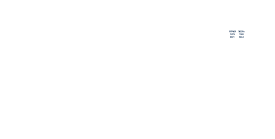
The deadly disease transmitted by mosquitoes is one of the leading causes of death in children. How did we eliminate the disease in some world regions and how can we continue progress against malaria?
By Max Roser and Hannah Ritchie
This article was first published in November 2015, and last revised in February 2024.
Malaria is a disease that is spread between people via infected mosquitoes. The bite of an infected Anopheles mosquito transmits a Plasmodium parasite that enters the victim’s blood and travels into the person’s liver, where it reproduces. The parasites then travel through the bloodstream and enter red blood cells, where they rapidly reproduce and burst red blood cells open; this repeats in cycles, leading to cycles of high fevers with shaking chills, and pain. In the worst cases, malaria leads to coma and death.
The parasites are single-celled microorganisms of the Plasmodium group. Plasmodium falciparum is the most lethal in humans, and responsible for most deaths.
Over half a million people died from the disease each year in the 2010s. Most were children, and the disease is one of the leading causes of child mortality .
For an overview of malaria — and how we can make progress against it — you can read the following essay:
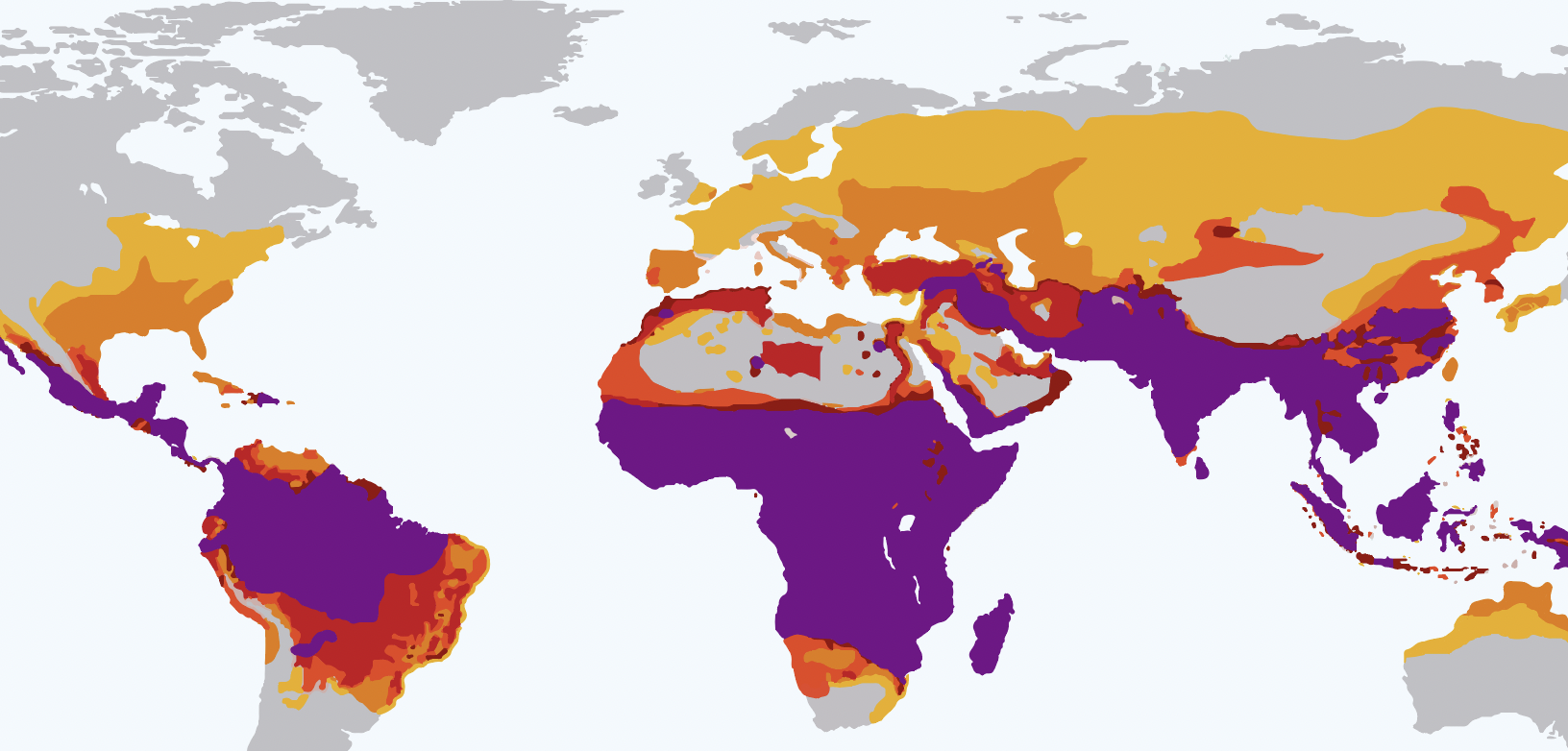
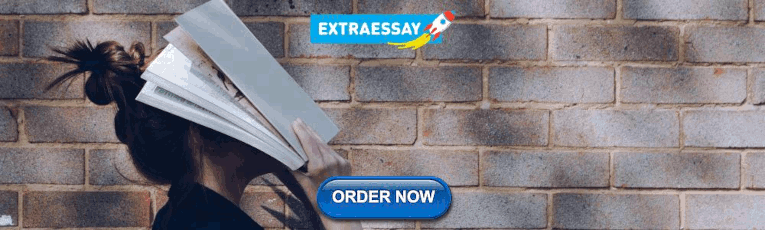
Malaria: One of the leading causes of child deaths, but progress is possible and you can contribute to it
We do not have to live in a world in which 1320 children die from a preventable disease every day.
See all interactive charts on malaria ↓
Malaria deaths
In the visualizations we provide estimates of the total number of deaths from the World Health Organization (WHO) from 2000 onwards, and the Institute of Health Metrics and Evaluation (IHME), Global Burden of Disease (GBD) from 1990 onwards.
These estimates are notably different across various countries which affects the total number of reported deaths. IHME figures, as shown, tend to be higher.
Malaria death estimates from WHO
The WHO has published estimates of the global number of people who die from malaria since 2000. This is shown in the chart.
The WHO estimates that the global death toll fell steadily between 2000 and 2015. Since then, progress has slowed. The number of deaths increased in 2020 as a result of disruptions during the COVID-19 pandemic . 1
Africa is the world region that is most affected by malaria. In recent years, the vast majority of deaths from malaria occurred on the African continent. But Africa is also the world region that has achieved most progress.
Malaria death estimates from IHME
The Institute of Health Metrics and Evaluation (IHME) has published estimates of the global number of people who die from malaria since 1990. This is shown in the chart.
The IHME estimates a rise, peak and fall between 1990 and 2017.
These estimates are notably higher than those of the WHO. Although divergent on the total number of malaria deaths, both the IHME and WHO estimate that the vast majority of deaths are in the African region.
Malaria deaths by age group
The chart below breaks down the annual number of deaths by age group.
These figures are those published by the IHME’s Global Burden of Disease study — the WHO does not publish country-level data on malaria deaths by age. Note that you can view these trends for any country in the chart.
It shows that the most vulnerable age group to malaria deaths are children under five years old.
Malaria death rates
The visualization shows the age-standardized death rate from malaria, measured as the death rate per 100,000 people in the population.
New cases of malaria
The map shows the number of new cases of malaria per 100,000 people across the world. As the chart shows, malaria cases are most common in the central part of Africa north and south of the equator.
The history of malaria
Humanity’s fight against malaria has a history of many thousand years. 2
For most of that time, humanity was exposed to the disease without any defense.
Malaria’s mark on the human body
The history of humanity is so closely entangled with the history of malaria that the disease left its mark on our bodies.
Sickle cell disorder is one of these marks. It is a genetic condition that deforms red blood cells to be sickle-shaped. Carriers suffer from attacks of pain from a young age and their lives are cut short by the condition. Tens of thousands of people globally die from the disease each year. 3
Why would such a genetic mutation not die out? The reason for the continued existence is that sickle cell disorder hinders the malaria parasite from reproducing, which means people who are carriers of the mutation — with one out of two mutations for it — have a protective advantage in environments where malaria exists.
The prevalence of sickle cell disorder today is a testament to the high-malaria environments in our species’ history. 4
Malaria’s mark on history
Malaria did not only leave its mark on our bodies, but in many ways also on our history. Malaria is thought to have been a secret ally that helped the Germanic and other tribes bring about the downfall of Rome.
By examining the teeth and bones of the Romans, archaeologists have brought together more and more evidence that the mosquito-borne fever once thrived in the warm, marshy river valleys of the Tiber and the Po in the Roman empire. The disease enfeebled the mightiest army of the ancient world directly through epidemics and indirectly by reducing the productivity in the agricultural sector and hastened the fall of Rome. 5
This disease that was powerful enough to afflict the mightiest armies also left its mark on the fate of societies and the lives of many other regions of the world. 6
Malaria’s mark on our drinks
One of the first treatments people discovered against malaria was quinine, which came from an extract from the bark of the cinchona tree in South America. 7
Quinine powder was either drunk with wine or dissolved in water, a mixture that became known as “tonic water”. But quinine is so bitter that tonic water, with concentrations high enough to be effective against malaria, was unpleasant to drink. The British colonial officers stationed in malarious India therefore started to add sugar, lime, and gin to it, and thereby created a drink that is still popular today: gin and tonic.
Malaria was common across half the world — since then it has been eliminated in many regions
Malaria left its mark on our history, our bodies, our drinks, and for thousands of years, caused the deaths of people all around the world.
But in the last few generations, humanity gained ground in this long-lasting battle against the disease. The map shows in which regions of the world malaria is prevalent today (in purple) and where it was prevalent in the past.
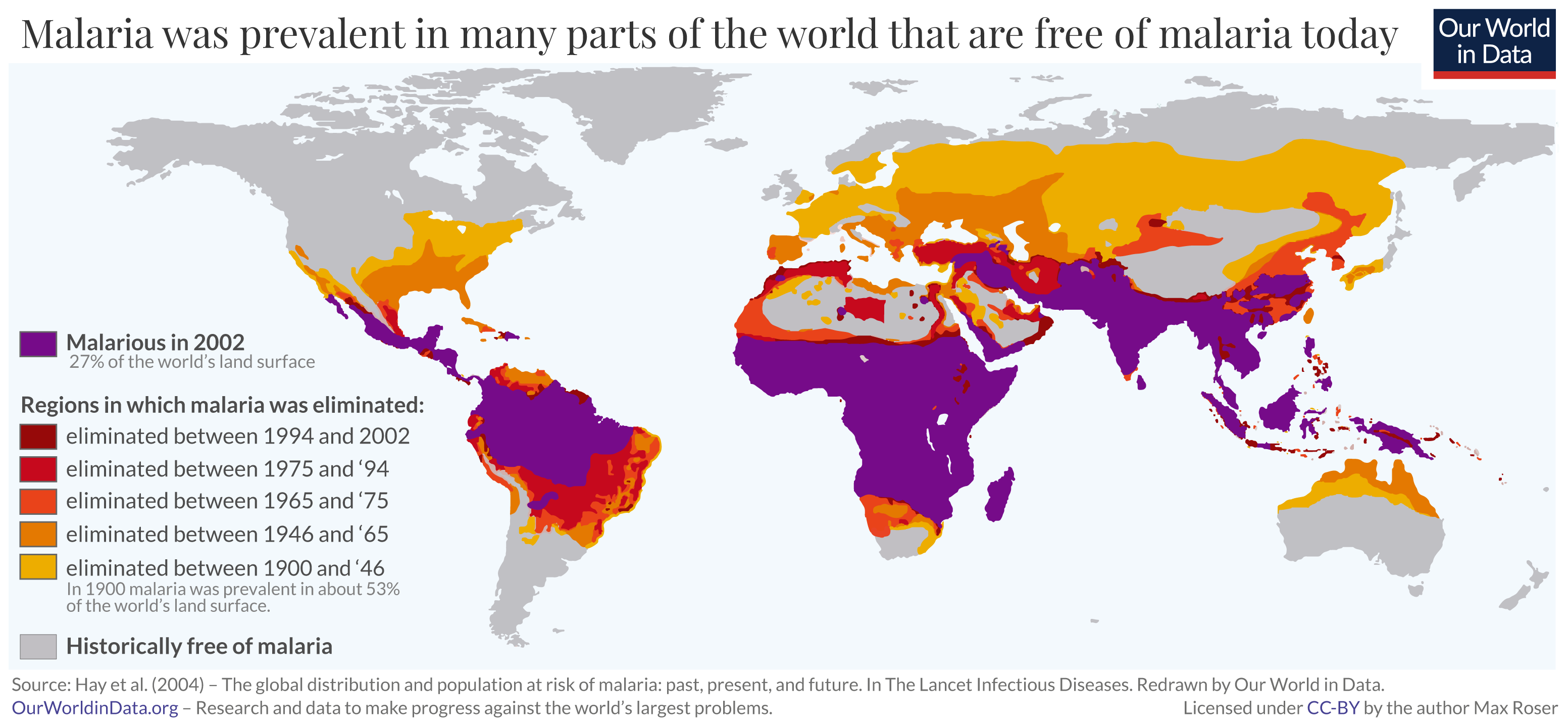
Just a few generations ago malaria was common in many more places around the world than it is today. Over the 20 th century, the disease was eliminated in many populous regions of the world, saving the lives of millions.
What the map makes clear is that malaria is not a tropical disease, but a disease that was eliminated everywhere except for the tropics.
Historically, malaria was prevalent in Europe and North America — the poet Friedrich Schiller contracted the disease in Mannheim, Oliver Cromwell in Ireland, and Abraham Lincoln in Illinois. 9
Since then the disease has been eliminated not only there, but also in East Asia and Australia and many parts in the Caribbean, South America, and Africa.
The researchers estimate that historically — and up to around 1900 — our ancestors were at risk from malaria across about half of the world’s land surface. By 2002, the area where humans are at risk of malaria contracted to a quarter (27%). 8
Why are these parts not malarious anymore?
Three factors were responsible for this global reduction of malarious regions: 10
First, public health measures, especially the widespread use of insecticides to attack the mosquito.
Second, drainage of swampland to expand agricultural land , which had the side effect of restricting breeding grounds for mosquitoes.
Third, social and economic development, which made treatment available to those who were infected, and also led to improvements in housing conditions and mosquito nets, which lowered the chances of infection in the first place.
All three factors — insecticides, land use change, and economic development — were major reasons that Europe and the other regions shown in shades of yellow, orange, and red are free of malaria today.
Malaria in the US
The map is taken from the US census from 1870. It shows in detail what the previous section discussed. Shown is the share of all deaths caused by malaria in the American Southeast at that time.
Malaria was very prevalent in this region in the past. Particularly along the coasts and along the Mississippi, malaria killed many Americans.
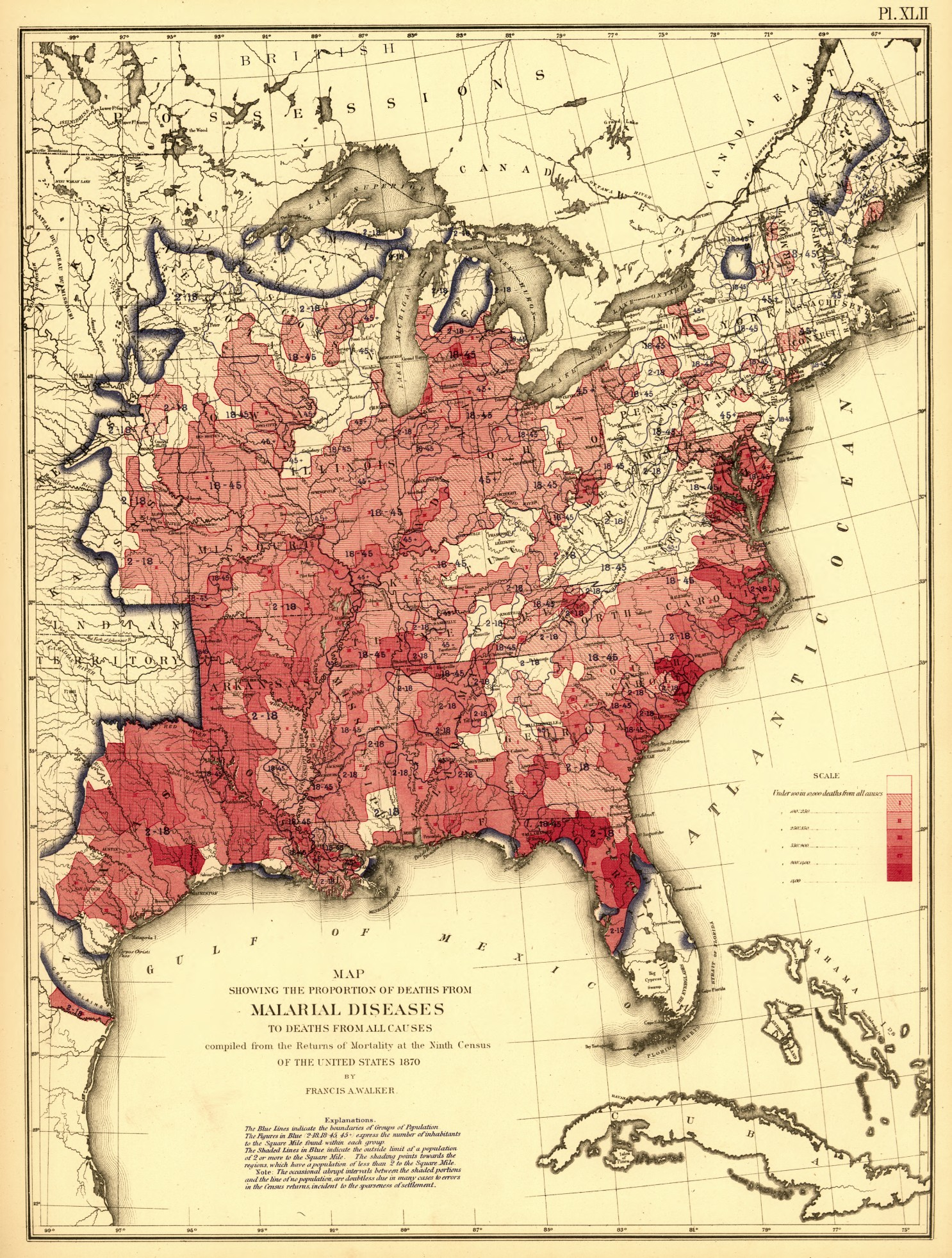
How can the world continue to make progress against malaria?
The world has already been very successful against this disease which is one of the deadliest diseases in humanity's history. How was this possible and what can we do to continue this progress?
Insecticide-treated bednets
The world is making progress against malaria thanks to insecticide-treated bednets.
Even after a century of progress against malaria, the disease remains devastating for millions. The World Health Organization estimates that 216 million suffered from the disease in 2016. 12
Fortunately, only a small fraction of malaria victims die of the disease. But those who die are the very weakest; three out of four malaria victims are children younger than 5 years old making it one of the leading causes of child mortality in the world today. 13
The world is making progress against malaria
One line of humanity’s attack on malaria is to progressively reduce the area in which malaria is prevalent. Another one is to prevent the transmission of the parasite in regions where it is still prevalent. It is a surprisingly simple technology that stopped transmission and saved the lives of millions in the last few years alone.
The years since the turn of the millennium were an extraordinarily successful era in the fight against malaria. The two maps show the change in malaria mortality for children in the region where the disease causes the highest death toll. 14
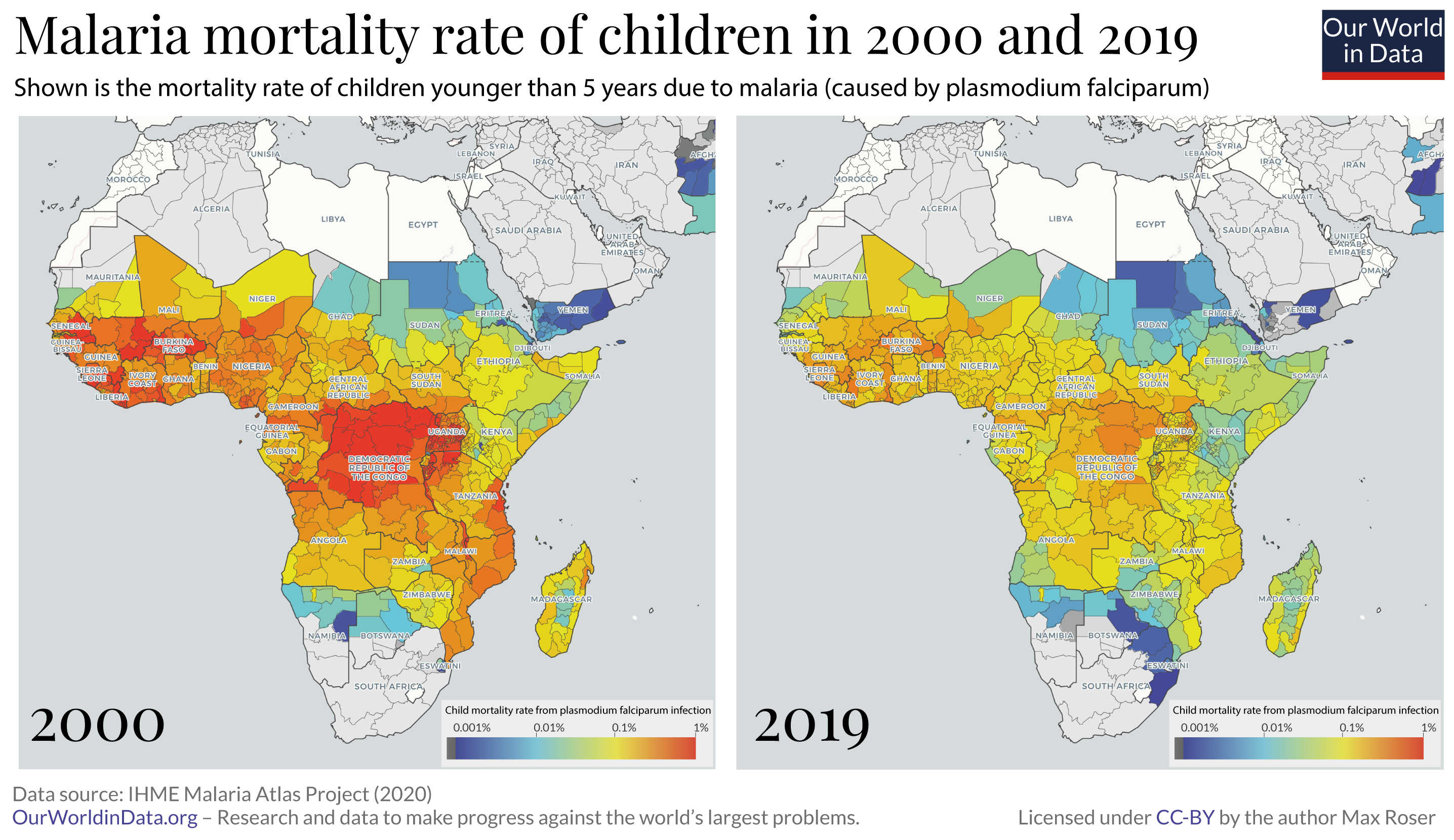
One of the most important contributors to the decline was the increased distribution of insecticide-treated bed nets.
Bed nets protect those who sleep under them, and the insecticide used on the bed nets kills the mosquitoes. So a community where a sufficiently high number of people sleep under bed nets the entire community is protected, regardless of whether they use the bed nets themselves. This is similar to the positive externality effect that vaccination has on communities.
Two other interventions that were important for the reduction in the disease burden of malaria were indoor residual spraying (IRS) of insecticides, and the treatment of malaria patients with artemisinin-based combination therapy (ACT).
Read more in our article:
Vaccines against malaria
Charles Alphonse Laveran discovered in 1880 that the Plasmodium parasite was the cause of malaria. 15 But all earlier attempts to develop vaccines were unsuccessful. Malaria vaccines such as SPf66 were insufficiently effective and until recently none of the scientific efforts led to a licensed vaccine. 16
This has changed with the malaria vaccine RTS,S , the world's first licensed malaria vaccine, which has been recommended for use by the WHO since 2021. The vaccine is undergoing pilot trials in select countries and it is hoped to protect against the parasite in the future. 17 Since then, another malaria vaccine has also been developed — the R21/Matrix-M vaccine, which has been recommended for use since 2023.
Malaria and economic prosperity
There are high economic costs of malaria.
Malaria was once prevalent in many of today's richest countries, but it was eliminated there over the last century. Today, malaria still affects some of the poorest countries in the world, as the chart shows.
This is partly because the poor finances make it hard to fight the disease, but also because the disease lowers the productivity of the economy.
In the chart below, you can see each country’s prevalence of malaria compared to their GDP per capita.
Interactive charts on malaria
World Malaria Report 2021 . Geneva: World Health Organization; 2021.
Wiesenfeld SL (1967) — Sickle-cell trait in human biological and cultural evolution. Science. 157:1134–40. Joy DA, Feng XR, Mu JB, et al. (2003) — Early origin and recent expansion of Plasmodium falciparum. Science. 300:318–21.
The Institute for Health Metrics and Evaluation’s 2019 Global Burden of Disease study estimated that around 40,000 people died from sickle cell disorder in 2019. Results are online here: https://vizhub.healthdata.org/gbd-compare/
See: Aidoo M, Terlouw DJ, Kolczak MS, McElroy PD, ter Kuile FO, Kariuki S, Nahlen BL, Lal AA, Udhayakumar V. (2002) — Protective Effects of the Sickle Cell Gene Against Malaria Morbidity and Mortality. In Lancet 2002; 359:1311-1312.
See Robert Sallares (2001) — Malaria and Rome: A History of Malaria in Ancient Italy and Robert Sallares, Abigail Bouwman and Cecilia Anderung (2012) — The Spread of Malaria to Southern Europe in Antiquity: New Approaches to Old Problems. Online here .
Daron Acemoglu, Simon Johnson, James A. Robinson (2002) — Reversal of Fortune: Geography and Institutions in the Making of the Modern World Income Distribution. In The Quarterly Journal of Economics, Vol. 117, No. 4 (Nov., 2002), pp. 1231-1294. Online here: ttps://economics.mit.edu/files/4127 John Luke Gallup and Jeffrey D. Sachs (2001) — The Economic Burden of Malaria https://www.ncbi.nlm.nih.gov/books/NBK2624/
On the history of the first effective treatments see Kennedy — A Brief History of Disease, Science and Medicine.
The original map was published in Simon I Hay, Carlos A Guerra, Andrew J Tatem, Abdisalan M Noor, and Robert W Snow (2004) — The global distribution and population at risk of malaria: past, present, and future. In The Lancet Infectious Diseases 2004 June; 4(6): 327–336. DOI: 10.1016/S1473-3099(04)01043-6. https://www.thelancet.com/journals/laninf/article/PIIS1473-3099(04)01043-6/fulltext I have digitized the Figure 1 using image tracing in Adobe Illustrator. The historical mapping of the prevalence of malaria is based on the pioneering work of Lysenko in the 1960s: Lysenko AJ, Semashko IN. Geography of malaria (1968) — A medico-geographic profile of an ancient disease. In: Lebedew AW, editor. Itogi Nauki: Medicinskaja Geografija. Academy of Sciences, USSR; Moscow: 1968. pp. 25–146. Lysenko AJ, Beljaev AE (1969) — An analysis of the geographical distribution of Plasmodium ovale. Bull World Health Organization; 40:383–94.
On the cause of Oliver Cromwell’s death see the FAQs at OliverCromwell.org , on Friedrich Schiller see Bayerischer Rundfunk here , on Abraham Lincoln see " The Physical Lincoln ". Other famous victims — although it is not always possible to diagnose the disease of historical figures — were German painter Albrecht Dürer, who contracted the disease in the Netherlands, and several popes, who died of the disease as malaria was very prevalent in Italy until recently.
See Hay et al (2004) above and de Zulueta J. (1994) — Malaria and ecosystems: from prehistory to posteradication. In Parassitologia. 1994 August. 36(1-2):7-15. World Bank (2009) — World Development Report (2009) - Part I: Reshaping Economic Geography. Washington, DC: World Bank. Online here .
This is taken from the Statistical Atlas from the 9th Census of the United States 1870 (published 1874). All maps and charts from the Statistical Atlas from the 9th Census of the United States 1870 are online at Radical Cartography here . This map shown here is available in high-resolution here .
Figures for 2016 according to the WHO: http://www.who.int/malaria/en/
The age-specific mortality figures are those published by the IHME’s Global Burden of Disease [the WHO does not publish country-level data on malaria deaths by age]. The share of children younger than 5 among malaria victims fell slightly over the course of the last generation, from 79% in 1990 to 72% in 2015. Here is the data: https://ourworldindata.org/grapher/malaria-deaths-by-age?stackMode=relative
Both the WHO and the IHME report a strong decline of malaria deaths since 2000. But throughout this period the IHME consistently estimates the number of annual deaths to be higher.
See Francis EG Cox (2010) — History of the discovery of the malaria parasites and their vectors. In Parasites and Vectors. Online here.
For an overview see Adrian V. S. Hill (2011) — Vaccines against malaria. In Philos Trans R Soc Lond B Biol Sci. 2011 Oct 12; 366(1579): 2806–2814. doi: 10.1098/rstb.2011.0091.
See RTS,S Clinical Trials Partnership (2015) — Efficacy and safety of RTS,S/AS01 malaria vaccine with or without a booster dose in infants and children in Africa: final results of a phase 3, individually randomised, controlled trial. In The Lancet, Volume 386, ISSUE 9988, P31-45, July 04, 2015. Online here .
Cite this work
Our articles and data visualizations rely on work from many different people and organizations. When citing this topic page, please also cite the underlying data sources. This topic page can be cited as:
BibTeX citation
Reuse this work freely
All visualizations, data, and code produced by Our World in Data are completely open access under the Creative Commons BY license . You have the permission to use, distribute, and reproduce these in any medium, provided the source and authors are credited.
The data produced by third parties and made available by Our World in Data is subject to the license terms from the original third-party authors. We will always indicate the original source of the data in our documentation, so you should always check the license of any such third-party data before use and redistribution.
All of our charts can be embedded in any site.
Our World in Data is free and accessible for everyone.
Help us do this work by making a donation.
- Open access
- Published: 06 June 2023
Predicting the risk of malaria re-introduction in countries certified malaria-free: a systematic review
- Guangyu Lu 1 , 2 na1 ,
- Dongying Zhang 3 na1 ,
- Juan Chen 4 na1 ,
- Yuanyuan Cao 5 ,
- Liying Chai 1 ,
- Kaixuan Liu 1 ,
- Zeying Chong 1 ,
- Yuying Zhang 1 ,
- Anna-Katharina Heuschen 7 ,
- Olaf Müller 7 ,
- Guoding Zhu 3 , 5 &
- Jun Cao 3 , 5
Malaria Journal volume 22 , Article number: 175 ( 2023 ) Cite this article
2775 Accesses
1 Citations
1 Altmetric
Metrics details
Predicting the risk of malaria in countries certified malaria-free is crucial for the prevention of re-introduction. This review aimed to identify and describe existing prediction models for malaria re-introduction risk in eliminated settings.
A systematic literature search following the PRISMA guidelines was carried out. Studies that developed or validated a malaria risk prediction model in eliminated settings were included. At least two authors independently extracted data using a pre-defined checklist developed by experts in the field. The risk of bias was assessed using both the prediction model risk of bias assessment tool (PROBAST) and the adapted Newcastle–Ottawa Scale (aNOS).
A total 10,075 references were screened and 10 articles describing 11 malaria re-introduction risk prediction models in 6 countries certified malaria free. Three-fifths of the included prediction models were developed for the European region. Identified parameters predicting malaria re-introduction risk included environmental and meteorological, vectorial, population migration, and surveillance and response related factors. Substantial heterogeneity in predictors was observed among the models. All studies were rated at a high risk of bias by PROBAST, mostly because of a lack of internal and external validation of the models. Some studies were rated at a low risk of bias by the aNOS scale.
Conclusions
Malaria re-introduction risk remains substantial in many countries that have eliminated malaria. Multiple factors were identified which could predict malaria risk in eliminated settings. Although the population movement is well acknowledged as a risk factor associated with the malaria re-introduction risk in eliminated settings, it is not frequently incorporated in the risk prediction models. This review indicated that the proposed models were generally poorly validated. Therefore, future emphasis should be first placed on the validation of existing models.
Despite the negative impact of the COVID-19 pandemic, there is progress toward malaria elimination. In 2021, 84 malaria-endemic countries compared to 108 in 2000 were identified [ 1 ]. Between 2000 and 2020, 23 countries were declared malaria-free based on zero indigenous malaria cases reported in 3 consecutive years [ 2 ]. In 2010–2021, total malaria cases in the E-2025 countries (malaria-eliminating countries for 2025) reduced by 82.8%, demonstrating continued efforts by countries toward their elimination goals [ 1 ]. With accelerated progress toward eliminating malaria in recent decades, the main concern is its re-emergence in areas where this disease was previously eliminated. Re-emergence can be facilitated through population movement from endemic countries, particularly due to the presence of competent vectors and favourable climatic conditions [ 3 ]. In recent years, reintroduced autochthonous malaria cases have even been sporadically reported from Italy, France, Spain and Greece [ 4 , 5 , 6 , 7 , 8 ].
Surveillance is a core and an effective intervention to support malaria elimination goal. The importation of parasites to an area with competent vectors makes the human population susceptible to risk. Thus, the rate of immigration of infected individuals and the prevalence of mosquito vectors are usually the focus of surveillance [ 3 , 9 , 10 , 11 , 12 ]. Moreover, meteorological conditions, including local temperatures, rainfall, and humidity, are frequently considered for predicting the risk of malaria re-introduction, as these strongly affect the life cycle and survival of parasites and vectors [ 13 , 14 , 15 , 16 , 17 ]. However, the risk of malaria re-introduction in eliminated settings depends on several factors; for example, the reduction of funding for malaria control programmes following successful elimination, inadequate awareness about the possibilities of parasite re-introduction to malaria-free regions, and socioeconomic parameters [ 13 , 18 ].
Winfried Schröder and Schmidt [ 19 ] established a malaria prediction model based on vector capacity and meteorological variables for northwestern Germany, where malaria was eradicated in the early 1950s. Linard et al. [ 20 ] assessed the risk of malaria re-emergence in southern France. Sainz-Elipe et al. [ 21 ] evaluated the transmission risk in Spain using the gradient model risk index. Romi et al . [ 22 ] assessed the risk of malaria re-introduction in central Italy through a multifactorial approach. Ranjbar et al . [ 9 ] predicted the risk of malaria re-introduction in two provinces in Iran. Such prediction models are crucial to facilitate the prioritization of allocation of the health system’s resources and take necessary action promptly to prevent the resurgence of malaria [ 9 ]. However, an up-to-date review of existing malaria re-introduction risk models in eliminated settings at a global level is lacking. Therefore, this study aimed to systematically review and critically appraise existing prediction models for malaria re-introduction risk in countries certified malaria-free.
This systematic review followed the Preferred Reporting Items for Systematic Reviews and Meta-Analyses (PRISMA) statement guidelines [ 23 ]. The protocol was registered on the international prospective register of systematic reviews (PROSPERO) database (CRD42022381245).
Search strategy
A literature search was performed in PubMed, Web of Science, Cochrane Library, China National Knowledge Infrastructure (CNKI), and reference lists of publications by using the following key words: “ malaria” , “ Malaria, Vivax ”, “ Malaria, Falciparum ”, “ acute malaria ”, “predict*”, “predictive model”, “prediction model”, “risk prediction”, “risk score”, “risk calculation”, and “risk assessment” (Additional file 1 : Appendix 1). This study initially searched databases on 01 June 2022, with an update on 16th March 2023. Citations of relevant articles were manually screened to identify additional studies.
Eligibility criteria
Studies that developed or validated prediction models for malaria re-introduction risk in countries that are already certifed as malaria-free, regardless of their design. Studies published in English or Chinese languages were included. The prediction rule was defined based on the combination of three or more risk factors. Studies that only analysed individual risk factors influencing malaria re-introduction without establishing prediction models were excluded. If more than two articles described one prediction model, the model was recorded once, and the information was extracted fully from all the relevant articles.
Data extraction and analysis
After eliminating duplicate entries, three researchers analyzed the titles and abstracts to confirm that all articles met the inclusion criteria and record the reasons for exclusion. Papers excluded for specific reasons were recorded. All studies were evaluated independently by at least two investigators. For potential relevant studies, the full text was obtained, and two investigators (JC and DYZ) independently assessed its eligibility. All data were independently characterized following a standardized protocol, including the title, first author name, year of publication, study location, source of data (surveillance data or cross-sectional survey), predictive variables in the established model, performance of the model (sensitivity and specificity), the internal and external validity of the established prediction model, and limitations of the model. Disagreements were resolved by consensus or through a discussion with a third reviewer. Considering the high heterogeneity in the prediction model, the limitations of the included studies were analyzed by thematic content analysis.
Quality assessment
Considering that the prediction model risk of bias assessment tool (PROBAST) is well acknowledged for a quality appraisal of prediction models, it was adopted to assess their risk of bias and applicability by the two independent reviewers. Moreover, the adapted Newcastle–Ottawa Scale (aNOS) scale was adapted to appraise the risk of bias in the included studies, as these were mainly observational [ 24 , 25 ]. PROBAST consisted of 20 items and included the specifics about the research design, study population, outcome of the model, predictors, handling of the data, and performance measures (Additional file 1 : Appendix 2). The aNOS scale included an assessment of the sample selection (4 criteria), comparability (1 criterion), and outcomes (2 criteria). Scores on this instrument range from 1 to 10, with higher scores indicating higher quality (Additional file 1 : Appendix 3). Any disagreements were resolved by mutual agreement.
A total of 8772 articles were identified after the electronic search of the databases and the removal of duplicates. The titles and abstracts of these articles were screened and 348 articles were subjected to full-text review (Fig. 1 ). Of these studies, 338 were excluded after reading their full texts because these did not fulfill the inclusion criteria (Additional file 1 : Appendix 4). Finally, 10 studies were included in the analysis [ 19 , 21 , 22 , 26 , 27 , 28 , 29 , 30 , 31 , 32 ].
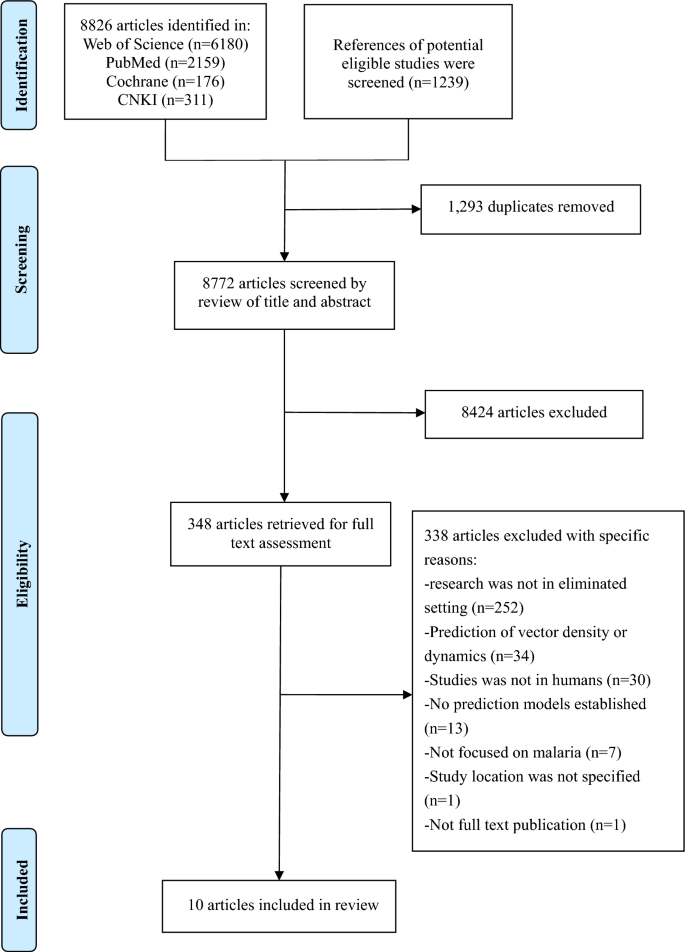
A summary flow of study selection process
General characteristics of the included studies
The general characteristics of the 10 articles reporting 11 malaria re-introduction risk prediction models in malaria eliminated settings were summarized in Table 1 . Among all included prediction models, seve n were developed for the European region (Germany, UK, Spain, Italy, and Greece), and four for China. The included studies were published between 2008 and 2023, with four (4/10, 40%) being published after 2020.
The methodology used in prediction models
Nearly half of the models (5/11, 45.6%) were developed by statistical and mathematical methods, followed by machining learning (3/11, 27.3%) and Delphi method (3/11, 27.3%). Of the 11 included malaria prediction models, the majority were developed based on routine surveillance data (8/11, 88.9%).
Variables included in the prediction model
Predictors included in the 11 prediction models were identified and classified into five categories, namely environmental and meteorological, vectorial, population migration, surveillance and response related factors, and other factors (Fig. 2 ).
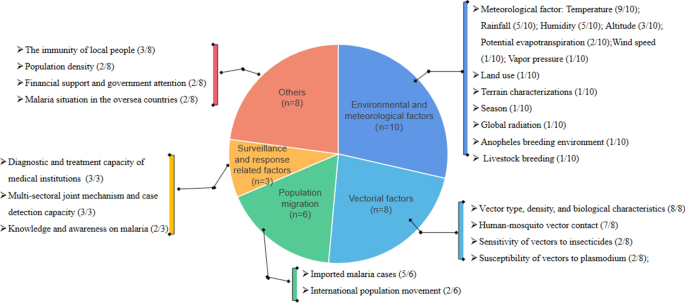
Five domains of predicting factors associated with malaria risk in eliminated settings
Environmental and meteorological factors
Environmental and meteorological factors were included in 10 models. Temperature parameters (9/10, 90%), rainfall (5/10, 50%), humidity (5/10, 50%) and altitude (3/10, 30%) were most frequently incorporated in the prediction models. Land use (1/10, 10%) was incorporated in one model, mainly including vegetation seasonal variations and land-cover categories [ 28 ]. Terrain characterizations, wind speed, global radiation, vapour pressure were identified in one model (1/10, 10%) [ 21 ].
Vectorial factors
Vector factors were incorporated in eight prediction models. The most commonly included vectorial factors identified included vectorial suitability (a combination of vector type, density, and biological characteristics) (8/8, 100%), human-vector contact (number of bites per person per day) (7/8, 87.5%), sensitivity of vectors to insecticides (2/8, 25%), and susceptibility of vectors to Plasmodium (2/8, 25%).
Population migration
Population migration was considered a predictor in six models. The socioeconomic status and epidemiological characteristics of imported cases of malaria were particularly interesting factors considered in predicting malaria re-introduction risk in countries certified malaria free and prevention of the re-introduction of malaria (5/6, 83.3%). Moreover, measurements of international population movement from malaria-endemic regions were considered in two models (33.3%).
Surveillance and response related factors
Surveillance and response related factors were considered in three models. Of which, diagnostic and treatment capacity was frequently incorporated, reflected by the capacity of medical institutions and stockpiling anti-malarial drugs. Moreover, researchers have acknowledged the capacity of cases detection and multi-sector joint mechanism is a significant predictor for malaria re-introduction risk in eliminated settings. Two models have included knowledge and awareness on malaria among the general population as a predictor for the risk of malaria re-introduction.
Other factors identified here included the immunity of the local people (3/8, 37.5%),financial support and government attention (2/8, 25%), population density (2/8, 25%), and malaria situation in oversea countries (2/8, 25%).
Performance and validation of the models
Of the 11 prediction models, two studies reported their model performance. Of which, one reported sensitivity and specificity of 98% and 98%, respectively [ 28 ], while one reported accuracy of 0.873 [ 27 ].
Only one model were externally validated by comparing potential distribution of malaria between 1961–1990 and 1859–1864 [ 26 ].
Limitations of the included studies
Of the 10 included studies, 5 reported their limitations of the established prediction models, which could be summarized into the following five aspects: (1) The model based on the Delphi method had the limitation of subjectivity (3/10, 30%). (2) The variables included in the prediction model were not comprehensive (2/10, 20%). (3) There is missing information in the data used in the model (1/10, 10%). (4) Modelling methods by mathematical approaches were time-consuming (1/10, 10%). (5) Predictive models lacked external validation (1/10, 10%) (Additional file 1 : Appendix 5).
All models showed a high risk of bias according to the PROBAST assessment, suggesting that their predictive performance, when used in practice, is probably more limited than expected. In particular, the predictions using the models may further lose reliability if not closely fitted to the local context. Bias was introduced by various sources, as summarized in Additional file 1 : Appendix 6. 11 models showed a high risk of bias for the analysis domain, mainly attributed to inappropriate handling of missing data, lack of model performance measure evaluation, and absence of validation. The quality of the included studies was also evaluated using aNOS. Of the 10 included studie s , scores on the aNOS scale ranged from three to eight, with nine models scoring seven and above, representing a generally favorable model quality performance. However, this scale only evaluated the aspects of sample selection, sample size, and the assessment of predictor variables (Additional file 1 : Appendix 7).
A total of 10 studies was included in this review and 11 prediction models for malaria re-introduction risk were identified in eliminated settings. Predictors mainly include environmental and meteorological, vectorial, population migration, and surveillance and response related factors. Models were mainly developed by statistical and mathematical, machining learning and delphi method. Most of the models were developed for the European region. All prediction models showed a high risk of bias owing to a combination of poor reporting, poor methodological conduct, and a lack of validation. Although published evidence for the routine use of the models in real malaria programmes is lacking, the findings provided a global overview of the existing prediction models and commonly used predictors for malaria risk in eliminated settings.
Approximately one third of the prediction models identified in this review were developed in China. China was certified as a malaria-free country in 2021 after several decades of active control and elimination efforts [ 33 ], and consequently, imported malaria cases and receptivity of certain areas posed a challenge to sustaining the success in elimination [ 34 ]. In recent years, the proportion of imported malaria cases in China is on the rise, especially those imported from sub-Saharan Africa (SSA), which have increased from 83% in 2017 to 91% in 2019 [ 35 ]. With the achievement of the malaria elimination goal in China in 2021, prevention of the re-introduction of malaria has attracted great research interest from scholars and public health workers [ 35 , 36 , 37 , 38 ]. Different with prediction models established in European countries mainly by statistical and mathematical method, Chinese scholars widely utilized Delphi expert consultation, through which expert opinions in the field could be obtained and integrated.
In countries cetificated malaria-free, malaria re-introduction may occur depending on multiple factors. Vectorial factors are frequently incorporated as indicators in predicting malaria risk in eliminated settings. Mosquito species, relative density, gonotrophic cycle, feeding behaviour, and biting activity (e.g., bites per person per day) are regularly considered during the evaluation of vector capacity. This further demonstrates that monitoring mosquito populations is important in malaria surveillance programmes in eliminated settings. However, ways to maintain high-quality mosquito surveillance to allow mosquito control experts to track exactly where the larval and adult mosquito populations are rising or falling is particularly challenging in eliminated settings, especially in resource-limited regions. Nevertheless, the competence of a malaria vector is strongly affected by climatic and environmental factors, which requires consideration [ 39 ]. Certain variables that can play a significant role in the complex dynamic processes of disease spread should be considered, including precipitation, humidity, availability of mosquito breeding sites, land use and land cover, and other ecological factors determining the developmental process of the vector mosquitoes [ 19 , 40 ]. For example, changes in land use and land cover changes were demonstrated to potentially exert a direct impact on the risk of re-emergence of the disease by affecting mosquito breeding grounds (e.g., the surface of marsh wetlands) and the contact rate between people and mosquitoes [ 20 ].
Climatic variables are considered environmental factors for increased risk of malaria because of their impacts on both the incubation rate of Plasmodium and mosquito vector activities. Previous researchers have employed a host of environmental factors and meteorological variables, including precipitation, temperature, altitude, and patterns of water availability to create computer models for predicting future malaria transmission [ 9 , 28 , 39 ]. However, the findings in this aspect are inconsistent. For example, elevated temperature was identified as the key meteorological factor correlated with malaria re-emergence in the Huang-huai River region of central China at the beginning of the twenty-first century and the re-emergence of malaria in Greece [ 29 , 41 ]. However, the increase in temperature was found not to mean an increase the malaria transmission risk, particularly if accompanied by a decrease in precipitation in Spain [ 21 ]. Although the temperature is important for parasite development, this indicator should be considered together with the variable related to water availability. Moreover, it is crucial and challenging for scientists to consider all available data and to communicate clearly about the complex interplay between climate and other factors in shaping disease trends [ 42 ]. In particular, considering that climate change is itself linked in multiple ways to emerging infectious diseases, global food security and public health [ 43 ], and routine surveillance of these predictors serves as an important basis for reemerging vectors and public health preparedness of potential malaria transmission risk of malaria.
In malaria-eliminated settings, malaria re-introduction is also affected by non-climatic factors including migration and human mobility. Previous scholars have emphasized that imported malaria is a particularly important factor and should be considered in the risk evaluation system in countries that have eliminated malaria but have the potency to supports local malaria transmission [ 44 ]. However, using such an indicator to predict malaria risk poses challenges. On the one hand, mobile and migrant populations may be underrepresented in routine case data and absent during household visits due to frequent travel [ 44 ], i.e., facility-based surveillance approaches may also fail to capture mobile and migrant populations who face barriers to accessing public health facilities, prefer private facilities, or those who do not seek care at all [ 45 ]. Moreover, quantifying population movement is not new but a daunting task in many contexts [ 46 ]. Travel history surveys, road traffic counts, border crossing questionnaires, shipping schedules, and question in census migration have long been used to obtain data on how people move[ 47 ], but each of these data types represents a snapshot of a small area, subpopulation or period, with limits on how much can be inferred beyond the collected data [ 47 ]. In addition to quantifying population movement, identifying vulnerable characteristics of migrating populations at high risk of importing malaria could provide an important basis for designing targeted interventions for the prevention of importation and further transmission in case of importation [ 48 ].
Malaria surveillance and response capacity are key for evaluation and preparedness for potential re-introduction in eliminated settings. In Europe, despite the substantial number of imported malaria cases and the documented presence of suitable anopheline vectors, autochthonous transmission has not been observed widely, probably due to early diagnosis and treatment afforded by efficient healthcare systems [ 49 ]. In contrast, India’s efforts to eliminate malaria have been largely challenged by an acute shortage of health workforce and weak public health surveillance systems [ 50 ]. Sri Lanka was once in the malaria pre-elimination phase, but as dichlorodiphenyltrichloroethane (DDT) spraying was discontinued, a disastrous malaria epidemic occurred occurred [ 18 ]. Health infrastructure and malaria surveillance capacity were the least commonly incorporated variables in predicting malaria risk in eliminated settings. This may be because of a lack of empirical measurement of competencies to assess public health infrastructure for infectious disease control and prevention, and the lack of easy-to-use instruments to assess the health capacity for infectious disease surveillance. Existing studies have mainly assessed the financial investment, diagnostic capacity of medical staff, diagnostic accuracy based on microscopy of public health workers, and implementation of a training program to reflect the surveillance capacity for malaria. In this regard, a well-acknowledged and user-friendly assessment tool of the surveillance capacity for infectious diseases in eliminated settings is necessitated.
Challenges to the methodology
The main aim of prediction models for malaria re-introduction risk is to increase preparedness for malaria risk and support public health decision-making. As very limited models were externally validated, it is important to assess the performance of risk prediction models by head-to-head comparison at a global level. The geography, social economy, and malaria epidemiology of the study setting must be carefully described so that the performance of the developed or validated model can be appraised in the given context, and users know which contexts the model applies to when making predictions. However, the included studies in the review mostly lacked an adequate description of their settings (e.g., study population), which leaves users of these models in doubt about the model’s applicability. It is recommend that all future prediction studies improve the description of their regional contexts and modelling choices.
Moreover, instead of developing and updating predictions in the local setting, compiling individual regional data from multiple countries with similar re-introductiom risks and healthcare systems might allow a better understanding of the general ability and implementation of prediction models. This approach could greatly improve the applicability and robustness of prediction models in routine surveillance, and multiple regional, national, and international collaborations are needed.
PROBAST is a risk-of-bias assessment tool designed for systematically reviewing diagnostic or prognostic prediction models, and therefore, does not always fit well with infectious disease transmission risk models. It is reflected in the participant, analytic domain, and reporting domains. Although the aNOS scale showed better results in evaluation, it is ultimately not designed for predictive modeling studies. As several modeling studies have been conducted in the field of infectious diseases, which mainly use routine surveillance data, a better fit and acknowledged quality appraisal tool for them is needed.
Implications for public health practice
All 11 reviewed prediction models showed a high risk of bias, and evidence from independent external validation of these models is currently lacking. Therefore, no any models could be recommended for use in practice at this point. It is anticipated that in the future, compiling individual regional data from multiple countries with similar transmission risks and healthcare systems could facilitate a better understanding of the general ability and implementation of prediction models. These data could be used to validate and update the currently available prediction models.
When building a new prediction model, it is recommend building on literature and expert opinion to select predictors rather than selecting them in a purely data-driven manner [ 51 ]. This is especially true for datasets with limited sample sizes. However, the application of data mining techniques may provide a way to generate potential predictors that are objective and reproducible. Based on multiple models identified in this review, researchers were encouraged to consider incorporating the following candidate predictors to build prediction models: vectorial, environmental and climatic, migration, surveillance and response related factors. By pointing to the most important methodological challenges and issues in designing and reporting by the currently available models, this review has provided potentially useful summarization for further studies aiming to develop new and improved models or validate and update existing ones.
Strengths and limitations
Although this is the first study to summarize the existing prediction models on malaria re-introduction risk in eliminated settings, some limitations need to be acknowledged. First, although this review included studies published in English and Chinese, an exclusion of study published in other language may potentially lead to the exclusion of models that could otherwise have been included in the review. Second, the strategy was broad and required the screening of a relatively large number of titles and abstracts. This review will be updated continuously to provide up-to-date information for healthcare decision-makers and professionals as more international research emerges over time. Moreover, the large heterogeneity in the methodology used for included prediction models did not allow a meta-analysis.
Models predicting malaria re-introduction risk in eliminated settings identified in this review had similar predictors, including climatic and environmental, vectorial factors, population mobility, malaria surveillance and response capacity. Although population movement is well acknowledged as a risk factor associated with malaria re-introduction risk in eliminated settings, it is not frequently incorporated in the risk prediction models. The existing evidence on prediction models for malaria risk in eliminated settings is lacking generalizability due to the lack of external validation. Therefore, future emphasis should be placed on the external validation of the existing models.
Availability of data and materials
All data generated or analysed during this study are included in this published article and its Additional file 1 .
WHO. World malaria report 2022. Geneva: World Health Organization; 2022.
Google Scholar
WHO. More malaria cases and deaths in 2020 linked to COVID-19 disruptions. Geneva: World Health Organization; 2021.
WHO. Guidelines on prevention of the reintroduction of malaria. Geneva: World Health Organization; 2007.
Baldari M, Tamburro A, Sabatinelli G, Romi R, Severini C, Cuccagna G, et al. Malaria in Maremma. Italy Lancet. 1998;351:1246–7.
Article CAS PubMed Google Scholar
Kampen H, Proft J, Etti S, Maltezos E, Pagonaki M, Maier WA, et al. Individual cases of autochthonous malaria in Evros Province, northern Greece: entomological aspects. Parasitol Res. 2003;89:252–8.
Article PubMed Google Scholar
Cuadros J, Calvente MJ, Benito A, Arévalo J, Calero MA, Segura J, et al. Plasmodium ovale malaria acquired in central Spain. Emerg Infect Dis. 2002;8:1506–8.
Article PubMed PubMed Central Google Scholar
Danis K, Baka A, Lenglet A, Van Bortel W, Terzaki I, Tseroni M, et al. Autochthonous Plasmodium vivax malaria in Greece, 2011. Euro Surveill. 2011. https://doi.org/10.2807/ese.16.42.19993-en .
Doudier B, Bogreau H, DeVries A, Ponçon N, Stauffer WM, Fontenille D, et al. Possible autochthonous malaria from Marseille to Minneapolis. Emerg Infect Dis. 2007;13:1236–8.
Ranjbar M, Shoghli A, Kolifarhood G, Tabatabaei SM, Amlashi M, Mohammadi M. Predicting factors for malaria re-introduction: an applied model in an elimination setting to prevent malaria outbreaks. Malar J. 2016;15:138.
Craig MH, Kleinschmidt I, Nawn JB, Le Sueur D, Sharp BL. Exploring 30 years of malaria case data in KwaZulu-Natal, South Africa: part I the impact of climatic factors. Trop Med Int Health. 2004;9:1247–57.
Thway AM, Rotejanaprasert C, Sattabongkot J, Lawawirojwong S, Thi A, Hlaing TM, et al. Bayesian spatiotemporal analysis of malaria infection along an international border: Hlaingbwe Township in Myanmar and Tha-Song-Yang District in Thailand. Malar J. 2018;17:428.
Tatarsky A, Aboobakar S, Cohen JM, Gopee N, Bheecarry A, Moonasar D, et al. Preventing the reintroduction of malaria in Mauritius: a programmatic and financial assessment. PLoS ONE. 2011;6: e23832.
Article CAS PubMed PubMed Central Google Scholar
Cohen JM, Smith DL, Cotter C, Ward A, Yamey G, Sabot OJ, et al. Malaria resurgence: a systematic review and assessment of its causes. Malar J. 2012;11:122.
Delon F, Mayet A, Thellier M, Kendjo E, Michel R, Ollivier L, et al. Assessment of the French national health insurance information system as a tool for epidemiological surveillance of malaria. J Am Med Inform Assoc. 2017;24:588–95.
Loha E, Lindtjørn B. Model variations in predicting incidence of Plasmodium falciparum malaria using 1998–2007 morbidity and meteorological data from south Ethiopia. Malar J. 2010;9:166.
Li T, Yang Z, Wang M. Temperature, relative humidity and sunshine may be the effective predictors for occurrence of malaria in Guangzhou, southern China, 2006–2012. Parasit Vectors. 2013;6:155.
Zhao X, Chen F, Feng Z, Li X, Zhou XH. The temporal lagged association between meteorological factors and malaria in 30 counties in south-west China: a multilevel distributed lag non-linear analysis. Malar J. 2014;13:57.
Nasir SMI, Amarasekara S, Wickremasinghe R, Fernando D, Udagama P. Prevention of re-establishment of malaria: historical perspective and future prospects. Malar J. 2020;19:452.
Schröder W, Schmidt G. Mapping the potential temperature-dependent tertian malaria transmission within the ecoregions of Lower Saxony (Germany). Int J Med Microbiol. 2008;298:38–49.
Article Google Scholar
Linard C, Ponçon N, Fontenille D, Lambin EF. A multi-agent simulation to assess the risk of malaria re-emergence in southern France. Ecol Modell. 2009;220:160–74.
Sainz-Elipe S, Latorre JM, Escosa R, Masià M, Fuentes MV, Mas-Coma S, et al. Malaria resurgence risk in southern Europe: climate assessment in an historically endemic area of rice fields at the Mediterranean shore of Spain. Malar J. 2010;9:221.
Romi R, Boccolini D, Vallorani R, Severini F, Toma L, Cocchi M, et al. Assessment of the risk of malaria re-introduction in the Maremma plain (Central Italy) using a multi-factorial approach. Malar J. 2012;11:98.
Moher D, Liberati A, Tetzlaff J, Altman DG. Preferred reporting items for systematic reviews and meta-analyses: the PRISMA statement. BMJ. 2009;339: b2535.
Wolff RF, Moons KGM, Riley RD, Whiting PF, Westwood M, Collins GS, et al. PROBAST: a tool to assess the risk of bias and applicability of prediction model studies. Ann Intern Med. 2019;170:51–8.
Modesti PA, Reboldi G, Cappuccio FP, Agyemang C, Remuzzi G, Rapi S, et al. Panethnic differences in blood pressure in Europe: a systematic review and meta-analysis. PLoS ONE. 2016;11: e0147601.
Lindsay SW, Hole DG, Hutchinson RA, Richards SA, Willis SG. Assessing the future threat from vivax malaria in the United Kingdom using two markedly different modelling approaches. Malar J. 2010;9:70.
Kamana E, Zhao J, Bai D. Predicting the impact of climate change on the re-emergence of malaria cases in China using LSTMSeq2Seq deep learning model: a modelling and prediction analysis study. BMJ Open. 2022;12: e053922.
Sudre B, Rossi M, Van Bortel W, Danis K, Baka A, Vakalis N, et al. Mapping environmental suitability for malaria transmission. Greece Emerg Infect Dis. 2013;19:784–6.
PubMed Google Scholar
Pergantas P, Tsatsaris A, Malesios C, Kriparakou G, Demiris N, Tselentis Y. A spatial predictive model for malaria resurgence in central Greece integrating entomological, environmental and social data. PLoS ONE. 2017;12: e0178836.
Lan ZY, Li Y, Huang YT, et al. Construction of a risk assessment indicator system for re-establishment of imported malaria. Chin J Schisto Control. 2022;34:163–71.
CAS Google Scholar
Li GQ, Liao Y, Chen MX, Shen XJ, Li WT, Zhou N, et al. Imported malaria in Changsha municipality –prevalence characteristics and re-transmission risk, 2010–2019. Chin J Public Health. 2022;38:485–9.
Liu Q, Liu M, Liu J. Construction of an indicator system for risk assessment on imported malaria during post-elimination period in China Malaria. J. 2023;44:67–73.
WHO. From 30 million cases to zero: China is certified malaria-free by WHO. Geneva: World Health Organization; 2021.
Cao J, Newby G, Cotter C, Hsiang MS, Larson E, Tatarsky A, et al. Achieving malaria elimination in China. Lancet Public Health. 2021;6:e871–2.
Hu X, Wu S, Weng Y, Han H, Tian L, Lu Y, et al. Epidemiology of global malaria and imported malaria in China. Dis Surveill. 2021;36:1057–62.
Lei L. Risk assessment of secondary transmission by imported malaria in Jiangxi province. Chin Center Dis Cont Prev. 2017;29(2):182.
Li GQ, Liao Y, Hong XQ, Chen MX, Zhou N, Ren X, et al. Risk prediction of imported malaria re-transmission in Changsha based on SEI-SEI model. Modern Preventive Medicine. 2021;48:1921–4.
Li Q. Risk analysis of preventing re-transmission after malaria elimination in Fujian Province. Fuzhou: Fujian Medical University; 2021.
Chen Z, Shi L, Zhou XN, Xia ZG, Bergquist R, Jiang QW. Elimination of malaria due to Plasmodium vivax in central part of the People’s Republic of China: analysis and prediction based on modelling. Geospat Health. 2014;9:169–77.
Gao HW, Wang LP, Liang S, Liu YX, Tong SL, Wang JJ, et al. Change in rainfall drives malaria re-emergence in Anhui Province. China PLoS One. 2012;7: e43686.
Zhou SS, Huang F, Wang JJ, Zhang SS, Su YP, Tang LH. Geographical, meteorological and vectorial factors related to malaria re-emergence in Huang-Huai River of central China. Malar J. 2010;9:337.
Rillig MC, Lehmann A, Bank MS, Gould KA, Heekeren HR. Scientists need to better communicate the links between pandemics and global environmental change. Nat Ecol Evol. 2021;5:1466–7.
Patz JA, Epstein PR, Burke TA, Balbus JM. Global climate change and emerging infectious diseases. JAMA. 1996;275:217–23.
Chuang TW, Soble A, Ntshalintshali N, Mkhonta N, Seyama E, Mthethwa S, et al. Assessment of climate-driven variations in malaria incidence in Swaziland: toward malaria elimination. Malar J. 2017;16:232.
Smith JL, Ghimire P, Rijal KR, Maglior A, Hollis S, Andrade-Pacheco R, et al. Designing malaria surveillance strategies for mobile and migrant populations in Nepal: a mixed-methods study. Malar J. 2019;18:158.
Feacham RGA. Shrinking the malaria map A Guide on Malaria Elimination for Policy Makers. San Francisco, USA: University of California; 2009.
Tatem AJ. Mapping population and pathogen movements. Int Health. 2014;6:5–11.
Tatem AJ, Smith DL. International population movements and regional Plasmodium falciparum malaria elimination strategies. Proc Natl Acad Sci USA. 2010;107:12222–7.
Piperaki ET, Daikos GL. Malaria in Europe: emerging threat or minor nuisance? Clin Microbiol Infect. 2016;22:487–93.
Dash AP, Valecha N, Anvikar AR, Kumar A. Malaria in India: challenges and opportunities. J Biosci. 2008;33:583–92.
Zinszer K, Verma AD, Charland K, Brewer TF, Brownstein JS, Sun Z, et al. A scoping review of malaria forecasting: past work and future directions. BMJ Open. 2012;2: e001992.
Buczak AL, Baugher B, Guven E, Ramac-Thomas LC, Elbert Y, Babin SM, et al. Fuzzy association rule mining and classification for the prediction of malaria in South Korea. BMC Med Inform Decis Mak. 2015;15:47.
Download references
Acknowledgements
Not applicable
This work was supported by the National Natural Science Foundation of China [Grant no. 71904165], the Open Project Program of Jiangsu Key Laboratory of Zoonosis [Grant no. R2208], the Open Project Program of International Research Laboratory of Prevention and Control of Important Animal Infectious Diseases and Zoonotic Diseases of Jiangsu Higher Education Institutions [01], and the Open Project Program of National Health Commission Key Laboratory of Parasitic Disease Control and Prevention and Jiangsu Provincial Key Laboratory on Parasite and Vector Control Technology [Grant no. wk023-007]. The funding sources had no role in the design and conduct of the study; the collection, management, analysis, and interpretation of the data; and preparation, review, or approval of the manuscript.
Author information
Guangyu Lu, Dongying Zhang and Juan Chen contributed equally.
Authors and Affiliations
School of Public Health, Medical College of Yangzhou University, Yangzhou University, Yangzhou, 225007, China
Guangyu Lu, Liying Chai, Kaixuan Liu, Zeying Chong & Yuying Zhang
Jiangsu Key Laboratory of Zoonosis, Yangzhou, China
Center for Global Health, School of Public Health, Nanjing Medical University, Nanjing, China
Dongying Zhang, Guoding Zhu & Jun Cao
School of Nursing, Medical College of Yangzhou University, Yangzhou University, Yangzhou, China
National Health Commission Key Laboratory of Parasitic Disease Control and Prevention, Jiangsu Provincial Key Laboratory On Parasite and Vector Control Technology, Jiangsu Institute of Parasitic Diseases, Wuxi, China
Yuanyuan Cao, Guoding Zhu & Jun Cao
Nanjing Health and Customs Quarantine Office, Nanjing, China
Institute of Global Health, Medical School, Ruprecht-Karls-University, Heidelberg, Germany
Anna-Katharina Heuschen & Olaf Müller
You can also search for this author in PubMed Google Scholar
Contributions
The study has been designed by GYL, GDZ and JC. All authors conceived and planned the work that led to the manuscript or played an important role in the acquisition, analysis and interpretation of the data or both. All authors wrote the paper and/or made substantive suggestions for revision. All authors approved the final submitted version.
Corresponding authors
Correspondence to Guangyu Lu , Guoding Zhu or Jun Cao .
Ethics declarations
Ethics approval and consent to participate.
Not applicable.
Consent for publication
Competing interests.
The authors declare that they have no competing interests.
Additional information
Publisher's note.
Springer Nature remains neutral with regard to jurisdictional claims in published maps and institutional affiliations.
Supplementary Information
Additional file 1: appendix 1..
Search strategy and databases. Appendix 2. PROBAST: Assessment of Risk of Bias and Concerns Regarding Applicability. Appendix 3. Adapted version of the Newcastle-Ottawa Scalechecklist for assessing the quality of cross-sectional studies. Appendix 4. Exclude after reading the whole article. Appendix 5. Limitation of included studies. Appendix 6. Quality assessment of included studies using the PROBAST scale for predictive modeling studies. Appendix 7. The aNOS for assessing the quality of cross-sectional studies.
Rights and permissions
Open Access This article is licensed under a Creative Commons Attribution 4.0 International License, which permits use, sharing, adaptation, distribution and reproduction in any medium or format, as long as you give appropriate credit to the original author(s) and the source, provide a link to the Creative Commons licence, and indicate if changes were made. The images or other third party material in this article are included in the article's Creative Commons licence, unless indicated otherwise in a credit line to the material. If material is not included in the article's Creative Commons licence and your intended use is not permitted by statutory regulation or exceeds the permitted use, you will need to obtain permission directly from the copyright holder. To view a copy of this licence, visit http://creativecommons.org/licenses/by/4.0/ . The Creative Commons Public Domain Dedication waiver ( http://creativecommons.org/publicdomain/zero/1.0/ ) applies to the data made available in this article, unless otherwise stated in a credit line to the data.
Reprints and permissions
About this article
Cite this article.
Lu, G., Zhang, D., Chen, J. et al. Predicting the risk of malaria re-introduction in countries certified malaria-free: a systematic review. Malar J 22 , 175 (2023). https://doi.org/10.1186/s12936-023-04604-4
Download citation
Received : 10 January 2023
Accepted : 22 May 2023
Published : 06 June 2023
DOI : https://doi.org/10.1186/s12936-023-04604-4
Share this article
Anyone you share the following link with will be able to read this content:
Sorry, a shareable link is not currently available for this article.
Provided by the Springer Nature SharedIt content-sharing initiative
- Malaria elimination
- Re-introduction risk
- Surveillance
- Prediction models
- Systematic review
Malaria Journal
ISSN: 1475-2875
- Submission enquiries: [email protected]
- Research article
- Open access
- Published: 28 July 2020
A five-year trend analysis of malaria surveillance data in selected zones of Amhara region, Northwest Ethiopia
- Damtie Lankir 1 ,
- Samrawit Solomon 1 &
- Addisu Gize 2
BMC Public Health volume 20 , Article number: 1175 ( 2020 ) Cite this article
5599 Accesses
19 Citations
Metrics details
Trend analysis of malaria surveillance data is essential to inform stakeholders on progress towards malaria control. From the total 387,096 cases of malaria reported in Amhara region in 2017, 167,079 (43.2%) cases were in Central, North and West Gondar zones. From this total figure of zones, 15,445 (9.2%) were ≤ 5 years which contributes 4% of cases in the region. So, the purpose of this study was to analyze trends of malaria parasite in Selected Zones of Amhara Region, Northwest Ethiopia.
A Retrospective study was conducted on purposely selected Central, North and West Gondar zones from July 1–30/ 2018. Data were collected, entered, cleaned, analyzed and interpreted using Microsoft Excel-2010. Different tables, figures and maps were used to present results.
A total of 2,827,722 cases have been received a diagnostic test of; Microscopy 1,712,193(60.56%) and Rapid Diagnostic Test (RDT) 1,115,529(39.44%). Trends of total patients treated as confirmed and clinical malaria cases in July 2017–June 2018 were decreased to 139,297 (14%) as compared from July 2015–June 2016, 249,571(25%). From total cases received diagnostic tests only 1,003,391 (36%) were confirmed and clinical cases treated with antimalaria. Of these Plasmodium falciparum and vivax malaria cases were confirmed to be 1002,946 (99.96%) and clinical malaria cases were 445(0.04%), respectively.
Risk of infection and diagnostic effort were high in West Gondar Zone. The Amhara public health institute including health Bureau, stakeholders and all responsible bodies should give special standing to highest malaria districts of West Gondar zone.
Peer Review reports
Malaria is the most important parasitic and vector born disease caused by the four species ( Plasmodium vivax, Plasmodium falciparum, Plasmodium malariae, Plasmodium ovale ) in Africa [ 1 ] which is a life-threatening disease. In areas with high transmission of malaria, children under 5 are particularly susceptible to infection, illness and death; more than two thirds (70%) of all malaria deaths occur in this age group. The number of under-5 malaria deaths has declined from 440,000 in 2010 to 285,000 in 2016. However, malaria remains a major killer of children under five years old, taking the life of a child every two minutes [ 2 ].
There have been over 9 million cases of malaria in the East African nation since January 2016, according to the report by the United Nation humanitarian office. In Zambia 119,593 (7.7/1000 population) cases were recorded in the first 11 weeks of 2017 compared to 73,019 and 82,328 cases for the same periods in 2016 and 2015. Even though Malaria prevention and control interventions have recently undergone major scale-up in Africa, Malaria disease burden is reported in several countries including Ethiopia and other East African countries. There is complexity within countries, including large geographical variation incidence and differing upward or downward trends between indicators [ 3 ].
Ethiopia is one of the most malaria endemic countries in Africa [ 4 ]. The population is reaching 105,350,020 in July 2017. Sixty eight percent of the population is at risk of malaria [ 5 ]. Approximately 75% of the geographic regions have significant malaria transmission risk. Among the leading communicable disease in Ethiopia, malaria accounts for about 30% of the overall Disability Adjusted Life Years lost [ 4 ].
According to the objective of National Malaria Policies and Strategies of Ethiopia, Program for Alternative Technology in Health (PATH), Malaria Control and Evaluation Partnership in Africa (MACEPA), December 2015 report; by 2017 and beyond, 100% of suspected malaria cases are diagnosed using Rapid Diagnostic Tests (RDTs) or microscopy within 24 h of fever onset (4–6). From 2009 to 2013 a total estimate of 13 million confirmed and clinical cases of malaria were reported in Ethiopia.
Stronger malaria surveillance systems could be enabled in endemic regions, to prevent outbreaks and resurgences, to track progress, and to hold governments and the global malaria community accountable from the updated information [ 5 ]. The information also needed to prioritize the most affected areas by malaria in different years and months and also may give baseline information for further study. So, the purpose of this study was to describe a five years malaria trend of Central, North and West Gondar zones and its districts (woreda’s) in Amhara region, Northwest Ethiopia.
Study setting
The study was conducted on purposively selected Central, North and West Gondar zones of Amhara regional state. Amhara region is one of the low land malarious regions of Ethiopia. Amhara National Regional state is one of the Ethiopia Regions with total population of 23,442,192 in 2017 estimate. The region consists of three major geographical zones, highlands or “Dega” (beyond 2300 m above sea level), semi-highlands or “Woyna Dega” (1500 to 2300 m above sea level) and lowlands “Kolla” or hot climatic zones (below 1500 m above sea level) accounting 25%, 44%, and 31% respectively [ 6 ]. The regional state was made up of 13 administrative zones.
Central, North and West Gondar Zones (previous North Gondar zone) are under the thirteen zones. These zones are located at a Latitude of 12° 44′ 59.99“ N and Longitude of 37° 00’ 0.00” E with an elevation of 1422 m above sea level. It is bounded by bordered on the South by lake Tana, West Gojjam, Awi and the Benishangul-Gumuz Region, on the West by South Sudan, on the North by the Tigray Region, on the East by Wag Hemra and on the South East by South Gondar Zone. Central Gondar Zone cover thirteen woreda, North Gondar zone cover seven Woereda and West Gondar zone cover six woredas. The first Health center in Ethiopia Kolla Diba is found in central Gondar zone. The total populations residing in Central Gondar were 2,896,928, North Gondar was 912,112 and West Gondar was 328,006. Generally a total of 4,137,046 populations were lived in these three zones. Of these Males were 2,107,981 and Female were 2,029,064 with a ratio of 1:1.
Study design and period
A retrospective study design was conducted in Central, North and West Gondar zones form weekly reported malaria surveillance data of Amhara Public Health Institute (APHI), Public Health Emergency Management (PHEM) directorate from July 1–30/ 2018.
All Outpatient Department (OPD) and Inpatient Department (IPD) confirmed and clinical reported malaria cases residing in all districts/woredas of Central, North and West Gondar zones which covers 5 years period.
Data collection instrument
Secondary data collection checklist was prepared and used to extract the documented malaria surveillance data of weekly reported cases from PHEM directorate office. This checklist contain variables like: Zone, Woreda, total population, Budget Year, Month, Reporting date, Total numbers in Outpatient department (OPD), Total incidence of parasite (IP), Total number of death, Blood Film (BF) test, Rapid Diagnostic Test (RDT), Total number of malaria (confirmed and clinical) in OPD, and age groups, etc.
Inclusion and exclusion criteria
Since it was retrospectively study, a total of five year period confirmed and clinically treated cases for malaria were included in the study and all incomplete data were excluded.
Data collection process
A regional weekly collected malaria data base was extracted to zones and woredas through filtering of important variables and filtered data were used for analysis. Total confirmed and clinically treated cases were cheeked as total inpatient and outpatient visited cases to have a quality data.
Data processing and analysis
Data were entered, processed and analyzed into Microsoft office Excel 2010. A descriptive analysis, using mean and percentage was calculated. Different graphs and tables were used to present trends of malaria cases and total population.
Ethical consideration
Permission letter was gained from Amhara public health institute; research and technology transfer directorate; and permission letter was secured from Amhara Public Health Institute (APHI) stated the ethics number as (APHI/ 5011, 06/02/11). The purposes and the importance of the study were stated this unique objective of the study for the region which serve as base line information to Public Health Emergency Management (PHEM) directorate office, and for different stake holders. PHEM directorate is the owner of this five years data and it direct stakeholder or programmer for malaria prevention and intervention measures. Confidentiality was assured at all levels of the study using password protected computer and through deleting all identifiers.
Operational definition
Suspected malaria case: - Clinical diagnosis of malaria is made in a patient who has fever or history of fever in the last 48 h and lives in malaria-endemic areas or has a history of travel within the last 30 days to malaria-endemic areas [ 7 ].
Confirmed malaria case: - A suspected case of malaria in which malaria parasites have been verified by microscopy or RDT.
Annual blood examination rate: - Smears examined in a year X 100 / Total population.
Annual falciparum incidence: - Total positive PF in a year × 1000 / Total population.
Annual parasite incidence: - Total number of positive slides for malaria parasite in a year × 1000 / Total population.
Trends of malaria cases by zones and years
Within five years from July 2013–June 2018 a total of 5,735,065 populations were attended health facilities in Central, North and west Gondar zones. The average annual health facility attendance rate of these zone were a total of 1,147,013 patients (285/1000 population) each year. In five years period a total of 2,827,722 cases were receiving a diagnostic test of Microscopy 1,712,193 (60.56%) and RDT 1115529 (39.44%). From total cases received diagnostic tests only 1,003,391 (36%) were confirmed and clinical cases treated with antimalaria. Of these treated with antimalarial cases, Plasmodium falciparum and vivax malaria cases were 1002,946 (99.96%) and clinical malaria cases were 445(0.04%) in Central, North and West Gondar zones. Among the total confirmed malaria cases 8623 (0.86%) were pregnant women positive for malaria. Average annual malaria cases were 200,589 (55 cases per 1000 population). The trends of total clinically and confirmed malaria cases were increased from 220,406(22%) in July 2013–June 2014 to 249,474 (25%) in July 2015–June 2016 in Central, North and West Gondar zone. In July 2017–June 2018 total patients treated as confirmed malaria cases were decreased by 139,296 (14%) as compared to July 2015–June 2016; 249,474(25%). Trends of malaria cases by zones were presented in Fig. 1 .
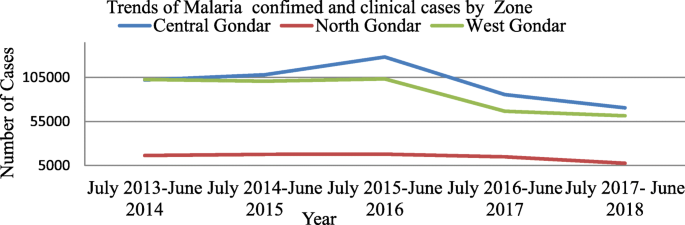
Five years trends of total confirmed and clinical malaria Cases in Central, North and West Gondar Zones from July 2013–June 2018
Trends of confirmed malaria species by years and zones
From a total of 1002, 946 confirmed malaria cases in Central, North and West Gondar zones; Plasmodium falciparum species were 736,149 (73.4%) and Plasmodium vivax were 266,797 (26.6%). Within five years period in West, Central and North Gondar Zones Plasmodium falciparum accounts 337,807(77.7%), 351,433(71.1%) and 46,909(63.2%), respectively. Plasmodium vivax infection were 96,836(22.3%) in West Gondar, 142,646(28.9%) in Central Gondar and 27,315(36.8%) in North Gondar zones. On average the number of malaria cases per year were 86,929(273/1000 population), 98,816 (35/1000 population) and 14,845(17/1000 population) in West, Central and North Gondar zones respectively. Total confirmed PF and PV species by years were presented in Fig. 2 .
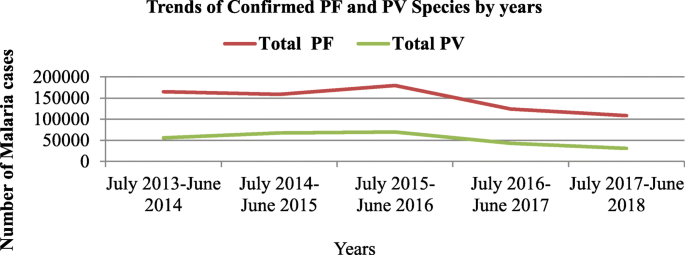
Trends of PF and PV cases in Central, North and West Gondar zones from July 2013–June 2018
Trends of malaria cases by months and epidemiological (EPI) weeks
The trends of malaria cases in July 2014–June 2015 and July 2016–June 2017 were high on November and lowest on April in both years. In July 2017–June 2018 highest number of cases was registered on October and lowest numbers of cases were reported on April. The highest malaria cases were reported in EPI week of 45, 46 and 47 in these three zones within five years period. Trends of malaria transmission by months and EPI week were presented in Figs. 3 and 4 respectively.
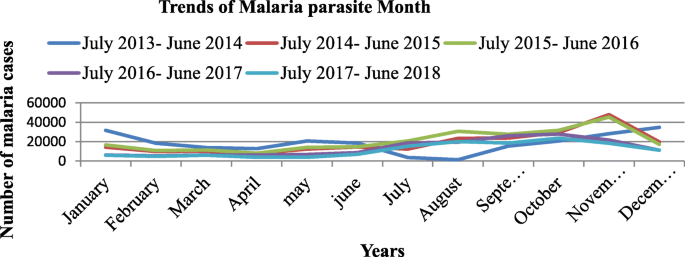
Transmition of malaria parasite by month in Centeral, North and West Gondar Zones from July 2013–June 2018
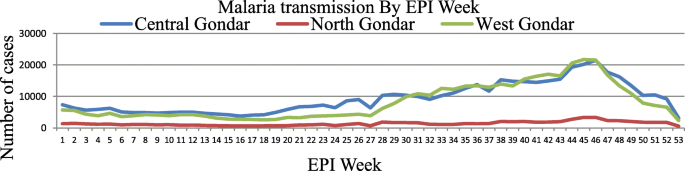
Malaria transmission by EPI weeks in Centeral, North and West Gondar Zones from July 2013–June 2018
Trends of PF and PV transmission by months
Annual average highest monthly Plasmodium falciparum transmission were observed in November with 24,993(17%) cases followed by October 20,873(14%) and September 16,892(11%) cases respectively. Plasmodium vivax transmission were also high in November, October and September with 7365(14%), 5813(11%) and 5431(10%) cases respectively at Central, North and West Gondar zones. Average Seasonal transmissions of falciparum and vivax malaria by months were presented in Fig. 5 .
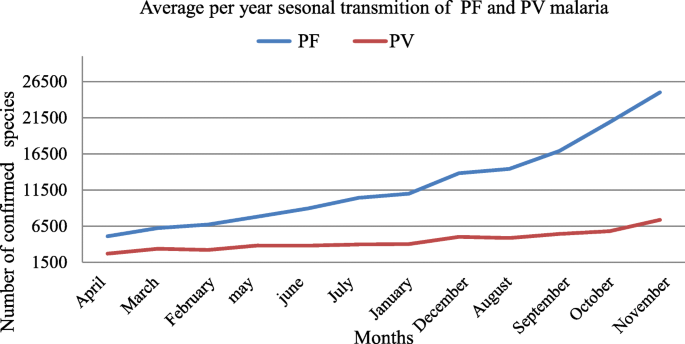
Monthly confirmed malaria cases average distribution over five years by Plasmodium falciparum and vivax Species in Central, North and west Gondar zone, Ethiopia, July 2013–June 2018
Malaria morbidity and mortality
Morbidity due to malaria was reported in all age groups including pregnant mothers. Within five years period > 15 years of age were more affected by malaria. Out of 1,003,391 total confirmed and clinical malaria cases obtaining outpatient and inpatient service, > 15 years of age accounts 721,430 (71.89%) followed by 5–14 years of age 183,656 (18.31%), < 5 years 89,676 (8.94%) and pregnant women 8629 (0.86%). All malaria cases treated in inpatient and OPD by age group were presented in Fig. 6 . Within five years period a total of 1770 (0.18%) cases received inpatient malaria service. Average annual inpatient malaria cases in West Gondar zone were 265 (83 per 100,000 population) followed by Central Gondar 69 (three per 100,000 populations) and North Gondar 20 (two per 100,000 populations). There were a total of 20 deaths in five years period. Case Fatality Rate (CFR) in North Gondar Zone were three (4/100,000 population), West Gondar zone were 13(3/100,000 population) and Central Gondar zone were four (0.89/100,000 population). In patient of malaria case and inpatient of all disease by zone were presented in Fig. 7 .
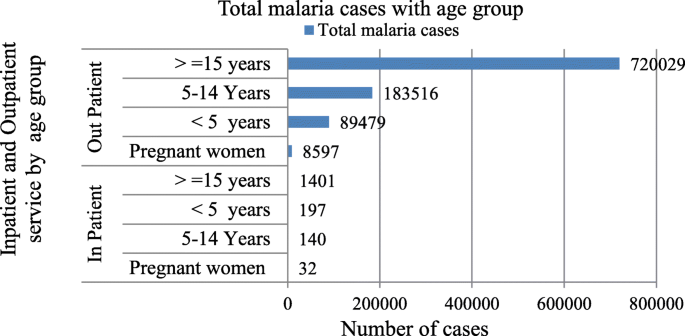
Number of outpatient and inpatient clinical and confirmed malaria cases by age group in Central, North and West Gondar Zones from July 2013–June 2018
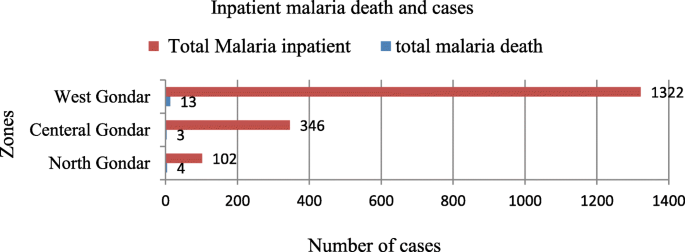
Inpatient malaria cases and inpatients to all disease in Central, North and West Gondar zones from July 2013–June 2018
Plasmodium falciparum and vivax species transmission
Plasmodium falciparum cases in West Gondar Zone of; Matema were 152,017 (45%), Quara were 79,894(24%) and Mirab Armachio were 71,137(23%) within five years period compared to other woredas in the zone. Plasmodium vivax in Metema were 35,649(37%) followed by Mirab Armachio 27,843(29%) from west Gondar zone and Tegede Woreda 25,220 (18%) from central Gondar zone. Transmissions of PF and PV in Central, North and West Gondar zone by its woredas were presented in Fig. 8 .
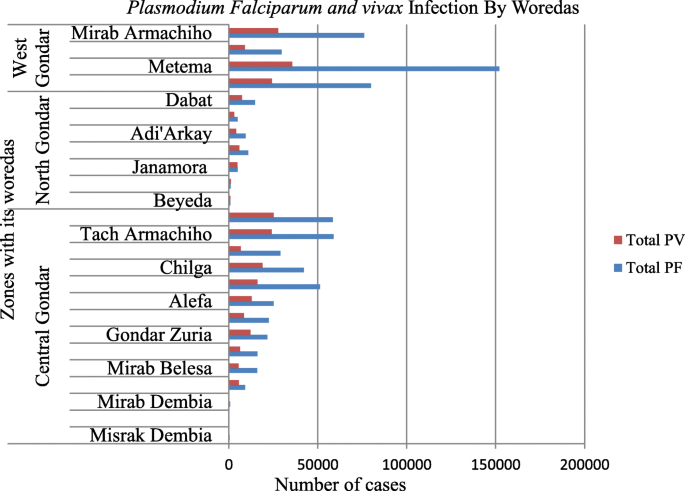
Distribution of Plasmodium falciparum and vivax cases of districts from July 2013–June 2018
Annual parasite incidence (API) by zones and districts (woredas)
Annual parasite incidence of malaria case in West Gondar zone were 86,929 (273/1000 population), Central Gondar zone were 98,816 (40/1000 population) and North Gondar zone were 14,845 (17/1000 population). The annual parasite incidence of Mirab Armachio, Gendawuha, Metema and Quara woreda were 20,796 (481/1000 population), 7754 (298/1000 population), 37,553 (269/1000 population) and 20,846(191/1000 population) cases respectively from West Gondar Zone. Annual parasite incidences by zones were presented in Fig. 9 .
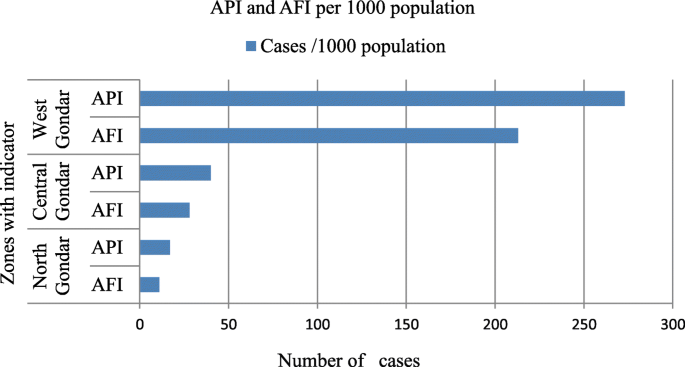
API of malaria in Central, North and West Gondar zones from July 2013–June 2018
Annual blood examination and test positivity rates
The Annual blood examination rate (ABER) of West Gondar zone was 237,675 (75%) followed by Central Gondar 286,527 (12%) and North Gondar zone 41,342 (5%). Test positivity rate of was greater in West Gondar zone. ABER test and falciparum positivity rate of zone were presented in Fig. 10 .
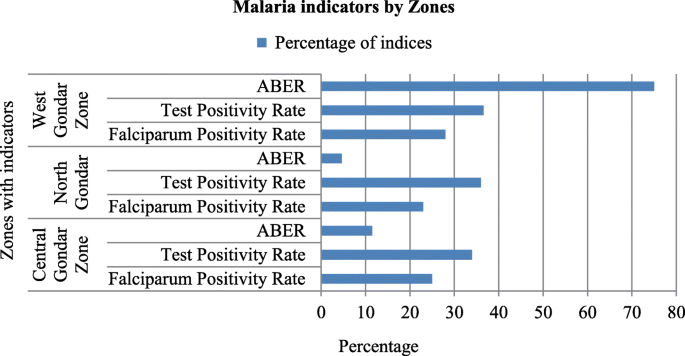
Average ABER and test positivity rate in Central, North and West Gondar zones from July 2013–June 2018
The overall five years Malaria trend analysis of Central, North and West Gondar zones indicates that the incidence of confirmed outpatient and inpatient malaria cases were increased from 220,406 (22%) in July 2013–June 2014 to 249,474 (25%) in July 2015–June 2016 and decreased to 139,296 (14%) in July 2017–June 2018. Average annual malaria cases of 200,589 (55 cases per 1000 population) were identified and treated with anti-malaria in Central, North and west Gondar zones which contributes 2.23% of East Africa nations malaria cases. These results shows that there were an increment of cases compared to Namibia 24,682 (12.33/1000 population) cases in 2016, Zambia 119,593 (7.7/1000 population) and highly affected regions of Botswana 794(0.36/1000 population) malaria cases 2017 WHO report [ 3 , 8 ]. This variation may be due to strong commitment of malaria controlling strategies of the countries, geographical variation and accessibility of controlling materials.
The number of malaria death were also decreased from 14 (0.34/100,000 Population) in July 2013–June 2014 to three (0.07/100,000 Population) in July 2017–June 2018 in the central, North and West Gondar zones. This result shows a different report in Burundi 4000(4/10,000 population) cases killed due to malaria and similar to five (0.23/100,000 Population) deaths in Botswana 2017 WHO report [ 3 , 5 , 8 ]. This significant difference may be due to outbreak conditions of the country and not timely treatment or arrival of cases to treatment centers and it requires further investigations.
A total of 1770 (0.18%) cases received inpatient malaria service within five years period. Average annual inpatient malaria cases in West Gondar zone were high 265 (83 per 100,000 population) as compared to Central Gondar 69 (three per 100,000 populations) and North Gondar 20 (two per 100,000 populations). From 1770 inpatient cases only 20 deaths were recorded in five years period in these zones. Case Fatality Rate (CFR) in West Gondar zone were 13(3/100,000 population), which is high as compared to Central Gondar zone four (0.89/100,000 population). This zonal inpatient death variation may be due to high 265 (83/100,000 population) inpatient cases in related to central Gondar Zone 69(3/100,000 Population) inpatient cases and not severely arrival of cases to the health institutions and continuous follow up cases in the inpatient service.
Within five years period > 15 years of age were more affected by malaria. Out of 103,391 total confirmed and clinical malaria cases obtaining outpatient and inpatient service, > 15 years of age accounts 720,029(71.76%) followed by 5–14 years of age 183,516(18.29%) in outpatient visit. Within five years period in Central, North and West Gondar zones inpatient malaria cases were 1770 (17.64/10,000 population) with a discharge rate of 1750 (98.87%). Next to > 15 years of age 1401(14/10,000 population), < 5 years of age 197(2/10,000 population) were more affected compared to 5–14 years of age 140(1.4/10,000 population) in inpatient service. This study shows slightly similar to studies conducted in other part of Ethiopia in 2015 and in North Shoa, Ethiopia between 2013 and 2017 [ 9 ]. This may be due to geographical similarity of reported zones and woredas and similarity of study design.
Highest monthly Plasmodium falciparum transmission were observed in November with 249, 93(17%) cases followed by October 20,873(14%) and September 16,892(11%) cases respectively. Plasmodium vivax transmission were also high in November, October and September with 7365(14%), 5813(11%) and 5431(10%) cases respectively at Central, North and West Gondar zones. This result showed that a different study conducted in Sibu Sire District, East wolega zone, with a highest peak in June 18.9% followed by May, November, and July with prevalence rate, 13.3, 13.2, and 11.2%, respectively. The prevalence rate in October, August, and September were 9.4, 8.7, and 7%, respectively [ 10 ]. The variation may be due to amount of rain fall, climatic variation, geographical difference and year of study.
From a total of 1002, 946 confirmed malaria cases in Central, North and West Gondar zones; Plasmodium falciparum species were 736,149 (73.4%) and Plasmodium vivax were 266,797 (26.6%). This result shows difference in the study conducted in South Wollo zone Kombolcha health center from January to December 2016, Plasmodium falciparum accounted for 1243 (60.2%) while P. vivax accounted for 734 (35.5%) cases [ 11 ]. In this study PF in West and Central Gondar zone was the most frequently reported species. The reason for this variation is not clear; however, may be due to the previous study focus on health center and the current study cover zonal level and may need further study.
API and ABER of West Gondar zone were High 86,929(273/1000 population) and 237,675 (75%) respectively compared to Central Gondar zone of API 98,816 (40/1000 population) and ABER 286,527(12%). Annual parasite incidence were high in Mirab Armachiho, Genda wuha and Metema woreda 20,796(481/1000 population), 7753 (298/1000 population) and 37,553 (269/1000 population) respectively compared to central and North Gondar zones of its woredas. Annual blood examination rate of Gendawuha 35,983(138%) were high compared to Mirab Armachiho 285,743 (132%) and Metema woreda 90,723(65%). This result shows that rate of infection with transmission and diagnostic efforts were high in West Gondar zone of all woredas. This high risk may be due to geographical location of the woredas and increased the number of daily labors to investment area.
Annual Falciparum incidence were high in West Gondar zone 67,561 (213/ 1000 population) compared to central Gondar Zone 70,287 (28/1000 population) and North Gondar 9382 (11/1000 population). Tsegede 11,770 (135/1000 population) and Tacharmachiho Woreda 111,770 (110/1000 population) were have high annual falciparum incidence relative to other central Gondar zone Woredas. From west Gondar zone; Mirabaremachio 15,222(352/1000 population), Gendawuha 35,985(229/1000 population) and Metema 90,723(218/1000 population) were the leading woreda by Annual falciparum incidence. This result shows that a similar report to 167,079 (43.2%) cases of previous North Gondar Zone 2017 Health Bureau PHEM report [ 7 ]. This difference may be due to study period and study design variation and it also need further investigation.
Limitations of the study
Plasmodium falciparum and vivax infection by pregnancy status, age categories and death of malaria with species infection were not analayzed. Distrbution of malaria by investement areas with its population at risk were not identified. Additionally, any malaria intervention activities that had been taken to control malaria were not collected from the study area.
Trends of total clinical and confirmed malaria cases were decreased from year to year. Rate of infection and diagnostic effort were high in West Gondar Zone. Malaria transmission were high in Mirabaremachiho 15,222 (352/1000 population), Gendawuha 35,985 (229/1000 population), Metema 90,723 (218/1000 population) from West Gondar zone and Tsegede 11,770 (135/1000 population) followed by Tacharmachiho 111,770 (110/1000 population) woreda from central Gondar zone. Amhra Public Health Institute and Regional Health Bureau should give special standing to highest annual parasite incidence area of west Gondar zone and highly transmitted districts/ woredas specially Mirabarmachio, Gendawuha and Metema woredas.
Availability of data and materials
The authors confirm that all data underlying the findings are fully available without restriction. All relevant data are within the manuscript.
Abbreviations
Annual Blood Examination Rate
Annual Falciparum Incidence
Amhara Public Health Institute
Annual Parasite Incidence
Case Fatality Rate
Epidemiology
In Patient Department
Malaria Control and Evaluation Partnership in Africa
Out Patient Department
Program for Alternative Technology in Health
Plasmodium falciparum
Public Health Department
Public Health Emergency Management
Plasmodium vivax
Rapid Diagnostic Test
St. Paul’s Hospital Millennium Medical College
United Nation
World Health Organization
Chaparro P, Padilla J, Vallejo AF, Herrera S. Characterization of a malaria outbreak in Colombia in 2010. Malar J. 2013;12(1):330.
Article PubMed PubMed Central Google Scholar
Alelign A, Tekeste Z, Petros B. Prevalence of malaria in Woreta town, Amhara region, Northwest Ethiopia over eight years. BMC Public Health. 2018;18(1):990.
Jima D, Wondabeku M, Alemu A, Teferra A, Awel N, Deressa W, et al. Analysis of malaria surveillance data in Ethiopia: what can be learned from the integrated disease surveillance and response system? Malar J. 2012;11(1):330.
Ayele DG, Zewotir TT, Mwambi HG. Prevalence and risk factors of malaria in Ethiopia. Malar J. 2012;11(1):195.
Sheet WF. World Malaria Report 2015. Geneva World Health Organ 2016;.
Ethiopia Regional State Government Amhara | Land Portal | Securing Land Rights Through Open Data [Internet]. [cited 2019 Apr 1]. Available from: https://landportal.org/organization/ethiopia-regional-state-government-amhara .
Organization WH. Disease surveillance for malaria control: an operational manual. 2012;.
Google Scholar
Cibulskis RE, Alonso P, Aponte J, Aregawi M, Barrette A, Bergeron L, et al. Malaria: global progress 2000–2015 and future challenges. Infect Dis Poverty. 2016;5(1):61.
Feleke DG, Gebretsadik D, Gebreweld A. Analysis of the trend of malaria prevalence in Ataye, north Shoa, Ethiopia between 2013 and 2017. Malar J. 2018;17(1):323.
Gemechu T, Samuel A, Yewhalaw D. Ten years trend analysis of malaria prevalence and its correlation with climatic variables in Sibu Sire District, east Wollega zone, Oromia regional state, Western Ethiopia: a retrospective study. Sci Technol Arts Res J. 2015;4(4):99 -105–105.
Article Google Scholar
Gebretsadik D, Feleke DG, Fiseha M. Eight-year trend analysis of malaria prevalence in Kombolcha, south Wollo, north-Central Ethiopia: a retrospective study. Parasit Vectors. 2018;11(1):55.
Download references
Acknowledgements
Authors would like to thank Amhara Public Health Institute PHEM officers and staffs for their technical support and programs related issues.
This research work has no any fund.
Author information
Authors and affiliations.
Department of Public Health, St. Paul’s Hospital Millennium Medical College, Addis Ababa, Ethiopia
Damtie Lankir & Samrawit Solomon
Department of Microbiology, St. Paul’s Hospital Millennium Medical College, Addis Ababa, Ethiopia
Addisu Gize
You can also search for this author in PubMed Google Scholar
Contributions
DL was involved in study conception, data analysis, drafting the manuscript. AG and SS were involved in the study design, and drafting the manuscript. AG critically revised and edited the manuscript for intellectual content. All authors have read and approved the manuscript.
Corresponding author
Correspondence to Addisu Gize .
Ethics declarations
Ethics approval and consent to participate.
Ethical clearance was obtained from the Department of Public Health, St. Paul’s Hospital. Millennium Medical College, Addis Ababa, Ethiopia, and submitted to Amhara Public Health. Institute (APHI); research and technology transfer directorate. Permission letter was secured from APHI which states its number as (APHI/ 5011, 06/02/11). The purposes and the importance of the study were explained to PHEM directorate. Confidentiality was assured at all levels of the study using password protected computer and through deleting all identifiers.
Consent for publication
Not applicable. This study does not contain any individual or personal data.
Competing interests
The authors declare that they have no competing interests.
Additional information
Publisher’s note.
Springer Nature remains neutral with regard to jurisdictional claims in published maps and institutional affiliations.
Rights and permissions
Open Access This article is licensed under a Creative Commons Attribution 4.0 International License, which permits use, sharing, adaptation, distribution and reproduction in any medium or format, as long as you give appropriate credit to the original author(s) and the source, provide a link to the Creative Commons licence, and indicate if changes were made. The images or other third party material in this article are included in the article's Creative Commons licence, unless indicated otherwise in a credit line to the material. If material is not included in the article's Creative Commons licence and your intended use is not permitted by statutory regulation or exceeds the permitted use, you will need to obtain permission directly from the copyright holder. To view a copy of this licence, visit http://creativecommons.org/licenses/by/4.0/ . The Creative Commons Public Domain Dedication waiver ( http://creativecommons.org/publicdomain/zero/1.0/ ) applies to the data made available in this article, unless otherwise stated in a credit line to the data.
Reprints and permissions
About this article
Cite this article.
Lankir, D., Solomon, S. & Gize, A. A five-year trend analysis of malaria surveillance data in selected zones of Amhara region, Northwest Ethiopia. BMC Public Health 20 , 1175 (2020). https://doi.org/10.1186/s12889-020-09273-5
Download citation
Received : 16 December 2019
Accepted : 19 July 2020
Published : 28 July 2020
DOI : https://doi.org/10.1186/s12889-020-09273-5
Share this article
Anyone you share the following link with will be able to read this content:
Sorry, a shareable link is not currently available for this article.
Provided by the Springer Nature SharedIt content-sharing initiative
- Malaria surveillance
- Data analysis
- West Gondar zones
- Northwest Ethiopia
BMC Public Health
ISSN: 1471-2458
- Submission enquiries: [email protected]
- General enquiries: [email protected]
- Ecology of Malaria
- Human Factors and Malaria
- Sickle Cell
The natural history of malaria involves cyclical infection of humans and female Anopheles mosquitoes. In humans, the parasites grow and multiply first in the liver cells and then in the red cells of the blood. In the blood, successive broods of parasites grow inside the red cells and destroy them, releasing daughter parasites (“merozoites”) that continue the cycle by invading other red cells.
The blood stage parasites are those that cause the symptoms of malaria. When certain forms of blood stage parasites (gametocytes, which occur in male and female forms) are ingested during blood feeding by a female Anopheles mosquito, they mate in the gut of the mosquito and begin a cycle of growth and multiplication in the mosquito. After 10-18 days, a form of the parasite called a sporozoite migrates to the mosquito’s salivary glands. When the Anopheles mosquito takes a blood meal on another human, anticoagulant saliva is injected together with the sporozoites, which migrate to the liver, thereby beginning a new cycle.
Thus the infected mosquito carries the disease from one human to another (acting as a “vector”), while infected humans transmit the parasite to the mosquito, In contrast to the human host, the mosquito vector does not suffer from the presence of the parasites.
More on: Anopheles Mosquitoes
More on: Malaria Parasites
Human Factors And Malaria
Biologic characteristics and behavioral traits can influence an individual’s risk of developing malaria and, on a larger scale, the intensity of transmission in a population.
More on: Human Factors and Malaria
Where does malaria transmission occur?
For malaria transmission to occur, conditions must be such so that all three components of the malaria life cycle are present:
- Anopheles mosquitoes, which able to feed on humans humans, and in which the parasites can complete the “invertebrate host” half of their life cycle
- Humans. who can be bitten by Anopheles mosquitoes, and in whom the parasites can complete the “vertebrate host” half of their life cycle
- Malaria parasites.
In rare cases malaria parasites can be transmitted from one person to another without requiring passage through a mosquito (from mother to child in "congenital malaria" or through transfusion, organ transplantation, or shared needles.)
Climate is a key determinant of both the geographic distribution and the seasonality of malaria. Without sufficient rainfall, mosquitoes cannot survive, and if not sufficiently warm, parasites cannot survive in the mosquito.
Anopheles lay their eggs in a variety of fresh or brackish bodies of water, with different species having different preferences. Eggs hatch within a few days, with resulting larvae spending 9-12 days to develop into adults in tropical areas. If larval habitats dry up before the process is completed, the larvae die; if rains are excessive, they may be flushed and destroyed. Life is precarious for mosquito larvae, with most perishing before becoming adults.
Life is usually short for adult mosquitoes as well, with temperature and humidity affecting longevity. Only older females can transmit malaria, as they must live long enough for sporozoites to develop and move to the salivary glands. This process takes a minimum of nine days when temperatures are warm (30°C or 86°F) and will take much longer at cooler temperatures. If temperatures are too cool (15°C or 59°F for Plasmodium vivax , 20°C or 68°F for P. falciparum ), development cannot be completed and malaria cannot be transmitted. Thus, malaria transmission is much more intense in warm and humid areas, with transmission possible in temperate areas only during summer months.
In warm climates people are more likely to sleep unprotected outdoors, thereby increasing exposure to night-biting Anopheles mosquitoes. During harvest seasons, agricultural workers might sleep in the fields or nearby locales, without protection against mosquito bites.
Anopheles Mosquitoes
The types (species) of Anopheles present in an area at a given time will influence the intensity of malaria transmission. Not all Anopheles are equally efficient vectors for transmitting malaria from one person to another. Those species that are most prone to bite humans are the most dangerous, as bites inflicted on animals that cannot be infected with human malaria break the chain of transmission. If the mosquito regularly bites humans, the chain of transmission is unbroken and more people will become infected. Some species are biologically unable to sustain development of human malaria parasites, while others are readily infected and produce large numbers of sporozoites (the parasite stage that is infective to humans).
Many of the most dangerous species bite human indoors. For these species insecticide treated mosquito nets and indoor residual spray (whereby the inner walls of dwellings are coated with a long-lasting insecticide) are effective interventions. Both of these interventions require attention to insecticide resistance, which will evolve if the same insecticide is used continuously in the same area.
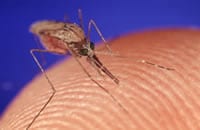
Biologic characteristics (inborn and acquired) and behavioral traits can influence an individual’s malaria risk and, on a larger scale, the overall malaria ecology.
More on: Human Factors And Malaria
Characteristics of the malaria parasite can influence the occurrence of malaria and its impact on human populations, for example:
- Areas where P. falciparum predominates (such as Africa south of the Sahara) will suffer more disease and death than areas where other species, which tend to cause less severe manifestations, predominate
- P. vivax and P. ovale have stages (“hypnozoites”) that can remain dormant in the liver cells for extended periods of time (months to years) before reactivating and invading the blood. Such relapses can result in resumption of transmission after apparently successful control efforts, or can introduce malaria in an area that was malaria-free
- P. falciparum (and to a lesser extent P. vivax ) have developed strains that are resistant to antimalarial drugs. Such strains are not uniformly distributed. Constant monitoring of the susceptibility of these two parasite species to drugs used locally is critical to ensure effective treatment and successful control efforts. Travelers to malaria-risk areas should use for prevention only those drugs that will be protective in the areas to be visited.
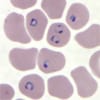
Plasmodium falciparum predominates in Africa south of the Sahara, one reason why malaria is so severe in that area.
Animal Reservoirs
A certain species of malaria called P. knowlesi has recently been recognized to be a cause of significant numbers of human infections. P. knowlesi is a species that naturally infects macaques living in Southeast Asia. Humans living in close proximity to populations of these macaques may be at risk of infection with this zoonotic parasite.
Areas Where Malaria Is No Longer Endemic
Malaria transmission has been eliminated in many countries of the world, including the United States. However, in many of these countries (including the United States) Anopheles mosquitoes are still present. Also, cases of malaria still occur in non-endemic countries, mostly in returning travelers or immigrants (“imported malaria”). Thus the potential for reintroduction of active transmission of malaria exists in many non-endemic parts of the world. All patients must be diagnosed and treated promptly for their own benefit but also to prevent the reintroduction of malaria.
Genetic Factors
Biologic characteristics present from birth can protect against certain types of malaria. Two genetic factors, both associated with human red blood cells, have been shown to be epidemiologically important. Persons who have the sickle cell trait (heterozygotes for the abnormal hemoglobin gene HbS) are relatively protected against P. falciparum malaria and thus enjoy a biologic advantage. Because P. falciparum malaria has been a leading cause of death in Africa since remote times, the sickle cell trait is now more frequently found in Africa and in persons of African ancestry than in other population groups. In general, the prevalence of hemoglobin-related disorders and other blood cell dyscrasias, such as Hemoglobin C, the thalassemias and G6PD deficiency, are more prevalent in malaria endemic areas and are thought to provide protection from malarial disease.
Persons who are negative for the Duffy blood group have red blood cells that are resistant to infection by P. vivax . Since the majority of Africans are Duffy negative, P. vivax is rare in Africa south of the Sahara, especially West Africa. In that area, the niche of P. vivax has been taken over by P. ovale , a very similar parasite that does infect Duffy-negative persons.
Other genetic factors related to red blood cells also influence malaria, but to a lesser extent. Various genetic determinants (such as the “HLA complex,” which plays a role in control of immune responses) may equally influence an individual’s risk of developing severe malaria.
More on: Sickle Cell and Malaria
Acquired Immunity
Acquired immunity greatly influences how malaria affects an individual and a community. After repeated attacks of malaria a person may develop a partially protective immunity. Such “semi-immune” persons often can still be infected by malaria parasites but may not develop severe disease, and, in fact, frequently lack any typical malaria symptoms.
In areas with high P. falciparum transmission (most of Africa south of the Sahara), newborns will be protected during the first few months of life presumably by maternal antibodies transferred to them through the placenta. As these antibodies decrease with time, these young children become vulnerable to disease and death by malaria. If they survive repeated infections to an older age (2-5 years) they will have reached a protective semi-immune status. Thus in high transmission areas, young children are a major risk group and are targeted preferentially by malaria control interventions.
In areas with lower transmission (such as Asia and Latin America), infections are less frequent and a larger proportion of the older children and adults have no protective immunity. In such areas, malaria disease can be found in all age groups, and epidemics can occur.
Anemia in young children in Asembo Bay, a highly endemic area in western Kenya. Anemia occurs most between the ages of 6 and 24 months. After 24 months, it decreases because the children have built up their acquired immunity against malaria (and its consequence, anemia).

Mother and her newborn in Jabalpur Hospital, State of Madhya Pradesh, India. The mother had malaria, with infection of the placenta.
Pregnancy and Malaria
Pregnancy decreases immunity against many infectious diseases. Women who have developed protective immunity against P. falciparum tend to lose this protection when they become pregnant (especially during the first and second pregnancies). Malaria during pregnancy is harmful not only to the mothers but also to the unborn children. The latter are at greater risk of being delivered prematurely or with low birth weight, with consequently decreased chances of survival during the early months of life. For this reason pregnant women are also targeted (in addition to young children) for protection by malaria control programs in endemic countries.
More on: Malaria During Pregnancy
Behavioral Factors
Human behavior, often dictated by social and economic reasons, can influence the risk of malaria for individuals and communities. For example:
- Poor rural populations in malaria-endemic areas often cannot afford the housing and bed nets that would protect them from exposure to mosquitoes. These persons often lack the knowledge to recognize malaria and to treat it promptly and correctly. Often, cultural beliefs result in use of traditional, ineffective methods of treatment.
- Travelers from non-endemic areas may choose not to use insect repellent or medicines to prevent malaria. Reasons may include cost, inconvenience, or a lack of knowledge.
- Human activities can create breeding sites for larvae (standing water in irrigation ditches, burrow pits)
- Agricultural work such as harvesting (also influenced by climate) may force increased nighttime exposure to mosquito bites
- Raising domestic animals near the household may provide alternate sources of blood meals for Anopheles mosquitoes and thus decrease human exposure
- War, migrations (voluntary or forced) and tourism may expose non-immune individuals to an environment with high malaria transmission.
Human behavior in endemic countries also determines in part how successful malaria control activities will be in their efforts to decrease transmission. The governments of malaria-endemic countries often lack financial resources. As a consequence, health workers in the public sector are often underpaid and overworked. They lack equipment, drugs, training, and supervision. The local populations are aware of such situations when they occur, and cease relying on the public sector health facilities. Conversely, the private sector suffers from its own problems. Regulatory measures often do not exist or are not enforced. This encourages private consultations by unlicensed, costly health providers, and the anarchic prescription and sale of drugs (some of which are counterfeit products). Correcting this situation is a tremendous challenge that must be addressed if malaria control and ultimately elimination is to be successful.
Protective Effect of Sickle Cell Trait Against Malaria
The sickle cell gene is caused by a single amino acid mutation (valine instead of glutamate at the 6th position) in the beta chain of the hemoglobin gene. Inheritance of this mutated gene from both parents leads to sickle cell disease and people with this disease have shorter life expectancy. On the contrary, individuals who are carriers for the sickle cell disease (with one sickle gene and one normal hemoglobin gene, also known as sickle cell trait) have some protective advantage against malaria. As a result, the frequencies of sickle cell carriers are high in malaria-endemic areas.
CDC’s birth cohort studies (Asembo Bay Cohort Project in western Kenya) conducted in collaboration with the Kenya Medical Research Institute allowed an investigation into this issue. It was found that that the sickle cell trait provides 60% protection against overall mortality. Most of this protection occurs between 2-16 months of life, before the onset of clinical immunity in areas with intense transmission of malaria.
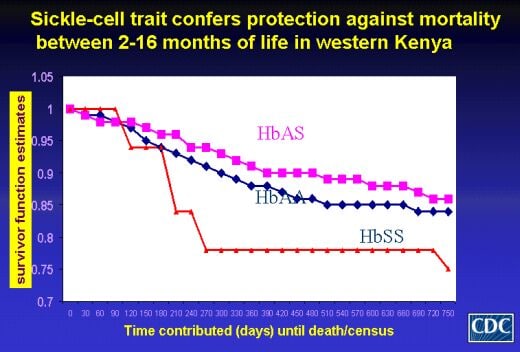
Graph of survival curves (“survival function estimates”) of children without any sickle cell genes (HbAA), children with sickle cell trait (HbAS), and children with sickle cell disease (HbSS). Those who had the sickle cell trait (HbAS) had a slight survival advantage over those without any sickle cell genes (HbAA), with children with sickle cell disease (HbSS) faring the worst.
Reference: Protective Effects of the Sickle Cell Gene Against Malaria Morbidity and Mortality. Aidoo M, Terlouw DJ, Kolczak MS, McElroy PD, ter Kuile FO, Kariuki S, Nahlen BL, Lal AA, Udhayakumar V. Lancet 2002; 359:1311-1312.
Malaria is transmitted to humans by female mosquitoes of the genus Anopheles. Female mosquitoes take blood meals for egg production, and these blood meals are the link between the human and the mosquito hosts in the parasite life cycle. The successful development of the malaria parasite in the mosquito (from the “gametocyte” stage to the “sporozoite” stage) depends on several factors. The most important is ambient temperature and humidity (higher temperatures accelerate the parasite growth in the mosquito) and whether the Anopheles survives long enough to allow the parasite to complete its cycle in the mosquito host (“sporogonic” or “extrinsic” cycle, duration 9 to 18 days). In contrast to the human host, the mosquito host does not suffer noticeably from the presence of the parasites.
Diagram of Adult Female Mosquito
Map of the world showing the distribution of predominant malaria vectors
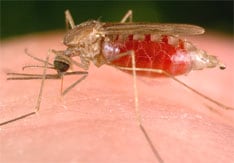
Anopheles freeborni mosquito pumping blood Larger Picture
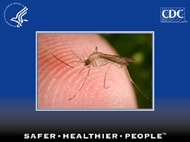
Sequential images of the mosquito taking its blood meal
General Information
There are approximately 3,500 species of mosquitoes grouped into 41 genera. Human malaria is transmitted only by females of the genus Anopheles . Of the approximately 430 Anopheles species, only 30-40 transmit malaria (i.e., are “vectors”) in nature. The rest either bite humans infrequently or cannot sustain development of malaria parasites.
Geographic Distribution
Anophelines are found worldwide except Antarctica. Malaria is transmitted by different Anopheles species in different geographic regions. Within geographic regions, different environments support a different species.
Anophelines that can transmit malaria are found not only in malaria-endemic areas, but also in areas where malaria has been eliminated. These areas are thus at risk of re-introduction of the disease.
Life Stages
Like all mosquitoes, anopheles mosquitoes go through four stages in their life cycle: egg, larva, pupa, and adult. The first three stages are aquatic and last 7-14 days, depending on the species and the ambient temperature. The biting female Anopheles mosquito may carry malaria. Male mosquitoes do not bite so cannot transmit malaria or other diseases. The adult females are generally short-lived, with only a small proportion living long enough (more than 10 days in tropical regions) to transmit malaria.
Adult females lay 50-200 eggs per oviposition. Eggs are laid singly directly on water and are unique in having floats on either side. Eggs are not resistant to drying and hatch within 2-3 days, although hatching may take up to 2-3 weeks in colder climates.
Mosquito larvae have a well-developed head with mouth brushes used for feeding, a large thorax, and a segmented abdomen. They have no legs. In contrast to other mosquitoes, Anopheles larvae lack a respiratory siphon and for this reason position themselves so that their body is parallel to the surface of the water.
Top: Anopheles Egg; note the lateral floats. Bottom: Anopheles eggs are laid singly.
Larvae breathe through spiracles located on the 8th abdominal segment and therefore must come to the surface frequently.
The larvae spend most of their time feeding on algae, bacteria, and other microorganisms in the surface microlayer. They do so by rotating their head 180 degrees and feeding from below the microlayer. Larvae dive below the surface only when disturbed. Larvae swim either by jerky movements of the entire body or through propulsion with the mouth brushes.
Larvae develop through 4 stages, or instars, after which they metamorphose into pupae. At the end of each instar, the larvae molt, shedding their exoskeleton, or skin, to allow for further growth.
Anopheles Larva. Note the position, parallel to the water surface.
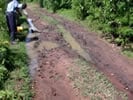
The larvae occur in a wide range of habitats but most species prefer clean, unpolluted water. Larvae of Anopheles mosquitoes have been found in fresh- or salt-water marshes, mangrove swamps, rice fields, grassy ditches, the edges of streams and rivers, and small, temporary rain pools. Many species prefer habitats with vegetation. Others prefer habitats that have none. Some breed in open, sun-lit pools while others are found only in shaded breeding sites in forests. A few species breed in tree holes or the leaf axils of some plants.
The pupa is comma-shaped when viewed from the side. This is a transitional stage between larva and adult. The pupae does not feed, but undergoes radical metamorphosis. The head and thorax are merged into a cephalothorax with the abdomen curving around underneath. As with the larvae, pupae must come to the surface frequently to breathe, which they do through a pair of respiratory trumpets on the cephalothorax. After a few days as a pupa, the dorsal surface of the cephalothorax splits and the adult mosquito emerges onto the surface of the water.
The duration from egg to adult varies considerably among species and is strongly influenced by ambient temperature. Mosquitoes can develop from egg to adult in as little as 7 days but usually take 10-14 days in tropical conditions.
Anopheles Pupa
Anopheles Adults. Note (bottom row) the typical resting position.
Like all mosquitoes, adult anopheles have slender bodies with 3 sections: head, thorax and abdomen.
The head is specialized for acquiring sensory information and for feeding. The head contains the eyes and a pair of long, many-segmented antennae. The antennae are important for detecting host odors as well as odors of aquatic larval habitats where females lay eggs. The head also has an elongate, forward-projecting proboscis used for feeding, and two sensory palps.
The thorax is specialized for locomotion. Three pairs of legs and a single pair of wings are attached to the thorax.
The abdomen is specialized for food digestion and egg development. This segmented body part expands considerably when a female takes a blood meal. The blood is digested over time serving as a source of protein for the production of eggs, which gradually fill the abdomen.
Anopheles mosquitoes can be distinguished from other mosquitoes by the palps, which are as long as the proboscis, and by the presence of discrete blocks of black and white scales on the wings. Adult Anopheles can also be identified by their typical resting position: males and females rest with their abdomens sticking up in the air rather than parallel to the surface on which they are resting .
Adult mosquitoes usually mate within a few days after emerging from the pupal stage. In some species, the males form large swarms, usually around dusk, and the females fly into the swarms to mate. The mating habitats of many species remain unknown.
Males live for about a week, feeding on nectar and other sources of sugar. Females will also feed on sugar sources for energy but usually require a blood meal for the development of eggs. After obtaining a full blood meal, the female will rest for a few days while the blood is digested and eggs are developed. This process depends on the temperature but usually takes 2-3 days in tropical conditions. Once the eggs are fully developed, the female lays them then seeks blood to sustain another batch of eggs.
The cycle repeats itself until the female dies. Females can survive up to a month (or longer in captivity) but most do not live longer than 1-2 weeks in nature. Their chances of survival depend on temperature and humidity, but also upon their ability to successfully obtain a blood meal while avoiding host defenses.
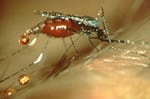
Female Anopheles dirus feeding
Factors Involved in Malaria Transmission and Malaria Control
Understanding the biology and behavior of Anopheles mosquitoes can aid in designing appropriate control strategies. Factors that affect a mosquito’s ability to transmit malaria include its innate susceptibility to Plasmodium , its host choice, and its longevity. Long-lived species that prefer human blood and support parasite development are the most dangerous. Factors that should be taken into consideration when designing a control program include the susceptibility of malaria mosquitoes to insecticides and the preferred feeding and resting location of adult mosquitoes.
More on: How to Reduce Malaria’s Impact
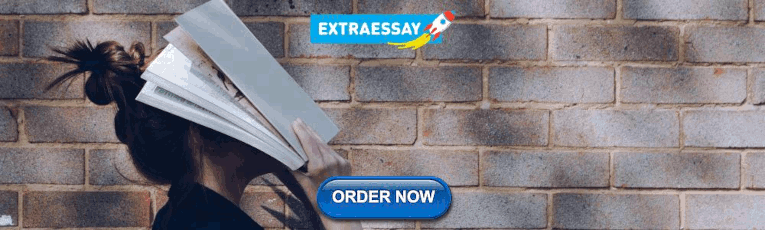
Preferred Sources for Blood Meals
One important behavioral factor is the degree to which an Anopheles species prefers to feed on humans (anthropophily) or animals such as cattle (zoophily). Anthrophilic Anopheles are more likely to transmit the malaria parasites from one person to another. Most Anopheles mosquitoes are not exclusively anthropophilic or zoophilic; many are opportunistic and feed upon whatever host is available. However, the primary malaria vectors in Africa, An. gambiae and An. funestus , are strongly anthropophilic and, consequently, are two of the most efficient malaria vectors in the world.
Once ingested by a mosquito, malaria parasites must undergo development within the mosquito before they are infectious to humans. The time required for development in the mosquito (the extrinsic incubation period) takes 9 days or longer, depending on the parasite species and the temperature. If a mosquito does not survive longer than the extrinsic incubation period, then she will not be able to transmit any malaria parasites.
It is not possible to measure directly the life span of mosquitoes in nature, but many studies have indirectly measured longevity by examination of their reproductive status or via marking, releasing, and recapturing adult mosquitoes. The majority of mosquitoes do not live long enough to transmit malaria, but some may live as long as three weeks in nature. Though evidence suggests that mortality rate increases with age, most workers estimate longevity in terms of the probability that a mosquito will live one day. Usually these estimates range from a low of 0.7 to a high of 0.9. If survivorship is 90% daily, then a substantial proportion of the population would live longer than 2 weeks and would be capable of transmitting malaria. Any control measure that reduces the average lifespan of the mosquito population will reduce transmission potential. Insecticides thus need not kill the mosquitoes outright, but may be effective by limiting their lifespan.
Patterns of Feeding and Resting
Most Anopheles mosquitoes are crepuscular (active at dusk or dawn) or nocturnal (active at night). Some Anopheles mosquitoes feed indoors (endophagic) while others feed outdoors (exophagic). After blood feeding, some Anopheles mosquitoes prefer to rest indoors (endophilic) while others prefer to rest outdoors (exophilic). Biting by nocturnal, endophagic Anopheles mosquitoes can be markedly reduced through the use of insecticide-treated bed nets (ITNs) or through improved housing construction to prevent mosquito entry (e.g., window screens). Endophilic mosquitoes are readily controlled by indoor spraying of residual insecticides. In contrast, exophagic/exophilic vectors are best controlled through source reduction (destruction of larval habitats).
Insecticide Resistance
Insecticide-based control measures (e.g., indoor spraying with insecticides, ITNs) are the principal way to kill mosquitoes that bite indoors. However, after prolonged exposure to an insecticide over several generations, mosquitoes, like other insects, may develop resistance, a capacity to survive contact with an insecticide. Since mosquitoes can have many generations per year, high levels of resistance can arise very quickly. Resistance of mosquitoes to some insecticides has been documented within a few years after the insecticides were introduced. There are over 125 mosquito species with documented resistance to one or more insecticides. The development of resistance to insecticides used for indoor residual spraying was a major impediment during the Global Malaria Eradication Campaign. Judicious use of insecticides for mosquito control can limit the development and spread of resistance, particularly via rotation of different classes of insecticides used for control. Monitoring of resistance is essential to alert control programs to switch to more effective insecticides.
Susceptibility/Refractoriness
Some Anopheles species are poor vectors of malaria, as the parasites do not develop well (or at all) within them. There is also variation within species. In the laboratory, it has been possible to select for strains of An. gambiae that are refractory to infection by malaria parasites. These refractory strains have an immune response that encapsulates and kills the parasites after they have invaded the mosquito’s stomach wall. Scientists are studying the genetic mechanism for this response. It is hoped that some day, genetically modified mosquitoes that are refractory to malaria can replace wild mosquitoes, thereby limiting or eliminating malaria transmission.
Malaria Parasites
Malaria parasites are micro-organisms that belong to the genus Plasmodium . There are more than 100 species of Plasmodium , which can infect many animal species such as reptiles, birds, and various mammals. Four species of Plasmodium have long been recognized to infect humans in nature. In addition there is one species that naturally infects macaques which has recently been recognized to be a cause of zoonotic malaria in humans. (There are some additional species which can, exceptionally or under experimental conditions, infect humans.)
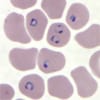
Ring-form trophozoites of P. falciparum in a thin blood smear.
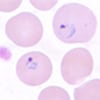
Ring-form trophozoites of P. vivax in a thin blood smear.
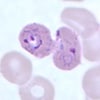
Trophozoites of P. ovale in a thin blood smear.
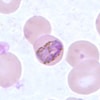
Band-form trophozoites of P. malariae in a thin blood smear.
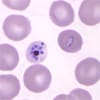
Schizont and ring-form trophozoite of P. knowlesi in a thin blood smear.
(All photos courtesy of DPDx )
The species infecting humans are the following:
- P. falciparum , which is found worldwide in tropical and subtropical areas, and especially in Africa where this species predominates. P. falciparum can cause severe malaria because it multiples rapidly in the blood, and can thus cause severe blood loss (anemia). In addition, the infected parasites can clog small blood vessels. When this occurs in the brain, cerebral malaria results, a complication that can be fatal.
- P. vivax , which is found mostly in Asia, Latin America, and in some parts of Africa. Because of the population densities especially in Asia it is probably the most prevalent human malaria parasite. P. vivax (as well as P. ovale ) has dormant liver stages (“hypnozoites”) that can activate and invade the blood (“relapse”) several months or years after the infecting mosquito bite.
- P. ovale is found mostly in Africa (especially West Africa) and the islands of the western Pacific. It is biologically and morphologically very similar to P. vivax . However, differently from P. vivax , it can infect individuals who are negative for the Duffy blood group, which is the case for many residents of sub-Saharan Africa. This explains the greater prevalence of P. ovale (rather than P. vivax ) in most of Africa.
- P. malariae , found worldwide, is the only human malaria parasite species that has a quartan cycle (three-day cycle). (The three other species have a tertian, two-day cycle.) If untreated, P. malariae causes a long-lasting, chronic infection that in some cases can last a lifetime. In some chronically infected patients P. malariae can cause serious complications such as the nephrotic syndrome.
- P. knowlesi is found throughout Southeast Asia as a natural pathogen of long-tailed and pig-tailed macaques. It has recently been shown to be a significant cause of zoonotic malaria in that region, particularly in Malaysia. P. knowlesi has a 24-hour replication cycle and so can rapidly progress from an uncomplicated to a severe infection; fatal cases have been reported.
- Disease caused by human malaria parasites.
- More images of the various stages of development of these 5 malaria species
To receive email updates about this page, enter your email address:
New! Locally Acquired Cases of Malaria in Florida, Texas, Maryland, and Arkansas
New! Update to Guidance for use of Artemether-Lumefantrine (Coartem®) in Pregnancy for Uncomplicated Malaria New! Discontinuation of CDC’s Distribution of Intravenous Artesunate as Commercial Drug Guidance for Malaria Diagnosis in Patients Suspected of Ebola Infection in the United States -->
See all Malaria Notices
- New! Malaria is a Serious Disease
- New! La malaria (paludismo) es una enfermedad grave
- How to Report a Case of Malaria
- CDC Yellow Book
- Red Pages: Malaria-endemic areas by country
- Drugs for Prevention
- Choosing a Drug to Prevent Malaria
- Drugs for Treatment in the U.S.
- Frequently Asked Questions (FAQs)
- Blood Banks
Click here for contact information
Exit Notification / Disclaimer Policy
- The Centers for Disease Control and Prevention (CDC) cannot attest to the accuracy of a non-federal website.
- Linking to a non-federal website does not constitute an endorsement by CDC or any of its employees of the sponsors or the information and products presented on the website.
- You will be subject to the destination website's privacy policy when you follow the link.
- CDC is not responsible for Section 508 compliance (accessibility) on other federal or private website.
Health Case study in a developing country- malaria
Case studies illustrate the causes of health issues. They explain their growth, effects on the population and the strategies involved in managing development and health in the 21st century.
Part of Geography Global issues
Case study in a developing country- malaria
Malaria is usually found between the Tropic of Cancer close Tropic of Cancer An important line of latitude located at 23.5° north of the Equator. in the north and the Tropic of Capricorn close Tropic of Capricorn An important line of latitude located at 23.5° south of the Equator. in the south.
There are a number of human close human factors A man-made thing or an aspect to do with humans, eg poor sanitation. and physical factors close physical factors Natural factors such as the type of land, the climate and the presence of water. which contribute to the worldwide distribution of malaria.
Causes of malaria
- Temperatures must be between 15 and 40°C with high rainfall – this makes the tropics ideal.
- The presence of the female anopheles mosquito close anopheles mosquito The type of mosquitoes that spread malaria. .
- Humidity close humidity The amount of water vapour in the atmosphere measured as a percentage. of 60 per cent and over.
- Stagnant water close stagnant water Water that is still and does not move. , eg a lake or even a puddle, needed to lay larvae close larvae The newly hatched, wingless and wormlike form of a mosquito before metamorphosis into an insect. .
- Areas of vegetation close vegetation Trees and plants. for shade to digest blood.
- Houses create areas of shade for the mosquitoes close mosquitoes A small flying insect. to rest.
- Global air travel contributes to the spread – ' airport malaria close airport malaria When malaria is brought into a country on aircraft. '.
- Settlements provide large populations for blood meals.
- Poor sanitation close sanitation Measures to help cleanliness and hygiene. in shanty towns.
- Man-made areas of stagnant water, eg paddy fields close paddy fields Fields where rice is grown. .
Malaria is spread as the female anopheles mosquito bites a person and passes the parasite close parasite An organism that lives in another organism such as humans. into their bloodstream, infecting them with malaria. Mosquitoes can also pick up the parasite from an infected human and then pass it on when they bite someone else.
More guides on this topic
- Climate change
- Natural regions
- Environmental hazards
- Trade and globalisation
- Global tourism
- Classroom Videos
Related links
- BBC Weather
- BBC News: Science, Environment
- BBC Two: Landward
- SQA National 5 Geography
- Planet Diary
- Scotland's Environment
- Royal Geographical Society
- Alzheimer's disease & dementia
- Arthritis & Rheumatism
- Attention deficit disorders
- Autism spectrum disorders
- Biomedical technology
- Diseases, Conditions, Syndromes
- Endocrinology & Metabolism
- Gastroenterology
- Gerontology & Geriatrics
- Health informatics
- Inflammatory disorders
- Medical economics
- Medical research
- Medications
- Neuroscience
- Obstetrics & gynaecology
- Oncology & Cancer
- Ophthalmology
- Overweight & Obesity
- Parkinson's & Movement disorders
- Psychology & Psychiatry
- Radiology & Imaging
- Sleep disorders
- Sports medicine & Kinesiology
- Vaccination
- Breast cancer
- Cardiovascular disease
- Chronic obstructive pulmonary disease
- Colon cancer
- Coronary artery disease
- Heart attack
- Heart disease
- High blood pressure
- Kidney disease
- Lung cancer
- Multiple sclerosis
- Myocardial infarction
- Ovarian cancer
- Post traumatic stress disorder
- Rheumatoid arthritis
- Schizophrenia
- Skin cancer
- Type 2 diabetes
- Full List »
share this!
April 24, 2024
This article has been reviewed according to Science X's editorial process and policies . Editors have highlighted the following attributes while ensuring the content's credibility:
fact-checked
peer-reviewed publication
trusted source
New study uses AI to predict malaria outbreaks in South Asia
by University of Oxford
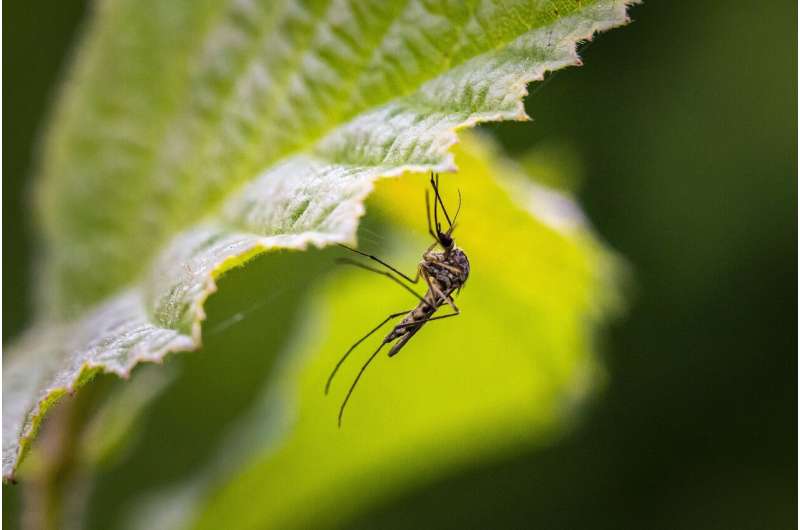
Researchers from NDORMS in collaboration with international institutions have demonstrated the potential of using environmental measurements and deep learning modeling to predict malaria outbreaks in South Asia. The study offers promising insights into improving early warning systems for one of the world's deadliest diseases.
Malaria remains a significant global health challenge, with approximately half of the world's population at risk of infection, particularly in African and South Asian countries. Despite being preventable, the changing nature of the climate, socio-demographic, and environmental risk factors have made outbreak prediction difficult.
Led by Associate Professor Sara Khalid of the Planetary Health Informatics Group at NDORMS, University of Oxford in collaboration with the Lahore University of Management Sciences, the research team wanted to address this challenge and explore whether an environment-based machine learning approach could offer the potential for location-specific early warning tools for malaria.
They developed a multi-dimensional LSTM (M-LSTM) model that simultaneously analyzed environmental indicators including temperature, rainfall, vegetation measures, and night-time light data to predict malaria incidence in the South Asian belt spanning Pakistan, India, and Bangladesh.
The data was plotted against district-level malaria incidence rates for each country between 2000 and 2017, obtained from the US Agency for International Development's Demographic and Health Survey datasets.
The results, which have been published in The Lancet Planetary Health , show that the proposed M-LSTM model consistently outperforms the state-of-the-art conventional LSTM model with 94.5%, 99.7%, and 99.8% lower error rates for Pakistan, India, and Bangladesh, respectively.
In general, higher accuracy and reduced error rates were achieved with increased model complexity, highlighting the effectiveness of the approach.
Sara explained, "This approach is generalizable and as such our modeling carries significant implications for public health policy. For example, it could be applied to other infectious diseases , or be scaled up to other high-risk areas with a disproportionately high burden of malaria cases and deaths in WHO Africa regions. It has the potential to help decision-makers implement more proactive measures to manage malaria outbreaks early and accurately.
"The real attraction is the ability to analyze pretty much anywhere and everywhere on earth, thanks to the rapid advancements in earth observation, deep learning and AI, and the availability of high-performance computers. This could lead to more targeted interventions and a better allocation of resources in the ongoing efforts to eradicate malaria and enhance public health outcomes worldwide."
Explore further
Feedback to editors

Link between depression and cardiovascular disease explained: They partly develop from same gene module
3 hours ago

Study highlights increased risk of second cancers among breast cancer survivors
8 hours ago

Opioids during pregnancy not linked to substantially increased risk of psychiatric disorders in children
9 hours ago

Research identifies pitfalls and opportunities for generative AI in patient messaging systems

A closed-loop drug-delivery system could improve chemotherapy
11 hours ago

It's easier now to treat opioid addiction with medication—but use has changed little, study finds

Solving the riddle of the sphingolipids in coronary artery disease

New AI technology estimates brain age using low-cost EEG device

Alteration of brain network condition could predict painful vaso-occlusive crisis in patients with sickle cell disease
12 hours ago

Trials reveal that internet-based conversations help sustain brain function in older adults
Related stories.

New nets 'prevent' 13 mn malaria cases in Africa: Project
Apr 17, 2024

First evidence of how the Asian malaria mosquito is spreading drug-resistant malaria in Africa
Nov 8, 2023

Data from satellite imagery useful for malaria early warning systems
Jun 7, 2017

WHO: Malaria reductions stall after progress
Nov 19, 2018

New analysis could help forecast malaria outbreaks
Feb 22, 2023

Climate change could upturn world malaria fight: WHO
Nov 30, 2023
Recommended for you

New algorithm could provide early warning for asthma attacks
13 hours ago

Researchers discover biology behind Fontan-operation-associated liver disease
14 hours ago

Gene-based therapy restores cellular development and function in brain cells from people with Timothy syndrome

Study finds AI can develop treatments to prevent 'superbugs'
Let us know if there is a problem with our content.
Use this form if you have come across a typo, inaccuracy or would like to send an edit request for the content on this page. For general inquiries, please use our contact form . For general feedback, use the public comments section below (please adhere to guidelines ).
Please select the most appropriate category to facilitate processing of your request
Thank you for taking time to provide your feedback to the editors.
Your feedback is important to us. However, we do not guarantee individual replies due to the high volume of messages.
E-mail the story
Your email address is used only to let the recipient know who sent the email. Neither your address nor the recipient's address will be used for any other purpose. The information you enter will appear in your e-mail message and is not retained by Medical Xpress in any form.
Newsletter sign up
Get weekly and/or daily updates delivered to your inbox. You can unsubscribe at any time and we'll never share your details to third parties.
More information Privacy policy
Donate and enjoy an ad-free experience
We keep our content available to everyone. Consider supporting Science X's mission by getting a premium account.
E-mail newsletter
Addressing Malaria and Other Vector-Borne Diseases through GIS: Insights and Resources
As we mark World Malaria Day, I’d like to reflect on the significant strides made and the relentless efforts needed to combat malaria and other vector-borne diseases. Geographic Information Systems (GIS) have proven instrumental in these efforts, providing relevant insights that drive strategic decisions and interventions. This blog will delve into how GIS aids in mosquito surveillance and control, offering a range of educational materials and resources to further our collective endeavor.
The Role of GIS in Vector-Borne Disease Management
GIS technology offers a robust platform for mapping and analyzing the distribution of vector populations, environmental conditions, and human demographics. These capabilities enable public health officials to identify high-risk areas, optimize resource allocation, and implement targeted control measures. Whether it’s malaria, Zika, or even other vector-borne diseases, GIS provides a comprehensive toolkit for enhancing disease surveillance and response strategies.
Educational Materials and Resources
- Brochure : Learn about effective vector-borne disease control through resources like the Effective vector-borne disease control and surveillance brochure .
- Blogs : Explore case studies and articles on combating mosquito-borne diseases using GIS, such as Mapping our battle against mosquito-borne diseases and Infectious mosquitoes post increasing health threat .
Practical GIS Solutions
- Mosquito Service Requests : Engage the public in identifying mosquito activity through platforms like Mosquito Service Requests .
- Mosquito Treatments : Manage and communicate treatment plans effectively using tools like Mosquito Treatments .
- Mosquito Surveillance : Monitor and track mosquito populations and disease spread with Mosquito Surveillance .
Case Studies and Success Stories
- Discover how organizations like DC Health have improved data collection and sharing through GIS in the DC Health case study .
- Learn from Zambia’s approach to eradicating malaria using geospatial data in Seeking to eradicate malaria: Zambia turns to geospatial data .
Learning Opportunities
- Tutorials : Engage with lessons like Monitor Malaria Epidemics and Visualize Malaria Rates in Africa to hone your GIS skills in a public health context.
- Software Trials : Experiment with GIS tools by accessing free trials of ArcGIS Pro and ArcGIS Online .
Engagement and Further Learning
For those looking to deepen their understanding or inquire about specific projects, consider reaching out via [email protected] or exploring the ArcGIS access options . Additionally, the Global public health grant program for ArcGIS offers opportunities for those seeking to leverage GIS for health initiatives.
GIS is more than just a tool; it’s a part of the solution in our global fight against vector-borne diseases. By harnessing the power of geospatial data, we can create more effective interventions and a healthier future. Let’s continue to explore and expand the potential of GIS in public health, empowering professionals and communities alike to stand resilient against the threat of diseases like malaria.
About the author
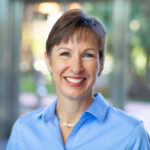
Este Geraghty
Dr. Este Geraghty, MD, MS, MPH, CPH, GISP, is the Chief Medical Officer at Esri where she leads strategy and messaging for the Health and Human Services sector. Dr. Geraghty has been with Esri since 2014 and has led business development and solution development in the market. During her time at Esri, Dr. Geraghty has helped organizations around the world use location intelligence to combat Zika virus, finish the fight against polio, grapple with the opioid crisis, combat homelessness, enhance health preparedness and response, inform strategic planning, optimize healthcare access, and traverse the COVID-19 pandemic while tackling inequity. Formerly the Deputy Director of the Center for Health Statistics and Informatics with the California Department of Public Health, Dr. Geraghty led the state vital records and public health informatics programs. There she engaged in statewide initiatives in meaningful use, health information exchange, open data and interoperability. While serving as an Associate Professor of Clinical Internal Medicine at the University of California at Davis she conducted research on geographic approaches to influencing health policy and advancing community development programs. In addition to her degrees in Medicine, Medical Informatics and Public Health, Dr. Geraghty is also a board-certified public health professional (CPH) and a Geographic Information Systems Professional (GISP).
Related Content:
- public health
- public health preparedness
- vector control
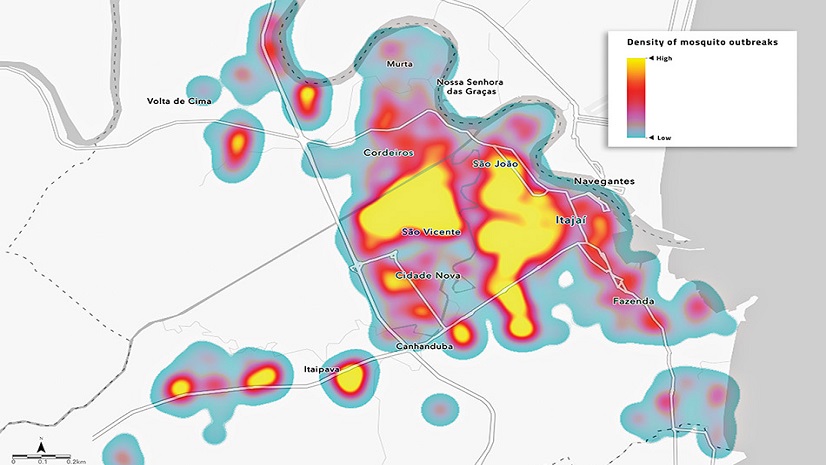
Mapping our Battle Against Mosquito-Borne Diseases with GIS Technology
Este Geraghty | Public Health | June 21, 2023
Recognizing National Mosquito Control week, Esri Chief Medical Officer highlights technology that can aid health departments.
Read this article
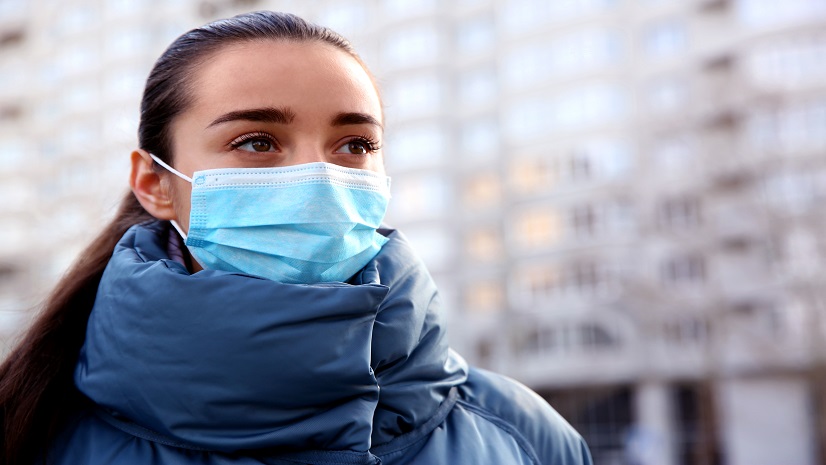
5 Ways to Apply GIS to Influenza Response
Este Geraghty | Health and Human Services | December 1, 2022
Flu season has a variety of impacts on a community. In order to contain the spread organizations can leverage GIS to strengthen their response.
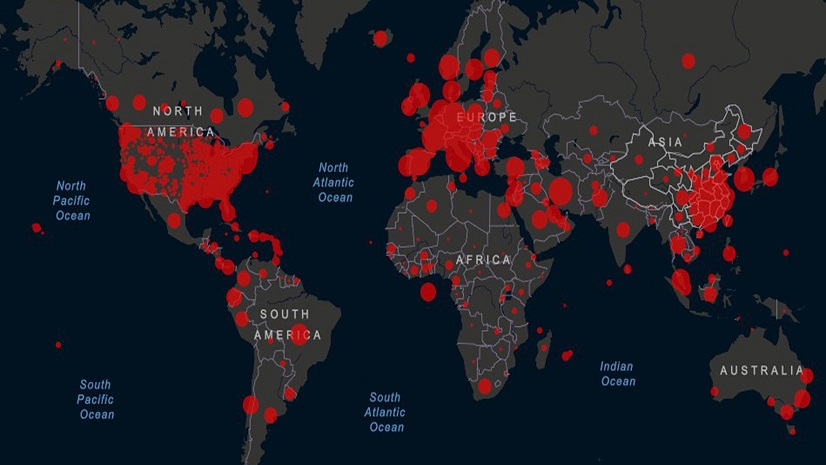
A Thank You to Johns Hopkins University
Este Geraghty | Health | March 11, 2023
Today marks the end of an era - the last update to the notable COVID-19 Dashboard that kept the world informed.
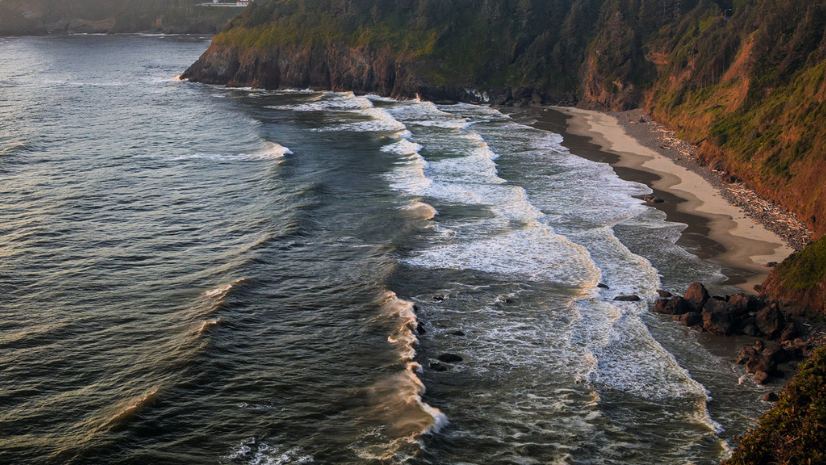
Next Article
Earth Day 2024: Embracing the 30x30 Initiative

An official website of the United States government
The .gov means it’s official. Federal government websites often end in .gov or .mil. Before sharing sensitive information, make sure you’re on a federal government site.
The site is secure. The https:// ensures that you are connecting to the official website and that any information you provide is encrypted and transmitted securely.
- Publications
- Account settings
Preview improvements coming to the PMC website in October 2024. Learn More or Try it out now .
- Advanced Search
- Journal List
- Wiley-Blackwell Online Open

Outcomes reported in trials of treatments for severe malaria: The need for a core outcome set
Lamprini lampro.
1 Medical Research Council Clinical Trials Unit at University College London, London UK
2 Intensive Care National Audit and Research Centre, London UK
Elizabeth C. George
Associated data.
Malaria is one of the most important parasitic infectious diseases worldwide. Despite the scale‐up of effective antimalarials, mortality rates from severe malaria (SM) remain significantly high; thus, numerous trials are investigating both antimalarials and adjunctive therapy. This review aimed to summarise all the outcome measures used in trials in the last 10 years to see the need for a core outcome set.
A systematic review was undertaken to summarise outcomes of individually randomised trials assessing treatments for SM in adults and children. We searched key databases and trial registries between 1 January 2010 and 30 July 2020. Non‐randomised trials were excluded to allow comparison of similar trials. Trial characteristics including phase, region, population, interventions, were summarised. All primary and secondary outcomes were extracted and categorised using a taxonomy table.
Twenty‐seven of 282 screened trials met our inclusion criteria, including 10,342 patients from 19 countries: 19 (70%) trials from Africa and 8 (30%) from Asia. A large amount of heterogeneity was observed in the selection of outcomes and instruments, with 101 different outcomes measures recorded, 78/101 reported only in a single trial. Parasitological outcomes (17 studies), neurological status (14 studies), death (14 studies) and temperature (10 studies), were the most reported outcomes. Where an outcome was reported in >1 study it was often measured differently: temperature (4 different measures), renal function (7 measures), nervous system (13 measures) and parasitology (10 measures).
Outcomes used in SM trials are inconsistent and heterogeneous. Absence of consensus for outcome measures used impedes research synthesis and comparability of different interventions. This systematic review demonstrates the need to develop a standardised collection of core outcomes for clinical trials of treatments for SM and next steps to include the development of a panel of experts in the field, a Delphi process, and a consensus meeting.
INTRODUCTION
Malaria is one of the most important parasitic infectious diseases worldwide. The vast majority of all malaria cases (>90%) are attributable to Plasmodium falciparum , which produces high levels of parasitaemia. This subsequently causes sequestration of mature‐staged infected erythrocytes in the microvasculature of organs, resulting in micro‐circulatory ischaemia and multi‐organ failure [ 1 ]. Severe or complicated malaria is the most life‐threatening and potentially fatal manifestation of the disease and most cases will develop severe neurological deficit or death in the absence of prompt and effective management [ 1 , 2 ].
Despite the efforts and progress over the past years in reducing malaria incidence and mortality, since 2015 the disease control has levelled off [ 3 , 4 ]. The estimated global incidence of severe malaria (SM) reaches 2 million reports every year [ 2 ], with children under 5 years and pregnant women living in sub‐Saharan Africa being the most vulnerable groups [ 3 , 5 ]. Even though the standard treatment of artesunate following the landmark SEAQUAMAT [ 6 ] and AQUAMAT [ 7 ] trials has improved outcomes in SM, the mortality rates remain significantly high, with rates ranging from 10% to 20% for in‐hospital care and up to 100% for patients unable to reach health facilities [ 8 , 9 ]. The heaviest burden falls on children from resource limited countries where very few will ever reach a high dependency or intensive care unit to receive specialised treatment [ 10 ]. The global emerging need for effective treatment strategies alongside antimalarials has also led to research in adjunctive treatments to improve clinical outcomes and a number of randomised controlled trials (RCTs) in adjunctive therapy in order to treat SM successfully [ 11 ].
A critical component in measuring and evaluating antimalarial or adjunctive treatment efficacy is the selection of appropriate outcomes for the RCTs [ 11 , 12 ]. However, to date there is no agreed uniform method to assess the efficacy of antimalarials or adjunctive treatments and no conclusive analysis of outcomes being used in this area has been published. In addition to that, there is a growing recognition that the lack of consensus with regard to the outcomes measured in trials leads to inconsistencies and high heterogeneity between reported outcomes [ 13 ]. Furthermore, only comparable and standardised outcomes can be pooled in meta‐analyses, providing meaningful trial comparisons and informing clinical practice.
A standardised minimum set of outcomes, known as a Core Outcome Set (COS), would help define clinically meaningful outcomes for the treatment of SM, facilitate transparency and reduce outcome reporting bias, while enhancing the credibility and validity of future trials [ 13 , 14 , 15 , 16 ]. Researchers are not restricted to the outcomes recommended in the COS, but rather use as a primary outcome those outlined in the COS, and continue exploring other relevant and important health outcomes, which may be at different points along the clinical pathway to serious illness and mortality [ 13 , 14 ].
The aim of this study is to describe the outcomes reported in individually randomised trials investigating treatments for SM in adults and children. This systematic review of trials in this area over the last 10 years will help further demonstrate the need for a core set and also provide a comprehensive synthesis of all outcomes published.
Search strategy
A systematic review in line with the 2009 Preferred Reporting Items for Systematic Reviews and Meta‐Analyses statement (PRISMA) guidelines was performed [ 17 ]. Planned, ongoing or completed interventional RCTs evaluating treatments for SM were considered eligible. Three key databases and three clinical trial registries were searched (May 2020), including ‘Cochrane Central Register of Controlled Trials’ (CENTRAL) published in The Cochrane Library, MEDLINE (OVID), ‘Literatura Latino Americana em Ciências da Saúde’ (LILACS), ‘International Standard Randomised Controlled Trial Number’ (ISRCTN), ClinicalTrials.gov and ‘Pan‐African Clinical Trial Registry’ (PACTR). In addition, the citation lists of retrieved trials were checked for additional eligible records.
Inclusion criteria were RCTs between 2010 and 2020 involving only hospitalised adults or children with SM (including cerebral malaria) following the WHO definition [ 2 ], and comparing antimalarial or adjunctive treatments for SM. Observational studies, literature reviews, secondary analyses of RCTs, non‐randomised trials, prevention or vaccine studies, trials including both severe and uncomplicated malaria, non‐human trials, conference abstracts, and posters were excluded.
The search terms were developed on the basis of four key concepts: SM, treatment, hospital and Randomised Controlled Trial. The search was limited to trials published in the English language. The time period restriction was to provide a comprehensive snapshot view of the outcomes from trials published in the last decade. A full list of search terms, concept construction and data extraction processes are provided in Table S1 .
Data extraction and analysis
The primary reviewer (LL) double‐screened potentially relevant records based on titles and abstracts, and reviewed the full text of selected trials to assess for eligibility. Data from eligible RCTs was then extracted and entered into tables created in Microsoft Word (Table S2 ). Eligible trials identified through the trial registries that did not include published results, were cross‐checked using registration number, primary investigator name, and country of study against the published studies in order to screen‐out duplicates. Trials requiring further review were discussed and resolved by consensus with the second reviewer (ECG). Trials that did not meet the inclusion criteria were excluded with reasons outlined in the PRISMA flowchart (Figure 1 ).

PRISMA flowchart
All primary and secondary outcome measures from eligible trials were summarised and tabulated (Table S2 ). Descriptions of all outcome measures were extracted verbatim from the study protocols or trial registries. Primary outcome measures that were not defined clearly in the trial publication were identified from the sample size calculations. The instruments used for the quantification of each outcome were also captured. The outcome measures were then grouped and categorised into the domains of the outcome taxonomy [ 15 ] recommended by the ‘Core Outcome Measures in Effectiveness Trials’ (COMET) initiative [ 14 ].
Our search retrieved 159 records from electronic bibliographic database searches and 119 from trial registries (Figure 1 ). Four additional trials were identified from the citation lists of the retrieved papers. After removing 18 duplicate trials, 264 unique records were processed through title and abstract screening. One hundred and ninety studies were then excluded after screening title and abstract (if published) or study characteristics (on trial registries). Finally, 74 full‐text studies were comprehensively reviewed, resulting in 27 records that met the inclusion criteria for this review (Figure 1 ).
Included studies
A total of 10,342 patients with SM were included in 27 trials [ 7 , 18 , 19 , 20 , 21 , 22 , 23 , 24 , 25 , 26 , 27 , 28 , 29 , 30 , 31 , 32 , 33 , 34 , 35 , 36 , 37 , 38 , 39 , 40 , 41 , 42 , 43 ], of which 7702 patients were included in trials testing primary antimalarial therapies and 2640 in trials testing adjunctive therapies in addition to standard antimalarial treatment. The majority of the trials conducted in the last decade studied the role of adjunctive therapy in the management of SM ( n = 18, 67%). The trial interventions are classified as drug‐related in 26 studies [ 7 , 18 , 20 , 21 , 22 , 23 , 24 , 25 , 26 , 27 , 28 , 29 , 30 , 31 , 32 , 33 , 34 , 35 , 36 , 37 , 38 , 39 , 40 , 41 , 42 , 43 ] and device‐related in only one study [ 19 ]. The control group was a placebo or no intervention in 12 trials (44%) and active treatment in 13 trials (56%). Two further trials [ 23 , 25 ] had dose‐comparison concurrent control. Fifteen (56%) trials included patients aged 3 months‐15 years and 12 (44%) included patients of all ages. Overall, 18 trials were open‐label and 7 used blinding; 2 further trials did not report any blinding methods. Full details with study characteristics are provided in Table S2 .
As shown in Table 1 and Figure 2 , the highest proportion of studies was conducted in sub‐Saharan Africa ( n = 19, 70%). Three additional trials were multi‐centre enrolling patients across several sub‐Saharan countries. Most of these studies ( n = 11, 60%) were RCTs studying adjunctive therapies for the treatment of SM, while eight ( n = 8, 40%) were on antimalarial treatments. Eight trials in total were conducted in Asia ( n = 8, 30%), with 7 (88%) studying adjunctive therapies for SM and only 1 trial was on antimalarial treatment.
Baseline characteristics of randomised controlled trials in treatment of severe malaria

As summarised in Table 1 , nine RCTs in the period 2010–2020 were studying antimalarial drug treatments for SM [ 7 , 18 , 25 , 27 , 29 , 31 , 37 , 38 , 40 ]. The largest proportion (7/9) of the antimalarial trials studied the efficacy of intravenous artesunate over intravenous quinine for the treatment of SM, one phase 2 trial checked the bioavailability of rectal artesunate and one other checked the superiority of intramuscular artesunate over intramuscular artemether.
The majority ( n = 18, 67%) of SM trials conducted in the period 2010–2020 studied the role of adjunctive treatments for the management of SM (Table 1 ) [ 19 , 20 , 21 , 22 , 23 , 24 , 26 , 28 , 30 , 32 , 33 , 34 , 35 , 36 , 39 , 41 , 42 , 43 ]. The adjunctive treatments that were tested in these trials were: azithromycin, paracetamol (2 trials), paracetamol versus ibuprofen, hypertonic saline versus mechanical ventilation, rosiglitazone, levetiracetam, iron supplements, nitric oxide (2 trials), dextran versus hydroxyethyl starch, enteral feeding, levamisole, vitamin A, mannitol, ursoxycholic acid and l ‐arginine. However, to date none of the published studies have shown clear efficacy results while a few are still ongoing.
Outcomes assessed
Overall, 101 distinct outcomes are evaluated in the included trials, and were inconsistently reported and measured among the 27 RCTs (Figure 3 ). Twenty‐three (85%) of the trials pre‐defined their primary and secondary outcomes. We identified 11 generic outcome categories, ordered following the taxonomy [ 15 ]. Death was a frequent outcome domain measured in 14/27 trials, with 11 of them testing adjunctive therapies. Parasite clearance time, neurological abnormalities and fever, the most commonly assessed outcomes, were only assessed in 17, 14 and 10 studies, respectively. All remaining outcomes were evaluated in less than half of the trials, and 78/101 were only reported in a single trial, highlighting an important heterogeneity in outcomes selection. The least reported outcomes were life impact and resource use.

Hierarchical representation of outcomes recorded in 27 severe malaria trials. Outcomes ordered by domain. Numbers in brackets illustrates number of studies reporting outcome. All outcomes corresponding to each study are listed in full in appendix. Outcomes in green reported in only a single study. AKI, acute kidney injury; BCS, Blantyre Coma scale; EEG, electroencephalogram; FBC, full blood count; SAE, serious adverse events. Figure design taken from Webbe et al. [ 44 ]
Studies reported using a variety of methods, sometimes in combination, to measure the outcomes within the same outcome domain. Also, each of the outcome domains included different and inconsistent outcome measures across the trials and more than 90 outcomes appeared only once or twice (Figure 3 ). The highest inconsistency was seen in the biomarkers/laboratory values domain, where 20 unique outcome measures were used.
Where trials had reported the same outcome, for example seizures, these may not be comparable as different outcome measures were used; for example, time for convulsions to stop after initiating treatment [ 34 ] , minutes with seizures on electroencephalogram (EEG) 72 h after treatment allocation [ 39 ], or seizures detected clinically or on daily EEG during the study duration [ 24 ]. Renal function was measured using 7 outcomes: Acute Kidney Injury (AKI) development, AKI progression, AKI duration, Major Adverse Kidney Events (MAKE), Assessment of Acute Kidney Injury, haemodialysis trends, urine colour chart, creatinine concentration, and AKI biomarkers. Where trials reported the AKI definition, this was also different as one defined AKI as ‘creatinine ≥26.5 μmol/L or ≥1.5× baseline’ [ 43 ], while another defined it as ‘(i) an absolute increase in serum creatinine of >26.5 μmol/L from enrolment creatinine; (ii) a percentage increase in serum creatinine of >50% from enrolment; (iii) post‐enrolment onset of oliguria of less than 0.5 ml/kg/h for more than 6 h; (iv) 24 h urine output of <400 ml after rehydration and urinary obstruction excluded’ [ 30 ]. AKI development also differed in measurement timing: first 7 days of enrolment, over 72 h, and 14 days were used.
Outcome measures in fever comprised fever clearance time, mean maximum temperature, area above the fever versus time curve, and fever duration. Where fever clearance time was measured, the time frame included: during the first 7 days from enrolment, 72 h, and the time at which the axillary temperature first dropped below 37.5°C and remained below 37.5°C for 24 h. Parasite clearance time was defined and assessed also at different time points, including the first 7 days of enrolment, 72 h, time (in h) from the onset of treatment to the time of the first of two successive negative blood smears through hospital discharge, first 24 h after initiating treatment, start of the treatment until the first negative blood film, with the blood film then remaining negative for 24 h (Figure 3 , Table S3 ).
Furthermore, same phase trials measured mortality distinctively; for instance mortality within 48 h post hospital admission and 14 days post hospital admission [ 36 ], mortality within 7 days of randomisation [ 19 ], mortality within 4 weeks [ 42 ], mortality at 48 h [ 20 ], mortality at 48 days and 90 days [ 23 ].
Among 27 included studies and after reviewing published papers and protocols where available, we found that no study reported patient or public involvement in outcome selection.
This study is, to our knowledge, the first comprehensive systematic review of outcome measures used in RCTs studying treatments for SM, summarising data from 27 trials [ 7 , 18 , 19 , 20 , 21 , 22 , 23 , 24 , 25 , 26 , 27 , 28 , 29 , 30 , 31 , 32 , 33 , 34 , 35 , 36 , 37 , 38 , 39 , 40 , 41 , 42 , 43 ] and 10,342 patients. Our review shows that the focus over the last 10 years for the management of SM is on adjunctive drug treatments. To date, parenteral artesunate remains the standard antimalarial treatment for SM, while no new antimalarial agents have been investigated in an RCT. Importantly, we found that although SM still accounts for substantial morbidity and mortality worldwide and there are trials in the pipeline for SM treatments, we highlight that there is no uniform method of measuring outcomes in this area, which subsequently results in many different and heterogeneous outcomes.
The highest proportion of SM trials between 2010 and 2020 were conducted in sub‐Saharan Africa ( n = 19, 70%), where most of the cases are reported. Although children under 5 years of age are the most vulnerable group affected by SM [ 5 , 10 , 45 ], our findings suggest that the past decade's trials were not focused entirely on the pediatric population, with 15/27 (56%) of the SM trials enrolling patients aged 3 months‐15 years. One explanation could be that few of the children with SM will reach health centres and be cared for in specialised units, so most will die before reaching hospital facilities [ 46 , 47 , 48 ]. Also, the sociocultural differences, values and beliefs around medical research, and barriers of obtaining informed consent in critically ill paediatric population, are only some of the factors hindering clinical trials in socioeconomic deprived regions such as sub‐Saharan Africa and Asia [ 49 , 50 ]. Lastly, despite the substantial morbidity and mortality of maternal SM [ 51 ], pregnant women were not included in randomised trials of treatment drugs or adjunctive therapy over the past decade, and therefore there would need to be careful consideration of the generalisability of findings onto this population [ 52 ].
This review aimed to identify all outcomes that have been used in RCTs studying treatments for SM in order to inform the design and development of a COS in light of the COMET initiative [ 14 ]. For this reason, we summarised all the primary and secondary outcomes of published and unpublished RCTs studying the treatment of SM. While the selected trials for analysis are comparable to each other with regards to their design, scope and patient inclusion criteria, as they are all individually randomised RCTs studying treatment options for patients with SM in hospital settings, the outcome measures showed wide variability. Due to this, we attempted to organise and group the outcome measures into main outcome domains by using the outcome taxonomy [ 14 ] recommended by the COMET initiative [ 15 ].
This review quantifies the high degree of inconsistency and heterogeneity around outcome measures used SM trials (Figure 3 ). This could be explained by the fact that malaria is a disease that affects multiple organs and hence different treatments are targeting different outcome domains. However, it should be noted that the large variability was not only evident between the outcome domains across trials, but within each subdomain too. More precisely, outcomes such as parasite clearance time, neurological abnormalities and fever were inconsistently measured and reported at multiple time points (which were often poorly specified). In addition, even an outcome as crucial as survival has not been universally reported. Heterogeneity of outcome selection is further illustrated by the large number of outcome measures only reported in a single trial (78/101), which reflects the high degree of variety between outcomes measured and reported in SM.
Therefore, the heterogeneity between the selected outcomes, as well as how the outcomes were measured and reported, identifies a clear obstacle to evidence synthesis. This finding is not something new in clinical research as several reports have previously highlighted this as a major problem [ 13 , 16 , 53 ]. Various areas of health have had a COS developed and successfully implemented, particularly in rheumatology and chronic pain [ 54 , 55 ], however, to date no work has been done in SM. Using a validated and standardised tool such as COS in this area, would limit outcome reporting biases, prevent research waste, fewer trials would have to be excluded in meta‐analyses and less heterogeneity would be seen in reported outcomes [ 13 , 14 ]. Also, the COS includes the ‘minimum set of outcomes’, and therefore researchers are still able to explore other relevant and important health outcomes [ 13 ]. The outcome measures summarised in this review can be used as the starting point and catalyst for the development of a COS.
Like sepsis, SM has a complex clinical presentation including several complications [ 10 ], that require a range of targeted adjunctive treatments which poses a challenge to standardising outcomes. This challenge has been discussed with experts from the Severe Malaria—A Research and Trials (SMAART) Consortium (funded by the Wellcome Trust [209265/Z/17/Z]) and they noted that part of the development of new therapies targeting each complication requires Phase II trials. These smaller trials require endpoints that lead to different outcomes that reflect the treatment's impact on the organ or syndrome that is being targeted, as clinical surrogate outcomes for prognosis. However, it has been noted that even if surrogate endpoints are being used to help address the issue of underpowered studies, mortality should always be measured as a secondary endpoint [ 56 ]. These trials also are not often able to capture continuing mortality and morbidity during the months following admission for SM. For antimalarials, lactate concentration was found to be a valid surrogate endpoint at 8 or 12 h after admission if studies were aiming to improve microcirculation, but measures of coma recovery were not valid as surrogates for mortality [ 57 ]. The validation of biomarkers as surrogate endpoints is challenging, as meta‐analyses of many trials of drugs in the same class are required measuring both the surrogate and the clinical endpoint [ 57 ], which may also have delayed the assessment of the need for a COS. To develop a COS evidence for the validity of surrogate outcomes would also need to be reviewed in any consensus meeting along with definitions and how each would be measured using the guidance on developing outcome measurement instruments [ 58 ]. Any Delphi process to create a COS would need to be focussed on outcomes and how to standardise measurement and definitions across the different clinical syndromes, rather than research processes or impact as has been done previously [ 59 ].
A key strength of our review was the comprehensive synthesis of evidence around outcomes used in SM trials, which goes beyond anything previously published in the literature of this field. This analysis provides also a descriptive assessment of the current portfolio of all RCTs that have been done in SM over the last decade, including phases, population characteristics, region, treatment features, and outcome measures. This review adhered to systematic review methods, including screening a wide range of electronic bibliographic databases as well as clinical trial registries, and appraising a large body of published and unpublished trials from 13 countries. By comprehensively searching the trial registries we were able to assess all the current registered trials, despite their publication status and therefore we aimed to minimise publication bias. Lastly, the inclusion and exclusion criteria were built around the WHO definition for SM, which utilised a standardised format in order to summarise data from similar studies and allow fair comparisons.
Our review has limitations. We did not examine other types of literature such as reviews nor certain types of studies, such as observational studies and non‐randomised trials. However, their exclusion allowed extrapolation of conclusions from comparing similar trials. Furthermore, the restriction to English language trials does have the risk of missing some trials. The restriction of studies from the 10‐year period 2010 to 2020 is also a potential limitation and some information on outcomes from trials published prior to this may have been missed. Finally, the included trials were assessed for relevance by only a single person, however, uncertainties and missing information were resolved by consensus with the author ECG.
To the best of our knowledge, this is the first study to systematically review all outcomes measured in RCTs of SM treatment and inform the need for a COS in this field. Given the lack of universal adoption of a minimum set of core outcomes and the consequently high variability in outcomes measured, it becomes evident that development of a COS for SM treatment is an urgent task. Also, since SM has a large incidence globally, these results are crucial and need to be highlighted in order to conceive future work; especially given the trials in the pipeline of drug development which would benefit from a COS. Next steps include the development of a panel of experts in the field, a Delphi process, and a consensus meeting. Lastly, a key stage in the development of a high quality COS would include established international collaboration between key stakeholders, including health professionals, researchers, and patient representatives, in the selection and specification of these outcomes [ 13 , 14 , 60 ].
FUNDING INFORMATION
Funding for ECG is provided by the Medical Research Council as core support to the MRC CTU at UCL (MC_UU_00004/05).
Supporting information
Appendix S1 Supporting Information
ACKNOWLEDGEMENTS
We thank experts from the Severe Malaria ‐ A Research and Trials (SMAART) Consortium for their time in discussing this work and the challenges in standardising outcomes.
Lampro L, George EC. Outcomes reported in trials of treatments for severe malaria: The need for a core outcome set . Trop Med Int Health . 2022; 27 ( 9 ):767–775. 10.1111/tmi.13803 [ PMC free article ] [ PubMed ] [ CrossRef ] [ Google Scholar ]
Sustainable Development Goal: Good Health and Wellbeing
Funding information Medical Research Council, Grant/Award Number: MC_UU_00004/05
Thank you for visiting nature.com. You are using a browser version with limited support for CSS. To obtain the best experience, we recommend you use a more up to date browser (or turn off compatibility mode in Internet Explorer). In the meantime, to ensure continued support, we are displaying the site without styles and JavaScript.
- View all journals
- My Account Login
- Explore content
- About the journal
- Publish with us
- Sign up for alerts
- Open access
- Published: 22 April 2024
Estimating the effects of temperature on transmission of the human malaria parasite, Plasmodium falciparum
- Eunho Suh ORCID: orcid.org/0000-0003-1761-0019 1 na1 ,
- Isaac J. Stopard ORCID: orcid.org/0000-0003-4612-5004 2 na1 ,
- Ben Lambert ORCID: orcid.org/0000-0003-4274-4158 3 ,
- Jessica L. Waite 1 nAff5 ,
- Nina L. Dennington 1 ,
- Thomas S. Churcher ORCID: orcid.org/0000-0002-8442-0525 2 &
- Matthew B. Thomas 1 , 4 nAff6
Nature Communications volume 15 , Article number: 3230 ( 2024 ) Cite this article
134 Accesses
10 Altmetric
Metrics details
- Ecological epidemiology
- Epidemiology
- Parasite development
Despite concern that climate change could increase the human risk to malaria in certain areas, the temperature dependency of malaria transmission is poorly characterized. Here, we use a mechanistic model fitted to experimental data to describe how Plasmodium falciparum infection of the African malaria vector, Anopheles gambiae , is modulated by temperature, including its influences on parasite establishment, conversion efficiency through parasite developmental stages, parasite development rate, and overall vector competence. We use these data, together with estimates of the survival of infected blood-fed mosquitoes, to explore the theoretical influence of temperature on transmission in four locations in Kenya, considering recent conditions and future climate change. Results provide insights into factors limiting transmission in cooler environments and indicate that increases in malaria transmission due to climate warming in areas like the Kenyan Highlands, might be less than previously predicted.
Similar content being viewed by others
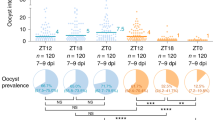
The influence of feeding behaviour and temperature on the capacity of mosquitoes to transmit malaria
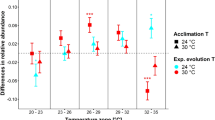
Unexpected behavioural adaptation of yellow fever mosquitoes in response to high temperatures
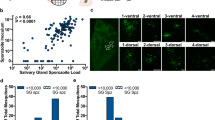
Revisiting the Plasmodium sporozoite inoculum and elucidating the efficiency with which malaria parasites progress through the mosquito
Introduction.
Malaria is a leading cause of morbidity and mortality in many tropical and sub-tropical regions 1 . The transmission of malaria parasites is inextricably linked to the biology of the mosquito vector. Classic models that estimate the Basic Reproduction Number (R 0 ) or Vectorial Capacity 2 , 3 , 4 indicate that traits such as mosquito life expectancy, vector competence, per-mosquito biting rate, and the Extrinsic Incubation Period (EIP; the duration of sporogony) are important determinants of malaria transmission. Despite their epidemiological importance, these traits are poorly characterised for most malaria vectors. A limited understanding of these traits and their interaction with environmental variables, such as temperature, constrains our ability to understand current patterns of transmission or future responses to climate change.
The EIP describes the time it takes for a mosquito to become infectious following an initial infected blood meal. This process involves parasites infecting the midgut, undergoing obligate sexual and asexual reproduction via multiple life stage transitions and then migrating to the mosquito salivary glands where they may be secreted into saliva 5 , 6 . Mosquitoes are ectothermic and although the EIP can be influenced by different biotic and abiotic factors 7 , the single biggest known determinant is ambient temperature 8 , 9 , 10 , 11 , 12 . For many decades, the temperature dependence of the EIP for Plasmodium falciparum , the most important human malaria-causing parasite, has been characterised using a single degree-day model 7 , 13 . This model assumes there is a fixed number of degree-days ( D ), or heat units, that need to be accumulated for the completion of sporogony such that,
where T is the mean daily temperature and T min is the lower temperature threshold for parasite development. For P. falciparum , D was estimated over 60 years ago to be 111 with T min equal to 16 °C 7 , 13 . Existing climate sensitive malaria models that account for the effects of temperature on the EIP when predicting malaria endemicity or transmission intensity commonly rely on this model 14 , 15 , 16 , 17 , 18 , 19 , 20 , 21 , 22 . Despite its extensive application, the established degree-day model remains poorly validated, and several potential limitations have been identified 7 , 14 , 15 , 16 , 17 , 18 , 19 , 20 , 21 , 22 . First, the model was parameterised based on a single minimally replicated experiment 23 , with the EIP measured as the first day at which an individual mosquito was observed to have parasites in the salivary glands 7 , 13 . Recent evidence, however, indicates variation in the EIP between mosquitoes exposed to the same environment 24 , 25 . Second, there were no empirical measures of the EIP below 19-20 °C, meaning the degree-day model estimates at cooler temperatures are based on extrapolation. However, exploratory experiments measuring EIP below 20 °C indicate this extrapolation may overestimate the EIP 25 . Finally, the original experiment was conducted with Anopheles maculipennis , a mosquito species complex responsible for malaria transmission in Eurasia 7 . The EIP is known to vary between Plasmodium species 13 , 26 , but it is unclear whether it varies between different mosquito species 7 , 25 and there has never been an EIP model developed for any dominant vector species in Africa.
Our understanding of the effects of environmental temperature on other important traits, such as the vector competence (the proportion of mosquitoes successfully develop infectious parasites in the salivary glands following an initial infectious blood meal) and mosquito longevity are similarly incomplete. A limited number of studies indicate that parasite establishment in the mosquito is sensitive to temperature 27 , 28 . There is, however, insufficient data on subsequent P. falciparum development in Anopheles gambiae , and mechanistic modelling studies examining the influence of climate on malaria transmission tend to either assume the vector competence is temperature invariant, or utilise data from infection experiments using inappropriate mosquito-parasite pairings such as species of North American vector infected with P. vivax 9 , 22 , 29 , 30 , 31 . Similarly, data on the effects of temperature on the survival of key malaria vectors are also scant, particularly in the field 32 .
Here, we generate a comprehensive set of experimental infection data for P. falciparum in the dominant African malaria vector, A. gambiae , across a range of temperatures. Mosquitoes are dissected to determine the presence of different parasite stages, so it is not possible to observe the parasite population dynamics within single mosquitoes. To estimate temperature-dependent changes in the EIP distribution, vector competence and parasite load among the mosquito population, we fit a mechanistic model 33 that simulates the temporal dynamics of sporogony. We use these estimates, together with data on temperature-dependent mosquito mortality rate, to parameterise a model of vectorial capacity and use this to investigate the effects of temperature on current and future transmission in four locations in Kenya.
Experimental infection of P. falciparum in A. gambiae mosquitoes at different temperatures
We began with a pilot study to refine sample sizes and to better understand the relationship between gametocytemia (percent gametocyte infection in red blood cells) and infection dynamics at different temperatures. A. gambiae mosquitoes (G3) were fed infectious blood meals with P. falciparum (NF54) gametocytemia of 0.024% within a standard membrane feeding assay and then maintained at constant temperatures of 17, 19, 21, 23, 25, 27 or 29 °C. The maximum oocyst infection was observed at 25 °C with oocyst prevalence of 45% (95% CI: 23.1–68.5%) and mean oocyst intensity of 5 (95% CI: 1.6–8.4; Supplementary Fig. 1a ). However, little or no infection was observed at 17 °C and 21 °C, and sporozoite infection was low at the temperature extremes (Supplementary Fig. 1b ). In the next two experimental infectious feeds (referred to herein as Feed1 and Feed2), gametocytemia in the blood meal and the mosquito sample size were increased to obtain more robust infection data. In Feed1, gametocytemia was adjusted to approximately 0.126% in the blood meal and the fed mosquitoes were kept at 17, 19, 21, 24, 25, 27, or 29 °C throughout. In Feed2, gametocytemia was increased to 0.139% and the blood-fed mosquitoes were kept at 18, 19, 20, 23, 25, 28, 29, and 30 °C. In these two feeds, maximum oocyst prevalence was observed at 23 and 25 °C for Feed1 (83.3%) and Feed2 (83.7%), respectively. For both feeds the mean oocyst intensity peaked at 23 °C, with 23.5 (Feed1) and 54.0 (Feed2) oocysts per mosquito with any observed oocysts. The lowest infection was observed at 17 and 18 °C, with oocyst prevalence of 13.3 to 25%, and mean oocyst intensities of 1.3 and 2.4 oocysts per oocyst-positive mosquito for Feed1 and Feed2, respectively (Supplementary Fig. 1a ). Sporozoite prevalence estimates from Feed1 & Feed2 are presented in Fig. 1 . There was little effect of parasite load on EIP in a preliminary study 34 , which is also consistent with another recent report 35 , so data from Feed1 & Feed2 were combined when modelling the EIP.
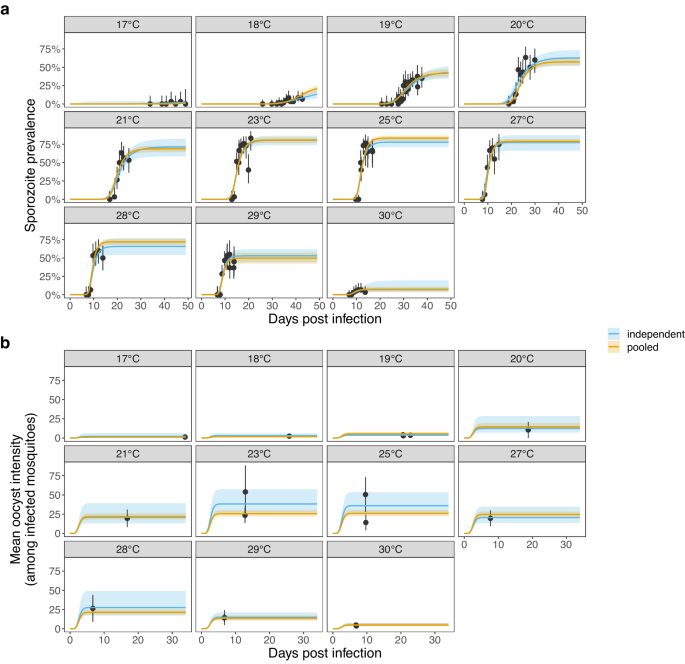
The temporal dynamics of sporogony is first fit to data from mosquitoes exposed to each constant temperature independently (independent model; blue lines). The model is also fit to all the standard membrane feeding assay mosquito dissection data simultaneously by fitting functional forms between temperature and certain model parameters (the independent model parameter estimates were used to guide the choice of functional forms) (pooled model; yellow lines). The actual (points) and predicted (lines) sporozoite prevalence and mean oocyst intensity are shown in ( a ) and ( b ) respectively. Uncertainty is shown by the 95% credible intervals of the posterior predictive means for the model estimates and 95% confidence intervals (error bars) for the experimental data, which was conducted with n = 4195 mosquitoes dissected for sporozoites ( a ) and n = 520 mosquitoes dissected for oocysts ( b ). For the binomial proportion data, confidence intervals were calculated using the Clopper-Pearson method. The facets indicate the constant temperature at which mosquitoes were maintained.
Extrinsic Incubation Period
Sporogony varies between individual A. gambiae mosquitoes exposed to the same temperature, which can be represented by a probability distribution with a mean and variance. It’s not possible to estimate the EIP of individual mosquitoes if they are dissected, but the cumulative increase in sporozoite prevalence with days post infection (DPI) is indicative of the cumulative distribution function (CDF), which represents the EIP variation across specimens. We estimate temperature-dependent changes in the EIP distribution and the human-to-mosquito-transmission probability (HMTP; the conditional probability of infection given the mosquito is alive) by fitting a mechanistic model of sporogony to the individual mosquito dissection data (oocyst intensity [counts] and sporozoite presence [1: any salivary gland sporozoites; 0: none]) from Feed1 and Feed2, in a Bayesian framework using Stan’s Markov Chain Monte Carlo (MCMC) sampler. We fitted two types of model: an independent model, based on models fitted separately to data from individual temperatures; and a pooled model, based on a single model fitted to data from all temperatures, where certain model parameters vary as a function of temperature. In Fig. 1a, b , we show these model fits to sporozoite prevalence and oocyst intensity data (among mosquitoes with observable oocysts), respectively. Except for sporozoite prevalence at 17 °C, the actual vs fitted plots and R 2 or Brier skill scores indicate the model reasonably predicts the sampled values (Supplementary Fig. 2 ).
Results indicate that the EIP decreases as temperature increases (Fig. 2a ). Our new EIP model enables us to describe the temporal variation in sporogony among mosquitoes at a given temperature: the time for 10%, 50% (the median) and 90% of the mosquito population to become infectious are denoted by EIP 10 , EIP 50 and EIP 90 . Consistent with the degree-day model, we see a non-linear increase in the EIP at lower temperatures. EIP 10 , for example, increased from 7.6 days (95% Credible Intervals, CrI: 7.2–8.0) at 30 °C to 49.1 days (95% CrI: 44.2–55.1) at 17 °C (pooled model estimates) (Fig. 2a ). The range between EIP 10 and EIP 90 decreased with increases in temperature: from 21.7 (95% CrI: 17.6–27.6) days at 17 °C to 3.7 (95% CrI: 3.4–4.0) days at 30 °C (Fig. 2a and Supplementary Fig. 3a ). Similarly, the EIP distribution variance decreased with increases in temperature (Supplementary Fig. 3b ). When the EIP standard deviation was normalised by the mean EIP there were little changes in this quantity with temperature, indicating that the variance scales with the mean (Supplementary Fig. 3c ). The standard degree-day model, however, assumes all mosquitoes have the same EIP at a given temperature (Fig. 2a ).
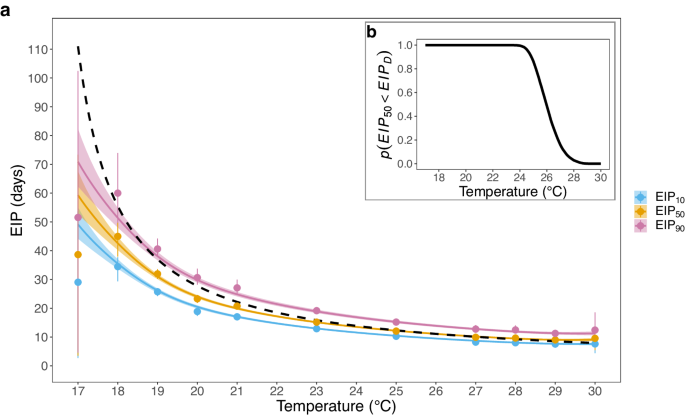
a The solid blue, yellow and pink lines show the relationship between temperature and pooled model EIP 10 , EIP 50 and EIP 90 estimates respectively. Points show the equivalent independent model estimates. Data are presented as the median posterior values with the 95% credible intervals. The dashed black line shows the degree day model estimates. b shows the probability the pooled model posterior EIP 50 estimates are less than the corresponding degree-day model values (EIP D ), which were estimated using the 10,000 posterior MCMC values.
Using the pooled model fits, we also calculated the probability that our EIP 50 estimates are less than the degree-day model estimate: \(p\left({{EIP}}_{50}\, < \,{{EIP}}_{D}\right)=0.95\) at approximately 24.6 days and \(p\left({{EIP}}_{50}\, < \,{{EIP}}_{D}\right)=0.05\) at approximately 27.7 days (Fig. 2b ). These metrics indicate the established degree-day model provides a reasonable approximation of our EIP estimates at intermediate temperatures but becomes increasingly inaccurate at the temperature extremes. This finding is consistent with the original empirical data to which the degree-day model was fitted, which is also lower than the EIP predicted by the degree-day model at the lowest experimental temperature (Supplementary Fig. 4 ).
Vector competence
The predicted mean oocyst load among infected mosquitoes ( μ ) varied unimodally with temperature: the pooled model peaked at 24.5 °C with a posterior value of 20.3 (95% CrI: 16.9–23.9) oocysts per mosquito (Fig. 3a ). The maximum HMTP determined by the presence of any oocysts ( δ O ) varied unimodally with temperature: the pooled model value peaked at 24.8 °C with a posterior value of 83% (95% CrI: 80–86) (Fig. 3b ). The probability that mosquitoes with any oocysts will mature any sporozoites ( δ S ; conversion of oocyst to sporozoite infection at the mosquito scale) is close to 100% at most of the temperatures investigated (Fig. 3c ). At temperature extremes (and especially high temperatures), however, this value decreased: at 30 °C, for example, the pooled model posterior estimate is 14% (95% CrI: 9–20). As a result, the vector competence (we define as the maximum HMTP measured by the presence of any salivary gland sporozoites, δ O δ S ) is unimodal and left-skewed, with a maximum at ~24.8 °C with a posterior value of 83% (95% CrI: 80–87; Fig. 3d ).
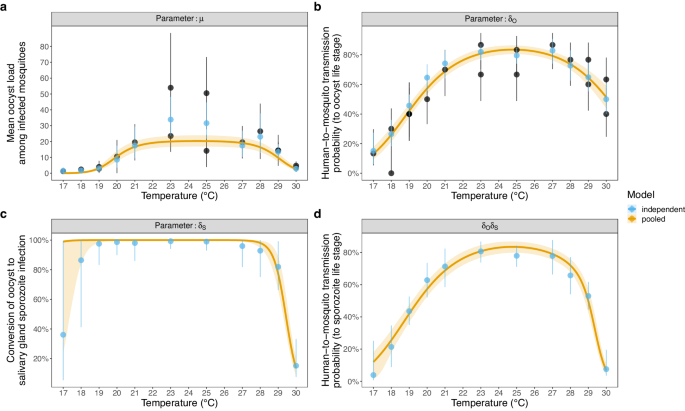
a μ (the mean oocyst load among infected mosquitoes), b \({{{{{\delta }}}}}_{{{{{O}}}}}\) (the human-to-mosquito transmission probability, as determined by the presence of oocysts), c \({{{{{\delta }}}}}_{{{{{S}}}}}\) (the probability a mosquito infected with any oocysts will develop any sporozoites at the mosquito scale: conversion), and d \({{{{{\delta }}}}}_{{{{{O}}}}}{{{{{\delta }}}}}_{{{{{S}}}}}\) (vector competence; the human-to-mosquito transmission probability, as determined by the presence of sporozoites). For ( a ) and ( b ) black points show the sampled mean oocyst load and oocyst prevalence disaggregated by day of dissection with the 95% confidence intervals respectively, which were estimated from n = 520 dissected mosquitoes. All mosquitoes were dissected at sufficient days post infection that all oocysts were expected to have developed. For all plots blue points show the independent model estimates and yellow lines show the pooled model median posterior estimates with the 95% credible intervals.
A . gambiae survival
Survival of mosquitoes fed with an infected blood meal was monitored across a range of temperatures from 17 to 30 °C. Cumulative survival was temperature-dependent (Fig. 4 ; Kaplan–Meier Log-Rank test for Feed1: χ 2 = 343.1, df = 6, P < 0.0001; Kaplan–Meier Log-Rank test for Feed2: χ 2 = 1464, df = 7, P < 0.0001), with median survival increasing as temperature decreased, at least across the temperature range studied (Supplementary Table 1 ). The cumulative survival data were best fitted by a Gompertz survival function, as indicated by the lowest corrected Akaike Information Criterion (AICc) values among four different survival models (Fig. 4 , Supplementary Table 2 and Supplementary Table 3 ), which indicates the laboratory-based mosquitoes die at a faster rate as they age (i.e. survival is age-dependent). Survival was higher in Feed2 at all four temperatures (Kaplan–Meier Log-Rank; χ 2 = 109.3, P < 0.0001 for 19 °C; χ 2 = 35, P < 0.0001 for 23 °C; χ 2 = 32, P < 0.0001 for 25 °C; χ 2 = 65.2, P < 0.0001 for 29 °C).
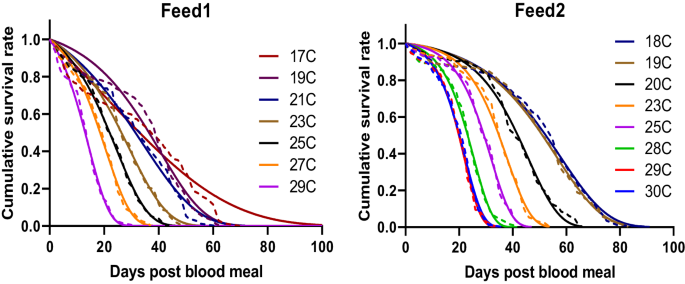
The cumulative survival was fitted with four representative survival models which were Gompertz, Weibull, loglogistic and negative exponential to determine best fit functions (see Supplementary Table 2 for model comparison). Gompertz model (solid lines) best describes cumulative survival (dashed lines) of the mosquitoes (see Supplementary Table 3 for parameter values of Gompertz model). Source data are provided as a Source Data file.
Implications for transmission and possible effects of climate warming
To explore the theoretical implications of our laboratory-derived parameter estimates for malaria transmission, we investigated how the Vectorial Capacity (VC) varied given recent and future mean temperatures for four locations in Kenya spanning a wide temperature range (Kericho, Kitale, Kisumu and Garissa, listed from coldest to warmest). Estimates of recent mean temperatures (1981–2000) and downscaled projections for future mean temperatures (2046–2065) for these locations were obtained from previous malaria-climate research 36 (Table 1 ). VC is a common transmission metric that describes the number of infectious mosquito bites arising per infectious human per day assuming complete transmission efficiency and a susceptible human population 37 . VC is related to the basic reproduction number, R 0 , but comprises a subset of the parameters to provide a measure of the entomological transmission potential of a mosquito population. Absolute VC estimates require measures of the vector-host ratio and how the vector-host ratio could change with climate, which are unknown for our locations. We therefore restrict our analysis to vector traits we have estimates for and calculate the expected number of infectious bites per a single mosquito, which we denote as the relative Vectorial Capacity: rVC. In the original derivation of VC, the per-capita mosquito mortality rate is assumed to be constant meaning the age when a mosquito is infected does not affect the results. Given the observed age-dependent mosquito mortality, the age at which a mosquito is infected can affect the number of infectious bites made per mosquito 38 . We therefore apply our temperature-dependent life history traits (EIP, age-dependent mosquito survival and biting rate) to estimate the expected number of infectious bites per infected mosquito, z , given it is infected at age t by adapting the methods developed by Iacovidou et al. 38 and conduct a sensitivity analysis for the age at which mosquitoes are infected (Supplementary Fig. 5 ). The sensitivity analysis indicates that adult mosquitoes infected at t 0 (i.e., at the youngest age upon emergence) generate the maximum number of infectious bites (Supplementary Fig. 5a ), so we give estimates of \({\mathbb{E}}\left({z|}{{{{{\rm{infected}}}}}}\; {{{{{\rm{at}}}}}}\; {{{{{\rm{age}}}}}}\,{t}_{0}\right)\) . This quantity does not account for vector density, but if density remains unchanged then the proportional difference in rVC estimates between EIP models and/or over time is equivalent to the proportional difference in absolute vectorial capacity within a given location. To propagate uncertainty in the EIP and biting rate estimates, we calculate rVC by sampling posterior parameters and estimating the EIP using our pooled model or the existing degree-day model using the recent and future mean temperatures for the four locations in Kenya. This approach enables us to compare the transmission consequences of our novel measures of EIP with the equivalent estimates from the degree-day model. Given that we did not measure biting rate in our current study, we estimated temperature-dependent changes in the posterior biting rates for another malaria vector, A. stephensi , using data presented in 24 (Supplementary Fig. 6 ) and sampled from these posteriors to calculate the rVC. Further methods and summary of the analysis are provided in Supplementary Fig. 7 and Supplementary Table 4 for determining survival distributions.
The predicted estimates for rVC using values of EIP derived from our model and the degree-day model for each of the locations in Kenya are presented in Table 1 (upper). Given there were differences in adult mosquito survival between Feed1 and Feed2, we present results for these experiments separately. Under recent climate conditions in Kericho, which is the coldest environment of the four locations, the rVC using our EIP model was 13 ( \(\frac{0.38}{0.03}\) ; see these rVC values in Table 1 upper) and 26 ( \(\frac{0.79}{0.03}\) ) times higher than that of the degree-day model for Feed1 and Feed2, respectively (Table 1 upper). Under warmer conditions the EIP models converge, so the differences in predictions of rVC become progressively smaller from Kitale to Kisumu, regardless of feed. As temperatures increase further, our EIP estimates are shorter than the degree-day model, so for the warmest location, Garissa, the predicted rVC was 0.92 for Feed1 and 0.95 for Feed2 of the equivalent values derived using the degree-day model. For the future climates, the rVC predictions were qualitatively similar, but because temperatures are shifted right along the EIP curve, the differences between models in Kericho were smaller (rVC based on our EIP model was now just 1.35 and 1.26 times higher than the degree-day model for Feed1 and Feed2, respectively) and slightly larger in Garissa (rVC values using our EIP model were 0.82 that of the degree-day model for Feed1 and 0.88 for Feed2) (Table 1 upper, Supplementary Fig. 5a ).
To explore the overall implications for climate change, we examine the relative difference in rVC using the ratio of rVC in recent and future conditions (Table 1 bottom). Differences between the ratio of future:recent rVC estimated using our EIP distributions and the degree-day model were consistently predicted irrespective of age of mosquito infection (Supplementary Fig. 5b ). With the degree-day model, we estimate transmission intensity to increase 43.1 (40.8–49.6 95% CrI) (Feed1) and 79.0 (75.2–88.8 95% CrI) (Feed2) times in Kericho due to climate warming. Using our model of EIP, the increase in transmission is only 3.93 (3.12–5.34 95% CrI) (Feed1) and 3.63 (2.88–5.96 95% CrI) (Feed2) times. These results indicate that the degree-day model over-estimates increase in future transmission potential by 11 (Feed1: \(\frac{43.1}{3.93}\) ) to 22 times (Feed2: \(\frac{79.0}{3.63}\) ) relative to our EIP model. For the other locations, there is much better agreement between EIP models (Table 1 bottom).
For over 60 years, the development time of the most deadly human malaria-causing parasite, P. falciparum , in anopheline mosquitoes has been approximated using a single fit of a degree-day model 13 . In this study, we generated sporogony data for P. falciparum in a dominant African vector species, A. gambiae . These data were then analysed using a mechanistic model of sporogony to fully characterise the kinetics of parasite development across a range of constant temperatures. Our EIP 50 estimates align well with the established degree-day model EIP estimates for constant temperatures between approximately 24 to 28 °C (Fig. 2 ). Outside this temperature range, the degree-day model estimates are not within the 95% CrIs of EIP 50 ; at 17 °C, for example, while the uncertainty of the pooled model was relatively large because the low infection rates resulted in poor model fitting, we estimated EIP 50 as 59 days with a 95% CrI of 53-68 days, whereas the degree-day model predicts 111 days. The reasons for the marked differences between our EIP estimates and those of the degree-day model at cooler temperatures are unclear. The initial study of Nikolaev 23 that was used to fit the degree-day model did not measure EIP below 19-20 °C so it could be a true difference between the experimental systems, a consequence of the degree-day model functional form, or because the model was fitted to limited data. Studies in other systems suggest non-linear effects of temperature under cool conditions which could violate the linear growth rate assumption of the degree-day model 39 , 40 , and also contribute to the relatively poor fit of our mechanistic model at the thermal limits. There could also be differences between mosquito species, or even populations. Preliminary studies suggest there can be differences in thermal performance of vector competence and EIP between species of malaria vector infected with the same parasite strain 25 , 41 . Our data suggest sporogony can be completed more rapidly in A. gambiae at cooler temperatures than predicted by the Detinova degree-day model but other data (albeit limited) on An. elutus , suggest longer EIP than the Detinova model at warmer temperatures 42 . Yet the prevailing assumption within mathematical models has been that the EIP is a property of the parasite species and temperature alone 7 . Future studies on other key malaria vectors are therefore important to further determine the role of vector species.
Consistent with other recent studies 24 , 25 , our research highlights variation in the EIP among mosquitoes exposed to the same environment. The mechanisms that generate this variation are unclear but could include intraspecific variation of individual mosquito characteristics such as body size 43 , nutritional condition 44 , or density-dependent effects among individual parasites 45 , 46 , 47 . This biological variation challenges a classic assumption of mechanistic malaria transmission models that typically assume all mosquitoes become infectious at the same time point following an infectious blood meal 37 , and highlights the methodological requirement to sample sufficient mosquitoes over time to enable estimation of the mean or median EIP (EIP 50 ).
In addition, our study provides insights into the temperature-dependence of the HMTP as measured by the presence of oocysts and sporozoites (vector competence). The maximum prevalence of oocysts and the mean number of oocysts per mosquito both had a unimodal relationship with temperature, with much less parasite establishment at thermal extremes. These findings support other experimental infection studies suggesting the earlier stages of parasite development are most temperature sensitive 27 , 28 , 48 . Our vector competence estimates also demonstrate a unimodal relationship, with an optimum of 24.8 °C. The asymmetric nature of this relationship appears to result in part from a strong effect of higher temperatures on the probability that an infected mosquito will develop sporozoites. To our knowledge, this is the first parameterisation of conversion efficiency of oocyst to sporozoite-infection across different constant temperatures. The conversion efficiency is 100% at most temperatures, meaning oocyst prevalence is a good measure of the prevalence of infectious mosquitoes. Many studies assume that infection prevalence at the oocyst stage (percent infected) can be used as a proxy for infection prevalence at the sporozoite stage (percent infectious) 27 , 49 , 50 , 51 . Our data suggest this relationship is not robust at higher or possibly lower temperatures and could be an important factor determining the edges of the thermal range for transmission. At high temperatures, for example, our laboratory strains showed a precipitous decline of this conversion efficiency to 14% between 28 and 30 °C (Fig. 3c ), meaning at these temperatures the presence of oocysts might not be an accurate measure of infectivity. Accurately quantifying sporozoite load is challenging, so we measured sporozoite prevalence, meaning we could only model this conversion efficiency at the mosquito scale. Understanding conversion probability and the mechanisms involved at the parasite scale will require further experiments and model fitting, ideally with field-derived sympatric parasite and mosquito strains.
Finally, there is limited research on the effects of temperature on A. gambiae survival, particularly towards the lower thermal limits of transmission, or including the influence of infectious blood meals 52 . Our data show that across the temperature range studied, survival increases as temperatures decrease, though at even cooler temperatures it is likely that survival will decrease 9 , 53 . Given the controlled insectary conditions, the differences in survival between Feed 1 & 2 were surprising and could suggest possible interactions between temperature and blood meal quality (different batches of blood, human serum etc.) or parasite load within the blood. Regardless, survival was best characterised using Gompertz functions, consistent with age-dependent mortality. An increasing number of studies highlight the importance of considering age-dependent mortality 38 , 54 , 55 , 56 , 57 , 58 , although most transmission models assume that mortality remains constant with age. The extent of age-dependent mortality in the field where mosquitoes are subject to greater causes of daily mortality (e.g. predation, swatting during blood feeding, stress associated with searching for oviposition sights, control tools) remains an important question 58 , 59 .
Incorporating our data into a transmission metric that captures variability in the EIP and age-dependence of mosquito mortality reveals important implications for understanding current and future transmission intensity. Across the four locations in Kenya, rVC is low in Kericho, which approaches the observed lower thermal limit for transmission, increases through Kitale and Kisumu which are at intermediate temperatures, and then declines slightly in Garissa, which is towards the upper limits for transmission. This pattern is consistent with a previously identified unimodal thermal performance curve for transmission 9 , 60 . However, our shorter EIPs result in higher rVC than predicted using estimates of EIP from the established degree-day model under cooler conditions. This result suggests that all else being equal, models of transmission that use the standard predictions of EIP are likely to underestimate transmission intensity in low temperature environments where differences between EIP models are greatest. At the other extreme, because there is a cross-over in the EIP models, our results suggest that conventional models will tend to marginally overestimate transmission at high temperatures. The unimodal relationship between temperature and transmission is present using either model of EIP, which means that the effects of climate change are predicted to be ‘two-tailed’ 41 , 61 , 62 , with transmission potential increasing in cooler environments, remaining largely unchanged at intermediate temperatures, and declining in warm environments. However, the predicted relative increase in transmission in Kericho was 11-22 times greater when using the degree-day estimates of EIP than predicted by our new estimates of EIP. Numerous studies predict variation in the dynamics and distribution of malaria due to climate warming, with increases in transmission of particular concern for colder environments such as the Kenyan Highlands 22 , 30 , 63 , 64 , 65 , 66 , 67 . Our results suggest that current predictions of the impact of climate change likely overestimate the increase in transmission risk in cooler areas because the Detinova degree-day model predicts both slower rates of parasite development under current conditions, and a greater rate of change in response to future warming, relative to our model. The quantitative effect could vary depending on other factors such as the exact pattern of mosquito mortality in the field or changes in vector density, but because our temperature-dependent EIP model is flatter than the degree-day model at the cooler end of the scale, we expect the overestimation to be qualitatively robust. Given the potential public health significance, these insights highlight the need to understand the effects of temperature at the fringes of transmission.
As in the original empirical work used to parameterise the Detinova degree-day model, our study manipulated temperatures experienced by the adult mosquitoes as these are known to affect parasite development rate directly. Yet, larval rearing conditions can have indirect transstadial effects that can further influence vector competence 68 , 69 , 70 and parasite development 44 . Thus, it could be valuable to extend research to include variation in larval conditions, such as temperature and resource availability, as well as variation in adult conditions. It should be noted, however, that temperatures in larval habitats need not be the same as those experienced by the adults 71 and adult mosquitoes in a single feeding and resting location could in principle derive from diverse larval habitats, adding complexity to both experimental design and interpretation.
A further potential limitation of our study is that we considered only one strain of A. gambiae and one strain of P. falciparum , both of which have been maintained under controlled lab settings for many generations. However, there could be intrinsic differences between strains 35 , or differences as a result of adaptation to local environments; at present, there is limited research on the extent of thermal adaption in mosquitoes or parasites, but in principle this could shape the influence of temperature on local sympatric mosquito and parasite pairings 72 . Other factors could also be important, such as the influence of multiple blood meals on EIP 73 , 74 , the effects of daily temperature fluctuation in addition to mean temperature 25 , 28 , 71 , and possible effects of parasite load on infection kinetics in the mosquito 33 (but see 35 , which showed no effect). Nonetheless, our highly resolved sporogony data, together with data on the temperature-dependence of vector competence and mosquito survival, provide new insights that challenge current understanding. Our findings highlight the importance of better characterising the thermal dependence of mosquito and parasite traits.
Anopheles gambiae (G3 strain) mosquitoes were used throughout the experiments. Mosquitoes for all developmental stages were reared under standard insectary conditions at 27 °C ± 0.5 °C, 80% ± 5% relative humidity, and a 12 h:12 h light-dark photoperiod. Larval density and amount of larval food (ground TetraFin TM ; Tetra, Blacksburg, VA) were standardised to ensure uniform adult size. Adult mosquitoes were maintained on 10% glucose solution supplemented with 0.05% para-aminobenzoic acid (PABA). For the infectious feeds, 5-6-day-old female mosquitoes were randomly aspirated into cardboard cup containers covered with netting and starved for approximately 6 h before the infectious feed. Individual containers contained 150-200 mosquitoes. All experiments were conducted under Penn State Institutional Biosafety Committee protocol # 48219, which covers protocols for handling mosquitoes during rearing, blood feeding and infection. We used blood from a commercial biospecimen supplier (BioIVT, corp) that derived from de-identified human donors and was not collected specifically for our study.
Mosquito transmission and survival studies
In vitro cultured Plasmodium falciparum (NF54 isolate, MR4) was provided by the Parasitology Core Lab ( http://www.parasitecore.org/ ) at Johns Hopkins University as described previously 28 . In brief, gametocyte culture in stage four to five (day 14 after gametocyte initiation) was transported to Penn State overnight, and gametocyte-infected erythrocytes were maintained > 24 h before the infectious feed to allow additional maturation of gametocytes.
Mosquitoes were fed on day 16 post gametocyte initiation. The proportion of erythrocytes infected with mature gametocytes (i.e., gametocytemia) generally ranged between 1–3% in the culture. An infectious blood meal was prepared by mixing gametocyte-infected erythrocytes with fresh human serum and erythrocytes at 40% haematocrit on the day of blood feeding as previously described 28 .
All infectious feeds were conducted in a walk-in environment-controlled chamber. Glass bell jars were uniformly covered with Parafilm to serve as membrane feeders and heated to 37 °C with continuously circulating water as described in 44 . Containers of mosquitoes were randomly allocated to bell jars to minimise any effect of position or feeder. Mosquitoes were fed for 20 min at 27 °C after acclimating at 27 °C for an hour, and > 95% mosquitoes were fully engorged in all infectious feeds. Immediately after blood feeding, mosquitoes were placed into incubators (Percival Scientific Inc., Perry, Iowa) with appropriate temperature treatment conditions (90% ± 5% relative humidity, and 12 h:12 h light-dark photoperiod) and provided daily with fresh 10% glucose solution supplemented with 0.05% PABA.
Approximately 300 to 1200 mosquitoes in two-to-six containers (150 to 200 in each container) were fed infectious blood meals depending on temperature treatment in three independent experiments. In each experiment, seven to eight temperatures were selected between 17 °C and 30 °C as these temperatures span the temperature range for P. falciparum infection to be observed in previous studies 25 , 28 . Two infectious feed experiments (Feed1 & Feed2) were conducted after an initial pilot trial. In the pilot study, gametocytemia of parasite-infected blood meal was 0.024% and blood-fed mosquitoes were kept at constant temperatures of 17, 19, 21, 23, 25, 27, and 29 °C throughout. This pilot generated little or no infections at lower temperatures. In the main two infectious feeds (i.e., Feed1 and Feed2) gametocytemia and mosquito sample size were increased. In Feed1, gametocytemia of blood meals was adjusted to 0.126% and blood-fed mosquitoes were kept at constant temperatures of 17, 19, 21, 23, 25, 27, and 29 °C throughout. In Feed2, gametocytemia was adjusted to 0.139% and blood-fed mosquitoes were kept at constant temperatures of either 18, 19, 20, 23, 25, 28, 29, or 30 °C to further increase the sample size of infected mosquitoes for low and high temperatures. The air temperature of the incubators (Percival Scientific Inc., Perry, Iowa) was monitored closely using HOBO data loggers (Onset Computer Corporation, Bourne, MA; error range = ± 0.1 °C) at 15 min intervals, and the accuracy of temperature was maintained at ±0.2 °C. Data loggers and incubators were rotated throughout all infectious feed experiments to minimise potential equipment effects. The number of mosquitoes and containers varied by experiments as presented in Supplementary Table 5 . In general, sample size of mosquito dissections and number of containers were increased for low and high temperatures to ensure sufficient sporozoite infections to estimate the EIP distribution.
To determine parasite infections, mosquitoes were randomly collected by aspirating into 95% ethanol, and midguts and salivary glands were dissected in 1× phosphate-buffered saline solution under a standard dissecting scope as previously 25 . Presence or absence of parasite infection was determined by examining midguts for oocysts or salivary glands for sporozoites using a compound microscope. Visible oocysts in midguts were counted. To ensure correct scoring, oocysts and sporozoites were inspected under 40× magnification and cross-checked by a second person. Oocyst or sporozoite prevalence was calculated as the total number of infected mosquitoes divided by the total number of dissected mosquitoes by combining dissection data from replicated containers of mosquitoes for each temperature treatment. Oocyst intensity and prevalence was determined on the days when maximum infection is expected based on previous studies 25 . To determine the EIP, ~10–60 salivary glands of mosquitoes were dissected daily (10–15 per container). Dissection days were determined to capture the first sporozoite infection followed by increase in prevalence to maximum within each temperature treatment. Detailed sample size and dissection days are reported in Supplementary Table 5 .
To obtain survival data, dead mosquitoes were scored daily in Feed1 and Feed2 experiment until all mosquitoes were dead. The mosquito containers were rotated daily within each incubator throughout the experiment to minimise potential effect of container position on survival.
Estimating the Extrinsic Incubation Period and human-to-mosquito transmission probability
Mechanistic model of sporogony.
Visualising the developmental stage of Plasmodium parasites typically requires mosquito dissection (but see ref. 75 for alternative experimental methods to determine sporozoite infection). The EIP of individual mosquitoes cannot be estimated from mosquito dissection data because dissection kills the mosquito. Consequently, the EIP is typically estimated among the mosquito and parasite populations as a whole using a statistical or mechanistic model 33 . In previous work, we demonstrated how the cumulative increase in sporozoite prevalence in standard membrane feeding assays with time, n , can, however, be used to estimate the CDF (F( n )) of EIPs among individual mosquitoes using a mechanistic model that simulates the development times of individual parasites within the mosquito 33 . To estimate the EIP, we modified and fitted this model 33 . The model consists of five key parameters: the HMTP (as determined by the presence of oocysts; δ O ), the parasite load (as determined by the number of oocysts per mosquito) distribution, which is described by the mean ( μ ) and overdispersion ( k ) parameters of a zero-truncated negative binomial distribution, and the parasite development time parameters (shape, α , and rate, β , of the gamma distribution). These development time parameters are constituted by the sum of two independent development times from inoculation ( G ) to first oocyst appearance ( O ) and from O to salivary gland sporozoites ( S ). This model allows the estimation of two quantities related to parasite development times: (1) the distribution of individual parasite development times from inoculation to sporozoite (parasite numbers are equivalent to the number of oocysts) and (2) the distribution of development times for individual mosquitoes to have completed sporogony (EIP distribution). According to this model the CDF of the EIP distribution, n , is calculated as,
where \({{{{{\rm{Q}}}}}}\left(\alpha,0,\beta n\right)=\frac{\Gamma \left(\alpha,0,\beta n\right)}{\Gamma \left({{{{{\rm{\alpha }}}}}}\right)}\) (the regularised gamma function), \(\Gamma \left({{{{{\rm{\alpha }}}}}}\right)={\int }_{0}^{\infty }{t}^{\alpha -1}{e}^{-t}{dt}\) (the complete gamma function) and \(\Gamma \left(\alpha,0,\beta n\right)={\int }_{0}^{\beta n}{t}^{\alpha -1}{e}^{-t}{dt}\) (the generalised incomplete gamma function). The modelled sporozoite prevalence at time, n , therefore depends on the proportion of infected mosquitoes that are infectious (F( n )) and the proportion of mosquitoes that are infected. P. falciparum infection of A. gambiae is unlikely to increase mosquito mortality because the parasite is highly adapted to this sympatric mosquito vector 41 , 76 , and examination of our raw data provided no evidence of a decline in sporozoite prevalence observed over time. We therefore assumed the relative survival of infected to uninfected mosquito parameter, which fitted in 33 , was equal to 1. To facilitate model fitting, an additional parameter that estimates the probability a mosquito infected with oocysts will develop any observable sporozoites ( δ S ) was incorporated at the mosquito scale to account for complete clearance of oocyst infection or complete lack of oocyst bursting, which was observed for certain temperature treatments. It would be preferable to account for this observation at the parasite scale: specifically, the loss of individual oocysts would be modelled, however, sporozoite load data was unavailable meaning it was not possible to parameterise this model.
We used a binomial likelihood to fit the probability of observing a mosquito with salivary gland sporozoites such that,
where I n is the number of infectious mosquitoes at days post infection n and D is the number of dissected mosquitoes at time n . We calculated the likelihood of observing a mosquito dissected with Y oocysts at time n as,
where Γ GO is the CDF of the individual parasite development times from G to O . The appearance of early oocysts was not available for these data as mosquitoes were all dissected when oocysts were late stage. This means that the distribution of transition times between G and O (and its dependence on temperature) was unidentifiable. We therefore specified informative priors on α GO and β GO such that the modelled oocyst prevalence peaked prior to the days post infection mosquitoes were dissected for oocysts. The mean prior values for α GO and β GO were determined by the corresponding values obtained from previously published model fits 1 .
Modelling the effects of temperature on sporogony
To identify suitable functional forms for the relationship between model parameters and temperature, first, we fit the model to data from mosquitoes exposed to each constant temperature independently. The independent model parameter estimates were then used to guide functional relationships between temperature and the model parameter values, which were estimated by fitting the model and temperature-dependent functions to all data (pooled model). All model fits were checked visually. To incorporate differences in development time from G to S , we allowed α OS and β OS to vary with temperature. In doing so, the model aims to estimate the complete development time from G to S , but not the constituent times from G to O and O to S . We fitted the model with the following functional relationships between the model parameters and temperature ( T ):
We fitted both the pooled and independent models to experimentally infected A. gambiae mosquitoes that were dissected for oocysts number or salivary gland sporozoites on different days post infection in a Bayesian framework using the No U-turn Markov-chain Monte Carlo (MCMC) sampler 77 , with four chains each with 5500 iterations (inclusive of 3000 iterations warmup). Convergence is determined by \(\hat{R} < 1.01\) for all parameters. Temperature was scaled so that the mean temperature was 0 and its standard deviation was 1. To account for uncertainty in the parameter estimates for all quantities, we calculate the estimates for each MCMC sample and give the median and 95% credible intervals (CrI) of these values. Independent and pooled model priors are given in Supplementary Table 6 .
Quantifying the EIP distribution
Differentiating F( n ) we obtain the EIP probability distribution function (PDF; f( n )):
The time for different percentiles, q , of the mosquito population to have completed the EIP (denoted by EIP q ) is given by the inverse CDF ( \({{{{{{\rm{F}}}}}}}^{-1}\left(t\right)\) ), which is calculated thus:
where QI is the inverse of the regularised gamma function.
The mean and variance of the EIP distributions were estimated at each temperature by numerical integration using the Cuhre algorithm (R calculus package 78 ): \({\mathbb{E}}\left[n\right]{{{{{\boldsymbol{=}}}}}}{\int }_{0}^{\infty }n{{{{{\rm{f}}}}}}\left(n\right)\,{dn}\) and \({Var}\left(n\right)={\mathbb{E}}\left[{n}^{2}\right]{{{{{\boldsymbol{-}}}}}}{\left({\mathbb{E}}\left[n\right]\right)}^{2}\) respectively.
Determining survival distribution of A. gambiae mosquitoes fed with parasite-infected blood meals
To characterise the mosquito survival distribution, the cumulative survival was fitted with four survival models: Gompertz, Weibull, loglogistic and negative exponential. These models have been used to describe survival curves of a diversity of insects in laboratory and field settings 56 , 79 , 80 , 81 , 82 , 83 , 84 . The corrected Akaike Information Criterion (AICc) value for these models were compared, indicating the Gompertz model is the best fit for all temperature treatments in Feed1 and Feed2. The equation used for Gompertz model was,
where S g (x) is cumulative proportion surviving at age x , a is the initial mortality rate, and b is the age-dependent mortality rate 84 , 85 , 86 .
Estimating the effects of EIP on expected number of infectious bites per infected mosquito under recent and future temperature conditions
To estimate the effects of the different EIP estimates on the relative vectorial capacity, we adapted the methods developed by Iacovidou et al. 38 to calculate the expected number of infectious bites per infected mosquito, z , given the mosquito age at infection and a Gompertz survival model. In all cases, n is the EIP; t 0 , t 1 and t 2 are the mosquito ages at infection, at completion of the EIP and when death occurs, respectively; a and b are the Gompertz survival model parameters; B is the per-mosquito biting rate per day; j is the number of bites. In the original methods the probability a mosquito survives the EIP given it is infected at age t 0 is calculated thus,
The probability a mosquito bites z times given it completes the EIP at age t 1 is then calculated,
These equations are combined to estimate the probability a mosquito bites z times given it is infected at age t 0 ,
And the expectation of \({\mathbb{P}}\left(z={j|}{{{{{\rm{infected}}}}}}\; {{{{{\rm{at}}}}}}\; {{{{{\rm{age}}}}}}\,{t}_{0}\right)\) is calculated thus,
We modified this model to calculate the equivalent quantity for the degree-day model, which assumes all mosquitoes have the same EIP:
\({\mathbb{E}}\left({z|}{{{{{\rm{infected}}}}}}\; {{{{{\rm{at}}}}}}\; {{{{{\rm{age}}}}}}\,{t}_{0}\right)\) is an estimate of the lifetime number of infectious bites per mosquito (what we call rVC). To quantify temperature-dependent changes in transmission risk, we calculate how this quantity changes given the temperature-dependent changes in the constituent parameters estimated in the laboratory. We do not have measures of vector-host densities but assume the vector-host ratios and per-mosquito biting rates remain the same within a location. The predicted future mean temperature for one location, Garissa (30.7 °C), is marginally above our experimental range, so predicted future mean temperature for this location we used the upper temperature limit for which we have empirical data (30 °C). Analytical solutions in the integrands in Eqs. 9, 10, 11 and 15 were not available, so these were integrated numerically using the Cuhre method with an absolute tolerance of 10 −7 (calculus R package 78 ).
Since the temperatures we used in our studies do not align exactly with the estimates of temperature in the field settings, to parameterise mosquito survival when calculating the \({\mathbb{E}}\left({z|}{{{{{\rm{infected}}}}}}\; {{{{{\rm{at}}}}}}\; {{{{{\rm{age}}}}}}\,{t}_{0}\right)\) , we fit temperature-dependent functions to the survival model parameter estimates, which we fit to data from each temperature independently. As mosquito survival differed between experiments, we estimate transmission potential separately for Feed1 and Feed2. To estimate the number of mosquitoes alive, we interpolate the Gompertz survival distributions to estimate daily cumulative survival rate at different temperatures. Values of parameter a (initial mortality) of the Gompertz functions were fitted to a quadratic function,
where \({G}_{q}\) ( T ) is parameter a of Gompertz function at a given temperature, T . Values of parameter b (age-dependent mortality rate) fitted to a linear function,
where \({G}_{l}\) ( T ) is parameter b of Gompertz function at a given temperature, T . From these modelled quadratic and linear functions, Gompertz survival distributions were estimated for each temperature in the four Kenyan locations under current and future climate change scenarios.
For daily biting rate, we used individual mosquito gonotrophic cycle length data from a previously published study conducted on A. stephensi 24 . The temperature-dependence of the biting rate was estimated by fitting a Brière function,
to these data, where B (T) is biting rate at given temperature, T, T 0 and T m are the lower and upper threshold temperatures of biting rate, respectively. To do so, we assumed complete gonotrophic concordance and calculated the predicted gonotrophic cycle length as the inverse of the predicted per mosquito biting rate. We fitted the Brière function parameters in a Bayesian framework, assuming that the gonotrophic cycle lengths were geometrically distributed with the mean 1/B( T ), using the Stan MCMC sampler (four chains each with 2500 iterations inclusive of 1250 warmup iterations; convergence was determined by \(\hat{R}\, < \,1.01\) for all parameters and by visually checking the trace plots). For the biting rate parameters, we assume weakly informative normal priors with the means equivalent to the values estimated by Mordecai et al. 9 ( c ~ normal (0.000203, 0.01), T 0 ~ normal (11.7, 4.5) and T m ~ normal (42.3, 4.5)). The Brière function forces the biting rate to decline at temperature extremes, which is uncertain as the empirical data show no marked decline over the temperature range used. We note that A. stephensi is a different species, but this earlier research was conducted in the same insectary and rearing conditions as the current study, and biting rate is applied equivalently to the different models in any case.
Statistical analysis
Mosquito survival was analysed using Kaplan–Meier Log Rank test to determine median survival and the effect of temperature on the survival (JMP 8.0, SAS). The mosquitoes collected for dissection were censored in the survival analysis. Least square estimation was used (Prism 8.02, GraphPad) for determining best fit survival function on survival data from Feed1 and Feed2 and for modelling temperature dependency of parameter values of the Gompertz survival functions.
Reporting summary
Further information on research design is available in the Nature Portfolio Reporting Summary linked to this article.
Data availability
All relevant data are available from the manuscript or https://github.com/IsaacStopard/mSOS_gambiae_only ( https://doi.org/10.5281/zenodo.10688356 ). Source data are provided with this paper.
Code availability
Code for the modelling study is available from https://github.com/IsaacStopard/mSOS_gambiae_only ( https://doi.org/10.5281/zenodo.10688356 ).
Organization, W. H. World Malaria Report 2022 (World Health Organization, Geneva, Switzerland, 2022).
Garrett-Jones, C. & Shidrawi, G. R. Malaria vectorial capacity of a population of Anopheles gambiae : an exercise in epidemiological entomology. Bull. World Health Organ. 40 , 531–545 (1969).
CAS PubMed PubMed Central Google Scholar
Macdonald, G. The Epidemiology and Control of Malaria (Oxford University Press, 1957).
Ross, R. Some quantitative studies in epidemiology. Nature 87 , 466–467 (1911).
Article ADS Google Scholar
Beier, J. C. Malaria parasite development in mosquitoes. Annu. Rev. Entomol. 43 , 519–543 (1998).
Article CAS PubMed Google Scholar
Baton, L. A. & Ranford-Cartwright, L. C. Spreading the seeds of million-murdering death: metamorphoses of malaria in the mosquito. Trends Parasitol. 21 , 573–580 (2005).
Article PubMed Google Scholar
Ohm, J. R. et al. Rethinking the extrinsic incubation period of malaria parasites. Parasit Vectors 11 , 178 (2018).
Article PubMed PubMed Central Google Scholar
Tesla, B. et al. Temperature drives Zika virus transmission: evidence from empirical and mathematical models. Proc. Biol. Sci. https://doi.org/10.1098/rspb.2018.0795 (2018).
Mordecai, E. A. et al. Optimal temperature for malaria transmission is dramatically lower than previously predicted. Ecol. Lett. 16 , 22–30 (2013).
Mordecai, E. A. et al. Thermal biology of mosquito-borne disease. Ecol. Lett. 22 , 1690–1708 (2019).
Mordecai, E. A. et al. Detecting the impact of temperature on transmission of Zika, dengue, and chikungunya using mechanistic models. PLoS Negl. Trop. Dis. 11 , e0005568 (2017).
Shocket, M. S. et al. Transmission of West Nile and five other temperate mosquito-borne viruses peaks at temperatures between 23 °C and 26 °C. Elife https://doi.org/10.7554/eLife.58511 (2020).
Detinova, T. Age-grouping Methods In Diptera Of Medical Importance, With Special Reference To Some Vectors Of Malaria . Vol. 47 (World Health Organization, 1962).
Yamana, T., Bomblies, A. & Eltahir, E. Climate change unlikely to increase malaria burden in West Africa. Nat. Clim. Change 6 , 1009-1013 (2016).
Gething, P. W. et al. Modelling the global constraints of temperature on transmission of Plasmodium falciparum and P. vivax. Parasit. Vectors 4 , 92 (2011).
Craig, M. H., Snow, R. W. & le Sueur, D. A climate-based distribution model of malaria transmission in sub-Saharan Africa. Parasitol. Today 15 , 105–111 (1999).
Hoshen, M. B. & Morse, A. P. A weather-driven model of malaria transmission. Malar J. 3 , 32 (2004).
Ermert, V., Fink, A. H., Jones, A. E. & Morse, A. P. Development of a new version of the Liverpool Malaria Model. II. Calibration and validation for West Africa. Malar J. 10 , 62 (2011).
Tompkins, A. M. & Ermert, V. A regional-scale, high resolution dynamical malaria model that accounts for population density, climate and surface hydrology. Malar J. 12 , 65 (2013).
Garske, T., Ferguson, N. M. & Ghani, A. C. Estimating air temperature and its influence on malaria transmission across Africa. PLoS ONE 8 , e56487 (2013).
Article ADS CAS PubMed PubMed Central Google Scholar
Weiss, D. J. et al. Air temperature suitability for Plasmodium falciparum malaria transmission in Africa 2000-2012: a high-resolution spatiotemporal prediction. Malar J. 13 , 171 (2014).
Caminade, C. et al. Impact of climate change on global malaria distribution. Proc Natl Acad Sci USA 111 , 3286–3291 (2014).
Nikolaev, B. P. [On the influence of temperature on the development of malaria plasmodia in the mosquito]. Trans. Pasteur Inst. Epi. Bact. Leningrad 2 , 1–5 (1935).
Shapiro, L. L. M., Whitehead, S. A. & Thomas, M. B. Quantifying the effects of temperature on mosquito and parasite traits that determine the transmission potential of human malaria. PLoS Biol. 15 , e2003489 (2017).
Waite, J. L., Suh, E., Lynch, P. A. & Thomas, M. B. Exploring the lower thermal limits for development of the human malaria parasite, Plasmodium falciparum. Biol. Lett. 15 , 20190275 (2019).
Vaughan, J. A. Population dynamics of Plasmodium sporogony. Trends Parasitol. 23 , 63–70 (2007).
Noden, B. H., Kent, M. D. & Beier, J. C. The impact of variations in temperature on early Plasmodium falciparum development in Anopheles stephensi. Parasitology 111 , 539–545 (1995).
Suh, E. et al. The influence of feeding behaviour and temperature on the capacity of mosquitoes to transmit malaria. Nat. Ecol. Evol. 4 , 940–951 (2020).
Parham, P. E. & Michael, E. Modelling climate change and malaria transmission. Adv. Exp. Med. Biol. 673 , 184–199 (2010).
Mordecai, E. A., Ryan, S. J., Caldwell, J. M., Shah, M. M. & LaBeaud, A. D. Climate change could shift disease burden from malaria to arboviruses in Africa. Lancet Planet Health 4 , e416–e423 (2020).
Villena, O. C., Ryan, S. J., Murdock, C. C. & Johnson, L. R. Temperature impacts the environmental suitability for malaria transmission by Anopheles gambiae and Anopheles stephensi. Ecology 103 , e3685 (2022).
Siria, D. J. et al. Rapid age-grading and species identification of natural mosquitoes for malaria surveillance. Nat. Commun. 13 , 1501 (2022).
Stopard, I. J., Churcher, T. S. & Lambert, B. Estimating the extrinsic incubation period of malaria using a mechanistic model of sporogony. PLoS Comput. Biol. 17 , e1008658 (2021).
Stopard, I. J. The Importance Of The Extrinsic Incubation Period For Malaria Transmission, Surveillance And Control. Ph.D. thesis (Imperial College London, 2023).
Guissou, E. et al. Intervention reducing malaria parasite load in vector mosquitoes: No impact on Plasmodium falciparum extrinsic incubation period and the survival of Anopheles gambiae. PloS Pathogens https://doi.org/10.1371/journal.ppat.1011084 (2023).
Paaijmans, K. P. et al. Downscaling reveals diverse effects of anthropogenic climate warming on the potential for local environments to support malaria transmission. Climate Change . 125 , 479–488 (2014).
Smith, D. L. et al. Ross, macdonald, and a theory for the dynamics and control of mosquito-transmitted pathogens. PLoS Pathog. 8 , e1002588 (2012).
Article CAS PubMed PubMed Central Google Scholar
Iacovidou, M. A. et al. Omitting age-dependent mosquito mortality in malaria models underestimates the effectiveness of insecticide-treated nets. PLoS Comput. Biol. 18 , e1009540 (2022).
Stinner, R. E., Gutierrez, A. P. & Butler, J., G. D. An algorithm for temperature-dependent growth ratee simulation Can. Entomol. 106 , 519–524 (1974).
Zhou, G. & Wang, Q. A new nonlinear method for calculating growing degree days. Sci. Rep. 8 , 10149 (2018).
Article ADS PubMed PubMed Central Google Scholar
Murdock, C. C., Sternberg, E. D. & Thomas, M. B. Malaria transmission potential could be reduced with current and future climate change. Sci. Rep. 6 , 27771 (2016).
Kligler, I. & Mer, G. Studies on the effect of various factors on the Infection rate of Anopheles elutus with different species of Plasmodium . Ann. Trop. Med. Parasitol. 31 , 71 (1937).
Article Google Scholar
Lyimo, E. O. & Koella, J. C. Relationship between body size of adult Anopheles gambiae s.l. and infection with the malaria parasite Plasmodium falciparum. Parasitology 104 , 233–237 (1992).
Shapiro, L. L., Murdock, C. C., Jacobs, G. R., Thomas, R. J. & Thomas, M. B. Larval food quantity affects the capacity of adult mosquitoes to transmit human malaria. Proc. Biol. Sci. https://doi.org/10.1098/rspb.2016.0298 (2016).
Mendes, A. M. et al. Infection intensity-dependent responses of Anopheles gambiae to the African malaria parasite Plasmodium falciparum. Infect. Immun. 79 , 4708–4715 (2011).
Sinden, R. E. et al. Progression of Plasmodium berghei through Anopheles stephensi is density-dependent. PLoS Pathog. 3 , e195 (2007).
Vaughan, J. A., Noden, B. H. & Beier, J. C. Population dynamics of Plasmodium falciparum sporogony in laboratory-infected Anopheles gambiae. J. Parasitol. 78 , 716–724 (1992).
Okech, B. A. et al. Resistance of early midgut stages of natural Plasmodium falciparum parasites to high temperatures in experimentally infected Anopheles gambiae (Diptera: Culicidae). J. Parasitol. 90 , 764–768 (2004).
Birkholtz, L. M., Alano, P. & Leroy, D. Transmission-blocking drugs for malaria elimination. Trends Parasitol. 38 , 390–403 (2022).
Stone, W. J. et al. The relevance and applicability of oocyst prevalence as a read-out for mosquito feeding assays. Sci. Rep. 3 , 3418 (2013).
Eling, W., Hooghof, J., van de Vegte-Bolmer, M., Sauerwein, R. V., & Gemert, G. J. Tropical temperatures can inhibit development of the human malaria parasite Plasmodium falciparum in the mosquito. Proc. sect. Exp. Appl. Entomol. Neth. Entomol. Soc. 12 , 151–156 (2001).
Google Scholar
Lunde, T. M., Bayoh, M. N. & Lindtjørn, B. How malaria models relate temperature to malaria transmission. Parasit. Vectors 6 , 20 (2013).
Bayoh, M. N. Studies On The Development And Survival Of Anopheles Gambiae Sensu Stricto At Various Temperatures And Relative Humidities (PhD thesis) Doctor of Philosophy thesis, (University of Durham, 2001).
Styer, L. M., Carey, J. R., Wang, J. L. & Scott, T. W. Mosquitoes do senesce: departure from the paradigm of constant mortality. Am. J. Trop. Med. Hyg. 76 , 111–117 (2007).
Dawes, E. J., Churcher, T. S., Zhuang, S., Sinden, R. E. & Basáñez, M. G. Anopheles mortality is both age- and Plasmodium-density dependent: implications for malaria transmission. Malar J. 8 , 228 (2009).
Bellan, S. E. The importance of age dependent mortality and the extrinsic incubation period in models of mosquito-borne disease transmission and control. PLoS ONE 5 , e10165 (2010).
Novoseltsev, V. N. et al. An age-structured extension to the vectorial capacity model. PLoS ONE 7 , e39479 (2012).
Ryan, S., Ben-Horin, T. & Johnson, L. Malaria control and senescence: the importance of accounting for the pace and shape of aging in wild mosquitoes. Ecosphere 6 , 1–13 (2015).
ADS CAS Google Scholar
Lambert, B., North, A. & Godfray, H. C. J. A Meta-analysis of longevity estimates of mosquito vectors of disease. bioRxiv https://doi.org/10.1101/2022.05.30.494059 (2022).
Parham, P. E. & Michael, E. Modeling the effects of weather and climate change on malaria transmission. Environ. Health Perspect. 118 , 620–626 (2010).
Thomas, M. B. Epidemics on the move: climate change and infectious disease. PLoS Biol. 18 , e3001013 (2020).
Lafferty, K. D. The ecology of climate change and infectious diseases. Ecology 90 , 888–900 (2009).
Siraj, A. S. et al. Altitudinal changes in malaria incidence in highlands of Ethiopia and Colombia. Science 343 , 1154–1158 (2014).
Article ADS CAS PubMed Google Scholar
Pascual, M., Ahumada, J. A., Chaves, L. F., Rodó, X. & Bouma, M. Malaria resurgence in the East African highlands: temperature trends revisited. Proc. Natl Acad. Sci. USA 103 , 5829–5834 (2006).
Paaijmans, K. P., Read, A. F. & Thomas, M. B. Understanding the link between malaria risk and climate. Proc. Natl Acad. Sci. USA 106 , 13844–13849 (2009).
Parham, P. E. et al. Modeling the role of environmental variables on the population dynamics of the malaria vector Anopheles gambiae sensu stricto. Malar J. 11 , 271 (2012).
Parham, P. E. & Hughes, D. A. Climate influences on the cost-effectiveness of vector-based interventions against malaria in elimination scenarios. Philos. Trans. R. Soc. Lond. B Biol. Sci. https://doi.org/10.1098/rstb.2013.0557 (2015).
Alto, B. W., Lounibos, L. P., Mores, C. N. & Reiskind, M. H. Larval competition alters susceptibility of adult Aedes mosquitoes to dengue infection. Proc. R. Soc. B-Biol. Sci. 275 , 463–471 (2008).
Westbrook, C. J., Reiskind, M. H., Pesko, K. N., Greene, K. E. & Lounibos, L. P. Larval environmental temperature and the susceptibility of aedes albopictus skuse (diptera: culicidae) to chikungunya virus. Vector-Borne Zoonotic Dis. 10 , 241–247 (2010).
Okech, B. A., Gouagna, L. C., Yan, G. Y., Githure, J. I. & Beier, J. C. Larval habitats of Anopheles gambiae s.s. (Diptera: Culicidae) influences vector competence to Plasmodium falciparum parasites. Malar. J. 6 , 7 (2007).
Paaijmans, K. P. et al. Influence of climate on malaria transmission depends on daily temperature variation. Proc. Natl Acad. Sci. USA 107 , 15135–15139 (2010).
Sternberg, E. D. & Thomas, M. B. Local adaptation to temperature and the implications for vector-borne diseases. Trends Parasitol. 30 , 115–122 (2014).
Shaw, W. R. et al. Multiple blood feeding in mosquitoes shortens the Plasmodium falciparum incubation period and increases malaria transmission potential. PLoS Pathog. 16 , e1009131 (2020).
Kwon, H., Simões, M. L., Reynolds, R. A., Dimopoulos, G. & Smith, R. C. Additional feeding reveals differences in immune recognition and growth of plasmodium parasites in the mosquito host. mSphere https://doi.org/10.1128/mSphere.00136-21 (2021).
Guissou, E. et al. A non-destructive sugar-feeding assay for parasite detection and estimating the extrinsic incubation period of Plasmodium falciparum in individual mosquito vectors. Sci. Rep. 11 , 9344 (2021).
Ferguson, H. M. & Read, A. F. Why is the effect of malaria parasites on mosquito survival still unresolved? Trends Parasitol. 18 , 256–261 (2002).
Carpenter, B. et al. Stan: a probabilistic programming language. J. Stat. Softw. https://doi.org/10.18637/jss.v076.i01 (2017).
Guidotti, E. calculus: high-dimensional numerical and symbolic calculus in R. J. Stat. Softw. 104 , 1–37 (2022).
Harrington, L. C. et al. Analysis of survival of young and old Aedes aegypti (Diptera: Culicidac) from Puerto Rico and Thailand. J. Med. Entomol. 38 , 537–547 (2001).
Pujol-Lereis, L. M., Rabossi, A. & Quesada-Allué, L. A. Lipid profiles as indicators of functional senescence in the medfly. Exp. Gerontol. 47 , 465–472 (2012).
Damos, P. & Soulopoulou, P. Do insect populations die at constant rates as they become older? Contrasting demographic failure kinetics with respect to temperature according to the weibull model. PLoS ONE 10 , e0127328 (2015).
Brady, O. J. et al. Modelling adult Aedes aegypti and Aedes albopictus survival at different temperatures in laboratory and field settings. Parasit. Vectors 6 , 351 (2013).
Srygley, R. B. Ontogenetic changes in immunity and susceptibility to fungal infection in Mormon crickets Anabrus simplex. J. Insect. Physiol. 58 , 342–347 (2012).
Wilson, D. L. The analysis of survival (mortality) data: fitting Gompertz, Weibull, and logistic functions. Mech. Ageing Dev. 74 , 15–33 (1994).
Pitchaimani, M. & Eakin, T. Unique estimation of Gompertz parameters with mortality deceleration rate. Math. Comput. Model. 47 , 104–114 (2008).
Article MathSciNet Google Scholar
Gompertz, B. On the nature of the function expressive of the law of human mortality and on a new mode of determining the value of life contingencies. Philos. Trans. R. Soc. 115 , 513–585 (1825).
Download references
Acknowledgements
We thank Deonna C. Soergel and Janet L. Teeple for technical assistance. This study was supported by NIH NIAID grant # R01AI110793 and National Science Foundation Ecology and Evolution of Infectious Diseases grant (DEB-1518681) awarded by M.B.T.; I.J.S. was supported by PhD funding from the Natural Environment Research Council (NE/P012345/1) administered through the Quantitative Methods in Ecology and Evolution Centre for Doctoral Training. I.J.S. & T.S.C. received support from Wellcome Trust (226072/Z/22/Z) and the MRC Centre for Global Infectious Disease Analysis (reference MR/X020258/1), funded by the UK Medical Research Council (MRC). This UK funded award is carried out in the frame of the Global Health EDCTP3 Joint Undertaking; and acknowledges funding by Community Jameel. The funders had no role in study design, data collection and analysis, decision to publish, or preparation of the manuscript.
Author information
Jessica L. Waite
Present address: Research Development, University of Vermont, Burlington, VT, USA
Matthew B. Thomas
Present address: Invasion Science Research Institute and Department of Entomology and Nematology, University of Florida, Gainesville, FL, USA
These authors contributed equally: Eunho Suh, Isaac J. Stopard.
Authors and Affiliations
Center for Infectious Disease Dynamics, Department of Entomology, The Pennsylvania State University, University Park, PA, USA
Eunho Suh, Jessica L. Waite, Nina L. Dennington & Matthew B. Thomas
MRC Centre for Global Infectious Disease Analysis, School of Public Health, Faculty of Medicine, Imperial College London, London, UK
Isaac J. Stopard & Thomas S. Churcher
Department of Statistics, University of Oxford, Oxford, UK
Ben Lambert
Department of Biology, University of York, York, UK
You can also search for this author in PubMed Google Scholar
Contributions
E.S., I.J.S., B.L., J.L.W., T.S.C. and M.B.T. designed the research; E.S., J.L.W. and N.L.D. conducted the experiment; E.S. and I.J.S. analysed the data; and E.S., I.J.S., B.L., T.S.C. and M.B.T. wrote the manuscript with inputs from J.L.W. and N.L.D.
Corresponding author
Correspondence to Eunho Suh .
Ethics declarations
Competing interests.
The authors declare no competing interests.
Peer review
Peer review information.
Nature Communications thanks Isobel Routledge, Miranda Teboh-Ewungkem and the other, anonymous, reviewer(s) for their contribution to the peer review of this work. A peer review file is available.
Additional information
Publisher’s note Springer Nature remains neutral with regard to jurisdictional claims in published maps and institutional affiliations.
Supplementary information
Supplementary information, peer review file, reporting summary, source data, source data, rights and permissions.
Open Access This article is licensed under a Creative Commons Attribution 4.0 International License, which permits use, sharing, adaptation, distribution and reproduction in any medium or format, as long as you give appropriate credit to the original author(s) and the source, provide a link to the Creative Commons licence, and indicate if changes were made. The images or other third party material in this article are included in the article’s Creative Commons licence, unless indicated otherwise in a credit line to the material. If material is not included in the article’s Creative Commons licence and your intended use is not permitted by statutory regulation or exceeds the permitted use, you will need to obtain permission directly from the copyright holder. To view a copy of this licence, visit http://creativecommons.org/licenses/by/4.0/ .
Reprints and permissions
About this article
Cite this article.
Suh, E., Stopard, I.J., Lambert, B. et al. Estimating the effects of temperature on transmission of the human malaria parasite, Plasmodium falciparum . Nat Commun 15 , 3230 (2024). https://doi.org/10.1038/s41467-024-47265-w
Download citation
Received : 25 September 2023
Accepted : 26 March 2024
Published : 22 April 2024
DOI : https://doi.org/10.1038/s41467-024-47265-w
Share this article
Anyone you share the following link with will be able to read this content:
Sorry, a shareable link is not currently available for this article.
Provided by the Springer Nature SharedIt content-sharing initiative
By submitting a comment you agree to abide by our Terms and Community Guidelines . If you find something abusive or that does not comply with our terms or guidelines please flag it as inappropriate.
Quick links
- Explore articles by subject
- Guide to authors
- Editorial policies
Sign up for the Nature Briefing newsletter — what matters in science, free to your inbox daily.

Click through the PLOS taxonomy to find articles in your field.
For more information about PLOS Subject Areas, click here .
Loading metrics
Open Access
Peer-reviewed
Research Article
Modeling the shared risks of malaria and anemia in Rwanda
Roles Conceptualization, Data curation, Formal analysis, Investigation, Validation, Visualization, Writing – review & editing
* E-mail: [email protected]
¶ ☯ All these authors are contributed equally to this work.
Affiliation African Institute for Mathematical Sciences (AIMS), Kigali, Rwanda

Roles Methodology
Affiliation African Centre of Excellence in Data Science, University of Rwanda, Kigali, Rwanda
Roles Supervision
Affiliations African Institute for Mathematical Sciences (AIMS), Kigali, Rwanda, Department of Statistics, Federal University of Technology, Akure, Nigeria
- Pacifique Karekezi,
- Jean Damascene Nzabakiriraho,
- Ezra Gayawan
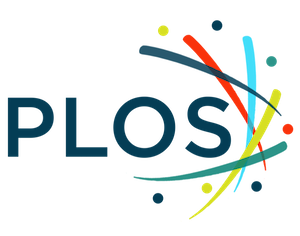
- Published: April 22, 2024
- https://doi.org/10.1371/journal.pone.0298259
- Peer Review
- Reader Comments
In sub-Saharan Africa, malaria and anemia contribute substantially to the high burden of morbidity and mortality among under-five children. In Rwanda, both diseases have remained public health challenge over the years in spite of the numerous intervention programs and policies put in place. This study aimed at understanding the geographical variations between the joint and specific risks of both diseases in the country while quantifying the effects of some socio-demographic and climatic factors. Using data extracted from Rwanda Demographic and Health Survey, a shared component model was conceived and inference was based on integrated nested Laplace approximation. The study findings revealed similar spatial patterns for the risk of malaria and the shared risks of both diseases, thus confirming the strong link between malaria and anaemia. The spatial patterns revealed that the risks for contracting both diseases are higher among children living in the districts of Rutsiro, Nyabihu, Rusizi, Ruhango, and Gisagara. The risks for both diseases are significantly associated with type of place of residence, sex of household head, ownership of bed net, wealth index and mother’s educational attainment. Temperature and precipitation also have substantial association with both diseases. When developing malaria intervention programs and policies, it is important to take into account climatic and environmental variability in Rwanda. Also, potential intervention initiatives focusing on the lowest wealth index, children of uneducated mothers, and high risky regions need to be reinforced.
Citation: Karekezi P, Nzabakiriraho JD, Gayawan E (2024) Modeling the shared risks of malaria and anemia in Rwanda. PLoS ONE 19(4): e0298259. https://doi.org/10.1371/journal.pone.0298259
Editor: Clement Ameh Yaro, University of Uyo, NIGERIA
Received: February 28, 2023; Accepted: January 22, 2024; Published: April 22, 2024
Copyright: © 2024 Karekezi et al. This is an open access article distributed under the terms of the Creative Commons Attribution License , which permits unrestricted use, distribution, and reproduction in any medium, provided the original author and source are credited.
Data Availability: All relevant data are within the paper and its Supporting information files.
Funding: The authors received no specific funding for this work.
Competing interests: The authors have declared that no competing interests exist.
Introduction
In sub-Saharan Africa, malaria and anemia contribute substantially to the high burden of morbidity and mortality among under-five children. In Rwanda, both diseases have remained as public health challenge over the years in spite of the numerous intervention programs and policies put in place. The well-being and survival chances of women and young children have continued to be endangered by the diseases [ 1 , 2 ]. The occurrence and magnitude of other diseases and malnutrition are exacerbated by malaria parasite leading to severe morbidity and mortality in some extreme cases. Malaria parasites can consume iron molecules in an individual’s red blood cell causing low functional hemoglobin concentration. In the case of young children, low hemoglobin concentration results in severe anemia and prevents quick recovery [ 3 ]. Anemia, commonly caused by iron deficiency and this occurs in situation where there is insufficient amount of iron in the red blood cells [ 4 ]. Malaria parasites feed on iron in the blood cell, leading to shortage of hemoglobin (Hb) concentration level. Thus, there are synergies between the causal relationship of malaria and anemia. Plasmodium falciparum can encroach into the red blood cell leading to acute hemolysis thereby hindering the proper development of the red cell, causing severe anemia [ 5 ]. On the other hand, severe anemia worsens morbidity from malaria particularly in children [ 6 ].
In both the tropics and subtropics, anemia is considered a health risk for under-five children particularly for those who simultaneously suffer from malaria [ 7 ].
It is a condition in which the blood is in short of healthy red blood cells, which could be due to malfunctioning of existing red blood cells that affects the flow of oxygen to the body tissue [ 8 ]. Under-five children and pregnant women are most affected by the global public health issue; with the World Health Organization estimating that 42% of children under this group and 40% of pregnant women are anemic worldwide [ 9 ]. Children who suffer from anemia experience increased heart rate, poor wound and tissue healing, poor cognitive development all of which compromise the child’s quality of life [ 10 ].
Malaria and anemia put direct pressure on the already fragile health care systems of developing countries, and also negatively affect individuals and households because of the loss of income and other economic consequences that arise due to loss of man-hour occasioned by the time spent during the recovery process or in taking care of the sick one [ 2 ]. Many developing countries are malaria endemic, where a huge proportion of the annual documented 300 million clinical cases and 2 million deaths take place. For instance, in 2019, 95% of the 229 million reported malaria cases came from 29 developing, while malaria deaths were put at 409, 000 and about 67% of this number occurred among under-five children [ 11 ].
Sub-Saharan African countries jointly account for about 93% of all malaria cases and 94% of malaria deaths that took place in 2018 with majority of the death reported among under-five children [ 9 ]. This is in spite of the multiple evolving government policies coupled with interventions from international development agencies. In Rwanda, malaria is the second leading cause of morbidity and is responsible for about 7.4% per cent of outpatient hospital visits and 4.3% of malaria proportional mortality [ 12 ]. Prior to year 2012, the country experienced a downward trend in malaria cases culminating to a record low of 48 per 1,000. However, between this period and 2016, there was a reversal in the gains that saw the number of reported cases rising to about 403 per 1,000 in 2016 [ 13 ].
Consequently, malaria gains in the country can be considered fragile and sustained policies that can push back new cases need to be scaled up. Human activities and behaviour, climatic change, environmental modification, and insecticide resistance play important roles in the sudden surge in cases [ 13 , 14 ]
The incidence of malaria has been linked to altitude, with a higher prevalence in lowlands than in highlands settings, and more cases occur around May-June and November-December. In addition to favorable climate, closeness to marshlands, irrigation schemes, and movement of people within and across geographical border influence the transmission, particularly in the Southern and Eastern fringes of the country [ 14 , 15 ]. As earlier argued, higher malaria risk in a given location would also imply higher risk of anemia particularly in children.
Several studies have been undertaken to evaluate the incidence and determinants of malaria and anaemia among under-five children in Rwanda including their geographical distributions [ 1 , 14 , 16 – 18 ]. However, limited efforts have gone into modeling the possible co-morbidity of the two disease, and there exists a persistent knowledge gap in their spatial overlap that specifically highlight districts with high and low risks of the combined diseases. Moreover, only few studies have mapped malaria and anaemia prevalence while controlling for climatic conditions particularly temperature and rainfall in the country. It is important to understand the patterns of the spatial overlap of both (shared) and specific risks from malaria and anaemia at small scale levels across the country, and to quantify the dynamics of climatic conditions for the two diseases as these could effectively aid intervention programs that are aimed at addressing their occurrence among young children. Consequently, this study was designed to estimate the shared and specific spatial patterns of malaria and anemia among children under five years of age in Rwanda using data extracted from the 2014–2015 Rwanda Demographic and Health Survey (RDHS). We use a shared-component modeling approach within a Bayesian framework allowing for simultaneous estimation of metrical variables as nonlinear effects, location-specific random effects that account for spatial heterogeneity, and the usual linear effects of categorical variables. The model enables the distinct estimation of the underlying risk surface for the diseases in the form of a component shared by both diseases (refered to as the shared-component) and others that are specific to each disease (disease-specific components). The shared spatial component can be viewed as a surrogate for unobserved covariates that influence the spatial structure of both diseases. We considered the effects of temperature and rainfall as climatic in addition to other demographic factors on the shared risks of the two diseases. To produce approximations to the posterior marginals of all parameters of interest, we adopt the integrated nested Laplace approximations (INLA) approach.
Materials and methods
The study used data obtained through the 2014–2015 Rwanda Demographic and Health Survey (RDHS). The survey, implemented by the National Institute of Statistics of Rwanda in partnership with the Rwanda Biomedical Center (RBC) and Rwanda Ministry of Health (MoH) between November 2014 and April 2015, provide essential data for quantifying and monitoring important demographic and health indicators at the national level, the five provinces, each of Rwanda’s 30 districts and for urban and rural areas. The survey was executed through a two-stage sampling design where enumeration areas (EAs), referred to as clusters, were selected at the first stage from the sampling frame used during the 2012 Rwanda Population and Housing Census (RPHC). A total of 492 clusters comprising 113 in urban and 379 in rural areas were selected. The second stage involves the selection of households from the listed EAs, which were picked at random. A total of 12,793 households were selected comprising of about twenty-six households from each EA.
Eligible women for interview were those aged 15 to 49 years who were the permanent residents of the selected households or those who slept in the households the night before the survey. There were 13,564 eligible women in the selected households and 13,497 were successfully interviewed, yielding a response rate of 99.5 percent. Information was also collected on children younger than five years of age from the caregivers in all the households. Anemia and malaria testing were executed in the sub-sample of households not selected for the male survey. After informed consent was obtained from the caregiver, children in these households were tested for malaria and anemia. The malaria test was executed through the rapid diagnostic test (RTD) and thick and thin blood smears. A drop of blood was taken by pricking the end of the finger in the case of RDTs and this was also used for anemia testing.
The response variables of interest were created from the malaria and anemia indicators with each variable taking the value of one if the child suffers from the ailment and zero if otherwise.
The independent variables considered include type of place of residence, sex of a household head, bed-net ownership, bed-nets usage, household wealth index, mother’s educational attainment, child’s sex, child’s age, and age of household head. The district of residence was geo-referenced. Furthermore, climate-related factors including temperature and rainfall were obtained from the GPS data set of the 2014–2015 RDHS to examine their effect on malaria and anemia co-morbidity. We extracted the shapefile of Rwanda from the Spatial Data Repository put together by The DHS Program [ 19 ]. This was used in constructing the adjacency matrix for the districts of Rwanda used in our Bayesian model and for plotting the estimates on maps.
Ethics statement
The study used a secondary data obtained from the Demographic and Health Survey after approval was secured. It should be noted that DHS adopts a coordinate displacement process to ensure the preservation of the identity of the respondents. Urban clusters are displaced on a distance of up to two kilometers and up to five kilometers for rural clusters. However, this would have minimal effect on our analysis since we used an area-level spatial approach that considers the districts of Rwanda hence every sampled individual would belong to a district notwithstanding the displacement of the coordinate.
Statistical analysis
Shared component model..
We implemented the model through a Bayesian inference that relies on the integrated nested Laplace approximation (INLA) as proposed by [ 21 ]. The prior distribution for σ d was log-normal with a mean of 0 and accuracy of 0.1. A weakly informative Gaussian prior with small precision τ β on the identity matrix was considered, and used for the fixed parameters and constant term, that is β ∼ N (0, τ β I ).
Data preparation and frequency tables were generated using Stata 14 while the Bayesian model was implemented using the R-INLA package.
Table 1 presents the descriptive analysis of the co-morbidity of malaria and anemia based on household characteristics of the under-five children. Overall, about 49% (1673/3446) of the children had malaria, anemia, or both. About 7.5% (259/3446) had malaria, 35.6% (1228/3446) had anemia, and another 5.4% (186/3446) suffered both malaria and anemia. Among the under-five children from rural areas, a greater proportion had malaria 8.7%, (239/2738), 36.9% (1009/2738) suffered anemia whereas 6.2% (169/2738) had both malaria and anemia. Among the children from female headed households, 10.4% (74/709) suffered malaria while 7.8% (55/709) had anemia and malaria. On other hand, slightly high proportion (35.7%, 976/2737) of children from male-headed households suffered anemia when compared with with female-headed households (35.5%, 252/709). Among children from households with no bed nets, 9.9% (53/538) suffered malaria, 37.7% (203/538) had anemia, and 8.6% (46/538) suffered both malaria and anemia. The proportion of the children who suffered malaria, anemia, or both illnesses varies with the use of bed nets such that 9.5% (27/284) of children from households in which some slept under bed nets suffered malaria, whereas 7.8% (75/967) of children who did not sleep under bed nets suffered both malaria and anemia. Children from the richest households were less likely to suffer the episodes of both malaria and anemia (0.5%, 3/627). Contrarily, among children from poorest households, about 10.1% (86/852) suffered both malaria and anemia. Children whose mothers attained at least primary education are less likely to suffer malaria, anemia, or both. The study findings also show that the proportion of children who had malaria, or both malaria and anemia did not vary with child’s sex. However, slightly high proportion of male children had anemia (36.7%, 645/1757).
- PPT PowerPoint slide
- PNG larger image
- TIFF original image
https://doi.org/10.1371/journal.pone.0298259.t001
Table 2 presents results of the linear effects for malaria and anemia showing the posterior means, standard deviations, and 95% credible intervals. When compared with children from rural areas, those from urban settings are less likely to develop both diseases, though the estimate is not significant. Children from male-headed households are less likely to have both diseases compared with those from female-headed households. Whereas ownership of bed nets in households shows lower likelihood for contracting both diseases, the estimates for usage are not significant. The study findings revealed an association between wealth quintile and coexistence of the two diseases such that children from at least the poorer households are less likely to contract both diseases when compared with those from the poorest households. Children whose mothers attained primary level of education are less likely to suffer from both diseases when compared with those with no education but the result for secondary/higher education is not significant. The findings on the sex of the children show non-significant effects.
https://doi.org/10.1371/journal.pone.0298259.t002
The results for the spatial effects presented in Figs 1 to 12 , show the maps for the posterior means, standard deviations, and 95% credible intervals, respectively for the shared effects of malaria and anemia, specific-effects for malaria and for anemia, with the results displaying uneven distributions of the burden of the diseases across the districts of Rwanda. The findings from the shared random effects (Figs 1 to 4 ) reveal that the highest risks of contracting malaria and anemia can be found among children from Rutsiro, Nyabihu, Ruhango, Gisagara, and Rusizi districts, while those from Rubavu, Karongi, Ngororero, Kayonza, Gatsibo, Rwamagana, Gasabo and Rulindo have moderate risks. The joint effects of Malaria and anemia seem to be negligible across the other districts of the country. The standard deviation and credible intervals maps show that there is more uncertainty about the risk of malaria and anemia in various parts of the country, particularly in the districts of Nyagatare, Rubavu, Ruhango and Nyamasheke.
https://doi.org/10.1371/journal.pone.0298259.g001
https://doi.org/10.1371/journal.pone.0298259.g002
https://doi.org/10.1371/journal.pone.0298259.g003
https://doi.org/10.1371/journal.pone.0298259.g004
https://doi.org/10.1371/journal.pone.0298259.g005
https://doi.org/10.1371/journal.pone.0298259.g006
https://doi.org/10.1371/journal.pone.0298259.g007
https://doi.org/10.1371/journal.pone.0298259.g008
https://doi.org/10.1371/journal.pone.0298259.g009
https://doi.org/10.1371/journal.pone.0298259.g010
https://doi.org/10.1371/journal.pone.0298259.g011
https://doi.org/10.1371/journal.pone.0298259.g012
In terms of the specific components, the spatial distributions for malaria (Figs 5 to 8 ) reveal a pattern that is somewhat similar to the shared spatial pattern obtained for the combined diseases. Specifically, the risks for malaria are highest among children living in Rutsiro, Nyabihu, Ruhango, Rusizi and Gisagara districts but moderate for those residing in Gatsibo, Kayonza, Rwamagana, Gasabo, Rulindo, Karongi, Ngororero, and Rubavu. However, the risks are lowest among those children living in Gakenke, Nyagatare, and Kirehe districts. The standard deviation and credible interval maps reflect high uncertainty in the districts of Rubavu, Kirehe, Nyagatare, Rusizi, Nyamasheke, Nyaruguru and Gisagara. In the case of anemia (Figs 9 to 12 ), the risks are higher among the children living in Rutsiro, Nyabihu, Rulindom, Ngoma, and Gisagara, followed by the districts of Rusizi, Huye, Nyanza, Ruhango, Ngororero, Gakenke, Gasabo, Nyarugenge, Kicukiro, and Kayonza. However, the risks for anemia are lower in the districts of Nyamasheke, Nyamagabe, Karongi, Muhanga, Kamonyi, Bugesera, Rwamagana, Gatsibo, Gicumbi, Nyagatare, Burera, Musanze and Rubavu. Nyaruguru and Kirehe.
The nonlinear effects of child’s age, age of household head, annual precipitation and mean temperature for the shared effects are presented in Figs 13 to 16 while those for the specific effects of malaria and anemia are presented in Figs 17 to 24 respectively. The Figures show the posterior means (middle lines) surrounded by the 95% credible intervals. The findings show a negative relationship between the likelihood of suffering from both diseases and the age of the child, indicating that as a child gets older, the likelihood of suffering from both diseases decreases. The estimates for household head show higher likelihood among children whose head of household is 75 years or older. For precipitation, the likelihood of shared risk increases with precipitation up to 120 mm/day, but starts decreasing when precipitation exceeds 120 mm/day though the credible intervals are generally wider. The findings revealed a positive association between temperature and risk of contracting both diseases, such that as temperature increases, the children become more prone to the two illnesses.
https://doi.org/10.1371/journal.pone.0298259.g013
https://doi.org/10.1371/journal.pone.0298259.g014
https://doi.org/10.1371/journal.pone.0298259.g015
https://doi.org/10.1371/journal.pone.0298259.g016
https://doi.org/10.1371/journal.pone.0298259.g017
https://doi.org/10.1371/journal.pone.0298259.g018
https://doi.org/10.1371/journal.pone.0298259.g019
https://doi.org/10.1371/journal.pone.0298259.g020
https://doi.org/10.1371/journal.pone.0298259.g021
https://doi.org/10.1371/journal.pone.0298259.g022
https://doi.org/10.1371/journal.pone.0298259.g023
https://doi.org/10.1371/journal.pone.0298259.g024
The findings for malaria Figs 17 to 20 reveal a positive association between the likelihood of having malaria and child’s age up to around 30 months but beyond this age, the likelihood decreases. As for the shared pattern, the likelihood of suffering from malaria increases for children whose household heads are older than 75 years, but lower before this age. The chances of having malaria increases with precipitation below 135 mm/day, but beyond this point, the chances of having malaria decreases. As for temperature, the study findings reveal wider credible intervals reflecting higher uncertainty around the estimates thus, the estimates may need to be interpreted with caution. The non-linear effects of child’s age, age of household head, annual precipitation and mean temperature estimated for anemia are presented in Figs 21 to 24 . The findings show a negative association between the risk of having anemia and the child’s age similar to what was obtained for the shared component, while in the case of age of household head, the risks are similar throughout the ages. Moreover, the risk of having anemia rises with increase in precipitation while the estimates for temperature appears flat but with wider credible interval.
A shared component modeling approach was utilized to map co-morbidity of malaria and anaemia among under-five children in Rwanda. The Bayesian spatial model allows the mapping of spatial phenomena across small geographical units and to quantify the effects of both socio-demographic and climatic factors on co-morbidity of the diseases. The findings from the study have practical relevance in a country like Rwanda where children still suffer co-morbidity from several diseases. Moreover, they provide evidence for effective and efficient implementation of intervention programs and policies designed to eliminate malaria and anaemia in Rwanda. The linear effects have shown the significant association between socio-demographic characteristics of the children and co-morbidity from the two diseases. The findings show no significant difference in the likelihood of suffering from both disease among the under-five children residing in urban and rural areas. However, findings from some African countries have shown that under-five children from urban settings are less likely to suffer from both malaria and anaemia when compared with those from rural settings [ 23 , 24 ], while a Rwandan study has demonstrated lower likelihood for malaria among urban children [ 1 ]. In most rural settings in Rwanda, where more than two-thirds of the population reside, the majority of the caregivers are farmers and small scale traders who have limited access to information on disease control and prevention methods when viewed in respect to the urban dwellers. They equally suffer from acute presence of health professionals and healthcare facilities as is the case in many developing countries, and thus, overstretching the available few [ 1 ].
The findings of the study demonstrate inverse relationship between household wealth and the likelihood of co-morbidity from malaria and anaemia indicating that as wealth increases, the chances of co-morbidity reduces. These findings are consistent with those of [ 25 , 26 ], indicating that children from impoverished households have higher prevalence of malaria compared with those from wealthy families. This may be attributed to lack of ability to afford adequate and balanced diet for children from poor families. Moreover, wealthy households would have adequate resources to acquire preventive and curative measures that safe guide their children from diseases. The similar significant association between household wealth and likelihood of having malaria and anaemia among under-five children have been reported in several other places [ 2 , 24 ].
We found that bed nets ownership reduce the risk of co-morbidity from malaria and anaemia but this was not the case for usage. Regular use of bed nets especially insecticide treated nets is a major vector control measure to prevent malaria and by extension, anemia [ 25 ]. A previous analysis of the data used in this study but where only malaria was consider revealed that both ownership and usage of bed nets reduce the risk of malaria [ 1 ]. The non-significant estimate obtained for usage in this case might have indicated that the usage of bed nets is not sufficient to cause a difference in co-morbidity from both diseases as it does for only malaria. Similar to some previous studies, we found children from male-headed household to be less prone to co-morbidity from the two diseases [ 26 , 27 ]. As with most sub-Saharan African countries the majority of Rwandan households are male-headed who often provide protective leads to members of the family than what can be obtained in households headed by female. We however found no substantial difference when the sex of the child was considered.
The findings show an association between mother’s educational attainment and likelihood of co-morbidity from the diseases. Children whose mothers completed at least primary education are less likely to suffer from both diseases. This association may be linked with the fact that higher educational attainment lead to improved knowledge, perception, and practice about strategies for preventing and treating diseases [ 28 , 29 ]. Educated mothers are at a vintage position to know where to find and how to expressed themselves to physicians such that their children get the best of treatments than the uneducated ones will do.
The study findings revealed spatial heterogeneity in the shared risks of malaria and anaemia, and also from the individual diseases among under-five children in Rwanda. It is interesting to note that the spatial pattern obtained for malaria is similar to that observed for the shared risks of the two diseases more than does the map obtained for anemia. This finding further reinforces the synergy between the epidemiology causal pathways of malaria and anemia, which ensures that anemia is often a complication that could arise from multiple episodes of malaria [ 2 ]. Districts with substantial high risks include Rutsiro, Nyabihu, Ruhango, Gisagara, and Rusizi, while moderate risks were estimated for Rubavu, Karongi, Ngororero, Kayonza, Gatsibo, Rwamagana, Gasabo and Rulindo. The majority of the districts with high risks are places with high rates of illiteracy and poverty which would limit caregivers ability of protect their children from infectious diseases [ 22 ]. The existence of Gishwati forest, in which many agricultural and farming activities take place, could aggravate the risks for both diseases in the districts of Rutsiro and Nyabihu [ 30 ]. Also, the higher risk of co-morbidity of these diseases in Rusizi district may be compounded by the abundance of breeding sites for anopheles mosquitoes in the Nyungwe forest and Bugarama plain as reported by [ 31 ]. The high temperature often experienced in the Bugarama plain, a rice farming environment, could also increase the risk of malaria transmission by mosquitoes [ 32 ]. The presence of several agricultural activities in Ruhango and Gisagara, coupled with cross border movement can define the increased likelihood of co-morbidity of malaria and anaemia. The irrigation-based agricultural practices mainly in the wetlands of the Eastern and Southern parts of the country has been reported to influence malaria through the creation of vector breeding sites [ 33 ]. The observed spatial variation could be also linked with numerous factors including spatial disparity in human behaviour, awareness, and media exposure to malaria, child feeding practices, and disease prevention strategies. The high prevalence of malaria across the western provinces can be further explained by the presence of Lake Kivu which has been reported to speed up malaria transmission [ 34 ].
The findings established nonlinear relationships between the shared risk of the two diseases and child’s age, age of household head, annual precipitation, and mean temperature, which were considered as metrical covariates. The findings show high likelihood for the shared risks of malaria and anemia among children below 24 months of age, and this is similar to what was obtained for the specific component of anemia. Findings from other African countries have similarly exhibited this pattern of relationship [ 4 ]. The first two years of life is a period of intense growth and development, making the children to have high demand for iron, but which may not be available in the required quantity particularly for children from low socio-economic settings. Weaning food may also be obtained from some unhygienic sources which could aggravate the risk of illness and subjecting the child to anemia. Moreover, due to the fragile nature of these children, they are highly susceptible to infections.
Temperature was revealed to be positively associated with the shared risks of malaria and anaemia. An increase in temperature could shorten the time period it takes for new generations of mosquitoes to emerge, as well as the parasite’s incubation period in mosquitoes [ 35 ]. Using an ecological model with malaria transmission data across African countries, [ 36 ] revealed that malaria transmission is at its optimal around temperature of 25° C , but this decreases substantially when temperature exceeds 28° C . The findings on annual precipitation reveals that when it is below 120 mm/day, the likelihood of co-morbidity of malaria and anemia increases with precipitation but when it is exceeds 120 mm/day, the risk decreases. Precipitation greater than 140mm destroys mosquitoes sites and leads to decrease in malaria transmission. The amount of precipitation determines the ample quantity and length of malaria transmission across different locations in a manner that in lower altitude regions, increase in precipitation might go with increase of available anopheles sites [ 37 , 38 ].
This study suffers from some limitations that need to be mentioned. The study was based on district level data but this could mask fine-scale variations because the districts are composed of several spatial units. A further analysis that creates maps at a continuous scale could provide more information on local variations than is obtainable here. As with similar studies that use data from cross-sectional survey, we were unable to make causal inference from our findings. An observational study in each of the districts could provide more information regarding the pattern of infection from malaria and anemia and why they vary from place to place. Furthermore, there are possibilities of bias responses to questions that seek to elicit information on ownership and use of bed nets as the accuracy depends solely on the willingness of the respondents to provide the right response. The wealth index variable that classified the children into different wealth strata, was also computed based on households assets commonly found in urban areas whereas, most rural dwellers who could posses other wealth indicators like livestock or other farm machinery would be classified in the lowest categories resulting in mis-classification. All these notwithstanding, the data source provide adequate information that allow for spatial analysis as done here and this provide information that can enhance intervention in the country.
This study aimed at understanding the shared spatial risks of malaria and anemia among under-five children in Rwanda using a shared component model while controlling for other important determinants. The study reveals that socio-demographic characteristics such as place of residence, mother’s educational level, wealth index, sex of household head, bed net ownership are among the major risk factors of the shared risk of the two diseases among Rwandan children. Rising precipitation particularly above 120 mm/day is associated with decreasing risk of co-morbidity of diseases. Furthermore, the risk of developing both diseases increases as the temperature rises. Findings from the study allow for the identification of the uneven spatial variations in joint and specific risks of suffering from malaria and anemia among young children in the country. Specifically, children from Rutsiro, Nyabihu, Ruhango, Rusizi, and Rusizi districts are particularly at higher risks. The regional variation of malaria is nearly identical to the spatial pattern of the shared risk of both diseases, indicating that malaria could the leading driver of anemia among children in the country. The study recommends considerable efforts to improve the existing intervention programs and control strategies that prioritize higher risk regions. Moreover, special attention needs to be accorded to individuals living in lowest wealth quintiles, female headed households, and children of uneducated mothers when developing malaria and anemia intervention programs and policies. Also, the study recommends that when formulating malaria and anemia intervention programs, climate and environmental factors particularly temperature and precipitation should be taken into account.
Supporting information
https://doi.org/10.1371/journal.pone.0298259.s001
Acknowledgments
PK is grateful to AIMS Rwanda for scholarship towards a master program during which the study was undertaken. The authors appreciate permission from The DHS Program to utilize the data set analysed in the study.
- View Article
- PubMed/NCBI
- Google Scholar
- 8. Bryant R. Anemias. In: Pediatric oncology nursing. Springer; 2010. p. 141–72.
- 9. World Health Organization and others. WHO urges countries to move quickly to save lives from malaria in sub-Saharan Africa. quickly to save lives from malaria in sub-Saharan Africa.
- 12. World Health Organization and others. Health policy and system support to optimize community health worker programmes for HIV, TB and malaria services: an evidence guide.2020.
- 19. https://spatialdata.dhsprogram.com/boundaries/#view=table&countryId=RW .
- 33. WHO. Prevention and Control of Malaria Epidemics: Tutor’s Guide. Geneva:. World Health Organiza- tion. 2003.
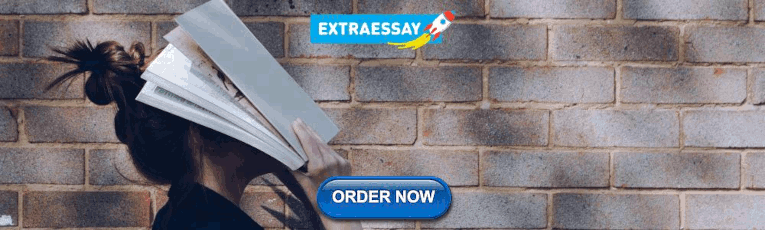
IMAGES
COMMENTS
One study from East Timor in 2006 reported a co-infection with Plasmodium falciparum malaria and dengue in a 7-year-old girl who subsequently died. 6 Three further descriptive studies and one case control study were published from India 7 8 and French Guiana, 9 10 all patients in these studies being adult patients. Malaria and dengue co ...
This case‑study is part of a series of malaria elimination case‑studies conducted by the WHO Global Malaria Programme and the Global Health Group at the University of California, San Francisco (UCSF/GHG). The two groups wish to acknowledge the financial support of the Bill & Melinda Gates Foundation in
INTRODUCTION. Malaria is a frequent parasitic infection prevalent in Africa. Around 300 million are infected annually in Africa by malaria and 1 to 2 million will die from the disease. 1 Of the 4 human parasitic species that have been identified, Plasmodium falciparum has been known to cause significant morbidity and mortality, particularly in children and pregnant women. 1 Strategies to ...
A community-based case-control study with a 1:1 ratio was employed at Waghemra Zone from September 14 to November 27, 2022. ... the aim of this study was to assess the occurrence of malaria ...
Malaria is a disease that is spread between people via infected mosquitoes. The bite of an infected Anopheles mosquito transmits a Plasmodium parasite that enters the victim's blood and travels into the person's liver, where it reproduces.The parasites then travel through the bloodstream and enter red blood cells, where they rapidly reproduce and burst red blood cells open; this repeats in ...
The patient presented in this case had severe malaria, specifically cerebral malaria, 18 days after returning to the United States from a 10-day trip to Ghana. ... The TropNet Severe Malaria Study. Clin Infect Dis. 2015; 61 (9):1441-14. [Google Scholar] 17. Tan KR, Arguin PM. Chapter 4 Travel-related infectious diseases Malaria In: CDC yellow ...
1. Introduction. Malaria affected an estimated 219 million people causing 435,000 deaths in 2017 globally. This burden of morbidity and mortality is a result of more than a century of global effort and research aimed at improving the prevention, diagnosis, and treatment of malaria [].Malaria is the most common disease in Africa and some countries in Asia with the highest number of indigenous ...
We conducted a prospective case-control study to identify risk factors associated with this sudden rise in malaria in the city (Goro Health Center) and DDU (Fig. 1b). In the city we recruited 48 ...
P. vivax malaria should be treated with chloroquine, except when acquired in Papua New Guinea and Indonesia, areas with high prevalence of chloroquine-resistant P. vivax. After a normal G6PD test, patients should get a radical cure with primaquine (30 mg per day for 14 days). Interactive clinical case-study dealing with a 44 year old man with ...
Imported malaria case study: Brazil's malaria epidemiological surveillance information system ... regardless of residence location. An imported case was defined as a case in which the ...
Malaria is resurging in many African and South American countries, exacerbated by COVID-19-related health service disruption. In 2021, there were an estimated 247 million malaria cases and 619 000 deaths in 84 endemic countries. Plasmodium falciparum strains partly resistant to artemisinins are entrenched in the Greater Mekong region and have emerged in Africa, while Anopheles mosquito vectors ...
Passively reported malaria data was collected from the government's Malaria Information System (MIS), which collects malaria case data from all villages in Mondulkiri. These health facilities reported the highest malaria caseloads in Mondulkiri in 2019-2020, which was the reason they were selected for this study.
A prospective population-based surveillance study was conducted through Eswatini's national passive and reactive malaria case detection program. Study Site Eswatini is a very low-transmission setting with annual malaria incidence of 0.7−1.3 per 1000 population from 2012 to 2015 and parasite prevalence of 0.2% in 2010 [ 31 , 32 ].
In the case of malaria, accessibility (distance or travel time) ... In this case study, location-allocation analysis can leverage a relatively standard risk factor model to create actionable decisions by integrating other pieces of information such as population distribution, estimated travel time, and prevalence to incidence conversion. ...
Malaria is a life-threatening disease spread to humans by some types of mosquitoes. It is mostly found in tropical countries. It is preventable and curable. The infection is caused by a parasite and does not spread from person to person. Symptoms can be mild or life-threatening.
Background Malaria is a public health concern worldwide. A figure of 3.2 billion people is at risk of malaria a report of World Health Organization in 2013. A proportion of 89 and 91 cases of malaria reported during 2015 were respectively attributed to malaria cases and malaria deaths in Sub-Saharan Africa. Rwanda is among the Sub-Saharan Africa located in East Africa. The several reports ...
Global interest in malaria elimination has prompted research on active test and treat (TaT) strategies. A systematic review and meta-analysis were conducted to assess the effectiveness of TaT strategies to reduce malaria transmission. A total of 72 empirical research and 24 modelling studies were identified, mainly focused on proactive mass TaT (MTaT) and reactive case detection (RACD) in ...
Malaria is a Serious Disease. CDC is collaborating with three state health departments on an investigation of seven locally acquired cases of Plasmodium vivax (P. vivax) malaria in Sarasota County, FL, one case of P.vivax in Cameron County, TX, and one case of P. falciparum malaria in a Maryland resident who lives in the National Capital Region.
This study analysed historical malaria cases in India from 1990 to 2022 to assess the annual trends and the impact of key anti-malarial interventions on malaria incidence. Factors associated with malaria incidence were identified using univariate and multivariate linear regression analyses. ... From 2001 to 2010, there was an annual malaria ...
Where Malaria Occurs. Where malaria is found depends mainly on climatic factors such as temperature, humidity, and rainfall. Malaria is transmitted in tropical and subtropical areas, where. Anopheles mosquitoes can survive and multiply, and. Malaria parasites can complete their growth cycle in the mosquitoes ("extrinsic incubation period").
Predicting the risk of malaria in countries certified malaria-free is crucial for the prevention of re-introduction. This review aimed to identify and describe existing prediction models for malaria re-introduction risk in eliminated settings. A systematic literature search following the PRISMA guidelines was carried out. Studies that developed or validated a malaria risk prediction model in ...
Trend analysis of malaria surveillance data is essential to inform stakeholders on progress towards malaria control. From the total 387,096 cases of malaria reported in Amhara region in 2017, 167,079 (43.2%) cases were in Central, North and West Gondar zones. From this total figure of zones, 15,445 (9.2%) were ≤ 5 years which contributes 4% of cases in the region.
The malaria parasite life cycle involves two hosts. During a blood meal, a malaria-infected female Anopheles mosquito inoculates sporozoites into the human host .Sporozoites infect liver cells and mature into schizonts , which rupture and release merozoites . (Of note, in P. vivax and P. ovale a dormant stage [hypnozoites] can persist in the liver (if untreated) and cause relapses by invading ...
This case-control study verified the occurrence of a malaria outbreak in the Waghemra zone. Age, the availability of artificial water-holding bodies, nearby stagnant water, sleeping outside overnight, and a lack of knowledge about malaria transmission and prevention all contributed to the epidemic's existence.
National 5; Health Case study in a developing country- malaria. Case studies illustrate the causes of health issues. They explain their growth, effects on the population and the strategies ...
More information: Usman Nazir et al, Predicting malaria outbreaks using earth observation measurements and spatiotemporal deep learning modelling: a South Asian case study from 2000 to 2017, The ...
Case Studies and Success Stories. Discover how organizations like DC Health have improved data collection and sharing through GIS in the DC Health case study. Learn from Zambia's approach to eradicating malaria using geospatial data in Seeking to eradicate malaria: Zambia turns to geospatial data. Learning Opportunities
Methods. A systematic review was undertaken to summarise outcomes of individually randomised trials assessing treatments for SM in adults and children. We searched key databases and trial registries between 1 January 2010 and 30 July 2020. Non‐randomised trials were excluded to allow comparison of similar trials.
There is, however, insufficient data on subsequent P. falciparum development in Anopheles gambiae, and mechanistic modelling studies examining the influence of climate on malaria transmission tend ...
In sub-Saharan Africa, malaria and anemia contribute substantially to the high burden of morbidity and mortality among under-five children. In Rwanda, both diseases have remained public health challenge over the years in spite of the numerous intervention programs and policies put in place. This study aimed at understanding the geographical variations between the joint and specific risks of ...