Exam Cheating, Its Causes and Effects
Introduction, definition of cheating, works cited.
The ability of a nation to compete effectively on the international front hinges on the quality of its education. With this in mind, it is okay to conclude that cheating in exams undermines the standard of education in a country and consequently hinders its ability to compete at the world stage. Indeed, students who cheat in exams become poor decision makers in their careers. Their productivity and level of integrity is adversely dented by their belief of having everything the easy way. Academic dishonesty is not new but with the increase in competition for jobs, most students have resorted to cheating in order to qualify for these jobs (Anderman and Johnston 75). The purpose of this paper is to research in detail the causes and effects of cheating in exams.
In the education fraternity, cheating entails: copying from someone, Plagiarizing of academic work and paying someone to do your homework. There are numerous reasons why students cheat in exams however; this action elicits harsh repercussions if one is caught. This may include: suspension, dismissal and/or cancellation of marks (Davis, Grover, Becker and McGregor 16).
One of the major reasons that make students cheat in exams is the over-emphasis that has been placed on passing exams. Apparently, more effort has been directed towards passing of exams than learning due to the high competition in the job market. Similarly, most interviewers focus more on certificates rather than the knowledge of the candidate. It is no wonder most learning institutions these days focus on teaching how to pass an exam and completely disregard impacting knowledge to students.
In some cases, students cheat because they are not confident of their ability or skills in academics. Whenever this feeling is present, students resort to cheating as a way of avoiding ridicule in case of failure. In essence, some of these students are very bright but the fear of failure and the lack of adequate preparations compel them to cheat. The paradox is that when cheating, most students swear that they will never do it again but this only serves as the beginning of a vicious cycle of cheating (Anderman and Johnston 76).
Societal pressure is another major cause for cheating in schools. Parents, teachers and relatives always, with good intentions, mount too much pressure on students to get good grades in order to join good schools and eventually get high paying jobs. All this pressure creates innate feelings that it is okay to cheat in exams if only to satisfy their parents and teachers egos.
There are times when students justify cheating because others do it. In most cases, if the head of the class is cheating then most of the other students will feel they have enough reason to also cheat. The system of education is such that it does not sufficiently reprimand those who cheat and tends to hail those who pass exams regardless of how they have done—the end justifies the means.
With the advent of the internet, it has become very easy to access information from a website using a phone or a computer. Search engines such as Google and Yahoo have made it very easy for students to buy custom-made papers for their class work. It is very easy for students from all over the world to have the same answer for an assignment as they all use a similar website. Indeed, plagiarism is the order of the day, all on has to do is to have the knowledge to search for the different reports and essays on the net (Davis, Grover, Becker and McGregor 18).
Nowadays, most tutors spend most of their class time giving lectures. In fact, it is considered old fashioned to give assignments during class time. Consequently, these assignments are piled up and given during certain durations of the semester. This poses a big challenge to students who have to strike a balance between attending to their homework and having fun. As a result, the workload becomes too much such that it is easier to pay for it to be done than actually do it—homework then becomes as demanding as a full-time job (Jordan 234).
From a tender age, children are taught that cheating is wrong; yet most of them divert from this course as they grow up. In fact, most of them become so addicted to the habit that they feel the need to perfect it. Most often, if a student cheats and never gets caught, he is likely to cheat all his life. Research has shown that students who cheat in high school are twice likely to cheat in college. The bigger problem is that this character is likely to affect one’s career in future consequently tarnishing his/her image.
Cheating in exams poses a great problem in one’s career. To get a good grade as a result of cheating is a misrepresentation of facts. Furthermore, it is difficult for a tutor to isolate students who genuinely need specialized coaching. It becomes a huge embarrassment when a cheating student is expected to give a perfect presentation and fails to demonstrate his ability as indicated by his/her grades. In addition, students who cheat in examination do not get a chance to grasp important concepts in class and are likely to face difficulties in the future when the same principles are applied in higher levels of learning.
The worst-case scenario in cheating in an exam is being caught. Once a student is caught, his reputation is dealt a huge blow. It is likely that such a student will be dismissed or suspended from school. This hinders his/her ability to land a good job or join graduate school. It can also lead to a complete damage of one’s reputation making it hard for others to trust you including those who cheat (Jordan 235).
Cheating in exams and assignments can be attributed to many reasons. To begin with, teaching today concentrates so much on the exams and passing rather than impacting knowledge. Lack of confidence in one’s ability and societal pressure is another reason why cheating is so wide spread. Cheating cannot solely be blamed on the students; lecturers have also played their part in this. Apparently, most lectures concentrate on teaching than giving assignments during class time. This leaves the students with loads of work to cover during their free time.
Technology has also played its part in cheating—many students turn to the internet in a bid to complete their assignments. On the other hand, it is important to note than choices have consequences and the repercussions of cheating in an exams are dire. First, it completely ruins one’s reputation thereby hindering chances of joining college or getting a good job. It also leads to suspensions and/or expulsion from school. Furthermore, the habit is so addictive that it is likely to replicate in all aspects of life—be it relationships, work, business deals etc. It is important to shun this habit as nothing good can come out of it.
Anderman, Erick and Jerome Johnston. “TV News in the Classroom: What are Adolescents Learning?” Journal of Adolescent Research , 13 (1998): 73-100. Print.
Davis, Stephen, Cathy Grover, Angela, Becker, and Loretta McGregor. “Academic Dishonesty: Prevalence, Determinants, Techniques, and Punishments”. Teaching of Psychology , 19 (1) (1996): 16–20. Print.
Jordan, Augustus E. “College Student Cheating: The Role of Motivation, Perceived Norms, Attitudes, and Knowledge of Institutional Policy. Ethics and Behavior , 11, (2001): 233–247. Print.
Cite this paper
- Chicago (N-B)
- Chicago (A-D)
StudyCorgi. (2020, November 25). Exam Cheating, Its Causes and Effects. https://studycorgi.com/exam-cheating-its-causes-and-effects/
"Exam Cheating, Its Causes and Effects." StudyCorgi , 25 Nov. 2020, studycorgi.com/exam-cheating-its-causes-and-effects/.
StudyCorgi . (2020) 'Exam Cheating, Its Causes and Effects'. 25 November.
1. StudyCorgi . "Exam Cheating, Its Causes and Effects." November 25, 2020. https://studycorgi.com/exam-cheating-its-causes-and-effects/.
Bibliography
StudyCorgi . "Exam Cheating, Its Causes and Effects." November 25, 2020. https://studycorgi.com/exam-cheating-its-causes-and-effects/.
StudyCorgi . 2020. "Exam Cheating, Its Causes and Effects." November 25, 2020. https://studycorgi.com/exam-cheating-its-causes-and-effects/.
This paper, “Exam Cheating, Its Causes and Effects”, was written and voluntary submitted to our free essay database by a straight-A student. Please ensure you properly reference the paper if you're using it to write your assignment.
Before publication, the StudyCorgi editorial team proofread and checked the paper to make sure it meets the highest standards in terms of grammar, punctuation, style, fact accuracy, copyright issues, and inclusive language. Last updated: November 8, 2023 .
If you are the author of this paper and no longer wish to have it published on StudyCorgi, request the removal . Please use the “ Donate your paper ” form to submit an essay.
- Our Mission
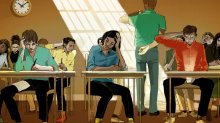
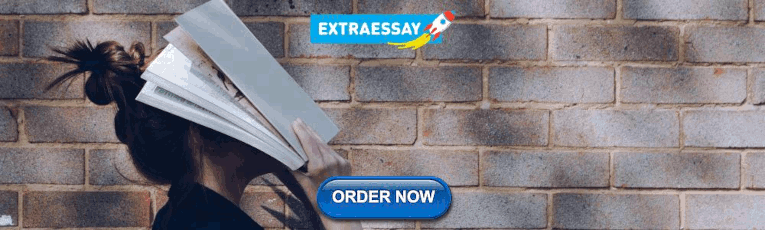
Why Students Cheat—and What to Do About It
A teacher seeks answers from researchers and psychologists.
“Why did you cheat in high school?” I posed the question to a dozen former students.
“I wanted good grades and I didn’t want to work,” said Sonya, who graduates from college in June. [The students’ names in this article have been changed to protect their privacy.]
My current students were less candid than Sonya. To excuse her plagiarized Cannery Row essay, Erin, a ninth-grader with straight As, complained vaguely and unconvincingly of overwhelming stress. When he was caught copying a review of the documentary Hypernormalism , Jeremy, a senior, stood by his “hard work” and said my accusation hurt his feelings.
Cases like the much-publicized ( and enduring ) 2012 cheating scandal at high-achieving Stuyvesant High School in New York City confirm that academic dishonesty is rampant and touches even the most prestigious of schools. The data confirms this as well. A 2012 Josephson Institute’s Center for Youth Ethics report revealed that more than half of high school students admitted to cheating on a test, while 74 percent reported copying their friends’ homework. And a survey of 70,000 high school students across the United States between 2002 and 2015 found that 58 percent had plagiarized papers, while 95 percent admitted to cheating in some capacity.
So why do students cheat—and how do we stop them?
According to researchers and psychologists, the real reasons vary just as much as my students’ explanations. But educators can still learn to identify motivations for student cheating and think critically about solutions to keep even the most audacious cheaters in their classrooms from doing it again.
Rationalizing It
First, know that students realize cheating is wrong—they simply see themselves as moral in spite of it.
“They cheat just enough to maintain a self-concept as honest people. They make their behavior an exception to a general rule,” said Dr. David Rettinger , professor at the University of Mary Washington and executive director of the Center for Honor, Leadership, and Service, a campus organization dedicated to integrity.
According to Rettinger and other researchers, students who cheat can still see themselves as principled people by rationalizing cheating for reasons they see as legitimate.
Some do it when they don’t see the value of work they’re assigned, such as drill-and-kill homework assignments, or when they perceive an overemphasis on teaching content linked to high-stakes tests.
“There was no critical thinking, and teachers seemed pressured to squish it into their curriculum,” said Javier, a former student and recent liberal arts college graduate. “They questioned you on material that was never covered in class, and if you failed the test, it was progressively harder to pass the next time around.”
But students also rationalize cheating on assignments they see as having value.
High-achieving students who feel pressured to attain perfection (and Ivy League acceptances) may turn to cheating as a way to find an edge on the competition or to keep a single bad test score from sabotaging months of hard work. At Stuyvesant, for example, students and teachers identified the cutthroat environment as a factor in the rampant dishonesty that plagued the school.
And research has found that students who receive praise for being smart—as opposed to praise for effort and progress—are more inclined to exaggerate their performance and to cheat on assignments , likely because they are carrying the burden of lofty expectations.
A Developmental Stage
When it comes to risk management, adolescent students are bullish. Research has found that teenagers are biologically predisposed to be more tolerant of unknown outcomes and less bothered by stated risks than their older peers.
“In high school, they’re risk takers developmentally, and can’t see the consequences of immediate actions,” Rettinger says. “Even delayed consequences are remote to them.”
While cheating may not be a thrill ride, students already inclined to rebel against curfews and dabble in illicit substances have a certain comfort level with being reckless. They’re willing to gamble when they think they can keep up the ruse—and more inclined to believe they can get away with it.
Cheating also appears to be almost contagious among young people—and may even serve as a kind of social adhesive, at least in environments where it is widely accepted. A study of military academy students from 1959 to 2002 revealed that students in communities where cheating is tolerated easily cave in to peer pressure, finding it harder not to cheat out of fear of losing social status if they don’t.
Michael, a former student, explained that while he didn’t need to help classmates cheat, he felt “unable to say no.” Once he started, he couldn’t stop.
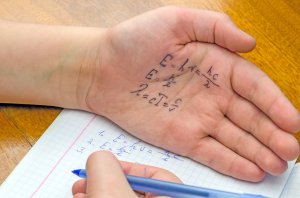
Technology Facilitates and Normalizes It
With smartphones and Alexa at their fingertips, today’s students have easy access to quick answers and content they can reproduce for exams and papers. Studies show that technology has made cheating in school easier, more convenient, and harder to catch than ever before.
To Liz Ruff, an English teacher at Garfield High School in Los Angeles, students’ use of social media can erode their understanding of authenticity and intellectual property. Because students are used to reposting images, repurposing memes, and watching parody videos, they “see ownership as nebulous,” she said.
As a result, while they may want to avoid penalties for plagiarism, they may not see it as wrong or even know that they’re doing it.
This confirms what Donald McCabe, a Rutgers University Business School professor, reported in his 2012 book ; he found that more than 60 percent of surveyed students who had cheated considered digital plagiarism to be “trivial”—effectively, students believed it was not actually cheating at all.
Strategies for Reducing Cheating
Even moral students need help acting morally, said Dr. Jason M. Stephens , who researches academic motivation and moral development in adolescents at the University of Auckland’s School of Learning, Development, and Professional Practice. According to Stephens, teachers are uniquely positioned to infuse students with a sense of responsibility and help them overcome the rationalizations that enable them to think cheating is OK.
1. Turn down the pressure cooker. Students are less likely to cheat on work in which they feel invested. A multiple-choice assessment tempts would-be cheaters, while a unique, multiphase writing project measuring competencies can make cheating much harder and less enticing. Repetitive homework assignments are also a culprit, according to research , so teachers should look at creating take-home assignments that encourage students to think critically and expand on class discussions. Teachers could also give students one free pass on a homework assignment each quarter, for example, or let them drop their lowest score on an assignment.
2. Be thoughtful about your language. Research indicates that using the language of fixed mindsets , like praising children for being smart as opposed to praising them for effort and progress , is both demotivating and increases cheating. When delivering feedback, researchers suggest using phrases focused on effort like, “You made really great progress on this paper” or “This is excellent work, but there are still a few areas where you can grow.”
3. Create student honor councils. Give students the opportunity to enforce honor codes or write their own classroom/school bylaws through honor councils so they can develop a full understanding of how cheating affects themselves and others. At Fredericksburg Academy, high school students elect two Honor Council members per grade. These students teach the Honor Code to fifth graders, who, in turn, explain it to younger elementary school students to help establish a student-driven culture of integrity. Students also write a pledge of authenticity on every assignment. And if there is an honor code transgression, the council gathers to discuss possible consequences.
4. Use metacognition. Research shows that metacognition, a process sometimes described as “ thinking about thinking ,” can help students process their motivations, goals, and actions. With my ninth graders, I use a centuries-old resource to discuss moral quandaries: the play Macbeth . Before they meet the infamous Thane of Glamis, they role-play as medical school applicants, soccer players, and politicians, deciding if they’d cheat, injure, or lie to achieve goals. I push students to consider the steps they take to get the outcomes they desire. Why do we tend to act in the ways we do? What will we do to get what we want? And how will doing those things change who we are? Every tragedy is about us, I say, not just, as in Macbeth’s case, about a man who succumbs to “vaulting ambition.”
5. Bring honesty right into the curriculum. Teachers can weave a discussion of ethical behavior into curriculum. Ruff and many other teachers have been inspired to teach media literacy to help students understand digital plagiarism and navigate the widespread availability of secondary sources online, using guidance from organizations like Common Sense Media .
There are complicated psychological dynamics at play when students cheat, according to experts and researchers. While enforcing rules and consequences is important, knowing what’s really motivating students to cheat can help you foster integrity in the classroom instead of just penalizing the cheating.
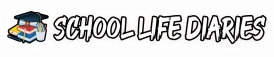
Consequences Of Cheating In Exams: Examples And Effects
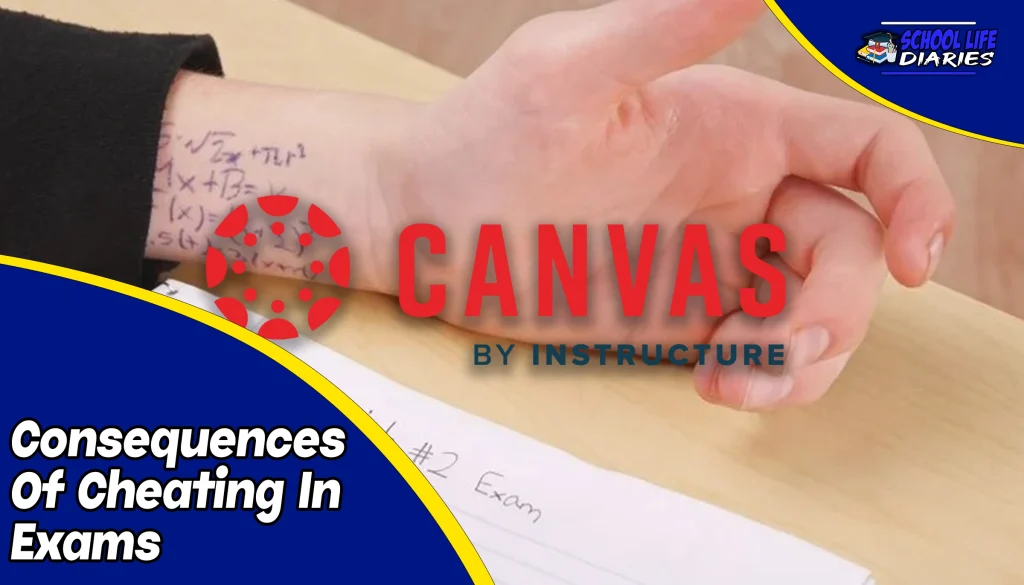
Cheating in exams is a serious issue that has far-reaching consequences for both individuals and society as a whole. It undermines the integrity of the education system, diminishes the value of qualifications, and erodes trust between students, teachers, and institutions.
Consequently, deserving students may miss out on opportunities such as scholarships or admission into competitive programs due to unfair competition from those who cheat.
Consequences of Cheating in College
Cheating in college exams can have serious consequences for students .
1. Cheating can lead to Class Failure
Academic dishonesty, such as cheating during exams, has the potential to result in students failing their classes. When students resort to cheating as a means to achieve better class performance, they not only compromise their academic integrity but also put their future at risk. The consequences of cheating can extend beyond immediate academic repercussions and have long-lasting effects on a student’s educational journey.
One of the primary academic consequences of cheating is the failure to grasp essential concepts and skills that are necessary for success in subsequent courses. Cheating stains a student’s reputation and raises questions about their character and reliability. A failed class due to cheating may leave a permanent mark on their academic transcript, potentially limiting opportunities for internships or postgraduate studies.
2. Legal consequences
The legal consequences of cheating in exams can have a long-lasting impact on one’s future. When employers or educational institutions discover that an individual has been involved in academic dishonesty, it raises questions about their character and ability to follow ethical practices. This can severely damage their reputation and hinder their chances of securing employment or admission into higher education programs. Moreover, having a criminal record for cheating can limit one’s opportunities for professional licensure or certification in certain fields where integrity is highly valued.
Cheating in exams not only undermines educational integrity but also carries significant legal consequences. Those who engage in such practices risk facing legal actions that can have far-reaching effects on their future prospects. It is important for individuals to understand the gravity of these consequences and make ethical choices when it comes to academic pursuits.
3. Cheating leads to Suspension and expulsion
Suspension and expulsion are disciplinary measures commonly imposed in response to dishonest practices that compromise the integrity of the educational system. When students engage in cheating during exams, they not only undermine their own learning but also violate the trust and fairness upon which academic institutions are built.
The consequences of suspension can be severe, as it involves a temporary removal from school for a specified period of time. During this period, students are barred from attending classes, participating in extracurricular activities , and accessing resources provided by the institution. This interruption in education can significantly impact a student’s academic progress and overall development.
Expulsion is an even more drastic repercussion of academic dishonesty. It entails a permanent dismissal from the educational institution, effectively ending any further enrollment or association with the school. Expulsion carries long-lasting consequences beyond just missing out on education opportunities. It tarnishes one’s academic record and reputation, making it difficult to gain admission into other institutions or pursue certain career paths that require a clean disciplinary history.
4. Academic reputation
A strong academic reputation is built upon a foundation of integrity and ethical conduct in educational institutions. Academic integrity refers to the honesty, trustworthiness, and ethical behavior expected from students and faculty members within an academic setting. It encompasses various aspects such as avoiding plagiarism, citing sources correctly, and conducting research with honesty and transparency.
Reputation management plays a crucial role in maintaining the academic reputation of an institution. Ethical behavior is essential for creating a conducive learning environment where knowledge is valued and respected. When students engage in cheating during exams, it undermines the principles of fairness and equal opportunities for all learners. To uphold academic integrity and manage their reputation effectively, educational institutions need to emphasize ethical behavior among their students through awareness campaigns, workshops on proper citation techniques, and clear guidelines on acceptable conduct during exams.
5. Cheating makes it hard to secure a Job
Cheating during exams not only undermines a student’s academic integrity but also raises serious concerns about their ethical values, which can have far-reaching consequences on their future career opportunities. Employers value honesty and integrity as fundamental qualities in potential employees, and discovering a candidate’s history of cheating can severely tarnish their chances of securing a job.
With competition for employment becoming increasingly fierce, employers are constantly seeking candidates who possess strong moral character and uphold the principles of fairness and trustworthiness. Consequently, those who succumb to the allure of cheating must grapple with the long-term impact on their personal growth, self-esteem, and ability to make ethically sound choices in future endeavors.
6. Cheating can cost you a scholarship
Scholarship opportunities can be lost as a result of engaging in dishonest practices during academic evaluations. Cheating not only undermines the integrity of the evaluation process but also has long-term consequences that can impact one’s future prospects. Many scholarships require applicants to demonstrate academic excellence and ethical conduct, making cheating a significant deterrent.
The impact on future prospects cannot be overstated. Scholarships provide financial support for students pursuing higher education and open doors to various opportunities such as internships, research projects, or study abroad programs. Cheating not only disqualifies individuals from immediate consideration but also diminishes their reputation and credibility over time. The impact extends beyond financial aid as it hinders access to valuable experiences and raises doubts about one’s abilities in competitive environments where integrity is paramount.
7. Creation of a false character
When students resort to creating a false character in order to cheat in exams, they not only undermine their own personal growth but also compromise the principles upon which academic institutions are built. The consequences of creating a false character extend beyond personal growth and affect the broader notion of academic integrity. It creates an unfair advantage for those who engage in such deceitful practices while disadvantaging honest students who have diligently worked towards achieving genuine success.
8. Cheating in school erodes your independence
When students resort to cheating in school, they are essentially relinquishing their independence by relying on illicit means to achieve academic success. By not putting in the necessary effort and taking shortcuts, students miss out on valuable opportunities for personal growth and development. In essence, cheating prevents them from learning essential skills such as critical thinking, problem-solving, and perseverance that are crucial for their future endeavors.
The impact of cheating on personal growth extends beyond the educational setting and can have severe consequences in adulthood. Students who habitually cheat may struggle with decision-making and lack confidence in their abilities to tackle challenges independently. This eroded sense of independence can hinder their professional development as they enter the workforce or pursue higher education.
9. Cheating in school prevents progress
Academic dishonesty in educational settings hinders the forward momentum of personal and intellectual growth, creating a stagnant environment where genuine progress becomes elusive. When students resort to cheating in school , they bypass the essential process of learning and understanding the material. By taking shortcuts, they deprive themselves of valuable opportunities to develop critical thinking skills, problem-solving abilities, and a deep understanding of the subject matter.
To prevent cheating and promote integrity in schools, academic institutions have implemented various measures such as academic integrity programs. These programs aim to educate students about the importance of ethical behavior in academia and provide resources for developing good study habits. By instilling a sense of responsibility and emphasizing honesty, these initiatives encourage students to take ownership of their education and learn through legitimate means.
10. Cheating in universities causes stress
Cheating in universities contributes to heightened levels of stress among students. The pressure to perform well academically can lead some students to resort to cheating as a means of achieving success. However, the consequences of such actions often result in increased stress levels. Students who cheat may experience constant anxiety and fear of getting caught, which can negatively impact their mental well-being.
One major factor contributing to the stress caused by cheating is the lack of effective stress management techniques. When students rely on cheating instead of developing their skills and knowledge, they miss out on opportunities for personal growth and self-improvement. This reliance on dishonest practices creates a cycle of stress and dependence, as students become increasingly anxious about maintaining their academic performance through unethical means.
11. Cheating in school brings Embarrassment
Embarrassment is a common emotion experienced by students who engage in dishonest practices within the educational system. Cheating in school not only undermines the integrity of the academic environment but also has significant psychological and social consequences for those involved.
When students resort to cheating, they often experience a profound sense of embarrassment, knowing that their actions go against established norms and values. The psychological impact of cheating-induced embarrassment can be profound. Students may feel guilty and ashamed for their dishonesty, leading to increased stress and anxiety levels. This emotional burden can affect their overall well-being and academic performance, as it becomes difficult to focus on learning when plagued by feelings of embarrassment.
12. Cheating is a form of disrespect.
One of the key aspects to consider when examining the act of cheating is the underlying disrespect it displays towards the educational system and its values. Cheating in exams is a form of disrespectful behavior that undermines the principles of academic integrity and moral values. This disrespectful behavior not only compromises their own personal growth but also diminishes the credibility and value of education as a whole.
Cheating reflects a lack of appreciation for the learning process and devalues the efforts put forth by both educators and students who adhere to ethical principles. It sends a message that shortcuts and deceitful practices are acceptable means to achieve success, undermining the foundational basis upon which education stands.
Effect of Cheating on the Learning Process
The impact of dishonesty during exams can have significant implications for the overall educational experience. Cheating not only undermines the integrity of the learning process but also has detrimental effects on motivation. When students resort to cheating, they are essentially bypassing the opportunity to engage with the material and develop a deep understanding of the subject matter. This lack of genuine effort and comprehension can lead to a decrease in intrinsic motivation, as students become more focused on achieving high grades rather than truly mastering the content
Examples of cheating in college
Cheating in college can take various forms, including copying from fellow students during exams or assignments. Another example of cheating is when someone pays another person to write essays or papers for them.
1. Copying from fellow students
Copying from fellow students during exams undermines the integrity of the assessment process and compromises the fairness of grading. This act not only has serious consequences for the individuals involved but also poses ethical implications and challenges academic integrity. When students resort to copying, they disregard the importance of genuine learning and academic growth. Consequently, their education becomes superficial and lacks the necessary depth that would prepare them for future challenges.
Copying from fellow students during exams not only has immediate consequences for those involved but also raises important ethical concerns regarding academic integrity. The act itself undermines genuine learning opportunities and inhibits personal growth in critical areas such as problem-solving and independent thinking. Moreover, it disrupts fairness in grading processes and erodes trust within educational institutions.
2. When someone writes essays or papers for you.
Outsourcing the writing of essays or papers undermines the authenticity of academic work and hinders the development of critical thinking skills and independent research abilities. When someone else writes an essay or paper on behalf of a student, it not only compromises their academic integrity but also deprives them of valuable learning opportunities.
Plagiarism detection tools have become increasingly sophisticated in recent years, making it easier for educators to identify instances of outsourced writing. The prevalence of such unethical practices raises serious ethical implications within educational institutions.
Outsourcing the writing of essays or papers has severe consequences on both individual students’ academic growth as well as broader educational systems’ integrity. It undermines authenticity by compromising academic rigor while hindering critical thinking skills and independent research abilities. The use of plagiarism detection tools serves as a deterrent against such practices but cannot completely eradicate them entirely.
3. Using textbooks, notes, and formula lists on exams
Utilizing textbooks, notes, and formula lists during examinations can significantly impact the educational integrity of the assessment process while potentially hindering the development of critical thinking skills and a deep understanding of the subject matter. Academic dishonesty is a serious concern in educational institutions, as it goes against the principles of fairness and equality.
Allowing students to rely on external resources during exams undermines the purpose of assessing their knowledge and proficiency in a particular subject. When students have access to textbooks, notes, or formula lists during exams, they may rely solely on these materials instead of actively engaging with the course content. This reliance not only diminishes their ability to think critically but also prevents them from fully comprehending complex concepts. Exams are designed not just to test factual recall but also to assess students’ analytical skills and their ability to synthesize information.
Allowing students to use textbooks, notes, and formula lists during examinations can compromise educational integrity by promoting academic dishonesty. It limits opportunities for critical thinking development and impedes a thorough grasp of course material. To ensure an effective assessment process that fosters genuine learning outcomes, it is crucial for educational institutions to discourage such exam preparation methods that undermine intellectual growth and hinder academic progress.
4. Collaborating in tests or exams without permission
Collaborating with others during tests or exams without proper authorization can compromise the integrity of the assessment process and undermine the principles of fairness and equality in education. Collaborative learning, when properly facilitated and authorized by instructors, can be a valuable educational tool that promotes critical thinking and problem-solving skills.
Collaborating in tests or exams without proper authorization is detrimental to both individuals involved and the integrity of education as a whole. It is important for students to understand that academic success should be based on one’s own efforts and abilities rather than relying on unauthorized collaboration. Upholding ethical behavior is crucial for maintaining fairness, equality, and credibility within educational institutions.
5. Copying from Online Tutors
Online tutoring has become increasingly popular due to its convenience and accessibility. Students can seek help from qualified tutors anytime, anywhere, and access a wide range of study materials. While online tutoring offers numerous benefits for students, it is important to recognize the potential risks associated with this practice. Copying answers directly from online tutors not only undermines the purpose of examinations but also violates academic integrity. Plagiarism prevention strategies play a crucial role in addressing this issue.
Educational institutions should prioritize implementing strict policies against cheating and plagiarism, educating students about the importance of academic honesty, and providing resources for developing effective study skills. Implementing plagiarism prevention strategies and comprehensive academic integrity programs can help instill a sense of responsibility among students while promoting ethical conduct in their educational journey.
Solutions to cheating in school
In order to address the issue of cheating in school, it is crucial to educate students on the importance of honesty and integrity. This can be done through regular discussions and workshops that highlight the negative consequences of cheating and emphasize the value of ethical behavior.
1. Educating students on the importance of honesty
To instill principles of honesty in students, it is imperative to educate them about the significance of integrity during examinations. Emphasizing the importance of ethical behavior in academic settings helps foster a culture of integrity and promotes academic honesty. By educating students on the consequences of cheating and highlighting the value of honesty, educational institutions can create an environment where students understand the long-term benefits of maintaining their integrity.
Educating students about the importance of ethical behavior lays the foundation for fostering a culture of integrity within educational institutions. When students are aware that dishonesty can have serious repercussions not only on their academic journey but also on their personal growth and development, they are more likely to adhere to guidelines promoting honesty. Promoting academic honesty goes beyond just preventing cheating; it encourages critical thinking skills, self-discipline, and intellectual growth.
2. Creating anti-cheating pledges
Creating anti-cheating pledges can be a powerful tool in addressing the issue of cheating in exams. These pledges serve as a visible reminder for students to uphold their integrity and make ethical choices when faced with academic challenges. By signing such a pledge, students publicly declare their commitment to honest practices, creating awareness not only among themselves but also among their peers. This collective effort towards maintaining academic honesty can have a profound impact on reducing incidents of cheating.
Creating anti-cheating pledges is an effective strategy for discouraging cheating in exams as it creates awareness about its consequences, promotes student accountability, and builds trust within educational institutions. By encouraging students to actively commit themselves to uphold academic honesty through these pledges, a culture of integrity and personal responsibility can be fostered. Implementing such measures not only deters cheating but also instills valuable life skills and values in students that extend beyond the academic realm.
3. Instructors changing the definition of success
An alternative approach employed by instructors involves redefining the criteria for achieving success within an academic context. Rather than solely focusing on exam performance, instructors are changing expectations and placing greater emphasis on alternative assessments to evaluate students’ understanding and knowledge.
This shift in mindset aims to reduce the pressure that often leads to cheating and encourages students to engage more deeply with the material. By changing the definition of success, instructors aim to create a learning environment that focuses on growth and understanding rather than simply memorizing information for exams. This change has a significant impact on students as it encourages them to develop critical thinking skills, problem-solving abilities, and a deeper comprehension of the subject matter.
Cheating in exams has serious consequences in college. Students who are caught cheating may face disciplinary actions such as receiving a failing grade for the exam or even being expelled from the institution. To combat cheating, schools can implement various solutions. Firstly, they can promote a culture of academic honesty by educating students about the negative consequences of cheating and emphasizing the importance of ethical conduct.
Schools can implement strict monitoring measures during exams to deter and detect instances of cheating. This can include using proctors or invigilators during exams or employing technology tools such as anti-plagiarism software to identify plagiarized content. By promoting academic integrity and implementing effective preventive measures, we can ensure that exams serve their intended purpose – assessing students’ true abilities and preparing them for success in their future endeavors without resorting to dishonest practices.
Related Posts
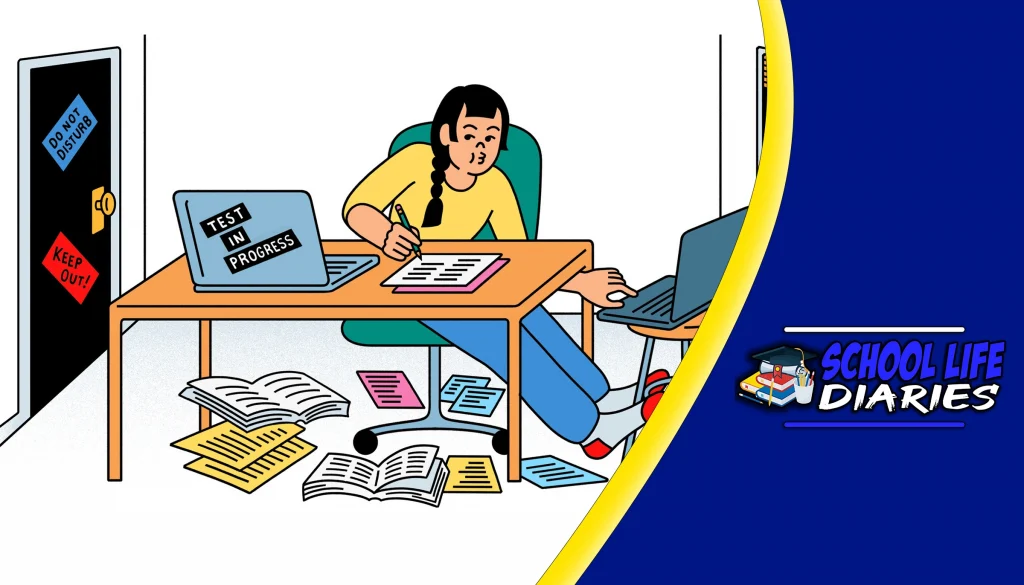
Can Online Exams Detect Cheating?
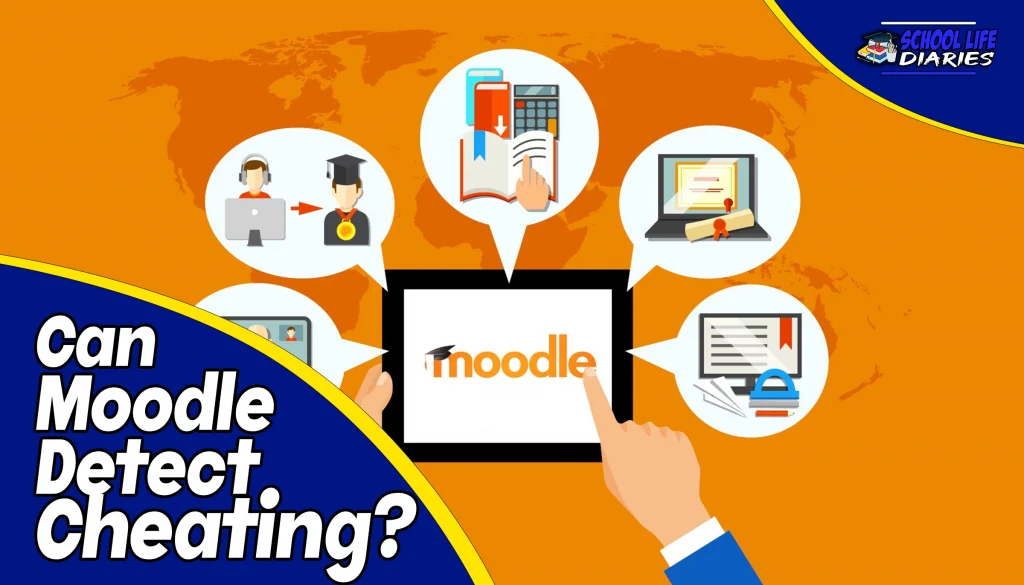
How Can Moodle Help Detect and Prevent Cheating?
Leave a comment cancel reply.
Your email address will not be published. Required fields are marked *
Save my name, email, and website in this browser for the next time I comment.
School Life Diaries
Our website provides interesting and informative content related to school life, teachers, and students. Our articles are written by experienced professionals and provide valuable insights into the world of education.
Whether you’re a student looking for advice or a teacher searching for new ideas, our website is a great resource for anyone interested in learning more about the school experience.
- Teachers Tote
Legal Pages
- Privacy Policy
- Terms of Use
Social Media
Would you like to explore a topic?
- LEARNING OUTSIDE OF SCHOOL
Or read some of our popular articles?
Free downloadable english gcse past papers with mark scheme.
- 19 May 2022
How Will GCSE Grade Boundaries Affect My Child’s Results?
- Akshat Biyani
- 13 December 2021
The Best Free Homeschooling Resources UK Parents Need to Start Using Today
- Joseph McCrossan
- 18 February 2022
What are the consequences of cheating in exams?
- August 23, 2022
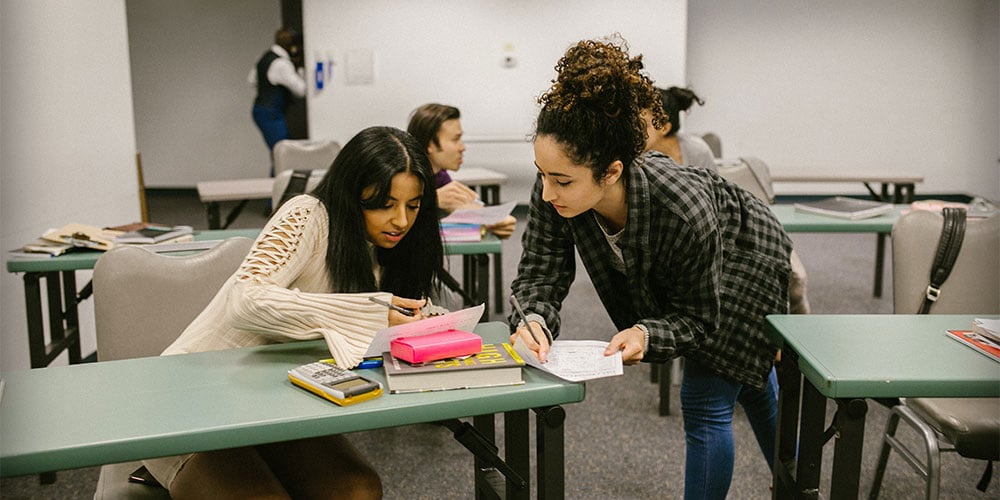
What is cheating?
How to cheat in an exam, what are the consequences of cheating in schools.
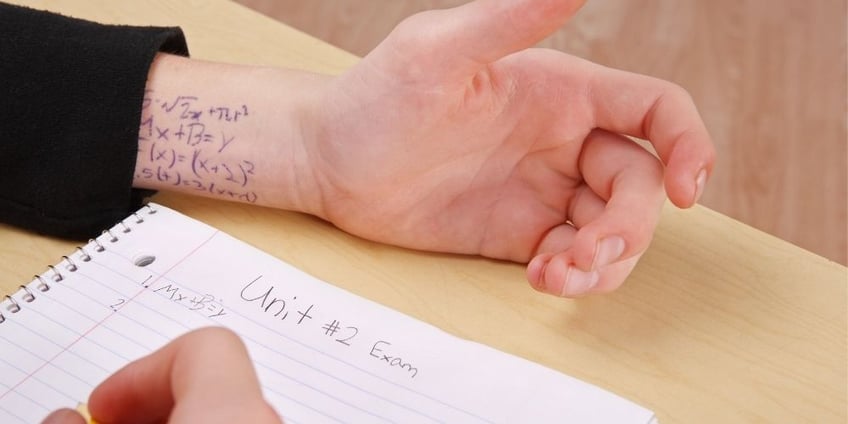
Cheating definition:
Cheating is the act of obtaining advantages or rewards without following the rules that apply to others. Examples include cheating in a board game or in a test at school.
What causes cheating?
- The problem is related to the issue of merit and rising competition. Students have to fight for the best possible grades in order to get into the most valued courses at university. Cheating in an exam is seen as an easy solution.
- This spirit of competition usually translates into the idea that all is fair, that only the result counts. But this idea is challenged by well-known sportsmen and women who are regularly involved in doping cases, even though they are role models for the younger generation . 🚴🏼
- When a child is under family pressure and is not allowed to make mistakes , or there is no room for failure, fraud and cheating may appear to be the only way to live up to expectations.
- Finally, a student may start cheating to join a group of peers or friends who encourage this malpractice, to try and rebel or to challenge the school system.
But seriously, do you really think I'm going to encourage your child to commit a crime? Certainly not.
- We all know the trick of the scientific calculator on which it is possible to record formulas or even definitions (but this does not work in French or English lessons!)
- A cheat sheet can be written behind the label on the water bottle or hidden in the bottom of a pencil case
- The mobile phone wallpaper acts as a notepad, which we pretend to use to look at the time 👀
All these tricks are well known classics that sometimes work during an assessment but are incredibly risky, and evidence of malpractice and cheating in exams.
Online tools
During school isolation periods in the past few years, even the most serious students enjoyed the thrill of cheating on online exams, which were much more difficult to monitor by teachers and schools.
This may have been collaborative fraud via chat groups with classmates on platforms such as Snapchat or WhatsApp. Others even paid more advanced candidates to take the exams for them. Or, more simply, they took their tests online with a second computer open on the Google homepage.
But keep in mind that whether online or face-to-face, the cheating student still faces the same risk of punishment! 👮
Cases of cheating in class
If a student hasn’t managed to revise , wants to cheat and is caught in class, it can seriously damage his or her results, as well as reputation or even place at the school. They could get a zero on their assessment, contact home or even detentions and exclusion from the school.
Parents and guardians even make an agreement with the school before their child starts to never cheat or be involved in any malpractice, which schools are serious about throughout their time there.
What are the penalties for exam fraud?
National qualifications such as GCSEs , A-Levels and exams at university are all official exams recognised by the government. If an exam invigilator catches your child cheating in the examination room, a verbal and written report will be drawn up and an investigation will begin.
Depending on the seriousness of the situation and the exam board or school, cheating at the exam can lead to different types of sanctions:
- Reprimand. This is a disciplinary sanction, a call to order without too serious consequences, usually reserved for internal school exams only (not National qualifications where consequences are more serious)
- Removal from the course or overall diploma, sometimes resulting in having to restart completely
- Immediate contact to the exams regulation authority, JCQ , who monitor most exam boards like AQA or Edexcel
- No awarded mark, grade, GCSE, A-Level or points for the course at university in that subject (usually named an ‘Ungraded’ or U)
- Sometimes a ban on taking any National exams for a number of years
- Sometimes, but infrequently, a ban on enrolling into a higher education institution for 5 years
Exam malpractice is rising; in 2019 Ofqual reported an 11% rise in malpractice reports, and this includes teachers and schools helping students to cheat!
Can you pass your driving test if you cheat at your exam?
I've always heard that a student who is caught cheating on the exam is then likely to be barred from taking any other official exam, including the assessment to get their UK driving licence. 🚗
Today, I can officially tell you that this is an urban legend. As the driving licence is not linked with our education certification system, it is not affected by the sanctions. But, cheating in an exam (and getting caught) can change your mindset to all other exams you go on to sit for the worse.
But rather than having to ask yourself this question, the best thing to do is to book some lessons online with our tutors. They will help your child to prepare well for challenging exams like GCSEs, without the need to cheat!
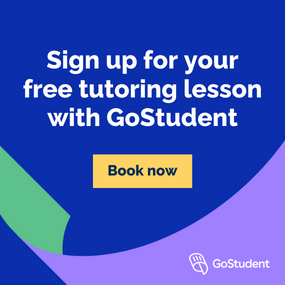
Popular posts
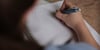
- By Guy Doza
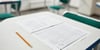
- By Akshat Biyani
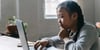
- By Joseph McCrossan
- In LEARNING TRENDS
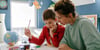
4 Surprising Disadvantages of Homeschooling
- By Andrea Butler
The 12 Best GCSE Revision Apps to Supercharge Your Revision
More great reads:.
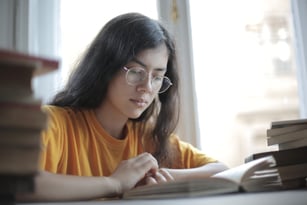
Advice From a Teacher: How Can I Help My Child If They Fail Their Mock Exams?
- By Natalie Lever
- January 9, 2024
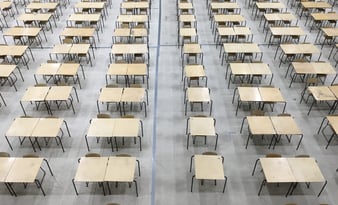
What Are Mock Exams and Why Are They Important?
- January 8, 2024
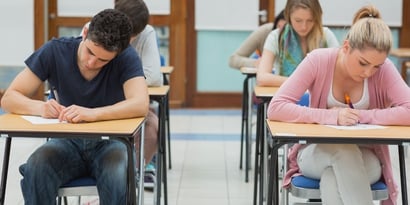
SQA Results Day 2023: Important Information About How to Get Scottish Exam Results
- By Sharlene Matharu
- August 3, 2023
Book a free trial session
Sign up for your free tutoring lesson..
What Are the Negative Impacts of Cheating on Students in College?
February 8, 2023 | Blog Articles | Share: Twitter Facebook Linkedin
Cheating on exams in college has never been a more serious problem, with scandals in institutions ranging from Harvard to the U.S. Naval Academy . The negative impacts of academic dishonesty affect institutions, administrators, professors, and test-takers alike, devaluing their degrees and leading to possible legal and financial repercussions . Oftentimes the testing industry doesn’t talk about negative repercussions that can affect students if a culture of cheating persists in an institution. But because of the negative outcomes that can result from cheating, it’s vital for institutions to invest in security measures to prevent and detect cheating on exams.
In this article, we will explore the reasons test-takers have for cheating on exams and how it affects those test-takers. We’ll also look at ways institutions can mitigate the prevalence of cheating and prevent unwanted fallout.
Moral Disengagement and Cheating Culture
There are a number of methods that test-takers employ to gain an unfair advantage on exams. They also have a number of motives for doing so. These motives can range from the personal to the professional. We’ll focus on two of the major reasons here.
Academic Pressure
Because of the high stakes of many exams, test-takers may feel increased pressure to do well. They may believe—and it may well be true—that passing an exam is necessary for them to continue with their major, graduate on time, or even get a job.
The belief that failing an exam could lead to negative consequences can cause test-takers to feel enormous pressure to cheat on exams in order to avoid those consequences. A recent study by Turnitin shows that 90% of students believe their peers cheat on exams. Though the same study says students understand how academic integrity is crucial for the assessment to be meaningful, they are still tempted to cheat because they believe that cheating is common.
Moral Disengagement
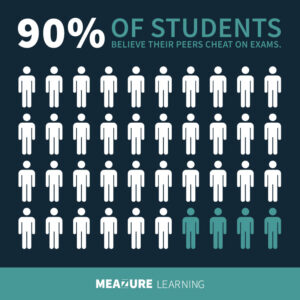
This perception of a culture of cheating can lead test-takers to believe they will be at a disadvantage if they do not cheat, since so many of their peers are. A culture of cheating, combined with this belief that not cheating puts a test-taker at a disadvantage, can lead to moral disengagement .
Moral disengagement is a process by which a person—in this case, the test-taker—gradually disengages from their personal morality. This process can lead to a test-taker cheating on exams, even though they believe cheating to be wrong. When test-takers perceive a culture of cheating, they see the value of the exam being lowered. In combination with their perception that their peers are cheating on the exam, this devaluation can lead them to put less moral weight on the concept of cheating, making it easier for them to engage in cheating behavior.
The same Turnitin study referenced previously asked students and faculty to determine whether certain behaviors counted as cheating. The study found that 18% of students did not think the use of unauthorized materials on an exam counted as cheating. A staggering 31% of students believed that hiring someone to write an essay for them is not cheating or is only somewhat cheating. Whether or not a student views a behavior as cheating can have a direct impact on how likely they are to engage in behavior that may lead to a culture of cheating at an institution.
How Test-Takers Can Be Affected by Cheating on Exams
The impacts of cheating on test-takers can be varied and consequential, both on those who engage in cheating behavior and those who don’t. For those who do cheat, the most obvious consequences are academic. Cheating on an exam can result in failing that test or—in many cases—failing the class entirely. Academic consequences can go beyond one class as well, sometimes leading to suspension or expulsion from the program or the institution entirely.
Impacts on Students Who Cheat
If allowed to continue, cheating behaviors can be habit-forming. Even if a test-taker feels that cheating is wrong, they may be tempted to engage in cheating behavior on a specific exam or assignment that is of significant value to their degree or program. If they give in to that temptation, it will be easier to do so the next time. Like many forms of dishonesty, cheating can be a slippery slope that may lead to more substantial behaviors in the long run.
When test-takers who cheat on exams are not found out, the effects can spread out into the wider world, beyond the test, class, and college degree. When a test-taker who cheats passes their exam, there can be dire consequences for their employment. They may find themselves hired for a job they are unqualified for, which could lead to a variety of unwanted results. Being unqualified could cost them a promotion, an assignment, or even their job. From the employer’s perspective, hiring an unqualified candidate leads to increased acquisition and firing costs, requiring them to replace incompetent workers. It could also lead to legal and financial repercussions for both employee and employer in a high-stakes job in an industry like medicine or finance, ultimately eroding the employer’s confidence in the exam program.
“The impacts of cheating on a program’s credibility and the students in that program can be devastating, but a robust academic honesty policy and clear communication of expectations can help prevent a culture of cheating. “ Ashley Norris, PhD, SVP, Strategic Communications and Policy
According to a recent study conducted by the ATP Workforce Skills Credentialing Security and Privacy Committee, over 80% of employers surveyed agreed that it is very important to be able to verify credential authenticity. If there is a history of test-takers cheating on a particular exam or a particular program’s exams, employers are much less likely to trust in that program’s credentials, which ends up hurting everyone associated with that program—test-takers and instructors included.
Impacts on Students Who Don’t Cheat
The effects of cheating don’t only touch those who engage in cheating behavior. The reputational damage done to a program through a culture of cheating affects everyone in the program. In addition, students who don’t cheat can face unique consequences when cheating is allowed or perceived to be allowed.
When faculty believe there is a culture of cheating, they adjust their policies and responses accordingly. Often instructors will begin to police student behavior much more strictly when there is a perceived culture of cheating. This can mean putting undue pressure on academically honest students, causing them unnecessary stress and anxiety. This can negatively affect their performance on the exam and in the program as a whole.
When students believe there is a culture of cheating, it can affect the way they view their program and their participation in it. With enough of a change in this view, students may leave the program entirely. Students who believe their peers regularly cheat may begin to feel they are at a disadvantage if they do not also cheat. It can also lead them to question the accuracy of their own assessments. They may begin to feel that in order to succeed in their program, they also need to cheat. This can lead to the moral disengagement we discussed previously.
Strategies for Reducing Cheating on Exams
While the effects of cheating are numerous and can reach test-takers and institutions alike, there are ways to mitigate the incidents of cheating and, therefore, the consequences of that attempted cheating. Here are some strategies to consider:
- Mitigation Strategy 1: Evidence shows that the mere inclusion of a human proctor can lessen incidents of cheating. Adding a human element lets students know you take academic honesty seriously.
- Mitigation Strategy 2: Be proactive in communicating your program’s misconduct policies. Knowing that an institution, instructor, or exam deliverer is aware of the potential for cheating can both curtail possible cheating and assure honest students that those who attempt to cheat are being watched and—hopefully—caught.
- Mitigation Strategy 3: Be clear in your communication, both verbal and written, about what test-takers can expect on exam day. A simple reminder that there is a process for the exam indicates that the instructor or exam administrator cares about the outcome of their exam.
Cheating and other forms of academic dishonesty may be on the rise, but a proactive approach, a clearly communicated academic dishonesty policy, and an open discussion of consequences of cheating can help. With these measures in place, and an online proctoring platform that works with you to prevent cheating rather than punish it, your program can avoid the negative impacts discussed previously. Your test-takers will have an overall better experience, and your exam program will maintain its integrity.
Learn more about how to prepare your test-takers with better communication by watching our recent webinar on the topic.
- ZW-HA, Harare
- ZW-HA, Mount Pleasant
- VN-HN, Hanoi
- VN-SG, Ho Chi Minh
- VN-SG, Ho Chi Minh City
- VE-A, El Rosal Chacao, Caracas
- GB-LND, Bethnal Green
- GB-CHS, Chesterfield
- GB-EDH, Edinburgh
- GB-GRE, Eltham
- GB-ESS, Essex
- GB-SCB, Glasgow
- GB-SOM, Highbridge
- GB-BNH, Hove
- GB-LND, Ilford, London
- GB-HLD, Inverness
- GB-LDS, Leeds
- GB-LIV, Liverpool
- GB-LND, London
- GB-MDB, Middlesborough
- GB-NWP, Newport
- GB-CAM, Peterborough
- GB-LAN, Preston
- GB-RDG, Reading
- GB-GAT, The River Tyne
- GB-CHS, Warrington
- GB-WLV, Wolverhampton
- AE-AZ, Abu Dhabi
- AE-AJ, Ajman
- AE-AZ, Al Ain
- AE-DU, Dubai
- AE-SH, Sharjah
- UA-12, Dnipro
- UA-30, Kyiv
- UA-46, Lviv
- TR-34, Istanbul
- TR-34, Maslak
- TN-11, Tunis
- TT-ARI, Arima
- TT-CHA, Chaguanas
- TT-SJL, Champs Fleurs
- TH-10, Bangkok
- TH-12, Muang Nonthaburi
- TH-10, Sathorn
- TZ-01, Dar Es Salaam
- TW-TPQ, Hsinchu
- TW-TPQ, Taichung City
- TW-TPQ, Taipei
- CH-GE, Geneva
- CH-VD, Lausanne
- CH-ZH, Zurich
- SE-O, Gothenburg
- SE-M, Malmo
- LK-11, Dehiwala-Mount Lavinia
- ES-B, Barcelona
- ES-BI, Bilbao – Bizkaia
- ES-M, Madrid
- ES-SE, Sevilla
- ES-VA, Valladolid
- ZA-WC, Cape Town
- ZA-NL, Durban
- ZA-GT, Johannesburg
- SI-061, Ljubljana
- SK-BL, Bratislava
- SG-X1~, Singapore
- RS-00, Novi Beograd
- SA-02, Jeddah
- SA-04, Khobar
- SA-03, Madinah
- SA-01, Riyadh
- LC-LC, Castries
- KN-03, Basseterre
- RO-B, Bucharest
- QA-DA, Doha
- PT-11, Lisboa
- PL-KP, Bydgoszcz
- PL-MZ, Warsaw
- PH-07, Cebu City
- PH-00, Makati City
- PH-00, Mandayulong
- PH-00, San Juan
- PE-LIM, Lima
- PALESTINIAN TERRITORY, OCCUPIED, Al-Bireh
- PK-IS, Islamabad
- PK-SD, Karachi
- PK-PB, Lahore
- OM-MA, Muscat
- NO-12, Bergen
- NO-03, Oslo
- NO-16, Trondheim
- NG-FC, Abuja
- NG-OY, Ibadan
- NG-LA, Ikeja
- NG-KD, Kaduna
- NG-LA, Lagos
- NG-RI, Port Harcourt
- NG-LA, Yaba, Lagos
- NZ-AUK, Auckland
- NZ-WGN, Christchurch
- NZ-AUK, Ponsonby
- NZ-WGN, Wellington
- NL-ZH, Barendrecht
- NL-NB, Den Bosch
- NL-NH, Diemen
- NL-FR, Drachten
- NL-NB, Eindhoven
- NL-LI, Maastricht
- NL-GE, Nijmegen
- NP-BA, Kathmandu
- NP-BA, Lalitpur
- NA-KH, Windhoek
- MN-1, Ulaanbaater
- MX-DIF, Col. San Jose Insurgentes, Del. Benito JuÁrez
- MX-DIF, Mexico City
- MX-NLE, Monterrey
- MU-MO, Moka
- MU-QB, Quatre Bornes
- MY-14, Kuala Lumpur
- MY-13, Kuching
- MY-10, Petaling Jaya
- MK-X1~, Skopje
- LT-VL, Vilnius
- LB-BA, Beirut
- LV-RI, Riga
- KW-HA, Hawally
- KW-KU, Kuwait (Authorized Testers Only)
- KW-JA, Kuwait City
- KW-KU, Safat
- KW-HA, Salmiya
- KR-11, Seoul
- KE-110, Mombasa
- KE-110, Nairobi
- KE-110, Nakuru
- KAZAKHSTAN, Almat
- JO-AM, Amman
- JO-IR, Irbid
- JO-AM, Shmeisani
- JP-13, Fussa (Authorized Testers Only)
- JP-14, Kanagawa
- JP-13, Minato-Ku
- JP-01, Misawa
- JP-47, Okinawa (Authorized Testers Only)
- JP-13, Tokyo
- JP-14, Yokosuka (Authorized Testers Only)
- JM-01, Kingston
- IT-78, Catanzaro
- IT-MI, Milano
- IT-72, Naples
- IT-36, Porcia
- IT-62, Rome
- IT-72, Sigonella (Authorized Testers Only)
- IL-TA, Tel Aviv
- IE-C, Bishopstown, Cork
- IE-D, Dublin
- IE-G, Galway
- IE-KE, Kildare
- IE-D, Sandyford
- ID-JK, Jakarta
- ID-JK, Jakarta Pusat
- ID-JK, North Jakarta
- IN-UP, Agra
- IN-GJ, Ahmedabad
- IN-PB, Amritsar
- IN-MH, Aundh, Pune
- IN-MH, Auranggabad
- IN-KA, Bangalore
- IN-MH, Belapur
- IN-KA, Bengaluru
- IN-MP, Bhopal
- IN-OR, Bhubaneshwar
- IN-KL, Calicut
- IN-CH, Chandigarh
- IN-TN, Chennai
- IN-TN, Coimbatore
- IN-MH, Dahisar
- IN-UL, Dehradun
- IN-DL, Delhi
- IN-MH, Dharampeth, Nagpur
- IN-TN, Dindigul
- IN-TN, Erode
- IN-HR, Gurugram
- IN-AS, Guwahati
- IN-MP, Gwalior
- IN-HR, Hissar
- IN-WB, Howrah
- IN-AP, Hyderabad
- IN-MP, Indore
- IN-RJ, Jaipur
- IN-PB, Jalandhar
- IN-JK, Jammu
- IN-UP, Kanpur
- IN-HR, Karnal
- IN-MH, Kolhapur
- IN-WB, Kolkata
- IN-UP, Lucknow
- IN-PB, Ludhiana
- IN-TN, Madurai
- IN-MH, Maharashtra
- IN-KA, Mangalore
- IN-KA, Manglore
- IN-MH, Mumbai
- IN-KA, Mysore
- IN-TN, Nagercoil
- IN-MH, Nagpur
- IN-MH, Nashik
- IN-DL, New Delhi
- IN-UP, Noida
- IN-GA, Panjim
- IN-BR, Patna
- IN-PY, Puducherry
- IN-MH, Pune
- IN-CT, Raipur
- IN-GJ, Rajkot
- IN-TN, Ramnad
- IN-JH, Ranchi
- IN-TN, Salem
- IN-GJ, Surat
- IN-MH, Thane
- IN-TN, Theni
- IN-KL, Thiruvananthapuram
- IN-TN, Tirunelveli
- IN-TN, Trichy
- IN-AP, Vadodara
- IN-UP, Varanasi
- IN-GJ, Vastrapur, Ahmedabad
- IN-AP, Vijayawada
- IN-AP, Visakhapatnam
- IS-0, Reykjavik
- HU-BU, Budapest
- HK-HK, Kowloon
- HK-HK, Kowloon Mong Kok
- HK-HK, Kwun Tong, Kowloon
- HT-OU, Port Au Prince
- GY-DE, Demerara
- GT-GU, Guatemala City
- GD-03, St. George’s
- GR-73, Komotini
- GR-54, Thessaloniki
- GH-AA, Accra
- GH-AA, Dansoman-Accra
- DE-BE, Berlin
- DE-NW, Bonn
- DE-HE, Darmstadt
- DE-HE, Frankfurt
- DE-SN, Leipzig
- DE-BY, Munich
- DE-BW, Neckarsulm
- DE-RP, Ramstein
- DE-BB, Spangdahlem (Authorized Testers Only)
- DE-BW, Stuttgart
- GA-1, Libreville
- FR-33, Bordeaux
- FR-67, Strasbourg
- FI-ES, Espoo
- FI-ES, Helsinki
- ET-AA, Addis Ababa
- SV-SS, San Salvador
- EG-C, 6 October City
- EG-C, Cairo
- EG-LX, Luxor
- EG-DK, Mansoura
- EG-C, Nasr City, Cairo
- EG-C, Sheraton Elmatar
- EC-P, Quito
- DO-01, Santo Domingo De Guzman
- CI-01, Abidjan
- CZ-PR, Prague
- CY-02, Limassol
- CY-01, Strovolos
- CO-CUN, Bogota
- CN-11, Beijing
- CN-22, Changchun
- CN-43, Changsha
- CN-32, Changzhou
- CN-51, Chengdu
- CN-50, Chongqing
- CN-21, Dalian
- CN-44, Foshan
- CN-35, Fuzhou
- CN-44, Guangzhou
- CN-52, Guiyang
- CN-23, Ha’erbin
- CN-46, Haikou
- CN-33, Hangzhou
- CN-34, Hefei
- CN-11, Hohhot
- CN-15, Huhot
- CN-37, Jinan
- CN-33, Jinhua
- CN-53, Kunming
- CN-62, Lanzhou
- CN-41, Luoyang
- CN-36, Nanchang
- CN-21, Nanjing
- CN-13, Nanning
- CN-33, Ningbo
- CN-11, Ningxia
- CN-35, Putian
- CN-37, Qingdao
- CN-11, Quanzhou
- CN-31, Shanghai
- CN-21, Shenyang
- CN-44, Shenzhen
- CN-21, Suzhou
- CN-14, Taiyuan
- CN-33, Taizhou
- CN-12, Tianjin
- CN-65, Urumqi
- CN-21, Wenzhou
- CN-42, Wuhan
- CN-32, Wuxi
- CN-61, Xi’an
- CN-35, Xiamen
- CN-64, Yinchaun
- CN-41, Zhengzhou
- CN-44, Zhuhai
- MB, Brandon
- BC, Burnaby
- AB, Calgary
- BC, Castlegar
- PE, Charlottetown
- NL, Corner Brook
- BC, Courtenay
- NS, Dartmouth
- AB, Edmonton
- AB, Fort Mcmurray
- NS, Halifax
- ON, Hamilton
- BC, Kamloops
- BC, Kelowna
- ON, Kitchener
- AB, Lethbridge
- AB, Medicine Hat
- ON, Mississauga
- BC, Nanaimo
- SK, Prince Albert
- BC, Richmond
- BC, Salt Spring Island
- SK, Saskatoon
- ON, Scarborough
- NL, Stephenville
- ON, Toronto
- BC, Vancouver
- BC, Victoria
- QC, Westmount
- YT, Whitehorse
- ON, Windsor
- CM-CE, Yoaunda
- BG-22, Sofia
- BR-MG, Belo Horizonte
- BR-DF, Brasilia
- BR-BA, Pituba, Salvador
- BR-RJ, Rio De Janeiro
- BR-SP, Sao Paulo
- BW-SE, Gaborone
- BO-C, Cochabamba
- BO-L, La Paz
- BO-S, Santa Cruz
- BO-S, Santa Cruz De La Sierra
- BM-BM, Paget
- BE-BRU, Brussels
- BB-01, Christ Church
- BD-13, Dhaka
- BH-13, Al Seef
- BH-13, Manama
- BS-AK, Nassau
- AZ-BA, Baku
- AT-9, Vienna
- AT-9, Wien (Vienna)
- AU-SA, Adelaide
- AU-VIC, Melbourne
- AU-NSW, Parramatta
- AU-WA, Perth
- AU-NSW, Seven Hills
- AU-NSW, Sydney
- AU-SA, Thebarton
- AU-VIC, West Melbourne
- AM-ER, Yerevan
- AR-B, Martinez, Buenos Aires
- AL-TR, Tirane
- WY, Cheyenne
- WY, Green River
- WY, Laramie
- WY, Riverton
- WY, Sheridan
- WI, Appleton
- WI, Elkhorn
- WI, Green Bay
- WI, Kenosha
- WI, Lacrosse
- WI, Madison
- WI, Milwaukee
- WI, Oshkosh
- WI, Pewaukee
- WI, Rhinelander
- WI, Stevens Point
- WI, Superior
- WI, Waukesha
- WI, Whitewater
- WI, Wisconsin Rapids
- WV, Beckley
- WV, Fairmont
- WV, Princeton
- WV, South Charleston
- WA, Bellingham
- WA, Ellensburg
- WA, Federal Way
- WA, Liberty Lake
- WA, Longview
- WA, Port Angeles
- WA, Walla Walla
- VA, Abingdon
- VA, Alexandria
- VA, Annandale
- VA, Ashburn
- VA, Chantilly
- VA, Fairfax
- VA, Falls Church
- VA, Fredericksburg
- VA, Harrisonburg
- VA, Herndon
- VA, Manassas
- VA, Midlothian
- VA, Norfolk
- VA, Richmond
- VA, Roanoke
- VA, Sterling
- VA, Tazewell
- VA, Virginia Beach
- VI, St Croix
- VI, St Thomas
- VT, Lyndonville
- UT, Cedar City
- UT, Monticello
- UT, Salt Lake City
- UT, St George
- TX, Abilene
- TX, Amarillo
- TX, Arlington
- TX, Beaumont
- TX, Bedford
- TX, Brownsville
- TX, Brownwood
- TX, College Station
- TX, Commerce
- TX, Corpus Christi
- TX, El Paso
- TX, Fairfield
- TX, Fort Worth
- TX, Ft Worth
- TX, Galveston
- TX, Glen Rose
- TX, Harlingen
- TX, Houston
- TX, Huntsville
- TX, Killeen
- TX, Lewisville
- TX, Lubbock
- TX, Mount Pleasant
- TX, Nacogdoches
- TX, Port Lavaca
- TX, Round Rock
- TX, San Antonio
- TX, San Marcos
- TX, Stephenville
- TX, Texarkana
- TX, Victoria
- TX, Weatherford
- TN, Chattanooga
- TN, Clarksville
- TN, Cookeville
- TN, Gallatin
- TN, Jackson
- TN, Memphis
- TN, Murfreesboro
- TN, Nashville
- SD, Brookings
- SD, Sioux Falls
- SD, Spearfish
- SC, Clemson
- SC, Columbia
- SC, Graniteville
- SC, Greenville
- SC, North Charleston
- SC, Rock Hill
- PR, San Juan
- PA, Allentown
- PA, Bloomsburg
- PA, Blue Bell
- PA, Camp Hill
- PA, Harrisburg
- PA, Newtown
- PA, Oil City
- PA, Philadelphia
- PA, Pittsburgh
- PA, Pottstown
- PA, Sewickley
- PA, Washington
- OR, Grants Pass
- OR, Klamath Falls
- OR, Roseburg
- OR, White City
- OK, Oklahoma City
- OK, Okmulgee
- OK, Weatherford
- OH, Beachwood
- OH, Chillicothe
- OH, Cincinnati
- OH, Cleveland
- OH, Columbus
- OH, Defiance
- OH, Hamilton
- OH, Kirtland
- OH, Lewis Center
- OH, Marietta
- OH, Nelsonville
- OH, Perrysburg
- OH, Ravenna
- OH, Rio Grande
- OH, Van Wert
- OH, Youngstown
- OH, Zanesville
- ND, Bismarck
- ND, Grand Forks
- NC, Asheville
- NC, Charlotte
- NC, Clinton
- NC, Cullowhee
- NC, Elizabeth City
- NC, Fayetteville
- NC, Greensboro
- NC, Greenville
- NC, Kannapolis
- NC, Lumberton
- NC, Morrisville
- NC, New Bern
- NC, Raleigh
- NC, Sanford
- NC, Spruce Pine
- NC, Wilmington
- NC, Winston Salem
- NY, Bohemia
- NY, Brewster
- NY, Brooklyn
- NY, Buffalo
- NY, Corning
- NY, Flushing
- NY, Forest Hills
- NY, Hempstead
- NY, Johnson City
- NY, Kew Gardens
- NY, Melville
- NY, New York
- NY, Poughkeepsie
- NY, Rochester
- NY, Staten Island
- NY, Syracuse
- NY, Westbury
- NM, Albuquerque
- NM, Farmington
- NM, Santa Fe
- NJ, Branchburg
- NJ, Eatontown
- NJ, Jersey City
- NJ, Lincroft
- NJ, Lyndhurst
- NJ, Mount Laurel
- NJ, South Plainfield
- NJ, Swedesboro
- NJ, Toms River
- NJ, Union City
- NJ, West Windsor
- NH, Concord
- NH, Manchester
- NH, Newington
- NV, Las Vegas
- NV, North Las Vegas
- NE, North Platte
- MT, Bozeman
- MT, Great Falls
- MT, Kalispell
- MT, Missoula
- MO, Bridgeton
- MO, Cape Girardeau
- MO, Carthage
- MO, Columbia
- MO, Kirksville
- MO, Lee’s Summit
- MO, Poplar Bluff
- MO, Sedalia
- MO, Springfield
- MO, St. Louis
- MO, St. Peters
- MO, Warrensburg
- MS, Meridian
- MS, Raymond
- MS, Starkville
- MN, Grand Marais
- MN, Inver Grove Heights
- MN, Rochester
- MN, St. Cloud
- MN, St. Paul
- MI, Ann Arbor
- MI, Auburn Hills
- MI, Berrien Springs
- MI, Dearborn
- MI, Dowagiac
- MI, East Lansing
- MI, Gaylord
- MI, Grand Rapids
- MI, Houghton
- MI, Lansing
- MI, Mount Pleasant
- MI, Port Huron
- MI, Traverse City
- MI, University Center
- MA, Holyoke
- MA, Milford
- MA, Norwood
- MA, Pittsfield
- MA, Rockland
- MA, South Boston
- MA, Springfield
- MA, West Boylston
- MA, Worcester
- MD, Baltimore
- MD, Catonsville
- MD, Columbia
- MD, Ellicott City
- MD, Frederick
- MD, Hagerstown
- MD, La Plata
- MD, Leonardtown
- MD, Ownings Mills
- MD, Prince Frederick
- MD, Reisterstown
- MD, Rockville
- MD, Salisbury
- MD, Wye Mills
- ME, Farmington
- ME, Presque Isle
- ME, South Portland
- ME, Windham
- LA, Alexandria
- LA, Baton Rouge
- LA, Bossier City
- LA, Hammond
- LA, Natchitoches
- LA, New Orleans
- LA, Shreveport
- LA, St. Rose
- LA, Sulphur
- KY, Ashland
- KY, Bowling Green
- KY, Elizabethtown
- KY, Florence
- KY, Highland Heights
- KY, Lexington
- KY, Louisville
- KY, Madisonville
- KY, Morehead
- KY, Prestonsburg
- KS, Lawrence
- KS, Manhattan
- KS, Overland Park
- KS, Pittsburg
- KS, Wichita
- IA, Cedar Falls
- IA, Des Moines
- IA, Dubuque
- IA, Iowa City
- IA, Mason City
- IA, Ottumwa
- IA, Waterloo
- IN, Anderson
- IN, Bloomington
- IN, Columbus
- IN, East Chicago
- IN, Evansville
- IN, Fort Wayne
- IN, Hammond
- IN, Indianapolis
- IN, Lafayette
- IN, Lawrenceburg
- IN, Madison
- IN, Merrillville
- IN, New Albany
- IN, Richmond
- IN, South Bend
- IN, Terre Haute
- IN, Valparaiso
- IL, Carbondale
- IL, Carol Stream
- IL, Champaign
- IL, Chicago
- IL, Chicago Heights
- IL, Collinsville
- IL, Danville
- IL, Des Plaines
- IL, East Peoria
- IL, Galesburg
- IL, Glen Ellyn
- IL, Godfrey
- IL, Kankakee
- IL, Midlothian
- IL, Naperville
- IL, Oak Forest
- IL, Oglesby
- IL, Park Ridge
- IL, Schaumburg
- IL, Springfield
- IL, Vernon Hills
- IL, Westmont
- ID, Idaho Falls
- ID, Lewiston
- ID, Pocatello
- ID, Twin Falls
- HI, Honolulu
- HI, Kahului
- HI, Kapolei
- GU, Mangilao
- GA, Alpharetta
- GA, Atlanta
- GA, Augusta
- GA, Brunswick
- GA, Columbus
- GA, Dahlonega
- GA, Decatur
- GA, Kennesaw
- GA, Lawrenceville
- GA, Marietta
- GA, Milledgeville
- GA, Savannah
- GA, Statesboro
- GA, Valdosta
- GA, Warner Robins
- GA, Waycross
- FL, Avon Park
- FL, Boca Raton
- FL, Casselberry
- FL, Chiefland
- FL, Daytona Beach
- FL, Eglin Afb (Authorized Testers Only)
- FL, Fort Lauderdale
- FL, Fort Myers
- FL, Ft. Myers
- FL, Gainesville
- FL, Hialeah
- FL, Jacksonville
- FL, Key West
- FL, Kissimmee
- FL, Lake Worth
- FL, Lecanto
- FL, Melbourne
- FL, Miami (Kendall)
- FL, North Miami
- FL, Orlando
- FL, Pembroke Pines
- FL, Pensacola
- FL, Pompano Beach
- FL, Port Charlotte
- FL, Riverview
- FL, Riviera Beach
- FL, Sanford
- FL, Tallahassee
- DC, Washington
- DE, Georgetown
- DE, Wilmington
- CT, Hartford
- CT, Meriden
- CT, Plantsville
- CT, Woodbridge
- CO, Centennial
- CO, Colorado Springs
- CO, Durango
- CO, Fort Collins
- CO, Fort Morgan
- CO, Grand Junction
- CO, Lakewood
- CO, Longmont
- CA, Anaheim
- CA, Bakersfield
- CA, Burbank
- CA, Burlingame
- CA, Calimesa
- CA, Ceres (Modesto)
- CA, Glendale
- CA, Hayward
- CA, Inglewood
- CA, Lakewood
- CA, Long Beach
- CA, Los Angeles
- CA, Northridge
- CA, Oroville
- CA, Palm Desert
- CA, Riverside
- CA, Sacramento
- CA, Salinas
- CA, San Bernardino
- CA, San Diego
- CA, San Francisco
- CA, San Jose
- CA, Santa Ana
- CA, Santa Clara
- CA, Santa Maria
- CA, Stockton
- CA, Woodland Hills
- CA, Yucaipa
- AR, Arkadelphia
- AR, Barling
- AR, Bentonville
- AR, Fayetteville
- AR, Hot Springs
- AR, Jonesboro
- AR, Little Rock
- AR, North Little Rock
- AZ, Avondale
- AZ, Flagstaff
- AZ, Glendale
- AZ, Phoenix
- AZ, Prescott
- AZ, Queen Creek
- AZ, Sierra Vista
- AZ, Surprise
- AZ, Thatcher
- AK, Anchorage
- AK, Fairbanks
- AK, Ketchikan
- AK, Soldotna
- AL, Birmingham
- AL, Daleville
- AL, Decatur
- AL, Huntsville
- AL, Millbrook
- AL, Montgomery
- AL, Phenix City
- AL, Tuscaloosa

Best Universities in Luxembourg

Streamlining Student Management: The Power of Automated Systems

All You Need to Know about Scholarship to Study Abroad

International School Fees: Unveiling the True Value

Top 10 SEO company in Bangalore Specialized in SEO Educational Institute .
- Career & Jobs
- Career Guidance
- Study Abroad
- Personality Development

Cheating in Examinations. Why Do Some Students Do It?
- What is cheating?
- Reasons why students cheat in exams
- Effects of cheating on students
Cheating can be defined as a dishonest act to gain an undue advantage. In educational parlance, cheating is usually associated with examinations.
It includes various forms of cheating like plagiarizing content, copying, or even impersonating another person to write an exam.
In The Cheating Culture: Why More Americans Are Doing Wrong To Get Ahead , David Callahan, co-founder and research director of Manhattan-based public policy think-tank Demos, demonstrates cheating through different means.
Professional athletes’ use of performance-enhancing steroids, reporters’ disguise of fiction as journalism, physicians’ promotion of drugs of questionable efficacy in exchange for payments from pharmaceutical companies, students’ cheating on exams and submitting plagiarized work, and music fans’ piracy of CDs on the internet, as well as theft by employees and high-stakes corporate crime.
Why Do Students Cheat in Exams?
In schools and colleges, cheating is not unheard of. There have been numerous instances where students have been caught cheating in examinations. The important question to answer here is why do students cheat?
There are many reasons why students cheat in exams. We have enumerated a few of these reasons below.
1.Poor Time Management
One of the main reasons why students cheat in exams is because they are pressed for time. With lack of time, students resort to cheating because they are unable to finish studying portions.
To overcome this problem, students must get into the practice of making realistic timetables . A timetable can help you set time for each subject, giving more time to tougher subjects and a bit more relaxed time for easier subjects.

This stress is one of the main reasons for students to cheat in exams. Unable to bear the stress and tension, students may resort to unlawful means like cheating during examinations.
3. Fear of Failure
No one likes to fail. It is a known fact that failure is looked upon as a taboo in our society. Students who fail are ridiculed by society.
These students are often looked down upon with disdain. Therefore, to avoid being ostracized by the community, students often resort to cheating in exams.
4. Educational System Pressure
The educational system today does not exactly have a scientific approach. It is not cut out for individual minds.
The education system caters to the bulk of the student population, leaving out a section of students unable to cope with the system.
So, what happens to these students? Well, these students are pressured to keep pace with the syllabus even if they cannot do so.
What do these students do then? These students resort to cheating and other dishonest methods of keeping up with the syllabus.
5. Family Expectations
Another reason why students cheat in exams is because of the pressure put on them by parents and other members of the family. This is one of the main reasons why students cheat.
Let’s take a typical example of a parent, who has a very high educational qualification and runs a successful business or enterprise.
Such a parent will also expect their child to study well and secure higher degrees and educational qualifications.
However, what if the child is incapable of performing to the parent’s expectation? What if the child is an average student?
The child may also want to prove to his or her parents that they can be as successful as their parents. This can lead to cheating to perform to parents’ expectations.
6. Comparison with Friends

Another reason why students cheat in exams is that they want to perform as well as their classmates and friends.
Healthy competition is not a bad concept. However, if this competition turns more serious, then it can lead to cheating and unfair means to achieve higher scores.
7. Cut-Offs and Limited Seats in Prestigious Institutions
Many institutions have a minimum cut-off below which students will not get admission into the institute.
Therefore students resort to cheating in exams to achieve high scores and get into prestigious institutions or pass prestigious administrative examinations.
8. “Everybody Is Doing It” Attitude
Another reason why students cheat in exams is because of the “everybody is doing it” attitude. This attitude discourages diligent and truthful students from studying and working hard.
Such students may think if others are cheating and getting marks then why should they study hard and get the same marks. So, they give in, even though they can study and achieve great success.
Cheating in Exams Causes and Effects
These were just some of the answers to the question why do students cheat? To understand this better, we can try and decipher some of the causes and effects of cheating in exams and find possible solutions for the problem of students cheating in examinations.
As mentioned above, some of the reasons why students cheat in exams are also the causes of cheating.
In addition to the above reasons, we can also mention media as one of the main causes of cheating
amongst students. You may ask how the media can play a role in this? The answer is simple.
Over the years, the means of communication have increased tenfold. Students have access to more than one means of communication.
Today, apart from television and radio, students have social media, cellular mobile phones, and facetime to communicate. This communication , though a boon in many cases can also be a bane.
Many times, media houses showcase successful people from prestigious institutes. Unknowingly, media houses indirectly advertise that the only way to reach success is through a particular channel of education and no other way.
Thus, this undue showcase of successful careers can influence students the wrong way. Students come to think that the only way to succeed is by getting into a particular university for a particular course. Thus, paving the way for students to think about getting it through hook or by crook.
The media portrays these successful personalities with a lot of wealth, fame, and respect in society . This blindly encourages students to try and achieve that success even if it means by unfair methods.
Therefore, we can safely conclude that one of the causes of cheating is the socio-economic disparity between classes and sections of people.
In an urge to become rich and successful overnight, students may employ shortcut methods like cheating.
An article published by the Carnegie Mellon University states several reasons why students cheat in exams:
- Unfair tests and unprofessional teachers
- Obligation to help other students fair better in examinations
- Poor study skills
- Competition
- Exam anxiety
- Lack of knowledge on the consequences of cheating
- The perception of escaping punishment
The causes and effects of cheating in exams are straightforward. There are numerous ill effects of cheating.
They can have a deep impact on the career and life of students. We have enumerated some of the effects of cheating on students.
1. Admonishments
Students caught cheating in examinations are severely punished. These punishments can range from getting debarred in exams, expelled from school, or even suspended for the rest of the academic year.
Consequently, these severe punishments can have grave repercussions on the student’s wellbeing.
2. Lifelong Record
When a student cheats on an exam, the incident is put on record and becomes a bad black spot on the student’s life ahead. It can affect a student’s career and life.
3. Meaningless Careers

Getting a job through unfair means can only take you through the first step. Sustaining yourself in the job will purely rest on the knowledge you have acquired. Through cheating, you can pass examinations but you will not acquire knowledge.
4. Loss of Reputation
Your reputation defines your character . It takes a lifetime to build a good reputation but a minute to lose it all.
Your single moment of cheating can ruin your reputation for the rest of your life. So, remember, the reputation for a thousand years can depend on your conduct in a single moment.
These are the causes and effects of cheating in exams, it can be rightly said that cheating can lead to worse situations that can affect your entire life and career ahead.
So, remember, it is up to you to decide if you want to make it the right way. Cheating is a choice, not a mistake.
You Might Also Like
Leave a reply cancel reply.
Your email address will not be published. Required fields are marked *
Save my name, email, and website in this browser for the next time I comment.
Weekly Newsletter
subscribe to our latest blog and weekly newsletter
Popular News

Importance of Objective Setting in Life
- Advertisement -

- Certifications
Top Categories
Subscribe us, for quick admission assistance.

Sign in to your account
Username or Email Address
Remember Me
How Cheating in College Hurts Students
Academic integrity is important, experts say, as plagiarism and other cheating may have severe consequences.

Getty Images | Westend61
Experts say the number of students engaging in academic dishonesty during the coronavirus pandemic is soaring.
Cheating in college is risky business loaded with potential consequences – failing classes, suspension, possible expulsion – yet it's common and perhaps more accessible than ever.
"A lot of people cheat a little," says David Pritchard, a physics professor emeritus at Massachusetts Institute of Technology who has studied academic dishonesty in online classes. "There's also a few people who cheat a lot."
Though it may be tempting and feel harmless, experts caution college students to think twice before cheating on coursework. Here's how to know what is typically considered cheating and the potential consequences.
How College Students Cheat
Cheating is a multibillion-dollar business, with some educational technology companies making money off students who use their products to break or bend academic integrity rules and others earning revenue from colleges trying to prevent academic dishonesty.
Students also use classic classroom moves like scribbling hidden notes somewhere or using technology such as smartwatches. Copying a classmate's assignment or plagiarizing parts of published works for a paper remain popular methods.
Many of those tactics appear to have been replaced by artificial intelligence and generative language models like ChatGPT and Google Bard, which offer some services like writing, editing and idea generation for free.
Pritchard notes that ChatGPT has performed well on exams in certain subjects, and the American Bar Association reported in March 2023 that it passed the Uniform Bar Exam by "a significant margin." While some professors say they're keeping an open mind about ChatGPT and similar tools, others say it's impossible to ignore the reality that students are using them to cheat.
ChatGPT "is the future of cheating," Pritchard says.
Rebecca Hamlin, a professor of legal studies and political science at the University of Massachusetts—Amherst , recently joined the university's academic honesty board and has seen cases of students caught cheating with ChatGPT. She caught 12 in her own classes during the spring 2023 semester.
“If students are genuinely interested in learning how to become writers, I’m very resistant to the idea that ChatGPT can help them," she says. “It’s really risky because it’s actually way more obvious to someone who reads really good writing all day long. I can immediately tell."
But plenty of students slip through undetected or cheat in other inconspicuous ways, she says.
Most instructors underestimate just how rampant the issue is, says Eric Anderman, a professor at The Ohio State University and interim dean at its Mansfield campus. "We think we're underestimating it because people don't want to admit to it."
Here's what academic integrity experts say college students should know about the immediate and long-term consequences of cheating.
The Consequences of Cheating in College
Regardless of the cheating method, students are only harming themselves and their learning process, experts say.
“I know that sounds really cheesy, but I kind of don’t really understand why someone is going to waste their time and money going to college if they don’t want to learn how to write," Hamlin says. "That’s probably one of the top two to three skills that you gain when you go to college."
Students also deprive themselves of a genuine feeling of achievement when they cheat, says Russell Monroe, director of academic integrity at Liberty University in Virginia.
"There’s a sense of dignity in knowing that I got a grade that I earned, whether that’s for an assignment or a class," he says. "You can look at your degree with pride knowing this is something I achieved on my own merit and didn’t have to outsource anything to anyone else or steal or plagiarize."
Some penalties can have a lasting effect and financial repercussions. They are often less severe for first-time offenders, but colleges keep records of such behavior. Students who continue to cheat and get caught risk failing a class, receiving academic suspension or being expelled from the school, which may come with a note on their transcript explaining why they were dismissed. This designation will likely make it harder to enroll at another college , experts say.
Students who fail a class due to academic dishonesty are usually allowed to retake it. If it's a class required for graduation, they don't have a choice. Either way, that means more money out of pocket, perhaps in student loans .
Failing a course also typically harms a student's GPA , particularly if they don't retake it and earn a higher grade. This could jeopardize eligibility for financial aid or scholarships and lead to academic probation .
Each school has its own policies and disciplinary measures, and professors may vary in how they address academic dishonesty. Some may handle it on their own while others may send it to a disciplinary committee. It often depends on the severity of cheating, Monroe says. For example, cheating on a discussion board assignment isn't seen as as serious as plagiarizing a dissertation or final exam paper, or cheating on a credential or certification exam, he says.
Plagiarizing on capstone course papers or other assignments tied to graduation is a particularly egregious offense that could jeopardize a student's ability to graduate, experts say.
“We are putting our stamp of approval on you to move on to the next step," Monroe says. "That next step might be graduation, but if we’re doing that based upon bad information or false information, that’s a serious problem.”
Even students who think they got away with cheating may suffer consequences, such as missing out on foundational information that they need to learn and apply in higher-level classes.
Additionally, graduates who cheated and perhaps even ended up with good grades may find themselves starting their career unprepared and lacking needed knowledge and skills. And for jobs that have a safety component, unprepared workers could put themselves and others at risk.
Then there are occasions when academic dishonesty is revealed later and torpedoes a career, sometimes in a public and humiliating way.
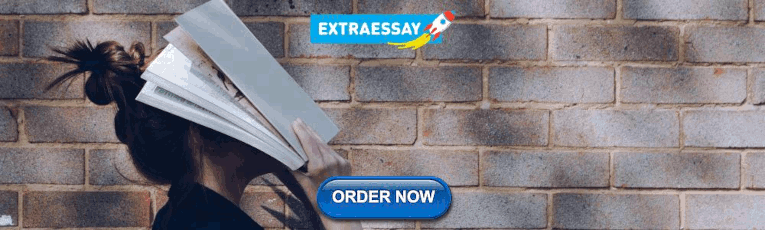
Know What Is and Isn't Cheating
While some students are well aware that they're cheating and see it as merely a means to an end, not all forms of academic dishonesty are intentional. In many cases, it's an accident made while under stress or when a student has procrastinated , experts say.
Sometimes students make mistakes because they aren't properly prepared to engage with college-level work. For example, improperly citing sources on a term paper can lead to charges of plagiarism.
"I think part of what happens is students aren't always taught in high school how to cite and evaluate information from the internet," Anderman says. "And I think a lot of them, when they get to college – and this is not an excuse – truly don't realize that you can't just look something up on the internet and put it in your paper, that you still have to cite it, and they get caught."
Colleges commonly use a variety of plagiarism-checking software, such as Turnitin, which flags written work that may be uncited or improperly cited. These tools help keep students honest and significantly decrease plagiarism, experts say.
Some forms of cheating, such as intentional plagiarism, buying papers online or paying someone to complete course work, should be fairly obvious, experts say. This is often referred to as "contract cheating," Monroe says, and it's an offense that can lead to expulsion from Liberty.
"It’s very difficult for us to know when that’s happening, but when we do find out, we view that very seriously because there are significant portions of your entire degree that may not have been done by the student at all," he says.
Other areas aren't as clear-cut, particularly what is permissible when it comes to collaborating with classmates, sharing information and using AI products. Monroe says Liberty doesn't ban the use of AI or tools like ChatGPT, but there are boundaries around their ethical use. Students can use these tools to edit and get inspiration, but any assignment turned in must be the student's original work.
Experts also caution against using online companies that position themselves as tutoring organizations but largely help students cheat. Colleges offer many academic resources that students can use instead, and at no extra cost.
“I would definitely encourage a student who’s facing a tough situation or feels that they can’t do their work on time to contact their professor and see if there’s some kind of alternate arrangement that can be made," Monroe says.
Many professors are willing to accept work late, he says. Liberty’s policy is to take 10% off of an assignment's overall grade if it’s late.
“We definitely prefer a timely submission of work," Monroe says, "but contact your professor. They are definitely willing to work with students within the scope that they’re allowed to. That would definitely be a better situation than turning to cheating."
Searching for a college? Get our complete rankings of Best Colleges.
10 Steps to Choosing the Right College

Tags: colleges , students , education , academics , cheating , college admissions , college applications , high school
2024 Best Colleges

Search for your perfect fit with the U.S. News rankings of colleges and universities.
College Admissions: Get a Step Ahead!
Sign up to receive the latest updates from U.S. News & World Report and our trusted partners and sponsors. By clicking submit, you are agreeing to our Terms and Conditions & Privacy Policy .
Ask an Alum: Making the Most Out of College
You May Also Like
How to decide if an mba is worth it.
Sarah Wood March 27, 2024

What to Wear to a Graduation
LaMont Jones, Jr. March 27, 2024

FAFSA Delays Alarm Families, Colleges
Sarah Wood March 25, 2024

Help Your Teen With the College Decision
Anayat Durrani March 25, 2024

Toward Semiconductor Gender Equity
Alexis McKittrick March 22, 2024

March Madness in the Classroom
Cole Claybourn March 21, 2024

20 Lower-Cost Online Private Colleges
Sarah Wood March 21, 2024

How to Choose a Microcredential
Sarah Wood March 20, 2024

Basic Components of an Online Course
Cole Claybourn March 19, 2024

Can You Double Minor in College?
Sarah Wood March 15, 2024


An official website of the United States government
The .gov means it’s official. Federal government websites often end in .gov or .mil. Before sharing sensitive information, make sure you’re on a federal government site.
The site is secure. The https:// ensures that you are connecting to the official website and that any information you provide is encrypted and transmitted securely.
- Publications
- Account settings
Preview improvements coming to the PMC website in October 2024. Learn More or Try it out now .
- Advanced Search
- Journal List
- v.7(10); 2021 Oct

Exam cheating and academic integrity breaches during the COVID-19 pandemic: An analysis of internet search activity in Spain
Rubén comas-forgas.
a University of the Balearic Islands, Spain
Thomas Lancaster
b Imperial College London, UK
Aina Calvo-Sastre
Jaume sureda-negre, associated data.
Data will be made available on request.
Academic institutions worldwide have had to adapt their methods of teaching and assessment due to COVID-19. In many Spanish universities exams moved online, affording students with opportunities to breach academic integrity that were not previously available to them. This paper uses the emerging research method of search engine data analysis to investigate the extent of requests for exam cheating information in Spain in the time period surrounding adjustments for the pandemic. The Internet data analytics technique is one that the paper proposes should be used more widely as an academic integrity research method.
For this study, search engine activity data on exam cheating in Spain was collected and analysed for the five-year period between 2016 and 2020 inclusive. The data suggests that students are searching for information about ways to cheat in exams, including how to create cheat sheets. Most strikingly, the results show a significant increase in requests for information on cheating on online exams during the COVID-19 timeframe and the Spanish lockdown period. Based on the findings, academic institutions in other regions should be wary about the opportunities that their students have to commit exam fraud.
Assessment; Exam cheating; Academic integrity; Spain; COVID-19.
1. Introduction
The COVID-19 pandemic has seen an international movement towards online teaching and assessment. The move online has often been completed at short notice and with little opportunity for plans to be put into place to ensure that academic integrity is preserved. Many default assessment methods are used to allow instructors to evaluate the competencies, skills and knowledge of students, including holding examinations and requiring students to write papers ( Stiggins, 2017 ; Wiggins, 2011 ). The results obtained by students during these assessments can be crucial to their future lives and careers, determining both their economic status and their position in society ( Fontaine et al., 2020 ).
The nature of assessment is such that, whether it is held in person or online, students have a personal incentive to try and obtain the best grades that they can. This means that some may resort to using unfair means, or as the academic integrity literature may declare this, they may act with academic dishonesty or commit academic misconduct. In other situations, such integrity breaches may be labelled as cheating or fraud.
Student cheating is not a new phenomenon. Comas et al. (2011) discuss a wide range of methods that students can use to get an unethical advantage over their peers, including using cheat sheets, copying from classmates during written tests, committing plagiarism and falsifying data ( Comas et al., 2011 ). Students taking exams have revealed a more recent tendency to hire external contacts to secretly communicate answers to them ( Lancaster et al., 2019 ).
This paper focuses specifically on the student interest in cheating in exams during the COVID-19 pandemic, an area in which it has been observed that an increasing number of students are contract cheating and requesting exam answers ( Lancaster and Cotarlan, 2021 ). Two novel contributions are made to the academic integrity literature. First, the study focuses on academic integrity in Spain, an area that has been little explored. Second, the emerging research technique of search engine analytics is used to conduct the study, suggesting a method that could be more widely applied to academic integrity research in the future.
2. Background
2.1. academic integrity and the pandemic.
The word pandemic is not a new word when considered in relation to the academic integrity situation. Authors have previously expressed concerned that fraudulent practices are widespread when assessment is considered ( Baran and Jonason, 2020 ; Brown and McInerney, 2008 ; Josien et al., 2015 ). This could already be said to represent a pandemic at the global level. Others commentators have suggested that academic misconduct is becoming a normative behaviour among students ( Jensen et al., 2002 ).
When assessment processes cannot be trusted to provide accurate results, this can pose a challenge to the validity of qualifications and the credibility of certificates and degrees ( Goff et al., 2020 ; Martin, 2017 ). An associated risk can see the trust that society has in academic institutions being called into question.
There is a relationship between academic dishonesty and professional dishonesty. As studies such as those of Nonis and Swift (2001) , Carpenter et al. (2004) and Guerrero-Dib et al. (2020) show, students who have acted fraudulently in academic environments are more likely, in the future, to carry out dishonest behaviours in their professional workplace.
Moreno (1999) has suggested that educational institutions are the first test bed of corruption and dishonest behaviour. This is particularly relevant in the context of COVID-19, where many institutions have been forced to adopt didactic models based on distance teaching and online student assessments ( Crawford et al., 2020 ; Raje and Stitzel, 2020 ; White, 2020 ). In some cases, students have been required to take tests and exams online in an environment which is not supervised and where it is difficult to verify that the student is completing the assessment of their abilities without resorting to unfair means.
Studies about the integrity of online assessments may be less developed than those relating to face-to-face assessments, but indications that online assessments pose high risks to academic integrity are beginning to emerge. The academic publisher Willey (2020) surveyed almost 800 university teachers from around the world and found that 93% of participants believed that students have more opportunities to cheat on online assessments and that this problem has never been as severe as during the COVID-19 pandemic.
Literature reviews such as the one conducted by Chen et al. (2020) show that academic dishonesty in online teaching models is widespread and that the factors that cause it are related to components such as personality, cognitive aspects and teaching styles. A further review ( Butler-Henderson and Crawford, 2020 ) confirms that the topic of assessment test fraud is the most addressed in the literature on online assessments. Its prevalence, as Sullivan (2016) has shown, is at an alarming level. In this regard, there is the suggestion that the online assessment environment has more drawbacks than the classroom environment for students and teachers ( Hobsons, 2021 ; Joshi et al., 2020 ). It also appears to offer greater facilitation for cheating ( Fask et al., 2014 ).
Although online proctoring of remote exams is possible, an early study of academic integrity during COVID-19 has shown that shown that this can be detrimental to students’ mental health ( Eaton and Turner, 2020 ). Online proctoring does not seen to have been widely adopted across the sector as a solution for preventing misconduct. Amzalag et al. (2021) also warn about a growing mistrust between students and instructors during this time.
2.2. The Spain context
The study of academic integrity in Spain, the geographical scope of this paper, does not have a tradition as established as that of the Anglo-Saxon environment or central and northern Europe ( Comas, 2009 ). Regarding studies that focus on dishonest student behaviours seen in the course of them taking exams, the work that can be cited is very scarce.
A study focused on nursing students ( Blanch-Mur et al., 2006 ) showed that 28% of students claimed to have copied during an exam. Data from a second study, based on a sample of Spanish university students, show that approximately 45% of students claimed to have used cheat sheets and material not allowed during exams ( Sureda-Negre et al., 2009 ). A later study carried out by the same group of researchers, found that almost 50% of university students reported having copied at least once during a face-to-face exam ( Comas et al., 2011 ).
A Spanish panel of experts, stated that the most serious dishonest behaviours that university students can commit on their assessments were: impersonating another person on an assessment; stealing tests or exams, manipulating their grades and changing them for others; obtaining exam or assessment questions before taking an exam; turning in an exam taken by another student as one's own; cheating on a face-to-face exam through technological devices, such as mobile phones and earpieces, then, finally, presenting work by another student as their own ( Sureda-Negre et al., 2020 ). The relationship and assessment of dishonest behaviours show the concern about exam fraud by the group of participating experts.
The adaptation of the Spanish university education system to the context caused by the pandemic has led, among other developments, to an increase in concerns about exam fraud. Such concern has resulted in the development of guidelines and recommendations by political and academic authorities on non-face-to-face assessment procedures ( Conferencia General de Política Universitaria, 2020 ; CRUE, 2020 ; Ministerio de Universidades, 2020 ). In the Conference of Rectors of Spanish Universities (Conferencia de Rectores de las Universidades Españolas - CRUE) guideline, there is no explicit reference to cheating on exams, but on up to twenty occasions, the word “security” appears, with honesty being one of its fundamental dimensions. Specifically, the following is stated:
Other important aspects to consider are measures to preserve academic integrity and the use of available legal mechanisms (expulsion from the test, qualification of suspension or, where appropriate, institution of disciplinary proceedings) in the case of fraudulent tests or assignments ( CRUE, 2020 , p. 5, p. 5).
In a handbook prepared by the Ministerio de Universidades (Ministry of Universities), a section is dedicated to presenting recommendations to avoid the use of fraudulent means and another to presenting systems to guarantee the authorship of exams ( Ministerio de Universidades, 2020 ).
The handbook of recommendations developed by the Group of Online Teaching Authorities of the Public Universities of Castilla y León is worth noting ( García-Peñalvo et al., 2020 ). Among its recommendations are detecting impersonation throughout an exam as a requirement that can be requested from an e-proctoring system, blocking the browser of the examinee so that they cannot access content outside the exam, detecting elements other than those necessary to perform a test; and, finally, encouraging the obtainment of objective evidence about the completion of exams by students without help or collaboration from third parties.
Concern about the issue of fraud on online exams in the context of COVID-19 is also reflected in the media in Spain, echoing numerous cases of fraud on online assessments during 2020 ( Alías, 2020 ; Asensio, 2021 ; García, 2020 ; Ortega, 2020 ; Peiro, 2020 ). In most of these journalistic articles, online assessments have been presented with a negative viewpoint due to the potential ease of fraud. In the opinion of Goberna, a Professor of Mathematics at the University of Alicante, “Online exams are a scam; they will basically cheat” ( Bueno, 2021 ). A Professor of Italian Philology at the University of Oviedo, de Sande, maintains a similar position stating “With the telematic exams, you give away the course” ( Rodríguez, 2021 ).
A final indicator of the extent of the phenomenon of fraud on assessments in the COVID-19 context can be obtained by searching YouTube with the descriptor “ copiar examen online ” (“ cheat online exam ”). A large number of videos are found in which experiences of cheating on exams are related to direct titles, such as “ Ayudo a mi hermana a copiar en un examen online! ” ( “I help my sister cheat in an online exam! ”), which gained over 3.7 million views in under nine months ( YoSoyPlex, 2020 ), and others openly give advice on cheating on online assessments, such as “ Cómo saber las respuestas de un examen online ” (“How to know the answers to an online exam”), which accumulated nearly 850,000 views from April 2020 to February 2021 ( SPOTTWAIS XD, 2020 ).
Considering the above, the relevance of obtaining new knowledge about exam fraud in the COVID-19 era is clear. This study addresses the issue from a perspective rarely used until now, namely data analysis from Internet searches or search analytics.
3. Objectives
This study addresses the issue of exam misconduct in the scenario that occurred in Spanish universities due to COVID-19. It does so by aiming to provide evidence that improves the knowledge about dishonest behaviours available to students during the pandemic. The study is based on the hypothesis that interest in exam fraud has experienced a substantial boom during the COVID-19 crisis and that this increase is reflected in Internet searches. Thus, the following objectives are provided:
- - To identify, analyse and classify the descriptors used in the Internet searches carried out in Spain about cheating on exams in 2020;
- - To analyse the volume of activity and the search trends for descriptors related to cheating on exams in Spain in 2020; and
- - To compare the trends for and volume of Internet searches about cheating on exams in Spain between 2016-2019 and 2020.
4. Methodology
4.1. methodological underpinning.
The study presented in this paper uses search engine analytics ( Walcott et al., 2011 ) or web log analysis ( Jansen and Spink, 2006 ) as the basis for a methodological analysis of academic dishonesty during the COVID-19 pandemic. This research approach is used to address many of the flaws that can be seen in other studies, examples of which are considered here.
The majority of research into academic integrity is based on experimental studies in which dishonest behaviours are analysed. Some examples include Beaussart et al. (2013) and Gino et al. (2009) . Many studies are based on self-reported responses by the participants, for example Cronan et al. (2018) and Schwartz et al. (2013) . Other research has been based on the analysis of detected cases of academic dishonesty as an indicator of its prevalence, an example of which is Thomas and Jeffers (2020) .
Each of these approaches has its own limitations. For instance, experimental or quasi-laboratory investigations can be based on recreated situations that do not correspond to the real context and the practical consequences of the dishonest behaviour studied ( Comas, 2009 ). Further, studies based on self-reported responses may be inaccurate or contaminated by social desirability bias ( Fask et al., 2014 ; Lee et al., 2020 ).
There are also shortcomings in the work focused on the detection of fraud because not all examples of academic dishonesty are discovered. This can be seen in the contributions of Foltýnek et al. (2020) , where the use of automatic plagiarism detection software can provide inaccurate measures of this malpractice, including both false positives and false negatives.
The study reported in this paper provides a novel way of addressing the methodological difficulties outlined above, namely the data analysis of queries in a search engine. It can be considered to complement existing research techniques used in academic integrity well.
The analysis of data from Internet searches is an expanding research method that has been used in various fields. First, it has been applied in medium-term forecasting studies. Some examples include the analysis of unemployment trends ( Vicente et al., 2015 ), the analysis of consumer good preferences ( Dimpfl and Jank, 2016 ), preferences between tourist destinations ( Yang et al., 2015 ), in estimations of affluence to spaces or cultural and sports activities ( Martínez et al., 2016 ), and in studies of electoral result predictions ( Prado-Román et al., 2020 ). Second, it has been used in immediate prediction studies to allow obtaining relevant information much earlier than through traditional data collection techniques ( Fantazzini, 2014 ). Third, it has been used in studies detecting problems related to health ( Clemente et al., 2019 ; Zhang et al., 2018 ), the environment ( Funk and Rusowsky, 2014 ) and well-being ( Arnold, 2020 ). Finally, it has been used for the measurement of complex processes where traditional data presents known deficits, for example, for international migration studies ( Böhme et al., 2020 ).
Search engine analytics not been widely applied in academic integrity research, but has potential to improve knowledge within this field. There are some educational studies that analyse Internet searches of academic institutions to predict their recognition and reputation, for example Vaughan and Romero-Frías (2014) , but they are tangential to the study presented here. One of the closest examples within the academic integrity field is the work by Daly (2020) . This analysed the search engine optimisation techniques used by contract cheating websites, but the focus there was primarily on the provision of services rather than the demand for them. Following the conclusions of a study by Ginsberg et al. (2009) this paper argues that web search data can be used to track various social phenomena and provide more timely and updated information than can traditional data.
4.2. Dataset formation
Two datasets were collected related to search terms used by students looking to cheat in exams. Data was gathered relating to exam cheating in general, as well as relating to specific requests to cheat in online exams. All of the data was restricted to searches from Spain.
4.2.1. First dataset
The first dataset related to monthly search volumes in 2020 and was prepared using the “Keyboard magic tool” function within the SEMrush software application. This allowed the exploration of organic search terms used in the Google search engine.
Two initial search terms in Spanish were applied. The descriptor “copiar examen” (“cheat exam”) was used to generate a list of 140 keywords. The keyword “chuletas examen” (“test cheat sheets”) generated a second list of 127 keywords. This list was manually filtered to remove duplicates (n = 73) and keywords not related to cheating in exams (n = 100), leaving 94 keywords for the first dataset.
For each of the 94 keywords, two measures were collected for each month between January and December 2020:
- 1) The average monthly volume of Google searches for each keyword throughout 2020
- 2) Trend data that measures the interest in the determined keyword during each month between January and December 2020. This is scaled by SEMrush between 0 and 1 based on the changes in the number of searches per month.
4.2.2. Second dataset
For the second dataset, figures were obtained through Google Trends related to longer term search figures between 2016 and 2020. This data was collected on a weekly basis. For this dataset, the searches in Spanish “copiar examen” (“cheat exam”), “chuletas examen” (“exam cheat sheets”), “copiar examen online” (“cheat online exam”) and “copiar online” (“cheat online”) were applied.
The Google Trends results are provided in a scaled format, with all values given between 0 and 100. 100 indicates the week with the highest frequency of searches between 2016 and 2020 in proportion to the total number of searches performed in Spain.
Two further examples are useful to indicate how the Google Trends data works.
The value of 50 indicates the weeks where the popularity of the term is half that of the maximum value.
The value of 0 represents the case where the search numbers were too low to provide enough data for the calculation.
4.2.3. Data processing
The process and data analysis were carried out using the statistical analysis programme SPSS V.20. The following statistical tests were used: frequency analysis, Student's t-test to compare means and one-way ANOVA.
5.1. Exam cheating search descriptors and volumes
Table 1 shows a high-level analysis of the 94 organic search keywords from the first dataset, grouped by the volume of searches. The total number of searches generated by the set of descriptors considered represents an average of almost 35,000 monthly searches in Spain, resulting in an annual volume close to 420,000 searches on this topic for 2020. The search for cheat sheet related terms are prominent in the dataset.
Table 1
List of analysed keywords and monthly search volume.
The 94 keywords were coded into five categories, classifying and counting the types of searches performed. As shown in Table 2 , the highest percentage (40.4%) was focused on locating information about how to cheat. The second group of most frequent searches (26.6%) was focused on generic concepts, while the other three refer, in this order, to searches for information about devices, electronic and non-electronic, and applications, programmes and webpages to cheat on exams.
Table 2
Search categories based on the analysed keywords.
In a second level of analysis, keywords were classified into two large blocks: searches related to cheating on online exams and searches related to cheating on exams in general. A total of 27.7% of the keywords identified searches for information related to online test fraud, while 73.3% were searches related to cheating on tests in general.
5.2. Monthly search trends
The 2020 exam cheating monthly keyword search trends for Spain were established from the first dataset. The cumulative results are shown in Figure 1 , which demonstrates that December, April and March 2020, in this order, were the months with the highest search volume. The lowest volumes occurred in September, August and January 2020.

Monthly search trends for the set of keywords during 2020.
The monthly trends for keywords based on cheating on online exams were compared with those based on cheating in general. Student's t-test was applied. The results are shown in Table 3 .
Table 3
Comparison of monthly trends for the keywords analysed with SEMrush based on searches related to cheating on exams in general vs. cheating on online exams.
∗ Significant at p < 0.05 level.
As Table 3 demonstrates, until May 2020, the search trend for keywords related to cheating on exams in general was higher than the trend for searches related to cheating on online tests, while in the months of May to July, the trend was reversed. The search trend for keywords related to cheating on exams in general in the last five months of the year was again higher. These differences were statistically significant for the March, April, May, June, July, November and December data.
5.3. Search trends between 2016 and 2020
The second dataset, containing data collected weekly between January 2016 and December 2020 and relating to general and online forms of exam cheating, was analysed. A one-way ANOVA test was used to assess the presence of significant differences among the annual means of searches for four keywords. Two keywords related to cheating on online exams (“Copiar online” (“Cheat online”) and “Copiar examen online” (“Cheat online exam”)) and two related to cheating on exams in general (“Copiar examen” (“Cheat exam”) and “Chuletas examen” (“Exam cheat sheets”)). The results are shown in Table 4 (see Table 5 ).
Table 4
Comparison of 2016–2020 annual means of weekly searches for the keywords analysed, based on data from Google Trends.
Table 5
Comparison of mean search interest figures in 2020 and in the 2016–2019 period for the keywords analysed, based on data from Google Trends.
As the data shown in Table 4 indicates, searches for exam cheating related terms increased substantially each year between 2016 and 2020, peaking in 2020. The search for “Copiar examen” (“Cheat exam”), showed high levels of interest in 2016 and 2020.
To calculate the difference between the searches performed during 2020, a year including the COVID-19 pandemic period, and the previous 4 years, the means for 2020 and the 2016–2019 period were compared using Student's t-test. There were significant differences in three of the four keywords analysed. The search interest for the descriptors “Copiar online” ("Cheat online"), “Copiar examen online” ("Cheat exam online") and “Copiar examen” ("Cheat exam") was significantly higher in 2020 than in previous years.
5.4. Searches during COVID-19 lockdown
A final study using the second dataset aimed to investigate the interest in exam cheating throughout 2020, comparing the period when the Spanish population was under lockdown with the remainder of the year. The lockdown period lasted for 15 weeks between March 14 and June 21. The remainder of the year, 37 weeks, comprised the non-lockdown period.
Table 6 shows a comparison of mean weekly search volumes for the four keywords over the lockdown and non-lockdown period. Student's t-test was applied.
Table 6
Comparison of weekly means of searches for keywords analysed for 2020 (period of lockdown vs. non-lockdown), based on data from Google Trends.
The results from Table 6 show the existence of significant differences in the search volumes for the selected keywords: during the lockdown. The search trends for the keywords “Copiar online” (“Cheat online”) (average interest, 63 points during lockdown versus 12 points during non-lockdown), “Copiar examen online” (“Cheat online exam”) (search interest, 42 points during lockdown versus three points during non-lockdown) and “Copiar examen” (“Cheat exam”) (search interest, 48 points during lockdown and 13 points during non-lockdown) were significantly higher than those during the non-lockdown period.
6. Limitations, discussion and conclusions
The data presented in this paper has shown an increase in interest in exam cheating in Spain which aligns with the period of the COVID-19 pandemic. This matches research that has been conducted using other methodologies, but which has focused mainly on exam cheating in English ( Lancaster and Cotarlan, 2021 ), thus suggesting that online exams in all languages are susceptible to breaches of academic integrity.
The research presented has relied on Internet search metrics. These provide valuable information on the interests on Internet users and can be used to predict population behaviour, but some limitations of this methodology should be noted. The largest limitation is that available data is anonymised, and therefore, there is no certainty about who is behind each search. This study identified approximately 400,000 annual searches in Spain related to cheating on exams, but there is no certainty that these were all conducted by students. The analysis also rely on trust of the data supplied by SEMrush and Google Trends. It could be argued that this is an indirect measure, but so is the predominant approach based on responses to questionnaires.
The Internet is central to the lives of everyone involved in education and beyond. It has become an important source of information for tracking and monitoring activities that take place online. In this context, the data related to internet searches is especially important as this reflects the “needs, wants, interest, and concerns” of people ( Ettredge et al., 2005 , p. 87). It has the potential to help improve the knowledge of human behaviour.
Using Internet search metrics has great value for research into academic integrity and education in general. This data describes what a population does when they think they are "alone" looking for information for their particular use ( Orduña-Malea, 2019 ). For sensitive research, such as the data presented here on exam misconduct, search engine analytics are suitable to both expand upon and complement existing evidence and methodologies.
Another limitation of the study is related to methodological issues; what have been detected with the methodology used are interests and trends about cheating practices during exams, but not the practices themselves. In the present study we are working on the basis of analysing a scenario of pre-conduct: we can estimate the level of interest on cheating in exams, but we cannot estimate the real number of cheating behaviours carried out, which is a very complex topic that has generated long discussions and arguments between researchers due to the intrinsic characteristics of the phenomenon analysed and the evident desirability bias that has ( Winrow et al., 2015 ).
The services used as data sources for this paper could also be useful for monitoring academic misconduct and predicting how this could develop in the future. Through Google Trends, for example, it should be possible to establish warning signs about new types of dishonest behaviours within weeks of discussion of such behaviours emerging. This would allow educational institutions to put early academic integrity interventions into place. Such monitoring and trend prediction could even take place on a regional or national level.
Most importantly, this paper has confirmed that Spain has not been immune to the academic integrity implications of the COVID-19 pandemic. The data presented has shown a significant increase in Internet searches for cheating on exams, especially on online exams.
This phenomenon was verified, first, through the volume of organic searches carried out in Spain in 2020 with keywords associated with “copiar en pruebas de evaluación” ("cheat on assessment tests"), which was very high (420,000). This magnitude can be better calibrated when compared with other descriptors associated with exams, such as “técnicas de estudio” (“study techniques”), whose annual search volume in 2020, based on SEMrush data, was approximately 152,000 in Spain.
Furthermore, the data obtained show that this interest in cheating on exams had been directed towards those taken online. Compared with previous years, the increase in such interest was significant. This trend is considered to be intimately related to the fact that during the pandemic, a large part of educational activities in Spain, including assessments, were virtual.
To be able to more accurately calibrate the relationship between interest in cheating on online exams and the educational context caused by the pandemic, it would be instructive to monitor the evolution of trends and search volumes in the coming years. Given the ease of access and processing of data from almost any country in the world, it would also be useful to perform international comparative analyses. This would allow a clearer snapshot to be obtained of what happens at the international level in relation to fraud in assessment processes in the context of the pandemic and the massive adoption of online assessment procedures. Unfortunately, the data provided in this study on Spain hints at an unflattering scenario for academic integrity.
It would be interesting, for the future, analysing and comparing the data gathered in our study with data generated by studies on the same topic carried out using other methodologies during the outbreak so as to be able to measuring the correlation between different data series and estimating the validity of the method used in our study.
Declarations
Author contribution statement.
Rubén Comas-Forgas, Thomas Lancaster, Aina Calvo-Sastre and Jaume Sureda-Negre: Conceived and designed the experiments; Performed the experiments; Analyzed and interpreted the data; Contributed reagents, materials, analysis tools or data; Wrote the paper.
Funding statement
This work was supported by the Spanish Ministry of Science and Innovation (MCI), the National Research Agency (AEI) and the European Regional Development Fund (ERDF) (Grant number: RTI2018-098314-B-I00).
Data availability statement
Declaration of interests statement.
The authors declare no conflict of interest.
Additional information
No additional information is available for this paper.
- Alías M. La Universidad española a la caza de los "copiones" que se aprovechan de la pandemia. Vozpopuli. 2020 https://www.vozpopuli.com/espana/universidad-examenes-plagio-copiar_0_1389761827.html [ Google Scholar ]
- Amzalag M., Shapira N., Dolev N. Two sides of the coin: lack of academic integrity in exams during the corona pandemic, students' and lecturers' perceptions. J. Acad. Ethics. 2021:1–21. [ PMC free article ] [ PubMed ] [ Google Scholar ]
- Arnold I.J. Internet search volumes of UK banks during the crisis: the role of banking structure and business model. Global Finance J. 2020; 45 :100472. [ Google Scholar ]
- Asensio E. Granada Hoy; 2021. La Universidad de Granada investiga dos denuncias por copiar en los exámenes. https://www.granadahoy.com/granada/Universidad-Granada-investiga-denuncias-copiar_0_1543946982.html [ Google Scholar ]
- Baran L., Jonason P.K. Academic dishonesty among university students: the roles of the psychopathy, motivation, and self-efficacy. PLoS One. 2020; 15 (8) [ PMC free article ] [ PubMed ] [ Google Scholar ]
- Beaussart M.L., Andrews C.J., Kaufman J.C. Creative liars: the relationship between creativity and integrity. Think. Skills Creativ. 2013; 9 :129–134. [ Google Scholar ]
- Blanch-Mur C., Rey-Abella F., Folch-Soler A. Nivel de conducta académica deshonesta entre los estudiantes de una escuela de ciencias de la salud. Enferm. Clín. 2006; 16 (2):57–61. [ Google Scholar ]
- Böhme M.H., Gröger A., Stöhr T. Searching for a better life: predicting international migration with online search keywords. J. Dev. Econ. 2020; 142 :102347. [ Google Scholar ]
- Brown B., McInerney T. Changes in academic dishonesty among business students in the United States, 1999-2006. Int. J. Manag. 2008; 25 (4):621–632. [ Google Scholar ]
- Bueno V. Los exámenes online son una estafa, van a copiar básicamente. Información. 2021 https://www.informacion.es/alicante/2021/01/16/examenes-online-estafa-29242243.html [ Google Scholar ]
- Butler-Henderson K., Crawford J. A systematic review of online examinations: a pedagogical innovation for scalable authentication and integrity. Comput. Educ. 2020; 159 [ PMC free article ] [ PubMed ] [ Google Scholar ]
- Carpenter D.D., Harding T.S., Finelli C.J., Passow H.J. Does academic dishonesty relate to unethical behaviour in professional practice? An exploratory study. Sci. Eng. Ethics. 2004; 10 (2):311–324. [ PubMed ] [ Google Scholar ]
- Chen C., Long J., Liu J., Wang Z., Wang L., Zhang J. 2020 International Conference on Advanced Education, Management and Social Science (AEMSS2020) Atlantis Press; 2020. Online academic dishonesty of college students: a review; pp. 156–161. [ Google Scholar ]
- Clemente L., Lu F., Santillana M. Improved real-time influenza surveillance: using internet search data in eight Latin American countries. JMIR Public Health Surveill. 2019; 5 (2):e12214. [ PMC free article ] [ PubMed ] [ Google Scholar ]
- Comas R. Palma de Mallorca: Universidad de las Islas Baleares (Spain); 2009. El ciberplagio y otras formas de deshonestidad académica entre el alumnado universitario (Doctoral Dissertation) https://dspace.uib.es/xmlui/handle/11201/153195 [ Google Scholar ]
- Comas R., Sureda J., Casero A., Morey M. La integridad académica entre el alumnado universitario español. Estud. Pedagog. 2011; 37 (1):207–225. [ Google Scholar ]
- Conferencia-General-de-Política-Universitaria . 2020. Recomendaciones sobre criterios generales para la adaptación del sistema universitario español ante la pandemia del Covid-19, durante el curso 2019-2020. https://www.madrimasd.org/uploads/recomendaciones_adaptacion_universidades_cgpu_de_15_de_abril.pdf [ Google Scholar ]
- Crawford J., Butler-Henderson K., Rudolph J., Malkawi B., Glowatz M., Burton R.,., Lam S. COVID-19: 20 countries' higher education intra-period digital pedagogy responses. J. Appl. Learn. Teach. 2020; 3 (1):1–20. [ Google Scholar ]
- Cronan T.P., Mullins J.K., Douglas D.E. Further understanding factors that explain freshman business students’ academic integrity intention and behavior: plagiarism and sharing homework. J. Bus. Ethics. 2018; 147 (1):197–220. [ Google Scholar ]
- CRUE . 2020. Informe sobre Procedimientos de Evaluación no Presewncial. Estudio del Impacto de su Implantación en las Universidades Españolas y Recomendaciones. https://tic.crue.org/wp-content/uploads/2020/05/Informe-procedimientos-evaluaci%C3%B3n-no-presencial-CRUE-16-04-2020.pdf [ Google Scholar ]
- Daly T. Bait and switch: the search engine optimization content practises of contract cheating websites. Proc. Plag. Across Eur. Beyond. 2020; 2020 :57–62. https://academicintegrity.eu/conference/proceedings/2020/daly20.pdf [ Google Scholar ]
- Dimpfl T., Jank S. Can internet search queries help to predict stock market volatility? Eur. Financ. Manag. 2016; 22 (2):171–192. [ Google Scholar ]
- Eaton S., Turner K. Exploring academic integrity and mental health during covid-19: rapid review. J. Contemp. Educ. Theo. Res. 2020; 4 (2) [ Google Scholar ]
- Ettredge M., Gerdes J., Karuga G. Using web-based search data to predict macroeconomic statistics. Commun. ACM. 2005; 48 (11):87–92. [ Google Scholar ]
- Fantazzini D. Nowcasting and forecasting the monthly food stamps data in the US using online search data. PLoS One. 2014; 9 (11) [ PMC free article ] [ PubMed ] [ Google Scholar ]
- Fask A., Englander F., Wang Z. Do online exams facilitate cheating? An experiment designed to separatre possible cheating from the effect of the online test taking environment. J. Acad. Ethics. 2014; 12 :101–112. [ Google Scholar ]
- Foltýnek T., Dlabolová D., Anohina-Naumeca A., Razı S., Kravjar J., Kamzola L.…Weber-Wulff D. Testing of support tools for plagiarism detection. Int. J. Technol. High. Educ. 2020; 17 (1):1–31. [ Google Scholar ]
- Fontaine S., Frenette E., Hébert M.H. Exam cheating among Quebec’s preservice teachers: the influencing factors. Int. J. Educ. Integ. 2020; 16 (1):1–18. [ Google Scholar ]
- Funk S.M., Rusowsky D. The importance of cultural knowledge and scale for analysing internet search data as a proxy for public interest toward the environment. Biodivers. Conserv. 2014; 23 (12):3101–3112. [ Google Scholar ]
- García C. 2020. ¿Cuáles son los trucos del alumnado para copiar en un examen online? El Economista . https://www.eleconomista.es/ecoaula/noticias/10540162/05/20/Cuales-son-los-trucos-del-alumnado-para-copiar-en-un-examen-online.html [ Google Scholar ]
- García-Peñalvo F.J., Corell A., Abella-García V., Grande M. La evaluación online en la educación superior en tiempos de la COVID-19. Educ. Know. Soc. 2020; 21 [ Google Scholar ]
- Gino F., Ayal S., Ariely D. Contagion and differentiation in unethical behavior: the effect of one bad apple on the barrel. Psychol. Sci. 2009; 20 (3):393–398. [ PubMed ] [ Google Scholar ]
- Ginsberg J., Mohebbi M.H., Patel R.S., Brammer L., Smolinski M.S., Brilliant L. Detecting influenza epidemics using search engine query data. Nature. 2009; 457 (7232):1012–1014. [ PubMed ] [ Google Scholar ]
- Goff D., Johnston J., Bouboulis B.S. Maintaining academic standards and integrity in online business courses. Int. J. High. Educ. 2020; 9 (2):248–257. [ Google Scholar ]
- Guerrero-Dib J.G., Portales L., Heredia-Escorza Y. Impact of academic integrity on workplace ethical behaviour. Int. J. Educ. Integ. 2020; 16 (1):1–18. [ Google Scholar ]
- Hobsons . 2021. Higher Ed Student Success Survey Fall 2020. https://www.hobsons.com/wp-content/uploads/2021/02/Higher-Ed-Student-Success-Survey-Fall-2020.pdf [ Google Scholar ]
- Jansen B.J., Spink A. How are we searching the World Wide Web? A comparison of nine search engine transaction logs. Inf. Process. Manag. 2006; 42 (1):248–263. [ Google Scholar ]
- Jensen L.A., Arnett J.J., Feldman S.S., Cauffman E. It's wrong, but everybody does it: academic dishonesty among high school and college students. Contemp. Educ. Psychol. 2002; 27 (2):209–228. [ Google Scholar ]
- Joshi A., Vinay M., Bhaskar P. Impact of coronavirus pandemic on the Indian education sector: perspectives of teachers on online teaching and assessments. Interact. Technol. Smart Educ. 2020 [ Google Scholar ]
- Josien L., Seeley E., Csipak J., Rampal R. Cheating: students and faculty's perception on potential cheating activity. J. Leg. Ethical Regul. Issues (JLERI) 2015; 18 (2) [ Google Scholar ]
- Lancaster T., Cotarlan C. Contract cheating by STEM students through a file sharing website: a Covid-19 pandemic perspective. Int. J. Educ. Integ. 2021; 17 (1):1–16. [ Google Scholar ]
- Lancaster T., Glendinning I., Foltýnek T., Dlabolová D., Linkeschová D. The perceptions of higher education students on contract cheating and educational corruption in south east Europe. J. Educ. Thought. 2019; 52 (3):209–227. [ Google Scholar ]
- Lee S.D., Kuncel N.R., Gau J. Personality, attitude, and demographic correlates of academic dishonesty: a meta-analysis. Psychol. Bull. 2020; 146 (11):1042. [ PubMed ] [ Google Scholar ]
- Martin B. Defending university integrity. Int. J. Educ. Integ. 2017; 13 (1):1–14. [ Google Scholar ]
- Martínez R.G., Herráez B.R., Yábar D. Actividad de búsquedas en internet como variable para determinar la afluencia a museos. Cuad. Tur. 2016; 38 :207–223. [ Google Scholar ]
- Ministerio de Universidades . 2020. Informe de iniciativas y herramientas de evaluación online universitaria en el contexto del COVID-19. http://www.feccoo-madrid.org/965dc797a0c3208f893b9a217b3ce1d5000063.pdf [ Google Scholar ]
- Moreno J.M. Con trampa y con cartón: el fraude en la educación, o cómo la corrupción también se aprende. Cuad. Pedagog. 1999; 283 :71–77. [ Google Scholar ]
- Nonis S., Swift C.O. An examination of the relationship between academic dishonesty and workplace dishonesty: a multicampus investigation. J. Educ. Bus. 2001; 77 (2):69–77. [ Google Scholar ]
- Orduña-Malea E. Google Trends: analítica de búsquedas al servicio del investigador, del profesional y del curioso. Anu. Think EPI. 2019; 13 :1–14. [ Google Scholar ]
- Ortega E. Computer Hoy; 2020. Copiar en un examen online: estos son los trucos que se están utilizando. https://computerhoy.com/noticias/life/copiar-examen-online-trucos-658423 [ Google Scholar ]
- Peiró R. La Razón; 2020. Discípulos del Lazarillo en la era digital. https://www.larazon.es/comunidad-valenciana/20200608/ncyax5cdtzc6jjdk74s5askjji.html [ Google Scholar ]
- Prado-Román C., Gómez-Martínez R., Orden-Cruz C. American Behavioral Scientist; 2020. Google Trends as a Predictor of Presidential Elections: the United States versus Canada. [ Google Scholar ]
- Raje S., Stitzel S. Strategies for effective assessments while ensuring academic integrity in general chemistry courses during COVID-19. J. Chem. Educ. 2020; 97 (9):3436–3440. [ Google Scholar ]
- Rodríguez E. 2021. Con los exámenes telemáticos regalas la asignatura. La voz de Asturias . https://www.lavozdeasturias.es/noticia/asturias/2021/01/13/examenes-telematicos-regalas-asignatura/00031610555975688520570.htm [ Google Scholar ]
- Schwartz B.M., Tatum H.E., Hageman M.C. College students’ perceptions of and responses to cheating at traditional, modified, and non-honor system institutions. Ethics Behav. 2013; 23 (6):463–476. [ Google Scholar ]
- SPOTTWAIS XD . 2020. Como saber las respuestas de un examen online. https://youtu.be/-WXVLiwmdDY [ Google Scholar ]
- Stiggins R. ASCD; 2017. The Perfect Assessment System. [ Google Scholar ]
- Sullivan D.P. An integrated approach to preempt cheating on asynchronous, objective, online assessments in graduate business classes. Online Learn. 2016; 20 (3):195–209. [ Google Scholar ]
- Sureda-Negre J., Cerdá-Navarro A., Calvo-Sastre A., Comas-Forgas R. Las conductas fraudulentas del alumnado universitario español en las evaluaciones: valoración de su gravedad y propuestas de sanciones a partir de un panel de expertos. Rev. Invest. Educ. 2020; 38 (1):201–219. [ Google Scholar ]
- Sureda-Negre J., Comas-Forgas R., Gili-Planas M. Prácticas académicas deshonestas en el desarrollo de exámenes entre el alumnado universitario español. Estud. Sobre Educ. ESE. 2009; 17 :103–122. [ Google Scholar ]
- Thomas J., Jeffers A. Mobile eye tracking and academic integrity: a proof-of-concept study in the United Arab Emirates. Account. Res. 2020; 27 (5):247–255. [ PubMed ] [ Google Scholar ]
- Vaughan L., Romero-Frías E. Web search volume as a predictor of academic fame: an exploration of Google trends. J. Assoc. Info. Sci. Technol. 2014; 65 (4):707–720. [ Google Scholar ]
- Vicente M.R., López-Menéndez A.J., Pérez R. Forecasting unemployment with internet search data: does it help to improve predictions when job destruction is skyrocketing? Technol. Forecast. Soc. Change. 2015; 92 :132–139. [ Google Scholar ]
- Walcott B.P., Nahed B.V., Kahle K.T., Redjal N., Coumans J.V. Determination of geographic variance in stroke prevalence using Internet search engine analytics. Neurosurg. Focus. 2011; 30 (6) [ PubMed ] [ Google Scholar ]
- White A. May you live in interesting times: a reflection on academic integrity and accounting assessment during COVID19 and online learning. Account. Res. J. 2020 [ Google Scholar ]
- Winrow A.R., Reitmaier-Koehler A., Winrow B.P. Social desirability bias in relation to academic cheating behaviors of nursing students. J. Nurs. Educ. Pract. 2015; 5 (8):1–14. [ Google Scholar ]
- Wiggins G. A true test: toward more authentic and equitable assessment. Phi Delta Kappan. 2011; 92 (7):81–93. [ Google Scholar ]
- Willey . 2020. Academic Integrity in the Age of Online Learning. http://read.uberflip.com/i/1272071-academic-integrity-in-the-age-of-online-learning/0 [ Google Scholar ]
- Yang X., Pan B., Evans J.A., Lv B. Forecasting Chinese tourist volume with search engine data. Tourism Manag. 2015; 46 :386–397. [ Google Scholar ]
- YoSoyPlex . 2020. Ayudo a mi hermana a copiar en un examen online! https://youtu.be/owFcIGgmS9E [ Google Scholar ]
- Zhang Q., Chai Y., Li X., Young S.D., Zhou J. Using internet search data to predict new HIV diagnoses in China: a modelling study. BMJ Open. 2018; 8 (10) [ PMC free article ] [ PubMed ] [ Google Scholar ]
Cheating on College Exams is Demoralizing Cause and Effect Essay
Introduction.
Cheating on exams is a violation of school’s policies. The research focuses on the effect of cheating on the college exams. The research discusses three distinct effects of cheating on the college exams. Cheating on the college exams is demoralising.
Another Test Will Uncover the Dishonest Act.
After passing the exams, Tim West (West, 2004) emphasized that another test will uncover the cheating activity. Normally, the teacher doubts the cheating student’s high college exam score. Consequently, the teacher may investigate the truthfulness of the student’s high college test score. The teacher may conduct another test to determine the validity of the student’s high score. The follow-up quiz shows strong evidence of the cheating occurrence.
Cheating shames the students.
Depending on the cheating student’s cultural background, Tibbetts (1999) reiterated that the teacher’s spotting of the cheating in progress brings shame on the student involved. Normally, the teacher takes the cheating student’s paper and marks it as cheating. The student receives an automatic failed grade.
The teacher reports the students to the school administrators for disciplinary action. The school administration officer or guidance officer reprimands the students. The repeated acts of cheating force the school administrators and teachers to implement severe punishment on the erring student.
Cheating affects other classmates
In terms of ethics, Tim West (2004) theorized that cheating in class affects the other students. One student feels that cheating in class is unfair to the other students. The cheater copies the answers of the nearest intelligent room seatmate. In some instances, the cheater unintentionally copies the wrong answers of the less intelligent classmate.
In the nutshell, the post agrees with the thesis statement. Cheating on the college academic test is demoralizing. After getting a passing score, another test exposes the student’s cheating activity. The teacher’s spotting of a student cheating on college exams lowers the cheating student’s self esteem. Classmate eyewitnesses often spread the news of the cheating activity. Cheating on class tests causes the other students to cry foul. Indeed, cheating on the college tests is a transgression of the school’s policies.
Tibbetts, S. G.,(1999). Differences Between Women and Men Regarding Decisions To Commit Test Cheating. Research in Higher Education, 40 (1), 323 -342.
West, T. S., (2004). Cheating and Moral Judgment in the College Classroom. Journal of Business Ethics , 54 (2), 173 -183.
- Chicago (A-D)
- Chicago (N-B)
IvyPanda. (2023, November 25). Cheating on College Exams is Demoralizing. https://ivypanda.com/essays/cheating-on-college-exams-is-demoralizing/
"Cheating on College Exams is Demoralizing." IvyPanda , 25 Nov. 2023, ivypanda.com/essays/cheating-on-college-exams-is-demoralizing/.
IvyPanda . (2023) 'Cheating on College Exams is Demoralizing'. 25 November.
IvyPanda . 2023. "Cheating on College Exams is Demoralizing." November 25, 2023. https://ivypanda.com/essays/cheating-on-college-exams-is-demoralizing/.
1. IvyPanda . "Cheating on College Exams is Demoralizing." November 25, 2023. https://ivypanda.com/essays/cheating-on-college-exams-is-demoralizing/.
Bibliography
IvyPanda . "Cheating on College Exams is Demoralizing." November 25, 2023. https://ivypanda.com/essays/cheating-on-college-exams-is-demoralizing/.
- Exploring Junot Díaz's Narrative Nonfiction
- Sex and Death in Stoker’s Dracula
- Human vs. Divine Ego: What Is the Difference?
- "The Harvest-La Cosecha" by Angus McLennan
- The Detriment of Participation Trophies
- "Tom Torlino Student File" Photograph Analysis
- Should Pay Policies Be Secret or Open?
- “The Corporation” a Film by Mark Achbar, Jennifer Abbott and Joel Bakan
- North and South Korea Unification Process: Issues and Probability
- Male Dominance as Impeding Female Sexual Freedom
- Students Procrastination Problem
- Marginal Analysis of Cheating
- Conclusion of Smoking Should Be Banned on College Campuses Essay
- Comprehensive Sex Education
- American History: The Problem of Education in American Culture
- Undergraduate
- High School
- Architecture
- American History
- Asian History
- Antique Literature
- American Literature
- Asian Literature
- Classic English Literature
- World Literature
- Creative Writing
- Linguistics
- Criminal Justice
- Legal Issues
- Anthropology
- Archaeology
- Political Science
- World Affairs
- African-American Studies
- East European Studies
- Latin-American Studies
- Native-American Studies
- West European Studies
- Family and Consumer Science
- Social Issues
- Women and Gender Studies
- Social Work
- Natural Sciences
- Pharmacology
- Earth science
- Agriculture
- Agricultural Studies
- Computer Science
- IT Management
- Mathematics
- Investments
- Engineering and Technology
- Engineering
- Aeronautics
- Medicine and Health
- Alternative Medicine
- Communications and Media
- Advertising
- Communication Strategies
- Public Relations
- Educational Theories
- Teacher's Career
- Chicago/Turabian
- Company Analysis
- Education Theories
- Shakespeare
- Canadian Studies
- Food Safety
- Relation of Global Warming and Extreme Weather Condition
- Movie Review
- Admission Essay
- Annotated Bibliography
- Application Essay
- Article Critique
Article Review
- Article Writing
- Book Review
- Business Plan
- Business Proposal
- Capstone Project
- Cover Letter
- Creative Essay
- Dissertation
- Dissertation - Abstract
- Dissertation - Conclusion
- Dissertation - Discussion
- Dissertation - Hypothesis
- Dissertation - Introduction
- Dissertation - Literature
- Dissertation - Methodology
- Dissertation - Results
- GCSE Coursework
- Grant Proposal
- Marketing Plan
- Multiple Choice Quiz
- Personal Statement
- Power Point Presentation
- Power Point Presentation With Speaker Notes
- Questionnaire
- Reaction Paper
- Research Paper
- Research Proposal
- SWOT analysis
- Thesis Paper
- Online Quiz
- Literature Review
- Movie Analysis
- Statistics problem
- Math Problem
- All papers examples
- How It Works
- Money Back Policy
- Terms of Use
- Privacy Policy
- We Are Hiring
Cheating in Exams, Essay Example
Pages: 3
Words: 821
Hire a Writer for Custom Essay
Use 10% Off Discount: "custom10" in 1 Click 👇
You are free to use it as an inspiration or a source for your own work.
Cheating in exams can be defined as committing acts of dishonesty during an exam in order to score good grades. This is normally done by students when they fail to prepare for the exams or when they feel that the test is too hard for them and they want to score good grades.
Various acts are considered as cheating: first when a student gets access to exam papers be it part of them or all the exam papers before the exam is considered as cheating. Another way of cheating is by having materials that are not authorized in the exam room either electronic or non electronic in their reach from which the students copy or even copying answers from scripts of other candidates or allowing your script to be copied from by other candidates. Such materials include phones in which they store data; some phones have memory cards that store huge amounts of data and thus a student can even carry the whole syllabus in their phones from which they copy. Other electronic materials are calculators in which students store formulas especially for science and math exams. Science and math formulas may also be written on the desktop which they hide from their supervisors by covering with the answer sheet. Non electronic materials include small notes which the students make on something they suspect will be tested. Such writings are made on small pieces of paper, on the palm or on sole tapes which the students stick on their clothes. Another way of cheating is when a student impersonates another one and ends up doing the exam for them or even communicating with fellow candidates during an exam session. These forms don’t exhaust the many ways of cheating.
When students succeed in their first attempt of cheating they will always be tempted to repeat the act since it enables them to pass exams without struggling however this may bring serious consequences for the students. The problems may be short lived or long term. Short term consequences include being awarded a zero score by the lecturer because they believe that the candidate does not know anything. Getting a fail forces the student to repeat the unit .This means an addition on the other terms work a burden which may make the student fail other units hence causing a cycle of failing. Other lecturers punish these students by suspending them for a given period of time .Such students get it rough in explaining to their parents the reasons for being suspended. They may also become the laughing stock in the village when fellow students spread the rumours. Another short term consequence is when the lecturer forces the students to take remedial studies as others go for holiday hence denying them the opportunity to enjoy their holidays.
Long term consequences include being expelled from school. This means the student has to look for another school and hence the student delays from finishing college which consequently affects their chances in the job market because most job advertisements specify age limit. Cheating students also gain bad reputation from fellow students and lectrurers.Fellow students always see you as a liar and lecturers lose faith in you and it becomes difficulty to convince them that you didn’t cheat at times when you pass.
In the long term a student who passed her exams through cheating may have problems when it comes to delivering services in a job. This is because a student may cheat in exams, graduate from college but have difficulties when solving problems touching on their field of study in work environment since the certificates they present don’t really show their capability but what they pretend to be. When it comes to giving ideas during discussions in the office the cheaters will strain to contribute and also the manner in which they present themselves in such meetings will be affected since they fear that fellow workers will notice their dormancy. Without a question poor performance in the job will lead to job loss.
Cheating in an exam also denies a student important knowledge in their lives which they would have gained if they take their studies seriously A student may escape being caught cheating and get good grades which would sound okay but the truth is they may lie to their teachers and parents but they cannot cheat themselves .the truth will remain that they waste their money and time in college but at the end of it they wont gain any knowledge since what they show to have gained is not theirs. In some colleges like the ones offering ACCA when a candidate is caught cheating they are discontinued from doing the other papers and this may kill the student’s dream of venturing in such a field.
The consequences of cheating in an exam are just too much to bear and so students should avoid such instances by ensuring they revise utilise their time well and revise thoroughly for their exams.
Stuck with your Essay?
Get in touch with one of our experts for instant help!
Abortion Is Murder of Innocent, Defenseless and Helpless, Article Review Example
Conceptualizing a Business, Essay Example
Time is precious
don’t waste it!
Plagiarism-free guarantee
Privacy guarantee
Secure checkout
Money back guarantee
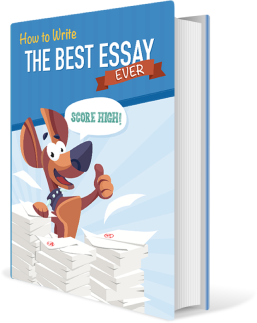
Related Essay Samples & Examples
Voting as a civic responsibility, essay example.
Pages: 1
Words: 287
Utilitarianism and Its Applications, Essay Example
Words: 356
The Age-Related Changes of the Older Person, Essay Example
Pages: 2
Words: 448
The Problems ESOL Teachers Face, Essay Example
Pages: 8
Words: 2293
Should English Be the Primary Language? Essay Example
Pages: 4
Words: 999
The Term “Social Construction of Reality”, Essay Example
Words: 371
- No category
Essay on Cheating in EXAM
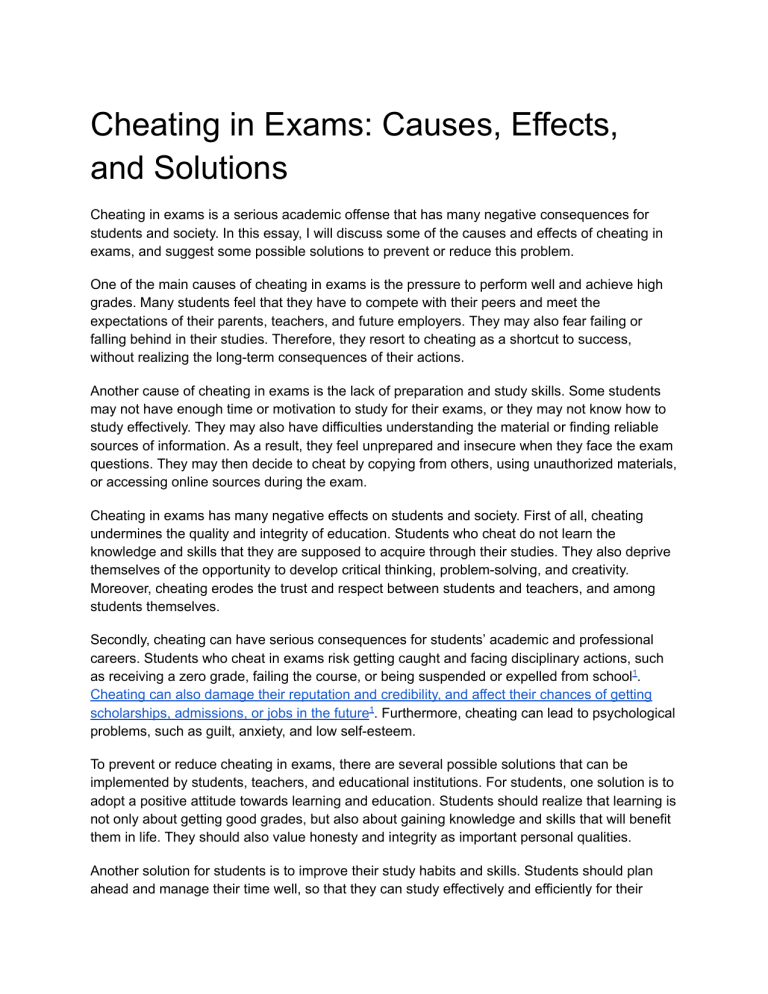
Related documents
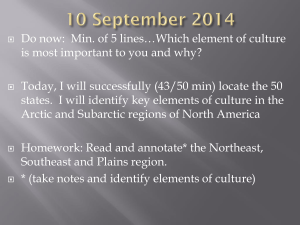
Add this document to collection(s)
You can add this document to your study collection(s)
Add this document to saved
You can add this document to your saved list
Suggest us how to improve StudyLib
(For complaints, use another form )
Input it if you want to receive answer
Paragraph on Cheating in Exam – by Rajan Karle
Introduction:
Cheating in exam has become a serious issue these days. Exam plays an important role in every student’s life.
Unfortunately, some circumstances come up that lead student to start cheating in exam.
The actual and foremost reason that drives students for cheating in exam is the desire to secure higher scores. It has found that many students start cheating in exam only because they are pressurized to score good marks; this pressure might come from any direction, from their family, teachers, relatives, or any other person who has direct impact on his or her career.
They get frightened with the feelings of getting punished by the parents, receiving insulting look from the friends or getting snubbed in front of the relatives. Under this kind of pressure, they cannot imagine the bright future.
ADVERTISEMENTS:
One more thing that drives students for cheating in exam is to see the higher scores obtained by other classmates. As a part of human nature, it feels bad when someone of our age does a good job and people start making comparisons. One of the most common things that drive students for cheating in exam is the inability to prepare well for the exams. Some students actually possess the low brain power or IQ that makes them completely blank during the exams.
Prevention:
It is necessary to make them realize the power hidden inside them. Psychological studies have shown that the students who secure average or higher marks in exam have positive attitude. They consider exams as an opportunity to shape their goals. Conversely, the students who cannot perform well in the exams have a little different approach to face the exams. Once this approach is changed via concentration, counseling and hard work, they can also perform even better than the studious students. Severe punishment to be ensured examination centers where rampant cheating takes place must be scrapped and action should be taken against the authorities.
Conclusion:
Whatever the reasons are; cheating in exam is always considered as a mal practice. A few marks obtained through the hard work have more importance than the larger marks obtained by cheating in exam. Cheating might help at the outset but it contains a lot of long-standing impact on future. As no knowledge is gained through cheating in examination.
Cheating in exam is not a good habit and it must be controlled at its starting face. This can be achieved in many ways. First of all, parents should stop burdening their child to score good marks. Giving complete freedom to a child will definitely result in gradual but growing progress. Another thing to follow is to stop making comparisons among two students.
Instead, it is preferable to generate self-confidence in his or her mind. There should be a separate lecture in every school or college that can pass the message of being honest into the minds of students. The disadvantages of cheating in exam should be highlighted in front of the students at least once in a month. It is pretty simple to restore good morals inside a child at his or her younger age.
Related Articles:
- Short Paragraph on Exam Phobia – by Supriya
- Paragraph on the Days of Preparation for Exam – By Silki
- Paragraph on a Unhappy Day of My Life – by Rajan
- Short Paragraph on Respect for Teachers
Re-evaluating GPT-4’s bar exam performance
- Original Research
- Open access
- Published: 30 March 2024
Cite this article
You have full access to this open access article
- Eric Martínez ORCID: orcid.org/0000-0003-2180-6268 1
79 Accesses
137 Altmetric
Explore all metrics
Perhaps the most widely touted of GPT-4’s at-launch, zero-shot capabilities has been its reported 90th-percentile performance on the Uniform Bar Exam. This paper begins by investigating the methodological challenges in documenting and verifying the 90th-percentile claim, presenting four sets of findings that indicate that OpenAI’s estimates of GPT-4’s UBE percentile are overinflated. First, although GPT-4’s UBE score nears the 90th percentile when examining approximate conversions from February administrations of the Illinois Bar Exam, these estimates are heavily skewed towards repeat test-takers who failed the July administration and score significantly lower than the general test-taking population. Second, data from a recent July administration of the same exam suggests GPT-4’s overall UBE percentile was below the 69th percentile, and \(\sim\) 48th percentile on essays. Third, examining official NCBE data and using several conservative statistical assumptions, GPT-4’s performance against first-time test takers is estimated to be \(\sim\) 62nd percentile, including \(\sim\) 42nd percentile on essays. Fourth, when examining only those who passed the exam (i.e. licensed or license-pending attorneys), GPT-4’s performance is estimated to drop to \(\sim\) 48th percentile overall, and \(\sim\) 15th percentile on essays. In addition to investigating the validity of the percentile claim, the paper also investigates the validity of GPT-4’s reported scaled UBE score of 298. The paper successfully replicates the MBE score, but highlights several methodological issues in the grading of the MPT + MEE components of the exam, which call into question the validity of the reported essay score. Finally, the paper investigates the effect of different hyperparameter combinations on GPT-4’s MBE performance, finding no significant effect of adjusting temperature settings, and a significant effect of few-shot chain-of-thought prompting over basic zero-shot prompting. Taken together, these findings carry timely insights for the desirability and feasibility of outsourcing legally relevant tasks to AI models, as well as for the importance for AI developers to implement rigorous and transparent capabilities evaluations to help secure safe and trustworthy AI.
Similar content being viewed by others
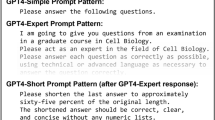
The model student: GPT-4 performance on graduate biomedical science exams
Daniel Stribling, Yuxing Xia, … Rolf Renne
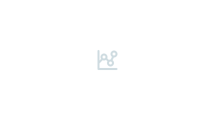
No Surprises
Benchmarking Partial Credit Grading Algorithms for Proof Blocks Problems
Avoid common mistakes on your manuscript.
1 Introduction
On March 14th, 2023, OpenAI launched GPT-4, said to be the latest milestone in the company’s effort in scaling up deep learning (OpenAI 2023a ). As part of its launch, OpenAI revealed details regarding the model’s “human-level performance on various professional and academic benchmarks” (OpenAI 2023a ). Perhaps none of these capabilities was as widely publicized as GPT-4’s performance on the Uniform Bar Examination, with OpenAI prominently displaying on various pages of its website and technical report that GPT-4 scored in or around the “90th percentile,” (OpenAI 2023a , b , n.d.) or “the top 10% of test-takers,” (OpenAI 2023a , b ) and various prominent media outlets (Koetsier 2023 ; Caron 2023 ; Weiss 2023 ; Wilkins 2023 ; Patrice 2023 ) and legal scholars (Schwarcz and Choi 2023 ) resharing and discussing the implications of these results for the legal profession and the future of AI.
Of course, assessing the capabilities of an AI system as compared to those of a human is no easy task (Hernandez-Orallo 2020 ; Burden and Hernández-Orallo 2020 ; Raji et al. 2021 ; Bowman 2022 , 2023 ; Kojima et al. 2022 ), and in the context of the legal profession specifically, there are various reasons to doubt the usefulness of the bar exam as a proxy for lawyerly competence (both for humans and AI systems), given that, for example: (a) the content on the UBE is very general and does not pertain to the legal doctrine of any jurisdiction in the United States (National Conference of Bar Examiners n.d.-h), and thus knowledge (or ignorance) of that content does not necessarily translate to knowledge (or ignorance) of relevant legal doctrine for a practicing lawyer of any jurisdiction; and (b) the tasks involved on the bar exam, particularly multiple-choice questions, do not reflect the tasks of practicing lawyers, and thus mastery (or lack of mastery) of those tasks does not necessarily reflect mastery (or lack of mastery) of the tasks of practicing lawyers.
Moreover, although the UBE is a closed-book exam for humans, GPT-4’s huge training corpus largely distilled in its parameters means that it can effectively take the UBE “open-book”, indicating that UBE may not only be an accurate proxy for lawyerly comptetence but is also likely to provide an overly favorable estimate of GPT-4’s lawyerly capabilities relative to humans.
Notwithstanding these concerns, the bar exam results appeared especially startling compared to GPT-4’s other capabilities, for various reasons. Aside from the sheer complexity of the law in form (Martinez et al. 2022a , b , in press) and content (Katz and Bommarito 2014 ; Ruhl et al. 2017 ; Bommarito and Katz 2017 ), the first is that the boost in performance of GPT-4 over its predecessor GPT-3.5 (80 percentile points) far exceeded that of any other test, including seemingly related tests such as the LSAT (40 percentile points), GRE verbal (36 percentile points), and GRE Writing (0 percentile points) (OpenAI 2023b , n.d.).
The second is that half of the Uniform Bar Exam consists of writing essays (National Conference of Bar Examiners n.d.-h), Footnote 1 and GPT-4 seems to have scored much lower on other exams involving writing, such as AP English Language and Composition (14th–44th percentile), AP English Literature and Composition (8th–22nd percentile) and GRE Writing ( \(\sim\) 54th percentile) (OpenAI 2023a , b ). In each of these three exams, GPT-4 failed to achieve a higher percentile performance over GPT-3.5, and failed to achieve a percentile score anywhere near the 90th percentile.
Moreover, in its technical report, GPT-4 claims that its percentile estimates are “conservative” estimates meant to reflect “the lower bound of the percentile range,” (OpenAI 2023b , p. 6) implying that GPT-4’s actual capabilities may be even greater than its estimates.
Methodologically, however, there appear to be various uncertainties related to the calculation of GPT’s bar exam percentile. For example, unlike the administrators of other tests that GPT-4 took, the administrators of the Uniform Bar Exam (the NCBE as well as different state bars) do not release official percentiles of the UBE (JD Advising n.d.-b; Examiner n.d.-b), and different states in their own releases almost uniformly report only passage rates as opposed to percentiles (National Conference of Bar Examiners n.d.-c; The New York State Board of Law Examiners n.d.), as only the former are considered relevant to licensing requirements and employment prospects.
Furthermore, unlike its documentation for the other exams it tested (OpenAI 2023b , p. 25), OpenAI’s technical report provides no direct citation for how the UBE percentile was computed, creating further uncertainty over both the original source and validity of the 90th percentile claim.
The reliability and transparency of this estimate has important implications on both the legal practice front and AI safety front. On the legal practice front, there is great debate regarding to what extent and when legal tasks can and should be automated (Winter et al. 2023 ; Crootof et al. 2023 ; Markou and Deakin 2020 ; Winter 2022 ). To the extent that capabilities estimates for generative AI in the context law are overblown, this may lead both lawyers and non-lawyers to rely on generative AI tools when they otherwise wouldn’t and arguably shouldn’t, plausibly increasing the prevalence of bad legal outcomes as a result of (a) judges misapplying the law; (b) lawyers engaging in malpractice and/or poor representation of their clients; and (c) non-lawyers engaging in ineffective pro se representation.
Meanwhile, on the AI safety front, there appear to be growing concerns of transparency Footnote 2 among developers of the most powerful AI systems (Ray 2023 ; Stokel-Walker 2023 ). To the extent that transparency is important to ensuring the safe deployment of AI, a lack of transparency could undermine our confidence in the prospect of safe deployment of AI (Brundage et al. 2020 ; Li et al. 2023 ). In particular, releasing models without an accurate and transparent assessment of their capabilities (including by third-party developers) might lead to unexpected misuse/misapplication of those models (within and beyond legal contexts), which might have detrimental (perhaps even catastrophic) consequences moving forward (Ngo 2022 ; Carlsmith 2022 ).
Given these considerations, this paper begins by investigating some of the key methodological challenges in verifying the claim that GPT-4 achieved 90th percentile performance on the Uniform Bar Examination. The paper’s findings in this regard are fourfold. First, although GPT-4’s UBE score nears the 90th percentile when examining approximate conversions from February administrations of the Illinois Bar Exam, these estimates appear heavily skewed towards those who failed the July administration and whose scores are much lower compared to the general test-taking population. Second, using data from a recent July administration of the same exam reveals GPT-4’s percentile to be below the 69th percentile on the UBE, and \(\sim\) 48th percentile on essays. Third, examining official NCBE data and using several conservative statistical assumptions, GPT-4’s performance against first-time test takers is estimated to be \(\sim\) 62nd percentile, including 42 percentile on essays. Fourth, when examining only those who passed the exam, GPT-4’s performance is estimated to drop to \(\sim\) 48th percentile overall, and \(\sim\) 15th percentile on essays.
Next, whereas the above four findings take for granted the scaled score achieved by GPT-4 as reported by OpenAI, the paper then proceeds to investigate the validity of that score, given the importance (and often neglectedness) of replication and reproducibility within computer science and scientific fields more broadly (Cockburn et al. 2020 ; Echtler and Häußler 2018 ; Jensen et al. 2023 ; Schooler 2014 ; Shrout and Rodgers 2018 ). The paper successfully replicates the MBE score of 158, but highlights several methodological issues in the grading of the MPT + MEE components of the exam, which call into question the validity of the essay score (140).
Finally, the paper also investigates the effect of adjusting temperature settings and prompting techniques on GPT-4’s MBE performance, finding no significant effect of adjusting temperature settings on performance, and some significant effect of prompt engineering on model performance when compared to a minimally tailored baseline condition.
Taken together, these findings suggest that OpenAI’s estimates of GPT-4’s UBE percentile, though clearly an impressive leap over those of GPT-3.5, are likely overinflated, particularly if taken as a “conservative” estimate representing “the lower range of percentiles,” and even moreso if meant to reflect the actual capabilities of a practicing lawyer. These findings carry timely insights for the desirability and feasibility of outsourcing legally relevant tasks to AI models, as well as for the importance for generative AI developers to implement rigorous and transparent capabilities evaluations to help secure safer and more trustworthy AI.
2 Evaluating the 90th Percentile estimate
2.1 evidence from openai.
Investigating the OpenAI website, as well as the GPT-4 technical report, reveals a multitude of claims regarding the estimated percentile of GPT-4’s Uniform Bar Examination performance but a dearth of documentation regarding the backing of such claims. For example, the first paragraph of the official GPT-4 research page on the OpenAI website states that “it [GPT-4] passes a simulated bar exam with a score around the top 10% of test takers” (OpenAI 2023a ). This claim is repeated several times later in this and other webpages, both visually and textually, each time without explicit backing. Footnote 3
Similarly undocumented claims are reported in the official GPT-4 Technical Report. Footnote 4 Although OpenAI details the methodology for computing most of its percentiles in A.5 of the Appendix of the technical report, there does not appear to be any such documentation for the methodology behind computing the UBE percentile. For example, after providing relatively detailed breakdowns of its methodology for scoring the SAT, GRE, SAT, AP, and AMC, the report states that “[o]ther percentiles were based on official score distributions,” followed by a string of references to relevant sources (OpenAI 2023b , p. 25).
Examining these references, however, none of the sources contains any information regarding the Uniform Bar Exam, let alone its “official score distributions” (OpenAI 2023b , pp. 22–23). Moreover, aside from the Appendix, there are no other direct references to the methodology of computing UBE scores, nor any indirect references aside from a brief acknowledgement thanking “our collaborators at Casetext and Stanford CodeX for conducting the simulated bar exam” (OpenAI 2023b , p. 18).
2.2 Evidence from GPT-4 passes the bar
Another potential source of evidence for the 90th percentile claim comes from an early draft version of the paper, “GPT-4 passes the bar exam,” written by the administrators of the simulated bar exam referenced in OpenAI’s technical report (Katz et al. 2023 ). The paper is very well-documented and transparent about its methodology in computing raw and scaled scores, both in the main text and in its comprehensive appendices. Unlike the GPT-4 technical report, however, the focus of the paper is not on percentiles but rather on the model’s scaled score compared to that of the average test taker, based on publicly available NCBE data. In fact, one of the only mentions of percentiles is in a footnote, where the authors state, in passing: “Using a percentile chart from a recent exam administration (which is generally available online), ChatGPT would receive a score below the 10th percentile of test-takers while GPT-4 would receive a combined score approaching the 90th percentile of test-takers”. (Katz et al. 2023 , p. 10)
2.3 Evidence online
As explained by JD Advising (n.d.-b), The National Conference of Bar Examiners (NCBE), the organization that writes the Uniform Bar Exam (UBE) does not release UBE percentiles. Footnote 5 Because there is no official percentile chart for UBE, all generally available online estimates are unofficial. Perhaps the most prominent of such estimates are the percentile charts from pre-July 2019 Illinois bar exam. Pre-2019, Footnote 6 Illinois, unlike other states, provided percentile charts of their own exam that allowed UBE test-takers to estimate their approximate percentile given the similarity between the two exams (JD Advising n.d.-b). Footnote 7
Examining these approximate conversion charts, however, yields conflicting results. For example, although the percentile chart from the February 2019 administration of the Illinois Bar Exam estimates a score of 300 (2–3 points higher thatn GPT-4’s score) to be at the 90th percentile, this estimate is heavily skewed compared to the general population of July exam takers, Footnote 8 since the majority of those who take the February exam are repeat takers who failed the July exam (Examiner n.d.-a), Footnote 9 and repeat takers score much lower Footnote 10 and are much more likely to fail than are first-timers. Footnote 11
Indeed, examining the latest available percentile chart for the July exam estimates GPT-4’s UBE score to be \(\sim\) 68th percentile, well below the 90th percentile figure cited by OpenAI (Illinois Board of Admissions to the Bar 2018 ).
3 Towards a more accurate percentile estimate
Although using the July bar exam percentiles from the Illinois Bar would seem to yield a more accurate estimate than the February data, the July figure is also biased towards lower scorers, since approximately 23% of test takers in July nationally are estimated to be re-takers and score, for example, 16 points below first-timers on the MBE (Reshetar 2022 ). Limiting the comparison to first-timers would provide a more accurate comparison that avoids double-counting those who have taken the exam again after failing once or more.
Relatedly, although (virtually) all licensed attorneys have passed the bar, Footnote 12 not all those who take the bar become attorneys. To the extent that GPT-4’s UBE percentile is meant to reflect its performance against other attorneys, a more appropriate comparison would not only limit the sample to first-timers but also to those who achieved a passing score.
Moreover, the data discussed above is based on purely Illinois Bar exam data, which (at the time of the chart) was similar but not identical to the UBE in its content and scoring (JD Advising n.d.-b), whereas a more accurate estimate would be derived more directly from official NCBE sources.
3.1 Methods
To account for the issues with both OpenAI’s estimate as well the July estimate, more accurate estimates (for GPT-3.5 and GPT-4) were sought to be computed here based on first-time test-takers, including both (a) first-time test-takers overall, and (b) those who passed.
To do so, the parameters for a normal distribution of scores were separately estimated for the MBE and essay components (MEE + MPT), as well as the UBE score overall. Footnote 13
Assuming that UBE scores (as well as MBE and essay subscores) are normally distributed, percentiles of GPT’s score can be directly computed after computing the parameters of these distributions (i.e. the mean and standard deviation).
Thus, the methodology here was to first compute these parameters, then generate distributions with these parameters, and then compute (a) what percentage of values on these distributions are lower than GPT’s scores (to estimate the percentile against first-timers); and (b) what percentage of values above the passing threshold are lower than GPT’s scores (to estimate the percentile against qualified attorneys).
With regard to the mean, according to publicly available official NCBE data, the mean MBE score of first-time test-takers is 143.8 (Reshetar 2022 ).
As explained by official NCBE publications, the essay component is scaled to the MBE data (Albanese 2014 ), such that the two components have approximately the same mean and standard deviation (Albanese 2014 ; Illinois Board of Admissions to the Bar 2018 , 2019 ). Thus, the methodology here assumed that the mean first-time essay score is 143.8. Footnote 14
Given that the total UBE score is computed directly by adding MBE and essay scores (National Conference of Bar Examiners n.d.-h), an assumption was made that mean first-time UBE score is 287.6 (143.8 + 143.8).
With regard to standard deviations, information regarding the SD of first-timer scores is not publicly available. However, distributions of MBE scores for July scores (provided in 5 point-intervals) are publicly available on the NCBE website (The National Bar Examiner n.d.).
Under the assumption that first-timers have approximately the same SD as that of the general test-taking population in July, the standard deviation of first-time MBE scores was computed by (a) entering the publicly available distribution of MBE scores into R; and (b) taking the standard deviation of this distribution using the built-in sd() function (which calculates the standard deviation of a normal distribution).
Given that, as mentioned above, the distribution (mean and SD) of essay scores is the same as MBE scores, the SD for essay scores was computed similarly as above.
With regard to the UBE, Although UBE standard deviations are not publicly available for any official exam, they can be inferred from a combination of the mean UBE score for first-timers (287.6) and first-time pass rates.
For reference, standard deviations can be computed analytically as follows:
x is the quantile (the value associated with a given percentile, such as a cutoff score),
\(\mu\) is the mean,
z is the z-score corresponding to a given percentile,
\(\sigma\) is the standard deviation.
Thus, by (a) subtracting the cutoff score of a given administration ( x ) from the mean ( \(\mu\) ); and (b) dividing that by the z-score ( z ) corresponding to the percentile of the cutoff score (i.e., the percentage of people who did not pass), one is left with the standard deviation ( \(\sigma\) ).
Here, the standard deviation was calculated according to the above formula using the official first-timer mean, along with pass rate and cutoff score data from New York, which according to NCBE data has the highest number of examinees for any jurisdiction (National Conference of Bar Examiners 2023 ). Footnote 15
After obtaining these parameters, distributions of first-timer scores for the MBE component, essay component, and UBE overall were computed using the built-in rnorm function in R (which generates a normal distribution with a given mean and standard deviation).
Finally, after generating these distributions, percentiles were computed by calculating (a) what percentage of values on these distributions were lower than GPT’s scores (to estimate the percentile against first-timers); and (b) what percentage of values above the passing threshold were lower than GPT’s scores (to estimate the percentile against qualified attorneys).
With regard to the latter comparison, percentiles were computed after removing all UBE scores below 270, which is the most common score cutoff for states using the UBE (National Conference of Bar Examiners n.d.-a). To compute models’ performance on the individual components relative to qualified attorneys, a separate percentile was likewise computed after removing all subscores below 135. Footnote 16
3.2 Results
3.2.1 performance against first-time test-takers.
Results are visualized in Tables 1 and 2 . For each component of the UBE, as well as the UBE overall, GPT-4’s estimated percentile among first-time July test takers is less than that of both the OpenAI estimate and the July estimate that include repeat takers.
With regard to the aggregate UBE score, GPT-4 scored in the 62nd percentile as compared to the \(\sim\) 90th percentile February estimate and the \(\sim\) 68th percentile July estimate. With regard to MBE, GPT-4 scored in the \(\sim\) 79th percentile as compared to the \(\sim\) 95th percentile February estimate and the 86th percentile July estimate. With regard to MEE + MPT, GPT-4 scored in the \(\sim\) 42nd percentile as compared to the \(\sim\) 69th percentile February estimate and the \(\sim\) 48th percentile July estimate.
With regard to GPT-3.5, its aggregate UBE score among first-timers was in the \(\sim\) 2nd percentile, as compared to the \(\sim\) 2nd percentile February estimate and \(\sim\) 1st percentile July estimate. Its MBE subscore was in the \(\sim\) 6th percentile, compared to the \(\sim\) 10th percentile February estimate \(\sim\) 7th percentile July estimate. Its essay subscore was in the \(\sim\) 0th percentile, compared to the \(\sim\) 1st percentile February estimate and \(\sim\) 0th percentile July estimate.
3.2.2 Performance against qualified attorneys
Predictably, when limiting the sample to those who passed the bar, the models’ percentile dropped further.
With regard to the aggregate UBE score, GPT-4 scored in the \(\sim\) 45th percentile. With regard to MBE, GPT-4 scored in the \(\sim\) 69th percentile, whereas for the MEE + MPT, GPT-4 scored in the \(\sim\) 15th percentile.
With regard to GPT-3.5, its aggregate UBE score among qualified attorneys was 0th percentile, as were its percentiles for both subscores (Table 3 ).
4 Re-evaluating the raw score
So far, this analysis has taken for granted the scaled score achieved by GPT-4 as reported by OpenAI—that is, assuming GPT-4 scored a 298 on the UBE, is the 90th-percentile figure reported by OpenAI warranted?
However, given calls for the replication and reproducibility within the practice of science more broadly (Cockburn et al. 2020 ; Echtler and Häußler 2018 ; Jensen et al. 2023 ; Schooler 2014 ; Shrout and Rodgers 2018 ), it is worth scrutinizing the validity of the score itself—that is, did GPT-4 in fact score a 298 on the UBE?
Moreover, given the various potential hyperparameter settings available when using GPT-4 and other LLMs, it is worth assessing whether and to what extent adjusting such settings might influence the capabilities of GPT-4 on exam performance.
To that end, this section first attempts to replicate the MBE score reported by OpenAI ( 2023a ) and Katz et al. ( 2023 ) using methods as close to the original paper as reasonably feasible.
The section then attempts to get a sense of the floor and ceiling of GPT-4’s out-of-the-box capabilities by comparing GPT-4’s MBE performance using the best and worst hyperparameter settings.
Finally, the section re-examines GPT-4’s performance on the essays, evaluating (a) the extent to which the methodology of grading GPT-4’s essays deviated that from official protocol used by the National Conference of Bar Examiners during actual bar exam administrations; and (b) the extent to which such deviations might undermine one’s confidence in the the scaled essay scores reported by OpenAI ( 2023a ) and Katz et al. ( 2023 ).
4.1 Replicating the MBE score
4.1.1 methodology.
As in Katz et al. ( 2023 ), the materials used here were the official MBE questions released by the NCBE. The materials were purchased and downloaded in pdf format from an authorized NCBE reseller. Afterwards, the materials were converted into TXT format, and text analysis tools were used to format the questions in a way that was suitable for prompting, following Katz et al. ( 2023 ).
To replicate the MBE score reported by OpenAI ( 2023a ), this paper followed the protocol documented by Katz et al. ( 2023 ), with some minor additions for robustness purposes.
In Katz et al. ( 2023 ), the authors tested GPT-4’s MBE performance using three different temperature settings: 0, .5 and 1. For each of these temperature settings, GPT-4’s MBE performance was tested using two different prompts, including (1) a prompt where GPT was asked to provide a top-3 ranking of answer choices, along with a justification and authority/citation for its answer; and (2) a prompt where GPT-4 was asked to provide a top-3 ranking of answer choices, without providing a justification or authority/citation for its answer.
For each of these prompts, GPT-4 was also told that it should answer as if it were taking the bar exam.
For each of these prompts / temperature combinations, Katz et al. ( 2023 ) tested GPT-4 three different times (“experiments” or “trials”) to control for variation.
The minor additions to this protocol were twofold. First, GPT-4 was tested under two additional temperature settings: .25 and .7. This brought the total temperature / prompt combinations to 10 as opposed to 6 in the original paper.
Second, GPT-4 was tested 5 times under each temperature / prompt combination as opposed to 3 times, bringing the total number of trials to 50 as opposed to 18.
After prompting, raw scores were computed using the official answer key provided by the exam. Scaled scores were then computed following the method outlined in JD Advising (n.d.-a), by (a) multiplying the number of correct answers by 190, and dividing by 200; and (b) converting the resulting number to a scaled score using a conversion chart based on official NCBE data.
After scoring, scores from the replication trials were analyzed in comparison to those from Katz et al. ( 2023 ) using the data from their publicly available github repository.
To assess whether there was a significant difference between GPT-4’s accuracy in the replication trials as compared to the Katz et al. ( 2023 ) paper, as well as to assess any significant effect of prompt type or temperature, a mixed-effects binary logistic regression was conducted with: (a) paper (replication vs original), temperature and prompt as fixed effects Footnote 17 ; and (b) question number and question category as random effects. These regressions were conducted using the lme4 (Bates et al. 2014 ) and lmertest (Kuznetsova et al. 2017 ) packages from R.
4.1.2 Results
Results are visualized in Table 4 . Mean MBE accuracy across all trials in the replication here was 75.6% (95% CI: 74.7 to 76.4), whereas the mean accuracy across all trials in Katz et al. ( 2023 ) was 75.7% (95% CI: 74.2 to 77.1). Footnote 18
The regression model did not reveal a main effect of “paper” on accuracy ( \(p=.883\) ), indicating that there was no significant difference between GPT-4’s raw accuracy as reported by Katz et al. ( 2023 ) and GPT-4’s raw accuracy as performed in the replication here.
There was also no main effect of temperature ( \(p>.1\) ) Footnote 19 or prompt ( \(p=.741\) ). That is, GPT-4’s raw accuracy was not significantly higher or lower at a given temperature setting or when fed a certain prompt as opposed to another (among the two prompts used in Katz et al. ( 2023 ) and the replication here) (Table 5 ).
4.2 Assessing the effect of hyperparameters
4.2.1 methods.
Although the above analysis found no effect of prompt on model performance, this could be due to a lack of variety of prompts used by Katz et al. ( 2023 ) in their original analysis.
To get a better sense of whether prompt engineering might have any effect on model performance, a follow-up experiment compared GPT-4’s performance in two novel conditions not tested in the original (Katz et al. 2023 ) paper.
In Condition 1 (“minimally tailored” condition), GPT-4 was tested using minimal prompting compared to Katz et al. ( 2023 ), both in terms of formatting and substance.
In particular, the message prompt in Katz et al. ( 2023 ) and the above replication followed OpenAI’s Best practices for prompt engineering with the API (Shieh 2023 ) through the use of (a) helpful markers (e.g. ‘```’) to separate instruction and context; (b) details regarding the desired output (i.e. specifying that the response should include ranked choices, as well as [in some cases] proper authority and citation; (c) an explicit template for the desired output (providing an example of the format in which GPT-4 should provide their response); and (d) perhaps most crucially, context regarding the type of question GPT-4 was answering (e.g. “please respond as if you are taking the bar exam”).
In contrast, in the minimally tailored prompting condition, the message prompt for a given question simply stated “Please answer the following question,” followed by the question and answer choices (a technique sometimes referred to as “basic prompting”: Choi et al., 2023 ). No additional context or formatting cues were provided.
In Condition 2 (“maximally tailored” condition), GPT-4 was tested using the highest performing prompt settings as revealed in the replication section above, with one addition, namely that: the system prompt, similar to the approaches used in Choi ( 2023 ), Choi et al. ( 2023 ), was edited from its default (“you are a helpful assistant”) to a more tailored message that included included multiple example MBE questions with sample answer and explanations structured in the desired format (a technique sometimes referred to as “few-shot prompting”: Choi et al. ( 2023 )).
As in the replication section, 5 trials were conducted for each of the two conditions. Based on the lack of effect of temperature in the replication study, temperature was not a manipulated variable. Instead, both conditions featured the same temperature setting (.5).
To assess whether there was a significant difference between GPT-4’s accuracy in the maximally tailored vs minimally tailored conditions, a mixed-effects binary logistic regression was conducted with: (a) condition as a fixed effect; and (b) question number and question category as random effects. As above, these regressions were conducted using the lme4 (Bates et al. 2014 ) and lmertest (Kuznetsova et al. 2017 ) packages from R.
4.2.2 Results
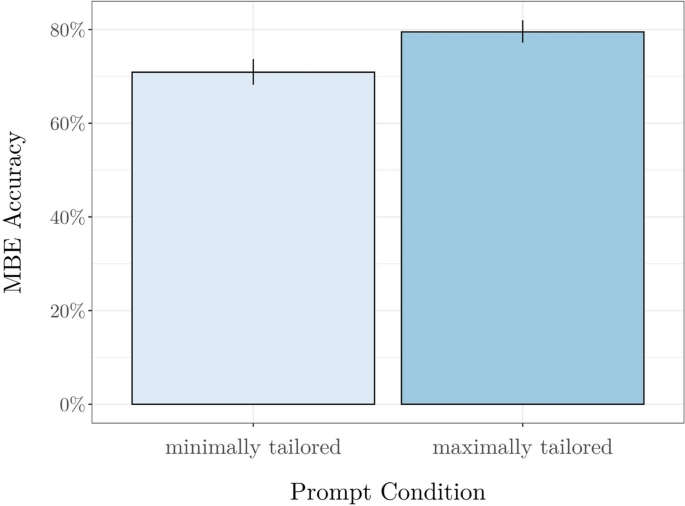
GPT-4’s MBE Accuracy in minimally tailored vs. maximally tailored prompting conditions. Bars reflect the mean accuracy. Lines correspond to 95% bootstrapped confidence intervals
Mean MBE accuracy across all trials in the maximally tailored condition was descriptively higher at 79.5% (95% CI: 77.1–82.1), than in the minimally tailored condition at 70.9% (95% CI: 68.1–73.7).
The regression model revealed a main effect of condition on accuracy ( \(\beta =1.395\) , \(\textrm{SE} =.192\) , \(p<.0001\) ), such that GPT-4’s accuracy in the maximally tailored condition was significantly higher than its accuracy in the minimally tailored condition.
In terms of scaled score, GPT-4’s MBE score in the minimally tailored condition would be approximately 150, which would place it: (a) in the 70th percentile among July test takers; (b) 64th percentile among first-timers; and (c) 48th percentile among those who passed.
GPT-4’s score in the maximally tailored condition would be approximately 164—6 points higher than that reported by Katz et al. ( 2023 ) and OpenAI ( 2023a ). This would place it: (a) in the 95th percentile among July test takers; (b) 87th percentile among first-timers; and (c) 82th percentile among those who passed.
4.3 Re-examining the essay scores
As confirmed in the above subsection, the scaled MBE score (not percentile) reported by OpenAI was accurately computed using the methods documented in Katz et al. ( 2023 ).
With regard to the essays (MPT + MEE), however, the method described by the authors significantly deviates in at least three aspects from the official method used by UBE states, to the point where one may not be confident that the essay scores reported by the authors reflect GPT models’ “true” essay scores (i.e., the score that essay examiners would have assigned to GPT had they been blindly scored using official grading protocol).
The first aspect relates to the (lack of) use of a formal rubric. For example, unlike NCBE protocol, which provides graders with (a) (in the case of the MEE) detailed “grading guidelines” for how to assign grades to essays and distinguish answers for a given MEE; and (b) (for both MEE and MPT) a specific “drafters’ point sheet” for each essay that includes detailed guidance from the drafting committee with a discussion of the issues raised and the intended analysis (Olson 2019 ), Katz et al. ( 2023 ) do not report using an official or unofficial rubric of any kind, and instead simply describe comparing GPT-4’s answers to representative “good” answers from the state of Maryland.
Utilizing these answers as the basis for grading GPT-4’s answers in lieu of a formal rubric would seem to be particularly problematic considering it is unclear even what score these representative “good” answers received. As clarified by the Maryland bar examiners: “The Representative Good Answers are not ‘average’ passing answers nor are they necessarily ‘perfect’ answers. Instead, they are responses which, in the Board’s view, illustrate successful answers written by applicants who passed the UBE in Maryland for this session” (Maryland State Board of Law Examiners 2022 ).
Given that (a) it is unclear what score these representative good answers received; and (b) these answers appear to be the basis for determining the score that GPT-4’s essays received, it would seem to follow that (c) it is likewise unclear what score GPT-4’s answers should receive. Consequently, it would likewise follow that any reported scaled score or percentile would seem to be insufficiently justified so as to serve as a basis for a conclusive statement regarding GPT-4’s relative performance on essays as compared to humans (e.g. a reported percentile).
The second aspect relates to the lack of NCBE training of the graders of the essays. Official NCBE essay grading protocol mandates the use of trained bar exam graders, who in addition to using a specific rubric for each question undergo a standardized training process prior to grading (Gunderson 2015 ; Case 2010 ). In contrast, the graders in Katz et al. ( 2023 ) (a subset of the authors who were trained lawyers) do not report expertise or training in bar exam grading. Thus, although the graders of the essays were no doubt experts in legal reasoning more broadly, it seems unlikely that they would have been sufficiently ingrained in the specific grading protocols of the MEE + MPT to have been able to reliably infer or apply the specific grading rubric when assigning the raw scores to GPT-4.
The third aspect relates to both blinding and what bar examiners refer to as “calibration,” as UBE jurisdictions use an extensive procedure to ensure that graders are grading essays in a consistent manner (both with regard to other essays and in comparison to other graders) (Case 2010 ; Gunderson 2015 ). In particular, all graders of a particular jurisdiction first blindly grade a set of 30 “calibration” essays of variable quality (first rank order, then absolute scores) and make sure that consistent scores are being assigned by different graders, and that the same score (e.g. 5 of 6) is being assigned to exams of similar quality (Case 2010 ).
Unlike this approach, as well as efforts to assess GPT models’ law school performance (Choi et al. 2021 ), the method reported by Katz et al. ( 2023 ) did not initially involve blinding. The method in Katz et al. ( 2023 ) did involve a form of inter-grader calibration, as the authors gave “blinded samples” to independent lawyers to grade the exams, with the assigned scores “match[ing] or exceed[ing]” those assigned by the authors. Given the lack of reporting to the contrary, however, the method used by the graders would presumably be plagued by issue issues as highlighted above (no rubric, no formal training with bar exam grading, no formal intra-grader calibration).
Given the above issues, as well as the fact that, as alluded in the introduction, GPT-4’s performance boost over GPT-3 on other essay-based exams was far lower than that on the bar exam, it seems warranted not only to infer that GPT-4’s relative performance (in terms of percentile among human test-takers) was lower than that reported by OpenAI, but also that GPT-4’s reported scaled score on the essay may have deviated to some degree from GPT-4’s “true” essay (which, if true, would imply that GPT-4’s “true” percentile on the bar exam may be even lower than that estimated in previous sections).
Indeed, Katz et al. ( 2023 ) to some degree acknowledge all of these limitations in their paper, writing: “While we recognize there is inherent variability in any qualitative assessment, our reliance on the state bars’ representative “good” answers and the multiple reviewers reduces the likelihood that our assessment is incorrect enough to alter the ultimate conclusion of passage in this paper”.
Given that GPT-4’s reported score of 298 is 28 points higher than the passing threshold (270) in the majority of UBE jurisdictions, it is true that the essay scores would have to have been wildly inaccurate in order to undermine the general conclusion of Katz et al. ( 2023 ) (i.e., that GPT-4 “passed the [uniform] bar exam”). However, even supposing that GPT-4’s “true” percentile on the essay portion was just a few points lower than that reported by OpenAI, this would further call into question OpenAI’s claims regarding the relative performance of GPT-4 on the UBE relative to human test-takers. For example, supposing that GPT-4 scored 9 points lower on the essays, this would drop its estimated relative performance to (a) 31st percentile compared to July test-takers; (b) 24th percentile relative to first-time test takers; and (c) less than 5th percentile compared to licensed attorneys.
5 Discussion
This paper first investigated the issue of OpenAI’s claim of GPT-4’s 90th percentile UBE performance, resulting in four main findings. The first finding is that although GPT-4’s UBE score approaches the 90th percentile when examining approximate conversions from February administrations of the Illinois Bar Exam, these estimates are heavily skewed towards low scorers, as the majority of test-takers in February failed the July administration and tend to score much lower than the general test-taking population. The second finding is that using July data from the same source would result in an estimate of \(\sim\) 68th percentile, including below average performance on the essay portion. The third finding is that comparing GPT-4’s performance against first-time test takers would result in an estimate of \(\sim\) 62nd percentile, including \(\sim\) 42nd percentile on the essay portion. The fourth main finding is that when examining only those who passed the exam, GPT-4’s performance is estimated to drop to \(\sim\) 48th percentile overall, and \(\sim\) 15th percentile on essays.
In addition to these four main findings, the paper also investigated the validity of GPT-4’s reported UBE score of 298. Although the paper successfully replicated the MBE score of 158, the paper also highlighted several methodological issues in the grading of the MPT + MEE components of the exam, which call into question the validity of the essay score (140).
Finally, the paper also investigated the effect of adjusting temperature settings and prompting techniques on GPT-4’s MBE performance, finding no significant effect of adjusting temperature settings on performance, and some effect of prompt engineering when compared to a basic prompting baseline condition.
Of course, assessing the capabilities of an AI system as compared to those of a practicing lawyer is no easy task. Scholars have identified several theoretical and practical difficulties in creating accurate measurement scales to assess AI capabilities and have pointed out various issues with some of the current scales (Hernandez-Orallo 2020 ; Burden and Hernández-Orallo 2020 ; Raji et al. 2021 ). Relatedly, some have pointed out that simply observing that GPT-4 under- or over-performs at a task in some setting is not necessarily reliable evidence that it (or some other LLM) is capable or incapable of performing that task in general (Bowman 2022 , 2023 ; Kojima et al. 2022 ).
In the context of legal profession specifically, there are various reasons to doubt the usefulness of UBE percentile as a proxy for lawyerly competence (both for humans and AI systems), given that, for example: (a) the content on the UBE is very general and does not pertain to the legal doctrine of any jurisdiction in the United States (National Conference of Bar Examiners n.d.-g), and thus knowledge (or ignorance) of that content does not necessarily translate to knowledge (or ignorance) of relevant legal doctrine for a practicing lawyer of any jurisdiction; (b) the tasks involved on the bar exam, particularly multiple-choice questions, do not reflect the tasks of practicing lawyers, and thus mastery (or lack of mastery) of those tasks does not necessarily reflect mastery (or lack of mastery) of the tasks of practicing lawyers; and (c) given the lack of direct professional incentive to obtain higher than a passing score (typically no higher than 270) (National Conference of Bar Examiners n.d.-a), obtaining a particularly high score or percentile past this threshold is less meaningful than for other exams (e.g. LSAT), where higher scores are taken into account for admission into select institutions (US News and World Report 2022 ).
Setting these objections aside, however, to the extent that one believes the UBE to be a valid proxy for lawyerly competence, these results suggest GPT-4 to be substantially less lawyerly competent than previously assumed, as GPT-4’s score against likely attorneys (i.e. those who actually passed the bar) is \(\sim\) 48th percentile. Moreover, when just looking at the essays, which more closely resemble the tasks of practicing lawyers and thus more plausibly reflect lawyerly competence, GPT-4’s performance falls in the bottom \(\sim\) 15th percentile. These findings align with recent research work finding that GPT-4 performed below-average on law school exams (Blair-Stanek et al. 2023 ).
The lack of precision and transparency in OpenAI’s reporting of GPT-4’s UBE performance has implications for both the current state of the legal profession and the future of AI safety. On the legal side, there appear to be at least two sets of implications. On the one hand, to the extent that lawyers put stock in the bar exam as a proxy for general legal competence, the results might give practicing lawyers at least a mild temporary sense of relief regarding the security of the profession, given that the majority of lawyers perform better than GPT on the component of the exam (essay-writing) that seems to best reflect their day-to-day activities (and by extension, the tasks that would likely need to be automated in order to supplant lawyers in their day-to-day professional capacity).
On the other hand, the fact that GPT-4’s reported “90th percentile” capabilities were so widely publicized might pose some concerns that lawyers and non-lawyers may use GPT-4 for complex legal tasks for which it is incapable of adequately performing, plausibly increasing the rate of (a) misapplication of the law by judges; (b) professional malpractice by lawyers; and (c) ineffective pro se representation and/or unauthorized practice of law by non-lawyers. From a legal education standpoint, law students who overestimate GPT-4’s UBE capabilities might also develop an unwarranted sense of apathy towards developing critical legal-analytical skills, particularly if under the impression that GPT-4’s level of mastery of those skills already surpasses that to which a typical law student could be expected to reach.
On the AI front, these findings raise concerns both for the transparency Footnote 20 of capabilities research and the safety of AI development more generally. In particular, to the extent that one considers transparency to be an important prerequisite for safety (Brundage et al. 2020 ), these findings underscore the importance of implementing rigorous transparency measures so as to reliably identify potential warning signs of transformative progress in artificial intelligence as opposed to creating a false sense of alarm or security (Zoe et al. 2021 ). Implementing such measures could help ensure that AI development, as stated in OpenAI’s charter, is a “value-aligned, safety-conscious project” as opposed to becoming “a competitive race without time for adequate safety precautions” (OpenAI 2018 ).
Of course, the present study does not discount the progress that AI has made in the context of legally relevant tasks; after all, the improvement in UBE performance from GPT-3.5 to GPT-4 as estimated in this study remains impressive (arguably equally or even more so given that GPT-3.5’s performance is also estimated to be significantly lower than previously assumed), even if not as flashy as the 10th–90th percentile boost of OpenAI’s official estimation. Nor does the present study discount the seemingly inevitable future improvement of AI systems to levels far beyond their present capabilities, or, as phrased in GPT-4 Passes the Bar Exam , that the present capabilities “highlight the floor, not the ceiling, of future application” (Katz et al. 2023 , 11).
To the contrary, given the inevitable rapid growth of AI systems, the results of the present study underscore the importance of implementing rigorous and transparent evaluation measures to ensure that both the general public and relevant decision-makers are made appropriately aware of the system’s capabilities, and to prevent these systems from being used in an unintentionally harmful or catastrophic manner. The results also indicate that law schools and the legal profession should prioritize instruction in areas such as law and technology and law and AI, which, despite their importance, are currently not viewed as descriptively or normatively central to the legal academy (Martínez and Tobia 2023 ).
Note that Uniform Bar Exam (UBE) has multiple components, including: (a) the Multistate Bar Exam (MBE), a 6 h, 200-question multiple choice test (National Conference of Bar Examiners n.d.-c, d) the Multistate Essay Exam (MEE), a 3 h, six-part essay exam (National Conference of Bar Examiners n.d.-e); and (c) the Multistate Practice Exam (MPT), a 3 h, two-part “closed universe” essay exam (National Conference of Bar Examiners n.d.-f). The exam is graded on a scale of 400. The MBE and essays (MEE + MPT) are each graded on a scale of 200 (National Conference of Bar Examiners n.d.-g). Thus, essays and multiple choice are each worth half of an examinee’s score.
Note that transparency here is not to be confused with the interpretability or explainability of AI systems themselves, as is often used in the AI safety literature. For a discussion of the term as used more along the lines of these senses, see (Bostrom and Yudkowsky 2018 , p. 2) (arguing that making an AI system “transparent to inspection” by the programmer is one of “many socially important properties”).
For example, near the top of the GPT-4 product page is displayed a reference to GPT-4’s 90th percentile Uniform Bar Exam performance as an illustrative example of how “GPT-4 outperforms ChatGPT by scoring in higher approximate percentiles among test-takers” (OpenAI n.d.).
As with the official website, the technical report (page 6) claims that GPT-4 “passes a simulated version of the Uniform Bar Examination with a score in the top 10% of test takers” (OpenAI 2023b ). This attested result is presented visually in Table 1 and Fig. 1 .
As the website JD Advising points out: “The National Conference of Bar Examiners (NCBE), the organization that writes the Uniform Bar Exam (UBE) does not release UBE percentiles” (JD Advising n.d.-b). Instead, the NCBE and state bar examiners tend to include in their press releases much more general and limited information, such as mean MBE scores and the percentage of test-takers who passed the exam in a given administration (Examiner n.d.-c; National Conference of Bar Examiners n.d.-c; The New York State Board of Law Examiners n.d.)
Note that Starting in July 2019, Illinois began administering the Uniform Bar Exam (University of Illinois Chicago n.d.), and accordingly stopped releasing official percentile charts. Thus, the generally available Illinois percentile charts are based on pre-UBE Illinois bar exam data.
In addition to the Illinois conversion chart, some sources often make claims about percentiles of certain scores without clarifying the source of those claims. See, for example (Lang 2023 ). There are also several generally available unofficial online calculators, which either calculate an estimated percentile of an MBE score based on official NCBE data (UBEEssays.com 2019 ), or make other non-percentile-related calculations, such as estimated scaled score (Rules.com n.d.)
For example, according to (National Conference of Bar Examiners n.d.-b), the pass rate in Illinois for the February 2023 administration was 43%, compared to 68% for the July administration.
According to (Examiner n.d.-a), for the 2021 February administration in Illinois, 284 takers were first-time takers, as compared to 426 repeaters.
For example, for the July administration, the 50th-percentile UBE-converted score was approximately 282 (Illinois Board of Admissions to the Bar 2019 ), whereas for the February exam, the 50th-percentile UBE-converted score was approximately 264 (Illinois Board of Admissions to the Bar 2019 )
For example, according to (National Conference of Bar Examiners n.d.-b), the pass rate among first-timers in the February 2023 administration in Illinois was 62%, compared to 35% for repeat takers.
One notable exception was made in 2020 due to COVID, for example, as the Supreme Court of the state of Washington granted a “diploma privilege” which allowed recent law graduates “to be admitted to the Washington State Bar Association and practice law in the state without taking the bar exam.”: (Washington State Bar Association 2020 )
A normal distribution of scores was assumed, given that (a) standardized tests are normalized and aim for a normal distribution (Kubiszyn and Borich 2016 ), (b) UBE is a standardized test, and (c) official visual estimates of MBE scores, both for February and July, appear to follow an approximately normal distribution. (The National Bar Examiner n.d.)
If anything, this assumption would lead to a conservative (that is, generous) estimate of GPT-4’s percentile, since percentiles for a given essay score tend to be slightly lower than those for a given MBE score. For example, according to the conversion chart of the Illinois bar exam for the July administration, a score of 145 on the MBE was estimated to be at the 61st percentile, while the same score on the essay component was estimated to be at the 59th percentile (Illinois Board of Admissions to the Bar 2018 )
Note that in a previous version of the paper, the standard deviation of overall UBE scores was instead computed using the estimated standard deviation of Illinois Bar exam data (estimated by feeding the values and percentiles of the July Illinois Bar exam data into an optimization function in R, using the optim() function using R’s “stats” package). This analysis was supplanted by the current method due to the latter having fewer/more plausible statistical assumptions, though both versions of the analysis yield converging results. For robustness purposes, the results of the old version can be found and replicated using the code available in the OSF repository.
Note that this assumes that all those who “failed” a subsection failed the bar overall. Since scores on the two portions of the exam are likely to be highly but not directly correlated, this assumption is implausible. However, its percentile predictions would still hold true, on average, for the two subsections—that is, to the extent that it leads to a slight underestimate of the percentile on one subsection it would lead to a commensurate overestimate on the other.
All fixed effect predictors were coded as factors, with treatment coding.
As a sanity check, note that the original mean accuracy originally reported by Katz et al. ( 2023 ) was also 75.7%, indicating that there were no errors here in reading the original data or computing the mean.
Note that because temperature was coded as a factor (categorical variable) as opposed to numeric (continuous variable), there were multiple \(\beta\) coefficients and p values (one for each level, not including the reference level). The p values for all levels were higher than .1.
As noted above, “transparency” here is not to be confused with the interpretability or explainability of the AI system, as is often used in the AI safety literature.
Albanese MA (2014) The testing column: scaling: it’s not just for fish or mountains. Bar Exam 83(4):50–56
Google Scholar
Bates D, Mächler M, Bolker B, Walker S (2014) Fitting linear mixed-effects models using LME4. arXiv preprint arXiv:1406.5823
Blair-Stanek A, Carstens A-M, Goldberg DS, Graber M, Gray DC, Stearns ML (2023) Gpt-4’s law school grades, Partnership tax b, property b-, tax b. Crim C-, Law & Econ C, Partnership Tax B, Property B-, Tax B
Bommarito MJ II, Katz DM (2017) Measuring and modeling the us regulatory ecosystem. J Stat Phys 168:1125–1135
Article Google Scholar
Bostrom N, Yudkowsky E (2018) The ethics of artificial intelligence. Artificial intelligence safety and security. Chapman and Hall/CRC, New York, pp 57–69
Bowman S (2022) The dangers of underclaiming: Reasons for caution when reporting how NLP systems fail. In: Proceedings of the 60th annual meeting of the association for computational linguistics (vol 1: Long papers) pp 7484–7499
Bowman SR (2023) Eight things to know about large language models. arXiv preprint arXiv:2304.00612
Brundage M, Avin S, Wang J, Belfield H, Krueger G, Hadfield G, et al (2020) Toward trustworthy AI development: mechanisms for supporting verifiable claims. arXiv preprint arXiv:2004.07213
Burden J, Hernández-Orallo J (2020) Exploring AI safety in degrees: generality, capability and control. In: Proceedings of the workshop on artificial intelligence safety (safeai 2020) co-located with 34th AAAI conference on artificial intelligence (AAAI 2020). pp 36–40
Carlsmith J (2022) Is power-seeking AI an existential risk? arXiv preprint arXiv:2206.13353
Caron P (2023) GPT-4 Beats 90% of aspiring lawyers on the bar exam. TaxProf Blog. https://taxprof.typepad.com/taxprof_blog/2023/03/gpt-4-beats-90-of-aspiring-lawyers-on-the-bar-exam.html . Accessed on 24 Apr 2023
Case SM (2010) Procedure for grading essays and performance tests. The Bar Examiner. https://thebarexaminer.ncbex.org/wp-content/uploads/PDFs/790410_TestingColumn.pdf
Choi JH (2023) How to use large language models for empirical legal research. J Instit Theor Econ (Forthcoming)
Choi JH, Monahan A, Schwarcz D (2023) Lawyering in the age of artificial intelligence. Available at SSRN 4626276
Choi JH, Hickman KE, Monahan AB, Schwarcz D (2021) Chatgpt goes to law school. J Legal Educ 71:387
Cockburn A, Dragicevic P, Besançon L, Gutwin C (2020) Threats of a replication crisis in empirical computer science. Commun ACM 63(8):70–79
Crootof R, Kaminski ME, Price II WN (2023) Humans in the loop. Vanderbilt Law Review, (Forthcoming)
Echtler F, Häußler M (2018) Open source, open science, and the replication crisis in HCI. Extended abstracts of the 2018 chi conference on human factors in computing systems. pp 1–8
Examiner TB (n.d.-a) First-time exam takers and repeaters in 2021. The Bar Examiner. https://thebarexaminer.ncbex.org/2021-statistics/first-time-exam-takers-and-repeaters-in-2021/ . Accessed on 24 Apr 2023
Examiner TB (n.d.-b) Statistics. The Bar Examiner. https://thebarexaminer.ncbex.org/statistics/ . Accessed on 24 Apr 2023
Gunderson JA (2015) The testing column: essay grading fundamentals. Bar Exam 84(1):54–56
Hernandez-Orallo J (2020) AI evaluation: on broken yardsticks and measurement scales. In: Workshop on evaluating evaluation of AI systems at AAAI
Illinois Board of Admissions to the Bar. (2018) https://www.ilbaradmissions.org/percentile-equivalent-charts-july-2018 . Accessed on 24 Apr 2023
Illinois Board of Admissions to the Bar. (2019) https://www.ilbaradmissions.org/percentile-equivalent-charts-february-2019 . Accessed on 24 Apr 2023
JD Advising (n.d.) MBE raw score conversion chart. https://jdadvising.com/mbe-raw-score-conversion-chart/ . Accessed on 01 Jan 2024
JD Advising. (n.d.) https://jdadvising.com/july-2018-ube-percentiles-chart/ . Accessed on 24 Apr 2023
Jensen TI, Kelly B, Pedersen LH (2023) Is there a replication crisis in finance? J Finance 78(5):2465–2518
Katz DM, Bommarito MJ, Gao S, Arredondo P (2023) GPT-4 passes the bar exam. Available at SSRN 4389233
Katz DM, Bommarito MJ (2014) Measuring the complexity of the law: the United States code. Artif Intell Law 22:337–374
Koetsier J (2023) GPT-4 Beats 90% of Lawyers Trying to Pass the Bar. Forbes. https://www.forbes.com/sites/johnkoetsier/2023/03/14/gpt-4-beats-90-of-lawyers-trying-to-pass-the-bar/?sh=b40c88d30279
Kojima T, Gu SS, Reid M, Matsuo Y, Iwasawa Y (2022) Large language models are zero-shot reasoners. arXiv preprint arXiv:2205.11916
Kubiszyn T, Borich GD (2016) Educational testing and measurement. John Wiley & Sons, Hoboken
Kuznetsova A, Brockhoff PB, Christensen RHB (2017) lmertest package: tests in linear mixed effects models. J Stat Software 82:13
Lang C (2023) What is a good bar exam score? Test Prep Insight. https://www.testprepinsight.com/what-is-a-good-bar-exam-score
Li B, Qi P, Liu B, Di S, Liu J, Pei J, Zhou B (2023) Trustworthy AI: From principles to practices. ACM Comput Surv 55(9):1–46
Markou C, Deakin S (2020) Is law computable? From rule of law to legal singularity. From Rule of Law to Legal Singularity. University of Cambridge Faculty of Law Research Paper
Martínez E, Tobia K (2023) What do law professors believe about law and the legal academy? Geo LJ 112:111
Martinez E, Mollica F, Gibson E (2022) Poor writing, not specialized concepts, drives processing difficulty in legal language. Cognition 224:105070
Martinez E, Mollica F, Gibson E (2022b) So much for plain language: An analysis of the accessibility of united states federal laws (1951–2009). In: Proceedings of the annual meeting of the cognitive science society, vol 44
Martinez E, Mollica F, Gibson E (in press) Even lawyers don’t like legalese. In: Proceedings of the national academy of sciences
Maryland State Board of Law Examiners (2022) July 2022 uniform bar examination (UBE) in maryland—representative good answers. https://mdcourts.gov/sites/default/files/import/ble/examanswers/2022/202207uberepgoodanswers.pdf
National Conference of Bar Examiners (2023) Bar exam results by jurisdiction. https://www.ncbex.org/statistics-research/bar-exam-results-jurisdiction . Accessed on 01 Jan 2024
National Conference of Bar Examiners (n.d.-a) https://www.ncbex.org/exams/ube/scores/ . Accessed on 03 May 2023
National Conference of Bar Examiners (n.d.-b) https://www.ncbex.org/exams/ube/score-portability/minimum-scores/ . Accessed on 24 Apr 2023
National Conference of Bar Examiners (n.d.-c) Bar Exam Results by Jurisdiction. National Conference of Bar Examiners. https://www.ncbex.org/statistics-and-research/bar-exam-results/ . Accessed on 24 Apr 2023
National Conference of Bar Examiners (n.d.-d) Multistate bar exam. https://www.ncbex.org/exams/mbe . Accessed on 01 Jan 2024
National Conference of Bar Examiners (n.d.-e) Multistate essay exam. https://www.ncbex.org/exams/mee . Accessed on 01 Jan 2024
National Conference of Bar Examiners (n.d.-f) Multistate performance test. https://www.ncbex.org/exams/mpt . Accessed on 01 Jan 2024
National Conference of Bar Examiners (n.d.-g) Uniform bar exam. Accessed on 01 Jan 2024
National Conference of Bar Examiners (n.d.-h) Uniform Bar Examination. National Conference of Bar Examiners. https://www.ncbex.org/exams/ube/ . Accessed on 24 Apr 2023
Ngo R (2022) The alignment problem from a deep learning perspective. arXiv preprint arXiv:2209.00626
Olson S (2019) 13 best practices for grading essays and performance tests. Bar Exam 88(4):8–14
OpenAI (2018) OpenAI Charter. https://openai.com/charter
OpenAI (2023) GPT 4. https://openai.com/research/gpt-4 . Accessed on 24 Apr 2023
OpenAI (2023) GPT-4 Technical Report. arXiv:2303.08774 . (Preprint submitted to arXiv)
OpenAI (n.d.) GPT-4 is OpenAI’s most advanced system, producing safer and more useful responses. https://openai.com/product/gpt-4 . Accessed on 24 Apr 2023
Patrice J (2023) New GPT-4 Passes All Sections Of The Uniform Bar Exam. Maybe This Will Finally Kill The Bar Exam. Above the Law. https://abovethelaw.com/2023/03/new-gpt-4-passes-all-sections-of-the-uniform-bar-exam-maybe-this-will-finally-kill-the-bar-exam/
Raji ID, Bender EM, Paullada A, Denton E, Hanna A (2021) Ai and the everything in the whole wide world benchmark. arXiv preprint arXiv:2111.15366
Ray T (2023) With GPT-4, OpenAI opts for secrecy versus disclosure. ZDNet. https://www.zdnet.com/article/with-gpt-4-openai-opts-for-secrecy-versus-disclosure/
Reshetar R (2022) The testing column: Why are February bar exam pass rates lower than July pass rates? Bar Exam 91(1):51–53
Ruhl J, Katz DM, Bommarito MJ (2017) Harnessing legal complexity. Science 355(6332):1377–1378
Rules.com M (n.d.) Bar Exam Calculators. https://mberules.com/bar-exam-calculators/?__cf_chl_tk=lTwxFyYWOZqBwTAenLs0TzDfAuvawkHeH2GaXU1PQo0-1683060961-0-gaNycGzNDBA . Accessed on 02 May 2023
Schooler JW (2014) Metascience could rescue the replication crisis. Nature 515(7525):9
Schwarcz D, Choi JH (2023) Ai tools for lawyers: a practical guide. Available at SSRN
Shieh J (2023) Best practices for prompt engineering with openai api. https://help.openai.com/en/articles/6654000-best-practices-for-prompt-engineering-with-openai-api . OpenAI. Accessed on 01 Jan 2024
Shrout PE, Rodgers JL (2018) Psychology, science, and knowledge construction: broadening perspectives from the replication crisis. Ann Rev Psychol 69:487–510
Stokel-Walker C (2023) Critics denounce a lack of transparency around GPT-4’s tech. Fast Company. https://www.fastcompany.com/90866190/critics-denounce-a-lack-of-transparency-around-gpt-4s-tech
The National Bar Examiner (n.d.) https://thebarexaminer.ncbex.org/2022-statistics/the-multistate-bar-examination-mbe/#step3 . Accessed on 24 Apr 2023
The New York State Board of Law Examiners (n.d.) NYS Bar Exam Statistics. The New York State Board of Law Examiners. https://www.nybarexam.org/examstats/estats.htm
UBEEssays.com. (2019) https://ubeessays.com/feb-mbe-percentiles/
University of Illinois Chicago (n.d.) https://law.uic.edu/student-support/academic-achievement/bar-exam-information/illinois-bar-exam/ . Accessed on 24 Apr 2023
US News and World Report (2022) https://www.usnews.com/best-graduate-schools/top-law-schools/law-rankings
Washington State Bar Association (2020) https://wsba.org/news-events/latest-news/news-detail/2020/06/15/state-supreme-court-grants-diploma-privilege . Accessed on 24 Apr 2023
Weiss DC (2023) Latest version of ChatGPT aces bar exam with score nearing 90th percentile. ABA Journal. https://www.abajournal.com/web/article/latest-version-of-chatgpt-aces-the-bar-exam-with-score-in-90th-percentile . Accessed on 24 Apr 2023
Wilkins S (2023) How GPT-4 mastered the entire bar exam, and why that matters. Law.com. https://www.law.com/legaltechnews/2023/03/17/how-gpt-4-mastered-the-entire-bar-exam-and-why-that-matters/?slreturn=20230324023302 . Accessed on 24 Apr 2023
Winter CK (2022) The challenges of artificial judicial decision-making for liberal democracy. Judicial decision-making: Integrating empirical and theoretical perspectives. Springer, Berlin, pp 179–204
Winter C, Hollman N, Manheim D (2023) Value alignment for advanced artificial judicial intelligence. Am Philos Quart 60(2):187–203
Zoe Cremer C, Whittlestone J (2021) Artificial canaries: early warning signs for anticipatory and democratic governance of AI
Download references
Acknowledgements
Acknowledgements omitted for anonymous review.
'Open Access funding provided by the MIT Libraries'.
Author information
Authors and affiliations.
Department of Brain and Cognitive Sciences, Massachusetts Institute of Technology (MIT), Cambridge, MA, 02138, USA
Eric Martínez
You can also search for this author in PubMed Google Scholar
Corresponding author
Correspondence to Eric Martínez .
Ethics declarations
Conflict of interest.
The author declares no financial nor non-financial interests that are directly or indirectly related to the work submitted for publication.
Additional information
Note that all code for this paper is available at the following repository link: https://osf.io/c8ygu/?view only=dcc617accc464491922b77414867a066 .
Rights and permissions
Open Access This article is licensed under a Creative Commons Attribution 4.0 International License, which permits use, sharing, adaptation, distribution and reproduction in any medium or format, as long as you give appropriate credit to the original author(s) and the source, provide a link to the Creative Commons licence, and indicate if changes were made. The images or other third party material in this article are included in the article's Creative Commons licence, unless indicated otherwise in a credit line to the material. If material is not included in the article's Creative Commons licence and your intended use is not permitted by statutory regulation or exceeds the permitted use, you will need to obtain permission directly from the copyright holder. To view a copy of this licence, visit http://creativecommons.org/licenses/by/4.0/ .
Reprints and permissions
About this article
Martínez, E. Re-evaluating GPT-4’s bar exam performance. Artif Intell Law (2024). https://doi.org/10.1007/s10506-024-09396-9
Download citation
Accepted : 30 January 2024
Published : 30 March 2024
DOI : https://doi.org/10.1007/s10506-024-09396-9
Share this article
Anyone you share the following link with will be able to read this content:
Sorry, a shareable link is not currently available for this article.
Provided by the Springer Nature SharedIt content-sharing initiative
- Legal analytics
- Natural language processing
- Machine learning
- Artificial intelligence
- Artificial intelligence and law
- Law and technology
- Legal profession
- Find a journal
- Publish with us
- Track your research
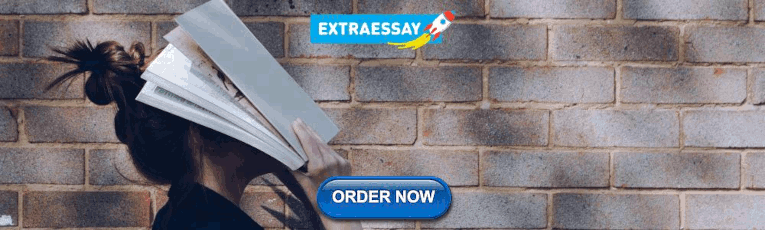
IMAGES
VIDEO
COMMENTS
In the education fraternity, cheating entails: copying from someone, Plagiarizing of academic work and paying someone to do your homework. There are numerous reasons why students cheat in exams however; this action elicits harsh repercussions if one is caught. This may include: suspension, dismissal and/or cancellation of marks (Davis, Grover ...
But students also rationalize cheating on assignments they see as having value. High-achieving students who feel pressured to attain perfection (and Ivy League acceptances) may turn to cheating as a way to find an edge on the competition or to keep a single bad test score from sabotaging months of hard work. At Stuyvesant, for example, students ...
The most common method of cheating was "letting others look at their papers while taking exams." The most common reason for cheating was "not being ready for the exam." As for inferential statistics, one-way analysis of variance, an independent t -test, and correlational analyses were used to test the effect and relationship of ...
6. Cheating can cost you a scholarship. Scholarship opportunities can be lost as a result of engaging in dishonest practices during academic evaluations. Cheating not only undermines the integrity of the evaluation process but also has long-term consequences that can impact one's future prospects.
If an exam invigilator catches your child cheating in the examination room, a verbal and written report will be drawn up and an investigation will begin. Depending on the seriousness of the situation and the exam board or school, cheating at the exam can lead to different types of sanctions: Reprimand. This is a disciplinary sanction, a call to ...
One of the consequences involves failure in the specific course which leads to the overall failure. This is because cheating may result in getting a zero mark. Apart from the failure, the student may also face the academic examination and disciplinary body which may make severe judgment for instance suspension or probation in case of first ...
Academic misconduct is a threat to the validity and reliability of online examinations, and media reports suggest that misconduct spiked dramatically in higher education during the emergency shift to online exams caused by the COVID-19 pandemic. This study reviewed survey research to determine how common it is for university students to admit cheating in online exams, and how and why they do ...
First, an evaluation of students' behavior during an examination is an educational practice that is necessary to establish behaviors associated with examination cheating. Students are aware of guidelines that restrict certain behaviors in an examination room. In this regard, they are likely to take caution to avoid cases of examination ...
Several findings emerged as a result of the research synthesis of the selected fifty-eight records on online cheating. The selected studies were categorized into four main topics, namely Cheating reasons, Cheating types, Cheating detection, and Cheating prevention, as shown in Fig. 2.All subsequent classifications reported in this paper have been provided by the authors.
A summary of online cheating research papers and their study themes is presented in Table ... (2019). Exam cheating among Cambodian students : when , how , and why it happens. Compare: ... Asmatulu R. Modern Cheating Techniques, Their Adverse Effects on Engineering Education and preventions.
This can negatively affect their performance on the exam and in the program as a whole. When students believe there is a culture of cheating, it can affect the way they view their program and their participation in it. With enough of a change in this view, students may leave the program entirely. Students who believe their peers regularly cheat ...
For a student, the pressure or tension is felt when the student is unable to cope with the syllabus. This stress is one of the main reasons for students to cheat in exams. Unable to bear the stress and tension, students may resort to unlawful means like cheating during examinations. 3. Fear of Failure.
Some forms of cheating, such as intentional plagiarism, buying papers online or paying someone to complete course work, should be fairly obvious, experts say. This is often referred to as ...
The 2020 exam cheating monthly keyword search trends for Spain were established from the first dataset. The cumulative results are shown in Figure 1, which demonstrates that December, April and March 2020, in this order, were the months with the highest search volume. The lowest volumes occurred in September, August and January 2020.
He thought the "anxiety of cheating would equalise with the happiness of getting a higher grade". Therefore, he wrote an entire essay of Chinese characters on his legs.
Introduction. Cheating on exams is a violation of school's policies. The research focuses on the effect of cheating on the college exams. The research discusses three distinct effects of cheating on the college exams. Cheating on the college exams is demoralising. We will write a custom essay on your topic. 809 writers online.
Cheating harms society by: (1) creating an-environment of broken-trust, which then limits the-ability of students and faculty, to-work-together, meaningfully and collaboratively, (2) leading to more-cheating and a lowering of standards, as cheating becomes 'normal' and the only-way, to-compete, in the-school-culture, (3)
Cheating in exams can be defined as committing acts of dishonesty during an exam in order to score good grades. This is normally done by students when they fail to prepare for the exams or when they feel that the test is too hard for them and they want to score good grades. Various acts are considered as cheating: first when a student gets ...
The findings of a previous study on 200 dental students in Jordan performed by Al-Dwair et al demonstrated that about 70% of students cheated on the exams (21). In general, "not being ready for ...
Cheating in exams is a serious academic offense that has many negative consequences for. students and society. In this essay, I will discuss some of the causes and effects of cheating in. exams, and suggest some possible solutions to prevent or reduce this problem. One of the main causes of cheating in exams is the pressure to perform well and ...
The most common method of cheating was "letting others look at their papers while taking exams." The most common reason for cheating was "not being ready for the exam." As for inferential statistics, one-way analysis of variance, an independent t-test, and correlational analyses were used to test the effect and relationship
A recent study has shown that in 1940, 20% of college students have admitted to cheating in their exams, but now, there is up to 80% drastic increase. Cheating in exams happens on a daily basis, not just in schools, but also in colleges. Students opt to cheat academically, as it is an easier way out of studying or managing their time for school ...
Introduction: Cheating in exam has become a serious issue these days. Exam plays an important role in every student's life. Unfortunately, some circumstances come up that lead student to start cheating in exam. Causes: The actual and foremost reason that drives students for cheating in exam is the desire to secure higher scores. It has found that many students start cheating in exam only ...
Perhaps the most widely touted of GPT-4's at-launch, zero-shot capabilities has been its reported 90th-percentile performance on the Uniform Bar Exam. This paper begins by investigating the methodological challenges in documenting and verifying the 90th-percentile claim, presenting four sets of findings that indicate that OpenAI's estimates of GPT-4's UBE percentile are overinflated ...