- Tools and Resources
- Customer Services
- Affective Science
- Biological Foundations of Psychology
- Clinical Psychology: Disorders and Therapies
- Cognitive Psychology/Neuroscience
- Developmental Psychology
- Educational/School Psychology
- Forensic Psychology
- Health Psychology
- History and Systems of Psychology
- Individual Differences
- Methods and Approaches in Psychology
- Neuropsychology
- Organizational and Institutional Psychology
- Personality
- Psychology and Other Disciplines
- Social Psychology
- Sports Psychology
- Share This Facebook LinkedIn Twitter
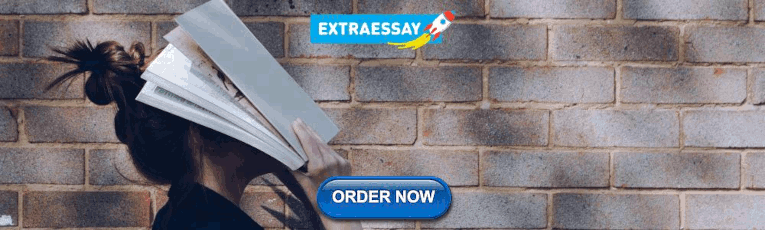
Article contents
Working memory.
- Tom Hartley Tom Hartley University of York
- and Graham J. Hitch Graham J. Hitch University of York
- https://doi.org/10.1093/acrefore/9780190236557.013.768
- Published online: 19 October 2022
Working memory is an aspect of human memory that permits the maintenance and manipulation of temporary information in the service of goal-directed behavior. Its apparently inelastic capacity limits impose constraints on a huge range of activities from language learning to planning, problem-solving, and decision-making. A substantial body of empirical research has revealed reliable benchmark effects that extend to a wide range of different tasks and modalities. These effects support the view that working memory comprises distinct components responsible for attention-like control and for short-term storage. However, the nature of these components, their potential subdivision, and their interrelationships with long-term memory and other aspects of cognition, such as perception and action, remain controversial and are still under investigation. Although working memory has so far resisted theoretical consensus and even a clear-cut definition, research findings demonstrate its critical role in both enabling and limiting human cognition and behavior.
- short-term memory
- serial order
- intelligence
Introduction
The term working memory refers to human memory functions that serve to maintain and manipulate temporary information. There is believed to be a limited capacity to support these functions which combine to play a key role in cognitive processes such as thinking and reasoning, problem-solving, and planning. A common illustration is mental calculation which typically involves maintaining some initial numerical information whilst carrying out a series of arithmetical operations on parts and maintaining any interim results. However, the range of activities that depend on working memory is very much wider than that example might suggest. Thus, perception and action can also depend critically on maintaining and manipulating temporary information, as for instance when identifying a familiar constellation in the night sky, or when preparing a meal.
Information about a stimulus remains available for a few seconds after it is perceived (short-term memory) but without active maintenance it rapidly becomes inaccessible ( Peterson & Peterson, 1959 ; Posner & Konick, 1966 ). Conceptually, working memory extends short-term memory by adding the active, attentional processes required to hold information in mind and to manipulate that information in the service of goal-directed behavior.
The short-term storage required for working memory can be distinguished from long-term memory, which is concerned with more permanent information acquired through learning or experience and includes declarative memory (retention of factual information and events) and procedural memory (underpinning skilled behavior; see Cohen & Squire, 1980 ). Notably, and in contrast to short-term memory, these forms of long-term memory are passive in the sense that, once acquired, memory for facts, events, and well-learned skills can persist over very long periods without moment-to-moment awareness. For example, a vocabulary of many thousands of words, including the relationship between their spoken forms and meanings, can be retained effortlessly over a lifetime. Similarly, once acquired through practice, complex and initially challenging behaviors such as swimming or riding a bicycle can become almost automatic and can be carried out with relatively little conscious control.
In early models of the human memory system (e.g., Atkinson & Shiffrin, 1968 ; see Logie, 1996 ) short-term memory was seen as a staging post or gateway to long-term memory, and it was recognized that it could also support more complex operations, such as reasoning, thus acting as a working memory. Subsequent research has attempted to refine the concept of working memory, characterizing its functional role, limits, and substructure, and distinguishing the processes involved in maintenance and manipulation of information from the storage systems with which they interact.
It has proven difficult, however, to disentangle working memory function from other aspects of cognition with which it overlaps. First, as described in more detail in the section “ Substructure and Relationship to Other Aspects of Cognition ,” many current accounts view the mechanisms of working memory as contributing to other perhaps more fundamental functions such as attention, long-term memory, perception, action, and representation. It is also notable that many informal descriptions of working memory emphasize consciousness and awareness as key features. Intuitively, many working memory functions are accessible to consciousness, and concepts such as mental manipulation, rehearsal, and losing track of information through inattention are subjectively encountered as characteristics of the conscious mind. Of course, by definition, people cannot be subjectively aware of any unconscious contributions to working memory (although they can potentially be inferred from behavior). Some theorists have argued that working memory is central to conscious thought (e.g., Baars, 2005 ; Carruthers, 2017 ), while other empirical researchers have sought to demonstrate nonconscious processes operating in what would typically be considered working memory tasks (e.g., Hassin et al., 2009 ; Soto et al., 2011 ). It is not clear whether, how, or to what extent consciousness is essential for working memory functions, or whether indeed the definition of working memory ought to include, or avoid, aspects of conscious experience. This article steers away from the topic, but the current status of the debate is captured in reviews such as Persuh et al. (2018) . Overall, it is difficult to precisely delineate the boundaries of working memory, whether with other cognitive functions or with consciousness and awareness; in philosophical terms it may not constitute a “natural kind” ( Gomez-Lavin, 2021 ).
These challenges make it difficult to establish a clear-cut and uncontroversial definition of working memory itself, its function, and substructure. Yet it is clear that working memory describes a cluster of related abilities that play a critical role in everyday thinking, placing important constraints on what we can and cannot do. Research on the topic has proved fruitful and although there remain many theoretical controversies about how working memory should be defined and analyzed, these mainly relate to the way in which its operations and substrates can be usefully subdivided, and their interrelationships with other cognitive systems such as those responsible for long-term memory and attention (see Logie et al., 2021 for in-depth discussion).
The following sections begin by identifying relatively uncontroversial characteristics of working memory and its temporal and capacity limits before outlining the main theoretical perspectives on the structure of working memory and its relationship to other forms of cognition. This is followed by a summary of the main experimental tasks and key empirical observations which underpin current understanding. Finally, a brief discussion of the importance of working memory beyond the laboratory is provided.
Temporal Limits
It is broadly agreed that its temporary or labile character is a defining characteristic of working memory. In contrast with established declarative and procedural memories that can be retained indefinitely, recently presented novel information is typically lost after a few seconds unless actively maintained. This active maintenance of short-term memory in order to complete a task is one of the core functions of working memory. As discussed further (see “ Limiting Mechanisms ”), it is less clear how such information is lost over time, or whether forgetting is strictly linked to the passage of time (decay) or merely correlated with it (for example, through an accumulation of interfering information). Nonetheless the vulnerability of short-term memory to degradation over time constrains the uses to which it can be put. Active maintenance processes include rehearsal—covertly subvocalizing verbal material, and attentional refreshing—selectively attending to an item that has not yet become inactive (see e.g., Camos et al., 2009 ). These active processes are themselves limited by the modality and quantity of the stored material, so that for instance subvocal rehearsal is disrupted by speaking aloud at the same time (“articulatory suppression”; Murray, 1967 ), and attentional refreshing can only be directed at a limited number of items in a given period of time ( Camos et al., 2018 ). Even though such active maintenance processes extend the temporal limits of short-term memory, when they do so at the cost of limited attentional resources, this reduces the availability of those resources for other goals.
Capacity Limits
It is also agreed that the limited capacity of working memory is a defining characteristic; in subjective terms, only a limited number of items can be “held in mind” at once. For example, in the classic digit span test of short-term memory capacity, participants are asked to briefly store, and then recall in order, arbitrary sequences of digits of gradually increasing length. In this type of task, accurate performance is typically only possible for very short sequences of up to three or four items beyond which errors of ordering become ever more frequent. Memory span is defined as the sequence length at which recall is correct half the time and is found to be between six and seven for digits, and even less for items such as unrelated words ( Crannell & Parrish, 1957 ). Similar capacity constraints are evident in nonverbal tasks requiring the recall of spatial sequences or the locations or visual properties of objects in spatial arrays. For instance, in the Corsi Block task, participants follow an assessor in tapping out a sequence of blocks in a tabletop array or a sequence of highlighted squares on a computer display. In the standard task, nine blocks are used in a fixed configuration and healthy participants can only recall sequences of around six taps even when tested immediately after presentation ( Corsi, 1972 ; Milner, 1971 ). Such tasks are helpful in identifying the fundamental capacity constraints on short-term memory but working memory capacity is also constrained by the active processes that maintain and manipulate information. This is typically assessed using complex span tasks which measure how many items can be held in mind while carrying out an attention-demanding concurrent task, leading to far lower estimates than simple spans ( Daneman & Carpenter, 1980 ). Similarly, participants show greatly reduced performance on a backward digit span task where mental manipulation is required to reverse the original sequence at recall. (Interestingly the Corsi span is the same in both directions; Kessels et al., 2008 ). Notably, forward and backward digit span and Corsi Block tasks are all used in the clinical assessment of neuropsychological patients as well as in research studies, highlighting the importance of working memory capacity in characterizing healthy and impaired cognitive function.
Just as the temporal limits of short-term memory can be extended by active maintenance processes, its capacity limits can be mitigated through strategic processing. Although it is clear that the number of items that can be stored in working memory is limited, there is some flexibility about what constitutes an item. For example, the sequence “1-0-0” might constitute three digits or might be represented as a single item, “hundred.” The possibility of more efficient forms of coding depends on interactions with long-term memory and can be exploited strategically to extend working memory capacity through “chunking” ( Miller, 1956 ). Thus, for an IT professional, the sequence “CPUBIOSPC” is more easily maintained as the familiar acronyms “CPU,” “BIOS,” and “PC” than as an arbitrary sequence of 10 letters.
While the previous example exploits long-term knowledge, even arbitrary grouping can extend the capacity of working memory, for example, in the immediate serial recall of verbal sequences, performance is improved when items are presented in groups. A spoken sequence of digits like “352-168” (i.e., with a pause between the two groups of digits) is recalled more easily than the ungrouped sequence “352168” ( Ryan, 1969 ). Again, this effect can be deployed strategically, and there is evidence that participants spontaneously group verbal material in memory.
More generally, prior learning and experience can not only expand effective storage capacity but can also contribute to efficient active processing operations. For example, children may initially use a counting-on strategy to perform simple sums such as 2 + 3 = 5, but later typically learn arithmetic number facts that automate such operations, in turn permitting more demanding mental arithmetic to be carried out within working memory ( Raghubar et al., 2010 ). In the extreme, expert calculators may collect extraordinarily large “mental libraries” of number facts ( Pesenti et al., 1999 ). Another powerful strategy for extending working memory capacity is seen in expert abacus operators who in mental calculation are able to use visual imagery to internalize algorithms learned from using the physical device ( Stigler, 1984 ).
Limiting Mechanisms
Despite the clear consensus that limited capacity and duration are defining characteristics of working memory, distinguishing it from other forms of memory and learning, there is less agreement about the mechanisms through which information is limited and forgotten.
In one account, the ultimate capacity limits of the system are determined by its access to a limited number of discrete slots, each of which can be used to hold a chunk of information ( Cowan, 2001 ; Luck & Vogel, 1997 ). However, an alternative and increasingly influential view is that working memory has access to a continuous resource which can be flexibly deployed to support a greater number of chunks or items on the one hand, or greater fidelity and precision on the other ( Bays & Husain, 2008 ; see Ma et al., 2014 for discussion).
The loss of information from working memory over time can similarly be attributed to different mechanisms, although here they do not amount to mutually exclusive models of the same phenomenon. One potential mechanism is decay, assumed to be a fundamental property of the substrate of short-term memory, through which information is lost due to the passage of time alone. In this view the attentional/executive component of working memory is typically deployed to extend its capacity by strategically (but effortfully) refreshing or rehearsing the content of short-term memory before it decays irretrievably. A further potential mechanism is interference. In this account, memory traces are prone to be confused with, or gradually corrupt one another. Several current models incorporate a combination of decay and interference ( Baddeley et al., 2021 ; Barrouillet & Camos, 2021 ; Cowan et al., 2021 ; Vandierendonck, 2021 ), while Oberauer (2021) stands out in rejecting time-based forgetting and maintenance processes, proposing in their place loss due to interference, and requiring a process dedicated to the active removal of outdated information from working memory.
Substructure and Relationship to Other Aspects of Cognition
Because it is linked to such a wide range of cognitive capacities, it can be difficult to clearly distinguish mechanisms of working memory from those of its specialized subcomponents or of general-purpose cognitive mechanisms which contribute to nonmemory functions. There is a broad consensus that working memory involves the interaction of an active process (corresponding to “attention” or “executive control”) with a substrate that can represent the content of memory and thus act as a short-term store. Authors disagree, or are sometimes agnostic, as to the extent to which these components can be usefully subdivided and the degree to which they are uniquely involved in working memory or more generally in cognition. Authors also differ in the emphasis they put on different modalities and tasks. These different emphases may sometimes mask a deeper consensus in which models are complementary rather than incompatible ( Miyake & Shah, 1999 ).
Although the term working memory had already been applied to the use of short-term memory in goal-directed behavior ( Atkinson & Shiffrin, 1968 ), it was the influential work of Baddeley and Hitch ( Baddeley, 1986 ; Baddeley & Hitch, 1974 ), that introduced the separation of attentional control processes (governed by a “central executive”) and short-term storage systems (thought of as “buffers,” i.e., distinct and specialized systems). They further identified a distinction between verbal and visual buffers which were subject to different forms of disruption and appeared to use distinct codes. In particular, verbal information could be stored in a speech-based system (termed the “phonological loop”), in which similar sounding items were more likely to be confused and which was disrupted by concurrent articulation. This work led to the development of the multicomponent model, which subsequently incorporated a richer characterization of the visuo-spatial store (the “visuospatial sketchpad,” see e.g., Baddeley & Logie, 1999 ; Logie, 1995) and, later, an additional store—the “episodic buffer” which holds amodal information and interacts with episodic long-term memory ( Baddeley, 2000 ). The possibility of further substructure within these core components is also recognized (e.g., Logie, 1995 on distinguishing visual and spatial subcomponents; see also Logie et al., 2021 on the possibility of multiple substrates within a multicomponent perspective).
An alternative view, the embedded processes model put forward by Cowan (1999) , is that working memory can be seen as the controlled, temporary activation of long-term memory representations, with access to awareness being limited to three to four items or chunks. A key distinction with the multicomponent model hangs on whether working memory relies on a distinct substrate (as implied by the term “buffer”), or whether the substrate is shared with long-term memory. Oberauer (2002) similarly identifies working memory with activated representations in long-term memory. In this account, the activated region forms a concentric structure within which a subset of individual chunks inside a “region of direct access” compete to be selected as the focus of attention.
Other more recent theoretical accounts have also emphasized the role of attentional control in determining the limits of working memory. For example, Engle (2002) regarded capacity constraints as reflecting the limited ability to control domain-general executive attention in situations where there is the potential for interference among conflicting responses. The time-based resource sharing account ( Barrouillet & Camos, 2004 ) highlights the need to balance the active refreshing of short-term with concurrent processing demands. In this view, constraints arise from the necessary trade-off between maintenance and manipulation, both of which rely on common attentional resources.
Many theoretical approaches to working memory do not follow Baddeley and Hitch in identifying modality-specific substrates for the temporary storage of information and assume instead a unitary system in which many different types of feature can be represented (e.g., Cowan et al., 2021 ; Oberauer, 2021 ). In such accounts, modality-specific phenomena are attributed to differences in the extent to which such features overlap within and between modalities. On the other hand, some authors acknowledge the possibility that there may be many alternative substrates, and that even within a modality further subdivisions may be possible. So, for example substrates supporting memory of verbal/linguistic content might further distinguish auditory-verbal, lexical, and semantic levels of representation ( Barnard, 1985 ; Martin, 1993 ).
Neuroscientific investigations have tended to support the consensus idea of a broad separation between executive and attentional control processes on the one hand, and (often modality-specific) stores on the other, but if anything have highlighted even more extensive overlap of the neural substrate of working memory with other cognitive functions including sensory–perceptual and action–motor representation, and greater granularity and fractionation of function within both storage and control systems. This led Postle (2006) to argue that working memory should be seen as an emergent property of the mind and brain rather than a specialized system in its own right:
Working memory functions arise through the coordinated recruitment, via attention, of brain systems that have evolved to accomplish sensory-, representation-, and action-related functions. ( Postle, 2006 ), p. 23
Even in this view it is clear that the mechanisms of working memory (however they overlap with other cognitive functions) involve the interaction of distinct components (at minimum “attention” is distinguished from sensory/representation and action-related function, and these latter functions may also be further subdivided).
Empirical Investigation and Key Findings
A variety of tasks have been developed to investigate working memory in the laboratory. These tasks, of course, always require participants to briefly retain some novel information, often the identity of a set of items which might be visual (for example, colored shapes) or verbal (digits, words, letters). However, they vary quite considerably in the extent to which they require memory for the structure of the set (such as, for verbal stimuli, their order or the spatial layout of an array of items), the degree to which they place an ongoing or concurrent load on memory and attention, and the precision with which sensory and perceptual properties of the individual items must be represented. An excellent overview of these techniques and associated benchmark findings can be found in Oberauer et al. (2018) .
In an item recognition task, participants determine whether a specific item was in a set (a sequentially presented list or simultaneously displayed array) that they previously studied ( McElree & Dosher, 1989 ). In probed recall , they are provided with a cue that uniquely specifies a given item from a previously presented set, which they are then required to recall ( Fuchs, 1969 ). In free recall tasks, typically employed with verbal stimuli, participants are presented with an ordered list, but are allowed to recall the items in any order ( Postman & Phillips, 1965 ), whereas in serial recall ( Jahnke, 1963 ) they are required to retain the original order of presentation.
The preceding tasks place increasing demands on short-term memory for the structure as well as the content of the presented stimuli, but place relatively little requirement for attention or the manipulation of memory content. To address these aspects of working memory, a range of additional tasks have been developed. In complex span tasks the to-be-remembered items are interleaved with a processing task, placing a greater concurrent load on the attentional system ( Daneman & Carpenter, 1980 ). In the N-back task , items are presented rapidly and continuously, with the participant being required to decide whether each new item repeats one encountered exactly n-items earlier in the sequence; to do this they must not only maintain the order of the previous n-items, but also manage the capacity-limited short-term memory resource as every new item arrives. These demands become increasingly taxing as the value of n increases, again giving an indication of the effects of load on performance or, since it is particularly amenable to neuroimaging, brain activity (see Owen et al., 2005 for review). 1 As mentioned, the manipulation requirements of serial recall can be increased by reversing the order in which items are to be recalled. More involved forms of mental manipulation are explicitly tested in memory updating paradigms ( Morris & Jones, 1990 ), within which, after being presented with an array or description, participants are instructed to carry out a sequence of operations before retrieving the result.
To assess its fidelity over brief intervals, tasks that require memory for detailed properties of the items are useful. In change detection tasks (e.g., Luck & Vogel, 1997 ), participants are required to respond to alterations in the stimulus (typically a visually presented array) between presentation and testing. These alterations can be made arbitrarily small, thus testing the precision of the underlying memory representation. Going beyond recognition -like responses to change, in continuous reproduction or delayed estimation tasks , participants are asked to recall continuous features of the stimuli such as the precise color or orientation of a shape within a previously-studied array (e.g., Bays & Husain, 2008 ). These tasks allow researchers to go beyond the question of whether information is merely retained or lost; they can be used to characterize and quantify the quality of the underlying representation, which in turn can shed light on the potential trade-off between capacity and precision in working memory.
The preceding tasks provide a very useful set of tools for investigating working memory in the laboratory. To investigate the structure and operation of the system, experiments typically manipulate characteristics of the items to be stored, and often employ concurrent tasks devised to selectively disrupt putative components or processes. In their standard forms, the individual items are treated as equally valuable or important, but it is also possible to cue specific items, locations, or serial positions in order to encourage participants to prioritize specific content (e.g., Hitch et al., 2020 ; Myers et al., 2017 ). Improved recall for such prioritized items can then reveal the operation of strategic processes. Overall, such manipulations show a range of replicable effects, not just on overall performance and response times, but also on patterns of error. In turn these benchmark effects have provided the impetus for current theories and provide important constraints for emerging computational models of working memory ( Oberauer et al., 2018 ).
Set Size and Retention Interval Effects
The most important effects relate to capacity and temporal limits that have already been discussed, and these apply across all applicable experimental paradigms and modalities. Specifically, in terms of capacity limits, task accuracy is impaired as the number of items (set size) is increased (response times also generally increase with set size), and in terms of temporal limits, accuracy declines monotonically with the duration of a delay between presentation and testing. The latter effect is reliably seen for both verbal and spatial materials when the retention interval is filled with a distracting task. It does not apply to unfilled delays in tasks with verbal materials, and only sometimes occurs with spatial materials. The difference between filled and unfilled delays forms part of the evidence in favor of the core working memory concept of active executive/attentional processes in sustaining otherwise fleeting short-term memories.
Primacy and Recency Effects
Another signature of working memory is that items are retrieved with greater accuracy if they are presented at the beginning (primacy) or end (recency) of a sequence relative to other items. The operation of primacy and recency effects is seen in immediate serial recall and other tasks where the presentation order is well-defined, and for both verbal and visuo-spatial content. This leads to a serial position curve (in which accuracy is plotted for each serial position in a list) with a characteristic bowed shape. The effect suggests that a shared or general serial ordering mechanism privileges access to these serial positions in an ordered list and/or impairs access to other serial positions. It is important to note that primacy and recency effects are also observed in the immediate free recall of lists of words when the capacity of working memory is greatly exceeded and where they may have a very different explanation (see e.g., Baddeley & Hitch, 1993 ).
Errors and Effects of Similarity
Working memory errors frequently involve confusion between items in the memory set. This is evident in a wide range of tasks (including variants of recognition, change-detection, and continuous reproduction tasks), but is perhaps clearest in immediate serial recall, where the most common forms of error involve the misordering of items. These errors most frequently involve local transpositions in which an item moves to a nearby list position, often exchanging with the item in that position. For example, a sequence like “D, F, E, O, P, Q” might be recalled as “D, F, O, E, P, Q.” Items are most likely to transpose to immediately adjacent list positions, with the probability of a transposition decreasing monotonically as the distance within the sequence increases. Note that there are fewer opportunities for local transpositions at the beginning and end of a sequence so the locality constraint on transpositions likely plays at least some role in primacy and recency effects.
In a verbal working memory task, when items from the memory set are confused with one another, they are most likely to be confused with phonologically similar items making performance for lists of similar sounding items poorer than for phonologically distinct items. In serial recall, this effect manifests itself as an increased tendency for phonologically similar items to transpose with one another, so that in the preceding example, items “D,” “E” and “P” (because they rhyme) would be more likely to transpose with one another than items “F,” “O,” and “Q.” Although these similarity effects are largely reported in verbal paradigms, analogous findings are sometimes observed with visual materials (for example, a sequence of similar colored shapes is harder to reconstruct than a sequence of distinctively colored shapes; Jalbert et al., 2008 ).
The analysis of errors and confusion has been critical in understanding the nature of representation in verbal working memory (for example, demonstrating the importance of speech-based rather than semantic codes), in developing the concept of the phonological loop, and in developing computational models which account for these findings in terms of underpinning serial ordering mechanisms.
Individual Differences and Links With Other Facets of Cognition
Speaking to questions about the relationship between working memory and other aspects of cognition, another set of benchmark findings is concerned with correlations between performance on working memory tasks and other measures. In particular, working memory is correlated with measures of attention and fluid intelligence (the capacity to solve novel problems independent of prior learning; see e.g., Engle, 2002 ) suggesting that all three constructs involve common resources. There is consensus that aspects of attention contribute to working memory, but attention is also relevant to tasks that make minimal demands on memory. At the same time, working memory plays an important role in problem solving in the absence of relevant prior learning, but it can also be applied to tasks that do not involve complex problems. This suggests a hierarchical relationship in which limited cognitive resources (i.e., attention) are applied to maintain and manipulate information in memory (attention + short-term memory = working memory) in the context of demanding problems (working memory + problem solving = fluid intelligence).
This somewhat simplistic sketch of the relationship between constructs omits the contribution of long-term memory and learning to working memory. That contribution is evident in several empirical phenomena. For example, the beneficial effect of chunking on recall often depends on familiarity with the chunks, as in the examples given previously. It is easily overlooked that the familiarity of the materials themselves is also important. For example, familiar words are recalled much better than nonwords ( Hulme et al., 1991 ) suggesting that words act as specialized phonological/semantic “chunks.” Similarly, grammatical sentences are recalled better than arbitrarily ordered lists or jumbled sentences ( Brener, 1940 ). The word–nonword and sentence superiority effects show that well-learned constraints on serial order (whether through syntax or phonotactics) can benefit recall. A related phenomenon, the Hebb repetition effect ( Hebb, 1961 ), can be seen in the laboratory: immediate serial recall for a specific random list gradually improves over successive trials when it becomes more familiar through being repeatedly but covertly presented interleaved among other lists.
The Importance of Working Memory
The laboratory tasks and benchmark findings outlined in the section “ Empirical Investigation and Key Findings ” have established its key characteristics, but the practical significance of working memory extends well beyond these phenomena into everyday cognition and learning. Notably the limits of working memory constrain what we can think about on a moment-to-moment basis and hence how quickly we can learn and what we can ultimately understand. An appreciation of the impact of working memory and its limitations is thus vitally important in the context of education (see e.g., Alloway & Gathercole, 2006 for a review). For example, individual differences in the capacity of phonological storage in verbal working memory are reciprocally linked to vocabulary acquisition in early childhood; children’s ability to repeat nonwords at age four (i.e., unfamiliar phonological sequences) predicts their vocabulary a year later. In turn, the emergence of vocabulary (i.e., phonological chunks) is associated with later improvements in nonword repetition ( Gathercole et al., 1992 ). It is not hard to imagine that this process amplifies the initial effect of variation in capacity, affecting literacy and then more advanced learning (potentially well beyond language abilities) that depends on reading. Working memory can similarly exert an influence on the emergence of numeracy and through it more advanced skills in arithmetic and mathematics. For example, kindergartners’ performance on a backward digit span task predicts their scores on a mathematics test a year later ( Gersten et al., 2005 ). In addition to these effects on the acquisition of foundational skills such as literacy and numeracy, working memory is important in maintaining and manipulating the information needed to carry out complex tasks in the classroom. Thus, students with lower working memory capacity can have difficulty retaining and following instructions ( Gathercole et al., 2008 ) again potentially hampering their ability to build more advanced skills and knowledge. Because of its critical involvement in classroom learning, working memory plays a central role in Cognitive Load Theory” ( Sweller, 2011 ) an influential educational framework which aims to incorporate principles derived from the architecture of human cognition into teaching methods.
Many measures of short-term memory and working memory show marked year-on-year improvement in childhood, with developmental change likely reflecting the maturation of several components that underpin performance ( Gathercole, 1999 ; Gathercole et al., 2004 ). These include changes in processes such as verbal recoding, subvocal rehearsal, the activation of temporary information and executive attentional control ( Camos & Barrouillet, 2011 ; Cowan et al., 2002 ; Hitch & Halliday, 1983 ). As might be expected given the centrality of working memory in the acquisition of language and numeracy, developmental disorders are commonly associated with reduced short-term or working memory capacity. Prominent examples include dyslexia ( Berninger et al., 2008 ), developmental language disorder ( Archibald & Gathercole, 2006 ; Montgomery et al., 2010 ), and dyscalculia ( Fias et al., 2013 ; McLean & Hitch, 1999 ). However, the nature of any causal role for working memory in developmental disorders has been controversial (see e.g., Masoura, 2006 ).
In adulthood, working memory capacity continues to limit the bandwidth that is available for cognitive operations, for example affecting planning and decision-making ( Gilhooly, 2005 ; Hinson et al., 2003 ). As we grow older, working memory capacity tends to decline, and there are some indications that this is associated with failing attention and greater vulnerability to distraction ( Hasher & Zacks, 1988 ; McNab et al., 2015 ; Park & Payer, 2006 ) rather than a mere reversal of earlier developmental gains. Across the entire lifespan, as it waxes and wanes, working memory plays an important part in shaping our daily experience.
Given its central role in constraining human cognitive abilities, extensive efforts have been made to develop interventions that can improve working memory, for example through computerized training programs. However, these efforts have so far met with limited success. Some working memory tasks show improvements with practice, but these effects tend to reflect near or intermediate transfer , specific to the trained task or (often closely-related) direct measures of working memory, rather than far transfer extending to more general improvements in other tasks thought to depend on working memory, such as reading comprehension or arithmetic ( Melby-Lervåg et al., 2016 ; Owen et al., 2010 ; Sala & Gobet, 2017 ). It has been argued that near and intermediate transfer effects arise through improvements in task-specific efficiency via refinement of strategies and long-term memory support (e.g., chunking) whereas more general benefits and far transfer would be expected to depend on the underlying capacity of attentional and storage systems ( von Bastian & Oberauer, 2014 ). The absence of clear evidence for far transfer despite such extensive research thus suggests that working memory capacity limits are a fundamental and unalterable feature of the human cognitive system.
Although it is perhaps premature to rule out the possibility of interventions that achieve increased working memory capacity, it appears at present that it can only be extended in specific contexts through more specialized training with particular tasks and materials. Paradoxically, this resistance to more general training may be what makes working memory so important; to the extent that its capacity limits are unavoidable, working memory helps to determine the scope of human cognition and spurs us to find strategies, technologies and cultural tools that allow us to go beyond them.
In conclusion, through the development of a powerful toolkit of experimental methods and of replicable empirical phenomena, the study of working memory function has provided many useful insights into interactions between attention and short-term memory. On the one hand these interactions can be used strategically to enhance goal-directed behavior and long-term learning while on the other they provide fundamental limits on cognition across the lifespan. Ongoing controversy over the structure of working memory relates to the difficulty in isolating these interactions from other facets of cognition, but there is little doubt about their importance in governing what we can and cannot do.
- Alloway, T. P. , & Gathercole, S. E. (2006). How does working memory work in the classroom? Education Research and Reviews , 1 (4), 134–139.
- Archibald, L. M. D. , & Gathercole, S. E. (2006). Short-term and working memory in specific language impairment . International Journal of Language & Communication Disorders , 41 (6), 675–693.
- Atkinson, R. C. , & Shiffrin, R. M. (1968). Human memory: A proposed system and its control processes In K. W. Spence & J. T. Spence (Eds.), The psychology of learning and motivation: Advances in research and theory (Vol. 2, pp. 89–195). Academic Press.
- Baars, B. J. (2005). Global workspace theory of consciousness: Toward a cognitive neuroscience of human experience . In S. Laureys (Ed.), Progress in brain research (Vol. 150, pp. 45–53). Elsevier.
- Baddeley, A. (1986). Working memory (pp. xi, 289). Clarendon Press.
- Baddeley, A. (2000). The episodic buffer: A new component of working memory ? Trends in Cognitive Sciences , 4 (11), 417–423.
- Baddeley, A. D. , & Hitch, G. (1974). Working memory . In G. H. Bower (Ed.), Psychology of learning and motivation (Vol. 8, pp. 47–89). Academic Press.
- Baddeley, A. D. , & Hitch, G. (1993). The recency effect: Implicit learning with explicit retrieval? Memory & Cognition , 21 (2), 146–155.
- Baddeley, A. , Hitch, G. , & Allen, R. (2021). A multicomponent model of working memory . In R. Logie , V. Camos , & N. Cowan (Eds.), Working memory: The state of the science . Oxford University Press.
- Baddeley, A. , & Logie, R. (1999). Working memory: The multiple-component model . In A. Miyake & P. Shah (Eds.), Models of working memory: Mechanisms of active maintenance and executive control (pp. 28–61). Cambridge University Press.
- Barnard, P. (1985). Interacting cognitive subsystems: A psycholinguistic approach to short-term memory. In A. W. Ellis (Ed.), Progress in the psychology of language (Vol. 2, pp. 197–258).
- Barrouillet, P. , & Camos, V. (2004). Time constraints and resource sharing in adults’ working memory spans. Journal of Experimental Psychology: General , 133 , 83–100.
- Barrouillet, P. , & Camos, V. (2021). The time-based resource-sharing model of working memory . In R. Logie , V. Camos , & N. Cowan (Eds.), Working memory . Oxford University Press.
- Bays, P. M. , & Husain, M. (2008). Dynamic shifts of limited working memory resources in human vision . Science , 321 (5890), 851–854.
- Berninger, V. W. , Raskind, W. , Richards, T. , Abbott, R. , & Stock, P. (2008). A multidisciplinary approach to understanding developmental dyslexia within working-memory architecture: Genotypes, phenotypes, brain, and instruction . Developmental Neuropsychology , 33 (6), 707–744.
- Brener, R. (1940). An experimental investigation of memory span . Journal of Experimental Psychology , 26 (5), 467–482.
- Camos, V. , & Barrouillet, P. (2011). Developmental change in working memory strategies: From passive maintenance to active refreshing . Developmental Psychology , 47 (3), 898–904.
- Camos, V. , Johnson, M. , Loaiza, V. , Portrat, S. , Souza, A. , & Vergauwe, E. (2018). What is attentional refreshing in working memory? Annals of the New York Academy of Sciences , 1424 (1), 19–32.
- Camos, V. , Lagner, P. , & Barrouillet, P. (2009). Two maintenance mechanisms of verbal information in working memory . Journal of Memory and Language , 61 (3), 457–469.
- Carruthers, P. (2017). The centered mind: What the science of working memory shows us about the nature of human thought (Reprint ed.). Oxford University Press.
- Cohen, N. J. , & Squire, L. R. (1980). Preserved learning and retention of pattern-analyzing skill in amnesia: Dissociation of knowing how and knowing that . Science , 210 (4466), 207–210.
- Corsi, P. (1972). Memory and the medial temporal region of the brain . McGill University.
- Cowan, N. (1999). An Embedded-Processes Model of working memory . In A. Miyake & P. Shah (Eds.), Models of working memory: Mechanisms of active maintenance and executive control (pp. 62–101). Cambridge University Press.
- Cowan, N. (2001). The magical number 4 in short-term memory: A reconsideration of mental storage capacity . Behavioral and Brain Sciences , 24 (1), 87–114.
- Cowan, N. , Morey, C. C. , & Naveh-Benjamin, M. (2021). An embedded-processes approach to working memory: How is it distinct from other approaches, and to what ends ? In R. Logie , V. Camos , & N. Cowan (Eds.), Working memory . Oxford University Press.
- Cowan, N. , Saults, J. S. , & Elliott, E. M. (2002). The search for what is fundamental in the development of working memory . In R. V. Kail & H. W. Reese (Eds.), Advances in child development and behavior (Vol. 29, pp. 1–49). JAI.
- Crannell, C. W. , & Parrish, J. M. (1957). A comparison of immediate memory span for digits, letters, and words . The Journal of Psychology: Interdisciplinary and Applied , 44 , 319–327.
- Daneman, M. , & Carpenter, P. A. (1980). Individual differences in working memory and reading . Journal of Verbal Learning and Verbal Behavior , 19 (4), 450–466.
- Engle, R. W. (2002). Working memory capacity as executive attention . Current Directions in Psychological Science , 11 (1), 19–23.
- Fias, W. , Menon, V. , & Szucs, D. (2013). Multiple components of developmental dyscalculia . Trends in Neuroscience and Education , 2 (2), 43–47.
- Fuchs, A. H. (1969). Recall for order and content of serial word lists in short-term memory . Journal of Experimental Psychology , 82 (1, Pt.1), 14–21.
- Gathercole, S. E. (1999). Cognitive approaches to the development of short-term memory . Trends in Cognitive Sciences , 3 (11), 410–419.
- Gathercole, S. E. , Durling, E. , Evans, M. , Jeffcock, S. , & Stone, S. (2008). Working memory abilities and children’s performance in laboratory analogues of classroom activities . Applied Cognitive Psychology , 22 (8), 1019–1037.
- Gathercole, S. E. , Pickering, S. J. , Ambridge, B. , & Wearing, H. (2004). The structure of working memory from 4 to 15 years of age . Developmental Psychology , 40 (2), 177–190.
- Gathercole, S. E. , Willis, C. S. , Emslie, H. , & Baddeley, A. D. (1992). Phonological memory and vocabulary development during the early school years: A longitudinal study . Developmental Psychology , 28 (5), 887–898.
- Gersten, R. , Jordan, N. C. , & Flojo, J. R. (2005). Early identification and interventions for students with mathematics difficulties . Journal of Learning Disabilities , 38 (4), 293–304.
- Gilhooly, K. J. (2005). Working memory and planning. The Cognitive Psychology of Planning , 71–88.
- Gomez-Lavin, J. (2021). Working memory is not a natural kind and cannot explain central cognition . Review of Philosophy and Psychology , 12 (2), 199–225.
- Hasher, L. , & Zacks, R. T. (1988). Working memory, comprehension, and aging: A review and a new view . In G. H. Bower (Ed.), The psychology of learning and motivation: Advances in research and theory (Vol. 22, pp. 193–225). Academic Press.
- Hassin, R. R. , Bargh, J. A. , Engell, A. D. , & McCulloch, K. C. (2009). Implicit working memory . Consciousness and Cognition , 18 (3), 665–678.
- Hebb, D. O. (1961). Distinctive features of learning in the higher animal. In J. F. Delafresnaye (Ed.), Brain mechanisms and learning (pp. 37–46). Blackwell.
- Hinson, J. M. , Jameson, T. L. , & Whitney, P. (2003). Impulsive decision making and working memory. Journal of Experimental Psychology: Learning, Memory, and Cognition , 29 (2), 298.
- Hitch, G. J. , Allen, R. J. , & Baddeley, A. D. (2020). Attention and binding in visual working memory: Two forms of attention and two kinds of buffer storage. Attention, Perception, & Psychophysics , 82 (1), 280–293.
- Hitch, G. J. , & Halliday, M. S. (1983). Working memory in children . Philosophical Transactions of the Royal Society of London. B, Biological Sciences , 302 (1110), 325–340.
- Hulme, C. , Maughan, S. , & Brown, G. D. A. (1991). Memory for familiar and unfamiliar words: Evidence for a long-term memory contribution to short-term memory span . Journal of Memory and Language , 30 (6), 685–701.
- Jahnke, J. C. (1963). Serial position effects in immediate serial recall . Journal of Verbal Learning and Verbal Behavior , 2 (3), 284–287.
- Jalbert, A. , Saint-Aubin, J. , & Tremblay, S. (2008). Short article: Visual similarity in short-term recall for where and when . Quarterly Journal of Experimental Psychology , 61 (3), 353–360.
- Kane, M. J. , Conway, A. R. , Miura, T. K. , & Colflesh, G. J. (2007). Working memory, attention control, and the N-back task: A question of construct validity. Journal of Experimental Psychology: Learning, Memory, and Cognition , 33 (3), 615.
- Kessels, R. P. C. , van den Berg, E. , Ruis, C. , & Brands, A. M. A. (2008). The backward span of the Corsi Block-Tapping Task and its association with the WAIS-III Digit Span . Assessment , 15 (4), 426–434.
- Logie R.H. (1995). Visuo-Spatial Working Memory . Psychology Press.
- Logie, R. H. (1996). The seven ages of working memory. In J. T. E. Richardson , R. W. Engle , L. Hasher , R. H. Logie , E. R. Stoltzfus , & R. T. Zacks (Eds.), Working memory and human cognition (pp. 31–65).
- Logie, R. , Camos, V. , & Cowan, N. (Eds.). (2021). Working memory: The state of the science (1st ed.). Oxford University Press.
- Luck, S. J. , & Vogel, E. K. (1997). The capacity of visual working memory for features and conjunctions . Nature , 390 (6657), 279–281.
- Ma, W. J. , Husain, M. , & Bays, P. M. (2014). Changing concepts of working memory . Nature Neuroscience , 17 (3), 347–356.
- Martin, C. (1993). Short-term memory and sentence processing: Evidence from neuropsychology. Memory & Cognition , 21 (2), 176–183.
- Masoura, E. V. (2006). Establishing the link between working memory function and learning disabilities. Learning Disabilities: A Contemporary Journal , 4 (2), 29–41.
- McElree, B. , & Dosher, B. A. (1989). Serial position and set size in short-term memory: The time course of recognition . Journal of Experimental Psychology: General , 118 (4), 346–373.
- McLean, J. F. , & Hitch, G. J. (1999). Working memory impairments in children with specific arithmetic learning difficulties . Journal of Experimental Child Psychology , 74 (3), 240–260.
- McNab, F. , Zeidman, P. , Rutledge, R. B. , Smittenaar, P. , Brown, H. R. , Adams, R. A. , & Dolan, R. J. (2015). Age-related changes in working memory and the ability to ignore distraction . Proceedings of the National Academy of Sciences , 112 (20), 6515–6518.
- Melby-Lervåg, M. , Redick, T. S. , & Hulme, C. (2016). Working memory training does not improve performance on measures of intelligence or other measures of “far transfer”: Evidence From a meta-analytic review . Perspectives on Psychological Science , 11 (4), 512–534.
- Miller, G. A. (1956). The magical number seven, plus or minus two: Some limits on our capacity for processing information . Psychological Review , 63 (2), 81–97.
- Milner, B. (1971). Interhemispheric differences in the localization of psychological processes in man . British Medical Bulletin , 27 (3), 272–277.
- Miyake, A. , & Shah, P. (Eds.). (1999). Models of working memory: Mechanisms of active maintenance and executive control . Cambridge University Press.
- Montgomery, J. W. , Magimairaj, B. M. , & Finney, M. C. (2010). Working memory and specific language impairment: An update on the relation and perspectives on assessment and treatment . American Journal of Speech-Language Pathology , 19 (1), 78–94.
- Morris, N. , & Jones, D. M. (1990). Memory updating in working memory: The role of the central executive . British Journal of Psychology , 81 (2), 111–121.
- Murray, D. J. (1967). The role of speech responses in short-term memory . Canadian Journal of Psychology/Revue Canadienne de Psychologie , 21 (3), 263.
- Myers, N. E. , Stokes, M. G. , & Nobre, A. C. (2017). Prioritizing information during working memory: Beyond sustained internal attention . Trends in Cognitive Sciences , 21 (6), 449–461.
- Oberauer, K. (2002). Access to information in working memory: Exploring the focus of attention. Journal of Experimental Psychology: Learning, Memory, and Cognition , 28 , 411–421.
- Oberauer, K. (2021). Towards a theory of working memory: From metaphors to mechanisms . In R. Logie , V. Camos , & N. Cowan (Eds.), Working memory . Oxford University Press.
- Oberauer, K. , Lewandowsky, S. , Awh, E. , Brown, G. D. A. , Conway, A. , Cowan, N. , Donkin, C. , Farrell, S. , Hitch, G. J. , Hurlstone, M. J. , Ma, W. J. , Morey, C. C. , Nee, D. E. , Schweppe, J. , Vergauwe, E. , & Ward, G. (2018). Benchmarks for models of short-term and working memory . Psychological Bulletin , 144 (9), 885–958.
- Owen, A. M. , Hampshire, A. , Grahn, J. A. , Stenton, R. , Dajani, S. , Burns, A. S. , Howard, R. J. , & Ballard, C. G. (2010). Putting brain training to the test . Nature , 465 (7299), 775–778.
- Owen, A. M. , McMillan, K. M. , Laird, A. R. , & Bullmore, E. (2005). N-back working memory paradigm: A meta-analysis of normative functional neuroimaging studies . Human Brain Mapping , 25 (1), 46–59.
- Park, D. C. , & Payer, D. (2006). Working memory across the adult lifespan . In Lifespan cognition: Mechanisms of change (pp. 128–142). Oxford University Press.
- Persuh, M. , LaRock, E. , & Berger, J. (2018). Working memory and consciousness: The current state of play . Frontiers in Human Neuroscience , 12 .
- Pesenti, M. , Seron, X. , Samson, D. , & Duroux, B. (1999). Basic and exceptional calculation abilities in a calculating prodigy: A case study . Mathematical Cognition , 5 (2), 97–148.
- Peterson, L. , & Peterson, M. J. (1959). Short-term retention of individual verbal items . Journal of Experimental Psychology , 58 (3), 193–198.
- Posner, M. I. , & Konick, A. F. (1966). On the role of interference in short-term retention . Journal of Experimental Psychology , 72 (2), 221–231.
- Postle, B. R. (2006). Working memory as an emergent property of the mind and brain . Neuroscience , 139 (1), 23–38.
- Postman, L. , & Phillips, L. W. (1965). Short-term temporal changes in free recall . Quarterly Journal of Experimental Psychology , 17 (2), 132–138.
- Raghubar, K. P. , Barnes, M. A. , & Hecht, S. A. (2010). Working memory and mathematics: A review of developmental, individual difference, and cognitive approaches . Learning and Individual Differences , 20 (2), 110–122.
- Ryan, J. (1969). Grouping and short-term memory: Different means and patterns of grouping . Quarterly Journal of Experimental Psychology , 21 (2), 137–147.
- Sala, G. , & Gobet, F. (2017). Working memory training in typically developing children: A meta-analysis of the available evidence . Developmental Psychology , 53 (4), 671–685.
- Soto, D. , Mäntylä, T. , & Silvanto, J. (2011). Working memory without consciousness . Current Biology , 21 (22), R912–R913.
- Stigler, J. W. (1984). “Mental abacus”: The effect of abacus training on Chinese children’s mental calculation . Cognitive Psychology , 16 (2), 145–176.
- Sweller, J. (2011). Cognitive load theory . In J. P. Mestre & B. H. Ross (Eds.), Psychology of learning and motivation (Vol. 55, pp. 37–76). Academic Press.
- Vandierendonck, A. (2021). Multicomponent working memory system with distributed executive control . In R. Logie , V. Camos , & N. Cowan (Eds.), Working memory . Oxford University Press.
- von Bastian, C. C. , & Oberauer, K. (2014). Effects and mechanisms of working memory training: A review . Psychological Research , 78 (6), 803–820.
1. However, note that, in at least one study ( Kane et al., 2007 ) n-back performance correlated only weakly with a measure of span, suggesting that, despite face validity, it may tax distinct cognitive resources.
Related Articles
- Working Memory and Cognitive Aging
- Memory Rehabilitation in Healthy Aging
- Culture and memory
Printed from Oxford Research Encyclopedias, Psychology. Under the terms of the licence agreement, an individual user may print out a single article for personal use (for details see Privacy Policy and Legal Notice).
date: 17 April 2024
- Cookie Policy
- Privacy Policy
- Legal Notice
- Accessibility
- [66.249.64.20|185.39.149.46]
- 185.39.149.46
Character limit 500 /500
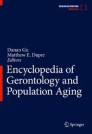
Encyclopedia of Gerontology and Population Aging pp 5457–5463 Cite as
Working Memory
- Nicole R. Nissim 3 , 4 &
- Adam J. Woods 4 , 5
- Reference work entry
- First Online: 01 January 2022
67 Accesses
Cognition ; Cognitive function ; Executive function ; Short-term memory
Working memory is an executive function that enables the temporary online storage, mental manipulation, and/or reorganization of information that is no longer present in the environment.
Working memory is a frontal lobe-mediated cognitive process that underlies everyday decision-making, planning, and problem solving. As a fundamental cognitive process, working memory is thought to serve as the bridge between perception and action. Working memory aids in processes of voluntary or goal directed behaviors, through short-term maintenance of relevant information, manipulation, and mental organization of impending sequences of action (Boisgueheneuc et al. 2006 ; Baddeley 2010 ). Decades of research on working memory in humans have indicated the importance of this complex brain system in complex cognitive tasks (e.g., learning, language comprehension, reasoning) (Baddeley 1992 ). In healthy older...
This is a preview of subscription content, log in via an institution .
Andrews-Hanna JR, Snyder AZ, Vincent JL et al (2007) Disruption of large-scale brain systems in advanced aging. Neuron 56:924–935. https://doi.org/10.1016/j.neuron.2007.10.038
Article Google Scholar
Baddeley A (1992) Working memory. Curr Biol 255:556–559. https://doi.org/10.1016/j.cub.2009.12.014
Baddeley A (2000) The episodic buffer: a new component of working memory? Trends Cogn Sci 4:417–423. https://doi.org/10.1016/S1364-6613(00)01538-2
Baddeley A (2003) Working memory: looking back and looking forward. Nat Rev Neurosci 4:829–839. https://doi.org/10.1038/nrn1201
Baddeley A (2010) Working memory. Curr Biol 20:136–140
Google Scholar
Baddeley AD, Hitch G (1974) Working memory. Psychol Learn Motiv 8:47–89
Barbey AK, Koenigs M, Grafman J (2013) Dorsolateral prefrontal contributions to human working memory. Cortex 49:1195–1205. https://doi.org/10.1016/j.cortex.2012.05.022
Boisgueheneuc FD, Levy R, Volle E et al (2006) Functions of the left superior frontal gyrus in humans: a lesion study. Brain 129:3315–3328. https://doi.org/10.1093/brain/awl244
Bueti D, Walsh V (2009) The parietal cortex and the representation of time, space, number and other magnitudes. Philos Trans R Soc Lond 364:1831–1840. https://doi.org/10.1098/rstb.2009.0028
Cabeza R (2002) Hemispheric asymmetry reduction in older adults: The HAROLD model. Psychology and Aging 17(1):85–100
Cabeza R, Dennis NA (2013) Frontal lobes and aging: Deterioration and compensation. In: D.T. Stuss D & R.T. Knight (Eds). Principles of Frontal Lobe Function, 2nd Edition. Oxford University Press, New York.
Craik FIM, Salthouse TA, Raz N (2000) Aging of the brain and its impact on cognitive performance: integration of structural and functional findings BT. In: Handbook of aging and cognition, vol 2. Lawrence Erlbaum Associates, Mahwah, pp 1–90
Duncan J, Owen AM (2000) Common regions of the human frontal lobe recruited by diverse cognitive demands. Trends Neurosci 23:475–483
Gill J, Shah-Basak PP, Hamilton R (2015) It’s the thought that counts: examining the task-dependent effects of transcranial direct current stimulation on executive function. Brain Stimul 8:253–259. https://doi.org/10.1016/j.brs.2014.10.018
Goldman-Rakic PS (1996) Regional and cellular fractionation of working memory. Proc Natl Acad Sci U S A 93:13473–13480. https://doi.org/10.1073/pnas.93.24.13473
Gomes-Osman J, Indahlastari A, Fried PJ et al (2018) Non-invasive brain stimulation: probing intracortical circuits and improving cognition in the aging brain. Front Aging Neurosci 10:Article 177. https://doi.org/10.3389/fnagi.2018.00177
Hartley A, Speer N (2000) Locating and fractionating working memory using functional neuroimaging: storage, maintenance, and executive functions. Microsc Res Tech 51:45–53
Jones KT, Stephens JA, Alam M et al (2015) Longitudinal neurostimulation in older adults improves working memory. PLoS One 10:e0121904. https://doi.org/10.1371/journal.pone.0121904
Keller JB, Hedden T, Thompson TW et al (2015) Resting-state anticorrelations between medial and lateral prefrontal cortex: association with working memory, aging, and individual differences. Cortex 64:271–280. https://doi.org/10.1016/j.cortex.2014.12.001
Kirchner WK (1958) Age differences in short-term retention of rapidly changing information. J Exp Psychol 55:352–358
Kuo MF, Nitsche MA (2015) Exploring prefrontal cortex functions in healthy humans by transcranial electrical stimulation. Neurosci Bull 31:198–206. https://doi.org/10.1007/s12264-014-1501-9
Linden DEJ (2007) The working memory networks of the human brain. Neuroscientist 13:257–267. https://doi.org/10.1177/1073858406298480
Malenka RC, Bear MF (2004) LTP and LTD: an embarrassment of riches. Neuron 44:5–21
Manoach DS, Schlaug CAG, Siewert B et al (1997) Prefrontal cortex fMRI signal changes are correlated with working memory load. Neuroreport 8:545–549
McLaren ME, Nissim NR, Woods AJ (2018) The effects of medication use in transcranial direct current stimulation: a brief review. Brain Stimul 11:52–58. https://doi.org/10.1016/j.brs.2017.10.006
Mograbi DC, Faria C d A, Fichman HC et al (2014) Relationship between activities of daily living and cognitive ability in a sample of older adults with heterogeneous educational level. Ann Indian Acad Neurol 17:71–76. https://doi.org/10.4103/0972-2327.128558
Nagel IE, Preuschhof C, Li S, Nyberg L (2011) Load modulation of BOLD response and connectivity predicts working memory performance in younger and older adults. J Cogn Neurosci 23:2030–2045. https://doi.org/10.1162/jocn.2010.21560
Nissim NR, O’Shea AM, Bryant V et al (2017) Frontal structural neural correlates of working memory performance in older adults. Front Aging Neurosci 8:1–9. https://doi.org/10.3389/fnagi.2016.00328
Nissim NR, O’Shea A, Indahlastari A et al (2019) Effects of in-scanner bilateral frontal tDCS on functional connectivity of the working memory network in older adults. Front Aging Neurosci 11:1–10. https://doi.org/10.3389/fnagi.2019.00051
Nitsche MA, Paulus W (2000) Excitability changes induced in the human motor cortex by weak transcranial direct current stimulation. J Physiol 527(Pt 3):633–639. PHY_1055 [pii]. https://doi.org/10.1111/j.1469-7793.2000.t01-1-00633.x
Nitsche MA, Nitsche MS, Klein CC et al (2003) Level of action of cathodal DC polarisation induced inhibition of the human motor cortex. Clin Neurophysiol 114:600–604. https://doi.org/10.1016/S1388-2457(02)00412-1
Owen AM, Mcmillan KM, Laird AR (2005) N-back working memory paradigm: a meta-analysis of normative functional neuroimaging studies. Hum Brain Mapp 25:46–59. https://doi.org/10.1002/hbm.20131
Park S-H, Seo J-H, Kim Y-H, Ko M-H (2013) Long-term effects of transcranial direct current stimulation combined with computer-assisted cognitive training in healthy older adults. Neuroreport 25:122–126. https://doi.org/10.1097/WNR.0000000000000080
Richmond LL, Wolk D, Chein J, Olson IR (2014) Transcranial direct current stimulation enhances verbal working memory training performance over time and near transfer outcomes. J Cogn Neurosci 26:2443–2454. https://doi.org/10.1162/jocn_a_00657
Salat DH, Buckner RL, Snyder AZ et al (2004) Thinning of the cerebral cortex in aging. Cereb Cortex 14:721–730. https://doi.org/10.1093/cercor/bhh032
Steffener J, Brickman AM, Rakitin BC et al (2009) The impact of age-related changes on working memory functional activity. Brain Imaging Behav 3:142–153. https://doi.org/10.1007/s11682-008-9056-x
Stephens JA, Berryhill ME (2016) Older adults improve on everyday tasks after working memory training and neurostimulation. Brain Stimul 9:553–559. https://doi.org/10.1016/j.brs.2016.04.001
Toepper M, Gebhardt H, Bauer E et al (2014) The impact of age on load-related dorsolateral prefrontal cortex activation. Front Aging Neurosci 6:1–10. https://doi.org/10.3389/fnagi.2014.00009
Whitfield-Gabrieli S, Nieto-Castanon A (2012) Conn: a functional connectivity toolbox for correlated and anticorrelated brain networks. Brain Connect 2:125–141. https://doi.org/10.1089/brain.2012.0073
Woods AJ, Antal A, Bikson M et al (2016) A technical guide to tDCS, and related non-invasive brain stimulation tools. Clin Neurophysiol 127:1031–1048. https://doi.org/10.1016/j.clinph.2015.11.012
Woods AJ, Cohen R, Marsiske M et al (2018) Augmenting cognitive training in older adults (The ACT study): design and methods of a phase III tDCS and cognitive training trial. Contemp Clin Trials 65:19–32. https://doi.org/10.1016/j.cct.2017.11.017
Download references
Author information
Authors and affiliations.
Department of Neuroscience, McKnight Brain Institute, University of Florida, Gainesville, FL, USA
Nicole R. Nissim
Department of Clinical and Health Psychology, College of Public Health and Health Professions, Center for Cognitive Aging and Memory, McKnight Brain Institute, University of Florida, Gainesville, FL, USA
Nicole R. Nissim & Adam J. Woods
Department of Neuroscience, University of Florida, Gainesville, FL, USA
Adam J. Woods
You can also search for this author in PubMed Google Scholar
Corresponding author
Correspondence to Adam J. Woods .
Editor information
Editors and affiliations.
Population Division, Department of Economics and Social Affairs, United Nations, New York, NY, USA
Department of Population Health Sciences, Department of Sociology, Duke University, Durham, NC, USA
Matthew E. Dupre
Section Editor information
Rights and permissions.
Reprints and permissions
Copyright information
© 2021 Springer Nature Switzerland AG
About this entry
Cite this entry.
Nissim, N.R., Woods, A.J. (2021). Working Memory. In: Gu, D., Dupre, M.E. (eds) Encyclopedia of Gerontology and Population Aging. Springer, Cham. https://doi.org/10.1007/978-3-030-22009-9_721
Download citation
DOI : https://doi.org/10.1007/978-3-030-22009-9_721
Published : 24 May 2022
Publisher Name : Springer, Cham
Print ISBN : 978-3-030-22008-2
Online ISBN : 978-3-030-22009-9
eBook Packages : Social Sciences Reference Module Humanities and Social Sciences Reference Module Business, Economics and Social Sciences
Share this entry
Anyone you share the following link with will be able to read this content:
Sorry, a shareable link is not currently available for this article.
Provided by the Springer Nature SharedIt content-sharing initiative
- Publish with us
Policies and ethics
- Find a journal
- Track your research
How does chunking help working memory?
Affiliation.
- 1 Department of Psychology, Cognitive Psychology Unit, University of Zurich.
- PMID: 29698045
- DOI: 10.1037/xlm0000578
Chunking is the recoding of smaller units of information into larger, familiar units. Chunking is often assumed to help bypassing the limited capacity of working memory (WM). We investigate how chunks are used in WM tasks, addressing three questions: (a) Does chunking reduce the load on WM? Across four experiments chunking benefits were found not only for recall of the chunked but also of other not-chunked information concurrently held in WM, supporting the assumption that chunking reduces load. (b) Is the chunking benefit independent of chunk size? The chunking benefit was independent of chunk size only if the chunks were composed of unique elements, so that each chunk could be replaced by its first element (Experiment 1), but not when several chunks consisted of overlapping sets of elements, disabling this replacement strategy (Experiments 2 and 3). The chunk-size effect is not due to differences in rehearsal duration as it persisted when participants were required to perform articulatory suppression (Experiment 3). Hence, WM capacity is not limited to a fixed number of chunks regardless of their size. (c) Does the chunking benefit depend on the serial position of the chunk? Chunks in early list positions improved recall of other, not-chunked material, but chunks at the end of the list did not. We conclude that a chunk reduces the load on WM via retrieval of a compact chunk representation from long-term memory that replaces the representations of individual elements of the chunk. This frees up capacity for subsequently encoded material. (PsycINFO Database Record (c) 2018 APA, all rights reserved).
- Memory, Long-Term / physiology*
- Memory, Short-Term / physiology*
- Mental Recall / physiology*
- Young Adult
Grants and funding
- Swiss National Science Foundation
- Reference Manager
- Simple TEXT file
People also looked at
Review article, a multisensory perspective of working memory.
- Department of Experimental Psychology, Ghent University, Ghent, Belgium
Although our sensory experience is mostly multisensory in nature, research on working memory representations has focused mainly on examining the senses in isolation. Results from the multisensory processing literature make it clear that the senses interact on a more intimate manner than previously assumed. These interactions raise questions regarding the manner in which multisensory information is maintained in working memory. We discuss the current status of research on multisensory processing and the implications of these findings on our theoretical understanding of working memory. To do so, we focus on reviewing working memory research conducted from a multisensory perspective, and discuss the relation between working memory, attention, and multisensory processing in the context of the predictive coding framework. We argue that a multisensory approach to the study of working memory is indispensable to achieve a realistic understanding of how working memory processes maintain and manipulate information.
A Multisensory Perspective of Working Memory
In everyday life we experience a continuous stream of information that we perceive through sight, sound, smell, taste, and touch. Even though this experience is mostly multisensory, that is, we receive information from multiple senses simultaneously, psychological research has primarily focused on studying our senses in isolation. While we are beginning to understand how our senses interact at various stages of processing (for an overview see, e.g., Wallace et al., 1993 ; Beauchamp, 2005 ; Ghazanfar and Schroeder, 2006 ; Stein and Stanford, 2008 ; Klemen and Chambers, 2012 ) it is still heavily debated whether the higher-order mental representations that are derived from these sensory inputs still contain modality- specific information or not. For instance, in working memory, research has focused on resolving whether information is memorized in the form of separate, modality or domain specific representations ( Baddeley and Hitch, 1974 ; Schneider and Detweiler, 1988 ), or as integrated representations ( Atkinson and Shiffrin, 1968 ; Cowan, 2001 ).
Multisensory processing refers to the interaction of signals arriving nearly simultaneously from different sensory modalities. This implies that information from one modality can influence information processing in another modality. Information from different sensory modalities can also be combined into a single multisensory event, a process that is referred to as multisensory integration ( Stein et al., 2010 ). In accordance with the suggestions of Stein et al. (2010) we will use the terms “modality-specific” or “cross-modal” when describing the properties of objects and “unisensory” or “multisensory” when referring to neural or behavioral processes associated with a single or multiple sensory modalities.
The aim of this paper is to discuss the current status of research on multisensory processing and the implications of these findings for our theoretical understanding of working memory. To do so, we will focus on reviewing working memory research conducted from a multisensory perspective. We will argue that a multisensory approach to the study of working memory is indispensable to achieve a realistic understanding of how working memory processes maintain and manipulate information.
Working Memory and the Multisensory Brain
In their seminal work, Atkinson and Shiffrin (1968) devised a model for the flow of information in human memory, which subsequently became known as the modal model. They suggested that environmental information is processed by various modality-specific sensory registers before it is combined into a single, modality-independent, or more formally amodal , percept and transferred into a short-term store. According to this view, the short-term store is an amodal, general-purpose mechanism. Atkinson and Shiffrin referred to this mechanism as “working memory”, as it was considered to be responsible for a variety of operations, such as the selection, manipulation, and rehearsal of the memorized items.
A few years later, Baddeley and Hitch (1974) proposed a multiple-component model of working memory where information is assumed to be stored in two domain-specific subsystems (the phonological loop and the visuo-spatial sketchpad) that are directed by a general control mechanism (the central executive). The phonological loop is responsible for short-term maintenance of speech-based and acoustic items. The visuo-spatial sketchpad maintains visually and/or spatially encoded items. In contrast to Atkinson and Shiffrin’s (1968) idea of a domain-independent (i.e., amodal) store, Baddeley and Hitch (1974) assume that information (e.g., verbal or spatial) is maintained in its corresponding domain-specific store.
Over the years it has become clear that information from different domains showed more interaction in working memory than one would expect from a strongly domain-specific perspective (e.g., Jiang et al., 2000 ; Logie et al., 2000 ; Prabhakaran et al., 2000 ). An episodic buffer was added to Baddeley and Hitch (1974) original working memory model to account for, amongst other things, the apparent interaction between phonological and visual processes ( Baddeley, 2000 ). The episodic buffer can be conceived as an amodal storage component, which was estimated to hold up to four chunks of information. Additionally, it was proposed to act as a link between all the other working memory components described above. For this revised model, Baddeley (2000) suggested that the episodic buffer integrates memory traces that may originate from different senses into a coherent perceptual scene.
On the basis of several studies, Postle (2006) has proposed that the brain areas involved in sensory perception are also responsible for the short-term storage of sensory information. For instance, functional magnetic resonance imaging (fMRI) studies showed object-specific memorization effects for faces in the posterior fusiform gyrus (e.g., Druzgal and D’Esposito, 2003 ; Ranganath et al., 2004 ), an area considered to be vital for face recognition. Postle and D’Esposito (1999) found activity related to memorization of visual object location and depiction in ventral temporal and occipital visual brain areas. Similarly, event-related potential (ERP) modulations can be seen in posterior and occipital recording sites during short-term memorization of visual objects contralateral to the to-be-remembered objects (e.g., Klaver et al., 1999 ; Vogel and Machizawa, 2004 ). Such findings (for an overview see, Postle, 2006 ; D’Esposito and Postle, 2015 ) indicate that memorizing modality-specific sensory information involves the same brain areas as those involved in the initial sensory processing of that information. This idea is compatible with the classical view that integration of the senses would take place at a later stage of processing, after initial unisensory processing has taken place (see Talsma, 2015 , for a discussion). Indeed, using neurophysiological methods with animals (e.g., Wallace et al., 1993 ; Fuster et al., 2000 ) and fMRI with humans (e.g., Calvert et al., 2000 ; Wright et al., 2003 ; Beauchamp et al., 2004 ) several higher-order brain areas have been identified that seem to be dedicated to integrating information from multiple unisensory sources. Brain areas typically regarded as multisensory in the human brain can for example be found in the lateral occipital-temporal cortex, such as the superior temporal sulcus (STS; Beauchamp, 2005 ).
An increasing number of studies now suggest, however, that multisensory processing can already take place in brain areas that were considered to be strictly unisensory (see for a review, Foxe and Schroeder, 2005 ; Macaluso and Driver, 2005 ). For example, Giard and Peronnet (1999) found multisensory ERP effects as early as 40 ms post-stimulus over occipital scalp areas, suggesting that multisensory interactions take place much earlier than previously assumed. Using fMRI, Foxe et al. (2002) showed integration related effects of auditory and somatosensory stimuli within a region of the auditory cortex previously thought to be unisensory. This brain area was more strongly activated by multisensory stimuli than what might be expected on the basis of a mere summation of either auditory or tactile stimulation alone. Likewise, Dionne et al. (2010) found increased BOLD signal in the right primary somatosensory cortex during a delayed sensory-to-motor task for cross-modal visual-somatosensory stimuli compared to modality-specific stimuli.
These findings also have implications for the memorization of multisensory information. If indeed, as Postle (2006) proposes, the brain areas responsible for perceptual processing are the same as those involved in memorization, and if multisensory effects can already be observed in the primary sensory cortices, then we would expect that cross-modal information is stored as a unified representation in working memory. We specifically aim to focus on the questions regarding how multisensory information is encoded in working memory and whether we memorize the individual unisensory representations separately and integrate them at a later stage, or whether they are memorized as part of an integrated, multimodal representation instead.
Feature Binding in Working Memory
To fully understand the importance of considering working memory from a multisensory perspective, it is necessary to discuss how information is organized within working memory. An important question here is whether each feature of an object is remembered separately or not (e.g., Luck and Vogel, 1997 ; Klaver et al., 1999 ; Vogel et al., 2001 , 2005 ; Wheeler and Treisman, 2002 ; Olsson and Poom, 2005 ; Luria et al., 2010 ; Diamantopoulou et al., 2011 ; Luria and Vogel, 2011 ). For example, Luck and Vogel (1997) used a change detection task to examine the capacity of working memory for visual objects. Participants were presented with an array of stimuli, which they had to remember during an interval without the stimuli being present. After this retention interval a second array was presented and participants responded by indicating whether any visual changes had occurred between the second and the first array. Varying the number of visual objects that need to be memorized allows estimating the capacity of visual working memory. Luck and Vogel (1997) found that capacity was limited to approximately four objects, regardless of the number of feature dimensions, or individual features that needed to be remembered per object. This led them to conclude that visual working memory has an object-based and not a feature-based organization. It is important to note that these findings have not been replicated ( Oberauer and Eichenberger, 2013 ; Hardman and Cowan, 2015 ). At the very least this suggests that feature binding can, but does not always, occur automatically.
Interestingly, research has shown that an asymmetry exists in binding the visual and spatial features of an object. Multiple studies have shown that processing the visual features of an object automatically bind this object to its spatial location (e.g., Jiang et al., 2000 ; Olson and Marshuetz, 2005 ). However, processing an object’s spatial location does not result in the automatic binding of that object’s visual features ( Jiang et al., 2000 ). While these findings show that binding of multiple features can occur within the visuo-spatial domain, other studies have shown that binding of features can even occur across domains.
Prabhakaran et al. (2000) showed that participants memorized verbal and spatial information in an integrated fashion. Participants in this study performed faster and more accurate on a verbal-spatial delayed-match-to-sample task when the probe was a letter-location combination that was presented together in the sample array compared to a letter-location combination that was presented separately. The findings on binding of verbal and spatial information have been replicated and extended in multiple studies ( Bao et al., 2007 ; Campo et al., 2008 , 2010 ; Elsley and Parmentier, 2009 ; Guérard et al., 2013 ; Meier et al., 2014 ). For example, Bao et al. (2007) found that switching attention between verbal and spatial features was faster when they were features from one object than when they were features from separate objects. Additionally, Guérard et al. (2013) showed that phonological similarity of verbal material can carry over to the recall of spatial locations in a combined verbal-spatial serial recall task. Participants were sequentially presented with letters in specific locations and were asked to either recall the order of spatial locations shown or the order of letters shown. They found that the harmful effect of phonological similarity on verbal recall carried over to spatial recall, but that the harmful effect of spatial complexity on spatial recall did not carry over to verbal recall. While the question remains under which exact circumstances automatic binding or integration of cross-domain information occurs, the asymmetry found in visual feature and location binding as well as verbal and spatial binding, suggest that the automatic integration of information across domains can occur.
Multisensory Working Memory Representations
Despite the evidence for integration of information from different domains, surprisingly little research has examined how multisensory information is represented in working memory. One of the first studies to use cross-modal stimuli was done by Thompson and Paivio (1994) . Participants memorized three different types of items: visual, auditory, or audiovisual for a later free-recall test. Thompson and Paivio found an improvement of free recall of cross-modal audiovisual stimuli compared to modality-specific, audio or visual stimuli. This superior audiovisual performance was not simply due to the double presentation of information in audiovisual conditions (audio and visual dual presentation), because picture-picture and sound-sound dual presentation conditions did not yield a similar improvement. When pictures in the picture-picture dual presentation condition were two different exemplars of the same item a slight improvement in free recall was found but audiovisual performance still resulted in higher recall rates. Goolkasian and Foos (2005) also found that recall rates were higher for picture/spoken word and written/spoken word dual presentation conditions compared to the double visual presentation of pictures and written words. These findings suggest that the improved memory performance is due to the combination of information from different modalities and not because of the redundancy of the information itself.
In the multisensory literature, additive effects, such as for example linear increases of brain activity for multisensory stimuli (For an overview see; Calvert, 2001 ), are considered to be exemplary of multisensory processing. By contrast, in working memory research, similar additive effects, such as an increase in capacity for audiovisual material compared to modality-specific material, are considered evidence for the independence of the two modalities. For example, the advantage of cross-modal object recall, in the study of Thompson and Paivio (1994) was explained by Paivio (1971 , 1986) “dual coding” theory. This theory states that a memory trace for a cross-modal stimulus is a combination of the independent sensory traces that were encoded, which in turn can be recalled separately when the task so requires. While information from different modalities can interact to provide certain behavioral benefits, this information is in fact independent.
Originally, the dual coding theory was developed to explain the independent, simultaneous processing of verbal and non-verbal information, but has later also been used to explain the independent, simultaneous processing of auditory and visual information. It is important to note that these forms of information can interact. Verbal information can be both visual (e.g., written words) and/or auditory (e.g., spoken words), and nonverbal information can also be visual (e.g., complex visual scenes) and/or auditory (e.g., white noise). We can make a distinction between the format of a working memory representation, i.e., the sensory modality in which the information is perceived and/or processed (e.g., auditory—visual), and the content of the representation, i.e., the actual information that is transferred (verbal–non-verbal). For example, when memorizing an array of blue squares or a picture of a cat, it might be more efficient to memorize this verbally as the verbal code “blue squares” or “red cat”. However, when the task requires one to describe the exact spatial location of each square, or point out a specific cat in an array of red cat pictures, it would be more efficient to use a visual code. We assume that information is processed in the format code that is most optimal for the current task. This implies that multiple format codes might be used for one and the same object, if that is more effective for memorizing that object.
Delogu et al. (2009) investigated how verbal and non-verbal auditory, visual, and audiovisual material is encoded in working memory. Participants were tested on immediate serial recall for sequentially presented visual, auditory, or audiovisual stimuli in either a non-verbal or verbal condition. In the non-verbal condition, stimuli were either pictures, environmental sounds, or a combination of both, and in the verbal condition, stimuli were either written words, spoken words, or a combination of both. Results showed that in the non-verbal condition serial recall for audiovisual stimuli was higher than recall for auditory or visual stimuli. In the verbal condition, recall for audiovisual material was still higher than recall for visual material, but auditory and audiovisual recall did not differ. The authors also found that preventing participants from articulating reduced memory performance in both the verbal and non-verbal conditions. This suggests that both in the verbal and in the non-verbal presentation conditions, the actual content of the representation was encoded in a verbal code. Furthermore, the verbal content seemed to play a key part in memorizing the stimuli in all conditions. This shows that the format in which information is presented is not necessarily the format in which the information is encoded. For example, when a participant is presented with an auditory stimulus of a meowing cat, it is possible that this sound calls forth a picture of a cat, or the word “cat”, which is then kept in working memory instead of the auditory features of the original meowing sound that was presented. It is a requirement that the participant recognizes the presented sound as the meowing produced by a cat in order to “recode” the sound into a visual or verbal representation. This requires semantic information from long term memory to be integrated with the working memory representation. Delogu et al. (2009) concluded that their findings are compatible with Baddeley’s (2000) working memory model where the existence of an episodic buffer integrates information from different modalities and combines this with semantic information from long term memory. Other studies have also shown the influence of semantic information from long term memory on visual working memory object representations (e.g., Olsson and Poom, 2005 ; Diamantopoulou et al., 2011 ) suggesting that information outside the pure visual domain can affect early visual object working memory. Similarly, Darling et al. (2012) found that accuracy on a digit serial recall task improved when the locations of presented digits matched the spatial configuration of a typical, numeral keypad (as found on a telephone or television remote) in a process they call visuospatial bootstrapping. They confirmed that this effect was due to the integration of the typical keypad representation from long-term memory with the working memory representation and not only to the binding between verbal and spatial information.
Thus far, the main goal of the studies discussed above was to provide insights into the dual code theory ( Paivio, 1971 , 1986 ) and/or the multiple component theory ( Baddeley and Hitch, 1974 ; Baddeley, 2000 ) mainly by looking at recall performance for a wide variety of stimuli. To better understand how multisensory information interacts in working memory we can look at working memory capacity for cross-modal objects. As mentioned before, estimates of working memory capacity for features and objects have been used to infer that visual working memory representations are object based ( Luck and Vogel, 1997 ). Likewise, by assuming that not only features within a modality but also across modalities are integrated into object representations, examining the number of cross-modal objects one can hold in memory compared to modality-specific objects could give insight into the organization of multisensory working memory. For instance, Saults and Cowan (2007) found that working-memory capacity for audiovisual material can exceed working-memory capacity for modality-specific material under certain conditions. In a series of five experiments, participants were presented with visual arrays of four to eight colored squares and auditory arrays of four spoken digits. They were instructed to memorize the visual array, the auditory array, or both. Interestingly, the performance advantage for audiovisual arrays disappeared when masks were used to block access to previously formed sensory memory traces. In this case, capacity for cross-modal stimuli was as high as the capacity of the highest modality-specific object, indicating that memory traces from an accessory sensory memory (echoic and/or iconic memory) contributed to the improvement of task performance. Since auditory and visual information did not additively contribute to memory performance when sensory memory traces were excluded, Saults and Cowan (2007) concluded that auditory and visual information share a common storage. Fougnie and Marois (2011) contested this interpretation by arguing that the formula used by Saults and Cowan (2007) to estimate the maximum number of object representations one can hold in working memory, might not adequately reflect the combined capacity of modality-specific stores. Fougnie and Marois argued that one item of auditory information generally places a larger load on memory than one item of visual information, suggesting that these modality-specific differences should be weighted accordingly in such a capacity estimate. Using an adapted formula in a series of three experiments, they found that even when using masks to exclude contributions of sensory memory traces, capacity for cross-modal items was superior to the capacity for modality-specific items. Contrary to Saults and Cowan (2007) , they concluded that auditory and visual objects were stored in their own respective stores and contributed to performance without interfering.
Overall, there seems to be a performance benefit for the memorization of audiovisual stimuli compared to the memorization of modality-specific stimuli. It remains under debate, however, whether this benefit exists because these stimuli are integrated into a new amodal representation or because the independent storage of auditory and visual information contributes to performance in an additive fashion because they do not interfere. At this time the same effect is used to argue for both sides of the debate. Where some see the additive performance of audiovisual objects as proof for an interaction or even integration of information in working memory (e.g., Delogu et al., 2009 ), others see it as proof that sensory information is memorized in its own separate store (e.g., Fougnie and Marois, 2011 ).
In addition to examining performance benefits for the combination of auditory and visual processing, we can also study the disruption of processing for the combination of auditory and visual information. In traditional working memory research, interference paradigms have been used to show a double dissociation between two separate processing mechanisms. Meaning that when two processes use the same underlying system, interference will occur which impairs performance on both processes. The disruption of performance between modalities is referred to as cross-modal interference and would suggest that information from the different modalities interact at a certain level. For multisensory working memory this could mean that information from different modalities is maintained in a single, multisensory store. Evidence for cross-modal interference is still somewhat ambiguous, however. For instance, using a visual-pattern-recall and auditory-digit-recall dual task, Cocchini et al. (2002) did not find evidence for cross-modal interference on performance accuracy in working memory. The absence of such interference suggests that working memory operates in a domain-specific manner and is in accordance with the notion of parallel processing without interaction of information from different modalities. In contrast, Goolkasian and Foos (2005) showed that spoken words could interfere with the recall of pictures and written words when using long sequences of incongruent dually presented items. Likewise, Morey and Cowan (2004 , 2005) , did find cross-modal interference on performance accuracy when memory load was sufficiently high. They examined digit span using a verbal-visual dual task and found that participants showed interference for visual memory recall but only when the verbal load was sufficiently high (a load of 7 digits instead of 2). The interference patterns observed in audio-visual dual tasks are as of yet inconclusive on whether visual and auditory information share a limited capacity storage. Although interference paradigms could give us an answer on the question of whether information from different modalities share a limited capacity storage or not, they cannot answer whether the information from different modalities is integrated in this single storage, or maintained as independent modality-specific traces.
Thus far, research on multisensory working memory has shown that recall is better for cross-modal objects compared to modality-specific objects ( Thompson and Paivio, 1994 ; Goolkasian and Foos, 2005 ; Delogu et al., 2009 ), working memory capacity is higher for cross-modal objects under certain circumstances ( Saults and Cowan, 2007 ; Fougnie and Marois, 2011 ), and visual and auditory information can interfere with each other ( Morey and Cowan, 2004 , 2005 ; Goolkasian and Foos, 2005 ) but not always ( Cocchini et al., 2002 ). Although a performance benefit for cross-modal objects is seen as evidence for integration in multisensory research, in working memory research it has traditionally been seen as evidence that modality-specific information from cross-modal objects is stored in separate stores. While we cannot definitively conclude that cross-modal objects are stored as fully integrated objects in working memory, it is apparent that cross-modal information interacts in working memory beyond what would be expected from modality-specific stores. The question is: at what stage or stages in the processing stream do these interactions occur?
Multisensory Processing, Selective Attention, and Working Memory
To answer this question we turn to research on multisensory processing and selective attention. The insights gained from this research could also inform questions about working memory for multisensory stimuli. In fact, more and more researchers have challenged the idea that working memory and attention are two separate systems ( Cowan, 2001 ; Awh et al., 2006 ; Olivers, 2008 ; Oberauer and Hein, 2012 ; Kiyonaga and Egner, 2013 ; Klaver and Talsma, 2013 ). For example, Olivers (2008) reviews evidence for the notion that working memory and attention share the same capacity, content and control processes, suggesting they might be two aspects of the same process. Likewise, Kiyonaga and Egner (2013) discuss the literature that examined the effects of external attention on working memory representations, as well as, the effects of working memory representations on directing selective attention. These studies indicate that a competitive interaction between working memory and selective attention exists, implying that they share a limited resource. Kiyonaga and Egner (2013) state that attention and working memory should no longer be regarded as two separate concepts, but instead as one concept, where attention can be directed externally (selective attention) and/or internally (working memory). The idea of working memory as internal attention is in line with Cowan’s (2001) original idea of working memory where a capacity limited focus of attention can shift between different levels of processing.
Given the above mentioned observations that working memory and attention are presumably two different aspects of the same underlying process, and considering that several studies have shown close ties between attention and multisensory processing, it is necessary to understand the implications of these ties for working memory. Instances where multisensory events guide and focus attention (also referred to as bottom-up effects) suggest an early integration of multisensory information, while instances where attention is needed for multisensory integration (also referred to as top-down effects) are indicative of late integration processes. There is evidence for both types of interaction between multisensory integration and attention. Factors that determine the predominance of either early and/or late interactions between information from different modalities are for example, task-relevancy (e.g., Busse et al., 2005 ), learned associations (e.g., Molholm et al., 2007 ), and saliency (e.g., Van der Burg et al., 2008 ).
An example of top-down influence of attention on multisensory integration was given by Talsma and colleagues (e.g., Senkowski et al., 2005 ; Talsma and Woldorff, 2005 ; Talsma et al., 2007 ). Using a rapid succession of task-relevant and irrelevant stimuli, they found that attention could influence the integration of cross-modal stimuli. Similarly, Alsius and colleagues ( Alsius et al., 2005 , 2007 ) have shown that attending elsewhere diminishes participants susceptibility to the McGurk illusion ( McGurk and MacDonald, 1976 ). Based on these findings it appears that attending to the relevant, to-be-integrated stimuli is necessary to build a robust, integrated representation ( Talsma et al., 2010 ).
However, evidence for bottom-up modulation of attention by multisensory integration has made it clear that multisensory processing can already happen in very early stages of perception ( Giard and Peronnet, 1999 ; Molholm et al., 2002 ; Van der Burg et al., 2011 ). For instance, Van der Burg et al. (2011) presented dynamic displays consisting of line elements that randomly changed orientation. When a target orientation change was synchronized with a short, spatially uninformative tone, visual search was strongly facilitated as compared to when the tone was absent. The interpretation given to these results was that the tone and the synchronized orientation change were bound together into one coherent event, thereby forming a cross-modal singleton that “popped out” between the non-synchronized visual distractors. EEG data showed that this multisensory benefit was apparent as early as 50 ms post-stimulus onset and that the strength of this effect predicted the magnitude of the behavioral benefit during visual search, due to the auditory signal.
The findings above imply that both top-down (task-relevance and learned associations) as well as bottom-up (salience) processes are involved in multisensory integration. To resolve this apparent contradiction between a bottom-up view of multisensory processing, where early multisensory effects seem to drive attention, and a top-down view of multi-sensory processing, where attention seems to be required to integrate cross-modal objects, Talsma et al. (2010) proposed a unified framework of attention and multisensory processing. According to this framework, early pre-attentive processes can bind multisensory inputs together, but only when competition among the individual inputs is low. Thus, the early latency processes serve to cross-feed low-level information between the individual sensory cortices involved in the integration processes. Early interactions might serve to realign auditory and visual input signals. Auditory information might give temporal information to visual cortex whereas visual information might provide spatial information to auditory processing.
This pre-attentive early integration would, according to Talsma et al. (2010) , only be possible, however, if the stimuli presented in one modality do not need to compete for processing capacity with other stimuli in that same modality. If there is competition among multiple stimuli in one modality, top-down attentional control may be required to filter out any stimulus that is not task relevant, thereby prioritizing those stimuli that are task relevant. Consistent with this view, Van der Burg et al. (2012) found that the earlier mentioned automatic capture by a synchronized cross-modal event can be modulated by the size of the attentional window, meaning that when participants were less focused the effect of the cross-modal pop out was stronger than when participants were forced to focus on a small cue before the synchronized cross-modal event. In conclusion, stimulus-driven, bottom-up processes can automatically capture attention towards multisensory events. Top-down attention can in turn facilitate the integration of multisensory information which leads to a spread of attention across sensory modalities.
Based on the previously mentioned idea that external attention and internal attention (working memory) are two aspects of the same process, findings in attentional research could be applied to working memory. It has been shown that spatial attention can actively influence working memory representations by facilitating encoding ( Uncapher et al., 2011 ) and improving the recall of memorized representations ( Murray et al., 2013 ). These effects are found not only within a single modality, but also across modalities. For instance, an auditory cue can draw attention to a visual object and vice versa ( Spence and Driver, 1997 ; Koelewijn et al., 2009 ). Similar effects for working memory have been found by Botta et al. (2011) . They examined the effect of visual, auditory, and audiovisual cues on working memory for arrays of colored squares in a change detection task. The cross-modal and modality-specific cues could either capture attention towards the hemifield which contained the to-be-remembered objects, or towards the opposite hemifield which contained the to-be-ignored objects. They found that audiovisual cues had a larger influence on performance accuracy than modality-specific visual or auditory cues. Memory accuracy was increased when an audiovisual cue was presented on the same side as the target and it was decreased when the audiovisual cue was presented on the opposite side. Both the facilitation and impairment of memory performance was larger for audiovisual cues compared to visual cues. Although these data do not directly address the question of how a cross-modal object is represented in working memory as such, they do tell us that multisensory information has a bottom-up effect on the subsequent memorization of a unisensory object.
Investigation of top-down effects of working memory on attention has revealed that working memory content can affect the allocation of visual selective attention ( Olivers et al., 2006 ). In a multisensory context, Murray et al. (2004) found that discrimination accuracy of visual objects, presented 20 s after initial presentation, improved when the initial presentation was a picture-sound combination compared to a unisensory picture. EEG data revealed that the neuronal response to a cross-modal stimulus happened as fast as 60–136 ms and predominantly influenced activation in the right lateral occipital complex. Where a semantically congruent picture-sound combination increased discrimination accuracy on a second presentation, a pure tone decreased discrimination accuracy on a second presentation ( Lehmann and Murray, 2005 ; Thelen et al., 2012 ). Thelen et al. (2015) replicate these earlier findings, while also showing the same effects in the auditory modality. Single-trial multisensory memories affect later auditory recognition. If cross-modal objects were congruent (visual and auditory information match semantically) accuracy was higher compared to unisensory stimuli but became worse if objects were incongruent or meaningless. Unisensory percepts seem to trigger the multisensory representations associated with them, suggesting at least a partially integrated storage in memory. Yet, it seems a multisensory representation stored in memory is only beneficial for memory performance when sounds and pictures are semantically congruent. These studies show that an internal representation is formed in which both the visual and auditory information is encoded. Moreover, they also indicate that information presented in a task irrelevant modality interferes with the task relevant representation. But although this still does not address the question of whether unisensory information is still accessible it does show that the original unisensory representations are closely related. Similar to the findings in research on attention and multisensory integration, it seems that top-down and bottom-up processes play an important part in the integration of cross-modal information in working memory representations.
Predictive Coding and Multisensory Working Memory
One influential framework that can explain the intricacies of top-down and bottom-up interactions in multisensory memory is that of predictive coding. The predictive coding framework states that the brain produces a Bayesian estimate of the environment ( Friston, 2010 ). According to this view, stochastic models of the environment exist in the brain, which are continuously updated on the basis of processed sensory information. Higher-order brain areas thus provide the lower areas with predictions (or in Bayesian terms “priors”) that influence the processing of ongoing sensory input. A strong mismatch between the prediction and the actual sensory input will then result in a major update of the internal model. Thus when we are in a complex environment with many stimuli competing for processing capacity, incongruence between the top-down predictions of the environment and the present incoming environmental information can determine the priority with which incoming stimuli need to be processed and integrated. The processed information changes the predictions and vice versa . Bottom-up sensory processing and top-down predictions mutually define each other continuously. In this way, the predictive coding view can explain how top-down and bottom-up processes interact in multisensory integration.
Talsma (2015) recently argued that the dynamic model of our environment provided by the aforementioned stochastic representations is essential to understanding the interaction between basic (multi)sensory processing on the one hand, and memory and attention on the other. For instance, Vetter et al. (2014) showed that actual auditory stimulation as well as imagined sounds could activate the visual cortex. Based on the predictive coding framework, these authors argued that visual cortex activation came about because either direct sensory information or a stored memory representation thereof could update the internal representation of the sound and therefore indirectly influence processing in visual cortex accordingly. This suggests that attention, memory, and multisensory processing are intrinsically intertwined. Similarly, Berger and Ehrsson (2013 , 2014) showed that imagined sounds can mimic the effects of actual sounds in a number of well-known multisensory illusions, such as the bounce-pass illusion ( Sekuler et al., 1997 ), the McGurk effect ( McGurk and MacDonald, 1976 ), or the ventriloquist illusion ( Howard and Templeton, 1966 ), and show independent of each other that visual cortex can be activated both by multisensory stimulation and by memory. Based on these findings, Talsma (2015) argued that despite the fact that several studies showed that auditory and visual inputs can interact at very early processing stages, the actual integration of the sensory inputs into a coherent mental representation occurs at later, higher-order processing stages.
An important consequence of applying the predictive coding framework is that our internal representation is assumed not only to be built on the basis of direct sensory input, but that it is also updated (and made consistent with) information stored in memory. Thus, attention is assumed to play an essential role in regulating how our sensory input is combined with these pre-existing representations stored in long-term memory. This is largely consistent with Cowan’s (2001) idea of the focus of attention, which is a part of activated long-term memory, as well as with Baddeley’s (2000) episodic buffer, although, Baddeley recently argued that attention in the form of the central executive was not necessary for the integration of multiple sources of information in the episodic buffer ( Baddeley et al., 2011 ).
A further consequence of applying the predictive coding framework is that the internal representation is by definition always multisensory. Moreover, the active representation integrates all possible sources of information, including semantic information from long-term memory. Thus, even when only a unisensory stimulus is presented, associated representations will be activated as well. These can include information from other modalities, prior experience with the stimulus, or learned associations. Because the formation of this internal mental representation is an active process that influences ongoing processes in the sensory cortices, this model can explain why memory traces in one modality can be strengthened or corrupted by traces in another one. Furthermore, because the active representation sends feedback information to the low-level processes in sensory cortices it can be assumed that the original unisensory memory traces are still present albeit in a relatively fragile state.
Multisensory Working Memory Representations in Current Models
The active internal environmental model as proposed by the predictive coding framework would be akin to what we would describe as a multisensory working memory representation. This memory representation does not only consist of information coming from different modalities but also includes information from long-term memory such as semantic knowledge or learned associations. Taking the previously mentioned example of memorizing a cat picture the multisensory representation includes not only the visual features of the cat, but also long-term semantic knowledge of cats, autobiographical knowledge (previous personal experience with cats), and information from modalities not presented with the picture (the sound a cat makes or the knowledge that its fur is soft to the touch). We assume that working memory has an amodal central storage component. Whether this is the main component of working memory as suggested by Cowan (2001) or a part of a bigger system like the episodic buffer in ( Baddeley’s, 2000 ; Baddeley et al., 2011 ) remains a point for further investigation.
The predictive coding framework would suggest that incoming sensory information is constantly used to update the internal environmental model, implying that incoming stimuli tend to integrate into a coherent multisensory representation. This framework can also explain why working memory is amodal in some cases and modality specific in others. For instance, Postle (2006) argued that working memory for modality-specific stimuli occurs in the sensory cortices. Recently, Yonelinas (2013) suggested that high-resolution bindings are stored in the hippocampus that can be used to support perception and working memory, specifically in memorizing (combinations of) complex features. In the latter case it is plausible that the multisensory representation will be activated, whereas in the former case it is not. Based on this, one important implication of the predictive coding approach is that differences in task and stimulus complexity can yield rather drastically different outcomes. With this in mind a recommendation for future research would be to consider effects of task and stimulus complexity on working memory activation.
Based on the above mentioned framework, we assume that sensory cortices can retain small amounts of modality-specific information (as suggested by Postle, 2006 ) and that this information supports a multisensory memory representation in higher order areas (e.g., the hippocampus; Yonelinas, 2013 ). Whether working memory for a specific task involves the higher-order areas or the sensory areas to retain information for limited time depends on the task and the information that needs to be memorized. For example, simple flashes and beeps could be retained in the sensory areas, whereas more complex information would also require the higher-order areas. In that sense the sensory cortices would retain information in a manner similar to separate slave systems ( Baddeley and Hitch, 1974 ) or the recently suggested peripheral storage ( Cowan et al., 2014 ).
Summary and Conclusions
In this paper we have reviewed recent developments in multisensory working memory research. Research has shown that cross-modal information interacts in working memory beyond what would be expected from the traditional modality-specific stores. Recall is better for cross-modal objects compared to modality-specific objects ( Thompson and Paivio, 1994 ; Goolkasian and Foos, 2005 ; Delogu et al., 2009 ), working memory capacity can be higher for cross-modal objects than for unimodal objects ( Saults and Cowan, 2007 ; Fougnie and Marois, 2011 ), and visual and auditory memory can interfere with each other ( Morey and Cowan, 2004 , 2005 ; Goolkasian and Foos, 2005 ). Furthermore, multisensory information has an effect on the subsequent memorization of a unisensory object ( Botta et al., 2011 ) and multisensory memory representations can influence subsequent unisensory stimulus discrimination ( Murray et al., 2004 ; Lehmann and Murray, 2005 ; Thelen et al., 2012 , 2015 ). Taken together, these studies show that sensory representations in multiple modalities interact more with each other than can be explained by classical modal models.
Paivio’s (1971 , 1986 ) dual coding theory states that although cross-modal information can interact it is in fact independent, because modality-specific information can still be retrieved in isolation. However, studies done by Thelen and colleagues ( Thelen et al., 2012 , 2015 ) show that this retrieval of modality-specific information from a cross-modal representation is more difficult than assumed, because a task irrelevant modality interferes with the task relevant representation. Moreover, higher-order representations of the external world built from memorized information have been shown to influence visual processing. Complex representations seem to be formed in working memory, consisting of the integration of several independent representations that can be sensory, and short- or long-term memory activations. Depending on task requirements either just the simple modal representation or the complex high-resolution binding of several features at once will become active. Therefore, we conclude that working memory is in essence multisensory, and that this must be taken into account to achieve a realistic understanding of how working memory processes maintain and manipulate information.
Conflict of Interest Statement
The authors declare that the research was conducted in the absence of any commercial or financial relationships that could be construed as a potential conflict of interest.
Acknowledgments
We would like to thank the editor and reviewers for their helpful comments and suggestions on earlier drafts of this paper.
Alsius, A., Navarra, J., Campbell, R., and Soto-Faraco, S. (2005). Audiovisual integration of speech falters under high attention demands. Curr. Biol. 15, 839–843. doi: 10.1016/j.cub.2005.03.046
PubMed Abstract | Full Text | CrossRef Full Text | Google Scholar
Alsius, A., Navarra, J., and Soto-Faraco, S. (2007). Attention to touch weakens audiovisual speech integration. Exp. Brain Res. 183, 399–404. doi: 10.1007/s00221-007-1110-1
Atkinson, R. C., and Shiffrin, R. M. (1968). Human memory: a proposed system and its control processes. Psychol. Learn. Motiv. 2, 89–195. doi: 10.1016/s0079-7421(08)60422-3
CrossRef Full Text | Google Scholar
Awh, E., Vogel, E. K., and Oh, S. H. (2006). Interactions between attention and working memory. Neuroscience 139, 201–208. doi: 10.1016/j.neuroscience.2005.08.023
Baddeley, A. (2000). The episodic buffer: a new component of working memory?. Trends Cogn. Sci. 4, 417–423. doi: 10.1016/s1364-6613(00)01538-2
Baddeley, A. D., Allen, R. J., and Hitch, G. J. (2011). Binding in visual working memory: the role of the episodic buffer. Neuropsychologia 49, 1393–1400. doi: 10.1016/j.neuropsychologia.2010.12.042
Baddeley, A. D., and Hitch, G. (1974). Working memory. Psychol. learn. Motiv. 8, 47–89. doi: 10.1016/S0079-7421(08)60452-1
Bao, M., Li, Z. H., and Zhang, D. R. (2007). Binding facilitates attention switching within working memory. J. Exp. Psychol. Learn. Mem. Cogn. 33, 959–969. doi: 10.1037/0278-7393.33.5.959
Beauchamp, M. S. (2005). See me, hear me, touch me: multisensory integration in lateral occipital-temporal cortex. Curr. Opin. Neurobiol. 15, 145–153. doi: 10.1016/j.conb.2005.03.011
Beauchamp, M. S., Lee, K. E., Argall, B. D., and Martin, A. (2004). Integration of auditory and visual information about objects in superior temporal sulcus. Neuron 41, 809–823. doi: 10.1016/s0896-6273(04)00070-4
Berger, C. C., and Ehrsson, H. H. (2013). Mental imagery changes multisensory perception. Curr. Biol. 23, 1367–1372. doi: 10.1016/j.cub.2013.06.012
Berger, C. C., and Ehrsson, H. H. (2014). The fusion of mental imagery and sensation in the temporal association cortex. J. Neurosci. 34, 13684–13692. doi: 10.1523/JNEUROSCI.0943-14.2014
Botta, F., Santangelo, V., Raffone, A., Sanabria, D., Lupiáñez, J., and Belardinelli, M. O. (2011). Multisensory integration affects visuo-spatial working memory. J. Exp. Psychol. Hum. Percept. Perform. 37, 1099–1109. doi: 10.1037/a0023513
Busse, L., Roberts, K. C., Crist, R. E., Weissman, D. H., and Woldorff, M. G. (2005). The spread of attention across modalities and space in a multisensory object. Proc. Natl. Acad. Sci. U S A 102, 18751–18756. doi: 10.1073/pnas.0507704102
Calvert, G. A. (2001). Crossmodal processing in the human brain: insights from functional neuroimaging studies. Cereb. Cortex 11, 1110–1123. doi: 10.1093/cercor/11.12.1110
Calvert, G. A., Campbell, R., and Brammer, M. J. (2000). Evidence from functional magnetic resonance imaging of crossmodal binding in the human heteromodal cortex. Curr. Biol. 10, 649–657. doi: 10.1016/s0960-9822(00)00513-3
Campo, P., Maestú, F., Capilla, A., Morales, M., Fernández, S., del Río, D., et al. (2008). Temporal dynamics of parietal activity during word-location binding. Neuropsychology 22, 85–99. doi: 10.1037/0894-4105.22.1.85
Campo, P., Poch, C., Parmentier, F. B., Moratti, S., Elsley, J. V., Castellanos, N. P., et al. (2010). Oscillatory activity in prefrontal and posterior regions during implicit letter-location binding. Neuroimage 49, 2807–2815. doi: 10.1016/j.neuroimage.2009.10.024
Cocchini, G., Logie, R. H., Della Sala, S., MacPherson, S. E., and Baddeley, A. D. (2002). Concurrent performance of two memory tasks: evidence for domain-specific working memory systems. Mem. Cognit. 30, 1086–1095. doi: 10.3758/bf03194326
Cowan, N. (2001). The magical number 4 in short-term memory: a reconsideration of mental storage capacity. Behav. Brain Sci. 24, 87–114; discussion 114–185. doi: 10.1017/s0140525x01003922
PubMed Abstract | Full Text | CrossRef Full Text
Cowan, N., Saults, J. S., and Blume, C. L. (2014). Central and peripheral components of working memory storage. J. Exp. Psychol. Gen. 143, 1806–1836. doi: 10.1037/a0036814
Darling, S., Allen, R. J., Havelka, J., Campbell, A., and Rattray, E. (2012). Visuospatial bootstrapping: long-term memory representations are necessary for implicit binding of verbal and visuospatial working memory. Psychon. Bull. Rev. 19, 258–263. doi: 10.3758/s13423-011-0197-3
Delogu, F., Raffone, A., and Belardinelli, M. O. (2009). Semantic encoding in working memory: is there a (multi) modality effect?. Memory 17, 655–663. doi: 10.1080/09658210902998054
D’Esposito, M., and Postle, B. R. (2015). The cognitive neuroscience of working memory. Annu. Rev. Psychol. 66, 115–142. doi: 10.1146/annurev-psych-010814-015031
Diamantopoulou, S., Poom, L., Klaver, P., and Talsma, D. (2011). Visual working memory capacity and stimulus categories: a behavioral and electrophysiological investigation. Exp. Brain Res. 209, 501–513. doi: 10.1007/s00221-011-2536-z
Dionne, J. K., Meehan, S. K., Legon, W., and Staines, W. R. (2010). Crossmodal influences in somatosensory cortex: interaction of vision and touch. Hum. Brain Mapp. 31, 14–25. doi: 10.1002/hbm.20841
Druzgal, T. J., and D’Esposito, M. (2003). Dissecting contributions of prefrontal cortex and fusiform face area to face working memory. J. Cogn. Neurosci. 15, 771–784. doi: 10.1162/089892903322370708
Elsley, J. V., and Parmentier, F. B. (2009). Is verbal-spatial binding in working memory impaired by a concurrent memory load?. Q. J. Exp. Psychol. (Hove) 62, 1696–1705. doi: 10.1080/17470210902811231
Fougnie, D., and Marois, R. (2011). What limits working memory capacity? Evidence for modality-specific sources to the simultaneous storage of visual and auditory arrays. J. Exp. Psychol. Learn. Mem. Cogn. 37, 1329–3141. doi: 10.1037/a0024834
Foxe, J. J., and Schroeder, C. E. (2005). The case for feedforward multisensory convergence during early cortical processing. Neuroreport 16, 419–423. doi: 10.1097/00001756-200504040-00001
Foxe, J. J., Wylie, G. R., Martinez, A., Schroeder, C. E., Javitt, D. C., Guilfoyle, D., et al. (2002). Auditory-somatosensory multisensory processing in auditory association cortex: an fMRI study. J. Neurophysiol. 88, 540–543. doi: 10.10.1152/jn.00694.2001
Friston, K. (2010). The free-energy principle: a unified brain theory? Nat. Rev. Neurosci. 11, 127–138. doi: 10.1038/nrn2787
Fuster, J., Bodner, M., and Kroger, J. (2000). Cross-modal and cross-temporal association in neurons of frontal cortex. Nature 405, 347–351. doi: 10.1038/35012613
Ghazanfar, A. A., and Schroeder, C. E. (2006). Is neocortex essentially multisensory? Trends Cogn. Sci. 10, 278–285. doi: 10.1016/j.tics.2006.04.008
Giard, M. H., and Peronnet, F. (1999). Auditory-visual integration during multimodal object recognition in humans: a behavioral and electrophysiological study. J. Cogn. Neurosci. 11, 473–490. doi: 10.1162/089892999563544
Goolkasian, P., and Foos, P. W. (2005). Bimodal format effects in working memory. Am. J. Psychol. 118, 61–77.
PubMed Abstract | Full Text | Google Scholar
Guérard, K., Morey, C. C., Lagacé, S., and Tremblay, S. (2013). Asymmetric binding in serial memory for verbal and spatial information. Mem. Cognit. 41, 378–391. doi: 10.3758/s13421-012-0275-4
Hardman, K. O., and Cowan, N. (2015). Remembering complex objects in visual working memory: do capacity limits restrict objects or features?. J. Exp. Psychol. Learn. Mem. Cogn. 41, 325–347. doi: 10.1037/xlm0000031
Howard, I. P., and Templeton, W. B. (1966). Human Spatial Orientation. Oxford, England: John Wiley and Sons.
Google Scholar
Jiang, Y., Olson, I. R., and Chun, M. M. (2000). Organization of visual short-term memory. J. Exp. Psychol. Learn. Mem. Cogn. 26, 683–702. doi: 10.1037/0278-7393.26.3.683
Kiyonaga, A., and Egner, T. (2013). Working memory as internal attention: toward an integrative account of internal and external selection processes. Psychon. Bull. Rev. 20, 228–242. doi: 10.3758/s13423-012-0359-y
Klaver, P., Smid, H. G., and Heinze, H. J. (1999). Representations in human visual short-term memory: an event-related brain potential study. Neurosci. Lett. 268, 65–68. doi: 10.1016/s0304-3940(99)00380-8
Klaver, P., and Talsma, D. (2013). Behind the scenes: how visual memory load biases selective attention during processing of visual streams. Psychophysiology 50, 1133–1146. doi: 10.1111/psyp.12126
Klemen, J., and Chambers, C. D. (2012). Current perspectives and methods in studying neural mechanisms of multisensory interactions. Neurosci. Biobehav. Rev. 36, 111–133. doi: 10.1016/j.neubiorev.2011.04.015
Koelewijn, T., Bronkhorst, A., and Theeuwes, J. (2009). Auditory and visual capture during focused visual attention. J. Exp. Psychol. Hum. Percept. Perform. 35, 1303–1315. doi: 10.1037/a0013901
Lehmann, S., and Murray, M. M. (2005). The role of multisensory memories in unisensory object discrimination. Brain Res. Cogn. Brain Res. 24, 326–334. doi: 10.1016/j.cogbrainres.2005.02.005
Logie, R. H., Della Sala, S., Wynn, V., and Baddeley, A. D. (2000). Visual similarity effects in immediate verbal serial recall. Q. J. Exp. Psychol. A 53, 626–646. doi: 10.1080/027249800410463
Luck, S. J., and Vogel, E. K. (1997). The capacity of visual working memory for features and conjunctions. Nature 390, 279–281. doi: 10.1038/36846
Luria, R., Sessa, P., Gotler, A., Jolicoeur, P., and Dell’Acqua, R. (2010). Visual short-term memory capacity for simple and complex objects. J. Cogn. Neurosci. 22, 496–512. doi: 10.1162/jocn.2009.21214
Luria, R., and Vogel, E. K. (2011). Shape and color conjunction stimuli are represented as bound objects in visual working memory. Neuropsychologia 49, 1632–1639. doi: 10.1016/j.neuropsychologia.2010.11.031
Macaluso, E., and Driver, J. (2005). Multisensory spatial interactions: a window onto functional integration in the human brain. Trends Neurosci. 28, 264–271. doi: 10.1016/j.tins.2005.03.008
McGurk, H., and MacDonald, J. (1976). Hearing lips and seeing voices. Nature 264, 746–748. doi: 10.1038/264746a0
Meier, T. B., Nair, V. A., Meyerand, M. E., Birn, R. M., and Prabhakaran, V. (2014). The neural correlates of age effects on verbal-spatial binding in working memory. Behav. Brain Res. 266, 146–152. doi: 10.1016/j.bbr.2014.03.005
Molholm, S., Martinez, A., Shpaner, M., and Foxe, J. J. (2007). Object-based attention is multisensory: co-activation of an object’s representations in ignored sensory modalities. Eur. J. Neurosci. 26, 499–509. doi: 10.1111/j.1460-9568.2007.05668.x
Molholm, S., Ritter, W., Murray, M. M., Javitt, D. C., Schroeder, C. E., and Foxe, J. J. (2002). Multisensory auditory-visual interactions during early sensory processing in humans: a high-density electrical mapping study. Brain Res. Cogn. Brain Res. 14, 115–128. doi: 10.1016/s0926-6410(02)00066-6
Morey, C. C., and Cowan, N. (2004). When visual and verbal memories compete: evidence of cross-domain limits in working memory. Psychon. Bull. Rev. 11, 296–301. doi: 10.3758/bf03196573
Morey, C. C., and Cowan, N. (2005). When do visual and verbal memories conflict? The importance of working-memory load and retrieval. J. Exp. Psychol. Learn. Mem. Cogn. 31, 703–713. doi: 10.1037/0278-7393.31.4.703
Murray, M. M., Michel, C. M., Grave de Peralta, R., Ortigue, S., Brunet, D., Gonzalez Andino, S., et al. (2004). Rapid discrimination of visual and multisensory memories revealed by electrical neuroimaging. Neuroimage 21, 125–135. doi: 10.1016/j.neuroimage.2003.09.035
Murray, A. M., Nobre, A. C., Clark, I. A., Cravo, A. M., and Stokes, M. G. (2013). Attention restores discrete items to visual short-term memory. Psychol. Sci. 24, 550–556. doi: 10.1177/0956797612457782
Oberauer, K., and Eichenberger, S. (2013). Visual working memory declines when more features must be remembered for each object. Mem. Cognit. 41, 1212–1227. doi: 10.3758/s13421-013-0333-6
Oberauer, K., and Hein, L. (2012). Attention to information in working memory. Curr. Dir. Psychol. Sci. 21, 164–169. doi: 10.1177/0963721412444727
Olivers, C. N. L. (2008). Interactions between visual working memory and visual attention. Front. Biosci. 13, 1182–1191. doi: 10.2741/2754
Olivers, C. N. L., Meijer, F., and Theeuwes, J. (2006). Feature-based memory-driven attentional capture: visual working memory content affects visual attention. J. Exp. Psychol. Hum. Percept. Perform. 32, 1243–1265. doi: 10.1037/0096-1523.32.5.1243
Olson, I. R., and Marshuetz, C. (2005). Remembering “what” brings along “where” in visual working memory. Percept. Psychophys. 67, 185–194. doi: 10.3758/bf03206483
Olsson, H., and Poom, L. (2005). Visual memory needs categories. Proc. Natl. Acad. Sci. U S A 102, 8776–8780. doi: 10.1073/pnas.0500810102
Paivio, A. (1971). Imagery and Verbal Processes. New York: Holt, Rinehart and Winston.
Paivio, A. (1986). Mental Representation: A Dual Coding Approach. New York: Oxford University Press.
Postle, B. R. (2006). Working memory as an emergent property of the mind and brain. Neuroscience 139, 23–38. doi: 10.1016/j.neuroscience.2005.06.005
Postle, B. R., and D’Esposito, M. (1999). Dissociation of human caudate nucleus activity in spatial and nonspatial working memory: an event-related fMRI study. Brain Res. Cogn. Brain Res. 8, 107–115. doi: 10.1016/s0926-6410(99)00010-5
Prabhakaran, V., Narayanan, K., Zhao, Z., and Gabrieli, J. D. E. (2000). Integration of diverse information in working memory within the frontal lobe. Nat. Neurosci. 3, 85–90. doi: 10.10.1038/71156
Ranganath, C., DeGutis, J., and D’Esposito, M. (2004). Category-specific modulation of inferior temporal activity during working memory encoding and maintenance. Brain Res. Cogn. Brain Res. 20, 37–45. doi: 10.1016/j.cogbrainres.2003.11.017
Saults, J. S., and Cowan, N. (2007). A central capacity limit to the simultaneous storage of visual and auditory arrays in working memory. J. Exp. Psychol. Gen. 136, 663–684. doi: 10.1037/0096-3445.136.4.663
Schneider, W., and Detweiler, M. (1988). A connectionist/control architecture for working memory. Psychol. Learn. Motiv. 21, 53–119. doi: 10.1016/s0079-7421(08)60026-2
Sekuler, R., Sekuler, A. B., and Lau, R. (1997). Sound alters visual motion perception. Nature 385:308. doi: 10.1038/385308a0
Senkowski, D., Talsma, D., Herrmann, C. S., and Woldorff, M. G. (2005). Multisensory processing and oscillatory gamma responses: effects of spatial selective attention. Exp. Brain Res. 166, 411–426. doi: 10.1007/s00221-005-2381-z
Spence, C., and Driver, J. (1997). Audiovisual links in exogenous covert spatial orienting. Percept. Psychophys. 59, 1–22. doi: 10.3758/bf03206843
Stein, B. E., Burr, D., Constantinidis, C., Laurienti, P. J., Alex Meredith, M., Perrault, T. J., et al. (2010). Semantic confusion regarding the development of multisensory integration: a practical solution. Eur. J. Neurosci. 31, 1713–1720. doi: 10.1111/j.1460-9568.2010.07206.x
Stein, B. E., and Stanford, T. R. (2008). Multisensory integration: current issues from the perspective of the single neuron. Nat. Rev. Neurosci. 9, 255–266. doi: 10.1038/nrn2331
Talsma, D. (2015). Predictive coding and multisensory integration: an attentional account of the multisensory mind. Front. Integr. Neurosci. 9:19. doi: 10.10.3389/fnint.2015.00019
Talsma, D., Doty, T. J., and Woldorff, M. G. (2007). Selective attention and audiovisual integration: is attending to both modalities a prerequisite for early integration? Cereb. Cortex 17, 679–690. doi: 10.1093/cercor/bhk016
Talsma, D., Senkowski, D., Soto-Faraco, S., and Woldorff, M. G. (2010). The multifaceted interplay between attention and multisensory integration. Trends Cogn. Sci. 14, 400–410. doi: 10.1016/j.tics.2010.06.008
Talsma, D., and Woldorff, M. G. (2005). Selective attention and multisensory integration: multiple phases of effects on the evoked brain activity. J. Cogn. Neurosci. 17, 1098–1114. doi: 10.1162/0898929054475172
Thelen, A., Cappe, C., and Murray, M. (2012). Electrical neuroimaging of memory discrimination based on single-trial multisensory learning. Neuroimage 62, 1478–1488. doi: 10.1016/j.neuroimage.2012.05.027
Thelen, A., Talsma, D., and Murray, M. M. (2015). Single-trial multisensory memories affect later auditory and visual object discrimination. Cognition 138, 148–160. doi: 10.10.1016/j.cognition.2015.02.003
Thompson, V., and Paivio, A. (1994). Memory for pictures and sounds: independence of auditory and visual codes. Can. J. Exp. Psychol. 48, 380–398. doi: 10.1037/1196-1961.48.3.380
Uncapher, M. R., Hutchinson, J. B., and Wagner, A. D. (2011). Dissociable effects of top-down and bottom-up attention during episodic encoding. J. Neurosci. 31, 12613–12628. doi: 10.1523/jneurosci.0152-11.2011
Van der Burg, E., Olivers, C. N. L., Bronkhorst, A. W., and Theeuwes, J. (2008). Pip and pop: nonspatial auditory signals improve spatial visual search. J. Exp. Psychol. Hum. Percept. Perform. 34, 1053–1065. doi: 10.1037/0096-1523.34.5.1053
Van der Burg, E., Olivers, C. N. L., and Theeuwes, J. (2012). The attentional window modulates capture by audiovisual events. PLoS One 7:e39137. doi: 10.1371/journal.pone.0039137
Van der Burg, E., Talsma, D., Olivers, C. N. L., Hickey, C., and Theeuwes, J. (2011). Early multisensory interactions affect the competition among multiple visual objects. Neuroimage 55, 1208–1218. doi: 10.1016/j.neuroimage.2010.12.068
Vetter, P., Smith, F. W., and Muckli, L. (2014). Decoding sound and imagery content in early visual cortex. Curr. Biol. 24, 1256–1262. doi: 10.1016/j.cub.2014.04.020
Vogel, E. K., and Machizawa, M. G. (2004). Neural activity predicts individual differences in visual working memory capacity. Nature 428, 748–751. doi: 10.1038/nature02447
Vogel, E. K., McCollough, A. W., and Machizawa, M. G. (2005). Neural measures reveal individual differences in controlling access to working memory. Nature 438, 500–503. doi: 10.1038/nature04171
Vogel, E. K., Woodman, G. F., and Luck, S. J. (2001). Storage of features, conjunctions and objects in visual working memory. J. Exp. Psychol. Hum. Percept. Perform. 27, 92–114. doi: 10.1037//0096-1523.27.1.92
Wallace, M. T., Meredith, M. A., and Stein, B. E. (1993). Converging influences from visual, auditory and somatosensory cortices onto output neurons of the superior colliculus. J. Neurophysiol. 69, 1797–1809.
Wheeler, M. E., and Treisman, A. M. (2002). Binding in short-term visual memory. J. Exp. Psychol. Gen. 131, 48–64. doi: 10.1037/0096-3445.131.1.48
Wright, T. M., Pelphrey, K. A., Allison, T., McKeown, M. J., and McCarthy, G. (2003). Polysensory interactions along lateral temporal regions evoked by audiovisual speech. Cereb. Cortex 13, 1034–1043. doi: 10.1093/cercor/13.10.1034
Yonelinas, A. P. (2013). The hippocampus supports high-resolution binding in the service of perception, working memory and long-term memory. Behav. Brain Res. 254, 34–44. doi: 10.1016/j.bbr.2013.05.030
Keywords: working memory, multisensory processing, multisensory integration, attention, top-down, bottom-up
Citation: Quak M, London RE and Talsma D (2015) A multisensory perspective of working memory. Front. Hum. Neurosci. 9:197. doi: 10.3389/fnhum.2015.00197
Received: 03 December 2014; Accepted: 25 March 2015; Published online: 21 April 2015.
Reviewed by:
Copyright © 2015 Quak, London and Talsma. This is an open-access article distributed under the terms of the Creative Commons Attribution License (CC BY) . The use, distribution and reproduction in other forums is permitted, provided the original author(s) or licensor are credited and that the original publication in this journal is cited, in accordance with accepted academic practice. No use, distribution or reproduction is permitted which does not comply with these terms.
*Correspondence: Michel Quak, Department of Experimental Psychology, Ghent University, Henri Dunantlaan 2, 9000, Ghent, Belgium [email protected]
This article is part of the Research Topic
Turning the Mind’s Eye Inward: The Interplay between Selective Attention and Working Memory
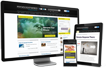
Learn More Psychology
- Memory Psychology
10 Influential Memory Theories and Studies in Psychology
Discover the experiments and theories that shaped our understanding of how we develop and recall memories..
Permalink Print |
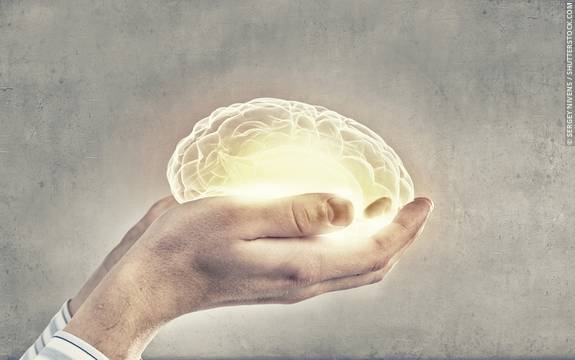
How do our memories store information? Why is it that we can recall a memory at will from decades ago, and what purpose does forgetting information serve?
The human memory has been the subject of investigation among many 20th Century psychologists and remains an active area of study for today’s cognitive scientists. Below we take a look at some of the most influential studies, experiments and theories that continue to guide our understanding of the function of memory.
1 Multi-Store Model
(atkinson & shiffrin, 1968).
An influential theory of memory known as the multi-store model was proposed by Richard Atkinson and Richard Shiffrin in 1968. This model suggested that information exists in one of 3 states of memory: the sensory, short-term and long-term stores . Information passes from one stage to the next the more we rehearse it in our minds, but can fade away if we do not pay enough attention to it. Read More
Information enters the memory from the senses - for instance, the eyes observe a picture, olfactory receptors in the nose might smell coffee or we might hear a piece of music. This stream of information is held in the sensory memory store , and because it consists of a huge amount of data describing our surroundings, we only need to remember a small portion of it. As a result, most sensory information ‘ decays ’ and is forgotten after a short period of time. A sight or sound that we might find interesting captures our attention, and our contemplation of this information - known as rehearsal - leads to the data being promoted to the short-term memory store , where it will be held for a few hours or even days in case we need access to it.
The short-term memory gives us access to information that is salient to our current situation, but is limited in its capacity.
Therefore, we need to further rehearse information in the short-term memory to remember it for longer. This may involve merely recalling and thinking about a past event, or remembering a fact by rote - by thinking or writing about it repeatedly. Rehearsal then further promotes this significant information to the long-term memory store, where Atkinson and Shiffrin believed that it could survive for years, decades or even a lifetime.
Key information regarding people that we have met, important life events and other important facts makes it through the sensory and short-term memory stores to reach the long-term memory .
Learn more about Atkinson and Shiffrin’s Multi-Store Model
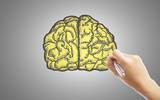
2 Levels of Processing
(craik & lockhart, 1972).
Fergus Craik and Robert Lockhart were critical of explanation for memory provided by the multi-store model, so in 1972 they proposed an alternative explanation known as the levels of processing effect . According to this model, memories do not reside in 3 stores; instead, the strength of a memory trace depends upon the quality of processing , or rehearsal , of a stimulus . In other words, the more we think about something, the more long-lasting the memory we have of it ( Craik & Lockhart , 1972). Read More
Craik and Lockhart distinguished between two types of processing that take place when we make an observation : shallow and deep processing. Shallow processing - considering the overall appearance or sound of something - generally leads to a stimuli being forgotten. This explains why we may walk past many people in the street on a morning commute, but not remember a single face by lunch time.
Deep (or semantic) processing , on the other hand, involves elaborative rehearsal - focusing on a stimulus in a more considered way, such as thinking about the meaning of a word or the consequences of an event. For example, merely reading a news story involves shallow processing, but thinking about the repercussions of the story - how it will affect people - requires deep processing, which increases the likelihood of details of the story being memorized.
In 1975, Craik and another psychologist, Endel Tulving , published the findings of an experiment which sought to test the levels of processing effect.
Participants were shown a list of 60 words, which they then answered a question about which required either shallow processing or more elaborative rehearsal. When the original words were placed amongst a longer list of words, participants who had conducted deeper processing of words and their meanings were able to pick them out more efficiently than those who had processed the mere appearance or sound of words ( Craik & Tulving , 1975).
Learn more about Levels of Processing here
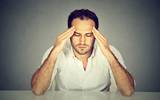
3 Working Memory Model
(baddeley & hitch, 1974).
Whilst the Multi-Store Model (see above) provided a compelling insight into how sensory information is filtered and made available for recall according to its importance to us, Alan Baddeley and Graham Hitch viewed the short-term memory (STM) store as being over-simplistic and proposed a working memory model (Baddeley & Hitch, 1974), which replace the STM.
The working memory model proposed 2 components - a visuo-spatial sketchpad (the ‘inner eye’) and an articulatory-phonological loop (the ‘inner ear’), which focus on a different types of sensory information. Both work independently of one another, but are regulated by a central executive , which collects and processes information from the other components similarly to how a computer processor handles data held separately on a hard disk. Read More
According to Baddeley and Hitch, the visuo-spatial sketchpad handles visual data - our observations of our surroundings - and spatial information - our understanding of objects’ size and location in our environment and their position in relation to ourselves. This enables us to interact with objects: to pick up a drink or avoid walking into a door, for example.
The visuo-spatial sketchpad also enables a person to recall and consider visual information stored in the long-term memory. When you try to recall a friend’s face, your ability to visualize their appearance involves the visuo-spatial sketchpad.
The articulatory-phonological loop handles the sounds and voices that we hear. Auditory memory traces are normally forgotten but may be rehearsed using the ‘inner voice’; a process which can strengthen our memory of a particular sound.
Learn more about Baddeley and Hitch’s working memory model here
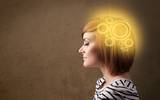
4 Miller’s Magic Number
(miller, 1956).
Prior to the working memory model, U.S. cognitive psychologist George A. Miller questioned the limits of the short-term memory’s capacity. In a renowned 1956 paper published in the journal Psychological Review , Miller cited the results of previous memory experiments, concluding that people tend only to be able to hold, on average, 7 chunks of information (plus or minus two) in the short-term memory before needing to further process them for longer storage. For instance, most people would be able to remember a 7-digit phone number but would struggle to remember a 10-digit number. This led to Miller describing the number 7 +/- 2 as a “magical” number in our understanding of memory. Read More
But why are we able to remember the whole sentence that a friend has just uttered, when it consists of dozens of individual chunks in the form of letters? With a background in linguistics, having studied speech at the University of Alabama, Miller understood that the brain was able to ‘chunk’ items of information together and that these chunks counted towards the 7-chunk limit of the STM. A long word, for example, consists of many letters, which in turn form numerous phonemes. Instead of only being able to remember a 7-letter word, the mind “recodes” it, chunking the individual items of data together. This process allows us to boost the limits of recollection to a list of 7 separate words.
Miller’s understanding of the limits of human memory applies to both the short-term store in the multi-store model and Baddeley and Hitch’s working memory. Only through sustained effort of rehearsing information are we able to memorize data for longer than a short period of time.
Read more about Miller’s Magic Number here
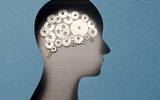
5 Memory Decay
(peterson and peterson, 1959).
Following Miller’s ‘magic number’ paper regarding the capacity of the short-term memory, Peterson and Peterson set out to measure memories’ longevity - how long will a memory last without being rehearsed before it is forgotten completely?
In an experiment employing a Brown-Peterson task, participants were given a list of trigrams - meaningless lists of 3 letters (e.g. GRT, PXM, RBZ) - to remember. After the trigrams had been shown, participants were asked to count down from a number, and to recall the trigrams at various periods after remembering them. Read More
The use of such trigrams makes it impracticable for participants to assign meaning to the data to help encode them more easily, while the interference task prevented rehearsal, enabling the researchers to measure the duration of short-term memories more accurately.
Whilst almost all participants were initially able to recall the trigrams, after 18 seconds recall accuracy fell to around just 10%. Peterson and Peterson’s study demonstrated the surprising brevity of memories in the short-term store, before decay affects our ability to recall them.
Learn more about memory decay here
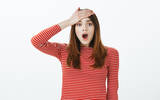
6 Flashbulb Memories
(brown & kulik, 1977).
There are particular moments in living history that vast numbers of people seem to hold vivid recollections of. You will likely be able to recall such an event that you hold unusually detailed memories of yourself. When many people learned that JFK, Elvis Presley or Princess Diana died, or they heard of the terrorist attacks taking place in New York City in 2001, a detailed memory seems to have formed of what they were doing at the particular moment that they heard such news.
Psychologists Roger Brown and James Kulik recognized this memory phenomenon as early as 1977, when they published a paper describing flashbulb memories - vivid and highly detailed snapshots created often (but not necessarily) at times of shock or trauma. Read More
We are able to recall minute details of our personal circumstances whilst engaging in otherwise mundane activities when we learnt of such events. Moreover, we do not need to be personally connected to an event for it to affect us, and for it lead to the creation of a flashbulb memory.
Learn more about Flashbulb Memories here
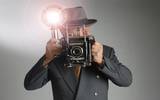
7 Memory and Smell
The link between memory and sense of smell helps many species - not just humans - to survive. The ability to remember and later recognize smells enables animals to detect the nearby presence of members of the same group, potential prey and predators. But how has this evolutionary advantage survived in modern-day humans?
Researchers at the University of North Carolina tested the olfactory effects on memory encoding and retrieval in a 1989 experiment. Male college students were shown a series of slides of pictures of females, whose attractiveness they were asked to rate on a scale. Whilst viewing the slides, the participants were exposed to pleasant odor of aftershave or an unpleasant smell. Their recollection of the faces in the slides was later tested in an environment containing either the same or a different scent. Read More
The results showed that participants were better able to recall memories when the scent at the time of encoding matched that at the time of recall (Cann and Ross, 1989). These findings suggest that a link between our sense of smell and memories remains, even if it provides less of a survival advantage than it did for our more primitive ancestors.
8 Interference
Interference theory postulates that we forget memories due to other memories interfering with our recall. Interference can be either retroactive or proactive: new information can interfere with older memories (retroactive interference), whilst information we already know can affect our ability to memorize new information (proactive interference).
Both types of interference are more likely to occur when two memories are semantically related, as demonstrated in a 1960 experiment in which two groups of participants were given a list of word pairs to remember, so that they could recall the second ‘response’ word when given the first as a stimulus. A second group was also given a list to learn, but afterwards was asked to memorize a second list of word pairs. When both groups were asked to recall the words from the first list, those who had just learnt that list were able to recall more words than the group that had learnt a second list (Underwood & Postman, 1960). This supported the concept of retroactive interference: the second list impacted upon memories of words from the first list. Read More
Interference also works in the opposite direction: existing memories sometimes inhibit our ability to memorize new information. This might occur when you receive a work schedule, for instance. When you are given a new schedule a few months later, you may find yourself adhering to the original times. The schedule that you already knew interferes with your memory of the new schedule.
9 False Memories
Can false memories be implanted in our minds? The idea may sound like the basis of a dystopian science fiction story, but evidence suggests that memories that we already hold can be manipulated long after their encoding. Moreover, we can even be coerced into believing invented accounts of events to be true, creating false memories that we then accept as our own.
Cognitive psychologist Elizabeth Loftus has spent much of her life researching the reliability of our memories; particularly in circumstances when their accuracy has wider consequences, such as the testimonials of eyewitness in criminal trials. Loftus found that the phrasing of questions used to extract accounts of events can lead witnesses to attest to events inaccurately. Read More
In one experiment, Loftus showed a group of participants a video of a car collision, where the vehicle was travelling at a one of a variety of speeds. She then asked them the car’s speed using a sentence whose depiction of the crash was adjusted from mild to severe using different verbs. Loftus found when the question suggested that the crash had been severe, participants disregarded their video observation and vouched that the car had been travelling faster than if the crash had been more of a gentle bump (Loftus and Palmer, 1974). The use of framed questions, as demonstrated by Loftus, can retroactively interfere with existing memories of events.
James Coan (1997) demonstrated that false memories can even be produced of entire events. He produced booklets detailing various childhood events and gave them to family members to read. The booklet given to his brother contained a false account of him being lost in a shopping mall, being found by an older man and then finding his family. When asked to recall the events, Coan’s brother believed the lost in a mall story to have actually occurred, and even embellished the account with his own details (Coan, 1997).
Read more about false memories here
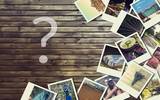
10 The Weapon Effect on Eyewitness Testimonies
(johnson & scott, 1976).
A person’s ability to memorize an event inevitably depends not just on rehearsal but also on the attention paid to it at the time it occurred. In a situation such as an bank robbery, you may have other things on your mind besides memorizing the appearance of the perpetrator. But witness’s ability to produce a testimony can sometimes be affected by whether or not a gun was involved in a crime. This phenomenon is known as the weapon effect - when a witness is involved in a situation in which a weapon is present, they have been found to remember details less accurately than a similar situation without a weapon. Read More
The weapon effect on eyewitness testimonies was the subject of a 1976 experiment in which participants situated in a waiting room watched as a man left a room carrying a pen in one hand. Another group of participants heard an aggressive argument, and then saw a man leave a room carrying a blood-stained knife.
Later, when asked to identify the man in a line-up, participants who saw the man carrying a weapon were less able to identify him than those who had seen the man carrying a pen (Johnson & Scott, 1976). Witnesses’ focus of attention had been distracted by a weapon, impeding their ability to remember other details of the event.
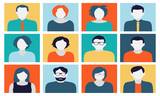
Which Archetype Are You?
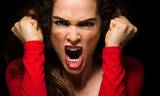
Are You Angry?
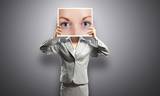
Windows to the Soul
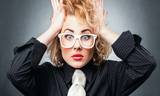
Are You Stressed?
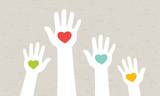
Attachment & Relationships
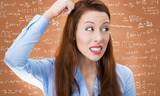
Memory Like A Goldfish?
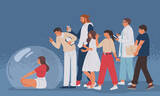
31 Defense Mechanisms
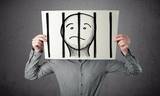
Slave To Your Role?
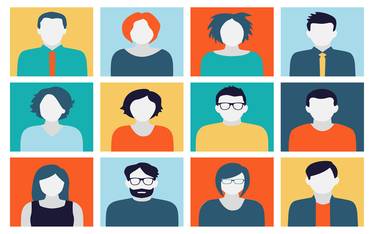
Are You Fixated?
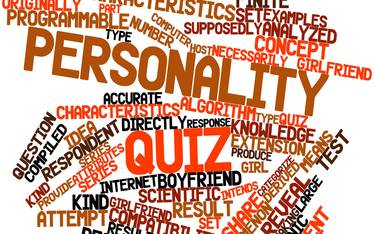
Interpret Your Dreams
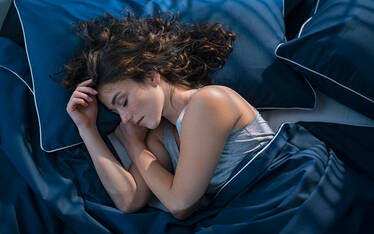
How to Read Body Language
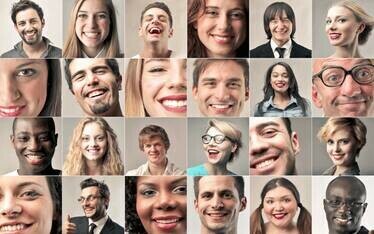
How to Beat Stress and Succeed in Exams
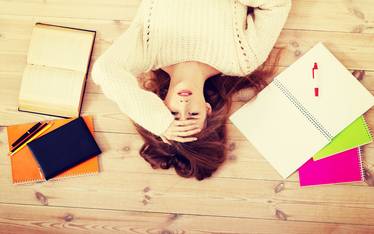
More on Memory Psychology
Test your short-term memory with this online feature.
Memory Test
False Memories
How false memories are created and can affect our ability to recall events.
Why Do We Forget?
Why do we forget information? Find out in this fascinating article exploring...
Conditioned Behavior
What is conditioning? What Pavlov's dogs experiment teaches us about how we...
Interrupt To Remember?
Explanation of the Zeigarnik effect, whereby interruption of a task can lead to...
Sign Up for Unlimited Access
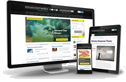
- Psychology approaches, theories and studies explained
- Body Language Reading Guide
- How to Interpret Your Dreams Guide
- Self Hypnosis Downloads
- Plus More Member Benefits
You May Also Like...
Dark sense of humor linked to intelligence, brainwashed, master body language, persuasion with ingratiation, psychology of color, making conversation, nap for performance, why do we dream, psychology guides.
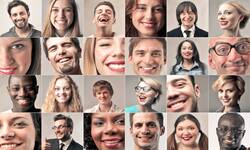
Learn Body Language Reading
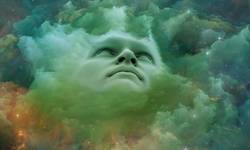
How To Interpret Your Dreams
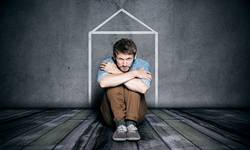
Overcome Your Fears and Phobias
Psychology topics, learn psychology.
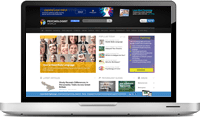
- Access 2,200+ insightful pages of psychology explanations & theories
- Insights into the way we think and behave
- Body Language & Dream Interpretation guides
- Self hypnosis MP3 downloads and more
- Behavioral Approach
- Eye Reading
- Stress Test
- Cognitive Approach
- Fight-or-Flight Response
- Neuroticism Test
© 2024 Psychologist World. Home About Contact Us Terms of Use Privacy & Cookies Hypnosis Scripts Sign Up
Working Memory Model (Baddeley and Hitch)
Saul Mcleod, PhD
Editor-in-Chief for Simply Psychology
BSc (Hons) Psychology, MRes, PhD, University of Manchester
Saul Mcleod, PhD., is a qualified psychology teacher with over 18 years of experience in further and higher education. He has been published in peer-reviewed journals, including the Journal of Clinical Psychology.
Learn about our Editorial Process
Olivia Guy-Evans, MSc
Associate Editor for Simply Psychology
BSc (Hons) Psychology, MSc Psychology of Education
Olivia Guy-Evans is a writer and associate editor for Simply Psychology. She has previously worked in healthcare and educational sectors.
On This Page:
The Working Memory Model, proposed by Baddeley and Hitch in 1974, describes short-term memory as a system with multiple components.
It comprises the central executive, which controls attention and coordinates the phonological loop (handling auditory information), and the visuospatial sketchpad (processing visual and spatial information).
Later, the episodic buffer was added to integrate information across these systems and link to long-term memory. This model suggests that short-term memory is dynamic and multifaceted.
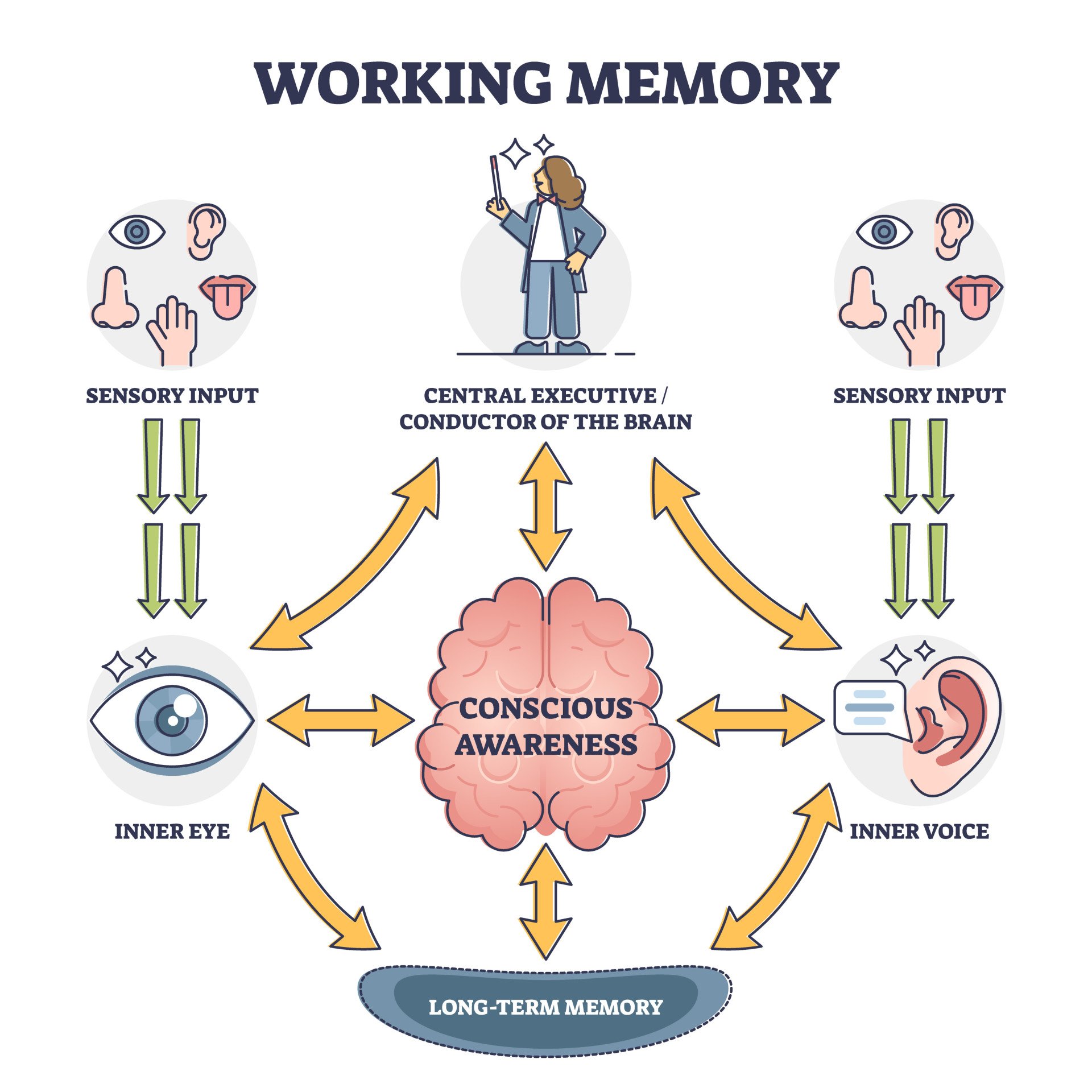
Take-home Messages
- Working memory is a limited capacity store for retaining information for a brief period while performing mental operations on that information.
- Working memory is a multi-component system that includes the central executive, visuospatial sketchpad, phonological loop, and episodic buffer.
- Working memory is important for reasoning, learning, and comprehension.
- Working memory theories assume that complex reasoning and learning tasks require a mental workspace to hold and manipulate information.
Atkinson’s and Shiffrin’s (1968) multi-store model was extremely successful in terms of the amount of research it generated. However, as a result of this research, it became apparent that there were a number of problems with their ideas concerning the characteristics of short-term memory.
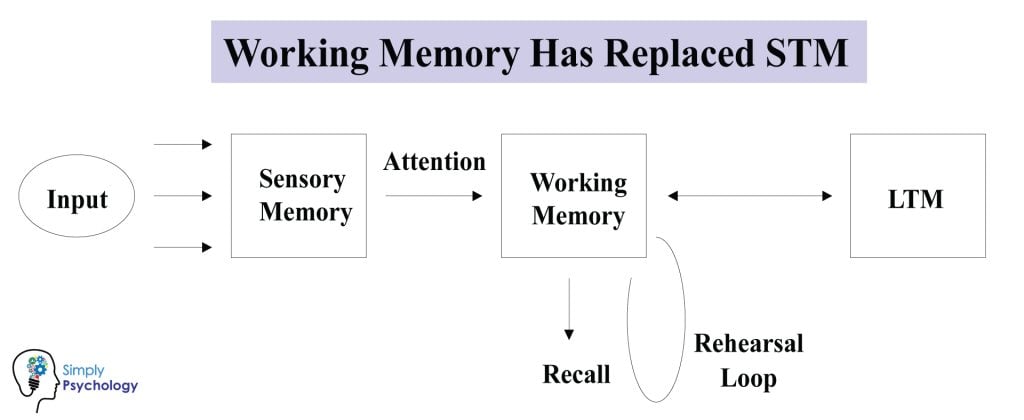
Fig 1 . The Working Memory Model (Baddeley and Hitch, 1974)
Baddeley and Hitch (1974) argue that the picture of short-term memory (STM) provided by the Multi-Store Model is far too simple.
According to the Multi-Store Model , STM holds limited amounts of information for short periods of time with relatively little processing. It is a unitary system. This means it is a single system (or store) without any subsystems. Whereas working memory is a multi-component system (auditory and visual).
Therefore, whereas short-term memory can only hold information, working memory can both retain and process information.
Working memory is short-term memory . However, instead of all information going into one single store, there are different systems for different types of information.
Central Executive
Visuospatial sketchpad (inner eye), phonological loop.
- Phonological Store (inner ear) processes speech perception and stores spoken words we hear for 1-2 seconds.
- Articulatory control process (inner voice) processes speech production, and rehearses and stores verbal information from the phonological store.
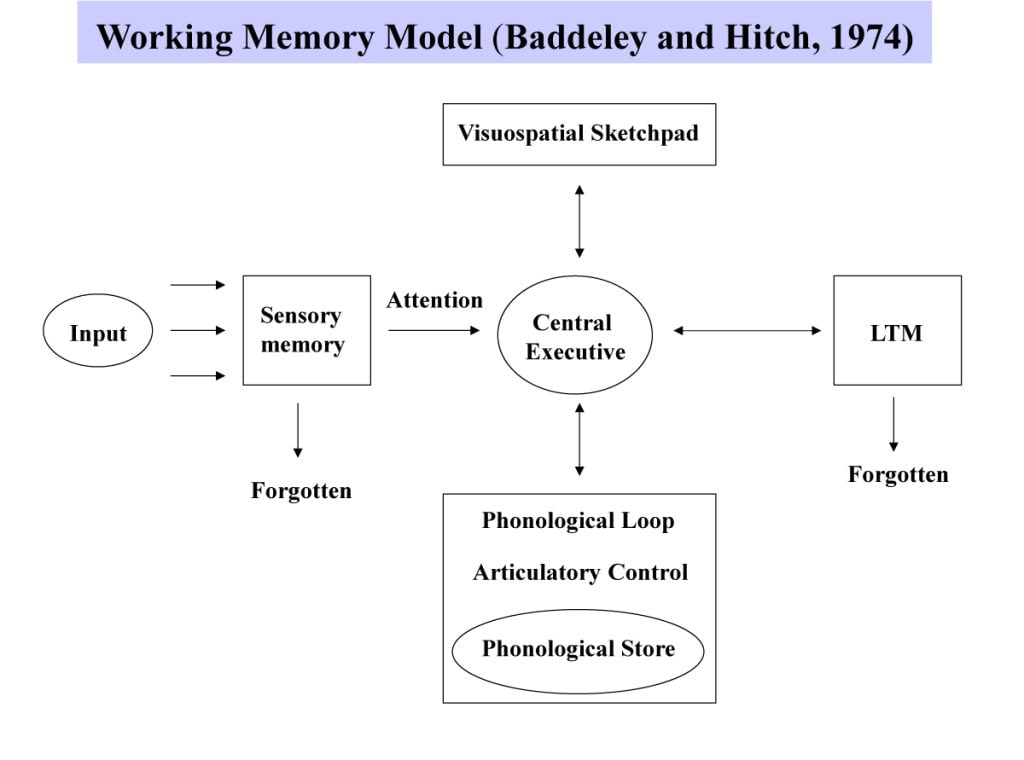
Fig 2 . The Working Memory Model Components (Baddeley and Hitch, 1974)
The labels given to the components (see Fig 2) of the working memory reflect their function and the type of information they process and manipulate.
The phonological loop is assumed to be responsible for the manipulation of speech-based information, whereas the visuospatial sketchpad is assumed to be responsible for manipulating visual images.
The model proposes that every component of working memory has a limited capacity, and also that the components are relatively independent of each other.
The Central Executive
The central executive is the most important component of the model, although little is known about how it functions. It is responsible for monitoring and coordinating the operation of the slave systems (i.e., visuospatial sketchpad and phonological loop) and relates them to long-term memory (LTM).
The central executive decides which information is attended to and which parts of the working memory to send that information to be dealt with. For example, two activities sometimes come into conflict, such as driving a car and talking.
Rather than hitting a cyclist who is wobbling all over the road, it is preferable to stop talking and concentrate on driving. The central executive directs attention and gives priority to particular activities.
p> The central executive is the most versatile and important component of the working memory system. However, despite its importance in the working-memory model, we know considerably less about this component than the two subsystems it controls.
Baddeley suggests that the central executive acts more like a system which controls attentional processes rather than as a memory store. This is unlike the phonological loop and the visuospatial sketchpad, which are specialized storage systems. The central executive enables the working memory system to selectively attend to some stimuli and ignore others.
Baddeley (1986) uses the metaphor of a company boss to describe the way in which the central executive operates. The company boss makes decisions about which issues deserve attention and which should be ignored.
They also select strategies for dealing with problems, but like any person in the company, the boss can only do a limited number of things at the same time. The boss of a company will collect information from a number of different sources.
If we continue applying this metaphor, then we can see the central executive in working memory integrating (i.e., combining) information from two assistants (the phonological loop and the visuospatial sketchpad) and also drawing on information held in a large database (long-term memory).
The Phonological Loop
The phonological loop is the part of working memory that deals with spoken and written material. It consists of two parts (see Figure 3).
The phonological store (linked to speech perception) acts as an inner ear and holds information in a speech-based form (i.e., spoken words) for 1-2 seconds. Spoken words enter the store directly. Written words must first be converted into an articulatory (spoken) code before they can enter the phonological store.
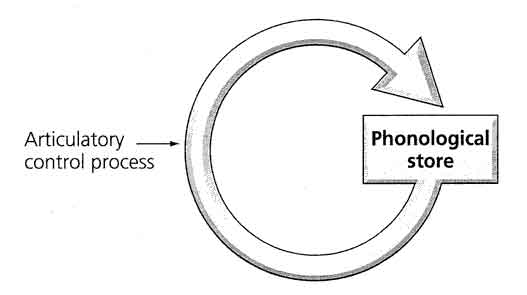
Fig 3 . The phonological loop
The articulatory control process (linked to speech production) acts like an inner voice rehearsing information from the phonological store. It circulates information round and round like a tape loop. This is how we remember a telephone number we have just heard. As long as we keep repeating it, we can retain the information in working memory.
The articulatory control process also converts written material into an articulatory code and transfers it to the phonological store.
The Visuospatial Sketchpad
The visuospatial sketchpad ( inner eye ) deals with visual and spatial information. Visual information refers to what things look like. It is likely that the visuospatial sketchpad plays an important role in helping us keep track of where we are in relation to other objects as we move through our environment (Baddeley, 1997).
As we move around, our position in relation to objects is constantly changing and it is important that we can update this information. For example, being aware of where we are in relation to desks, chairs and tables when we are walking around a classroom means that we don”t bump into things too often!
The sketchpad also displays and manipulates visual and spatial information held in long-term memory. For example, the spatial layout of your house is held in LTM. Try answering this question: How many windows are there in the front of your house?
You probably find yourself picturing the front of your house and counting the windows. An image has been retrieved from LTM and pictured on the sketchpad.
Evidence suggests that working memory uses two different systems for dealing with visual and verbal information. A visual processing task and a verbal processing task can be performed at the same time.
It is more difficult to perform two visual tasks at the same time because they interfere with each other and performance is reduced. The same applies to performing two verbal tasks at the same time. This supports the view that the phonological loop and the sketchpad are separate systems within working memory.
The Episodic Buffer
The original model was updated by Baddeley (2000) after the model failed to explain the results of various experiments. An additional component was added called the episodic buffer.
The episodic buffer acts as a “backup” store which communicates with both long-term memory and the components of working memory.
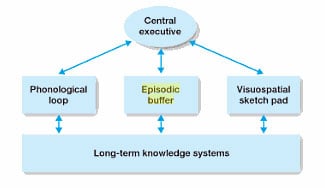
Fig 3 . Updated Model to include the Episodic Buffer
Critical Evaluation
Researchers today generally agree that short-term memory is made up of a number of components or subsystems. The working memory model has replaced the idea of a unitary (one part) STM as suggested by the multistore model.
The working memory model explains a lot more than the multistore model. It makes sense of a range of tasks – verbal reasoning, comprehension, reading, problem-solving and visual and spatial processing. The model is supported by considerable experimental evidence.
The working memory applies to real-life tasks:
- reading (phonological loop)
- problem-solving (central executive)
- navigation (visual and spatial processing)
The KF Case Study supports the Working Memory Model. KF suffered brain damage from a motorcycle accident that damaged his short-term memory.
KF’s impairment was mainly for verbal information – his memory for visual information was largely unaffected. This shows that there are separate STM components for visual information (VSS) and verbal information (phonological loop).
The working memory model does not over-emphasize the importance of rehearsal for STM retention, in contrast to the multi-store model.
Empirical Evidence for Working Memory
What evidence is there that working memory exists, that it comprises several parts, that perform different tasks? Working memory is supported by dual-task studies (Baddeley and Hitch, 1976).
The working memory model makes the following two predictions:
1 . If two tasks make use of the same component (of working memory), they cannot be performed successfully together. 2 . If two tasks make use of different components, it should be possible to perform them as well as together as separately.
Key Study: Baddeley and Hitch (1976)
Aim : To investigate if participants can use different parts of working memory at the same time.
Method : Conducted an experiment in which participants were asked to perform two tasks at the same time (dual task technique) – a digit span task which required them to repeat a list of numbers, and a verbal reasoning task which required them to answer true or false to various questions (e.g., B is followed by A?).
Results : As the number of digits increased in the digit span tasks, participants took longer to answer the reasoning questions, but not much longer – only fractions of a second. And, they didn”t make any more errors in the verbal reasoning tasks as the number of digits increased.
Conclusion : The verbal reasoning task made use of the central executive and the digit span task made use of the phonological loop.
Brain Imaging Studies
Several neuroimaging studies have attempted to identify distinct neural correlates for the phonological loop and visuospatial sketchpad posited by the multi-component model.
For example, some studies have found that tasks tapping phonological storage tend to activate more left-hemisphere perisylvian language areas, whereas visuospatial tasks activate more right posterior regions like the parietal cortex (Smith & Jonides, 1997).
However, the overall pattern of results remains complex and controversial. Meta-analyses often fail to show consistent localization of verbal and visuospatial working memory (Baddeley, 2012).
There is significant overlap in activation, which may reflect binding processes through the episodic buffer, as well as common executive demands.
Differences in paradigms and limitations of neuroimaging methodology further complicate mapping the components of working memory onto distinct brain regions or circuits (Henson, 2001).
While neuroscience offers insight into working memory, Baddeley (2012) argues that clear anatomical localization is unlikely given the distributed and interactive nature of working memory. Specifically, he suggests that each component likely comprises a complex neural circuit rather than a circumscribed brain area.
Additionally, working memory processes are closely interrelated with other systems for attention, perception and long-term memory . Thus, neuroimaging provides clues but has not yet offered definitive evidence to validate the separable storage components posited in the multi-component framework.
Further research using techniques with higher spatial and temporal resolution may help better delineate the neural basis of verbal and visuo-spatial working memory.
Lieberman (1980) criticizes the working memory model as the visuospatial sketchpad (VSS) implies that all spatial information was first visual (they are linked).
However, Lieberman points out that blind people have excellent spatial awareness, although they have never had any visual information. Lieberman argues that the VSS should be separated into two different components: one for visual information and one for spatial.
There is little direct evidence for how the central executive works and what it does. The capacity of the central executive has never been measured.
Working memory only involves STM, so it is not a comprehensive model of memory (as it does not include SM or LTM).
The working memory model does not explain changes in processing ability that occur as the result of practice or time.
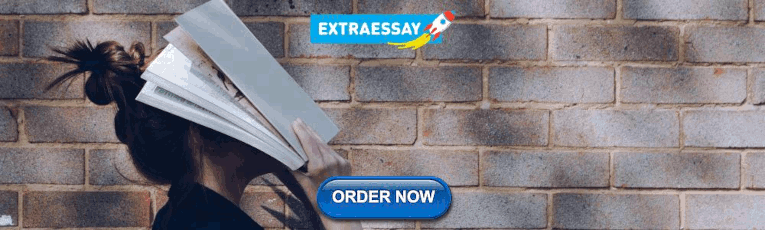
State-based models of WM
Early models of working memory proposed specialized storage systems, such as the phonological loop and visuospatial sketchpad, in Baddeley and Hitch’s (1974) influential multi-component model.
However, newer “state-based” models suggest working memory arises from temporarily activating representations that already exist in your brain’s long-term memory or perceptual systems.
For example, you activate your memory of number concepts to remember a phone number. Or, to remember where your keys are, you activate your mental map of the room.
According to state-based models, you hold information in mind by directing your attention to these internal representations. This gives them a temporary “boost” of activity.
More recent state-based models argue against dedicated buffers, and propose that working memory relies on temporarily activating long-term memory representations through attention (Cowan, 1995; Oberauer, 2002) or recruiting perceptual and motor systems (Postle, 2006; D’Esposito, 2007).
Evidence from multivariate pattern analysis (MVPA) of fMRI data largely supports state-based models, rather than dedicated storage buffers.
For example, Lewis-Peacock and Postle (2008) showed MVPA classifiers could decode information being held in working memory from patterns of activity associated with long-term memory for that content.
Other studies have shown stimulus-specific patterns of activity in sensory cortices support the retention of perceptual information (Harrison & Tong, 2009; Serences et al., 2009).
Atkinson, R. C., & Shiffrin, R. M. (1968). Chapter: Human memory: A proposed system and its control processes. In Spence, K. W., & Spence, J. T. The psychology of learning and motivation (Volume 2). New York: Academic Press. pp. 89–195.
Baddeley, A. D. (1986). Working memory . Oxford: Oxford University Press.
Baddeley, A. (1996). Exploring the central executive. The Quarterly Journal of Experimental Psychology Section A , 49 (1), 5-28.
Baddeley, A. D. (2000). The episodic buffer: A new component of working memory? Trends in Cognitive Sciences , 4, (11): 417-423.
Baddeley, A. (2010). Working memory. Current biology , 20 (4), R136-R140.
Baddeley, A. (2012). Working memory: Theories, models, and controversies. Annual review of psychology , 63 , 1-29.
Baddeley, A. D., & Hitch, G. (1974). Working memory. In G.H. Bower (Ed.), The psychology of learning and motivation: Advances in research and theory (Vol. 8, pp. 47–89). New York: Academic Press.
Baddeley, A. D., & Lieberman, K. (1980). Spatial working memory. ln R. Nickerson. Attention and Performance, VIII . Hillsdale, N): Erlbaum.
Borella, E., Carretti, B., Sciore, R., Capotosto, E., Taconnat, L., Cornoldi, C., & De Beni, R. (2017). Training working memory in older adults: Is there an advantage of using strategies?. Psychology and Aging , 32 (2), 178.
Chai, W. J., Abd Hamid, A. I., & Abdullah, J. M. (2018). Working memory from the psychological and neurosciences perspectives: a review. Frontiers in Psychology , 9 , 401.
Cowan, N. (1995). Attention and memory: An integrated framework . Oxford Psychology Series, No. 26. New York: Oxford University Press.
Cowan, N. (2005). Working memory capacity. Exp. Psychology, 54, 245–246.
Cowan, N. (2008). What are the differences between long-term, short-term, and working memory?. Progress in brain research , 169 , 323-338.
Curtis, C.E., & D’Esposito, M. (2003). Persistent activity in the prefrontal cortex during working memory. Trends in Cognitive Sciences, 7 (9), 415-423.
D’Esposito, M. (2007). From cognitive to neural models of working memory. Philosophical Transactions of the Royal Society B: Biological Sciences, 362 (1481), 761-772.
D’Esposito, M., & Postle, B. R. (2015). The cognitive neuroscience of working memory. Annual review of psychology , 66 , 115-142.
Fell, J., & Axmacher, N. (2011). The role of phase synchronization in memory processes. Nature Reviews Neuroscience, 12 (2), 105-118.
Harrison, S. A., & Tong, F. (2009). Decoding reveals the contents of visual working memory in early visual areas. Nature, 458 (7238), 632-635.
Henson, R. (2001). Neural working memory. In J. Andrade (Ed.), Working memory in perspective (pp. 151-174). Psychology Press.
Lewis-Peacock, J. A., & Postle, B. R. (2008). Temporary activation of long-term memory supports working memory. Journal of Neuroscience, 28 (35), 8765-8771.
Lieberman, M. D. (2000). Introversion and working memory: Central executive differences. Personality and Individual Differences , 28 (3), 479-486.
Osaka, M., Osaka, N., Kondo, H., Morishita, M., Fukuyama, H., Aso, T., & Shibasaki, H. (2003). The neural basis of individual differences in working memory capacity: an fMRI study. NeuroImage , 18 (3), 789-797.
Serences, J.T., Ester, E.F., Vogel, E.K., & Awh, E. (2009). Stimulus-specific delay activity in human primary visual cortex. Psychological Science, 20( 2), 207-214.
Smith, E.E., & Jonides, J. (1997). Working memory: A view from neuroimaging. Cognitive Psychology, 33 (1), 5-42.

Thank you for visiting nature.com. You are using a browser version with limited support for CSS. To obtain the best experience, we recommend you use a more up to date browser (or turn off compatibility mode in Internet Explorer). In the meantime, to ensure continued support, we are displaying the site without styles and JavaScript.
- View all journals
- My Account Login
- Explore content
- About the journal
- Publish with us
- Sign up for alerts
- Open access
- Published: 18 February 2023
Dynamics of working memory process revealed by independent component analysis in an fMRI study
- Magdalena Fafrowicz 1 ,
- Anna Ceglarek 1 ,
- Justyna Olszewska 2 ,
- Anna Sobczak 1 ,
- Bartosz Bohaterewicz 3 ,
- Monika Ostrogorska 4 ,
- Patricia Reuter-Lorenz 5 ,
- Koryna Lewandowska 1 ,
- Barbara Sikora-Wachowicz 1 ,
- Halszka Oginska 1 ,
- Magdalena Hubalewska-Mazgaj 6 &
- Tadeusz Marek 1
Scientific Reports volume 13 , Article number: 2900 ( 2023 ) Cite this article
2592 Accesses
3 Citations
2 Altmetric
Metrics details
- Cognitive neuroscience
- Learning and memory
- Neuroscience
Human memory is prone to errors in many everyday activities but also when cultivating hobbies such as traveling and/or learning a new language. For instance, while visiting foreign countries, people erroneously recall foreign language words that are meaningless to them. Our research simulated such errors in a modified Deese-Roediger-McDermott paradigm for short-term memory with phonologically related stimuli aimed at uncovering behavioral and neuronal indices of false memory formation with regard to time-of-day, a variable known to influence memory. Fifty-eight participants were tested in a magnetic resonance (MR) scanner twice. The results of an Independent Component Analysis revealed encoding-related activity of the medial visual network preceding correct recognition of positive probes and correct rejection of lure probes. The engagement of this network preceding false alarms was not observed. We also explored if diurnal rhythmicity influences working memory processes. Diurnal differences were seen in the default mode network and the medial visual network with lower deactivation in the evening hours. The GLM results showed greater activation of the right lingual gyrus, part of the visual cortex and the left cerebellum in the evening. The study offers new insight into the mechanisms associated with false memories, suggesting that deficient engagement of the medial visual network during the memorization phase of a task results in short-term memory distortions. The results shed new light on the dynamics of working memory processes by taking into account the effect of time-of-day on memory performance.
Similar content being viewed by others
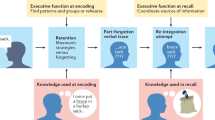
The roles of attention, executive function and knowledge in cognitive ageing of working memory
Moshe Naveh-Benjamin & Nelson Cowan
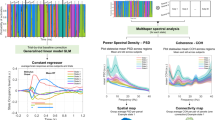
A data-driven network decomposition of the temporal, spatial, and spectral dynamics underpinning visual-verbal working memory processes
Chiara Rossi, Diego Vidaurre, … Jeroen Van Schependom
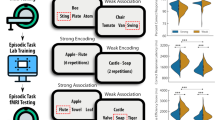
Varying demands for cognitive control reveals shared neural processes supporting semantic and episodic memory retrieval
Deniz Vatansever, Jonathan Smallwood & Elizabeth Jefferies
Introduction
In new language learners, errors that include the incorrect recall of foreign words are common. For example, in the Esperanto language, it is easy to confuse the words antidotoj (antidotes) and anekdotoj (anecdotes). The short-term memory task we use here resembles situations in a foreign country where we try to recall words that are foreign and thus relatively meaningless. These “pseudo” words are useful and important, for example, street or station names during sightseeing, but we fail by uttering phonetically similar words. We were interested in identifying neuronal activity associated with the performance of memory distortions that resemble the aforementioned mistakes and examining potential of time-of-day effects on these mechanisms. We use a modified version of the Deese-Roediger-McDermott (DRM) paradigm 1 , 2 and time-of-day approach to investigate this problem.
The concept of working memory has figured prominently in the study of cognition over the last 50 years. Working memory is responsible for the temporary retention and management of information required for a range of higher cognitive abilities such as problem-solving, comprehension, and reasoning 3 , 4 , 5 . Overall, working memory is thought to be important for goal-directed behaviors, which require maintenance, selection, and sequencing functions 6 , 7 . The terms “working memory” and “short-term memory” are becoming synonymous to some extent 8 with working memory seen as a "multi-component system that holds and manipulates information in short-term memory" 9 . Such a view is adopted in this work. The Multicomponent Working Memory Model proposed by Baddeley and Hitch (1974) 10 is one of the most influential early models and has been widely used to describe working memory processes 11 , 12 , 13 , 14 . According to this model, the central executive coordinates information stored in various independent “slave” subsystems, most notably, the visuospatial sketchpad and the phonological loop. The former is responsible for retaining and processing information in a visual and spatial form, and is typically used for navigation and visuospatial reasoning. The phonological loop consists of two distinct parts—the phonological store and the articulatory control process—that actively maintain spoken and written verbal material. Evidence suggests this subsystem also contributes to acquiring new words during the process of foreign language learning through the engagement of phonological short-term memory 15 , 16 . A conventional way to evaluate the above subsystem memory is the pseudoword repetition test, consisting of words which do not exist in a given language, however are phonologically similar to the ones that do.
Many experimental paradigms have been used to study human memory and its failings. One of the most popular is Deese-Roediger-McDermott (DRM) paradigm (for the review, see: REF 17 ), used for studying memory distortions, because of its simplicity, controllability, and flexibility 18 . In this paradigm, participants study a list of related words, each of which converges on a non-studied critical lure. When tested, participants often recall and/or recognize the critical lure as having been presented, resulting in a form of memory distortion 1 , 2 , 19 , 20 , 21 , 22 . In DRM adjusted to study short-term memory (ST-DRM), the delay between encoding and recall/recognition lasts for several seconds 23 , 24 . This variant of the DRM task allows to effectively separate the encoding (presentation of the memory set) and retrieval processes (remembering whether or not the recognition probe was presented in the preceding memory set). While most DRM tasks focus on the distorting effects of semantic similarity, memory distortions due to phonological similarity (based on surface/structural content) are also investigated 17 , 18 . In a review by Chang and Brainerd (2021) 18 , the authors showed that there are differences between semantic and phonological distortions examined using DRM paradigm under both short-term and long-term memory conditions as well as due to the test modality (auditory versus visually presented items). McBride et al. (2019) 25 demonstrated that false memories are stronger for phonological than for semantic lists in short-term memory. Regarding the stimulus modality, Olszewska et al. (2015) 26 showed that phonological illusions were lower when the list was presented visually.
While numerous neurocognitive models of working memory have been proposed 27 , the recent dynamic-processing model of working memory endeavors to account for a differing sets of brain-based findings that have been difficult to reconcile with any single model 28 . The dynamic-processing model proposes that the relevant information is retained based on dynamically-coded representations and maintenance processes that vary according to the task demands (e.g. interference), task rules, and behavioral goals. Likewise, the associated neural substrates vary dynamically according to the representational and processing demands of the tasks and associated behavioral goals 29 .
Various brain imaging studies have established the neural bases of human working memory and documented the critical involvement of the fronto-parietal network in these process 14 , 30 , 31 . Logie et al. (2003) 32 showed brain activation in the inferior parietal gyrus as well as the left middle frontal gyrus while processing phonological material in working memory. In the work utilizing laminar functional MRI (lfMRI), Sharoh and collegues (2019) 33 revealed unique connectivity patterns during visually presented both words and pseudowords reading. The difference was seen in the depth-dependent BOLD signals of the left occipitotemporal sulcus in a way that to words responded deep parts of this structure and to pseudowords—superficial and middle parts. The majority of studies in the magnetic resonance (MR) environment aimed to investigate the distortions of long-term memory (for the reviews, see: REF 34 , 35 ). Several regions such as the medial superior frontal gyrus, left precentral gyrus as well as left inferior parietal cortex were related with false memory retrieval, regardless of the type of material. Regarding short-term false memory, activity of the dorsolateral prefrontal cortex was revealed during correct rejection of related lures in semantic material while true recognition was associated with the activation in the left fusiform gyrus 26 as well as in figural material demonstrating hippocampal areas activations related to fewer correct rejections of lures 36 and higher false recognition-related activity of prefrontal and visual cortices in young adults 37 . Many neuroimaging studies investigated the formation of true and false memories focusing on the sensory reactivation theory 38 , however recent evidence strongly transforming this concept, showing that memory representations differ from perceptual representations and pointing out memory retrieval as constructive not reproductive act 39 .
Previous resting-state fMRI studies revealed that brain functional architecture also changes dynamically according to the time-of-day 40 . Accordingly, the other aim of the present study is to determine if there are diurnal changes in the dynamic of working memory processes. Many studies revealed the time-of-day effects on cognition, in which the optimal time for the performance of a certain task was evaluated (for the reviews, see: Ref 41 , 42 ). Three experimental procedures—constant routine, forced desynchrony, and time-of-day protocols—are essentially used for the investigation of daily fluctuations of cognitive processes 41 , 43 . When constant routine and forced desynchrony procedures are performed in the strictly controlled, laboratory environment, in the time-of-day paradigm participants (examined at optimal or/and nonoptimal time-of-day) between experimental sessions carry out their routine activity. One of the experimental designs here is the chronotype-based paradigm, which allows investigating over the normal working day the temporal fluctuations of performance related to individual’s circadian preference (chronotype). In addition, we considered the genetic factors, which have been shown to contribute both diurnal preference and homeostatic regulation of sleep. There is some evidence that polymorphism of PER3 gene is associated with individual differences in circadian and sleep phenotypes. The PER3 4-repeat allele has been linked with “eveningess”, whereas PER3 5-repeat allele with “morningness” and greater homeostatic sleep pressure (e.g. 44 , 45 , 46 ). More information regarding the chronotypes-based paradigm used in our research is presented in the Supplementary Information. Although several studies indicated a diurnal variation in the performance of working memory tasks 36 , 47 , 48 , 49 , the results of these studies are not always consistent and it is still an underexplored area of research. From the neuroimaging perspective, the studies revealed differences in brain activation according to the time-of-day. Schmidt et al. (2015) 48 showed higher thalamic activity in the morning and decreased activity in the ventrolateral prefrontal cortex and premotor areas from the morning to the evening hours using the n-back paradigm. Singh et al. (2022) 50 demonstrated higher brain activations in the morning session during working memory task performance.
The literature reviewed showed that most fMRI results related to both short-term and long-term false memory were obtained with use of the general linear model (GLM). Yet, exploratory approaches, such as the Independent Component Analysis (ICA), may shed light on the underexplored aspects of diurnal cognitive variability. ICA is a data-driven approach, which aims for decomposition of a multivariate signal into additive subcomponents 51 . The method is considered a special case of blind source separation that resembles the cocktail party effect where one sound is isolated from the noise 52 . To the best of our knowledge, neither brain imaging nor an exploratory analytic approach like ICA has been used to investigate time-of-day effects on short-term memory processes.
Taken together, the present study was designed to investigate the neural mechanisms underlying the generation of distortions in working memory and to evaluate if the time-of-day influences these mechanisms using the DRM paradigm, together with a time-of-day protocol, and the ICA approach to the fMRI data. We expect that the obtained results will offer new insights into the dynamics of working memory processes. These results may also be interesting in the context of learning new languages to the extent such processes are similar to the memorization of a random group of syllables.
Materials and methods
Participants.
Online advertisement on the Jagiellonian University website and Facebook was the primary recruitment method for the study. A total of the 5354 young, healthy volunteers participated in the first stage of selection and completed assessment of diurnal preference as measured by the Chronotype Questionnaire 53 , night sleep quality as measured by the Pittsburgh Sleep Quality Index (PSQI 54 ), and daytime sleepiness as measured by the Epworth Sleepiness Scale (ESS 55 ). Four hundred fifty-one participants identified as morning or evening chronotypes reporting no excessive daytime sleepiness (ESS score ≤ 10 points) and good sleep quality (PSQI score ≤ 5 points) went through the next stage of selection. The selection criteria for chronotypes were: above 15 points in amplitude scale from Chronotype Questionnaire and between 8 and 19 for morning types and between 26 and 32 for evening types in morningness/eveningness scale from Chronotype Questionnaire.
In the next stage of selection all participants underwent genotyping to identify PER3 VNTR polymorphisms affecting circadian typology and sleep homeostatic drive (for the review, see: Ref 46 ). To perform genotyping, mouth swabs were collected from all of the participants. More information about this procedure is presented in the Supplementary Information. Only individuals who were homozygous for the PER3 4- alleles (evening chronotypes) and PER3 5-alleles (morning chronotypes) were included in the study. Further exclusion criteria included dyslexia (four participants eliminated), drug, caffeine, alcohol or nicotine dependence (three participants excluded), shift work (one person excluded), and having been on a flight passing more than two time zones within the past 2 months (one participant eliminated). The total sample for the fMRI study consisted of 66 young, healthy native Polish speakers who reported no dyslexic symptoms and fulfilled the selection criteria: age between 20 and 35 years, right-handedness according to the Edinburgh Handedness Inventory (EHI 56 ), normal or corrected-to-normal vision, regular time-of-day schedule without sleep debt, no neurological or psychiatric disorders, and no MRI contraindications. Informed, written consent was provided by all participants prior to completion of the study procedures. The sleep–wake schedule of participants was monitored using the MotionWatch 8 actigraphy during the week preceding the study as well as the days of brain imaging. Imaging data from eight participants were excluded, three due to the excessive movements, defined as exceeding a 4° rotation or 4 mm translation on any axis and five due to the lack of errors in at least one condition of the task. Hence, 58 participants (37 females; mean age = 24.4, SD = 3.68) divided into two groups: 29 morning types (MT) and 29 evening types (ET) were included in the analyses (see above for the selection criteria). The participants were remunerated for participation in the experiment. The study was conducted in accordance with the Declaration of Helsinki and approved by the Research Ethics Committee at the Institute of Applied Psychology at the Jagiellonian University. Demographics, questionnaires and actigraphy results are provided in Table 1 .
The experimental task was based on the modified short-term memory DRM paradigm 23 , 24 using pseudowords as memoranda, with a distractor task filling the brief retention interval.
Memory items: 550 pseudowords—pronounceable meaningless letter strings—containing Polish syllables were used to create memory sets characterized by phonological (and visual) similarity. All stimuli in one set were made up of similar Polish morphemes and started or ended with the same syllable. The number of characters in the stimuli varied from 5 to 9 for two syllables pseudowords (mean length 6.11), from 5 to 10 for three syllables pseudowords (mean length 7.68), and from 7 to 11 for four syllables pseudowords (mean length 8.65).
Distractor items: 120 concrete Polish adjectives and nouns served as distractors occurring between memory sets and probes (see Fig. 1 ). The number of characters in two-syllable words varied from 5 to 9 (mean length 6.5), in the three-syllable words from 5 to 12 (mean length 8.02), and in the four-syllable words from 8 to 11 (mean length 9.75).
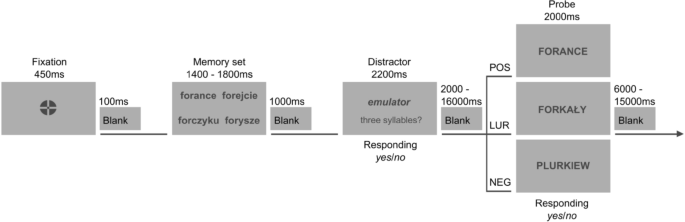
The sequence of stimuli in the task with an example set of pseudowords and distractor. POS positive probe, LUR lure probe, NEG negative probe.
All stimuli (memory sets, distractors and memory probes) were presented in Calibri 22-point font via a mirror on an MR-compatible LCD screen (NordicNeuroLab, Bergen, Norway) with a refresh rate of 60 Hz and a resolution of 800 × 600 pixels. Each item in the memory set appeared at one of four possible locations at 11° and 18° of visual angle in x-axis and 7° and 9° in y-axis, whereas the distractor and memory probe were presented at the center of the screen. The colors used were dark gray (RGB 72, 72, 72) in the foreground and light gray (RGB 176, 176, 176) in the background.
Two equivalent versions (version A and version B) of the experimental task were used, each consisting of 60 trials and differing only in terms of stimuli sets and distracting words. In total there were 120 memory sets consisting of four pseudowords (two, three, or four syllables) each and 120 distractors consisting of two, three, or four syllable words. As distractors we used low frequency words in Polish language based on the dictionary by Kazojc (2011) 57 .
Each participant performed the task twice—during morning (MS) and evening (ES) sessions in a 3 T Siemens Magnetom Skyra MRI scanner. Counterbalancing took place allowing all four possible pairing between the two task versions (A and B) and the two sessions (MS and ES) across participants, in a manner that half of participants performed version A in the morning and version B in the evening, whereas the opposite was true for the other half of participants. The possibility of the practice effect was rejected, no differences were found in both the error ratio and the reaction times between the first session held for each participant (MS or ES) and the second. Each experimental trial began with a fixation point for 450 ms and was followed by a blank screen presented for 100 ms. A set of four pseudowords was presented simultaneously for 1400, 1600 or 1800 ms (encoding phase), depending on the number of syllables (two, three or four syllables, respectively). 1000 ms followed offset of the memory set, then a distractor item (word) appeared at the center of the screen for 2200 ms. An interval of 2000–16,000 ms (mean duration 6097 ms) followed the distractor task, and then a single stimulus/pseudoword served as the memory probe, which appeared for 2000 ms (retrieval phase). The memory probe was of three possible types: positive probe (a studied item from the immediately preceding list), lure (a pseudoword phonologically similar to the items studied in the immediately preceding list) and a negative probe (a new pseudoword, unrelated to those previously studied in the immediately preceding list). For each set, the lure was selected on the basis of a pilot study (a stimulus that was the closest one to the average of a whole set) and the negative probe-based on specific criteria (number of syllables and stimuli beginnings/ends). Mean duration of the inter-trial interval was 8403 ms (range: 6000–15,000 ms). The task consisted of 60 sets of stimuli followed by 25 positive probes, 25 lures, and ten negative probes. The number of sets in each version of task was as follows: two and four syllables—15 sets each; three syllables—30 sets. The number of distractors in each task: two- and four-syllables words—6 each; three-syllables words—48. The experimental task lasted 22 min. Figure 1 shows the sequence of events in the task.
Participants were instructed to memorize each set of four stimuli in order to recognize the presented pseudoword a few seconds later. During the distractor task, participants were asked to indicate whether or not a word consisted of three syllables. In response to the memory probe participants indicated whether they remember that the item appeared in the directly preceding set. For both the distractor task and the memory task, participants responded by pressing a button in their right hand for ‘yes’ responses and a button in their left hand for ‘no’ responses.
Within each version of the experiment, there were six lists created in a manner such that each stimulus could be followed by each probe type (e.g. in each list, out of 60 sets presented to a participant, ten different probes were followed by a negative probe). The lists were counterbalanced across participants. Within each list the order of stimuli was randomized but the order of probe types (positive, lure or negative), inter-stimulus intervals and inter-trial interval was fixed and the same for each participant (using the Optseq, FS-Fast analysis tools by D. Greve, Charlestown, MA). During the task, a single memory set could appear only once. The tasks were prepared using E-Prime 2.0 (Psychology Software Tools, Pittsburgh, PA, USA).
Participants completed the study in three visits: a training session, a morning session, and an evening one. During the training session—started at 5 PM one day before the first experimental session—the participants were extensively trained on the experimental procedure and types of stimuli to avoid the influence of a learning process on their performance. The training session consisted of three parts. In the first each participant was informed about the course of the experiment. Next sample trials were used to familiarize the participant with the experimental task procedure and trial types. Six experimental trials (2 positive probes, 2 lure probes, and 2 negative ones) were presented to the participant. There was no time limit to familiarize with each trial component—participant pressed a key to proceed to the next part of the trial. The subject could familiarize with trial components as many times as he/she needed. In the third part of the training session a whole-task training approach was used. The participants responded to both the probe and distractor by pressing a key with the right hand (response ‘yes’) or left hand (response ‘no'). Stimuli for training differed to those used for the experimental tasks. The tasks were presented on a HP Compaq laptop with a 15.4-in LCD monitor, with the screen resolution set at 1280 × 768 pixels and a screen refresh rate of 60 Hz. The presentation and data recording were controlled with E-prime 2.0 software (Psychology Software Tools, Pittsburgh, PA, USA). A research assistant who accompanied the participants during the training clarified any questions about the task. The participant could complete the task as many times as he/she needed. No feedback for correct or incorrect response was given, but the research assistant who assisted the participant during the training session encouraged him/her to practice the task until they felt well-trained and comfortable with the task. Participants abstained from alcohol (48 h) and caffeine (24 h) before each fMRI session and on the experimental days. During experimental days participants could engage in non-strenuous activities. The night before the morning session, they slept in rooms located in the building of the MR laboratory. The tasks were performed by morning-type participants between 09:49 AM and 10:11 AM ( SD: 1 h 08 min) in the morning and between 18:44 PM and 19:06 PM ( SD: 1 h 12 min) in the evening. Evening-type participants performed the tasks between 11:09 AM and 11:31 AM ( SD: 1 h 24 min) in the morning and between 20:17 PM and 20:39 PM ( SD: 1 h 25 min) in the evening.
fMRI data acquisition
MRI data was acquired using a 3 T Siemens Skyra MR System with a 64-channel coil. For anatomical reference, a T1-weighted MPRAGE sequence was performed (TR = 2.3 s, TE = 2.98 ms, FA = 9°, 176 sagittal slices, slice thickness = 1.1 mm, FOV = 256 × 256 mm). For the BOLD imaging, a T2*—weighted EPI sequence was used (TR = 1.8 s, TE = 27 ms, FA = 75°, 34 slices with interleaved acquisition, voxel size = 4 × 4 × 4 mm, slice thickness = 4 mm, inter-slice gap = 0 mm, FOV = 256 × 256 mm). The 736 volumes were acquired during task performance. Participants’ eye movements were monitored using an eye tracking system (Eyelink 1000, SR research, Mississauga, ON, Canada).
Behavioral data analysis
Statistical analyses were performed using Statistica 6.0 software (StatSoft, Inc., 2001) and R software version 4.0.0 (R Core Team, 2017). Behavioral analyses focused on hits ( yes responses to positive probes) and false alarms ( yes responses to critical lures and yes responses to negative probes) as well as on reaction times (RTs) to: hits, misses, correctly rejected critical lures and negative probes, and false alarms for critical lures and negative probes.
We performed signal detection analyses using dʹ as an estimate of sensitivity 58 . Following other studies 59 , 60 , 61 , 62 two measures of sensitivity were computed using the individual participants’ data: (1) estimates of sensitivity comparing hits ( yes responses to studied probes) to false alarms ( yes responses to critical lures), which reflects item-specific memory and (2) estimates of sensitivity where false alarms were treated as hits ( yes responses to critical lures) and were compared to unrelated probes treated as false alarms ( yes responses to unrelated probes), which reflects gist-based memory. Each of these indices of sensitivity was treated as dependent variables in separate analyses of variance (ANOVAs), with chronotype (MT vs. ET) as a between-subjects factor and time-of-day (morning vs. evening) as a within-subjects factor.
fMRI data analysis
Preprocessing.
Data preprocessing was performed using the Statistical Parametric Mapping software package (SPM12, Welcome Department of Imaging Neuroscience, UCL, London, UK; www.fil.ion.ucl.ac.uk/spm/ ) and DPABI (V4.2) implemented on MATLAB (Mathworks, Inc., MA, USA). Scans were slice-timed corrected and realigned by inclusion of field maps. Following motion correction, each individual’s structural T1-weighted image was co-registered and spatially normalized to Montreal Neurological Institute (MNI) space, as well as resampled at 3 mm 3 using B-Spline Interpolation. The normalized volumes were smoothed using 6-mm FWHM Gaussian kernel to increase the signal-to-noise ratio of the data. Then the signal from white matter and cerebrospinal fluid was regressed out and the band-pass filtering (0.01–0.08 Hz) was applied.
Preprocessed images were analyzed with the Group ICA of fMRI Toolbox (GIFT) software package (v4.0b; Medical Image Analysis Lab, University of New Mexico; http://icatb.sourceforge.net/groupica.html ). Spatial ICA was performed for two sessions and the spatial maps, as well as associated time courses, were produced separately for morning and evening sessions. The optimal number of components was estimated using the Minimum Description Length (MDL) criteria in the GIFT software package. A two-step principal component analysis (PCA) was executed to lower the imaging data dimensionality. Independent component estimation was performed using the Infomax algorithm and repeated 50 times in ICASSO ( http://research.ics.tkk.fi/ica/icasso ) to assess the consistency of the components. For each independent component (IC), the ”centroid” (i.e. the most stable result) was determined following the agglomerative hierarchical clustering with average-linkage criterion and its consistency was calculated with a cluster quality index (Iq) ranging from 0 to 1. Subject-specific spatial maps and associated time courses were back-reconstructed using the GICA3 method provided in the GIFT software package. To identify non-artifactual ICs, the visual inspection of IC spatial patterns as well as frequency inspection of time courses was performed, based on the prior 63 , 64 , 65 . Then the time courses of thirteen selected intrinsic connectivity networks (ICNs) were regressed with the design matrix (see GLM section) created with regressors from the task (onsets of probes—hits, misses, false alarms, correct rejection of lure and negative probes for encoding and retrieval phases) to establish which networks were engaged in the task. The regression step was done using ‘mvregress’ function in Matlab environment. Next, the beta values were averaged for every ICN across the subjects and modelled separately for encoding and retrieval phases. Positive beta values meant the activation of a given network, while negative ones—its deactivation. The one-sample t -test on mean beta values for MS and ES was performed to see which networks were engaged in the task with false discovery rate (FDR) correction for multiple comparisons at p < 0.05 level using the Benjamini–Hochberg procedure. The paired t -tests for MS and ES were conducted for every ICN to define the differences in activation/deactivation of ICNs in distinct time-of-day with FDR correction for multiple comparisons at p < 0.05 level using the Benjamini–Hochberg procedure.
Additionally, the preprocessed data were analyzed using the General Linear Model (GLM) implemented on SPM12. In the first level of statistical analyses, the conditions were defined by their stimulus onsets, for each subject and session the design matrix was created with regressors from the task (hits, correct rejection of lure and negative probes, erroneous responses for positive (misses) and lure (false alarms) probes for encoding and retrieval phase and “others” regressor which contain individual erroneous responses for negative probe and events without the response and the realignment parameters as the regressors of no interest. The regressors were modeled by a boxcar function (locked to the onset of the memory set in the encoding phase and memory probe in the retrieval phase with duration of 2000 ms) convolved with a hemodynamic response function and contrasted against each other, which resulted in eight contrasts for each subject and session (hits > false alarms, false alarms > hits, correct rejection of lures > false alarms and false alarms > correct rejection of lures at encoding and retrieval). The negative probe was treated as a control condition due to the small number used in the task (10) and was not included in contrasts. In the second level of analysis (group-level), the paired t-tests using the above-mentioned contrasts for MS vs. ES were conducted to ascertain the differences in activations of brain structures in distinct time-of-day. The results are presented at cluster-wise p < 0.05 level with FDR correction for multiple comparisons and a cluster size of at least 20 voxels. Anatomical labeling of significantly activated clusters was performed using WFU Pickatlas software, extension to SPM12 (Functional MRI laboratory—Wake Forest University School of Medicine, Winston Salem).
Ethics statement
The study was conducted in accordance with the Declaration of Helsinki and approved by the Research Ethics Committee at the Institute of Applied Psychology at the Jagiellonian University. The participants signed the written informed consent before the data gathering.
Behavioral results
The linear mixed model was conducted with six response types (hits; misses; correct rejection and false alarms for lure probes; correct rejection and false alarms for negative probes) as fixed effects and participant as random effects to test for any differences in RTs between conditions of the task. The model was constructed using the lme function of the nlme R package (version 4.0.0) with restricted maximum likelihood. The probes without the response were not included in the analysis. An ANOVA was performed on the model. The significant effect of condition F(1,5) = 113.46, p < 0.0001 was revealed. The model indicated the significant differences between all response types (p < 0.001). The post hoc HSD Tukey test indicated the differences between correct rejection of negative and misses (p = 0.002) and between correct rejection of negative and false alarms for lure (p = 0.01). Table 2 showed mean RTs for all response types.
A two by two ANOVA with time of day (morning vs. evening) as a within-subjects factor and chronotype (MT vs. ET) as a between-subjects factor was conducted on measures of sensitivity (d') for item-specific memory and gist-based memory. Table 3 presents measures of sensitivity (d') calculated for morning and evening time-of-day as well as morning and evening types.
Item-specific memory
There was no main effect of chronotype F(1, 56) = 0.05, p = 0.82, no main effect of time-of-day F(1, 56) = 1.78, p = 0.19 and no interaction F(1, 56) = 1.024, p = 0.32 in sensitivity associated with discriminating positive probes from critical lures.
Gist-based memory
There was a main effect of chronotype F(1, 56) = 8.25, p = 0.006, η 2 = 0.13 showing greater reliance on gist among evening types (M = 1.23, SD = 0.61) than morning ones (M = 0.84, SD = 0.74). Neither a main effect of time of day F(1, 56) = 2.62, p = 0.11 nor an interaction F(1, 56) = 0.78, p = 0.38 were significant.
Next, we tested RT differences between times of day at which the study was performed and between chronotypes for each probe type: correctly recognized positive probe, correctly rejected lure probe and correctly rejected negative probe. For each probe type we performed 2 (chronotype: MT vs. ET) × 2 (time-of-day: morning vs. evening) ANOVA on RT and for all three probe types we revealed no main effects and no interactions (all Fs < 2, ps > 0.1).
fMRI results
Ica results.
Thirteen ICNs were extracted from the data: the anterior (medial prefrontal cortex, anterior cingulate gyrus) and posterior DMN (angular gyrus, posterior cingulate gyrus, precuneus), the left and right fronto-parietal network (fronto-parietal cortices), auditory network (superior temporal gyrus, Heschl’s gyrus), language network (inferior frontal gyrus, superior temporal gyrus), the medial (fusiform gyrus) and lateral (superior and middle occipital gyrus) visual network, occipital pole (inferior occipital gyrus), insular network, sensory-motor network (precentral gyrus, supplementary motor area), executive network (medial frontal gyrus, anterior cingulate gyrus) and dorsal attention network (intraparietal sulcus). The MNI coordinates of structures belonging to the given network are presented in Supplementary Information (Table S1 ).
In the encoding phase, for all conditions (hits, correct rejection of lures and false alarms) the activation of executive, right fronto-parietal and sensory-motor networks and the deactivation of anterior default mode, auditory and dorsal attention networks was evident. Furthermore, the analysis revealed activation of the medial visual network and deactivation of the insular network for hits, deactivation of the language network and activation of the medial visual network—for correct rejection of lure, and deactivation of insular and language network—for false alarms (Fig. 2 a). For other conditions, no differences were found.
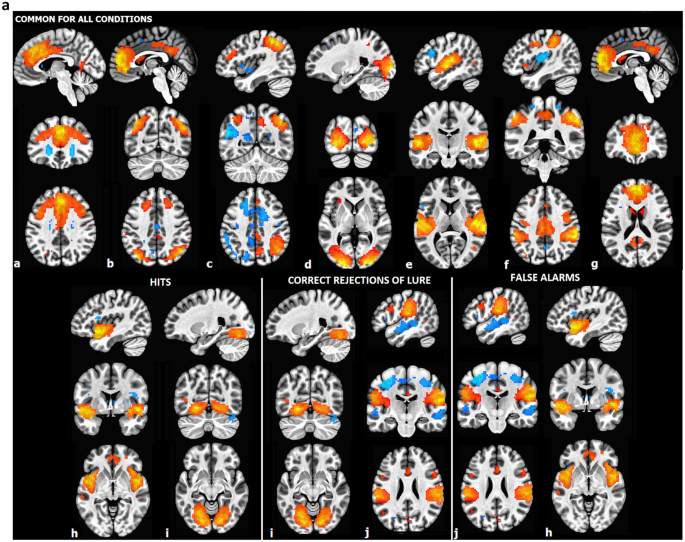
( a ) The brain networks engaged in the encoding phase of the phonological task, common for all conditions and distinct for each of them (FDR corrected at p < 0.05 level). a—Executive network, b—dorsal attention network, c—right fronto-parietal network, d—lateral visual network, e—auditory network, f—sensory-motor network, g—anterior default mode network, h—insular network, i—medial visual, j—language network. ( b ) The brain networks engaged in the retrieval phase of the phonological task, common for all conditions and specific for hits and correct rejection of lure probes (FDR corrected at p < .05 level). a—Executive network, b—right fronto-parietal network, c—lateral visual network, d—medial visual network, e—left fronto-parietal network, f—language network, g—anterior default mode network, h—posterior default mode network.
In the retrieval phase, for all conditions the activation of executive and right fronto-parietal networks and deactivation of lateral and medial visual networks was evident. Furthermore, for hits the activation of the left fronto-parietal networks was evident, and for correct rejection of lures—the deactivation of anterior and posterior default mode networks and activation of language network (Fig. 2 b). It is worth emphasizing that no distinguishing networks were detected for false alarms during the retrieval phase. No differences were evident for engagement of ICNs between morning- and evening-type participants. The diurnal differences (MS vs. ES) were evident only for correct rejection of lure in the retrieval phase for the anterior and posterior default mode networks and the medial visual network—all exhibited more deactivations in the morning (Fig. 3 ). For other conditions, no differences were found.
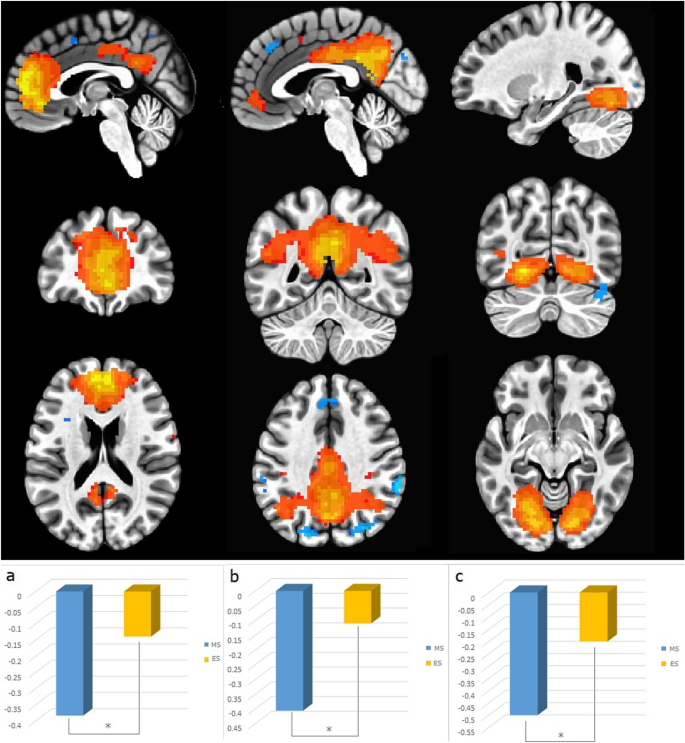
ICNs with diurnal differences during correct rejection of lure in the retrieval phase. Each network showed higher deactivation in the morning session compared to the evening session. The paired t-tests with FDR correction at p < 0.05 level were performed. ( a ) Anterior default mode network, ( b ) posterior default mode network, ( c ) medial visual network.
GLM results
The impact of time-of-day (MS vs. ES) was found for the contrast correct rejection of lure > false alarms. In the retrieval phase the right lingual gyrus (MNI: 15 −79 −7; t = 5.94; k = 154 voxels) and left cerebellum (MNI: −24 −79 −28; t = 5.40; k = 145 voxels) were activated in the evening session compared to the morning one. The significance level was established at p < 0.05, FDR corrected on the cluster level (Fig. 4 , Table 4 ).
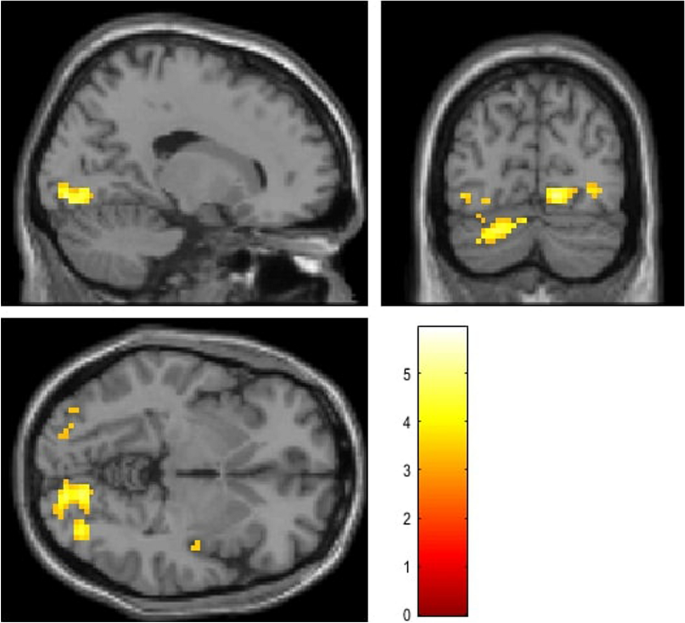
fMRI results of paired t-test for ES > MS for contrast correct rejection of lure > false alarms in the retrieval phase ( p < .05, FDR corrected on the cluster level).
The present study demonstrates the dynamics of working memory processes—differential engagement of brain networks during veridical recognition and false memories—in both encoding and retrieval phases. Also, modulatory effects of time-of-day on neural activity were observed during the retrieval phase of a visually presented phonological short-term memory task. We employed an exploratory approach—Independent Component Analysis—to investigate phonological DRM illusion formation and true recognition. This approach allowed us to assess the dynamic involvement of brain networks in encoding and retrieval phases in relation to working memory performance.
Many experimental paradigms were utilized to investigate memory illusions (for the reviews, see: REF 34 , 38 ). Numerous functional neuroimaging experiments that examine false recognition concentrate only on the retrieval phase as in the museum paradigm 66 , 67 , but some are focusing also on the encoding or/and storage processes (e.g. delayed-match-to-sample task). One of the paradigms, which allows investigating both the encoding and retrieval phases of the memorizing process, is utilized in our study the DRM paradigm.
Regarding the behavioral results, the differences in reaction times of the particular response types (the longest RTs for false alarms and correct rejection of lure probes, the shortest for correct rejection of negative probes) and between erroneous responses (misses, false alarms for lure and false alarms for negative probes) indicate the differences in information processing related to conflict monitoring 68 and when participants make errors 69 . Our results are in line with previous studies 24 , 70 .
In the context of neural activity, our findings point out that the networks activated in the encoding phase of the visual phonological task comprise executive, right fronto-parietal, visual and sensory-motor networks, whereas deactivated networks include the anterior default mode network, auditory and dorsal attention networks. The activation of executive and right fronto-parietal networks in the encoding phase is in line with results obtained from studies investigating the neural basis of visual short memory showing that the prefrontal and parietal association cortex are crucial structures that encode information 71 , 72 . Visual and sensory-motor network activated during encoding provides highly selective tuning of stimulus-specific features (for the review, see: Ref 71 ). Also, the deactivations of the anterior DMN, auditory and dorsal attention networks are consistent with previous results 73 . There is evidence that the deactivation of DMN during the encoding phase even enhances subsequent retrieval of maintained information 74 . Additionally, the analysis revealed activation of the medial visual network and deactivation of the insular network for hits, deactivation of the language network and activation of the medial visual network—for correct rejection of lure, and deactivation of insular and language network—for false alarms. We noticed the activity of the medial visual network (i.e. fusiform gyrus, BA 37) in the encoding phase that preceded accurate performance including hits and correct rejection of lure probes, whereas the engagement of the medial visual network during the encoding phase that preceded false alarms was not observed. The medial visual network is considered crucial in high-level visual processing for functionally-specialized processes related to object recognition, face perception, and language processing. A number of studies have also revealed the critical role of the left fusiform gyrus in both reading (e.g. 75 , 76 ) and learning to read 77 , 78 . Seeing words engages a highly specialized part of the middle fusiform gyrus, known as the visual word form area (VWFA). The VWFA is typically lateralized in the left hemisphere and activated by visually presented words and pseudowords but not by auditory ones 79 . This region is thought to be sensitive to orthographic information 80 , 81 , 82 . The study by Glezer and colleagues (2015) 83 employed fMRI-RA showed the VWFA as exclusively sensitive to orthography and not modulated by phonological information. Moreover, the study using PET 66 as well as fMRI 84 , revealed the role of bilateral VWFA in pseudowords processing, suggesting reduced lateralization in the pseudowords than in the words condition. Rauschecker (2012) 85 showed that the spatial pattern of VWFA response varies with stimulus position and that both the left and right middle fusiform gyri are involved in word processing, which together constitute a unified system that interfaces vision and language. Garoff and colleagues (2005) 86 found that the fusiform gyrus is activated during the encoding phase bilaterally, dependent on specific (related to processing of stimuli details) or non-specific (related to global processing of stimuli) recognition. Furthermore, the study by Ardila, Bernal, and Rosselli (2015) 87 utilizing a meta-analytic connectivity model to investigate the connectivity of the fusiform gyrus demonstrated the multimodality of this area. Two interconnected networks were found to be involved in different cognitive functions of language and visual processing. The interaction of the fusiform gyri with different brain areas points to the involvement of these structures in verbal working memory. This connectivity model also indicated a strong connection of fusiform gyri with the inferior frontal gyrus, a part of the language network.
At the retrieval phase, for all conditions, activation of the executive and right fronto-parietal networks and deactivation of the visual networks (medial and lateral) was observed. Additionally, for hits the activation of the left fronto-parietal networks was evident. Even though the left fronto-parietal network is involved in word processing, there are neuroimaging studies demonstrating the engagement of right frontal gyri and right parietal gyrus during the pseudowords reading 88 , 89 , as well as studies showing the involvement of right hemisphere in pseudowords visual processing 90 , 91 . The executive network comprising mainly of the prefrontal cortex and anterior cingulate cortex is responsible for cognitive control and optimizing goal-directed behaviors 92 . To sum up, the activation of executive and both right and left fronto-parietal networks supports true recognition. One of the most popular hypotheses in the functional neuroimaging experiments examining retrieval mechanisms of false memory is the sensory reactivation hypothesis, according to which the retrieval phase involves the reinstatement of processes that appeared in the encoding stage. This hypothesis assumes that true memory formation entails the activation of sensory areas (for the review, see: Ref 38 ). In experiments using visual stimuli the increased activity of early visual regions was linked with true but not false memories formation, whereas late visual regions (including BA 37, i.e. fusiform gyrus) showed both activities linked with true and false memories 93 . Also, Slotnick and Schacter (2004) 94 indicated that whereas early visual regions are primarily linked with true recognition (and seem to reflect sensory reactivation of previously seen stimuli), late visual structures were associated with both true and false recognition. The results of our short-term false memory study indicating deactivation of the visual networks (medial and lateral) at the retrieval phase for all conditions do not align with the sensory reactivation hypothesis. However, prior evidence supporting the hypothesis was obtained using long-term memory tasks and employing mainly visuo-spatial stimuli such as objects, photographs, or abstract shapes. In our short-term memory study even though the stimuli were presented visually, they were characterized by phonological similarity. Likewise, the results of an experiment employing stimuli that required mental imagery or orthographic-to-phonological transformation reported by Kahn, Davachi, and Wagner (2004) 95 also do not support the sensory reactivation hypothesis. Recent evidence transforming the concept of sensory recruitment in short-term/working memory. As reviewed by Favila, Lee, and Kuhl (2020) 39 , during memory retrieval the transformation of content representations from visual regions to frontoparietal regions is revealed.
During correct rejection of lures—the deactivation of anterior and posterior default mode networks and activation of language network was observed. The deactivation of DMN during external task performance is well-established 96 , along with greater deactivations as cognitive load increases 97 , 98 . Likewise, our results demonstrated that the correct rejection of lures condition seems more demanding than the other conditions.
The activation of the language network (i.e. inferior frontal gyrus and superior temporal gyrus) in the retrieval phase, in the case of more demanding condition (correct rejection of lures) compared to the true recognition needs to be discussed. This network in the encoding phase was deactivated preceding the correct rejection of lures and false alarms. The inferior frontal gyrus is recognized as a structure involved in coding articulatory phonological features 99 . A meta-analysis based on neuroimaging studies on language processing showed the involvement of the inferior frontal gyrus not only for semantic and phonological processing but also for working memory (for the review, see: Ref 100 ). As reviewed by Yi, Leonard, and Chang (2019) 101 , the superior temporal gyrus is considered one of the critical centers for phonological processing whereby meaningful linguistic features such as phonemes or syllables are extracted from the speech input. In light of our results, the dynamic interplay between medial visual and language networks for the more demanding probe (lure probe) supports the correct rejection of lures or false memory. Regarding the less demanding condition (encoding preceding hits), the activation of the executive and fronto-parietal network appears to be sufficient. Our results are in line with the dynamic-processing model of working memory, implicating the conversion and development of memory representations over time and showing that cognitive control induces engagement of different network to support goal-directed behavior 29 .
During the last decades, the false memory phenomenon has been extensively studied both in long-term and short-term/working memory, but the neural basis of false memory formation is rather underexplored. The main structures showing false recognition-related changes in the activity were the anterior cingulate cortex and the medial temporal gyrus (for a meta-analysis, see: Ref 34 ), as well as fronto-parietal regions, superior temporal gyrus, and insula (for a meta-analysis, see: Ref 35 ). Also, the amygdala activity was observed to differentiate false from true memories both at short and long time delays 102 , 103 , the same as the orbital cortices in long-term memory 102 . Yet, it should be noted that in these experiments, unlike in the current one, the morphed human faces were used, potentially increasing the stimuli saliency. Importantly, independently of the material type used in the experiment, the false memories were found to emerge based on both semantic and perceptual relatedness of these stimuli 104 , 105 . For instance, both semantically- and perceptually-related words were used 104 , 106 , the same as conceptually- and perceptually-related objects and scenes 93 , 105 , 107 , 108 , 109 . The concept of the dynamic-processing model of working memory utilized in our study allowed for a deeper insight into the neural basis of false memory formation.
Our findings highlight the complex interactions of memory representations during the encoding and retrieval phases, resulting in false memory, true recognitions, and correct rejection of lures. We demonstrated that phonological coding in the language network plays a role in working memory performance. Our results also have some parallels with the study by Zhu and colleagues employing DRM tasks in documenting complex interactions of memory representations during encoding and retrieval phases related to sensory modality 110 .
In the context of diurnal differences indicating an effect of circadian and/or homeostatic processes 111 on veridical memory and memory distortions, this effect was not found. We observed the deactivations of the anterior and posterior DMN and the medial visual network with higher deactivation in the morning hours during the correct rejection of lure probes (at the retrieval phase). Previous studies have shown involvement of the DMN during visual n-back task 112 as well as semantic and non-semantic declarative memory tasks 113 . Although here was studied short-term memory, we reported converging results, and additionally revealed involvement of the DMN that was dependent on the time-of-day. Blautzik et al. (2013) 114 demonstrated the rhythmic connectivity patterns of DMN across a day, other evidence is provided by the study using regional homogeneity in resting-state fMRI, revealed the diurnal variations in the DMN activity 115 . Here, we confirmed the engagement of DMN in a specific condition of short-term memory task—the correct rejection of lure during retrieval, as well as the change of deactivation of default mode network dependent on the time-of-day, which could be explained by the effect of homeostatic process after a day full of activities 111 .
The GLM analysis also revealed diurnal differences in some brain regions, in the contrast correct rejection of lures vs. false alarms namely, in the left cerebellum and right lingual gyrus. During the last few decades, many studies have confirmed that the cerebellum is involved not only in motor control but also in many cognitive functions, including learning 116 , 117 , as well as in the phonological short-term memory 118 . Although the right cerebellum was considered responsible for language processing including phonological word retrieval 119 , Murdoch and Whelan (2007) 120 demonstrated that left cerebellar lesions disrupt language processing and proposed model of bilateral involvement of the cerebellum in high-level linguistic processing. On the other hand, the right lingual gyrus is not typically recruited for short-term memory tasks but its function has been established for letter processing and words/pseudowords reading (for a meta-analysis, see: Ref 84 ). It was shown that a decrease of right lingual gyrus involvement in word processing was correlated with semantic deficits in patients with ALS when they used Japanese sign-words 121 . Our results showed increased activation of this structure in the evening, which might be related to the information processing strategy that engaged the right hemisphere at this time of day as suggested by researchers exploring the diurnal and/or circadian variability of cognitive processes (e.g. 122 , 123 , 124 ).
Taken together, the present study using Independent Component Analysis (ICA), DRM paradigm, and time-of-day protocol shed new light on the neural mechanism of false memory formation, dynamic process of veridical memory, and the modulatory effect of time-of-day on the retrieval process.
Limitations and future directions
One limitation of our study is the lack of objective control or measurement of the fatigue of participants, which would allow us to show even more impact of the homeostatic process. Another limitation is the use of FDR correction, which is less conservative than FEW (family-wise error), nevertheless, FDR correction is often applied in neuroimaging research. Also, future studies could include analysis involving false alarms to unrelated probes, in particular a comparison of false alarms to related versus unrelated probes. This challenging comparison was not possible in our experiment due to very low error rate on unrelated, clearly distinct probes. When applied in future studies, it could provide further insight into the false memory phenomenon. Finally, future studies could use meaningful verbal material to explore if the patterns of network activation/deactivation are similar. Last but not least, the recent study by Spets and colleagues (2021) 125 suggests sex differences in the neural correlates of retrieval processes, which is a relevant direction for future studies.
Conclusions
To our knowledge, this is the first study on veridical and false memory using the ICA approach, which offers new insight into the neural mechanisms of false memory formation in short-term memory. The main findings illustrate a deficiency of medial visual network activity during the encoding phase, contributing to false memory formation in short-term memory. Our results related to true recognition and correct rejection of lures support the dynamic-processing model of working memory showing that cognitive control induces different dynamics of networks to support goal-directed behavior. The results related to the modulatory effect of time-of-day suggest another important, yet under-appreciated neurobiological factor that can influence the dynamics of working memory.
Data availability
Data can be made available from the corresponding author on reasonable request. Data were analyzed with open source toolboxes: SPM12 (Welcome Department of Imaging Neuroscience, UCL, London, UK), DPABI (V4.2) implemented on MATLAB (Mathworks, Inc., MA, USA) and GIFT software package (v4.0b; Medical Image Analysis Lab, University of New Mexico).
Abbreviations
Amplitude scale from Chronotype Questionnaire
Blood oxygenation level dependent contrast
Default mode network
Deese–Roediger–McDermott
Edinburgh Handedness Inventory
Echo-planar imaging
Evening session
Epworth Sleepiness Scale
Evening type
False discovery rate
Functional magnetic resonance imaging
Field of view
General linear model
Independent component analysis
Intrinsic connectivity network
Laminar functional magnetic resonance imaging
Minimum description length
Morningness/eveningness scale from Chronotype Questionnaire
Montreal Neurological Institute
Magnetic resonance
Morning session
Morning type
Principal component analysis
Period circadian regulator 3
Pittsburgh Sleep Quality Index
Reaction time
Short-term memory DRM
Repetition time
Visual word form area
Deese, J. On the prediction of occurrence of particular verbal intrusions in immediate recall. J. Exp. Psychol. 58 , 17–22. https://doi.org/10.1037/h0046671 (1959).
Article CAS PubMed Google Scholar
Roediger, H. L. & McDermott, K. B. Creating false memories: Remembering words not presented in lists. J. Exp. Psychol. 21 , 803–814. https://doi.org/10.1037/0278-7393.21.4.803 (1995).
Article Google Scholar
Baddeley, A. Working memory. Curr. Biol. 20 , 136–140 (2010).
Cowan, N. Working memory underpins cognitive development, learning, and education. Educ. Psychol. Rev. 26 (2), 197–223 (2014).
Article PubMed Google Scholar
Cowan, N. The many faces of working memory and short-term storage. Psychon. Bull. Rev. 24 (4), 1158–1170 (2017).
Chai, W. J., Abd Hamid, A. I. & Abdullah, J. M. Working memory from the psychological and neurosciences perspectives: A review. Front. Psychol. 9 , 401 (2018).
Article PubMed PubMed Central Google Scholar
Oberauer, K. Working memory and attention–A conceptual analysis and review. J. Cogn. 2 (1), 36 (2019).
Unsworth, N. & Engle, R. W. On the division of short-term and working memory: An examination of simple and complex span and their relation to higher order abilities. Psychol. Bull. 133 (6), 1038–1066 (2007).
Cowan, N. What are the differences between long-term, short-term, and working memory?. Prog. Brain Res. 169 , 323–338 (2008).
Baddeley, A. & Hitch, G. Working memory. Psychol. Learn. Motiv. 8 , 47–89 (1974).
Baars, B. J. & Franklin, S. How conscious experience and working memory interact. Trends Cogn. Sci. 7 , 166–172 (2003).
Ashkenazi, S., Rosenberg-lee, M., Metcalfe, A. W. S., Swigart, A. G. & Menon, V. Visuo-spatial working memory is an important source of domain-general vulnerability. Neuropsychologia 51 (11), 2305–2317. https://doi.org/10.1016/j.neuropsychologia.2013.06.03 (2013).
D’Esposito, M. & Postle, B. R. The cognitive neuroscience of working memory. Annu. Rev. Psychol. 66 , 115–142 (2015).
Kim, C., Kroger, J. K., Calhoun, V. D. & Clark, V. P. The role of the frontopolar cortex in manipulation of integrated information in working memory. Neurosci. Lett. 595 , 25–29 (2015).
Article CAS PubMed PubMed Central Google Scholar
Baddeley, A., Gathercole, S. & Papagno, C. The phonological loop as a language learning device. Psychol. Rev. 105 (1), 158–173. https://doi.org/10.1037/0033-295x.105.1.158 (1998).
Hamada, M. & Koda, K. The role of the phonological loop in english word learning: A comparison of Chinese ESL learners and native speakers. J. Psycholinguist. Res. 40 (2), 75–92. https://doi.org/10.1007/s10936-010-9156-9 (2010).
Coane, J. H. et al. Manipulations of list type in the DRM paradigm: A review of how structural and conceptual similarity affect false memory. Front. Psychol. 12 , 668550. https://doi.org/10.3389/fpsyg.2021.668550 (2021).
Chang, M. & Brainerd, C. J. Semantic and phonological false memory: A review of theory and data. J. Mem. Lang. 119 , 104210. https://doi.org/10.1016/j.jml.2020.104210 (2021).
Dodson, C. S. & Schacter, D. L. “If I had said it I would have remembered it”: Reducing false memories with a distinctiveness heuristic. Psychon. Bull. Rev. 8 , 155–161 (2001).
Gallo, D. A., McDermott, K. B., Percer, J. M. & Roediger, H. L. Modality effects in false recall and false recognition. J. Exp. Psychol. Learn. Mem. Cogn. 27 , 339–353. https://doi.org/10.1037/0278-7393.27.2.339 (2001).
Maylor, E. & Mo, A. Effects of study-test modality on false recognition. Br. J. Psychol. 90 , 477–493 (1999).
Smith, R. & Hunt, E. Presentation modality affects false memory. Psychon. Bull. Rev. 5 , 710–715. https://doi.org/10.3758/BF03208850 (1998).
Atkins, A. S. & Reuter-Lorenz, P. A. False working memories? Semantic distortion in a mere 4 seconds. Mem. Cognit. 36 , 74–81. https://doi.org/10.3758/mc.36.1.74 (2008).
Atkins, A. S. & Reuter-Lorenz, P. A. Neural mechanisms of semantic interference and false recognition in short-term memory. Neuroimage 56 , 1726–1734. https://doi.org/10.1016/j.neuroimage.2011.02.048 (2011).
McBride, D. M., Coane, J. H., Xu, S., Feng, Y. & Yu, Z. Short-term false memories vary as a function of list type. Q. J. Exp. Psychol. 72 (12), 2726–2741. https://doi.org/10.1177/1747021819859880 (2019).
Olszewska, J. M., Reuter-Lorenz, P. A., Munier, E. & Bendler, S. A. Misremembering what you see or hear: Dissociable effects of modality on short- and long-term false recognition. J. Exp. Psychol. Learn. Mem. Cogn. 41 (5), 1316–1325. https://doi.org/10.1037/xlm0000115 (2015).
Logie, R. H., Belletier, C., & Doherty, J. M. Integrating theories of working memory. In Working Memory: State of the Science (Logie, R. H., Camos, V., & Cowan, N. Eds.). 389–429. (Oxford University Press, 2021).
Rose, N. S. The dynamic-processing model of working memory. Curr. Dir. Psychol. Sci. 29 (4), 378–387. https://doi.org/10.1177/0963721420922185 (2020).
Buschman, T. J. & Miller, E. K. Working memory is complex and dynamic, like your thoughts. J. Cogn. Neurosci. 35 (1), 17–23. https://doi.org/10.1162/jocn_a_01940 (2022).
Chein, J. M., Moore, A. B. & Conway, A. R. A. NeuroImage domain-general mechanisms of complex working memory span. Neuroimage 54 , 550–559 (2011).
Owen, A. M., McMillan, K. M., Laird, A. R. & Bullmore, E. N-back working memory paradigm: A meta-analysis of normative functional neuroimaging studies. Hum. Brain Mapp. 25 , 46–59 (2005).
Logie, R. H., Venneri, A., Della Sala, S., Redpath, T. W. & Marshall, I. Brain activation and the phonological loop: The impact of rehearsal. Brain Cogn. 53 (2), 293–296 (2003).
Sharoh, D. et al. Laminar specific fMRI reveals directed interactions in distributed networks during language processing. PNAS https://doi.org/10.1073/pnas.1907858116 (2019).
Kurkela, K. A. & Dennis, N. A. Event-related fMRI studies of false memory: An activation likelihood estimation meta-analysis. Neuropsychologia 81 , 149–167. https://doi.org/10.1016/j.neuropsychologia.2015.12.006 (2016).
Yu, J., Tao, Q., Zhang, R., Chan, C. C. H. & Lee, T. M. C. Can fMRI discriminate between deception and false memory? A meta-analytic comparison between deception and false memory studies. Neurosci. Biobehav. Rev. 104 , 43–55. https://doi.org/10.1016/j.neubiorev.2019.06.027 (2019).
Ceglarek, A. et al. Non-linear functional brain co-activations in short-term memory distortion tasks. Front. Neurosci. 15 , 778242. https://doi.org/10.3389/fnins.2021.778242 (2021).
Sikora-Wachowicz, B. et al. False recognitions in short-term memory–Age-differences in neural activity. Brain Cogn. 151 , 105728. https://doi.org/10.1016/j.bandc.2021.105728 (2021).
Abe, N. Neuroimaging studies of false memory; A selective review. Psychologia 55 , 131–145. https://doi.org/10.2117/psysoc.2012.131 (2012).
Favila, S. E., Lee, H. & Kuhl, B. A. Transforming the concept of memory reactivation. Trends Neurosci. 43 (12), 939–950 (2020).
Farahani, F. V. et al. Diurnal variations of resting-state fMRI data: A graph-based analysis. Neuroimage 256 , 119246. https://doi.org/10.1016/j.neuroimage.2022.119246 (2022).
Schmidt, C., Collette, F., Cajochen, C. & Peigneux, P. A time to think: circadian rhythms in human cognition. Cogn. Neuropsychol. 24 , 755–789. https://doi.org/10.1080/02643290701754158 (2007).
Smarr, B. L., Jennings, K. J., Driscoll, J. R. & Kriegsfeld, L. J. A time to remember: The role of circadian clocks in learning and memory. Behav. Neurosci. 128 (3), 283–303. https://doi.org/10.1037/a0035963 (2014).
Monteiro, F., Rodrigues, P., Nascimento, C. S., Simões, F. & Miguel, M. The daily rhythms of working memory and their methodological constraints: A critical overview. Biol. Rhythm Res. 53 , 1–28. https://doi.org/10.1080/09291016.2021.1907511 (2021).
Viola, A. U. et al. PER3 polymorphism predicts sleep structure and waking performance. Curr. Biol. 17 (7), 613–618. https://doi.org/10.1016/j.cub.2007.01.073 (2007).
Liberman, A. R. et al. Circadian clock model supports molecular link between PER3 and human anxiety. Sci. Rep. 7 (1), 1–10 (2017).
Article MathSciNet CAS Google Scholar
Archer, S. N., Schmidt, C., Vandewalle, G. & Dijk, D. J. Phenotyping of PER3 variants reveals widespread effects on circadian preference, sleep regulation, and health. Sleep Med. Rev. 40 , 109–126 (2018).
Fabbri, M., Mencarelli, C., Adan, A. & Natale, V. Time-of-day and circadian typology on memory retrieval. Biol. Rhythm Res. 44 (1), 125–142 (2013).
Schmidt, C. et al. Pushing the limits: Chronotype and time of day modulate working memory-dependent cerebral activity. Front. Neurol. https://doi.org/10.3389/fneur.2015.00199 (2015).
Lewandowska, K., Wachowicz, B., Marek, T., Oginska, H. & Fafrowicz, M. Would you say “yes” in the evening? Time-of-day effect on response bias in four types of working memory recognition tasks. Chronobiol. Int. 35 (1), 80–89 (2018).
Singh, P. et al. Analysis of fMRI signals from working memory tasks and resting-state of brain: Neutrosophic-entropy-based clustering algorithm. Int. J. Neural Syst. 32 (04), 2250012. https://doi.org/10.1142/S0129065722500125 (2022).
Article MathSciNet PubMed Google Scholar
Bijsterbosch, J., Smith, S. M. & Beckmann, C. F. Introduction to Resting State fMRI Functional Connectivity (Oxford University Press, 2017).
Google Scholar
McKeown, M. J., Hansen, L. K. & Sejnowsk, T. J. Independent component analysis of functional MRI: What is signal and what is noise?. Curr. Opin. Neurobiol. 13 (5), 620–629 (2003).
Oginska, H., Mojsa-Kaja, J. & Mairesse, O. Chronotype description: In search of a solid subjective amplitude scale. Chronobiol. Int. 34 , 1388–1400. https://doi.org/10.1080/07420528.2017.1372469 (2017).
Buysse, D. J., Reynolds, C. F. I., Monk, T. H., Berman, S. R. & Kupfer, D. J. The Pittsburgh Sleep Quality Index: A new instrument for psychiatric practice and research. Psychiatry Res. 28 , 193–213. https://doi.org/10.1016/0165-1781(89)90047-4 (1989).
Johns, M. W. A new method for measuring daytime sleepiness: The Epworth sleepiness scale. Sleep 14 , 540–545. https://doi.org/10.1093/sleep/14.6.540 (1991).
Oldfield, R. C. The assessment and analysis of handedness: The Edinburgh inventory. Neuropsychologia 9 , 97–113. https://doi.org/10.1016/0028-3932(71)90067-4 (1971).
Kazojc, J. Slownik frekwencyjny języka polskiego [ Polish Language Dictionary of Attendance ]. http://www.slowniki.org.pl/pol.html (2011).
McMillan, N. A. & Creelman, C. D. Detection Theory: A User’s Guide . 2nd edn. (Erlbaum, 2005).
Koutstaal, W. & Schacter, D. L. Gist-based false recognition of pictures in older and younger adults. J. Mem. Lang. 37 (4), 555–583. https://doi.org/10.1006/jmla.1997.2529 (1997).
Flegal, K. E., Atkins, A. S. & Reuter-Lorenz, P. A. False memories seconds later: The rapid and compelling onset of illusory recognition. J. Exp. Psychol. Learn. Mem. Cogn. 36 , 1331–1338. https://doi.org/10.1037/a0019903 (2010).
Arndt, J. The role of memory activation in creating false memories of encoding context. J. Exp. Psychol. Learn. Mem. Cogn. 36 (1), 66–79. https://doi.org/10.1037/a0017394 (2010).
Article MathSciNet PubMed PubMed Central Google Scholar
Olszewska, J. et al. Meaningful versus meaningless sounds and words: A false memories perspective. Exp. Psychol. 68 (1), 4–17. https://doi.org/10.1027/1618-3169/a000506 (2021).
Beckmann, C. F., DeLuca, M., Devlin, J. T. & Smith, S. M. Investigations into resting-state connectivity using independent component analysis. Philos. Trans. R. Soc. Lond. B. Biol. Sci. 360 (1457), 1001–1013 (2005).
Smith, S. M. et al. Correspondence of the brain’s functional architecture during activation and rest. PNAS 106 (31), 13040–13045 (2009).
Article ADS CAS PubMed PubMed Central Google Scholar
Laird, A. R. et al. Behavioral interpretations of intrinsic connectivity networks. J. Cogn. Neurosci. 23 (12), 4022–4037 (2011).
St. Jacques, P. L. & Schacter, D. L. Modifying memory. Psych. Sci. 24 (4), 537–543. https://doi.org/10.1177/0956797612457377 (2013).
Daviddi, S., Mastroberardino, S., St. Jacques, P. L., Schacter, D. L. & Santangelo, V. Remembering a virtual museum tour: Viewing time, memory reactivation, and memory distortion. Front. Psychol. 13 , 869336. https://doi.org/10.3389/fpsyg.2022.869336 (2022).
Brown, J. W. Beyond conflict monitoring. Curr. Dir. Psychol. Sci. 22 (3), 179–185. https://doi.org/10.1177/0963721412470685 (2013).
Hoffmann, S. & Beste, C. A perspective on neural and cognitive mechanisms of error commission. Front. Behav. Neurosci. 9 , 1–16. https://doi.org/10.3389/fnbeh.2015.00050 (2015).
Article CAS Google Scholar
Janik, R. A. et al. Neural spatio-temporal patterns of information processing related to cognitive conflict and correct or false recognitions. Sci. Rep. 12 , 5271. https://doi.org/10.1038/s41598-022-09141-9 (2022).
Serences, J. T. Neural mechanisms of information storage in visual short-term memory. Vis. Res. 128 , 53–67. https://doi.org/10.1016/j.visres.2016.09.010 (2016).
Jacob, S. N., Hähnke, D. & Nieder, A. Structuring of abstract working memory content by fronto-parietal synchrony in primate cortex. Neuron 99 (3), 588–597. https://doi.org/10.1016/j.neuron.2018.07.025 (2018).
Sato, N. & Mizuhara, H. Successful encoding during natural reading is associated with fixation-related potentials and large-scale network deactivation. eNeuro https://doi.org/10.1523/ENEURO.0122-18.2018 (2018).
Daselaar, S. M., Prince, S. E. & Cabeza, R. When less means more: Deactivations during encoding that predict subsequent memory. Neuroimage 23 (3), 921–927. https://doi.org/10.1016/j.neuroimage.2004.07.031 (2004).
Cohen, L. et al. The visual word form area. Brain 123 (2), 291–307. https://doi.org/10.1093/brain/123.2.291 (2000).
Qu, J. et al. Cross-language pattern similarity in the bilateral fusiform cortex is associated with reading proficiency in second language. Neuroscience 410 , 254–263 (2019).
Dehaene, S. et al. How learning to read changes the cortical networks for vision and language. Science 330 (6009), 1359–1364. https://doi.org/10.1126/science.1194140 (2010).
Article ADS CAS PubMed Google Scholar
Ben-Shachar, M., Dougherty, R. F., Deutsch, G. K. & Wandell, B. A. The development of cortical sensitivity to visual word forms. J. Cogn. Neurosci. 23 , 2387–2399 (2011).
Dehaene, S., Le Clec’H, G., Poline, J. B., Le Bihan, D. & Cohen, L. The visual word form area: A prelexical representation of visual words in the fusiform gyrus. NeuroReport 13 , 321–325 (2002).
Kronbichler, M. et al. Taxi vs. taksi: On orthographic word recognition in the left ventral occipitotemporal cortex. J. Cogn. Neurosci. 19 (10), 1584–1594 (2007).
Bruno, J. L., Zumberge, A., Manis, F. R., Lu, Z. L. & Goldman, J. G. Sensitivity to orthographic familiarity in the occipito-temporal region. Neuroimage 39 (4), 1988–2001 (2008).
Glezer, L. S. & Riesenhuber, M. Individual variability in location impacts orthographic selectivity in the “visual word form area”. J. Neurosci. 33 (27), 11221–11226 (2013).
Glezer, L. S., Kim, J., Rule, J., Jiang, X. & Riesenhuber, M. Adding words to the brain’s visual dictionary: Novel word learning selectively sharpens orthographic representations in the VWFA. J. Neurosci. 35 (12), 4965–4972 (2015).
Tagamets, M.-A., Novick, J. M., Chalmers, M. L. & Friedman, R. B. A parametric approach to orthographic processing in the brain: An fMRI study. J. Cogn. Neurosci. 12 (2), 281–297. https://doi.org/10.1162/089892900562101 (2000).
Rauschecker, J. P. Ventral and dorsal streams in the evolution of speech and language. Front. Evol. Neurosci. 4 , 7. https://doi.org/10.3389/fnevo.2012.00007 (2012).
Garoff, R. J., Slotnick, S. D. & Schacter, D. L. The neural origins of specific and general memory: The role of the fusiform gyrus. Neuropsychologia 43 (6), 847–859 (2005).
Ardila, A., Bernal, B. & Rosselli, M. Language and visual perception associations: Meta-analytic connectivity modeling of Brodmann area 37. Beh. Neurol. 2015 , 1–14. https://doi.org/10.1155/2015/565871 (2015).
Hagoort, P. et al. The neural circuitry involved in the reading of German words and pseudowords: A PET study. J. Cogn. Neurosci. 11 (4), 383–398 (1999).
Abutalebi, J. et al. Late acquisition of literacy in a native language. Hum. Brain Mapp. 28 , 19–33 (2007).
Rutherford, B. J. & Mathesius, J. R. The brain’s hemispheres and controlled search of the Lexicon: Evidence from fixated words and pseudowords. Brain Cognit. 79 (3), 188–199 (2012).
Ripamonti, E., Traficante, D., Crippa, F. & Luzzatti, C. Word and pseudoword superiority effects in a shallow orthography language: The role of hemispheric lateralization. Percept. Mot. Skills 118 (2), 411–428 (2014).
Friedman, N. P. & Robbins, T. W. The role of prefrontal cortex in cognitive control and executive function. Neuropsychopharmacology 47 (1), 72–89. https://doi.org/10.1038/s41386-021-01132-0 (2021).
Kim, H. & Cabeza, R. Differential contributions of prefrontal, medial temporal, and sensory-perceptual regions to true and false memory formation. Cereb. Cortex 17 (9), 2143–2150. https://doi.org/10.1093/cercor/bhl122 (2007).
Slotnick, S. D. & Schacter, D. L. A sensory signature that distinguishes true from false memories. Nat. Neurosci. 7 , 664–672. https://doi.org/10.1038/nn1252 (2004).
Kahn, I., Davachi, L. & Wagner, A. D. Functional-neuroanatomic correlates of recollection: Implications for models of recognition memory. J. Neurosci. 24 (17), 4172–4180. https://doi.org/10.1523/jneurosci.0624-04.2004 (2004).
Buckner, R. L., Andrews-Hanna, J. R. & Schacter, D. L. The brain’s default network. Ann. N. Y. Acad. Sci. 1124 (1), 1–38. https://doi.org/10.1196/annals.1440.011 (2008).
Article ADS PubMed Google Scholar
Singh, K. D. & Fawcett, I. P. Transient and linearly graded deactivation of the human default-mode network by a visual detection task. Neuroimage 41 (1), 100–112. https://doi.org/10.1016/j.neuroimage.2008.01.051 (2008).
Hu, Y., Chen, X., Gu, H. & Yang, Y. Resting-state glutamate and GABA concentrations predict task-induced deactivation in the default mode network. J. Neurosci. 33 (47), 18566–18573. https://doi.org/10.1523/jneurosci.1973-13.2013 (2013).
Okada, K., Matchin, W. & Hickok, G. Phonological feature repetition suppression in the left inferior frontal gyrus. J. Cogn. Neurosci. 30 (10), 1549–1557. https://doi.org/10.1162/jocn_a_01287 (2018).
Liakakis, G., Nickel, J. & Seitz, R. Diversity of the inferior frontal gyrus—A meta-analysis of neuroimaging studies. Behav. Brain Res. 225 (1), 341–347 (2011).
Yi, H. G., Leonard, M. K. & Chang, E. F. The encoding of speech sounds in the superior temporal gyrus. Neuron 102 (6), 1096–1110 (2019).
Iidaka, T., Harada, T., Kawaguchi, J. & Sadato, N. Neuroanatomical substrates involved in true and false memories for face. Neuroimage 62 (1), 167–176. https://doi.org/10.1016/j.neuroimage.2012.04.044 (2012).
Iidaka, T., Harada, T. & Sadato, N. False memory for face in short-term memory and neural activity in human amygdala. Brain Res. 1591 , 74–85. https://doi.org/10.1016/j.brainres.2014.10.003 (2014).
Ly, M., Murray, E. & Yassa, M. A. Perceptual versus conceptual interference and pattern separation of verbal stimuli in young and older adults. Hippocampus 23 (6), 425–430 (2013).
Pidgeon, L. M. & Morcom, A. M. Age-related increases in false recognition: the role of perceptual and conceptual similarity. Front. Aging Neurosci. 6 , 283 (2014).
Garoff-Eaton, R. J., Kensinger, E. A. & Schacter, D. L. The neural correlates of conceptual and perceptual false recognition. Learn. Mem. 14 (10), 684–692 (2007).
Gutchess, A. H. & Schacter, D. L. The neural correlates of gist-based true and false recognition. Neuroimage 59 (4), 3418–3426 (2012).
Garoff-Eaton, R. J., Slotnick, S. D. & Schacter, D. L. Not all false memories are created equal: The neural basis of false recognition. Cereb. Cortex 16 , 1645–1652. https://doi.org/10.1093/cercor/bhj101 (2005).
Miller, M. B. & Gazzaniga, M. S. Creating false memories for visual scenes. Neuropsychologia 36 (6), 513–520 (1998).
Zhu, B. et al. Multiple interactive memory representations underlie the induction of false memory. PNAS 116 (9), 3466–3475. https://doi.org/10.1073/pnas.1817925116 (2019).
Borbély, A. A., Daan, S., Wirz-Justice, A. & Deboer, T. The two-process model of sleep regulation: a reappraisal. J. Sleep Res. 25 (2), 131–143 (2016).
Xia, L., Qihong, Z., Yong, H. & Yihong, Y. Topologically reorganized connectivity architecture of default-mode, executive-control, and salience networks across working memory task loads. Cereb. Cortex 26 (4), 1501–1511 (2016).
Wirth, M. et al. Semantic memory involvement in the default mode network: A functional neuroimaging study using independent component analysis. Neuroimage 54 (4), 3057–3066. https://doi.org/10.1016/j.neuroimage.2010.10.039 (2011).
Blautzik, J. et al. Classifying fMRI-derived resting-state connectivity patterns according to their daily rhythmicity. Neuroimage 71 , 298–306. https://doi.org/10.1016/j.neuroimage.2012.08.010 (2013).
Jiang, C. et al. Diurnal variations in neural activity of healthy human brain decoded with resting-state blood oxygen level dependent fMRI. Front. Hum. Neurosci. https://doi.org/10.3389/fnhum.2016.00634 (2016).
Buckner, R. L. The cerebellum and cognitive function: 25 years of insight from anatomy and neuroimaging. Neuron 80 , 807–815. https://doi.org/10.1016/j.neuron.2013.10.044 (2013).
Christian, K. M. & Thompson, R. F. Neural substrates of eyeblink conditioning: Acquisition and retention. Learn Mem. 10 (6), 427–455. https://doi.org/10.1101/lm.59603 (2003).
Paulesu, E., Frith, C. D. & Frackowiak, R. S. J. The neural correlates of the verbal component of working memory. Nature 362 (6418), 342–345. https://doi.org/10.1038/362342a0 (1993).
Marien, P., Engelborghs, S., Fabbro, F. & De Deyn, P. P. The lateralized linguistic cerebellum: A review and a new hypothesis. Brain Lang. 79 (3), 580–600. https://doi.org/10.1006/brln.2001.2569 (2001).
Murdoch, B. E. & Whelan, B.-M. Language disorders subsequent to left cerebellar lesions: A case for bilateral cerebellar involvement in language?. Folia Phoniatr. Logop. 59 (4), 184–189. https://doi.org/10.1159/000102930 (2007).
Ogura, A. et al. Semantic deficits in ALS related to right lingual/fusiform gyrus network involvement. EBioMedicine 47 , 506–517. https://doi.org/10.1016/j.ebiom.2019.08.022 (2019).
Folkard, S. Time of day and level of processing. Mem. Cognit. 7 , 247–252 (1979).
Folkard, S. & Monk, T. H. Circadian rhythms in human memory. Br. J. Psychol. 71 , 295–307. https://doi.org/10.1111/j.2044-8295.1980.tb01746.x (1980).
Corbera, X., Grau, C. & Vendrell, P. Diurnal oscillations in hemispheric performance. J. Clin. Exp. Neuropsychol. 15 (2), 300–310 (1993).
Spets, D. S., Karanian, J. M. & Slotnick, S. D. False memories activate distinct brain regions in females and males. Neuroimage Rep. 1 (4), 100043. https://doi.org/10.1016/j.ynirp.2021.100043 (2021).
Download references
Acknowledgements
We thank Karolina Lukasik, Alicja Grochowska, and Paulina Kostrzewska for their support in the experimental task developing, Aleksandra Domagalik for her helpful suggestions during project realization process, Piotr Faba for his technical support on this project and help in data acquisition, Anna Beres for her assistance with data collection, Aleksandra Zyrkowska for help in process of participants selection, and Magdalena Debowska for help in collecting actigraphy data. Special thanks to Bernadette A. Woldt for her contribution to the language quality of the text.
This study was funded by the Polish National Science Centre through grant Harmonia (2013/08/M/HS6/00042) and supported by the Foundation for Polish Science project “Bio-inspired Artificial Neural Networks” (POIR.04.04.00-00-14DE/18-00).
Author information
Authors and affiliations.
Department of Cognitive Neuroscience and Neuroergonomics, Institute of Applied Psychology, Jagiellonian University, Lojasiewicza Street 4, 30-348, Krakow, Poland
Magdalena Fafrowicz, Anna Ceglarek, Anna Sobczak, Koryna Lewandowska, Barbara Sikora-Wachowicz, Halszka Oginska & Tadeusz Marek
Department of Psychology, University of Wisconsin-Oshkosh, Oshkosh, WI, USA
Justyna Olszewska
Department of Psychology of Individual Differences, Psychological Diagnosis and Psychometrics, Faculty of Psychology, SWPS University of Social Sciences and Humanities, Warsaw, Poland
Bartosz Bohaterewicz
Chair of Radiology, Medical College, Jagiellonian University, Krakow, Poland
Monika Ostrogorska
Department of Psychology, University of Michigan, Ann Arbor, MI, USA
Patricia Reuter-Lorenz
Department of Drug Addiction Pharmacology, Maj Institute of Pharmacology, Polish Academy of Sciences, Krakow, Poland
Magdalena Hubalewska-Mazgaj
You can also search for this author in PubMed Google Scholar
Contributions
M.F.: Conceptualization, Research design, Methodology, Data collection, Data Curation, Funding acquisition, Project administration, Results interpretation, Writing—original draft, Writing—review & editing, A.C.: Data curation, Methodology, Behavioral and fMRI data analysis, Visualization, Results interpretation, Writing—original draft, Writing—review & editing. J.O.: Conceptualization, Research design, Methodology, Behavioral data analysis, Writing—original draft, Writing—review & editing. A.S.: Writing—original draft, Writing—review & editing. B.B.: Data curation, Methodology, Writing—original draft, Writing—review & editing. M.O.: Data collection, Writing—original draft, Writing—review & editing. PRL: Conceptualization, Research design, Methodology, Funding acquisition, Writing—review & editing. K.L.: Conceptualization, Research design, Methodology, Data collection, Data curation, Visualization, Writing—review & editing. B.S.W.: Conceptualization, Research design, Methodology, Data collection, Data curation, Writing—review & editing. H.O.: Methodology, Data curation, Writing—review & editing. M.H.M.: Methodology, Genotyping procedure, Writing—review & editing. T.M.: Conceptualization, Research design, Methodology, Writing—review & editing, Supervision.
Corresponding authors
Correspondence to Magdalena Fafrowicz or Anna Ceglarek .
Ethics declarations
Competing interests.
The authors declare no competing interests.
Additional information
Publisher's note.
Springer Nature remains neutral with regard to jurisdictional claims in published maps and institutional affiliations.
Supplementary Information
Supplementary information., rights and permissions.
Open Access This article is licensed under a Creative Commons Attribution 4.0 International License, which permits use, sharing, adaptation, distribution and reproduction in any medium or format, as long as you give appropriate credit to the original author(s) and the source, provide a link to the Creative Commons licence, and indicate if changes were made. The images or other third party material in this article are included in the article's Creative Commons licence, unless indicated otherwise in a credit line to the material. If material is not included in the article's Creative Commons licence and your intended use is not permitted by statutory regulation or exceeds the permitted use, you will need to obtain permission directly from the copyright holder. To view a copy of this licence, visit http://creativecommons.org/licenses/by/4.0/ .
Reprints and permissions
About this article
Cite this article.
Fafrowicz, M., Ceglarek, A., Olszewska, J. et al. Dynamics of working memory process revealed by independent component analysis in an fMRI study. Sci Rep 13 , 2900 (2023). https://doi.org/10.1038/s41598-023-29869-2
Download citation
Received : 30 June 2022
Accepted : 11 February 2023
Published : 18 February 2023
DOI : https://doi.org/10.1038/s41598-023-29869-2
Share this article
Anyone you share the following link with will be able to read this content:
Sorry, a shareable link is not currently available for this article.
Provided by the Springer Nature SharedIt content-sharing initiative
By submitting a comment you agree to abide by our Terms and Community Guidelines . If you find something abusive or that does not comply with our terms or guidelines please flag it as inappropriate.
Quick links
- Explore articles by subject
- Guide to authors
- Editorial policies
Sign up for the Nature Briefing newsletter — what matters in science, free to your inbox daily.

- Open access
- Published: 15 April 2024
Complex PTSD symptom clusters and executive function in UK Armed Forces veterans: a cross-sectional study
- Natasha Biscoe ORCID: orcid.org/0000-0003-3471-6472 1 ,
- Emma New 2 &
- Dominic Murphy ORCID: orcid.org/0000-0002-9530-2743 1 , 3
BMC Psychology volume 12 , Article number: 209 ( 2024 ) Cite this article
Metrics details
Less is known about complex posttraumatic stress disorder (CPTSD) than postrraumatic stress disorder (PTSD) in military veterans, yet this population may be at greater risk of the former diagnosis. Executive function impairment has been linked to PTSD treatment outcomes. The current study therefore aimed to explore possible associations between each CPTSD symptom cluster and executive function to understand if similar treatment trajectories might be observed with the disorder.
A total of 428 veterans from a national charity responded to a self-report questionnaire which measured CPTSD symptom clusters using the International Trauma Questionnaire, and executive function using the Adult Executive Function Inventory. Single and multiple linear regression models were used to analyse the relationship between CPTSD symptom clusters and executive function, including working memory and inhibition.
Each CPTSD symptom cluster was significantly associated with higher executive function impairment, even after controlling for possible mental health confounding variables. Emotion dysregulation was the CPTSD symptom cluster most strongly associated with executive function impairment.
Conclusions
This is the first study to explore the relationship between executive function and CPTSD symptom clusters. The study builds on previous findings and suggests that executive function could be relevant to CPTSD treatment trajectories, as is the case with PTSD alone. Future research should further explore such clinical implications.
Peer Review reports
Military veterans face a greater risk of experiencing PTSD than the general UK population [ 1 ] and are more likely to meet criteria for Complex PTSD (CPTSD) than PTSD [ 2 ]. PTSD encompasses a set of symptoms which may be experienced following a traumatic event, including hyperarousal, re-experiencing (nightmares, intrusions), cognitive and behavioural avoidance and negative alterations in mood (DSM-V; [ 3 ]). CPTSD was added to the International Classification of Diseases in 2011 [ 4 ] as a distinct disorder. A diagnosis of CPTSD includes experiencing clusters of symptoms that encompass PTSD, as well as symptom clusters referred to as Disturbances in Self-Organisation (DSO), which are: emotion dysregulation, interpersonal difficulties, and negative self-concept, as well as functional impairment connected to both PTSD and DSO symptoms.
CPTSD has been linked with sustained and multiple traumas [ 5 ] as well as interpersonal trauma [ 6 ]. Military veterans appear to be at greater risk of CPTSD than PTSD [ 7 ]. Indeed, CPTSD appears to be more prevalent in UK treatment-seeking veterans than PTSD (with 80% meeting criteria for CPTSD compared to 20% for PTSD; [ 2 , 8 ]. Additionally, proportionally higher treatment dropout rates are reported for veterans with CPTSD [ 9 ]. It is therefore clinically important to understand factors which may be relevant to both PTSD and CPTSD, as interventions may need to be tailored to each disorder respectively.
PTSD and executive function
An association between impairments in executive function (EF), and posttraumatic stress disorder (PTSD) is well-established in the literature (for review see: [ 10 , 11 , 12 ]). EFs are a collection of abilities grouped together for their relevance to planning and executing complex, goal-directed behaviour [ 13 , 14 , 15 ]. There is significant variation in both definitions of the concept and how the construct is operationalised, although the current study follows Miyake and colleagues [ 16 ] as this conceptualisation aligns well with the self-report measure of executive function used in this study. These authors identify cognitive flexibility, working memory and inhibition as core EFs, deficits in all of which may be relevant to PTSD [ 17 , 18 , 19 , 20 , 21 ]. Furthermore, one study has reported that greater inhibitory control is associated with a better improvement in PTSD symptoms following psychological treatment, indicating the possible relevance of EF in PTSD recovery trajectories [ 22 ]. Less is known about whether similar trajectories would be observed in those with CPTSD. However, insight may be drawn from neurocognitive explanations of the observed associations between EF and PTSD.
Neurocognitive models of PTSD and EF
Several meta-analyses of lesion and neuroimaging studies implicate the prefrontal cortex (PFC) as key in supporting EF [ 23 , 24 , 25 ]. The PFC has been theorised as a control centre, mediating between sensory inputs and behavioural outputs via regulation of brain systems central to emotion processing such as the amygdala [ 26 ]. The PFC is also structurally associated with PTSD, as well as the amygdala, hippocampus, and cingulate cortex [ 27 ], with this system key to attaching emotional valence to memories relevant to the fear-based experiences that lead to PTSD [ 19 ].
The shared relevance of these brain systems to both EF and PTSD suggests a neurocognitive explanation for the overlap observed between the two constructs. For example, one neurocognitive model of PTSD posits that PFC (and associated deficits in EF) may be ineffectively regulating hyperarousal of the amygdala in individuals with PTSD when a perceive threat is observed in a safe environment [ 28 , 29 , 30 ]. Furthermore, elevated arousal – a symptom of PTSD – may deplete cognitive resources leading to deficits in EF as attention is focused instead on regulating hyperarousal [ 20 , 31 , 32 , 33 ].
EFs and CPTSD
Neuroimaging studies reinforce this theory and suggest functional connectivity between the PFC and brain regions relevant to emotion regulation are key to supporting EF [ 34 , 35 ]. Emotion dysregulation therefore may be pertinent to the observed overlap between PTSD and EF. Given emotion dysregulation is a DSO symptom of CPTSD, exploring associations between CPTSD and EFs could inform understanding of the disorder and how existing PTSD interventions could be tailored to improve treatment response in veterans seeking treatment for CPTSD. In a study using an adolescent sample, deficits in EFs were associated with greater CPTSD severity [ 36 ]. However, less is known about the relationship between CPTSD and EFs in veteran populations.
The current study
Given the potential relevance of EF to PTSD treatment outcomes in veterans, and the need to further understand CPTSD in this population, the current study explores the relationship between both PTSD and CPTSD and a self-report measure of EF (inhibition and working memory) in a clinical sample of UK veterans. Associations between each PTSD symptom cluster and EFs are separately investigated, including the DSO clusters that encompass CPTSD. In line with previous studies [ 36 ], it is hypothesised that lower executive functioning scores (both working memory and inhibition) will be associated with greater severity of CPTSD symptoms.
This study was approved by [blinded for review].
Participants
Of the veterans seeking treatment UK charity, a 20% random sample was selected to assess whether they met study inclusion criteria: (1) having a valid email address; (2) having provided consent to contact from the research team about studies; (3) had attended one or more appointments (classed as treatment-seeking). In total 989 veterans were emailed with the study link, to which 428/989 responded (43.3% response rate; M age =50.4, SD age =10.9). Participation was voluntary. No differences were found between those who returned completed questionnaires and non-responders [ 2 ]. We determined this by analysing predictors of returning a completed survey, including age, sex and service branch.
Eligible and consenting veterans were emailed the link to a self-report questionnaire hosted on Survey Monkey, which included demographic questions and the measures described below. Responses were collected between August and October 2020 and participants were emailed not more than five times. The questionnaire took approximately 20 min to complete. Full study procedure has been described previously [ 2 ].
The Adult Executive Function Inventory (ADEXI; [ 37 ]), measures EF on a 14-item self-report scale, with responses on a five-point Likert scale ranging from zero (definitely not true) to four (definitely true). Items 1, 2, 5, 7, 8, 9, 11, 12 and 13 comprise the working memory subscale, e.g.: “I have difficulty remembering lengthy instructions” and “when someone asks me to do several things, I sometimes only remember the first or last”. The remaining items make up the inhibition subscale, e.g.: “I have a tendency to do things without first thinking about what could happen” and “I sometimes have difficulty stopping myself from doing something that I like even though someone tells me that it is not allowed”. A higher score on the scale or each of the subscales indicates greater impairment. The ADEXI has good internal consistency and test-retest reliability, but poor convergent validity with neuropsychological tests of EF [ 37 ]. The ADEXI has good internal consistency (α = 0.68–0.72; [ 37 ]).
Symptoms of PTSD and CPTSD were measured using the International Trauma Questionnaire [ 38 ], an 18-item scale with responses on a 5-point Likert scale ranging from zero (not at all) to four (extremely). Two items measure each of the three PTSD symptom clusters: hyperarousal, re-experiencing and avoidance. Two items measure each of the three disturbances in self-organisation (DSO) symptom clusters that comprise CPTSD: negative self-concept, interpersonal relationships and affect dysregulation. Three identical items then measure functional impairment related to the PTSD and DSO symptom clusters respectively. The ITQ has strong psychometric properties [ 39 ]. Possible caseness for PTSD is indicated by a score of two or higher on at least one of each item measuring each PTSD symptom cluster, as well scoring two or higher on one of the three functional impairment items relating to PTSD symptom clusters. Possible caseness for CPTSD is indicated by meeting the criteria for PTSD, as well as scoring two or higher on at least one of the two items for each DSO symptom cluster, and at least a two on one of the functional impairment items relating to DSO symptoms. The ITQ has good internal consistency (α = 0.90; [ 39 ]).
Symptoms of generalised anxiety and depression were measured with the General Health Questionnaire (GHQ-12; [ 40 ]), a 12-item scale where a score of four or higher is indicative of potential caseness for common mental health difficulties (CMDs). The GHQ-9 has good internal consistency (α = 0.72; [ 41 ]).
Somatic symptoms were measured using the Patient Health Questionnaire (PHQ-15; [ 41 ]), a 15-item scale where a score above 15 indicates higher severity of somatic symptoms. The PHQ-15 has good internal consistency (α = 0.80; [ 42 ]).
Symptoms of poor sleep quality were measured using the Sleep Condition Indicator (SCI; [ 43 ]), an eight-item scale where a score below 16 is indicative of a potential insomnia disorder. The SCI has good internal consistency (α = 0.86; [ 44 ]).
Symptoms of difficulties with anger were measured using the Dimensions of Anger Reactions (DAR-5; [ 45 ]), a five-item scale where a score higher than 12 is indicative of possible anger difficulties. The DAR-5 has good internal consistency (α = 0.89–0.90; [ 46 ]).
Symptoms of alcohol misuse were measured using the Alcohol Use Disorders Identification Test (AUDIT; [ 47 ]), a 10-item scale where scores higher than eight and 16 respectively are classified as possible hazardous and harmful alcohol use. The AUDIT has good internal consistency (α = 0.60–0.80; [ 48 ]).
Data analysis
Data were prepared in STATA 13.0 and analysed in SPSS v.26. Continuous variables were ADEXI scores and subscale scores. These were averaged so that comparisons could be made across scores calculated from different numbers of items. All other variables were categorical, divided into case and no case or high severity and lower severity for each health outcome, and no PTSD, PTSD, and CPTSD for the ITQ variable. To understand the relationship between mental health variables, including PTSD and EF, single linear regression models were used with demographic and mental health caseness variables as predictors, and ADEXI and inhibition and working memory subscale scores as outcome variables in separate analyses. This was to understand possible confounding variables for any relationship between PTSD and CPTSD with EF. Multiple linear regression models were then used with PTSD and CPTSD caseness as predictor variables, and ADEXI score, and subscale scores as outcome variables. Those variables which were significant in the single linear regression models were included in the multiple regression models to adjust for possible confounding factors. Single linear regression models explored the relationships between individual PTSD and DSO symptom clusters with EF. ‘Caseness’ for each symptom cluster was calculated as a score of two or higher on at least one of the two items measuring each cluster. The sample met assumptions for multiple linear regression: the data were normally distributed (W = 0.96, p = 0.23), there was low multicollinearity and there is a linear relationship between the variables used in the regression models. As described in [ 2 ], analyses were restricted to responders only and missing data were not included in the models due to the assumption that data were missing at random. A power analysis was not conducted for the present study as the analysis was exploratory and data were collected through convenience sampling [ 49 ]. In regression analysis, B values below 0.1. between 0.1 and 0.5 and above 0.5 are broadly considered small, medium and high respectively [ 50 ].
Demographic characteristics are described in Table 1 , as well as descriptive statistics for the variables included in regression models.
Single regression models
Single linear regression models for demographic and mental health factors are presented in Table 2 . Being unemployed and having an ethnicity other than white were significantly associated with higher overall EF, inhibition and working memory impairment. Having high somatic symptoms and meeting caseness for probable common mental health difficulties were also associated with higher overall EF, inhibition and working memory impairment. In addition, scores indicating hazardous alcohol use were associated with working memory and inhibition impairment, and sleep disturbances were associated with a higher working memory impairment.
Multiple regression models
Multiple regression models for PTSD adjusted for all other significant variables besides CPTSD caseness observed in the single regression models. The same models were analysed including CPTSD as a predictor and not PTSD caseness. These models are displayed in Table 3 . Across all adjusted models, both PTSD and CPTSD remained significant predictors for EF, inhibition and working memory.
PTSD and DSO symptom clusters
Linear regression models for each of the PTSD and DSO symptom clusters and EF, inhibition and working memory are displayed in Table 4 . In line with our hypothesis, each symptom cluster was significantly associated with EF, as well as inhibition and working memory subscales.
The aim of the current study was to explore the associations between CPTSD symptom clusters and EF in a clinical sample of UK veterans. Both PTSD and CPTSD caseness were significantly associated with greater impairment in inhibition and working memory, in line with our hypothesis. All PTSD symptom clusters, and the DSO symptom clusters which encompass CPTSD, were associated with inhibition and working memory. In particular, the DSO symptom emotion dysregulation was most strongly associated with EF impairment. PTSD encompasses symptoms hyperarousal, re-experiencing and avoidance. CPTSD is a relatively new separate diagnosis which includes PTSD symptoms as well as DSO symptoms: emotion dysregulation, negative self-concept and interpersonal difficulties, as well as functional impairment relating to these domains [ 4 ].
These associations remained after controlling for the following possible confounders, which were also found to be associated with greater EF impairment: employment status, ethnicity, somatisation severity, common mental health disorders, alcohol misuse and for working memory, sleep function. The finding that EF impairment is associated with worse health coheres with previous research, which has observed relationships between EF deficits and both depression [ 51 ] and somatisation disorder [ 52 ]. Additionally, sleep deprivation is consistently associated with impairments in working memory [ 53 , 54 ].
Emotion dysregulation and EF impairment
Our finding that emotion dysregulation was the CPTSD symptom cluster most associated with EF coheres with and builds on neurocognitive models espoused in the literature. Previous research has suggested functional connectivity between the PFC and limbic system is key in the overlap observed between PTSD chronicity, severity, and EF impairment [ 10 , 55 ]. In one study, those with greater functional connectivity in this system - termed the frontal parietal control and limbic network (FPCN) - were observed to have less chronicity of and greater reduction in PTSD symptoms [ 56 ]. The FPCN underlies emotion processing [ 57 ], mind wandering [ 58 ] and is neurally connected with the default mode network (DMN; [ 59 ]), all of which are associated with PTSD [ 60 ]. Moreover, the development of the DMN is particularly sensitive during childhood, with research suggesting its development could be affected by early and prolonged trauma [ 61 , 62 ]. Given these factors are more strongly associated with CPTSD than PTSD [ 5 ], the finding that DSO symptom cluster emotion dysregulation was most related to EF suggests similar neurobiological mechanisms may be involved in CPTSD as those espoused for the overlap between EF and PTSD.
Limitations
A number of limitations to the present study should be noted. Firstly, whilst the self-report measure of EF facilitated the collection of data from a larger sample, it has limited convergent validity with neuropsychological measures of EF [ 37 ]. However, as a self-report measure, the scale has strong psychometric properties [ 37 ] and self-report EF measures are strongly related to functional impairment [ 63 ]. Secondly, the scale does not include items measuring cognitive flexibility, although this would be difficult to capture on a self-report measure. Data were collected during the Covid-19 pandemic, and environmental factors related to restrictive measures at the time could have affected participants’ responses. However, our research suggests veterans’ mental health difficulties remained relatively stable throughout the pandemic. Finally, no causal relationships can be interpreted from the current findings due to the cross-sectional design of the study. However, the observed finding of an association between DSO symptom clusters and EF impairment builds on previous findings of similar association with PTSD clusters and this can inform future research and clinical studies.
Implications for treatment
Taken together, the findings of the present study suggest that CPTSD interventions may – as observed with PTSD treatment outcomes [ 22 ] – result in better symptom improvement in patients who display greater inhibitory control in neuropsychological tests. By separately analysing both PTSD and DSO symptom clusters, the current study has highlighted the potential role of emotion dysregulation in the overlap between EF impairment and PTSD observed in previous studies [ 10 , 11 , 12 ]. Future research might explore whether veterans with better inhibitory control and working memory respond better to CPTSD interventions. For example, Enhanced Skills Training in Affective and Interpersonal Regulation (ESTAIR; [ 64 ]) is a modular CPTSD treatment which sequentially targets each DSO symptom – including emotion dysregulation. Future studies might explore whether building skills in emotion regulation reduces impairment in EF and subsequently improves recovery trajectories.
This was the first study to explore the relationship between EF and CPTSD symptom clusters in a clinical sample of UK Armed Forces veterans. That DSO symptom clusters, in addition to PTSD clusters, were associated with EF builds on previous findings and suggests that CPTSD treatment outcomes could similarly be affected by levels of EF impairment in veteran patients. Future research should explore the clinical implications of these findings further.
Data availability
The datasets analysed during the current study are not publicly available due to patient confidentiality.
Abbreviations
Complex posttraumatic stress disorder
Default mode network
Disturbances in self-organisation
- Executive function
Posttraumatic stress disorder
Stevelink SAM, Jones M, Hull L, Pernet D, MacCrimmon S, Goodwin L, et al. Mental health outcomes at the end of the British involvement in the Iraq and Afghanistan conflicts: a cohort study. Br J Psychiatry. 2018;213(6):690–7.
Article PubMed PubMed Central Google Scholar
Williamson C, Baumann J, Murphy D. Exploring the health and well-being of a national sample of U.K. treatment-seeking veterans. Psychological Trauma: Theory, Research, Practice, and Policy [Internet]. 2022 Oct 10 [cited 2022 Nov 1]; http://doi.apa.org/getdoi.cfm?doi=10.1037/tra0001356 .
American Psychiatric Association. Diagnostic and statistical manual of mental disorders. 5th ed. Arlington, VA: Author; 2013.
International Classification of Diseases, Eleventh Revision (ICD-11), World Health Organization (WHO) 2019/2021. https://icd.who.int/browse11 .
Murphy D, Karatzias T, Busuttil W, Greenberg N, Shevlin M. ICD-11 posttraumatic stress disorder (PTSD) and complex PTSD (CPTSD) in treatment seeking veterans: risk factors and comorbidity. Soc Psychiatry Psychiatr Epidemiol. 2021;56(7):1289–98.
Article PubMed Google Scholar
Cloitre M, Garvert DW, Brewin CR, Bryant RA, Maercker A. Evidence for proposed ICD-11 PTSD and complex PTSD: a latent profile analysis. Eur J Psychotraumatology. 2013;4(1):20706.
Article Google Scholar
Maercker A, Brewin CR, Bryant RA, Cloitre M, Van Ommeren M, Jones LM, et al. Diagnosis and classification of disorders specifically associated with stress: proposals for ICD-11. World Psychiatry. 2013;12(3):198–206.
Murphy D, Busuttil W. Understanding the needs of veterans seeking support for mental health difficulties. BMJ Mil Health. 2020;166(4):211–3.
Karatzias T, Murphy P, Cloitre M, Bisson J, Roberts N, Shevlin M, et al. Psychological interventions for ICD-11 complex PTSD symptoms: systematic review and meta-analysis. Psychol Med. 2019;49(11):1761–75.
Aupperle RL, Melrose AJ, Stein MB, Paulus MP. Executive function and PTSD: disengaging from trauma. Neuropharmacology. 2012;62(2):686–94.
Vasterling JJ, Brewin CR, editors. Neuropsychology of PTSD: Biological, cognitive, and clinical perspectives. The Guilford; 2005.
Scott JC, Matt GE, Wrocklage KM, Crnich C, Jordan J, Southwick SM, et al. A quantitative meta-analysis of neurocognitive functioning in posttraumatic stress disorder. Psychol Bull. 2015;141(1):105–40.
RepovŠ G, Baddeley A. The multi-component model of working memory: explorations in experimental cognitive psychology. Neuroscience. 2006;139(1):5–21.
Stuss DT, Alexander MP. Executive functions and the frontal lobes: a conceptual view. Psychol Res. 2000;63(3–4):289–98.
Diamond A. Executive functions. Annu Rev Psychol. 2013;64(1):135–68.
Miyake A, Friedman NP, Emerson MJ, Witzki AH, Howerter A, Wager TD. The Unity and Diversity of Executive Functions and their contributions to Complex Frontal Lobe tasks: a latent variable analysis. Cogn Psychol. 2000;41(1):49–100.
Ben-Zion Z, Fine NB, Keynan NJ, Admon R, Green N, Halevi M, et al. Cognitive flexibility predicts PTSD symptoms: observational and interventional studies. Front Psychiatry. 2018;9:477.
Polak AR, Witteveen AB, Reitsma JB, Olff M. The role of executive function in posttraumatic stress disorder: a systematic review. J Affect Disord. 2012;141(1):11–21.
Bremner JD, Southwick SM, Johnson DR, Yehuda R, Charney DS. Childhood physical abuse and combat-related posttraumatic stress disorder in Vietnam veterans. Am J Psychiatry. 1993;150(2):235–9.
Vasterling JJ, Duke LM, Brailey K, Constans JI, Allain AN, Sutker PB. Attention, learning, and memory performances and intellectual resources in Vietnam veterans: PTSD and no disorder comparisons. Neuropsychology. 2002;16(1):5–14.
Vyas K, Murphy D, Greenberg N. Cognitive biases in military personnel with and without PTSD: a systematic review. J Mental Health. 2020;1–12.
Wild J, Gur RC. Verbal memory and treatment response in post-traumatic stress disorder. Br J Psychiatry. 2008;193(3):254–5.
Yuan P, Raz N. Prefrontal cortex and executive functions in healthy adults: a meta-analysis of structural neuroimaging studies. Neurosci Biobehavioral Reviews. 2014;42:180–92.
Buchsbaum BR, Greer S, Chang W, Berman KF. Meta-analysis of neuroimaging studies of the Wisconsin Card‐sorting task and component processes. Hum Brain Mapp. 2005;25(1):35–45.
Rottschy C, Langner R, Dogan I, Reetz K, Laird AR, Schulz JB, et al. Modelling neural correlates of working memory: a coordinate-based meta-analysis. NeuroImage. 2012;60(1):830–46.
Norman DA, Shallice T. Attention to Action: Willed and Automatic Control of Behavior. In: Davidson RJ, Schwartz GE, Shapiro D, editors. Consciousness and Self-Regulation [Internet]. Boston, MA: Springer US; 1986 [cited 2023 Oct 20]. pp. 1–18. http://link.springer.com/ https://doi.org/10.1007/978-1-4757-0629-1_1 .
Morey RA, Haswell CC, Hooper SR, De Bellis MD, Amygdala. Hippocampus, and Ventral Medial Prefrontal Cortex Volumes Differ in Maltreated Youth with and without chronic posttraumatic stress disorder. Neuropsychopharmacol. 2016;41(3):791–801.
Koenigs M, Grafman J. The functional neuroanatomy of depression: distinct roles for ventromedial and dorsolateral prefrontal cortex. Behav Brain Res. 2009;201(2):239–43.
Bremner JD, Bolus R, Mayer EA. Psychometric properties of the early trauma inventory–self report. J Nerv Mental Disease. 2007;195(3):211–8.
Pitman RK, Rasmusson AM, Koenen KC, Shin LM, Orr SP, Gilbertson MW, et al. Biological studies of post-traumatic stress disorder. Nat Rev Neurosci. 2012;13(11):769–87.
Eysenck MW, Derakshan N, Santos R, Calvo MG. Anxiety and cognitive performance: attentional control theory. Emotion. 2007;7(2):336–53.
Falconer E, Bryant R, Felmingham KL, Kemp AH, Gordon E, Peduto A, Olivieri G, Williams LM. The neural networks of inhibitory control in posttraumatic stress disorder. J Psychiatry Neurosci. 2008;33(5):413–22. PMID: 18787658; PMCID: PMC2527717.
PubMed PubMed Central Google Scholar
Etkin A, Gyurak A, O’Hara R. A neurobiological approach to the cognitive deficits of psychiatric disorders. Dialog Clin Neurosci. 2013;15(4):419–29.
Bressler SL, Menon V. Large-scale brain networks in cognition: emerging methods and principles. Trends Cogn Sci. 2010;14(6):277–90.
Gold AL, Morey RA, McCarthy G. Amygdala–Prefrontal Cortex Functional Connectivity during threat-Induced anxiety and goal distraction. Biol Psychiatry. 2015;77(4):394–403.
Shin YJ, Kim SM, Hong JS, Han DH. Correlations between cognitive functions and clinical symptoms in adolescents with Complex post-traumatic stress disorder. Front Public Health. 2021;9:586389.
Holst Y, Thorell LB. Adult executive functioning inventory (ADEXI): validity, reliability, and relations to ADHD. Int J Methods Psych Res. 2018;27(1):e1567.
Cloitre M, Shevlin M, Brewin CR, Bisson JI, Roberts NP, Maercker A, et al. The International Trauma Questionnaire: development of a self-report measure of ICD-11 PTSD and complex PTSD. Acta Psychiatr Scand. 2018;138(6):536–46.
Camden AA, Petri JM, Jackson BN, Jeffirs SM, Weathers FW. A psychometric evaluation of the International Trauma Questionnaire (ITQ) in a trauma-exposed college sample. Eur J Trauma Dissociation. 2023;7(1):100305.
Goldberg DP. General Health Questionnaire-12 [Internet]. American Psychological Association; 2011 [cited 2023 Jan 18]. http://doi.apa.org/getdoi.cfm?doi=10.1037/t00297-000 .
Kim YJ, Cho MJ, Park S, Hong JP, Sohn JH, Bae JN, et al. The 12-Item General Health Questionnaire as an effective Mental Health Screening Tool for General Korean Adult Population. Psychiatry Investig. 2013;10(4):352.
Kroenke K, Spitzer RL, Williams JBW. The PHQ-15: validity of a new measure for evaluating the severity of somatic symptoms. Psychosom Med. 2002;64(2):258–66.
Spitzer RL, Kroenke K, Williams JBW, Löwe B. A brief measure for assessing generalized anxiety disorder: the GAD-7. Arch Intern Med. 2006;166(10):1092.
Espie CA, Kyle SD, Hames P, Gardani M, Fleming L, Cape J. The Sleep Condition Indicator: a clinical screening tool to evaluate insomnia disorder. BMJ Open. 2014;4(3):e004183.
Forbes D, Alkemade N, Mitchell D, Elhai JD, McHugh T, Bates G, et al. UTILITY OF THE DIMENSIONS OF ANGER REACTIONS-5 (DAR-5) SCALE AS a BRIEF ANGER MEASURE: Research Article: utility of DAR-5. Depress Anxiety. 2014;31(2):166–73.
Kim HJ, Lee DH, Kim JH, Kang SE. Validation of the dimensions of anger reactions Scale (the DAR-5) in non-clinical South Korean adults. BMC Psychol. 2023;11(1):74.
Saunders JB, Aasland OG, Babor TF, De La Fuente JR, Grant M. Development of the Alcohol Use disorders Identification Test (AUDIT): WHO Collaborative Project on early detection of persons with harmful alcohol Consumption-II. Addiction. 1993;88(6):791–804.
Noorbakhsh S, Shams J, Faghihimohamadi M, Zahiroddin H, Hallgren M, Kallmen H. Psychometric properties of the Alcohol Use disorders Identification Test (AUDIT) and prevalence of alcohol use among Iranian psychiatric outpatients. Subst Abuse Treat Prev Policy. 2018;13(1):5.
Haile ZT. Power Analysis and Exploratory Research. J Hum Lact. 2023;39(4):579–83.
Aggarwal R, Ranganathan P. Common pitfalls in statistical analysis: Linear regression analysis. Perspect Clin Res. 2017;8(2):100.
Alves M, Yamamoto T, Arias-Carrion O, Rocha N, Nardi A, Machado S, et al. Executive function impairments in patients with Depression. CNSNDDT. 2014;13(6):1026–40.
Trivedi J. Cognitive deficits in psychiatric disorders: current status. Indian J Psychiatry. 2006;48(1):10.
Frenda SJ, Fenn KM. Sleep less, think worse: the effect of sleep deprivation on working memory. J Appl Res Memory Cognition. 2016;5(4):463–9.
Peng Z, Dai C, Ba Y, Zhang L, Shao Y, Tian J. Effect of Sleep Deprivation on the Working Memory-related N2-P3 components of the event-related potential waveform. Front Neurosci. 2020;14:469.
Dunsmoor JE, Cisler JM, Fonzo GA, Creech SK, Nemeroff CB. Laboratory models of post-traumatic stress disorder: the elusive bridge to translation. Neuron. 2022;110(11):1754–76.
Jagger-Rickels A, Rothlein D, Stumps A, Evans TC, Bernstein J, Milberg W, et al. An executive function subtype of PTSD with unique neural markers and clinical trajectories. Transl Psychiatry. 2022;12(1):262.
Dixon ML, De La Vega A, Mills C, Andrews-Hanna J, Spreng RN, Cole MW et al. Heterogeneity within the frontoparietal control network and its relationship to the default and dorsal attention networks. Proc Natl Acad Sci USA [Internet]. 2018 Feb 13 [cited 2023 Oct 23];115(7). https://doi.org/10.1073/pnas.1715766115 .
Kucyi A, Hove MJ, Esterman M, Hutchison RM, Valera EM. Dynamic Brain Network correlates of spontaneous fluctuations in attention. Cereb Cortex. 2016;bhw029.
Kucyi A, Esterman M, Capella J, Green A, Uchida M, Biederman J, et al. Prediction of stimulus-independent and task-unrelated thought from functional brain networks. Nat Commun. 2021;12(1):1793.
Daniels J. Default mode alterations in posttraumatic stress disorder related to early-life trauma: a developmental perspective. J Psychiatry Neurosci. 2011;36(1):56–9.
Fair DA, Cohen AL, Dosenbach NUF, Church JA, Miezin FM, Barch DM, et al. The maturing architecture of the brain’s default network. Proc Natl Acad Sci USA. 2008;105(10):4028–32.
Sherman LE, Rudie JD, Pfeifer JH, Masten CL, McNealy K, Dapretto M. Development of the default Mode and Central Executive Networks across early adolescence: a longitudinal study. Dev Cogn Neurosci. 2014;10:148–59.
Barkley RA, Murphy KR. Impairment in Occupational Functioning and adult ADHD: the predictive utility of executive function (EF) ratings Versus EF tests. Arch Clin Neuropsychol. 2010;25(3):157–73.
Karatzias T, Mc Glanaghy E, Cloitre M. Enhanced skills Training in Affective and Interpersonal Regulation (ESTAIR): a New Modular Treatment for ICD-11 Complex Posttraumatic stress disorder (CPTSD). Brain Sci. 2023;13(9):1300.
Download references
Acknowledgements
The authors have no acknowledgements to declare.
This research was unfunded.
Author information
Authors and affiliations.
Combat Stress, Leatherhead, Surrey, KT22 0BX, UK
Natasha Biscoe & Dominic Murphy
Birmingham and Solihull Mental Health NHS Foundation Trust, Birmingham, UK
King’s Centre for Military Health Research, King’s College London, London, SE5 9PR, UK
Dominic Murphy
You can also search for this author in PubMed Google Scholar
Contributions
DM conceptualised the study and prepared the data. NB analysed the data and drafted the manuscript. EN drafted the manuscript. All authors contributed to manuscript revision.
Corresponding author
Correspondence to Natasha Biscoe .
Ethics declarations
Ethics approval and consent to participate.
Approval for the study was granted by the Combat Stress Research Ethics Committee (ref. pn2020). When providing consent, participants agreed that anonymised survey responses could be used for research. The study was performed in accordance with relevant guidelines and regulations including the Declaration of Helsinki for research with human participants. informed consent was obtained from all participants.
Consent for publication
Not applicable.
Competing interests
The authors declare no competing interests.
Additional information
Publisher’s note.
Springer Nature remains neutral with regard to jurisdictional claims in published maps and institutional affiliations.
Rights and permissions
Open Access This article is licensed under a Creative Commons Attribution 4.0 International License, which permits use, sharing, adaptation, distribution and reproduction in any medium or format, as long as you give appropriate credit to the original author(s) and the source, provide a link to the Creative Commons licence, and indicate if changes were made. The images or other third party material in this article are included in the article’s Creative Commons licence, unless indicated otherwise in a credit line to the material. If material is not included in the article’s Creative Commons licence and your intended use is not permitted by statutory regulation or exceeds the permitted use, you will need to obtain permission directly from the copyright holder. To view a copy of this licence, visit http://creativecommons.org/licenses/by/4.0/ . The Creative Commons Public Domain Dedication waiver ( http://creativecommons.org/publicdomain/zero/1.0/ ) applies to the data made available in this article, unless otherwise stated in a credit line to the data.
Reprints and permissions
About this article
Cite this article.
Biscoe, N., New, E. & Murphy, D. Complex PTSD symptom clusters and executive function in UK Armed Forces veterans: a cross-sectional study. BMC Psychol 12 , 209 (2024). https://doi.org/10.1186/s40359-024-01713-w
Download citation
Received : 23 October 2023
Accepted : 05 April 2024
Published : 15 April 2024
DOI : https://doi.org/10.1186/s40359-024-01713-w
Share this article
Anyone you share the following link with will be able to read this content:
Sorry, a shareable link is not currently available for this article.
Provided by the Springer Nature SharedIt content-sharing initiative
- Mental health
- Complex PTSD
- Emotion dysregulation
BMC Psychology
ISSN: 2050-7283
- General enquiries: [email protected]

An official website of the United States government
The .gov means it’s official. Federal government websites often end in .gov or .mil. Before sharing sensitive information, make sure you’re on a federal government site.
The site is secure. The https:// ensures that you are connecting to the official website and that any information you provide is encrypted and transmitted securely.
- Publications
- Account settings
Preview improvements coming to the PMC website in October 2024. Learn More or Try it out now .
- Advanced Search
- Journal List
- HHS Author Manuscripts

Working memory and short-term memory deficits in ADHD: A bifactor modeling approach
Michael j. kofler.
1 Florida State University, Department of Psychology
Leah J. Singh
Elia f. soto, elizabeth s.m. chan, caroline e. miller, sherelle l. harmon.
2 Harvard University, Department of Psychology
Jamie A. Spiegel
Associated data.
Attention-deficit/hyperactivity disorder (ADHD) has been associated with large magnitude impairments in working memory, whereas short-term memory deficits, when detected, tend to be less pronounced. However, confidence in these findings is limited due to task impurity combined with methodological and statistical limitations of the current evidence base.
A well-characterized, clinically-evaluated sample of 172 children ages 8–13 years ( M =10.30, SD =1.42; 72 girls; 64% White/non-Hispanic) were administered multiple, counterbalanced working memory tests. Bifactor-(s-1) modeling was used to characterize the presence and magnitude of central executive working memory, phonological short-term memory, and visuospatial short-term memory deficits in pediatric ADHD.
ADHD status was associated with very large magnitude impairments in central executive working memory that are present in most pediatric cases ( d =1.63–2.03; 75%−81% impaired), and these deficits covaried with ADHD inattentive and hyperactive/impulsive symptom severity based on both parent and teacher report. There was also evidence for a unique, albeit significantly smaller, impairment in visuospatial short-term memory ( d =0.60; 38% impaired); however, visuospatial short-term memory abilities did not covary with ADHD symptom severity. There was no evidence linking ADHD with phonological short-term memory deficits across either the dimensional or categorical analyses.
These findings provide strong evidence that ADHD is associated with marked central executive working memory deficits that covary with their behavioral symptom presentation across settings. In contrast, visuospatial short-term memory deficits, when present, are likely epiphenomenal, and the most parsimonious conclusion appears to be that phonological short-term memory is intact in pediatric ADHD.
Working memory deficits are present in the majority of children with ADHD and have been shown to covary longitudinally and experimentally with ADHD behavioral symptoms (e.g., Karalunas et al., 2017 ; Kofler et al., 2018 ). In contrast, short-term memory deficits, when detected, tend to be smaller in magnitude, present in a smaller percentage of cases, and show weaker to nonsignificant associations with ADHD symptoms ( Rapport et al., 2013 ; Martinussen et al., 2005 ). However, the extent to which short-term memory abilities are intact in pediatric ADHD remains uncertain due to statistical and methodological limitations that characterize the current evidence base as described below (for review see Gibson et al., 2017, 2018 ). The current study addressed these limitations by applying the bifactor model ( Eid et al., 2018a ) to isolate latent estimates of working memory (i.e., the central executive), phonological short-term memory (storage/rehearsal), and visuospatial short-term memory (storage/rehearsal) and link them with ADHD both categorically and dimensionally in a large and carefully phenotyped sample of children with and without ADHD.
Working Memory Deficits in ADHD
Working memory refers to the active, top-down manipulation of information held in short-term memory, and includes interconnected functions of the mid-lateral prefrontal cortex that guide behavior via the updating, dual-processing, and temporal/sequential manipulation of internally-held information ( Nee et al., 2013 ; Wager & Smith, 2003 ). Among the diverse models of working memory (e.g., Engle et al., 1999 ; Gray et al., 2017; Nee et al., 2015; Wager & Smith, 2003 ), the Baddeley (2007) model has considerable empirical support and has been used extensively in the ADHD literature, where it has proven fruitful for advancing our understanding of both the disorder’s neurocognitive deficits and the limitations of extant ‘working memory’ treatment protocols targeted toward this population (for reviews, please see Kofler et al., 2019 ; Rapport et al., 2013 ). In the Baddeley (2007) model, working memory includes three functionally and anatomically distinct components: (a) the central executive, or ‘working’ component of working memory, which is responsible for acting upon information stored in short-term memory; (b) phonological short-term memory, which is responsible for the temporary storage and rehearsal of language-based, verbal/auditory information; and (c) visuospatial short-term memory, which provides temporary storage/rehearsal of visual and spatial information that cannot be coded verbally. Throughout the manuscript, we use the term ‘working memory’ to refer to the ‘ working ’ (i.e., central executive/active processing) components of the working memory system. Similarly, we use the term ‘short-term memory’ to refer to the ‘ memory ’ (i.e., temporary storage/rehearsal) components of the working memory system.
Working memory deficits are well established in ADHD (for review see Kasper et al., 2012 ); however, there remains significant debate regarding the magnitude of these deficits and the extent to which they reflect underlying mechanisms that produce ADHD behavioral symptoms as opposed to reflecting epiphenomenal symptoms or moderating recovery (for review see Chacko et al., 2014 ). For example, early meta-analytic estimates indicated medium magnitude impairments ( d =0.55–0.63; Willcutt et al., 2005 ), which suggest that only 35%−40% of children with ADHD are likely to have working memory impairments based on converting effect sizes into proportion non-overlap ( Zakzanis, 2001 ). Highly similar results were reported in at least two within-subject heterogeneity studies finding that only approximately 30% of children with ADHD were classified as impaired in working memory ( Coghill, Seth, & Matthews, 2014 ; Wahlstedt, Thorell, & Bohlin, 2009 ). In contrast, more recent meta-analytic evidence indicates very large effect sizes ( d =2.01–2.15 based on meta-regression) that suggest that up to 85% of children with ADHD have working memory deficits ( Kasper et al., 2012 ). Furthermore, highly similar results were reported in recent heterogeneity studies reporting that 62%−85% of children with ADHD were classified as impaired in working memory ( Fosco et al., in press ; Karalunas et al., 2017 ; Kofler, Irwin et al., 2019 ).
As argued by Fosco et al. (in press) , the variability in these reported estimates appears to reflect the challenges inherent in assessing children’s working memory. In particular, studies finding lower effect sizes/impairment estimates were more likely to rely on estimates from single tasks that have been criticized for poor construct validity because they primarily assess short-term memory rather than working memory (e.g., backward digit span; Snyder et al., 2015 ; Wells et al., 2018 ). In contrast, studies finding higher effect sizes and impairment estimates were more likely to use multiple tasks that involve active serial/temporal reordering of information from separate short-term storage/rehearsal modalities to generate latent/composite estimates that control for short-term memory ( Fosco et al., in press ). Because all working memory tasks inherently include demands on one or more short-term memory systems ( Baddeley, 2007 ), failure to control for short-term memory abilities is likely to suppress estimates of working memory deficits to the extent that short-term memory abilities are less- or un-impaired in ADHD as suggested below. The current study addresses this issue via latent estimation of working memory based on multiple, counterbalanced tasks with prominent working memory serial reordering demands but differing short-term memory demands (phonological vs. visuospatial).
Short-Term Memory Deficits in ADHD
Despite recent and compelling evidence that ADHD is associated with impairments in working memory, the extent to which ADHD is associated with impairments in the functionally and anatomically distinct phonological and visuospatial short-term memory systems is less clear. Meta-analytic estimates based primarily on simple span tasks suggest that ADHD may be associated with similar, medium magnitude deficits in phonological and visuospatial short-term memory ( d =0.55 vs. 0.63 in Willcutt et al., 2005 ; d =0.69 vs. 0.74 in Kasper et al., 2012 ) and/or smaller phonological than visuospatial short-term memory deficits ( d =0.47 vs. 0.85 in Martinussen et al., 2005 ), which correspond to impairment rates of 32% to 50% ( Zakzanis, 2001 ). Simple span tasks are designed to primarily assess short-term memory, and typically involve presenting children with a set of numbers, letters, or spatial locations and asking them to repeat the set in either the same or reversed order. 1 However, because ADHD is also associated with impaired working memory processes, these short-term memory estimates may be inflated because even simple span tasks (and potentially all cognitive tasks) require at least some working memory processes associated with controlled attention (e.g., Engle et al., 1999 ).
To more precisely evaluate short-term memory abilities in children with ADHD, several studies have adopted a regression-based (e.g., Rapport et al., 2008 ) or ANCOVA approach (e.g., Dovis et al., 2013 ) that uses phonological and visuospatial working memory tasks to statistically remove variance attributable to the domain-general central executive (working memory) component of the Baddeley (2007) model. These approaches are based on compelling evidence that the working memory system is characterized by a single central executive controller that operates on the functionally and anatomically separate phonological and visuospatial short-term memory subsystems ( Baddeley, 2007 ; Alloway et al., 2006 ; Smith et al., 1996 ). Thus, shared variance across phonological and visuospatial working memory tasks can be attributed to the domain-general central executive (referred to as ‘working memory’ in the current study), whereas unique variance in each task can be attributed to phonological and visuospatial short-term memory, respectively. Using these methods, Rapport et al. (2008) found that ADHD was associated with very large impairments in working memory ( d =2.76), large impairments in visuospatial short-term memory ( d =0.89), and medium impairments in phonological short-term memory ( d =0.55).
However, there are limitations to the use of residual scores for estimating short-term memory components (Gibson et al., 2017, 2018 ). For example, because error variance is retained in the residual but not predicted scores by definition, effect sizes for phonological/visuospatial short-term memory may be deflated relative to working memory. In addition, Gibson et al. (2017) demonstrated that the residual phonological and visuospatial scores will correlate with each other to the same degree as the predicted score estimates of working memory, but in the opposite direction. In other words, the residual phonological and visuospatial short-term memory scores will correlate negatively with each other – a finding that appears contradictory to theory and evidence for their structural and functional independence ( Baddeley, 2007 ; Gibson et al., 2017).
To address this limitation, Gibson et al (2018) used phonological and visuospatial immediate free recall (IFR) tasks, and scored children’s recall based on established primacy and recency effects, to differentiate between performance attributed to primary memory (i.e., short-term memory) and secondary memory (i.e., working memory). Consistent with regression-based methods, they found that children with ADHD displayed large magnitude impairments in working memory ( d =0.73–1.12) but showed small magnitude impairments or did not differ from controls in terms of phonological ( d =0.38) or visuospatial short-term memory ( d =0.29, ns ). However, a primary limitation of this method is that it involves explicitly instructing participants to adopt a specific strategy for completing the task, which may impact children’s performance and produces relatively high rates of excluded participant data (e.g., 21% of the ADHD group in Gibson et al., 2018 ). To address the limitations of both methods for examining short-term memory in ADHD, Gibson et al. (2017, 2018 ) called for a bifactor modeling approach to provide latent estimates that maximally distinguish between primary (short-term memory) and secondary memory (working memory). This is the approach used in the current study. As described below, the bifactor model is particularly well suited for this purpose because it provides latent estimates of our constructs of interest, therefore providing a direct test of the extent to which working memory test scores reflect reliable variance attributable to both domain-general working memory processing and domain-specific phonological and visuospatial short-term memory capacity ( Eid et al., 2018b ).
Current Study
The evidence base at this time suggests that ADHD is associated with large magnitude deficits in working memory but smaller or nonsignificant deficits in phonological and visuospatial short-term memory. However, methodological and statistical issues limit confidence in these findings and no ADHD study to date has maximally fractionated the short-term/working memory system into its functionally and anatomically distinct component processes. The current study is the first to address these limitations and uses bifactor-(s-1) modeling ( Eid et al., 2018b ) with a large and carefully phenotyped sample of children with and without ADHD to characterize the presence and magnitude of working memory, phonological short-term memory, and visuospatial short-term memory deficits in pediatric ADHD. Building on prior work, we hypothesized that (1) ADHD would be associated with large magnitude impairments in latent estimates of working memory, and that these impairments would be significantly larger than those seen for the short-term memory subsystems; (2) visuospatial short-term memory deficits, if present, would be larger than phonological short-term memory deficits in ADHD ( Martinussen et al., 2005 ); and (3) working memory abilities, but not short-term memory abilities, would covary with continuous estimates of parent- and teacher-reported inattentive and hyperactive symptoms ( Chacko et al., 2014 ; Rapport et al., 2013 ).
Open Data and Open Science Disclosure Statement
The de-identified dataset (.jasp), annotated results output (including test statistics), and lavaan analysis scripts are available for peer review: [ https://osf.io/mvkrc/ ]. We report how we determined our sample size, all data exclusions, all manipulations, and all measures in the study (Simmons et al., 2012). The work is part of ongoing clinical research investigating neurocognitive mechanisms underlying pediatric attention and behavioral problems. Performance data on the working memory tasks for subsets of the current sample were included in the datasets used for recent studies to investigate conceptually-distinct hypotheses (Groves et al., 2020). We have not previously applied the bifactor model to these task data or modeled/tested short-term memory deficits in ADHD with any participants in the current sample. To our knowledge, this is the first study in the ADHD literature to use multiple tasks and bifactor modeling to fractionate a key neurocognitive system implicated in ADHD (i.e., working memory) into its component processes.
Participants
The sample included 172 children aged 8–13 years ( M =10.30, SD =1.42; 72 girls) from the Southeastern United States, consecutively recruited through community resources from 2015–2019 ( Table 1 ). IRB approval was obtained/maintained, and all parents and children gave informed consent/assent. Sample ethnicity was mixed with 110 White/Non-Hispanic (64.0%), 20 Hispanic/English-speaking (11.6%), 20 African-American (11.6%), 7 Asian (4.1%), and 15 multiracial children (8.7%).
Sample and Demographic Variables
Working memory task performance is measured in stimuli correct per trial. BASC = Behavior Assessment System for Children. Ethnicity: AA = African American, A = Asian, C = Caucasian Non-Hispanic, H = Hispanic, M = Multiracial. FSIQ = Full Scale Intelligence (WISC-V Short Form), SES = Hollingshead socioeconomic status.
Group Assignment
All children with ADHD and their parents completed a comprehensive psychoeducational and diagnostic evaluation that included a detailed, semi-structured clinical interview using the Kiddie Schedule for Affective Disorders and Schizophrenia for School-Aged Children (K-SADS; Kaufman et al., 1997 ). The K-SADS (2013 Update) allows differential diagnosis according to symptom onset, course, duration, quantity, severity, and impairment in children and adolescents based on DSM-5 criteria ( APA, 2013 ), and was supplemented with parent and teacher ratings from the Behavior Assessment System for Children (BASC-2/3; Reynolds & Kamphaus, 2015 ) and ADHD Rating Scale-4/5 (ADHD-4/5; DuPaul et al., 2016 ). Please see the larger study’s preregistration for a detailed account of the comprehensive psychoeducational evaluation and study procedures ( https://osf.io/abwms ). A psychoeducational report was provided to parents.
Eighty-one children met all of the following criteria and were included in the ADHD group ( n =81; 32% girls): (1) DSM-5 diagnosis of ADHD Combined ( n =62), Inattentive ( n =17), or Hyperactive/Impulsive Presentation ( n =2) by the directing clinical psychologist based on K-SADS; (2) borderline/clinical elevations on at least one parent and one teacher ADHD subscale; and (3) current impairment based on parent report. All ADHD subtypes/presentations were eligible given the instability of ADHD subtypes ( Valo & Tannock, 2010 ). Psychostimulants ( n prescribed =25) were withheld ≥24 hours for testing. To improve generalizability, children with comorbidities were included. Comorbidities reflect clinical consensus best estimates ( Kosten & Rounsaville, 1992 ), and included anxiety (24%), oppositional defiant (10%) 2 , autism spectrum (9%), and depressive (5%) disorders. Positive screens for reading (15%) and math disability (10%) were defined based on score(s) >1.5 SD below age-norms on one or more KTEA-3 Academic Skills Battery reading and math subtests, as specified in DSM-5 ( APA, 2013 ).
The Non-ADHD group comprised 91 consecutive case-control referrals (46 girls) who did not meet ADHD criteria, and included both neurotypical children and children with psychiatric disorders other than ADHD. Neurotypical children (70%) had normal developmental histories and nonclinical parent/teacher ratings and were recruited through community resources. Clinically referred and evaluated children who did not meet ADHD criteria were also included in the Non-ADHD group. These Non-ADHD disorders were included to control for comorbidities in the ADHD group, and included best estimate diagnoses of anxiety (18%), autism spectrum (8%), depressive (3%), and oppositional defiant disorders (1%). None of the clinically-evaluated Non-ADHD cases screened positive for learning disorders in reading; 1 screened positive for a learning disorder in math. The clinically-evaluated Non-ADHD cases did not differ significantly from the ADHD group in the proportion of children diagnosed with anxiety ( p =.45), depression ( p =.59) or ASD ( p =.97); the ADHD group had a higher proportion of ODD cases as expected ( p =.03).
Of the Non-ADHD participants, 51 completed an identical evaluation as the ADHD group. Due to funding constraints, the remaining 40 Non-ADHD participants completed an abbreviated screening evaluation that included parent BASC-3, a 1-subtest IQ screener, and detailed developmental, medical, educational, and psychiatric histories. Neurotypical children did not differ significantly based on whether they received a full or abbreviated evaluation in terms of IQ, gender, ethnicity, age, or BASC hyperactivity T-scores (all p > .28). The abbreviated subgroup had, on average, slightly lower BASC inattention T-scores (M=48.0 vs. 55.3, p = .001) and SES (M=45.5 vs. 53.3, p = .01).
Children were excluded if they presented with gross neurological, sensory, or motor impairment; history of seizure disorder, psychosis, or intellectual disability; or non-stimulant medications that could not be withheld for testing. Additional exclusion criteria were added a priori for the abbreviated evaluation subgroup because we were unable to clinically evaluate these cases: previous diagnosis of ADHD or other psychiatric disorders, or BASC-3 inattention/hyperactivity T-scores > 1.5 SD above the normative sample mean for age and gender.
Working Memory Tasks
Working memory reordering.
The Rapport et al. (2009) computerized working memory tests and their administration instructions are identical to those described in Kofler et al. (2018) . These computerized phonological and visuospatial working memory tasks predict hyperactivity ( Rapport et al., 2009 ), attention ( Kofler et al., 2010 ), impulsivity ( Raiker et al., 2012 ), ADHD diagnostic group membership ( Tarle et al., 2017 ), and ADHD-related functional impairments ( Friedman et al., 2017 ; Kofler et al., 2011 , 2016 ). Reliability and validity evidence includes internal consistency (α=.82-.97; Kofler, Sarver et al., 2018 ), 1- to 3-week test-retest reliability (.76-.90; Sarver et al., 2015 ), and expected magnitude relations with criterion working memory complex span ( r =.69) and updating tasks ( r =.61; Wells et al., 2018 ). Internal consistency in the current sample was .81 (phonological) and .87 (visuospatial). Five practice trials were administered before each task (80% correct required).
Both tasks involve serial reordering of characters presented (numbers, black dot locations), and reordering of a target stimulus (letter, red dot location) into the final serial position. The phonological task involved mentally reordering and verbally recalling a jumbled series of sequentially presented numbers and letters (e.g., 4H62 is correctly recalled as 246H). The visuospatial task involved mentally reordering a sequentially presented series of spatial locations based on what color dot appeared in each location (black dots in serial order, red dot last) and responding on a modified keyboard. Six trials were administered at each set size for each task (3–6 stimuli/trial; 1 stimuli/second; 1 second between the final stimuli and the response phase; 1 second between trials). The 24 total trials per task were randomized, then grouped into 2 blocks of 12 trials each, with short breaks between each block (approximately 1 minute; Kofler et al., 2016 ). Partial-credit unit scoring (stimuli correct per trial) was used to derive performance estimates at each short-term memory load (set sizes of 3 to 6 items per trial) for each task as recommended (8 total variables: phonological memory loads 3–6, visuospatial memory loads 3–6; Conway et al., 2005 ). Higher scores indicate higher accuracy.
Bifactor-(s-1) Models
The bifactor model was selected a priori given our goal of identifying process pure, latent estimates of domain-general working memory and the two domain-specific short-term memory systems (phonological, visuospatial). This decision was guided by the Baddeley (2007) model, which posits a single central executive (working memory) controller that operates on distinct phonological and visuospatial short-term storage buffers. In this model, shared variance across working memory tasks with different stimulus modalities (i.e., phonological vs. visuospatial) is attributed to domain-general working memory (termed the ‘central executive’), whereas unique variance associated with each task is attributed to a domain-specific short-term memory system (termed the phonological and visuospatial ‘storage/rehearsal’ subsystems; for review, see Rapport et al., 2008 ). Support for this model includes replicated evidence that the phonological and visuospatial storage systems are functionally and anatomically distinct, as well as replicated evidence supporting a single, domain-general central executive rather than separate central executive components for processing phonological and visuospatial information ( Baddeley, 2007 ; Alloway et al., 2006 ; Smith et al., 1996 ).
Following recommendations for bifactor models by Eid et al. (2018a ), the current study used a bifactor-(s-1) structure such that all 8 indicators (VS and PH memory set sizes 3, 4, 5, 6) loaded onto a general factor (i.e., working memory) and a subset of indicators also loaded onto each specific short-term memory factor (i.e., phonological or visuospatial). As required to properly fit the bifactor model and interpret the general factor, one or more items must load onto the general factor but not onto any specific factor ( Eid et al., 2018b ). These reference facets serve as markers that define the meaning of the general factor (in this case, working memory). To ensure that the general factor reflected domain-general working memory, we selected 2 reference facets: one phonological and one visuospatial (Heinrich et al., 2018). We chose set size 3 from each task given Baddeley’s (2007) conceptualization that central executive demands remain relatively constant despite increasing set size; increasing set size is viewed as primarily a manipulation of short-term memory demands (see Kofler et al., 2010 for empirical support for this conceptualization in an ADHD sample). Exploratory analyses indicated that the pattern and interpretation of results was unchanged when different combinations of reference facets were selected, suggesting robustness of the findings to this methodological decision.
Importantly for our purposes, the general factor is modeled as uncorrelated with the specific factor(s) in the bifactor model, and the specific factors are also modeled as uncorrelated with each other, based on the underlying assumption that an individual’s score on an item reflects at least two distinct sources of reliable variance (i.e., attributable to the general factor and the specific factor). 3 This model differs from the traditional hierarchical approach, which assumes that the general factor affects the specific factor, which in turn affects the individual item ( Eid et al., 2018a ). Thus, the bifactor-(s-1) model allows maximal discrimination between our constructs of interest, therefore providing a direct test of the extent to which working memory test scores reflect reliable variance attributable to both domain-general central executive processing (working memory) and domain-specific phonological and visuospatial storage/rehearsal (short-term memory) processes ( Eid et al., 2018b ). By fractionating test performance into reliable variance associated with all three primary components of the Baddeley (2007) model, this approach provides the ideal test of the extent to which ADHD is associated with specific short-term memory deficits when accounting for their well-documented impairments in working memory, as recommended (Gibson et al., 2017, 2018 ).
Data Analysis Overview
Analyses were conducted using structural equation modeling (SEM) via the R package lavaan ( Rosseel, 2012 ) as implemented in JASP v.0.10.2 ( JASP Team, 2019 ). The software Omega v2 (Watkins, 2017) was used to assess the multidimensionality, construct reliability and replicability, and explained common variance of the Tier 2 bifactor model. Our primary analyses are organized into two analytic Tiers. In the first Tier, we built the single-factor model (all 8 task performance indicators loading onto a single ‘working memory’ factor). In Tier 2, we added the phonological and visuospatial short-term memory specific factors ( Figure 1 ).

Bifactor-(s-1) model of central executive working memory (general factor) and short-term memory (phonological and visuospatial specific factors). Standardized loadings are shown (all p <.02). Age, gender, and SES are controlled but not depicted for clarity.
Tiers 1 and 2 each included three models: First, we built the short-term/working memory measurement model to evaluate model fit. Second, we tested the extent to which ADHD diagnostic status was associated with impairments in each short-term/working memory component. This structural model involved adding ADHD status (no/yes) to each model and then correlating it with the short-term/working memory factor(s). Because the ADHD grouping variable was dichotomous, we then converted the standardized correlation coefficients ( r ) to Cohen’s d effect sizes ( Hayes, 2009 ) and the proportion of non-overlap ( Zakzanis, 2001 ) to aid interpretation. The ‘proportion of non-overlap’ statistic estimates the proportion of ADHD cases that fall outside of the Non-ADHD range and thus provides an estimate of the percentage of ADHD children with impairments in each short-term/working memory component ( Zakzanis, 2001 ); these estimates tend to align closely with values obtained from within-subject/heterogeneity methods for defining neurocognitive impairment rates in ADHD as seen above.
Third, we replaced the dichotomous ADHD status variable with continuous estimates of parent- and teacher-reported attention problems and hyperactivity/impulsivity (age and gender normed BASC-3 T-scores, allowed to correlate with each other) to probe the extent to which children’s abilities in each short-term/working memory component covary with ADHD symptom severity. We then used the test for dependent correlations as implemented in the R package cocor ( Diedenhofen & Musch, 2015 ) to test whether the associations between each short-term/working memory component and ADHD diagnosis/symptom severity differed significantly in magnitude (e.g., do children with ADHD have larger impairments in working memory than they have in visuospatial short-term memory?).
For all confirmatory models, absolute and relative fit were tested. Adequate model fit is indicated by CFI and TLI ≥ .90, and RMSEA ≤ .10. The χ 2 difference test was used to evaluate nested model fit; lower chi-square values indicate the preferred model ( Satorra & Bentler, 2010 ). Omega total (ω) and omega subscale (ωs) index the reliability of the general factor (working memory) and specific factors (phonological and visuospatial short-term memory) by providing estimates of the proportion of variance attributable to sources of common and specific variance, respectively; values >.70 are preferred ( Rodriguez et al., 2016b ). Explained common variance (ECV) indicates the proportion of reliable variance explained by each factor. The percentage of uncontaminated correlations (PUC) is used to assess potential bias from forcing unidimensional data into a multidimensional model. When general factor ECV > .70 and PUC > .70, bias is considered low and the instrument can be interpreted as primarily unidimensional (i.e., the increased complexity of the bifactor structure is likely not warranted; Rodriguez et al., 2016a ). Construct replicability (H) values > .80 suggest a well-defined latent variable that is more likely to be stable across studies (Watkins, 2017).
All items showed the expected range of scores and were screened for normality (all skewness < |2|; all kurtosis < |1| except phonological set size 3: kurtosis [SE] = 3.05 [0.37]). Delta scaling with maximum likelihood estimation with robust standard errors (MLR) were therefore used to handle non-normality ( Kline, 2016 ). Standardized residuals were inspected for magnitude (all positive and ≤ 1, indicating no evidence of localized ill fit). Directionality of parameter estimates were inspected.
Power Analysis
A series of Monte Carlo simulations were run using Mplus7 ( Muthén & Muthén, 2012 ) to estimate the power of our proposed bifactor models for detecting significant factor loadings of the expected magnitude, given a sample size of 172, power (1- β) ≥ .80, α=.05, and 10,000 simulations per model run. Briefly, this process compiled the percentage of model runs that resulted in statistically significant estimates of model parameters. Standardized factor loadings and expected residual variances for observed variables were imputed iteratively to delineate the proposed bifactor model. For Tier 1 analyses, results indicated that our model is powered to detect standardized factor loadings ≥ .52, which falls well below the loadings for these tasks in previous factor analytic studies (e.g., Kofler, Irwin et al., 2018 ). For the Tier 2 analyses, our model is powered to detect associations of r ≥.24 between ADHD status and each short-term/working memory component. Finally, based on the Rweb quantpsy utility, for α=.05 and 52 degrees of freedom for our most complex model, our N =172 is powered to differentiate between an adequate (RMSEA=.05) and poor fitting model (RMSEA=.10) at power (1- β) = .98. Thus, the study is sufficiently powered to address our primary aims ( Preacher & Coffman, 2006 ).
Preliminary Analyses
All parent and teacher ADHD rating scale scores were higher for the ADHD relative to Non-ADHD group as expected ( Table 1 ). The ADHD group demonstrated impairments on all 8 phonological and visuospatial working memory performance variables ( d = 0.87–1.65; all p <.001). There was no significant evidence to indicate between-group differences in socioeconomic status ( p =.44), whereas the ADHD group was slightly younger (10.1 vs. 10.5; p =.05) and had slightly lower IQ estimates (102.7 vs. 107.7, p =.02). Age, gender, and SES were controlled in all analyses; the pattern and interpretation of results is unchanged if these covariates are removed. 4 IQ was not included as a covariate based on compelling statistical, methodological, and conceptual rationale against covarying IQ when investigating cognitive processes in ADHD ( Dennis et al., 2009 ; Kofler et al., 2016 ). In other words, covarying IQ would preclude conclusions regarding ADHD as a neurodevelopmental disorder by fundamentally changing our grouping variable, and remove significant variance associated with the outcomes of interest ( Ackerman, Beier, & Boyle, 2005 ; Dennis et al., 2009 ).
Tier 1: Working Memory Deficits in ADHD
In Tier 1, all 8 indicators loaded significantly onto the domain-general working memory factor (β=.40-.81, all p <.001) and the model showed adequate fit ( Table 2 ).
Measurement models
Note: Model fits were highly similar for the structural models and are not depicted here but can be found on the study’s OSF site (linked in the Method section), which also presents individual item-factor loadings for all models. Omega (ω) and all columns to its right are only computed for bifactor models. PH = phonological short-term memory, VS = visuospatial short-term memory, WM = working memory (central executive). CFI = comparative fit index, ECV = explained common variance, H = construct replicability, ω = omega total (general WM factor), ωs = omega subscale (specific STM factors), PUC = percent of uncontaminated correlations, RMSEA = root mean square error of approximation, SRMR = standardized root mean square residual, TLI = Tucker-Lewis index.
ADHD diagnostic status
Adding ADHD status (no/yes) to the single-factor model revealed that children with ADHD demonstrated very large magnitude impairments in working memory ( r =.71, p <.001; Table 3 top/right triangle). An r of .71 corresponds to a Cohen’s d of 2.03 and an 81% population non-overlap estimate, suggesting that approximately 81% of children with ADHD have impaired working memory abilities as defined by scores that fall below the Non-ADHD range.
Latent correlations of each short-term/working memory component with ADHD status and continuous ADHD symptoms.
Note: The left/bottom triangle reflects associations with the working memory/short-term memory bifactor-(s−1) model (Tier 2). The top/right triangle reflects associations with the single-factor working memory model (Tier 1). The internal boxes highlight the associations of primary interest in the current study (significant correlations bolded). The correlations between working memory (general factor) and phonological/visuospatial short-term memory (specific factors) are set as .00 as required for bifactor modeling. The specific factors were also modeled as uncorrelated based on theory; exploratory analyses indicated that allowing them to correlate did not improve model fit, Δχ 2 [1]=0.48, p =.49, and as expected that they did not correlate significantly, r =.17, p =.40. ADHD status’s associations with the attention problems/hyperactivity T-scores are based on zero-order correlations (ADHD status was not included in any models with the continuous attention problems/hyperactivity T-scores).
ADHD symptom severity
With continuous estimates of ADHD symptoms added to the model (replacing the dichotomous ADHD status indicator), better developed working memory was associated with lower ADHD inattentive and hyperactive/impulsive symptom severity based on both parent and teacher report ( r = −.25 to −.30, all p <.009; Table 3 top/right triangle).
Tier 2: Short-Term Memory Deficits in ADHD
In Tier 2, we built the working memory/phonological short-term memory/visuospatial short-term memory bifactor-(s-1) model. This involved adding the phonological and visuospatial short-term memory specific factors to the Tier 1 model ( Figure 1 ). Results indicated excellent model fit, all indicators loaded significantly onto their hypothesized factor(s), and model fit was significantly improved relative to the Tier 1 single-factor model (all p <.001; Table 2 ). The proportion of uncontaminated correlations was <.70, supporting the multidimensionality of the data (PUC=.68; Rodriquez et al., 2016; Watkins, 2017). Reliability was high for the general factor (ω=.88) and both specific factors (ωs=.76–84). Total variance explained by the model was 53%, whereas unique (unexplained) variance was 47%, highlighting task impurity and the importance of using multiple indicators for valid assessment of neurocognitive processes (i.e., 47% of the variance in test scores was not attributable to the constructs of interest). The general working memory factor explained 75% of the common variance (ECV=.75) vs. 25% for the specific factors (phonological ECV = .12, visuospatial ECV = .13), indicating that the short-term memory factors explained modest but substantive portions of the reliable variance in task performance (Canivez, 2015).
The association between ADHD and working memory deficits remained very large ( r =.63, p <.001, d =1.63, population nonoverlap=75%; Table 3 bottom/left triangle). In addition, ADHD was associated with a unique impairment in visuospatial short-term memory ( r =.29, p =.004; Cohen’s d =0.60). This effect size suggests that approximately 38% of children with ADHD exhibit impaired visuospatial short-term memory ( Zakzanis, 2001 ). In contrast, there was no evidence for a unique impairment in phonological short-term memory ( r =.14, p =.27; d =0.28, 20% population non-overlap). Results of the tests of dependent correlations revealed that ADHD status showed a significantly higher association with working memory than it did with both phonological and visuospatial short-term memory ( p <.0001), which did not differ ( p =.15; WM>PH=VS).
Similar to the Tier 1 results, better-developed working memory was associated with lower ADHD symptom severity based on both parent and teacher report ( r = −.28 to −.35, all p <.002; Table 3 bottom/left triangle). In contrast, neither phonological short-term memory ( r = −.25 to .26, all p >.10) nor visuospatial short-term memory ( r = −.01 to .12, all p >.30) were associated with ADHD symptom severity based on parent or teacher report. Results of the tests of dependent correlations are as follows: In terms of associations with parent- and teacher-reported hyperactive/impulsive symptoms, working memory showed significantly stronger relations relative to both phonological and visuospatial short-term memory (all p <.004), which did not differ (both p >.18; WM>PH=VS). In terms of associations with parent- and teacher-reported attention problems, visuospatial short-term memory showed significantly weaker relations relative to both working memory and phonological short-term memory (all p <.04); the difference between working memory and phonological short-term memory did not reach significance for parent- ( r = −.28 vs. −.25; p =.77) or teacher-reported attention problems ( r = −.31 vs. −.12; p =.07; WM=PH>VS). 5
Taken together, the Tier 1 and 2 results confirm previous findings of large magnitude working memory deficits in pediatric ADHD ( d =1.62–2.03), with deficits apparent in approximately 75%−81% of these children and evidence that working memory difficulties covary with greater parent- and teacher-reported inattentive and hyperactive/impulsive symptoms. In addition, a sizable minority of children with ADHD (38%; d =0.60) exhibit an additional impairment in visuospatial short-term memory, but these difficulties were not associated with ADHD symptoms based on either parent or teacher report, suggesting that visuospatial short-term memory difficulties are likely epiphenomenal rather than contributory to core ADHD behavioral symptoms. Finally, there was no categorical or dimensional evidence to support a unique impairment in phonological short-term memory.
The current study was the first to apply the bifactor model to the study of short-term and working memory in pediatric ADHD in a large and carefully phenotyped sample of children with and without ADHD. Overall, the current findings indicate large magnitude working memory deficits in pediatric ADHD ( d =1.62–2.03) that covary with both inattentive and hyperactive/impulsive symptom severity, with population nonoverlap estimates suggesting that working memory deficits are present in approximately 75%−81% of these children. These estimates are highly consistent with meta-analytic ‘best case’ estimates based on tasks with high central executive demands ( d =2.01–2.15, 81%−84% impaired; Kasper et al., 2012 ), as well as with studies using latent or composite estimates ( d =1.41–1.67; 62%−85%; Karalunas et al., 2017 ; Kofler, Irwin et al., 2019 ). At the same time, these effect sizes and impairment estimates are significantly higher than omnibus meta-analytic estimates of d =0.55–0.74 ( Kasper et al., 2012 ; Willcutt et al., 2005 ) and impairment estimates of approximately 30% ( Coghill, Seth, & Matthews, 2014 ; Wahlstedt, Thorell, & Bohlin, 2009 ). As argued by Fosco et al. (in press) , the discrepancy between these ranges likely reflects the use of latent/composite estimates vs. relying on single tasks that have been criticized for suboptimal construct validity due to placing insufficient demands on the central executive ‘working’ component of working memory and/or that confound measurement of working memory and short-term memory ( Snyder et al., 2015 ).
Of primary interest in the current study was the extent to which pediatric ADHD is associated with unique impairments in the temporary storage and rehearsal of information (i.e., short-term memory). Understanding the presence and prevalence of short-term memory deficits in ADHD is critical given that they are the primary targets of extant ‘working memory’ training protocols for ADHD (for review see Rapport et al., 2013 ). Previous evidence suggested that short-term memory may be relatively less impaired than working memory in pediatric ADHD and that such deficits, if present, do not appear to covary with ADHD symptom severity (for review, see Chacko et al., 2014 ). However, the veracity of these conclusions was limited due to methodological and statistical limitations with the tasks and methods used to estimate phonological and visuospatial short-term memory in most previous studies (Gibson et al., 2017, 2018 ). By applying the bifactor model ( Eid et al., 2018a ), we were able to address these limitations and fractionate the working memory system to obtain latent, process-pure estimates of both phonological and visuospatial short-term memory.
Interestingly, ADHD appears to be uniquely associated with impairments in visuospatial short-term memory that are present in about 1/3 of pediatric ADHD cases ( d =0.60). This finding is highly consistent with the meta-analytic estimate of d =0.63 for visuospatial working memory obtained primarily from tasks that challenge visuospatial storage/rehearsal ( Willcutt et al., 2005 ), and extends prior findings via improved control for domain-general working memory abilities. Importantly, however, it is unlikely that visuospatial short-term memory deficits, when present, are contributing to the phenotypic expression of ADHD inattentive or hyperactive/impulsive behavioral symptoms. This conclusion is based on the current finding that visuospatial short-term memory was not associated with parent or teacher perceptions of ADHD inattentive or hyperactive/impulsive behavior. Although correlation does not equal causation, it is a necessary prerequisite, and as such the current findings suggest that visuospatial short-term memory deficits are likely epiphenomenal to the inattentive and hyperactive/impulsive behaviors that characterize ADHD ( Coghill et al., 2014 ). To that end, the current findings also suggest that attempts to improve visuospatial short-term memory are unlikely to produce meaningful changes in parent and teacher perceptions of core ADHD behavioral symptoms given that these domains do not appear to be significantly related ( Rapport et al., 2013 ), although of course this conclusion is speculative because the current study did not assess intervention effects. At the same time, it would be premature to conclude that visuospatial short-term memory deficits are unimportant for understanding ADHD. That is, despite its lack of association with core ADHD behavioral symptoms, visuospatial short-term memory deficits may be an important contributor to impairments in peer, family, and/or academic functioning for these children. For example, prior work has linked visuospatial memory with ADHD-related social difficulties ( Kofler et al., 2011 ) and underachievement in reading and math ( Sarver et al., 2012 ); however, the extent to which these associations are attributable specifically to visuospatial short-term memory, as opposed to uncontrolled domain-general working memory processes, remains unknown.
Finally, ADHD does not appear to be associated with a unique deficit in phonological short-term memory. This finding was consistent across categorical and dimensional indicators of ADHD, and suggests that phonological short-term memory is likely intact in most children with ADHD. In this context, it is likely that prior findings of medium magnitude phonological working memory deficits in ADHD ( Kasper et al., 2012 ; Willcutt et al., 2005 ) likely reflect domain-general impairments rather than impairments specific to the phonological system. Given that ADHD has been associated with impairments on tests intended to assess a broad range of constructs ( Willcutt et al., 2005 ), identifying neurocognitive components that are intact in pediatric ADHD is important for improving our understanding of the mechanisms and processes that underlie – and do not underlie – the disorder’s heterogeneous behavioral presentation ( Kofler et al., 2019 ). For example, Raiker and colleagues reported that pediatric ADHD may be associated with a strength in motor speed ( Raiker et al., 2017 ) and intact reward sensitivity ( Tenenbaum et al., 2018 ). Similarly, we have found that ADHD is likely not associated with impairments in set shifting (Irwin et al., 2019), response speeds ( Kofler et al., 2016 ), or episodic buffer processes ( Kofler, Spiegel et al., 2018 ) based in part on a subset of the current sample. In practice, identifying and harnessing these children’s intact cognitive abilities from a strengths-based perspective – in addition to remediating and/or accommodating their deficits – is likely to promote optimal functioning for children with ADHD ( Dvorsky & Langberg, 2016 ).
Limitations
The unique contribution of the current study was its latent assessment of all three primary components of the working memory system in a large and carefully phenotyped sample of children with and without ADHD. Additional strengths of the study include the use of multiple, well-validated tests and bifactor modeling that maximally isolated reliable variance associated with each short-term/working memory component. At the same time, several caveats merit consideration when interpreting results. Our estimates of short-term memory were derived from tasks with prominent executive components (storage + processing). Thus, although results were generally consistent with findings from alternative assessment methods (e.g., simple span, immediate free recall; Dovis et al., 2013 ; Gibson et al., 2018 ), it remains possible that short-term memory deficits would be detected/larger on tasks that require simple storage without active mental manipulation of the internally-stored information, although at the same time such tasks would be expected to significantly underestimate working memory ( Wells et al., 2018 ). Similarly, the current study did not evaluate the ability to maintain information in short-term memory over extended durations ( Bolden et al., 2012 ) or assess the episodic buffer. The episodic buffer was added to the Baddeley (2012) model relatively recently and is responsible for the temporary storage of bound information (e.g., visual + verbal). However, prior work suggests that the episodic buffer is likely intact in pediatric ADHD ( Alderson et al., 2015 ; Kofler, Spiegel et al., 2018 ). Approximately 30% of our ADHD sample was prescribed stimulant medication, which was somewhat lower than epidemiological estimates and may have dampened association magnitudes when juxtaposing short-term/working memory performance obtained off medication with parent/teacher perceptions that may be influenced by medication. Effect sizes for short-term/working memory links with ADHD symptoms may be further blunted by measuring cognitive abilities and overt behaviors in different settings, and these cross-sectional associations disallow causal attributions.
Clinical and Research Implications
Taken together, the current study provides evidence that ADHD is associated with impairments in both working memory and visuospatial short-term memory, but not phonological short-term memory. By addressing statistical limitations associated with previous methods used to estimate these abilities in ADHD, we were able to more precisely isolate performance attributable to each of the three primary components of the Baddeley (2007) model. Importantly, working memory deficits appear to be present in upwards of 3 out of every 4 ADHD cases and covary with their ADHD symptom severity based on both parent and teacher report. Combined with longitudinal and experimental evidence (e.g., Karalunas et al., 2017 ; Rapport et al., 2009 ; Patros et al., 2017 ), the evidence base at this time implicates working memory as a core mechanism that is involved, at least in part, in the phenotypic expression of ADHD behavioral symptoms. In contrast, visuospatial short-term memory deficits were present in fewer ADHD cases and did not covary with ADHD symptom severity. This pattern suggests that visuospatial short-term memory deficits, when present, are likely epiphenomenal to the inattentive and hyperactive/impulsive behaviors that characterize ADHD. When combined with meta-analytic evidence that current ADHD ‘working memory’ training protocols largely target short-term memory as opposed to working memory ( Rapport et al., 2013 ), the current findings provide further insight into why these training protocols fail to reduce ADHD symptomology in well-controlled studies. Nonetheless, future work is needed to determine the extent to which visuospatial short-term memory deficits contribute to the heterogeneous pattern of functional impairments associated with ADHD ( Sarver et al., 2012 ).
Most children with ADHD have impairments in working memory. However, it is unclear whether they also have reduced short-term memory capacities.
Most children with ADHD have intact short-term memory abilities. In contrast, most children with ADHD exhibit marked impairment in working memory, and only working memory abilities are related to ADHD inattentive and hyperactive/impulsive symptom severity.
These findings further implicate working memory deficits in the behavioral symptoms that define ADHD. In contrast, these findings suggest that reduced short-term memory capacity is not a viable candidate for explaining why children with ADHD have difficulties with attention and impulse control/hyperactivity.
Despite not being implicated in core ADHD behavioral symptoms, it is possible that individual differences in short-term memory capacity among children with ADHD may help explain the heterogeneity in functional impairments associated with the disorder.
Supplementary Material
Acknowledgements.
This work was supported in part by NIH grants (R34 MH102499-01, R01 MH115048; PI: Kofler). The sponsor had no role in design and conduct of the study; collection, management, analysis, and interpretation of the data; or preparation, review, or approval of the manuscript.
Conflict of Interest: The authors have no conflicts of interest to report.
Ethical Approval: All procedures performed in studies involving human participants were in accordance with the ethical standards of the institutional and/or national research committee and with the 1964 Helsinki declaration and its later amendments or comparable ethical standards.
Informed Consent: Informed consent was obtained from all individual participants included in the study.
1 Children’s performance when recalling stimuli in the order presented is often interpreted as short-term memory and their performance when recalling stimuli in reverse order is often interpreted as working memory. However, see Wells et al. (2018) for compelling evidence from child, adolescent, and adult clinical and non-clinical samples indicating that a simple reversal of order does not sufficiently engage central executive working memory processes. Please also see Wells et al. (2018) for a discussion of the distinction between reversal and reordering, such that only the latter sufficiently increases central executive demands despite high face validity for assuming they would function similarly.
2 As recommended in the K-SADS, oppositional defiant disorder was diagnosed clinically only with evidence of multi-informant/multi-setting symptoms. ODD comorbidity is 48% in the ADHD group and 16% in the Non-ADHD group based on parent-reported symptom counts.
3 Correlating specific factors with each other is permissible in bifactor-(s-1) models (Heinrich et al., 2018). We decided a priori to model our phonological and visuospatial short-term memory specific factors as uncorrelated based on their structural and functional independence as reviewed above. Exploratory analyses allowing them to covary indicated that they were not significantly correlated, r =.17, p =.40, and that adding this pathway did not improve model fit Δχ 2 [1]=0.48, p =.49.
4 In the Tier 2 models reported below, older age predicted better working memory abilities ( β =.38-.41, p <.001) and slightly lower likelihood of ADHD group membership ( β =.16, p =.03). Male gender predicted greater likelihood of ADHD group membership ( β =.20, p =.008) and better visuospatial short-term memory abilities ( β =.27, p =.02), whereas lower SES predicted less well developed visuospatial short-term memory abilities ( β =.31-.33, p <.001). Age, gender, and SES were not correlated significantly with the BASC-3 age- and gender-normed parent/teacher-reported ADHD symptom severity scores (all p >.07).
5 Exploratory analyses using the ADHD-RS-4/5 ADHD subscale T-scores (Attention Problems, Hyperactivity/Impulsivity) instead of the BASC-2/3 were added during the peer review process. Results for the ADHD symptom severity analyses were highly consistent with those reported in both Tiers 1 and 2. Specifically, working memory correlated significantly with all 4 ADHD-RS-4/5 subscales in Tier 1 ( r = −.19 to −.38, p <.05) and Tier 2 ( r = −.24 to −.41, p <.01). In Tier 2, neither phonological short-term memory ( r = −.23 to .25, all p >.09) nor visuospatial short-term memory ( r = −.02 to .07, all p >.59) were associated with ADHD symptom severity based on parent or teacher report. We also explored the relations between the BASC and ADHD-RS and found that same informant/subscale correlations between the ADHD-RS and BASC in the current study were r = .63-.80 (all p < .001).
- Ackerman PL, Beier ME, & Boyle MO (2005). Working memory and intelligence: The same or different constructs? . Psychological Bulletin , 131 ( 1 ), 30. [ PubMed ] [ Google Scholar ]
- Alderson RM, Kasper LJ, Patros CH, Hudec KL, Tarle SJ, & Lea SE (2015). Working memory deficits in boys with ADHD: An examination of orthographic coding and episodic buffer processes . Child Neuropsychology , 21 ( 4 ), 509–530. [ PubMed ] [ Google Scholar ]
- Alloway TP, Gathercole SE, & Pickering SJ (2006). Verbal and visuospatial short‐term and working memory in children: Are they separable? . Child Development , 77 ( 6 ), 1698–1716. [ PubMed ] [ Google Scholar ]
- APA (2013). Diagnostic and statistical manual of mental disorders (DSM-5) . APA. [ PubMed ] [ Google Scholar ]
- Baddeley A (2007). Working memory, thought, and action . New York: Oxford Press. [ Google Scholar ]
- Baddeley A (2012). Working memory: theories, models, and controversies . Annual Review of Psychology , 63 , 1–29. [ PubMed ] [ Google Scholar ]
- Bolden J, Rapport MD, Raiker JS, Sarver DE, & Kofler MJ (2012). Understanding phonological memory deficits in boys with ADHD: dissociation of short-term storage and articulatory rehearsal processes . Journal of Abnormal Child Psychology , 40 ( 6 ), 999–1011. [ PubMed ] [ Google Scholar ]
- Chacko A, Kofler M, & Jarrett M (2014). Improving outcomes for youth with ADHD: A conceptual framework for combined neurocognitive and skill-based treatment approaches . Clinical Child and Family Psychology Review , 17 ( 4 ), 368–384. [ PMC free article ] [ PubMed ] [ Google Scholar ]
- Coghill DR, Seth S, & Matthews K (2014). A comprehensive assessment of memory, delay aversion, timing, inhibition, decision making and variability in ADHD: advancing beyond the three-pathway models . Psychological Medicine , 44 , 1989–2001. [ PubMed ] [ Google Scholar ]
- Conway ARA, Kane MJ, Bunting MF, Hambrick DZ, Wilhelm O, & Engle RW (2005). Working memory span tasks: A methodological review and user’s guide . Psychonomic Bulletin and Review , 12 ( 5 ), 769–786. [ PubMed ] [ Google Scholar ]
- Dennis M, Francis DJ, Cirino PT, Schachar R, Barnes MA, & Fletcher JM (2009). Why IQ is not a covariate in cognitive studies of neurodevelopmental disorders . Journal of the International Neuropsychological Society , 15 ( 3 ), 331–343. [ PMC free article ] [ PubMed ] [ Google Scholar ]
- Diedenhofen B, & Musch J (2015). cocor: A comprehensive solution for the statistical comparison of correlations . PloS one , 10 ( 4 ), e0121945. [ PMC free article ] [ PubMed ] [ Google Scholar ]
- Dovis S, Van der Oord S, Wiers RW, & Prins PJ (2013). What part of working memory is not working in ADHD? Short-term memory, the central executive and effects of reinforcement . Journal of Abnormal Child Psychology , 41 ( 6 ), 901–917. [ PubMed ] [ Google Scholar ]
- DuPaul GJ, Power TJ, Anastopoulos AD, & Reid R (2016). ADHD rating scale-5 for children and adolescents: Checklists, norms, and clinical interpretation . New York: Guilford. [ Google Scholar ]
- Dvorsky MR, & Langberg JM (2016). A review of factors that promote resilience in youth with ADHD and ADHD symptoms . Clinical Child and Family Psychology Review , 19 ( 4 ), 368–391. [ PubMed ] [ Google Scholar ]
- Eid M, Geiser C, Koch T, & Heene M (2018). Anomalous results in G-factor models: Explanations and alternatives . Psychological Methods , 22 , 541–562. [ PubMed ] [ Google Scholar ]
- Eid M, Krumm S, Koch T, & Schulze J (2018). Bifactor models for predicting criteria by general and specific factors: Problems of nonidentifiability and alternative solutions . Journal of Intelligence , 6 ( 3 ), 42. [ PMC free article ] [ PubMed ] [ Google Scholar ]
- Engle RW, Tuholski SW, Laughlin JE, & Conway ARA (1999). Working memory, short-term memory, and general fluid intelligence: A latent variable approach . Journal of Experimental Psychology: General , 125 , 309–331. [ PubMed ] [ Google Scholar ]
- Fosco WD, Kofler MJ, *Groves NB, *Chan ESB, & Raiker JS (in press). Which ‘working’ components of working memory aren’t working in youth with ADHD? Journal of Abnormal Child Psychology . [ PMC free article ] [ PubMed ] [ Google Scholar ]
- Friedman LM, Rapport MD, Raiker JS, Orban SA, & Eckrich SJ (2017). Reading comprehension in boys with ADHD: The mediating roles of working memory and orthographic conversion . Journal of Abnormal Child Psychology , 45 ( 2 ), 273–287. [ PubMed ] [ Google Scholar ]
- Gibson BS, Gondoli DM, Flies A, Dobrzenski B, & Unsworth N (2010). Application of the dual-component model of working memory to ADHD . Child Neuropsychology , 16 , 60–79. [ PubMed ] [ Google Scholar ]
- Gibson BS, Gondoli DM, Ralph KJ, & Sztybel P (2018). Application of the dual-component model of working memory to ADHD: Greater secondary memory deficit despite confounded cognitive differences . Child Neuropsychology , 24 ( 1 ), 61–81. [ PubMed ] [ Google Scholar ]
- Hayes MH (2009). Statistical digital signal processing and modeling . John Wiley & Sons. [ Google Scholar ]
- JASP Team. (2019). JASP (Version 0.8.2 software) .
- Karalunas SL, Gustafsson HC, Dieckmann N, Tipsord J, Mitchell S, & Nigg JT (2017). Heterogeneity in development of aspects of working memory predicts longitudinal attention deficit hyperactivity disorder symptom change . Journal of Abnormal Psychology , 126 , 774. [ PMC free article ] [ PubMed ] [ Google Scholar ]
- Kasper LJ, Alderson RM, & Hudec KL (2012). Moderators of working memory deficits in children with attention-deficit/hyperactivity disorder (ADHD): a meta-analytic review . Clinical Psychology Review , 32 ( 7 ), 605–617. [ PubMed ] [ Google Scholar ]
- Kaufman J, Birmaher B, Brent D, Rao UMA, Flynn C, Moreci P, … & Ryan N (1997). Schedule for affective disorders and schizophrenia for school-age children-present and lifetime version (K-SADS-PL): initial reliability and validity data . Journal of the American Academy of Child & Adolescent Psychiatry , 36 ( 7 ), 980–988. [ PubMed ] [ Google Scholar ]
- Kline R (2016). Principles and practice of structural equation modeling (4th Ed.).NY: Guilford. [ Google Scholar ]
- Kofler MJ, Irwin LN, Soto EF, Groves NB, Harmon SL, & Sarver DE (2019). Executive functioning heterogeneity in pediatric ADHD . Journal of Abnormal Child Psychology , 47 , 273–86. [ PMC free article ] [ PubMed ] [ Google Scholar ]
- Kofler MJ, Rapport MD, Bolden J, Sarver DE, & Raiker JS (2010). ADHD and working memory: The impact of central executive deficits and exceeding storage/rehearsal capacity on observed inattentive behavior . Journal of Abnormal Child Psychology , 38 , 149–161. [ PubMed ] [ Google Scholar ]
- Kofler MJ, Rapport MD, Bolden J, Sarver DE, Raiker JS, & Alderson RM (2011). Working memory deficits and social problems in children with ADHD . Journal of Abnormal Child Psychology , 39 , 805–817. [ PubMed ] [ Google Scholar ]
- Kofler MJ, Sarver DE, Spiegel JA, Day TN, Harmon SL, & Wells EL (2016). Heterogeneity in ADHD: Neurocognitive predictors of peer, family, and academic functioning . Child Neuropsychology , 23 , 733–759. [ PMC free article ] [ PubMed ] [ Google Scholar ]
- Kofler MJ, Sarver DE, Harmon SL, Moltisanti A, Aduen PA, Soto EF, & Ferretti N (2018). Working memory and organizational skills problems in ADHD. Journal of Child Psychology and Psychiatry , 59 , 57–67. [ PMC free article ] [ PubMed ] [ Google Scholar ]
- Kofler MJ, Spiegel JA, Austin KE, Irwin LN, Soto EF, & Sarver DE (2018). Are episodic buffer processes intact in ADHD? Experimental evidence and linkage with hyperactive behavior . Journal of Abnormal Child Psychology , 46 ( 6 ), 1171–1185. [ PMC free article ] [ PubMed ] [ Google Scholar ]
- Kosten TA, & Rounsaville BJ (1992). Sensitivity of psychiatric diagnosis based on the best estimate procedure . The American Journal of Psychiatry , 149 ( 9 ), 1225. [ PubMed ] [ Google Scholar ]
- Martinussen R, Hayden J, Hogg-Johnson S, & Tannock R (2005). A meta-analysis of working memory impairments in children with attention-deficit/hyperactivity disorder . Journal of the American Academy of Child & Adolescent Psychiatry , 44 ( 4 ), 377–384. [ PubMed ] [ Google Scholar ]
- Muthén LK, & Muthén B (2012). MPlus: statistical analysis with latent variables user’s guide . [ Google Scholar ]
- Nee DE, Brown JW, Askren MK, Berman MG, Demiralp E, … & Jonides J (2013). A meta-analysis of executive components of working memory . Cerebral Cortex , 23 ( 2 ), 264–282. [ PMC free article ] [ PubMed ] [ Google Scholar ]
- Patros CHG, Alderson RM, Hudec KL, Tarle SJ, & Lea SE (2017). Hyperactivity in boys with ADHD: The influence of underlying visuospatial working memory and self-control processes . Journal of Experimental Child Psychology , 154 , 1–12. [ PubMed ] [ Google Scholar ]
- Preacher KJ, & Coffman DL (2006, May). Computing power and minimum sample size for RMSEA [Computer software ]. Available from http://quantpsy.org/ .
- Raiker JS, Rapport MD, Kofler MJ, & Sarver DE (2012). Objectively-measured impulsivity and ADHD: Testing competing predictions from the working memory and behavioral inhibition models of ADHD . Journal of Abnormal Child Psychology , 40 , 699–713. [ PubMed ] [ Google Scholar ]
- Raiker JS, Friedman LM, Orban S, Kofler MJ, Sarver D, & Rapport MD (2017). Phonological working memory deficits in ADHD revisited: The role of lower level information-processing deficits in impaired working memory performance . Journal of Attention Disorders , 1 , 13. [ PubMed ] [ Google Scholar ]
- Rapport MD, Alderson RM, Kofler MJ, Sarver DE, Bolden J, & Sims V (2008). Working memory deficits in boys with ADHD: The contribution of central executive and subsystem processes . Journal of Abnormal Child Psychology , 36 ( 6 ), 825–837. [ PubMed ] [ Google Scholar ]
- Rapport MD, Bolden J, Kofler MJ, Sarver DE, Raiker JS, & Alderson RM (2009). Hyperactivity in boys with ADHD: A ubiquitous core symptom or manifestation of working memory deficits? Journal of Abnormal Child Psychology , 37 , 521–534. [ PubMed ] [ Google Scholar ]
- Rapport MD, Orban SA, Kofler MJ, & Friedman LM (2013). Do programs designed to train working memory, other executive functions, and attention benefit children with ADHD? A meta-analytic review . Clinical Psychology Review , 33 , 1237–1252. [ PubMed ] [ Google Scholar ]
- Reynolds CR, & Kamphaus RW (2015). Behavior assessment system for children–Third Edition (BASC-3) . Bloomington, MN: Pearson. [ Google Scholar ]
- Rodriguez A, Reise SP, & Haviland MG (2016). Evaluating bifactor models: calculating and interpreting statistical indices . Psychological Methods , 21 ( 2 ), 137. [ PubMed ] [ Google Scholar ]
- Rodriguez A, Reise SP, & Haviland MG (2016). Applying bifactor statistical indices in the evaluation of psychological measures . Journal of Personality Assessment , 98 ( 3 ), 223–237. [ PubMed ] [ Google Scholar ]
- Rosseel Y (2012). Lavaan: An R package for structural equation modeling and more. Version 0.5–12 (BETA) . Journal of Statistical Software , 48 ( 2 ), 1–36. [ Google Scholar ]
- Sarver DE, Rapport MD, Kofler MJ, Scanlan SW, Raiker JS, Altro TA, & Bolden J (2012). Attention problems, phonological short-term memory, and visuospatial short-term memory: Differential effects on near-and long-term scholastic achievement . Learning and Individual Differences , 22 , 8–19. [ Google Scholar ]
- Sarver DE, Rapport MD, Kofler MJ, Raiker JS, & Friedman LM (2015). Hyperactivity in ADHD: Impairing deficit or compensatory behavior? Journal of Abnormal Child Psychology , 43 , 1219–1232. [ PubMed ] [ Google Scholar ]
- Satorra A, & Bentler PM (2010). Ensuring positiveness of the scaled difference chi-square test statistic . Psychometrika , 75 ( 2 ), 243–248. [ PMC free article ] [ PubMed ] [ Google Scholar ]
- Simmons JP, Nelson LD, & Simonsohn U (2011). False-positive psychology: Undisclosed flexibility in data collection and analysis allows presenting anything as significant . Psychological Science , 22 ( 11 ), 1359–1366. [ PubMed ] [ Google Scholar ]
- Smith EE, Jonides J, & Koeppe RA (1996). Dissociating verbal and spatial working memory using PET . Cerebral Cortex , 6 ( 1 ), 11–20. [ PubMed ] [ Google Scholar ]
- Snyder HR, Miyake A, & Hankin BL (2015). Advancing understanding of executive function impairments and psychopathology: bridging the gap between clinical and cognitive approaches . Frontiers in psychology , 6 , 328. [ PMC free article ] [ PubMed ] [ Google Scholar ]
- Tarle SJ, Alderson RM, Patros CHG, Lea SE, Hudec KL, & Arrington EF (2017). ADHD and phonological working memory: Methodological variability affects clinical and experimental performance metrics . Neuropsychology , 31 ( 4 ), 383–394. [ PubMed ] [ Google Scholar ]
- Tenenbaum RB, Musser ED, Raiker JS, Coles EK, Gnagy EM, & Pelham WE (2018). Specificity of reward sensitivity and parasympathetic-based regulation among children with ADHD and disruptive behavior disorders . Journal of Abnormal Child Psychology , 46 , 965–77. [ PMC free article ] [ PubMed ] [ Google Scholar ]
- Valo S, & Tannock R (2010). Diagnostic instability of DSM–IV ADHD subtypes: Effects of informant source, instrumentation, and methods for combining symptom reports . Journal of Clinical Child & Adolescent Psychology , 39 ( 6 ), 749–760. [ PubMed ] [ Google Scholar ]
- Wager TD, & Smith EE (2003). Neuroimaging studies of working memory . Cognitive, Affective, & Behavioral Neuroscience , 3 ( 4 ), 255–274. [ PubMed ] [ Google Scholar ]
- Wåhlstedt C, Thorell LB, & Bohlin G (2009). Heterogeneity in ADHD: Neuropsychological pathways, comorbidity and symptom domains . J Abnormal Child Psychology , 37 , 551–564. [ PubMed ] [ Google Scholar ]
- Wells EL, Kofler MJ, Soto EF, Schaefer HS, & Sarver DE (2018). Assessing working memory in children with ADHD: Minor administration and scoring changes may improve digit span backward’s construct validity . Research in developmental disabilities , 72 , 166–178. [ PMC free article ] [ PubMed ] [ Google Scholar ]
- Willcutt E, Doyle A, Nigg J, Faraone S, & Pennington B (2005). Validity of the executive function theory of ADHD: a meta-analytic review . Biological Psychiatry , 57 , 1336–46. [ PubMed ] [ Google Scholar ]
- Zakzanis KK (2001). Statistics to tell the truth, the whole truth, and nothing but the truth: Formulae, illustrative numerical examples, and heuristic interpretation of effect size analyses for neuropsychological researchers . Archives of Clinical Neuropsychology , 16 ( 7 ), 653–667. [ PubMed ] [ Google Scholar ]
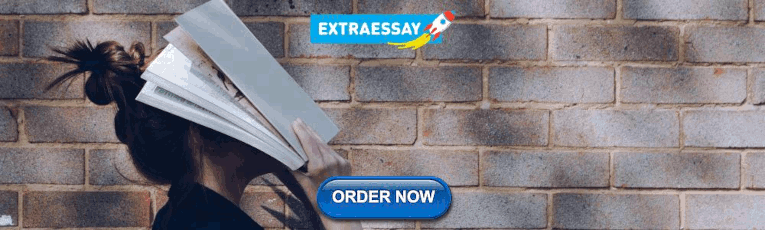
IMAGES
VIDEO
COMMENTS
Introduction. Working memory has fascinated scholars since its inception in the 1960's (Baddeley, 2010; D'Esposito and Postle, 2015).Indeed, more than a century of scientific studies revolving around memory in the fields of psychology, biology, or neuroscience have not completely agreed upon a unified categorization of memory, especially in terms of its functions and mechanisms (Cowan ...
Recent studies suggest that working memory is open to intervention (Diamond, Barnett, Thomas, & Munro, 2007).This raises exciting potential to overcome the limited working memory abilities of at-risk children (Vicari, Caravale, Carlesimo, Casadei, & Allemand, 2004).Nevertheless, questions have been raised about whether working memory training extends outside the laboratory to affect how ...
The Diseased Brain and Working Memory. Age is not the only factor influencing working memory. In recent studies, working memory deficits in populations with mental or neurological disorders were also being investigated (see Table 3).Having identified that the working memory circuitry involves the fronto-parietal region, especially the prefrontal and parietal cortices, in a healthy functioning ...
Early History of Working Memory Research. In 1690, John Locke distinguished between contemplation, or holding an idea in mind, and memory, or the power to revive an idea after it has disappeared from the mind ... the results of working memory studies seem rather replicable, but small differences in method produce large differences in results ...
COGNITIVE MODELS OF WORKING MEMORY. As we write this review the multicomponent model of working memory is marking its 40 th anniversary, and from roughly 1985 through 2005 - what one might consider the first 20 years of the cognitive neuroscience study of working memory - this was the dominant theoretical framework. More recently, however, what might be called state-based models have taken ...
Neurophysiological studies of working memory training in monkeys, however, reveal that training is associated with an increase in prefrontal pyramidal-cell firing rate and a flattening of tuning ...
Summary. Working memory is an aspect of human memory that permits the maintenance and manipulation of temporary information in the service of goal-directed behavior. Its apparently inelastic capacity limits impose constraints on a huge range of activities from language learning to planning, problem-solving, and decision-making.
Previous research has characterized a capacity-limited working memory (WM) store for brief maintenance of information and an unlimited long-term memory (LTM) store for sustained maintenance of ...
Working memory is the active and robust retention of multiple bits of information over the time-scale of a few seconds. It is distinguished from short-term memory by the involvement of executive ...
Recent research on intervention studies targeting working memory using noninvasive brain stimulation has shown promise at enhancing working memory performance in older adults. In particular, transcranial direct current stimulation (tDCS) has demonstrated potential in ameliorating age-related declines on working memory tasks in older adults ...
Working memory. Working memory is primarily associated with the prefrontal and posterior parietal cortex (Sarnthein et al., 1998; Todd and Marois, 2005).Working memory is not localized to a single brain region, and research suggests that it is an emergent property arising from functional interactions between the prefrontal cortex (PFC) and the rest of the brain (D'Esposito, 2007).
I present an account of the origins and development of the multicomponent approach to working memory, making a distinction between the overall theoretical framework, which has remained relatively stable, and the attempts to build more specific models within this framework. I follow this with a brief discussion of alternative models and their relationship to the framework.
Sleep deprivation has been shown to affect working memory first. Previous studies have used the n-back working memory paradigm in participants who underwent sleep deprivation and found that lack of sleep induces a decrease in metabolic activity in the brain's regional network, which is mainly effected information processing and reaction ...
Measuring W orking Memory. Zhisheng (Edward) W en, Alan Juffs, and Paula W inke. Macao Polytechnic Institute / University of Pittsburgh / Michigan State University. [email protected] / juffs ...
Chunking is the recoding of smaller units of information into larger, familiar units. Chunking is often assumed to help bypassing the limited capacity of working memory (WM). We investigate how chunks are used in WM tasks, addressing three questions: (a) Does chunking reduce the load on WM? Across four experiments chunking benefits were found ...
The age-related benchmark findings (Table 3) shed light on the nature of the ageing of working memory. However, most of the studies on ageing of working memory use a cross-sectional design, which ...
A multisensory perspective of working memory. Michel Quak * Raquel Elea London Durk Talsma. Department of Experimental Psychology, Ghent University, Ghent, Belgium. Although our sensory experience is mostly multisensory in nature, research on working memory representations has focused mainly on examining the senses in isolation.
An influential theory of memory known as the multi-store model was proposed by Richard Atkinson and Richard Shiffrin in 1968. This model suggested that information exists in one of 3 states of memory: the sensory, short-term and long-term stores. Information passes from one stage to the next the more we rehearse it in our minds, but can fade ...
The Working Memory Model, proposed by Baddeley and Hitch in 1974, describes short-term memory as a system with multiple components. It comprises the central executive, which controls attention and coordinates the phonological loop (handling auditory information) and the visuospatial sketchpad (processing visual and spatial information).
Previous research has demonstrated that sleep disturbances may lead to psychiatric disorders such as depression, ... The study found that working memory indirectly fosters resilience-enabling behaviors . Given that those students in our study with high GPA had a stronger working memory, it could be that such students are also capable of being ...
Recent studies have provided evidence for the concurrent encoding of sensory percepts and visual working memory contents (VWM) across visual areas; however, it has remained unclear how these two types of representations are concurrently present. Here, we reanalyzed an open-access fMRI dataset where participants memorized a sensory stimulus while simultaneously being presented with sensory ...
Accordingly, the other aim of the present study is to determine if there are diurnal changes in the dynamic of working memory processes. Many studies revealed the time-of-day effects on cognition ...
Participants included 38 children (4 - 13 years old) from Alberta, Canada in two groups: PAE (n = 20) and typically developing (n = 18). Significant improvements in areas of working memory and attentional control for both the PAE and the typically developing group were reported immediately after intervention completion (short-term impact).
Ethics. This study was approved by [blinded for review]. Participants. Of the veterans seeking treatment UK charity, a 20% random sample was selected to assess whether they met study inclusion criteria: (1) having a valid email address; (2) having provided consent to contact from the research team about studies; (3) had attended one or more appointments (classed as treatment-seeking).
Agustina Comedi (Argentina, 1986) is a filmmaker known for her exploration of the ties between archive, memory, and fabulation. In 2017, she premiered her debut film El Silencio es un Cuerpo que Cae which received multiple awards and went on to be screened at over sixty international festivals. Her 2019 film Playback, Ensayo de una Despedida ...
The work is part of ongoing clinical research investigating neurocognitive mechanisms underlying pediatric attention and behavioral problems. Performance data on the working memory tasks for subsets of the current sample were included in the datasets used for recent studies to investigate conceptually-distinct hypotheses (Groves et al., 2020 ...