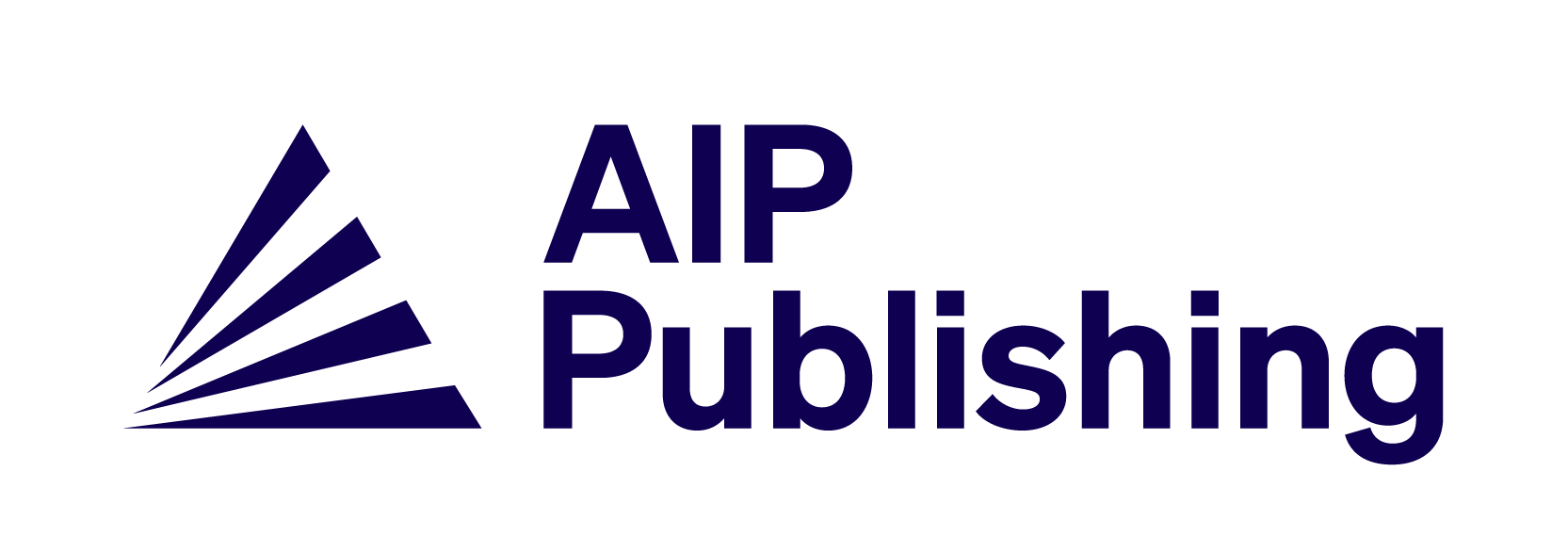
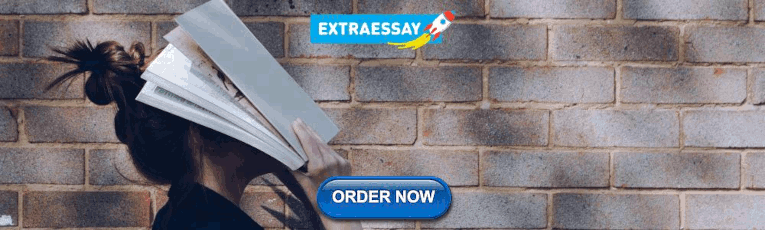
Factors affecting share prices: A literature revisit
- Article contents
- Figures & tables
- Supplementary Data
- Peer Review
- Reprints and Permissions
- Cite Icon Cite
- Search Site
Mohammad Almashaqbeh , Md Aminul Islam , Rosni Bakar; Factors affecting share prices: A literature revisit. AIP Conf. Proc. 3 May 2021; 2339 (1): 020143. https://doi.org/10.1063/5.0045110
Download citation file:
- Ris (Zotero)
- Reference Manager
The aim of the paper is to present key factors they are determinants of share prices in the stock markets globally. To achieve the aim of this study many of previous studies have been reviewed. This paper revisits previous studies by various researches on factors affecting the share price in both developed and developing economies. These researchers looked at the relationships between stock prices and selected internal and external factors affecting a firm’s share price. “Some of the factors affecting share prices can be generalized to both developed and developing economies. Some studies have concluded that company fundamentals such as institutional factors and the nature of the firm or business are major factors that affect stock prices”. “However, other studies indicated that firm’s boundaries, organization, governance, ownership pattern, auditing, and value relevance of accounting information is the most influencing factors of stock prices”. “In addition, the effect of inter related factors has been covered in some other studies”. As a revisit, this work derived from secondary sources. This revisit provides researchers and practitioners with the latest improvements of approaches by minimizing their effort in collecting the relevant material and a better understanding of each factor the affecting share prices, draw attention to specific areas for further research. Based on this result the study of ownership structures, audit quality and value relevance of accounting information and relations between them very important and they key factors to determinants of share prices.
Sign in via your Institution
Citing articles via, publish with us - request a quote.
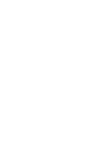
Sign up for alerts
- Online ISSN 1551-7616
- Print ISSN 0094-243X
- For Researchers
- For Librarians
- For Advertisers
- Our Publishing Partners
- Physics Today
- Conference Proceedings
- Special Topics
pubs.aip.org
- Privacy Policy
- Terms of Use
Connect with AIP Publishing
This feature is available to subscribers only.
Sign In or Create an Account
Click through the PLOS taxonomy to find articles in your field.
For more information about PLOS Subject Areas, click here .
Loading metrics
Open Access
Peer-reviewed
Research Article
The sustainability of stock price fluctuations: Explanation from a recursive dynamic model
Contributed equally to this work with: Jun Xie, Wenqian Xia, Bin Gao
Roles Funding acquisition, Methodology, Project administration, Supervision, Visualization, Writing – original draft, Writing – review & editing
Affiliation School of Economics, Guangxi University, Nanning, China
Roles Methodology, Validation, Writing – review & editing
Roles Formal analysis, Funding acquisition, Methodology, Validation, Writing – original draft, Writing – review & editing
* E-mail: [email protected]
Affiliation School of Economics, Guangxi University for Nationalities, Nanning, China

- Jun Xie,
- Wenqian Xia,
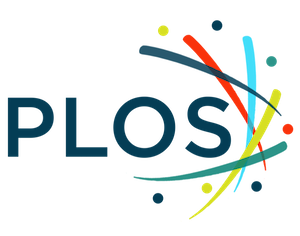
- Published: August 17, 2021
- https://doi.org/10.1371/journal.pone.0255081
- Reader Comments
The sustainability of stock price fluctuations indicated by many empirical studies hardly reconciles with the existing models in standard financial theories. This paper proposes a recursive dynamic asset pricing model based on the comprehensive impact of the sentiment investor, the information trader and the noise trader. The dynamic process of the asset price is characterized and a numerical simulation of the model is provided. The model captures the features of the actual stock price that are consistent with the empirical evidence on the sustainability of stock price fluctuations. It also offers a partial explanation for other financial anomalies, for example, asset price’s overreaction, asset bubble and the financial crisis. The major finding is that investor sentiment is the key factor to understand the sustainability of stock price fluctuations.
Citation: Xie J, Xia W, Gao B (2021) The sustainability of stock price fluctuations: Explanation from a recursive dynamic model. PLoS ONE 16(8): e0255081. https://doi.org/10.1371/journal.pone.0255081
Editor: Haijun Yang, BeiHang University School of Economics and Management, CHINA
Received: August 20, 2020; Accepted: July 11, 2021; Published: August 17, 2021
Copyright: © 2021 Xie et al. This is an open access article distributed under the terms of the Creative Commons Attribution License , which permits unrestricted use, distribution, and reproduction in any medium, provided the original author and source are credited.
Data Availability: All relevant data are within the paper and its Supporting Information files. In particular, we explain that the data used in the numerical simulation of this paper are all in the uploaded ZIP MATLAB program.
Funding: This study was supported by the National Natural Science Foundation of China to JX (No.72061002), Social Sciences and Humanities Youth Foundation of Chinese Ministry of Education to BG (18XJC790003), the Natural Science Foundation of Guangxi Province of China to BG (AD20159052), the Foundation for University Key Teacher by Guangxi Province of China to BG (2018), the Xiangsi Lake Youth Innovation Team Funds for Guangxi University for Nationalities to BG (2018RSCXSHQN05), the ‘Guangxi One Thousand Young and Middle-Aged College and University Backbone Teachers Cultivation Program’ Humanities and social sciences projects to JX (2021QGRW003) and the Foundation of Guangxi Development Strategy Institute, Key Research Base of Humanities and Social Sciences in Guangxi Universities to JX. The funders had no role in study design, data collection and analysis, decision to publish, or preparation of the manuscript.
Competing interests: The authors have declared that no competing interests exist.
1. Introduction
Stock price fluctuations are always higher than the expectation of the standard financial theory. In standard financial theories, such as CAPM [ 1 ], stock price changes only when new information appears, and volatility should be quite low. However, Schwert [ 2 ] uses monthly returns from 1802 to 2010, daily returns from 1885 to 2010, and intraday returns from 1982 to 2010 in the USA to show how stock price fluctuations have changed over time, and illustrate the historically high levels of stock price fluctuations in the USA. In fact, China has a similar situation. The Chinese stock market is a newly developing capital market where a dramatic fall in price always follows a soar and the price fluctuates repeatedly. For example, from June 6, 2005 to October 16, 2007, within two and a half years, Shanghai Composite Index (SCI) increased more than 6 times, rising from 998.23 points to 6124.04 points; from October 16, 2007 and August 11, 2008, within one year, SCI decreased more than half of the top points, dropping from 6124.04 points to 2470.07 (see Fig 1 ). The mean and standard deviation of SCI were 2720 and 868 respectively from 2005-01-01 to 2019-11-14. All these phenomena deviated from the Chinese stock market are unexplainable through standard financial theories.
- PPT PowerPoint slide
- PNG larger image
- TIFF original image
https://doi.org/10.1371/journal.pone.0255081.g001
The literature for possible clues of the sustainability of stock price fluctuations may go back to several seminal works. Abundant empirical evidences imply that stock price fluctuations are explained with the subsequent variation in the stock quality [ 3 ], economic fundamentals [ 4 – 6 ], overconfidence [ 7 – 9 ], investor attention [ 10 ], subjective beliefs [ 11 ], microeconomic foundation [ 12 ], industrial policy [ 13 ], corporate social responsibility [ 14 ], monetary policy [ 15 ] and sentiment [ 16 ]. The factors that affect the fluctuations of stock price may seem so numerous, but they can be roughly divided into two categories: (1) Fundamental factors (rational factors), which include the stock quality, economic fundamentals, industrial policy, monetary policy and so on. (2) Sentiment factors, which include overconfidence, investor attention, subjective beliefs, and so on, since overconfidence, investor attention and other subjective beliefs are always influenced by investor sentiment. In fact, lots of empirical literature [ 17 – 22 ] show that sentiment has a significant influence on the fluctuations of stock prices. In other words, the continuous fluctuation of a stock price is the result of the joint action of heterogeneous investors: rational investor and sentiment investor. The theoretical asset pricing model based on the heterogeneous is urgently needed to explain this phenomenon.
Asset pricing model based on the heterogeneous is a hot spot in the recent thirty years. DeLong, et al. [ 23 ] confirm that arbitrageurs buy in anticipation of positive-feedback trading by the Noise trader, and thus destabilize prices. Wang [ 24 ] presents a dynamic trading model with differentially informed investors and shows that less-informed investors can rationally behave like price chasers. Brock and Hommes [ 25 ] investigate the dynamics in a simple present discounted value asset pricing model with heterogeneous beliefs and show that price fluctuations are driven by the evolutionary dynamics between different expectation schemes (‘rational animal spirits’). Barlevy and Veronesi [ 26 ] consider a model with risk-neutral Outsiders trading with Noise trader and Insiders, optimally extracting information from the price of an asset. Mendel and Shleifer [ 27 ] present a model in which rational but uninformed traders occasionally chase noise as if it were information. In their model, noise trader can have an impact on market equilibrium disproportionate to their size in the market. Agliari, et al. [ 28 ] propose a stock market model in which participation depends upon an attractiveness measure related to the market activity and the fundamental value of the market. Oshima [ 29 ] builds a two-agent New Keynesian model with subjective and objective beliefs about capital gains from stock prices and shows that the presence of the two agents improves second moments of stock prices with realistic moments of business cycle properties. Schmitt, et al. [ 30 ] proposes a simple agent-based computational model in which speculators’ trading behavior may cause bubbles and crashes, excess volatility, serially uncorrelated returns, fat-tailed return distributions and volatility clustering. Few of them discuss the investor sentiment in their theoretical model based on the heterogeneous. In particular, there is no model based on the heterogeneous to describe the specific process of price changes under the influence of the sentiment investor. Li [ 31 ] examines the changes in price with the heterogeneous sentiment. But aforementioned empirical papers show that stock price fluctuations by the combined action of the rational investor (the information trader) and the sentiment investor, instead of heterogeneous sentiment investors. These phenomena indicate a gap between current theoretical models and empirical evidence.
To fill the gap between the asset pricing theory model and empirical model in the paper, a recursive dynamic asset pricing model with heterogeneous traders (the information trader, the sentiment trader and the noise trader) is proposed to explain in theory why the sustainability of stock price fluctuations appears in the real financial market. A similar model with heterogeneous agents is proposed by Hong and Stein [ 32 ]. This paper extends the analysis of the seminal work of Hong and Stein [ 32 ] in the following two aspects: (1) The effect of investor sentiment is included in our discussion whereas Hong and Stein [ 32 ] did not mention it. The paper analyzes investor sentiment instead of discussing the moment trader, since the seminal works [ 11 , 17 – 22 ] confirm that investor sentiment is a key factor in asset pricing. For example, Adam et al. [ 11 ] suggest that asset price dynamics are to a large extent influenced by investors’ subjective optimism and pessimism. (2) This paper uses a discrete recursive method to build the model, yet Hong and Stein [ 32 ] use the continuous method. This makes the stock price simulated by the model in this paper more intuitive to show the sustainability of stock price fluctuations, and it is found that the sustainability of stock price fluctuations is closely related to sentiment investor which is consistent with the results of empirical literature [ 17 – 22 ].
Our paper contributes three fresh insights. Firstly, the paper derives a recursive dynamic asset pricing model with heterogeneous traders, which include the information trader, the sentiment trader and the noise trader. Secondly, the paper finds that investor sentiment is the key factor that leads the stock price to fluctuate powerfully and causes the sustainability of stock price fluctuations anomaly. Our third result explores the source of financial anomalies. From our model, we show that overreaction, asset bubble and the financial crisis are attributable to investor sentiment.
The rest of this paper is organized as follows. Section 2 presents the assumptions of the model; Section 3 describes the dynamic recursive asset pricing model with heterogeneous traders; Section 4 analyzes the model thoroughly and displays the dynamic process of stock price; Section 5 presents a numerical example and Section 6 is the conclusion.
2. The model assumptions
In the standard finance theories, all investors are rational and have homogeneous cognition. However, in the real financial market, the situation is different. As aforementioned empirical papers show that stock price fluctuations by the combined action of the rational investor (the information trader) and the sentiment investor, we suppose that there are three investors in the market: the information trader, the sentiment trader and the noise trader. The information trader trades based on the accounting information, which means the information trader is rational. The sentiment trader buys the asset when the sentiment runs high and sells the asset when sentiment falls. We simply assume that investor sentiment rises when asset prices rise and vice versa, and when the information trader (or the sentiment trader) enters or exits the market, the entering or exiting behavior equally affects the stock price in opposite directions. For simplicity, we only analyze the impact of positive information.
(i) The information trader
The information trader optimizes his or her investment by collecting the information himself, known as the private information. This type of trader does not care about the effect of the price change or the other investors’ behavior. In other words, the information trader is rational. Let P t denote the stock price at the time t , and the stock price change is Δ P t , where Δ P t = P t − P t −1 . The information trader does investment after considering how private information affects the price P t , instead of the price change Δ P t . When new information shocks the stock, the information trader enters the market with high expectations and following the “buy and hold” principle, which implies that the information trader closes out the stock till survival. As the accounting information is uncovered quarterly, we assume that the information trader is interested in long-term investment and the survival of the information trader is much longer than the survival of the sentiment trader.
(ii) The sentiment trader
The sentiment trader optimizes his or her investment by the sentiment which is affected by the price change. This type of trader also follows the “buy and hold” principle and the survival period is k . As the price changes more frequently than the uncovered accounting information, we assume that the survival period k of the sentiment trader is much shorter than the survival period of the information trader, and also much shorter than the whole period T.
Furthermore, the sentiment trader is divided into the optimistic trader and the pessimistic trader. When the stock price is on the rise, the investor who feels optimistic and enters the market is the optimistic trader. When the stock price increases Δ P t −1 in t −1 time, the optimistic trader enters the market to buy the stock, which leads the stock price to increase W o Δ P t −1 at the time t . Where W o >0, W o is the elasticity coefficient that implies the level of price change caused by the optimistic trader. As the survival period of the sentiment trader is k , the optimistic trader who enters the market at the time t −1 should exit the market at the time t + k −1, which leads the stock price to decline W o Δ P t −1 . On the other hand, when the stock price is falling, the investor who feels pessimistic and enters into the market to short selling stock is the pessimistic trader. When the stock price decreases Δ P t −1 in t −1 time, the pessimistic trader enters the market to sell the stock, leading the stock price to decrease W p Δ P t −1 . Where W p >0, W p is the elasticity coefficient: the level of price change caused by the pessimistic trader. As the survival period of the sentiment trader is k , the pessimistic trader who enters the market in t −1 time exit the market in t + k −1 time, leading the stock price to increase W p Δ P t −1 . Furthermore, we assume W o + W p <1, which means that the effect of the sentiment trader on the stock price is less than the price change.
(iii) The noise trader
The noise trader is the investor who is the counterparty against the information trader or the sentiment trader and only provides stock quantity Q every time. This assumption is similar to the assumption in the HS model [ 33 ]. Considering the real financial market, the behavior of the noise trader only leads the stock price to fluctuate slightly and does not attract the sentiment trader to enter the market.
Finally, from the evidence provided by Hong and Stein [ 33 ], this paper assumes that the information spreads gradually among all information traders. The information spread process is uniformed and needs λ periods. The effect of information on the stock’s basic value is noted as ε t . According to the assumption, when the information ε t appears at the time t , only ε t /λ parts of the information are observed at the time t , which leads the stock price to change ε t /λ . At the time t +1, other ε t /λ parts of the information are observed, which leads that the stock price changes ε t /λ as well. Until after λ periods, at the time t + λ , the information ε t is observed completely. At the time t + λ , the effect of the information on stock price is accomplished. The overall effect of information on the stock price is ε t .
3. The asset pricing model
4. The dynamic process of price change
(I) Between periods [ t +2, t + λ ]
(II) Between periods [ t + λ +1, t + k +1]
(III) Between periods [ t + k +2, t +2 k +1]
For any t ′∈[ t +1, t + k ], from Eq ( 6 ), Δ P t ′ >0 is satisfied. The survival of the optimistic trader is k , Δ P x <0 for any x ∈[ t + k +2, t +2 k +1], which implies that the stock price should decline between periods [ t + k +2, t + k + λ ]. Furthermore, for any x ∈[ t + k +2, t + k + λ ], from Eq ( 14 ), we know that Δ P x −Δ P x −1 <0, which implies that the stock price accelerates to fall. On the other hand, from Eq ( 14 ), we know that Δ P t +2 k +1 ≈0, which implies that the change in the stock price is slight at the time t +2 k +1 and does not attract the pessimistic trader to enter the market.
(IV) Between periods [ t +2 k +3, t +3 k +1]
The above formula implies that the stock price changes slightly at the time t +3 k +1, which does not attract the sentiment investor to enter the market. The survival period of the sentiment investor is k , so the optimistic trader who enters the market at the time t +2 k +3 should exit the market by selling the stock at the time t +3 k +3. This behavior makes the stock price decline at the time t +3 k +4. Thus, the pessimistic trader enters the market by short-selling and leads the stock price to decline further. This is the declining wave of the second fluctuation. When the optimistic trader exits the market in the second fluctuation, the stock price changes slightly and does not attract the pessimistic trader to enter the market. After k periods, the pessimistic trader who enters the market by short-selling in the second fluctuation should exit the market by buying the stock, which leads the stock price to increase again and attracts the optimistic trader to enter the market by buying the stock again, etc. In other words, the sentiment investor enters and exits the market repeatedly, which causes the stock price to fluctuate. The repeated movement of sentiment investor in and out of the market is the main reason for the sustainability of stock price fluctuations.
(V) A summary of the stock price fluctuation
As discussed above, positive information shocks the stock price to fluctuate strongly and continuously under the effect of the sentiment investor. To understand the fluctuation process, a summary is presented as follows:
- Between periods [ t +1, t + k +1]. Under a shock of positive information, the information trader and the optimistic trader buy the stock, accelerating the increase of stock price.
- Between periods [ t + k +2, t +2 k +1]. The optimistic trader exits the market by selling the stock and the pessimistic trader enters the market by short-selling, causing the stock price to continue to decline. Furthermore, the stock price accelerates to fall between periods [ t + k +3, t + k + λ ].
- Between periods [ t +2 k +3, t +3 k +1]. The pessimistic trader exits the market by short-selling and the optimistic trader enters the market by short-selling, causing the stock price to increase continuously. Furthermore, the stock price accelerates to increase between periods [ t +2 k +4, t +2 k + λ ].
Finally, the behavior of the sentiment investor who enters or exits the market is the main factor that leads the stock price to fluctuate powerfully and continuously, causing the sustainability of stock price fluctuations anomaly. The result shows that the price fluctuation process of the model is in line with the real financial market.
Comparing Eqs ( 17 ) and ( 18 ) with the stock price Eq ( 1 ) which is affected by the sentiment investor, we find that if there is no sentiment trader in the market, the stock price fluctuates slightly under the shock of information. This fluctuation with no sentiment influence is consistent with the standard finance theory. But if there is a sentiment trader in the market, the stock price fluctuates strongly and causes the sustainability of stock price fluctuations anomaly. Therefore, unlike other classical theoretical asset pricing models based on the heterogeneous [ 23 – 30 ], we conclude that the sustainability of stock price fluctuations is caused by the sentiment investor, which is consistent with the empirical results of [ 17 – 22 ].
5. Examples
To describe the fluctuation of the stock price and explain the sustainability of stock price fluctuations and overreaction, an example is provided in this section.
(i) Numerical Simulation of the Model
In the numerical simulation, we assume that a piece of positive information shocks the stock price, and the stock price fluctuation is influenced by the heterogeneous traders (the information trader, the sentiment trader and the noise trader) as discussed in the theoretical model. As the information is positive, the optimistic trader has a greater elasticity coefficient for the stock price change than the pessimistic trader. Specifically, the parameters used in the numerical simulation are shown in Table 1 .
https://doi.org/10.1371/journal.pone.0255081.t001
Using the parameters in Table 1 , we know that the basic value of the stock price after the shock of information is 55 in the simulation. The numerical simulations of stock price fluctuation are presented in Figs 2 – 5 . The results are as follows:
https://doi.org/10.1371/journal.pone.0255081.g002
https://doi.org/10.1371/journal.pone.0255081.g003
https://doi.org/10.1371/journal.pone.0255081.g004
https://doi.org/10.1371/journal.pone.0255081.g005
The level that stock price deviates from the basic value is closely related to the elasticity coefficient of the sentiment trader on stock price change. The larger the elasticity coefficients are, the higher the level of deviation is. In Fig 3 , W 0 = 0.6 and W p = 0.2, the highest stock price is 62.5 (1.14 times over the basic value), and the lowest stock price is 51.79 (0.94 times of the basic value). Thus, we conclude that the asset bubble, overreaction and financial crisis are attributable to investor sentiment, since the stock price deviates from the basic value which is caused by sentiment investor.
The stock price convergence and the elasticity coefficient of the sentiment trader are negatively correlated. By comparing Fig 2 with Fig 3 , and Fig 4 with Fig 5 , we find that the larger the elasticity coefficient of the sentiment trader is, the slower the stock price convergence is. In particular, in Fig 2 , where W 0 = 0.6 and W p = 0.3, after 500 periods, the stock price is still fluctuating. Moreover, a particular simulation shows that the stock price converges after 1045 periods which is 21 times as much as the survival period of the sentiment trader. However, in Fig 5 , W 0 = 0.5 and W p = 0.2, the stock price converges after 368 periods which is only 7 times as much as the survival period of the sentiment trader. All these imply that investor sentiment is the key factor that leads the stock price to fluctuate powerfully and causes the sustainability of stock price fluctuations anomaly.
To sum up, the numerical simulation also indicates that investor sentiment is the key factor that causes the stock price to fluctuate. The effect level of the sentiment is positively correlated with the fluctuation level of the stock price, which leads to the sustainability of stock price fluctuations. Furthermore, we show that overreaction, asset bubble and the financial crisis are also attributable to investor sentiment.
(ii) Robustness
https://doi.org/10.1371/journal.pone.0255081.t002
6. Conclusions
This paper derives a recursive dynamic asset pricing model with heterogeneous traders: the information trader, the sentiment trader and the noise trader. A theoretical discussion and a numerical simulation for the fluctuation of the stock price under the shock of information are given. The paper finds that investor sentiment is the key factor that leads the stock price to fluctuate powerfully and causes the sustainability of stock price fluctuations anomaly. The level that the stock price deflects the basic value is closely related to the elasticity coefficient of the sentiment trader on the stock price change. The greater the elasticity coefficient is, the higher the level that the stock price deflects the basic value is. Furthermore, the stock price convergence is closely related to the elasticity coefficient of the sentiment trader on the stock price change. The greater the elasticity coefficient of the sentiment trader is, the slower the stock price converges.
In brief, unlike other classical theoretical asset pricing models based on the heterogeneous [ 23 – 30 ], this paper depicts the fluctuation process of stock price under the common-effect of the information trader, the sentiment trader and the noise trader, proving that the investor sentiment is the key factor that causes the sustainability of stock price fluctuations anomaly [ 22 ]. Meanwhile, the asset price affected by investor sentiment is always overreacting [ 35 – 37 ]. However, the paper restricts the value of the elasticity coefficients of the sentiment trader on the stock price change. If these restrictions are elevated, the stock price may no longer converge or the convergence time is too long, which could explain the asset bubble [ 38 ] and the financial crisis caused by investor sentiment. This paper adopts the recursive method to build a dynamic asset pricing model based on heterogeneous investors. Compared with seminal works [ 23 – 30 ], this model is easier to simulate the trend of asset prices after information shock. It can intuitively show the persistence of asset price fluctuation through numerical simulation, and also shows that the sustainability of stock price fluctuations, the overreaction, the asset bubble, even the financial crisis are mainly caused by sentiment investor. This paper provides a new perspective for academics to construct dynamic asset pricing model based on heterogeneous, and provides theoretical basis for professionals in the financial industry to construct their quantitative investment strategy, and they should all pay more attention to investor sentiment, which is an important factor in the asset pricing.
However, this paper only discusses a recursive dynamic asset pricing model with three types of investors traders (the information trader, the sentiment trader and the noise trader). For the further direction of research, one is to further study the impact of other types of investors on stock price by using recursive method. The second is combining with the latest data of the Covid-19’s impact on stock price to empirically test the conclusion of the paper.
Supporting information
S1 file. program for the numerical simulation..
https://doi.org/10.1371/journal.pone.0255081.s001
S1 Appendix.
https://doi.org/10.1371/journal.pone.0255081.s002
Acknowledgments
The authors wish to thank the editor and anonymous reviewers who make valuable suggestions for revision and contributions to significantly enhancing the quality of this work.
- View Article
- Google Scholar
- PubMed/NCBI
Thank you for visiting nature.com. You are using a browser version with limited support for CSS. To obtain the best experience, we recommend you use a more up to date browser (or turn off compatibility mode in Internet Explorer). In the meantime, to ensure continued support, we are displaying the site without styles and JavaScript.
- View all journals
- My Account Login
- Explore content
- About the journal
- Publish with us
- Sign up for alerts
- Open access
- Published: 18 July 2022
ESG performance and stock prices: evidence from the COVID-19 outbreak in China
- Zengfu Li 1 ,
- Liuhua Feng 1 ,
- Zheng Pan 2 &
- Hafiz M. Sohail ORCID: orcid.org/0000-0002-7885-5549 1
Humanities and Social Sciences Communications volume 9 , Article number: 242 ( 2022 ) Cite this article
19k Accesses
14 Citations
3 Altmetric
Metrics details
- Social science
This paper investigates the role of environmental, social, and governance (ESG) performance in stock prices during the market financial crisis caused by the COVID-19 pandemic. We use the Chinese listed company data as the bases for adopting an event-study method to identify the impact of ESG performance on cumulative abnormal returns. Empirical results suggest that ESG performance significantly increases firms’ cumulative abnormal returns and has asymmetric effects during the pandemic. Our results are robust to various robustness checks that consider the replacement of event window period, ESG measurement, adding other control variables, and sample exclusion of Hubei Province. We further find that reputation and insurance effects are important mechanisms through which ESG performance influences stock prices. Lastly, heterogeneous analyses show that ESG effects are considerably pronounced among firms with low human capital and bad image and in high-impact regions.
Similar content being viewed by others
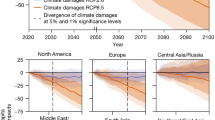
The economic commitment of climate change
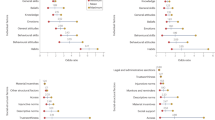
Determinants of behaviour and their efficacy as targets of behavioural change interventions
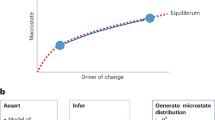
Entropy, irreversibility and inference at the foundations of statistical physics
Introduction.
In recent years, environmental, social, and governance (ESG) investments, frequently called ethical or sustainable investments, have rapidly increased globally (Galbreath, 2013 ). ESG investing is an investment process that integrates ESG considerations into investment decisions (Mǎnescu, 2011 ). Given the COVID-19 pandemic, the necessity of ESG investing has been highlighted again (Demers et al., 2021 ; Manabe and Nakagawa, 2022 ). Investors are interested in ESG investments for at least two reasons (Renneboog et al., 2008 ). First, by focusing on ESG investments, ethical investment practices are actively promoted (Baldini et al., 2018 ; Broadstock et al., 2021 ). Second, ESG investments are increasingly recognized as improving the performance of managed portfolios, reducing portfolio risks, and increasing returns (Albuquerque et al., 2020 ; Díaz et al., 2021 ; Broadstock et al., 2021 ).
Early literature on ESG investing has been partially inspired by studies of the eminent economist Milton Friedman (Friedman, 2007 ), who argued that ESG practices constitute a misallocation and misappropriation of valuable corporate resources. Renneboog et al. ( 2008 ) concluded that existing studies hint, but do not explicitly demonstrate, that ethical investors are willing to accept sub-optimal financial performance to pursue social or ethical objectives. Subsequently, a series of studies have expanded on the preceding literature. Some studies on ESG investing have focused on the application of returns and risk management. Hartzmark and Sussman ( 2019 ) found that investors make positive predictions on sustainable assets, steering money away from funds with low portfolio sustainability ratings to those with high ratings. They also found no evidence that high-sustainability funds outperform low-sustainability funds. Demers et al. ( 2021 ) determined that ESG performance facilitates the accumulation of intangible assets but does not serve as protection against downside risk.
However, emerging studies have supported the view that ESG-themed investments have low downside risks and are minimally volatile in price during turbulent times. Hoepner et al. ( 2021 ) and Pedersen et al. ( 2021 ) obtained empirical evidence that ESG engagement reduces firms’ downside risks and their exposure to downside risk factors. Albuquerque et al. ( 2020 ) developed a theoretical framework to show that stocks with high ESG ratings have significantly higher returns, lower return volatilities, and higher trading volumes than other stocks. Broadstock et al. ( 2021 ) showed that high-ESG portfolios typically outperform low-ESG portfolios, thereby mitigating financial risks during financial crises.
Although there is limited research on the specific role of ESG performance during times of crisis, some insights have been gained from the 2008–2009 global financial crisis. Nejati et al. ( 2010 ) noted that the root causes of the current economic crisis could be moderated by a global transparency and accountability system and a public reporting of ESG performance. Erragraguy and Revelli ( 2015 ) showed that the adoption of ESG standards by firms during the crisis increased transparency, mitigated information asymmetries, and improved stock market liquidity and quality. Henke ( 2016 ) demonstrated that high-ESG-rated funds outperformed low-ESG-rated funds during the crisis, further supporting the view that investors place intrinsic value on ESG investments.
In the first few months of 2020, the sudden market-wide financial crisis was triggered in response to the emerging global health crisis (i.e., COVID-19), the consequences of which were more severe than those of the Great Depression in 1929–1933 and the global financial crisis in 2007/2008 (Broadstock et al., 2021 ). The current study shows that stock prices are empirically tested for negative shocks during the COVID-19 pandemic in some products and firms but not in others (Al-Awadhi et al., 2020 ; Shen et al., 2020 ). This result leads to a compromise opinion that the role of ESG generally depends on exposure to crisis shocks. Moreover, we are motivated to question how ESG effects vary along with different products and firms during public crises. However, only a few studies have indicated the specific role of ESG performance in crisis periods. Therefore, the goal of this paper is to fill in this research gap.
We use the Chinese listed company data as the bases for adopting an event-study method to identify the impact of ESG performance on cumulative abnormal returns. The empirical results show that ESG performance is positively associated with cumulative abnormal returns during the COVID-19 pandemic. When decomposing firms with positive and negative shocks, we find that cumulative abnormal returns are positively related to ESG among firms with negative shocks but not positive shocks. These results suggest that the importance of ESG performance is reinforced in times of crisis, and is consistent with the inference that investors use ESG performance as a signal of future returns and risk mitigation.
Our work builds on the current literature on the role of ESG performance in stock prices (Duuren et al., 2016 ; Remmer Sassen et al., 2016 ; Jagannathan et al., 2017 ) and extends the results on ESG to public crisis events. We use an event study approach to analyze the volatility of ESG performance on stock prices during the COVID-19 pandemic. Compared with the existing literature (Nejati et al., 2010 ; Erragraguy and Revelli, 2015 ; Henke, 2016 ), this study provides a more comprehensive perspective on the importance of ESG performance in major crises. Understanding the importance of ESG is necessary because it is a crucial indicator of risk management, non-financial performance, and sustainability. Through ESG practices, firms can obtain significant reputation and risk protection to reduce price volatility in times of crisis, thereby contributing to their long-term operations and sustainability.
This study adds to the limited number of prior studies that have examined the impact of firms’ ESG performance on their stock prices but have provided conflicting results (Friedman, 2007 ; Renneboog et al., 2008 ; Hartzmark and Sussman, 2019 ; Demers et al., 2021 ). The current study also provides additional empirical evidence on the important mechanisms of ESG performance through further analysis, thereby opening a black box for a positive relationship between ESG performance and stock prices. The results of this study suggest that reputation and insurance effects are important mechanisms by which ESG performance influences stock prices. This outcome reflects the fact that sustainability investments have low downside risks and are minimally volatile in price during turbulent periods. This finding clarifies the important role of current ESG practices in guiding investors’ decision-making and provides empirical evidence for investors to focus on sustainable investment.
This paper also complements the literature on the effects of public crises on financial markets. Existing literature has discussed the influence on financial markets mainly in terms of the 2008–2009 financial crisis (Nejati et al., 2010 ; Erragraguy and Revelli, 2015 ; Henke, 2016 ). We differ from these studies by specifically focusing on the COVID-19 pandemic. Note that the subject of our study is Chinese listed companies. Given that China is the second-largest economy in the world where the COVID-19 public crisis spread earlier and was interrupted by containment measures, a reasonable undertaking is to investigate the influence of this crisis on financial markets in the Chinese sample we use compared with other countries.
The remainder of this paper is organized as follows. Section “Data and methodology” presents the sample and variables. Section “Empirical results” discusses the main empirical results and robustness analyses. Section “Further analysis” provides further mechanisms. Lastly, section “Conclusion” concludes the study.
Data and methodology
Data of this study cover Chinese non-financial A-share listed firms in 2020. We collect data from several resources. First, we acquire ESG data from China Sino-Securities Index Information Service (Shanghai) Company Limited, a third-party data provider based in China specializing in ESG data. Second, stock prices, firm financial data, and firm management data are obtained from China Securities Markets and Accounting Research Database. In particular, stock prices are measured using cumulative abnormal returns calculated by utilizing the event-study method. Third, we obtain media coverage data mainly from the Chinese Research Data Service database to measure the level of media attention.
Main variables
Data of this study cover Chinese non-financial A-share listed firms in 2020. We collect data from several resources. First, we acquire ESG data from China Sino-Securities Index Information Service (Shanghai) Company Limited, a third-party data provider based in China specializing in ESG data. Second, stock prices, firm financial data, and firm management data are obtained from the China Securities Markets and Accounting Research Database. Specifically, stock prices are measured using cumulative abnormal returns calculated by the event-study method. Third, we obtain media coverage data mainly from the Chinese Research Data Service database to measure the level of media attention.
Stock price (CAR)
Cumulative abnormal return (CAR) is widely used as a measure of stock price. We follow the previous literature (Demers et al., 2021 ; Zhang et al., 2021 ; Broadstock et al., 2021 ) and use CAR estimated through an event study approach as the dependent variable. CAR is an impartial estimate of additional or reduced firm value that accrues as a result of the occurrence (McWilliams and Siegel, 1997 ; Campbell et al., 1998 ; Fernando et al., 2012 ). In the COVID-19 context, this paper uses the event study method to analyze the impact of ESG performance on stock prices.
In an event study, we need to determine the event date, event window, estimation window, and estimation model. The details are as follows. (1) Event date : As COVID-19 received national attention on 20 January 2020, we draw on existing literature (Zhang et al., 2021 ) and use this date as an event date in the event study. On 20 January 2020, China’s central government provided important instructions on the prevention and containment of COVID-19. On the same day, panic ensued after the announcement by pulmonary experts that COVID-19 was more transmissible than previous diseases and could be passed from one person to another person. As of 20 January 2020, the Chinese National Health Council officially issued the number of new cases in each province and incorporated COVID-19 into the Infectious Diseases Act and Sanitary and Isolation Act (Zhang et al., 2021 ). (2) Event window : This paper follows previous studies (Kanas, 2005 ; Miyajima and Yafeh, 2007 ; Fernando et al., 2012 ) and selects the event window consisting of 11 days from t −5 to t +5 days. (3) Estimation window : Campbell et al. ( 1998 ) documented that the selection of estimation window for short-term events can be 120 days or even longer. Thus, we choose the estimated window period of 175 days from t −210 to t −36 days. (4) Estimation model : We use the existing literature (Campbell et al., 1998 ) as a basis to take the OLS market model for calculating the expected returns.
This study requires the calculation of expected, abnormal, and cumulative abnormal returns (Campbell et al., 1998 ). We first calculate each firm’s expected return (ER i,t ) during the event period:
where ER i,t is the expected return of firm i on day t during the event period, R M , t represents the market return on day t during the event period, and β 0 and β 0 are the estimated parameters in Model (1). Thereafter, we use expected returns (ER i , t ) gained from Model (1) to calculate the abnormal returns during the event period. This study estimates abnormal returns (AR i,t ) based on the Fama et al. ( 1969 ) market model:
where AR i,t is the abnormal return of firm i on day t during the event period, R i,t is the actual return of firm i on day t during the event period, and ER i , t is the expected return obtained from Model (1). Lastly, we use abnormal returns (AR i,t ) obtained from Model (2) to calculate the cumulative abnormal returns:
where CAR i,t is a calculation from the period of days between k and j . In this paper, the event windows to calculate CAR is [−5, 5].
ESG performance (ESG)
The Independent variable used in this study is the quarterly score of ESG performance, which is calculated based on three dimensions: environmental, social, and governance. Given that ESG data are disclosed quarterly, we calculate the average of ESG over four quarters to measure the annual ESG performance. We also follow the previous literature (Zhang et al., 2021 ; Broadstock et al., 2021 ; Díaz et al., 2021 ; Demers et al., 2021 ) and include the following set of control variables in the estimation: leverage ratio (Lev), firm profitability (Roa), firm size (Size), nature of equity (Soe), degree of risk (Beta), shareholder structure (Top1), number of board members (Board), the duality of CEO and chairman (Dual), the proportion of independent directors (Independ), intangible asset (Intangible), and tangible asset (Tangible). The detailed definitions of the variables are presented in Table 1 .
Summary statistics
In response to the COVID-19 pandemic, this paper selects Chinese A-share listed companies as a study sample. This study selects the sample based on the following considerations. First, the financial sector is excluded owing to the uniqueness of its business, financial reporting, and regulatory structure. Second, we exclude firms with losses and those specially treated by stock exchanges. Third, we exclude samples with an estimation window of under 175 trading days. Fourth, our article removes samples with missing values in the ESG, CAR, and control variables. Lastly, continuous variables are winsorized at the 1% and 99% levels to mitigate concerns with extreme values. The resulting sample in our study is 2188 observations.
Table 2 provides the descriptive statistics of the variables. CAR index ranges from −0.2496 to 0.4665. In addition, the mean and median of CAR are −0.0115 and −0.0367, respectively, with a standard deviation of 0.1223. This result suggests that the level of cumulative abnormal returns varies considerably across firms and that overall cumulative abnormal returns are low. Moreover, this situation implies that firms are generally subject to negative shocks during the COVID-19 pandemic. The average ESG is 0.8447, which is within the range of good. For the control variables, the average leverage is 44.21%, ROA is 5.13%, and firm size is 22.59. Our sampled chairman has ~27.06% of the CEO, and the largest shareholder holder accounts for 34.61% of firm stocks. The average board size is about 8 (= e 2.1260 ) members, 37.61% of whom are independent directors. This result is consistent with the CSRC requirement for board independence (Wang et al., 2021 ). The distribution of the control variables in this paper is similar to that reported in previous research (Zhang et al., 2021 ; Broadstock et al., 2021 ; Díaz et al., 2021 ).
Figure 1 shows a graphical representation of AARs and CARs for the event window [−5, 5]. As shown in Fig. 1 , the two trend lines rise initially and fall thereafter from the announcement date. In the 4 days from 20 to 23 January 2020, firms reacted positively to the pandemic market. In the early stage of the COVID-19 pandemic, the demand for products to prevent pandemic infection was greater than the supply. This phenomenon prompted speculators to use arbitrage opportunities to invest, thereby explaining the positive response of the capital market.
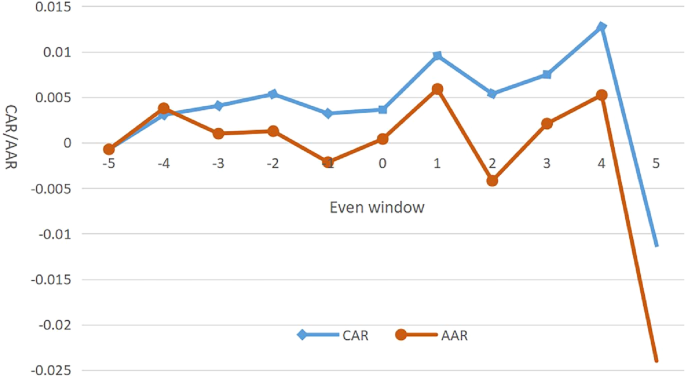
Trend of AARs and CARs for the event window ( t −5 – t +5 days).
On the 4th day of the COVID-19 outbreak (23 February 2020), the city of Wuhan, which was the most affected city, was closed. Shortly after the lockdown, the stock market was closed for the Chinese Spring Festival from 24 January to 2 February. The market reopened on 3 February. Note that on the 5th day of trading following the COVID-19 outbreak, which was the first trading day after the closure of Wuhan (3 February 2020), the response of firms to the pandemic was extremely negative. The pandemic caused chaos and weakened the economy of China, particularly after the closure of Wuhan. The closure of the city to curb the further spread of the disease will destroy the entire logistics and supply chain system (Tang et al., 2021 ). Most factories faced shutdowns, production stoppages, and even closures, thereby causing a significant downward trend across the capital markets. The downward trend of the stock market was consistent with the previous statistical description that the COVID-19 epidemic in 2019 has had a significant negative impact on the financial market.
Empirical results
Effect of esg performance on firms’ stock price.
We use the following multiple regression model to investigate the general relationship between ESG performance and firm stock prices during the COVID-19 pandemic:
where i denotes the firm, CAR represents the firm’s cumulative abnormal return during the COVID-19 pandemic, and ESG is the firm’s ESG performance measure proxied by its ESG score. Our results focus on β 1 , which captures how ESG performance affects a firm’s stock price. The vector Controls i stacks a series of control variables that account for the impact of firm characteristics on the stock price. Details of these variables are described in the section “ESG performance (ESG)”. We also include province-fixed effects to control for unobservable regional characteristics. ESG is a highly industry-related variable (Yu and Luu, 2021 ). To control for industry characteristics, we control for industry fixed effects, in which ε i is the error term. Variables and definitions used in the model are shown in Table 1 .
We report the results of the baseline OLS regressions in Table 3 . In column (1), we include only the ESG and CAR indicators after controlling for the province- and industry-fixed effects. The coefficient of ESG is 0.1479 ( t -stat 3.5072), which is positive and statistically significant at the 1% level. This result indicates that ESG practices play an important role in reducing price volatility in times of crisis. Our results show that ESG performance has a significant positive impact on the cumulative excess return. In other cases, we include firm control variables in column (2). However, estimates remain positive and statistically significant. For control variables, we find that the coefficient of Size is significantly positive, suggesting that large firms reduce their risk of stock price declines. Conversely, the coefficient of Lev is negative, implying that firms with more leverages will suffer a greater risk of stock price declines. Our results are consistent with those of previous studies regarding control variables (Demers et al., 2021 ; Broadstock et al., 2021 ). In summary, our results provide suggestive evidence that firms with high ESG ratings are conducive to mitigating the downside risk of stock prices during the COVID-19 pandemic.
To significantly understand whether or not the role of ESG depends on the risk exposure characteristics of crisis shock, this paper examines the influence of ESG performance on stock prices of firms subject to positive and negative shocks during the COVID-19 pandemic. We choose two approaches to classify the sample into firms with positive and negative shocks during the pandemic. On the one hand, this paper draws on the existing literature (Kanas, 2005 ; Miyajima and Yafeh, 2007 ) in classifying the positive and negative shock groups by determining whether or not the cumulative abnormal return is above 0. On the other hand, we use the previous literature (Al-Awadhi et al., 2020 ; Shen et al., 2020 ) as a basis for identifying the following industries as positive groups: information technology and medicine manufacturing industries. Moreover, we select the following industries to be identified as negative groups: tourism, transportation, restaurants, wholesale and retail trade, realty business, and export manufacturing industries.
Table 4 shows the results of the tests of exposure characteristics during the crisis. The first two columns and last two columns show that the results are similar whether the sample is divided in the first or second way. Coefficients of ESG for firms in the negative group are positive and statistically significant. Conversely, coefficients of ESG for firms in the positive group is not statistically significant. These results suggest that the positive effect of ESG performance on stock prices is more significant among firms that are more severely affected by negative shocks. That is, our findings provide evidence that ESG performance acts as a risk protection tool that contributes to the sustainability of operations in turbulent times, specifically among firms with severe negative shocks.
Robustness tests
Alternative event window periods.
In the baseline regression, we mainly use an event window of [−5, 5] for the test. However, the role of ESG performance for the shorter or longer event windows is unclear. This section investigates whether or not our results hold after replacing the shorter and longer event windows. These event windows are as follows: [−3, 3], [−7, 10], [−7, 11], and [−7, 14]. The first four columns of Table 5 present the results of the tests for alternative event window periods, in which each column represents a different event window. Column (1) shows that the coefficient of ESG is 0.0752 ( t -stat 2.8186) in the short-term event window [−3, 3], which is positive at the 1% level. We also find that the coefficient of ESG is 0.1327 ( t -stat 2.6966) in the long-term event window [−7, 10], which is positive at the 1% level. Coefficient of ESG is also significantly positive in the event windows [−7, 11] and [−7, 14], albeit at a weaker level of significance (5%, 10%). In summary, these findings provide strong evidence that ESG plays an important role in preventing downside risk during turbulent times.
Alternative proxies for ESG performances
Given that raw data for the ESG score is quarterly, this paper uses it for testing the annual data of firms that are susceptible to measurement error and raise concerns about their validity. To address these potential issues, we consider two alternative proxies for ESG performance. First, we choose the median of the quarterly data on ESG scores (ESG1) to measure ESG performance. Second, we measure ESG performance as ESG score in the first quarter of year t + 1 (ESG2). Similarly, we find a robust positive association between ESG performance and stock price, as shown in columns (5) and (6) of Table 5 .
Excluding the Hubei sample
This paper considers a subsample that excludes Hubei Province, which was the most affected by COVID-19. The early outbreak of COVID-19 in China was concentrated in Wuhan (Wuhan), Hubei Province, and spread rapidly to other provinces. Hubei has many listed firms. Thus, we draw on the existing literature (Ren et al., 2021 ) and exclude the Hubei sample to emphasize that our results are not driven by firms in the province most affected by COVID-19. We re-estimate our regression using the subsamples. Column (7) of Table 5 shows that the coefficient of ESG is positive and significant, which remains consistent with our findings.
Inclusion of other variables
Existing research has suggested that institutional investor ownership and cash holdings of firms may be associated with stock price volatility (Bushee and Noe, 2000 ; Chang et al., 2017 ). Whether or not our regression results are sensitive to include institutional investor ownership and cash holdings has aroused our concern. To mitigate this concern, we control for institutional investor ownership and cash holdings of firms in the regression process. In column (8) of Table 5 , empirical results remain positive and statistically significant for the cumulative abnormal returns. The inclusion of firms’ institutional investor ownership and cash holdings does not induce material change in coefficient magnitudes. That is, empirical evidence implies that the inclusion of institutional investor ownership and cash holdings should not be an issue in our research.
Further analysis
Potential mechanism.
This section extends the preceding results to clarify the potential mechanisms of why ESG performance positively affects firms’ cumulative abnormal returns.
Reputation effect
Demers et al. ( 2021 ) suggested that ESG performance is an intangible asset, which plays a positive role in optimizing supply chain partnerships, enhancing consumer product satisfaction, and improving employee productivity. Given the attention of social media and stakeholders, ESG practices are gradually becoming a tool for corporate impression management that can enhance social visibility and expand market share (Lokuwaduge and Heenetigala, 2017 ; Xie et al., 2019 ). Thus, we expect ESG performance to be conducive to increasing cumulative abnormal corporate returns through reputation enhancement.
In particular, we take the number of positive online media and financial newspaper reports to represent the reputation gained by the firm. Thereafter, we use the natural logarithm of the number of positive online media and financial newspaper reports as our variables New1 and New2, respectively. To test the reputation effect mechanism, we introduce an interaction term between ESG and New1 or New2 in our model. As shown in columns (1) and (2) of Table 6 , the coefficients of New1 and New2 are significantly positive, suggesting that ESG practices are beneficial in enhancing corporate reputation. We also find that the coefficients of ESG × New1 and ESG × New2 are significantly negative, implying that the reputation effect of ESG performance is more pronounced among firms with lower reputations. Our findings demonstrate that ESG practices are beneficial in enhancing corporate reputation to improve cumulative excess returns during turbulent times.
Insurance effect
Academics and practitioners agree that firms’ risk exposures are linked to their ESG profiles. Albuquerque et al. ( 2020 ) presented empirical evidence suggesting that firms’ increasing product differentiation through ESG investments reduces systemic risk and improves firm value. Hoepner et al. ( 2021 ) found corroborating evidence that ESG practices reduce firms’ exposure to downside risk factors. These results support practitioner arguments that including ESG factors in investment decisions can mitigate uncompensated portfolio risks (Jagannathan et al., 2017 ; Pandey and Kumari, 2021 ; Broadstock et al., 2021 ). Hence, we expect ESG performance to be considered a risk management tool during the COVID-19 pandemic to increase cumulative excess returns by reducing business risk.
This paper Utilizes the previous literature (Ghosh and Olsen, 2009 ) as basis in using the standard deviation of sales revenue over the past 5 years (Risk1) to measure business operating risk. To remove the effect of industry, we also use the standard deviation of sales revenue over the past 5 years adjusted for industry (Risk2) to measure business operating risk. We introduce an interaction term between ESG and EU1 or EU2 in the model to test the insurance effect mechanism. The results in columns (3) and (4) of Table 6 show that the coefficients of Risk1 and Risk2 are negative and significant. This result is consistent with findings of previous studies that ESG performance reduces downside risk. We also find that coefficients of ESG × Risk1 and ESG × Risk2 are significantly positive, suggesting that the insurance effect of ESG performance is markedly pronounced among firms with high operational risk. The results of this study indicate that ESG practices can be used as a risk management tool in turbulent times, thereby increasing cumulative abnormal returns. These findings further support the insurance-enhancing effects of ESG practices.
Cross-sectional analysis
In this subsection, we conduct several cross-sectional tests to examine how the impact of ESG performance on cumulative abnormal returns varies with firm characteristics during the COVID-19 pandemic.
Cross-sectional tests of human capital characteristics
We investigate whether or not the human capital characteristics of firms affect the contribution of ESG performance in terms of cumulative abnormal returns during the COVID-19 pandemic. Apart from the detrimental influence of the virus on staff safety, the lockdown and physical separation measures damage firms’ financial performance. Fahlenbrach et al. ( 2020 ) showed that labor-intensive firms, in which work-from-home policies are difficult to implement, have high exposure to COVID-19. By contrast, firms with high technological equipment are less affected and even have the opportunity to expand their business (Li et al., 2021 ). Thus, we predict that firms with low human capital are considerably at risk during the COVID-19 pandemic, thereby possibly increasing the sensitivity of ESG performance to cumulative abnormal returns.
This paper draws on prior literature (Li et al., 2021 ) and selects the ratio of the number of employees to sales as a measure of human capital intensity (Labor). The more value of Labor, the higher the productivity of employees and the higher the human capital of the firm. In addition, we divide the two groups of high and low human capital according to whether or not Labor is above the median value of the sample. Columns (1) and (2) of Table 7 show that the coefficient of ESG is significantly positive at the 1% level among firms with low human capital, but it is not statistically significant among firms with high human capital. Thus, the role of ESG performance in preventing stock price downside is markedly significant among firms with low human capital during the COVID-19 pandemic. This result is consistent with our expectations.
Cross-sectional test of public image characteristics
This subsection further investigates whether or not our main results are influenced by corporate image. The concepts of corporate image and trust have obtained special relevance, which significantly influence individual behavior (Rindell et al., 2015 ). Flavián et al. ( 2005 ) argued that the image perceived by consumers makes the factors existing in the transaction visible, thereby reducing the risk perceived by individuals and increasing the possibility of purchase. Compared with firms with bad image, firms with good image are expected to have stronger protection against downside risks (Lee et al., 2022 ). Consequently, we predict that firms with bad image have a high downside risk during the COVID-19 pandemic, thereby possibly increasing the sensitivity of ESG performance to cumulative abnormal returns.
This study chooses the number of negative reports in online media (Bad_image) measure corporate image. Columns (3) and (4) of Table 7 report that the coefficient of ESG is significantly positive at the 1% level among firms with numerous negative online media reports (firms with Bad_image above the sample median), but it is not statistically significant at a low number of negative online media reports. This finding is consistent with our expectation that firms with bad image face high downside risk during the COVID-19 pandemic, thereby increasing the sensitivity of ESG performance to cumulative abnormal returns.
Cross-sectional test of regional characteristics
Lastly, we explore the heterogeneous effect of ESG performance on cumulative abnormal returns for firms with different geographical locations. Shen et al. ( 2020 ) noted that by using the region as a criterion, COVID-19 has a major negative influence on the serious-impact regions. After China began its comprehensive campaign against COVID-19, seven provinces, namely, Hubei, Hunan, Henan, Jiangxi, Anhui, Guangdong, and Zhejiang, enforced harsh labor restrictions and the resumption of work. These restrictions have led to a decline in consumption levels and closure of many firms in high-impact areas. By contrast, for cities far from the infected areas, the resumption of operations will be significantly earlier. Fu and Shen ( 2021 ) showed that the early resumption of work sends a signal of reduced risk to stakeholders, thereby promoting firms to obtain more investment capital. In summary, we expect that the protection of ESG performance against downside risk during the COVID-19 pandemic is more significant in high-impact regions.
We draw on the previous literature (Shen et al., 2020 ) in selecting seven provinces (i.e., Hubei, Hunan, Henan, Jiangxi, Anhui, Guangdong, and Zhejiang) as high-impact regions and other provinces as low-impact regions. Columns (5) and (6) of Table 7 show that the coefficient of ESG is significantly positive at the 1% level for firms in high-impact regions, but it is not statistically significant for firms in low-impact regions. This result suggests that ESG practices of firms in high-impact regions play a key role in risk management and reduce stock price volatility during the COVID-19 pandemic.
During the COVID-19 pandemic, the significant decrease in global equity values reflects strong negative investor sentiments (Broadstock et al., 2021 ). Hence, we ask if this negative sentiment transfers asymmetrically across the firms, or whether or not ESG performance may be used as a valuable signal for systematically avoiding negative risk during the crisis. However, only a few studies have provided the specific role of ESG performance in this crisis period. Therefore, the goal of this paper is to fill in this research gap.
We use a unique environmental setting and find that ESG performance is positively associated with cumulative abnormal returns around the COVID-19 pandemic and has an asymmetric impact. We contribute to the literature with empirical evidence on the resilience of stocks with high ESG performance during financial crises. This finding is consistent with the view that investors may take ESG performance as a signal of risk mitigation during the crisis. We further find that the reputation and insurance effects are important mechanisms through which ESG performance influences stock price. Moreover, heterogeneous analyses show that ESG effects are considerably pronounced among firms with low human capital, bad image, and in high-impact regions. Overall, we conclude that ESG practices can be used as a risk management tool to enhance share price resilience, particularly in turbulent times.
Our findings are of particular relevance to business managers, investors, and policy makers. For managers, this paper provides empirical evidence supporting ESG investing as a value-enhancing strategy. In addition, we find evidence that ESG practices act as impression and risk management tools to reduce risk downside in turbulent times. Thus, firms should elevate their ESG performance to make them markedly attractive targets in the market to expand their market share. For investors, ESG investments improve the performance of managed portfolios, reduce portfolio risk, and increase returns. Investors should consider ESG factors in their investment decisions to enhance investment returns in turbulent times. Lastly, policy makers should advocate the adoption of ESG practices and encourage companies to disclose information on ESG performance, which are essential for economic sustainability.
Several limitations should be considered in future research. One of the limitations of this study is that the data set only includes large listed firms selected from the China Securities Market and Accounting Research Database. In our opinion, limited resources may play a key role in determining the ESG performance of small firms. Consequently, the inclusion of small- and medium-sized firms may provide different results, which will be left for future research. Furthermore, this study is mainly based on a sample of Chinese listed companies, thereby limiting the generalizability of this research. Lastly, future research could investigate whether or not these results are valid in the context of developed countries or international markets, in which business strategies and ESG disclosures of firms are influenced by the institutional environment.
Data availability
Data set used in this study is available from the corresponding author on the reasonable request. Further data is publically available on China Securities Markets and Accounting Research Databases.
Al-Awadhi AM, Alsaifi K, Al-Awadhi A, Alhammadi S (2020) Death and contagious infectious diseases: impact of the COVID-19 virus on stock market returns. J Behav Exp Finance 27:100326. https://doi.org/10.1016/j.jbef.2020.100326
Article PubMed PubMed Central Google Scholar
Albuquerque R, Koskinen Y, Yang S, Zhang C (2020) Love in the time of COVID-19: the resiliency of environmental and social stocks. CEPR Discussion Papers
Baldini M, Maso LD, Liberatore G, Mazzi F, Terzani S (2018) Role of country- and firm-level determinants in environmental, social, and governance disclosure. J Bus Eth 150:79–98. https://doi.org/10.1007/s10551-016-3139-1
Article Google Scholar
Broadstock DC, Chan K, Cheng LTW, Wang X (2021) The role of ESG performance during times of financial crisis: evidence from COVID-19 in China. Finance Res Lett 38:101716. https://doi.org/10.1016/j.frl.2020.101716
Bushee BJ, Noe CF (2000) Corporate disclosure practices, institutional investors, and stock return volatility. J Account Res 38:171–202. https://doi.org/10.2307/2672914
Campbell JY, Mackinlay AC, Lo AW (1998) The econometrics of financial markets. J Empir Finance 3(1):15–102
MATH Google Scholar
Chang Y, Benson K, Faff R (2017) Are excess cash holdings more valuable to firms in times of crisis? Financial constraints and governance matters. Pac-Basin Finance J 45:157–173
Demers E, Hendrikse J, Joos P, Lev B (2021) ESG did not immunize stocks during the COVID-19 crisis, but investments in intangible assets did. J Bus Finance Account 48:433–462. https://doi.org/10.1111/jbfa.12523
Díaz V, Ibrushi D, Zhao J (2021) Reconsidering systematic factors during the Covid-19 pandemic—the rising importance of ESG. Finance Res Lett 38:101870
Duuren EV, Plantinga A, Scholtens B (2016) ESG integration and the investment management process: fundamental investing reinvented. J Bus Eth 138:525–533
Erragraguy E, Revelli C (2015) Should Islamic investors consider SRI criteria in their investment strategies. Finance Res Lett 14:11–19. https://doi.org/10.1016/j.frl.2015.07.003
Fahlenbrach R, Rageth K, Stulz RM (2020) How valuable is financial flexibility when revenue stops? Evidence from the COVID-19 crisis. Working Paper Series
Fama E, Fisher L, Jensen M, Roll R (1969) The adjustment of stock prices to new information. Int Econ Rev 10:1–21. https://doi.org/10.2139/ssrn.321524
Fernando CS, May AD, Megginson WL (2012) The value of investment banking relationships: evidence from the collapse of lehman brothers. J Finance 67:235–270
Flavián C, Guinalíu M, Torres E (2005) The influence of corporate image on consumer trust: a comparative analysis in traditional versus internet banking. Internet Res 15:447–470. https://doi.org/10.1108/10662240510615191
Friedman M (2007) The social responsibility of business is to increase its profits. In: Zimmerli WCh, Holzinger M, Koordination CSR und Nachhaltigkeit (eds). Corporate ethics and corporate governance. Springer, pp. 173–178
Fu M, Shen H (2021) COVID-19 and corporate performance in the energy industry. Energy Res Lett 1:1–4. https://doi.org/10.46557/001c.12967
Galbreath J (2013) ESG in focus: the Australian evidence. J Bus Eth 118:529–541. https://doi.org/10.1007/s10551-012-1607-9
Ghosh D, Olsen L (2009) Environmental uncertainty and managers’ use of discretionary accruals. Account Organ Soc 34:188–205. https://doi.org/10.1016/j.aos.2008.07.001
Hartzmark SM, Sussman AB (2019) Do investors value sustainability? A natural experiment examining ranking and fund flows. J Finance 74:2789–2837. https://doi.org/10.1111/jofi.12841
Henke H-M (2016) The effect of social screening on bond mutual fund performance. J Bank Finance 67:69–84. https://doi.org/10.1016/j.jbankfin.2016.01.010
Hoepner AGF, Oikonomou I, Sautner Z, Starks LT, Zhou X (2021) ESG shareholder engagement and downside risk. Soc Sci Res Netw. Finance Working Paper No. 671/2020.
Jagannathan R, Ravikumar A, Sammon M (2017) Environmental, social, and governance criteria: why investors are paying attention. National Bureau of Economic Research
Kanas A (2005) Pure contagion effects in international banking: the case of BCCI’s failure. J Appl Econ 8:101–123. https://doi.org/10.1080/15140326.2005.12040620
Lee S, Lee D, Hong C, Park M-H (2022) Performance of socially responsible firms during the COVID-19 crisis and trading behavior by investor type: evidence from the Korean stock market. Finance Res Lett 45:102660. https://doi.org/10.1016/j.frl.2021.102660
Li K, Liu X, Mai F, Zhang T (2021) The role of corporate culture in bad times: evidence from the COVID-19 pandemic. J Financ Quant Anal 56:2545–2583
Lokuwaduge CSDS, Heenetigala K (2017) Integrating environmental, social and governance (ESG) disclosure for a sustainable development: an Australian study. Bus Strategy Environ 26:438–450. https://doi.org/10.1002/bse.1927
Manabe T, Nakagawa K (2022) The value of reputation capital during the COVID-19 crisis: evidence from Japan. Finance Res Lett 46:102370. https://doi.org/10.1016/j.frl.2021.102370
Mǎnescu C (2011) Stock returns in relation to environmental, social and governance performance: mispricing or compensation for risk? Sustain Dev19:95–118
McWilliams A, Siegel D (1997) Event studies in management research: theoretical and empirical issues. Acad Manag J 40:626–657. https://doi.org/10.2307/257056
Miyajima H, Yafeh Y (2007) Japan’s banking crisis: an event-study perspective. J Bank Finance 31:2866–2885. https://doi.org/10.1016/j.jbankfin.2007.03.006
Nejati M, Shah Bin A, Shahbudin, Bin Amran A (2010) Sustainable development: a competitive advantage or a threat? Bus Strategy Ser 11:84–89
Pandey DK, Kumari V (2021) Event study on the reaction of the developed and emerging stock markets to the 2019-nCoV outbreak. Int Rev Econ Finance 71:467–483. https://doi.org/10.1016/j.iref.2020.09.014
Pedersen LH, Fitzgibbons S, Pomorski L (2021) Responsible investing: the ESG-efficient frontier *. J Financ Econ 142:572–597. https://doi.org/10.1016/j.jfineco.2020.11.001
Ren Z, Zhang X, Zhang Z (2021) New evidence on COVID-19 and firm performance. Econ Anal Policy 72:213–225. https://doi.org/10.1016/j.eap.2021.08.002
Renneboog L, Ter Horst J, Zhang C (2008) Socially responsible investments: institutional aspects, performance, and investor behavior. J Bank Finance 32:1723–1742. https://doi.org/10.1016/j.jbankfin.2007.12.039
Rindell A, Santos FP, de Lima AP (2015) Two sides of a coin: connecting corporate brand heritage to consumers’ corporate image heritage. J Brand Manag 22:467–484. https://doi.org/10.1057/bm.2015.20
Sassen R, Hinze A-K, Hardeck I (2016) Impact of ESG factors on firm risk in Europe. J Bus Econ 86:867–904. https://doi.org/10.1007/s11573-016-0819-3
Shen H, Fu M, Pan H, Yu Z, Chen Y (2020) The impact of the COVID-19 pandemic on firm performance. Emerg Markets Finance Trade 56:2213–2230
Tang C-H, Chin C-Y, Lee Y-H (2021) Coronavirus disease outbreak and supply chain disruption: evidence from Taiwanese firms in China. Res Int Bus Finance 56:101355. https://doi.org/10.1016/j.ribaf.2020.101355
Article PubMed Google Scholar
Wang L, Dai Y, Kong D (2021) Air pollution and employee treatment. J Corporate Finance 70:102067. https://doi.org/10.1016/j.jcorpfin.2021.102067
Xie J, Nozawa W, Yagi M, Fujii H, Managi S (2019) Do environmental, social, and governance activities improve corporate financial performance? Bus Strategy Environ 28:286–300. https://doi.org/10.1002/bse.2224
Yu EP, Luu BV (2021) International variations in ESG disclosure—do cross-listed companies care more. Int Rev Financ Anal 75:101731. https://doi.org/10.1016/j.irfa.2021.101731
Zhang P, Gao J, Li X (2021) Stock liquidity and firm value in the time of COVID-19 pandemic. Emerg Mark Finance Trade 57:1578–1591. https://doi.org/10.1080/1540496X.2021.1898368
Download references
Acknowledgements
This research work was funded by National Social Science Fund of China under the grant (19BGL057).
Author information
Authors and affiliations.
School of Economics & Management, South China Normal University, Guangzhou, Guangdong, China
Zengfu Li, Liuhua Feng & Hafiz M. Sohail
College of Management, Zhongkai University of Agriculture and Engineering, Guangzhou, Guangdong, China
You can also search for this author in PubMed Google Scholar
Contributions
LHF conceptualized, conducted the econometric analysis, wrote the introduction and conducted the literature review. ZL conceptualized, conducted the econometric analysis and supervised the work. ZP was mainly responsible for writing structure guidance. HMS analyzed the findings and wrote the conclusions and recommendations and edited the entire draft.
Corresponding author
Correspondence to Zengfu Li .
Ethics declarations
Competing interests.
The author declares no competing interests.
Ethical approval
This article does not contain any studies with human participants or animals performed by any of the authors.
Informed consent
Additional information.
Publisher’s note Springer Nature remains neutral with regard to jurisdictional claims in published maps and institutional affiliations.
Rights and permissions
Open Access This article is licensed under a Creative Commons Attribution 4.0 International License, which permits use, sharing, adaptation, distribution and reproduction in any medium or format, as long as you give appropriate credit to the original author(s) and the source, provide a link to the Creative Commons license, and indicate if changes were made. The images or other third party material in this article are included in the article’s Creative Commons license, unless indicated otherwise in a credit line to the material. If material is not included in the article’s Creative Commons license and your intended use is not permitted by statutory regulation or exceeds the permitted use, you will need to obtain permission directly from the copyright holder. To view a copy of this license, visit http://creativecommons.org/licenses/by/4.0/ .
Reprints and permissions
About this article
Cite this article.
Li, Z., Feng, L., Pan, Z. et al. ESG performance and stock prices: evidence from the COVID-19 outbreak in China. Humanit Soc Sci Commun 9 , 242 (2022). https://doi.org/10.1057/s41599-022-01259-5
Download citation
Received : 01 March 2022
Accepted : 04 July 2022
Published : 18 July 2022
DOI : https://doi.org/10.1057/s41599-022-01259-5
Share this article
Anyone you share the following link with will be able to read this content:
Sorry, a shareable link is not currently available for this article.
Provided by the Springer Nature SharedIt content-sharing initiative
Quick links
- Explore articles by subject
- Guide to authors
- Editorial policies

Corporate Social Responsibility and Stock Prices After the Financial Crisis: The Role of Strategic CSR Activities
- Original Paper
- Published: 15 September 2021
- Volume 182 , pages 223–242, ( 2023 )
Cite this article
- Aneta Havlinova 1 &
- Jiri Kukacka ORCID: orcid.org/0000-0001-8680-2896 1 , 2
4123 Accesses
16 Citations
Explore all metrics
We analyze the relationship between corporate social responsibility and the stock market performance in the post-global financial crisis period. A new measure of social responsibility by Thomson Reuters, called the ESG Combined Score, is used. As a novel feature of our analysis, socially responsible engagement is divided into the strategic activities closely related to the examined companies’ core business and the remaining secondary activities. The results of the fixed effects regression show a positive and statistically, as well as economically, significant impact of the strategic activities on the corporate stock market performance of companies. This impact is up to 103% higher compared to the secondary activities. The empirical results suggest that if companies aim to increase their share prices via the corporate social responsibility channel, they should strategically select their socially responsible initiatives.
This is a preview of subscription content, log in via an institution to check access.
Access this article
Price includes VAT (Russian Federation)
Instant access to the full article PDF.
Rent this article via DeepDyve
Institutional subscriptions
Similar content being viewed by others
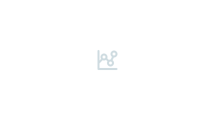
The impact of corporate governance on financial performance: a cross-sector study
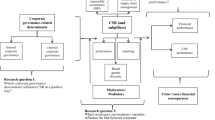
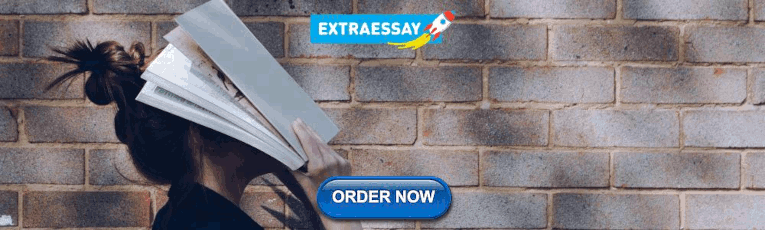
Meta-analyses on Corporate Social Responsibility (CSR): a literature review
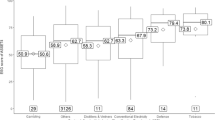
The Influence of Firm Size on the ESG Score: Corporate Sustainability Ratings Under Review
Data availability.
The data that support the findings of this study are available from the Refinitiv Eikon database (Thomson Reuters, 2021 , accessed 2021-01-28). Restrictions apply to the availability of these data, which were used under license for this study. Data are available at https://eikon.thomsonreuters.com with the permission of Thomson Reuters.
Please note that due to the logarithmic nature of the dependent variable, the precise interpretation of the estimated coefficient of the non-logaritmized independent variable follows: an increase of the independent variable by 1 (small) unit is associated with a change of the dependent variable by \(\big (exp({\widehat{\beta }})-1\big ) \times 100\) percent. This is often approximated by \({\widehat{\beta }} \times 100\) percent. However, such simplification holds well only for ‘very small’ values of \({\widehat{\beta }}\) .
Abbott, W. F., & Monsen, R. J. (1979). On the measurement of corporate social responsibility: self-reported disclosures as a method of measuring corporate social involvement. Acad. Manag. J., 22 (3), 501–515.
Article Google Scholar
Arellano, M. (1987). PRACTITIONERS’ CORNER: computing robust standard errors for within-groups estimators. Oxford Bull. Econ. Stat., 49 (4), 431–434.
Bansal, P., & Song, H.-C. (2017). Similar but not the same: differentiating corporate sustainability from corporate responsibility. Acad. Manag. Ann., 11 (1), 105–149.
Barnett, M. L., & Salomon, R. M. (2006). Beyond dichotomy: the curvilinear relationship between social responsibility and financial performance. Strategic Manag. J., 27 (11), 1101–1122.
Baron, D. P. (2001). Private politics, corporate social responsibility, and integrated strategy. J. Econ. Manag. Strategy, 10 (1), 7–45.
Barth, M. E., Beaver, W. H., & Landsman, W. R. (1992). The market valuation implications of net periodic pension cost components. J. Account. Econ., 15 (1), 27–62.
Bénabou, R., & Tirole, J. (2010). Individual and corporate social responsibility. Economica, 77 (305), 1–19.
Bowen, H. R. (1953). Social Responsibilities of the Businessman . New York: Harper & Row.
Google Scholar
Bowman, E. H., & Haire, M. (1975). A strategic posture toward corporate social responsibility. CA Manag. Rev., 18 (2), 49–58.
Brammer, S., & Millington, A. (2008). Does it pay to be different? An analysis of the relationship between corporate social and financial performance. Strategic Manag. J., 29 (12), 1325–1343.
Brown, S. J., Lajbcygier, P., & Li, B. (2008). Going negative: what to do with negative book equity stocks. J. Portfolio Manag., 35 (1), 95–102.
Burbano, V. C. (2016). Social responsibility messages and worker wage requirements: field experimental evidence from online labor marketplaces. Org. Sci., 27 (4), 1010–1028.
Carroll, A. B. (1981). Business and Society: Managing Corporate Social Performance . Boston: Little Brown and Company.
Chatterji, A. K., Durand, R., Levine, D. I., & Touboul, S. (2015). Do ratings of firms converge? Implications for managers, investors and strategy researchers. Strategic Manag. J., 37 (8), 1597–1614.
Chatterji, A. K., Levine, D. I., & Toffel, M. W. (2009). How well do social ratings actually measure corporate social responsibility? J. Econ. Manag. Strategy, 18 (1), 125–169.
Cheng, B., Ioannou, I., & Serafeim, G. (2014). Corporate social responsibility and access to finance. Strategic Manag. J., 35 (1), 1–23.
Clark, J. M. (1939). Social Control of Business . New York: McGraw-Hill.
Cochran, P. L., & Wood, R. A. (1984). Corporate social responsibility and financial performance. Acad. Manag. J., 27 (1), 42–56.
De Klerk, M., de Villiers, C., & van Staden, C. (2015). The influence of corporate social responsibility disclosure on share prices: evidence from the United Kingdom. Pac. Account. Rev., 27 (2), 208–228.
DesJardine, M., Bansal, P., & Yang, Y. (2019). Bouncing back: building resilience through social and environmental practices in the context of the 2008 global financial crisis. J. Manag., 45 (4), 1434–1460.
Durand, R., Paugam, L., & Stolowy, H. (2019). Do investors actually value sustainability indices? Replication, development, and new evidence on CSR visibility. Strategic Manag. J., 40 (9), 1471–1490.
Eccles, R. G., Ioannou, I., & Serafeim, G. (2014). The impact of corporate sustainability on organizational processes and performance. Manag. Sci., 60 (11), 2835–2857.
Fama, E. F., & French, K. R. (1993). Common risk factors in the returns on stocks and bonds. J. Financ. Econ., 33 (1), 3–56.
Flammer, C. (2015). Does corporate social responsibility lead to superior financial performance? A regression discontinuity approach. Manag. Sci., 61 (11), 2549–2568.
Flammer, C. (2018). Competing for government procurement contracts: the role of corporate social responsibility. Strategic Manag. J., 39 (5), 1299–1324.
Flammer, C., & Luo, J. (2017). Corporate social responsibility as an employee governance tool: evidence from a quasi-experiment. Strategic Manag. J., 38 (2), 163–183.
Freeman, R. E. (1984). Strategic Management: A Stakeholder Approach . Boston: Pitman.
Godfrey, P. C. (2005). The relationship between corporate philanthropy and shareholder wealth: a risk management perspective. Acad. Manag. Rev., 30 (4), 777–798.
Gond, J.-P., Palazzo, G., & Basu, K. (2009). Reconsidering instrumental corporate social responsibility through the Mafia metaphor. Business Ethics Q., 19 (1), 57–85.
Gregory, A., Tharyan, R., & Whittaker, J. (2014). Corporate social responsibility and firm value: disaggregating the effects on cash flow, risk and growth. J. Business Ethics, 124 (4), 633–657.
Griffin, J. M., & Lemmon, M. L. (2002). Book-to-market equity, distress risk, and stock returns. J. Finance, 57 (5), 2317–2336.
Hausman, J. A. (1978). Specification tests in econometrics. Econometrica, 46 (6), 1251–1271.
Hausman, J. A., & Taylor, W. E. (1981). Panel data and unobservable individual effects. Econometrica, 49 (6), 1377–1398.
Hawn, O., Chatterji, A. K., & Mitchell, W. (2018). Do investors actually value sustainability? New evidence from investor reactions to the Dow Jones sustainability index (DJSI). Strategic Manag. J., 39 (4), 949–976.
Hillman, A. J., & Keim, G. D. (2001). Shareholder value, stakeholder management, and social issues: what’s the bottom line? Strategic Manag. J., 22 (2), 125–139.
Hull, C. E., & Rothenberg, S. (2008). Firm performance: the interactions of corporate social performance with innovation and industry differentiation. Strategic Manag. J., 29 (7), 781–789.
Inoue, Y., & Lee, S. (2011). Effects of different dimensions of corporate social responsibility on corporate financial performance in tourism-related industries. Tour. Manag., 32 (4), 790–804.
Ioannou, I., & Serafeim, G. (2015). The impact of corporate social responsibility on investment recommendations: analysts’ perceptions and shifting institutional logics. Strategic Manag. J., 36 (7), 1053–1081.
Jan, C.-L., & Ou, J. A. (2012). Negative-book-value firms and their valuation. Account. Horizons, 26 (1), 91–110.
Khan, M., Serafeim, G., & Yoon, A. (2016). Corporate sustainability: first evidence on materiality. Account. Rev., 91 (6), 1697–1724.
KLD Research & Analytics, Inc., KLD database (2019). http://www.whartonwrds.com/datasets/kld/
Koh, P.-S., Reeb, D. M., & Zhao, W. (2018). CEO confidence and unreported R&D. Manag. Sci., 64 (12), 5725–5747.
Kramer, M. R., & Porter, M. (2011). Creating shared value. Harvard Business Rev., 89 (1/2), 62–77.
Kreps, T.J.: Measurement of the social performance of business. In: An Investigation of Concentration of Economic Power for the Temporary National Economic Committee, Monograph No. 7, Washington, DC: American Enterprise Institute for Public Policy Research (1940).
Luo, X., & Bhattacharya, C. (2006). Corporate social responsibility, customer satisfaction, and market value. J. Market., 70 (4), 1–18.
Lydenberg, S., Rogers, J., Wood, D.: From transparency to performance: industry-based sustainability reporting on key issues (2010). https://bit.ly/3ryITrp
Makni, R., Francoeur, C., & Bellavance, F. (2009). Causality between corporate social performance and financial performance: evidence from Canadian firms. J. Business Ethics, 89 (3), 409.
Refinitiv. (2020a) Refinitiv ESG Scores. https://www.refinitiv.com/en/sustainable-finance/esg-scores
Refinitiv. (2020b). Refinitiv ESG Scores (backup for 2020 methodology in .pdf). https://bit.ly/3hY2I8q
Sustainability Accounting Standards Board (2021), SASB Materiality Map. https://www.sasb.org/
Thomson Reuters (2017), Thomson Reuters ESG Scores 2017. https://bit.ly/2HAiBA2
Thomson Reuters (2021), Eikon database. https://eikon.thomsonreuters.com
McGuire, J. B., Sundgren, A., & Schneeweis, T. (1988). Corporate social responsibility and firm financial performance. Acad. Manag. J., 31 (4), 854–872.
McWilliams, A., & Siegel, D. (2000). Corporate social responsibility and financial performance: correlation or misspecification? Strategic Manag. J., 21 (5), 603–609.
Moneva, J. M., Rivera-Lirio, J. M., & Muñoz-Torres, M. J. (2007). The corporate stakeholder commitment and social and financial performance. Ind. Manag. Data Syst., 107 (1), 84–102.
Moskowitz, M. (1972). Choosing socially responsible stocks. Business Soc. Rev., 1 (1), 71–75.
Ohlson, J. A. (1995). Earnings, book values, and dividends in equity valuation. Contemp. Account. Res., 11 (2), 661–687.
Perrini, F., Russo, A., Tencati, A., & Vurro, C. (2011). Deconstructing the relationship between corporate social and financial performance. J. Business Ethics, 102 (1), 59–76.
Porter, M. E., & Kramer, M. R. (2006). The link between competitive advantage and corporate social responsibility. Harvard Business Rev., 84 (12), 78–92.
Qiu, Y., Shaukat, A., & Tharyan, R. (2016). Environmental and social disclosures: link with corporate financial performance. Br. Account. Rev., 48 (1), 102–116.
Revelli, C., & Viviani, J.-L. (2015). Financial performance of socially responsible investing (SRI): what have we learned? A meta-analysis. Business Ethics, 24 (2), 158–185.
Schadewitz, H., & Niskala, M. (2010). Communication via responsibility reporting and its effect on firm value in Finland. Corp. Soc. Resp. Environ. Manag., 17 (2), 96–106.
Scherer, A. G., & Palazzo, G. (2011). The new political role of business in a globalized world: a review of a new perspective on CSR and its implications for the firm, governance, and democracy. J. Manag. Stud., 48 (4), 899–931.
Seele, P., & Lock, I. (2015). Instrumental and/or deliberative? A typology of CSR communication tools. J. Business Ethics, 131 (2), 401–414.
Servaes, H., & Tamayo, A. (2013). The impact of corporate social responsibility on firm value: the role of customer awareness. Manag. Sci., 59 (5), 1045–1061.
Siegel, D. S., & Vitaliano, D. F. (2007). An empirical analysis of the strategic use of corporate social responsibility. J. Econ. Manag. Strategy, 16 (3), 773–792.
Spicer, B. H. (1978). Investors, corporate social performance and information disclosure: an empirical study. Account. Rev., 53 (1), 94–111.
Sturdivant, F. D., & Ginter, J. L. (1977). Corporate social responsiveness: management attitudes and economic performance. CA Manag. Rev., 19 (3), 30–39.
Tirole, J. (2001). Corporate governance. Econometrica, 69 (1), 1–35.
Van der Laan, G., Van Ees, H., & Van Witteloostuijn, A. (2008). Corporate social and financial performance: an extended stakeholder theory, and empirical test with accounting measures. J. Business Ethics, 79 (3), 299–310.
Vance, S. C. (1975). Are socially responsible corporations good investment risks. Manag. Rev., 64 (8), 19–24.
Vassalou, M., & Xing, Y. (2004). Default risk in equity returns. J. Finance, 59 (2), 831–868.
Waddock, S. A., & Graves, S. B. (1997a). The corporate social performance-financial performance link. Strategic Manag. J., 18 (4), 303–319.
Waddock, S. A., & Graves, S. B. (1997b). Quality of management and quality of stakeholder relations: are they synonymous? Business Soc., 36 (3), 250–279.
Zhao, X., & Murrell, A. J. (2016). Revisiting the corporate social performance-financial performance link: a replication of Waddock and Graves. Strategic Manag. J., 37 (11), 2378–2388.
Download references
Acknowledgements
Jiri Kukacka gratefully acknowledges financial support from the Charles University PRIMUS program [project PRIMUS/19/HUM/17] and from the Charles University UNCE program [project UNCE/HUM/035]. We are also indebted to I. Jupa and M. Walter for their professional consultation. Finally, we are very thankful to the two anonymous reviewers for their inspiring comments and detailed suggestions.
Author information
Authors and affiliations.
Charles University, Faculty of Social Sciences, Institute of Economic Studies, Opletalova 26, 110 00, Prague 1, Czechia
Aneta Havlinova & Jiri Kukacka
Czech Academy of Sciences, Institute of Information Theory and Automation, Pod Vodarenskou vezi 4, 182 00, Prague 8, Czechia
Jiri Kukacka
You can also search for this author in PubMed Google Scholar
Corresponding author
Correspondence to Jiri Kukacka .
Additional information
Publisher's note.
Springer Nature remains neutral with regard to jurisdictional claims in published maps and institutional affiliations.
Appendix A: Multicollinearity Assessment: Correlations
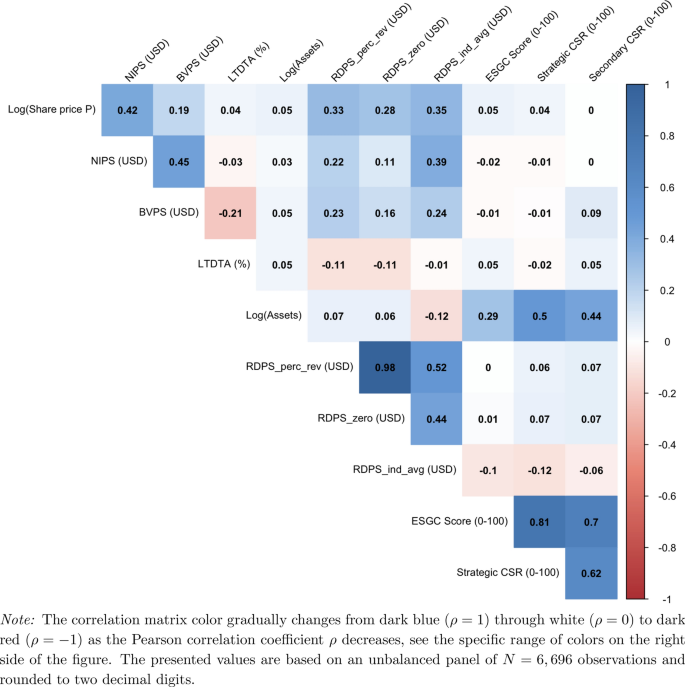
Descriptive statistics of the dataset: Correlations
Appendix B: Regression Results Con’t
Appendix c: regression results for the strategic and secondary csr con’t, rights and permissions.
Reprints and permissions
About this article
Havlinova, A., Kukacka, J. Corporate Social Responsibility and Stock Prices After the Financial Crisis: The Role of Strategic CSR Activities. J Bus Ethics 182 , 223–242 (2023). https://doi.org/10.1007/s10551-021-04935-9
Download citation
Received : 20 May 2020
Accepted : 26 August 2021
Published : 15 September 2021
Issue Date : January 2023
DOI : https://doi.org/10.1007/s10551-021-04935-9
Share this article
Anyone you share the following link with will be able to read this content:
Sorry, a shareable link is not currently available for this article.
Provided by the Springer Nature SharedIt content-sharing initiative
- Corporate social responsibility
- Strategic CSR
- Business ethics
- Corporate financial performance
- Fixed effects
JEL Classifications
- Find a journal
- Publish with us
- Track your research
- Open access
- Published: 28 August 2020
Short-term stock market price trend prediction using a comprehensive deep learning system
- Jingyi Shen 1 &
- M. Omair Shafiq ORCID: orcid.org/0000-0002-1859-8296 1
Journal of Big Data volume 7 , Article number: 66 ( 2020 ) Cite this article
270k Accesses
165 Citations
91 Altmetric
Metrics details
In the era of big data, deep learning for predicting stock market prices and trends has become even more popular than before. We collected 2 years of data from Chinese stock market and proposed a comprehensive customization of feature engineering and deep learning-based model for predicting price trend of stock markets. The proposed solution is comprehensive as it includes pre-processing of the stock market dataset, utilization of multiple feature engineering techniques, combined with a customized deep learning based system for stock market price trend prediction. We conducted comprehensive evaluations on frequently used machine learning models and conclude that our proposed solution outperforms due to the comprehensive feature engineering that we built. The system achieves overall high accuracy for stock market trend prediction. With the detailed design and evaluation of prediction term lengths, feature engineering, and data pre-processing methods, this work contributes to the stock analysis research community both in the financial and technical domains.
Introduction
Stock market is one of the major fields that investors are dedicated to, thus stock market price trend prediction is always a hot topic for researchers from both financial and technical domains. In this research, our objective is to build a state-of-art prediction model for price trend prediction, which focuses on short-term price trend prediction.
As concluded by Fama in [ 26 ], financial time series prediction is known to be a notoriously difficult task due to the generally accepted, semi-strong form of market efficiency and the high level of noise. Back in 2003, Wang et al. in [ 44 ] already applied artificial neural networks on stock market price prediction and focused on volume, as a specific feature of stock market. One of the key findings by them was that the volume was not found to be effective in improving the forecasting performance on the datasets they used, which was S&P 500 and DJI. Ince and Trafalis in [ 15 ] targeted short-term forecasting and applied support vector machine (SVM) model on the stock price prediction. Their main contribution is performing a comparison between multi-layer perceptron (MLP) and SVM then found that most of the scenarios SVM outperformed MLP, while the result was also affected by different trading strategies. In the meantime, researchers from financial domains were applying conventional statistical methods and signal processing techniques on analyzing stock market data.
The optimization techniques, such as principal component analysis (PCA) were also applied in short-term stock price prediction [ 22 ]. During the years, researchers are not only focused on stock price-related analysis but also tried to analyze stock market transactions such as volume burst risks, which expands the stock market analysis research domain broader and indicates this research domain still has high potential [ 39 ]. As the artificial intelligence techniques evolved in recent years, many proposed solutions attempted to combine machine learning and deep learning techniques based on previous approaches, and then proposed new metrics that serve as training features such as Liu and Wang [ 23 ]. This type of previous works belongs to the feature engineering domain and can be considered as the inspiration of feature extension ideas in our research. Liu et al. in [ 24 ] proposed a convolutional neural network (CNN) as well as a long short-term memory (LSTM) neural network based model to analyze different quantitative strategies in stock markets. The CNN serves for the stock selection strategy, automatically extracts features based on quantitative data, then follows an LSTM to preserve the time-series features for improving profits.
The latest work also proposes a similar hybrid neural network architecture, integrating a convolutional neural network with a bidirectional long short-term memory to predict the stock market index [ 4 ]. While the researchers frequently proposed different neural network solution architectures, it brought further discussions about the topic if the high cost of training such models is worth the result or not.
There are three key contributions of our work (1) a new dataset extracted and cleansed (2) a comprehensive feature engineering, and (3) a customized long short-term memory (LSTM) based deep learning model.
We have built the dataset by ourselves from the data source as an open-sourced data API called Tushare [ 43 ]. The novelty of our proposed solution is that we proposed a feature engineering along with a fine-tuned system instead of just an LSTM model only. We observe from the previous works and find the gaps and proposed a solution architecture with a comprehensive feature engineering procedure before training the prediction model. With the success of feature extension method collaborating with recursive feature elimination algorithms, it opens doors for many other machine learning algorithms to achieve high accuracy scores for short-term price trend prediction. It proved the effectiveness of our proposed feature extension as feature engineering. We further introduced our customized LSTM model and further improved the prediction scores in all the evaluation metrics. The proposed solution outperformed the machine learning and deep learning-based models in similar previous works.
The remainder of this paper is organized as follows. “ Survey of related works ” section describes the survey of related works. “ The dataset ” section provides details on the data that we extracted from the public data sources and the dataset prepared. “ Methods ” section presents the research problems, methods, and design of the proposed solution. Detailed technical design with algorithms and how the model implemented are also included in this section. “ Results ” section presents comprehensive results and evaluation of our proposed model, and by comparing it with the models used in most of the related works. “ Discussion ” section provides a discussion and comparison of the results. “ Conclusion ” section presents the conclusion. This research paper has been built based on Shen [ 36 ].
Survey of related works
In this section, we discuss related works. We reviewed the related work in two different domains: technical and financial, respectively.
Kim and Han in [ 19 ] built a model as a combination of artificial neural networks (ANN) and genetic algorithms (GAs) with discretization of features for predicting stock price index. The data used in their study include the technical indicators as well as the direction of change in the daily Korea stock price index (KOSPI). They used the data containing samples of 2928 trading days, ranging from January 1989 to December 1998, and give their selected features and formulas. They also applied optimization of feature discretization, as a technique that is similar to dimensionality reduction. The strengths of their work are that they introduced GA to optimize the ANN. First, the amount of input features and processing elements in the hidden layer are 12 and not adjustable. Another limitation is in the learning process of ANN, and the authors only focused on two factors in optimization. While they still believed that GA has great potential for feature discretization optimization. Our initialized feature pool refers to the selected features. Qiu and Song in [ 34 ] also presented a solution to predict the direction of the Japanese stock market based on an optimized artificial neural network model. In this work, authors utilize genetic algorithms together with artificial neural network based models, and name it as a hybrid GA-ANN model.
Piramuthu in [ 33 ] conducted a thorough evaluation of different feature selection methods for data mining applications. He used for datasets, which were credit approval data, loan defaults data, web traffic data, tam, and kiang data, and compared how different feature selection methods optimized decision tree performance. The feature selection methods he compared included probabilistic distance measure: the Bhattacharyya measure, the Matusita measure, the divergence measure, the Mahalanobis distance measure, and the Patrick-Fisher measure. For inter-class distance measures: the Minkowski distance measure, city block distance measure, Euclidean distance measure, the Chebychev distance measure, and the nonlinear (Parzen and hyper-spherical kernel) distance measure. The strength of this paper is that the author evaluated both probabilistic distance-based and several inter-class feature selection methods. Besides, the author performed the evaluation based on different datasets, which reinforced the strength of this paper. However, the evaluation algorithm was a decision tree only. We cannot conclude if the feature selection methods will still perform the same on a larger dataset or a more complex model.
Hassan and Nath in [ 9 ] applied the Hidden Markov Model (HMM) on the stock market forecasting on stock prices of four different Airlines. They reduce states of the model into four states: the opening price, closing price, the highest price, and the lowest price. The strong point of this paper is that the approach does not need expert knowledge to build a prediction model. While this work is limited within the industry of Airlines and evaluated on a very small dataset, it may not lead to a prediction model with generality. One of the approaches in stock market prediction related works could be exploited to do the comparison work. The authors selected a maximum 2 years as the date range of training and testing dataset, which provided us a date range reference for our evaluation part.
Lei in [ 21 ] exploited Wavelet Neural Network (WNN) to predict stock price trends. The author also applied Rough Set (RS) for attribute reduction as an optimization. Rough Set was utilized to reduce the stock price trend feature dimensions. It was also used to determine the structure of the Wavelet Neural Network. The dataset of this work consists of five well-known stock market indices, i.e., (1) SSE Composite Index (China), (2) CSI 300 Index (China), (3) All Ordinaries Index (Australian), (4) Nikkei 225 Index (Japan), and (5) Dow Jones Index (USA). Evaluation of the model was based on different stock market indices, and the result was convincing with generality. By using Rough Set for optimizing the feature dimension before processing reduces the computational complexity. However, the author only stressed the parameter adjustment in the discussion part but did not specify the weakness of the model itself. Meanwhile, we also found that the evaluations were performed on indices, the same model may not have the same performance if applied on a specific stock.
Lee in [ 20 ] used the support vector machine (SVM) along with a hybrid feature selection method to carry out prediction of stock trends. The dataset in this research is a sub dataset of NASDAQ Index in Taiwan Economic Journal Database (TEJD) in 2008. The feature selection part was using a hybrid method, supported sequential forward search (SSFS) played the role of the wrapper. Another advantage of this work is that they designed a detailed procedure of parameter adjustment with performance under different parameter values. The clear structure of the feature selection model is also heuristic to the primary stage of model structuring. One of the limitations was that the performance of SVM was compared to back-propagation neural network (BPNN) only and did not compare to the other machine learning algorithms.
Sirignano and Cont leveraged a deep learning solution trained on a universal feature set of financial markets in [ 40 ]. The dataset used included buy and sell records of all transactions, and cancellations of orders for approximately 1000 NASDAQ stocks through the order book of the stock exchange. The NN consists of three layers with LSTM units and a feed-forward layer with rectified linear units (ReLUs) at last, with stochastic gradient descent (SGD) algorithm as an optimization. Their universal model was able to generalize and cover the stocks other than the ones in the training data. Though they mentioned the advantages of a universal model, the training cost was still expensive. Meanwhile, due to the inexplicit programming of the deep learning algorithm, it is unclear that if there are useless features contaminated when feeding the data into the model. Authors found out that it would have been better if they performed feature selection part before training the model and found it as an effective way to reduce the computational complexity.
Ni et al. in [ 30 ] predicted stock price trends by exploiting SVM and performed fractal feature selection for optimization. The dataset they used is the Shanghai Stock Exchange Composite Index (SSECI), with 19 technical indicators as features. Before processing the data, they optimized the input data by performing feature selection. When finding the best parameter combination, they also used a grid search method, which is k cross-validation. Besides, the evaluation of different feature selection methods is also comprehensive. As the authors mentioned in their conclusion part, they only considered the technical indicators but not macro and micro factors in the financial domain. The source of datasets that the authors used was similar to our dataset, which makes their evaluation results useful to our research. They also mentioned a method called k cross-validation when testing hyper-parameter combinations.
McNally et al. in [ 27 ] leveraged RNN and LSTM on predicting the price of Bitcoin, optimized by using the Boruta algorithm for feature engineering part, and it works similarly to the random forest classifier. Besides feature selection, they also used Bayesian optimization to select LSTM parameters. The Bitcoin dataset ranged from the 19th of August 2013 to 19th of July 2016. Used multiple optimization methods to improve the performance of deep learning methods. The primary problem of their work is overfitting. The research problem of predicting Bitcoin price trend has some similarities with stock market price prediction. Hidden features and noises embedded in the price data are threats of this work. The authors treated the research question as a time sequence problem. The best part of this paper is the feature engineering and optimization part; we could replicate the methods they exploited in our data pre-processing.
Weng et al. in [ 45 ] focused on short-term stock price prediction by using ensemble methods of four well-known machine learning models. The dataset for this research is five sets of data. They obtained these datasets from three open-sourced APIs and an R package named TTR. The machine learning models they used are (1) neural network regression ensemble (NNRE), (2) a Random Forest with unpruned regression trees as base learners (RFR), (3) AdaBoost with unpruned regression trees as base learners (BRT) and (4) a support vector regression ensemble (SVRE). A thorough study of ensemble methods specified for short-term stock price prediction. With background knowledge, the authors selected eight technical indicators in this study then performed a thoughtful evaluation of five datasets. The primary contribution of this paper is that they developed a platform for investors using R, which does not need users to input their own data but call API to fetch the data from online source straightforward. From the research perspective, they only evaluated the prediction of the price for 1 up to 10 days ahead but did not evaluate longer terms than two trading weeks or a shorter term than 1 day. The primary limitation of their research was that they only analyzed 20 U.S.-based stocks, the model might not be generalized to other stock market or need further revalidation to see if it suffered from overfitting problems.
Kara et al. in [ 17 ] also exploited ANN and SVM in predicting the movement of stock price index. The data set they used covers a time period from January 2, 1997, to December 31, 2007, of the Istanbul Stock Exchange. The primary strength of this work is its detailed record of parameter adjustment procedures. While the weaknesses of this work are that neither the technical indicator nor the model structure has novelty, and the authors did not explain how their model performed better than other models in previous works. Thus, more validation works on other datasets would help. They explained how ANN and SVM work with stock market features, also recorded the parameter adjustment. The implementation part of our research could benefit from this previous work.
Jeon et al. in [ 16 ] performed research on millisecond interval-based big dataset by using pattern graph tracking to complete stock price prediction tasks. The dataset they used is a millisecond interval-based big dataset of historical stock data from KOSCOM, from August 2014 to October 2014, 10G–15G capacity. The author applied Euclidean distance, Dynamic Time Warping (DTW) for pattern recognition. For feature selection, they used stepwise regression. The authors completed the prediction task by ANN and Hadoop and RHive for big data processing. The “ Results ” section is based on the result processed by a combination of SAX and Jaro–Winkler distance. Before processing the data, they generated aggregated data at 5-min intervals from discrete data. The primary strength of this work is the explicit structure of the whole implementation procedure. While they exploited a relatively old model, another weakness is the overall time span of the training dataset is extremely short. It is difficult to access the millisecond interval-based data in real life, so the model is not as practical as a daily based data model.
Huang et al. in [ 12 ] applied a fuzzy-GA model to complete the stock selection task. They used the key stocks of the 200 largest market capitalization listed as the investment universe in the Taiwan Stock Exchange. Besides, the yearly financial statement data and the stock returns were taken from the Taiwan Economic Journal (TEJ) database at www.tej.com.tw/ for the time period from year 1995 to year 2009. They conducted the fuzzy membership function with model parameters optimized with GA and extracted features for optimizing stock scoring. The authors proposed an optimized model for selection and scoring of stocks. Different from the prediction model, the authors more focused on stock rankings, selection, and performance evaluation. Their structure is more practical among investors. But in the model validation part, they did not compare the model with existed algorithms but the statistics of the benchmark, which made it challenging to identify if GA would outperform other algorithms.
Fischer and Krauss in [ 5 ] applied long short-term memory (LSTM) on financial market prediction. The dataset they used is S&P 500 index constituents from Thomson Reuters. They obtained all month-end constituent lists for the S&P 500 from Dec 1989 to Sep 2015, then consolidated the lists into a binary matrix to eliminate survivor bias. The authors also used RMSprop as an optimizer, which is a mini-batch version of rprop. The primary strength of this work is that the authors used the latest deep learning technique to perform predictions. They relied on the LSTM technique, lack of background knowledge in the financial domain. Although the LSTM outperformed the standard DNN and logistic regression algorithms, while the author did not mention the effort to train an LSTM with long-time dependencies.
Tsai and Hsiao in [ 42 ] proposed a solution as a combination of different feature selection methods for prediction of stocks. They used Taiwan Economic Journal (TEJ) database as data source. The data used in their analysis was from year 2000 to 2007. In their work, they used a sliding window method and combined it with multi layer perceptron (MLP) based artificial neural networks with back propagation, as their prediction model. In their work, they also applied principal component analysis (PCA) for dimensionality reduction, genetic algorithms (GA) and the classification and regression trees (CART) to select important features. They did not just rely on technical indices only. Instead, they also included both fundamental and macroeconomic indices in their analysis. The authors also reported a comparison on feature selection methods. The validation part was done by combining the model performance stats with statistical analysis.
Pimenta et al. in [ 32 ] leveraged an automated investing method by using multi-objective genetic programming and applied it in the stock market. The dataset was obtained from Brazilian stock exchange market (BOVESPA), and the primary techniques they exploited were a combination of multi-objective optimization, genetic programming, and technical trading rules. For optimization, they leveraged genetic programming (GP) to optimize decision rules. The novelty of this paper was in the evaluation part. They included a historical period, which was a critical moment of Brazilian politics and economics when performing validation. This approach reinforced the generalization strength of their proposed model. When selecting the sub-dataset for evaluation, they also set criteria to ensure more asset liquidity. While the baseline of the comparison was too basic and fundamental, and the authors did not perform any comparison with other existing models.
Huang and Tsai in [ 13 ] conducted a filter-based feature selection assembled with a hybrid self-organizing feature map (SOFM) support vector regression (SVR) model to forecast Taiwan index futures (FITX) trend. They divided the training samples into clusters to marginally improve the training efficiency. The authors proposed a comprehensive model, which was a combination of two novel machine learning techniques in stock market analysis. Besides, the optimizer of feature selection was also applied before the data processing to improve the prediction accuracy and reduce the computational complexity of processing daily stock index data. Though they optimized the feature selection part and split the sample data into small clusters, it was already strenuous to train daily stock index data of this model. It would be difficult for this model to predict trading activities in shorter time intervals since the data volume would be increased drastically. Moreover, the evaluation is not strong enough since they set a single SVR model as a baseline, but did not compare the performance with other previous works, which caused difficulty for future researchers to identify the advantages of SOFM-SVR model why it outperforms other algorithms.
Thakur and Kumar in [ 41 ] also developed a hybrid financial trading support system by exploiting multi-category classifiers and random forest (RAF). They conducted their research on stock indices from NASDAQ, DOW JONES, S&P 500, NIFTY 50, and NIFTY BANK. The authors proposed a hybrid model combined random forest (RF) algorithms with a weighted multicategory generalized eigenvalue support vector machine (WMGEPSVM) to generate “Buy/Hold/Sell” signals. Before processing the data, they used Random Forest (RF) for feature pruning. The authors proposed a practical model designed for real-life investment activities, which could generate three basic signals for investors to refer to. They also performed a thorough comparison of related algorithms. While they did not mention the time and computational complexity of their works. Meanwhile, the unignorable issue of their work was the lack of financial domain knowledge background. The investors regard the indices data as one of the attributes but could not take the signal from indices to operate a specific stock straightforward.
Hsu in [ 11 ] assembled feature selection with a back propagation neural network (BNN) combined with genetic programming to predict the stock/futures price. The dataset in this research was obtained from Taiwan Stock Exchange Corporation (TWSE). The authors have introduced the description of the background knowledge in detail. While the weakness of their work is that it is a lack of data set description. This is a combination of the model proposed by other previous works. Though we did not see the novelty of this work, we can still conclude that the genetic programming (GP) algorithm is admitted in stock market research domain. To reinforce the validation strengths, it would be good to consider adding GP models into evaluation if the model is predicting a specific price.
Hafezi et al. in [ 7 ] built a bat-neural network multi-agent system (BN-NMAS) to predict stock price. The dataset was obtained from the Deutsche bundes-bank. They also applied the Bat algorithm (BA) for optimizing neural network weights. The authors illustrated their overall structure and logic of system design in clear flowcharts. While there were very few previous works that had performed on DAX data, it would be difficult to recognize if the model they proposed still has the generality if migrated on other datasets. The system design and feature selection logic are fascinating, which worth referring to. Their findings in optimization algorithms are also valuable for the research in the stock market price prediction research domain. It is worth trying the Bat algorithm (BA) when constructing neural network models.
Long et al. in [ 25 ] conducted a deep learning approach to predict the stock price movement. The dataset they used is the Chinese stock market index CSI 300. For predicting the stock price movement, they constructed a multi-filter neural network (MFNN) with stochastic gradient descent (SGD) and back propagation optimizer for learning NN parameters. The strength of this paper is that the authors exploited a novel model with a hybrid model constructed by different kinds of neural networks, it provides an inspiration for constructing hybrid neural network structures.
Atsalakis and Valavanis in [ 1 ] proposed a solution of a neuro-fuzzy system, which is composed of controller named as Adaptive Neuro Fuzzy Inference System (ANFIS), to achieve short-term stock price trend prediction. The noticeable strength of this work is the evaluation part. Not only did they compare their proposed system with the popular data models, but also compared with investment strategies. While the weakness that we found from their proposed solution is that their solution architecture is lack of optimization part, which might limit their model performance. Since our proposed solution is also focusing on short-term stock price trend prediction, this work is heuristic for our system design. Meanwhile, by comparing with the popular trading strategies from investors, their work inspired us to compare the strategies used by investors with techniques used by researchers.
Nekoeiqachkanloo et al. in [ 29 ] proposed a system with two different approaches for stock investment. The strengths of their proposed solution are obvious. First, it is a comprehensive system that consists of data pre-processing and two different algorithms to suggest the best investment portions. Second, the system also embedded with a forecasting component, which also retains the features of the time series. Last but not least, their input features are a mix of fundamental features and technical indices that aim to fill in the gap between the financial domain and technical domain. However, their work has a weakness in the evaluation part. Instead of evaluating the proposed system on a large dataset, they chose 25 well-known stocks. There is a high possibility that the well-known stocks might potentially share some common hidden features.
As another related latest work, Idrees et al. [ 14 ] published a time series-based prediction approach for the volatility of the stock market. ARIMA is not a new approach in the time series prediction research domain. Their work is more focusing on the feature engineering side. Before feeding the features into ARIMA models, they designed three steps for feature engineering: Analyze the time series, identify if the time series is stationary or not, perform estimation by plot ACF and PACF charts and look for parameters. The only weakness of their proposed solution is that the authors did not perform any customization on the existing ARIMA model, which might limit the system performance to be improved.
One of the main weaknesses found in the related works is limited data-preprocessing mechanisms built and used. Technical works mostly tend to focus on building prediction models. When they select the features, they list all the features mentioned in previous works and go through the feature selection algorithm then select the best-voted features. Related works in the investment domain have shown more interest in behavior analysis, such as how herding behaviors affect the stock performance, or how the percentage of inside directors hold the firm’s common stock affects the performance of a certain stock. These behaviors often need a pre-processing procedure of standard technical indices and investment experience to recognize.
In the related works, often a thorough statistical analysis is performed based on a special dataset and conclude new features rather than performing feature selections. Some data, such as the percentage of a certain index fluctuation has been proven to be effective on stock performance. We believe that by extracting new features from data, then combining such features with existed common technical indices will significantly benefit the existing and well-tested prediction models.
The dataset
This section details the data that was extracted from the public data sources, and the final dataset that was prepared. Stock market-related data are diverse, so we first compared the related works from the survey of financial research works in stock market data analysis to specify the data collection directions. After collecting the data, we defined a data structure of the dataset. Given below, we describe the dataset in detail, including the data structure, and data tables in each category of data with the segment definitions.
Description of our dataset
In this section, we will describe the dataset in detail. This dataset consists of 3558 stocks from the Chinese stock market. Besides the daily price data, daily fundamental data of each stock ID, we also collected the suspending and resuming history, top 10 shareholders, etc. We list two reasons that we choose 2 years as the time span of this dataset: (1) most of the investors perform stock market price trend analysis using the data within the latest 2 years, (2) using more recent data would benefit the analysis result. We collected data through the open-sourced API, namely Tushare [ 43 ], mean-while we also leveraged a web-scraping technique to collect data from Sina Finance web pages, SWS Research website.
Data structure
Figure 1 illustrates all the data tables in the dataset. We collected four categories of data in this dataset: (1) basic data, (2) trading data, (3) finance data, and (4) other reference data. All the data tables can be linked to each other by a common field called “Stock ID” It is a unique stock identifier registered in the Chinese Stock market. Table 1 shows an overview of the dataset.
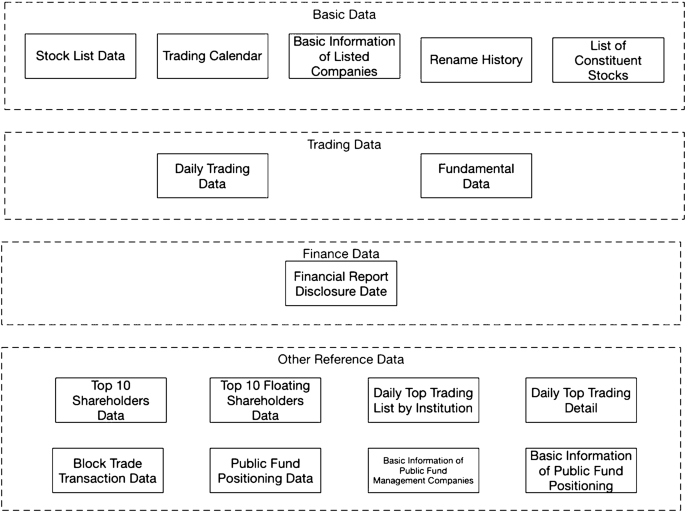
Data structure for the extracted dataset
The Table 1 lists the field information of each data table as well as which category the data table belongs to.
In this section, we present the proposed methods and the design of the proposed solution. Moreover, we also introduce the architecture design as well as algorithmic and implementation details.
Problem statement
We analyzed the best possible approach for predicting short-term price trends from different aspects: feature engineering, financial domain knowledge, and prediction algorithm. Then we addressed three research questions in each aspect, respectively: How can feature engineering benefit model prediction accuracy? How do findings from the financial domain benefit prediction model design? And what is the best algorithm for predicting short-term price trends?
The first research question is about feature engineering. We would like to know how the feature selection method benefits the performance of prediction models. From the abundance of the previous works, we can conclude that stock price data embedded with a high level of noise, and there are also correlations between features, which makes the price prediction notoriously difficult. That is also the primary reason for most of the previous works introduced the feature engineering part as an optimization module.
The second research question is evaluating the effectiveness of findings we extracted from the financial domain. Different from the previous works, besides the common evaluation of data models such as the training costs and scores, our evaluation will emphasize the effectiveness of newly added features that we extracted from the financial domain. We introduce some features from the financial domain. While we only obtained some specific findings from previous works, and the related raw data needs to be processed into usable features. After extracting related features from the financial domain, we combine the features with other common technical indices for voting out the features with a higher impact. There are numerous features said to be effective from the financial domain, and it would be impossible for us to cover all of them. Thus, how to appropriately convert the findings from the financial domain to a data processing module of our system design is a hidden research question that we attempt to answer.
The third research question is that which algorithms are we going to model our data? From the previous works, researchers have been putting efforts into the exact price prediction. We decompose the problem into predicting the trend and then the exact number. This paper focuses on the first step. Hence, the objective has been converted to resolve a binary classification problem, meanwhile, finding an effective way to eliminate the negative effect brought by the high level of noise. Our approach is to decompose the complex problem into sub-problems which have fewer dependencies and resolve them one by one, and then compile the resolutions into an ensemble model as an aiding system for investing behavior reference.
In the previous works, researchers have been using a variety of models for predicting stock price trends. While most of the best-performed models are based on machine learning techniques, in this work, we will compare our approach with the outperformed machine learning models in the evaluation part and find the solution for this research question.
Proposed solution
The high-level architecture of our proposed solution could be separated into three parts. First is the feature selection part, to guarantee the selected features are highly effective. Second, we look into the data and perform the dimensionality reduction. And the last part, which is the main contribution of our work is to build a prediction model of target stocks. Figure 2 depicts a high-level architecture of the proposed solution.
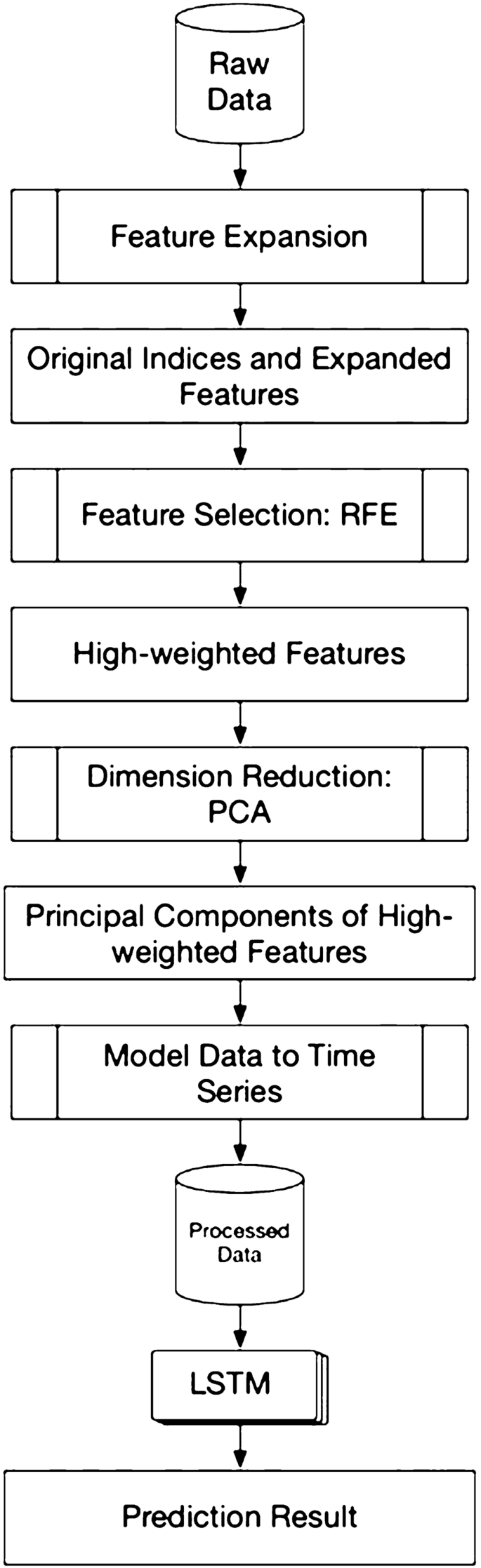
High-level architecture of the proposed solution
There are ways to classify different categories of stocks. Some investors prefer long-term investments, while others show more interest in short-term investments. It is common to see the stock-related reports showing an average performance, while the stock price is increasing drastically; this is one of the phenomena that indicate the stock price prediction has no fixed rules, thus finding effective features before training a model on data is necessary.
In this research, we focus on the short-term price trend prediction. Currently, we only have the raw data with no labels. So, the very first step is to label the data. We mark the price trend by comparing the current closing price with the closing price of n trading days ago, the range of n is from 1 to 10 since our research is focusing on the short-term. If the price trend goes up, we mark it as 1 or mark as 0 in the opposite case. To be more specified, we use the indices from the indices of n − 1 th day to predict the price trend of the n th day.
According to the previous works, some researchers who applied both financial domain knowledge and technical methods on stock data were using rules to filter the high-quality stocks. We referred to their works and exploited their rules to contribute to our feature extension design.
However, to ensure the best performance of the prediction model, we will look into the data first. There are a large number of features in the raw data; if we involve all the features into our consideration, it will not only drastically increase the computational complexity but will also cause side effects if we would like to perform unsupervised learning in further research. So, we leverage the recursive feature elimination (RFE) to ensure all the selected features are effective.
We found most of the previous works in the technical domain were analyzing all the stocks, while in the financial domain, researchers prefer to analyze the specific scenario of investment, to fill the gap between the two domains, we decide to apply a feature extension based on the findings we gathered from the financial domain before we start the RFE procedure.
Since we plan to model the data into time series, the number of the features, the more complex the training procedure will be. So, we will leverage the dimensionality reduction by using randomized PCA at the beginning of our proposed solution architecture.
Detailed technical design elaboration
This section provides an elaboration of the detailed technical design as being a comprehensive solution based on utilizing, combining, and customizing several existing data preprocessing, feature engineering, and deep learning techniques. Figure 3 provides the detailed technical design from data processing to prediction, including the data exploration. We split the content by main procedures, and each procedure contains algorithmic steps. Algorithmic details are elaborated in the next section. The contents of this section will focus on illustrating the data workflow.
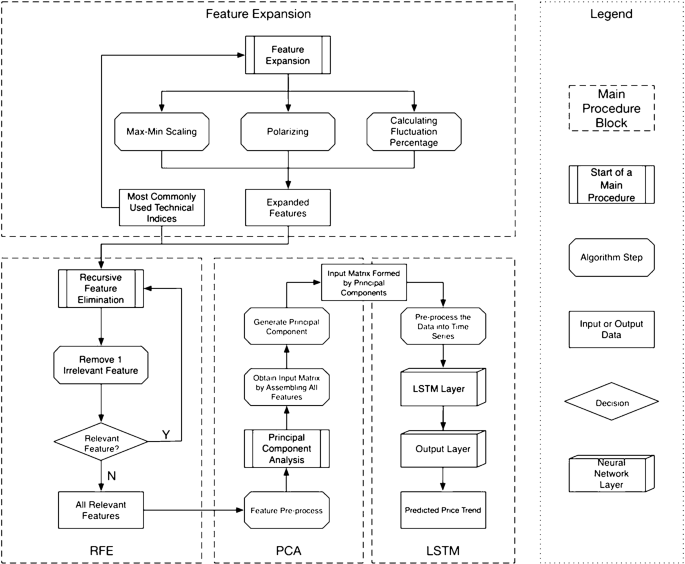
Detailed technical design of the proposed solution
Based on the literature review, we select the most commonly used technical indices and then feed them into the feature extension procedure to get the expanded feature set. We will select the most effective i features from the expanded feature set. Then we will feed the data with i selected features into the PCA algorithm to reduce the dimension into j features. After we get the best combination of i and j , we process the data into finalized the feature set and feed them into the LSTM [ 10 ] model to get the price trend prediction result.
The novelty of our proposed solution is that we will not only apply the technical method on raw data but also carry out the feature extensions that are used among stock market investors. Details on feature extension are given in the next subsection. Experiences gained from applying and optimizing deep learning based solutions in [ 37 , 38 ] were taken into account while designing and customizing feature engineering and deep learning solution in this work.
Applying feature extension
The first main procedure in Fig. 3 is the feature extension. In this block, the input data is the most commonly used technical indices concluded from related works. The three feature extension methods are max–min scaling, polarizing, and calculating fluctuation percentage. Not all the technical indices are applicable for all three of the feature extension methods; this procedure only applies the meaningful extension methods on technical indices. We choose meaningful extension methods while looking at how the indices are calculated. The technical indices and the corresponding feature extension methods are illustrated in Table 2 .
After the feature extension procedure, the expanded features will be combined with the most commonly used technical indices, i.e., input data with output data, and feed into RFE block as input data in the next step.
Applying recursive feature elimination
After the feature extension above, we explore the most effective i features by using the Recursive Feature Elimination (RFE) algorithm [ 6 ]. We estimate all the features by two attributes, coefficient, and feature importance. We also limit the features that remove from the pool by one, which means we will remove one feature at each step and retain all the relevant features. Then the output of the RFE block will be the input of the next step, which refers to PCA.
Applying principal component analysis (PCA)
The very first step before leveraging PCA is feature pre-processing. Because some of the features after RFE are percentage data, while others are very large numbers, i.e., the output from RFE are in different units. It will affect the principal component extraction result. Thus, before feeding the data into the PCA algorithm [ 8 ], a feature pre-processing is necessary. We also illustrate the effectiveness and methods comparison in “ Results ” section.
After performing feature pre-processing, the next step is to feed the processed data with selected i features into the PCA algorithm to reduce the feature matrix scale into j features. This step is to retain as many effective features as possible and meanwhile eliminate the computational complexity of training the model. This research work also evaluates the best combination of i and j, which has relatively better prediction accuracy, meanwhile, cuts the computational consumption. The result can be found in the “ Results ” section, as well. After the PCA step, the system will get a reshaped matrix with j columns.
Fitting long short-term memory (LSTM) model
PCA reduced the dimensions of the input data, while the data pre-processing is mandatory before feeding the data into the LSTM layer. The reason for adding the data pre-processing step before the LSTM model is that the input matrix formed by principal components has no time steps. While one of the most important parameters of training an LSTM is the number of time steps. Hence, we have to model the matrix into corresponding time steps for both training and testing dataset.
After performing the data pre-processing part, the last step is to feed the training data into LSTM and evaluate the performance using testing data. As a variant neural network of RNN, even with one LSTM layer, the NN structure is still a deep neural network since it can process sequential data and memorizes its hidden states through time. An LSTM layer is composed of one or more LSTM units, and an LSTM unit consists of cells and gates to perform classification and prediction based on time series data.
The LSTM structure is formed by two layers. The input dimension is determined by j after the PCA algorithm. The first layer is the input LSTM layer, and the second layer is the output layer. The final output will be 0 or 1 indicates if the stock price trend prediction result is going down or going up, as a supporting suggestion for the investors to perform the next investment decision.
Design discussion
Feature extension is one of the novelties of our proposed price trend predicting system. In the feature extension procedure, we use technical indices to collaborate with the heuristic processing methods learned from investors, which fills the gap between the financial research area and technical research area.
Since we proposed a system of price trend prediction, feature engineering is extremely important to the final prediction result. Not only the feature extension method is helpful to guarantee we do not miss the potentially correlated feature, but also feature selection method is necessary for pooling the effective features. The more irrelevant features are fed into the model, the more noise would be introduced. Each main procedure is carefully considered contributing to the whole system design.
Besides the feature engineering part, we also leverage LSTM, the state-of-the-art deep learning method for time-series prediction, which guarantees the prediction model can capture both complex hidden pattern and the time-series related pattern.
It is known that the training cost of deep learning models is expansive in both time and hardware aspects; another advantage of our system design is the optimization procedure—PCA. It can retain the principal components of the features while reducing the scale of the feature matrix, thus help the system to save the training cost of processing the large time-series feature matrix.
Algorithm elaboration
This section provides comprehensive details on the algorithms we built while utilizing and customizing different existing techniques. Details about the terminologies, parameters, as well as optimizers. From the legend on the right side of Fig. 3 , we note the algorithm steps as octagons, all of them can be found in this “ Algorithm elaboration ” section.
Before dive deep into the algorithm steps, here is the brief introduction of data pre-processing: since we will go through the supervised learning algorithms, we also need to program the ground truth. The ground truth of this research is programmed by comparing the closing price of the current trading date with the closing price of the previous trading date the users want to compare with. Label the price increase as 1, else the ground truth will be labeled as 0. Because this research work is not only focused on predicting the price trend of a specific period of time but short-term in general, the ground truth processing is according to a range of trading days. While the algorithms will not change with the prediction term length, we can regard the term length as a parameter.
The algorithmic detail is elaborated, respectively, the first algorithm is the hybrid feature engineering part for preparing high-quality training and testing data. It corresponds to the Feature extension, RFE, and PCA blocks in Fig. 3 . The second algorithm is the LSTM procedure block, including time-series data pre-processing, NN constructing, training, and testing.
Algorithm 1: Short-term stock market price trend prediction—applying feature engineering using FE + RFE + PCA
The function FE is corresponding to the feature extension block. For the feature extension procedure, we apply three different processing methods to translate the findings from the financial domain to a technical module in our system design. While not all the indices are applicable for expanding, we only choose the proper method(s) for certain features to perform the feature extension (FE), according to Table 2 .
Normalize method preserves the relative frequencies of the terms, and transform the technical indices into the range of [0, 1]. Polarize is a well-known method often used by real-world investors, sometimes they prefer to consider if the technical index value is above or below zero, we program some of the features using polarize method and prepare for RFE. Max-min (or min-max) [ 35 ] scaling is a transformation method often used as an alternative to zero mean and unit variance scaling. Another well-known method used is fluctuation percentage, and we transform the technical indices fluctuation percentage into the range of [− 1, 1].
The function RFE () in the first algorithm refers to recursive feature elimination. Before we perform the training data scale reduction, we will have to make sure that the features we selected are effective. Ineffective features will not only drag down the classification precision but also add more computational complexity. For the feature selection part, we choose recursive feature elimination (RFE). As [ 45 ] explained, the process of recursive feature elimination can be split into the ranking algorithm, resampling, and external validation.
For the ranking algorithm, it fits the model to the features and ranks by the importance to the model. We set the parameter to retain i numbers of features, and at each iteration of feature selection retains Si top-ranked features, then refit the model and assess the performance again to begin another iteration. The ranking algorithm will eventually determine the top Si features.
The RFE algorithm is known to have suffered from the over-fitting problem. To eliminate the over-fitting issue, we will run the RFE algorithm multiple times on randomly selected stocks as the training set and ensure all the features we select are high-weighted. This procedure is called data resampling. Resampling can be built as an optimization step as an outer layer of the RFE algorithm.
The last part of our hybrid feature engineering algorithm is for optimization purposes. For the training data matrix scale reduction, we apply Randomized principal component analysis (PCA) [ 31 ], before we decide the features of the classification model.
Financial ratios of a listed company are used to present the growth ability, earning ability, solvency ability, etc. Each financial ratio consists of a set of technical indices, each time we add a technical index (or feature) will add another column of data into the data matrix and will result in low training efficiency and redundancy. If non-relevant or less relevant features are included in training data, it will also decrease the precision of classification.
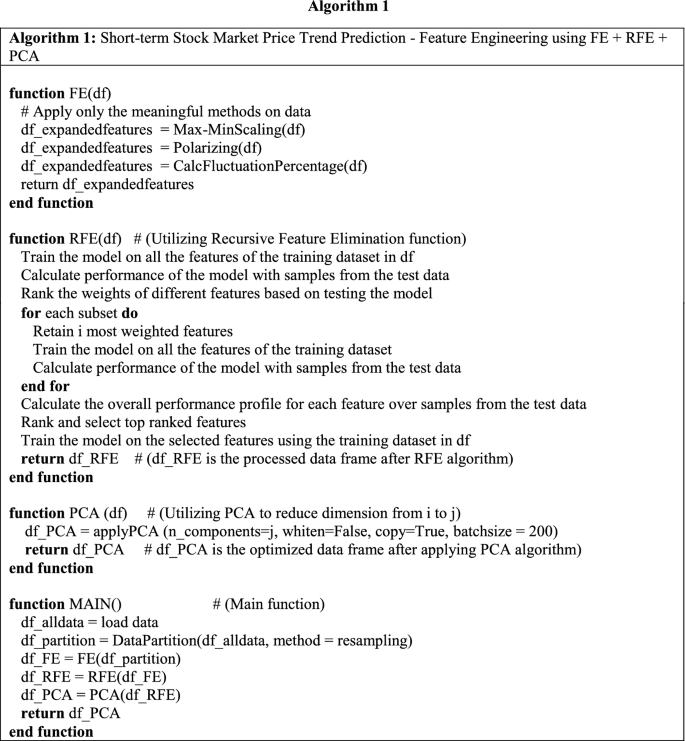
The above equation represents the explanation power of principal components extracted by PCA method for original data. If an ACR is below 85%, the PCA method would be unsuitable due to a loss of original information. Because the covariance matrix is sensitive to the order of magnitudes of data, there should be a data standardize procedure before performing the PCA. The commonly used standardized methods are mean-standardization and normal-standardization and are noted as given below:
Mean-standardization: \(X_{ij}^{*} = X_{ij} /\overline{{X_{j} }}\) , which \(\overline{{X_{j} }}\) represents the mean value.
Normal-standardization: \(X_{ij}^{*} = (X_{ij} - \overline{{X_{j} }} )/s_{j}\) , which \(\overline{{X_{j} }}\) represents the mean value, and \(s_{j}\) is the standard deviation.
The array fe_array is defined according to Table 2 , row number maps to the features, columns 0, 1, 2, 3 note for the extension methods of normalize, polarize, max–min scale, and fluctuation percentage, respectively. Then we fill in the values for the array by the rule where 0 stands for no necessity to expand and 1 for features need to apply the corresponding extension methods. The final algorithm of data preprocessing using RFE and PCA can be illustrated as Algorithm 1.
Algorithm 2: Price trend prediction model using LSTM
After the principal component extraction, we will get the scale-reduced matrix, which means i most effective features are converted into j principal components for training the prediction model. We utilized an LSTM model and added a conversion procedure for our stock price dataset. The detailed algorithm design is illustrated in Alg 2. The function TimeSeriesConversion () converts the principal components matrix into time series by shifting the input data frame according to the number of time steps [ 3 ], i.e., term length in this research. The processed dataset consists of the input sequence and forecast sequence. In this research, the parameter of LAG is 1, because the model is detecting the pattern of features fluctuation on a daily basis. Meanwhile, the N_TIME_STEPS is varied from 1 trading day to 10 trading days. The functions DataPartition (), FitModel (), EvaluateModel () are regular steps without customization. The NN structure design, optimizer decision, and other parameters are illustrated in function ModelCompile () .
Some procedures impact the efficiency but do not affect the accuracy or precision and vice versa, while other procedures may affect both efficiency and prediction result. To fully evaluate our algorithm design, we structure the evaluation part by main procedures and evaluate how each procedure affects the algorithm performance. First, we evaluated our solution on a machine with 2.2 GHz i7 processor, with 16 GB of RAM. Furthermore, we also evaluated our solution on Amazon EC2 instance, 3.1 GHz Processor with 16 vCPUs, and 64 GB RAM.
In the implementation part, we expanded 20 features into 54 features, while we retain 30 features that are the most effective. In this section, we discuss the evaluation of feature selection. The dataset was divided into two different subsets, i.e., training and testing datasets. Test procedure included two parts, one testing dataset is for feature selection, and another one is for model testing. We note the feature selection dataset and model testing dataset as DS_test_f and DS_test_m, respectively.
We randomly selected two-thirds of the stock data by stock ID for RFE training and note the dataset as DS_train_f; all the data consist of full technical indices and expanded features throughout 2018. The estimator of the RFE algorithm is SVR with linear kernels. We rank the 54 features by voting and get 30 effective features then process them using the PCA algorithm to perform dimension reduction and reduce the features into 20 principal components. The rest of the stock data forms the testing dataset DS_test_f to validate the effectiveness of principal components we extracted from selected features. We reformed all the data from 2018 as the training dataset of the data model and noted as DS_train_m. The model testing dataset DS_test_m consists of the first 3 months of data in 2019, which has no overlap with the dataset we utilized in the previous steps. This approach is to prevent the hidden problem caused by overfitting.
Term length
To build an efficient prediction model, instead of the approach of modeling the data to time series, we determined to use 1 day ahead indices data to predict the price trend of the next day. We tested the RFE algorithm on a range of short-term from 1 day to 2 weeks (ten trading days), to evaluate how the commonly used technical indices correlated to price trends. For evaluating the prediction term length, we fully expanded the features as Table 2 , and feed them to RFE. During the test, we found that different length of the term has a different level of sensitive-ness to the same indices set.
We get the close price of the first trading date and compare it with the close price of the n _ th trading date. Since we are predicting the price trend, we do not consider the term lengths if the cross-validation score is below 0.5. And after the test, as we can see from Fig. 4 , there are three-term lengths that are most sensitive to the indices we selected from the related works. They are n = {2, 5, 10}, which indicates that price trend prediction of every other day, 1 week, and 2 weeks using the indices set are likely to be more reliable.
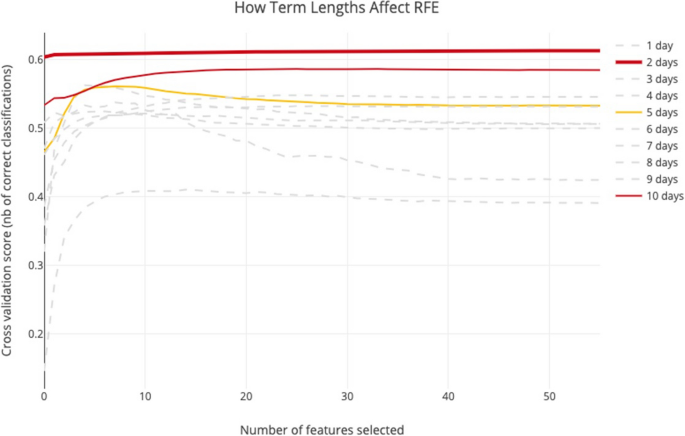
How do term lengths affect the cross-validation score of RFE
While these curves have different patterns, for the length of 2 weeks, the cross-validation score increases with the number of features selected. If the prediction term length is 1 week, the cross-validation score will decrease if selected over 8 features. For every other day price trend prediction, the best cross-validation score is achieved by selecting 48 features. Biweekly prediction requires 29 features to achieve the best score. In Table 3 , we listed the top 15 effective features for these three-period lengths. If we predict the price trend of every other day, the cross-validation score merely fluctuates with the number of features selected. So, in the next step, we will evaluate the RFE result for these three-term lengths, as shown in Fig. 4 .
We compare the output feature set of RFE with the all-original feature set as a baseline, the all-original feature set consists of n features and we choose n most effective features from RFE output features to evaluate the result using linear SVR. We used two different approaches to evaluate feature effectiveness. The first method is to combine all the data into one large matrix and evaluate them by running the RFE algorithm once. Another method is to run RFE for each individual stock and calculate the most effective features by voting.
Feature extension and RFE
From the result of the previous subsection, we can see that when predicting the price trend for every other day or biweekly, the best result is achieved by selecting a large number of features. Within the selected features, some features processed from extension methods have better ranks than original features, which proves that the feature extension method is useful for optimizing the model. The feature extension affects both precision and efficiency, while in this part, we only discuss the precision aspect and leave efficiency part in the next step since PCA is the most effective method for training efficiency optimization in our design. We involved an evaluation of how feature extension affects RFE and use the test result to measure the improvement of involving feature extension.
We further test the effectiveness of feature extension, i.e., if polarize, max–min scale, and calculate fluctuation percentage works better than original technical indices. The best case to leverage this test is the weekly prediction since it has the least effective feature selected. From the result we got from the last section, we know the best cross-validation score appears when selecting 8 features. The test consists of two steps, and the first step is to test the feature set formed by original features only, in this case, only SLOWK, SLOWD, and RSI_5 are included. The next step is to test the feature set of all 8 features we selected in the previous subsection. We leveraged the test by defining the simplest DNN model with three layers.
The normalized confusion matrix of testing the two feature sets are illustrated in Fig. 5 . The left one is the confusion matrix of the feature set with expanded features, and the right one besides is the test result of using original features only. Both precisions of true positive and true negative have been improved by 7% and 10%, respectively, which proves that our feature extension method design is reasonably effective.
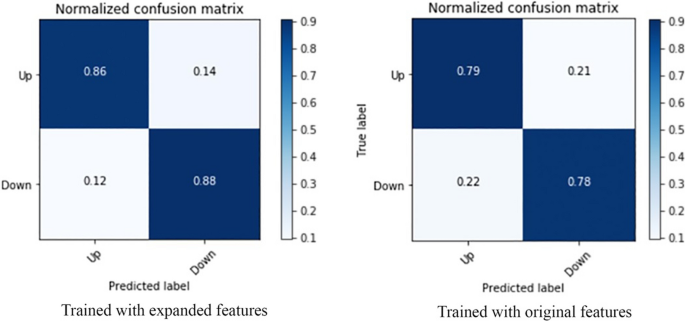
Confusion matrix of validating feature extension effectiveness
Feature reduction using principal component analysis
PCA will affect the algorithm performance on both prediction accuracy and training efficiency, while this part should be evaluated with the NN model, so we also defined the simplest DNN model with three layers as we used in the previous step to perform the evaluation. This part introduces the evaluation method and result of the optimization part of the model from computational efficiency and accuracy impact perspectives.
In this section, we will choose bi-weekly prediction to perform a use case analysis, since it has a smoothly increasing cross-validation score curve, moreover, unlike every other day prediction, it has excluded more than 20 ineffective features already. In the first step, we select all 29 effective features and train the NN model without performing PCA. It creates a baseline of the accuracy and training time for comparison. To evaluate the accuracy and efficiency, we keep the number of the principal component as 5, 10, 15, 20, 25. Table 4 recorded how the number of features affects the model training efficiency, then uses the stack bar chart in Fig. 6 to illustrate how PCA affects training efficiency. Table 6 shows accuracy and efficiency analysis on different procedures for the pre-processing of features. The times taken shown in Tables 4 , 6 are based on experiments conducted in a standard user machine to show the viability of our solution with limited or average resource availability.
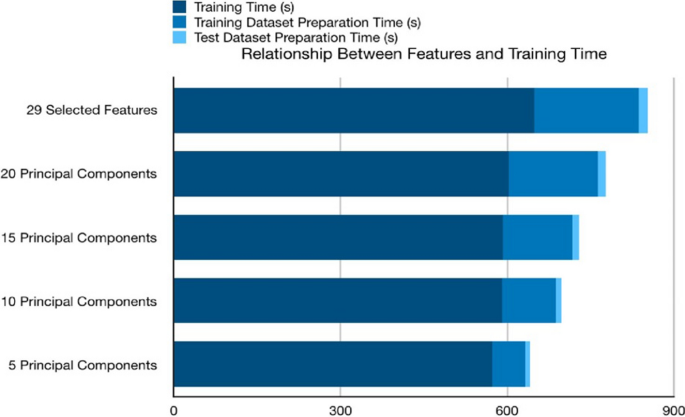
Relationship between feature number and training time
We also listed the confusion matrix of each test in Fig. 7 . The stack bar chart shows that the overall time spends on training the model is decreasing by the number of selected features, while the PCA method is significantly effective in optimizing training dataset preparation. For the time spent on the training stage, PCA is not as effective as the data preparation stage. While there is the possibility that the optimization effect of PCA is not drastic enough because of the simple structure of the NN model.
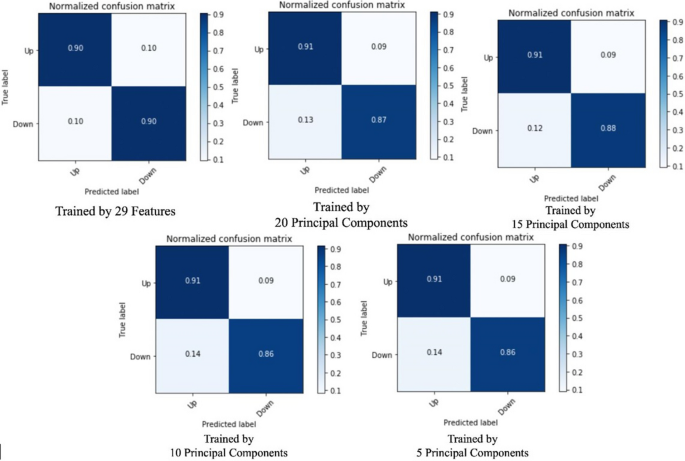
How does the number of principal components affect evaluation results
Table 5 indicates that the overall prediction accuracy is not drastically affected by reducing the dimension. However, the accuracy could not fully support if the PCA has no side effect to model prediction, so we looked into the confusion matrices of test results.
From Fig. 7 we can conclude that PCA does not have a severe negative impact on prediction precision. The true positive rate and false positive rate are barely be affected, while the false negative and true negative rates are influenced by 2% to 4%. Besides evaluating how the number of selected features affects the training efficiency and model performance, we also leveraged a test upon how data pre-processing procedures affect the training procedure and predicting result. Normalizing and max–min scaling is the most commonly seen data pre-procedure performed before PCA, since the measure units of features are varied, and it is said that it could increase the training efficiency afterward.
We leveraged another test on adding pre-procedures before extracting 20 principal components from the original dataset and make the comparison in the aspects of time elapse of training stage and prediction precision. However, the test results lead to different conclusions. In Table 6 we can conclude that feature pre-processing does not have a significant impact on training efficiency, but it does influence the model prediction accuracy. Moreover, the first confusion matrix in Fig. 8 indicates that without any feature pre-processing procedure, the false-negative rate and true negative rate are severely affected, while the true positive rate and false positive rate are not affected. If it performs the normalization before PCA, both true positive rate and true negative rate are decreasing by approximately 10%. This test also proved that the best feature pre-processing method for our feature set is exploiting the max–min scale.
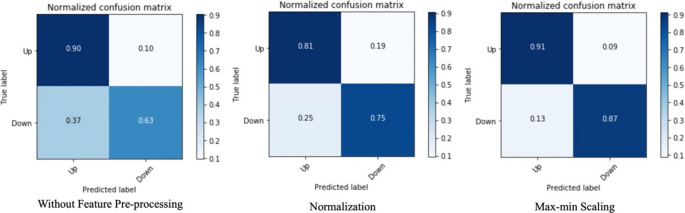
Confusion matrices of different feature pre-processing methods
In this section, we discuss and compare the results of our proposed model, other approaches, and the most related works.
Comparison with related works
From the previous works, we found the most commonly exploited models for short-term stock market price trend prediction are support vector machine (SVM), multilayer perceptron artificial neural network (MLP), Naive Bayes classifier (NB), random forest classifier (RAF) and logistic regression classifier (LR). The test case of comparison is also bi-weekly price trend prediction, to evaluate the best result of all models, we keep all 29 features selected by the RFE algorithm. For MLP evaluation, to test if the number of hidden layers would affect the metric scores, we noted layer number as n and tested n = {1, 3, 5}, 150 training epochs for all the tests, found slight differences in the model performance, which indicates that the variable of MLP layer number hardly affects the metric scores.
From the confusion matrices in Fig. 9 , we can see all the machine learning models perform well when training with the full feature set we selected by RFE. From the perspective of training time, training the NB model got the best efficiency. LR algorithm cost less training time than other algorithms while it can achieve a similar prediction result with other costly models such as SVM and MLP. RAF algorithm achieved a relatively high true-positive rate while the poor performance in predicting negative labels. For our proposed LSTM model, it achieves a binary accuracy of 93.25%, which is a significantly high precision of predicting the bi-weekly price trend. We also pre-processed data through PCA and got five principal components, then trained for 150 epochs. The learning curve of our proposed solution, based on feature engineering and the LSTM model, is illustrated in Fig. 10 . The confusion matrix is the figure on the right in Fig. 11 , and detailed metrics scores can be found in Table 9 .
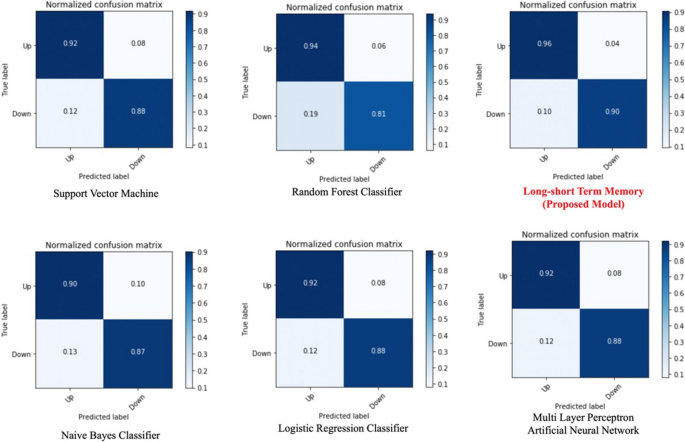
Model prediction comparison—confusion matrices
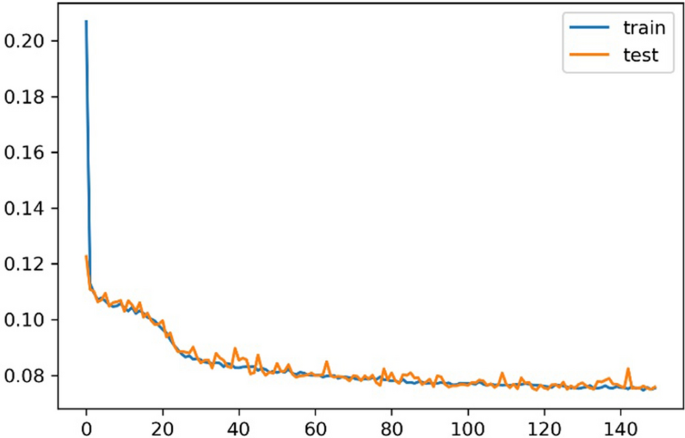
Learning curve of proposed solution
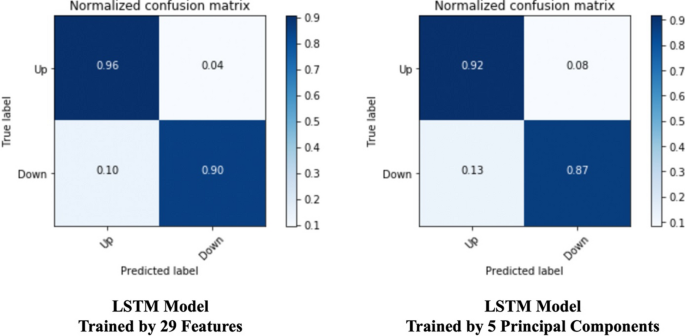
Proposed model prediction precision comparison—confusion matrices
The detailed evaluate results are recorded in Table 7 . We will also initiate a discussion upon the evaluation result in the next section.
Because the resulting structure of our proposed solution is different from most of the related works, it would be difficult to make naïve comparison with previous works. For example, it is hard to find the exact accuracy number of price trend prediction in most of the related works since the authors prefer to show the gain rate of simulated investment. Gain rate is a processed number based on simulated investment tests, sometimes one correct investment decision with a large trading volume can achieve a high gain rate regardless of the price trend prediction accuracy. Besides, it is also a unique and heuristic innovation in our proposed solution, we transform the problem of predicting an exact price straight forward to two sequential problems, i.e., predicting the price trend first, focus on building an accurate binary classification model, construct a solid foundation for predicting the exact price change in future works. Besides the different result structure, the datasets that previous works researched on are also different from our work. Some of the previous works involve news data to perform sentiment analysis and exploit the SE part as another system component to support their prediction model.
The latest related work that can compare is Zubair et al. [ 47 ], the authors take multiple r-square for model accuracy measurement. Multiple r-square is also called the coefficient of determination, and it shows the strength of predictor variables explaining the variation in stock return [ 28 ]. They used three datasets (KSE 100 Index, Lucky Cement Stock, Engro Fertilizer Limited) to evaluate the proposed multiple regression model and achieved 95%, 89%, and 97%, respectively. Except for the KSE 100 Index, the dataset choice in this related work is individual stocks; thus, we choose the evaluation result of the first dataset of their proposed model.
We listed the leading stock price trend prediction model performance in Table 8 , from the comparable metrics, the metric scores of our proposed solution are generally better than other related works. Instead of concluding arbitrarily that our proposed model outperformed other models in related works, we first look into the dataset column of Table 8 . By looking into the dataset used by each work [ 18 ], only trained and tested their proposed solution on three individual stocks, which is difficult to prove the generalization of their proposed model. Ayo [ 2 ] leveraged analysis on the stock data from the New York Stock Exchange (NYSE), while the weakness is they only performed analysis on closing price, which is a feature embedded with high noise. Zubair et al. [ 47 ] trained their proposed model on both individual stocks and index price, but as we have mentioned in the previous section, index price only consists of the limited number of features and stock IDs, which will further affect the model training quality. For our proposed solution, we collected sufficient data from the Chinese stock market, and applied FE + RFE algorithm on the original indices to get more effective features, the comprehensive evaluation result of 3558 stock IDs can reasonably explain the generalization and effectiveness of our proposed solution in Chinese stock market. However, the authors of Khaidem and Dey [ 18 ] and Ayo [ 2 ] chose to analyze the stock market in the United States, Zubair et al. [ 47 ] performed analysis on Pakistani stock market price, and we obtained the dataset from Chinese stock market, the policies of different countries might impact the model performance, which needs further research to validate.
Proposed model evaluation—PCA effectiveness
Besides comparing the performance across popular machine learning models, we also evaluated how the PCA algorithm optimizes the training procedure of the proposed LSTM model. We recorded the confusion matrices comparison between training the model by 29 features and by five principal components in Fig. 11 . The model training using the full 29 features takes 28.5 s per epoch on average. While it only takes 18 s on average per epoch training on the feature set of five principal components. PCA has significantly improved the training efficiency of the LSTM model by 36.8%. The detailed metrics data are listed in Table 9 . We will leverage a discussion in the next section about complexity analysis.
Complexity analysis of proposed solution
This section analyzes the complexity of our proposed solution. The Long Short-term Memory is different from other NNs, and it is a variant of standard RNN, which also has time steps with memory and gate architecture. In the previous work [ 46 ], the author performed an analysis of the RNN architecture complexity. They introduced a method to regard RNN as a directed acyclic graph and proposed a concept of recurrent depth, which helps perform the analysis on the intricacy of RNN.
The recurrent depth is a positive rational number, and we denote it as \(d_{rc}\) . As the growth of \(n\) \(d_{rc}\) measures, the nonlinear transformation average maximum number of each time step. We then unfold the directed acyclic graph of RNN and denote the processed graph as \(g_{c}\) , meanwhile, denote \(C(g_{c} )\) as the set of directed cycles in this graph. For the vertex \(v\) , we note \(\sigma_{s} (v)\) as the sum of edge weights and \(l(v)\) as the length. The equation below is proved under a mild assumption, which could be found in [ 46 ].
They also found that another crucial factor that impacts the performance of LSTM, which is the recurrent skip coefficients. We note \(s_{rc}\) as the reciprocal of the recurrent skip coefficient. Please be aware that \(s_{rc}\) is also a positive rational number.
According to the above definition, our proposed model is a 2-layers stacked LSTM, which \(d_{rc} = 2\) and \(s_{rc} = 1\) . From the experiments performed in previous work, the authors also found that when facing the problems of long-term dependency, LSTMs may benefit from decreasing the reciprocal of recurrent skip coefficients and from increasing recurrent depth. The empirical findings above mentioned are useful to enhance the performance of our proposed model further.
This work consists of three parts: data extraction and pre-processing of the Chinese stock market dataset, carrying out feature engineering, and stock price trend prediction model based on the long short-term memory (LSTM). We collected, cleaned-up, and structured 2 years of Chinese stock market data. We reviewed different techniques often used by real-world investors, developed a new algorithm component, and named it as feature extension, which is proved to be effective. We applied the feature expansion (FE) approaches with recursive feature elimination (RFE), followed by principal component analysis (PCA), to build a feature engineering procedure that is both effective and efficient. The system is customized by assembling the feature engineering procedure with an LSTM prediction model, achieved high prediction accuracy that outperforms the leading models in most related works. We also carried out a comprehensive evaluation of this work. By comparing the most frequently used machine learning models with our proposed LSTM model under the feature engineering part of our proposed system, we conclude many heuristic findings that could be future research questions in both technical and financial research domains.
Our proposed solution is a unique customization as compared to the previous works because rather than just proposing yet another state-of-the-art LSTM model, we proposed a fine-tuned and customized deep learning prediction system along with utilization of comprehensive feature engineering and combined it with LSTM to perform prediction. By researching into the observations from previous works, we fill in the gaps between investors and researchers by proposing a feature extension algorithm before recursive feature elimination and get a noticeable improvement in the model performance.
Though we have achieved a decent outcome from our proposed solution, this research has more potential towards research in future. During the evaluation procedure, we also found that the RFE algorithm is not sensitive to the term lengths other than 2-day, weekly, biweekly. Getting more in-depth research into what technical indices would influence the irregular term lengths would be a possible future research direction. Moreover, by combining latest sentiment analysis techniques with feature engineering and deep learning model, there is also a high potential to develop a more comprehensive prediction system which is trained by diverse types of information such as tweets, news, and other text-based data.
Abbreviations
Long short term memory
Principal component analysis
Recurrent neural networks
Artificial neural network
Deep neural network
Dynamic Time Warping
Recursive feature elimination
Support vector machine
Convolutional neural network
Stochastic gradient descent
Rectified linear unit
Multi layer perceptron
Atsalakis GS, Valavanis KP. Forecasting stock market short-term trends using a neuro-fuzzy based methodology. Expert Syst Appl. 2009;36(7):10696–707.
Article Google Scholar
Ayo CK. Stock price prediction using the ARIMA model. In: 2014 UKSim-AMSS 16th international conference on computer modelling and simulation. 2014. https://doi.org/10.1109/UKSim.2014.67 .
Brownlee J. Deep learning for time series forecasting: predict the future with MLPs, CNNs and LSTMs in Python. Machine Learning Mastery. 2018. https://machinelearningmastery.com/time-series-prediction-lstm-recurrent-neural-networks-python-keras/
Eapen J, Bein D, Verma A. Novel deep learning model with CNN and bi-directional LSTM for improved stock market index prediction. In: 2019 IEEE 9th annual computing and communication workshop and conference (CCWC). 2019. pp. 264–70. https://doi.org/10.1109/CCWC.2019.8666592 .
Fischer T, Krauss C. Deep learning with long short-term memory networks for financial market predictions. Eur J Oper Res. 2018;270(2):654–69. https://doi.org/10.1016/j.ejor.2017.11.054 .
Article MathSciNet MATH Google Scholar
Guyon I, Weston J, Barnhill S, Vapnik V. Gene selection for cancer classification using support vector machines. Mach Learn 2002;46:389–422.
Hafezi R, Shahrabi J, Hadavandi E. A bat-neural network multi-agent system (BNNMAS) for stock price prediction: case study of DAX stock price. Appl Soft Comput J. 2015;29:196–210. https://doi.org/10.1016/j.asoc.2014.12.028 .
Halko N, Martinsson PG, Tropp JA. Finding structure with randomness: probabilistic algorithms for constructing approximate matrix decompositions. SIAM Rev. 2001;53(2):217–88.
Article MathSciNet Google Scholar
Hassan MR, Nath B. Stock market forecasting using Hidden Markov Model: a new approach. In: Proceedings—5th international conference on intelligent systems design and applications 2005, ISDA’05. 2005. pp. 192–6. https://doi.org/10.1109/ISDA.2005.85 .
Hochreiter S, Schmidhuber J. Long short-term memory. J Neural Comput. 1997;9(8):1735–80. https://doi.org/10.1162/neco.1997.9.8.1735 .
Hsu CM. A hybrid procedure with feature selection for resolving stock/futures price forecasting problems. Neural Comput Appl. 2013;22(3–4):651–71. https://doi.org/10.1007/s00521-011-0721-4 .
Huang CF, Chang BR, Cheng DW, Chang CH. Feature selection and parameter optimization of a fuzzy-based stock selection model using genetic algorithms. Int J Fuzzy Syst. 2012;14(1):65–75. https://doi.org/10.1016/J.POLYMER.2016.08.021 .
Huang CL, Tsai CY. A hybrid SOFM-SVR with a filter-based feature selection for stock market forecasting. Expert Syst Appl. 2009;36(2 PART 1):1529–39. https://doi.org/10.1016/j.eswa.2007.11.062 .
Idrees SM, Alam MA, Agarwal P. A prediction approach for stock market volatility based on time series data. IEEE Access. 2019;7:17287–98. https://doi.org/10.1109/ACCESS.2019.2895252 .
Ince H, Trafalis TB. Short term forecasting with support vector machines and application to stock price prediction. Int J Gen Syst. 2008;37:677–87. https://doi.org/10.1080/03081070601068595 .
Jeon S, Hong B, Chang V. Pattern graph tracking-based stock price prediction using big data. Future Gener Comput Syst. 2018;80:171–87. https://doi.org/10.1016/j.future.2017.02.010 .
Kara Y, Acar Boyacioglu M, Baykan ÖK. Predicting direction of stock price index movement using artificial neural networks and support vector machines: the sample of the Istanbul Stock Exchange. Expert Syst Appl. 2011;38(5):5311–9. https://doi.org/10.1016/j.eswa.2010.10.027 .
Khaidem L, Dey SR. Predicting the direction of stock market prices using random forest. 2016. pp. 1–20.
Kim K, Han I. Genetic algorithms approach to feature discretization in artificial neural networks for the prediction of stock price index. Expert Syst Appl. 2000;19:125–32. https://doi.org/10.1016/S0957-4174(00)00027-0 .
Lee MC. Using support vector machine with a hybrid feature selection method to the stock trend prediction. Expert Syst Appl. 2009;36(8):10896–904. https://doi.org/10.1016/j.eswa.2009.02.038 .
Lei L. Wavelet neural network prediction method of stock price trend based on rough set attribute reduction. Appl Soft Comput J. 2018;62:923–32. https://doi.org/10.1016/j.asoc.2017.09.029 .
Lin X, Yang Z, Song Y. Expert systems with applications short-term stock price prediction based on echo state networks. Expert Syst Appl. 2009;36(3):7313–7. https://doi.org/10.1016/j.eswa.2008.09.049 .
Liu G, Wang X. A new metric for individual stock trend prediction. Eng Appl Artif Intell. 2019;82(March):1–12. https://doi.org/10.1016/j.engappai.2019.03.019 .
Liu S, Zhang C, Ma J. CNN-LSTM neural network model for quantitative strategy analysis in stock markets. 2017;1:198–206. https://doi.org/10.1007/978-3-319-70096-0 .
Long W, Lu Z, Cui L. Deep learning-based feature engineering for stock price movement prediction. Knowl Based Syst. 2018;164:163–73. https://doi.org/10.1016/j.knosys.2018.10.034 .
Malkiel BG, Fama EF. Efficient capital markets: a review of theory and empirical work. J Finance. 1970;25(2):383–417.
McNally S, Roche J, Caton S. Predicting the price of bitcoin using machine learning. In: Proceedings—26th Euromicro international conference on parallel, distributed, and network-based processing, PDP 2018. pp. 339–43. https://doi.org/10.1109/PDP2018.2018.00060 .
Nagar A, Hahsler M. News sentiment analysis using R to predict stock market trends. 2012. http://past.rinfinance.com/agenda/2012/talk/Nagar+Hahsler.pdf . Accessed 20 July 2019.
Nekoeiqachkanloo H, Ghojogh B, Pasand AS, Crowley M. Artificial counselor system for stock investment. 2019. ArXiv Preprint arXiv:1903.00955 .
Ni LP, Ni ZW, Gao YZ. Stock trend prediction based on fractal feature selection and support vector machine. Expert Syst Appl. 2011;38(5):5569–76. https://doi.org/10.1016/j.eswa.2010.10.079 .
Pang X, Zhou Y, Wang P, Lin W, Chang V. An innovative neural network approach for stock market prediction. J Supercomput. 2018. https://doi.org/10.1007/s11227-017-2228-y .
Pimenta A, Nametala CAL, Guimarães FG, Carrano EG. An automated investing method for stock market based on multiobjective genetic programming. Comput Econ. 2018;52(1):125–44. https://doi.org/10.1007/s10614-017-9665-9 .
Piramuthu S. Evaluating feature selection methods for learning in data mining applications. Eur J Oper Res. 2004;156(2):483–94. https://doi.org/10.1016/S0377-2217(02)00911-6 .
Qiu M, Song Y. Predicting the direction of stock market index movement using an optimized artificial neural network model. PLoS ONE. 2016;11(5):e0155133.
Scikit-learn. Scikit-learn Min-Max Scaler. 2019. https://scikit-learn.org/stable/modules/generated/sklearn.preprocessing.MinMaxScaler.html . Retrieved 26 July 2020.
Shen J. Thesis, “Short-term stock market price trend prediction using a customized deep learning system”, supervised by M. Omair Shafiq, Carleton University. 2019.
Shen J, Shafiq MO. Deep learning convolutional neural networks with dropout—a parallel approach. ICMLA. 2018;2018:572–7.
Google Scholar
Shen J, Shafiq MO. Learning mobile application usage—a deep learning approach. ICMLA. 2019;2019:287–92.
Shih D. A study of early warning system in volume burst risk assessment of stock with Big Data platform. In: 2019 IEEE 4th international conference on cloud computing and big data analysis (ICCCBDA). 2019. pp. 244–8.
Sirignano J, Cont R. Universal features of price formation in financial markets: perspectives from deep learning. Ssrn. 2018. https://doi.org/10.2139/ssrn.3141294 .
Article MATH Google Scholar
Thakur M, Kumar D. A hybrid financial trading support system using multi-category classifiers and random forest. Appl Soft Comput J. 2018;67:337–49. https://doi.org/10.1016/j.asoc.2018.03.006 .
Tsai CF, Hsiao YC. Combining multiple feature selection methods for stock prediction: union, intersection, and multi-intersection approaches. Decis Support Syst. 2010;50(1):258–69. https://doi.org/10.1016/j.dss.2010.08.028 .
Tushare API. 2018. https://github.com/waditu/tushare . Accessed 1 July 2019.
Wang X, Lin W. Stock market prediction using neural networks: does trading volume help in short-term prediction?. n.d.
Weng B, Lu L, Wang X, Megahed FM, Martinez W. Predicting short-term stock prices using ensemble methods and online data sources. Expert Syst Appl. 2018;112:258–73. https://doi.org/10.1016/j.eswa.2018.06.016 .
Zhang S. Architectural complexity measures of recurrent neural networks, (NIPS). 2016. pp. 1–9.
Zubair M, Fazal A, Fazal R, Kundi M. Development of stock market trend prediction system using multiple regression. Computational and mathematical organization theory. Berlin: Springer US; 2019. https://doi.org/10.1007/s10588-019-09292-7 .
Book Google Scholar
Download references
Acknowledgements
This research is supported by Carleton University, in Ottawa, ON, Canada. This research paper has been built based on the thesis [ 36 ] of Jingyi Shen, supervised by M. Omair Shafiq at Carleton University, Canada, available at https://curve.carleton.ca/52e9187a-7f71-48ce-bdfe-e3f6a420e31a .
NSERC and Carleton University.
Author information
Authors and affiliations.
School of Information Technology, Carleton University, Ottawa, ON, Canada
Jingyi Shen & M. Omair Shafiq
You can also search for this author in PubMed Google Scholar
Contributions
Yes. All authors read and approved the final manuscript.
Corresponding author
Correspondence to M. Omair Shafiq .
Ethics declarations
Competing interests.
The authors declare that they have no competing interests.
Additional information
Publisher's note.
Springer Nature remains neutral with regard to jurisdictional claims in published maps and institutional affiliations.
Rights and permissions
Open Access This article is licensed under a Creative Commons Attribution 4.0 International License, which permits use, sharing, adaptation, distribution and reproduction in any medium or format, as long as you give appropriate credit to the original author(s) and the source, provide a link to the Creative Commons licence, and indicate if changes were made. The images or other third party material in this article are included in the article's Creative Commons licence, unless indicated otherwise in a credit line to the material. If material is not included in the article's Creative Commons licence and your intended use is not permitted by statutory regulation or exceeds the permitted use, you will need to obtain permission directly from the copyright holder. To view a copy of this licence, visit http://creativecommons.org/licenses/by/4.0/ .
Reprints and permissions
About this article
Cite this article.
Shen, J., Shafiq, M.O. Short-term stock market price trend prediction using a comprehensive deep learning system. J Big Data 7 , 66 (2020). https://doi.org/10.1186/s40537-020-00333-6
Download citation
Received : 24 January 2020
Accepted : 30 July 2020
Published : 28 August 2020
DOI : https://doi.org/10.1186/s40537-020-00333-6
Share this article
Anyone you share the following link with will be able to read this content:
Sorry, a shareable link is not currently available for this article.
Provided by the Springer Nature SharedIt content-sharing initiative
- Deep learning
- Stock market trend
- Feature engineering
Stock Buybacks Have a Positive Impact on Stock Price Stabilization, New Research Shows
By Lacie Blankenship
Stock buybacks have been a hot topic in recent political conversations. Vanderbilt Business professors shed light on the impact of stock buybacks on stock liquidity and volatility and why that matters.
What is a stock buyback?
Stock buybacks, also known as share repurchases, occur when a company purchases its own shares from the marketplace. Buybacks increase the demand and value of company shares, providing investors with a return on their investment. In recent months, this financial tactic has come under heightened scrutiny for potentially having an outsized benefit for company executives with large stakes in their own companies.
Why does this matter?
The Biden Administration recently unveiled tax plan includes a provision that levies a 1% surcharge on repurchasing companies, adding fuel to the stock buyback debate.
In a recent report released by the U.S. Chamber of Commerce and published by the Center for Capital Markets , Craig M. Lewis , Madison S. Wigginton Professor of Finance, and Josh T. White , Assistant Professor of Finance and Brownlee O. Currey Jr. Dean’s Faculty Fellow, found that stock buybacks have an overlooked beneficial effect on stock liquidity (the ability for quick and low-impact transactions) and stock volatility (degree of price movement).
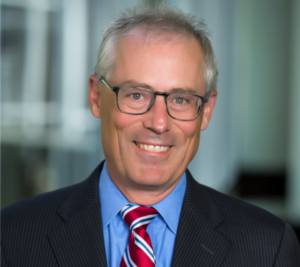
Professor Craig Lewis
“Much of the political rhetoric around stock buybacks focuses on benefits for executives or large investors,” notes Lewis. “Despite their growing importance, the implications of buybacks for independent retail investors are often absent from this conversation and we sought to explore that perspective in this report.”
What does the research tell us?
Study background, by using a large sample of 10,000+ u.s. companies over 17 years, the study, corporate liquidity provision and share repurchase programs , presents evidence that “managers [of public companies] strategically utilize share repurchases ([stock buybacks]) to increase stock liquidity and reduce volatility,” and therefore stabilize stock prices, benefiting all investors., the study found 6 key benefits associated with buybacks:.
- Greater Liquidity : Companies repurchasing stock provides substantial liquidity that facilitates orderly trading and reduces transaction costs for retail investors.
- Reduced Volatility : Stock buybacks significantly reduce realized and anticipated return volatility. Imposing limitations on buyback activity would increase stock market volatility and force retail investors to bear greater amounts of downside risk.
- Retail Investors Impact : Stock buybacks generate an economically large benefit for retail investors. Since 2004, buybacks have saved retail investors $2.1–4.2 billion in transaction and price impact costs.
- Proactive Repurchase Activity : Managers utilize market-based estimates of future volatility to inform their buyback decisions. When volatility is expected to be higher, managers increase their buyback intensity to stabilize stock prices, thus reducing costs for retail investors.
- Response To Uncertainty : Studies show that economic policy uncertainty increases stock price volatility and illiquidity. Managers respond to elevated policy uncertainty by strengthening their buyback activities. Retail investors benefit from price certainty about the value of their investments during periods of greater uncertainty.
- Strategic Liquidity Supplier : Managers expand stock buyback activity during critical periods when investors sell relatively large amounts of shares. Thus, managers use buybacks to actively mitigate price pressure during periods of net selling pressure.
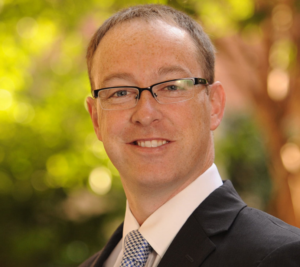
Professor Josh T. White
Lewis and White’s research demonstrates that stock buybacks positively contribute to the welfare of all investors and stock transactions, not just the large shareholders.
“It is important to understand how financial policies impact retail investors, who now account for over 20% of U.S. equity trading volume,” says White. “Our study demonstrates how buybacks lower trading costs and reduce downside risk for these key stakeholders.”
Study Implications
This research has powerful implications for policies regarding share repurchase regulation, taxation, and bans. Knowing that stock buybacks positively influence stock price stabilization, it is notable that limiting or taxing corporate buybacks will reduce opportunities to “supply liquidity and reduce volatility,” ultimately resulting in harm.
Faculty News Featured News News Press Releases
Other Stories
Which program is right for you?
- Work & Careers
- Life & Arts
Become an FT subscriber
Try unlimited access Only $1 for 4 weeks
Then $75 per month. Complete digital access to quality FT journalism on any device. Cancel anytime during your trial.
- Global news & analysis
- Expert opinion
- Special features
- FirstFT newsletter
- Videos & Podcasts
- Android & iOS app
- FT Edit app
- 10 gift articles per month
Explore more offers.
Standard digital.
- FT Digital Edition
Premium Digital
Print + premium digital, weekend print + standard digital, weekend print + premium digital.
Essential digital access to quality FT journalism on any device. Pay a year upfront and save 20%.
- Global news & analysis
- Exclusive FT analysis
- FT App on Android & iOS
- FirstFT: the day's biggest stories
- 20+ curated newsletters
- Follow topics & set alerts with myFT
- FT Videos & Podcasts
- 20 monthly gift articles to share
- Lex: FT's flagship investment column
- 15+ Premium newsletters by leading experts
- FT Digital Edition: our digitised print edition
- Weekday Print Edition
- Videos & Podcasts
- Premium newsletters
- 10 additional gift articles per month
- FT Weekend Print delivery
- Everything in Standard Digital
- Everything in Premium Digital
Complete digital access to quality FT journalism with expert analysis from industry leaders. Pay a year upfront and save 20%.
- 10 monthly gift articles to share
- Everything in Print
Terms & Conditions apply
Explore our full range of subscriptions.
Why the ft.
See why over a million readers pay to read the Financial Times.
International Edition
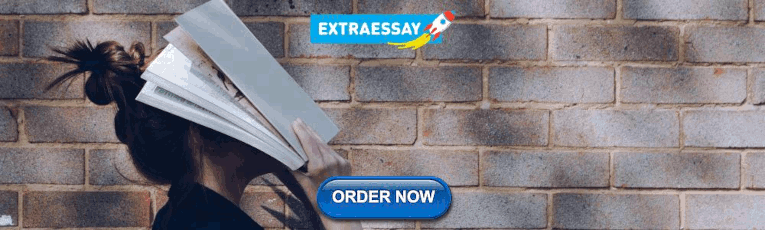
IMAGES
VIDEO
COMMENTS
A stock market is a place for trading stocks (equity) and other financial instruments of public listed companies, where the price of shares is termed "share" or "stock price" (Wanjawa and Muchemi 2014). In reality, the stock price level of a firm, to a large extent reflects how it "cuts its pie" (Chan et al. 2017).
The aim of the paper is to present key factors they are determinants of share prices in the stock markets globally. To achieve the aim of this study many of previous studies have been reviewed.
This paper attempts to provide empirical evidence on how EPS affect the share price movement. We have collected and analyzed 22 scheduled banks 110 firm year data and found that share price does ...
EPS is one of the measure to check the financial position of the firm [2]. Core et al. [7] concluded that in presence of ESP (Employee Stock Option) EPS will positively influence stock price of the company. Forecasting of earnings also influences the stock price. The study of Capstaff et al. [6] is first study in this regard.
This news tends to spread emotions. Investors are vulnerable to these market emotions when making investment decisions. Therefore, how to analyze the impact of news on the stock price has become a hot research topic. In this paper, we intend to establish a model to study the effects of news texts on stock prices.
This paper revisits previous studies by various researches on factors affecting the share price in both developed and developing economies. These researchers looked at the relationships between stock prices and selected internal and external factors affecting a firm's share price. "Some of the factors affecting share prices can be ...
The sustainability of stock price fluctuations indicated by many empirical studies hardly reconciles with the existing models in standard financial theories. This paper proposes a recursive dynamic asset pricing model based on the comprehensive impact of the sentiment investor, the information trader and the noise trader. The dynamic process of the asset price is characterized and a numerical ...
Share Repurchases on Trial: Large-Sample Evidence on Share Price Performance, Executive Compensation, and Corporate Investment. Forthcoming at Financial Management. 41 Pages Posted: 20 Jul 2022 Last revised: 29 Jan 2023. ... S&P Global Market Intelligence Research Paper Series. Follow.
Summary statistics. In response to the COVID-19 pandemic, this paper selects Chinese A-share listed companies as a study sample. This study selects the sample based on the following considerations.
This paper examines the impact of Corporate Social Responsibility (CSR) on companies' stock market performance, measured in terms of share price, in the period after the global financial crisis. We further distinguish between strategic socially responsible activities directly related to a company's business core, and the remaining CSR ...
In the era of big data, deep learning for predicting stock market prices and trends has become even more popular than before. We collected 2 years of data from Chinese stock market and proposed a comprehensive customization of feature engineering and deep learning-based model for predicting price trend of stock markets. The proposed solution is comprehensive as it includes pre-processing of ...
The rest of this paper proceeds in the following structure. Part 2 reviews the literature that explores the mechanism of interest rate on the stock price. ... Interest rates move in the opposite direction from share prices, and money supply is aligned with share prices. However, this effect is statistically asymmetrical, and the influence of ...
Firstly, taking the A-share listed enterprises from 2005 to 2020 as the research sample, this paper analyzed the factors that may affect the innovation behavior of enterprises, such as enterprise stock price information, peer stock price information, and IPO, and put forward relevant assumptions. ... Private enterprises can rely on the share ...
To examine the impact of the dividend policy as well as DPR of the share price. Descriptive statistics and least square regression method: The model is able to explain only 43.43% of the variation in the share price wherein DY, investment growth and earnings volatility insignificantly influence the changes in the company's share price. 2013
3.1. Sample selection. This paper examines a sample of all A-share listed companies in Shanghai and Shenzhen from Q1 2017 to Q3 2021. The data on open market share repurchases was determined through the following steps: Firstly, retain the open market repurchase of A-shares from the WIND database, and delete the directional repurchase, over-the-counter transactions, and repurchase programmes ...
Extending Gordon's approach, the dividend discounting approach hypothesized that the share price is the present value of all future dividend payments. ... International Review of business research Papers, 4(4), 208-222. Google Scholar. Lintner J. (1956). Distribution of incomes of corporations among dividends, retained earnings, and taxes.
The academic literature related to effect of dividend policy on share price has grown rapidly over the previous decade, due to its puzzling phenomena. ... Research paper. Th e Effect of Dividend ...
The conclusions of this research are going to equip policymakers, academicians, current and prospective investors with investing knowledge. ... This paper is structured in five sections including introduction. The second section is the review of existing literature on the subject. ... foreign reserves, exchange rate, US share price index and US ...
This research has powerful implications for policies regarding share repurchase regulation, taxation, and bans. Knowing that stock buybacks positively influence stock price stabilization, it is notable that limiting or taxing corporate buybacks will reduce opportunities to "supply liquidity and reduce volatility," ultimately resulting in harm.
The table has also captures impact of NIM on share prices that shows significantly positive impact. The significant positive impact has been found in earlier studies (Ben Naceur and Goaied, 2008). Moreover, CAR has also shown a positive significant impact on the share price as the t value is 3.29, which is significant at the 0.05 significance ...
There are many internal and external factors, which simultaneously affect stock prices and it is almost impossible to segregate the effect of each so the variations remain. This paper empirically estimates excess stock market returns for all the thirty banks listed in Dhaka Stock Exchange for the period of 2007 to 2011.
AsianJournalofAccounting Research Vol.8No.2,2023 pp.184-193 EmeraldPublishingLimited 2443-4175. DOI10.1108/AJAR-11-2020-0110. Sudhi Sharma, Indira Bhardwaj and Kamal Kishore. Published in Asian Journal of Accounting Research. Published by Emerald Publishing Limited.
This paper analyses the equity share fluctuations in India Selected Industry. It also measures the strength of the trend and the money involved in investing in the stocks. Simple moving average ...
According to a Financial Times analysis of hundreds of LinkedIn profiles as well as public job postings and research papers, the $2.7tn company has undertaken a hiring spree over recent years to ...
Patterns of illicit activity involving groups of bitcoin nodes and chains of transactions are described in a research paper by Elliptic and MIT-IBM Watson AI Lab. By Ian Allison May 1, 2024 at 1: ...