Open research in computer science
Spanning networks and communications to security and cryptology to big data, complexity, and analytics, SpringerOpen and BMC publish one of the leading open access portfolios in computer science. Learn about our journals and the research we publish here on this page.
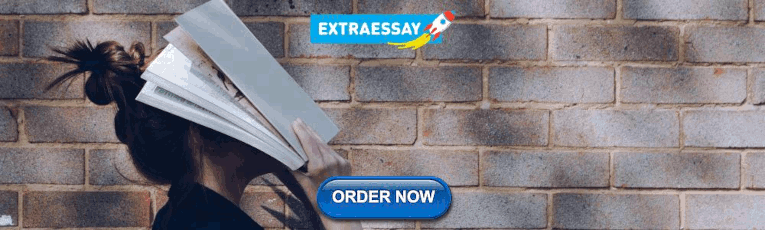
Highly-cited recent articles
Spotlight on.
EPJ Data Science
See how EPJ Data Science brings attention to data science
Reasons to publish in Human-centric Computing and Information Sciences
Download this handy infographic to see all the reasons why Human-centric Computing and Information Sciences is a great place to publish.
We've asked a few of our authors about their experience of publishing with us.
What authors say about publishing in our journals:
Fast, transparent, and fair. - EPJ Data Science Easy submission process through online portal. - Journal of Cloud Computing Patient support and constant reminder at every phase. - Journal of Cloud Computing Quick and relevant. - Journal of Big Data
How to Submit Your Manuscript
Your browser needs to have JavaScript enabled to view this video
Computer science blog posts
Read the latest from the SpringerOpen blog
The SpringerOpen blog highlights recent noteworthy research of general interest published in our open access journals.
Failed to load RSS feed.
Reference management. Clean and simple.
The top list of computer science research databases
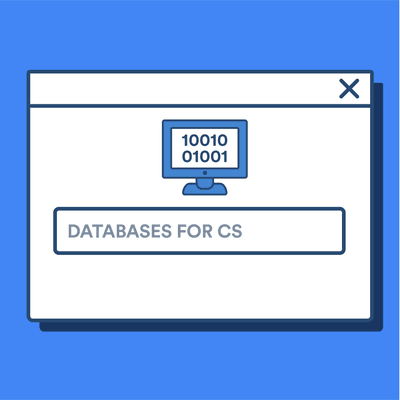
1. ACM Digital Library
2. ieee xplore digital library, 3. dblp computer science bibliography, 4. springer lecture notes in computer science (lncs), frequently asked questions about computer science research databases, related articles.
Besides the interdisciplinary research databases Web of Science and Scopus there are also academic databases specifically dedicated to computer science. We have compiled a list of the top 4 research databases with a special focus on computer science to help you find research papers, scholarly articles, and conference papers fast.
ACM Digital Library is the clear number one when it comes to academic databases for computer science. The ACM Full-Text Collection currently has 540,000+ articles, while the ACM Guide to Computing Literature holds more than 2.8+ million bibliographic entries.
- Coverage: 2.8+ million articles
- Abstracts: ✔
- Related articles: ✘
- References: ✔
- Cited by: ✔
- Full text: ✔ (requires institutional subscription)
- Export formats: BibTeX, EndNote
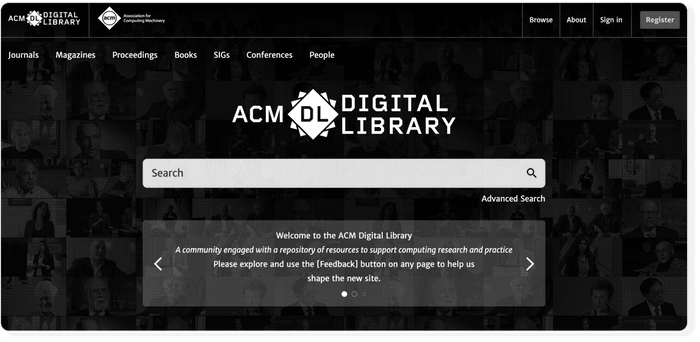
Pro tip: Use a reference manager like Paperpile to keep track of all your sources. Paperpile integrates with ACM Digital Library and many popular databases, so you can save references and PDFs directly to your library using the Paperpile buttons and later cite them in thousands of citation styles:
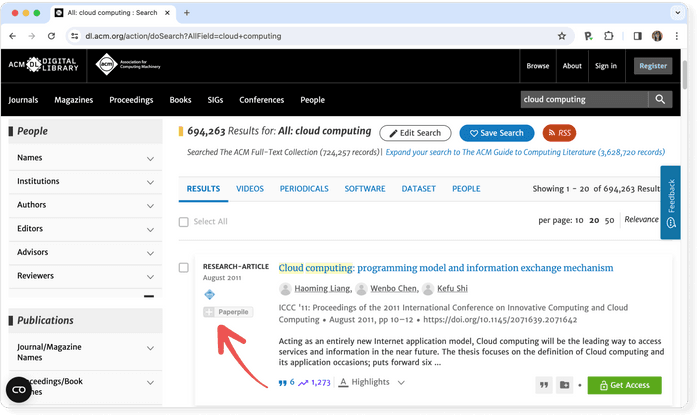
IEEE Xplore holds more than 4.7 million research articles from the fields of electrical engineering, computer science, and electronics. It not only covers articles published in scholarly journals, but also conference papers, technical standards, as well as some books.
- Coverage: 4.7+ million articles
- Export formats: BibTeX, RIS
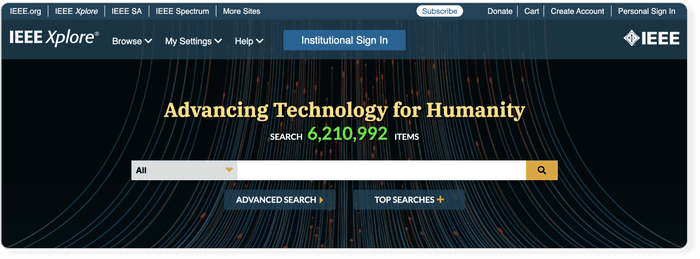
Hosted at the University of Trier, Germany, dbpl has become an indispensable resource in the field of computer science. Its index covers journal articles, conference and workshop proceedings, as well as monographs.
- Coverage: 4.3 million articles
- Abstracts: ✘
- References: ✘
- Cited by: ✘
- Full text: ✘ (Links to publisher websites available)
- Export formats: RIS, BibTeX
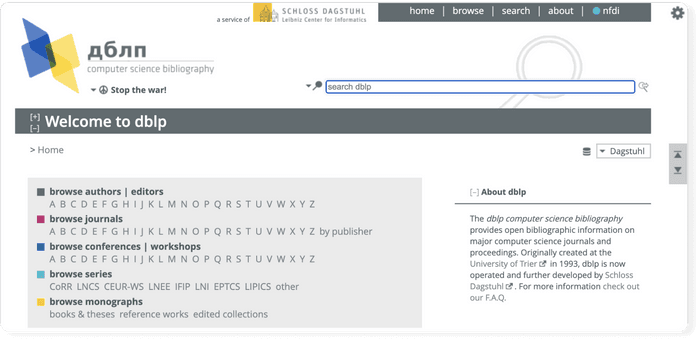
Springer's Lecture Notes in Computer Science is the number one publishing source for conference proceedings covering all areas of computer science.
- Coverage: 415,000+ articles
- Export formats: RIS, EndNote, BibTeX
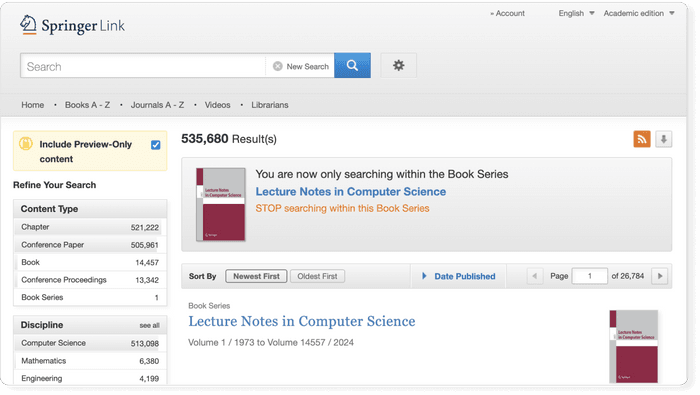
Hosted at the University of Trier, Germany, dbpl has become an indispensable resource in the field of computer science. It's index covers journal articles, conference and workshop proceedings, as well as monographs.
Microsoft Academic was a free academic search engine developed by Microsoft Research. It had more than 13.9 million articles indexed. It was shut down in 2022.
EEE Xplore holds more than 4.7 million research articles from the fields of electrical engineering, computer science, and electronics. It not only covers articles published in scholarly journals, but also conference papers, technical standards, as well as some books.
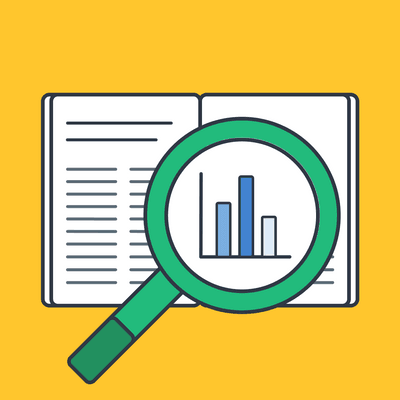
On computer science research and its temporal evolution
- Published: 30 July 2022
- Volume 127 , pages 4913–4938, ( 2022 )
Cite this article
- Camil Demetrescu ORCID: orcid.org/0000-0002-4686-6745 1 ,
- Irene Finocchi ORCID: orcid.org/0000-0002-6394-6798 2 ,
- Andrea Ribichini ORCID: orcid.org/0000-0002-0281-4257 1 &
- Marco Schaerf ORCID: orcid.org/0000-0002-2016-1966 1
627 Accesses
4 Citations
1 Altmetric
Explore all metrics
In this article, we study the evolution of the computer science research community over the past 30 years. Analyzing data from the full Scopus database, we investigate how aspects such as the community size, gender composition, and academic seniority of its members changed over time. We also shed light on the varying popularity of specific research areas, as derived from the ACM’s Special Interest Groups and IEEE classifications. Our analysis spans 19 nations (all members of the G20 group, excluding the EU) and involves a total of 728,374 authors and 8,412,543 publications. This work shows that the overall size of the computer science community has increased by a factor of ten in the time period 1991–2020, with China and India enjoying the highest growth. At the same time, this increase has not been uniform across research areas. Female participation has also increased, but more slowly than expected and not uniformly across countries and areas.
This is a preview of subscription content, log in via an institution to check access.
Access this article
Price includes VAT (Russian Federation)
Instant access to the full article PDF.
Rent this article via DeepDyve
Institutional subscriptions
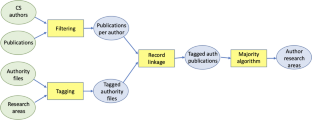
Similar content being viewed by others
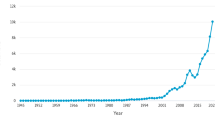
Literature reviews as independent studies: guidelines for academic practice
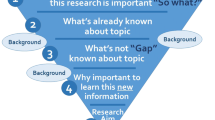
How to Write and Publish a Research Paper for a Peer-Reviewed Journal
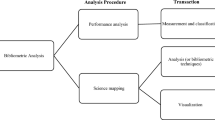
How to design bibliometric research: an overview and a framework proposal
This work uses Scopus data provided by Elsevier through ICSR Lab.
https://dblp.org/ .
Aleixandre-Benavent, R., Alonso-Arroyo, A., Chorro-Gascó, F., Alfonso-Manterola, F., González-Alcaide, G., Salvador, M., Bolaños-Pizarro, M., Areses, E., Valderrama-Zurián, J., Barón-Esquivias, G., Plaza-Celemín, L., Teresa-Galván, E., Macaya-Miguel, C., Pulpón-Rivera, L., Anguita-Sánchez, M., Pérez-Villacastín, J., Escosa-Royo, L., Martin-Burrieza, F. (2009) Cardiovascular Scientific Production in Spain and in the European and Global Context (2003-2007). Revista Espanola de Cardiologia 62 (12 2009), 1404–1417. https://doi.org/10.1016/S0300-8932(09)73126-4
Banshal, S.K., Uddin, A., & Singh, V.K. (2015) Identifying themes and trends in CS research output from India. In 2015 International Conference on Cognitive Computing and Information Processing(CCIP) (pp. 1–6). https://doi.org/10.1109/CCIP.2015.7100742
Cavero, J. M., Vela, B., & Cáceres, P. (2014). Computer science research: More production, less productivity. Scientometrics, 98 , 2103–2111. https://doi.org/10.1007/s11192-013-1178-2
Article Google Scholar
Chernysheva, N. A., Bakulina, A. A., & Bich, M. G. (2019). The new trends in the Chinese Hi-Tech industry: the evidence from Huawei. In Proceedings of the External Challenges and Risks for Russia in the Context of the World Community’s Transition to Polycentrism: Economics, Finance and Business (ICEFB 2019) . Atlantis Press (pp. 9�12). https://doi.org/10.2991/icefb-19.2019.3
Confraria, H., Godinho, M. M., & Wang, L. (2017). Determinants of citation impact: A comparative analysis of the Global South versus the Global North. Research Policy, 46 , 265–279. https://doi.org/10.1016/j.respol.2016.11.004
Courtioux, P., étivier, F., & Reberioux, A. (2019). Scientific Competition between Countries: Did China Get What It Paid for? https://halshs.archives-ouvertes.fr/halshs-02307534 Documents de travail du Centre d’Economie de la Sorbonne 2019.13.
Das, J., Do, Q.-T., Shaines, K., & Srikant, S. (2013). U.S. and them: The Geography of Academic Research. Journal of Development Economics, 105 , 112–130. https://doi.org/10.1016/j.jdeveco.2013.07.010
Demetrescu, C., Finocchi, I., Ribichini, A., & Schaerf, M. (2020). On bibliometrics in academic promotions: a case study in computer science and engineering in Italy. Scientometrics, 124 , 6. https://doi.org/10.1007/s11192-020-03548-9
Demetrescu, C., Finocchi, I., Ribichini, A., & Schaerf, M. (2022). Which conference is that? A case study in computer science. Journal of Data and Information Quality, 14 (3), 13. https://doi.org/10.1145/3519031
Demetrescu, C., Lupia, F., Mendicelli, A., Ribichini, A., Scarcello, F., & Schaerf, M. (2019). On the Shapley value and its application to the Italian VQR research assessment exercise. Journal of Informetrics, 13 , 87–104. https://doi.org/10.1016/j.joi.2018.11.008
Demetrescu, C., Ribichini, A., & Schaerf, M. (2018). Accuracy of Author Names in Bibliographic Data Sources: An Italian Case Study. Scientometrics, 11 , 1777–1791. https://doi.org/10.1007/s11192-018-2945-x
Fortnow, L. (2009). Viewpoint: Time for Computer Science to Grow Up. Communication on ACM, 52 , 33–35. https://doi.org/10.1145/1536616.1536631
Franceschini, F., & Maisano, D. (2017). Critical remarks on the Italian research assessment exercise VQR 2011–2014. Journal of Informetrics, 11 , 337–357. https://doi.org/10.1016/j.joi.2017.02.005
Glänzel, W., Schlemmer, B., Schubert, A., & Thijs, B. (2006). Proceedings literature as additional data source for bibliometric analysis. Scientometrics, 68 , 457–473. https://doi.org/10.1007/s11192-006-0124-y
Goodrum, A., McCain, K. W., Lawrence, S., & Giles, C. L. (2001). Scholarly publishing in the Internet age: A citation analysis of computer science literature. Information Processing & Management, 37 , 661–675. https://doi.org/10.1016/S0306-4573(00)00047-9
Article MATH Google Scholar
Guan, J., & Ma, N. (2004). A comparative study of research performance in computer science. Scientometrics, 61 , 339–359. https://doi.org/10.1023/b:scie.0000045114.85737.1b
Gul, S., Nisa, N., Shah, T., Gupta, S., Jan, A., & Ahmad, S. (2015). Middle East: research productivity and performance across nations. Scientometrics, 105 , 1157–1166. https://doi.org/10.1007/s11192-015-1722-3
Gupta, B. M., & Dhawan, S. (2005). Computer Science Research in India: A Scientometric Analysis of Research Output During the Period 1994-2001. DESIDOC Bulletin of Information Technology 25, 3–12. https://doi.org/10.14429/dbit.25.1.3644
He, Y., & Guan, J. (2008). Contribution of Chinese publications in computer science: A case study on LNCS. Scientometrics, 75 , 519–534. https://doi.org/10.1007/s11192-007-1781-1
Hoonlor, A., Szymanski, B. K., & Zaki, M. J. (2013). Trends in Computer Science Research. Communication on ACM, 56 , 74–83. https://doi.org/10.1145/2500892
Jaffe, K., Horst, E., Gunn, L. H., Zambrano, J. D., & Molina, G. (2020). A network analysis of research productivity by country, discipline, and wealth. PLoS ONE 15, 5 (2020). https://doi.org/10.1371/journal.pone.0232458
King, D. A. (2004). The scientific impact of nations. Nature, 430 , 311–316. https://doi.org/10.1038/430311a
Kulczycki, E. (2017). Assessing publications through a bibliometric indicator: The case of comprehensive evaluation of scientific units in Poland. Research Evaluation, 26 , 41–52. https://doi.org/10.1093/reseval/rvw023
Kumar, S., & Garg, K. (2005). Scientometrics of computer science research in India and China. Scientometrics, 64 , 121–132. https://doi.org/10.1007/s11192-005-0244-9
Leydesdorff, L., & Wagner, C. (2009). Is the United States Losing Ground in Science? A Global Perspective on the World Science System. Scientometrics, 78 , 11. https://doi.org/10.1007/s11192-008-1830-4
Liang, Z., Luo, X., Gong, F., Bao, H., Qian, H., Jia, Z., & Li, G. (2015). Worldwide Research Productivity in the Field of Arthroscopy: A Bibliometric Analysis. Arthroscopy: The Journal of Arthroscopic & Related Surgery . https://doi.org/10.1016/j.arthro.2015.03.009
Mantovani, A., Rinaldi, E., & Zusi, C. (2020). Country rankings on the scientific production in endocrinology and diabetology. Exploration of Medicine 1, 10. https://doi.org/10.37349/emed.2020.00020
Patterson, D., Snyder, L., Ullman, J. (1999). Evaluating Computer Scientists and Engineers For Promotion and Tenure. Computing Research News (September 1999). http://www.cra.org/resources/bp-view/evaluating_computer_scientists_and_engineers_for_promotion_and_tenure/
Rahman, M., & Fukui, T. (2003). Biomedical research productivity: factors across the countries. International Journal of Technology Assessment in Health Care, 19 , 249–252.
Singh, V., Uddin, A., & Pinto, D. (2015). Computer science research: The top 100 institutions in India and in the world. Scientometrics . https://doi.org/10.1007/s11192-015-1612-8
Singh, V. K., Banshal, S. K., Singhal, K., & Uddin, A. (2015). Scientometric Mapping of Research on ‘Big Data’. Scientometrics, 105 , 727–741. https://doi.org/10.1007/s11192-015-1729-9
Singhal, K., Banshal, S. K., Uddin, A., & Singh, V. K. (2015). A Scientometric analysis of computer science research in India. In 2015 Eighth International Conference on Contemporary Computing (IC3) (pp. 177–182). https://doi.org/10.1109/IC3.2015.7346675
Smith, K. M., Crookes, E., & Crookes, P. A. (2013). Measuring research ‘impact’ for academic promotion: Issues from the literature. Journal of Higher Education Policy and Management, 35 , 410–420. https://doi.org/10.1080/1360080X.2013.812173
Stuart, D. (2015). Finding “good enough’’ metrics for the UK’s Research Excellence Framework. Online Information Review, 39, 265–269.
Subramanyam, K. (1984). Research productivity and breadth of interest of computer scientists. Journal of the American Society for Information Science, 3 , 369–371. https://doi.org/10.1002/asi.4630350609
Uddin, A., Singh, V., Pinto, D., & Olmos, I. (2015). Scientometric mapping of computer science research in Mexico. Scientometrics . https://doi.org/10.1007/s11192-015-1654-y
Vardi, M. Y. (2009). Conferences vs. Journals in Computing Research. Communication on ACM 52, 5. https://doi.org/10.1145/1506409.1506410
Vrettas, G., & Sanderson, M. (2015). Conferences versus Journals in Computer Science. Journal of the Association for Information Science and Technology, 66 , 2674–2684. https://doi.org/10.1002/asi.23349
Wang, L. (2016). The structure and comparative advantages of China’s scientific research: quantitative and qualitative perspectives. Scientometrics, 106 , 435–452. https://doi.org/10.1007/s11192-015-1650-2
Zhang, J., Chen, X., Gao, X., Yang, H., Zhen, Z., Li, Y. L., & Zhao, X. (2017). Worldwide research productivity in the field of psychiatry. International Journal of Mental Health Systems . https://doi.org/10.1186/s13033-017-0127-5
Zhou, P., & Leydesdorff, L. (2006). The emergence of China as a leading nation in science. Research Policy, 35 , 83–104. https://doi.org/10.1016/j.respol.2005.08.006
Download references
Prof. Demetrescu, Prof. Finocchi and Dr. Ribichini were partially supported for this work by MIUR, the Italian Ministry of Education, University and Research, under PRIN Project n. 20174LF3T8 AHeAD (Efficient Algorithms for HArnessing Networked Data).
Author information
Authors and affiliations.
Department of Computer, Control, and Management Engineering “Antonio Ruberti”, Sapienza University of Rome, Via Ariosto 25, 00185, Rome, Italy
Camil Demetrescu, Andrea Ribichini & Marco Schaerf
Department of Business and Management, Luiss Guido Carli University, Viale Romania 32, 00197, Rome, Italy
Irene Finocchi
You can also search for this author in PubMed Google Scholar
Corresponding author
Correspondence to Andrea Ribichini .
Ethics declarations
Conflict of interest.
The authors have no conflicts of interest to declare.
Additional information
Publisher's note.
Springer Nature remains neutral with regard to jurisdictional claims in published maps and institutional affiliations.
Rights and permissions
Reprints and permissions
About this article
Demetrescu, C., Finocchi, I., Ribichini, A. et al. On computer science research and its temporal evolution. Scientometrics 127 , 4913–4938 (2022). https://doi.org/10.1007/s11192-022-04445-z
Download citation
Received : 08 January 2022
Accepted : 17 June 2022
Published : 30 July 2022
Issue Date : August 2022
DOI : https://doi.org/10.1007/s11192-022-04445-z
Share this article
Anyone you share the following link with will be able to read this content:
Sorry, a shareable link is not currently available for this article.
Provided by the Springer Nature SharedIt content-sharing initiative
- Computer science research
- Temporal evolution
- Gender gap in computer science
- National scientific productivity
- Research areas
- Find a journal
- Publish with us
- Track your research
computer science Recently Published Documents
Total documents.
- Latest Documents
- Most Cited Documents
- Contributed Authors
- Related Sources
- Related Keywords
Hiring CS Graduates: What We Learned from Employers
Computer science ( CS ) majors are in high demand and account for a large part of national computer and information technology job market applicants. Employment in this sector is projected to grow 12% between 2018 and 2028, which is faster than the average of all other occupations. Published data are available on traditional non-computer science-specific hiring processes. However, the hiring process for CS majors may be different. It is critical to have up-to-date information on questions such as “what positions are in high demand for CS majors?,” “what is a typical hiring process?,” and “what do employers say they look for when hiring CS graduates?” This article discusses the analysis of a survey of 218 recruiters hiring CS graduates in the United States. We used Atlas.ti to analyze qualitative survey data and report the results on what positions are in the highest demand, the hiring process, and the resume review process. Our study revealed that a software developer was the most common job the recruiters were looking to fill. We found that the hiring process steps for CS graduates are generally aligned with traditional hiring steps, with an additional emphasis on technical and coding tests. Recruiters reported that their hiring choices were based on reviewing resume’s experience, GPA, and projects sections. The results provide insights into the hiring process, decision making, resume analysis, and some discrepancies between current undergraduate CS program outcomes and employers’ expectations.
A Systematic Literature Review of Empiricism and Norms of Reporting in Computing Education Research Literature
Context. Computing Education Research (CER) is critical to help the computing education community and policy makers support the increasing population of students who need to learn computing skills for future careers. For a community to systematically advance knowledge about a topic, the members must be able to understand published work thoroughly enough to perform replications, conduct meta-analyses, and build theories. There is a need to understand whether published research allows the CER community to systematically advance knowledge and build theories. Objectives. The goal of this study is to characterize the reporting of empiricism in Computing Education Research literature by identifying whether publications include content necessary for researchers to perform replications, meta-analyses, and theory building. We answer three research questions related to this goal: (RQ1) What percentage of papers in CER venues have some form of empirical evaluation? (RQ2) Of the papers that have empirical evaluation, what are the characteristics of the empirical evaluation? (RQ3) Of the papers that have empirical evaluation, do they follow norms (both for inclusion and for labeling of information needed for replication, meta-analysis, and, eventually, theory-building) for reporting empirical work? Methods. We conducted a systematic literature review of the 2014 and 2015 proceedings or issues of five CER venues: Technical Symposium on Computer Science Education (SIGCSE TS), International Symposium on Computing Education Research (ICER), Conference on Innovation and Technology in Computer Science Education (ITiCSE), ACM Transactions on Computing Education (TOCE), and Computer Science Education (CSE). We developed and applied the CER Empiricism Assessment Rubric to the 427 papers accepted and published at these venues over 2014 and 2015. Two people evaluated each paper using the Base Rubric for characterizing the paper. An individual person applied the other rubrics to characterize the norms of reporting, as appropriate for the paper type. Any discrepancies or questions were discussed between multiple reviewers to resolve. Results. We found that over 80% of papers accepted across all five venues had some form of empirical evaluation. Quantitative evaluation methods were the most frequently reported. Papers most frequently reported results on interventions around pedagogical techniques, curriculum, community, or tools. There was a split in papers that had some type of comparison between an intervention and some other dataset or baseline. Most papers reported related work, following the expectations for doing so in the SIGCSE and CER community. However, many papers were lacking properly reported research objectives, goals, research questions, or hypotheses; description of participants; study design; data collection; and threats to validity. These results align with prior surveys of the CER literature. Conclusions. CER authors are contributing empirical results to the literature; however, not all norms for reporting are met. We encourage authors to provide clear, labeled details about their work so readers can use the study methodologies and results for replications and meta-analyses. As our community grows, our reporting of CER should mature to help establish computing education theory to support the next generation of computing learners.
Light Diacritic Restoration to Disambiguate Homographs in Modern Arabic Texts
Diacritic restoration (also known as diacritization or vowelization) is the process of inserting the correct diacritical markings into a text. Modern Arabic is typically written without diacritics, e.g., newspapers. This lack of diacritical markings often causes ambiguity, and though natives are adept at resolving, there are times they may fail. Diacritic restoration is a classical problem in computer science. Still, as most of the works tackle the full (heavy) diacritization of text, we, however, are interested in diacritizing the text using a fewer number of diacritics. Studies have shown that a fully diacritized text is visually displeasing and slows down the reading. This article proposes a system to diacritize homographs using the least number of diacritics, thus the name “light.” There is a large class of words that fall under the homograph category, and we will be dealing with the class of words that share the spelling but not the meaning. With fewer diacritics, we do not expect any effect on reading speed, while eye strain is reduced. The system contains morphological analyzer and context similarities. The morphological analyzer is used to generate all word candidates for diacritics. Then, through a statistical approach and context similarities, we resolve the homographs. Experimentally, the system shows very promising results, and our best accuracy is 85.6%.
A genre-based analysis of questions and comments in Q&A sessions after conference paper presentations in computer science
Gender diversity in computer science at a large public r1 research university: reporting on a self-study.
With the number of jobs in computer occupations on the rise, there is a greater need for computer science (CS) graduates than ever. At the same time, most CS departments across the country are only seeing 25–30% of women students in their classes, meaning that we are failing to draw interest from a large portion of the population. In this work, we explore the gender gap in CS at Rutgers University–New Brunswick, a large public R1 research university, using three data sets that span thousands of students across six academic years. Specifically, we combine these data sets to study the gender gaps in four core CS courses and explore the correlation of several factors with retention and the impact of these factors on changes to the gender gap as students proceed through the CS courses toward completing the CS major. For example, we find that a significant percentage of women students taking the introductory CS1 course for majors do not intend to major in CS, which may be a contributing factor to a large increase in the gender gap immediately after CS1. This finding implies that part of the retention task is attracting these women students to further explore the major. Results from our study include both novel findings and findings that are consistent with known challenges for increasing gender diversity in CS. In both cases, we provide extensive quantitative data in support of the findings.
Designing for Student-Directedness: How K–12 Teachers Utilize Peers to Support Projects
Student-directed projects—projects in which students have individual control over what they create and how to create it—are a promising practice for supporting the development of conceptual understanding and personal interest in K–12 computer science classrooms. In this article, we explore a central (and perhaps counterintuitive) design principle identified by a group of K–12 computer science teachers who support student-directed projects in their classrooms: in order for students to develop their own ideas and determine how to pursue them, students must have opportunities to engage with other students’ work. In this qualitative study, we investigated the instructional practices of 25 K–12 teachers using a series of in-depth, semi-structured interviews to develop understandings of how they used peer work to support student-directed projects in their classrooms. Teachers described supporting their students in navigating three stages of project development: generating ideas, pursuing ideas, and presenting ideas. For each of these three stages, teachers considered multiple factors to encourage engagement with peer work in their classrooms, including the quality and completeness of shared work and the modes of interaction with the work. We discuss how this pedagogical approach offers students new relationships to their own learning, to their peers, and to their teachers and communicates important messages to students about their own competence and agency, potentially contributing to aims within computer science for broadening participation.
Creativity in CS1: A Literature Review
Computer science is a fast-growing field in today’s digitized age, and working in this industry often requires creativity and innovative thought. An issue within computer science education, however, is that large introductory programming courses often involve little opportunity for creative thinking within coursework. The undergraduate introductory programming course (CS1) is notorious for its poor student performance and retention rates across multiple institutions. Integrating opportunities for creative thinking may help combat this issue by adding a personal touch to course content, which could allow beginner CS students to better relate to the abstract world of programming. Research on the role of creativity in computer science education (CSE) is an interesting area with a lot of room for exploration due to the complexity of the phenomenon of creativity as well as the CSE research field being fairly new compared to some other education fields where this topic has been more closely explored. To contribute to this area of research, this article provides a literature review exploring the concept of creativity as relevant to computer science education and CS1 in particular. Based on the review of the literature, we conclude creativity is an essential component to computer science, and the type of creativity that computer science requires is in fact, a teachable skill through the use of various tools and strategies. These strategies include the integration of open-ended assignments, large collaborative projects, learning by teaching, multimedia projects, small creative computational exercises, game development projects, digitally produced art, robotics, digital story-telling, music manipulation, and project-based learning. Research on each of these strategies and their effects on student experiences within CS1 is discussed in this review. Last, six main components of creativity-enhancing activities are identified based on the studies about incorporating creativity into CS1. These components are as follows: Collaboration, Relevance, Autonomy, Ownership, Hands-On Learning, and Visual Feedback. The purpose of this article is to contribute to computer science educators’ understanding of how creativity is best understood in the context of computer science education and explore practical applications of creativity theory in CS1 classrooms. This is an important collection of information for restructuring aspects of future introductory programming courses in creative, innovative ways that benefit student learning.
CATS: Customizable Abstractive Topic-based Summarization
Neural sequence-to-sequence models are the state-of-the-art approach used in abstractive summarization of textual documents, useful for producing condensed versions of source text narratives without being restricted to using only words from the original text. Despite the advances in abstractive summarization, custom generation of summaries (e.g., towards a user’s preference) remains unexplored. In this article, we present CATS, an abstractive neural summarization model that summarizes content in a sequence-to-sequence fashion while also introducing a new mechanism to control the underlying latent topic distribution of the produced summaries. We empirically illustrate the efficacy of our model in producing customized summaries and present findings that facilitate the design of such systems. We use the well-known CNN/DailyMail dataset to evaluate our model. Furthermore, we present a transfer-learning method and demonstrate the effectiveness of our approach in a low resource setting, i.e., abstractive summarization of meetings minutes, where combining the main available meetings’ transcripts datasets, AMI and International Computer Science Institute(ICSI) , results in merely a few hundred training documents.
Exploring students’ and lecturers’ views on collaboration and cooperation in computer science courses - a qualitative analysis
Factors affecting student educational choices regarding oer material in computer science, export citation format, share document.
University Library, University of Illinois at Urbana-Champaign

Computer Science Research Resources: Find Articles & Papers
- Find Articles & Papers
- High-Impact Journals
- Standards & Technical Reports
- Patents & Government Documents
- E-Books & Reference
- Dissertations & Theses
- Additional Resources
Engineering Easy Search
University library search engines.
- Grainger Engineering Library Homepage With specialized searches for Engineering and the Physical Sciences.
- Easy Search The easiest way to locate University Library resources, materials, and more!
- Find Online Journals Search by title or by subject to view our subscription details, including date ranges and where you can access full text.
- Journal and Article Locator Finds electronic or print copy of articles by using a citation.
Engineering Article Databases
- Engineering Village This link opens in a new window Search for articles, conference paper, and report information in all areas of engineering. Full-text is often available through direct download.
- Scopus This link opens in a new window Search periodicals, conference proceedings, technical reports, trade literature, patents, books, and press releases in all engineering fields. Some full-text available as direct downloads.
- Web of Science (Core Collection) This link opens in a new window Search for articles in science and engineering. Also provides Science Citation Index that tracks citations in science and technical journals published since 1981. Journal Citation Reports are also available through ISI.
Computer Science Article Databases
- ACM Digital Library This link opens in a new window This site provides access to tables of contents, abstracts, reviews, and full text of every article ever published by ACM and bibliograhic citations from major publishers in computing.
- Compendex This link opens in a new window Compendex is the most comprehensive bibliographic database of scientific and technical engineering research available, covering all engineering disciplines. It includes millions of bibliographic citations and abstracts from thousands of engineering journals and conference proceedings. When combined with the Engineering Index Backfile (1884-1969), Compendex covers well over 120 years of core engineering literature.
- IEEE Xplore This link opens in a new window Provides full-text access to IEEE transactions, IEEE and IEE journals, magazines, and conference proceedings published since 1988, and all current IEEE standards; brings additional search and access features to IEEE/IEE digital library users. Browsable by books & e-books, conference publications, education and learning, journals and magazines, standards and by topic. Also provides links to IEEE standards, IEEE spectrum and other sites.
Subject Guide

Ask a Librarian
- Next: High-Impact Journals >>
- Last Updated: Jun 16, 2023 9:35 AM
- URL: https://guides.library.illinois.edu/cs
Penn State University Libraries
Computer science and engineering.
- Reference Sources
- Finding Articles and Databases
- Finding Books
- Finding Websites
- Penn State Resources and Organizations
- Books, Articles, and Other Educational Resources
- Research Tips
- Main Parts of a Scientific/Technical Paper
- Technical Writing Resources
- Ten Tips for Technical Writing
- Professional Organizations
Parts of a Technical Paper
The basic parts of a scientific or technical paper are:
Title and Author Information Abstract Introduction Literature Review Methods Results Discussion Conclusions References and Appendices
Detailed Explanation for Each Part
Title and Author Information:
The title of your paper and any needed information about yourself (usually your name and institution).
A short (usually around 250-400 words) description of the paper. Should include what the purpose of the paper is (including the basic research question/problem), the basic design of your project, and the major findings.
Introduction:
A general introduction to your topic and what you expect to learn from your project or experiment. Your research question should be found here.
Literature Review:
An analysis of what has already been published about your chosen topic. Should be able to show how your research question fits into the context of your field.
A description of everything you did in your experiment or project, step-by-step. Needs to be detailed enough so that any reader would be able to repeat each step exactly on their own.
What actually happened during your project or what you found at the end of your experiment. This is usually the best part to include the majority of your graphs, photos, tables, and other visual aids, as long as they help explain the results of your work.
Discussion:
An analysis of the results that integrates what you found into the wider body of research in your field. Can also include future hypotheses to be tested or future projects to build from your own.
Conclusion:
Can be included in the discussion if necessary. A final summary of the paper, including whether or not you were able to answer your original research question.
References and Appendices:
The reference page(s) is a list of all the sources you used to research and create your project/experiment, including everything cited in the literature review and methods sections. Remember to use the same citation style throughout the paper. An appendix would include any additional information about your work that you were not able to include within the body of your paper (like large datasets and figures) that would help readers better understand your results.
- << Previous: Technical Writing
- Next: Technical Writing Resources >>
- Last Updated: Mar 1, 2024 1:52 PM
- URL: https://guides.libraries.psu.edu/compsciandengin
Computer Science
Overview - library research for computer science, quick start, need more help, tutorial: understanding journals, literature searches, what is libkey nomad, library article databases, browse journals using browzine, find computer science books, computer science books you can check out or read online, e-book collections, e-reference sources, find web sources, associations, organizations, & societies, interesting websites, find journals, finding a specific journal, browsing journals using browzine, interlibrary loan, library research for computer science.
This guide will help you get started searching the computer science "literature" for research papers on your topic and finding other resources such as books, journals, government websites, and published theses and dissertations.
Be sure to visit the different pages of this guide using the tabs on the left.
Library Search connects you to books, articles, and a variety of other library sources.
Library Search at Rowan University Libraries
Schedule an appointment with me:
- Request a Research Consultation
- Understanding Journals tutorial A short online tutorial for students on how to understand and use library journals.
Computer Science Articles
We recommend that you use the specialized subscription databases on the library website to search for engineering "literature" (papers) because you will see only content that the library subscribes to.
However if you prefer to use Google Scholar, you can create a Google Scholar profile with your Rowan University credentials , which will give you access to the library's subscription content.
Then when you search in Google Scholar, you will see links to the right of some results labeled Full Text @Rowan University . Selecting one of these links will take you to the subscribed content.

LibKey Nomad is a browser extension for the Chrome, Firefox, and Edge web browsers.
Once you install it, you can use it to download full text articles to which we subscribe, from any publisher website where you find them. (Otherwise you would need to go to the library website to access the full text if you are off campus.)

When the full text PDF is available to you, you will see a LibKey Download PDF icon in the bottom left corner of the page.
If it is not, you will see a button labeled Access Options which will direct you to our Interlibrary Loan system.
Watch the short video shown below to learn how to download the extension for the browser you use.
- LibKey Nomad Installation Video showing you how to install LibKey Nomad
The following subscription databases available through the library website contain scholarly articles in Computer Science.
- ACM Digital Library This link opens in a new window Academic journal articles in computer science. more... less... Full text of every article ever published by ACM (the professional organization for computer scientists) and bibliographic citations from major publishers in computing. (WALDO consortial access.)
- IEEE Xplore This link opens in a new window Journal and conference papers in electrical engineering. more... less... Full text access to journals and major conference proceedings published by the Institute of Electrical and Electronic Engineers (IEEE).
- MathSciNet This link opens in a new window The American Mathematical Society’s index to mathematical literature. more... less... Contains references, abstracts, and reviews from mathematical journals, conference proceedings, books, and dissertations.
- Web of Science This link opens in a new window Full text database of scientific journal articles with data on who has cited them. more... less... Web of Science® provides immediate data on who has cited research papers, covering over 12,000 of the highest impact journals worldwide, including Open Access journals and over 150,000 conference proceedings. You'll find current and retrospective coverage in the sciences, social sciences, arts, and humanities, with coverage to 1900.
- Scopus This link opens in a new window SciVerse Scopus is the world’s largest abstract and citation database of peer-reviewed literature and quality web sources.
- SIAM Journals Online Collection of journals published by the Society for Industrial and Applied Mathematics. more... less... Rowan account required.
Computer Science Books
This page explains ways to find computer science books through the library.
Computer science books are located in the QA76 section on the 4th floor of Campbell Library. Most programming books are in QA76 but some are in the TK (computer engineering) section.
- LibrarySearch Use Library Search to look up books by topic or title. Use the Books facet.
- Ebook Central ebook collection This link opens in a new window Collection of scholarly e-books in many academic disciplines. more... less... Multidisciplinary collection of scholarly ebooks, offering a strong collection of academic titles from leading scholarly publishers. Includes subscribed and purchased content. It is not a permanent acquisition of e-books, and is subject to change.
These subscription sources are available through the library website.
- Credo Reference - Science An online database which includes many science dictionaries and encyclopedias.
Computer Science Websites
This page highlights good websites for computer science research.
- American Association for Artificial Intelligence more... less... The AAAI, founded in 1979, is a scientific society devoted to advancing the scientific understanding of the mechanisms underlying thought and intelligent behavior and their embodiment in machines. Their site contains information on artificial intelligence, AAAI publications (book, journals, conference proceedings, and technical papers); conference, workshop, and symposia information; and membership benefits.
- ANSI standards search
- Association for Computing Machinery more... less... Billing itself as "the first society in computing," the ACM is the world's first educational and scientific computing society. Founded in 1947, its membership currently totals over 80,000 computing professionals and students world wide. The site includes information about ACM activities, services, conferences, publications, and policies. The ACM Digital Library contains full text of articles and papers from all of the their journals, magazines, and proceedings.
- IEEE Computer Society more... less... Founded in 1947, the IEEE Computer Society is the world's oldest and largest (98,000 members) professional association of people in computing. The site contains a full range of information about conferences, standards, publications, activities, education, certification, and employment. The Digital Library provides access to the Computer Society's magazines, transactions, and a growing body of conference proceedings.
- Society for Industrial and Applied Mathematics more... less... A group of professionals formed SIAM in 1952 to advance the application of mathematics to science and industry. SIAM members are computer scientists, mathematicians, engineers, statisticians, and engineers. This site includes information about their publications, conferences, meetings.
- Special Interest Group on Human-Computer Interaction
- ArXiv Pre-print repository of articles in Physics, Mathematics, and Computer Science maintained by Cornell University.
- Software Engineering Institute SEI is a federally-funded research and development center headquartered on the campus of Carnegie Mellon University.
- HCI Bibliography A site offering a bibliography of human-computer interaction resources.
- How to Get a Computer Science Job Commercial website with advice on getting your first professional job in computer science.
Computer Science Journals
This page offers several options for finding scientific journals containing research on computer science.
To discover whether the library subscribes to a specific journal, and see content in that journal, go to the Search Tools section of the Campbell Library home page. Click on the Journal Finder option.
Enter the complete name of the journal (no abbreviations) - for example, "Environmental & Engineering Geoscience."
If the journal is listed in the results, click on Available Online . Then scroll down to View Online and choose a database link. In this example there should be a link for the database GeoScienceWorld .
If you are looking for a specific issue of the journal, there is usually a way to browse volumes and issues to drill down to the desired issue.
Or, search within the journal using the Search box in the top right corner to find articles on a topic.
Journal articles to which we do not have full text access may be requested through our online Interlibrary Loan request system, Illiad. Articles are usually sent to your email address within a few days.
- Campbell Library Interlibrary Loan logon
- Last Updated: May 16, 2024 1:45 PM
- URL: https://libguides.rowan.edu/ComputerScience
University of Illinois Chicago
University library, search uic library collections.
Find items in UIC Library collections, including books, articles, databases and more.
Advanced Search
Search UIC Library Website
Find items on the UIC Library website, including research guides, help articles, events and website pages.
- Search Collections
- Search Website
- UIC Library
- Subject and Course Guides
- Computer Science
How to Read a Scientific Paper
Computer science: how to read a scientific paper.
- Research Databases
- Managing Your Data
- Journal and Conference Rankings
- Writing Help
Structure of a Technical Paper
- How to Read a Paper A short work on how to read academic papers, organized as an academic paper. Some of the advice on doing a literature survey works better in the author's field (CS) but most the material works for everyone.
- How to Read a Research Paper Part of an assignment on how to read academic papers for a CS class, it describes some strategies and lays out some expectations in terms of time and effort that should be useful.
- How to Read Scientific Papers Without Reading Every Word A blog post that gives a similar but differently worded take on the same issue.
- How to read Mathematics An article discussing how to go about reading a math article or chapter.

Health Promotion Practice. (2020). How to Read a Scholarly Article [Infographic].
http://healthpromotionpracticenotes.com/2020/07/new- tool-how-to-read-a-scholarly-article-infographic/
Assistant Professor and Reference & Liaison Librarian (STEM)
- << Previous: Managing Your Data
- Next: Journal and Conference Rankings >>
- Last Updated: Apr 15, 2024 2:56 PM
- URL: https://researchguides.uic.edu/computerscience
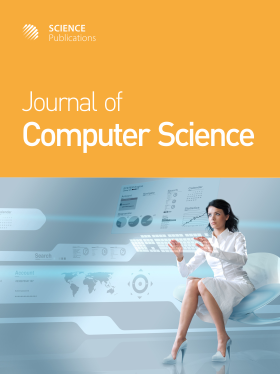
Journal of Computer Science
Aims and scope.
The Journal of Computer Science (JCS) is dedicated to advancing computer science by publishing high-quality research and review articles that span both theoretical foundations and practical applications in information, computation, and computer systems. With a commitment to excellence, JCS offers a platform for researchers, scholars, and industry professionals to share their insights and contribute to the ongoing evolution of computer science. Published on a monthly basis, JCS provides up-to-date insights into this ever-evolving discipline.
Science Publications is pleased to announce the launch of a new open access journal, Journal of Adaptive Structures. JAS brings together emerging technologies for adaptive smart structures, including advanced materials, smart actuation, sensing and control, to pursue the progressive adoption of the major scientific achievements in this multidisciplinary field on-board of commercial aircraft.
It is with great pleasure that we announce the SGAMR Annual Awards 2020. This award is given annually to Researchers and Reviewers of International Journal of Structural Glass and Advanced Materials Research (SGAMR) who have shown innovative contributions and promising research as well as others who have excelled in their Editorial duties.
This special issue "Neuroinflammation and COVID-19" aims to provide a space for debate in the face of the growing evidence on the affectation of the nervous system by COVID-19, supported by original studies and case series.
- Recently Published
- Most Viewed
- Most Downloaded
SCIgen - An Automatic CS Paper Generator
About Generate Examples Talks Code Donations Related People Blog
Generate a Random Paper
Related Work
- We initially based SCIgen on Chris Coyne's grammar for high school papers; Chris is now making neat pictures with context-free grammars.
May 16, 2024
How New Science Fiction Could Help Us Improve AI
We need to tell a new story about AI, and fiction has that power, humanities scholars say
By Nick Hilden
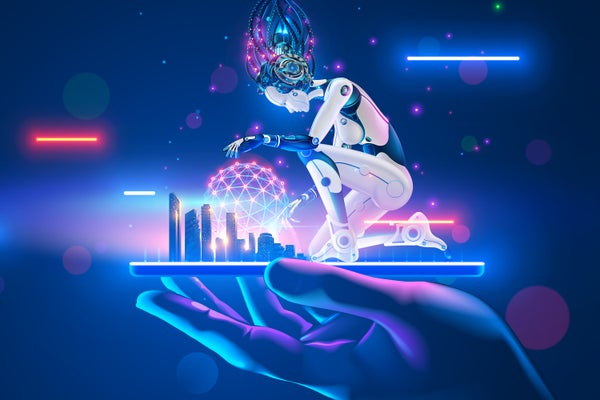
Andrey Suslov/Getty Images
For the past decade, a group called the Future of Life Institute has been campaigning for human welfare in public conversations around nuclear weapons, climate change, artificial intelligence and other evolving threats. The nonprofit organization aims to steer technological development away from the dystopian visions that so frequently haunt media. But when it comes to discussions about artificial intelligence, its team has had to face one especially persistent foe: the Terminator .
“When we first started talking about AI risk, every article that came out about our work had a Terminator in it,” says Emilia Javorsky, director of the institute’s Futures program. The Terminator film franchise’s specter of a powerful and antagonistic robot that is driven only by ruthless logic is hard to dispel. Ask people to imagine a powerful artificial intelligence, and they tend to think of the fictional archetype of a machine with a “Machiavellian soul,” Javorsky adds—even though actual AI systems inherently “have no malevolence, no human intent to them whatsoever.”
Recognizing the influence that popular narratives have on our collective perceptions, a growing number of AI and computer science experts now want to harness fiction to help imagine futures in which algorithms don’t destroy the planet. The arts and humanities, they argue, must play a role to ensure AI serves human goals. To that end, Nina Beguš, an AI researcher at the University of California, Berkeley, advocates for a new discipline that she calls the “artificial humanities.” In her upcoming book Artificial Humanities: A Fictional Perspective on Language in AI, she contends that the “responsibility of making these technologies is too big for the technologists to bear it alone.” The artificial humanities, she explains, would fuse science and the arts to leverage fiction and philosophy in the exploration of AI’s benevolent potential.
On supporting science journalism
If you're enjoying this article, consider supporting our award-winning journalism by subscribing . By purchasing a subscription you are helping to ensure the future of impactful stories about the discoveries and ideas shaping our world today.
“The humanities simply have to be part of the conversation, or this new world advances without our input,” says cultural historian Catherine Clarke of the University of London, who has studied the intersection of literature and AI.
Entertainment strongly shapes people’s perceptions of AI, as a recent public opinion study by researchers at the University of Texas at Austin shows. These depictions, however, frequently ignore positive technological potential in favor of portraying our worst fears. “We need fictional works that consider machines for what they are and articulate what their intelligence and creativity could be,” Beguš says. And because fiction is “not obliged to mirror actual technological developments,” it can be a “public space for experimentation and reflection.”
Importantly, it also turns out that our entertainment-fueled negative impressions of AI can, in turn, influence how the technology performs in the real world; the stories we tell ourselves about AI prime us to use it in certain ways. Preconceptions that an AI chatbot will answer like a manipulative machine initiate a hostile feedback loop so that the bot acts as expected, according to a recent study by researchers at the Massachusetts Institute of Technology Media Lab. A user’s internalized fears can be self-fulfilling, seasoning an algorithm with adversarial ingredients. So it may be that if fiction trains us to expect the worst from AI, that’s exactly what we’ll get.
But if we treat AI models with some finesse, they will respond in kind. Clarke, along with Murray Shanahan of Imperial College London and Google DeepMind, recently sought to determine whether a text-generating AI could be coached to deliver human-quality prose. They provided the beginning of a story to a chatbot and used prompts of varying detail and complexity to ask it to complete the narrative. As their preprint results found, stories composed by an AI that was given crude prompts fell flat, but more elegant and creatively refined prompts led to more literary prose. This suggests that what we give to a generative AI is returned to us.
“Why do we always imagine science fiction to be a dystopia? Why can’t we imagine science fiction that gives us hope?” — Pat Pataranutaporn, M.I.T. Media Lab
If these patterns hold true for more intelligent forms of AI, we need to instill them with scruples before we flip their “on” switches. The University of Oxford’s AI doomsayer Nick Bostrom has called this need “philosophy with a deadline.”
To pull more artists and thinkers into that discussion, the Future of Life Institute has sponsored multiple initiatives linking fiction writers and other creatives with technologists. “You can't mitigate risks that you can’t imagine,” Javorsky says. “You also can’t build positive futures with technology and steer toward those if you’re not imagining them.” The institute’s Worldbuilding Competition , for example, brings together multidisciplinary teams to conceptualize various friendly-AI futures. Those imagined tomorrows include a world in which a centralized AI manages the equitable distribution of goods. A second scenario suggests a system of digital nations that are free of geographic bounds. In yet another, artificial governance programs advocate for peace. In a fourth, AI helps us achieve a more inclusive society .
Merely imagining such worlds, where growth and innovation no longer depend on conventional human labor, allows fiction writers and other thinkers to ask provocative questions, Javorsky says: “What does meaning look like? What does aspiration look like? How do we rethink human purpose and agency in a world of shared abundance?”
The Future of Life Institute has also joined forces with an organization called Hollywood, Health & Society and other organizations to form the Blue Sky Scriptwriting Contest , which awards writers for creating television scripts that depict fair and equitable applications for artificial intelligence.
“We’ve all seen lots of dystopian and postapocalyptic futures in popular entertainment,” says Hollywood, Health & Society’s program director Kate Langrall Folb. There are “very few depictions of a greener, safer, more just future.” The inaugural contest was held in 2022, with prizes awarded last year. In that competition, the winning entry was set in a town where AI equally serves the needs of all residents, who are shaken when a once-in-a-generation murder complicates their potential techno-utopia. In another, AI-powered advisers equipped with Indigenous wisdom support a more sustainable society. Another tells of an Earth where AI has moved all manufacturing and heavy infrastructure off-planet, regenerating the terrestrial ecosystems below.
To further inspire these lines of thinking, the Future of Life Institute is in the process of producing a free, publicly available “Worldbuilding” course to train participants in hope rather than doom when it comes to AI. And once a person has managed to escape the doom loop, Javorsky says, it can be difficult to know where to direct efforts at developing positive AI. To address this, the institute is developing detailed scenario maps that suggest where different trajectories and decision points could lead this technology over the long run. The intention is to bring these scenarios to creative, artistic people who will then flesh out these stories, pursuing the crossover between technology and creativity—and providing AI developers with ideas about where different courses of action may take us.
This moment desperately needs “the power of storytelling and the humanities,” Javorsky says, to steer people away from the Terminator and toward a future where they’d be excited to live alongside AI—in peace and felicity.
“We need to come up with a new story,” says Pat Pataranutaporn, a researcher at the M.I.T. Media Lab and a co-author of the study on AI user preconceptions. “Why do we always imagine science fiction to be a dystopia? Why can’t we imagine science fiction that gives us hope?”
Thank you for visiting nature.com. You are using a browser version with limited support for CSS. To obtain the best experience, we recommend you use a more up to date browser (or turn off compatibility mode in Internet Explorer). In the meantime, to ensure continued support, we are displaying the site without styles and JavaScript.
- View all journals
- Explore content
- About the journal
- Publish with us
- Sign up for alerts
- Published: 02 August 2023
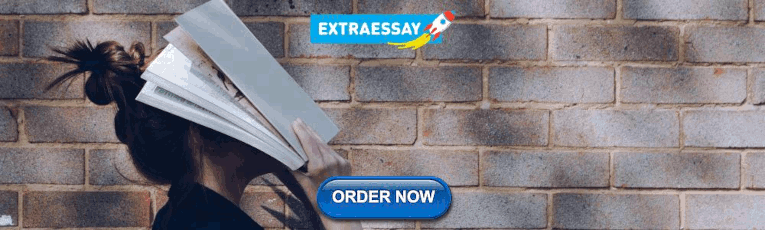
Scientific discovery in the age of artificial intelligence
- Hanchen Wang ORCID: orcid.org/0000-0002-1691-024X 1 , 2 na1 nAff37 nAff38 ,
- Tianfan Fu 3 na1 ,
- Yuanqi Du 4 na1 ,
- Wenhao Gao 5 ,
- Kexin Huang 6 ,
- Ziming Liu 7 ,
- Payal Chandak ORCID: orcid.org/0000-0003-1097-803X 8 ,
- Shengchao Liu ORCID: orcid.org/0000-0003-2030-2367 9 , 10 ,
- Peter Van Katwyk ORCID: orcid.org/0000-0002-3512-0665 11 , 12 ,
- Andreea Deac 9 , 10 ,
- Anima Anandkumar 2 , 13 ,
- Karianne Bergen 11 , 12 ,
- Carla P. Gomes ORCID: orcid.org/0000-0002-4441-7225 4 ,
- Shirley Ho 14 , 15 , 16 , 17 ,
- Pushmeet Kohli ORCID: orcid.org/0000-0002-7466-7997 18 ,
- Joan Lasenby 1 ,
- Jure Leskovec ORCID: orcid.org/0000-0002-5411-923X 6 ,
- Tie-Yan Liu 19 ,
- Arjun Manrai 20 ,
- Debora Marks ORCID: orcid.org/0000-0001-9388-2281 21 , 22 ,
- Bharath Ramsundar 23 ,
- Le Song 24 , 25 ,
- Jimeng Sun 26 ,
- Jian Tang 9 , 27 , 28 ,
- Petar Veličković 18 , 29 ,
- Max Welling 30 , 31 ,
- Linfeng Zhang 32 , 33 ,
- Connor W. Coley ORCID: orcid.org/0000-0002-8271-8723 5 , 34 ,
- Yoshua Bengio ORCID: orcid.org/0000-0002-9322-3515 9 , 10 &
- Marinka Zitnik ORCID: orcid.org/0000-0001-8530-7228 20 , 22 , 35 , 36
Nature volume 620 , pages 47–60 ( 2023 ) Cite this article
98k Accesses
164 Citations
598 Altmetric
Metrics details
- Computer science
- Machine learning
- Scientific community
A Publisher Correction to this article was published on 30 August 2023
This article has been updated
Artificial intelligence (AI) is being increasingly integrated into scientific discovery to augment and accelerate research, helping scientists to generate hypotheses, design experiments, collect and interpret large datasets, and gain insights that might not have been possible using traditional scientific methods alone. Here we examine breakthroughs over the past decade that include self-supervised learning, which allows models to be trained on vast amounts of unlabelled data, and geometric deep learning, which leverages knowledge about the structure of scientific data to enhance model accuracy and efficiency. Generative AI methods can create designs, such as small-molecule drugs and proteins, by analysing diverse data modalities, including images and sequences. We discuss how these methods can help scientists throughout the scientific process and the central issues that remain despite such advances. Both developers and users of AI tools need a better understanding of when such approaches need improvement, and challenges posed by poor data quality and stewardship remain. These issues cut across scientific disciplines and require developing foundational algorithmic approaches that can contribute to scientific understanding or acquire it autonomously, making them critical areas of focus for AI innovation.
This is a preview of subscription content, access via your institution
Access options
Access Nature and 54 other Nature Portfolio journals
Get Nature+, our best-value online-access subscription
24,99 € / 30 days
cancel any time
Subscribe to this journal
Receive 51 print issues and online access
185,98 € per year
only 3,65 € per issue
Buy this article
- Purchase on Springer Link
- Instant access to full article PDF
Prices may be subject to local taxes which are calculated during checkout
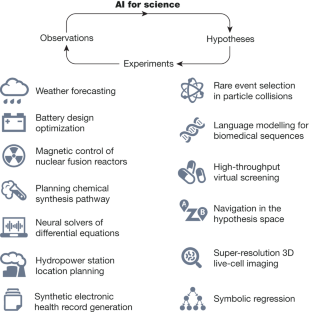
Similar content being viewed by others
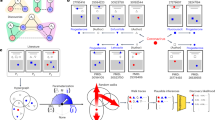
Accelerating science with human-aware artificial intelligence
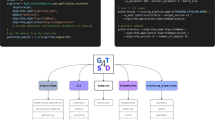
Accelerating material design with the generative toolkit for scientific discovery
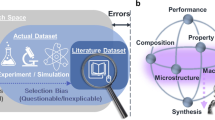
Why big data and compute are not necessarily the path to big materials science
Change history, 30 august 2023.
A Correction to this paper has been published: https://doi.org/10.1038/s41586-023-06559-7
LeCun, Y., Bengio, Y. & Hinton, G. Deep learning. Nature 521 , 436–444 (2015). This survey summarizes key elements of deep learning and its development in speech recognition, computer vision and and natural language processing .
Article ADS CAS PubMed Google Scholar
de Regt, H. W. Understanding, values, and the aims of science. Phil. Sci. 87 , 921–932 (2020).
Article MathSciNet Google Scholar
Pickstone, J. V. Ways of Knowing: A New History of Science, Technology, and Medicine (Univ. Chicago Press, 2001).
Han, J. et al. Deep potential: a general representation of a many-body potential energy surface. Commun. Comput. Phys. 23 , 629–639 (2018). This paper introduced a deep neural network architecture that learns the potential energy surface of many-body systems while respecting the underlying symmetries of the system by incorporating group theory.
Akiyama, K. et al. First M87 Event Horizon Telescope results. IV. Imaging the central supermassive black hole. Astrophys. J. Lett. 875 , L4 (2019).
Article ADS CAS Google Scholar
Wagner, A. Z. Constructions in combinatorics via neural networks. Preprint at https://arxiv.org/abs/2104.14516 (2021).
Coley, C. W. et al. A robotic platform for flow synthesis of organic compounds informed by AI planning. Science 365 , eaax1566 (2019).
Article CAS PubMed Google Scholar
Bommasani, R. et al. On the opportunities and risks of foundation models. Preprint at https://arxiv.org/abs/2108.07258 (2021).
Davies, A. et al. Advancing mathematics by guiding human intuition with AI. Nature 600 , 70–74 (2021). This paper explores how AI can aid the development of pure mathematics by guiding mathematical intuition.
Article ADS CAS PubMed PubMed Central MATH Google Scholar
Jumper, J. et al. Highly accurate protein structure prediction with AlphaFold. Nature 596 , 583–589 (2021). This study was the first to demonstrate the ability to predict protein folding structures using AI methods with a high degree of accuracy, achieving results that are at or near the experimental resolution. This accomplishment is particularly noteworthy, as predicting protein folding has been a grand challenge in the field of molecular biology for over 50 years.
Article ADS CAS PubMed PubMed Central Google Scholar
Stokes, J. M. et al. A deep learning approach to antibiotic discovery. Cell 180 , 688–702 (2020).
Article CAS PubMed PubMed Central Google Scholar
Bohacek, R. S., McMartin, C. & Guida, W. C. The art and practice of structure-based drug design: a molecular modeling perspective. Med. Res. Rev. 16 , 3–50 (1996).
Bileschi, M. L. et al. Using deep learning to annotate the protein universe. Nat. Biotechnol. 40 , 932–937 (2022).
Bellemare, M. G. et al. Autonomous navigation of stratospheric balloons using reinforcement learning. Nature 588 , 77–82 (2020). This paper describes a reinforcement-learning algorithm for navigating a super-pressure balloon in the stratosphere, making real-time decisions in the changing environment.
Tshitoyan, V. et al. Unsupervised word embeddings capture latent knowledge from materials science literature. Nature 571 , 95–98 (2019).
Zhang, L. et al. Deep potential molecular dynamics: a scalable model with the accuracy of quantum mechanics. Phys. Rev. Lett. 120 , 143001 (2018).
Deiana, A. M. et al. Applications and techniques for fast machine learning in science. Front. Big Data 5 , 787421 (2022).
Karagiorgi, G. et al. Machine learning in the search for new fundamental physics. Nat. Rev. Phys. 4 , 399–412 (2022).
Zhou, C. & Paffenroth, R. C. Anomaly detection with robust deep autoencoders. In ACM SIGKDD International Conference on Knowledge Discovery and Data Mining 665–674 (2017).
Hinton, G. E. & Salakhutdinov, R. R. Reducing the dimensionality of data with neural networks. Science 313 , 504–507 (2006).
Article ADS MathSciNet CAS PubMed MATH Google Scholar
Kasieczka, G. et al. The LHC Olympics 2020 a community challenge for anomaly detection in high energy physics. Rep. Prog. Phys. 84 , 124201 (2021).
Govorkova, E. et al. Autoencoders on field-programmable gate arrays for real-time, unsupervised new physics detection at 40 MHz at the Large Hadron Collider. Nat. Mach. Intell. 4 , 154–161 (2022).
Article Google Scholar
Chamberland, M. et al. Detecting microstructural deviations in individuals with deep diffusion MRI tractometry. Nat. Comput. Sci. 1 , 598–606 (2021).
Article PubMed PubMed Central Google Scholar
Rafique, M. et al. Delegated regressor, a robust approach for automated anomaly detection in the soil radon time series data. Sci. Rep. 10 , 3004 (2020).
Pastore, V. P. et al. Annotation-free learning of plankton for classification and anomaly detection. Sci. Rep. 10 , 12142 (2020).
Naul, B. et al. A recurrent neural network for classification of unevenly sampled variable stars. Nat. Astron. 2 , 151–155 (2018).
Article ADS Google Scholar
Lee, D.-H. et al. Pseudo-label: the simple and efficient semi-supervised learning method for deep neural networks. In ICML Workshop on Challenges in Representation Learning (2013).
Zhou, D. et al. Learning with local and global consistency. In Advances in Neural Information Processing Systems 16 , 321–328 (2003).
Radivojac, P. et al. A large-scale evaluation of computational protein function prediction. Nat. Methods 10 , 221–227 (2013).
Barkas, N. et al. Joint analysis of heterogeneous single-cell RNA-seq dataset collections. Nat. Methods 16 , 695–698 (2019).
Tran, K. & Ulissi, Z. W. Active learning across intermetallics to guide discovery of electrocatalysts for CO 2 reduction and H 2 evolution. Nat. Catal. 1 , 696–703 (2018).
Article CAS Google Scholar
Jablonka, K. M. et al. Bias free multiobjective active learning for materials design and discovery. Nat. Commun. 12 , 2312 (2021).
Roussel, R. et al. Turn-key constrained parameter space exploration for particle accelerators using Bayesian active learning. Nat. Commun. 12 , 5612 (2021).
Ratner, A. J. et al. Data programming: creating large training sets, quickly. In Advances in Neural Information Processing Systems 29 , 3567–3575 (2016).
Ratner, A. et al. Snorkel: rapid training data creation with weak supervision. In International Conference on Very Large Data Bases 11 , 269–282 (2017). This paper presents a weakly-supervised AI system designed to annotate massive amounts of data using labeling functions.
Butter, A. et al. GANplifying event samples. SciPost Phys. 10 , 139 (2021).
Brown, T. et al. Language models are few-shot learners. In Advances in Neural Information Processing Systems 33 , 1877–1901 (2020).
Ramesh, A. et al. Zero-shot text-to-image generation. In International Conference on Machine Learning 139 , 8821–8831 (2021).
Littman, M. L. Reinforcement learning improves behaviour from evaluative feedback. Nature 521 , 445–451 (2015).
Cubuk, E. D. et al. Autoaugment: learning augmentation strategies from data. In IEEE Conference on Computer Vision and Pattern Recognition 113–123 (2019).
Reed, C. J. et al. Selfaugment: automatic augmentation policies for self-supervised learning. In IEEE Conference on Computer Vision and Pattern Recognition 2674–2683 (2021).
ATLAS Collaboration et al. Deep generative models for fast photon shower simulation in ATLAS. Preprint at https://arxiv.org/abs/2210.06204 (2022).
Mahmood, F. et al. Deep adversarial training for multi-organ nuclei segmentation in histopathology images. IEEE Trans. Med. Imaging 39 , 3257–3267 (2019).
Teixeira, B. et al. Generating synthetic X-ray images of a person from the surface geometry. In IEEE Conference on Computer Vision and Pattern Recognition 9059–9067 (2018).
Lee, D., Moon, W.-J. & Ye, J. C. Assessing the importance of magnetic resonance contrasts using collaborative generative adversarial networks. Nat. Mach. Intell. 2 , 34–42 (2020).
Kench, S. & Cooper, S. J. Generating three-dimensional structures from a two-dimensional slice with generative adversarial network-based dimensionality expansion. Nat. Mach. Intell. 3 , 299–305 (2021).
Wan, C. & Jones, D. T. Protein function prediction is improved by creating synthetic feature samples with generative adversarial networks. Nat. Mach. Intell. 2 , 540–550 (2020).
Repecka, D. et al. Expanding functional protein sequence spaces using generative adversarial networks. Nat. Mach. Intell. 3 , 324–333 (2021).
Marouf, M. et al. Realistic in silico generation and augmentation of single-cell RNA-seq data using generative adversarial networks. Nat. Commun. 11 , 166 (2020).
Ghahramani, Z. Probabilistic machine learning and artificial intelligence. Nature 521 , 452–459 (2015). This survey provides an introduction to probabilistic machine learning, which involves the representation and manipulation of uncertainty in models and predictions, playing a central role in scientific data analysis.
Cogan, J. et al. Jet-images: computer vision inspired techniques for jet tagging. J. High Energy Phys. 2015 , 118 (2015).
Zhao, W. et al. Sparse deconvolution improves the resolution of live-cell super-resolution fluorescence microscopy. Nat. Biotechnol. 40 , 606–617 (2022).
Brbić, M. et al. MARS: discovering novel cell types across heterogeneous single-cell experiments. Nat. Methods 17 , 1200–1206 (2020).
Article PubMed Google Scholar
Qiao, C. et al. Evaluation and development of deep neural networks for image super-resolution in optical microscopy. Nat. Methods 18 , 194–202 (2021).
Andreassen, A. et al. OmniFold: a method to simultaneously unfold all observables. Phys. Rev. Lett. 124 , 182001 (2020).
Bergenstråhle, L. et al. Super-resolved spatial transcriptomics by deep data fusion. Nat. Biotechnol. 40 , 476–479 (2021).
Vincent, P. et al. Extracting and composing robust features with denoising autoencoders. In International Conference on Machine Learning 1096–1103 (2008).
Kingma, D. P. & Welling, M. Auto-encoding variational Bayes. In International Conference on Learning Representations (2014).
Eraslan, G. et al. Single-cell RNA-seq denoising using a deep count autoencoder. Nat. Commun. 10 , 390 (2019).
Goodfellow, I., Bengio, Y. & Courville, A. Deep Learning (MIT Press, 2016).
Olshausen, B. A. & Field, D. J. Emergence of simple-cell receptive field properties by learning a sparse code for natural images. Nature 381 , 607–609 (1996).
Bengio, Y. Deep learning of representations for unsupervised and transfer learning. In ICML Workshop on Unsupervised and Transfer Learning (2012).
Detlefsen, N. S., Hauberg, S. & Boomsma, W. Learning meaningful representations of protein sequences. Nat. Commun. 13 , 1914 (2022).
Becht, E. et al. Dimensionality reduction for visualizing single-cell data using UMAP. Nat. Biotechnol. 37 , 38–44 (2019).
Bronstein, M. M. et al. Geometric deep learning: going beyond euclidean data. IEEE Signal Process Mag. 34 , 18–42 (2017).
Anderson, P. W. More is different: broken symmetry and the nature of the hierarchical structure of science. Science 177 , 393–396 (1972).
Qiao, Z. et al. Informing geometric deep learning with electronic interactions to accelerate quantum chemistry. Proc. Natl Acad. Sci. USA 119 , e2205221119 (2022).
Bogatskiy, A. et al. Symmetry group equivariant architectures for physics. Preprint at https://arxiv.org/abs/2203.06153 (2022).
Bronstein, M. M. et al. Geometric deep learning: grids, groups, graphs, geodesics, and gauges. Preprint at https://arxiv.org/abs/2104.13478 (2021).
Townshend, R. J. L. et al. Geometric deep learning of RNA structure. Science 373 , 1047–1051 (2021).
Wicky, B. I. M. et al. Hallucinating symmetric protein assemblies. Science 378 , 56–61 (2022).
Kipf, T. N. & Welling, M. Semi-supervised classification with graph convolutional networks. In International Conference on Learning Representations (2017).
Veličković, P. et al. Graph attention networks. In International Conference on Learning Representations (2018).
Hamilton, W. L., Ying, Z. & Leskovec, J. Inductive representation learning on large graphs. In Advances in Neural Information Processing Systems 30 , 1024–1034 (2017).
Gilmer, J. et al. Neural message passing for quantum chemistry. In International Conference on Machine Learning 1263–1272 (2017).
Li, M. M., Huang, K. & Zitnik, M. Graph representation learning in biomedicine and healthcare. Nat. Biomed. Eng. 6 , 1353–1369 (2022).
Satorras, V. G., Hoogeboom, E. & Welling, M. E( n ) equivariant graph neural networks. In International Conference on Machine Learning 9323–9332 (2021). This study incorporates principles of physics into the design of neural models, advancing the field of equivariant machine learning .
Thomas, N. et al. Tensor field networks: rotation-and translation-equivariant neural networks for 3D point clouds. Preprint at https://arxiv.org/abs/1802.08219 (2018).
Finzi, M. et al. Generalizing convolutional neural networks for equivariance to lie groups on arbitrary continuous data. In International Conference on Machine Learning 3165–3176 (2020).
Fuchs, F. et al. SE(3)-transformers: 3D roto-translation equivariant attention networks. In Advances in Neural Information Processing Systems 33 , 1970-1981 (2020).
Zaheer, M. et al. Deep sets. In Advances in Neural Information Processing Systems 30 , 3391–3401 (2017). This paper is an early study that explores the use of deep neural architectures on set data, which consists of an unordered list of elements.
Cohen, T. S. et al. Spherical CNNs. In International Conference on Learning Representations (2018).
Gordon, J. et al. Permutation equivariant models for compositional generalization in language. In International Conference on Learning Representations (2019).
Finzi, M., Welling, M. & Wilson, A. G. A practical method for constructing equivariant multilayer perceptrons for arbitrary matrix groups. In International Conference on Machine Learning 3318–3328 (2021).
Dijk, D. V. et al. Recovering gene interactions from single-cell data using data diffusion. Cell 174 , 716–729 (2018).
Gainza, P. et al. Deciphering interaction fingerprints from protein molecular surfaces using geometric deep learning. Nat. Methods 17 , 184–192 (2020).
Hatfield, P. W. et al. The data-driven future of high-energy-density physics. Nature 593 , 351–361 (2021).
Bapst, V. et al. Unveiling the predictive power of static structure in glassy systems. Nat. Phys. 16 , 448–454 (2020).
Zhang, R., Zhou, T. & Ma, J. Multiscale and integrative single-cell Hi-C analysis with Higashi. Nat. Biotechnol. 40 , 254–261 (2022).
Sammut, S.-J. et al. Multi-omic machine learning predictor of breast cancer therapy response. Nature 601 , 623–629 (2022).
DeZoort, G. et al. Graph neural networks at the Large Hadron Collider. Nat. Rev. Phys . 5 , 281–303 (2023).
Liu, S. et al. Pre-training molecular graph representation with 3D geometry. In International Conference on Learning Representations (2022).
The LIGO Scientific Collaboration. et al. A gravitational-wave standard siren measurement of the Hubble constant. Nature 551 , 85–88 (2017).
Reichstein, M. et al. Deep learning and process understanding for data-driven Earth system science. Nature 566 , 195–204 (2019).
Goenka, S. D. et al. Accelerated identification of disease-causing variants with ultra-rapid nanopore genome sequencing. Nat. Biotechnol. 40 , 1035–1041 (2022).
Bengio, Y. et al. Greedy layer-wise training of deep networks. In Advances in Neural Information Processing Systems 19 , 153–160 (2006).
Hinton, G. E., Osindero, S. & Teh, Y.-W. A fast learning algorithm for deep belief nets. Neural Comput. 18 , 1527–1554 (2006).
Article MathSciNet PubMed MATH Google Scholar
Jordan, M. I. & Mitchell, T. M. Machine learning: trends, perspectives, and prospects. Science 349 , 255–260 (2015).
Devlin, J. et al. BERT: pre-training of deep bidirectional transformers for language understanding. In North American Chapter of the Association for Computational Linguistics 4171–4186 (2019).
Rives, A. et al. Biological structure and function emerge from scaling unsupervised learning to 250 million protein sequences. Proc. Natl Acad. Sci. USA 118 , e2016239118 (2021).
Elnaggar, A. et al. ProtTrans: rowards cracking the language of lifes code through self-supervised deep learning and high performance computing. In IEEE Transactions on Pattern Analysis and Machine Intelligence (2021).
Hie, B. et al. Learning the language of viral evolution and escape. Science 371 , 284–288 (2021). This paper modeled viral escape with machine learning algorithms originally developed for human natural language.
Biswas, S. et al. Low- N protein engineering with data-efficient deep learning. Nat. Methods 18 , 389–396 (2021).
Ferruz, N. & Höcker, B. Controllable protein design with language models. Nat. Mach. Intell. 4 , 521–532 (2022).
Hsu, C. et al. Learning inverse folding from millions of predicted structures. In International Conference on Machine Learning 8946–8970 (2022).
Baek, M. et al. Accurate prediction of protein structures and interactions using a three-track neural network. Science 373 , 871–876 (2021). Inspired by AlphaFold2, this study reported RoseTTAFold, a novel three-track neural module capable of simultaneously processing protein’s sequence, distance and coordinates.
Weininger, D. SMILES, a chemical language and information system. 1. Introduction to methodology and encoding rules. J. Chem. Inf. Comput. Sci. 28 , 31–36 (1988).
Lin, T.-S. et al. BigSMILES: a structurally-based line notation for describing macromolecules. ACS Cent. Sci. 5 , 1523–1531 (2019).
Krenn, M. et al. SELFIES and the future of molecular string representations. Patterns 3 , 100588 (2022).
Flam-Shepherd, D., Zhu, K. & Aspuru-Guzik, A. Language models can learn complex molecular distributions. Nat. Commun. 13 , 3293 (2022).
Skinnider, M. A. et al. Chemical language models enable navigation in sparsely populated chemical space. Nat. Mach. Intell. 3 , 759–770 (2021).
Chithrananda, S., Grand, G. & Ramsundar, B. ChemBERTa: large-scale self-supervised pretraining for molecular property prediction. In Machine Learning for Molecules Workshop at NeurIPS (2020).
Schwaller, P. et al. Predicting retrosynthetic pathways using transformer-based models and a hyper-graph exploration strategy. Chem. Sci. 11 , 3316–3325 (2020).
Tetko, I. V. et al. State-of-the-art augmented NLP transformer models for direct and single-step retrosynthesis. Nat. Commun. 11 , 5575 (2020).
Schwaller, P. et al. Mapping the space of chemical reactions using attention-based neural networks. Nat. Mach. Intell. 3 , 144–152 (2021).
Kovács, D. P., McCorkindale, W. & Lee, A. A. Quantitative interpretation explains machine learning models for chemical reaction prediction and uncovers bias. Nat. Commun. 12 , 1695 (2021).
Article ADS PubMed PubMed Central Google Scholar
Pesciullesi, G. et al. Transfer learning enables the molecular transformer to predict regio-and stereoselective reactions on carbohydrates. Nat. Commun. 11 , 4874 (2020).
Vaswani, A. et al. Attention is all you need. In Advances in Neural Information Processing Systems 30 , 5998–6008 (2017). This paper introduced the transformer, a modern neural network architecture that can process sequential data in parallel, revolutionizing natural language processing and sequence modeling.
Mousavi, S. M. et al. Earthquake transformer—an attentive deep-learning model for simultaneous earthquake detection and phase picking. Nat. Commun. 11 , 3952 (2020).
Avsec, Ž. et al. Effective gene expression prediction from sequence by integrating long-range interactions. Nat. Methods 18 , 1196–1203 (2021).
Meier, J. et al. Language models enable zero-shot prediction of the effects of mutations on protein function. In Advances in Neural Information Processing Systems 34 , 29287–29303 (2021).
Kamienny, P.-A. et al. End-to-end symbolic regression with transformers. In Advances in Neural Information Processing Systems 35 , 10269–10281 (2022).
Jaegle, A. et al. Perceiver: general perception with iterative attention. In International Conference on Machine Learning 4651–4664 (2021).
Chen, L. et al. Decision transformer: reinforcement learning via sequence modeling. In Advances in Neural Information Processing Systems 34 , 15084–15097 (2021).
Dosovitskiy, A. et al. An image is worth 16x16 words: transformers for image recognition at scale. In International Conference on Learning Representations (2020).
Choromanski, K. et al. Rethinking attention with performers. In International Conference on Learning Representations (2021).
Li, Z. et al. Fourier neural operator for parametric partial differential equations. In International Conference on Learning Representations (2021).
Kovachki, N. et al. Neural operator: learning maps between function spaces. J. Mach. Learn. Res. 24 , 1–97 (2023).
Russell, J. L. Kepler’s laws of planetary motion: 1609–1666. Br. J. Hist. Sci. 2 , 1–24 (1964).
Huang, K. et al. Artificial intelligence foundation for therapeutic science. Nat. Chem. Biol. 18 , 1033–1036 (2022).
Guimerà, R. et al. A Bayesian machine scientist to aid in the solution of challenging scientific problems. Sci. Adv. 6 , eaav6971 (2020).
Liu, G. et al. Deep learning-guided discovery of an antibiotic targeting Acinetobacter baumannii. Nat. Chem. Biol. https://doi.org/10.1038/s41589-023-01349-8 (2023).
Gómez-Bombarelli, R. et al. Design of efficient molecular organic light-emitting diodes by a high-throughput virtual screening and experimental approach. Nat. Mater. 15 , 1120–1127 (2016). This paper proposes using a black-box AI predictor to accelerate high-throughput screening of molecules in materials science.
Article ADS PubMed Google Scholar
Sadybekov, A. A. et al. Synthon-based ligand discovery in virtual libraries of over 11 billion compounds. Nature 601 , 452–459 (2022).
The NNPDF Collaboration Evidence for intrinsic charm quarks in the proton. Nature 606 , 483–487 (2022).
Graff, D. E., Shakhnovich, E. I. & Coley, C. W. Accelerating high-throughput virtual screening through molecular pool-based active learning. Chem. Sci. 12 , 7866–7881 (2021).
Janet, J. P. et al. Accurate multiobjective design in a space of millions of transition metal complexes with neural-network-driven efficient global optimization. ACS Cent. Sci. 6 , 513–524 (2020).
Bacon, F. Novum Organon Vol. 1620 (2000).
Schmidt, M. & Lipson, H. Distilling free-form natural laws from experimental data. Science 324 , 81–85 (2009).
Petersen, B. K. et al. Deep symbolic regression: recovering mathematical expressions from data via risk-seeking policy gradients. In International Conference on Learning Representations (2020).
Zhavoronkov, A. et al. Deep learning enables rapid identification of potent DDR1 kinase inhibitors. Nat. Biotechnol. 37 , 1038–1040 (2019). This paper describes a reinforcement-learning algorithm for navigating molecular combinatorial spaces, and it validates generated molecules using wet-lab experiments.
Zhou, Z. et al. Optimization of molecules via deep reinforcement learning. Sci. Rep. 9 , 10752 (2019).
You, J. et al. Graph convolutional policy network for goal-directed molecular graph generation. In Advances in Neural Information Processing Systems 31 , 6412–6422 (2018).
Bengio, Y. et al. GFlowNet foundations. Preprint at https://arxiv.org/abs/2111.09266 (2021). This paper describes a generative flow network that generates objects by sampling them from a distribution optimized for drug design.
Jain, M. et al. Biological sequence design with GFlowNets. In International Conference on Machine Learning 9786–9801 (2022).
Malkin, N. et al. Trajectory balance: improved credit assignment in GFlowNets. In Advances in Neural Information Processing Systems 35 , 5955–5967 (2022).
Borkowski, O. et al. Large scale active-learning-guided exploration for in vitro protein production optimization. Nat. Commun. 11 , 1872 (2020). This study introduced a dynamic programming approach to determine the optimal locations and capacities of hydropower dams in the Amazon Basin, balancing between energy production and environmental impact .
Flecker, A. S. et al. Reducing adverse impacts of Amazon hydropower expansion. Science 375 , 753–760 (2022). This study introduced a dynamic programming approach to determine the optimal locations and capacities of hydropower dams in the Amazon basin, achieving a balance between the benefits of energy production and the potential environmental impacts.
Pion-Tonachini, L. et al. Learning from learning machines: a new generation of AI technology to meet the needs of science. Preprint at https://arxiv.org/abs/2111.13786 (2021).
Kusner, M. J., Paige, B. & Hernández-Lobato, J. M. Grammar variational autoencoder. In International Conference on Machine Learning 1945–1954 (2017). This paper describes a grammar variational autoencoder that generates novel symbolic laws and drug molecules.
Brunton, S. L., Proctor, J. L. & Kutz, J. N. Discovering governing equations from data by sparse identification of nonlinear dynamical systems. Proc. Natl Acad. Sci. USA 113 , 3932–3937 (2016).
Article ADS MathSciNet CAS PubMed PubMed Central MATH Google Scholar
Liu, Z. & Tegmark, M. Machine learning hidden symmetries. Phys. Rev. Lett. 128 , 180201 (2022).
Article ADS MathSciNet CAS PubMed Google Scholar
Gabbard, H. et al. Bayesian parameter estimation using conditional variational autoencoders for gravitational-wave astronomy. Nat. Phys. 18 , 112–117 (2022).
Chen, D. et al. Automating crystal-structure phase mapping by combining deep learning with constraint reasoning. Nat. Mach. Intell. 3 , 812–822 (2021).
Gómez-Bombarelli, R. et al. Automatic chemical design using a data-driven continuous representation of molecules. ACS Cent. Sci. 4 , 268–276 (2018).
Anishchenko, I. et al. De novo protein design by deep network hallucination. Nature 600 , 547–552 (2021).
Fu, T. et al. Differentiable scaffolding tree for molecular optimization. In International Conference on Learning Representations (2021).
Sanchez-Lengeling, B. & Aspuru-Guzik, A. Inverse molecular design using machine learning: generative models for matter engineering. Science 361 , 360–365 (2018).
Huang, K. et al. Therapeutics Data Commons: machine learning datasets and tasks for drug discovery and development. In NeurIPS Datasets and Benchmarks (2021). This study describes an initiative with open AI models, datasets and education programmes to facilitate advances in therapeutic science across all stages of drug discovery and development.
Dance, A. Lab hazard. Nature 458 , 664–665 (2009).
Segler, M. H. S., Preuss, M. & Waller, M. P. Planning chemical syntheses with deep neural networks and symbolic AI. Nature 555 , 604–610 (2018). This paper describes an approach that combines deep neural networks with Monte Carlo tree search to plan chemical synthesis.
Gao, W., Raghavan, P. & Coley, C. W. Autonomous platforms for data-driven organic synthesis. Nat. Commun. 13 , 1075 (2022).
Kusne, A. G. et al. On-the-fly closed-loop materials discovery via Bayesian active learning. Nat. Commun. 11 , 5966 (2020).
Gormley,A. J. & Webb, M. A. Machine learning in combinatorial polymer chemistry. Nat. Rev. Mater. 6 , 642–644 (2021).
Ament, S. et al. Autonomous materials synthesis via hierarchical active learning of nonequilibrium phase diagrams. Sci. Adv. 7 , eabg4930 (2021).
Degrave, J. et al. Magnetic control of tokamak plasmas through deep reinforcement learning. Nature 602 , 414–419 (2022). This paper describes an approach for controlling tokamak plasmas, using a reinforcement-learning agent to command-control coils and satisfy physical and operational constraints.
Melnikov, A. A. et al. Active learning machine learns to create new quantum experiments. Proc. Natl Acad. Sci. USA 115 , 1221–1226 (2018).
Smith, J. S., Isayev, O. & Roitberg, A. E. ANI-1: an extensible neural network potential with DFT accuracy at force field computational cost. Chem. Sci. 8 , 3192–3203 (2017).
Wang, D. et al. Efficient sampling of high-dimensional free energy landscapes using adaptive reinforced dynamics. Nat. Comput. Sci. 2 , 20–29 (2022). This paper describes a neural network for reliable uncertainty estimations in molecular dynamics, enabling efficient sampling of high-dimensional free energy landscapes.
Wang, W. & Gómez-Bombarelli, R. Coarse-graining auto-encoders for molecular dynamics. npj Comput. Mater. 5 , 125 (2019).
Hermann, J., Schätzle, Z. & Noé, F. Deep-neural-network solution of the electronic Schrödinger equation. Nat. Chem. 12 , 891–897 (2020). This paper describes a method to learn the wavefunction of quantum systems using deep neural networks in conjunction with variational quantum Monte Carlo.
Carleo, G. & Troyer, M. Solving the quantum many-body problem with artificial neural networks. Science 355 , 602–606 (2017).
Em Karniadakis, G. et al. Physics-informed machine learning. Nat. Rev. Phys. 3 , 422–440 (2021).
Li, Z. et al. Physics-informed neural operator for learning partial differential equations. Preprint at https://arxiv.org/abs/2111.03794 (2021).
Kochkov, D. et al. Machine learning–accelerated computational fluid dynamics. Proc. Natl Acad. Sci. USA 118 , e2101784118 (2021). This paper describes an approach to accelerating computational fluid dynamics by training a neural network to interpolate from coarse to fine grids and generalize to varying forcing functions and Reynolds numbers.
Ji, W. et al. Stiff-PINN: physics-informed neural network for stiff chemical kinetics. J. Phys. Chem. A 125 , 8098–8106 (2021).
Smith, J. D., Azizzadenesheli, K. & Ross, Z. E. EikoNet: solving the Eikonal equation with deep neural networks. IEEE Trans. Geosci. Remote Sens. 59 , 10685–10696 (2020).
Waheed, U. B. et al. PINNeik: Eikonal solution using physics-informed neural networks. Comput. Geosci. 155 , 104833 (2021).
Chen, R. T. Q. et al. Neural ordinary differential equations. In Advances in Neural Information Processing Systems 31 , 6572–6583 (2018). This paper established a connection between neural networks and differential equations by introducing the adjoint method to learn continuous-time dynamical systems from data, replacing backpropagation.
Raissi, M., Perdikaris, P. & Karniadakis, G. E. Physics-informed neural networks: a deep learning framework for solving forward and inverse problems involving nonlinear partial differential equations. J. Comput. Phys. 378 , 686–707 (2019). This paper describes a deep-learning approach for solving forwards and inverse problems in nonlinear partial differential equations and can find solutions to differential equations from data.
Article ADS MathSciNet MATH Google Scholar
Lu, L. et al. Learning nonlinear operators via DeepONet based on the universal approximation theorem of operators. Nat. Mach. Intell. 3 , 218–229 (2021).
Brandstetter, J., Worrall, D. & Welling, M. Message passing neural PDE solvers. In International Conference on Learning Representations (2022).
Noé, F. et al. Boltzmann generators: sampling equilibrium states of many-body systems with deep learning. Science 365 , eaaw1147 (2019). This paper presents an efficient sampling algorithm using normalizing flows to simulate equilibrium states in many-body systems.
Rezende, D. & Mohamed, S. Variational inference with normalizing flows. In International Conference on Machine Learning 37 , 1530–1538, (2015).
Dinh, L., Sohl-Dickstein, J. & Bengio, S. Density estimation using real NVP. In International Conference on Learning Representations (2017).
Nicoli, K. A. et al. Estimation of thermodynamic observables in lattice field theories with deep generative models. Phys. Rev. Lett. 126 , 032001 (2021).
Kanwar, G. et al. Equivariant flow-based sampling for lattice gauge theory. Phys. Rev. Lett. 125 , 121601 (2020).
Gabrié, M., Rotskoff, G. M. & Vanden-Eijnden, E. Adaptive Monte Carlo augmented with normalizing flows. Proc. Natl Acad. Sci. USA 119 , e2109420119 (2022).
Article MathSciNet PubMed PubMed Central Google Scholar
Jasra, A., Holmes, C. C. & Stephens, D. A. Markov chain Monte Carlo methods and the label switching problem in Bayesian mixture modeling. Stat. Sci. 20 , 50–67 (2005).
Bengio, Y. et al. Better mixing via deep representations. In International Conference on Machine Learning 552–560 (2013).
Pompe, E., Holmes, C. & Łatuszyński, K. A framework for adaptive MCMC targeting multimodal distributions. Ann. Stat. 48 , 2930–2952 (2020).
Article MathSciNet MATH Google Scholar
Townshend, R. J. L. et al. ATOM3D: tasks on molecules in three dimensions. In NeurIPS Datasets and Benchmarks (2021).
Kearnes, S. M. et al. The open reaction database. J. Am. Chem. Soc. 143 , 18820–18826 (2021).
Chanussot, L. et al. Open Catalyst 2020 (OC20) dataset and community challenges. ACS Catal. 11 , 6059–6072 (2021).
Brown, N. et al. GuacaMol: benchmarking models for de novo molecular design. J. Chem. Inf. Model. 59 , 1096–1108 (2019).
Notin, P. et al. Tranception: protein fitness prediction with autoregressive transformers and inference-time retrieval. In International Conference on Machine Learning 16990–17017 (2022).
Mitchell, M. et al. Model cards for model reporting. In Conference on Fairness, Accountability, and Transparency 220–229 (2019).
Gebru, T. et al. Datasheets for datasets. Commun. ACM 64 , 86–92 (2021).
Bai, X. et al. Advancing COVID-19 diagnosis with privacy-preserving collaboration in artificial intelligence. Nat. Mach. Intell. 3 , 1081–1089 (2021).
Warnat-Herresthal, S. et al. Swarm learning for decentralized and confidential clinical machine learning. Nature 594 , 265–270 (2021).
Hie, B., Cho, H. & Berger, B. Realizing private and practical pharmacological collaboration. Science 362 , 347–350 (2018).
Rohrbach, S. et al. Digitization and validation of a chemical synthesis literature database in the ChemPU. Science 377 , 172–180 (2022).
Gysi, D. M. et al. Network medicine framework for identifying drug-repurposing opportunities for COVID-19. Proc. Natl Acad. Sci. USA 118 , e2025581118 (2021).
King, R. D. et al. The automation of science. Science 324 , 85–89 (2009).
Mirdita, M. et al. ColabFold: making protein folding accessible to all. Nat. Methods 19 , 679–682 (2022).
Doerr, S. et al. TorchMD: a deep learning framework for molecular simulations. J. Chem. Theory Comput. 17 , 2355–2363 (2021).
Schoenholz, S. S. & Cubuk, E. D. JAX MD: a framework for differentiable physics. In Advances in Neural Information Processing Systems 33 , 11428–11441 (2020).
Peters, J., Janzing, D. & Schölkopf, B. Elements of Causal Inference: Foundations and Learning Algorithms (MIT Press, 2017).
Bengio, Y. et al. A meta-transfer objective for learning to disentangle causal mechanisms. In International Conference on Learning Representations (2020).
Schölkopf, B. et al. Toward causal representation learning. Proc. IEEE 109 , 612–634 (2021).
Goyal, A. & Bengio, Y. Inductive biases for deep learning of higher-level cognition. Proc. R. Soc. A 478 , 20210068 (2022).
Deleu, T. et al. Bayesian structure learning with generative flow networks. In Conference on Uncertainty in Artificial Intelligence 518–528 (2022).
Geirhos, R. et al. Shortcut learning in deep neural networks. Nat. Mach. Intell. 2 , 665–673 (2020).
Koh, P. W. et al. WILDS: a benchmark of in-the-wild distribution shifts. In International Conference on Machine Learning 5637–5664 (2021).
Luo, Z. et al. Label efficient learning of transferable representations across domains and tasks. In Advances in Neural Information Processing Systems 30 , 165–177 (2017).
Mahmood, R. et al. How much more data do I need? estimating requirements for downstream tasks. In IEEE Conference on Computer Vision and Pattern Recognition 275–284 (2022).
Coley, C. W., Eyke, N. S. & Jensen, K. F. Autonomous discovery in the chemical sciences part II: outlook. Angew. Chem. Int. Ed. 59 , 23414–23436 (2020).
Gao, W. & Coley, C. W. The synthesizability of molecules proposed by generative models. J. Chem. Inf. Model. 60 , 5714–5723 (2020).
Kogler, R. et al. Jet substructure at the Large Hadron Collider. Rev. Mod. Phys. 91 , 045003 (2019).
Acosta, J. N. et al. Multimodal biomedical AI. Nat. Med. 28 , 1773–1784 (2022).
Alayrac, J.-B. et al. Flamingo: a visual language model for few-shot learning. In Advances in Neural Information Processing Systems 35 , 23716–23736 (2022).
Elmarakeby, H. A. et al. Biologically informed deep neural network for prostate cancer discovery. Nature 598 , 348–352 (2021).
Qin, Y. et al. A multi-scale map of cell structure fusing protein images and interactions. Nature 600 , 536–542 (2021).
Schaffer, L. V. & Ideker, T. Mapping the multiscale structure of biological systems. Cell Systems 12 , 622–635 (2021).
Stiglic, G. et al. Interpretability of machine learning-based prediction models in healthcare. Wiley Interdiscip. Rev. Data Min. Knowl. Discov. 10 , e1379 (2020).
Erion, G. et al. A cost-aware framework for the development of AI models for healthcare applications. Nat. Biomed. Eng. 6 , 1384–1398 (2022).
Lundberg, S. M. et al. Explainable machine-learning predictions for the prevention of hypoxaemia during surgery. Nat. Biomed. Eng. 2 , 749–760 (2018).
Sanders, L. M. et al. Beyond low Earth orbit: biological research, artificial intelligence, and self-driving labs. Preprint at https://arxiv.org/abs/2112.12582 (2021).
Gagne, D. J. II et al. Interpretable deep learning for spatial analysis of severe hailstorms. Mon. Weather Rev. 147 , 2827–2845 (2019).
Rudin, C. Stop explaining black box machine learning models for high stakes decisions and use interpretable models instead. Nat. Mach. Intell. 1 , 206–215 (2019).
Koh, P. W. & Liang, P. Understanding black-box predictions via influence functions. In International Conference on Machine Learning 1885–1894 (2017).
Mirzasoleiman, B., Bilmes, J. & Leskovec, J. Coresets for data-efficient training of machine learning models. In International Conference on Machine Learning 6950–6960 (2020).
Kim, B. et al. Interpretability beyond feature attribution: quantitative testing with concept activation vectors (TCAV). In International Conference on Machine Learning 2668–2677 (2018).
Silver, D. et al. Mastering the game of go without human knowledge. Nature 550 , 354–359 (2017).
Baum, Z. J. et al. Artificial intelligence in chemistry: current trends and future directions. J. Chem. Inf. Model. 61 , 3197–3212 (2021).
Finlayson, S. G. et al. Adversarial attacks on medical machine learning. Science 363 , 1287–1289 (2019).
Urbina, F. et al. Dual use of artificial-intelligence-powered drug discovery. Nat. Mach. Intell. 4 , 189–191 (2022).
Norgeot, B. et al. Minimum information about clinical artificial intelligence modeling: the MI-CLAIM checklist. Nat. Med. 26 , 1320–1324 (2020).
Download references
Acknowledgements
M.Z. gratefully acknowledges the support of the National Institutes of Health under R01HD108794, U.S. Air Force under FA8702-15-D-0001, awards from Harvard Data Science Initiative, Amazon Faculty Research, Google Research Scholar Program, Bayer Early Excellence in Science, AstraZeneca Research, Roche Alliance with Distinguished Scientists, and Kempner Institute for the Study of Natural and Artificial Intelligence. C.P.G. and Y.D. acknowledge the support from the U.S. Air Force Office of Scientific Research under Multidisciplinary University Research Initiatives Program (MURI) FA9550-18-1-0136, Defense University Research Instrumentation Program (DURIP) FA9550-21-1-0316, and awards from Scientific Autonomous Reasoning Agent (SARA), and AI for Discovery Assistant (AIDA). Any opinions, findings, conclusions or recommendations expressed in this material are those of the authors and do not necessarily reflect the views of the funders. We thank D. Hassabis, A. Davies, S. Mohamed, Z. Li, K. Ma, Z. Qiao, E. Weinstein, A. V. Weller, Y. Zhong and A. M. Brandt for discussions on the paper.
Author information
Hanchen Wang
Present address: Department of Research and Early Development, Genentech Inc, South San Francisco, CA, USA
Present address: Department of Computer Science, Stanford University, Stanford, CA, USA
These authors contributed equally: Hanchen Wang, Tianfan Fu, Yuanqi Du
Authors and Affiliations
Department of Engineering, University of Cambridge, Cambridge, UK
Hanchen Wang & Joan Lasenby
Department of Computing and Mathematical Sciences, California Institute of Technology, Pasadena, CA, USA
Hanchen Wang & Anima Anandkumar
Department of Computational Science and Engineering, Georgia Institute of Technology, Atlanta, GA, USA
Department of Computer Science, Cornell University, Ithaca, NY, USA
Yuanqi Du & Carla P. Gomes
Department of Chemical Engineering, Massachusetts Institute of Technology, Cambridge, MA, USA
Wenhao Gao & Connor W. Coley
Department of Computer Science, Stanford University, Stanford, CA, USA
Kexin Huang & Jure Leskovec
Department of Physics, Massachusetts Institute of Technology, Cambridge, MA, USA
Harvard-MIT Program in Health Sciences and Technology, Cambridge, MA, USA
Payal Chandak
Mila – Quebec AI Institute, Montreal, Quebec, Canada
Shengchao Liu, Andreea Deac, Jian Tang & Yoshua Bengio
Université de Montréal, Montreal, Quebec, Canada
Shengchao Liu, Andreea Deac & Yoshua Bengio
Department of Earth, Environmental and Planetary Sciences, Brown University, Providence, RI, USA
Peter Van Katwyk & Karianne Bergen
Data Science Institute, Brown University, Providence, RI, USA
NVIDIA, Santa Clara, CA, USA
Anima Anandkumar
Center for Computational Astrophysics, Flatiron Institute, New York, NY, USA
Department of Astrophysical Sciences, Princeton University, Princeton, NJ, USA
Department of Physics, Carnegie Mellon University, Pittsburgh, PA, USA
Department of Physics and Center for Data Science, New York University, New York, NY, USA
Google DeepMind, London, UK
Pushmeet Kohli & Petar Veličković
Microsoft Research, Beijing, China
Tie-Yan Liu
Department of Biomedical Informatics, Harvard Medical School, Boston, MA, USA
Arjun Manrai & Marinka Zitnik
Department of Systems Biology, Harvard Medical School, Boston, MA, USA
Debora Marks
Broad Institute of MIT and Harvard, Cambridge, MA, USA
Debora Marks & Marinka Zitnik
Deep Forest Sciences, Palo Alto, CA, USA
Bharath Ramsundar
BioMap, Beijing, China
Mohamed bin Zayed University of Artificial Intelligence, Abu Dhabi, United Arab Emirates
University of Illinois at Urbana-Champaign, Champaign, IL, USA
HEC Montréal, Montreal, Quebec, Canada
CIFAR AI Chair, Toronto, Ontario, Canada
Department of Computer Science and Technology, University of Cambridge, Cambridge, UK
Petar Veličković
University of Amsterdam, Amsterdam, Netherlands
Max Welling
Microsoft Research Amsterdam, Amsterdam, Netherlands
DP Technology, Beijing, China
Linfeng Zhang
AI for Science Institute, Beijing, China
Department of Electrical Engineering and Computer Science, Massachusetts Institute of Technology, Cambridge, MA, USA
Connor W. Coley
Harvard Data Science Initiative, Cambridge, MA, USA
Marinka Zitnik
Kempner Institute for the Study of Natural and Artificial Intelligence, Harvard University, Cambridge, MA, USA
You can also search for this author in PubMed Google Scholar
Contributions
All authors contributed to the design and writing of the paper, helped shape the research, provided critical feedback, and commented on the paper and its revisions. H.W., T.F., Y.D. and M.Z conceived the study and were responsible for overall direction and planning. W.G., K.H. and Z.L. contributed equally to this work (equal second authorship) and are listed alphabetically.
Corresponding author
Correspondence to Marinka Zitnik .
Ethics declarations
Competing interests.
The authors declare no competing interests.
Peer review
Peer review information.
Nature thanks Brian Gallagher and Benjamin Nachman for their contribution to the peer review of this work.
Additional information
Publisher’s note Springer Nature remains neutral with regard to jurisdictional claims in published maps and institutional affiliations.
Rights and permissions
Springer Nature or its licensor (e.g. a society or other partner) holds exclusive rights to this article under a publishing agreement with the author(s) or other rightsholder(s); author self-archiving of the accepted manuscript version of this article is solely governed by the terms of such publishing agreement and applicable law.
Reprints and permissions
About this article
Cite this article.
Wang, H., Fu, T., Du, Y. et al. Scientific discovery in the age of artificial intelligence. Nature 620 , 47–60 (2023). https://doi.org/10.1038/s41586-023-06221-2
Download citation
Received : 30 March 2022
Accepted : 16 May 2023
Published : 02 August 2023
Issue Date : 03 August 2023
DOI : https://doi.org/10.1038/s41586-023-06221-2
Share this article
Anyone you share the following link with will be able to read this content:
Sorry, a shareable link is not currently available for this article.
Provided by the Springer Nature SharedIt content-sharing initiative
This article is cited by
Antimicrobial resistance crisis: could artificial intelligence be the solution.
- Guang-Yu Liu
- Xiao-Fen Liu
Military Medical Research (2024)
Embracing data science in catalysis research
- Manu Suvarna
- Javier Pérez-Ramírez
Nature Catalysis (2024)
Artificial intelligence to predict soil temperatures by development of novel model
- Lakindu Mampitiya
- Kenjabek Rozumbetov
- Upaka Rathnayake
Scientific Reports (2024)
Automated BigSMILES conversion workflow and dataset for homopolymeric macromolecules
- Joonbum Lee
- Junhee Seok
Scientific Data (2024)
Techniques for supercharging academic writing with generative AI
- Zhicheng Lin
Nature Biomedical Engineering (2024)
By submitting a comment you agree to abide by our Terms and Community Guidelines . If you find something abusive or that does not comply with our terms or guidelines please flag it as inappropriate.
Quick links
- Explore articles by subject
- Guide to authors
- Editorial policies
Sign up for the Nature Briefing: AI and Robotics newsletter — what matters in AI and robotics research, free to your inbox weekly.

Help | Advanced Search
Computer Science > Computation and Language
Title: dalk: dynamic co-augmentation of llms and kg to answer alzheimer's disease questions with scientific literature.
Abstract: Recent advancements in large language models (LLMs) have achieved promising performances across various applications. Nonetheless, the ongoing challenge of integrating long-tail knowledge continues to impede the seamless adoption of LLMs in specialized domains. In this work, we introduce DALK, a.k.a. Dynamic Co-Augmentation of LLMs and KG, to address this limitation and demonstrate its ability on studying Alzheimer's Disease (AD), a specialized sub-field in biomedicine and a global health priority. With a synergized framework of LLM and KG mutually enhancing each other, we first leverage LLM to construct an evolving AD-specific knowledge graph (KG) sourced from AD-related scientific literature, and then we utilize a coarse-to-fine sampling method with a novel self-aware knowledge retrieval approach to select appropriate knowledge from the KG to augment LLM inference capabilities. The experimental results, conducted on our constructed AD question answering (ADQA) benchmark, underscore the efficacy of DALK. Additionally, we perform a series of detailed analyses that can offer valuable insights and guidelines for the emerging topic of mutually enhancing KG and LLM. We will release the code and data at this https URL .
Submission history
Access paper:.
- HTML (experimental)
- Other Formats

References & Citations
- Google Scholar
- Semantic Scholar
BibTeX formatted citation

Bibliographic and Citation Tools
Code, data and media associated with this article, recommenders and search tools.
- Institution
arXivLabs: experimental projects with community collaborators
arXivLabs is a framework that allows collaborators to develop and share new arXiv features directly on our website.
Both individuals and organizations that work with arXivLabs have embraced and accepted our values of openness, community, excellence, and user data privacy. arXiv is committed to these values and only works with partners that adhere to them.
Have an idea for a project that will add value for arXiv's community? Learn more about arXivLabs .
share this!
May 17, 2024
This article has been reviewed according to Science X's editorial process and policies . Editors have highlighted the following attributes while ensuring the content's credibility:
fact-checked
peer-reviewed publication
trusted source
The tentacles of retracted science reach deep into social media: A simple button could change that
by University of Sydney
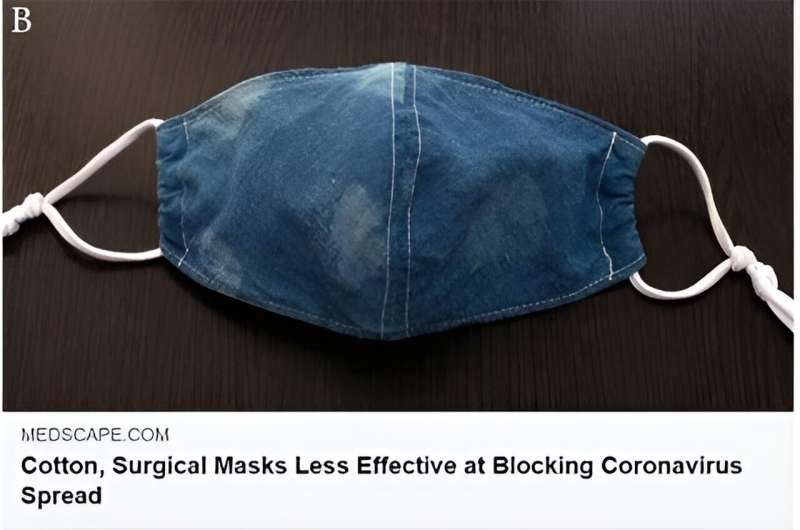
In 1998, a paper linking childhood vaccines with autism was published in the journal, The Lancet , only to be retracted in 2010 when the science was debunked.
Fourteen years since its retraction, the paper's original claim continues to flourish on social media, fueling misinformation and disinformation around vaccine safety and efficacy.
A University of Sydney team is hoping to help social media users identify posts featuring misinformation and disinformation arising from now-debunked science. They have developed and tested a new interface that helps users discover further information about potentially fraught claims on social media.
The study is published in the journal Proceedings of the ACM on Human-Computer Interaction .
They created and tested the efficacy of adding a "more information" button to social media posts . The button links to a drop down which allows users to see more details about claims or information in news posts, including information on whether that news is based on retracted science. The researchers say social media platforms could use an algorithm to link posts to details of retracted science.
Testing of the interface among a group of participants showed that when people understand the idea of retraction and can easily find when health news is based on a claim from retracted research, it can help reduce the impact and spread of misinformation as they are less likely to share it.
"Knowledge is power," said Professor Judy Kay from the School of Computer Science who led the research. "During the height of the COVID-19 pandemic, myths around the efficacy and safety of vaccines abounded. We want to help people to better understand when science has been debunked or challenged so they can make informed decisions about their health," she said.
"The ability to read and properly interpret often complex scientific papers is a very niche skill—not everybody has that literacy or is up to date on the latest science. Many people would have seen posts about now-debunked vaccine research and thought: 'It was published in a medical journal, so it must be true.' Sadly, that isn't the case for retracted publications."
"Social media platforms could do much better than they do now," said co-author and Ph.D. student Waheeb Yaqub. "During the height of the COVID-19 pandemic, myths around the efficacy and safety of vaccines spread like wildfire."
"Our approach shows that when people understand the idea of retraction and can find when health news is based on a retracted science article, it can reduce the impact and spread of misinformation," he said.
Tool boosts literacy of processes behind scientific research
The research was conducted with 44 participants who started with little or no understanding of scientific retraction. After completing a five-minute tutorial, they rated how various reasons for retraction make a paper's findings invalid.
The researchers then studied how participants used the "More Information" button. They found the new information altered the participants' beliefs on three health claims based on retracted papers shared on social media.
These claims were: whether masks are effective in limiting the spread of coronavirus; that the Mediterranean diet is effective in reducing heart disease ; and snacking while watching an action movie leads to overeating.
The first claim was based on two papers, one which had been retracted and one which hadn't. The other two claims were based on retracted papers. The researchers specifically chose papers of which participants would have differing knowledge.
"Participants confidently considered masks were effective. Most didn't know about the Mediterranean diet and so were unsure about whether it was true. Many people whose personal experience of snacking during films made them believe it was true."
The button influenced participants when they knew little about a topic to begin with. When the participants discovered the post was based on a retracted paper, they were less likely to like or share it.
On social media, both misinformation (the inadvertent spread of false information) and disinformation (false information deliberately spread with malicious intent), are rising.
Papers can be retracted when problems with methodology, results or experiments are found.
The researchers say it would be feasible for social media platforms to develop back-end software that links databases of retracted papers.
"If social media platforms want to maintain their quality and integrity, they should look to implement simple methods like ours," Professor Kay said.
Explore further
Feedback to editors

Q&A: Model disgorgement—the key to fixing AI bias and copyright infringement?
15 hours ago

Sun, sustainability, and silicon: A double dose of solar fuel research

Floating photovoltaics could limit Africa's future reliance on hydro-generated energy
17 hours ago

Orphan articles: The 'dark matter' of Wikipedia

Researchers find LLMs are easy to manipulate into giving harmful information
19 hours ago

A promising three-terminal diode for wireless communication and optically driven computing
23 hours ago

Scientists develop a soft robot that mimics a spider's leg
May 16, 2024

New research to make digital transactions quantum safe and 20 times faster

AI-powered noise-filtering headphones give users the power to choose what to hear

New advance in wireless communications could help precisely pinpoint the locations of people and objects
Related stories.

Flawed research not retracted fast enough to prevent spread of misinformation, study finds
Jun 15, 2022
Retracted scientific paper persists in new citations, study finds
Jan 5, 2021

Social media 'trust' or 'distrust' buttons could reduce spread of misinformation
Jun 6, 2023

Flawed scientific papers fueling COVID-19 misinformation
Jul 30, 2021

Stemming the spread of misinformation on social media
Jul 2, 2020

Understanding how news works can short-circuit the connection between social media use and vaccine hesitancy
Nov 3, 2022
Recommended for you

New browser extension empowers users to fight online misinformation

Managing screen time by making phones slightly more annoying to use
May 14, 2024

Google unleashes AI in search, raising hopes for better results and fears about less web traffic

Researchers develop a biomechanical dataset for badminton performance analysis
May 6, 2024

An affordable miniature car-like robot to test control and estimation algorithms
Apr 29, 2024
Let us know if there is a problem with our content
Use this form if you have come across a typo, inaccuracy or would like to send an edit request for the content on this page. For general inquiries, please use our contact form . For general feedback, use the public comments section below (please adhere to guidelines ).
Please select the most appropriate category to facilitate processing of your request
Thank you for taking time to provide your feedback to the editors.
Your feedback is important to us. However, we do not guarantee individual replies due to the high volume of messages.
E-mail the story
Your email address is used only to let the recipient know who sent the email. Neither your address nor the recipient's address will be used for any other purpose. The information you enter will appear in your e-mail message and is not retained by Tech Xplore in any form.
Your Privacy
This site uses cookies to assist with navigation, analyse your use of our services, collect data for ads personalisation and provide content from third parties. By using our site, you acknowledge that you have read and understand our Privacy Policy and Terms of Use .
E-mail newsletter
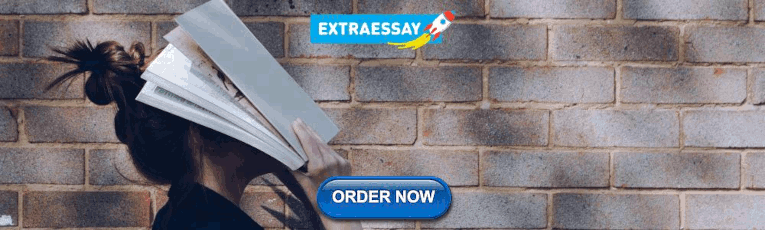
IMAGES
VIDEO
COMMENTS
Covers all theoretical and applied aspects at the intersection of computer science and game theory, including work in mechanism design, learning in games (which may overlap with Learning), foundations of agent modeling in games (which may overlap with Multiagent systems), coordination, specification and formal methods for non-cooperative computational environments.
Computer science is the study and development of the protocols required for automated processing and manipulation of data. This includes, for example, creating algorithms for efficiently searching ...
An artificial-intelligence graph neural network was trained on experimental data and used to identify chemical substructures that underlie selective antibiotic activity in more than 12 million ...
arXiv is a free distribution service and an open-access archive for nearly 2.4 million scholarly articles in the fields of physics, mathematics, computer science, quantitative biology, quantitative finance, statistics, electrical engineering and systems science, and economics. Materials on this site are not peer-reviewed by arXiv.
The increasing potential and challenges of digital twins. This issue of Nature Computational Science includes a Focus that highlights recent advancements, challenges, and opportunities in the ...
Explore the latest full-text research PDFs, articles, conference papers, preprints and more on COMPUTER SCIENCE. Find methods information, sources, references or conduct a literature review on ...
About the journal. Computer Science Review publishes research surveys and expository overviews of open problems in computer science. All articles are aimed at a general computer science audience seeking a full and expert overview of the latest developments across computer science research. Articles from other fields are welcome, as long as ...
Computers. Computers is an international, scientific, peer-reviewed, open access journal of computer science, including computer and network architecture and computer-human interaction as its main foci, published monthly online by MDPI. Open Access — free for readers, with article processing charges (APC) paid by authors or their institutions.
Open research in computer science. Spanning networks and communications to security and cryptology to big data, complexity, and analytics, SpringerOpen and BMC publish one of the leading open access portfolios in computer science. Learn about our journals and the research we publish here on this page.
Get 30 days free. 1. ACM Digital Library. ACM Digital Library is the clear number one when it comes to academic databases for computer science. The ACM Full-Text Collection currently has 540,000+ articles, while the ACM Guide to Computing Literature holds more than 2.8+ million bibliographic entries. Coverage: 2.8+ million articles. Abstracts: .
In this article, we study the evolution of the computer science research community over the past 30 years. Analyzing data from the full Scopus database, we investigate how aspects such as the community size, gender composition, and academic seniority of its members changed over time. We also shed light on the varying popularity of specific research areas, as derived from the ACM's Special ...
We also believe that highlighting excellent research will inspire others to enter the computing education field and make their own contributions.". The Top Ten Symposium Papers are: 1. " Identifying student misconceptions of programming " (2010) Lisa C. Kaczmarczyk, Elizabeth R. Petrick, University of California, San Diego; Philip East ...
Computer science ( CS ) majors are in high demand and account for a large part of national computer and information technology job market applicants. Employment in this sector is projected to grow 12% between 2018 and 2028, which is faster than the average of all other occupations. Published data are available on traditional non-computer ...
The ranking contains Impact Score values gathered on December 21st, 2022. The process for ranking journals involves examining more than 6,652 journals which were selected after detailed inspection and rigorous examination of over 99,245 scientific documents published during the last three years by 10,278 leading and well-respected scientists in the area of computer science.
AI-based predictive approach via FFB propagation in a driven-cavity of Ostwald de-Waele fluid using CFD-ANN and Levenberg-Marquardt. Ahmed Refaie Ali. , Rashid Mahmood. & Mohamed H. Behiry. Article.
Use these databases to find articles, papers from conference proceedings, and dissertations and theses ... A guide to finding articles and reference materials for students in the field of Computer Science. Find Articles & Papers; High-Impact Journals ... Compendex is the most comprehensive bibliographic database of scientific and technical ...
The Computer Science and Engineering guide provides links to information on all topics related to computer science and computer engineering in relevant databases, journals, conference proceedings, technical reports, websites, professional societies, etc. ... The basic parts of a scientific or technical paper are: Title and Author Information ...
This guide will help you get started searching the computer science "literature" for research papers on your topic and finding other resources such as books, journals, government websites, and published theses and dissertations. Be sure to visit the different pages of this guide using the tabs on the left.
A short work on how to read academic papers, organized as an academic paper. Some of the advice on doing a literature survey works better in the author's field (CS) but most the material works for everyone.
The Journal of Computer Science (JCS) is dedicated to advancing computer science by publishing high-quality research and review articles that span both theoretical foundations and practical applications in information, computation, and computer systems. With a commitment to excellence, JCS offers a platform for researchers, scholars, and ...
Large Language Models have recently gained significant attention in scientific discovery for their extensive knowledge and advanced reasoning capabilities. However, they encounter challenges in effectively simulating observational feedback and grounding it with language to propel advancements in physical scientific discovery. Conversely, human scientists undertake scientific discovery by ...
SCIgen is a program that generates random Computer Science research papers, including graphs, figures, and citations. It uses a hand-written context-free grammar to form all elements of the papers. Our aim here is to maximize amusement, rather than coherence. One useful purpose for such a program is to auto-generate submissions to conferences ...
3.3 million articles on ScienceDirect are open access. Articles published open access are peer-reviewed and made freely available for everyone to read, download and reuse in line with the user license displayed on the article. ScienceDirect is the world's leading source for scientific, technical, and medical research.
The journal Nature said in 2023 that an AI tool could not be a credited author on a research paper, and that any researchers using AI tools must document their use. Gray fears that these papers ...
Nick Hilden writes for the likes of the Washington Post, Esquire, Popular Science, National Geographic, the Daily Beast, and more.You can follow him on Twitter @nickhilden or Instagram @nick.hilden
Fig. 1: Science in the age of artificial intelligence. Scientific discovery is a multifaceted process that involves several interconnected stages, including hypothesis formation, experimental ...
Fake studies have flooded the publishers of top scientific journals, leading to thousands of retractions and millions of dollars in lost revenue. The biggest hit has come to Wiley, a 217-year-old ...
Recent advancements in large language models (LLMs) have achieved promising performances across various applications. Nonetheless, the ongoing challenge of integrating long-tail knowledge continues to impede the seamless adoption of LLMs in specialized domains. In this work, we introduce DALK, a.k.a. Dynamic Co-Augmentation of LLMs and KG, to address this limitation and demonstrate its ability ...
"Knowledge is power," said Professor Judy Kay from the School of Computer Science who led the research. "During the height of the COVID-19 pandemic, myths around the efficacy and safety of vaccines abounded. ... "The ability to read and properly interpret often complex scientific papers is a very niche skill—not everybody has that literacy or ...