
Researched by Consultants from Top-Tier Management Companies
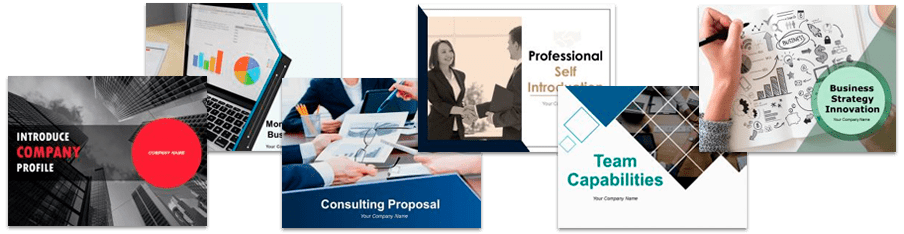
Powerpoint Templates
Icon Bundle
Kpi Dashboard
Professional
Business Plans
Swot Analysis
Gantt Chart
Business Proposal
Marketing Plan
Project Management
Business Case
Business Model
Cyber Security
Business PPT
Digital Marketing
Digital Transformation
Human Resources
Product Management
Artificial Intelligence
Company Profile
Acknowledgement PPT
PPT Presentation
Reports Brochures
One Page Pitch
Interview PPT
All Categories
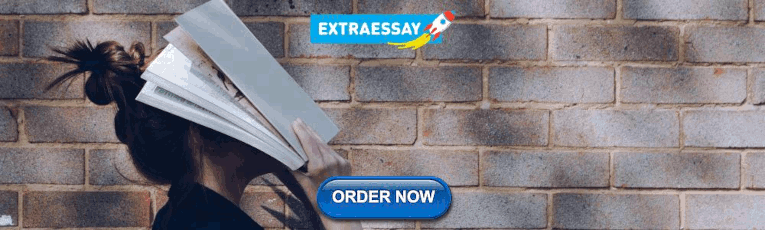
Top 7 Data Governance Templates with Examples and Samples
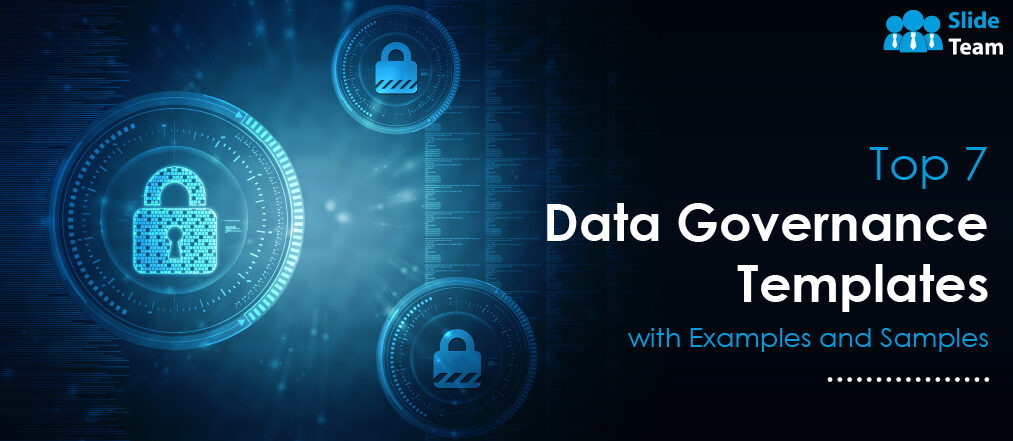
Mohammed Sameer

In the binary world of ones and zeros, where data is the key currency, data governance is the emerging business sunrise sector. With data emerging as the lifeblood of modern business, data governance has risen as its watchful, vigilant guardian, ensuring that the flow of information through data remains purposeful and productive.
To use this well, data governance templates emerge as the strategic anchors. Imagine your data as fundamental threads awaiting skillful interlacing into the intricate fabric of knowledge. These PPT Presentations serve as adept craftsmen, weaving data elements into a network of insights conducive to actionable decision-making. Whether you are a seasoned data strategist, a business analyst, or a researcher, these templates extend beyond just being a mere compass. These provide an all-encompassing map for informed and strategic navigation.
The 100% customizable nature of the templates provides you with the desired flexibility to edit your presentations. The content-ready slides give you the much-needed structure.
Let’s explore!
Template 1: Data Governance PPT Deck
This comprehensive PPT Template delves deep into core aspects of effective data governance. It offers insights, addressing crucial facets that include the necessity for robust data governance and the common challenges that companies face. The differentiating factors between manual and automated data governance approaches are also outlined. Use this template to navigate through a structured breakdown of the data governance framework, empowering you to comprehend its complex layers with ease. Have a better understanding of diverse roles and responsibilities that constitute a well-functioning data governance ecosystem. This PPT Set also illuminates strategies for initiating a successful data governance program. From outlining methodologies to establishing a governance structure, each step is covered to perfection. Explore an in-depth roadmap for enhancing your data governance endeavors, ensuring continuous improvements in line with your evolving business needs. Get it today!

Download this template
Template 2: Data Governance Strategy PPT Set
This PPT Set presents a roadmap for efficient data governance strategy implementation. This template guides you through the stages of data definition and migration, data standards, validation, automation, prevention of duplication, and resolution. This PPT Layout illustrates essential steps for strategic data governance. It covers every cornerstone, from the assessment of your organizational framework to developing a governance charter, an actionable implementation plan, and the strategic definition of roles and resource enablement. Get it today!
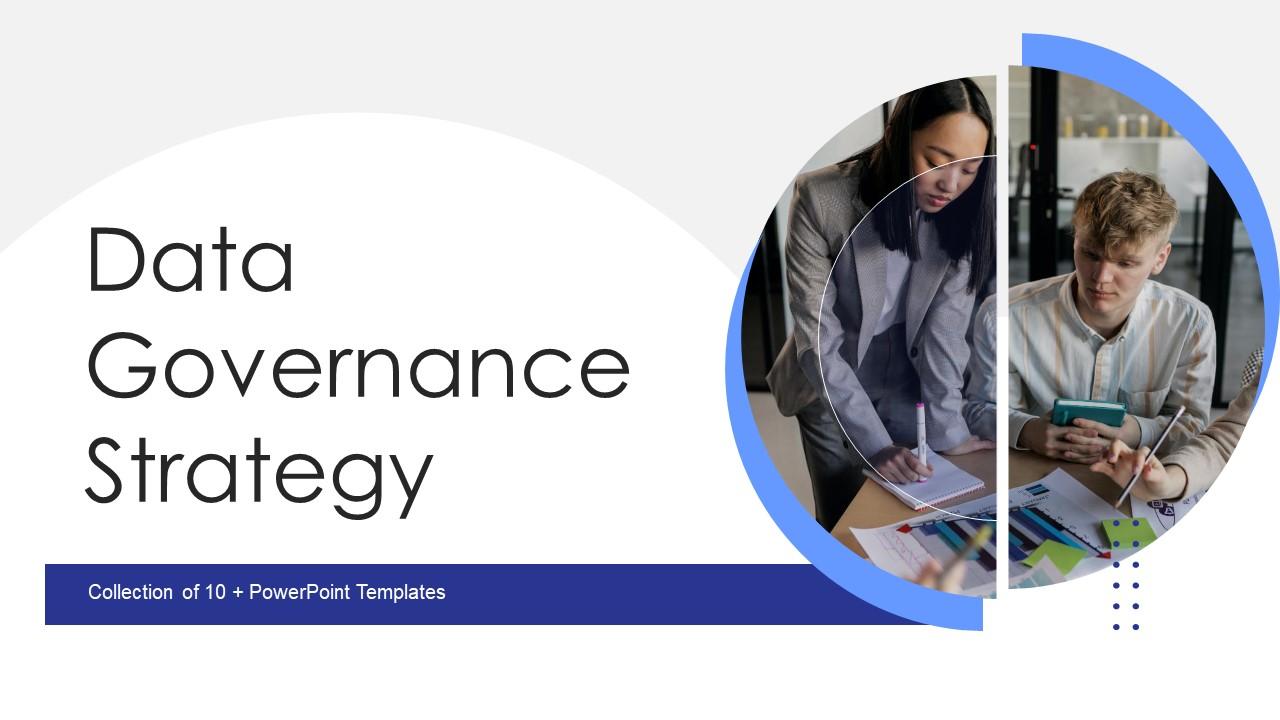
Get this template
Template 3: Data Governance Framework PPT Design
This carefully-crafted resource PPT Template simplifies the complexities of the data governance framework, while highlighting the theme of continuous improvement. It presents easy-to-understand insights covering Policies and Standards, Compliance and Security, Information Quality, and Architecture and Integration. Each facet is detailed to provide a holistic understanding. The presentation template goes beyond the surface to explore the bedrock principles that underpin an effective data governance framework.
Discover how Accountability and Right Decision-Making form the backbone, instilling a sense of responsibility that drives your data strategy forward. Delve into the Trust Model, Collaboration and Culture, and Transparency and Ethics, where you get the basic tenets that govern a robust data fabric. Security is paramount, and this PPT Set doesn’t shy away from addressing risk and security head-on. Learn how to safeguard your data assets, while fostering a culture of innovation. Use this template to discover the value that stems from data-driven insights. The Value and Outcomes principle ensures you’re not just managing data, but harnessing it to take your organization to success. Download now!

Grab this template
Template 4: Enterprise Data Governance Framework with Change Management
Use this PPT Set to explore crucial layers that pave the way for effective data management. It starts with the Strategic Layer, where Governance Strategy and Vision converge to provide a clear direction. Dive into the DG Stewardship and Core Layer to explore the intricacies of Program Management Organization, ensuring that every facet of your data governance is structured well. This PPT Template also provides you Data Governance Enablers, even dissecting the Process Model. Integrate processes that empower your data governance framework, ensuring smooth operations and strategic alignment. Lastly, explore the Data Management Layer, a domain dedicated to Information Lifecycle Management. Witness your data evolve, from creation to archival, guided by best practices that optimize its utility and lifespan. Download now!
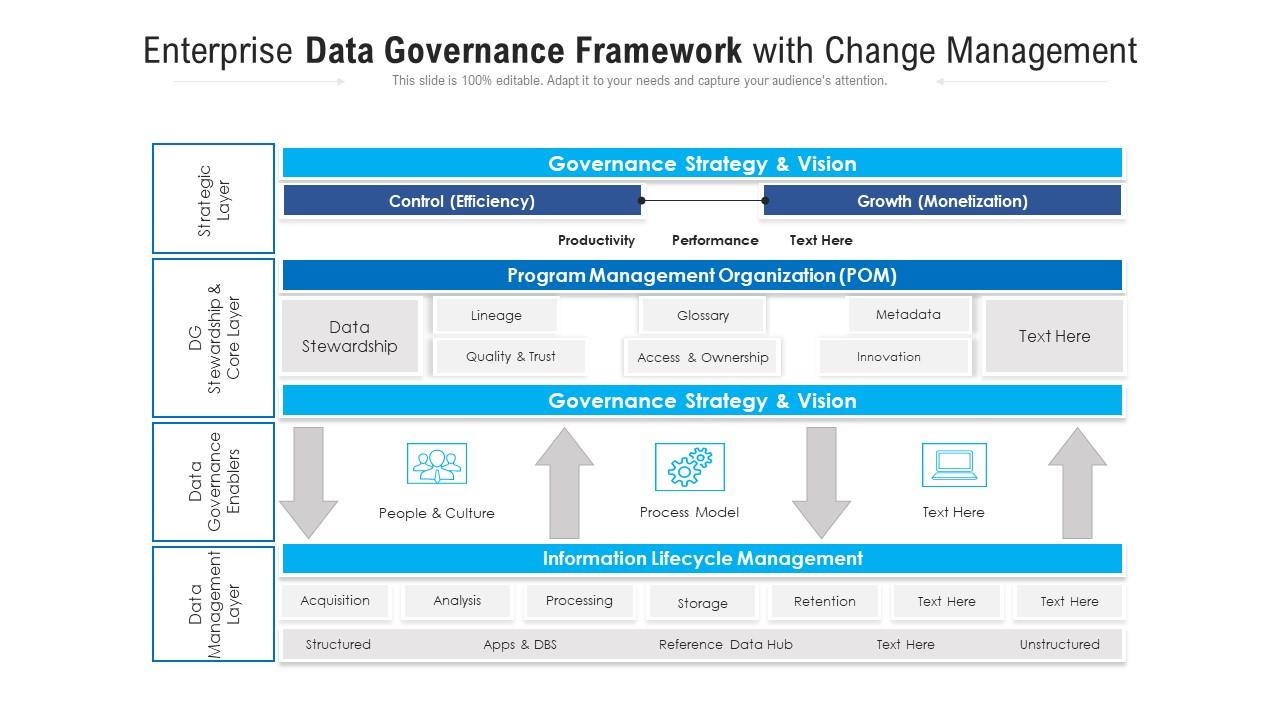
Template 5: Data Governance PPT Bundle
This comprehensive PPT Set explains the ins and outs of data governance services, offering profound insights into four key pillars: Consulting, workflow management, data organization, and privacy supervision. Use this template to articulate the essence of each service, explaining its significance within the data governance program. Embedded within this resourceful complete deck is a dedicated slide, revealing the very drivers and priorities steering the data governance program. Gain a nuanced understanding of the forces that fuel robust governance, while aligning with strategic priorities. Download now!
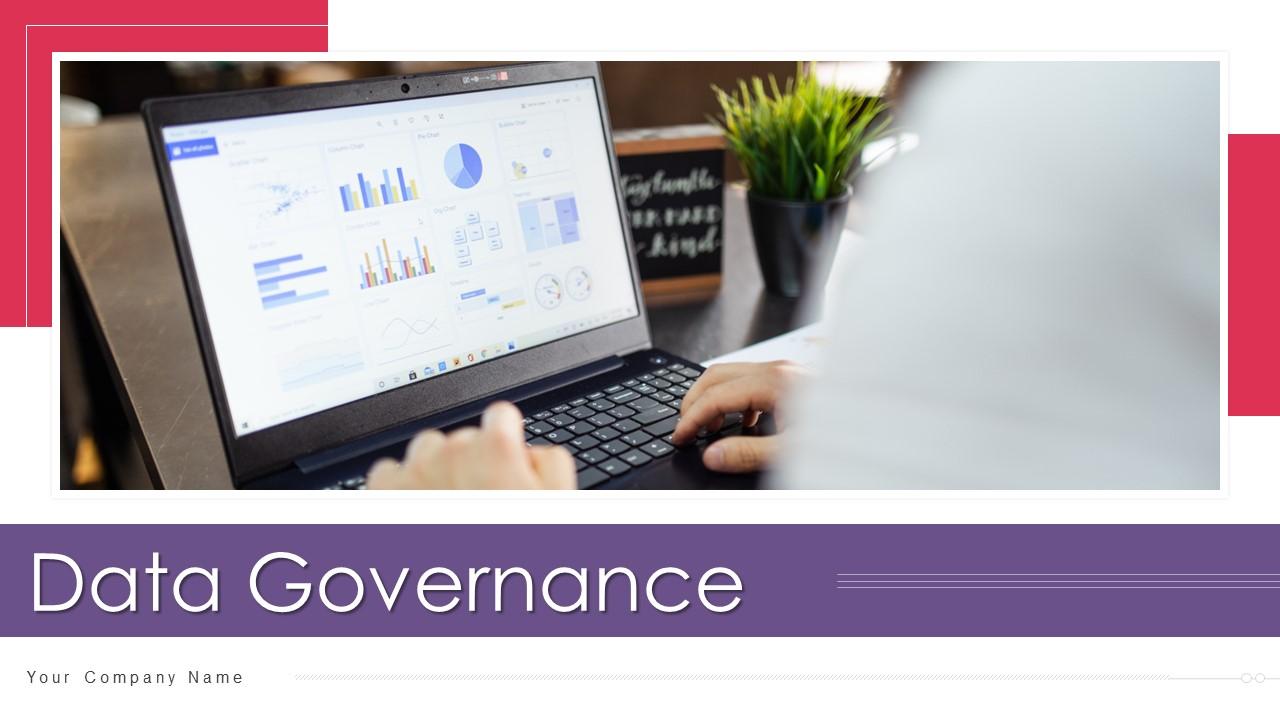
Template 6: Master Data Governance Management Framework
Get a comprehensive roadmap to effective data oversight with this PPT Layout. This structured report sheds light on the players involved — ranging from data shareholders to dedicated data governance departments and data stewardship personnel. Discover the core rationale driving your data management endeavors — an articulation of the rules of engagement through a compelling mission statement. Dive into the heart of your focus areas, gaining insights that enable you to make informed decisions and allocate resources. Master the data governance processes, understanding when and how to implement crucial steps. With our template as your guide, you'll unveil the 'Who, Why, What, and When' of data governance, empowering your organization to use its data as a strategic asset. Download now!

Template 7: Data Governance Maturity Model and Assessment
This PPT Preset guides you through the Data Governance Maturity Model, offering insights structured across five essential levels. Embark on your data journey with Level 1: Initiation, where the foundation of your governance practices takes shape.
Transition seamlessly to Level 2: Management, where the art of overseeing and directing data-related activities is under focus. Promote your practices further as you ascend to Level 3: A realm where well-structured processes and standards solidify your data governance framework.
In level 4, quantitative management takes centre-stage, where metrics and measurements steer your data processes toward optimal outcomes. Finally, reach the pinnacle of data governance in Level 5 of Optimizing. Here, continuous improvement and innovation enable you to harness data as a strategic asset.
This PPT Presentation stretches beyond the ordinary, providing not just a run-of-the-mill slide, but a holistic understanding of data governance maturity. As you navigate each level, you’ll find content that empowers you to enact meaningful changes, make informed decisions, and steer your organization toward data-driven excellence. Download now!
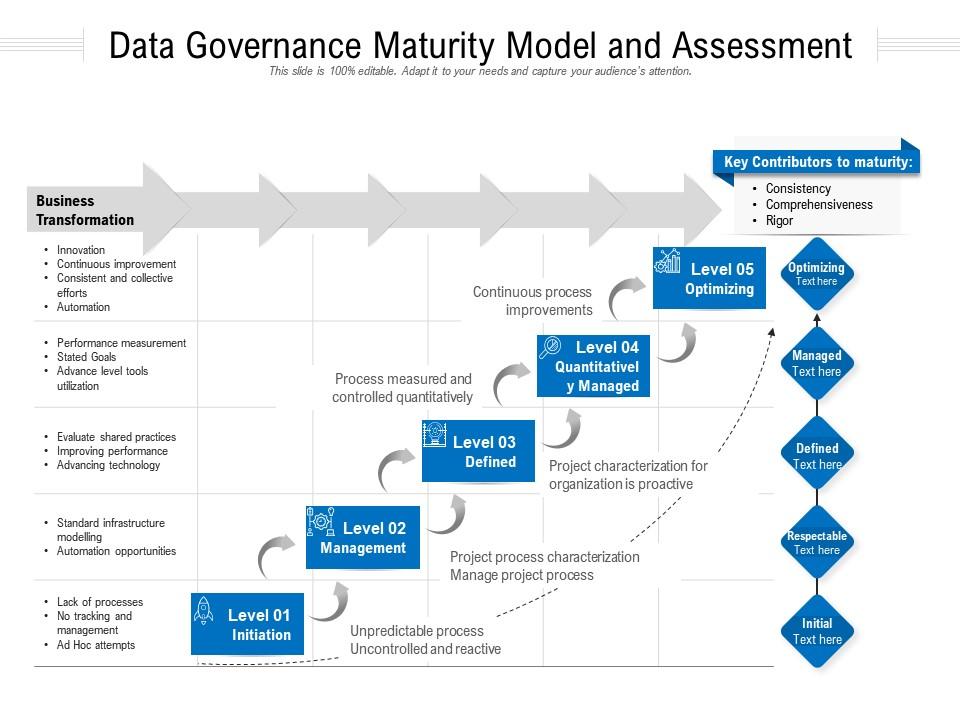
Precision in Every Byte
The digital landscape can be a maze, but fear not – our Data Governance Templates are always at hand. With them, you hold the tools to standardize, regulate, and optimize your data. These templates aren’t just tools; these are the engineers of effective decision-making, custodians of data accuracy, and architects of compliance. Equip yourself with the means to use the potential information that data holds, and shape it into an asset. Take action today, and embark on your journey toward mastering the art of data governance.
FAQs on Data Governance
What is data governance.
Data governance is the framework of processes, policies, and practices that ensure data is managed effectively and responsibly within an organization. It involves defining roles, establishing data standards, ensuring quality, security, and compliance, and managing data throughout its lifecycle. Data governance aims to enhance data accuracy, availability, and usability, while mitigating risks associated with data misuse or breaches. It provides the structure for informed decision-making, data-driven insights, and regulatory compliance.
What are the four pillars of data governance?
Data governance encompasses establishing structures and processes to ensure an organization’s effective management of data assets. The four essential pillars of data governance are:
- Data Quality: This pillar focuses on maintaining data accuracy, consistency, and reliability. Organizations must define data quality standards, implement data validation processes, and monitor and improve data quality.
- Data Security and Privacy: Protecting sensitive information is crucial. This pillar defines access controls, encryption, and authentication mechanisms to safeguard data. Compliance with data privacy regulations like GDPR or HIPAA also falls under this pillar.
- Data Lifecycle Management: Effective data governance involves managing data throughout its lifecycle – from creation to archival or deletion. This pillar includes defining data retention policies, data archival strategies, and proper data disposal procedures.
- Data Policy and Standards: Establishing clear policies and standards is essential to ensure consistent data usage across the organization. This pillar defines data ownership, classification, and usage guidelines to promote understanding and proper use.
What are the three key roles of data governance?
Three Key Roles of Data Governance:
- Data Steward: Data stewards are responsible for ensuring specific datasets’ quality, accuracy, and proper usage. These collaborate with business units, IT teams, and data owners to enforce data policies, resolve data-related issues, and drive data improvement initiatives.
- Data Custodian: Data custodians manage technical aspects of data governance. They handle tasks like data storage, access controls, and security measures. They work closely with data stewards to implement data policies and ensure data compliance.
- Data Governance Committee: Senior leaders, business representatives, and IT experts are part of this committee. It defines the strategic direction of data governance initiatives, decides on data policies, and resolves conflicts. It ensures that data governance aligns with the organization’s goals and objectives.
What is a data governance roadmap?
A data governance roadmap outlines the strategic plan for implementing and maturing data governance within an organization. It provides a structured approach to building a comprehensive data governance framework. The roadmap includes:
- Assessment and Planning: Evaluate the current state of data management, identify pain points, and define objectives. Create a data governance strategy that aligns with the organization's goals and compliance requirements.
- Framework Design: This includes defining data policies, roles and responsibilities, processes, and standards.
- Implementation: Roll out the data governance framework in phases. Start with a pilot project to establish processes, demonstrate value, and gather feedback. Expand to cover more data domains and business units.
- Education and Training: Provide training sessions to educate employees about data governance principles, roles, and responsibilities. Promote a culture of data awareness and ownership throughout the organization.
- Monitoring and Improvement: Monitor data governance activities, assess effectiveness, and make necessary adjustments. Regularly review and update policies, address emerging challenges, and ensure ongoing compliance.
- Integration with IT: Integrate data governance processes and tools with the IT infrastructure to streamline data management efforts and ensure data consistency and security.
Related posts:
- Why Does the Enterprise Security Management Concern You! 17 Best Layouts for Your Organization
- How to Design the Perfect Service Launch Presentation [Custom Launch Deck Included]
- Quarterly Business Review Presentation: All the Essential Slides You Need in Your Deck
- [Updated 2023] How to Design The Perfect Product Launch Presentation [Best Templates Included]
Liked this blog? Please recommend us

Top 10 Business Intelligence Dashboards to Enable Data-Driven Decision Making
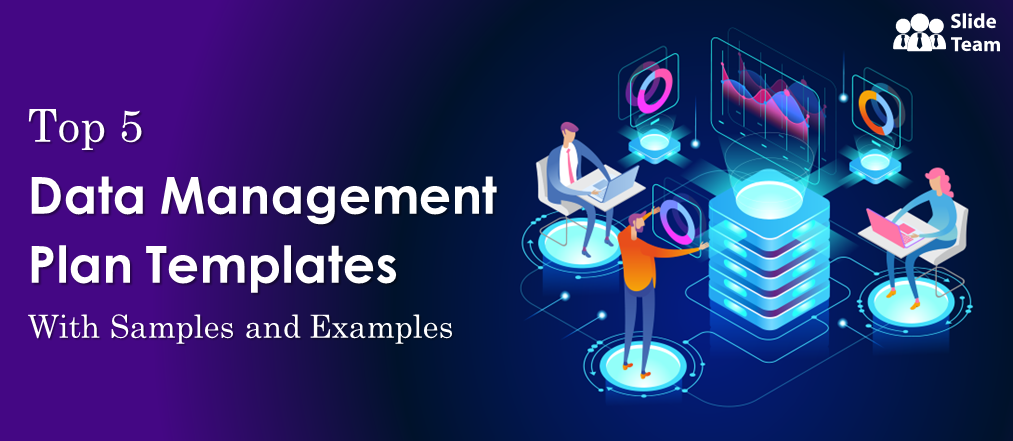
Top 5 Data Management Plan Templates With Samples and Examples
This form is protected by reCAPTCHA - the Google Privacy Policy and Terms of Service apply.
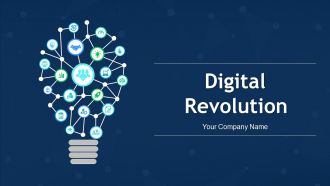
Digital revolution powerpoint presentation slides
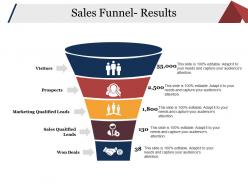
Sales funnel results presentation layouts
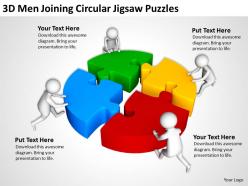
3d men joinning circular jigsaw puzzles ppt graphics icons
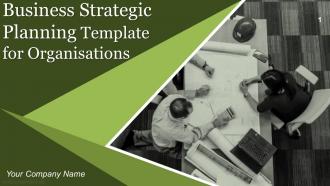
Business Strategic Planning Template For Organizations Powerpoint Presentation Slides
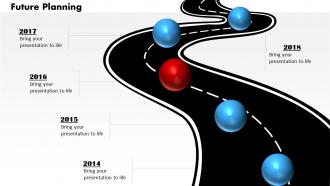
Future plan powerpoint template slide
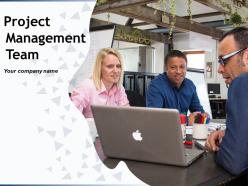
Project Management Team Powerpoint Presentation Slides
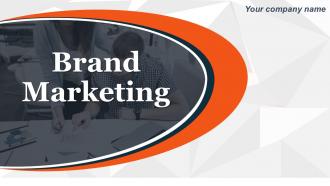
Brand marketing powerpoint presentation slides
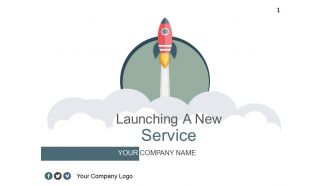
Launching a new service powerpoint presentation with slides go to market
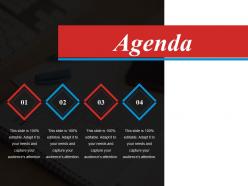
Agenda powerpoint slide show
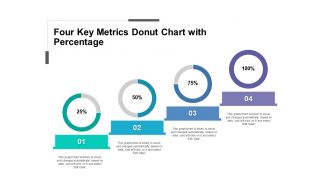
Four key metrics donut chart with percentage
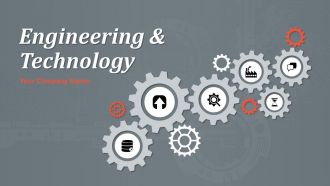
Engineering and technology ppt inspiration example introduction continuous process improvement
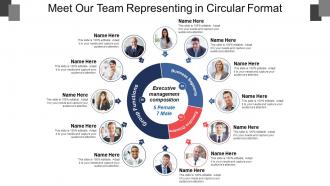
Meet our team representing in circular format

Data Topics
- Data Architecture
- Data Literacy
- Data Science
- Data Strategy
- Data Modeling
- Governance & Quality
- Uncategorized
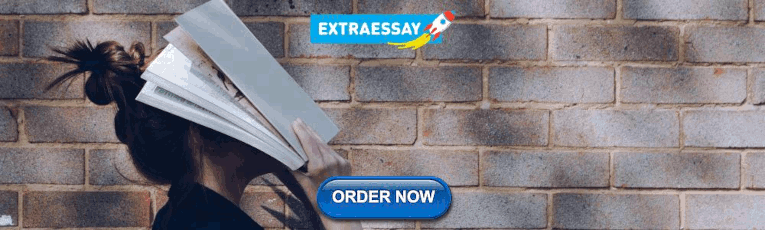
RWDG Slides: A Complete Set of Data Governance Roles & Responsibilities
RWDG Slides: A Complete Set of Data Governance Roles & Responsibilities from DATAVERSITY To view just the On Demand recording of this presentation, click HERE>> About the Webinar Roles and responsibilities are the backbone to a successful Data Governance program. The way you define and utilize the roles will be the biggest factor of program […]
To view just the On Demand recording of this presentation, click HERE>>
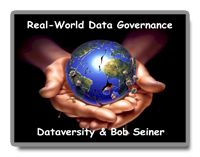
About the Webinar
Roles and responsibilities are the backbone to a successful Data Governance program. The way you define and utilize the roles will be the biggest factor of program success. From Data Stewards to the steering committee and everyone in between, people will need to understand the role they play, why they are in the role and how the role fits in with their existing job.
Join Bob Seiner for this RWDG webinar where he will provide a complete and detailed set of Data Governance roles and responsibilities. Bob will share an Operating Model of Roles and Responsibilities that can be customized to address the specific needs of your organization.
In this webinar, Bob will discuss:
- Executive, Strategic, Tactical, Operational, and Support Level Roles
- How to customize an Operating Model to fit your organization
- Detailed Responsibilities for each level
- Defining who participates at each level
- Using working teams to implement tactical solutions
About the Speaker
Robert s. seiner.
President and Principal, KIK Consulting and Educational Services
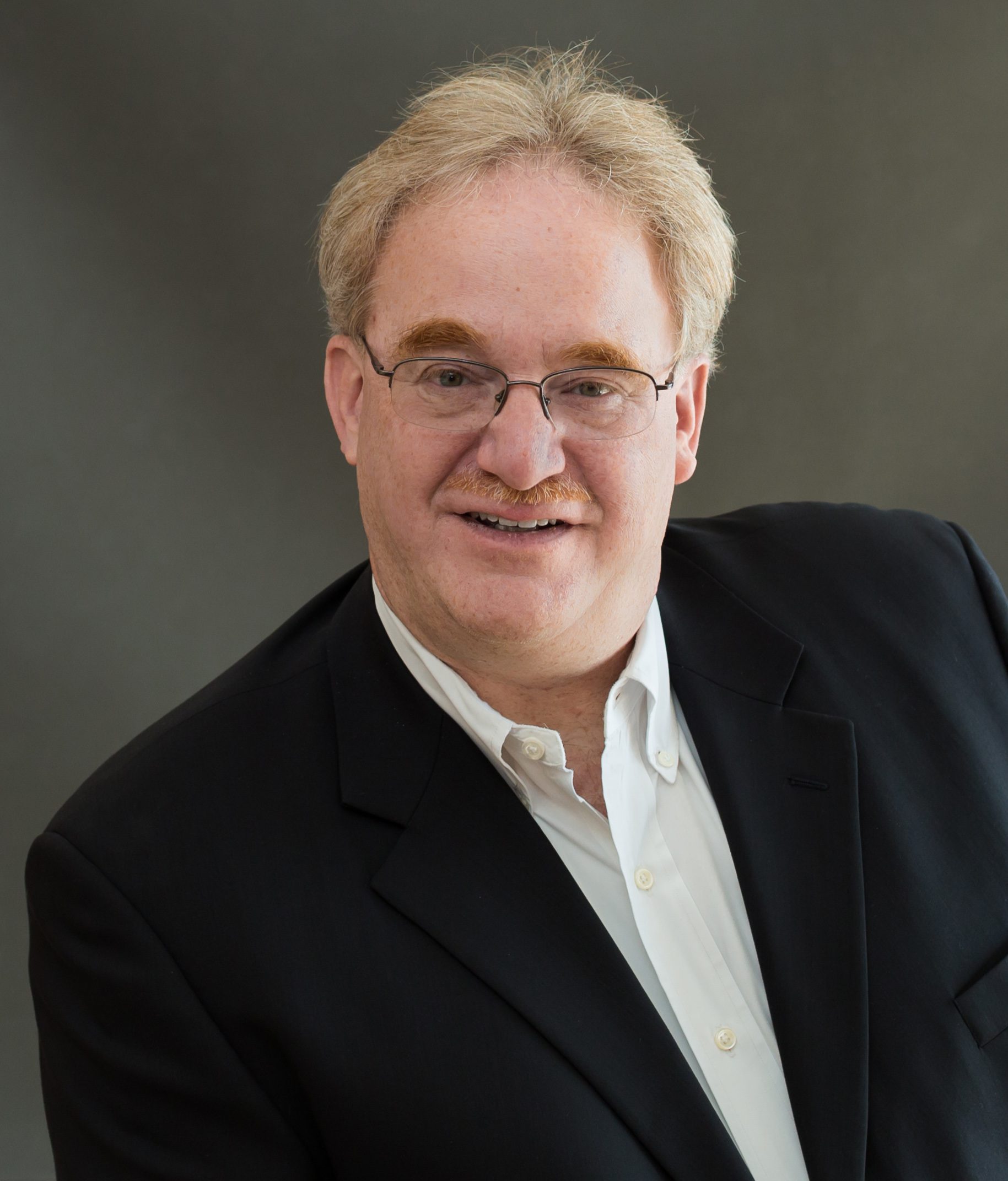
Robert S. Seiner is the President and Principal of KIK Consulting & Educational Services and the Publisher of The Data Administration Newsletter ( TDAN.com ). Bob was recently awarded the DAMA Professional Award for significant and demonstrable contributions to the data management industry. Bob specializes in “non-invasive data governance”, data stewardship, and meta-data management solutions.
This presentation is brought to you in partnership with:
and
Leave a Reply Cancel reply
You must be logged in to post a comment.
In our last blog , we delved into the seven most prevalent data challenges that can be addressed with effective data governance. Today we will share our approach to developing a data governance program to drive data transformation and fuel a data-driven culture.
Data governance is a crucial aspect of managing an organization’s data assets. The primary goal of any data governance program is to deliver against prioritized business objectives and unlock the value of your data across your organization.
Realize that a data governance program cannot exist on its own – it must solve business problems and deliver outcomes. Start by identifying business objectives, desired outcomes, key stakeholders, and the data needed to deliver these objectives. Technology and data architecture play a crucial role in enabling data governance and achieving these objectives.
- People refers to the organizational structure, roles, and responsibilities of those involved in data governance, including those who own, collect, store, manage, and use data.
- Policies provide the guidelines for using, protecting, and managing data, ensuring consistency and compliance.
- Process refers to the procedures for communication, collaboration and managing data, including data collection, storage, protection, and usage.
- Technology refers to the tools and systems used to support data governance, such as data management platforms and security solutions.
For example, if the goal is to improve customer retention, the data governance program should focus on where customer data is produced and consumed across the organization, ensuring that the organization’s customer data is accurate, complete, protected, and accessible to those who need it to make decisions that will improve customer retention.
It’s important to coordinate and standardize policies, roles, and data management processes to align them with the business objectives. This will ensure that data is being used effectively and that all stakeholders are working towards the same goal.
Starting a data governance program may seem like a daunting task, but by starting small and focusing on delivering prioritized business outcomes, data governance can become a natural extension of your day-to-day business.
Building a data governance program is an iterative and incremental process
Step 1: define your data strategy and data governance goals and objectives.
What are the business objectives and desired results for your organization? You should consider both long-term strategic goals and short-term tactical goals and remember that goals may be influenced by external factors such as regulations and compliance.
A data strategy identifies, prioritizes, and aligns business objectives across your organization and its various lines of business. Across multiple business objectives, a data strategy will identify data needs, measures and KPIs, stakeholders, and required data management processes, technology priorities and capabilities.
It is important to regularly review and update your data strategy as your business and priorities change. If you don’t have a data strategy, you should build one – it doesn’t take a long time, but you do need the right stakeholders to contribute.
Once you have a clear understanding of business objectives and data needs, set data governance goals and priorities. For example, an effective data governance program may:
- Improve data quality, which can lead to more accurate and reliable decision making
- Increase data security to protect sensitive information
- Enable compliance and reporting against industry regulations
- Improve overall trust and reliability of your data assets
- Make data more accessible and usable, which can improve efficiency and productivity.
Clearly defining your goals and objectives will guide the prioritization and development of your data governance program, ultimately driving revenue, cost savings, and customer satisfaction.
Step 2: Secure executive support and essential stakeholders
Identify key stakeholders and roles for the data governance program and who will need to be involved in its execution. This should include employees, managers, IT staff, data architects, and line of business owners, and data custodians within and outside your organization.
An executive sponsor is crucial – an individual who understands the significance and objectives of data governance, recognizes the business value that data governance enables, and who supports the investment required to achieve these outcomes.
With key sponsorship in place, assemble the team to understand the compelling narrative, define what needs to be accomplished, how to raise awareness, and how to build the funding model that will be used to support the implementation of the data governance program.
The following is an example of typical stakeholder levels that may participate in a data governance program:
By effectively engaging key stakeholders, identifying and delivering clear business value, the implementation of a data governance program can become a strategic advantage for your organization.
Step 3: Assess, build & refine your data governance program
With your business objectives understood and your data governance sponsors and stakeholders in place, it’s important to map these objectives against your existing People, Processes and Technology capabilities to achieve these objectives.
Data management frameworks such as the EDM Council’s DCAM and CDMC offer a structured way to assess your data maturity against industry benchmarks with a common language and set of data best practices.
Look at how data is currently being governed and managed within your organization. What are the strengths and weaknesses of your current approach? What is needed to deliver key business objectives?
Remember, you don’t have to (nor should you) do everything at once. Identify areas for improvement, in context of business objectives, to prioritize your efforts and focus on the most important areas to deliver results to the business in a meaningful way. An effective and efficient data governance program will support your organization’s growth and competitive advantage.
Step 4: Document your organization’s data policies
Data policies are a set of documented guidelines for how an organization’s data assets are consistently governed, managed, protected and used. Data policies are driven by your organization’s data strategy, align against business objectives and desired outcomes, and may be influenced by internal and external regulatory factors. Data policies may include topics such as data collection, storage, and usage, data quality and security:
Data policies ensure that your data is being used in a way that supports the overall goals of your organization and complies with relevant laws and regulations. This can lead to improved data quality, better decision making, and increased trust in the organization’s data assets, ultimately leading to a more successful and sustainable organization.
Step 5: Establish roles and responsibilities
Define clear roles and responsibilities of those involved in data governance, including those responsible for collecting, storing, and using data. This will help ensure that everyone understands their role and can effectively contribute to the data governance effort.
The structure of data governance can vary depending on the organization. In a large enterprise, data governance may have a dedicated team overseeing it (as in the table above), while in a small business, data governance may be part of existing roles and responsibilities. A hybrid approach may also be suitable for some organizations. It is crucial to consider company culture and to develop a data governance framework that promotes data-driven practices. The key to success is to start small, learn and adapt, while focusing on delivering and measuring business outcomes.
Having a clear understanding of the roles and responsibilities of data governance participants can ensure that they have the necessary skills and knowledge to perform their duties.
Step 6: Develop and refine data processes
Data governance processes ensure effective decision making and enable consistent data management practices by coordinating teams across (and outside of) your organization. Additionally, data governance processes can also ensure compliance with regulatory standards and protect sensitive data.
Data processes provide formal channels for direction, escalation, and resolution. Data governance processes should be lightweight to achieve your business goals without adding unnecessary burden or hindering innovation.
Processes may be automated through tools, workflow, and technology.
It is important to establish these processes early to prevent issues or confusion that may arise later in the data management implementation.
Step 7 – Implement, evaluate, and adapt your strategy
Once you have defined the components of your data governance program, it’s time to put them in action. This could include implementing new technologies or processes or making changes to existing ones.
It is important to remember that data governance programs can only be successful if they demonstrate value to the business, so you need to measure and report on the delivery of the prioritized business outcomes. Regularly monitoring and reviewing your strategy will ensure that it is meeting your goals and business objectives.
Continuously evaluate your goals and objectives and adjust as needed. This will allow your data governance program to evolve and adapt to the changing needs of the organization and the industry. An approach of continuous improvement will enable your data governance program to stay relevant and deliver maximum value to the organization.
Get started on your data governance program
In conclusion, by following an incremental structured approach and engaging key stakeholders, you can build a data governance program that aligns with the unique needs of your organization and supports the delivery of accelerated business outcomes.
Implementing a data governance program can present unique challenges such as limited resources, resistance to change and a lack of understanding of the value of data governance. These challenges can be overcome by effectively communicating the value and benefits of the program to all stakeholders, providing training and support to those responsible for implementation, and involving key decision-makers in the planning process.
By implementing a data governance program that delivers key business outcomes, you can ensure the success of your program and drive measurable business value from your organization’s data assets while effectively manage your data, improving data quality, and maintaining the integrity of data throughout its lifecycle.
So where are you in your data governance journey? Reach out to IBM Expert Labs – we’d be happy to help.
In case you missed them, check out our earlier blogs, Understanding Data Governanc e and Unlocking the power of data governance by understanding key challenges .
More from Artificial intelligence
Where to begin: 3 ibm leaders offer guidance to newly appointed chief ai officers.
4 min read - The number of chief artificial intelligence officers (CAIOs) has almost tripled in the last 5 years, according to LinkedIn. Companies across industries are realizing the need to integrate artificial intelligence (AI) into their core strategies from the top to avoid falling behind. These AI leaders are responsible for developing a blueprint for AI adoption and oversight both in companies and the federal government. Following a recent executive order by the Biden administration and a meteoric rise in AI adoption across…
Scaling generative AI with flexible model choices
5 min read - This blog series demystifies enterprise generative AI (gen AI) for business and technology leaders. It provides simple frameworks and guiding principles for your transformative artificial intelligence (AI) journey. In the previous blog, we discussed the differentiated approach by IBM to delivering enterprise-grade models. In this blog, we delve into why foundation model choices matter and how they empower businesses to scale gen AI with confidence. Why are model choices important? In the dynamic world of gen AI, one-size-fits-all approaches are…
Unlocking the power of AI across the hybrid cloud with watsonx and Fusion HCI
3 min read - AI adoption is paramount in today’s business organizations, but the path to AI adoption can be filled with curves, bumps and uncertainty. Enterprise-ready SaaS environments may not be appropriate for highly sensitive data and applications for some companies, especially those in regulated industries. Many organizations have built on-premises infrastructure and processes to accommodate their security and data sovereignty requirements. But while these systems have been engineered to support more traditional enterprise IT workloads, they are often ill-prepared for the intense…
IBM Newsletters
Designing data governance that delivers value
Executives in every industry know that data is important. Without it, there can be no digital transformation to propel the organization past competitors . There are no analytics driving new sources of revenue . Even running the basic business well isn’t possible. But for data to fuel these initiatives, it must be readily available, of high quality, and relevant. Good data governance ensures data has these attributes, which enable it to create value.
The problem is that most governance programs today are ineffective. The issue frequently starts at the top, with a C-suite that doesn’t recognize the value-creation potential in data governance. As a result, it becomes a set of policies and guidance relegated to a support function executed by IT and not widely followed—rendering the initiatives that data powers equally ineffective. In other cases, organizations try to use technology to solve the problem. While technology solutions such as data lakes and data-governance platforms can help, they aren’t a panacea.
Without quality-assuring governance, companies not only miss out on data-driven opportunities; they waste resources. Data processing and cleanup can consume more than half of an analytics team’s time, including that of highly paid data scientists, which limits scalability and frustrates employees. Indeed, the productivity of employees across the organization can suffer: respondents to our 2019 Global Data Transformation Survey reported that an average of 30 percent of their total enterprise time was spent on non-value-added tasks because of poor data quality and availability (Exhibit 1).
While it’s challenging to directly attribute value to data governance, there are multiple examples of its significant indirect value. Leading firms have eliminated millions of dollars in cost from their data ecosystems and enabled digital and analytics use cases worth millions or even billions of dollars. Data governance is one of the top three differences between firms that capture this value and firms that don’t. In addition, firms that have underinvested in governance have exposed their organizations to real regulatory risk , which can be costly.
Building the foundation for effective governance
While many organizations struggle to effectively scale data governance, some have excelled. For example, a leading global retailer, whose data governance was managed within IT, struggled to capture value from data for years. Then, as part of an enterprise-wide analytics transformation, it invested in educating and involving the entire senior-executive leadership team in data governance. It assigned to each executive leader (CFO, CMO, and so on) several data domains, or business-data subject areas, some of which, such as consumer transactions and employee data, spanned multiple functions or lines of business.
Once these leaders grasped the value of data governance, they became its champions. Within their domains, they selected representatives to act as data-domain owners and stewards and directly linked data-governance efforts to priority analytics use cases. They then worked in sprints to identify priority data based on the value they could deliver, checking in with the CEO and senior leadership team every few weeks. These efforts have begun to pay off, allowing the organization to stand up priority data domains over the course of a few months (versus years) and reduce the amount of time data scientists spend on data cleanup, accelerating analytics use-case delivery. The program continues to grow over time.
As the example demonstrates, effective data governance requires rethinking its organizational design. A typical governance structure includes three components:
- a central data management office (DMO), typically led by a chief data officer (CDO) , with a targeted data strategy and governance leaders who set the overall direction and standards
- governance roles organized by data domain where the day-to-day work is done
- a data council that brings domain leaders and the DMO together to connect the data strategy and priorities to the corporate strategy, approve funding, and address issues
This structure serves as the foundation for data governance, balancing central oversight, proper prioritization, and consistency while ensuring that the employees creating and using data are the ones leading its management (Exhibit 2).
Would you like to learn more about McKinsey Digital ?
Six ways to drive data-governance excellence.
The organizational foundation alone, however, is not enough. Six critical practices are needed to ensure data governance creates value.
1. Secure top management’s attention
As the aforementioned example highlights, success with data governance requires buy-in from business leadership. The first step is for the DMO to engage with the C-suite to understand their needs, highlight the current data challenges and limitations, and explain the role of data governance. The next step is to form a data-governance council within senior management (including, in some organizations, leaders from the C-suite itself), which will steer the governance strategy toward business needs and oversee and approve initiatives to drive improvement—for example, the appropriate design and deployment of an enterprise data lake—in concert with the DMO.
The DMO and the governance council should then work to define a set of data domains and select the business executives to lead them. These leaders drive governance efforts day-to-day by defining data elements and establishing quality standards. Companies need to invest the time to introduce these leaders to their new roles, which are typically added to their primary responsibilities. They should understand the value they will generate in these roles and be armed with the skills they need, including an understanding of the relevant regulations and core elements of the data architecture.
Critically, having top-down business-leadership buy-in will avoid the usual challenges around role clarity and empowerment. Data stewards on the business side will understand that the effort is an enterprise priority and make time to address it (which might be facilitated by a shift in their performance metrics or an adjustment in their other responsibilities). Top-down mandates also make it possible to immediately address conflicts over data ownership.
Leading organizations also create tangible ways to track progress and value creation. For example, they can measure the amount of time data scientists spend finding, curating, or enabling data for priority use cases, or the dollar losses associated with poor-quality data and associated business errors. Tracking impact metrics like these helps ensure the attention and continuing support of top management.
2. Integrate with primary transformation themes
To ensure that governance efforts create value, link them directly to continuing transformation efforts that already have CEO attention, such as digitization, omnichannel enablement, or enterprise-resource-planning modernization. These efforts typically depend on data availability and quality.
Linking governance to transformation themes simplifies senior leadership buy-in and changes the organizational construct. Rather than governance running on its own, such initiatives shift data responsibility and governance toward product teams, integrating it at the point of production and consumption.
For example, a European retailer embarked on a digital transformation of its core business and a rapid extension of its online business, which required significant redevelopment of the e-commerce stack, including back-end platforms. Data was identified as a critical enabler, and a DMO and a data council were set up to develop the core framing on the future ecosystem, as well as the structure of data domains, including the strategic goals on managing data in the future.
Lead product owners, who were heading several digital-transformation squads in dedicated functional areas, became data leaders within their area of responsibility. Product owners became data-domain owners. For example, the product owner working to drive process improvements around in-store checkout owned the sales and payment domains. This structure ensured that governance efforts were oriented primarily to enabling business needs and that the leaders creating and consuming data were actively shepherding it.
3. Prioritize data assets and focus data leadership accordingly
Many organizations approach data governance in a holistic manner, looking at all data assets at once. But such a large scope means slow relative progress in any given area and a risk that efforts aren’t linked directly to business needs. To succeed, data assets should be prioritized in two ways: by domains and by data within each domain.
The data council, supported by the DMO, should prioritize domains based on transformational efforts, regulatory requirements, and other inputs to create a road map for domain deployment. Then the organization should rapidly roll out priority domains, starting with two to three initially, and aim for each domain to be fully functional in several months.
For example, a North American retailer set a bold aspiration to transform the company over three years with advanced analytics. The company quickly realized that its current data would hold it back and established a DMO and data domains to scale governance. It identified ten domains across the enterprise and prioritized deployment of the first two—transactional data (logging in-store purchases) and product data (establishing a clear hierarchy of products and their details). This helped accelerate priority use cases around in-store assortment and inventory.
In addition to prioritizing domains, prioritize data assets within each domain by defining a level of criticality (and associated care) for each data element. Critical data typically represents no more than 10 to 20 percent of total data in most organizations. Critical elements, such as customer name or address, should receive a high level of care, including ongoing quality monitoring and clear tracking of flow across the organization, whereas for elements that are used less often in analytics, reporting, or business operations (such as a customer’s academic degree), ad hoc quality monitoring without tracking may suffice. This significantly narrows the scope of governance efforts and ensures that they are focused on the most important data.
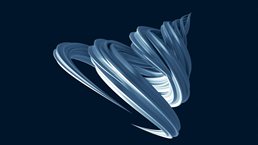
How chief data officers can navigate the COVID-19 response and beyond
4. apply the right level of governance.
Data-governance programs can vary dramatically across organizations and industries. Leading organizations take a “needs-based” approach, adopting the level of governance sophistication appropriate to their organization and then adjusting the level of rigor by data set.
It’s important to realize that data governance was largely first championed by banks under pressure from BCBS 239 1 Basel Committee on Banking Supervision’s standard number 239: “Principles for effective risk data aggregation and risk reporting.” and other regulations that required sophisticated governance models. Most other industries and organizations don’t face the same level of regulatory pressure, so the design of their programs should align with the level of regulation they uniquely face and the level of their data complexity. Organizations with multiple, distinct businesses spanning many geographies have more complex needs than those with a business in only one geography; similarly, a high pace of data change or low level of technology automation increases data complexity (Exhibit 3).
The most comprehensive governance model— say, for a global bank—will have a robust data-governance council (often with C-suite leaders involved) to drive it; a high degree of automation with metadata recorded in an enterprise dictionary or data catalog; data lineage tracked back to the source for many data elements; and a broader domain scope with ongoing prioritization as enterprise needs shift.
In contrast, targeted data governance for a regional technology company might have a data council that meets less frequently and includes C-suite leaders only periodically; metadata tracking that could even start in Excel; limited lineage tracking; and narrower domain scope, at least initially, to enable priority use cases.
In parallel with establishing the right level of governance for the organization as a whole, adjust the level of governance rigor across data sets. Many organizations’ legacy data standards set conservative restrictions on quality and access across the board. This minimizes risk but can stifle innovation. Leading organizations consciously balance opportunities and risks and differentiate governance by data set.
For example, organizations can apply light governance for data that is used only in an exploration setting and not beyond the boundaries of the science team. The team may also not need perfectly prepared and integrated data with full metadata available. Data masking may be appropriate to ensure privacy, together with strict internal non-disclosure agreements (NDAs). However, as soon as such data is used in a broader setting, such as in interactions with customers, stronger governance principles need to be applied.
An Asian financial institution took an aggressive approach to “free the data” using these principles. It agreed on the sensitivity level for each data set and was able to free the roughly 60 percent of enterprise data that was low risk, giving all employees access to use and explore it. On the other hand, highly sensitive data, such as personally identifiable information, was highly restricted both in terms of who could access it and how.
As organizations mature and their governance capabilities and technology continue to advance, scope becomes less important. A suite of tools is beginning to automate data-governance activities, and its coverage and cost-effectiveness will only improve over time. Both newer platforms, such as Octopai and erwin, and established organizations, such as Informatica and Collibra, are rolling out capabilities for automated metadata harvesting, lineage creation, data-quality management, and other governance functions.
5. Choose iterative and focused implementation
To ensure that data governance creates value fast, tailor governance priorities to the domain, and use iteration to adapt quickly. This goes beyond integrating governance with business needs, prioritizing use cases and domains, and applying needs-based governance; the key is to adopt iterative principles in day-to-day governance. For example, if there is a backlog of known data-quality issues, review and reprioritize daily, working to maximize the benefit to the business as priorities shift.
Push to enable priority use cases quickly even if the solution isn’t perfect. Longer-term development to make use cases production ready (by integrating with the core customer-relationship-management and operational customer master data) can occur once value has been demonstrated. For example, enhancing customer campaigns may not require a fully integrated set of data across the entire enterprise, but rather a tailored approach in a dedicated platform. Data governance should support and accelerate this tailored approach, focusing on solving issues around availability and quality in addition to establishing strong master-data management.
6. Generate excitement for data
When people are excited and committed to the vision of data enablement , they’re more likely to help ensure that data is high quality and safe. Leading organizations invest in change management to build data supporters and convert the skeptics. This can be the most difficult part of the program, as it requires motivating employees to use data and encouraging producers to share it (and ideally improve its quality at the source).
Successful organizations use a combination of interventions to drive the right behavior. These can include role modeling from the CEO and other senior leaders, recognition for high quality, responsive sources, and new demonstrated-use cases. Some organizations also offer training and qualifications, often as part of a larger academy approach , together with communicating about career opportunities in data jobs. Others have used successes in data and analytics to create excitement in the form of events, publications, or even data art. 2 TED compiled a series of talks on data art: ted.com/playlists/201/art_from_data. What works is highly dependent on the culture of the organization.
Getting started
Companies should begin their new data-governance approach by asking these six questions:
- What is the opportunity cost of not getting data governance right in terms of missed upside, extensive time lost in manually cleaning data, or incorrect and suboptimal business decisions?
- Who is leading governance efforts today, and what would it look like to elevate the conversation to the C-suite? Who should be involved?
- Where is governance most important? What domains and parts of domains does the organization most need right now?
- What governance archetype best fits the organization, and are current efforts aligned to that level of need?
- How can governance be accelerated by adjusting its focus and injecting iterative working concepts?
- Do you have the in-house capabilities to manage such a shift?
Data governance is critical to capturing value through analytics, digital, and other transformative opportunities. While many companies struggle to get it right, every company can succeed by shifting its mindset from thinking of data governance as frameworks and policies to embedding it strategically into the way the organization works every day.
Bryan Petzold is an associate partner in McKinsey’s Silicon Valley office, Matthias Roggendorf is a partner in the Berlin office, Kayvaun Rowshankish is a partner in the New York office, and Christoph Sporleder is a partner in the Frankfurt office.
Explore a career with us
Related articles.
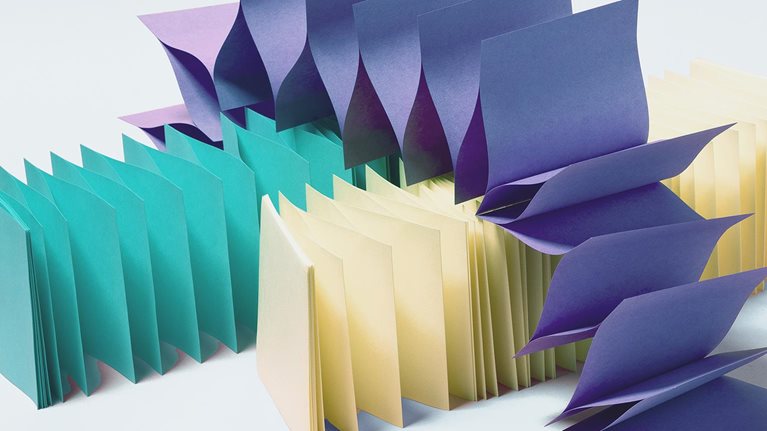
How to build a data architecture to drive innovation—today and tomorrow
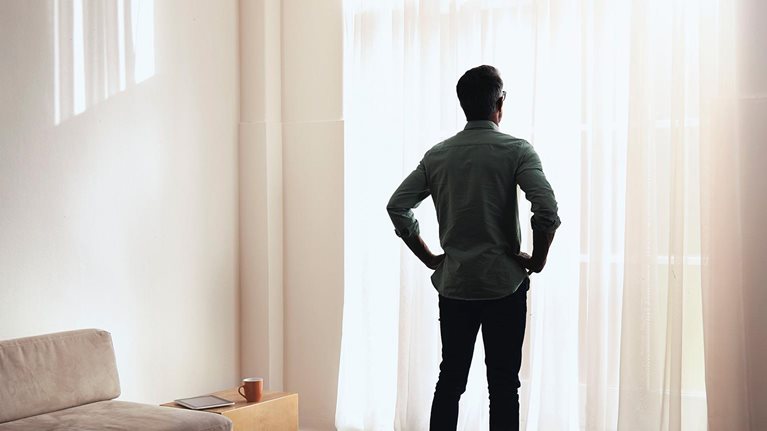
The digital-led recovery from COVID-19: Five questions for CEOs
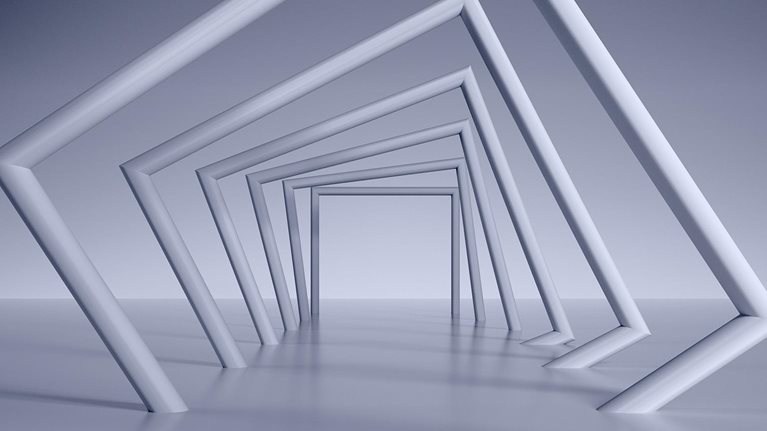
How to make the most of AI? Open up and share data
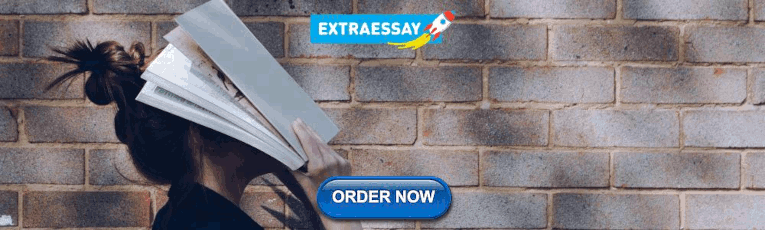
COMMENTS
Template 5: Data Governance PPT Bundle . This comprehensive PPT Set explains the ins and outs of data governance services, offering profound insights into four key pillars: Consulting, workflow management, data organization, and privacy supervision. Use this template to articulate the essence of each service, explaining its significance within ...
Christiana Nicole November 21, 2022. Real-World Data Governance: Data Governance Best Practices, Assessments, and Roadmaps from DATAVERSITY To view just the slides from this presentation, click HERE>> This webinar is sponsored by About the Webinar When starting or evaluating the present state of your Data Governance program, it is important to ...
TO DATA GOVERNANCE THE CASE FOR DATA GOVERNANCE Data is the lifeblood of an increasingly digital economy. Emerging technologies have helped streamline and optimize processes with new and innovative uses of data, but they also expose businesses to significant risk across a continually shifting landscape of threats and vulnerabilities.
scale data governance, some have excelled. For example, a leading global retailer, whose data governance was managed within IT, struggled to capture value from data for years. Then, as part of an enterprise-wide analytics transformation, it invested in educating and involving the entire senior-executive leadership team in data governance.
Justifications for Data Governance - Cost (3) The average financial impact of poor data quality on a typical businesses is $9.7 million per year. Opportunity costs, loss of reputation and low confidence in data may push these costs higher. Forbes (2017).
This presentation reports on data governance best practices. Based on a definition of fundamental terms and the business rationale for data governance, a set of case studies from leading companies is presented. The content of this presentation is a result of the Competence Center Corporate Data Quality (CC CDQ) at the University of St. Gallen ...
Bob was recently awarded the DAMA Professional Award for significant and demonstrable contributions to the data management industry. Bob specializes in "non-invasive data governance", data stewardship, and meta-data management solutions. This presentation is brought to you in partnership with:
This presentation reports on data governance best practices. Based on a definition of fundamental terms and the business rationale for data governance, a set of case studies from leading companies is presented. The content of this presentation is a result of the Competence Center Corporate Data Quality (CC CDQ) at the University of St. Gallen ...
Data governance promotes data democratization by ensuring data accuracy, consistency, and trustworthiness. It helps data users find high-quality data quickly, promoting a better understanding of the data's meaning and context, leading to increased productivity and faster decision-making. Enhanced collaboration and value realization.
It is critical that all agencies make progress on data governance and maturity. This playbook describes these activities in a recommended order: 1. Play 1 - Data Governance a. Step 1: Establishing a data governance body b. Step 2: Setting the vision 2. Play 2 - Data and Related Infrastructure Maturity a. Step 1: Conducting a data maturity ...
Step 5: Establish roles and responsibilities. Define clear roles and responsibilities of those involved in data governance, including those responsible for collecting, storing, and using data. This will help ensure that everyone understands their role and can effectively contribute to the data governance effort.
The most comprehensive governance model— say, for a global bank—will have a robust data-governance council (often with C-suite leaders involved) to drive it; a high degree of automation with metadata recorded in an enterprise dictionary or data catalog; data lineage tracked back to the source for many data elements; and a broader domain scope with ongoing prioritization as enterprise needs ...
Data governance (DG) is the overall management of the availability, usability, integrity and security of data used in an enterprise. A sound data governance program includes a governing body or council, a defined set of procedures and a plan to execute those procedures.
This presentation was part of the IDS Webinar on Data Governance. It gives a brief overview of the history on Data Governance, describes how governing data has to be further developed in the era of business and data ecosystems, and outlines the contribution of the International Data Spaces Association on the topic.
No. 3: Implement trust-based governance. Data and analytics assets exist everywhere across an enterprise and vary in nature, so making business decisions based on the assumption that "all information is equal" is no longer a good approach. Instead, establish a trust-based governance model that: Supports a distributed D&A ecosystem.
Data governance is organizing, securing, managing, and presenting data using methods and technologies that ensure it remains correct, consistent, and accessible to verified users. Let's break that down. Data governance is: Organizing — identifying all your data sources and getting all your data in one place.
Data engineers and data governance officers will find these slides helpful in illustrating the components, foundational pillars, core elements, and functions of this framework. Deliver an engaging presentation with the help of our PPT to depict the stepwise process of creating a data governance framework.
This presentation reports on data governance best practices. Based on a definition of fundamental terms and the business rationale for data governance, a set of case studies from leading companies is presented. The content of this presentation is a result of the Competence Center Corporate Data Quality (CC CDQ) at the University of St. Gallen ...
F÷&¾ aï0Z Òi 6&Mܨõ nàÎ" ¸#PuÞ^¬Ú´ÉÌÄ…³„ ' 0 XÃÞÐ M®à£,ç $¥]Uð—ÕýÙ5g!‚S`ÈaÁ |>;=™>¡ ˜6…µ® K). | c}+D k´ 2ªÑ%R'· ÓÐW¢ ¹ Å8Ï' fðÙQ&[ª‚û¥:çlÕÔø—l*K-qArkÑÅ-#„ÒPy° ÐÐ6¦`ð Æ‚gÙ/ ÂìØM-²¹hW ´€ íB*'^ Ñ ê £ ¶ôs0 ´hÜg6é0³Zz TÆL'ý'ú ...