, inherent to the phenomenon in question, and misleading features resulting from noise contamination can be very challenging. Topology, by design, is blind to matters of scale or dimensionality. Its tools are aiming to extract geometric features which are robust in comparison to scale or dimensionality distortions. TDA seeks to exploit the inherent noise-blindness of topology by employing topological techniques to data analysis.
TDA represents a unique intersection point of pure mathematics with applied mathematics. In recent work such a formalism for topology was identified and is being developed. The advantages of the formalism to the foundations of topology are demonstrated while more advanced features are being tested. Alongside the topological gains, applications to the foundations of TDA are emerging as well. The main aims of the project are: 1) further enhance and develop the new mathematical foundations of topology; and 2) investigate the flow of ideas between topology and TDA along this new bridge, with new applications of TDA in mind.
The project aims to investigate alternative formalisms of topology with the aim of facilitating a smoother transition of topological tools to TDA, thereby enlarging the scope of applicability of TDA, while at the same time adjusting the classical topological methodologies to allow insights from TDA to filter back to topology. Using this improved language and tools new algorithms will be developed and tested for tackling TDA problems.
Fees and funding
Visit the research subject area page for fees and funding information for this project.
Funding availability: Self-funded PhD students only.
PhD full-time and part-time courses are eligible for the UK Government Doctoral Loan (UK and EU students only).
Entry Requirements Accordian Panel
General admissions.
- You'll need a good first degree from an internationally recognised university (minimum second class or equivalent, depending on your chosen course) or a Master’s degree in a relevant subject area
- In exceptional cases, we may consider equivalent professional experience and/or Qualifications
- English language proficiency at a minimum of IELTS band 6.5 with no component score below 6.0
Specific Admissions
Familiarity with formal proof based mathematics, and in particular topology.
How to apply
When you are ready to apply, please follow the 'Apply now' link on the Mathematics PhD subject area page and select the link for the relevant intake. Make sure you submit a personal statement, proof of your degrees and grades, details of two referees, proof of your English language proficiency and an up-to-date CV. Our ‘ How to Apply ’ page offers further guidance on the PhD application process.
Maia Fraser
Associate Professor
Department of Mathematics & Statistics School of E. Eng. & Comp. Sci. (cross-appointed) Brain and Mind Research Institute (member) University of Ottawa

Recent publications and preprints
- MAT4155: Elementary Manifold Theory
- MAT4373/5314: Statistical Machine Learning
- MAT3555/MAT3155: Géométrie Différentielle/Differential Geometry
- MAT2355: Introduction to Geometry
- MAT2143: Algebraic Structures: Intro. to Group Theory
- MAT1741: Algèbre linéaire
- MAT3153: Introduction to Topology
- CSC263: Data Structures and Analysis
- MAT102: Intro. to Mathematical Proofs
- CMSC28100 Introduction to Complexity Theory
- CMSC25010 Introduction to AI
- CMSC15300 Foundations of Software

An official website of the United States government
The .gov means it’s official. Federal government websites often end in .gov or .mil. Before sharing sensitive information, make sure you’re on a federal government site.
The site is secure. The https:// ensures that you are connecting to the official website and that any information you provide is encrypted and transmitted securely.
- Publications
- Account settings
Preview improvements coming to the PMC website in October 2024. Learn More or Try it out now .
- Advanced Search
- Journal List
- Front Artif Intell

Applications of Topological Data Analysis in Oncology
Anuraag bukkuri.
1 Department of Integrated Mathematical Oncology, Moffitt Cancer Center, Tampa, FL, United States
Noemi Andor
Isabel k. darcy.
2 Department of Mathematics, University of Iowa, Iowa City, IA, United States
The emergence of the information age in the last few decades brought with it an explosion of biomedical data. But with great power comes great responsibility: there is now a pressing need for new data analysis algorithms to be developed to make sense of the data and transform this information into knowledge which can be directly translated into the clinic. Topological data analysis (TDA) provides a promising path forward: using tools from the mathematical field of algebraic topology, TDA provides a framework to extract insights into the often high-dimensional, incomplete, and noisy nature of biomedical data. Nowhere is this more evident than in the field of oncology, where patient-specific data is routinely presented to clinicians in a variety of forms, from imaging to single cell genomic sequencing. In this review, we focus on applications involving persistent homology, one of the main tools of TDA. We describe some recent successes of TDA in oncology, specifically in predicting treatment responses and prognosis, tumor segmentation and computer-aided diagnosis, disease classification, and cellular architecture determination. We also provide suggestions on avenues for future research including utilizing TDA to analyze cancer time-series data such as gene expression changes during pathogenesis, investigation of the relation between angiogenic vessel structure and treatment efficacy from imaging data, and experimental confirmation that geometric and topological connectivity implies functional connectivity in the context of cancer.
1. Introduction
With the advent of next-generation high-throughput sequencing (Roychowdhury et al., 2011 ; Reuter et al., 2015 ), improved medical imaging (Wang, 2016 ; Tahmassebi et al., 2018 ; Aiello et al., 2019 ), and an increased focus on personalized medicine (Dilsizian and Siegel, 2014 ; Gu and Taylor, 2014 ; Alyass et al., 2015 ; Suwinski et al., 2019 ), more data is being collected than ever before. Efficient data analysis techniques are critically needed to convert this data into meaningful, clinically translatable information. Topological data analysis (TDA) focuses on the shape of data, identifying both local and global structures at multiple scales. Consider a trivial example: suppose data points lie on a circle. The data points could represent customers' preferences or patient gene expression. In this case if a product or drug were targeted to the average person, the target would be the center of the circle and would thus miss the data set entirely. While this is a simple made-up example, it illustrates the importance of understanding the shape of data. TDA can be applied to high-dimensional and noisy data. While the output of TDA can be affected by incomplete data, it is still effective at distinguishing between data sets that have different shapes.
TDA has been successfully applied in a variety of medical contexts including to discover phenotype-biomarker associations in traumatic brain injury (Nielson et al., 2017 ), identify diagnostic factors for pulmonary embolism (Rucco et al., 2015 ), discriminate between healthy patients and those with diabetic retinopathy from retinal imaging (Garside et al., 2019 ), map human recombination at fine scales (Camara et al., 2016 ), identify novel pathological phenotypes of asthma (Siddiqui et al., 2018 ), and characterize the structure of chromatin conformation inside the nucleus (Emmett et al., 2016 ). In this review, we shall focus our attention on some recent applications of persistent homology, a main tool of TDA, to oncology. We specifically discuss treatment responses, clinical outcomes, disease classification, biomarker identification, and cellular architecture in cancer. We will also provide insights into possible future fruitful avenues of research, including analysis of time-series data to help with disease classification and identification of selection events, investigation of the relation between angiogenic vessel structure and treatment efficacy from imaging data, and experimental confirmation that geometric and topological connectivity implies functional connectivity in the context of cancer. Though we focus on persistent homology here, it is worth noting that there have been many notable successes of the application of other TDA methods, such as the Mapper algorithm (Singh et al., 2007 ). For example, Mapper was recently used to extract information from high-throughput microarray data and define a new subtype of breast cancer, c-MYB+, characterized by high c-MYB expression and low levels of innate inflammatory genes, with corresponding patients exhibiting 100% survival and no metastasis (Nicolau et al., 2007 ). In another study, Mapper was used to discover 38 new cancer-associated genes across tumor types, some of which were then confirmed to play a key role in tumorigenesis in mouse models (Rabadán et al., 2020 ). Before delving into the applications of persistent homology in cancer, we introduce some of the key mathematical underpinnings needed to understand these results.
2. What Is Persistent Homology?
The mathematical definition of homology/homologous is very precise and often differs from the English common usage. Homology uses algebra to detect topological shapes. Topology is sometimes called rubber sheet geometry as two objects are topologically equivalent to each other if one can be deformed into the other without tearing or puncturing the objects. For example, the spherical and cubical surfaces are topologically equivalent per Figure 1A . The sphere is topologically different from the 3-dimensional ball that the sphere bounds. Homology detects this difference by noting that the 2-dimensional spherical surface bounds a void while the 3-dimensional ball is solid and thus does not bound any voids.

(A) The solid ball and solid cube are topologically equivalent and thus have the same homology. Their surface boundaries also have the same homology since these surfaces are topologically equivalent. The solid ball has one connected component and thus β 0 = 1. The solid ball does not contain any voids, and thus β i = 0 for all i > 0. The sphere, which is the boundary of the ball, has β 0 = 1 since it is connected, and β 2 = 1 since the 2-dimensional sphere bounds a void, while β i = 0 for all other i since there are no lower or higher dimensional voids. For an n + 1-dimensional ball (for example, all points of distance less than or equal to 1 from the origin in R n +1 ), β i = 0 for all i > 0 since it does not contain any voids. The n -dimensional sphere, which is the boundary of the n + 1-dimensional ball, has β i = 1 for i = 0, n and β i = 0 for all other i . Since the n -dimensional sphere contains a void, it is the n -dimensional object that generates β n . (B) A surface with boundary that is topologically equivalent to an annulus. The annulus is a 2-dimensional surface that has the same homology as a 1-dimensional circle. Since this object has one connected component, β 0 = 1. We can use addition to represent a cycle. The cycle e 5 + e 9 + e 12 + e 8 = e 5 + e 8 + e 9 + e 12 is homologous to 0 since it bounds a surface (the light green waning crescent moon). Since all 1-dimensional cycles are either homologous to 0 or to (a multiple of) the rectangle cycle e 1 + e 2 + e 3 + e 4 , β 1 = 1. Since this object lives in the 2-dimensional plane, β i = 0 for all i > 1. (C) The solid torus has β i = 1 for i = 0, 1 and β i = 0 for all other i while its boundary, the torus, has β i = 1 for i = 0, 2, β 1 = 2, and β i = 0 for all other i . The thick blue cycle is a 1-dimensional homology generator for both the solid torus and its boundary. The thiner black cycle is a homologous to 0 in the solid torus as it bounds a meridinal disk, while this black circle is a homology generator in the torus which is not homologous to the blue circle. The torus surface generates the 2-dimensional homology.
To describe homology, we will first focus on two quantities: β 0 = the number of connected components and β 1 = the number of 1-dimensional holes (a circle that has not been filled in). One does not need to understand the algebra of homology in order to understand the basics of persistent homology, thus we will only briefly introduce some concepts for the interested reader. Two points are homologous if they are in the same connected component. Thus, β 0 = 1 if the object is connected. To describe β 1 , we will focus on Figure 1B . We can use addition to represent topological objects. For example, the rectangle in Figure 1 is represented by the sum of edges: e 1 + e 2 + e 3 + e 4 . Two 1-dimensional cycles are homologous to each other if they form the boundary of a surface. Thus, the rectangle is homologous to the cycle e 5 + e 8 + e 10 + e 11 since these two cycles bound the green surface. The cycles e 5 + e 6 + e 7 + e 8 and e 9 + e 10 + e 11 + e 12 are also homologous since they bound the light green surface consisting of two crescent moons. In fact all these cycles are homologous to the rectangle e 1 + e 2 + e 3 + e 4 . One can see that this object contains many cycles, many of which are homologous to the rectangle (or a multiple of the rectangle, for example, ∑ i = 5 12 e i is homologous to 2 ∑ i = 1 4 e i ). A 1-dimensional cycle is homologous to 0 if it bounds a surface. Thus the cycles e 5 + e 9 + e 12 + e 8 and e 6 + e 7 + e 11 + e 10 are both homologous to 0 since they each form the boundary of a surface (the two crescent moons, waning or waxing, respectively). Since each of the cycles in this figure are homologous to 0 or to a multiple of the rectangle, its homology is generated by a single cycle (for example, the rectangle) and thus β 1 = 1.
The intuitive definition of homology is that β n equals the number of n −dimensional holes 1 . Per the Figure 1 caption, homology can be used to distinguish the following objects from each other: solid ball, sphere, higher dimensional balls and spheres, solid torus, and torus. Homology cannot distinguish all objects that are topologically different. For example,the 1-dimensional circle, the 2-dimensional surface in Figure 1B , and the 3-dimensional solid torus ( Figure 1C ) all have the same homology. For more on the mathematical definition of homology (please see Munkres, 1984 ; Hatcher, 2002 ; Ghrist, 2014 ).
We will illustrate with an elementary example how persistent homology can detect shape at multiple scales by noting the birth and death of topological features. Our dataset will consist of 5 points from a circle as shown in Figure 2 . To detect the circle, we need to connect these points in some manner. For example, we could connect all points whose distance is less than some fixed ϵ. If one can visualize the data set, then the choice of ϵ may be clear. But more often, there is no obvious choice, so instead we analyze the data at multiple scales using persistent homology. The first box in Figure 2 shows the five data points. At this stage, we have five components, one for each data point (β 0 = 5). These components are represented by the five red lines in the top part of this figure. These five red lines along with the blue segment is called the barcode for the data set. The barcode keeps track of the number of components (red bars) and number of 1-dimensional holes (blue bar) as the threshold for connecting data points increases. We can visualize the increasing threshold (or proximity parameter) by growing balls around each data point and connecting pairs of points as soon as their respective balls intersect. Thus, in the second box, an edge joins the two closest points, reducing the number of connected components by one. Thus, one bar ends (dies), and only 4 bars (β 0 = 4) continue past this threshold. Observe that every time an edge joins two components, a bar dies (and β 0 reduces by one). In the timepoint just before 1.5 (box labeled 5), two edges are added. One connects two components, but the third forms a triangle with two previously created edges. These three edges surround a small hole, but we fill in this hole (shaded in pink) as we only want to detect large holes. We are forming a Rips complex where whenever a triangle is formed, it is immediately filled in and thus triangles do not contribute to β 1 . In the timepoint after 1.5 (box labeled 6), a cycle containing four edges is formed. This is indicated in the barcode by the start (birth) of the blue bar. As more edges are added, eventually this region is divided into two triangles and the blue bar dies at timepoint close to 2 (corresponding to box labeled 7). Note we have one infinitely long bar (top red bar with arrow) since after time 1.5 we have one connected component.

A barcode captures topological features in a dataset at multiple scales. The topology of a dataset at a fixed scale is determined by joining pairs of data points with an edge if the distances between the pair of points is less than the fixed scale. If three edges form a triangle, then the triangle is filled in. This process is shown in the seven boxes as the scale for joining vertices increases from box 1 to box 7. The corresponding barcode is shown at the top of the figure. The persistence of a feature over multiple scales determines the length of the bar corresponding to that feature. The number of components (β 0 ) that exist at a particular scale is represented by the number of red bars that exists at the corresponding Rips diameter. The creation of the 1-dimensional cycle in box 6 is represented by the birth of the blue bar. The blue bar dies when this cycle is filled in with triangles (box 7). This figure was created by modifying the output of the R package TDAstat (Wadhwa et al., 2018 ) and latex code written by Catalina Betancourt.
To summarize, this example of a TDA pipeline consists of taking a dataset, creating a sequence of Rips complexes, and outputting a barcode (Edelsbrunner et al., 2002 ; Carlsson et al., 2005 ; Zomorodian and Carlsson, 2005 ). A Rips complex is a generalization of a graph. While in our example we only looked at adding edges and triangles, we can also add higher dimensional simplices. A n -simplex in a Rips complex is a collection of n + 1 points where each pair of points is connected by an edge. Thus an edge is a 1-simplex, a triangle is a 2-simplex, and a tetrahedron is a 3-simplex. In our circle example, when all pairs of the 5 points are connected by edges, we add a 4-simplex even though the data set lives in 2-dimensions. The existence of an n -simplex means that (all pairs of) n + 1 points are close together according to a given threshold. The Rips complex is also called a clique complex, the latter term coming from graph theory where a clique is a graph where every pair of vertices is connected. Thus, our simplices correspond to clique subgraphs. Other names for Rips complex include Vietoris-Rips complex and flag complex.
There are other ways to form a simplicial complex from data. For the Rips complex, an n -simplex is formed at threshold r when all pairs of n + 1 points are of distance less than r (so that each pair of points is connected by an edge). This is equivalent to requiring every pair of balls of radius r centered around the n + 1 points to intersect. If we require the intersection of all these balls to be nonempty in order to form an n -simplex, we instead form the Čech complex. Thus, to form a 2-simplex (triangle), the Rips complex only requires non-empty pairwise intersection of three balls while the Čech complex requires the intersection of all three balls to be nonempty. Thus, the Čech complex is similar to the Rips complex, but an n -simplex is formed at a slightly larger threshold in the Čech complex. Under certain conditions, the Čech complex is guaranteed to have the same homology as the union of all balls of radius r centered around data points (Hatcher, 2002 ). But the Rips complex has much smaller computer memory requirements as only the edges need to be stored to determine the Rips complex, and thus the Rips complex is normally used when calculating persistent homology. A very different TDA technique called Mapper uses a completely different method to create a simplicial complex from data (Singh et al., 2007 ). For Mapper, each vertex represents a cluster of data points. If n + 1 of these clusters have a common intersection, then an n -simplex is formed. Mapper can be used to reduce the size of a data set and to visualize it.
The example in Figure 2 focused on β 0 and β 1 . For data that lives in a higher dimensional space, we can similarly calculate β n = the number of n-dimensional holes. For example, β 2 = 1 for both the sphere and torus as these are 2-dimensional surfaces that bound voids in space. For more details regarding persistent homology and barcodes (please see Ghrist, 2008 ; Carlsson, 2009 ; Edelsbrunner and Harer, 2010 ; Otter et al., 2017 ).
In order to use persistent homology in machine learning, we need a distance between barcodes. We first convert barcodes to persistence diagrams as described in the next section and use these diagrams to define a distance between barcodes. In this section, we show how persistent homology is stable with respect to noise: small perturbations in the data have only a small effect on the barcode (Cohen-Steiner et al., 2007 ). In section 2.2, we discuss the advantages/disadvantages of persistent homology with regard to how it handles noise, incomplete data, and computational complexity. In section 2.3, we discuss one method (persistent images) of converting a persistence diagram into a vector that can be used in machine learning. We also give references to many other methods for using persistent homology in machine learning.
While we have discussed the basic method for converting Euclidean data into barcodes, there are a number of other methods for obtaining barcodes from data. All one needs is a method to determine when to add an edge between pairs of data points. Thus, the data do not need to live in Euclidean space. We also assumed that small holes correspond to noise, but there are applications where the point of using persistent homology is to detect small holes (Bendich et al., 2016 ). We also had only one infinite bar corresponding to the one connected component we obtained when all our data points were connected by edges. If one is working with Euclidean data, eventually all holes will be filed in and thus eventually a Rips complex with only one component and no holes will be formed. But in other applications, holes may persist forever, resulting in infinite bars. One can also obtain additional information by looking at the group structure of the filtered homology groups, and prove stability properties using interleaving distance (Bauer and Lesnick, 2014 ; Bubenik and Scott, 2014 ; Oudot, 2015 ; Chazal et al., 2016 ).
2.1. Persistence Diagrams and Stability
While barcodes are useful for visualizing changes in homology, barcodes are generally converted into persistence diagrams for statistical and machine learning analysis (Edelsbrunner et al., 2002 ; Mileyko et al., 2011 ). The start of a bar represents the birth of a cycle while the end represents its death. The plot of the points (birth time, death time) in 2-dimensional space is called the persistent diagram (PD). The persistent diagram corresponding to the barcode in Figure 2 is shown in Figure 3 . A persistence diagram also includes the diagonal as shown in this figure as the diagonal is used when computing distances between PDs. A PD can be a multiset if multiple bars have the same birth time b and death time d , so that the point ( b, d ) occurs multiple times in the PD.

A barcode can be converted into a persistent diagram. Each bar with finite length in a barcode is represented by a point in the persistent diagram. If a bar is born at time b and dies at time d , then the bar is represented by the point ( b, d ). In Figure 2 , there are four finite red bars plus one infinite red bar. These bars are all born at time 0. In the persistent diagram, the four finite red bars are represented by the four red points all of which have b = 0. The one blue bar in Figure 2 is represented by the blue triangle in this persistent diagram. This figure was created using the R package TDAstat (Wadhwa et al., 2018 ).
The formula for the bottleneck distance for a fixed β i between two persistence diagrams, P 1 and P 2 , is d B ( P 1 , P 2 ) : = inf γ : P 1 → P 2 sup x ∈ P 1 ∥ x - γ ( x ) ∥ ∞ . To compute this distance we first create a matching γ between these diagrams for the fixed β i as shown in Figure 4 . In this figure the blue triangles represent features with the fixed β i from one data set while the purple stars represent features from a different data set for the same β i . A matching γ: P 1 → P 2 is a bijective function from P 1 to P 2 where both persistence diagrams include the diagonal. Features that are close to the diagonal get matched to the diagonal unless they are closer to another feature that does not have a better matching than to the diagonal. If x = ( b, d ) ∈ P 1 is matched to the point (β, δ), then the distance between these features is ‖ x − γ( x )‖ ∞ = max(| b − β|, | d − δ|). To find the distance for a particular matching γ, we calculate sup x ∈ P 1 ∥ x - γ ( x ) ∥ ∞ = the largest distance between a point x in P 1 and its match γ( x ) in P 2 . The bottleneck distance is obtained by taking the infimum of this distance over all possible matchings. In Figure 4 , red dotted lines indicate best matches between features from P 1 and P 2 .

Two persistence diagram, P 1 and P 2 , are shown for a single dimension (for example, β 1 ). The blue triangles correspond to P 1 while purple stars are used for P 2 . Both persistence diagrams include the diagonal. A matching between P 1 and P 2 is shown where the red dotted lines indicate features that have been matched where some of the features are matched to the diagonal. The length of the thicker dark red dotted lines indicate the distance between matched features. The distance between a feature and the diagonal is the persistence of the feature, d − b , where b = birth time and d = death time of that feature. If feature ( b, d ) is matched with feature (β, δ), then the distance between these features is max(| b − β|, | d − δ|). Since the best matching is shown, d B ( P 1 , P 2 ) equals the length of the longest of the thick dark red dotted lines. Any other matching would have a matched pair of features with larger distance.
If P 1 is the PD for the data set X and P 2 is the PD for the data set Y , the stability theorem states that d B ( P 1 , P 2 ) ≤ d H ( X, Y ) = inf{ε ≥ 0; X ⊆ Y ε and Y ⊆ X ε } where X ε : = ∪ x ∈ X { z ∈ M ; d ( z , x ) ≤ ε } (Cohen-Steiner et al., 2007 ). In other words, if each data point is perturbed by at most a distance ϵ, then the persistence of a feature will change by at most 2ϵ since the birth and death times can change by at most ϵ. Features with persistence <2ϵ may disappear, while new features with persistence less than 2ϵ may be created.
2.2. Benefits and Limitations of Persistent Homology
That persistent homology is stable with respect to noise is, of course, a major advantage. But any method that uses Euclidean distance is affected by the curse of dimensionality due to the effect of noise on distance. For example, suppose a data point should be at the origin, but due to noise, each coordinate is perturbed by 0.01 units, then the point which should be at the origin is now Σ i = 1 n ( 0 . 0 1 2 ) units away from the origin if the data lives in ℝ n . Thus, for example if n = 10, 000, then the data point is perturbed by a distance Σ i = 1 10 , 000 ( 0 . 0 1 2 ) = 1. While the change in persistent homology is bounded by the distance between the original data set and the perturbed data set, the latter can be quite large, depending on the amount of noise and the dimension of the dataset. Thus, performing PCA or t-SNE or other dimension reduction technique first may lead to stronger results.
In order to recover the shape of an object, one must have sufficient coverage. Some holes detected by persistent homology may be due to incomplete data. If these are small, then they only result in short bars which may be considered noise. But in high dimensional spaces, one has many degrees of freedom, so even recovering the shape of simple objects in high dimensions can be impossible as obtaining a sufficient number of data points may not be feasible. However, differences between data sets may still be detected even if coverage is lacking. For example, one may have insufficient coverage to recover the topology of a torus if one uniformly under-samples data points from a torus. However, the resulting barcode will likely be very different than the barcode obtained from uniformly under-sampling points from a sphere. Also, coverage can be less of an issue if you have some information regarding the shape of the data such as periodicity (for example, Dequeant et al., 2008 ). Thus, in practice, topological data analysis has proven to be quite robust. For more on complexity and topological inference (see Weinberger, 2014 ).
Due to computational complexity, most analysis using TDA restricts to the use of β i for i ≤ 4. Often only β 0 and β 1 are used, but faster algorithms such as Ripser (Bauer, 2019 ) are becoming available. To calculate persistent homology of a point cloud, one first needs to create simplicial complexes. The number of simplices grows rapidly with the number of data points as well as the homology dimension (not the dimension of the data set, but the dimension of the holes one wishes to detect—in order to calculate β i , one needs i -dimensional and i + 1 dimensional simplices). The TDA pipeline also requires the computation of distances between data points. The dimension in which the data lives can affect this step, but after distances are calculated, it is the shape of the data that can have the largest effect, sometimes even larger than the number of data points as there are several algorithms that can greatly simplify the simplicial complex (Zomorodian, 2010 ; Mischaikow and Nanda, 2013 ; Wilkerson et al., 2014 ; Boissonnat and Pritam, 2020 ). The effectiveness of these simplification algorithms depends on both the topology and geometry of the data set. For example, suppose one takes n data points equally spaced on a straight line. The topology of the line is the same as the topology of a point. Thus, to calculate the homology of the line, one can remove all simplices except for a single vertex. For more on computational complexity of persistent homology (see Otter et al., 2017 ).
If all the data points enter at time 0, the β 0 bars all start at time 0. Thus the barcode for β 0 can be created from a single linkage hierarchical clustering dendrogram as the merge heights of the dendrogram become the lengths of the β 0 bars. Hence the β 0 barcode contains less information than a single linkage hierarchical clustering dendrogram. However, there are applications where the data points enter at different times such as time series data. Thus, the β 0 barcode can be applied to a wider variety of applications than standard clustering techniques. Clustering also cannot capture holes and voids; the higher dimensional barcodes capture structure that other methods such as clustering miss.
2.3. Persistent Homology and Machine Learning
The barcode can be used as a topological signature to identify structure in data. While homology is built to detect topology and not geometry, persistent homology can be implemented in a variety of ways to distinguish geometrical shapes (e.g., Turner et al., 2014 ; Li et al., 2018 ; Bubenik et al., 2020 ). Machine learning can be applied to a collection of persistent diagrams to distinguish between data sets with different structures. Many machine learning algorithms take a vector as input. There are many ways to create a vector from persistent homology. A pipeline to create a vector using persistence images (Adams et al., 2017 ) is illustrated in Figure 5 . A persistent diagram is first rotated by 45° so that the diagonal becomes the horizontal axis (2nd panel of Figure 5 ). Thus the horizontal axis represents the birth time, while the vertical axis represents persistence = death - birth. A heat map is then created using a Gaussian distribution (or other weight function) about each point (3rd panel). The height of the Gaussian distribution is indicated with color in the heat map and is dependent on the persistence of the feature. Points closest to the diagonal are considered to be the result of noise and are thus given no intensity. Hence the bottom of the heat map will always have the color corresponding to zero intensity, in this case blue. In other words, points close to the diagonal have no effect on the heat map. Observe that the point furthest from the diagonal in the first panel corresponds to a feature with the largest persistence per second panel. Thus, in the heat map in the 3rd panel, the color at this point is given the highest intensity (yellow). As shown in the fourth panel, the heat map is discretized by partitioning the heat map into n × n squares where the color of each square corresponds to the average value of the corresponding square in the heat map. In the discretized heat map (4th panel), the yellowish region from the 3rd panel corresponding to the most persistent feature is partitioned between two squares with the yellow square in the top row of this heat map containing a larger portion than the pinkish square next to it in the same row. In the final panel, an n 2 -dimensional vector is created by concatenating the rows of the discretized heat map.

Pipeline for vectorizing a persistent diagram using persistent images. This figure is a modification of Figure 1 from Adams et al. ( 2017 ) which is licensed under CC BY 4.0 .
Other methods for using persistent homology in machine learning include persistent landscapes (Bubenik, 2015 , 2020 ), persistent curves (Chung and Lawson, 2019 ), and kernel functions (for example, Reininghaus et al., 2015 ; Kusano et al., 2016 ; Carrière et al., 2017 ; Chazal et al., 2017 ).
3. Treatment Responses and Prognosis
What impedes the success of cancer therapies is often the coexistence of therapy resistant cells along with therapy sensitive tumor cell populations. When administered separately, all currently adopted therapeutic strategies—ranging from cytotoxic chemotherapies to molecular targeted therapies—impose a dramatic, yet homogeneous selective pressure on an often heterogeneous group of tumor cells. Despite varying resistance mechanisms contingent upon therapy-type and tumor composition, every therapeutic intervention inevitably selects for resistant cells, which expand and become the dominant cell type of recurrent tumors, that cease to respond to therapy (Maley and Reid, 2005 ; Aparicio and Caldas, 2013 ; Bukkuri, 2020 ). The increased resolution on the clonal architecture of intermixed tumor cell populations that has just now become available calls for prognostic and therapeutic benefits. High intra-tumor diversity in pre-malignant lesions has been shown to predict progression to malignant growths and poor outcome (Maley et al., 2006 ; Laurie et al., 2012 ). The therapeutic significance of intratumoral heterogeneity (ITH) is exemplified in a recent study that measured genetic and transcriptional diversity of breast cancer tumors before and after therapy based on four genetic markers and two transcriptional markers. The study provided proof-of-principle that therapy-induced phenotypic changes can be predicted based on the characterization of coexisting tumor subpopulations (Almendro et al., 2014 ). Another recent study used RNA interference to model heterogeneous tumors and tested the efficacy of predicted drug combinations in eliminating coexisting tumor subpopulations (Zhao et al., 2014 ). Their findings suggest that the most effective drug combination for a given tumor cannot be achieved by targeting the predominant subpopulation alone, but requires detailed characterization of the genetic makeup of branched subpopulations and their contribution to the tumor bulk.
Techniques from computational homology have been used to develop a new algorithm to characterize comparative genomic hybridization (CGH) profiles and identify the frequency of cancer recurrence in early stage breast cancer patients through identification of recurrent copy number aberrations (CNAs) in cancer (DeWoskin et al., 2010 ), which serve as markers of genomic instability and thus cancer prognosis (Hanahan and Weinberg, 2000 ; Han et al., 2006 ). Specifically, the method uses a sliding window algorithm to associate a set of point clouds to each array CGH. Different window sizes allow one to analyze the data at various scales by considering different dimensional point clouds. Then, persistent homology is applied to these point clouds for classification. It was found, in accordance with prior results (Climent et al., 2007 ), that the Betti numbers of the zero dimensional homology groups (β 0 ) can distinguish between recurrent and non-recurrent groups in patients who did not receive anthracycline-based chemotherapy after surgery but not in patients who were treated with anthracycline. Note that, in this approach, no segmentation of the data was required.
In another study, a novel statistic called the smooth Euler characteristic transform (SECT), which allows shape information to be integrated into traditional statistical models, was developed and applied to predict disease free survival in glioblastoma multiforme (GBM) based on tumor shape from post-contrast T1 axial magnetic resonance imaging (MRI) (Crawford et al., 2020 ). SECT is a variation of the persistent homology transform (PHT) introduced in Turner et al. ( 2014 ) that was created to overcome the difficulties in integration with traditional statistical models. Specifically, the output of SECT is a collection of smooth vectors, while the output of PHT is a collection of persistence diagrams (Edelsbrunner et al., 2002 ), thus having a complicated representation and geometry which does not lend itself easily into integration with statistical models. In the GBM application, the statistical model used was a Bayesian linear mixed model (BLMM) (Ishwaran and Rao, 2005 ; Guan and Stephens, 2011 ; Zhou et al., 2013 ). When this topological approach was applied to the GBM MRI data, it was found to outperform gene expression, volumetric, and morphological summaries in predicting disease free survival.
Clinically, there is a great importance in the identification of biomarkers which can serve as predictors for metastasis and patient prognosis in cancer. To this end, researchers have recently used persistent homology techniques, in an exploratory data analysis fashion, to identify biologically meaningful geometric properties of single cell data (Lockwood and Krishnamoorthy, 2015 ). In this method, data was first transposed and analyzed in its dual space with each gene represented in a much lower dimensional sample space, thus circumventing the problem of high dimensionality that is typical of single cell data. A small set of genes (120–200) were then selected as landmarks (De Silva and Carlsson, 2004 ) and a family of nested simplicial complexes was constructed, indexed by a proximity parameter. Unlike many other methods which focus on the analysis of zero dimensional homology groups (DeWoskin et al., 2010 ; Nicolau et al., 2011 ), thus performing analyses which are topologically equivalent to clustering, this study focused their efforts on identifying loops of one dimensional homology groups which persist over a large range of values of the proximity parameter, hypothesizing that connections around holes imply nontrivial interactions among genes and biological functions which could have implications for tumorigenesis. Repeating this process for various landmarks, features which remain stable over large ranges of both the proximity parameter and number of landmarks could be detected. Applying these techniques to five different cancer data sets from brain, breast, ovarian, and acute myeloid leukemia cancers, many members of the significant loops in the one dimensional homology groups that were found have been previously shown to be accurate biomarkers for cancer biogenesis, while others serve as potential new markers which have yet to be experimentally validated.
4. Tumor Segmentation and Computer-Aided Diagnosis
Computerized methods can efficiently and effectively identify quantitative image features that are otherwise difficult to spot by manual inspection (Yu et al., 2016 ). Quantitative morphological features extracted from H&E stained slides, such as Zernike shape features, have been shown to predict survival in lung adeno- and squamous cell carcinoma (Yu et al., 2016 ). Recent advances in next-generation sequencing technologies gave rise to a plethora of approaches that quantify and characterize the genotypic diversity within a given tumor. Evidence supporting a quantitative relation between genotypic and morphological ITH followed. A quantitative image analysis approach that complements genomic profiling with geographical information was developed (Yuan et al., 2012 ; Andor et al., 2016 ). Furthermore, the authors characterized cellular heterogeneity by distinguishing between well-defined cell-populations (stromal cells, lymphocytes, cancer cells). However, so far qualitative details of how this diversity in morphology is structured (i.e., how many subpopulations are present and what their geographical boundaries are on the H&E slide) are unknown.
As a step toward a computer-aided cancer diagnosis system, persistent homology has been used to develop an automated tumor segmentation approach for Hematoxylin & Eosin (H&E) stained colorectal cancer histology whole slide images (WSI) (Qaiser et al., 2016 ). The authors exploit the fact that nuclei in tumor regions have atypical characteristics such as non-uniform chromatin texture, irregularity in shape and size, and clustering of nuclei, and use persistent homology profiles to characterize the degree of connectivity among nuclei and to classify cancerous regions based on this information. Specifically, once a WSI has been obtained, it is first divided into patches, each of which has a persistent homology profile. Given two patches, the symmetrized Kullback-Leibler divergence (KLD) can be computed between the respective persistent homology profiles, which serves as a metric for interpatch distance. Then an input patch is classified as cancerous or non-cancerous by a kNN classifier, based on KLD distances between its persistent homology patch and those of each representative patches. These exemplar patches are chosen by training a CNN and selecting patches whose activation during training is large (separately for cancerous and non-cancerous classes). The benefit of this approach over previous approaches is that only the subset of highly activated patches from the convolutional layers are used as exemplars rather than the set of all patches in the training data. This method was compared against standard CNN and HyMaP (Khan et al., 2013 ) approaches on 74 H&E stained WSIs of colorectal cancers; in addition to being computationally less expensive than the other two methods, it was also shown to have better precision and segmentation accuracy.
Another example of tumor segmentation and algorithmic diagnosis is a recent study which aimed to segment a diseased area of skin and classify the type of skin lesion into one of seven classes in a given dermatoscopic image (Tschandl et al., 2018 ) using persistent homology (Chung et al., 2018 ). Like the colorectal image segmentation study (Qaiser et al., 2016 ), the segmentation algorithm used is a concept similar to persistent homology (Edelsbrunner et al., 2002 ). Linear support vector machines (SVMs) were used for classification on the persistence statistics (Chung et al., 2018 ) and persistence curves (Chung and Lawson, 2019 ) were derived from persistence diagrams. Specifically, given an image, a segmentation algorithm was first implemented to obtain an image mask: a binary image in which each pixel is colored either white (if it part of the healthy skin) or black (if it is part of a lesion). Once the mask was applied to the original image, the RGB color space is transformed into an RGB, HSV, or XYZ color space and each channel was extracted. Persistent homology software was then used to compute persistence diagrams for each channel; from each diagram, persistence statistics and curves were computed as features. Finally, a multi-class SVM was used to classify the input into one of the seven types of skin lesions. When this approach was applied to a validation set of 5,000 images, the highest resulting accuracy scores were 65.6, 66, and 67.2%.
Similar persistent homology techniques were used to classify H&E stained stage T3 and stage T4 colorectal adenocarcinomas images as benign or malignant (Chittajallu et al., 2018 ). To do this, given an image, it was first color normalized (Reinhard et al., 2001 ) and the nuclear stain and minimum cross entropy thresholding (Li and Tam, 1998 ) for nuclear foreground segmentation were extracted using an unsupervised color deconvolution method (Macenko et al., 2009 ). Then, a fast difference-of-Gaussian implementation of the scale-adaptive Laplacian-of-Gaussian filter of Al-Kofahi et al. ( 2010 ) was performed to detect nuclei centroids. Then, by considering the set of nuclei centroids as a point cloud, the persistence diagram of its Vietoris-Rips filtration for the one dimensional homology groups (loops) was computed using a fast multiscale approach (Doyle et al., 2008 ). Then, persistence landscape (Bubenik, 2015 ) and image (Adams et al., 2017 ) representations were computed and used as features to characterize loops formed by glandular epithelial cell nuclei. Then given training images with benign/malignant labels, a random forest classifier was trained using these topological features. PCA was used to reduce the dimensionality of each feature group so as to preserve 99% of the variance. Hyperparameter optimization was also performed via cross-validation using a tree-structured parzen estimator (Bergstra et al., 2011 ). When this method was applied to testing data consisting of 80 images, an accuracy of 85%, AUC of 0.85, precision of 78%, and recall of 95% was obtained, an improvement over the traditional cell graph property approach in all areas (Doyle et al., 2008 ).
5. Disease Classification
Cancers of unknown primary represent 3–5% of all cancer cases, whereby physicians find one or multiple metastases but fail to locate the primary tumor. Pathologic evaluation of a metastatic biopsy often does not provide a definitive answer. Molecular data ranging from gene expression to somatic mutations have been shown to significantly aid classification of metastatic biopsies to their corresponding primary tumor site (Ferracin et al., 2011 ; Marquard et al., 2015 ; Vikeså et al., 2015 ; Moran et al., 2016 ; Søndergaard et al., 2017 ).
One study used persistent homology on 150 non-contrast-enhanced fat-suppressed 3D T1-weighted magnetic resonance (MR) images to classify hepatic tumors into three classes: hepatocellular carcinomas (HCC), metastatic tumors (MT), and hepatic hemangiomas (HH) (Oyama et al., 2019 ). To do this, for each image, a 3D region of interest (ROI) in the shape of a rectangular solid enclosing the entire lesion was created by an experienced radiologist. Then, gray-scale values of the voxels in each ROI were normalized and persistence diagrams were created for dimensions 0, 1, and 2 using HomCloud (Kimura et al., 2018 ; Obayashi and Hiraoka, 2018 ). These diagrams were vectorized into persistence images (Adams et al., 2015 ). Feature vectors were then obtained from these images and inputted into logistic regression with an elastic net penalty and extreme gradient boosting machine learning models for classification. The results from classification showed that dimension 1 persistence images had the highest accuracy rates: 85% for classifying HCC and MT, 84% for HCC and HH, and 74% for HH and MT.
An alternative method to accurately classify tumor subtypes is through the use of high throughput genomics (Nutt et al., 2003 ; Freije et al., 2004 ). Aiming to produce more robust algorithms than traditional classification methods, given gene expression profile data, researchers used statistical invariants and persistent homology to identify core patient groups associated with the classical, mesenchymal, and proneural subtypes of GBM and a compact set of genes most useful for this partitioning (Seemann et al., 2012 ). To do this, a sufficient, but compact, panel of genes to be used for clustering was predetermined using non-dimensionalized standard deviation (to ensure bimodality of gene expression distribution across patient samples; Phillips et al., 2006 ; Verhaak et al., 2010 ) and persistent homology (to find groups of genes whose expression levels change coherently among patient samples; Carlsson, 2009 ; Horak et al., 2009 ). Then, a hierarchical partitioning of patient samples based on gene expression levels is performed using persistent homology; specifically, samples are repeatedly bisected until further partitioning is not possible, thus obtaining the number of clusters that exists and some notion of genetic proximity of the clusters. Each bisection was implemented using 30 genes. A predictive model was then implemented to assign cancer subtypes to each cluster. Applying this approach to the 20 GBM test samples, fifteen predictions were in accordance with results from standard clustering calculations (Verhaak et al., 2010 ), five of which were unassigned by both algorithms. Of the remaining five samples, four were classified as “neural” by the clustering algorithm, but were unassigned by this approach since the neural group was not found in a single cluster.
Another example of the use of persistent and computational homology on gene expression data is in Arsuaga et al. ( 2012 ), whereby, upon application to a breast cancer gene expression dataset, the algorithm was able to distinguish among most breast cancer subtypes. This paper extended the work of DeWoskin et al. ( 2010 ) to gene expression data, under the assumption that gene expression is a measure of the underlying copy number changes (Neve et al., 2006 ; Horlings et al., 2010 ). Before applying the sliding window algorithm developed in DeWoskin et al. ( 2010 ) to gene expression data, theoretical work was done to show that under idealized conditions, the point cloud defined by the algorithm is a good representation of the original data. Hence, analysis of the point cloud is applicable to the original data set. This was done using Taken's embedding theorem, an extension of Whitney's embedding theorem to dynamical systems theory, and a circularization technique. To apply the sliding window algorithm to gene expression data, instead of pre-selecting differentially expressed genes like traditional clustering algorithms, all genes were ordered by their location in the genomes. Then, the sliding window algorithm was applied to generate point clouds, upon which topological and statistical analysis was performed. It was shown that when only β 0 was used, the algorithm could distinguish between less aggressive subtypes, like normal and luminal-A, and more aggressive ones, such as luminal B, basal-like, and Her2. It was also noted that the algorithm could not distinguish luminal B from Her2 and basal-like, implying the close similarities among these subtypes. Thus, it was noted that breast cancer subtypes can not only be classified by specific sets of genes, but also by certain global relationships among all genes.
6. Cellular Architecture
Imaging is an essential part of cancer clinical protocols, providing physicians with morphological, structural, and metabolic information about patient tumors, thereby assisting in clinical decision making and treatment planning (Fass, 2008 ). The development of new image segmentation tools (Zhang et al., 2001 ; Hong and Brady, 2003 ; Xiaohua et al., 2004 ) and quantitative multiplex immunofluorescence (Stack et al., 2014 ; Dimitriou et al., 2019 ; Abousamra et al., 2020 ) have set the stage for topological data analysis and persistent homology techniques to be harnessed for interpretation of high-dimensional information in histopathological imaging data.
One example of this is using persistent homology techniques to investigate architectural characteristics of cellular organization and nuclear arrangements from microarray tissue samples to distinguish among genetically derived breast cancer subtypes (Basal, Luminal A, Luminal B, and HER2; Singh et al., 2014 ). This was done through distinct topological characterizations such as nuclear connectivity (generators of zero dimensional homology groups) and loops (generators of one dimensional homology groups) based on Vietoris-Rips filtration of nuclei centers (Mischaikow and Nanda, 2013 ). When its performance was compared to a standard distance weighted discrimination classifier (Marron and Todd, 2007 ), nearly a four times improvement in classification accuracy was noted. Furthermore, for certain combinations of feature weightings, it was shown that topological features provide complementary information to patch based image appearance features. By using such topological features, they solve/address two main challenges in obtaining accurate cellular architectural characterization: the heterogeneity of spatial arrangements, both among patients and within single tumor samples, and differences in stain intensity which require manually determined phenotypic thresholds (Engers, 2007 ; Truesdale et al., 2011 ; Goodman et al., 2012 ; Helpap et al., 2012 ; Truong et al., 2013 ; Epstein et al., 2016 ; Evans et al., 2016 ). This improves performance over existing standard classifiers, which are more sensitive to noise, cannot model stain concentration variations, and have issues with larger cell arrangements (Aukerman et al., 2020 ).
In another paper, researchers used TDA to cluster prostate cancer histology into architectural groups consistent with the continuum of Gleason patterns, the most widely accepted system for evaluating prostate cancer architecture (Humphrey, 2004 ; Lawson et al., 2019 ). Persistent homology was used to compute persistence intensity diagrams (of zero and one dimensional components) of purely graded prostate cancer histopathology images of Gleason patterns 3–5. This revealed key insights into characteristics such as nuclei density, glandular shape, and inter-glandular arrangement. Furthermore, persistent homology was able to cluster these images into architectural groups through a rank descending persistence vector–the six resulting clusters provided a stable architectural continuum from well differentiated to poorly differentiated adenocarcinoma at an even finer level than the standard Gleason scale.
Persistent homology has also been used to characterize the spatial arrangement of immune and epithelial (tumor) cells within the breast cancer immune microenvironment from quantitative multiplex immunofluorescence (qmIF) imaging (Aukerman et al., 2020 ). Stain intensities and spatial coordinates of individual cells were collected from qmIF through nuclear segmentation, cytoplasmic definition, and stain quantification. In order to incorporate these stain intensities, instead of directly using a Rips or Cech filtration on the point cloud data (Chazal et al., 2009 ), a discretization process was first implemented to convert the point cloud data with stain intensity values into an image. Then, persistence diagrams were created from these images by using the opposite of the pixel stain intensity as the filter function. These diagrams were assessed as potential biomarkers of cancer subtype and prognostic biomarkers of overall survival using kernel mean embeddings (Gretton et al., 2012 ) with the sliced Wasserstein kernel (Carrière et al., 2017 ) and were shown to outperform the standard nearest neighbor analysis with a standard Gaussian kernel. Furthermore, a correlation analysis using constrained covariance (Herbrich et al., 2005 ) showed that the correlation between nearest neighbor and persistence diagrams were always <0.1, implying the features are nearly statistically independent and thus complementary.
7. Discussion
As we have seen in this paper, TDA has proven to be a powerful tool, yielding critical insights in the treatment prognosis, tumor segmentation and diagnosis, disease classification, and cellular architecture of cancer. But despite the many recent successes of TDA in the field of oncology, it is still a nascent field with much fruitful work yet to be done. Experimentally, to biologically validate the TDA methodology and results, it would be worth performing thorough studies to assess whether geometric and topological connectivity implies functional connectivity. Computationally, one area which deserves further exploration is the use of TDA to analyze time-series data (Ravishanker and Chen, 2019 ) in cancer. This has been done extensively in several other fields including climate analysis (Berwald et al., 2014 ), tracking stability of dynamical systems (Khasawneh and Munch, 2016 ), clustering populations of Tribolium flour beetles (Pereira and de Mello, 2015 ), analyzing motion sensor data during sports activities (Stolz et al., 2017 ), and financial time series data (Gidea, 2017 ; Truong, 2017 ; Gidea and Katz, 2018 ; Gidea et al., 2020 ). Though time series oncological data have been analyzed with varying degrees of success (Aoto et al., 2018 ; Kourou et al., 2020 ), TDA techniques of any sort have yet to be applied. Applying persistent homology techniques to time series microarray, cell anatomy imaging, or gene/pathway expression data, for example, may further help in disease classification, identifying intra-tumoral selection events, and contribute to a greater understanding of tumorigenesis. Another possible avenue of research is to investigate the process of angiogenesis, an inherently geometric and spatially dependent process, using persistent homology techniques. Specifically, we anticipate that TDA will help us understand the changes that occur in tumor vasculature morphology during cancer progression and under treatments. More importantly, we hope that connections between cancer vessel network and treatment prognosis can be found, such as by testing vessel normalization theory (Jain, 2005 ). In addition to the ideas presented above, it is worth noting that research into the use of TDA in oncology is sparse and, as such, there is much important and clinically relevant work to be done in simply applying well-understood persistent homology algorithms to broader classes of cancer data sets (note that most TDA analyses have been concentrated in just melanoma, brain, breast, and colorectal cancers) and in performing longitudinal studies across several cancer types.
Author Contributions
AB conceptualized the project and wrote the sections 3–7. AB and ID wrote the section 1. ID wrote the section 2. NA wrote the sections 1, 3, 4, and 5. All authors contributed to the article and approved the submitted version.
Conflict of Interest
The authors declare that the research was conducted in the absence of any commercial or financial relationships that could be construed as a potential conflict of interest.
Acknowledgments
The authors would like to thank Ethan Rooke and Hind Benmerabet for their insightful comments on a draft of this manuscript.
1 While the intuitive definition will suffice for this paper, we have left out a number of details. For example if we use addition with ℤ 2 coefficients, we can detect the Klein bottle surface (β 2 = 1), while if we use ℤ coefficients, β 2 = 0 since the Klein bottle does not bound a void. For computational speed, ℤ 2 coefficients are frequently used when computing persistent homology.
Funding. AB was supported by the National Science Foundation Graduate Research Fellowship Program under Grant No. 1746051. Any opinions, findings, and conclusions or recommendations expressed in this material are those of the author and do not necessarily reflect the views of the National Science Foundation.
- Abousamra S., Fassler D., Hou L., Zhang Y., Gupta R., Kurc T., et al.. (2020). “Weakly-supervised deep stain decomposition for multiplex IHC images,” in 2020 IEEE 17th International Symposium on Biomedical Imaging (ISBI) , 481–485. 10.1109/ISBI45749.2020.9098652 [ CrossRef ] [ Google Scholar ]
- Adams H., Chepushtanova S., Emerson T., Hanson E., Kirby M., Motta F., et al.. (2015). Persistence images: a stable vector representation of persistent homology . J. Mach. Learn. Res . 18 , 1–35. Available online at: http://jmlr.org/papers/v18/16-337.html [ Google Scholar ]
- Adams H., Emerson T., Kirby M., Neville R., Peterson C., Shipman P., et al.. (2017). Persistence images: a stable vector representation of persistent homology . J. Mach. Learn. Res . 18 , 1–35. Available online at: http://jmlr.org/papers/v18/16-337.html [ Google Scholar ]
- Aiello M., Cavaliere C., D'Albore A., Salvatore M. (2019). The challenges of diagnostic imaging in the era of big data . J. Clin. Med . 8 :316. 10.3390/jcm8030316 [ PMC free article ] [ PubMed ] [ CrossRef ] [ Google Scholar ]
- Al-Kofahi Y., Lassoued W., Lee W., Roysam B. (2010). Improved automatic detection and segmentation of cell nuclei in histopathology images . IEEE Trans. Bio-Med. Eng . 57 , 841–852. 10.1109/TBME.2009.2035102 [ PubMed ] [ CrossRef ] [ Google Scholar ]
- Almendro V., Cheng Y. K., Randles A., Itzkovitz S., Marusyk A., Ametller E., et al.. (2014). Inference of tumor evolution during chemotherapy by computational modeling and in situ analysis of genetic and phenotypic cellular diversity . Cell Rep . 6 , 514–527. 10.1016/j.celrep.2013.12.041 [ PMC free article ] [ PubMed ] [ CrossRef ] [ Google Scholar ]
- Alyass A., Turcotte M., Meyre D. (2015). From big data analysis to personalized medicine for all: challenges and opportunities . BMC Med. Genomics 8 :33. 10.1186/s12920-015-0108-y [ PMC free article ] [ PubMed ] [ CrossRef ] [ Google Scholar ]
- Andor N., Graham T. A., Jansen M., Xia L. C., Aktipis C. A., Petritsch C., et al.. (2016). Pan-cancer analysis of the extent and consequences of intratumor heterogeneity . Nat. Med . 22 , 105–113. 10.1038/nm.3984 [ PMC free article ] [ PubMed ] [ CrossRef ] [ Google Scholar ]
- Aoto Y., Okumura K., Hachiya T., Hase S., Wakabayashi Y., Ishikawa F., et al.. (2018). Time-series analysis of tumorigenesis in a murine skin carcinogenesis model . Sci. Rep . 8 :12994. 10.1038/s41598-018-31349-x [ PMC free article ] [ PubMed ] [ CrossRef ] [ Google Scholar ]
- Aparicio S., Caldas C. (2013). The implications of clonal genome evolution for cancer medicine . N. Engl. J. Med . 368 , 842–851. 10.1056/NEJMra1204892 [ PubMed ] [ CrossRef ] [ Google Scholar ]
- Arsuaga J., Baas N. A., Daniel DeWoskin Mizuno H., Pankov A., Park C. (2012). Topological analysis of gene expression arrays identifies high risk molecular subtypes in breast cancer . Applicable Algebra in Engineering, Communications and Comput . 23 , 3–15. 10.1007/s00200-012-0166-8 [ CrossRef ] [ Google Scholar ]
- Aukerman A., Carriére M., Chen C., Gardner K., Rabadán R., Vanguri R. (2020). “Persistent homology based characterization of the breast cancer immune microenvironment: a feasibility study,” in 36th International Symposium on Computational Geometry, Vol. 11 (Dagstuhl: ), 1–11. [ Google Scholar ]
- Bauer U. (2019). Ripser: efficient computation of Vietoris-Rips persistence barcodes . arXiv: 1908.02518v1 . [ Google Scholar ]
- Bauer U., Lesnick M. (2014). “Induced matchings of barcodes and the algebraic stability of persistence,” in Computational Geometry (SoCG'14) (New York, NY: ACM; ), 355–364. 10.1145/2582112.2582168 [ CrossRef ] [ Google Scholar ]
- Bendich P., Marron J. S., Miller E., Pieloch A., Skwerer S. (2016). Persistent homology analysis of brain artery trees . Ann. Appl. Stat . 10 , 198–218. 10.1214/15-AOAS886 [ PMC free article ] [ PubMed ] [ CrossRef ] [ Google Scholar ]
- Bergstra J., Bardenet R., Bengio Y., Kégl B. (2011). Algorithms for hyper-parameter optimization . Adv. Neural Inform. Process. Syst . 24 , 1–9. Available online at: https://proceedings.neurips.cc/paper/2011/file/86e8f7ab32cfd12577bc2619bc635690-Paper.pdf [ Google Scholar ]
- Berwald J. J., Gidea M., Vejdemo-Johansson M. (2014). Automatic recognition and tagging of topologically different regimes in dynamical systems . Discont. Nonlin. Complex . 3 , 413–426. 10.5890/DNC.2014.12.004 [ CrossRef ] [ Google Scholar ]
- Boissonnat J.-D., Pritam S. (2020). “Edge collapse and persistence of flag complexes,” in 36th International Symposium on Computational Geometry (SoCG 2020), Vol. 164 of Leibniz International Proceedings in Informatics (LIPIcs) , eds S. Cabello and D. Z. Chen (Dagstuhl: Schloss Dagstuhl-Leibniz-Zentrum für Informatik; ), 19 :1–19:15. [ Google Scholar ]
- Bubenik P. (2015). Statistical topological data analysis using persistence landscapes . J. Mach. Learn. Res . 16 , 77–102. Available online at: http://jmlr.org/papers/v16/bubenik15a.html [ Google Scholar ]
- Bubenik P. (2020). “The persistence landscape and some of its properties,” in Topological Data Analysis , eds N. Baas, G. Carlsson, G. Quick, M. Szymik, M. Thaule (Geiranger: Springer; ), 97–117. 10.1007/978-3-030-43408-3_4 [ CrossRef ] [ Google Scholar ]
- Bubenik P., Hull M., Patel D., Whittle B. (2020). Persistent homology detects curvature . Inverse Probl . 36 :025008. 10.1088/1361-6420/ab4ac0 [ CrossRef ] [ Google Scholar ]
- Bubenik P., Scott J. A. (2014). Categorification of persistent homology . Discrete Comput. Geom . 51 , 600–627. 10.1007/s00454-014-9573-x [ CrossRef ] [ Google Scholar ]
- Bukkuri A. (2020). Optimal control analysis of combined chemotherapy-immunotherapy treatment regimens in a PKPD cancer evolution model . Biomath 9 , 1–12. 10.11145/j.biomath.2020.02.137 [ CrossRef ] [ Google Scholar ]
- Camara P. G., Rosenbloom D. I., Emmett K. J., Levine A. J., Rabadan R. (2016). Topological data analysis generates high-resolution, genome-wide maps of human recombination . Cell Syst . 3 , 83–94. 10.1016/j.cels.2016.05.008 [ PMC free article ] [ PubMed ] [ CrossRef ] [ Google Scholar ]
- Carlsson G. (2009). Topology and data . Bull. Am. Math. Soc . 46 , 255–308. 10.1090/S0273-0979-09-01249-X [ CrossRef ] [ Google Scholar ]
- Carlsson G., Zomorodian A., Collins A., Guibas L. J. (2005). Persistence barcodes for shapes . Int. J. Shape Model . 11 , 149–187. 10.1142/S0218654305000761 [ CrossRef ] [ Google Scholar ]
- Carriére M., Cuturi M., Oudot S. (2017). “Sliced Wasserstein kernel for persistence diagrams,” in Proceedings of Machine Learning Research (Sydney, NSW: ). [ Google Scholar ]
- Chazal F., Cohen-Steiner D., Glisse M., Guibas L., Oudot S. (2009). “Proximity of persistence modules and their diagrams,” in Proceedings of the Twenty-Fifth Annual Symposium on Computational Geometry (Aarhus: ACM; ), 237–246. 10.1145/1542362.1542407 [ CrossRef ] [ Google Scholar ]
- Chazal F., de Silva V., Glisse M., Oudot S. (2016). The Structure and Stability of Persistence Modules . SpringerBriefs in Mathematics. Cham: Springer. 10.1007/978-3-319-42545-0_2 [ CrossRef ] [ Google Scholar ]
- Chazal F., Fasy B., Lecci F., Michel B., Rinaldo A., Wasserman L. (2017). Robust topological inference: distance to a measure and kernel distance . J. Mach. Learn. Res . 18 :40. Available online at: http://jmlr.org/papers/v18/15-484.html [ Google Scholar ]
- Chittajallu D. R., Siekierski N., Lee S., Gerber S., Beezley J., Manthey D., et al.. (2018). “Vectorized persistent homology representations for characterizing glandular architecture in histology images,” in 2018 IEEE 15th International Symposium on Biomedical Imaging (Washington, DC: ). 10.1109/ISBI.2018.8363562 [ CrossRef ] [ Google Scholar ]
- Chung Y.-M., Hu C.-S., Lawson A., Smyth C. (2018). “Topological approaches to skin disease image analysis,” in IEEE International Conference on Big Data (Big Data) (Seattle, WA: ), 100–105. 10.1109/BigData.2018.8622175 [ CrossRef ] [ Google Scholar ]
- Chung Y.-M., Lawson A. (2019). Persistence curves: a canonical framework for summarizing persistence diagrams . arXiv: 1904.07768 . [ Google Scholar ]
- Climent J., Dimitrow P., Fridlyand J., Palacios J., Siebert R., Albertson D. G., et al.. (2007). Deletion of chromosome 11q predicts response to anthracycline-based chemotherapy in early breast cancer . Cancer Res . 67 , 818–826. 10.1158/0008-5472.CAN-06-3307 [ PubMed ] [ CrossRef ] [ Google Scholar ]
- Cohen-Steiner D., Edelsbrunner H., Harer J. (2007). Stability of persistence diagrams . Discrete Comput. Geom . 37 , 103–120. 10.1007/s00454-006-1276-5 [ CrossRef ] [ Google Scholar ]
- Crawford L., Monod A., Chen A. X., Mukherjee S., Rabadán R. (2020). Predicting clinical outcomes in glioblastoma: an application of topological and functional data analysis . J. Am. Stat. Assoc . 115 , 1139–1150. 10.1080/01621459.2019.1671198 [ CrossRef ] [ Google Scholar ]
- De Silva V., Carlsson G. (2004). “Topological estimation using witness complexes,” in Eurographics Symposium on Point-Based Graphics (Zurich: ), 157–166. 10.2312/SPBG/SPBG04/157-166 [ CrossRef ] [ Google Scholar ]
- Dequeant M.-L., Ahnert S., Edelsbrunner H., Fink T. M., Glynn E. F., Hattem G., et al.. (2008). Comparison of pattern detection methods in microarray time series of the segmentation clock . PLoS ONE 3 :e2856. 10.1371/journal.pone.0002856 [ PMC free article ] [ PubMed ] [ CrossRef ] [ Google Scholar ]
- DeWoskin D., Climent J., Cruz-White I., Vazquez M., Park C., Arsuaga J. (2010). Applications of computational homology to the analysis of treatment response in breast cancer patients . Topol. Appl . 157 , 157–164. 10.1016/j.topol.2009.04.036 [ CrossRef ] [ Google Scholar ]
- Dilsizian S. E., Siegel E. L. (2014). Artificial intelligence in medicine and cardiac imaging: harnessing big data and advanced computing to provide personalized medical diagnosis and treatment . Curr. Cardiol. Rep . 16 :441. 10.1007/s11886-013-0441-8 [ PubMed ] [ CrossRef ] [ Google Scholar ]
- Dimitriou N., Arandjelović O., Caie P. D. (2019). Deep learning for whole slide image analysis: an overview . Front. Med . 6 :264. 10.3389/fmed.2019.00264 [ PMC free article ] [ PubMed ] [ CrossRef ] [ Google Scholar ]
- Doyle S., Agner S., Madabhushi A., Feldman M., Tomaszewski J. (2008). “Automated grading of breast cancer histopathology using spectral clustering with textural and architectural image features,” in 5th IEEE International Symposium on Biomedical Imaging: From Nano to Macro (Paris: ), 496–499. 10.1109/ISBI.2008.4541041 [ CrossRef ] [ Google Scholar ]
- Edelsbrunner H., Harer J. (2010). Computational Topology: An Introduction . Providence, RI: American Mathematical Society. 10.1090/mbk/069 [ CrossRef ] [ Google Scholar ]
- Edelsbrunner H., Letscher D., Zomorodian A. (2002). Topological persistence and simplification . Discr. Comput. Geom . 28 , 511–533. 10.1007/s00454-002-2885-2 [ CrossRef ] [ Google Scholar ]
- Emmett K., Schweinhart B., Rabadan R. (2016). “Multiscale topology of chromatin folding,” in Proceedings of the 9th EAI Conference on Bio-inspired Information and Communications Technologies (New York, NY: ), 177–180. 10.4108/eai.3-12-2015.2262453 [ CrossRef ] [ Google Scholar ]
- Engers R. (2007). Reproducibility and reliability of tumor grading in urological neoplasms . World J. Urol . 25 , 595–605. 10.1007/s00345-007-0209-0 [ PubMed ] [ CrossRef ] [ Google Scholar ]
- Epstein J. I., Zelefsky M. J., Sjoberg D. D., Nelson J. B., Egevad L., Magi-Galluzzi C., et al.. (2016). A contemporary prostate cancer grading system: a validated alternative to the Gleason score . Eur. Urol . 69 , 428–435. 10.1016/j.eururo.2015.06.046 [ PMC free article ] [ PubMed ] [ CrossRef ] [ Google Scholar ]
- Evans S. M., Patabendi Bandarage V., Kronborg C., Earnest A., Millar J., Clouston D. (2016). Gleason group concordance between biopsy and radical prostatectomy specimens: a cohort study from Prostate Cancer Outcome Registry-Victoria . Prost. Int . 4 , 145–151. 10.1016/j.prnil.2016.07.004 [ PMC free article ] [ PubMed ] [ CrossRef ] [ Google Scholar ]
- Fass L. (2008). Imaging and cancer: a review . Mol. Oncol . 2 , 115–152. 10.1016/j.molonc.2008.04.001 [ PMC free article ] [ PubMed ] [ CrossRef ] [ Google Scholar ]
- Ferracin M., Pedriali M., Veronese A., Zagatti B., Gafá R., Magri E., et al.. (2011). MicroRNA profiling for the identification of cancers with unknown primary tissue-of-origin . J. Pathol . 225 , 43–53. 10.1002/path.2915 [ PMC free article ] [ PubMed ] [ CrossRef ] [ Google Scholar ]
- Freije W. A., Castro-Vargas F. E., Fang Z., Horvath S., Cloughesy T., Liau L. M., et al.. (2004). Gene expression profiling of gliomas strongly predicts survival . Cancer Res . 64 , 6503–6510. 10.1158/0008-5472.CAN-04-0452 [ PubMed ] [ CrossRef ] [ Google Scholar ]
- Garside K., Henderson R., Makarenko I., Masoller C. (2019). Topological data analysis of high resolution diabetic retinopathy images . PLoS ONE 14 :e217413. 10.1371/journal.pone.0217413 [ PMC free article ] [ PubMed ] [ CrossRef ] [ Google Scholar ]
- Ghrist R. (2008). Barcodes: the persistent topology of data . Bull. Am. Math. Soc . 45 , 61–75. 10.1090/S0273-0979-07-01191-3 [ CrossRef ] [ Google Scholar ]
- Ghrist R. W. (2014). Elementary Applied Topology, Vol. 1 . Createspace Seattle. [ Google Scholar ]
- Gidea M. (2017). “Topology data analysis of critical transitions in financial networks,” in 3rd International Winter School and Conference on Network Science (Tel Aviv: ), 47–59. 10.1007/978-3-319-55471-6_5 [ CrossRef ] [ Google Scholar ]
- Gidea M., Goldsmith D., Katz Y., Roldan P., Shmalo Y. (2020). Topological recognition of critical transitions in time series of cryptocurrencies . Phys. A 548 :123843. 10.1016/j.physa.2019.123843 [ CrossRef ] [ Google Scholar ]
- Gidea M., Katz Y. (2018). Topological data analysis of financial time series: landscapes of crashes . Phys. A 491 , 820–834. 10.1016/j.physa.2017.09.028 [ CrossRef ] [ Google Scholar ]
- Goodman M., Ward K. C., Osunkoya A. O., Datta M. W., Luthringer D., Young A. N., et al.. (2012). Frequency and determinants of disagreement and error in gleason scores: a population-based study of prostate cancer . Prostate 72 , 1389–1398. 10.1002/pros.22484 [ PMC free article ] [ PubMed ] [ CrossRef ] [ Google Scholar ]
- Gretton A., Borgwardt K. M., Rasch M. J., Smola A., Schölkopf B., Smola Gretton A. (2012). A kernel two-sample test . J. Mach. Learn. Res . 13 , 723–773. Available online at: http://jmlr.org/papers/v13/gretton12a.html [ Google Scholar ]
- Gu J., Taylor C. R. (2014). Practicing pathology in the era of big data and personalized medicine . Appl. Immunohistochem. Mol. Morphol . 22 , 1–9. 10.1097/PAI.0000000000000022 [ PMC free article ] [ PubMed ] [ CrossRef ] [ Google Scholar ]
- Guan Y., Stephens M. (2011). Bayesian variable selection regression for genome-wide association studies and other large-scale problems . Ann. Appl. Stat . 5 , 1780–1815. 10.1214/11-AOAS455 [ CrossRef ] [ Google Scholar ]
- Han W., Han M. R., Kang J. J., Bae J. Y., Lee J. H., Bae Y. J., et al.. (2006). Genomic alterations identified by array comparative genomic hybridization as prognostic markers in tamoxifen-treated estrogen receptor-positive breast cancer . BMC Cancer 6 :92. 10.1186/1471-2407-6-92 [ PMC free article ] [ PubMed ] [ CrossRef ] [ Google Scholar ]
- Hanahan D., Weinberg R. A. (2000). The Hallmarks of Cancer . Technical report. 100 , 57–70. 10.1016/S0092-8674(00)81683-9 [ PubMed ] [ CrossRef ] [ Google Scholar ]
- Hatcher A. (2002). Algebraic Topology . Cambridge: Cambridge University Press. [ Google Scholar ]
- Helpap B., Kristiansen G., Beer M., Köllermann J., Oehler U., Pogrebniak A., et al.. (2012). Improving the reproducibility of the gleason scores in small foci of prostate cancer - Suggestion of diagnostic criteria for glandular fusion . Pathol. Oncol. Res . 18 , 615–621. 10.1007/s12253-011-9484-6 [ PubMed ] [ CrossRef ] [ Google Scholar ]
- Herbrich R., Smola A., Bousquet O., Schölkopf Bernhardschoelkopf B., Gretton A., Schölkopf Gretton B. (2005). Kernel methods for measuring independence . J. Mach. Learn. Res . 6 , 2075–2129. Available online at: http://jmlr.org/papers/v6/gretton05a.html [ Google Scholar ]
- Hong B.-W., Brady M. (2003). “A topographic representation for mammogram segmentation,” in International Conference on Medical Image Computing and Computer-Assisted Intervention (Montreal, QC: ), 730–737. 10.1007/978-3-540-39903-2_89 [ CrossRef ] [ Google Scholar ]
- Horak D., Maletić S., Rajković M. (2009). Persistent homology of complex networks . J. Stat. Mech . 2009 :P03034. 10.1088/1742-5468/2009/03/P03034 [ CrossRef ] [ Google Scholar ]
- Horlings H. M., Lai C., Nuyten D. S., Halfwerk H., Kristel P., Van Beers E., et al.. (2010). Integration of DNA copy number alterations and prognostic gene expression signatures in breast cancer patients . Clin. Cancer Res . 16 , 651–663. 10.1158/1078-0432.CCR-09-0709 [ PubMed ] [ CrossRef ] [ Google Scholar ]
- Humphrey P. A. (2004). Gleason grading and prognostic factors in carcinoma of the prostate . Modern Pathol . 17 , 292–306. 10.1038/modpathol.3800054 [ PubMed ] [ CrossRef ] [ Google Scholar ]
- Ishwaran H., Rao J. S. (2005). Spike and slab variable selection: frequentist and bayesian strategies . Ann. Stat . 33 , 730–773. 10.1214/009053604000001147 [ CrossRef ] [ Google Scholar ]
- Jain R. K. (2005). Normalization of tumor vasculature: an emerging concept in antiangiogenic therapy . Sci. Rev . 307 , 58–62. 10.1126/science.1104819 [ PubMed ] [ CrossRef ] [ Google Scholar ]
- Khan A., El-Daly H., Simmons E., Rajpoot N. (2013). HyMaP: A hybrid magnitude-phase approach to unsupervised segmentation of tumor areas in breast cancer histology images . J. Pathol. Inform . 4 ( Suppl ):S1. 10.4103/2153-3539.109802 [ PMC free article ] [ PubMed ] [ CrossRef ] [ Google Scholar ]
- Khasawneh F. A., Munch E. (2016). Chatter detection in turning using persistent homology . Mech. Syst. Signal Process . 70–71, 527–541. 10.1016/j.ymssp.2015.09.046 [ CrossRef ] [ Google Scholar ]
- Kimura M., Obayashi I., Takeichi Y., Murao R., Hiraoka Y. (2018). Non-empirical identification of trigger sites in heterogeneous processes using persistent homology . Sci. Rep . 8 :3553. 10.1038/s41598-018-21867-z [ PMC free article ] [ PubMed ] [ CrossRef ] [ Google Scholar ]
- Kourou K., Rigas G., Papaloukas C., Mitsis M., Fotiadis D. I. (2020). Cancer classification from time series microarray data through regulatory Dynamic Bayesian Networks . Comput. Biol. Med . 116 :103577. 10.1016/j.compbiomed.2019.103577 [ PubMed ] [ CrossRef ] [ Google Scholar ]
- Kusano G., Hiraoka Y., Fukumizu K. (2016). “Persistence weighted Gaussian kernel for topological data analysis,” in International Conference on Machine Learning (New York, NY: ), 2004–2013. [ Google Scholar ]
- Laurie C. C., Laurie C. A., Rice K., Doheny K. F., Zelnick L. R., McHugh C. P., et al.. (2012). Detectable clonal mosaicism from birth to old age and its relationship to cancer . Nat. Genet . 44 , 642–650. 10.1038/ng.2271 [ PMC free article ] [ PubMed ] [ CrossRef ] [ Google Scholar ]
- Lawson P., Sholl A. B., Brown J. Q., Fasy B. T., Wenk C. (2019). Persistent homology for the quantitative evaluation of architectural features in prostate cancer histology . Sci. Rep . 9 :1139. 10.1038/s41598-018-36798-y [ PMC free article ] [ PubMed ] [ CrossRef ] [ Google Scholar ]
- Li C. H., Tam P. K. (1998). An iterative algorithm for minimum cross entropy thresholding . Pattern Recogn. Lett . 19 , 771–776. 10.1016/S0167-8655(98)00057-9 [ CrossRef ] [ Google Scholar ]
- Li M., An H., Angelovici R., Bagaza C., Batushansky A., Clark L., Coneva V., et al.. (2018). Topological data analysis as a morphometric method: Using persistent homology to demarcate a leaf morphospace . Front. Plant Sci . 9 :553. 10.3389/fpls.2018.00553 [ PMC free article ] [ PubMed ] [ CrossRef ] [ Google Scholar ]
- Lockwood S., Krishnamoorthy B. (2015). “Topological features in cancer gene expression data,” in Pacific Symposium on Biocomputing (Kohala Coast: ). [ PubMed ] [ Google Scholar ]
- Macenko M., Niethammer M., Marron J., Borland D., Woosley J. T., Guan X. (2009). “A method for normalizing histology slides for quantitative analysis,” in IEEE International Symposium on Biomedical Imaging: From Nano to Macro (Boston, MA: ), 1107–1110. 10.1109/ISBI.2009.5193250 [ CrossRef ] [ Google Scholar ]
- Maley C. C., Galipeau P. C., Finley J. C., Wongsurawat V. J., Li X., Sanchez C. A., et al.. (2006). Genetic clonal diversity predicts progression to esophageal adenocarcinoma . Nat. Genet . 38 , 468–473. 10.1038/ng1768 [ PubMed ] [ CrossRef ] [ Google Scholar ]
- Maley C. C., Reid B. J. (2005). Natural selection in neoplastic progression of Barrett's esophagus . Semin. Cancer Biol . 15 , 474–483. 10.1016/j.semcancer.2005.06.004 [ PubMed ] [ CrossRef ] [ Google Scholar ]
- Marquard A. M., Birkbak N. J., Thomas C. E., Favero F., Krzystanek M., Lefebvre C., et al.. (2015). TumorTracer: a method to identify the tissue of origin from the somatic mutations of a tumor specimen . BMC Med. Genomics 8 :58. 10.1186/s12920-015-0130-0 [ PMC free article ] [ PubMed ] [ CrossRef ] [ Google Scholar ]
- Marron J. S., Todd M. (2007). Distance-weighted discrimination . J. Am. Stat. Assoc . 102 , 1267–1271. 10.1198/016214507000001120 [ PMC free article ] [ PubMed ] [ CrossRef ] [ Google Scholar ]
- Mileyko Y., Mukherjee S., Harer J. (2011). Probability measures on the space of persistence diagrams . Inverse Probl . 27 :124007. 10.1088/0266-5611/27/12/124007 [ CrossRef ] [ Google Scholar ]
- Mischaikow K., Nanda V. (2013). Morse theory for filtrations and efficient computation of persistent homology . Discr. Comput. Geom . 50 , 330–353. 10.1007/s00454-013-9529-6 [ CrossRef ] [ Google Scholar ]
- Moran S., Martínez-Cardús A., Sayols S., Musulén E., Bala ná C., Estival-Gonzalez A., et al.. (2016). Epigenetic profiling to classify cancer of unknown primary: a multicentre, retrospective analysis . Lancet Oncol . 17 , 1386–1395. 10.1016/S1470-2045(16)30297-2 [ PubMed ] [ CrossRef ] [ Google Scholar ]
- Munkres J. R. (1984). Elements of Algebraic Topology . Menlo Park, CA: Addison-Wesley Publishing Company. [ Google Scholar ]
- Neve R. M., Chin K., Fridlyand J., Yeh J., Baehner F. L., Fevr T., et al.. (2006). A collection of breast cancer cell lines for the study of functionally distinct cancer subtypes . Cancer Cell 10 , 515–527. 10.1016/j.ccr.2006.10.008 [ PMC free article ] [ PubMed ] [ CrossRef ] [ Google Scholar ]
- Nicolau M., Levine A. J., Carlsson G. (2011). Topology based data analysis identifies a subgroup of breast cancers with a unique mutational profile and excellent survival . Proc. Natl. Acad. Sci. U.S.A . 108 , 7265–7270. 10.1073/pnas.1102826108 [ PMC free article ] [ PubMed ] [ CrossRef ] [ Google Scholar ]
- Nicolau M., Tibshirani R., Børresen-Dale A. L., Jeffrey S. S. (2007). Disease-specific genomic analysis: identifying the signature of pathologic biology . Bioinformatics 23 , 957–965. 10.1093/bioinformatics/btm033 [ PubMed ] [ CrossRef ] [ Google Scholar ]
- Nielson J. L., Cooper S. R., Yue J. K., Sorani M. D., Inoue T., Yuh E. L., et al.. (2017). Uncovering precision phenotype-biomarker associations in traumatic brain injury using topological data analysis . PLoS ONE 12 :e169490. 10.1371/journal.pone.0169490 [ PMC free article ] [ PubMed ] [ CrossRef ] [ Google Scholar ]
- Nutt C. L., Mani D. R., Betensky R. A., Tamayo P., Cairncross J. G., Ladd C., et al.. (2003). Gene expression-based classification of malignant gliomas correlates better with survival than histological classification . Cancer Res . 63 , 1602–1607. [ PubMed ] [ Google Scholar ]
- Obayashi I., Hiraoka Y. (2018). Persistence diagrams with linear machine learning models . J. Appl. Comput. Topol . 1 , 421–449. 10.1007/s41468-018-0013-5 [ CrossRef ] [ Google Scholar ]
- Otter N., Porter M. A., Tillmann U., Grindrod P., Harrington H. A. (2017). A roadmap for the computation of persistent homology . EPJ Data Sci . 6 :17. 10.1140/epjds/s13688-017-0109-5 [ PMC free article ] [ PubMed ] [ CrossRef ] [ Google Scholar ]
- Oudot S. Y. (2015). Persistence Theory: From Quiver Representations to Data Analysis, Vol. 209 of Mathematical Surveys and Monographs . Providence, RI: American Mathematical Society. 10.1090/surv/209 [ CrossRef ] [ Google Scholar ]
- Oyama A., Hiraoka Y., Obayashi I., Saikawa Y., Furui S., Shiraishi K., et al.. (2019). Hepatic tumor classification using texture and topology analysis of non-contrast-enhanced three-dimensional T1-weighted MR images with a radiomics approach . Sci. Rep . 9 :8764. 10.1038/s41598-019-45283-z [ PMC free article ] [ PubMed ] [ CrossRef ] [ Google Scholar ]
- Pereira C. M., de Mello R. F. (2015). Persistent homology for time series and spatial data clustering . Expert Syst. Appl . 42 , 6026–6038. 10.1016/j.eswa.2015.04.010 [ CrossRef ] [ Google Scholar ]
- Phillips H. S., Kharbanda S., Chen R., Forrest W. F., Soriano R. H., Wu T. D., et al.. (2006). Molecular subclasses of high-grade glioma predict prognosis, delineate a pattern of disease progression, and resemble stages in neurogenesis . Cancer Cell 9 , 157–173. 10.1016/j.ccr.2006.02.019 [ PubMed ] [ CrossRef ] [ Google Scholar ]
- Qaiser T., Sirinukunwattana K., Nakane K., Tsang Y. W., Epstein D., Rajpoot N. (2016). “Persistent homology for fast tumor segmentation in whole slide histology images,” in Procedia Computer Science, Vol. 90 (Loughborough: Elsevier B.V.), 119–124. 10.1016/j.procs.2016.07.033 [ CrossRef ] [ Google Scholar ]
- Rabadán R., Mohamedi Y., Rubin U., Chu T., Alghalith A. N., Elliott O., et al.. (2020). Identification of relevant genetic alterations in cancer using topological data analysis . Nat. Commun . 11 , 1–10. 10.1101/2020.01.30.922310 [ PMC free article ] [ PubMed ] [ CrossRef ] [ Google Scholar ]
- Ravishanker N., Chen R. (2019). Topological data analysis (TDA) for time series . arXiv: 1909.10604 . [ Google Scholar ]
- Reinhard E., Ashikhmin M., Gooch B., Shirley P. (2001). Color transfer between images . IEEE Comput. Graph. Appl . 21 , 34–41. 10.1109/38.946629 [ CrossRef ] [ Google Scholar ]
- Reininghaus J., Huber S., Bauer U., Kwitt R. (2015). “A stable multi-scale kernel for topological machine learning,” in Proceedings of the IEEE Conference on Computer Vision and Pattern Recognition (Boston, MA: ), 4741–4748. 10.1109/CVPR.2015.7299106 [ CrossRef ] [ Google Scholar ]
- Reuter J. A., Spacek D. V., Snyder M. P. (2015). High-throughput sequencing technologies . Mol. Cell 58 , 586–597. 10.1016/j.molcel.2015.05.004 [ PMC free article ] [ PubMed ] [ CrossRef ] [ Google Scholar ]
- Roychowdhury S., Iyer M. K., Robinson D. R., Lonigro R. J., Wu Y. M., Cao X., et al.. (2011). Personalized oncology through integrative high-throughput sequencing: a pilot study . Sci. Transl. Med . 3 , 1–12. 10.1126/scitranslmed.3003161 [ PMC free article ] [ PubMed ] [ CrossRef ] [ Google Scholar ]
- Rucco M., Merelli E., Herman D., Ramanan D., Petrossian T., Falsetti L., et al.. (2015). Using Topological Data Analysis for diagnosis pulmonary embolism . arXiv:1409.5020v1 . 9 , 41–55. [ Google Scholar ]
- Seemann L., Shulman J., Gunaratne G. H. (2012). A robust topology-based algorithm for gene expression profiling . ISRN Bioinform . 2012 :381023. 10.5402/2012/381023 [ PMC free article ] [ PubMed ] [ CrossRef ] [ Google Scholar ]
- Siddiqui S., Shikotra A., Richardson M., Doran E., Choy D., Bell A., et al.. (2018). Airway pathological heterogeneity in asthma: visualization of disease microclusters using topological data analysis . J. Aller. Clin. Immunol . 142 , 1457–1468. 10.1016/j.jaci.2017.12.982 [ PubMed ] [ CrossRef ] [ Google Scholar ]
- Singh G., Mémoli F., Carlsson G. (2007). “Topological methods for the analysis of high dimensional data sets and 3D object recognition,” in Eurographics Symposium on Point-Based Graphics (Prague: ). [ Google Scholar ]
- Singh N., Couture H. D., Marron J. S., Perou C., Niethammer M. (2014). “Topological descriptors of histology images,” in Machine Learning in Medical Imaging (Boston, MA: ). 10.1007/978-3-319-10581-9_29 [ CrossRef ] [ Google Scholar ]
- Søndergaard D., Nielsen S., Pedersen C. N., Besenbacher S. (2017). Prediction of primary tumors in cancers of unknown primary . J. Integr. Bioinform . 14 :20170013. 10.1515/jib-2017-0013 [ PMC free article ] [ PubMed ] [ CrossRef ] [ Google Scholar ]
- Stack E. C., Wang C., Roman K. A., Hoyt C. C. (2014). Multiplexed immunohistochemistry, imaging, and quantitation: a review, with an assessment of Tyramide signal amplification, multispectral imaging and multiplex analysis . Methods 70 , 46–58. 10.1016/j.ymeth.2014.08.016 [ PubMed ] [ CrossRef ] [ Google Scholar ]
- Stolz B. J., Harrington H. A., Porter M. A. (2017). Persistent homology of time-dependent functional networks constructed from coupled time series . Chaos 27 :047410. 10.1063/1.4978997 [ PubMed ] [ CrossRef ] [ Google Scholar ]
- Suwinski P., Ong C. K., Ling M. H., Poh Y. M., Khan A. M., Ong H. S. (2019). Advancing personalized medicine through the application of whole exome sequencing and big data analytics . Front. Genet . 10 :49. 10.3389/fgene.2019.00049 [ PMC free article ] [ PubMed ] [ CrossRef ] [ Google Scholar ]
- Tahmassebi A., Schulte M. H., Gandomi A. H., Goudriaan A. E., McCann I., Meyer-Baese A. (2018). “Deep learning in medical imaging: FMRI big data analysis via convolutional neural networks,” in ACM International Conference Proceeding Series (Pittsburgh, PA: Association for Computing Machinery; ), 1–4. 10.1145/3219104.3229250 [ CrossRef ] [ Google Scholar ]
- Truesdale M. D., Cheetham P. J., Turk A. T., Sartori S., Hruby G. W., Dinneen E. P., et al.. (2011). Gleason score concordance on biopsy-confirmed prostate cancer: is pathological re-evaluation necessary prior to radical prostatectomy? BJU Int . 107 , 749–754. 10.1111/j.1464-410X.2010.09570.x [ PubMed ] [ CrossRef ] [ Google Scholar ]
- Truong M., Slezak J. A., Lin C. P., Iremashvili V., Sado M., Razmaria A. A., et al.. (2013). Development and multi-institutional validation of an upgrading risk tool for Gleason 6 prostate cancer . Cancer 119 , 3992–4002. 10.1002/cncr.28303 [ PMC free article ] [ PubMed ] [ CrossRef ] [ Google Scholar ]
- Truong P. (2017). An exploration of topological properties of high-frequency onedimensional financial time series data using TDA (Ph.D. thesis). KTH Royal Institute of Technology, Stockholm, Sweden. [ Google Scholar ]
- Tschandl P., Rosendahl C., Kittler H. (2018). Data descriptor: the HAM10000 dataset, a large collection of multi-source dermatoscopic images of common pigmented skin lesions . Sci. Data 5 :180161. 10.1038/sdata.2018.161 [ PMC free article ] [ PubMed ] [ CrossRef ] [ Google Scholar ]
- Turner K., Mukherjee S., Boyer D. M. (2014). Persistent homology transform for modeling shapes and surfaces . Inf. Inference 3 , 310–344. 10.1093/imaiai/iau011 [ CrossRef ] [ Google Scholar ]
- Verhaak R. G., Hoadley K. A., Purdom E., Wang V., Qi Y., Wilkerson M. D., et al.. (2010). Integrated genomic analysis identifies clinically relevant subtypes of glioblastoma characterized by abnormalities in PDGFRA, IDH1, EGFR, and NF1 . Cancer Cell 17 , 98–110. 10.1016/j.ccr.2009.12.020 [ PMC free article ] [ PubMed ] [ CrossRef ] [ Google Scholar ]
- Vikeså J., Møller A. K., Kaczkowski B., Borup R., Winther O., Henao R., et al.. (2015). Cancers of unknown primary origin (CUP) are characterized by chromosomal instability (CIN) compared to metastasis of know origin . BMC Cancer 15 :151. 10.1186/s12885-015-1128-x [ PMC free article ] [ PubMed ] [ CrossRef ] [ Google Scholar ]
- Wadhwa R. R., Williamson D. F. K., Dhawan A., Scott J. G. (2018). TDAstats: R pipeline for computing persistent homology in topological data analysis . J. Open Source Softw . 3 :860. 10.21105/joss.00860 [ PMC free article ] [ PubMed ] [ CrossRef ] [ Google Scholar ]
- Wang G. (2016). A perspective on deep imaging . IEEE Access 4 , 8914–8924. 10.1109/ACCESS.2016.2624938 [ CrossRef ] [ Google Scholar ]
- Weinberger S. (2014). The complexity of some topological inference problems . Found. Comput. Math . 14 , 1277–1285. 10.1007/s10208-013-9152-1 [ CrossRef ] [ Google Scholar ]
- Wilkerson A. C., Chintakunta H., Krim H. (2014). “Computing persistent features in big data: a distributed dimension reduction approach,” in 2014 IEEE International Conference on Acoustics, Speech and Signal Processing (ICASSP) (Florence: ), 11–15. 10.1109/ICASSP.2014.6853548 [ CrossRef ] [ Google Scholar ]
- Xiaohua C., Brady M., Rueckert D. (2004). “Simultaneous segmentation and registration for medical image,” in International Conference on Medical Image Computing and Computer-Assisted Intervention (Saint-Malo: ), 663–670. 10.1007/978-3-540-30135-6_81 [ CrossRef ] [ Google Scholar ]
- Yu K. H., Zhang C., Berry G. J., Altman R. B., Ré C., Rubin D. L., et al.. (2016). Predicting non-small cell lung cancer prognosis by fully automated microscopic pathology image features . Nat. Commun . 7 , 1–10. 10.1038/ncomms12474 [ PMC free article ] [ PubMed ] [ CrossRef ] [ Google Scholar ]
- Yuan Y., Failmezger H., Rueda O. M., Raza Ali H., Gräf S., Chin S. F., et al.. (2012). Quantitative image analysis of cellular heterogeneity in breast tumors complements genomic profiling . Sci. Transl. Med . 4 :157ra143. 10.1126/scitranslmed.3004330 [ PubMed ] [ CrossRef ] [ Google Scholar ]
- Zhang Y., Brady M., Smith S. (2001). Segmentation of brain MR images through a hidden markov random field model and the expectation-maximization algorithm . IEEE Trans. Med. Imaging 20 :45. 10.1109/42.906424 [ PubMed ] [ CrossRef ] [ Google Scholar ]
- Zhao B., Pritchard J. R., Lauffenburger D. A., Hemann M. T. (2014). Addressing genetic tumor heterogeneity through computationally predictive combination therapy . Cancer Discov . 4 , 166–174. 10.1158/2159-8290.CD-13-0465 [ PMC free article ] [ PubMed ] [ CrossRef ] [ Google Scholar ]
- Zhou X., Carbonetto P., Stephens M. (2013). Polygenic modeling with bayesian sparse linear mixed models . PLoS Genet . 9 :e1003264. 10.1371/journal.pgen.1003264 [ PMC free article ] [ PubMed ] [ CrossRef ] [ Google Scholar ]
- Zomorodian A. (2010). “The tidy set: a minimal simplicial set for computing homology of clique complexes [extended abstract],” in Computational Geometry (SCG'10) (New York, NY: ACM; ), 257–266. 10.1145/1810959.1811004 [ CrossRef ] [ Google Scholar ]
- Zomorodian A., Carlsson G. (2005). Computing persistent homology . Discrete Comput. Geom . 33 , 249–274. 10.1007/s00454-004-1146-y [ CrossRef ] [ Google Scholar ]
Parameterized Topological Data Analysis
Brad nelson, institute for computational and mathematical engineering, stanford university.
May 5, 2020 bnels.github.io/phd-talk
Collaborators/Support
- Gunnar Carlsson
- Anjan Dwaraknath
- DoD through NDSEG fellowship
- DOE through SLAC/Neutrino group
- Introduction to Topological Data Analysis (TDA).
- Matrix factorization algorithms and quiver representations for TDA.
- Parametrization with cover complexes.
- The topology of 3-dimensional image patches.
Topological Data Analysis (TDA)
Concerned with understanding and using data through topology. Data sets as topological spaces. Data points as topological spaces. Compute algebraic signatures of shapes.
Application of homology to data begun by Robins [R 00] , furthered by [ELZ 02] , [ZC 05] , [CdS 10] .
Applications (necessarily incomplete list): Data visualization (mapper) [SMC 07] , neuroscience [GGB 16] , molecular properties [CWD 17] , materials discovery [H+ 16] , regularization [G N D+ 20] , genetics [CGR 13]
Topological Features

Figure from "Potentially highly potent drugs for 2019-nCoV" Nguyen et al. [N+ 20]
Topology of Data Sets

Topology in Image Patches

- Patches from [vHvdS 98] natural images.
- Investigation of statistics [LPM 02]
- Topological investigations in [dSC 04]
- Klein bottle model [C+ 08]
- Compression scheme [MSC 08]
- Texture classification [PC 14]
- Analysis of CNNs [GC 19]
- Range Images [AC 09]
- Optical flow [A+ 20]
Algebraic Topology Review
Data set (discrete topological space) $X$. $k$-simplex $(x_0,\dots,x_k)$ is the span of $k+1$ points in $X$. Simplicial complex (topological space) $\mathcal{X}$ built from simplices.
Chain complex $C_\ast(\mathcal{X})$ (assume field coefficients). $C_k(\mathcal{X})$ vector space with basis vector for every $k$-simplex. Differential maps $\partial_k: C_k(\mathcal{X}) \to C_{k-1}(\mathcal{X})$, $\partial_k \circ \partial_{k+1} = 0$.
Homology $H_k(\mathcal{X}) = \ker \partial_k / \text{img} \partial_{k+1}$ quotient vector space. $\dim H_0(\mathcal{X})$ = # connected components, $\dim H_1(\mathcal{X})$ = # of loops, ... Maps $f:\mathcal{X}\to \mathcal{Y}$ produce induced maps $H_k(f) : H_k(\mathcal{X}) \to H_k(\mathcal{Y})$ Homotopy invariance: if $\mathcal{X}\simeq \mathcal{Y}$, $H_\ast(\mathcal{X}) \cong H_\ast(\mathcal{Y})$.
Persistent and Zigzag Homology

Parameterized TDA

Data is typically discrete. Sometimes more sensible to talk about covers $\mathcal{U}$, and pullbacks of covers $p^{-1}(\mathcal{U})$.
Why? Lots of structure to use - gives more ways to compare different data. Can we bring computational tools (long exact and spectral sequences) to setting of data?
Topological Simplification
Simplicial complexes often contain much more information than is needed. This can make computations much more expensive than necessary.
One idea is to use a cover $\mathcal{U}$ of $X$, and use the nerve $\mathcal{N}(\mathcal{U})$.
Nerve Theorem [B 48] (informal): For nice $\mathcal{U}$ and nice $X$, $\mathcal{N}(\mathcal{U}) \simeq X$. Unfortunately, this isn't immediately useful for discrete data.
Computing Zigzag Homology

We'll call $\cal N(\cal U, \cal V)$ the bivariate nerve. Something like this proposed in [CdS 10] for Witness Complexes
Prior Work on Zigzag Homology

- Zigzag homology introduced by [CdS 10]
- Algorithm for special case of inclusions [CdSM 09]
- Only widely available implementation in Dionysus [M]
- Work on applications to Rips complexes [OS 15]
- Examples of type-A quiver representations [G 72]
No computational tools for bivariate Nerve diagram (maps are not inclusions)
Contributions
- New computational framework for computing persistent and zigzag homology
- Handles arbitrary maps between spaces
- Multiple opportunities for parallelization
We can compute zigzag homology of bivariate nerve diagrams! Persistent and Zigzag Homology: A Matrix Factorization Viewpoint [CD N 19] Code: BATS and BATS.py See also Anjan's forthcoming thesis [D 20] .
Parallelization of Homology Functor

- Computing Homology of each space embarassingly parallel
- Computing each induced map embarassingly parallel
Existing TDA packages work on chain complexes (Makes sense if all maps are inclusions) We take advantage of this parallelization.
Quiver Representations

- Diagram of vector spaces, linear transformations
- type-A quiver representations are on a line graph
- [G 72] : type-A quiver representations have finite indecomposables
- [CdS 10] : barcodes are the indecomposable forms
Example: Indecomposable for bar with birth at 1, death at 2: $I[1,2] = 0 \leftrightarrow \mathbb{F} \overset{\cong}\leftrightarrow \mathbb{F} \leftrightarrow 0 \leftrightarrow \dots$ Type-A quiver representations are isomorphic to something in the form $\bigoplus_i I[b_i, d_i]$
The Companion Matrix

Acts on $V_0 \oplus V_1 \oplus V_2$
The Barcode Factorization

$E_i$ have at most 1 nonzero in each row and column Can read barcode off from $\Lambda$ by tracking basis vectors [CD N 19] : the barcode form $\Lambda$ doesn't depend on choice of basis in each vector space
Further Details
- Reversed arrows use $PU\hat{E}_L L$ factorization instead (zigzag)
- Can start at other end of diagram using $PLE_U U$ factorizations
- Combine to get divide-and-conquer scheme to parallelize
Review and Future Directions
- New computational framework for persistent/zigzag homology.
- Can be applied to any maps.
- New applications.
- Quiver representations other than type-A?
- Combine with other optimizations for persistent homology.
Cover Complexes
Rips filtrations $\mathcal{R}(X; r)$ are expensive to use. Difficult to compute high dimensional homology in most situations. True for other constructions as well.
To compute $PH_3(\mathcal{R}(X; r))$ when $X$ has 1000 points, need $\binom{1000}{5}$, about 10 trillion, 4-simplices.
May need even more than 1000 samples to resolve interesting structure.
[S 13] Linear-size approximations to Rips complex. Constant factor may be large.
[GS 17, CS 18] Approximations using filtered Nerves. Focus is on when Nerve is good approximation.
[LM 15] Mayer Vietoris spectral sequence [Y 18] Leray spectral sequence + sheaf cohomology
We'll analyze the cover filtrations $\mathcal{X}(\mathcal{U}; r)$. Idea: restrict simplices in a filtration $\mathcal{X}(r)$ to open sets in $\mathcal{U}$. If we cover 1000 points with 40 overlapping sets of 50 points each, get $\le 40 \binom{50}{5}$, or about 100 million 4-simplices (instead of 10 trillion).
- New method of constructing complexes from point cloud data using a cover.
- Reduce the size of geometric complexes.
- Understanding of how this relates to different constructions.
Implementations available in BATS .
Comparison using Interleavings
We can compare $PH_k(\mathcal{X}(t))$ and $PH_k(\mathcal{Y}(s))$ using an interleaving

In this case, we say $PH_k(\mathcal{X}(t))$ and $PH_k(\mathcal{Y}(s))$ are $(\alpha,\beta)$-interleaved.
Equivalent to the bottleneck distance on persistence diagrams [L 15] .
How to Construct Maps?
In general can be difficult to write down "by hand".
Acyclic carrier theorem [M 80] can be used to algorithmically generate maps from initial data (easier).
[ N 20] extends the acyclic carrier theorem to filtrations.
Basic idea: carrier $\mathscr{C}$ assigns simplices in $\mathcal{X}$ to sub-complexes of $\mathcal{Y}$. We can always generate maps when these subcomplexes satisfy acyclic condition. $\ker \partial_k = \text{img}\, \partial_{k+1} \Rightarrow H_k = 0$, or contractible.
A Nerve Theorem
Let $\bar{\mathcal{U}}$ denote the collection of all non-empty intersections of sets in $\mathcal{U}$.
[ N 20] (Informal) Suppose $PH_\ast(\mathcal{X}(U; r))$ is interleaved with something acyclic for all $U\in \bar{\mathcal{U}}$. Then $PH_\ast(\mathcal{X}(\mathcal{U}; r))$ and $PH_\ast(\mathcal{N}(\mathcal{U}))$ are also interleaved.
If points in $X$ are perturbed, how does $\mathcal{R}(X,\mathcal{U};r)$ change?
[ N 20] (Informal) Let $X,Y$ be samples, and $\mathcal{U}$ a cover of $X\sqcup Y$. If all the sets in $\bar{\mathcal{U}}$ restricted to $X$ are close (in Hausdorff distance) to the sets restricted to $Y$, then the cover complexes have close persistent homology.
$\mathcal{R}(X, \mathcal{U}; r)$ vs. $\mathcal{R}(X; r)$
Different regimes [ N 20] :
- For some $R \ge 0$, $\mathcal{R}(X, \mathcal{U}; r) = \mathcal{R}(X; r)$ for $r\le R$.
- For some $R' \ge 0$, $\mathcal{R}(X, \mathcal{U}; r)$ and $\mathcal{R}(X; r)$ are not interleaved for $r\ge R'$, unless $\mathcal{N}(\mathcal{U})$ is contractible.
- Intermediate regime where $\mathcal{R}(X, \mathcal{U}; r)$ and $\mathcal{R}(X; r)$ are non-trivially interleaved.
Flat torus sampled on $20 \times 10$ grid (200 points). Cover pulled back from cover of 1st circular coordinate.

- Cover complexes can drastically reduce the size of geometric complexes.
- Filtered version of acylic carrier theorem for constructing interleavings.
- We've seen how this compares to the "full" complex for Rips construction.
- Cover complexes fix geometry of cover. Use this in ML pipelines?
- Amenable to parallelization. Adapt scheme from [Y 18] ?
- Algorithmic applications of filtered acyclic carrier theorem?
The Topology of Image Patches
2D images: $\ell \times \ell$ pixel squares sampled from images.
3D images: sample $\ell \times \ell \times \ell$ voxel cubes.
Patches are sampled from natural (non-random) images, so there is structure in the data.
Filter for high-contrast, dense subsets of data. k, p: take top p% ranked by distance to k-nearest neighbor.

- [LPM 02] find annulus when investigating patch statistics
- Preliminary topological investigations in [dSC 04]
- Klein bottle model proposed in [C+ 08]
- Compression [MSC 08] Texture recognition [PC 14] Neural nets [GC 19]
- Range images [AC 09] Flow [A+ 20]
- Topological modeling of 3D image patch data.
- Analyze a fiber bundle model that generalizes klein bottle for 2D patches.
- Use this to understand the distribution of patches in different data sets.
Van Hateren data set [vHvdS 98] - data base of 2D natural images.
BRATS MRI data set [BRATS]
Penobscot seismic interpetation data [Pen]

Van Hateren, k=100 p=20

Penobscot, k=30 p=40
2-dimensional PCA embedding with eigenpatches.

BRATS k=100 p=40
The model space $\cal k^d$.
Consider $d$-dimensional patches. We'll consider idealized patches as functions on the disk $D^d$ We'd like to capture primary spheres and secondary circles in a topological model.
The primary $(d-1)$ sphere: $\{(v_\phi^T x) \mid v_\phi\in S^{d-1}\}$
Fix $v_\phi$. A secondary circle: $\{\cos(\theta)(v_\phi^T x)^2 + \sin(\theta)(v_\phi^T x)\mid \theta \in [0,2\pi)\}$
Note that $v_\phi$ and $-v_\phi$ share the same secondary circle.

The Harris Map
Harris corner/edge detector [HS 88] analyzes eigenvalues of: $M(x) = \sum_i \Delta(x_i) \Delta(x_i)^T$, $\Delta$ finite difference gradient. Harris map $h: x\mapsto \text{MaxEigVec}(M(x))$

There is a sign/scale ambiguity for eigenvectors, so range is naturally $\mathbb{R}P^{d-1}$
Continuous limit $M(f(x)) = \int_x dx\, \nabla(f(x))\nabla(f(x))^T$ For $k(v_\phi, \theta; x)\in \mathcal{K}^d$, $\nabla(k(x)) = k'(x) v_\phi$ so $M(k(x))$ is rank-1, and $h(k(v_\phi, \theta; x)) = [v_\phi]$
$\mathcal{K}^d$ is a fiber bundle over $\mathbb{R}P^{d-1}$ (non-trivial because of twist in identification) The Harris map $h$ is a fibration for $\mathcal{K}^d$ Fibers $h^{-1}([v])$ are secondary circles.
Homology of $\cal K^d$
Because of fibration structure, we can use Leray-Serre spectral sequence to compute homology. We'll use integer ($\mathbb{Z}$) coefficients, which can be used to obtain field coefficients.
$H_\ast(\mathcal{K}^2) = (\mathbb{Z}, \mathbb{Z}\oplus \mathbb{Z}_2, 0)$ $H_\ast(\mathcal{K}^3) = (\mathbb{Z}, \mathbb{Z}_2\oplus \mathbb{Z}_2, 0, \mathbb{Z})$
BRATS patches on $\cal K^3$

Penobscot patches on $\cal K^3$

Review & Future Directions
- Useful for interpreting subsets of the data.
- Captures topology of high-dimensional data with small number of dimensions.
Potential applications: 3D compression, texture, neural nets [MSC 08, PC 14, GC 19]
If we have higher-dimensional patches, we could perform similar analyses. Where would a 4-dimensional image come from?
Other applications where we might use a map: Evasion [AC 14] , Time series, Control systems.
Acknowledgements
- Parameterization by cover when building complexes.
- Parameterization of 3D patch model to understand data.
Where to next?
- We're just scratching the surface of potential "structured" methods for TDA.
- Parameterized invariants for ML pipelines?
- How can we use induced maps more effectively in TDA?

What are barriers to matrix factorization frameworks for other types of quiver representations?
How tight are the interleaving bounds for cover complexes?
What happens for different sized image patches?
Technical Credits
- Slides made with reveal.js
- Computations done with BATS and BATS.py
- Video animations made with manim
- manimtda (uses BATS) manim_reveal (thanks Anjan!)
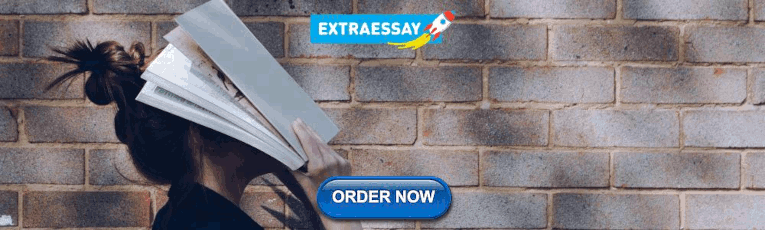
Bibliography
The contents of this talk, with complete references, can be found in: [ N 20] Bradley Nelson. Parameterized Topological Data Analysis. Ph.D. Dissertation. Stanford University. 2020.
[A+ 20] Adams, Bush, Carr, Kassab, Mirth. A torus model for optical flow. 2020. [AC 09] Adams, Carlsson. On the Nonlinear Statistics of Range Image Patches. 2009. [AC 14] - Adams, Carlsson. Evasion Paths in Mobile Sensor Networks. 2015. [BRATS] - BRATS data set. https://www.med.upenn.edu/sbia/brats2018/registration.html [B 48] Borsuk. On the imbedding of systems of compacta in simplicial complexes. 1948. [CWD 17] Cang, Wei, Dunbrack. TopologyNet: Topology based deep convolutional and multi-task neural networks for biomolecular property predictions. 2017. [C+ 08] Carlsson, Ishkhanov, de Silva, Zomorodian. On the Local Behavior of Spaces of Natural Images. 2008. [CD N 19] Carlsson, Dwaraknath, Nelson. Persistent and Zigzag Homology: A Matrix Factorization Viewpoint. 2019. [CdS 10] Carlsson, de Silva. Zigzag Persistence. 2010. [CdSM 09] Carlsson, de Silva, Morozov. Zigzag Persistent Homology and Real-valued Functions. 2009. [CS 18] Cavanna, Sheehy. The Generlized Persistent Nerve Theorem. 2018. [CGR 13] Chan, Carlsson, Rabadan. Topology of viral evolution. 2013. [D 20] Dwaraknath. Quiver Theory, Zigzag Homology, and Deep Learning. 2020. [G 72] Gabriel. Unzerlegbare Darstellungen I. 1972. [GC 19] Gabrielsson, Carlsson. Exposition and Interpretation of the Topology of Neural Networks. 2019. [G N D+ 20] Gabrielsson, Nelson, Dwaraknath, Skraba, Carlsson, Guibas. A Topology Layer for Machine Learning. 2020. [GGB 16] Two’s company, three (or more) is a simplex: Algebraic-topological tools for understanding higher-order structure in neural data. 2016. [GS 17] Govc, Skraba. An Approximate Nerve Theorem. 2017. [HS 88] Harris, Stephens. A combined corner and edge detector. 1988. [H+ 16] Hiraoka, Nakamura, Hirata, Escolar, Matsue, Nishiura. Hierarchical structures of amorphous solids characterized by persistent homology. 2016. [vHvdS 98] van Hateren, van der Schaaf. Independent component filters of natural images compared with simple cells in primary visual cortex. 1998. [LPM 02] Lee, Pedersen, Mumford. The Nonlinear Statistics of High-Contrast Patches in Natural Images. 2002. [dSC 04] de Silva, Carlsson. Topological estimation using witness complexes. 2004. [ELZ 02] Edelsbrunner, Letscher, Zomorodian. Topological Persistence and Simplification. 2002. [L 15] Lesnick. The Theory of the Interleaving Distance on Multidimensional Persistence Modules. 2015. [LM 13] Lewis, Morozov. Parallel Computaiton of Persistent Homology using the Blowup Complex. 2015. [MSC 08] Maleki, Shahram, Carlsson. A Near Optimal Coder For Image Geometry With Adaptive Partitioning. 2008. [M] Morozov. Dionysus2. https://mrzv.org/software/dionysus2/ [M 80] Munkres. Algebraic Topology. 1980. [N+ 20] Nguyen, Gao, Chen, Wang, Wei. Potentially highly potent drugs for 2019-nCoV. 2020. [OS 15] Oudot, Sheehy. Zigzag Zoology: Rips Zigzags for Homology Inference. 2015. [Pen] Penobscot Interpretation Dataset. https://zenodo.org/record/1341774 [PC 14] Perea, Carlsson. A Klein-Bottle-Based Dictionary for Texture Representation. 2014. [R 00] Robins. Computational Topology at Multiple Resolutions: Foundations and Applications to Fractals and Dynamics. 2000. [S 13] Sheehy. Linear-Size Approximations to the Vietoris–Rips Filtration. 2013. [SMC 07] Singh, Memoli, Carlsson. Topological Methods for the Analysis of High Dimensional Data Sets and 3D Object Recognition. 2007. [Y 18] Yoon. Celluar Sheaves and Cosheaves fo Distributed Topological Data Analysis. 2018. [ZC 05] Zomorodian, Carlsson. Computing Persistent Homology. 2005.
Department of Mathematics
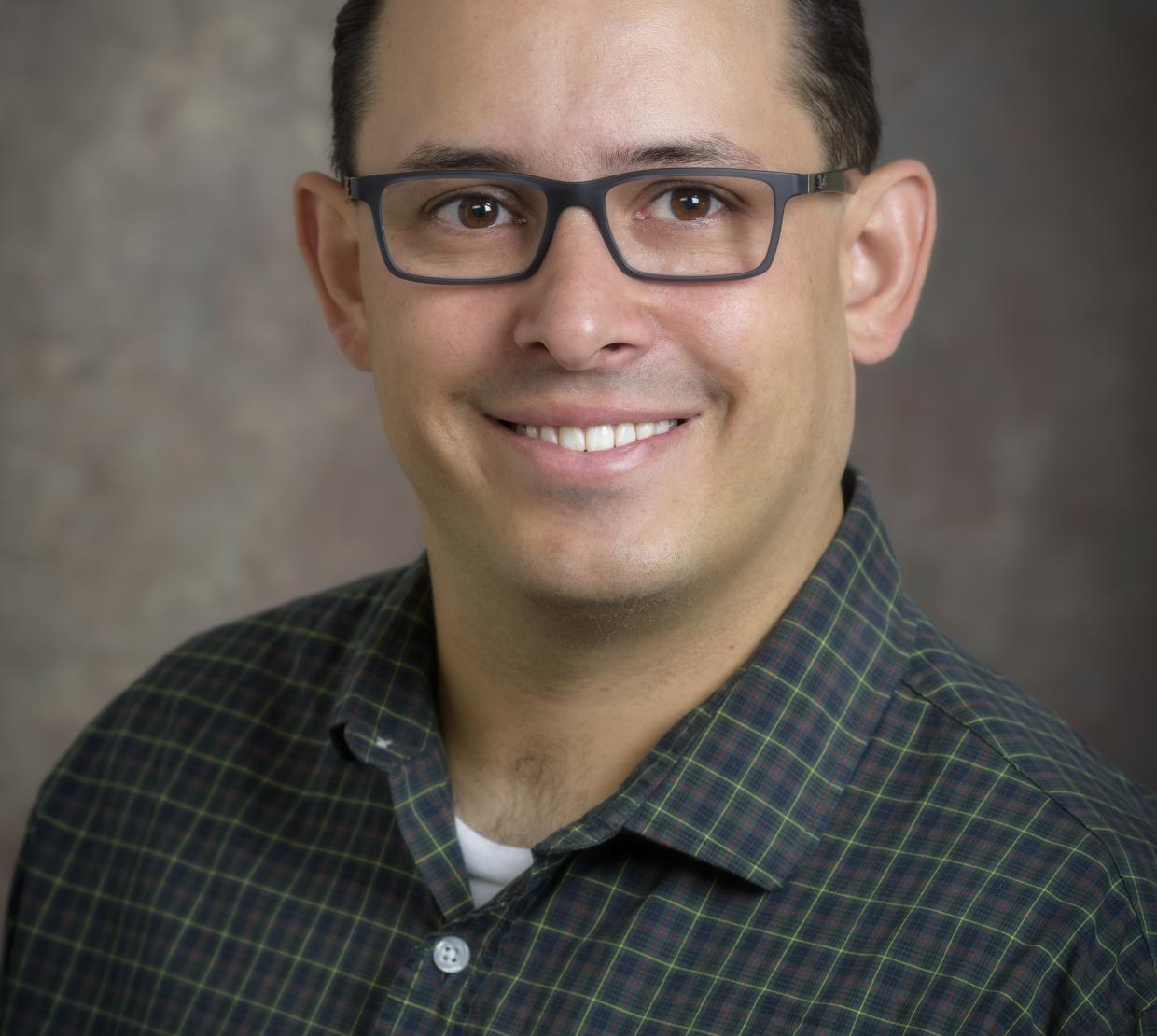
Precision Problem Solving: Topological Data Analysis Driving Advances in Medicine and Biology
Mathematician Chad Giusti spoke with MAA FOCUS, the news magazine of the Mathematical Association of America.
Chad Giusti is an assistant professor of mathematics at Oregon State University. He works in pure and applied topology, with applications principally in neuroscience and complex systems. His work has appeared in journals such as PNAS and Crelle’s and has been supported by the NSF, AFOSR, and AFRL. Here, we learn about the fascinating work Chad has done in applying the tools of topological data analysis to problems in medicine and biology.
1. You are an expert in topological data analysis (TDA), a field that many people in our community are unfamiliar with. How would you describe TDA to someone who just finished the calculus sequence? How would you describe TDA to someone who has taken a standard introductory course in topology?
The usual quip is that topological data analysis characterizes complex systems or data in terms of qualitative notions of “shape.” I think this is at the same time too vague and too specific.
Calculus students are adept at describing shape in qualitative ways. A common exercise is to read off various information about a polynomial by looking at its graph or the graph of its derivative. By counting extrema and roots, examining behavior “at the ends,” and so on, we can determine things like the minimum possible degree, sign of the leading coefficient, and so on. While these are, in principle, numeric answers, they aren’t exact measurements—they’re bounds and ranges of possible values. Even if I only provide a scattering of points on the graph of the polynomial, it’s not much harder to provide the same data about the underlying polynomial.
For students, I would say that topology, particularly algebraic topology, provides a set of mathematical tools for a similarly qualitative characterization of more complex shapes: surfaces and higher dimensional analogues called manifolds, and more abstract structures like graphs. We most commonly formalize “qualitative” as meaning “up to continuous deformation” – stretching or compressing, without cutting or gluing. A circle remains a circle, topologically, even if we stretch it into a wiggly mess as we might do with a rope, so long as we don’t cut it open into a long strand or glue distant points together. This flexibility reduces the specificity of what we can say about systems, but it makes these descriptors more applicable in the presence of noise or incomplete data, both of which are particularly pernicious in biological and medical applications.
Students in an undergraduate topology course might not recognize much of what we do in TDA immediately. However, many will have seen the fundamental group of a topological space, or the topological classification of smooth surfaces, which are cousins of the kind of measurements and classifications we employ when studying “shape” in applications. However, data is rarely given to us in the form of a topological space—we must build approximations of our spaces from things like finite collections of points sampled on (or noisily near) a surface we want to study.
Currently, the most common tool used in TDA is called persistent homology, which characterizes how qualitative features of a shape evolve as some parameter changes. The parameter can be a measure of size (“how big are the features”), time (“when do the features appear”), or something more esoteric and domain dependent. Persistent homology gives us a collection of vector spaces associated to the space, much like the fundamental group gives us a group. By comparing these vector spaces across different data sets—results of some experiment under different conditions, for example—we can use the similarity or differences between the evolution of features to reason about how the underlying systems compare. Differences in shape can point to differences in organization in a complex system. For example, neural activity that encodes the head direction of a mouse is well-described by a circle, but that which describes the head direction of a bat generally requires a shape that can encode three dimensions of motion. (In fact, experimentally it appears to be a torus, not a sphere!)
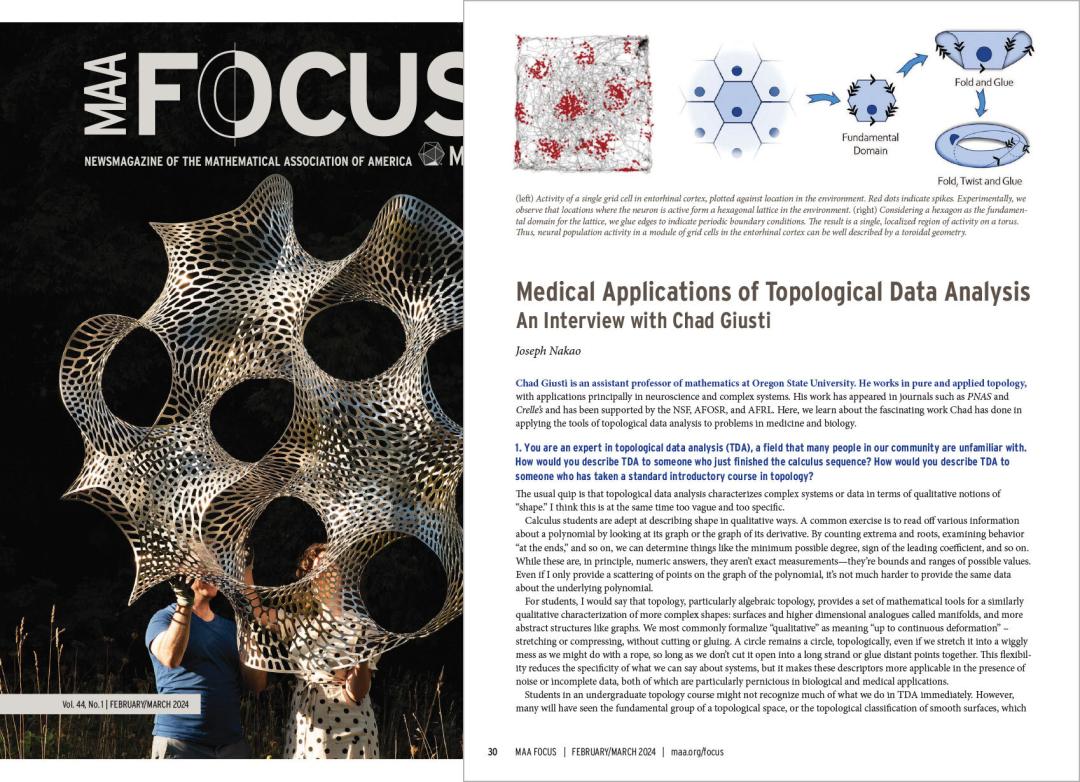
Image of Chad Giusti's MAA FOCUS magazine article.
2. You apply TDA to current problems and systems that arise in biology and medicine. Can you elaborate more on those applications and what got you interested in pursuing them?
When I think about my applied work, I usually place it in the field of theoretical neuroscience, in the context of developing a theory of how neural populations encode information and perform computations. It turns out that many of the models that neuroscientists have developed to describe these phenomena “look” topological in the sense that it’s easy (for an applied topologist like me) to imagine formalizing them using language from TDA.
In fact, this is how I first got started in the area. As a graduate student, I worked in pure algebraic and geometric topology studying spaces of knots, though my projects always had a computational bent. One year on the job market, I had two offers: one to go to Belgium and work on this very theoretical type of mathematics, and another to go to Lincoln, Nebraska and try to apply topology to the study of neural codes. The PIs on that project, Vladimir Itskov and Carina Curto, showed me some pictures of place fields, which diagram how individual neurons in the hippocampus respond to an animal’s location in its environment.
These look a great deal like the topological notion of a “cover” of a space, which is one of our fundamental tools for studying shape. Their notion, which turned out to be an excellent one, was that we should be able to use tools from TDA to study this structure in neural activity, providing a platform for mathematically formalizing some of these informal models. The idea of developing an entirely new way of studying how the brain works—and doing it using all of the abstract math I’d fallen in love with in graduate school—was a very compelling offer.
I think it’s important to note that, as compelling as the offer was, pursuing this route was a risky decision. Novel applications of mathematics, particularly areas of math that aren’t well established for applications, very often don’t gain traction or take many years to do so, and a postdoc project that doesn’t go anywhere usually doesn’t lead to further employment. I had the privilege to be able to take that risk in large part because I had a strong economic and personal support system, including skills that would allow me to seek alternative employment if the project didn’t work out. It would behoove us to provide more support to early career academics so it’s easier to take these big risks.
Lastly, I should note that my own narrow conception of my work is not exactly accurate: I’ve done or supervised projects in human neuroscience/neurology, physics of granular media, plant/pollinator networks, collective behavior of swarms, and elsewhere. I’m currently working with researchers on problems in climate science and cancer genetics. I suppose the point is that it doesn’t take a lot of persuasion to get me interested in a good problem.
To read the rest of the article click here.
Read more stories about: news , faculty and staff , mathematics
Related Stories
Across the department, explore related stories.
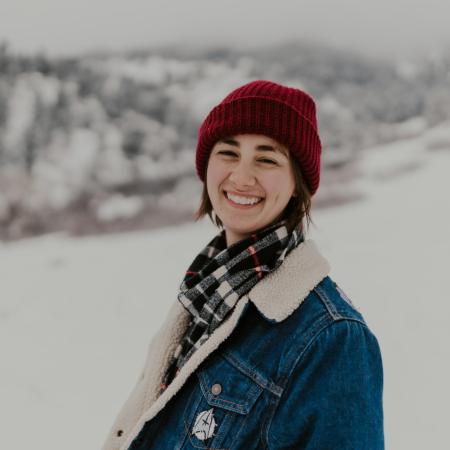
What do mathematicians do? This mathematics grad began a technical writing career at Amazon
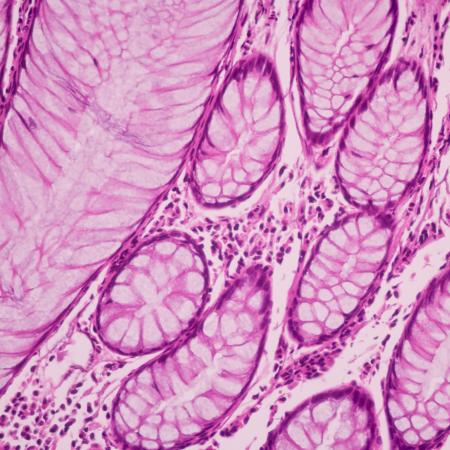
Innovation in cancer treatment and mathematics: SciRIS awardees lead the way
Mathematics graduate thrives with simple philosophy: ‘Why not?’
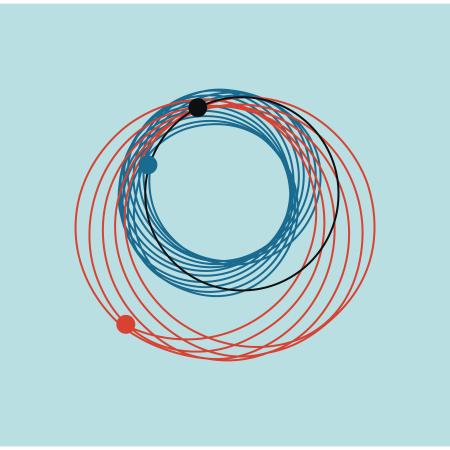
Classroom puzzles to cosmic insights: Students and professor demystify mathematical theorem
Introduction to Topological Data Analysis
Course Overview
- Course days: 15-19 January 2024
- Attendance: online
- Course level: Master's, PhD candidates, and professionals from all disciplines
- Course curriculum: see the full course curriculum
- Coordinating lecturers: Senja Barthel , Magnus Botnan , Renee Hoekzema
- Forms of tuition: tutorials, pre-recorded lectures, practical tasks, feedback sessions
- Language of tuition: English
- Forms of assessment: theory questions, practical tasks, daily assignments, final mini project
- Credits: 2 ECTS
- Contact hours: 15 hours
- Self-study hours: 41 hours
- How to apply: read more about our fees and application process
This online course is part of VU Amsterdam Graduate Winter School online learning, short courses targeted at graduates and professionals.
This website uses cookies
You can accept all cookies or set your preferences per cookie category. You can always alter your choice by removing the cookies from your browser. VU Amsterdam and others use cookies to: 1) analyse website use; 2) personalise the website; 3) connect to social media networks; 4) show relevant advertisements. More information about the cookies we use
Cookie preferences
You can accept all cookies or you can set your preferences per cookie category. You can always alter your choice by removing the cookies from your browser. See more information in the cookie statement.
Personal settings:
These cookies are used to ensure that our website operates properly.
These cookies help to analyse the use of the website. These measurement data are subsequently used to improve the website.
Personalisation
These cookies are used to analyse how you use our website. This enables us to adapt our website content with information that suits your interests.
Social media
These cookies are placed by social media networks. For example, if you watch a YouTube video embedded in the website, or use the social media buttons on our website to share or like a post. This allows social media networks to track your internet behaviour and use that for their own purposes.
Advertising
These cookies are placed by advertising partners. They are used to show you relevant advertisements for Vrije Universiteit Amsterdam on other websites that you visit. They enable advertising networks to track your internet behaviour.

- Scalar Field Visualization
- Vector Field Visualization
- Tensor Field Visualization
- Feature-Based Visualization
Topological Analysis
- Volume Rendering
- Higher-Order Visualization
- Information Visualization
- Astrophysics Visualization
- Point-Based Graph. & Vis.
- Computational Photography
Publications
- ParaView Plugins
- Open Positions
- Achievements
- Legal Information
Magnet.me - The smart network where hbo and wo students find their internship and first job.
The smart network where hbo and wo students find their internship and first job.
Postdoc In Topological Data Analysis
Your career starts on Magnet.me
Create a profile and receive smart job recommendations based on your liked jobs.
Do you have an inquisitive mind and a passion for topology and its applications? Please apply for a Postdoctoral position at Vrije Universiteit Amsterdam.
Your function
The Department of Mathematics of Vrije Universiteit Amsterdam welcomes applications for a three-year Postdoctoral position in topological data analysis. The topic is the development of techniques to study data through topology and the mathematical and algorithmic foundations for these techniques. This encompasses topics such as persistent homology, knot-theoretic invariants for data, developing methodology for analyzing biomedical data, and topological aspects of phylogenetic trees and solid-state materials. The exact project will be chosen according to the strengths of the applicant. The postdoc will work within the Center for Topology and Applications Amsterdam (https://vu.nl/en/about-vu/more-about/geometry-and-topology). Preference will be given to candidates who can connect to existing research strengths in the department. We are an inclusive, interdisciplinary group, and diversity and internationalism are at the heart of our research principles and teaching practice.
The preferred starting date is 01.09.2024.
Applications from all groups currently under-represented in academic posts are especially encouraged. We particularly welcome applications from women and people with an ethnic minority background.
Your duties
- conducting research in the area of topological data analysis (85%)
- contributing to the teaching portfolio of the Department of Mathematics (15%)
Your profile
- a PhD in mathematics with expertise in topology, geometry, or related areas
- some experience with programming
- strong independent research skills
- good communication skills in English
What do we offer?
A rewarding position in a socially involved organization. On full-time basis the remuneration amounts to a minimum gross monthly salary of €4,036 (scale 10) and a maximum of €5,090 (scale 10). The job profile is based on the university job ranking system and is vacant for 0.8-1.0 FTE.
The appointment will initially be for 2 years. After a satisfactory evaluation, the contract can be extended for another year. Additionally, Vrije Universiteit Amsterdam offers excellent fringe benefits and various schemes and regulations to promote a good work/life balance, such as:
- 8% holiday allowance and 8.3% end-of-year bonus
- contribution to commuting expenses
- optional model for designing a personalized benefits package
- a maximum of 41 days of annual leave based on full-time employment
- good paid parental leave scheme
The Department of Mathematics
The Department of Mathematics strives for excellence in research. The department balances pure mathematical research with mathematical research motivated by applications. Researchers in the department are on one hand active at a fundamental and theoretical level, and, on the other hand, work on applications with links to business, the sciences, and societal issues. The department has a strong international research staff with expertise in dynamical systems, topology, geometry and algebra, as well as in stochastics (statistics, data analytics, probability).
Faculty of Science
Researchers and students at VU Amsterdam’s Faculty of Science tackle fundamental and complex scientific problems to help pave the way for a sustainable and healthy future. From forest fires to big data, from obesity to malnutrition, and from molecules to the moon: we cover the full spectrum of the natural sciences. Our teaching and research have a strong experimentally technical, computational and interdisciplinary nature.
We work on new solutions guided by value-driven, interdisciplinary methodologies. We are committed to research, valorisation and training socially engaged citizens of the world who will make valuable contributions to a sustainable, healthy future.
Are you interested in joining the Faculty of Science? You will join undergraduate students, PhD candidates and researchers at the biggest sciences faculty in the Netherlands. You will combine a professional focus with a broad view of the world. We are proud of our collegial working climate, characterised by committed staff, a pragmatic attitude and engagement in the larger whole. The faculty is home to over 11,000 students enrolled in 40 study programmes. It employs over 1,600 professionals spread across 10 academic departments.
Vrije Universiteit Amsterdam
Vrije Universiteit Amsterdam stands for values-driven education and research. We are open-minded experts with the ability to think freely. - a broader mind. Maintaining an entrepreneurial perspective and concentrating on diversity, significance and humanity, we work on sustainable solutions with social impact. By joining forces, across the boundaries of disciplines, we work towards a better world for people and planet. Together we create a safe and respectful working and study climate, and an inspiring environment for education and research.
We are located on one physical campus, in the heart of Amsterdam's Zuidas business district, with excellent location and accessibility. Over 5,500 staff work at the VU and over 30,000 students attend academic education.
Diversity is the driving force of the VU. The VU wants to be accessible and receptive to diversity in disciplines, cultures, ideas, nationalities, beliefs, preferences and worldviews. We believe that trust, respect, interest and differences lead to new insights and innovation, to sharpness and clarity, to excellence and a broader understanding.
We stand for an inclusive community and believe that diversity and internationalisation contribute to the quality of education, research and our services. Therefore, we are always searching for people whose backgrounds and experience contribute to the diversity of the VU community.
At Vrije Universiteit Amsterdam, we attach great importance to the societal impact of our education and research. Personal development and social involvement are key parts of our vision on education, in which individual differences are seen as a strength. This allows us to develop innovations and insights that contribute to a better world.
People also viewed
Risk and Finance Data Management & Analysis Program
Amsterdam, NL
Data Engineer
Takeaway.com
Junior Market Risk Reporting & Data Analysis
Postdoc in SuperGPS-2 Project
Delft University of Technology
Data Analyst
Digital Power
Engineering Analysis Intern
Young Professional – Financial Planning & Analysis
View what's on offer:
- Traineeships
- HBO Traineeships
- WO Traineeships
- Internships
- HBO Internships
- WO Internships
- Graduate internships
- Business courses
- Inhouse days
- All locations
Quickly browse to:
- Communication internships
- IT internships
- Marketing internships
- Consulting internships
- IT traineeships
- Consulting traineeships
- Internship interview - A complete guide
- How to choose a company to work for after graduating
- 9 things to know before starting an internship
- How to rock your video interview
- Skills for CV: Teamwork
- 4 tips that guarantee you to ace your first job interview in the Netherlands
- Careers guide
- About us (press)
- For employers
- Privacy Policy & Terms of Service
- Careers at Magnet.me

Change language to: Dutch
This page is optimised for people from the Netherlands. View the version optimised for people from the UK.
An official website of the United States government
Here's how you know
Official websites use .gov A .gov website belongs to an official government organization in the United States.
Secure .gov websites use HTTPS. A lock ( Lock Locked padlock ) or https:// means you've safely connected to the .gov website. Share sensitive information only on official, secure websites.

- Search Awards
- Recent Awards
- Presidential and Honorary Awards
- About Awards
- How to Manage Your Award
- Grant General Conditions
- Cooperative Agreement Conditions
- Special Conditions
- Federal Demonstration Partnership
- Policy Office Website

Note: When clicking on a Digital Object Identifier (DOI) number, you will be taken to an external site maintained by the publisher. Some full text articles may not yet be available without a charge during the embargo (administrative interval). Some links on this page may take you to non-federal websites. Their policies may differ from this site.
Please report errors in award information by writing to: [email protected] .
Thank you for visiting nature.com. You are using a browser version with limited support for CSS. To obtain the best experience, we recommend you use a more up to date browser (or turn off compatibility mode in Internet Explorer). In the meantime, to ensure continued support, we are displaying the site without styles and JavaScript.
- View all journals
- My Account Login
- Explore content
- About the journal
- Publish with us
- Sign up for alerts
- Open access
- Published: 11 April 2024
Nonlinearity-induced topological phase transition characterized by the nonlinear Chern number
- Kazuki Sone ORCID: orcid.org/0000-0003-4382-3544 1 ,
- Motohiko Ezawa ORCID: orcid.org/0000-0002-3629-5643 1 ,
- Yuto Ashida ORCID: orcid.org/0000-0002-9812-0320 2 , 3 ,
- Nobuyuki Yoshioka ORCID: orcid.org/0000-0001-6094-8635 1 , 4 , 5 &
- Takahiro Sagawa ORCID: orcid.org/0000-0002-1274-0829 1 , 6
Nature Physics ( 2024 ) Cite this article
2305 Accesses
23 Altmetric
Metrics details
- Nonlinear optics
- Nonlinear phenomena
- Topological matter
As first demonstrated by the characterization of the quantum Hall effect by the Chern number, topology provides a guiding principle to realize the robust properties of condensed-matter systems immune to the existence of disorder. The bulk–boundary correspondence guarantees the emergence of gapless boundary modes in a topological system whose bulk exhibits non-zero topological invariants. Although some recent studies have suggested a possible extension of the notion of topology to nonlinear systems, the nonlinear counterpart of a topological invariant has not yet been understood. Here we propose a nonlinear extension of the Chern number based on the nonlinear eigenvalue problems in two-dimensional systems and show the existence of bulk–boundary correspondence beyond the weakly nonlinear regime. Specifically, we find nonlinearity-induced topological phase transitions, in which the existence of topological edge modes depends on the amplitude of oscillatory modes. We propose and analyse a minimal model of a nonlinear Chern insulator whose exact bulk solutions are analytically obtained. The model exhibits the amplitude dependence of the nonlinear Chern number, for which we confirm the nonlinear extension of the bulk–boundary correspondence. Thus, our result reveals the existence of genuinely nonlinear topological phases that are adiabatically disconnected from the linear regime.
Similar content being viewed by others
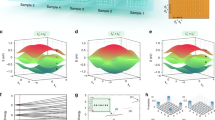
Quantum superposition demonstrated higher-order topological bound states in the continuum
Yao Wang, Bi-Ye Xie, … Xian-Min Jin
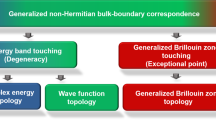
Topological phase transitions of generalized Brillouin zone
Sonu Verma & Moon Jip Park
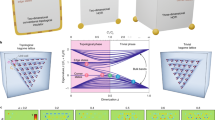
Nonlinear second-order photonic topological insulators
Marco S. Kirsch, Yiqi Zhang, … Matthias Heinrich
Topology is utilized to realize the robust properties of materials that are immune to disorders 1 , 2 . A prototypical example of topological materials is the quantum Hall effect 3 , 4 , which was discovered in a two-dimensional semiconductor under a magnetic field. In such a two-dimensional system, the Chern number characterizes the topology of the band structure and the corresponding gapless boundary modes. This bulk–boundary correspondence lies at the heart of the robustness of topological devices utilizing boundary modes. Recent studies have also explored topological phenomena in a variety of platforms, such as photonics 5 , electrical circuits 6 , ultracold atoms 7 , fluids 8 and mechanical lattices 9 .
Although band topology has been well explored in linear systems, nonlinear dynamics is ubiquitous in classical 10 , 11 , 12 , 13 , 14 , 15 and interacting bosonic systems 16 , 17 . For example, nonlinear interactions can naturally emerge in the mean-field analysis of bosonic many-body systems, such as the Gross–Pitaevskii equations. Recent research has also studied the nonlinear effects on topological edge modes 11 , 12 , 18 , 19 , 20 , 21 , 22 , 23 , 24 , 25 , 26 , 27 , 28 and revealed unique topological phenomena intertwined with solitons 29 , 30 , 31 , 32 , 33 , 34 , 35 and synchronization 36 , 37 , 38 . Nonlinearity can further modify the conventional notion of topological phases; it has been found that one-dimensional systems can exhibit nonlinearity-induced topological phase transitions, where the existence of topological edge modes depends on the amplitude of the oscillatory modes 39 , 40 , 41 , 42 , 43 . Although these previous studies have indicated the existence of topological edge modes in nonlinear systems, one cannot straightforwardly extend the topological invariants to nonlinear systems because they have no band structures, at least in the conventional sense. In addition, nonlinear topology in two-dimensional systems 18 , 30 , 31 , 35 is much less understood than that in one-dimensional systems.
In this paper, we introduce the notion of the nonlinear Chern number and reveal its relation to the bulk–boundary correspondence. To define the nonlinear Chern number of two-dimensional systems, we consider the nonlinear extension of the eigenvalue problem 41 , 43 , 44 and make an analogy to band structures. Although it is not obvious that the nonlinear eigenvalue problem elucidates the bulk–boundary correspondence in nonlinear topological insulators, we theoretically prove the bulk–boundary correspondence of the nonlinear Chern number in weakly nonlinear systems. Furthermore, in stronger nonlinear regimes where nonlinear terms are larger compared with the linear bandgap, we find that the nonlinearity-induced topological phase transition can occur in two-dimensional systems (Fig. 1 ). We analytically show the nonlinear bulk–boundary correspondence, which states that the non-zero nonlinear Chern number predicts the existence of localized edge modes in semi-infinite systems even under strong nonlinearity. Since the nonlinearity-induced topological phases are disconnected from the linear limits under adiabatic deformations, our results show the existence of genuinely nonlinear topological phases.
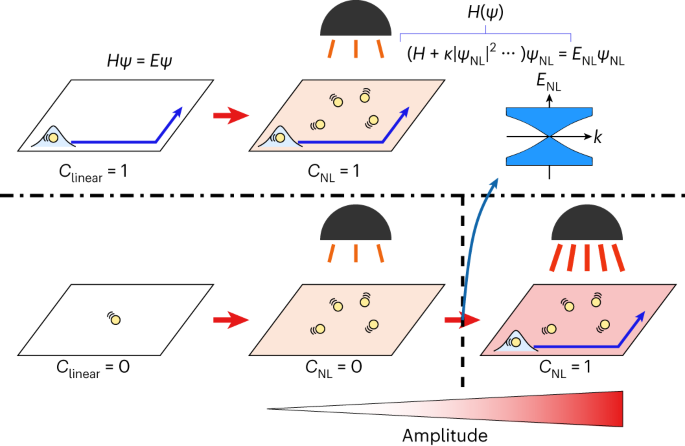
Although the topology of a non-interacting linear system can be characterized by the Chern number that is computed from its eigenvectors, the topology of a nonlinear system is classified by the nonlinear Chern number, which utilizes the nonlinear extension of the eigenequation. In weakly nonlinear regions (that is, small amplitude), the nonlinear Chern number predicts the existence of edge modes corresponding to those in linear systems. Specifically, when nonlinear systems exhibit edge-localized steady states, both nonlinear and linear Chern numbers are non-zero (top). If we inject higher energy into the system and consider the eigenmodes with large amplitudes, the nonlinear band structure can become gapless. At such a gapless point, a nonlinearity-induced topological phase transition can occur, where topological boundary modes appear with the non-zero nonlinear Chern number (bottom). The nonlinearity-induced topological phases exhibit boundary modes that cannot be predicted from the linear Chern number. Therefore, such topological phases are genuinely unique to nonlinear systems.
The scope of this paper applies to a broad class of two-dimensional systems with U (1) gauge and spatial translation symmetries, which can be realized in a variety of experimental setups. Similar to how the Thouless–Kohmoto–Nightingale–den Nijs formula 4 triggered the research of a variety of topological materials, the nonlinear Chern number is expected to open up the research stream of nonlinear topological materials including their systematic classification. From the experiment point of view, one can realize nonlinear Chern insulators with the U (1)-gauge symmetry in, for example, photonics 11 , 12 , 18 , 20 , 22 , 24 , 27 , 30 , 31 , 32 , 33 , 34 , ultracold atoms 16 , 17 , 27 and electrical circuits 6 , 36 , 37 , where both linear band topology and nonlinear effects have been investigated. In particular, since the Kerr nonlinearity 10 is fairly common in photonic systems, it should be possible to extend the current topological photonic devices to nonlinear ones.
Nonlinear eigenvalue problem and nonlinear Chern number
We consider the nonlinear extension of the eigenvalue equations 41 , 43 , 44 and define the nonlinear Chern number by using nonlinear eigenvectors. We start from the general nonlinear dynamics:
where Ψ j ( r ) is the state variable and f j ( ⋅ ; r ) is a nonlinear function of the state vector Ψ . In lattice systems, r denotes a representative point in each unit cell of the lattice (Fig. 2a ). Then, j represents the internal degrees of freedom that include, for example, sublattices and effective spin degrees of freedom. When we consider continuum systems, r should simply represent the location and j corresponds to the internal degrees of freedom such as spins. For example, the Gross–Pitaevskii equation in the continuous space is given by f ( Ψ ; r ) = − ∇ 2 Ψ ( r )/(2 m ) + VΨ ( r ) + (4π a / m ) ∣ Ψ ( r ) ∣ 2 Ψ ( r ), where V is a potential and m and a are the mass and scattering length, respectively. The nonlinear function f ( ⋅ ; r ) depends on Ψ ( r ) and its derivative, and has no internal degrees of freedom. Since the quantum Hall system has U (1) and translation symmetries, we impose them on the nonlinear equation to study the analogy of such a prototypical topological insulator. Concretely, the U (1) symmetry is represented as f j (e i θ Ψ ; r ) = e i θ f j ( Ψ ; r ), which is satisfied in, for example, the Kerr-like nonlinearity κ ∣ Ψ j ( r ) ∣ 2 Ψ j ( r ) (ref. 10 ). The translational symmetry in lattice systems is defined as f j ( Ψ ; r + a ) = f j ( Φ ; r ), where a is a lattice vector and Φ is the translated state variable: Φ j ( r ) = Ψ j ( r + a ). The translational symmetry in continuum systems is also defined in the same equation, whereas f j ( Φ ; r ) still remains dependent on r due to, for example, the periodic potential. We also focus on conservative dynamics analogous to Hermitian Hamiltonians where the sum of squared amplitudes ∑ j , r ∣ Ψ j ( r ) ∣ 2 is preserved.
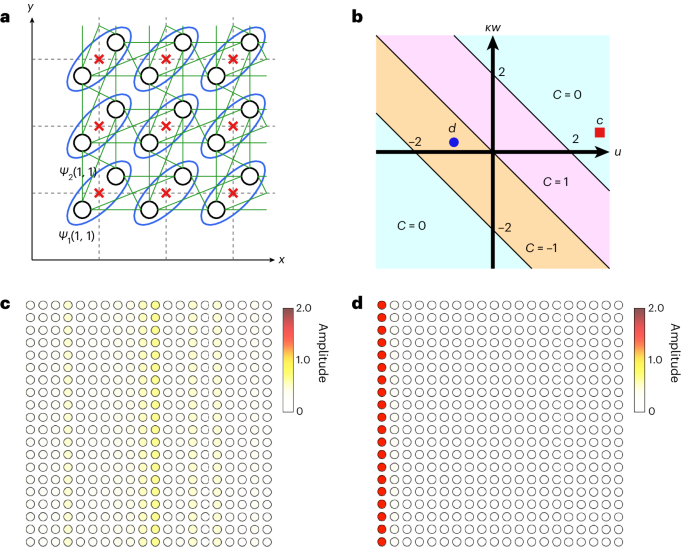
a , Schematic of the nonlinear QWZ model. The model has two sublattices (black circles) at each lattice point encircled by the blue ellipse. The green lines represent the linear couplings. We use the notation Ψ i ( x , y ) to represent the state variable at each sublattice, where ( x , y ) is the location of the representative point of each lattice point denoted by the red cross. b , Analytical demonstration of the phase diagram of the nonlinear QWZ model. The horizontal axis represents the parameter of the mass term and the vertical axis corresponds to the strength of nonlinearity. The colour of each separated region represents the difference in the nonlinear Chern number. c , Numerical demonstration of the absence of edge modes in the topologically trivial parameter region. We simulate the dynamics of the prototypical model of a nonlinear Chern insulator starting from an initial state localized at the left edge. We impose the open boundary condition in the x direction and the periodic boundary condition in the y direction. The figure shows the snapshot at t = 1. The colour shows the absolute value of the components of the state vector at each site. The parameters used are u = 3 and κ = 0.1, and the average amplitude is w = 0.1, which corresponds to the red square in b . d , Numerical demonstration of the existence of the long-lived localized state in the weakly nonlinear topological insulator. The figure shows the snapshot of the simulation at t = 1. The sites at the left edge show large amplitudes, which indicates the existence of the edge-localized state. The parameters used are u = −1 and κ = 0.1, and the average amplitude is w = 0.1, which corresponds to the blue circle in b .
In accordance with the nonlinear dynamical system in equation ( 1 ), the nonlinear eigenvector and eigenvalue are defined as the state vector Ψ with components Ψ j ( r ) and the constant E that satisfy
We term the equation as a nonlinear eigenequation and analyse its bulk–boundary correspondence below. We note that we can regard the nonlinear eigenvector as a periodically oscillating steady state Ψ j ( r ; t ) = e −i E t Ψ j ( r ) of the nonlinear system when the eigenvalue is real.
To extend the Chern number to nonlinear systems, we introduce the eigenvalue problems in the wavenumber space, which is analogous to the linear eigenequation of the Bloch Hamiltonian. In a lattice system with translation symmetries, we assume an ansatz state 41 that we name the Bloch ansatz: Ψ j ( r ) = e i k · r ψ j ( k ). In linear systems, the Bloch theorem guarantees that every eigenvector is given by the form of the Bloch ansatz. On the other hand, in nonlinear systems, there can be nonlinear eigenvectors out of the description of the Bloch ansatz, including bulk-localized ones. Despite the existence of such localized modes, here we only focus on nonlinear bulk eigenvectors described by the Bloch ansatz and show that even such periodic bulk solutions can exhibit topological phenomena inherent to nonlinear systems, namely, the nonlinearity-induced topological phase transition. Under this ansatz and U (1) symmetry, one can rewrite the nonlinear eigenequation as f j ( k , ψ ( k )) = E ( k ) ψ j ( k ) parametrized by k (Supplementary Note 1 provides a detailed derivation). We note that in finite periodic systems, the Bloch-ansatz solution is still an eigenvector, whereas it can be unstable under superposition with other eigenstates.
To capture the nonlinearity-induced topological phase transition depending on amplitudes, we focus on a special solution of the nonlinear eigenvector at each k whose sum of the squared amplitudes ∑ j ∣ ψ j ( k ) ∣ 2 = w is fixed independently of wavenumber k . We note that the assumption of such fixed-amplitude Bloch-ansatz solutions is consistent with the perturbative calculation of the nonlinear eigenvectors ( Methods ). By using fixed-amplitude nonlinear eigenvectors, we define the nonlinear Chern number as
We note that this definition is reduced to the conventional linear Chern number if f defined in equation ( 2 ) is a linear function. It is also noteworthy that since special solutions of nonlinear eigenvectors should exist at arbitrary w in ordinary nonlinear systems, we can define the nonlinear Chern number at any positive w except for gap-closing points. One can prove that the nonlinear Chern number is an integer by embedding the nonlinear eigenvectors into an eigenspace of a linear Bloch Hamiltonian (Supplementary Note 2 ). The main purpose of this paper is to show the bulk–boundary correspondence for this nonlinear Chern number. Since the eigenvector can be changed by the amplitude w , the nonlinear Chern number also depends on w (Fig. 1 ). Therefore, the nonlinear Chern number can predict the nonlinearity-induced topological phase transition by the change in amplitude of nonlinear systems, which is absent in linear systems 22 , 25 , 39 , 40 , 41 , 42 , 43 . Although here we define the nonlinear Chern number in lattice systems, we can also define that in continuum systems ( Methods ).
Since the parameter w is unique to nonlinear systems, it is non-trivial how to relate the amplitude w under the periodic boundary condition to that under the open boundary condition to formulate the bulk–boundary correspondence. There are two possible choices: one can equate w under the periodic boundary condition to either the average amplitude w ave ≡ ∑ r , j ∣ Ψ j ( r ) ∣ 2 / L or the edge amplitude w edge ≡ ∑ j ∣ Ψ j ( r edge ) ∣ 2 in the model under the open boundary condition, the latter of which is used to calculate the corresponding edge modes. As shown later, both definitions can predict the topological phase transition in the continuum limit.
Previous research on one-dimensional nonlinear systems 43 has indicated the appearance of higher-order correction terms in the topological invariant due to multifrequency effects in nonlinear eigenvectors. However, under the U (1) symmetry, higher-order terms are excluded from the nonlinear Chern number. We also note that the Bloch ansatz does not describe bulk-localized solutions that can be obtained in strongly nonlinear systems, whereas the ansatz still captures nonlinearity-induced topological phenomena. Therefore, we mainly focus on weakly and more strongly nonlinear systems where the nonlinear terms are smaller than the linear terms (Supplementary Note 3 shows the Bloch-wave-like solutions of the bulk modes in this parameter region).
Nonlinear Chern number calculated from exact solutions
To investigate the bulk–boundary correspondence, that is, the correspondence between the non-zero nonlinear Chern number and the existence of the edge-localized steady state, we propose and analyse the nonlinear extension of the Qi–Wu–Zhang (QWZ) model 45 ( Methods provides the real-space description of the model). By using the Bloch ansatz, we rigorously obtain its wavenumber-space description:
where w is the squared amplitude w = ∣ ψ 1 ( k ) ∣ 2 + ∣ ψ 2 ( k ) ∣ 2 ; u and κ are dimensionless parameters of the linear and nonlinear mass terms, respectively. Here we introduce the staggered Kerr-like nonlinearity ± κ w to the linear Chern insulator model 45 .
To calculate the nonlinear Chern number, we focus on special solutions where the squared amplitude w is fixed independent of wavenumber k . Then, we can regard equation ( 4 ) as a linear equation and thus can analytically obtain the exact bulk solutions of the nonlinear eigenvalues and eigenvectors as the linear QWZ model. Using the exactly obtained nonlinear eigenvectors, we calculate the nonlinear Chern number and obtain the phase diagram shown in Fig. 2b ( Methods shows the detailed calculation and Supplementary Note 6 provides the numerical confirmation). The amplitude dependence of the nonlinear Chern number indicates the existence of the nonlinearity-induced topological phase transition in the nonlinear QWZ model. We note that since we calculate the nonlinear Chern number from the exact nonlinear eigenvectors, our result shows the existence of the nonlinearity-induced topological phase transition without any approximations. Such an analytical demonstration of the nonlinearity-induced topological phase transition is achieved by considering nonlinear equations with the form
where ψ j and k are the state variables and wavenumber, respectively. Under the existence of a more general nonlinear term, we may also define and calculate the nonlinear Chern number by appropriately defining w (Supplementary Notes 4 and 5 ).
Bulk–boundary correspondence in weakly nonlinear systems
We first numerically confirm the bulk–boundary correspondence in weakly nonlinear systems. We simulate the dynamics of the nonlinear QWZ model (equation ( 4 )) with weak nonlinearity, where the nonlinear Chern number is the same as that in the linear limit κ w → 0. In the topological phase ( C NL = ±1; Fig. 2d ), we find a long-lived localized state that corresponds to a topological edge mode in the QWZ model. Meanwhile, in the case of C NL = 0 (Fig. 2c ), the edge-localized initial state is spread to the bulk, which indicates the absence of edge modes. We also confirm the bulk–boundary correspondence from the perspective of the nonlinear band structure (Fig. 3 ; Methods provides the numerical method), which implies the utility of the nonlinear band structure to detect topological edge modes.
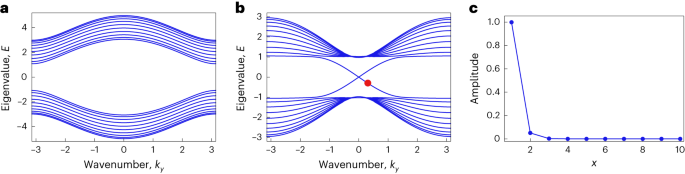
a , We numerically calculate the nonlinear band structure of the topologically trivial system under the open boundary condition in the x direction and the periodic boundary condition in the y direction. In the data shown in a and b , we relate the average amplitude w ave to the amplitude of the bulk modes w . One can confirm the absence of gapless modes. The parameters used are u = 3, κ = 0.1 and w = 0.1. b , Nonlinear band structure of the topologically non-trivial system is numerically calculated. There are gapless modes that connect the upper and lower bulk bands. The parameters used are u = −1, κ = 0.1 and w = 0.1. c , Spatial distribution of the gapless mode is presented. The eigenvalue of the localized mode corresponds to the red circle in the band structure in b .
In fact, the bulk–boundary correspondence between the nonlinear Chern number and the gapless edge modes can be established in general weakly nonlinear systems. We mathematically show the bulk–boundary correspondence under weak nonlinearity compared with the linear bandgap. Methods and Supplementary Note 7 describe the details of the theorem and its proof.
Nonlinearity-induced topological phase transition
We next show that nonlinearity-induced topological phase transitions occur in the stronger nonlinear regime, where the nonlinear Chern number becomes nonzero and topological edge modes appear at a critical amplitude. First, we consider continuum systems and analytically show such nonlinearity-induced topological phase transitions and the bulk–boundary correspondence under stronger nonlinearity. Specifically, we derive the effective theory of the low-energy dispersion of the nonlinear QWZ model as
where m = u + 2 and Ψ ( x , y ) = ( Ψ 1 ( x , y ), Ψ 2 ( x , y )) T is the state-vector function at location ( x , y ) ( Methods and Supplementary Note 8 provide the derivation). This state-dependent Hamiltonian has a similar structure to the Dirac Hamiltonian, except for the nonlinear mass term κ ( ∣ Ψ 1 ∣ 2 + ∣ Ψ 2 ∣ 2 ), and thus, we term it the nonlinear Dirac Hamiltonian. In general, the nonlinear Dirac Hamiltonian should describe the low-energy dispersions of a broad class of nonlinear topological insulators, and thus, its localized modes unveil the existence of topological edge modes in various continuum systems. By considering the right semi-infinite system which has an open boundary at x = 0 and are periodic in the y direction, and assuming the ansatz \({({{\varPsi}}_{1}(x,y),{{\varPsi}}_{2}(x,y))}^{{\rm{T}}}\) \(={{\rm{e}}}^{{\rm{i}}{k}_{y}y}\phi (x){(1/\sqrt{2},-{\rm{i}}/\sqrt{2})}^{{\rm{T}}}\) ( E = k y ), we can analytically obtain the spatial distribution of the gapless mode of the nonlinear Dirac Hamiltonian as
where D is the integral constant and –( κ / m ) + D e −2 m x must be positive.
Figure 4 summarizes the nonlinear Chern numbers ( Methods ) and the behaviours of gapless modes in the nonlinear Dirac Hamiltonian at different parameters. In the case of m < 0, where the Chern number is C = 1/2 in the linear limit, we obtain the localized states at the left side as in the linear case. These localized states are consistent with the bulk–boundary correspondence in weakly nonlinear systems, as shown earlier. We can also check that no localized modes appear in the case of positive m and κ , where the nonlinear Chern number is C NL = −1/2 at any amplitude.
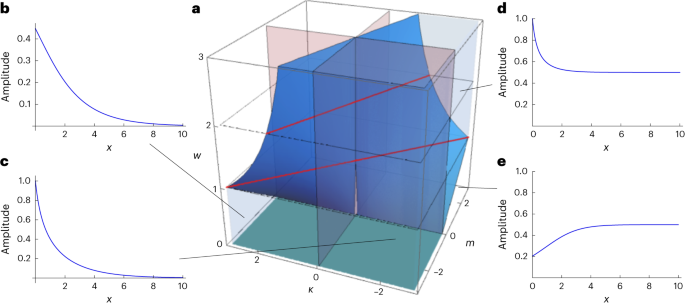
a , Phase diagram of the nonlinear Dirac Hamiltonian, which demonstrates the nonlinear bulk–boundary correspondence in the continuum model. The vertical axis represents the amplitude, and the horizontal axes correspond to the parameters of the nonlinear Dirac Hamiltonian. The blue curved surface shows the phase boundary that separates a trivial phase without boundary modes and a topological phase exhibiting localized modes at the left boundary. The red surfaces present the boundaries where the sign of the parameters of the Dirac Hamiltonian changes. The red lines show the phase boundaries at the surfaces of w = 1 and w = 2. In the linear limit ( w = 0), the topological phases are separated by the m = 0 axis, whereas the nonlinearity modifies the boundary of the topological phases. b – d , Representative shape of the localized mode in each of the topologically non-trivial parameter regions. b , When m is negative and κ is positive, we obtain a localized mode in the small-amplitude region, which is regarded as a counterpart of a conventional topological edge mode. We set m , κ and D as m = −0.5, κ = 1 and D = 3. c , When both m and κ are negative, a localized mode appears independent of the amplitude as in linear topological insulators. We set m = −0.5, κ = −1 and D = 3. d , When m is positive and κ is negative, the nonlinear Dirac Hamiltonian exhibits the nonlinearity-induced topological phase transition. We obtain an unconventional localized mode if the amplitude is larger than a critical value. In this localized mode, there exist non-vanishing amplitudes even in the limit of x → ∞. We set m = 0.5, κ = −2 and D = −3. e , We can also obtain anti-localized modes in the topologically trivial phase, which are unique to nonlinear systems. We set m = 0.5, κ = −2 and D = −20.
To discuss the bulk–boundary correspondence under stronger nonlinearity, we must relate the amplitude w under the periodic boundary condition to \({w}_{{{{\rm{ave}}}}}=\int\nolimits_{0}^{L}{\rm{d}}x| \phi (x){| }^{2}/L\) or w edge = ∣ ϕ (0) ∣ 2 of the edge-localized mode obtained under the open boundary condition. In fact, either choice can exactly predict the phase boundary as shown in the case of m > 0, κ < 0, where we obtain the localized state with the residual amplitude \(\sqrt{| m/\kappa | }\) in the limit of x → ∞. In this case, we obtain a gapless homogeneous mode \(\phi (x)=\sqrt{| m/\kappa | }\) at the phase boundary. Since both w ave and w edge of such a homogeneous mode are \({w}_{{{{\rm{ave/edge}}}}}\) \(=\sqrt{| m/\kappa | }\) and satisfy m + κ w ave/edge = 0, both definitions of the amplitude predict the nonlinearity-induced topological phase transition associated with the amplitude-dependent Chern number (Supplementary Note 9 ). We note that the non-vanishing amplitude in the limit of x → ∞ indicates that it is impossible to normalize the edge mode. In finite systems, however, such a non-vanishing localized mode can be normalized and thus can robustly emerge. We also obtain anti-localized modes satisfying ∣ ϕ (0) ∣ < ∣ ϕ ( x ) ∣ for D > 0 (Fig. 4e ), which is unique to nonlinear systems.
We can also confirm the bulk–boundary correspondence in lattice systems (Supplementary Notes 10 and 12 – 17 ). Specifically, by making the correspondence between the bulk amplitude w and edge amplitude w edge , the non-zero nonlinear Chern number corresponds to the existence of localized zero modes. We analytically show such bulk–boundary correspondence in semi-infinite systems and numerically confirm it in finite systems of the nonlinear QWZ model.
Observation protocol of edge modes via quench dynamics
One can observe the topological properties via quench dynamics, which directly detects the existence of topological edge modes. In quench dynamics, one only has to excite the edge sites at homogeneous amplitudes and observe the dynamics without any other external interactions (Fig. 5a ). To confirm the correspondence between the existence of nonlinear edge modes and the localized states in quench dynamics, we numerically simulate the quench dynamics of a nonlinear QWZ model (equation ( 4 )) at various parameters. Figure 5b,c shows the time evolution of the quench dynamics with and without nonlinear edge modes. We also obtain the phase diagrams (Fig. 5d,e ), which are classified by the amplitude at the edge of the sample in the long-time limit. Here we consider two initial states equivalent to the nonlinear edge modes at u + κ w = ±1 ( Methods ). We confirm that the localized states remain in the topological cases and vanish in the trivial cases. Identifying the bulk amplitude w as w edge = ∑ j , y ∣ Ψ j (0, y ) ∣ 2 / L y ( L y is the system size in the y direction) of the initial state, we confirm that the nonlinear Chern number C NL ( w ) roughly corresponds to the phase boundaries in the quench dynamics, which indicates that the nonlinear Chern number and its nonlinearity-induced topological phase transition can predict the existence of experimentally observable localized states.
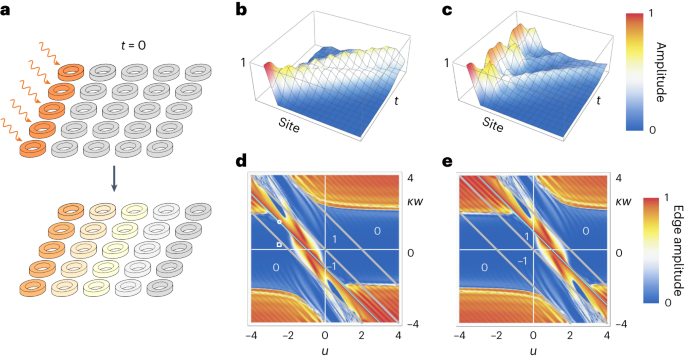
a , Schematic of the experimental protocol of the quench dynamics. First, one excites the edge sites by, for example, applying lasers to the edge resonators in nonlinear topological photonic insulators. Then, one observes the nonlinear dynamics without external fields and confirms the existence or absence of a long-lived localized state. b , Time evolution of the quench dynamics in a trivial phase. We use the parameters u = −2.5 and κ = 0.25, and set the initial edge amplitude as w = 1, which corresponds to the white square in d . We confirm the absence of localized edge modes. c , Time evolution of the quench dynamics of nonlinear edge modes. We use the parameters u = −2.5 and κ = 1.5, and set the initial edge amplitude as w = 1, which corresponds to the white circle in d . We confirm that a localized state remains for a long time, which indicates the existence of edge modes. d , e , Simulation of the quench dynamics and a plot of the amplitude remaining at the edge sites in the long-term limit after a quench in the u – κ w plane. The light-blue lines indicate the parameters where we can obtain the exact edge-localized solutions. The white numbers show the nonlinear Chern number, and the grey lines represent their phase boundaries, where we relate the amplitude w to w edge = ∑ j ∣ Ψ j ( x = 0) ∣ 2 at t = 0. These lines agree with the boundary of the topological phase, and thus, the quench dynamics shows the shift in the phase boundary by nonlinearity. We take the different initial configurations corresponding to the edge modes in the linear limit at u = −1 in d and u = 1 in e . Thus, the phase diagrams of the quench dynamics reproduce the topological phases with different Chern numbers in these panels ( C = −1 for d and C = 1 for e ).
Possible experimental setups of nonlinear Chern insulators
Nonlinear Chern insulators are expected to be realized by using topological photonics with the Kerr nonlinearity. In particular, we can replace the nonlinear term in equation ( 4 ) by on-site Kerr nonlinear terms, that is, κ ∣ Ψ j ( x , y ) ∣ 2 Ψ j ( x , y ), and such Kerr nonlinearity is feasible in various photonic systems. Supplementary Note 18 further discusses a possible optical setup and the analytical demonstration of the nonlinearity-induced topological phase transition under the on-site Kerr nonlinearity.
Discussions
Our results indicate the existence of unique topological phenomena beyond the weakly nonlinear regime. There remain intriguing issues to establish the topological classification of nonlinear systems including further strongly nonlinear cases where nonlinearity is even stronger than the linear couplings. Since such strong nonlinearity can induce bulk-localized modes 18 , 35 , 42 , 46 and topological edge solitons 30 , 31 , 32 , 33 , 34 , 35 that are out of the description of the Bloch ansatz, it is unclear whether or not the nonlinear Chern number still fully works. We can also discuss the linear stability of the Bloch-ansatz state, which may be related to the topological phase transition 28 (Supplementary Note 19 ). It may also be intriguing to investigate the connection to many-body quantum physics because nonlinear terms can be derived from the mean-field approximation 16 , 17 or the Kohn–Sham equation 47 , 48 of interacting systems in general (Supplementary Note 20 ). Therefore, the nonlinear topology may also be useful to understand the topology of interacting systems.
Justification of the Bloch ansatz via perturbation analysis
If the nonlinear term is small compared with the linear bandgap, one can regard the nonlinear effect as a perturbation to the linear band structure. Under such an assumption, one can perturbatively calculate the nonlinear eigenvectors. To show the perturbation calculation protocol of the nonlinear eigenvalue problem, we rewrite the nonlinear eigenequation as
We consider the perturbation expansion by κ as follows:
One can determine Ψ (0) and E (0) from the eigenvalue and eigenvector of the linear Hamiltonian H 0 as
Then, the first-order perturbation is calculated from the eigenequation of ( H 0 + κ H NL ( Ψ (0) )) as
One can confirm the consistency between equations ( 9 ) and ( 13 ) by substituting equations ( 10 ) and ( 11 ) into the former equation.
In translation-invariant systems, the non-perturbed eigenvector Ψ (0) is described by a Bloch wave Ψ (0) = e i k · x ψ (0) , due to the Bloch theorem of linear systems. Since the Bloch wave exhibits no site dependence of the amplitude, one can assume that the nonlinear term κ H NL ( Ψ ) is also uniform, and thus, the whole effective Hamiltonian H 0 + κ H NL ( Ψ ) still has translational symmetry. Therefore, in weakly nonlinear systems, one can believe that all of the nonlinear eigenvectors are described by the Bloch ansatz. We note that in strongly nonlinear regimes, there can be localized modes that cannot be described by the Bloch ansatz 35 , 42 , 46 . However, the periodic solutions obtained from the Bloch ansatz are still exact nonlinear eigenvectors under the periodic boundary conditions.
Nonlinear Chern number in continuum systems
In continuum systems with a periodic potential, the Bloch ansatz should read Ψ j ( r ) = e i k· r ψ j ( k , r ), where ψ j ( k , r ) is a periodic function of r whose period is equal to that of the periodic potential. Then, the wavenumber-space representation of the nonlinear eigenequation becomes f j ( k , ψ ( k ); r ) = E ( k ) ψ j ( k , r ), and the nonlinear Chern number can be written as
where S represents the unit cell of the periodic system. The squared amplitude is defined as w = ∑ j ∫ S d 2 r ∣ ψ j ( k , r ) ∣ 2 in this continuum case.
Real-space description of the nonlinear QWZ model
To investigate the existence or absence of topological edge modes in lattice systems, we construct a minimal lattice model of a nonlinear Chern insulator, which we term the nonlinear QWZ model (equation ( 4 ) shows the wavenumber-space description). Its real-space dynamics is described as
where ( j , l ) and ( x , y ) represent the internal degree of freedom and location, respectively, and Ψ j ( x , y ) is the j th component of the state vector at location ( x , y ). The values of \({({\sigma }_{i})}_{jl}\) are the ( j , l )th component of the i th Pauli matrix. This lattice model introduces the staggered Kerr-like nonlinearity −(−1) j κ ( ∣ Ψ 1 ( x , y ) ∣ 2 + ∣ Ψ 2 ( x , y ) ∣ 2 ) Ψ i ( x , y ) to the linear QWZ model 45 .
Exact bulk solutions of the nonlinear QWZ model
To obtain the phase diagram shown in Fig. 2b , we analytically solve the nonlinear eigenequation in equation ( 4 ). If we focus on special solutions where the squared amplitude ∣ Ψ 1 ( k ) ∣ 2 + ∣ Ψ 2 ( k ) ∣ 2 = w has no k dependence, the nonlinear eigenequation (equation ( 4 )) exactly corresponds to a linear one. Therefore, by solving the corresponding linear eigenequation, we obtain the following exact bulk eigenvalues and eigenvectors as
where \({c}_{\pm }\left({{{\bf{k}}}}\right)=\sqrt{{\left(u+\kappa w+\cos {k}_{x}+\cos {k}_{y}+{E}_{\pm }\left({k}_{x},{k}_{y}\right)\right)}^{2}+{\sin }^{2}{k}_{x}+{\sin }^{2}{k}_{y}}\) is a normalization constant. By using these nonlinear eigenvectors, we analytically obtain the nonlinear Chern number as
as summarized in Fig. 2b . We note that one can generally obtain exact bulk solutions if the nonlinear equation has the form in equation ( 5 ) (Supplementary Note 4 ).
Perturbation analysis of the bulk modes of the nonlinear QWZ model
Although we calculate the exact solutions of the bulk modes of the nonlinear QWZ model in the main text, we can also obtain the same bulk modes from the perturbation analysis or self-consistent calculations. Specifically, if we conduct the perturbation analysis described earlier, the calculation stops at the first-order perturbation and derives the same bulk modes as the exact solutions.
The zeroth-order solutions, that is, the linear solutions of the bulk modes of the QWZ model are
where we fix the norm as | ψ 1± ( k )| 2 + | ψ 2± ( k )| 2 = w . Then, substituting these solutions into the state-dependent Hamiltonian of the nonlinear QWZ model, one can obtain the first-order perturbation solutions. Due to the nonlinear terms depending only on the norm of the nonlinear eigenvector, the substituted effective Hamiltonian is described as
independently of the wavenumber. The eigenvalues and eigenvectors of this Hamiltonian are the same as those of the nonlinear QWZ model (equations ( 16 ) and ( 17 )), and thus, the first-order perturbation calculation is consistent with the exact solutions.
The self-consistent calculation is equivalent to the higher-order perturbation calculation (as shown later). However, if one substitutes the first-order solutions into the state-dependent Hamiltonian of the nonlinear QWZ model, one obtains the same effective Hamiltonian as equation ( 22 ). Therefore, the nonlinear eigenvectors and eigenvalues obtained from the self-consistent calculation are the same as those obtained from the first-order perturbation and exact solutions.
Numerical simulations of the real-space dynamics of the nonlinear QWZ model
In Fig. 2 , we numerically calculate the dynamics of the nonlinear QWZ model by using the fourth-order Runge–Kutta method. We consider a 20 × 20 square lattice, where each lattice point has two internal degrees of freedom. We impose the open boundary condition in the x direction and the periodic boundary condition in the y direction. We set the time step d t = 0.005. The simulation starts from the localized initial states that have non-zero values \({{\varPsi}}_{1}(x,y)=w/\sqrt{2}\) and \({{\varPsi}}_{2}(x,y)={\rm{i}}w/\sqrt{2}\) only at x = 1. We use the parameters u = 3 and κ = 0.1 in Fig. 2c and u = −1 and κ = 0.1 in Fig. 2d . We also set the initial amplitude at the edge site as w = 1. In these figures, we plot the square root of the sum of the square of absolute values of the first and second components.
Self-consistent calculation of nonlinear band structures
To obtain the nonlinear band structures in Fig. 3 , we numerically calculate the nonlinear eigenvalue problem by using the self-consistent method. We first rewrite the nonlinear dynamics as i∂ t Ψ = f ( Ψ ) = H ( Ψ ) Ψ , where H ( Ψ ) is a state-dependent effective Hamiltonian. Then, we conduct the self-consistent calculation in the following procedure. (1) We numerically diagonalize \(H(\bf{0})\) and set the initial guess of the eigenvalue and eigenvector Ψ 0 and E 0 , respectively, by adopting a pair of the obtained eigenvalue and eigenvector of \(H(\bf{0})\) . We fix the norm of Ψ 0 to be ∣ ∣ Ψ 0 ∣ ∣ 2 = w L , where L is the system size. (2) We substitute the guessed eigenvector Ψ i after i iterations into H ( Ψ ) and diagonalize H ( Ψ i ). (3) We choose the obtained eigenvalue that is the closest to the previous guess E i , and the corresponding eigenvector as the next guess E i +1 and Ψ i +1 . (4) We iterate steps (2) and (3) until the distance between Ψ i and Ψ i +1 becomes smaller than the threshold of ∣ ∣ Ψ i +1 − Ψ i ∣ ∣ < ϵ , or the iteration reaches a fixed number. We also perform these calculations starting from all the eigenvectors of \(H(\bf{0})\) and obtain a set of nonlinear eigenvectors and eigenvalues of f ( Ψ ).
In Fig. 3 , we consider the parametrized state-dependent Hamiltonian that corresponds to the nonlinear Chern insulator under the assumptions of the y -periodic Bloch ansatz and the open boundary condition in the x direction. The state-dependent Hamiltonian is described as
where Δ x and \({\Delta }_{x}^{2}\) are the difference operators defined as Δ x Ψ ( x ) = [ Ψ ( x + 1) − Ψ ( x )]/2 and \({\Delta }_{x}^{2}{{\varPsi}} (x)={{\varPsi}} (x+1)+{{\varPsi}} (x-1)-2{{\varPsi}} (x)\) , respectively. Then, we calculate the nonlinear eigenvalues of this state-dependent Hamiltonian at k y = n Δ k , where n = − N , − N + 1… N − 1, N , and Δ k = π/ N ( N = 50). Here we set the system size in the x direction as L = 10 and fix the average amplitude of the nonlinear eigenvector as ∑ x , j ∣ Ψ j ( x ; k y ) ∣ 2 / L = w independent of wavenumber k y . We note that the self-consistent calculation is only stable at weak nonlinearity. To calculate the band structures in strongly nonlinear systems than those analysed in the main text, one should instead use the Newton method ( Supplementary Methods ).
Theorem of bulk–boundary correspondence in weakly nonlinear systems
We mathematically show the bulk–boundary correspondence in weakly nonlinear systems. Here we use a simple notation \(f\,(\bf{\Psi })=E\,\bf{\Psi }\) instead of f j ( Ψ ; r ) = EΨ j ( r ), where \(\bf{\Psi }\) is the nonlinear eigenvector whose components correspond to the state variables at locations r and internal degrees of freedom j . The claim of the theorem is as follows:
Suppose \(f\,(\bf{\Psi })=E\,\bf{\Psi }\) is a nonlinear eigenvalue problem on a two-dimensional lattice system that satisfies the following assumptions. (1) When we rewrite the nonlinear function f as \(f\,(\bf{\Psi })=H(\bf{\Psi })\,\bf{\Psi }\) , there exists a positive real number c < 1 that satisfies \(| | H(\bf{\Psi })-H(\bf{0})| | < gc/2\) for any complex vector \(\bf{\Psi }\) , where g is the bandgap of \(H(\bf{0})\) and ∣ ∣ ⋅ ∣ ∣ is the operator norm. (2) There exists a positive real number c ′ < 1 such that for any pairs of complex vectors Ψ and Ψ + Δ Ψ with the norm w , they satisfy \(| | H(\,\bf{\Psi }+\Delta \bf{\Psi })-H(\,\bf{\Psi })| | \le g(1-c){c}^{{\prime} }{(6\sqrt{2}w)}^{-1}| | \Delta \bf{\Psi }| |\) . (3) For any complex vector \(\bf{\Psi }\) , one can rewrite the nonlinear function \(f(\bf{\Psi })\) as \(f(\bf{\Psi })=\tilde{H}(\bf{\Psi })\;\bf{\Psi }\) , where \(\tilde{H}\) is a Hermitian matrix. (4) The nonlinear equation satisfies the U (1) symmetry, \(f({{\rm{e}}}^{{\rm{i}}\theta }\bf{\Psi })={{\rm{e}}}^{{\rm{i}}\theta }f(\bf{\Psi })\) . We also assume that the number of nonlinear eigenvectors is equal to that of the linear eigenvectors of \(H(\bf{0})\) . Then, the nonlinear eigenequation \(f\,(\bf{\Psi })=E\,\bf{\Psi }\) exhibits robust gapless boundary modes if and only if its nonlinear Chern number is non-zero.
We note that here we relate the average amplitude w ave = ∑ r , j ∣ Ψ j ( r ) ∣ 2 / L to the amplitude used in the definition of the nonlinear Chern number. To prove the theorem, we first show the following proposition.
Proposition 1
In the nonlinear eigenvalue problem that satisfies the assumptions in the theorem, the self-consistent calculation converges, namely, E i → E ∞ and \({\bf{\Psi }}_{i}\to {\bf{\Psi }}_{\infty }\) . Furthermore, there exists an eigenvector \({\bf{\Psi }}_{0}\) and eigenvalue E 0 of \(H(\bf{0})\) that satisfy ∣ ∣ E ∞ – E 0 ∣ ∣ < gc /2(1 – c ′) and \(| |\;{\bf{\Psi }}_{\infty }-{\bf{\Psi }}_{0}| | < {2}^{-1/2}c{(1-2{c}^{{\prime} })}^{-1}w\) .
To show this proposition, we utilize the perturbation theorem of the eigenvectors in linear systems. Suppose H is a non-degenerate Hermitian matrix and g is the minimum difference between its two eigenvalues. If ∣ ∣ A ∣ ∣ < g /2 in terms of the operator norm, for an arbitrary eigenvector \(\bf{\Psi }\) of H + A , there exists an eigenvector \({\bf{\Psi }}_{0}\) of H that satisfies \(| |\;\bf{\Psi }-{\bf{\Psi }}_{0}| | \le D{g}^{-1}| | A| | \,| |\;{\bf{\Psi }}_{0}| |\) ( \(D=2\sqrt{2}\) ). We iteratively use this theorem and evaluate the distance between the guess of eigenvectors \({\bf{\Psi }}_{i}\) and \({\bf{\Psi }}_{i+1}\) at each step.
We also show that the resulting eigenvalue and eigenvector, namely, E ∞ and \({\bf{\Psi }}_{\infty }\) , respectively, are indeed a pair of nonlinear eigenvalue and eigenvector of \(f(\bf{\Psi })\) , which is summarized below.
Proposition 2
The converged solutions of the self-consistent calculation E ∞ and \({\bf{\Psi }}_{\infty }\) satisfy \(f(\,{\bf{\Psi }}_{\infty })={E}_{\infty }{\bf{\Psi }}_{\infty }\) .
We show this proposition by using simple inequalities and limit evaluations. By using these propositions, we finally show the following lemma to prove the theorem.
For an arbitrary eigenvector of a nonlinear eigenvalue problem \((H+f(\,\bf{\Psi }))\,\bf{\Psi }=E\,\bf{\Psi }\) that satisfies the conditions in the theorem, there exists the eigenvector of \([H+(1-\epsilon )\,f(\,{\bf{\Psi }}_{\epsilon })]\,{\bf{\Psi }}_{\epsilon }=E\,{\bf{\Psi }}_{\epsilon }\) that satisfies \(| |\,\bf{\Psi }-{\bf{\Psi }}_{\epsilon }| | < Cw\epsilon\) (0 < ε < g ′/ g , where g ′ is the minimum difference of the eigenvalues of \(H+f(\bf{\Psi })\) ), where w is the norm of \(\bf{\Psi }\) and \({\bf{\Psi }}_{\epsilon }\) and C is a constant independent of the eigenvector \(\bf{\Psi }\) and constant ϵ .
This lemma indicates that in weakly nonlinear systems, one can map the nonlinear eigenvalues and eigenvectors onto those of a linear eigenvalue problem. Thus, we can show the bulk–boundary correspondence in weakly nonlinear systems.
Derivation of the nonlinear Dirac Hamiltonian and its gapless mode
We have derived the nonlinear Dirac Hamiltonian (equation ( 25 )) as the effective theory of the low-energy dispersion of the nonlinear QWZ model around the critical amplitude. For example, if we focus on the critical amplitude w c = (−2 − u )/ κ , the nonlinear band structure of the model closes the gap at ( k x , k y ) = (0, 0). Then, around the critical amplitude w ≈ w c and the gap-closing point ( k x , k y ) ≈ (0, 0), w remains the leading order term of the wavenumber-space description of the nonlinear QWZ model. Finally, by substituting the wavenumbers with the derivative, we derive the real-space description of the nonlinear Dirac Hamiltonian in equation ( 25 ) as
Starting from other critical amplitudes w c = − u / κ and w c = (2 − u )/ κ , one can derive similar state-dependent Hamiltonians (Supplementary Note 8 ).
We have also analytically calculated the spatial distribution of the gapless modes of the nonlinear Dirac Hamiltonian. Here we assume the Bloch ansatz \({{\varPsi}}_{i}(x,y)={{\rm{e}}}^{{\rm{i}}{k}_{y}y}{{\varPsi}}_{i}^{{\prime} }(x)\) that is periodic in the y direction and consider the semi-infinite system that has an open boundary at x = 0 and is extended to x → ∞. We calculate the localized mode with the wavenumber k y and the eigenvector E = k y . Constructing an analogy to the linear case, one can use an ansatz \({({{\varPsi}}_{1}(x,y),{{\varPsi}}_{2}(x,y))}^{{{T}}}={{\rm{e}}}^{{\rm{i}}{k}_{y}y}\phi (x){(1/\sqrt{2},-{{i}}/\sqrt{2})}{\,}^{{{T}}}\) to describe the localized mode. Then, ϕ ( x ) should satisfy ∂ x ϕ ( x ) = m ϕ ( x ) + κ ∣ ϕ ( x ) ∣ 2 ϕ ( x ). We can analytically obtain the solution of this equation as
where D is the integral constant and −( κ / m ) + D e −2 m x must be positive.
Nonlinear Chern number of the nonlinear Dirac Hamiltonian
To calculate the nonlinear Chern number of the nonlinear Dirac Hamiltonian (equation ( 25 )), we use the Bloch ansatz Ψ i ( x , y ) = ψ i ( k )exp(i( k x x + k y y )) (without explicit ( x , y ) dependence because of the continuous translational symmetry). Then, we analytically obtain the nonlinear Chern number of the nonlinear Dirac Hamiltonian as
where w = ∫ S d S [ ∣ Ψ 1 ( x , y ) ∣ 2 + ∣ Ψ 2 ( x , y ) ∣ 2 ]/ ∣ S ∣ is the average squared amplitude of plane waves in this nonlinear system. We note that the nonlinear Chern number of the nonlinear QWZ model corresponds to the sum of those of the nonlinear Dirac Hamiltonians obtained from the expansion around the gap-closing points ( k x , k y ) = (0, 0), (0, π), (π, 0), (π, π).
Numerical calculations of the quench dynamics
We have numerically solved the nonlinear Schrödinger equation (equation ( 15 )) with the initial condition localized at the left edge (Fig. 5a ). As the initial conditions, we study the two cases. One is
which corresponds to the solution with u = −1 of the linear model ( κ = 0). The other is
which corresponds to the solution with u = 1 of the linear model ( κ = 0). Supplementary Note 13 shows the derivation. For the numerical calculation, we used the NDSolve function in Mathematica (version 13.3). We consider a L × L lattice (we set L = 10 in the numerical calculation) under the open boundary condition in the x direction and the periodic boundary condition in the y direction.
We solve the nonlinear Schrödinger equation (equation ( 15 )) under the two initial conditions in equations ( 28 ) and ( 29 ). The time evolution of | Ψ 1 ( x , y )| 2 + | Ψ 2 ( x , y )| 2 is shown in Fig. 5d,e . We define the phase indicator P as
where T = 10. We have P ≈ 0 in the trivial phase (Fig. 5b ), which implies the absence of localized edge states. On the other hand, we have P ≈ 1 in a topological phase (Fig. 5c ), which implies the presence of a localized edge mode. To elucidate the topological phase diagram, we plot P in the u – κ w plane (Fig. 5d,e ). We find that the phase indicator is 1 along the blue line, where the exact solution is valid. It shows that the exact solution is realized by the quench dynamics.
To compare the phase boundary obtained from the quench dynamics and the nonlinear Chern number, we relate the bulk amplitude w with the edge amplitude w edge = 1. Then, grey lines in Fig. 5 show the phase boundary of the nonlinear Chern number. This definition of the amplitude under the open boundary condition is different from that used in the calculation of the nonlinear band structures because we should choose the proper definition to observe the bulk–boundary correspondence in each numerical method for demonstrating the nonlinear edge modes ( Supplementary Methods ).
Data availability
All relevant data to interpret the results of this study are included in the figures. All other data that support the plots within this paper and other findings of this study are available from the corresponding author upon reasonable request.
Kane, C. L. & Mele, E. J. Z 2 topological order and the quantum spin Hall effect. Phys. Rev. Lett. 95 , 146802 (2005).
Article ADS Google Scholar
Hazan, M. Z. & Kane, C. L. Colloquium: topological insulators. Rev. Mod. Phys. 82 , 3045–3067 (2010).
Klitzing, K. V., Dorda, G. & Pepper, M. New method for high-accuracy determination of the fine-structure constant based on quantized Hall resistance. Phys. Rev. Lett. 45 , 494–497 (1980).
Thouless, D. J., Kohmoto, M., Nightingale, M. P. & Nijs, M. D. Quantized Hall conductance in a two-dimensional periodic potential. Phys. Rev. Lett. 49 , 405–408 (1982).
Lu, L., Joannopoulos, J. D. & Soljačić, M. Topological photonics. Nat. Photon. 8 , 821–829 (2014).
Ningyuan, J., Owens, C., Sommer, A., Schuster, D. & Simon, J. Time- and site-resolved dynamics in a topological circuit. Phys. Rev. X 5 , 021031 (2015).
Google Scholar
Jotzu, G. et al. Experimental realization of the topological Haldane model with ultracold fermions. Nature 515 , 237–240 (2014).
Yang, Z. et al. Topological acoustics. Phys. Rev. Lett. 114 , 114301 (2015).
Kane, C. L. & Lubensky, T. C. Topological boundary modes in isostatic lattices. Nat. Phys. 10 , 39–45 (2013).
Article Google Scholar
Boyd, R. W. Nonlinear Optics (Academic Press, 2003).
Smirnova, D., Leykam, D., Chong, Y. & Kivshar, Y. Nonlinear topological photonics. Appl. Phys. Rev. 7 , 021306 (2020).
Ota, Y. et al. Active topological photonics. Nanophotonics 9 , 547–567 (2020).
Acebrón, J. A., Bonilla, L. L., Pérez, V. C. J., Ritort, F. & Spigler, R. The Kuramoto model: a simple paradigm for synchronization phenomena. Rev. Mod. Phys. 77 , 137–185 (2005).
Strogatz, S. H. Nonlinear Dynamics and Chaos with Student Solutions Manual: With Applications to Physics, Biology, Chemistry, and Engineering (CRC Press, 2018).
Marchetti, M. C. et al. Hydrodynamics of soft active matter. Rev. Mod. Phys. 85 , 1143–1189 (2013).
Gross, E. P. Structure of a quantized vortex in boson systems. Nuovo Cim. 20 , 454–477 (1961).
Article ADS MathSciNet Google Scholar
Pitaevskii, L. P. Vortex lines in an imperfect Bose gas. Sov. Phys. JETP 13 , 451–454 (1961).
MathSciNet Google Scholar
Lumer, Y., Plotnik, Y., Rechtsman, M. C. & Segev, M. Self-localized states in photonic topological insulators. Phys. Rev. Lett. 111 , 243905 (2013).
Bomantara, R. W., Zhao, W., Zhou, L. & Gong, J. Nonlinear Dirac cones. Phys. Rev. B 96 , 121406 (2017).
Harari, G. et al. Topological insulator laser: theory. Science 359 , 1230 (2018).
Zangeneh-Nejad, F. & Fleury, R. Nonlinear second-order topological insulators. Phys. Rev. Lett. 123 , 053902 (2019).
Maczewsky, L. J. et al. Nonlinearity-induced photonic topological insulator. Science 370 , 701–704 (2020).
Lo, P. W. et al. Topology in nonlinear mechanical systems. Phys. Rev. Lett. 127 , 076802 (2021).
Jürgensen, M., Mukherjee, S. & Rechtsman, M. C. Quantized nonlinear Thouless pumping. Nature 596 , 63–67 (2021).
Mochizuki, K., Mizuta, K. & Kawakami, N. Fate of topological edge states in disordered periodically driven nonlinear systems. Phys. Rev. Research 3 , 043112 (2021).
Fu, Q., Wang, P., Kartashov, Y. V., Konotop, V. V. & Ye, F. Nonlinear Thouless pumping: solitons and transport breakdown. Phys. Rev. Lett. 128 , 154101 (2022).
Mostaan, N., Grusdt, F. & Goldman, N. Quantized topological pumping of solitons in nonlinear photonics and ultracold atomic mixtures. Nat. Commun. 13 , 5997 (2022).
Leykam, D., Smolina, E., Maluckov, A., Flach, S. & Smirnova, D. A. Probing band topology using modulational instability. Phys. Rev. Lett. 126 , 073901 (2021).
Chen, B. G., Upadhyaya, N. & Vitelli, V. Nonlinear conduction via solitons in a topological mechanical insulator. Proc. Natl Acad. Sci. USA 111 , 13004–13009 (2014).
Leykam, D. & Chong, Y. D. Edge solitons in nonlinear-photonic topological insulators. Phys. Rev. Lett. 117 , 143901 (2016).
Zhang, Z. et al. Observation of edge solitons in photonic graphene. Nat. Commun. 11 , 1902 (2020).
Ivanov, S. K., Kartashov, Y. V., Maczewsky, L. J., Szameit, A. & Konotop, V. V. Edge solitons in Lieb topological Floquet insulator. Opt. Lett. 45 , 1459–1462 (2020).
Mukherjee, S. & Rechtsman, M. C. Observation of unidirectional solitonlike edge states in nonlinear Floquet topological insulators. Phys. Rev. X 11 , 041057 (2021).
Li, R. et al. Topological bulk solitons in a nonlinear photonic Chern insulator. Commun. Phys. 5 , 275 (2022).
Ezawa, M. Nonlinearity-induced chiral solitonlike edge states in Chern systems. Phys. Rev. B 106 , 195423 (2022).
Kotwal, T. et al. Active topolectrical circuits. Proc. Natl Acad. Sci. USA 118 , e2106411118 (2021).
Sone, K., Ashida, Y. & Sagawa, T. Topological synchronization of coupled nonlinear oscillators. Phys. Rev. Research 4 , 023211 (2022).
Wächtler, C. W. & Platero, G. Topological synchronization of quantum van der Pol oscillators. Phys. Rev. Research 5 , 023021 (2023).
Hadad, Y., Khanikaev, A. B. & Alù, A. Self-induced topological transitions and edge states supported by nonlinear staggered potentials. Phys. Rev. B 93 , 155112 (2016).
Darabi, A. & Leamy, M. J. Tunable nonlinear topological insulator for acoustic waves. Phys. Rev. Appl. 12 , 044030 (2019).
Tuloup, T., Bomantara, R. W., Lee, C. H. & Gong, J. Nonlinearity induced topological physics in momentum space and real space. Phys. Rev. B 102 , 115411 (2020).
Ezawa, M. Nonlinearity-induced transition in the nonlinear Su-Schrieffer-Heeger model and a nonlinear higher-order topological system. Phys. Rev. B 104 , 235420 (2021).
Zhou, D., Rocklin, D. Z., Leamy, M. & Yao, Y. Topological invariant and anomalous edge modes of strongly nonlinear systems. Nat. Commun. 13 , 3379 (2022).
Li, F., Wang, J., Cui, D., Xue, K. & Yi, X. X. Bloch band structures and linear response theory of nonlinear systems. Int. J. Mod. Phys. B 0 , 2450322 (2023).
Qi, X. L., Wu, Y. S. & Zhang, S. C. Topological quantization of the spin Hall effect in two-dimensional paramagnetic semiconductors. Phys. Rev. B 74 , 085308 (2006).
Eilbeck, J. C., Lomdahl, P. S. & Scott, A. C. The discrete self-trapping equation. Phys. D 16 , 318–338 (1985).
Article MathSciNet Google Scholar
Strandberg, T. O., Canali, C. M. & MacDonald, A. H. Calculation of Chern number spin Hamiltonians for magnetic nano-clusters by DFT methods. Phys. Rev. B 77 , 174416 (2008).
Dongbin, S. et al. Unraveling materials Berry curvature and Chern numbers from real-time evolution of Bloch states. Proc. Natl Acad. Sci. USA 116 , 4135–4140 (2019).
Download references
Acknowledgements
We thank Z. Gong, T. Morimoto, T. Sawada, H. Watanabe and T. Yoshida for valuable discussions. K.S. is supported by the World-Leading Innovative Graduate Study Program for Materials Research, Information, and Technology (MERIT-WINGS) of the University of Tokyo. K.S. is also supported by JSPS KAKENHI grant no. JP21J20199. M.E. is supported by JST, CREST grant no. JPMJCR20T2 and Grants-in-Aid for Scientific Research from MEXT KAKENHI (grant no. 23H00171). Y.A. acknowledges support from the Japan Society for the Promotion of 511 Science (JSPS) through grant no. JP19K23424 and from JST FOREST Program (grant no. 512 JPMJFR222U, Japan) and JST CREST (grant no. JPMJCR23I2, Japan). N.Y. acknowledges support from the Japan Science and Technology Agency (JST) PRESTO under grant no. JPMJPR2119 and JST grant no. JPMJPF2221. T.S. is supported by JSPS KAKENHI grant no. JP19H05796, JST, CREST grant no. JPMJCR20C1 and JST ERATO-FS grant no. JPMJER2204. N.Y. and T.S. are also supported by the Institute of AI and Beyond of the University of Tokyo and JST ERATO grant no. JPMJER2302, Japan.
Author information
Authors and affiliations.
Department of Applied Physics, The University of Tokyo, Bunkyo-ku, Japan
Kazuki Sone, Motohiko Ezawa, Nobuyuki Yoshioka & Takahiro Sagawa
Department of Physics, University of Tokyo, Bunkyo-ku, Japan
Yuto Ashida
Institute for Physics of Intelligence, University of Tokyo, Hongo, Japan
Theoretical Quantum Physics Laboratory, RIKEN Cluster for Pioneering Research (CPR), Wako-shi, Japan
Nobuyuki Yoshioka
Japan Science and Technology Agency (JST), PRESTO, Kawaguchi, Japan
Quantum-Phase Electronics Center (QPEC), The University of Tokyo, Bunkyo-ku, Japan
Takahiro Sagawa
You can also search for this author in PubMed Google Scholar
Contributions
K.S., M.E., Y.A., N.Y. and T.S. planned the project. K.S. and M.E. performed the analytical and numerical calculations. K.S., M.E., Y.A., N.Y. and T.S. analysed and interpreted the results and wrote the paper.
Corresponding author
Correspondence to Kazuki Sone .
Ethics declarations
Competing interests.
The authors declare no competing interests.
Peer review
Peer review information.
Nature Physics thanks the anonymous reviewers for their contribution to the peer review of this work.
Additional information
Publisher’s note Springer Nature remains neutral with regard to jurisdictional claims in published maps and institutional affiliations.
Supplementary information
Supplementary information.
Supplementary Figs. 1–13, Notes 1–20, Table 1 and Methods.
Supplementary Video 1
Numerical simulation for the data in Fig. 2c.
Supplementary Video 2
Numerical simulation for the data in Fig. 2d.
Supplementary Video 3
Numerical simulation for the data in Supplementary Fig. 7a.
Supplementary Video 4
Numerical simulation for the data in Supplementary Fig. 7b.
Rights and permissions
Open Access This article is licensed under a Creative Commons Attribution 4.0 International License, which permits use, sharing, adaptation, distribution and reproduction in any medium or format, as long as you give appropriate credit to the original author(s) and the source, provide a link to the Creative Commons licence, and indicate if changes were made. The images or other third party material in this article are included in the article’s Creative Commons licence, unless indicated otherwise in a credit line to the material. If material is not included in the article’s Creative Commons licence and your intended use is not permitted by statutory regulation or exceeds the permitted use, you will need to obtain permission directly from the copyright holder. To view a copy of this licence, visit http://creativecommons.org/licenses/by/4.0/ .
Reprints and permissions
About this article
Cite this article.
Sone, K., Ezawa, M., Ashida, Y. et al. Nonlinearity-induced topological phase transition characterized by the nonlinear Chern number. Nat. Phys. (2024). https://doi.org/10.1038/s41567-024-02451-x
Download citation
Received : 27 October 2023
Accepted : 22 February 2024
Published : 11 April 2024
DOI : https://doi.org/10.1038/s41567-024-02451-x
Share this article
Anyone you share the following link with will be able to read this content:
Sorry, a shareable link is not currently available for this article.
Provided by the Springer Nature SharedIt content-sharing initiative
Quick links
- Explore articles by subject
- Guide to authors
- Editorial policies
Sign up for the Nature Briefing newsletter — what matters in science, free to your inbox daily.


Data to accompany microseismic analysis, Clearfield County, PA hydraulic fracturing operation (PhD Thesis and Paper)
This passive seismic dataset was analyzed as part of the PhD Thesis by David Rampton, "A Comprehensive Geophysical Analysis to Determine Induced Fracture Distribution from a Hydraulic Fracturing Operation in the Marcellus Shale Formation", March 2014 and will be presented in an upcoming paper. This data was acquired in conjunction with a timelapse crosswell dataset, data also available on Kilthub. The entire dataset is available on NETL's data exchange EDX, but this paper only analyzed four stages. The raw data is included, along with contractor information, final results, and scripts used to generate intermediate and final results.
This research was supported in part by an appointment to the U.S. Department of Energy (DOE) Postgraduate Research Program at the National Energy Technology Laboratory administered by the Oak Ridge Institute for Science and Education.
Usage metrics.

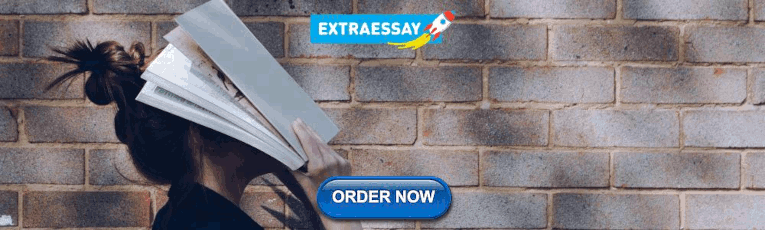
IMAGES
VIDEO
COMMENTS
Additional PhD funding opportunities at Swansea though CDTs that could cover projects in topological data analysis (both of these are unfortunately open only to EU/UK residents: • EPSRC multi-disciplinary Centre for Doctoral Training in Human-Centred AI and Data Science.
Andrew received his PhD in 2005 from University of Chicago. Andrew has broad research interests in mathematics and computer science. His research includes work in algebraic topology, topological data analysis, and computer security and privacy.
1 Introduction and Motivation. Topological data analysis (tda) is a recent field that emerged from various works in applied (algebraic) topology and computational geometry during the first decade of the century.Although one can trace back geometric approaches to data analysis quite far into the past, tda really started as a field with the pioneering works of Edelsbrunner et al. (2002) and ...
The emerging field of topological data analysis (TDA) provides a number of potential avenues for addressing some of these issues. TDA encompasses a set of tools for data visualization, exploration, and analysis that are grounded in topology, an area of mathematics that studies abstract notions of shape and connectivity.
Our research. We study a range of problems in topological data analysis. At the graduate level and beyond, our main focus is on developing tools and their underlying mathematics. At the undergraduate level the main focus is on applying topological data analysis and computations. However, there is a mix of these aspects at all levels.
3.8 Euler Characteristics in Topological Data Analysis 228 3.9 Exploratory Data Analysis with Mapper 231 3.10 Summary 233 3.11 Suggestions for Further Reading 234 4 Dimensionality Reduction, Manifold Learning, and Metric Geometry 235 4.1 A Quick Refresher on Eigenvectors and Eigenvalues 238 4.2 Background on PCA and MDS 239 4.3 Manifold ...
Topological Data Analysis (TDA) is an emerging and highly successful approach to Big Data problems. TDA represents a unique intersection point of pure mathematics with applied mathematics; the underlying idea is to employ topological techniques in the analysis of large quantities of data. One of the main difficulties with real-world data is ...
Supervisor: Dr Omer Bobrowski. Project description: Recently discovered, the phenomenon of universality in Topological Data Analysis (TDA) offers a new direction to data analysis. This project will explore its applications and develop new methodologies on top of this phenomenon. There are many possible directions but the initial focus will be on:
Hyunnam Ryu is a PhD candidate in the Department of Statistics at the University of Georgia. She received a bachelor's degree in statistics and mathematics and a master's degree in statistics at Kyungpook National University, South Korea. She is currently working on topological data analysis for network data.
Topological data analysis (tda) is a recent and fast-growing field providing a set of new topological and geometric tools to infer relevant features for possibly complex data. It proposes new well-founded mathematical theories and computational tools that can be used independently or in combination with other data analysis and statistical ...
The PhD will be based in the Faculty of Technology, and will be supervised by Dr Ittay Weiss. The work on this project could involve: Developing new techniques in frontiers of topological data analysis. Synergising advanced methods of algebraic topology to facilitate new applications and enhance existing ones. Developing new algorithms based on ...
topology, and he has spent the last 20 years on the development of topological data analysis. He is also passionate about the transfer of scientiÞc Þndings to real-world applications, leading him to found the topological data analysis-based company Ayasdi in 2008. Mikael Vejdemo-Johansson is Assistant Professor in the Department of Mathematics
M. Fraser, Group Actions in Topological Data Analysis and Hierarchical Learning. PhD Thesis, Dept. of Computer Science, University of Chicago, August 2013. M. Fraser, Tight Linear Lower Memory Bound for Local Routing in Planar Digraphs. In Proceedings of Canadian Conference on Computational Geometry (CCCG12), August 2012. (proceedings pdf)
Figure 2. A barcode captures topological features in a dataset at multiple scales. The topology of a dataset at a fixed scale is determined by joining pairs of data points with an edge if the distances between the pair of points is less than the fixed scale. If three edges form a triangle, then the triangle is filled in.
Parameterized Topological Data Analysis. Brad Nelson Institute for Computational and Mathematical Engineering Stanford University. May 5, 2020 bnels.github.io/phd-talk. Collaborators/Support. Collaborators who appear in this work Gunnar Carlsson; Anjan Dwaraknath; Funding I've received while working on these topics DoD through NDSEG fellowship
Chad Giusti is an assistant professor of mathematics at Oregon State University. He works in pure and applied topology, with applications principally in neuroscience and complex systems. Here, we learn about the fascinating work Chad has done in applying the tools of topological data analysis to problems in medicine and biology.
Topological data analysis (tda) is a recent and fast-growing field providing a set of new topological and geometric tools to infer relevant features for possibly complex data. It proposes new well ...
Keywords. Topological data analysis, Deep learning, Gene expression, Cancer Phenotype prediction 1 Introduction 1.1 Topology Overview Topological data analysis (TDA) is a powerful method for extracting a set of refined, robust quantitative features on the structure of data by translating the data and encoding it into shape [1].
Topological data analysis refers to approaches for system-atically and reliably computing abstract 'shapes' of complex data sets. There are various applications of topological data analysis in life and data sciences, with growing interest among physicists. We present a concise review of applica-tions of topological data analysis to physics ...
Most of the data science done in the current corporate setting concerns itself with the analysis of a finite collection of points in a vector space. Granted, there are (sometimes many) difficult ...
In this work, we want to explore how Topological Data Analysis [8] can be used for the exploitation of feature presence probability fields generated by deep- learning algorithms, in the context of fault extraction for geosciences. In particular, we would like to focus on the Morse-Smale complex [9], which is a topolog- ical object that is, in ...
The field of Topological Data Analysis (TDA) applies higher-level techniques from the mathematical field of Topology to the study of datasets. In this course we will introduce mathematical theory of TDA as well as practicing the actual application through programming. Course Overview. This online course is part of VU Amsterdam Graduate ...
In Proceedings of 11th International Symposium on Parallel and Distributed Computing (ISPDC), pp. 87-94, 2012. In Topological Methods in Data Analysis and Visualization II, Springer Berlin Heidelberg, pp. 269-281, 2012. IEEE Transactions on Visualization and Computer Graphics, vol. 17, no. 6, pp. 781-794, 2011.
conducting research in the area of topological data analysis (85%) contributing to the teaching portfolio of the Department of Mathematics (15%) ... PhD candidates and researchers at the biggest sciences faculty in the Netherlands. You will combine a professional focus with a broad view of the world. We are proud of our collegial working ...
This work will be integrated into the educational program of the PI through the creation of an online TDA (Topological Data Analysis) academy, with the dual purpose of lowering the barrier of entry into the field for data scientists and academics, as well as increasing the representation of underserved communities in the field of computational ...
As first demonstrated by the characterization of the quantum Hall effect by the Chern number, topology provides a guiding principle to realize the robust properties of condensed-matter systems ...
This passive seismic dataset was analyzed as part of the PhD Thesis by David Rampton, "A Comprehensive Geophysical Analysis to Determine Induced Fracture Distribution from a Hydraulic Fracturing Operation in the Marcellus Shale Formation", March 2014 and will be presented in an upcoming paper. This data was acquired in conjunction with a timelapse crosswell dataset, data also available on ...