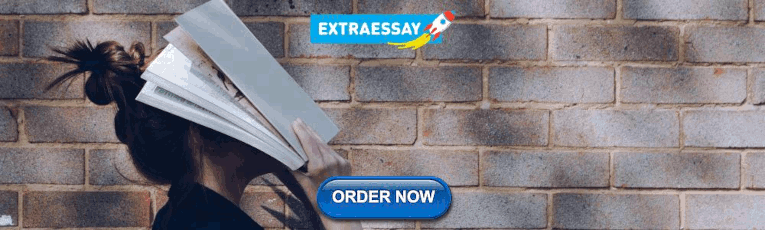
Hypothesis Generation and Interpretation
Design Principles and Patterns for Big Data Applications
- © 2024
- Hiroshi Ishikawa 0
Department of Systems Design, Tokyo Metropolitan University, Hino, Japan
You can also search for this author in PubMed Google Scholar
- Provides an integrated perspective on why decisions are made and how the process is modeled
- Presentation of design patterns enables use in a wide variety of big-data applications
- Multiple practical use cases indicate the broad real-world significance of the methods presented
Part of the book series: Studies in Big Data (SBD, volume 139)
1553 Accesses
This is a preview of subscription content, log in via an institution to check access.
Access this book
- Available as EPUB and PDF
- Read on any device
- Instant download
- Own it forever
- Durable hardcover edition
- Dispatched in 3 to 5 business days
- Free shipping worldwide - see info
Tax calculation will be finalised at checkout
Other ways to access
Licence this eBook for your library
Institutional subscriptions
Table of contents (8 chapters)
Front matter, basic concept.
Hiroshi Ishikawa
Science and Hypothesis
Machine learning and integrated approach, hypothesis generation by difference, methods for integrated hypothesis generation, interpretation, back matter.
- Hypothesis Generation
- Hypothesis Interpretation
- Data Engineering
- Data Science
- Data Management
- Machine Learning
- Data Mining
- Design Patterns
- Design Principles
About this book
The novel methods and technologies proposed in Hypothesis Generation and Interpretation are supported by the incorporation of historical perspectives on science and an emphasis on the origin and development of the ideas behind their design principles and patterns.
Authors and Affiliations
About the author.
He has published actively in international, refereed journals and conferences, such as ACM Transactions on Database Systems , IEEE Transactions on Knowledge and Data Engineering , The VLDB Journal , IEEE International Conference on Data Engineering, and ACM SIGSPATIAL and Management of Emergent Digital EcoSystems (MEDES). He has authored and co-authored a dozen books, including Social Big Data Mining (CRC, 2015) and Object-Oriented Database System (Springer-Verlag, 1993).
Bibliographic Information
Book Title : Hypothesis Generation and Interpretation
Book Subtitle : Design Principles and Patterns for Big Data Applications
Authors : Hiroshi Ishikawa
Series Title : Studies in Big Data
DOI : https://doi.org/10.1007/978-3-031-43540-9
Publisher : Springer Cham
eBook Packages : Computer Science , Computer Science (R0)
Copyright Information : The Editor(s) (if applicable) and The Author(s), under exclusive license to Springer Nature Switzerland AG 2024
Hardcover ISBN : 978-3-031-43539-3 Published: 02 February 2024
Softcover ISBN : 978-3-031-43542-3 Due: 31 January 2024
eBook ISBN : 978-3-031-43540-9 Published: 01 January 2024
Series ISSN : 2197-6503
Series E-ISSN : 2197-6511
Edition Number : 1
Number of Pages : XII, 372
Number of Illustrations : 52 b/w illustrations, 125 illustrations in colour
Topics : Theory of Computation , Database Management , Data Mining and Knowledge Discovery , Machine Learning , Big Data , Complex Systems
- Publish with us
Policies and ethics
- Find a journal
- Track your research
Society Homepage About Public Health Policy Contact
Data-driven hypothesis generation in clinical research: what we learned from a human subject study, article sidebar.
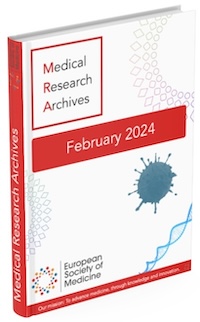
Submit your own article
Register as an author to reserve your spot in the next issue of the Medical Research Archives.
Join the Society
The European Society of Medicine is more than a professional association. We are a community. Our members work in countries across the globe, yet are united by a common goal: to promote health and health equity, around the world.
Join Europe’s leading medical society and discover the many advantages of membership, including free article publication.
Main Article Content
Hypothesis generation is an early and critical step in any hypothesis-driven clinical research project. Because it is not yet a well-understood cognitive process, the need to improve the process goes unrecognized. Without an impactful hypothesis, the significance of any research project can be questionable, regardless of the rigor or diligence applied in other steps of the study, e.g., study design, data collection, and result analysis. In this perspective article, the authors provide a literature review on the following topics first: scientific thinking, reasoning, medical reasoning, literature-based discovery, and a field study to explore scientific thinking and discovery. Over the years, scientific thinking has shown excellent progress in cognitive science and its applied areas: education, medicine, and biomedical research. However, a review of the literature reveals the lack of original studies on hypothesis generation in clinical research. The authors then summarize their first human participant study exploring data-driven hypothesis generation by clinical researchers in a simulated setting. The results indicate that a secondary data analytical tool, VIADS—a visual interactive analytic tool for filtering, summarizing, and visualizing large health data sets coded with hierarchical terminologies, can shorten the time participants need, on average, to generate a hypothesis and also requires fewer cognitive events to generate each hypothesis. As a counterpoint, this exploration also indicates that the quality ratings of the hypotheses thus generated carry significantly lower ratings for feasibility when applying VIADS. Despite its small scale, the study confirmed the feasibility of conducting a human participant study directly to explore the hypothesis generation process in clinical research. This study provides supporting evidence to conduct a larger-scale study with a specifically designed tool to facilitate the hypothesis-generation process among inexperienced clinical researchers. A larger study could provide generalizable evidence, which in turn can potentially improve clinical research productivity and overall clinical research enterprise.
Article Details
The Medical Research Archives grants authors the right to publish and reproduce the unrevised contribution in whole or in part at any time and in any form for any scholarly non-commercial purpose with the condition that all publications of the contribution include a full citation to the journal as published by the Medical Research Archives .
CIAO DATE: 8/00
Case Study Methods in International Political Economy
- AI Case Study Generator
Generate professional and engaging case studies effortlessly with our free AI Case Study creator. Simplify the process and showcase your success.
- Browse all Apps
- Acronym Generator
- Active to Passive Voice Converter
- AI Answer Generator
- AI Essay Generator
- AI Language Translator
- AI Letter Generator
- AI Product Roadmap Generator
- AI Content & Copy Generator
- Amazon Ad Headline Generator
- Amazon Product Bullet Points Generator
- Amazon Product Title Generator
- Article Rewriter
- Author Bio Generator
- Blog Heading Expander
- Blog Outline Generator
- Blog Ideas Generator
- Blog Insights Generator
- Blog Introduction Generator
- Blog Post Generator
- Blog Section Writer
- Blog Title Alternatives Generator
- Blog Title Generator
- Bullet Points Generator
- Bullet Points to Paragraph Generator
- AI Case Study Title Generator
- Checklists Ideas Generator
- Compelling Bullet Points Generator
- Conclusion Generator
- Paragraph Expander
- Content Idea Generator
- Content Readability Improver
- Content Tone Changer
- Courses Ideas Generator
- eBay Ad Headline Generator
- eBay Product Bullet Points Generator
- eBay Product Description Generator
- eBay Product Title Generator
- Ebooks Ideas Generator
- Editorial Calendar Generator
- Essay Title Generator
- Etsy Ad Headline Generator
- Etsy Product Bullet Points Generator
- Etsy Product Description Generator
- Etsy Product Title Generator
- First to Third Person Converter
- Flipkart Ad Headline Generator
- Flipkart Product Bullet Points Generator
- Flipkart Product Description Generator
- Flipkart Product Title Generator
- Headline Generator - 100% Free AI Tool
- Headline Intro Generator
- Hook Generator
- Idiom Explainer
- Local Services Description Generator
- Negative Keyword List Generator
- Pain Agitate Solution Generator
- Paragraph Generator
- Paragraph Shredder
- Paraphrasing Tool (Paraphraser)
- Passive to Active Voice Converter
- Personal Bio Generator (Profile Bio)
- Poll Question and Answers Generator
- Post and Caption Ideas Generator
- Product or Service Generator
- Product Review Generator
- Sentence Rewriter
- Subheadings Generator
- AI Summarizing Tool: Summary Generator
- Synopsis Generator
- Text Extender
- Topic Sentence Generator
- Video Marketing Strategy Generator
- Add Emoji to Text
- Amazon Product Description Generator
- Baby Name Generator: Find the Unique Baby Names
- Chat Message Reply Writer
- Course Description Generator
- Course Name Generator
- Email Response Generator
- Email Subject Line Generator
- Email Writer & Generator
- Excel Formula Generator
- FAQs Generator
- Fitness Exercise & Workout Generator
- Grammar Checker
- Image Generator
- Lyrics Interepreter
- Restaurant Review Generator
- Resume Bullet Points Generator
- Resume Headline Generator
- Resume Objective Generator
- Resume Summary Generator
- Song Lyrics Generator
- Story Plot Generator
- Storyteller | Storymaker
- Text Rewriter
- What to do?
- Facebook Bio Generator
- Facebook Group Post Comment Generator
- Facebook Group Post Generator
- Facebook Hashtag Generator
- Facebook Poll Questions Generator
- Facebook Post Comment Generator
- Facebook Post Generator
- Hacker News Post Comment Generator
- Hacker News Post Generator
- Hashtag Generator
- IndieHackers Post Generator
- IndieHackers Post Comment Generator
- Instagram Bio Generator
- Instagram Caption Generator
- Instagram Hashtag Generator
- Instagram Reels Ideas Generator
- Instagram Reel Script Generator
- Instagram Threads Bio Generator
- Instagram (meta) Threads Generator
- LinkedIn Hashtag Generator
- LinkedIn Poll Questions Generator
- Linkedin Summary Generator
- LinkedIn Experience Description Generator
- Linkedin Headline Generator
- LinkedIn Post Comment Generator
- LinkedIn Post Generator
- LinkedIn Recommendation Generator
- Pinterest Bio Generator
- Pinterest Board Name Generator
- Pinterest Description Generator
- Pinterest Hashtag Generator
- Poem Generator
- Quora Answer Generator
- Quora Questions Generator
- Reddit Post Generator
- Social Media Content Calendar Generator
- TikTok Bio Generator
- TikTok Caption Generator
- TikTok Content Ideas Generator
- TikTok Hashtag Generator
- TikTok Script Generator
- TikTok Ads Generator
- Tweet Generator AI Tool
- Tweet Ideas Generator
- Tweet Reply Generator
- Twitter Bio Generator
- Twitter Hashtag Generator
- Twitter Poll Questions Generator
- Whatsapp Campaign Template
- YouTube Channel Name Generator
- Youtube Hashtag Generator
- Youtube Shorts Ideas Generator
- Youtube Shorts Script Generator
- YouTube Tags Generator
- YouTube Title Generator
- YouTube Video Script Generator
- About Us Page Generator
- Advertisement Script Generator
- Advertising Campaign Generator
- AI Email Newsletter Generator
- AI Marketing Assistant
- AI SWOT Analysis Generator
- AIDA Generator
- Before After Bridge Copy Generator
- Buyer Challenges Generator
- Buyer Persona Generator
- Call to Action Generator
- Content Brief Generator
- Content Calendar Generator
- Digital Marketing Strategy Generator
- Elevator Pitch Generator
- Email Campaign Template
- Podcast Episode Title Generator AI Tool
- Facebook Ads Generator
- Feature Advantage Benefit Generator
- Feature to Benefit Converter
- Glossary Generator
- Go To Market Strategy Generator
- Google Ad Headline Generator
- AI Google Ads Copy Generator
- AI Google Sheets Formula Generator
- Identify Popular Questions
- Landing Page and Website Copies Generator
- Lead Magnet Generator
- LinkedIn Ad Generator
- Listicle Generator
- Marketing Plan Generator
- Marketing Segmentation Generator
- Newsletter Name Generator
- AI Podcast Name Generator Tool
- Podcast Questions Generator
- Customer Persona Generator
- Press Release Ideas Generator
- Press Release Quote Generator
- Press release Writer
- Product Launch Checklist Generator
- Product Name Generator
- AI Q&A Generator
- Questions and Answers Generator
- Reply to Reviews and Messages Generator
- Slide Decks Ideas Generator
- Slideshare presentations Ideas Generator
- SMS Campaign Template
- Survey Question Generator
- Talking Points Generator
- Twitter Ads Generator
- Youtube Channel Description Generator
- Youtube Video Description Generator
- Youtube Video Ideas Generator
- Webinar Title Generator
- Webinars Ideas Generator
- Whitepapers Ideas Generator
- YouTube Video Topic Ideas Generator
- Keyword Research Strategies Generator
- Keywords Extractor
- Keywords Generator
- Long Tail Keyword Generator
- Meta Description Generator
- SEO Meta Title Generator
- SEO Topical Map Generator
- Sales & Cold Calling Script Generator
- Content Comparison Generator
- AI Follow-Up Email Generator
- Icebreaker Generator
- LinkedIn Connection Request Generator
- LinkedIn Followup Message Generator
- LinkedIn Inmail Generator
- LinkedIn Message Generator
- Pain Point Generator
- Proposal Generator
- Sales Qualifying Questions Generator
- AI Sales Email Generator
- Sales Pitch Deck Generator
- Voice Message Generator
- Closing Ticket Response Writer
- Request for Testimonial Email Generator
- Support Ticket Auto Reply Writer
- AI Support Ticket Explainer
- Support Ticket Reply Writer
- Ticket Resolution Delay Response Writer
- Billing Reminder Email Writer
- Customer Contract Summarizer
- Customer QBR Presentation Writer
- Business Meeting Summary Generator
- Monthly Product Newsletter Writer
- Product Questions for Customer Generator
- AI Backlink Outreach Email
- Business Name Generator
- Brand Story Generator
- Business idea pitch generator
- Business ideas generator
- Company Slogan Generator
- Core Values Generator
- AI Customer Retention Strategy Generator
- Domain Name Generator
- Event Description generator
- Event ideas generator
- Metaphor Generator
- Micro SaaS ideas Generator
- Project Plan Generator
- Startup ideas Generator
- Vision and Mission Generator
- Product Description Generator AI Tool
- Product Description with Bullet Points Generator
- SMS and Notifications Generator
- Tagline and Headline Generator
- Testimonial and Review Generator
- Value Prop Generator
- Convincing Premium
- Critical Premium
- Funny Premium
- Humble Premium
- Humorous Premium
- Informative Premium
- Inspirational Premium
- Joyful Premium
- Passionate Premium
- Thoughtful Premium
- Urgent Premium
- Worried Premium
- English (US)
- German (Germany)
- Italian (Italy)
- Japanese (Japan)
- Russian (Russia)
- Portuguese (Portugal)
- Hindi (India)
- Urdu (Pakistan)
- Arabic (Saudi Arabia)
- French (France)
- English (UK) Premium
- English (Australia) Premium
- English (Canada) Premium
- English (India) Premium
- English (Singapore) Premium
- English (New Zealand) Premium
- English (South Africa) Premium
- Spanish (Spain) Premium
- Spanish (Mexico) Premium
- Spanish (United States) Premium
- Arabic (Egypt) Premium
- Arabic (United Arab Emirates) Premium
- Arabic (Kuwait) Premium
- Arabic (Bahrain) Premium
- Arabic (Qatar) Premium
- Arabic (Oman) Premium
- Arabic (Jordan) Premium
- Arabic (Lebanon) Premium
- Danish (Denmark) Premium
- German (Switzerland) Premium
- German (Austria) Premium
- French (Canada) Premium
- French (Switzerland) Premium
- French (Belgium) Premium
- Italian (Switzerland) Premium
- Dutch (Netherlands) Premium
- Dutch (Belgium) Premium
- Portuguese (Brazil) Premium
- Chinese (China) Premium
- Chinese (Taiwan) Premium
- Chinese (Hong Kong) Premium
- Chinese (Singapore) Premium
- Korean (South Korea) Premium
- Finnish (Finland) Premium
- Greek (Greece) Premium
- Czech (Czech Republic) Premium
- Swedish (Sweden) Premium
- Norwegian (Norway) Premium
- Turkish (Turkey) Premium
- Polish (Poland) Premium
- Romanian (Romania) Premium
- Hungarian (Hungary) Premium
- Thai (Thailand) Premium
- Hebrew (Israel) Premium
- Indonesian (Indonesia) Premium
- Vietnamese (Vietnam) Premium
- Malay (Malaysia) Premium
- Tagalog (Philippines) Premium
- Swahili (Kenya) Premium
- Swahili (Tanzania) Premium
- Zulu (South Africa) Premium
- Xhosa (South Africa) Premium
- Amharic (Ethiopia) Premium
- Tamil (India) Premium
- Tamil (Sri Lanka) Premium
- Bengali (Bangladesh) Premium
- Bengali (India) Premium
- Punjabi (Pakistan) Premium
- Punjabi (India) Premium
- Marathi (India) Premium
- Telugu (India) Premium
- Kannada (India) Premium
- Gujarati (India) Premium
- Oriya (India) Premium
- Malayalam (India) Premium
- Urdu (India) Premium
- Persian (Iran) Premium
- Azerbaijani (Azerbaijan) Premium
- Ukrainian (Ukraine) Premium
- Belarusian (Belarus) Premium
- Catalan (Spain) Premium
- Basque (Spain) Premium
- Galician (Spain) Premium
- Slovak (Slovakia) Premium
- Lithuanian (Lithuania) Premium
- Latvian (Latvia) Premium
- Estonian (Estonia) Premium
- Bulgarian (Bulgaria) Premium
- Albanian (Albania) Premium
- Croatian (Croatia) Premium
- Slovenian (Slovenia) Premium
- Bosnian (Bosnia and Herzegovina) Premium
- Serbian (Serbia) Premium
- Macedonian (North Macedonia) Premium
- Montenegrin (Montenegro) Premium
- Maltese (Malta) Premium
- Irish (Ireland) Premium
- Welsh (United Kingdom) Premium
- Scots Gaelic (United Kingdom) Premium
- Icelandic (Iceland) Premium
- Luxembourgish (Luxembourg) Premium
- Afrikaans (South Africa) Premium
- Hausa (Nigeria) Premium
- Yoruba (Nigeria) Premium
- Somali (Somalia) Premium
- Tigrinya (Eritrea) Premium
- Kinyarwanda (Rwanda) Premium
- Sesotho (Lesotho) Premium
- Shona (Zimbabwe) Premium
- Sinhala (Sri Lanka) Premium
- Dhivehi (Maldives) Premium
- Burmese (Myanmar) Premium
- Lao (Laos) Premium
- Khmer (Cambodia) Premium
- Mongolian (Mongolia) Premium
- Tibetan (China) Premium
- Uighur (China) Premium
- Pashto (Afghanistan) Premium
- Dari (Afghanistan) Premium
- Nepali (Nepal) Premium
- Dzongkha (Bhutan) Premium
- Sesotho (South Africa) Premium
- Setswana (Botswana) Premium
- Seselwa Creole (Seychelles) Premium
- Mauritian Creole (Mauritius) Premium
- Haitian Creole (Haiti) Premium
- Greenlandic (Greenland) Premium
- Faroese (Faroe Islands) Premium
- Samoan (Samoa) Premium
- Tongan (Tonga) Premium
Popular Writing Apps
Case study generator.
Unlock the power of our case study creator tool—Generate compelling case studies effortlessly with our creator and captivate your audience. With just a few clicks, our smart technology helps you understand data, find trends, and make insightful reports, making your experience better and improving your SEO strategy.
What is a Case Study
A case study is like a detailed story that looks closely at a particular situation, person, or event, especially in the business world. It's a way to understand how things work in real life and learn valuable lessons. For instance, if a business wanted to figure out how another one became successful, they might study that business as a case study.
Let's say there's a small company that started selling handmade products online and became successful. A case study about this business could explain the challenges they faced, the strategies they used to grow, and the results they achieved. By reading this case study, other businesses could learn useful tips and apply them to their situations to improve and succeed.
7 Tips For Writing Great Case Studies
- Pick a Familiar Topic: Choose a client or project that your audience can relate to. This makes it easier for them to see how your solutions might work for their situations.
- Clear Structure: Start with a concise introduction that sets the stage for the case study. Clearly outline the problem, solution, and results to make your case study easy to follow.
- Engaging Storytelling: Turn your case study into a compelling narrative. Use real-world examples, anecdotes, and quotes to make it relatable and interesting for your audience.
- Focus on the Problem: Clearly define the problem or challenge your case study addresses. This helps readers understand the context and sets the foundation for the solution.
- Highlight Solutions: Showcase the strategies or solutions implemented to overcome the problem. Provide details about the process, tools used, and any unique approaches that contributed to the success.
- Optimize for SEO: By incorporating your case study into a blog post using a blog post generator, you enhance its visibility and reach. This, in turn, improves the search engine rankings of your blog post, attracting more organic traffic.
- Quantify Results: Use data and metrics to quantify the impact of your solutions. Whether it's increased revenue, improved efficiency, or customer satisfaction, concrete results add credibility and demonstrate the value of your case study.
What is a Case Study Creator
A free case study generator is a tool or system designed to automatically create detailed case studies. It typically uses predefined templates and may incorporate artificial intelligence (AI) to generate comprehensive analyses of specific situations, events, or individuals.
This tool streamlines the process of crafting informative case studies by extracting key details, analyzing data, and presenting the information in a structured format.
Case study generators are valuable for businesses, students, or professionals seeking to efficiently produce well-organized and insightful case studies without the need for extensive manual effort.
Benefits of Using Case Study Generator
In today's competitive landscape, showcasing your product or service successes is vital. While case studies offer a compelling way to do this, starting from scratch can be time-consuming. That's where case study generators step in, providing a robust solution to streamline the process and unlock various advantages.
- Easy and Quick: A case study generator makes it simple to create detailed studies without spending a lot of time. It's a fast and efficient way to compile information.
- Accessible Online: As an online case study generator, you can use it from anywhere with an internet connection. No need for installations or downloads.
- Free of Cost: Many case study creators are free to use, eliminating the need for any financial investment. This makes it budget-friendly for businesses or individuals.
- AI-Powered Insights: Some generators use AI (artificial intelligence) to analyze data and provide valuable insights. This adds depth and accuracy to your case studies.
- Save Time and Effort: Generate a polished case study in minutes, automating tasks like data analysis and content creation. This frees up your time to focus on other aspects of your business.
- Enhance Quality and Consistency: Case study creators offer templates and AI-powered suggestions, ensuring your studies are well-structured and visually appealing. Consistent quality strengthens your brand image.
- Improve Brand Awareness and Credibility: Sharing case studies on your platforms increases brand awareness and builds trust. Positive impacts on others establish you as a credible provider.
- Boost Lead Generation and Sales: Compelling case studies build trust and showcase your value, attracting leads and converting them into customers, ultimately boosting your sales.
- Increase Customer Engagement and Loyalty: Case studies provide insights into your company, fostering deeper connections, increasing engagement, and promoting long-term loyalty.
- Improve Your Writing Skills: Free AI Case study generators act as learning tools, offering guidance on structure, content, and storytelling. Studying generated drafts refines your writing skills for crafting impactful case studies in the future.
How AI Case Study Generator Works
An online case study generator works by leveraging artificial intelligence algorithms to analyze and synthesize information, creating comprehensive case studies. Here's a simplified explanation of its functioning:
Data Input:
Algorithm analysis:, content generation:, language processing:, who needs a case study creator.
Anyone looking to create informative and detailed case studies can benefit from using an online case study generator. This tool is useful for
Businesses:
Professionals:, individuals:, marketing professionals:, researchers:, why opt for our case study creator.
Are you on the lookout for a top-notch case study generator that combines outstanding features with user-friendliness, all at no cost and without the need for registration? Your search ends here. Our AI-driven case study generator is the ideal solution for you. Here's why you should choose our tool:
Craft Case Study in 50+ Languages:
Incorporate keywords in case study:, user-friendly interface:, 100% free, no registration:, 20+ diverse tones for versatile styles:, how much does your case study creator cost, do i need any writing experience to use a case study generator, what types of case studies can i create with a case study creator, what are some common mistakes people make when creating case studies.
- Not focusing on the benefits to the reader.
- Not using data and results to support their claims.
- Not telling a compelling story.
- Not using visuals effectively.
- Not promoting their case study.
Can I customize the generated case study?
Is the generated content unique.
Results from a hypothesis generating case-control study: herpes family viruses and schizophrenia among military personnel
Affiliation.
- 1 Division of Preventive Medicine, Department of Epidemiology, Walter Reed Army Institute of Research, Silver Spring, MD 20901, USA. [email protected]
- PMID: 18156638
- PMCID: PMC2632504
- DOI: 10.1093/schbul/sbm139
Background: Herpes family viruses can cause central nervous system inflammatory changes that can present with symptoms indistinguishable from schizophrenia and therefore are of interest in schizophrenia research. Most existing studies of herpes viruses have used small populations and postdiagnosis specimens. As part of a larger research program, we conducted a hypothesis-generating case-control study of selected herpes virus antibodies among individuals discharged from the US military with schizophrenia and pre- and postdiagnosis sera.
Methods: Cases (n = 180) were servicemembers hospitalized and discharged from military service with schizophrenia. Controls, 3:1 matched on several factors, were members not discharged. The military routinely collects and stores members' serum specimens. We used microplate enzyme immunoassay to measure immunoglobulin G (IgG) antibody levels to 6 herpes viruses in pre- and postdiagnosis specimens. Conditional logistic regression was used, and the measure of association was the hazard ratio (HR).
Results: Overall, we found a significant association between human herpes virus type 6 and schizophrenia, with an HR of 1.17 (95% confidence interval [CI] = 1.04, 1.32). Women and blacks had significant negative associations with herpes simplex virus type 2 and cytomegalovirus; among blacks, there was a significant positive association with herpes simplex virus type 1. Among men, there was a HHV-6 temporal effect with an HR of 1.41 (95% CI = 1.02, 1.96) for sera drawn 6-12 months before diagnosis.
Discussion: Findings from previous studies of herpes family viruses and schizophrenia have been inconsistent. Our study is based on a larger population than most previous studies and used serum specimens collected before onset of illness. This study adds to the body of knowledge and provides testable hypotheses for follow-on studies.
Publication types
- Research Support, Non-U.S. Gov't
- Research Support, U.S. Gov't, Non-P.H.S.
- Antibodies, Viral / blood*
- Black People / psychology
- Black People / statistics & numerical data
- Case-Control Studies
- Cytomegalovirus / immunology
- Encephalitis, Herpes Simplex / ethnology
- Encephalitis, Herpes Simplex / immunology
- Encephalitis, Herpes Simplex / psychology
- Herpesviridae / immunology*
- Herpesvirus 1, Human / immunology
- Herpesvirus 2, Human / immunology
- Herpesvirus 3, Human / immunology
- Herpesvirus 4, Human / immunology
- Herpesvirus 6, Human / immunology
- Immunoglobulin G / blood
- Military Personnel / psychology*
- Military Personnel / statistics & numerical data
- Schizophrenia / ethnology
- Schizophrenia / immunology*
- Schizophrenia / virology
- Sex Factors
- White People / psychology
- White People / statistics & numerical data
- Young Adult
- Antibodies, Viral
- Immunoglobulin G

A .gov website belongs to an official government organization in the United States.
A lock ( ) or https:// means you've safely connected to the .gov website. Share sensitive information only on official, secure websites.
- Signs and Symptoms
- How It Spreads
- Shigella Surveillance
- Publications
- Clinical Overview
- Clinical Care and Treatment
- Guidance for Health Departments
- Shigella Prevention and Control Toolkit
- National Shigella surveillance data are collected through passive surveillance of laboratory-confirmed human Shigella infections.
- Clinical diagnostic laboratories submit Shigella isolates to state and territorial public health laboratories, where they are confirmed, speciated, and subtyped.
- Unusual or untypable isolates are forwarded to CDC; results are then reported back to public health laboratories.
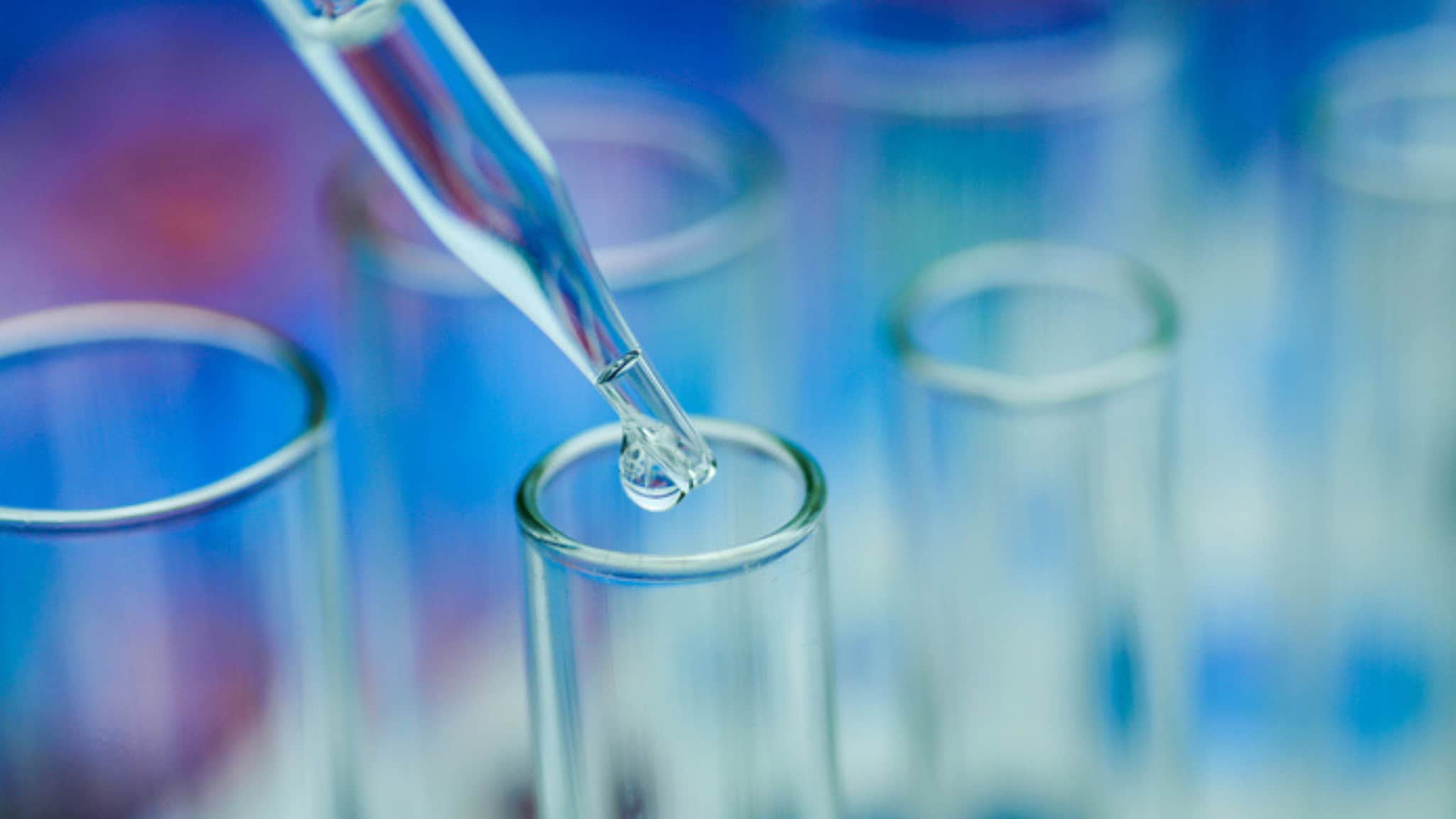
Surveillance overview
State and territorial public health laboratories report Shigella infections electronically to CDC through a variety of mechanisms. Data are collected into the Laboratory-Based Enteric Disease Surveillance (LEDS) system. The annual summaries of these data are the national source of species and subtype information for Shigella .
National Shigella Surveillance Overview [PDF – 2 pages]
Shigella Hypothesis Generating Questionnaire
Download questionnaire:
English [PDF - 8 pages]
Spanish [PDF - 9 pages]
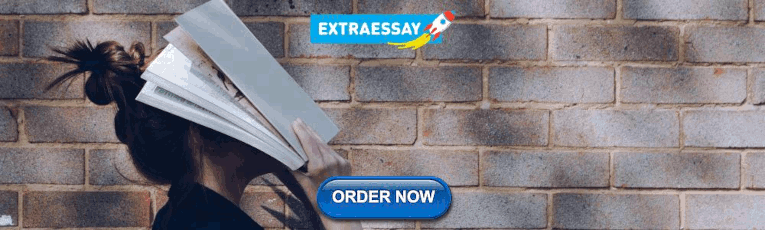
Annual Summaries
- 2016 Summary [PDF – 18 pages]
- 2015 Summary [PDF – 18 pages]
- 2014 Summary [PDF – 18 pages]
- 2013 Summary [PDF – 18 pages]
- 2012 Summary [PDF – 9 pages] , Appendix [PDF – 8 pages]
- 2011 Summary [PDF – 9 pages] , Appendix [PDF – 8 pages]
- 2010 Summary [PDF – 10 pages] , Appendix [PDF – 8 pages]
- 2009 Summary [PDF – 3 pages] , Summary Tables [PDF – 23 pages]
- 2008 Summary [PDF – 3 pages] , Summary Tables [PDF – 21 pages]
- 2007 Summary [PDF – 3 pages] , Summary Tables [PDF – 21 pages]
Shigella - Shigellosis
Shigella bacteria cause an infection called shigellosis that can spread easily from one person to another.
For Everyone
Health care providers, public health.

Outbreak Toolkit
Hypothesis generation, on this page, questionnaires and interviewing, exposure analysis.
Generating hypotheses is an important, but often challenging, step in an outbreak investigation . When generating hypotheses, it is best to keep an open mind and to cast a wide net. A good starting place would be to identify exposures that have been previously been associated with the pathogen under investigation. This can be done by:
- searching an outbreak database such as Outbreak Summaries , the Marler-Clark database, and the CDC Foodborne Outbreak Online database (see Tools for links to these databases)
- reviewing the published literature using a search engine such as PubMed or Google Scholar.
If the case definition for the illnesses under investigation includes laboratory information in the form of Whole Genome Sequencing (WGS) results, consider investigating where and when the sequence has been seen before. Provincial and federal public health laboratories maintain WGS databases that can contain valuable information for outbreak investigation purposes. PulseNet Canada can provide information about how common or rare the serotype or sequence is nationally, where and when it was last seen, and if it has been detected in any food samples in the past. PulseNet Canada will also be able to check the United States’ PulseNet WGS databases for matches. FoodNet Canada can provide information about whether the sequence has previously been seen in farm or retail samples from its sentinel sites.
While it is important to gather such historical information, the most effective way to generate a high-quality hypothesis is to identify common exposures amongst cases. This can be achieved by interviewing cases using a hypothesis generating questionnaire and analysing exposures.
Back to top
Hypothesis generating questionnaires
Hypothesis generating questionnaires (or shotgun questionnaires) are intended to obtain detailed information on what a person’s exposures were in the days leading up to their illness. They are typically quite long and ask about many exposures such as travel history, contact with animals, restaurants, events attended, and a comprehensive food history. The time period of interest varies between pathogens, as the exposure period is equal to the maximum incubation period of the pathogen.
When designing a questionnaire, it is important to ensure that the questions are gathering the intended information. Questions should be concise, informal, and specific. Before interviewing cases, questionnaires should be tested to ensure clarity and identify any potential errors.
Read more – Questionnaire Design
Case interviewing
Once the questionnaire is developed and piloted, it should be administered to cases in a consistent and unbiased manner. Case interviews can be conducted by one or multiple interviewers. A centralized approach allows a single interviewer to standardize interviews, detect patterns, and probe for items of interest. However, a multiple- interviewer approach is more time-efficient and allows for multiple perspectives when it comes time to identify the source.
Although case interviewing is an important outbreak investigation tool, it is not without its challenges. By the time the outbreak team is ready to conduct the interview, it could be weeks to months after the onset of symptoms. It is difficult for people to recall what they ate over a month ago. Sometimes cases might need to be interviewed multiple times as the hypothesis is developed and refined.
Read more- Case interviews
Once the interviews are complete, the data can be entered into a database or line list . The frequency of exposures for the cases is then obtained (e.g., % of cases that consumed each food item).
It is tempting to conclude that the most commonly consumed food items are the most likely suspects, but it is possible that these foods are commonly consumed amongst the general population as well. What is needed is a baseline proportion to compare the exposure frequencies to. Reference population studies, such as the CDC Food Atlas, the Nesbitt Waterloo study and Foodbook (see Tools ), can be used for this purpose. These studies provide investigators with the expected food frequencies based on 7-day food histories from thousands of respondents. These data can be used as a point of comparison for questionnaire data to identify exposures such as food items with higher than expected frequencies. Statistical tests (e.g., binomial probability tests) can then be used to test whether the differences between the proportion of cases exposed is significantly different from the proportion of “controls” (i.e., people included in the population studies) (see Tools ).
There are many limitations to using expected food frequencies, such as some studies not accounting for:
- Seasonality (e.g., consumption of cherries is higher in the summer, however the expected levels are the same year-round)
- Differences in consumption between sexes, adults and children
- Geographic location
- Various ethnic/religious/cultural groups
Further, since specific questions differ among surveys, it is often difficult to find the most appropriate comparison group. For example, the CDC Atlas of Exposures differentiates between hamburgers eaten at home or outside the home, while questionnaires used in investigations typically do not. Such differences in food definitions can make it challenging to determine which reference variable is the most appropriate to use as an “expected” level.
It is important to keep in mind that some foods with high expected consumption levels (e.g., chicken) may not flag statistically, but could still be potential sources. Further, there are other common exposures amongst cases that can carry important clues about the source of the outbreak. Cases that report common restaurants, events, or grocery stores can be considered sub-clusters. These sub-clusters should be investigated thoroughly by obtaining menus, receipts, or shopper card information if possible.
- Case study, Module 2: Hypothesis generation
- Case study, Module 2 – Exercise 3: Food frequency analysis in Excel
- Case study, Module 2: Interpreting hypothesis generation results
- Hypothesis generation through case exposures in multiple restaurant clusters example: Barton Behravesh C, et al . 2012. Multistate outbreak of Salmonella serotype Typhimurium infections associated with consumption of restaurant tomatoes, USA, 2006: hypothesis generation through case exposures in multiple restaurant clusters. Epidemiol Infect . 140 (11): 2053-2061 .
- Case-case study example: Galanis, E., et al . 2014. The association between campylobacteriosis, agriculture and drinking water: a case-case study in a region of British Columbia, Canada, 2005–2009. Epidemiol Infect . 142 (10): 2075-2084 .
- Exact Probability Calculation and Case-Case Study example: Gaulin, C., et al. 2012. Escherichia coli O157:H7 Outbreak Linked to Raw Milk Cheese in Quebec, Canada: Use of Exact Probability Calculation and Case-Case Study Approaches to Foodborne Outbreak Investigation. J Food Prot . 5: 812-818 .
Toolkit binomial probability calculation tool for food exposures
- This Microsoft Excel document allows users to enter outbreak case food exposure numbers for 300 food items and automatically calculates binomial probabilities using two reference populations and flags exposures of interest for follow-up (Reference populations: CDC Population Survey Atlas of Exposures, 2006-2007 and Waterloo Region, Ontario Food Consumption Survey, November 2005 to March 2006).
Toolkit Outbreak Summaries overview
- This PDF document provides an overview of the Outbreak Summaries application, its key features and benefits, and an example of how it can be used during an outbreak investigation.
CDC National Outbreak Reporting System (NORS) Dashboard
- The NORS dashboard allows users to view and download data on disease outbreaks reported to CDC.Data can be filtered by type of outbreak, year, state, etiology (genus only), setting, food/ingredient, water exposure, and water type.
Food Consumption Patterns in the Waterloo Region
- This food frequency study by Nesbitt et. al. was conducted in Waterloo, Ontario in 2005-2006. The study collected 7-day food consumption data from 2,332 Canadians.
CDC Food Atlas 2006-2007
- This study by CDC was conducted in 10 U.S. states in 2006-2007. The study asked 17,000 respondents about their exposure to a comprehensive list of foods as well as animal exposure.
FoodNet Canada Reports and Publications
- FoodNet Canada reports and publications provide information on the areas of greatest risk to human health to help direct food safety actions and programming as well as public health interventions, and to evaluate their effectiveness.
CDC FoodNet Reports
- The Foodborne Diseases Active Surveillance Network (FoodNet) Annual Reports are summaries of information collected through active surveillance of nine pathogens.
Marler Clark Foodborne Illness Outbreak Database
- This database provides summaries of food and water related outbreaks caused by various enteric pathogens dating back to 1984.
FDA Foodborne Illness-Causing Organisms Cheat Sheet
- A quick summary chart on foodborne illnesses, organisms involved, symptom onset times, signs and symptoms to expect, and food sources.
CFIA: Canada’s 10 Least Wanted Foodborne Pathogens
- This infographic prepared by the CFIA includes information on symptoms, onset time, transmission, potential sources, and preventative measures for ten foodborne pathogens.
Foodbook: Canadian Food Exposure Study to Strengthen Outbreak Response
- Foodbook is a population-based telephone survey that was conducted in all Canadian provinces and territories. It provides essential data on food, animal and water exposure which is used by the Agency, as well as other federal, provincial, and territorial (F/P/T) partners to understand, respond to, control and prevent enteric illness in Canada.
Toolkit outbreak response database*
*Due to the Government of Canada’s Standard on Web Accessibility, this tool cannot be posted, but it is available upon request. Please contact us at [email protected] to request a copy. Please let us know if you need support or an accessible format.
- Open access
- Published: 15 May 2024
Learning together for better health using an evidence-based Learning Health System framework: a case study in stroke
- Helena Teede 1 , 2 na1 ,
- Dominique A. Cadilhac 3 , 4 na1 ,
- Tara Purvis 3 ,
- Monique F. Kilkenny 3 , 4 ,
- Bruce C.V. Campbell 4 , 5 , 6 ,
- Coralie English 7 ,
- Alison Johnson 2 ,
- Emily Callander 1 ,
- Rohan S. Grimley 8 , 9 ,
- Christopher Levi 10 ,
- Sandy Middleton 11 , 12 ,
- Kelvin Hill 13 &
- Joanne Enticott ORCID: orcid.org/0000-0002-4480-5690 1
BMC Medicine volume 22 , Article number: 198 ( 2024 ) Cite this article
Metrics details
In the context of expanding digital health tools, the health system is ready for Learning Health System (LHS) models. These models, with proper governance and stakeholder engagement, enable the integration of digital infrastructure to provide feedback to all relevant parties including clinicians and consumers on performance against best practice standards, as well as fostering innovation and aligning healthcare with patient needs. The LHS literature primarily includes opinion or consensus-based frameworks and lacks validation or evidence of benefit. Our aim was to outline a rigorously codesigned, evidence-based LHS framework and present a national case study of an LHS-aligned national stroke program that has delivered clinical benefit.
Current core components of a LHS involve capturing evidence from communities and stakeholders (quadrant 1), integrating evidence from research findings (quadrant 2), leveraging evidence from data and practice (quadrant 3), and generating evidence from implementation (quadrant 4) for iterative system-level improvement. The Australian Stroke program was selected as the case study as it provides an exemplar of how an iterative LHS works in practice at a national level encompassing and integrating evidence from all four LHS quadrants. Using this case study, we demonstrate how to apply evidence-based processes to healthcare improvement and embed real-world research for optimising healthcare improvement. We emphasize the transition from research as an endpoint, to research as an enabler and a solution for impact in healthcare improvement.
Conclusions
The Australian Stroke program has nationally improved stroke care since 2007, showcasing the value of integrated LHS-aligned approaches for tangible impact on outcomes. This LHS case study is a practical example for other health conditions and settings to follow suit.
Peer Review reports
Internationally, health systems are facing a crisis, driven by an ageing population, increasing complexity, multi-morbidity, rapidly advancing health technology and rising costs that threaten sustainability and mandate transformation and improvement [ 1 , 2 ]. Although research has generated solutions to healthcare challenges, and the advent of big data and digital health holds great promise, entrenched siloes and poor integration of knowledge generation, knowledge implementation and healthcare delivery between stakeholders, curtails momentum towards, and consistent attainment of, evidence-and value-based care [ 3 ]. This is compounded by the short supply of research and innovation leadership within the healthcare sector, and poorly integrated and often inaccessible health data systems, which have crippled the potential to deliver on digital-driven innovation [ 4 ]. Current approaches to healthcare improvement are also often isolated with limited sustainability, scale-up and impact [ 5 ].
Evidence suggests that integration and partnership across academic and healthcare delivery stakeholders are key to progress, including those with lived experience and their families (referred to here as consumers and community), diverse disciplines (both research and clinical), policy makers and funders. Utilization of evidence from research and evidence from practice including data from routine care, supported by implementation research, are key to sustainably embedding improvement and optimising health care and outcomes. A strategy to achieve this integration is through the Learning Health System (LHS) (Fig. 1 ) [ 2 , 6 , 7 , 8 ]. Although there are numerous publications on LHS approaches [ 9 , 10 , 11 , 12 ], many focus on research perspectives and data, most do not demonstrate tangible healthcare improvement or better health outcomes. [ 6 ]
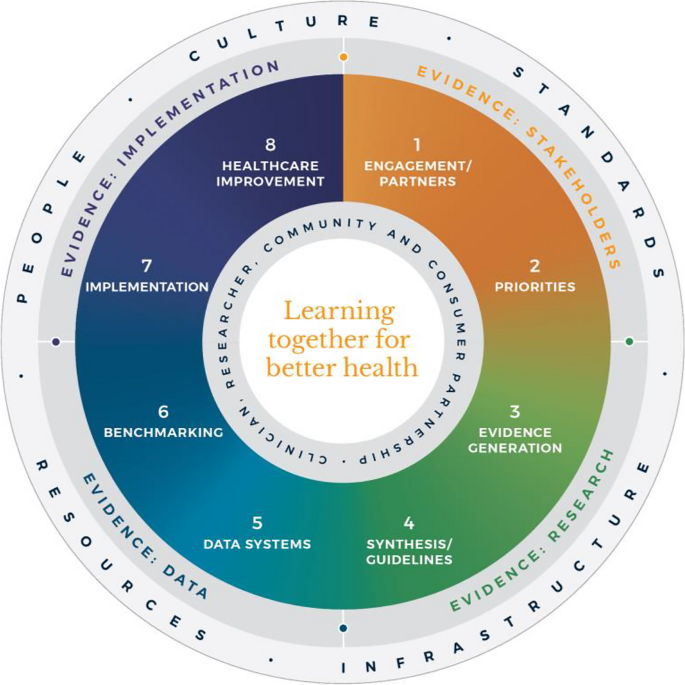
Monash Learning Health System: The Learn Together for Better Health Framework developed by Monash Partners and Monash University (from Enticott et al. 2021 [ 7 ]). Four evidence quadrants: Q1 (orange) is evidence from stakeholders; Q2 (green) is evidence from research; Q3 (light blue) is evidence from data; and, Q4 (dark blue) is evidence from implementation and healthcare improvement
In developed nations, it has been estimated that 60% of care provided aligns with the evidence base, 30% is low value and 10% is potentially harmful [ 13 ]. In some areas, clinical advances have been rapid and research and evidence have paved the way for dramatic improvement in outcomes, mandating rapid implementation of evidence into healthcare (e.g. polio and COVID-19 vaccines). However, healthcare improvement is challenging and slow [ 5 ]. Health systems are highly complex in their design, networks and interacting components, and change is difficult to enact, sustain and scale up. [ 3 ] New effective strategies are needed to meet community needs and deliver evidence-based and value-based care, which reorients care from serving the provider, services and system, towards serving community needs, based on evidence and quality. It goes beyond cost to encompass patient and provider experience, quality care and outcomes, efficiency and sustainability [ 2 , 6 ].
The costs of stroke care are expected to rise rapidly in the next decades, unless improvements in stroke care to reduce the disabling effects of strokes can be successfully developed and implemented [ 14 ]. Here, we briefly describe the Monash LHS framework (Fig. 1 ) [ 2 , 6 , 7 ] and outline an exemplar case in order to demonstrate how to apply evidence-based processes to healthcare improvement and embed real-world research for optimising healthcare. The Australian LHS exemplar in stroke care has driven nationwide improvement in stroke care since 2007.
An evidence-based Learning Health System framework
In Australia, members of this author group (HT, AJ, JE) have rigorously co-developed an evidence-based LHS framework, known simply as the Monash LHS [ 7 ]. The Monash LHS was designed to support sustainable, iterative and continuous robust benefit of improved clinical outcomes. It was created with national engagement in order to be applicable to Australian settings. Through this rigorous approach, core LHS principles and components have been established (Fig. 1 ). Evidence shows that people/workforce, culture, standards, governance and resources were all key to an effective LHS [ 2 , 6 ]. Culture is vital including trust, transparency, partnership and co-design. Key processes include legally compliant data sharing, linkage and governance, resources, and infrastructure [ 4 ]. The Monash LHS integrates disparate and often siloed stakeholders, infrastructure and expertise to ‘Learn Together for Better Health’ [ 7 ] (Fig. 1 ). This integrates (i) evidence from community and stakeholders including priority areas and outcomes; (ii) evidence from research and guidelines; (iii) evidence from practice (from data) with advanced analytics and benchmarking; and (iv) evidence from implementation science and health economics. Importantly, it starts with the problem and priorities of key stakeholders including the community, health professionals and services and creates an iterative learning system to address these. The following case study was chosen as it is an exemplar of how a Monash LHS-aligned national stroke program has delivered clinical benefit.
Australian Stroke Learning Health System
Internationally, the application of LHS approaches in stroke has resulted in improved stroke care and outcomes [ 12 ]. For example, in Canada a sustained decrease in 30-day in-hospital mortality has been found commensurate with an increase in resources to establish the multifactorial stroke system intervention for stroke treatment and prevention [ 15 ]. Arguably, with rapid advances in evidence and in the context of an ageing population with high cost and care burden and substantive impacts on quality of life, stroke is an area with a need for rapid research translation into evidence-based and value-based healthcare improvement. However, a recent systematic review found that the existing literature had few comprehensive examples of LHS adoption [ 12 ]. Although healthcare improvement systems and approaches were described, less is known about patient-clinician and stakeholder engagement, governance and culture, or embedding of data informatics into everyday practice to inform and drive improvement [ 12 ]. For example, in a recent review of quality improvement collaborations, it was found that although clinical processes in stroke care are improved, their short-term nature means there is uncertainty about sustainability and impacts on patient outcomes [ 16 ]. Table 1 provides the main features of the Australian Stroke LHS based on the four core domains and eight elements of the Learning Together for Better Health Framework described in Fig. 1 . The features are further expanded on in the following sections.
Evidence from stakeholders (LHS quadrant 1, Fig. 1 )
Engagement, partners and priorities.
Within the stroke field, there have been various support mechanisms to facilitate an LHS approach including partnership and broad stakeholder engagement that includes clinical networks and policy makers from different jurisdictions. Since 2008, the Australian Stroke Coalition has been co-led by the Stroke Foundation, a charitable consumer advocacy organisation, and Stroke Society of Australasia a professional society with membership covering academics and multidisciplinary clinician networks, that are collectively working to improve stroke care ( https://australianstrokecoalition.org.au/ ). Surveys, focus groups and workshops have been used for identifying priorities from stakeholders. Recent agreed priorities have been to improve stroke care and strengthen the voice for stroke care at a national ( https://strokefoundation.org.au/ ) and international level ( https://www.world-stroke.org/news-and-blog/news/world-stroke-organization-tackle-gaps-in-access-to-quality-stroke-care ), as well as reduce duplication amongst stakeholders. This activity is built on a foundation and culture of research and innovation embedded within the stroke ‘community of practice’. Consumers, as people with lived experience of stroke are important members of the Australian Stroke Coalition, as well as representatives from different clinical colleges. Consumers also provide critical input to a range of LHS activities via the Stroke Foundation Consumer Council, Stroke Living Guidelines committees, and the Australian Stroke Clinical Registry (AuSCR) Steering Committee (described below).
Evidence from research (LHS quadrant 2, Fig. 1 )
Advancement of the evidence for stroke interventions and synthesis into clinical guidelines.
To implement best practice, it is crucial to distil the large volume of scientific and trial literature into actionable recommendations for clinicians to use in practice [ 24 ]. The first Australian clinical guidelines for acute stroke were produced in 2003 following the increasing evidence emerging for prevention interventions (e.g. carotid endarterectomy, blood pressure lowering), acute medical treatments (intravenous thrombolysis, aspirin within 48 h of ischemic stroke), and optimised hospital management (care in dedicated stroke units by a specialised and coordinated multidisciplinary team) [ 25 ]. Importantly, a number of the innovations were developed, researched and proven effective by key opinion leaders embedded in the Australian stroke care community. In 2005, the clinical guidelines for Stroke Rehabilitation and Recovery [ 26 ] were produced, with subsequent merged guidelines periodically updated. However, the traditional process of periodic guideline updates is challenging for end users when new research can render recommendations redundant and this lack of currency erodes stakeholder trust [ 27 ]. In response to this challenge the Stroke Foundation and Cochrane Australia entered a pioneering project to produce the first electronic ‘living’ guidelines globally [ 20 ]. Major shifts in the evidence for reperfusion therapies (e.g. extended time-window intravenous thrombolysis and endovascular clot retrieval), among other advances, were able to be converted into new recommendations, approved by the Australian National Health and Medical Research Council within a few months of publication. Feedback on this process confirmed the increased use and trust in the guidelines by clinicians. The process informed other living guidelines programs, including the successful COVID-19 clinical guidelines [ 28 ].
However, best practice clinical guideline recommendations are necessary but insufficient for healthcare improvement and nesting these within an LHS with stakeholder partnership, enables implementation via a range of proven methods, including audit and feedback strategies [ 29 ].
Evidence from data and practice (LHS quadrant 3, Fig. 1 )
Data systems and benchmarking : revealing the disparities in care between health services. A national system for standardized stroke data collection was established as the National Stroke Audit program in 2007 by the Stroke Foundation [ 30 ] following various state-level programs (e.g. New South Wales Audit) [ 31 ] to identify evidence-practice gaps and prioritise improvement efforts to increase access to stroke units and other acute treatments [ 32 ]. The Audit program alternates each year between acute (commencing in 2007) and rehabilitation in-patient services (commencing in 2008). The Audit program provides a ‘deep dive’ on the majority of recommendations in the clinical guidelines whereby participating hospitals provide audits of up to 40 consecutive patient medical records and respond to a survey about organizational resources to manage stroke. In 2009, the AuSCR was established to provide information on patients managed in acute hospitals based on a small subset of quality processes of care linked to benchmarked reports of performance (Fig. 2 ) [ 33 ]. In this way, the continuous collection of high-priority processes of stroke care could be regularly collected and reviewed to guide improvement to care [ 34 ]. Plus clinical quality registry programs within Australia have shown a meaningful return on investment attributed to enhanced survival, improvements in quality of life and avoided costs of treatment or hospital stay [ 35 ].
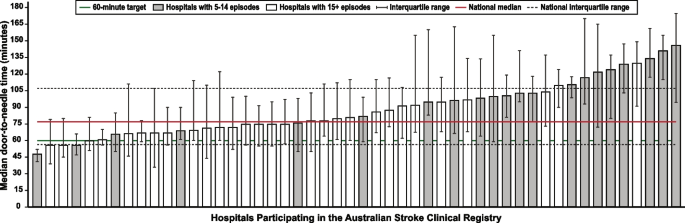
Example performance report from the Australian Stroke Clinical Registry: average door-to-needle time in providing intravenous thrombolysis by different hospitals in 2021 [ 36 ]. Each bar in the figure represents a single hospital
The Australian Stroke Coalition endorsed the creation of an integrated technological solution for collecting data through a single portal for multiple programs in 2013. In 2015, the Stroke Foundation, AuSCR consortium, and other relevant groups cooperated to design an integrated data management platform (the Australian Stroke Data Tool) to reduce duplication of effort for hospital staff in the collection of overlapping variables in the same patients [ 19 ]. Importantly, a national data dictionary then provided the common data definitions to facilitate standardized data capture. Another important feature of AuSCR is the collection of patient-reported outcome surveys between 90 and 180 days after stroke, and annual linkage with national death records to ascertain survival status [ 33 ]. To support a LHS approach, hospitals that participate in AuSCR have access to a range of real-time performance reports. In efforts to minimize the burden of data collection in the AuSCR, interoperability approaches to import data directly from hospital or state-level managed stroke databases have been established (Fig. 3 ); however, the application has been variable and 41% of hospitals still manually enter all their data.
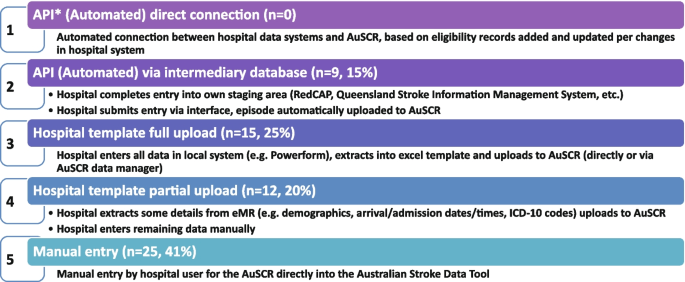
Current status of automated data importing solutions in the Australian Stroke Clinical Registry, 2022, with ‘ n ’ representing the number of hospitals. AuSCR, Australian Stroke Clinical Registry; AuSDaT, Australian Stroke Data Tool; API, Application Programming Interface; ICD, International Classification of Diseases; RedCAP, Research Electronic Data Capture; eMR, electronic medical records
For acute stroke care, the Australian Commission on Quality and Safety in Health Care facilitated the co-design (clinicians, academics, consumers) and publication of the national Acute Stroke Clinical Care Standard in 2015 [ 17 ], and subsequent review [ 18 ]. The indicator set for the Acute Stroke Standard then informed the expansion of the minimum dataset for AuSCR so that hospitals could routinely track their performance. The national Audit program enabled hospitals not involved in the AuSCR to assess their performance every two years against the Acute Stroke Standard. Complementing these efforts, the Stroke Foundation, working with the sector, developed the Acute and Rehabilitation Stroke Services Frameworks to outline the principles, essential elements, models of care and staffing recommendations for stroke services ( https://informme.org.au/guidelines/national-stroke-services-frameworks ). The Frameworks are intended to guide where stroke services should be developed, and monitor their uptake with the organizational survey component of the Audit program.
Evidence from implementation and healthcare improvement (LHS quadrant 4, Fig. 1 )
Research to better utilize and augment data from registries through linkage [ 37 , 38 , 39 , 40 ] and to ensure presentation of hospital or service level data are understood by clinicians has ensured advancement in the field for the Australian Stroke LHS [ 41 ]. Importantly, greater insights into whole patient journeys, before and after a stroke, can now enable exploration of value-based care. The LHS and stroke data platform have enabled focused and time-limited projects to create a better understanding of the quality of care in acute or rehabilitation settings [ 22 , 42 , 43 ]. Within stroke, all the elements of an LHS culminate into the ready availability of benchmarked performance data and support for implementation of strategies to address gaps in care.
Implementation research to grow the evidence base for effective improvement interventions has also been a key pillar in the Australian context. These include multi-component implementation interventions to achieve behaviour change for particular aspects of stroke care, [ 22 , 23 , 44 , 45 ] and real-world approaches to augmenting access to hyperacute interventions in stroke through the use of technology and telehealth [ 46 , 47 , 48 , 49 ]. The evidence from these studies feeds into the living guidelines program and the data collection systems, such as the Audit program or AuSCR, which are then amended to ensure data aligns to recommended care. For example, the use of ‘hyperacute aspirin within the first 48 h of ischemic stroke’ was modified to be ‘hyperacute antiplatelet…’ to incorporate new evidence that other medications or combinations are appropriate to use. Additionally, new datasets have been developed to align with evidence such as the Fever, Sugar, and Swallow variables [ 42 ]. Evidence on improvements in access to best practice care from the acute Audit program [ 50 ] and AuSCR is emerging [ 36 ]. For example, between 2007 and 2017, the odds of receiving intravenous thrombolysis after ischemic stroke increased by 16% 9OR 1.06 95% CI 1.13–1.18) and being managed in a stroke unit by 18% (OR 1.18 95% CI 1.17–1.20). Over this period, the median length of hospital stay for all patients decreased from 6.3 days in 2007 to 5.0 days in 2017 [ 51 ]. When considering the number of additional patients who would receive treatment in 2017 in comparison to 2007 it was estimated that without this additional treatment, over 17,000 healthy years of life would be lost in 2017 (17,786 disability-adjusted life years) [ 51 ]. There is evidence on the cost-effectiveness of different system-focussed strategies to augment treatment access for acute ischemic stroke (e.g. Victorian Stroke Telemedicine program [ 52 ] and Melbourne Mobile Stroke Unit ambulance [ 53 ]). Reciprocally, evidence from the national Rehabilitation Audit, where the LHS approach has been less complete or embedded, has shown fewer areas of healthcare improvement over time [ 51 , 54 ].
Within the field of stroke in Australia, there is indirect evidence that the collective efforts that align to establishing the components of a LHS have had an impact. Overall, the age-standardised rate of stroke events has reduced by 27% between 2001 and 2020, from 169 to 124 events per 100,000 population. Substantial declines in mortality rates have been reported since 1980. Commensurate with national clinical guidelines being updated in 2007 and the first National Stroke Audit being undertaken in 2007, the mortality rates for men (37.4 deaths per 100,000) and women (36.1 deaths per 100,0000 has declined to 23.8 and 23.9 per 100,000, respectively in 2021 [ 55 ].
Underpinning the LHS with the integration of the four quadrants of evidence from stakeholders, research and guidelines, practice and implementation, and core LHS principles have been addressed. Leadership and governance have been important, and programs have been established to augment workforce training and capacity building in best practice professional development. Medical practitioners are able to undertake courses and mentoring through the Australasian Stroke Academy ( http://www.strokeacademy.com.au/ ) while nurses (and other health professionals) can access teaching modules in stroke care from the Acute Stroke Nurses Education Network ( https://asnen.org/ ). The Association of Neurovascular Clinicians offers distance-accessible education and certification to develop stroke expertise for interdisciplinary professionals, including advanced stroke co-ordinator certification ( www.anvc.org ). Consumer initiative interventions are also used in the design of the AuSCR Public Summary Annual reports (available at https://auscr.com.au/about/annual-reports/ ) and consumer-related resources related to the Living Guidelines ( https://enableme.org.au/resources ).
The important success factors and lessons from stroke as a national exemplar LHS in Australia include leadership, culture, workforce and resources integrated with (1) established and broad partnerships across the academic-clinical sector divide and stakeholder engagement; (2) the living guidelines program; (3) national data infrastructure, including a national data dictionary that provides the common data framework to support standardized data capture; (4) various implementation strategies including benchmarking and feedback as well as engagement strategies targeting different levels of the health system; and (5) implementation and improvement research to advance stroke systems of care and reduce unwarranted variation in practice (Fig. 1 ). Priority opportunities now include the advancement of interoperability with electronic medical records as an area all clinical quality registry’s programs needs to be addressed, as well as providing more dynamic and interactive data dashboards tailored to the need of clinicians and health service executives.
There is a clear mandate to optimise healthcare improvement with big data offering major opportunities for change. However, we have lacked the approaches to capture evidence from the community and stakeholders, to integrate evidence from research, to capture and leverage data or evidence from practice and to generate and build on evidence from implementation using iterative system-level improvement. The LHS provides this opportunity and is shown to deliver impact. Here, we have outlined the process applied to generate an evidence-based LHS and provide a leading exemplar in stroke care. This highlights the value of moving from single-focus isolated approaches/initiatives to healthcare improvement and the benefit of integration to deliver demonstrable outcomes for our funders and key stakeholders — our community. This work provides insight into strategies that can both apply evidence-based processes to healthcare improvement as well as implementing evidence-based practices into care, moving beyond research as an endpoint, to research as an enabler, underpinning delivery of better healthcare.
Availability of data and materials
Not applicable
Abbreviations
Australian Stroke Clinical Registry
Confidence interval
- Learning Health System
World Health Organization. Delivering quality health services . OECD Publishing; 2018.
Enticott J, Braaf S, Johnson A, Jones A, Teede HJ. Leaders’ perspectives on learning health systems: A qualitative study. BMC Health Serv Res. 2020;20:1087.
Article PubMed PubMed Central Google Scholar
Melder A, Robinson T, McLoughlin I, Iedema R, Teede H. An overview of healthcare improvement: Unpacking the complexity for clinicians and managers in a learning health system. Intern Med J. 2020;50:1174–84.
Article PubMed Google Scholar
Alberto IRI, Alberto NRI, Ghosh AK, Jain B, Jayakumar S, Martinez-Martin N, et al. The impact of commercial health datasets on medical research and health-care algorithms. Lancet Digit Health. 2023;5:e288–94.
Article CAS PubMed PubMed Central Google Scholar
Dixon-Woods M. How to improve healthcare improvement—an essay by Mary Dixon-Woods. BMJ. 2019;367: l5514.
Enticott J, Johnson A, Teede H. Learning health systems using data to drive healthcare improvement and impact: A systematic review. BMC Health Serv Res. 2021;21:200.
Enticott JC, Melder A, Johnson A, Jones A, Shaw T, Keech W, et al. A learning health system framework to operationalize health data to improve quality care: An Australian perspective. Front Med (Lausanne). 2021;8:730021.
Dammery G, Ellis LA, Churruca K, Mahadeva J, Lopez F, Carrigan A, et al. The journey to a learning health system in primary care: A qualitative case study utilising an embedded research approach. BMC Prim Care. 2023;24:22.
Foley T, Horwitz L, Zahran R. The learning healthcare project: Realising the potential of learning health systems. 2021. Available from https://learninghealthcareproject.org/wp-content/uploads/2021/05/LHS2021report.pdf . Accessed Jan 2024.
Institute of Medicine. Best care at lower cost: The path to continuously learning health care in America. Washington: The National Academies Press; 2013.
Google Scholar
Zurynski Y, Smith CL, Vedovi A, Ellis LA, Knaggs G, Meulenbroeks I, et al. Mapping the learning health system: A scoping review of current evidence - a white paper. 2020:63
Cadilhac DA, Bravata DM, Bettger J, Mikulik R, Norrving B, Uvere E, et al. Stroke learning health systems: A topical narrative review with case examples. Stroke. 2023;54:1148–59.
Braithwaite J, Glasziou P, Westbrook J. The three numbers you need to know about healthcare: The 60–30-10 challenge. BMC Med. 2020;18:1–8.
Article Google Scholar
King D, Wittenberg R, Patel A, Quayyum Z, Berdunov V, Knapp M. The future incidence, prevalence and costs of stroke in the UK. Age Ageing. 2020;49:277–82.
Ganesh A, Lindsay P, Fang J, Kapral MK, Cote R, Joiner I, et al. Integrated systems of stroke care and reduction in 30-day mortality: A retrospective analysis. Neurology. 2016;86:898–904.
Lowther HJ, Harrison J, Hill JE, Gaskins NJ, Lazo KC, Clegg AJ, et al. The effectiveness of quality improvement collaboratives in improving stroke care and the facilitators and barriers to their implementation: A systematic review. Implement Sci. 2021;16:16.
Australian Commission on Safety and Quality in Health Care. Acute stroke clinical care standard. 2015. Available from https://www.safetyandquality.gov.au/our-work/clinical-care-standards/acute-stroke-clinical-care-standard . Accessed Jan 2024.
Australian Commission on Safety and Quality in Health Care. Acute stroke clinical care standard. Sydney: ACSQHC; 2019. Available from https://www.safetyandquality.gov.au/publications-and-resources/resource-library/acute-stroke-clinical-care-standard-evidence-sources . Accessed Jan 2024.
Ryan O, Ghuliani J, Grabsch B, Hill K, G CC, Breen S, et al. Development, implementation, and evaluation of the Australian Stroke Data Tool (AuSDaT): Comprehensive data capturing for multiple uses. Health Inf Manag. 2022:18333583221117184.
English C, Bayley M, Hill K, Langhorne P, Molag M, Ranta A, et al. Bringing stroke clinical guidelines to life. Int J Stroke. 2019;14:337–9.
English C, Hill K, Cadilhac DA, Hackett ML, Lannin NA, Middleton S, et al. Living clinical guidelines for stroke: Updates, challenges and opportunities. Med J Aust. 2022;216:510–4.
Cadilhac DA, Grimley R, Kilkenny MF, Andrew NE, Lannin NA, Hill K, et al. Multicenter, prospective, controlled, before-and-after, quality improvement study (Stroke123) of acute stroke care. Stroke. 2019;50:1525–30.
Cadilhac DA, Marion V, Andrew NE, Breen SJ, Grabsch B, Purvis T, et al. A stepped-wedge cluster-randomized trial to improve adherence to evidence-based practices for acute stroke management. Jt Comm J Qual Patient Saf. 2022.
Elliott J, Lawrence R, Minx JC, Oladapo OT, Ravaud P, Jeppesen BT, et al. Decision makers need constantly updated evidence synthesis. Nature. 2021;600:383–5.
Article CAS PubMed Google Scholar
National Stroke Foundation. National guidelines for acute stroke management. Melbourne: National Stroke Foundation; 2003.
National Stroke Foundation. Clinical guidelines for stroke rehabilitation and recovery. Melbourne: National Stroke Foundation; 2005.
Phan TG, Thrift A, Cadilhac D, Srikanth V. A plea for the use of systematic review methodology when writing guidelines and timely publication of guidelines. Intern Med J . 2012;42:1369–1371; author reply 1371–1362
Tendal B, Vogel JP, McDonald S, Norris S, Cumpston M, White H, et al. Weekly updates of national living evidence-based guidelines: Methods for the Australian living guidelines for care of people with COVID-19. J Clin Epidemiol. 2021;131:11–21.
Grimshaw JM, Eccles MP, Lavis JN, Hill SJ, Squires JE. Knowledge translation of research findings. Implement Sci. 2012;7:50.
Harris D, Cadilhac D, Hankey GJ, Hillier S, Kilkenny M, Lalor E. National stroke audit: The Australian experience. Clin Audit. 2010;2:25–31.
Cadilhac DA, Purvis T, Kilkenny MF, Longworth M, Mohr K, Pollack M, et al. Evaluation of rural stroke services: Does implementation of coordinators and pathways improve care in rural hospitals? Stroke. 2013;44:2848–53.
Cadilhac DA, Moss KM, Price CJ, Lannin NA, Lim JY, Anderson CS. Pathways to enhancing the quality of stroke care through national data monitoring systems for hospitals. Med J Aust. 2013;199:650–1.
Cadilhac DA, Lannin NA, Anderson CS, Levi CR, Faux S, Price C, et al. Protocol and pilot data for establishing the Australian Stroke Clinical Registry. Int J Stroke. 2010;5:217–26.
Ivers N, Jamtvedt G, Flottorp S, Young J, Odgaard-Jensen J, French S, et al. Audit and feedback: Effects on professional practice and healthcare outcomes. Cochrane Database Syst Rev . 2012
Australian Commission on Safety and Quality in Health Care. Economic evaluation of clinical quality registries. Final report. . 2016:79
Cadilhac DA, Dalli LL, Morrison J, Lester M, Paice K, Moss K, et al. The Australian Stroke Clinical Registry annual report 2021. Melbourne; 2022. Available from https://auscr.com.au/about/annual-reports/ . Accessed 6 May 2024.
Kilkenny MF, Kim J, Andrew NE, Sundararajan V, Thrift AG, Katzenellenbogen JM, et al. Maximising data value and avoiding data waste: A validation study in stroke research. Med J Aust. 2019;210:27–31.
Eliakundu AL, Smith K, Kilkenny MF, Kim J, Bagot KL, Andrew E, et al. Linking data from the Australian Stroke Clinical Registry with ambulance and emergency administrative data in Victoria. Inquiry. 2022;59:469580221102200.
PubMed Google Scholar
Andrew NE, Kim J, Cadilhac DA, Sundararajan V, Thrift AG, Churilov L, et al. Protocol for evaluation of enhanced models of primary care in the management of stroke and other chronic disease (PRECISE): A data linkage healthcare evaluation study. Int J Popul Data Sci. 2019;4:1097.
CAS PubMed PubMed Central Google Scholar
Mosalski S, Shiner CT, Lannin NA, Cadilhac DA, Faux SG, Kim J, et al. Increased relative functional gain and improved stroke outcomes: A linked registry study of the impact of rehabilitation. J Stroke Cerebrovasc Dis. 2021;30: 106015.
Ryan OF, Hancock SL, Marion V, Kelly P, Kilkenny MF, Clissold B, et al. Feedback of aggregate patient-reported outcomes (PROs) data to clinicians and hospital end users: Findings from an Australian codesign workshop process. BMJ Open. 2022;12:e055999.
Grimley RS, Rosbergen IC, Gustafsson L, Horton E, Green T, Cadigan G, et al. Dose and setting of rehabilitation received after stroke in Queensland, Australia: A prospective cohort study. Clin Rehabil. 2020;34:812–23.
Purvis T, Middleton S, Craig LE, Kilkenny MF, Dale S, Hill K, et al. Inclusion of a care bundle for fever, hyperglycaemia and swallow management in a national audit for acute stroke: Evidence of upscale and spread. Implement Sci. 2019;14:87.
Middleton S, McElduff P, Ward J, Grimshaw JM, Dale S, D’Este C, et al. Implementation of evidence-based treatment protocols to manage fever, hyperglycaemia, and swallowing dysfunction in acute stroke (QASC): A cluster randomised controlled trial. Lancet. 2011;378:1699–706.
Middleton S, Dale S, Cheung NW, Cadilhac DA, Grimshaw JM, Levi C, et al. Nurse-initiated acute stroke care in emergency departments. Stroke. 2019:STROKEAHA118020701.
Hood RJ, Maltby S, Keynes A, Kluge MG, Nalivaiko E, Ryan A, et al. Development and pilot implementation of TACTICS VR: A virtual reality-based stroke management workflow training application and training framework. Front Neurol. 2021;12:665808.
Bladin CF, Kim J, Bagot KL, Vu M, Moloczij N, Denisenko S, et al. Improving acute stroke care in regional hospitals: Clinical evaluation of the Victorian Stroke Telemedicine program. Med J Aust. 2020;212:371–7.
Bladin CF, Bagot KL, Vu M, Kim J, Bernard S, Smith K, et al. Real-world, feasibility study to investigate the use of a multidisciplinary app (Pulsara) to improve prehospital communication and timelines for acute stroke/STEMI care. BMJ Open. 2022;12:e052332.
Zhao H, Coote S, Easton D, Langenberg F, Stephenson M, Smith K, et al. Melbourne mobile stroke unit and reperfusion therapy: Greater clinical impact of thrombectomy than thrombolysis. Stroke. 2020;51:922–30.
Purvis T, Cadilhac DA, Hill K, Reyneke M, Olaiya MT, Dalli LL, et al. Twenty years of monitoring acute stroke care in Australia from the national stroke audit program (1999–2019): Achievements and areas of future focus. J Health Serv Res Policy. 2023.
Cadilhac DA, Purvis T, Reyneke M, Dalli LL, Kim J, Kilkenny MF. Evaluation of the national stroke audit program: 20-year report. Melbourne; 2019.
Kim J, Tan E, Gao L, Moodie M, Dewey HM, Bagot KL, et al. Cost-effectiveness of the Victorian Stroke Telemedicine program. Aust Health Rev. 2022;46:294–301.
Kim J, Easton D, Zhao H, Coote S, Sookram G, Smith K, et al. Economic evaluation of the Melbourne mobile stroke unit. Int J Stroke. 2021;16:466–75.
Stroke Foundation. National stroke audit – rehabilitation services report 2020. Melbourne; 2020.
Australian Institute of Health and Welfare. Heart, stroke and vascular disease: Australian facts. 2023. Webpage https://www.aihw.gov.au/reports/heart-stroke-vascular-diseases/hsvd-facts/contents/about (accessed Jan 2024).
Download references
Acknowledgements
The following authors hold National Health and Medical Research Council Research Fellowships: HT (#2009326), DAC (#1154273), SM (#1196352), MFK Future Leader Research Fellowship (National Heart Foundation #105737). The Funders of this work did not have any direct role in the design of the study, its execution, analyses, interpretation of the data, or decision to submit results for publication.
Author information
Helena Teede and Dominique A. Cadilhac contributed equally.
Authors and Affiliations
Monash Centre for Health Research and Implementation, 43-51 Kanooka Grove, Clayton, VIC, Australia
Helena Teede, Emily Callander & Joanne Enticott
Monash Partners Academic Health Science Centre, 43-51 Kanooka Grove, Clayton, VIC, Australia
Helena Teede & Alison Johnson
Stroke and Ageing Research, Department of Medicine, School of Clinical Sciences at Monash Health, Monash University, Level 2 Monash University Research, Victorian Heart Hospital, 631 Blackburn Rd, Clayton, VIC, Australia
Dominique A. Cadilhac, Tara Purvis & Monique F. Kilkenny
Stroke Theme, The Florey Institute of Neuroscience and Mental Health, University of Melbourne, Heidelberg, VIC, Australia
Dominique A. Cadilhac, Monique F. Kilkenny & Bruce C.V. Campbell
Department of Neurology, Melbourne Brain Centre, Royal Melbourne Hospital, Parkville, VIC, Australia
Bruce C.V. Campbell
Department of Medicine, Faculty of Medicine, Dentistry and Health Sciences, University of Melbourne, Victoria, Australia
School of Health Sciences, Heart and Stroke Program, University of Newcastle, Hunter Medical Research Institute, University Drive, Callaghan, NSW, Australia
Coralie English
School of Medicine and Dentistry, Griffith University, Birtinya, QLD, Australia
Rohan S. Grimley
Clinical Excellence Division, Queensland Health, Brisbane, Australia
John Hunter Hospital, Hunter New England Local Health District and University of Newcastle, Sydney, NSW, Australia
Christopher Levi
School of Nursing, Midwifery and Paramedicine, Australian Catholic University, Sydney, NSW, Australia
Sandy Middleton
Nursing Research Institute, St Vincent’s Health Network Sydney and and Australian Catholic University, Sydney, NSW, Australia
Stroke Foundation, Level 7, 461 Bourke St, Melbourne, VIC, Australia
Kelvin Hill
You can also search for this author in PubMed Google Scholar
Contributions
HT: conception, design and initial draft, developed the theoretical formalism for learning health system framework, approved the submitted version. DAC: conception, design and initial draft, provided essential literature and case study examples, approved the submitted version. TP: revised the manuscript critically for important intellectual content, approved the submitted version. MFK: revised the manuscript critically for important intellectual content, provided essential literature and case study examples, approved the submitted version. BC: revised the manuscript critically for important intellectual content, provided essential literature and case study examples, approved the submitted version. CE: revised the manuscript critically for important intellectual content, provided essential literature and case study examples, approved the submitted version. AJ: conception, design and initial draft, developed the theoretical formalism for learning health system framework, approved the submitted version. EC: revised the manuscript critically for important intellectual content, approved the submitted version. RSG: revised the manuscript critically for important intellectual content, provided essential literature and case study examples, approved the submitted version. CL: revised the manuscript critically for important intellectual content, provided essential literature and case study examples, approved the submitted version. SM: revised the manuscript critically for important intellectual content, provided essential literature and case study examples, approved the submitted version. KH: revised the manuscript critically for important intellectual content, provided essential literature and case study examples, approved the submitted version. JE: conception, design and initial draft, developed the theoretical formalism for learning health system framework, approved the submitted version. All authors read and approved the final manuscript.
Authors’ Twitter handles
@HelenaTeede
@DominiqueCad
@Coralie_English
@EmilyCallander
@EnticottJo
Corresponding authors
Correspondence to Helena Teede or Dominique A. Cadilhac .
Ethics declarations
Ethics approval and consent to participate, consent for publication, competing interests, additional information, publisher's note.
Springer Nature remains neutral with regard to jurisdictional claims in published maps and institutional affiliations.
Rights and permissions
Open Access This article is licensed under a Creative Commons Attribution 4.0 International License, which permits use, sharing, adaptation, distribution and reproduction in any medium or format, as long as you give appropriate credit to the original author(s) and the source, provide a link to the Creative Commons licence, and indicate if changes were made. The images or other third party material in this article are included in the article's Creative Commons licence, unless indicated otherwise in a credit line to the material. If material is not included in the article's Creative Commons licence and your intended use is not permitted by statutory regulation or exceeds the permitted use, you will need to obtain permission directly from the copyright holder. To view a copy of this licence, visit http://creativecommons.org/licenses/by/4.0/ . The Creative Commons Public Domain Dedication waiver ( http://creativecommons.org/publicdomain/zero/1.0/ ) applies to the data made available in this article, unless otherwise stated in a credit line to the data.
Reprints and permissions
About this article
Cite this article.
Teede, H., Cadilhac, D.A., Purvis, T. et al. Learning together for better health using an evidence-based Learning Health System framework: a case study in stroke. BMC Med 22 , 198 (2024). https://doi.org/10.1186/s12916-024-03416-w
Download citation
Received : 23 July 2023
Accepted : 30 April 2024
Published : 15 May 2024
DOI : https://doi.org/10.1186/s12916-024-03416-w
Share this article
Anyone you share the following link with will be able to read this content:
Sorry, a shareable link is not currently available for this article.
Provided by the Springer Nature SharedIt content-sharing initiative
- Evidence-based medicine
- Person-centred care
- Models of care
- Healthcare improvement
BMC Medicine
ISSN: 1741-7015
- Submission enquiries: [email protected]
- General enquiries: [email protected]

An official website of the United States government
The .gov means it’s official. Federal government websites often end in .gov or .mil. Before sharing sensitive information, make sure you’re on a federal government site.
The site is secure. The https:// ensures that you are connecting to the official website and that any information you provide is encrypted and transmitted securely.
- Publications
- Account settings
Preview improvements coming to the PMC website in October 2024. Learn More or Try it out now .
- Advanced Search
- Journal List
- Acta Orthop
- v.90(4); 2019 Aug
Hypothesis-generating and confirmatory studies, Bonferroni correction, and pre-specification of trial endpoints
A p-value presents the outcome of a statistically tested null hypothesis. It indicates how incompatible observed data are with a statistical model defined by a null hypothesis. This hypothesis can, for example, be that 2 parameters have identical values, or that they differ by a specified amount. A low p-value shows that it is unlikely (a high p-value that it is not unlikely) that the observed data are consistent with the null hypothesis. Many null hypotheses are tested in order to generate study hypotheses for further research, others to confirm an already established study hypothesis. The difference between generating and confirming a hypothesis is crucial for the interpretation of the results. Presenting an outcome from a hypothesis-generating study as if it had been produced in a confirmatory study is misleading and represents methodological ignorance or scientific misconduct.
Hypothesis-generating studies differ methodologically from confirmatory studies. A generated hypothesis must be confirmed in a new study. An experiment is usually required for confirmation as an observational study cannot provide unequivocal results. For example, selection and confounding bias can be prevented by randomization and blinding in a clinical trial, but not in an observational study. Confirmatory studies, but not hypothesis-generating studies, also require control of the inflation in the false-positive error risk that is caused by testing multiple null hypotheses. The phenomenon is known as a multiplicity or mass-significance effect. A method for correcting the significance level for the multiplicity effect has been devised by the Italian mathematician Carlo Emilio Bonferroni. The correction (Bender and Lange 2001 ) is often misused in hypothesis-generating studies, often ignored when designing confirmatory studies (which results in underpowered studies), and often inadequately used in laboratory studies, for example when an investigator corrects the significance level for comparing 3 experimental groups by lowering it to 0.05/3 = 0. 017 and believes that this solves the problem of testing 50 null hypotheses, which would have required a corrected significance level of 0.05/50 = 0.001.
In a confirmatory study, it is mandatory to show that the tested hypothesis has been pre-specified. A study protocol or statistical analysis plan should therefore be enclosed with the study report when submitted to a scientific journal for publication. Since 2005 the ICMJE (International Committee of Medical Journal Editors) and the WHO also require registration of clinical trials and their endpoints in a publicly accessible register before enrollment of the first participant. Changing endpoints in a randomized trial after its initiation can in some cases be acceptable, but this is never a trivial problem (Evans 2007 ) and must always be described to the reader. Many authors do not understand the importance of pre-specification and desist from registering their trial, use vague or ambiguous endpoint definitions, redefine the primary endpoint during the analysis, switch primary and secondary outcomes, or present completely new endpoints without mentioning this to the reader. Such publications are simply not credible, but are nevertheless surprisingly common (Ramagopalan et al. 2014 ) even in high impact factor journals (Goldacre et al. 2019 ). A serious editorial evaluation of manuscripts presenting confirmatory results should always include a verification of the endpoint’s pre-specification.
Hypothesis-generating studies are much more common than confirmatory, because the latter are logistically more complex, more laborious, more time-consuming, more expensive, and require more methodological expertise. However, the result of a hypothesis-generating study is just a hypothesis. A hypothesis cannot be generated and confirmed in the same study, and it cannot be confirmed with a new hypothesis-generating study. Confirmatory studies are essential for scientific progress.
- Bender R, Lange S. Adjusting for multiple testing: when and how? J Clin Epidemiol 2001; 54 : 343–9. [ PubMed ] [ Google Scholar ]
- Evans S. When and how can endpoints be changed after initiation of a randomized clinical trial? PLoS Clin Trials 2007; 2 : e18. [ PMC free article ] [ PubMed ] [ Google Scholar ]
- Goldacre B, Drysdale H, Milosevic I, Slade E, Hartley P, Marston C, Powell-Smith A, Heneghan C, Mahtani K R. COMPare: a prospective cohort study correcting and monitoring 58 misreported trials in real time . Trials 2019; 20 : 118. [ PMC free article ] [ PubMed ] [ Google Scholar ]
- Ramagopalan S, Skingsley A P, Handunnetthi L, Klingel M, Magnus D, Pakpoor J, Goldacre B. Prevalence of primary outcome changes in clinical trials registered on ClinicalTrials.gov: a cross-sectional study . F1000Research 2014, 3 : 77. [ PMC free article ] [ PubMed ] [ Google Scholar ]
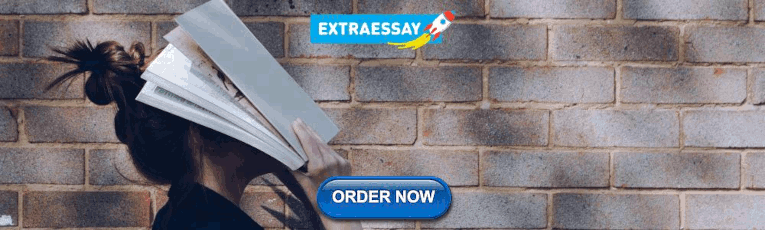
IMAGES
VIDEO
COMMENTS
However, hypothesis-generating case studies contribute to the process of theory construction rather than to theory itself (Ravenswood, 2011). For instance, it is particularly useful in explaining cases that do not fit into existing brief notes on Case Study (Collier, 1999, as cited in Levy, 2008 , p. 5).
Formulating Hypotheses for Different Study Designs. Generating a testable working hypothesis is the first step towards conducting original research. Such research may prove or disprove the proposed hypothesis. Case reports, case series, online surveys and other observational studies, clinical trials, and narrative reviews help to generate ...
the theoretical purposes of case studies and the case selection strategies or research de. signs used to advance those objectives. I construct a typology of case studies based on their purposes: idiographic (inductive and theory-guided), hypothesis-generating, hypothesis-testing, and plausibility probe case studies.
A case study is one of the most commonly used methodologies of social research. This article attempts to look into the various dimensions of a case study research strategy, the different epistemological strands which determine the particular case study type and approach adopted in the field, discusses the factors which can enhance the effectiveness of a case study research, and the debate ...
Case studies can be distinguished according to their purpose. Levy suggests a typology with four types: idiographic, hypothesis generating, hypothesis testing, and plausibility probes. Idiographic case studies aim to describe, explain, or interpret a singular historical epi - sode with no intention of generalizing beyond the case.
Although case studies have been discussed extensively in the literature, little has been written about the specific steps one may use to conduct case study research effectively (Gagnon, 2010; Hancock & Algozzine, 2016).Baskarada (2014) also emphasized the need to have a succinct guideline that can be practically followed as it is actually tough to execute a case study well in practice.
I distinguish between the theoretical purposes of case studies and the case selection strategies or research designs used to advance those objectives. I construct a typology of case studies based on their purposes: idiographic (inductive and theory-guided), hypothesis-generating, hypothesis-testing, and plausibility probe case studies.
theoretical types is the hypothesis-generating case study. Of studies of this type, Lijphart says: 'They start out with a more or less vague notion of possible hypotheses, and attempt to formulate definite hypotheses to be tested subsequently among a large number of cases.'2 The study upon which this paper is based satisfies entirely the first
In a recent article on the comparative method in the study of politics, 1 Arend Lijphart discusses the place of the case study and suggests that there are six types of case study, four of which may be characterized as theoretical, two as atheoretical. The first of the theoretical types is the hypothesis-generating case study.
Practical case studies are used to demonstrate how hypothesis-generation and -interpretation technologies work. These are based on "social infrastructure" applications like in-bound tourism, disaster management, lunar and planetary exploration, and treatment of infectious diseases.
Hypothesis generation is an early and critical step in any hypothesis-driven clinical research project. Because it is not yet a well-understood cognitive process, the need to improve the process goes unrecognized. Without an impactful hypothesis, the significance of any research project can be questionable, regardless of the rigor or diligence applied in other steps of the study, e.g., study ...
1. An Overview. Broadly, preclinical research can be classified into two distinct categories depending on the aim and purpose of the experiment, namely, "hypothesis generating" (exploratory) and "hypothesis testing" (confirmatory) research (Fig. 1).Hypothesis generating studies are often scientifically-informed, curiosity and intuition-driven explorations which may generate testable ...
The hypothesis-generating mode of research has been primarily practiced in basic science but has recently been extended to clinical-translational work as well. Just as in basic science, this approach to research can facilitate insights into human health and disease mechanisms and provide the crucially needed data set of the full spectrum of ...
Case Study: Hypothesis Generation with NYC Taxi Trip Duration Prediction . What is Hypothesis Generation? Hypothesis generation is an educated "guess" of various factors that are impacting the business problem that needs to be solved using machine learning. In framing a hypothesis, the data scientist must not know the outcome of the ...
A case study is an in-depth, ... Hypothesis-generating (or heuristic) case studies aim to inductively identify new variables, hypotheses, causal mechanisms, and causal paths. Theory testing case studies aim to assess the validity and scope conditions of existing theories.
The hypothesis-generating case study. A case study begun for any purpose can become a hypothesis-generating case study as well. One of the most valuable contributions of any method would be the generation of a new hypothesis that turned out to be valid or fruitful of fresh lines of investigation.
FUTURE DIRECTIONS. In assembling this long list of techniques for creative hypothesis generating I have tried to be inclusive, allowing some of the heuristics to overlap, e.g. several heuristics use variants of interaction effects or of deviant-case analysis as a creative device.
The problem with transposing the concepts of "hypothesis generation" and "hypothesis testing" is that hypothesis generation is relatively easy. It's basically coming up with testable ...
5. Phrase your hypothesis in three ways. To identify the variables, you can write a simple prediction in if…then form. The first part of the sentence states the independent variable and the second part states the dependent variable. If a first-year student starts attending more lectures, then their exam scores will improve.
and emphasize that one of the main tasks of case study analysis is to generate as many testable implications of one's hypotheses as possible in a given case (King et al., 1994).7 ... hypothesis generating case studies; hypothesis testing cases, which combine Lijphart's theory-confirming and theory-informing categories; ...
A free case study generator is a tool or system designed to automatically create detailed case studies. It typically uses predefined templates and may incorporate artificial intelligence (AI) to generate comprehensive analyses of specific situations, events, or individuals. This tool streamlines the process of crafting informative case studies ...
As part of a larger research program, we conducted a hypothesis-generating case-control study of selected herpes virus antibodies among individuals discharged from the US military with schizophrenia and pre- and postdiagnosis sera. Methods: Cases (n = 180) were servicemembers hospitalized and discharged from military service with schizophrenia ...
The Shigella Hypothesis Generating Questionnaire, modeled after the National Hypothesis Generating Questionnaire, is a tool created for public health professionals to interview people who are experiencing diarrheal illness and are suspected or confirmed to have Shigella infection. The questionnaire investigates exposures related to shigellosis ...
Case study, Module 2: Interpreting hypothesis generation results; Hypothesis generation through case exposures in multiple restaurant clusters example: Barton Behravesh C, et al. 2012. Multistate outbreak of Salmonella serotype Typhimurium infections associated with consumption of restaurant tomatoes, USA, 2006: hypothesis generation through ...
A case study is a research approach that is used to generate an in-depth, multi-faceted understanding of a complex issue in its real-life context. It is an established research design that is used extensively in a wide variety of disciplines, particularly in the social sciences. A case study can be defined in a variety of ways (Table.
Internationally, health systems are facing a crisis, driven by an ageing population, increasing complexity, multi-morbidity, rapidly advancing health technology and rising costs that threaten sustainability and mandate transformation and improvement [1, 2].Although research has generated solutions to healthcare challenges, and the advent of big data and digital health holds great promise ...
This paper proposes a method for conducting quantitative inductive research on survey data when the variable of interest follows an ordinal distribution. A methodology based on novel and traditional penalising models is described. The main aim of this study is to pedagogically present the method utilising the new penalising methods in a new application. A case was employed to outline the ...
First, we assessed the structural similarities of the dyes, generating a grouping hypothesis with DY3 and two Sudan dyes within one group. Daphnia magna were exposed acutely to equi-effective doses of all seven dyes (each at 3 doses and 3 time points), transcriptomics and metabolomics data were generated from 760 samples.
The purpose of this study is to highlight the relationship between historical monuments, viewed as factors generating tourist motivation and tourism. This was made possible by conducting a spatial analysis (at the level of territorial administrative units and localities) of the defining criteria for historical monuments and tourism in Bihor ...
Hypothesis-generating studies differ methodologically from confirmatory studies. A generated hypothesis must be confirmed in a new study. An experiment is usually required for confirmation as an observational study cannot provide unequivocal results. For example, selection and confounding bias can be prevented by randomization and blinding in a ...