College Students’ Time Management: a Self-Regulated Learning Perspective
- Review Article
- Published: 27 October 2020
- Volume 33 , pages 1319–1351, ( 2021 )
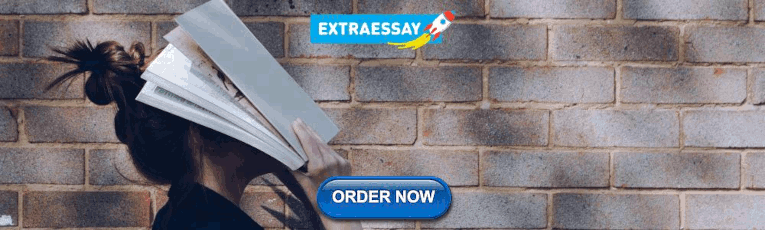
Cite this article
- Christopher A. Wolters ORCID: orcid.org/0000-0002-8406-038X 1 &
- Anna C. Brady 1
25k Accesses
58 Citations
10 Altmetric
Explore all metrics
Despite its recognized importance for academic success, much of the research investigating time management has proceeded without regard to a comprehensive theoretical model for understanding its connections to students’ engagement, learning, or achievement. Our central argument is that self-regulated learning provides the rich conceptual framework necessary for understanding college students’ time management and for guiding research examining its relationship to their academic success. We advance this larger purpose through four major sections. We begin by describing work supporting the significance of time management within post-secondary contexts. Next, we review the limited empirical findings linking time management and the motivational and strategic processes viewed as central to self-regulated learning. We then evaluate conceptual ties between time management and processes critical to the forethought, performance, and post-performance phases of self-regulated learning. Finally, we discuss commonalities in the antecedents and contextual determinants of self-regulated learning and time management. Throughout these sections, we identify avenues of research that would contribute to a greater understanding of time management and its fit within the framework of self-regulated learning. Together, these efforts demonstrate that time management is a significant self-regulatory process through which students actively manage when and for how long they engage in the activities deemed necessary for reaching their academic goals.
This is a preview of subscription content, log in via an institution to check access.
Access this article
Price includes VAT (Russian Federation)
Instant access to the full article PDF.
Rent this article via DeepDyve
Institutional subscriptions
Similar content being viewed by others
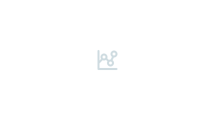
Examining the relations of time management and procrastination within a model of self-regulated learning
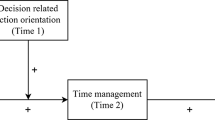
Willing, able, and engaged: roles of action-state orientation, intrinsic academic motivation, and time management on academic engagement
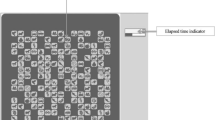
Are Mastery-Oriented College Students Better Time Managers?
Adams, G. A., & Jex, S. M. (1999). Relationships between time management, control, work family conflict, and strain. Journal of Occupational Health Psychology, 4 (1), 72–77.
Google Scholar
Aeon, B., & Aguinis, H. (2017). It’s about time: new perspectives and insights on time management. Academy of Management Perspectives, 31 , 309–330.
Ames, C. (1992). Classrooms: goals, structures, and student motivation. Journal of Educational Psychology, 84 , 261–271.
Asikainen, H., & Gijbels, D. (2017). Do students develop towards more deep approaches to learning during studies? A systematic review on the development of students’ deep and surface approaches to learning in higher education. Educational Psychology Review, 29 , 205–234.
Balduf, M. (2009). Underachievement among college students. Journal of Advanced Academics, 20 , 274–294.
Banahan, L., & Mullendore, R. (2014). Navigating the first college year. A guide for parents and families . Columbia: University of South Carolina, National Resource Center for The First-Year Experience and Students in Transition.
Bandura, A. (1986). The explanatory and predictive scope of self-efficacy theory. Journal of Social and Clinical Psychology, 4 , 359–373.
Bartels, J. M., Magun-Jackson, S., & Ryan, J. J. (2010). Dispositional approach-avoidance achievement motivation and cognitive self-regulated learning: the mediation of achievement goals. Individual Differences Research, 8 , 97–110.
Basila, C. (2014). Good time management and motivation level predict student academic success in college on-line courses. International Journal of Cyber Behavior, Psychology and Learning, 4 , 45–52.
Bembenutty, H. (2009). Academic delay of gratification, self-regulation of learning, gender differences, and expectancy-value. Personality and Individual Differences, 46 , 347–352.
Beuhler, R., Griffin, D., & Peetz, J. (2010). The planning fallacy: cognitive, motivational, and social origins. Advances in Experimental Social Psychology, 43 , 1–62.
Bidjerano, T., & Dai, D. Y. (2007). The relationship between the big-five model of personality and self-regulated learning strategies. Learning and Individual Differences, 17 , 69–81.
Boekaerts, M. (1996). Self-regulated learning at the junction of cognition and motivation. European Psychologist, 1 , 100–112.
Boekaerts, M., & Corno, L. (2005). Self-regulation in the classroom: a perspective on assessment and intervention. Applied Psychology: An International Review, 54 , 199–231.
Bond, M. J., & Feather, N. T. (1988). Some correlates of structure and purpose in the use of time. Journal of Personality and Social Psychology, 55 , 321–329.
Britton, B. K., & Glynn, S. M. (1989). Mental management and creativity: a cognitive model of time management for intellectual productivity. In J. Glover, R. Ronning, & C. Reynolds (Eds.), Handbook of creativity (pp. 429–440). New York: Plenum.
Britton, B. K., & Tesser, A. (1991). Effects of time-management practices on college grades. Journal of Educational Psychology, 83 , 405–410.
Buehler, R., & Griffin, D. (2015). When plans lead to optimistic forecasts. In M. D. Mumford & M. Frese (Eds.), The psychology in planning in organizations: research and applications (pp. 31–57). New York: Routledge.
Burlison, J. D., Murphy, C. S., & Dwyer, W. O. (2009). Evaluation of the Motivated Strategies for Learning Questionnaire for predicting academic performance in college students of varying scholastic aptitude. College Student Journal, 43 , 1313–1323.
Burnette, J., O’Boyle, E., VanEpps, E., Pollack, J., & Finkel, E. (2013). Mind-sets matter: a meta-analytic review of implicit theories and self-regulation. Psychological Bulletin, 139 (3), 655–701.
Burt, C. D., Weststrate, A., Brown, C., & Champion, F. (2010). Development of the time management environment (TiME) scale. Journal of Managerial Psychology, 25 , 649–668.
Butler, D., & Winne, P. (1995). Feedback and self-regulated learning: a theoretical synthesis. Review of Educational Research, 65 , 245–281.
Cano, F. (2006). An in-depth analysis of the Learning and Study Strategies Inventory (LASSI). Educational and Psychological Measurement, 66 , 1023–1038.
Capdeferro, N., Romero, M., & Barberà, E. (2014). Polychronicity: review of the literature and a new configuration for the study of this hidden dimension of online learning. Distance Education, 35 , 294–310.
Cassidy, S. (2011). Self-regulated learning in higher education: identifying key component processes. Studies in Higher Education, 36 , 989–1000.
Chang, A., & Nguyen, L. T. (2011). The mediating effects of time structure on the relationships between time management behaviour, job satisfaction, and psychological well-being. Australian Journal of Psychology, 63 , 187–197.
Choi, B. (2016). How people learn in an asynchronous online learning environment: the relationships between graduate students’ learning strategies and learning satisfaction. Canadian Journal of Learning and Technology, 42 , 1–15.
Chuderski, A. (2016). Time pressure prevents relational learning. Learning and Individual Differences, 49 , 361–365.
Claessens, B. J. C., van Eerde, W., Rutte, C. G., & Roe, R. A. (2007). A review of the time management literature. Personnel Review, 36 , 255–276.
Conti, R. (2001). Time flies: investigating the connection between intrinsic motivation and the experience of time. Journal of Personality, 69 (1), 1–26.
Corno, L., Cronbach, L. J., Kupermintz, H., Lohman, D. F., Mandinach, E. B., Porteus, A. W., & Talbert, J. E. (2002). Remaking the concept of aptitude: extending the legacy of Richard E Snow . Mahwah: Lawrence Erlbaum Associates Publishers.
Crede, M., & Kuncel, N. R. (2008). Study habits, skills, and attitudes: the third pillar supporting collegiate academic performance. Perspectives on Psychological Science, 3 (6), 425–453.
Crede, M., & Phillips, L. A. (2011). A meta-analytic review of the Motivated Strategies for Learning Questionnaire. Learning and Individual Differences, 21 , 337–346.
Crede, M., Roch, S. G., & Kieszczynka, U. M. (2010). Class attendance in college: a meta-analytic review of the relationship of class attendance with grades and student characteristics. Review of Educational Research, 80 , 272–295.
Csikszentmihalyi, M. (1996). Creativity: flow and the psychology of discovery and invention . New York: HarperCollins Publishers.
Dembo, M. H., & Eaton, M. J. (2000). Self-regulation of academic learning in middle-level schools. The Elementary School Journal, 100 , 473–490.
Dent, A., & Koenka, A. (2016). The relation between self-regulated learning and academic achievement across childhood and adolescence: a meta-analysis. Educational Psychology Review, 28 , 425–474.
Díaz-Morales, J. F., Ferrari, J. R., & Cohen, J. R. (2008). Indecision and avoidant procrastination: the role of morningness—eveningness and time perspective in chronic delay lifestyles. The Journal of General Psychology, 135 (3), 228–240.
Donker, A., de Boer, H., Kostons, D., Dignath van Ewijk, C., & van der Werf, M. (2014). Effectiveness of learning strategy instruction on academic performance: a meta-analysis. Educational Psychology Review, 11 , 1–26.
Douglas, H. E., Bore, M., & Munro, D. (2016). Coping with university education: the relationships of time management behaviour and work engagement with the five factor model aspects. Learning and Individual Differences, 45 , 268–274.
Doumen, S., Broeckmans, J., & Masui, C. (2014). The role of self-study time in freshmen’s achievement. Educational Psychology, 34 , 385–402.
Duncan, T. G., & McKeachie, W. J. (2005). The making of the motivated strategies for learning questionnaire. Educational Psychologist, 40 , 117–128.
Duncheon, J. C., & Tierney, W. G. (2013). Changing conceptions of time: implications for educational research and practice. Review of Educational Research, 83 , 236–272.
Dunlosky, J., & Ariel, R. (2011). Self-regulated learning and the allocation of study time. In B. Ross (Ed.), Psychology of learning and motivation (Vol. 54, pp. 103–140). San Diego: Academic Press.
Dunning, D., Heath, C., & Suls, J. (2004). Flawed self-assessments. Psychological Science in the Public Interest, 5 (3), 69–106.
Efklides, A. (2011). Interactions of metacognition with motivation and affect in self-regulated learning: the MASRL model. Educational Psychologist, 46 , 6–25.
Eilam, B., & Aharon, I. (2003). Students’ planning in the process of self-regulated learning. Contemporary Educational Psychology, 28 , 304–334.
Ferrari, J. R., & Díaz-Morales, J. F. (2007). Procrastination: different time orientations reflect different motives. Journal of Research in Personality, 41 , 707–714.
Flake, J. K., Barron, K. E., Hulleman, C., McCoach, B. D., & Welsh, M. E. (2015). Measuring cost: the forgotten component of expectancy-value theory. Contemporary Educational Psychology, 41 , 232–244.
Flanigan, A. E., & Kiewra, K. A. (2018). What college instructors can do about student cyber-slacking. Educational Psychology Review, 30 , 585–597.
Francis-Smythe, J. A., & Robertson, I. T. (1999a). On the relationship between time management and time estimation. British Journal of Psychology, 90 , 333–347.
Francis-Smythe, J. A., & Robertson, I. T. (1999b). Time-related individual differences. Time & Society, 8 , 273–292.
Fromme, K., Corbin, W., & Kruse, M. (2008). Behavioral risks during the transition from high school to college. Developmental Psychology, 44 (5), 1497–1504.
Gable, P. A., & Poole, B. D. (2012). Time flies when you’re having approach-motivated fun: effects of motivational intensity on time perception. Psychological Science, 23 (8), 879–886.
Gevers, J. M., Rutte, C. G., & Van Eerde, W. (2006). Meeting deadlines in work groups: implicit and explicit mechanisms. Applied Psychology, 55 , 52–72.
Green, P., & Skinner, D. (2005). Does time management training work? An evaluation. International Journal of Training and Development, 9 , 124–139.
Gulec, M., Selvi, Y., Boysan, M., Aydin, A., Oral, E., & Aydin, E. F. (2013). Chronotype effects on general well-being and psychopathology levels in healthy young adults. Biological Rhythm Research, 44 , 457–468.
Guzman, G., Goldberg, T., & Swanson, H. (2018). A meta-analysis of self-monitoring on reading performance of K-12 students. School Psychology Quarterly, 33 (1), 160–168.
Hadwin, A., & Oshige, M. (2011). Self-regulation, coregulation, and socially shared regulation: exploring perspectives of social in self-regulated learning theory. Teachers College Record, 113 , 240–264.
Hafner, A., Stock, A., Pinneker, L., & Strohle, S. (2014). Stress prevention through a time management training intervention: an experimental study. Educational Psychology, 34 , 403–416.
Hahn, C., Cowell, J. M., Wiprzycka, U. J., Goldstein, D., Ralph, M., Hasher, L., & Zelazo, P. D. (2012). Circadian rhythms in executive function during the transition to adolescence: the effect of synchrony between chronotype and time of day. Developmental Science, 15 (3), 408–416.
Harkin, B., Webb, T. L., Chang, B. P. I., Prestwich, A., Conner, M., Kellar, I., et al. (2016). Does monitoring goal progress promote goal attainment? A meta-analysis of the experimental evidence. Psychological Bulletin, 142 (2), 198–229.
Hattie, J., Biggs, J., & Purdie, N. (1996). Effects of learning skills interventions on student learning: a meta-analysis. Review of Educational Research, 66 , 99–136.
Haynes, N., Comer, J., & Hamilton-Lee, M. (1988). Gender and achievement status differences on learning factors among Black high school students. Journal of Educational Research, 81 , 233–237.
Hensley, L. C., Wolters, C. A., Won, S., & Brady, A. C. (2018). Academic probation, time management, and time use in a college success course. Journal of College Reading and Learning, 48 , 105–123.
Hicks, T., & Heastie, S. (2008). High school to college transition: a profile of the stressors, physical and psychological health issues that affect the first-year on-campus college students. Journal of Cultural Diversity, 15 (3), 143–147.
Hilbrecht, M., Zuzanek, J., & Mannell, R. C. (2008). Time use, time pressure and gendered behavior in early and late adolescence. Sex Roles, 58 , 342–357.
Hofer, B. K., Yu, S. L., & Pintrich, P. R. (1998). Teaching college students to be self-regulated learners. In D. H. Schunk & B. J. Zimmerman (Eds.), Self-regulated learning: from teaching to self-reflective practice (pp. 57–85). New York: Guilford Press.
Horstmanshof, L., & Zimitat, C. (2007). Future time orientation predicts academic engagement among first-year university students. British Journal of Educational Psychology, 77 (Pt 3), 703–718.
Huie, F. C., Winsler, A., & Kitsantas, A. (2014). Employment and first-year college achievement: the role of self-regulation and motivation. Journal of Education and Work, 27 , 110–135.
Husman, J., & Lens, W. (1999). The role of the future in student motivation. Educational Psychologist, 34 , 113–125.
Kanfer, R., Frese, M., & Johnson, R. (2017). Motivation related to work: a century of progress. Journal of Applied Psychology, 102 (3), 338–355.
Kantrowitz, T. M., Grelle, D. M., Beaty, J. C., & Wolf, M. B. (2012). Time is money: polychronicity as a predictor of performance across job levels. Human Performance, 25 , 114–137.
Kauderer, S., & Randler, C. (2013). Differences in time use among chronotypes in adolescents. Biological Rhythm Research, 44 , 601–608.
Kaufman-Scarborough, C., & Lindquist, J. D. (1999). Time management and polychronicity: comparisons, contrasts, and insights for the workplace. Journal of Managerial Psychology, 14 , 288–312.
Keating, D. P. (2012). Cognitive and brain development in adolescence. Enfance, 64 , 267–279.
Kesici, Ş., Baloğlu, M., & Deniz, M. E. (2011). Self-regulated learning strategies in relation with statistics anxiety. Learning and Individual Differences, 21 , 472–477.
Kim, K. R., & Seo, E. H. (2015). The relationship between procrastination and academic performance: a meta-analysis. Personality and Individual Differences, 82 , 26–33.
Kitsantas, A., Winsler, A., & Huie, F. (2008). Self-regulation and ability predictors of academic success during college: a predictive validity study. Journal of Advanced Academics, 20 , 42–68.
Koch, C. J., & Kleinmann, M. (2002). A stitch in time saves nine: behavioural decision-making explanations for time management problem. European Journal of Work and Organizational Psychology, 11 , 199–217.
Komarraju, M., Karau, S. J., & Schmeck, R. R. (2009). Role of the Big Five personality traits in predicting college students’ academic motivation and achievement. Learning and Individual Differences, 19 , 47–52.
Konig, C. J., & Waller, M. J. (2010). Time for reflection: a critical examination of polychronicity. Human Performance, 23 , 173–190.
Kooij, D. T. A. M., Kanfer, R., Betts, M., & Rudolph, C. W. (2018). Future time perspective: a systematic review and meta-analysis. Journal of Applied Psychology, 103 (8), 867–893.
Krumrei-Mancuso, E. J., Newton, F. B., Kim, E., & Wilcox, D. (2013). Psychosocial factors predicting first-year college student success. Journal of College Student Development, 54 , 247–266.
Landrum, R. E., Turrisi, R., & Brandel, J. M. (2006). College students’ study time: course level, time of semester, and grade earned. Psychological Reports, 98 (3), 675–682.
Liborius, P., Bellhauser, H., & Schmitz, B. (2017). What makes a good study day? An intraindividual study on university students’ time investment by means of time-series analyses. Learning and Instruction.
Linnenbrink-Garcia, L., & Patall, E. (2016). Motivation. In L. Corno & E. Anderman (Eds.), Handbook of educational psychology (3rd ed., pp. 91–1030). New York: Routledge.
Liu, O. L., Rijmen, F., MacCann, C., & Roberts, R. (2009). The assessment of time management in middle-school students. Personality and Individual Differences, 47 , 174–179.
Locke, E., & Latham, G. (2019). The development of goal setting theory: a half-century retrospective. Motivation Science, 5 , 93–105.
Loureiro, F., & Garcia-Marques, T. (2015). Morning or evening person? Which type are you? Self-assessment of chronotype. Personality and Individual Differences, 86 , 168–171.
Lynch, D. J. (2010). Application of online discussion and cooperative learning strategies to online and blended college courses. College Student Journal, 44 , 920–927.
Macan, T. H. (1994). Time management: test of a process model. Journal of Applied Psychology, 79 , 381–391.
Macan, T. H., Shahani, C., Dipboye, R. L., & Phillips, A. P. (1990). College students’ time management: correlations with academic performance and stress. Journal of Educational Psychology, 82 , 760–768.
MacCann, C., & Roberts, R. D. (2010). Do time management, grit, and self-control relate to academic achievement independently of conscientiousness? In R. E. Hicks (Ed.), Personality and individual differences: current directions (pp. 79–90). Bowen Hills: Australian Academic Press.
MacCann, C., Fogarty, G. J., & Roberts, R. D. (2012). Strategies for success in education: time management is more important for part-time than full-time community college students. Learning and Individual Differences, 22 , 618–623.
McCrae, R., & Lockenhoff, C. (2010). Self-regulation and the five-factor model of personality traits. In R. Hoyle (Ed.), Handbook of personality and self-regulation (pp. 143–168). New York: Wiley.
McInerney, D., & King, R. (2018). Culture and self-regulation in educational contexts. In D. Schunk & J. Greene (Eds.), Handbook of self-regulation of learning and performance (2nd ed., pp. 485–502). New York: Routledge.
Meeuwisse, M., Born, M. P., & Severiens, S. E. (2013). Academic performance differences among ethnic groups: do the daily use and management of time offer explanations? Social Psychology of Education, 16 , 599–615.
Melancon, J. G. (2002). Reliability, structure, and correlates of Learning and Study Strategies Inventory scores. Educational and Psychological Measurement, 62 , 1020–1027.
Miele, D. B., & Scholer, A. A. (2018). The role of metamotivational monitoring in motivation regulation. Educational Psychologist, 53 , 1–21.
Miller, R. B., & Brickman, S. J. (2004). A model of future-oriented motivation and self-regulation. Educational Psychology Review, 16 , 9–33.
Muis, K. R. (2007). The role of epistemic beliefs in self-regulated learning. Educational Psychologist, 42 , 173–190.
Nelson, T., & Narens, L. (1990). Metamemory: a theoretical framework and some new findings. In G. H. Bower (Ed.), The psychology of learning and motivation (pp. 125–173). New York: Academic Press.
Nonis, S., Teng, J., & Ford, C. (2005). A cross-cultural investigation of time management practices and job outcomes. International Journal of Intercultural Relations, 29 , 409–428.
Nonis, S. A., Philhours, M. J., & Hudson, G. I. (2006). Where does the time go? A diary approach to business and marketing students’ time use. Journal of Marketing Education, 28 , 121–134.
Olaussen, B. S., & Bråten, I. (1998). Identifying latent variables measured by the Learning and Study Strategies Inventory (LASSI) in Norwegian college students. The Journal of Experimental Education, 67 , 82–96.
Olejnik, S., & Nist, S. L. (1992). Identifying latent variables measured by the Learning and Study Strategies Inventory (LASSI). The Journal of Experimental Education, 60 , 151–159.
Panadero, E. (2017). A review of self-regulated learning: six models and four directions for research. Frontiers in Psychology, 8 , 1–28.
Panadero, E., Brown, G., & Strijbos, J. (2016). The future of student self-assessment: a review of known and unknowns and potential directions. Educational Psychology Review, 28 (803), 830.
Panek, E. (2014). Left to their own devices: college students’ “guilty pleasure” media use and time management. Communication Research, 41 , 561–577.
Pekrun, R., Goetz, T., Titz, W., & Perry, R. P. (2002). Academic emotions in students’ self-regulated learning and achievement: a program of qualitative and quantitative research. Educational Psychologist, 37 , 91–105.
Pintrich, P. R. (2000). The role of goal orientation in self-regulated learning. In M. Boekaerts, P. R. Pintrich, & M. Zeidner (Eds.), Handbook of self-regulation (pp. 451–502). San Diego: Academic.
Pintrich, P. R. (2004). A conceptual framework for assessing motivation and self-regulated learning in college students. Educational Psychology Review, 16 , 385–407.
Pintrich, P. R., & Zusho, A. (2007). Student motivation and self-regulated learning in the college classroom. In R. P. Perry & J. C. Smart (Eds.), The scholarship of teaching and learning in higher education: an evidence based perspective (pp. 731–810). New York: Springer.
Pintrich, P. R., Smith, D. A., Garcia, T., & McKeachie, W. J. (1993). Reliability and predictive validity of the Motivated Strategies for Learning Questionnaire. Educational and Psychological Measurement, 53 , 801–813.
Pintrich, P. R., Wolters, C. A., & Baxter, G. P. (2000). Assessing metacognition and self-regulated learning. In G. Schraw & J. Impara (Eds.), Issues in the measurement of metacognition (pp. 43–97). Lincoln: Buros Institute of Mental Measurement.
Plant, E. A., Ericsson, K. A., Hill, L., & Asberg, K. (2005). Why study time does not predict grade point average across college students: implications of deliberate practice for academic performance. Contemporary Educational Psychology, 30 , 96–116.
Prevatt, F., Petscher, Y., Proctor, B. E., Hurst, A., & Adams, K. (2006). The revised Learning and Study Strategies Inventory: an evaluation of competing models. Educational and Psychological Measurement, 66 (3), 448–458.
Pychyl, T. A., Morin, R. W., & Salmon, B. R. (2000). Procrastination and the planning fallacy: an examination of the study habits of university students. Journal of Social Behavior and Personality, 15 , 135–150.
Ranellucci, J., Hall, N. C., & Goetz, T. (2015). Achievement goals, emotions, learning, and performance: a process model. Motivation Science, 1 , 98–120.
Rhodes, M., & Tauber, S. (2011). The influence of delaying judgments of learning on metacognitive accuracy: a meta-analytic review. Psychological Bulletin, 137 (1), 131–148.
Richards, J. H. (1987). Time management—a review. Work & Stress, 1 , 73–78.
Rickert, N. P., Meras, I. L., & Witkow, M. R. (2014). Theories of intelligence and students’ daily self-handicapping behaviors. Learning and Individual Differences, 36 , 1–8.
Roskes, M., Elliot, A. J., Nijstad, B. A., & De Dreu, C. K. (2013). Time pressure undermines performance more under avoidance than approach motivation. Personality and Social Psychology Bulletin, 39 , 803–813.
Rytkonen, H., Parpala, A., Lindblom-Ylanne, S., Virtanen, V., & Postareff, L. (2012). Factors affecting bioscience students’ academic achievement. Instructional Science, 40 , 241–256.
Sanders, L., Reedy, D., & Frizell, M. (Eds.). (2018). Learning centers in the 21 st century: a modern guide for learning assistance professionals in higher education . Bentonville: AR. Iona Press.
Sansgiry, S. S., Bhosle, M., & Sail, K. (2006). Factors that affect academic performance among pharmacy students. American Journal of Pharmaceutical Education, 70 , 1–9.
Schell, K. L., & Conte, J. M. (2008). Associations among polychronicity, goal orientation, and error orientation. Personality and Individual Differences, 44 , 288–298.
Schraw, G. (2006). Knowledge: structures and processes. In P. Alexander & P. Winne (Eds.), Handbook of educational psychology (2nd ed., pp. 245–263). Mahwah: Erlbaum Associates.
Schunk, D. H., & Zimmerman, B. J. (1997). Social origins of self-regulatory competence. Educational Psychologist, 32 , 195–208.
Schunk, D. H., & Zimmerman, B. J. (Eds.). (1998). Self-regulated learning: from teaching to self-reflective practice . New York: Guilford Press.
Sen, S., & Yilmaz, A. (2016). Devising a structural equation model of relationships between preservice teachers’ time and study environment management, effort regulation, self-efficacy, control of learning beliefs, and metacognitive self-regulation. Science Education International, 27 , 301–316.
Shaunessy-Dedrick, E., Suldo, S. M., Roth, R. A., & Fefer, S. A. (2015). Students’ perceptions of factors that contribute to risk and success in accelerated high school courses. The High School Journal, 98 , 109–137.
Shell, D. F., & Husman, J. (2001). The multivariate dimensionality of personal control and future time perspective beliefs in achievement and self-regulation. Contemporary Educational Psychology, 26 (4), 481–506.
Simons, J., Vansteenkiste, M., Lens, W., & Lacante, M. (2004). Placing motivation and future time perspective theory in a temporal perspective. Educational Psychology Review, 16 , 121–139.
Sinatra, G., Kienhues, D., & Hofer, B. (2014). Addressing challenges to public understanding of science: epistemic cognition, motivated reasoning, and conceptual change. Educational Psychologist, 49 , 123–138.
Sirois, F. M. (2014). Procrastination and stress: exploring the role of self-compassion. Self and Identity, 13 , 128–145.
Sitzmann, T., & Ely, K. (2011). A meta-analysis of self-regulated learning in work-related training and educational attainment: what we know and where we need to go. Psychological Bulletin, 137 (3), 421–442.
Sitzmann, T., Ely, K., Brown, K., & Bauer, K. (2010). Self-assessment of knowledge: a cognitive learning or affective measure? Academy of Management Learning & Education, 9 , 169–191.
Snow, R. (1989). Cognitive-conative aptitude interactions in learning. In R. Kanfer, P. Ackerman, & R. Cudeck (Eds.), Abilities, motivation, and methodology: the Minnesota symposium on learning and individual differences (pp. 435–474). Hillsdale: Erlbaum Associates.
Snow, R. E., Corno, L., & Jackson III, D. (1996). Individual differences in affective and conative functions. In D. C. Berliner & R. C. Calfee (Eds.), Handbook of educational psychology (pp. 243–310). New York: Macmillan Library Reference.
Soderstrom, N. C., & Bjork, R. A. (2014). Testing facilitates the regulation of subsequent study time. Journal of Memory and Language, 73 , 99–115.
Steel, P. (2007). The nature of procrastination: a meta-analytic and theoretical review of quintessential self-regulatory failure. Psychological Bulletin, 133 (1), 65–94.
Stevens, T., & Tallent-Runnels, M. K. (2004). The learning and study strategies inventory-high school version: issues of factorial invariance across gender and ethnicity. Educational and Psychological Measurement, 64 , 332–346.
Strunk, K. K., Cho, Y., Steele, M. R., & Bridges, S. L. (2013). Development and validation of a 2× 2 model of time-related academic behavior: procrastination and timely engagement. Learning and Individual Differences, 25 , 35–44.
Tanner, J. R., Stewart, G., Maples, G. M., & Totaro, M. W. (2009). How business students spend their time—do they really know? Research in Higher Education Journal, 3 , 1–9.
Terenzini, P., Rendon, L., Upcraft, M. L., Millar, S., Allison, K., Gregg, P., & Jalomo, R. (1994). The transition to college: diverse students, diverse stories. Research in Higher Education, 35 , 57–73.
Trueman, M., & Hartley, J. (1996). A comparison between the time-management skills and academic performance of mature and traditional-entry university students. Higher Education, 32 , 199–215.
Truschel, J., & Reedy, D. L. (2009). National survey—what is a learning center in the 21st century? Learning Assistance Review, 14 , 9–22.
Tsai, H. C., & Liu, S. H. (2015). Relationships between time-management skills, Facebook interpersonal skills and academic achievement among junior high school students. Social Psychology of Education, 18 , 503–516.
U.S. Department of Education, Institute of Education Sciences, What Works Clearinghouse (2016). Studies of interventions for students in developmental education intervention report: first year experience courses for students in developmental education . Retrieved from http://whatworks.ed.gov . Accessed 20 Jan 2020.
Urdan, T., & Schoenfelder, E. (2006). Classroom effects on student motivation: goal structures, social relationships, and competence beliefs. Journal of School Psychology, 44 , 331–349.
Usher, E. L., & Pajares, F. (2008). Sources of self-efficacy in school: critical review of the literature and future directions. Review of Educational Research, 78 , 751–796.
van Den Hurk, M. (2006). The relation between self-regulated strategies and individual study time, prepared participation and achievement in a problem-based curriculum. Active Learning in Higher Education, 7 , 155–169.
van der Meer, J., Jansen, E., & Torenbeek, M. (2010). ‘It’s almost a mindset that teachers need to change’: first-year students’ need to be inducted into time management. Studies in Higher Education, 35 , 777–791.
van Eerde, W. (2015). Time management and procrastination. In M. D. Mumford & M. Frese (Eds.), The psychology of planning in organizations: research and applications (pp. 312–333). New York: Routledge.
Wagner, P., Schober, B., & Spiel, C. (2008). Time students spend working at home for school. Learning and Instruction, 18 , 309–320.
Weiner, B. (2005). Motivation from an attribution perspective and the social psychology of perceived competence. In A. Elliot & C. Dweck (Eds.), Handbook of competence and motivation (pp. 73–84). New York: Guildford.
Weinstein, C. E., Palmer, D. R., & Acee, T. W. (2016). LASSI user’s manual (3th ed.). Clearwater: H & H Pub.
Winne, P. H. (1995). Inherent details in self-regulated learning. Educational Psychologist, 30 , 173–187.
Winne, P. H., & Baker, R. (2013). The potentials of educational data mining for researching metacognition, motivation, and self-regulated learning. Journal of Educational Data Mining, 5 , 1–8.
Winne, P., & Hadwin, A. (1998). Studying as self-regulated learning. In D. Hacker, J. Dunlosky, & A. Graesser (Eds.), Metacognition in educational theory and practice (pp. 277–304). Mahwah: Erlbaum.
Winne, P. H., & Hadwin, A. F. (2008). The weave of motivation and self-regulated learning. Motivation and self-regulated learning: theory, research, and applications (pp. 297–314). New York: Taylor & Francis.
Winne, P., & Perry, N. (2000). Measuring self-regulated learning. In M. Boekaerts, P. Pintrich, & M. Zeidner (Eds.), Handbook of self-regulation (pp. 531–566). San Diego: Academic Press.
Witkow, M. R. (2009). Academic achievement and adolescents’ daily time use in the social and academic domains. Journal of Research on Adolescence, 19 , 151–172.
Wolters, C. A. (2003). Regulation of motivation: evaluating an underemphasized aspect of self-regulated learning. Educational Psychologist, 38 , 189–205.
Wolters, C. A. (2011). Regulation of motivation: contextual and social aspects. Teachers College Record, 113 , 265–283.
Wolters, C. A., & Gonzalez, A. L. (2008). Classroom climate and motivation: a step toward integration. Advances in Motivation and Achievement: Social Psychological Influences, 15 , 493–519.
Wolters, C. A., & Hoops, L. D. (2015). Self-regulated learning interventions for motivationally disengaged college students. In T. L. Cleary (Ed.), Self-regulated learning interventions with at-risk youth: Enhancing adaptability, performance, and well-being (pp. 67–88). Washington: American Psychological Association.
Wolters, C., & Taylor, D. (2012). A self-regulated learning perspective on student engagement. In S. L. Christenson, A. Reschly, & C. Wylie (Eds.), Handbook of research on student engagement (pp. 635–651). New York: Springer.
Wolters, C. A., & Won, S. (2018). Validity and the use of self-report questionnaires to assess self-regulated learning. In D. H. Schunk & J. A. Greene (Eds.), Handbook of self-regulation of learning and performance (pp. 307–322). New York: Routledge.
Wolters, C. A., Won, S., & Hussain, M. (2017). Examining the relations of time management and procrastination within a model of self-regulated learning. Metacognition and Learning, 12 , 381–399.
Won, S., & Yu, S. L. (2018). Relations of perceived parental autonomy support and control with adolescents’ academic time management and procrastination. Learning and Individual Differences, 61 , 205–215.
Woolfolk, A. E., & Woolfolk, R. L. (1986). Time management: an experimental investigation. Journal of School Psychology, 24 , 267–275.
Xie, K., Heddy, B. C., & Greene, B. A. (2019). Affordances of using mobile technology to support experience-sampling method in examining college students’ engagement. Computers & Education, 128 , 183–198.
Xu, J. (2008). Models of secondary school students’ interest in homework: a multilevel analysis. American Educational Research Journal, 45 , 1180–1205.
Xu, J. (2010). Predicting homework time management at the secondary school level: a multilevel analysis. Learning and Individual Differences, 20 , 34–39.
Xu, J., Du, J., & Fan, X. (2013). “Finding our time”: predicting students’ time management in online collaborative groupwork. Computers & Education, 69 , 139–147.
Xu, J., Yuan, R., Xu, B., & Xu, M. (2014). Modeling students’ time management in math homework. Learning and Individual Differences, 34 , 33–42.
Yamada, M., Goda, Y., Matsuda, T., Saito, Y., Kato, H., & Miyagawa, H. (2016). How does self-regulated learning relate to active procrastination and other learning behaviors? Journal of Computing in Higher Education, 28 , 326–343.
Young, D. G., & Hopp, J. M. (2014). 2012-2013 national survey of first-year seminars: exploring high-impact practices in the first college year (Research report No. 4) . Columbia: University of South Carolina.
Zimbardo, P., & Boyd, J. (1999). Putting time in perspective: a valid, reliable individual-differences metric. Journal of Personality and Social Psychology, 77 , 1271–1288.
Zimmerman, B. J. (1989). A social cognitive view of self-regulated academic learning. Journal of Educational Psychology, 81 , 329–339.
Zimmerman, B. J. (2000). Attaining self-regulation: a social cognitive perspective. In M. Boekaerts, P. R. Pintrich, & M. Zeidner (Eds.), Handbook of self-regulation: theory, research, and applications (pp. 13–29). San Diego: Academic Press.
Zimmerman, B. J., & Risemberg, R. (1997). Becoming a self-regulated writer: a social cognitive perspective. Contemporary Educational Psychology, 22 , 73–101.
Zimmerman, B. J., & Schunk, D. (2012). Motivation: an essential dimension of self-regulated learning. In D. Schunk & B. Zimmerman (Eds.), Motivation and self-regulated learning: theory, research and applications (pp. 1–30). New York: Routledge.
Zimmerman, B. J., Greenberg, D., & Weinstein, C. E. (1994). Self-regulating academic study time: a strategy approach. In D. H. Schunk & B. J. Zimmerman (Eds.), Self-regulation of learning and performance: issues and educational applications (pp. 181–199). Hillsdale: Erlbaum.
Zusho, A. (2017). Toward an integrated model of student learning in the college classroom. Educational Psychology Review, 29 , 301–324.
Zusho, A., & Pintrich, P. (2003). Skill and will: the role of motivation and cognition in the learning of college chemistry. International Journal of Science Education, 25 , 1081–1094.
Download references
Author information
Authors and affiliations.
Dennis Learning Center, The Ohio State University, 250B Younkin Success Building, 1640 Neil Ave., Columbus, OH, 43201, USA
Christopher A. Wolters & Anna C. Brady
You can also search for this author in PubMed Google Scholar
Corresponding author
Correspondence to Christopher A. Wolters .
Ethics declarations
Conflict of interest.
The authors declare that they have no conflict of interest.
Additional information
Publisher’s note.
Springer Nature remains neutral with regard to jurisdictional claims in published maps and institutional affiliations.
Rights and permissions
Reprints and permissions
About this article
Wolters, C.A., Brady, A.C. College Students’ Time Management: a Self-Regulated Learning Perspective. Educ Psychol Rev 33 , 1319–1351 (2021). https://doi.org/10.1007/s10648-020-09519-z
Download citation
Published : 27 October 2020
Issue Date : December 2021
DOI : https://doi.org/10.1007/s10648-020-09519-z
Share this article
Anyone you share the following link with will be able to read this content:
Sorry, a shareable link is not currently available for this article.
Provided by the Springer Nature SharedIt content-sharing initiative
- Self-regulated learning
- Time management
- Postsecondary students
- Find a journal
- Publish with us
- Track your research

An official website of the United States government
The .gov means it’s official. Federal government websites often end in .gov or .mil. Before sharing sensitive information, make sure you’re on a federal government site.
The site is secure. The https:// ensures that you are connecting to the official website and that any information you provide is encrypted and transmitted securely.
- Publications
- Account settings
Preview improvements coming to the PMC website in October 2024. Learn More or Try it out now .
- Advanced Search
- Journal List
- Behav Sci (Basel)

Assessing Cognitive Factors of Modular Distance Learning of K-12 Students Amidst the COVID-19 Pandemic towards Academic Achievements and Satisfaction
Yung-tsan jou.
1 Department of Industrial and Systems Engineering, Chung Yuan Christian University, Taoyuan 320, Taiwan; wt.ude.ucyc@uojty (Y.-T.J.); moc.oohay@enimrahcrolfas (C.S.S.)
Klint Allen Mariñas
2 School of Industrial Engineering and Engineering Management, Mapua University, Manila 1002, Philippines
3 Department of Industrial Engineering, Occidental Mindoro State College, San Jose 5100, Philippines
Charmine Sheena Saflor
Associated data.
Not applicable.
The COVID-19 pandemic brought extraordinary challenges to K-12 students in using modular distance learning. According to Transactional Distance Theory (TDT), which is defined as understanding the effects of distance learning in the cognitive domain, the current study constructs a theoretical framework to measure student satisfaction and Bloom’s Taxonomy Theory (BTT) to measure students’ academic achievements. This study aims to evaluate and identify the possible cognitive capacity influencing K-12 students’ academic achievements and satisfaction with modular distance learning during this new phenomenon. A survey questionnaire was completed through an online form by 252 K-12 students from the different institutions of Occidental Mindoro. Using Structural Equation Modeling (SEM), the researcher analyses the relationship between the dependent and independent variables. The model used in this research illustrates cognitive factors associated with adopting modular distance learning based on students’ academic achievements and satisfaction. The study revealed that students’ background, experience, behavior, and instructor interaction positively affected their satisfaction. While the effects of the students’ performance, understanding, and perceived effectiveness were wholly aligned with their academic achievements. The findings of the model with solid support of the integrative association between TDT and BTT theories could guide decision-makers in institutions to implement, evaluate, and utilize modular distance learning in their education systems.
1. Introduction
The 2019 coronavirus is the latest infectious disease to develop rapidly worldwide [ 1 ], affecting economic stability, global health, and education. Most countries have suspended thee-to-face classes in order to curb the spread of the virus and reduce infections [ 2 ]. One of the sectors impacted has been education, resulting in the suspension of face-to-face classes to avoid spreading the virus. The Department of Education (DepEd) has introduced modular distance learning for K-12 students to ensure continuity of learning during the COVID-19 pandemic. According to Malipot (2020), modular learning is one of the most popular sorts of distance learning alternatives to traditional face-to-face learning [ 3 ]. As per DepEd’s Learner Enrolment and Survey Forms, 7.2 million enrollees preferred “modular” remote learning, TV and radio-based practice, and other modalities, while two million enrollees preferred online learning. It is a method of learning that is currently being used based on the preferred distance learning mode of the students and parents through the survey conducted by the Department of Education (DepEd); this learning method is mainly done through the use of printed and digital modules [ 4 ]. It also concerns first-year students in rural areas; the place net is no longer available for online learning. Supporting the findings of Ambayon (2020), modular teaching within the teach-learn method is more practical than traditional educational methods because students learn at their own pace during this modular approach. This educational platform allows K-12 students to interact in self-paced textual matter or digital copy modules. With these COVID-19 outbreaks, some issues concerned students’ academic, and the factors associated with students’ psychological status during the COVID-19 lockdown [ 5 ].
Additionally, this new learning platform, modular distance learning, seems to have impacted students’ ability to discover and challenged their learning skills. Scholars have also paid close attention to learner satisfaction and academic achievement when it involves distance learning studies and have used a spread of theoretical frameworks to assess learner satisfaction and educational outcomes [ 6 , 7 ]. Because this study aimed to boost academic achievement and satisfaction in K-12 students, the researcher thoroughly applied transactional distance theory (TDT) to understand the consequences of distance in relationships in education. The TDT was utilized since it has the capability to establish the psychological and communication factors between the learners and the instructors in distance education that could eventually help researchers in identifying the variables that might affect students’ academic achievement and satisfaction [ 8 ]. In this view, distance learning is primarily determined by the number of dialogues between student and teacher and the degree of structuring of the course design. It contributes to the core objective of the degree to boost students’ modular learning experiences in terms of satisfaction. On the other hand, Bloom’s Taxonomy Theory (BTT) was applied to investigate the students’ academic achievements through modular distance learning [ 6 ]. Bloom’s theory was employed in addition to TDT during this study to enhance students’ modular educational experiences. Moreover, TDT was utilized to check students’ modular learning experiences in conjuction with enhacing students’ achievements.
This study aimed to detect the impact of modular distance learning on K-12 students during the COVID-19 pandemic and assess the cognitive factors affecting academic achievement and student satisfaction. Despite the challenging status of the COVID-19 outbreak, the researcher anticipated a relevant result of modular distance learning and pedagogical changes in students, including the cognitive factors identified during this paper as latent variables as possible predictors for the utilization of K-12 student academic achievements and satisfaction.
1.1. Theoretical Research Framework
This study used TDT to assess student satisfaction and Bloom’s theory to quantify academic achievement. It aimed to assess the impact of modular distance learning on academic achievement and student satisfaction among K-12 students. The Transactional Distance Theory (TDT) was selected for this study since it refers to student-instructor distance learning. TDT Moore (1993) states that distance education is “the universe of teacher-learner connections when learners and teachers are separated by place and time.” Moore’s (1990) concept of ”Transactional Distance” adopts the distance that occurs in all linkages in education, according to TDT Moore (1993). Transactional distance theory is theoretically critical because it states that the most important distance is transactional in distance education, rather than geographical or temporal [ 9 , 10 ]. According to Garrison (2000), transactional distance theory is essential in directing the complicated experience of a cognitive process such as distance teaching and learning. TDT evaluates the role of each of these factors (student perception, discourse, and class organization), which can help with student satisfaction research [ 11 ]. Bloom’s Taxonomy is a theoretical framework for learning created by Benjamin Bloom that distinguishes three learning domains: Cognitive domain skills center on knowledge, comprehension, and critical thinking on a particular subject. Bloom recognized three components of educational activities: cognitive knowledge (or mental abilities), affective attitude (or emotions), and psychomotor skills (or physical skills), all of which can be used to assess K-12 students’ academic achievement. According to Jung (2001), “Transactional distance theory provides a significant conceptual framework for defining and comprehending distance education in general and a source of research hypotheses in particular,” shown in Figure 1 [ 12 ].

Theoretical Research Framework.
1.2. Hypothesis Developments and Literature Review
This section will discuss the study hypothesis and relate each hypothesis to its related studies from the literature.
There is a significant relationship between students’ background and students’ behavior .
The teacher’s guidance is essential for students’ preparedness and readiness to adapt to a new educational environment. Most students opt for the Department of Education’s “modular” distance learning options [ 3 ]. Analyzing students’ study time is critical for behavioral engagement because it establishes if academic performance is the product of student choice or historical factors [ 13 ].
There is a significant relationship between students’ background and students’ experience .
Modules provide goals, experiences, and educational activities that assist students in gaining self-sufficiency at their speed. It also boosts brain activity, encourages motivation, consolidates self-satisfaction, and enables students to remember what they have learned [ 14 ]. Despite its success, many families face difficulties due to their parents’ lack of skills and time [ 15 ].
There is a significant relationship between students’ behavior and students’ instructor interaction .
Students’ capacity to answer problems reflects their overall information awareness [ 5 ]. Learning outcomes can either cause or result in students and instructors behavior. Students’ reading issues are due to the success of online courses [ 16 ].
There is a significant relationship between students’ experience and students’ instructor interaction .
The words “student experience” relate to classroom participation. They establish a connection between students and their school, teachers, classmates, curriculum, and teaching methods [ 17 ]. The three types of student engagement are behavioral, emotional, and cognitive. Behavioral engagement refers to a student’s enthusiasm for academic and extracurricular activities. On the other hand, emotional participation is linked to how children react to their peers, teachers, and school. Motivational engagement refers to a learner’s desire to learn new abilities [ 18 ].
There is a significant relationship between students’ behavior and students’ understanding .
Individualized learning connections, outstanding training, and learning culture are all priorities at the Institute [ 19 , 20 ]. The modular technique of online learning offers additional flexibility. The use of modules allows students to investigate alternatives to the professor’s session [ 21 ].
There is a significant relationship between students’ experience and students’ performance .
Student conduct is also vital in academic accomplishment since it may affect a student’s capacity to study as well as the learning environment for other students. Students are self-assured because they understand what is expected [ 22 ]. They are more aware of their actions and take greater responsibility for their learning.
There is a significant relationship between students’ instructor interaction and students’ understanding .
Modular learning benefits students by enabling them to absorb and study material independently and on different courses. Students are more likely to give favorable reviews to courses and instructors if they believe their professors communicated effectively and facilitated or supported their learning [ 23 ].
There is a significant relationship between students’ instructor interaction and students’ performance.
Students are more engaged and active in their studies when they feel in command and protected in the classroom. Teachers play an essential role in influencing student academic motivation, school commitment, and disengagement. In studies on K-12 education, teacher-student relationships have been identified [ 24 ]. Positive teacher-student connections improve both teacher attitudes and academic performance.
There is a significant relationship between students’ understanding and students’ satisfaction .
Instructors must create well-structured courses, regularly present in their classes, and encourage student participation. When learning objectives are completed, students better understand the course’s success and learning expectations. “Constructing meaning from verbal, written, and graphic signals by interpreting, exemplifying, classifying, summarizing, inferring, comparing, and explaining” is how understanding is characterized [ 25 ].
There is a significant relationship between students’ performance and student’s academic achievement .
Academic emotions are linked to students’ performance, academic success, personality, and classroom background [ 26 ]. Understanding the elements that may influence student performance has long been a goal for educational institutions, students, and teachers.
There is a significant relationship between students’ understanding and students’ academic achievement .
Modular education views each student as an individual with distinct abilities and interests. To provide an excellent education, a teacher must adapt and individualize the educational curriculum for each student. Individual learning may aid in developing a variety of exceptional and self-reliant attributes [ 27 ]. Academic achievement is the current level of learning in the Philippines [ 28 ].
There is a significant relationship between students’ performance and students’ satisfaction .
Academic success is defined as a student’s intellectual development, including formative and summative assessment data, coursework, teacher observations, student interaction, and time on a task [ 29 ]. Students were happier with course technology, the promptness with which content was shared with the teacher, and their overall wellbeing [ 30 ].
There is a significant relationship between students’ academic achievement and students’ perceived effectiveness .
Student satisfaction is a short-term mindset based on assessing students’ educational experiences [ 29 ]. The link between student satisfaction and academic achievement is crucial in today’s higher education: we discovered that student satisfaction with course technical components was linked to a higher relative performance level [ 31 ].
There is a significant relationship between students’ satisfaction and students’ perceived effectiveness.
There is a strong link between student satisfaction and their overall perception of learning. A satisfied student is a direct effect of a positive learning experience. Perceived learning results had a favorable impact on student satisfaction in the classroom [ 32 ].
2. Materials and Methods
2.1. participants.
The principal area under study was San Jose, Occidental Mindoro, although other locations were also accepted. The survey took place between February and March 2022, with the target population of K-12 students in Junior and Senior High Schools from grades 7 to 12, aged 12 to 20, who are now implementing the Modular Approach in their studies during the COVID-19 pandemic. A 45-item questionnaire was created and circulated online to collect the information. A total of 300 online surveys was sent out and 252 online forms were received, a total of 84% response rate [ 33 ]. According to several experts, the sample size for Structural Equation Modeling (SEM) should be between 200 and 500 [ 34 ].
2.2. Questionnaire
The theoretical framework developed a self-administered test. The researcher created the questionnaire to examine and discover the probable cognitive capacity influencing K-12 students’ academic achievement in different parts of Occidental Mindoro during this pandemic as well as their satisfaction with modular distance learning. The questionnaire was designed through Google drive as people’s interactions are limited due to the effect of the COVID-19 pandemic. The questionnaire’s link was sent via email, Facebook, and other popular social media platforms.
The respondents had to complete two sections of the questionnaire. The first is their demographic information, including their age, gender, and grade level. The second is about their perceptions of modular learning. The questionnaire is divided into 12 variables: (1) Student’s Background, (2) Student’s Experience, (3) Student’s Behavior, (4) Student’s Instructor Interaction, (5) Student’s Performance, (6) Student’s Understanding, (7) Student’s Satisfaction, (8) Student’s Academic Achievement, and (9) Student’s Perceived Effectiveness. A 5-point Likert scale was used to assess all latent components contained in the SEM shown in Table 1 .
The construct and measurement items.
2.3. Structural Equation Modeling (SEM)
All the variables have been adapted from a variety of research in the literature. The observable factors were scored on a Likert scale of 1–5, with one indicating “strongly disagree” and five indicating “strongly agree”, and the data were analyzed using AMOS software. Theoretical model data were confirmed by Structural Equation Modeling (SEM). SEM is more suitable for testing the hypothesis than other methods [ 53 ]. There are many fit indices in the literature, of which the most commonly used are: CMIN/DF, Comparative Fit Index (CFI), AGFI, GFI, and Root Mean Square Error (RMSEA). Table 2 demonstrates the Good Fit Values and Acceptable Fit Values of the fit indices, respectively. AGFI and GFI are based on residuals; when sample size increases, the value of the AGFI also increase. It takes a value between 0 and 1. The fit is good if the value is more significant than 0.80. GFI is a model index that spans from 0 to 1, with values above 0.80 deemed acceptable. An RMSEA of 0.08 or less suggests a good fit [ 54 ], and a value of 0.05 to 0.08 indicates an adequate fit [ 55 ].
Acceptable Fit Values.
3. Results and Discussion
Figure 2 demonstrates the initial SEM for the cognitive factors of Modular Distance learning towards academic achievements and satisfaction of K-12 students during the COVID-19 pandemic. According to the figure below, three hypotheses were not significant: Students’ Behavior to Students’ Instructor Interaction (Hypothesis 3), Students’ Understanding of Students’ Academic Achievement (Hypothesis 11), and Students’ Performance to Students’ Satisfaction (Hypothesis 12). Therefore, a revised SEM was derived by removing this hypothesis in Figure 3 . We modified some indices to enhance the model fit based on previous studies using the SEM approach [ 47 ]. Figure 3 demonstrates the final SEM for evaluating cognitive factors affecting academic achievements and satisfaction and the perceived effectiveness of K-12 students’ response to Modular Learning during COVID-19, shown in Table 3 . Moreover, Table 4 demonstrates the descriptive statistical results of each indicator.

Initial SEM with indicators for evaluating the cognitive factors of modular distance learning towards academic achievements and satisfaction of K-12 students during COVID-19 pandemic.

Revised SEM with indicators for evaluating the cognitive factors of modular distance learning towards academic achievements and satisfaction of K-12 students during the COVID-19 pandemic.
Summary of the Results.
Descriptive statistic results.
The current study was improved by Moore’s transactional distance theory (TDT) and Bloom’s taxonomy theory (BTT) to evaluate cognitive factors affecting academic achievements and satisfaction and the perceived effectiveness of K-12 students’ response toward modular learning during COVID-19. SEM was utilized to analyze the correlation between Student Background (SB), Student Experience (SE), Student Behavior (SBE), Student Instructor Interaction (SI), Student Performance (SP), Student Understanding (SAU), Student Satisfaction (SS), Student’s Academic achievement (SAA), and Student’s Perceived effectiveness (SPE). A total of 252 data samples were acquired through an online questionnaire.
According to the findings of the SEM, the students’ background in modular learning had a favorable and significant direct effect on SE (β: 0.848, p = 0.009). K-12 students should have a background and knowledge in modular systems to better experience this new education platform. Putting the students through such an experience would support them in overcoming all difficulties that arise due to the limitations of the modular platforms. Furthermore, SEM revealed that SE had a significant adverse impact on SI (β: 0.843, p = 0.009). The study shows that students who had previous experience with modular education had more positive perceptions of modular platforms. Additionally, students’ experience with modular distance learning offers various benefits to them and their instructors to enhance students’ learning experiences, particularly for isolated learners.
Regarding the Students’ Interaction—Instructor, it positively impacts SAU (β: 0.873, p = 0.007). Communication helps students experience positive emotions such as comfort, satisfaction, and excitement, which aim to enhance their understanding and help them attain their educational goals [ 62 ]. The results revealed that SP substantially impacted SI (β: 0.765; p = 0.005). A student becomes more academically motivated and engaged by creating and maintaining strong teacher-student connections, which leads to successful academic performance.
Regarding the Students’ Understanding Response, the results revealed that SAA (β: 0.307; p = 0.052) and SS (β: 0.699; p = 0.008) had a substantial impact on SAU. Modular teaching is concerned with each student as an individual and with their specific capability and interest to assist each K-12 student in learning and provide quality education by allowing individuality to each learner. According to the Department of Education, academic achievement is the new level for student learning [ 63 ]. Meanwhile, SAA was significantly affected by the Students’ Performance Response (β: 0.754; p = 0.014). It implies that a positive performance can give positive results in student’s academic achievement, and that a negative performance can also give negative results [ 64 ]. Pekrun et al. (2010) discovered that students’ academic emotions are linked to their performance, academic achievement, personality, and classroom circumstances [ 26 ].
Results showed that students’ academic achievement significantly positively affects SPE (β: 0.237; p = 0.024). Prior knowledge has had an indirect effect on academic accomplishment. It influences the amount and type of current learning system where students must obtain a high degree of mastery [ 65 ]. According to the student’s opinion, modular distance learning is an alternative solution for providing adequate education for all learners and at all levels in the current scenario under the new education policy [ 66 ]. However, the SEM revealed that SS significantly affected SPE (β: 0.868; p = 0.009). Students’ perceptions of learning and satisfaction, when combined, can provide a better knowledge of learning achievement [ 44 ]. Students’ perceptions of learning outcomes are an excellent predictor of student satisfaction.
Since p -values and the indicators in Students’ Behavior are below 0.5, therefore two paths connecting SBE to students’ interaction—instructor (0.155) and students’ understanding (0.212) are not significant; thus, the latent variable Students’ Behavior has no effect on the latent variable Students’ Satisfaction and academic achievement as well as perceived effectiveness on modular distance learning of K12 students. This result is supported by Samsen-Bronsveld et al. (2022), who revealed that the environment has no direct influence on the student’s satisfaction, behavior engagement, and motivation to study [ 67 ]. On the other hand, the results also showed no significant relationship between Students’ Performance and Students’ Satisfaction (0.602) because the correlation p -values are greater than 0.5. Interestingly, this result opposed the other related studies. According to Bossman & Agyei (2022), satisfaction significantly affects performance or learning outcomes [ 68 ]. In addition, it was discovered that the main drivers of the students’ performance are the students’ satisfaction [ 64 , 69 ].
The result of the study implies that the students’ satisfaction serves as the mediator between the students’ performance and the student-instructor interaction in modular distance learning for K-12 students [ 70 ].
Table 5 The reliabilities of the scales used, i.e., Cronbach’s alphas, ranged from 0.568 to 0.745, which were in line with those found in other studies [ 71 ]. As presented in Table 6 , the IFI, TLI, and CFI values were greater than the suggested cutoff of 0.80, indicating that the specified model’s hypothesized construct accurately represented the observed data. In addition, the GFI and AGFI values were 0.828 and 0.801, respectively, indicating that the model was also good. The RMSEA value was 0.074, lower than the recommended value. Finally, the direct, indirect, and total effects are presented in Table 7 .
Construct Validity Model.
Direct effect, indirect effect, and total effect.
Table 6 shows that the five parameters, namely the Incremental Fit Index, Tucker Lewis Index, the Comparative Fit Index, Goodness of Fit Index, and Adjusted Goodness Fit Index, are all acceptable with parameter estimates greater than 0.8, whereas mean square error is excellent with parameter estimates less than 0.08.
4. Conclusions
The education system has been affected by the 2019 coronavirus disease; face-to-face classes are suspended to control and reduce the spread of the virus and infections [ 2 ]. The suspension of face-to-face classes results in the application of modular distance learning for K-12 students according to continuity of learning during the COVID-19 pandemic. With the outbreak of COVID-19, some issues concerning students’ academic Performance and factors associated with students’ psychological status are starting to emerge, which impacted the students’ ability to learn. This study aimed to perceive the impact of Modular Distance learning on the K-12 students amid the COVID-19 pandemic and assess cognitive factors affecting students’ academic achievement and satisfaction.
This study applied Transactional Distance Theory (TDT) and Bloom Taxonomy Theory (BTT) to evaluate cognitive factors affecting students’ academic achievements and satisfaction and evaluate the perceived effectiveness of K-12 students in response to modular learning. This study applied Structural Equation Modeling (SEM) to test hypotheses. The application of SEM analyzed the correlation among students’ background, experience, behavior, instructor interaction, performance, understanding, satisfaction, academic achievement, and student perceived effectiveness.
A total of 252 data samples were gathered through an online questionnaire. Based on findings, this study concludes that students’ background in modular distance learning affects their behavior and experience. Students’ experiences had significant effects on the performance and understanding of students in modular distance learning. Student instructor interaction had a substantial impact on performance and learning; it explains how vital interaction with the instructor is. The student interacting with the instructor shows that the student may receive feedback and guidance from the instructor. Understanding has a significant influence on students’ satisfaction and academic achievement. Student performance has a substantial impact on students’ academic achievement and satisfaction. Perceived effectiveness was significantly influenced by students’ academic achievement and student satisfaction. However, students’ behavior had no considerable effect on students’ instructor interaction, and students’ understanding while student performance equally had no significant impact on student satisfaction. From this study, students are likely to manifest good performance, behavior, and cognition when they have prior knowledge with regard to modular distance learning. This study will help the government, teachers, and students take the necessary steps to improve and enhance modular distance learning that will benefit students for effective learning.
The modular learning system has been in place since its inception. One of its founding metaphoric pillars is student satisfaction with modular learning. The organization demonstrated its dedication to the student’s voice as a component of understanding effective teaching and learning. Student satisfaction research has been transformed by modular learning. It has caused the education research community to rethink long-held assumptions that learning occurs primarily within a metaphorical container known as a “course.” When reviewing studies on student satisfaction from a factor analytic perspective, one thing becomes clear: this is a complex system with little consensus. Even the most recent factor analytical studies have done little to address the lack of understanding of the dimensions underlying satisfaction with modular learning. Items about student satisfaction with modular distance learning correspond to forming a psychological contract in factor analytic studies. The survey responses are reconfigured into a smaller number of latent (non-observable) dimensions that the students never really articulate but are fully expected to satisfy. Of course, instructors have contracts with their students. Studies such as this one identify the student’s psychological contact after the fact, rather than before the class. The most important aspect is the rapid adoption of this teaching and learning mode in Senior High School. Another balancing factor is the growing sense of student agency in the educational process. Students can express their opinions about their educational experiences in formats ranging from end-of-course evaluation protocols to various social networks, making their voices more critical.
Furthermore, they all agreed with latent trait theory, which holds that the critical dimensions that students differentiate when expressing their opinions about modular learning are formed by the combination of the original items that cannot be directly observed—which underpins student satisfaction. As stated in the literature, the relationship between student satisfaction and the characteristic of a psychological contract is illustrated. Each element is translated into how it might be expressed in the student’s voice, and then a contract feature and an assessment strategy are added. The most significant contributor to the factor pattern, engaged learning, indicates that students expect instructors to play a facilitative role in their teaching. This dimension corresponds to the relational contract, in which the learning environment is stable and well organized, with a clear path to success.
5. Limitations and Future Work
This study was focused on the cognitive capacity of modular distance learning towards academic achievements and satisfaction of K-12 students during the COVID-19 pandemic. The sample size in this study was small, at only 252. If this study is repeated with a larger sample size, it will improve the results. The study’s restriction was to the province of Occidental Mindoro; Structural Equation Modeling (SEM) was used to measure all the variables. Thus, this will give an adequate solution to the problem in the study.
The current study underlines that combining TDT and BTT can positively impact the research outcome. The contribution the current study might make to the field of modular distance learning has been discussed and explained. Based on this research model, the nine (9) factors could broadly clarify the students’ adoption of new learning environment platform features. Thus, the current research suggests that more investigation be carried out to examine relationships among the complexity of modular distance learning.
Funding Statement
This research received no external funding.
Author Contributions
Data collection, methodology, writing and editing, K.A.M.; data collection, writing—review and editing, Y.-T.J. and C.S.S. All authors have read and agreed to the published version of the manuscript.
Institutional Review Board Statement
Informed consent statement.
Informed consent was obtained from all subjects involved in the study.
Data Availability Statement
Conflicts of interest.
The authors declare no conflict of interest.
Publisher’s Note: MDPI stays neutral with regard to jurisdictional claims in published maps and institutional affiliations.
International Journal of Learning, Teaching and Educational Research
- Announcements
- Editorial Board
- Submit a Paper
- Publication Ethics
- ##PAPER TEMPLATE##
- ##Retraction Policy##
Students’ Time Management, Academic Procrastination, and Performance during Online Science and Mathematics Classes
COVID-19 affected all sectors, including academia, which resulted in an increase in online learning. While education continued through online platforms, various students-related problems arose, including improper time management, procrastination, and fluctuating academic performance. It is in this context that this quantitative study was carried out to determine how time management and procrastination affected students’ performance in science and mathematics during the pandemic. We surveyed 650 Filipino high school students using the Procrastination Assessment Scale-Students and Wayne State University’s Time Management questionnaire with a 0.93 reliability coefficient. The findings revealed that in science and mathematics, female students outperformed males. Eleven 12-year-olds had the highest mean grades in science and mathematics, while 15 16-year-olds had the lowest. Younger respondents (11-14) were more likely to have better time management in than older ones. Further, older respondents (15-18) procrastinate more than younger ones. Time management correlates positively with success in science and mathematics. Achievement in science and mathematics is the highest among students with good time management. Procrastination negatively affects achievement. High school students who procrastinated less fare better in mathematics. With this, the study opens possibilities for teaching older learners in time management to boost their performance. Students across ages should be urged to avoid procrastinating as it negatively affects academic performance. As reinforcement, schools may educate learners on time management and procrastination avoidance through orientations and other platforms.
https://doi.org/10.26803/ijlter.21.12.8
Ahmad, S., Batool, A., & Ch, A. H. (2020). Path relationship of time management and academic achievement of students in distance learning institutions. Pakistan Journal of Distance and Online Learning, 5(2). http://journal.aiou.edu.pk/journal1/index.php/PJDOL/article/view/441/89
Ahmad, S., Sultana, N., & Jamil, S. (2022). Students’ Attitude towards Biology and Academic Achievement in Biology at Secondary Level, in Islamabad, Pakistan. American Journal of Educational Research, 10(5), 268-275. https://doi.org/10.12691/education-10-5-1
Alay, S., & Kocak, S. (2003). Relationship between time management and academic achievement of university students. Journal of Educational Management in Theory and Practice, 35, 326-335. https://doi.org/10.1177/014572179902500411
Al-Balushi, S. M., Al-Harthy, I. S., & Almehrizi, R. S. (2022). Attention Drifting Away While Test-Taking: Mind-Wandering in Students with Low-and High-Performance Levels in TIMSS-Like Science Tests. International Journal of Science and Mathematics Education, 1-22. https://doi.org/10.1007/s10763-022-10258-6
Al-Bahrani, A., Apostolova-Mihaylova, M., & Marshall, E. C. (2022). Helping some and harming others: Homework frequency and tradeoffs in student performance. The Journal of Economic Education, 53(3), 197-209. https://doi.org/10.1080/00220485.2022.2075506
Batbaatar, N., & Amin, G. (2021). Students’ time management during online CLASS. In International Conference Universitas Pekalongan 2021 (Vol. 1, No. 1, pp. 189-194). https://proceeding.unikal.ac.id/index.php/icunikal2021/article/view/627
Bernard, R. M., Abrami, P. C., Borokhovski, E., Wade, C. A., Tamim, R. M., Surkes, M. A., & Bethel, E. C. (2009). A meta-analysis of three types of interaction treatments in distance education. Review of Educational research, 79(3), 1243-1289. https://doi.org/10.3102/0034654309333844
bin Mohammed Al-Subaie, A. (2022). Academic Procrastination and Metacognitive Strategies Among Prince Sattam Bin Abdulaziz University Students. Journal of Positive School Psychology, 6(8), 7601-7610. https://journalppw.com/index.php/jpsp/article/view/11109
Bocar, A., & Daga-ang, MG (2013). Time management of work scholars. https://www.researchgate.net/publication/270566011
Capinding, A. T. (2022). Impact of Modular Distance Learning on High School Students Mathematics Motivation, Interest/Attitude, Anxiety and Achievement during the COVID-19 Pandemic. Impact of Modular Distance Learning on High School Students Mathematics Motivation, Interest/Attitude, Anxiety and Achievement during the COVID-19 Pandemic, 11(2), 917-934. https://doi.org/10.12973/eu-jer.11.2.917
DeBeliso, M., Gauthier, H., Sevene, T., Adams, K. J., Lawrence, M. M., Climstein, M., ... & Navalta, J. W. (2022). The time management matrix re-tooled: an instrument for academics navigating the tenure process. International Journal of Multidisciplinary Perspectives in Higher Education, 7(1), 31-51. https://researchportal.scu.edu.au/discovery/delivery/61SCU_INST:ResearchRepository/991012998198802368#1395510230002368
Demuyakor, J. (2020). Analysis of social media utilization by students in higher education: A critical literature review of Ghana. Journal of New Media and Mass Communication, 6(1), 1–7. https://doi.org/10.18488/journal.91.2020.61.1.7
Desmond, A., & Amadi, K. (2022). Influence of Time Management on academic performance of Business Education Students in Universities in Rivers State. Journal homepage: www. ijrpr. com ISSN, 2582, 7421. https://ijrpr.com/uploads/V3ISSUE6/IJRPR5096.pdf
de Vera, J. V., Peteros, E. D., Peconcillo Jr, L. B., Alcantara, G. A., Villarin, E. R., & Fulgencio, M. D. (2022). Assessing differences on students’ attributes in mathematics based on their learning sessions. Open Journal of Social Sciences, 10(3), 170-185. https://doi.org/10.4236/jss.2022.103012
De Paola, M., Gioia, F., & Scoppa, V. (2022). Online teaching, procrastination and students’ achievement: evidence from COVID-19 induced remote learning. http://dx.doi.org/10.2139/ssrn.4114561
Fentaw, Y., Moges, B. T., & Ismail, S. M. (2022). Academic Procrastination Behavior among Public University Students. Education Research International, 2022. https://doi.org/10.1155/2022/1277866
Gagnier, K. M., Holochwost, S. J., & Fisher, K. R. (2022). Spatial thinking in science, technology, engineering, and mathematics: Elementary teachers' beliefs, perceptions, and self?efficacy. Journal of Research in Science Teaching, 59(1), 95-126. https://doi.org/10.1002/tea.21722
Garcia, M. I., Caraig, D. J., Carator, K., Oyco, M. T., Tababa, G. A., Linaugo, J., & De Oca, P. R. (2022). The Influence of Gadget Dependency on the Academic Procrastination Levels of Grade 12 STEM Students. International Journal of Multidisciplinary: Applied Business and Education Research, 3(6), 1197-1210. https://doi.org/10.11594//ijmaber.03.06.22
Gargari, R. B., Sabouri, H., & Norzad, F. (2011) Academic procrastination: The relationship between causal attribution styles and behavioral postponement. Iranian Journal of Psychiatry and Behavioral Sciences, 5(2), 76-82. https://www.ncbi.nlm.nih.gov/pmc/articles/PMC3939975/
Geverola, I. J. R., Mutya, R. C., Siason, L. M. B., & Bonotan, A. (2022). Challenges and struggles of public senior high school science teachers during the new normal. Journal of Research, Policy & Practice of Teachers and Teacher Education, 12(1), 49-68. https://doi.org/10.37134/jrpptte.vol12.1.4.2022
Harefa, S., & Sihombing, G. L. A. (2021). Students’ perception of online learning amidst the Covid-19 pandemic: A study of junior, senior high school and college students in a remote area. F1000Research, 10. https://doi.org/10.12688/f1000research.52152.2
Hinderliter, H., Xie, Y., Ladendorf, K., & Muehsler, H. (2022). Path Analysis of Internal and External Factors Associated with Parental Satisfaction over K-12 Online Learning. Computers in the Schools, 38(4), 354-383. https://doi.org/10.1080/07380569.2021.1988319
Karagiannopoulou, E., Milienos, F. S., & Rentzios, C. (2022). Grouping learning approaches and emotional factors to predict students’ academic progress. International Journal of School & Educational Psychology, 10(2), 258-275. https://doi.org/10.1080/21683603.2020.1832941
Kulal, A., & Nayak, A. (2020). A study on perception of teachers and students toward online classes in Dakshina Kannada and Udupi District. Asian Association of Open Universities Journal. https://doi.org/10.1108/AAOUJ-07-2020-0047
Laurie, A., & Hellsten, M. (2002). What Do We Know About Time Management? A Review of the Literature and a Psychometric Critique of Instruments Assessing Time Management University of Saskatchewan, Canada. https://doi.org/10.5772/37248
Lopez-Agudo, L. A., & Marcenaro-Gutierrez, O. D. (2022). Instruction time and students’ academic achievement: a cross-country comparison. Compare: A Journal of Comparative and International Education, 52(1), 75-91. https://doi.org/10.1080/03057925.2020.1737919
Miertschin, S. L., Goodson, C. E., & Stewart, B. L. (2015). Time management skills and student performance in online courses. In 2015 ASEE Annual Conference & Exposition (pp. 26-1585). https://doi.org/10.18260/p.24921
Migliozzi, C. L. (2022). Engaging the Adult College Student: A Case Study on Improving Nontraditional Students’ Persistence. https://scholarworks.umb.edu/instruction_capstone/82
Narci, M. (2022). The Relationship Between Problematic Internet Use and Academic Procrastination and Life Satisfaction of University Students (Doctoral dissertation, University of Alabama Libraries). https://www.proquest.com/openview/59be06329f73db36012c76a7fe6f6745/1.pdf?pq-origsite=gscholar&cbl=18750&diss=y
Naturil-Alfonso, C., Peñaranda, D., Vicente, J., & Marco-Jiménez, F. (2018, October). Procrastination: the poor time management among university students. In 4th International Conference on Higher Education Advances (HEAD'18) (pp. 1-8). Editorial Universitat Politècnica de València. https://doi.org/10.4995/HEAD18.2018.8167
Njuguna, M. N. (2022). Antecedents of Academic Procrastination and its Relationship to Academic Achievement among form Three Students in Kiambu County, Kenya. https://ir-library.ku.ac.ke/bitstream/handle/123456789/23953/Antecedents%20of%20Academic%20Procrastination…..pdf?sequence=1
Noran, F. Y. (2000). Procrastination among students in institutes of higher learning: Challenges for K-Economy. Retrieved December, 30, 2008. https://files.eric.ed.gov/fulltext/EJ1066019.pdf
Ocak, G., & Boyraz, S. (2016). Examination of the Relation between Academic Procrastination and Time Management Skills of Undergraduate Students in Terms of Some Variables. Journal of education and training studies, 4(5), 76-84. https://eric.ed.gov/?id=EJ1092698
Pagaran, G. M., Loremas, M. L., Gultiano, J. D., & Etcuban, J. O. (2022). Mathematics Performance of Senior High School Students in Blended Learning Amidst the Covid-19 Pandemic. Journal of Positive School Psychology, 10593-10613. https://journalppw.com/index.php/jpsp/article/view/9686/6321
Parantika, I. W. A., Suniasih, N. W., & Kristiantari, M. R. (2020). Differences in academic procrastination attitude between fifth grade male and female students. Journal of Psychology and Instruction, 4(1), 10-15. https://doi.org/10.23887/jpai.v4i1.24451
Pilotti, M. A., El-Moussa, O. J., & Abdelsalam, H. M. (2022). Measuring the impact of the pandemic on female and male students’ learning in a society in transition: A must for sustainable education. Sustainability, 14(6), 3148. https://doi.org/10.3390/su14063148
Rabin, A. L., Fogel, J., & Upham-Nutter, E. K. (2011). Academic procrastination in college students: The role of self-reported executive function. Journal of Clinical and Experimental Neuropsychology, 3(3), 344-357. https://doi.org/10.1080/13803395.2010.518597
Shaked, L., & Altarac, H. (2022). The possible contribution of procrastination and perception of self-efficacy to academic achievement. Journal of Further and Higher Education, 1-17. https://doi.org/10.1080/0309877X.2022.2102414
Shepperd, R. S. (2002). Predictors of student success in distance education courses. West Virginia University. https://www.proquest.com/openview/d4c1195ea0c6ffe90fef5b104ea60872/1?pq-origsite=gscholar&cbl=18750&diss=y
Solomon, L. J., & Rothblum, E. (1988). Procrastination assessment scale-students. Dictionary of behavioral assessment techniques, 358-360. https://web.archive.org/web/20091222073641id_/http://www-rohan.sdsu.edu:80/~rothblum/doc_pdf/procrastination/ProcrastinationAssessmentScaleStudents.pdf
Soysal, D., Bani-Yaghoub, M., & Riggers-Piehl, T. A. (2022). Analysis of anxiety, motivation, and confidence of STEM students during the COVID-19 pandemic. International Electronic Journal of Mathematics Education, 17(2), em0684. https://doi.org/10.29333/iejme/11836
Steel, P. (2007). The nature of procrastination: A meta-analytic and theoretical review of quintessential self-regulatory failure, Psychological Bulletin, 133, 65–94. https://doi.org/10.1037/0033-2909.133.1.65
Tezer, M., Çavu?, S., Orkun, M. A., Osum, A., & Ture, A. (2021). Examination of opinions of elementary school students on Mathematics course in the COVID-19 pandemic process. International Journal of Learning and Teaching, 13(1), 42-53. https://doi.org/10.18844/ijlt.v13i1.5279
Time Management Questionnaire. (2014). Wayne State University. Retrieved July 05, 2022 from http://advising.wayne.edu/hndbk/time.php
Treceñe, J. K. D. (2022). COVID-19 and Remote Learning in the Philippine Basic Education System: Experiences of Teachers, Parents, and Students. In Socioeconomic Inclusion During an Era of Online Education (pp. 92-110). IGI Global. https://www.igi-global.com/chapter/covid-19-and-remote-learning-in-the-philippine-basic-education-system/307359
Vooren, M., Haelermans, C., Groot, W., & van den Brink, H. M. (2022). Comparing success of female students to their male counterparts in the STEM fields: an empirical analysis from enrollment until graduation using longitudinal register data. International Journal of STEM Education, 9(1), 1-17. https://doi.org/10.1186/s40594-021-00318-8
Winanda, R. S., Mikail, A., Ahmad, D., Agustina, D., & Rahmawati, R. (2022). University Students' Procrastination: A Mathematical Model (Case Studies: Student in Mathematics Department Universitas Negeri Padang). Eksakta: Berkala Ilmiah Bidang MIPA (E-ISSN: 2549-7464), 23(02), 98-105. https://doi.org/10.24036/eksakta/vol23-iss02/315
Xu, J. (2022). More than minutes: A person-centered approach to homework time, homework time management, and homework procrastination. Contemporary Educational Psychology, 70, 102087. https://doi.org/10.1016/j.cedpsych.2022.102087
Žnidarši?, A., Brezavš?ek, A., Rus, G., & Jerebic, J. (2022). Has the COVID-19 Pandemic Affected Mathematics Achievement? A Case Study of University Students in Social Sciences. Mathematics, 10(13),
https://doi.org/10.3390/math10132314
- There are currently no refbacks.
e-ISSN: 1694-2116
p-ISSN: 1694-2493
- Research article
- Open access
- Published: 06 February 2017
Blended learning effectiveness: the relationship between student characteristics, design features and outcomes
- Mugenyi Justice Kintu ORCID: orcid.org/0000-0002-4500-1168 1 , 2 ,
- Chang Zhu 2 &
- Edmond Kagambe 1
International Journal of Educational Technology in Higher Education volume 14 , Article number: 7 ( 2017 ) Cite this article
759k Accesses
223 Citations
37 Altmetric
Metrics details
This paper investigates the effectiveness of a blended learning environment through analyzing the relationship between student characteristics/background, design features and learning outcomes. It is aimed at determining the significant predictors of blended learning effectiveness taking student characteristics/background and design features as independent variables and learning outcomes as dependent variables. A survey was administered to 238 respondents to gather data on student characteristics/background, design features and learning outcomes. The final semester evaluation results were used as a measure for performance as an outcome. We applied the online self regulatory learning questionnaire for data on learner self regulation, the intrinsic motivation inventory for data on intrinsic motivation and other self-developed instruments for measuring the other constructs. Multiple regression analysis results showed that blended learning design features (technology quality, online tools and face-to-face support) and student characteristics (attitudes and self-regulation) predicted student satisfaction as an outcome. The results indicate that some of the student characteristics/backgrounds and design features are significant predictors for student learning outcomes in blended learning.
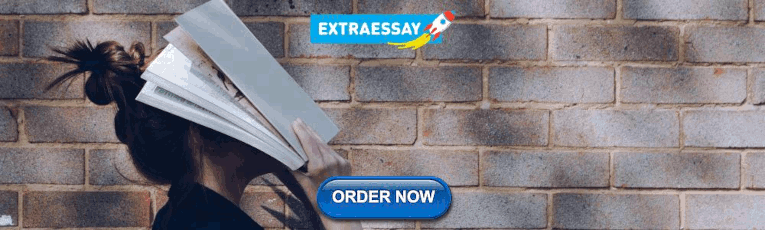
Introduction
The teaching and learning environment is embracing a number of innovations and some of these involve the use of technology through blended learning. This innovative pedagogical approach has been embraced rapidly though it goes through a process. The introduction of blended learning (combination of face-to-face and online teaching and learning) initiatives is part of these innovations but its uptake, especially in the developing world faces challenges for it to be an effective innovation in teaching and learning. Blended learning effectiveness has quite a number of underlying factors that pose challenges. One big challenge is about how users can successfully use the technology and ensuring participants’ commitment given the individual learner characteristics and encounters with technology (Hofmann, 2014 ). Hofmann adds that users getting into difficulties with technology may result into abandoning the learning and eventual failure of technological applications. In a report by Oxford Group ( 2013 ), some learners (16%) had negative attitudes to blended learning while 26% were concerned that learners would not complete study in blended learning. Learners are important partners in any learning process and therefore, their backgrounds and characteristics affect their ability to effectively carry on with learning and being in blended learning, the design tools to be used may impinge on the effectiveness in their learning.
This study tackles blended learning effectiveness which has been investigated in previous studies considering grades, course completion, retention and graduation rates but no studies regarding effectiveness in view of learner characteristics/background, design features and outcomes have been done in the Ugandan university context. No studies have also been done on how the characteristics of learners and design features are predictors of outcomes in the context of a planning evaluation research (Guskey, 2000 ) to establish the effectiveness of blended learning. Guskey ( 2000 ) noted that planning evaluation fits in well since it occurs before the implementation of any innovation as well as allowing planners to determine the needs, considering participant characteristics, analyzing contextual matters and gathering baseline information. This study is done in the context of a plan to undertake innovative pedagogy involving use of a learning management system (moodle) for the first time in teaching and learning in a Ugandan university. The learner characteristics/backgrounds being investigated for blended learning effectiveness include self-regulation, computer competence, workload management, social and family support, attitude to blended learning, gender and age. We investigate the blended learning design features of learner interactions, face-to-face support, learning management system tools and technology quality while the outcomes considered include satisfaction, performance, intrinsic motivation and knowledge construction. Establishing the significant predictors of outcomes in blended learning will help to inform planners of such learning environments in order to put in place necessary groundwork preparations for designing blended learning as an innovative pedagogical approach.
Kenney and Newcombe ( 2011 ) did their comparison to establish effectiveness in view of grades and found that blended learning had higher average score than the non-blended learning environment. Garrison and Kanuka ( 2004 ) examined the transformative potential of blended learning and reported an increase in course completion rates, improved retention and increased student satisfaction. Comparisons between blended learning environments have been done to establish the disparity between academic achievement, grade dispersions and gender performance differences and no significant differences were found between the groups (Demirkol & Kazu, 2014 ).
However, blended learning effectiveness may be dependent on many other factors and among them student characteristics, design features and learning outcomes. Research shows that the failure of learners to continue their online education in some cases has been due to family support or increased workload leading to learner dropout (Park & Choi, 2009 ) as well as little time for study. Additionally, it is dependent on learner interactions with instructors since failure to continue with online learning is attributed to this. In Greer, Hudson & Paugh’s study as cited in Park and Choi ( 2009 ), family and peer support for learners is important for success in online and face-to-face learning. Support is needed for learners from all areas in web-based courses and this may be from family, friends, co-workers as well as peers in class. Greer, Hudson and Paugh further noted that peer encouragement assisted new learners in computer use and applications. The authors also show that learners need time budgeting, appropriate technology tools and support from friends and family in web-based courses. Peer support is required by learners who have no or little knowledge of technology, especially computers, to help them overcome fears. Park and Choi, ( 2009 ) showed that organizational support significantly predicts learners’ stay and success in online courses because employers at times are willing to reduce learners’ workload during study as well as supervisors showing that they are interested in job-related learning for employees to advance and improve their skills.
The study by Kintu and Zhu ( 2016 ) investigated the possibility of blended learning in a Ugandan University and examined whether student characteristics (such as self-regulation, attitudes towards blended learning, computer competence) and student background (such as family support, social support and management of workload) were significant factors in learner outcomes (such as motivation, satisfaction, knowledge construction and performance). The characteristics and background factors were studied along with blended learning design features such as technology quality, learner interactions, and Moodle with its tools and resources. The findings from that study indicated that learner attitudes towards blended learning were significant factors to learner satisfaction and motivation while workload management was a significant factor to learner satisfaction and knowledge construction. Among the blended learning design features, only learner interaction was a significant factor to learner satisfaction and knowledge construction.
The focus of the present study is on examining the effectiveness of blended learning taking into consideration learner characteristics/background, blended learning design elements and learning outcomes and how the former are significant predictors of blended learning effectiveness.
Studies like that of Morris and Lim ( 2009 ) have investigated learner and instructional factors influencing learning outcomes in blended learning. They however do not deal with such variables in the contexts of blended learning design as an aspect of innovative pedagogy involving the use of technology in education. Apart from the learner variables such as gender, age, experience, study time as tackled before, this study considers social and background aspects of the learners such as family and social support, self-regulation, attitudes towards blended learning and management of workload to find out their relationship to blended learning effectiveness. Identifying the various types of learner variables with regard to their relationship to blended learning effectiveness is important in this study as we embark on innovative pedagogy with technology in teaching and learning.
Literature review
This review presents research about blended learning effectiveness from the perspective of learner characteristics/background, design features and learning outcomes. It also gives the factors that are considered to be significant for blended learning effectiveness. The selected elements are as a result of the researcher’s experiences at a Ugandan university where student learning faces challenges with regard to learner characteristics and blended learning features in adopting the use of technology in teaching and learning. We have made use of Loukis, Georgiou, and Pazalo ( 2007 ) value flow model for evaluating an e-learning and blended learning service specifically considering the effectiveness evaluation layer. This evaluates the extent of an e-learning system usage and the educational effectiveness. In addition, studies by Leidner, Jarvenpaa, Dillon and Gunawardena as cited in Selim ( 2007 ) have noted three main factors that affect e-learning and blended learning effectiveness as instructor characteristics, technology and student characteristics. Heinich, Molenda, Russell, and Smaldino ( 2001 ) showed the need for examining learner characteristics for effective instructional technology use and showed that user characteristics do impact on behavioral intention to use technology. Research has dealt with learner characteristics that contribute to learner performance outcomes. They have dealt with emotional intelligence, resilience, personality type and success in an online learning context (Berenson, Boyles, & Weaver, 2008 ). Dealing with the characteristics identified in this study will give another dimension, especially for blended learning in learning environment designs and add to specific debate on learning using technology. Lin and Vassar, ( 2009 ) indicated that learner success is dependent on ability to cope with technical difficulty as well as technical skills in computer operations and internet navigation. This justifies our approach in dealing with the design features of blended learning in this study.
Learner characteristics/background and blended learning effectiveness
Studies indicate that student characteristics such as gender play significant roles in academic achievement (Oxford Group, 2013 ), but no study examines performance of male and female as an important factor in blended learning effectiveness. It has again been noted that the success of e- and blended learning is highly dependent on experience in internet and computer applications (Picciano & Seaman, 2007 ). Rigorous discovery of such competences can finally lead to a confirmation of high possibilities of establishing blended learning. Research agrees that the success of e-learning and blended learning can largely depend on students as well as teachers gaining confidence and capability to participate in blended learning (Hadad, 2007 ). Shraim and Khlaif ( 2010 ) note in their research that 75% of students and 72% of teachers were lacking in skills to utilize ICT based learning components due to insufficient skills and experience in computer and internet applications and this may lead to failure in e-learning and blended learning. It is therefore pertinent that since the use of blended learning applies high usage of computers, computer competence is necessary (Abubakar & Adetimirin, 2015 ) to avoid failure in applying technology in education for learning effectiveness. Rovai, ( 2003 ) noted that learners’ computer literacy and time management are crucial in distance learning contexts and concluded that such factors are meaningful in online classes. This is supported by Selim ( 2007 ) that learners need to posses time management skills and computer skills necessary for effectiveness in e- learning and blended learning. Self-regulatory skills of time management lead to better performance and learners’ ability to structure the physical learning environment leads to efficiency in e-learning and blended learning environments. Learners need to seek helpful assistance from peers and teachers through chats, email and face-to-face meetings for effectiveness (Lynch & Dembo, 2004 ). Factors such as learners’ hours of employment and family responsibilities are known to impede learners’ process of learning, blended learning inclusive (Cohen, Stage, Hammack, & Marcus, 2012 ). It was also noted that a common factor in failure and learner drop-out is the time conflict which is compounded by issues of family , employment status as well as management support (Packham, Jones, Miller, & Thomas, 2004 ). A study by Thompson ( 2004 ) shows that work, family, insufficient time and study load made learners withdraw from online courses.
Learner attitudes to blended learning can result in its effectiveness and these shape behavioral intentions which usually lead to persistence in a learning environment, blended inclusive. Selim, ( 2007 ) noted that the learners’ attitude towards e-learning and blended learning are success factors for these learning environments. Learner performance by age and gender in e-learning and blended learning has been found to indicate no significant differences between male and female learners and different age groups (i.e. young, middle-aged and old above 45 years) (Coldwell, Craig, Paterson, & Mustard, 2008 ). This implies that the potential for blended learning to be effective exists and is unhampered by gender or age differences.
Blended learning design features
The design features under study here include interactions, technology with its quality, face-to-face support and learning management system tools and resources.
Research shows that absence of learner interaction causes failure and eventual drop-out in online courses (Willging & Johnson, 2009 ) and the lack of learner connectedness was noted as an internal factor leading to learner drop-out in online courses (Zielinski, 2000 ). It was also noted that learners may not continue in e- and blended learning if they are unable to make friends thereby being disconnected and developing feelings of isolation during their blended learning experiences (Willging & Johnson, 2009). Learners’ Interactions with teachers and peers can make blended learning effective as its absence makes learners withdraw (Astleitner, 2000 ). Loukis, Georgious and Pazalo (2007) noted that learners’ measuring of a system’s quality, reliability and ease of use leads to learning efficiency and can be so in blended learning. Learner success in blended learning may substantially be affected by system functionality (Pituch & Lee, 2006 ) and may lead to failure of such learning initiatives (Shrain, 2012 ). It is therefore important to examine technology quality for ensuring learning effectiveness in blended learning. Tselios, Daskalakis, and Papadopoulou ( 2011 ) investigated learner perceptions after a learning management system use and found out that the actual system use determines the usefulness among users. It is again noted that a system with poor response time cannot be taken to be useful for e-learning and blended learning especially in cases of limited bandwidth (Anderson, 2004 ). In this study, we investigate the use of Moodle and its tools as a function of potential effectiveness of blended learning.
The quality of learning management system content for learners can be a predictor of good performance in e-and blended learning environments and can lead to learner satisfaction. On the whole, poor quality technology yields no satisfaction by users and therefore the quality of technology significantly affects satisfaction (Piccoli, Ahmad, & Ives, 2001 ). Continued navigation through a learning management system increases use and is an indicator of success in blended learning (Delone & McLean, 2003 ). The efficient use of learning management system and its tools improves learning outcomes in e-learning and blended learning environments.
It is noted that learner satisfaction with a learning management system can be an antecedent factor for blended learning effectiveness. Goyal and Tambe ( 2015 ) noted that learners showed an appreciation to Moodle’s contribution in their learning. They showed positivity with it as it improved their understanding of course material (Ahmad & Al-Khanjari, 2011 ). The study by Goyal and Tambe ( 2015 ) used descriptive statistics to indicate improved learning by use of uploaded syllabus and session plans on Moodle. Improved learning is also noted through sharing study material, submitting assignments and using the calendar. Learners in the study found Moodle to be an effective educational tool.
In blended learning set ups, face-to-face experiences form part of the blend and learner positive attitudes to such sessions could mean blended learning effectiveness. A study by Marriot, Marriot, and Selwyn ( 2004 ) showed learners expressing their preference for face-to-face due to its facilitation of social interaction and communication skills acquired from classroom environment. Their preference for the online session was only in as far as it complemented the traditional face-to-face learning. Learners in a study by Osgerby ( 2013 ) had positive perceptions of blended learning but preferred face-to-face with its step-by-stem instruction. Beard, Harper and Riley ( 2004 ) shows that some learners are successful while in a personal interaction with teachers and peers thus prefer face-to-face in the blend. Beard however dealt with a comparison between online and on-campus learning while our study combines both, singling out the face-to-face part of the blend. The advantage found by Beard is all the same relevant here because learners in blended learning express attitude to both online and face-to-face for an effective blend. Researchers indicate that teacher presence in face-to-face sessions lessens psychological distance between them and the learners and leads to greater learning. This is because there are verbal aspects like giving praise, soliciting for viewpoints, humor, etc and non-verbal expressions like eye contact, facial expressions, gestures, etc which make teachers to be closer to learners psychologically (Kelley & Gorham, 2009 ).
Learner outcomes
The outcomes under scrutiny in this study include performance, motivation, satisfaction and knowledge construction. Motivation is seen here as an outcome because, much as cognitive factors such as course grades are used in measuring learning outcomes, affective factors like intrinsic motivation may also be used to indicate outcomes of learning (Kuo, Walker, Belland, & Schroder, 2013 ). Research shows that high motivation among online learners leads to persistence in their courses (Menager-Beeley, 2004 ). Sankaran and Bui ( 2001 ) indicated that less motivated learners performed poorly in knowledge tests while those with high learning motivation demonstrate high performance in academics (Green, Nelson, Martin, & Marsh, 2006 ). Lim and Kim, ( 2003 ) indicated that learner interest as a motivation factor promotes learner involvement in learning and this could lead to learning effectiveness in blended learning.
Learner satisfaction was noted as a strong factor for effectiveness of blended and online courses (Wilging & Johnson, 2009) and dissatisfaction may result from learners’ incompetence in the use of the learning management system as an effective learning tool since, as Islam ( 2014 ) puts it, users may be dissatisfied with an information system due to ease of use. A lack of prompt feedback for learners from course instructors was found to cause dissatisfaction in an online graduate course. In addition, dissatisfaction resulted from technical difficulties as well as ambiguous course instruction Hara and Kling ( 2001 ). These factors, once addressed, can lead to learner satisfaction in e-learning and blended learning and eventual effectiveness. A study by Blocker and Tucker ( 2001 ) also showed that learners had difficulties with technology and inadequate group participation by peers leading to dissatisfaction within these design features. Student-teacher interactions are known to bring satisfaction within online courses. Study results by Swan ( 2001 ) indicated that student-teacher interaction strongly related with student satisfaction and high learner-learner interaction resulted in higher levels of course satisfaction. Descriptive results by Naaj, Nachouki, and Ankit ( 2012 ) showed that learners were satisfied with technology which was a video-conferencing component of blended learning with a mean of 3.7. The same study indicated student satisfaction with instructors at a mean of 3.8. Askar and Altun, ( 2008 ) found that learners were satisfied with face-to-face sessions of the blend with t-tests and ANOVA results indicating female scores as higher than for males in the satisfaction with face-to-face environment of the blended learning.
Studies comparing blended learning with traditional face-to-face have indicated that learners perform equally well in blended learning and their performance is unaffected by the delivery method (Kwak, Menezes, & Sherwood, 2013 ). In another study, learning experience and performance are known to improve when traditional course delivery is integrated with online learning (Stacey & Gerbic, 2007 ). Such improvement as noted may be an indicator of blended learning effectiveness. Our study however, delves into improved performance but seeks to establish the potential of blended learning effectiveness by considering grades obtained in a blended learning experiment. Score 50 and above is considered a pass in this study’s setting and learners scoring this and above will be considered to have passed. This will make our conclusions about the potential of blended learning effectiveness.
Regarding knowledge construction, it has been noted that effective learning occurs where learners are actively involved (Nurmela, Palonen, Lehtinen & Hakkarainen, 2003 , cited in Zhu, 2012 ) and this may be an indicator of learning environment effectiveness. Effective blended learning would require that learners are able to initiate, discover and accomplish the processes of knowledge construction as antecedents of blended learning effectiveness. A study by Rahman, Yasin and Jusoff ( 2011 ) indicated that learners were able to use some steps to construct meaning through an online discussion process through assignments given. In the process of giving and receiving among themselves, the authors noted that learners learned by writing what they understood. From our perspective, this can be considered to be accomplishment in the knowledge construction process. Their study further shows that learners construct meaning individually from assignments and this stage is referred to as pre-construction which for our study, is an aspect of discovery in the knowledge construction process.
Predictors of blended learning effectiveness
Researchers have dealt with success factors for online learning or those for traditional face-to-face learning but little is known about factors that predict blended learning effectiveness in view of learner characteristics and blended learning design features. This part of our study seeks to establish the learner characteristics/backgrounds and design features that predict blended learning effectiveness with regard to satisfaction, outcomes, motivation and knowledge construction. Song, Singleton, Hill, and Koh ( 2004 ) examined online learning effectiveness factors and found out that time management (a self-regulatory factor) was crucial for successful online learning. Eom, Wen, and Ashill ( 2006 ) using a survey found out that interaction, among other factors, was significant for learner satisfaction. Technical problems with regard to instructional design were a challenge to online learners thus not indicating effectiveness (Song et al., 2004 ), though the authors also indicated that descriptive statistics to a tune of 75% and time management (62%) impact on success of online learning. Arbaugh ( 2000 ) and Swan ( 2001 ) indicated that high levels of learner-instructor interaction are associated with high levels of user satisfaction and learning outcomes. A study by Naaj et al. ( 2012 ) indicated that technology and learner interactions, among other factors, influenced learner satisfaction in blended learning.
Objective and research questions of the current study
The objective of the current study is to investigate the effectiveness of blended learning in view of student satisfaction, knowledge construction, performance and intrinsic motivation and how they are related to student characteristics and blended learning design features in a blended learning environment.
Research questions
What are the student characteristics and blended learning design features for an effective blended learning environment?
Which factors (among the learner characteristics and blended learning design features) predict student satisfaction, learning outcomes, intrinsic motivation and knowledge construction?
Conceptual model of the present study
The reviewed literature clearly shows learner characteristics/background and blended learning design features play a part in blended learning effectiveness and some of them are significant predictors of effectiveness. The conceptual model for our study is depicted as follows (Fig. 1 ):
Conceptual model of the current study
Research design
This research applies a quantitative design where descriptive statistics are used for the student characteristics and design features data, t-tests for the age and gender variables to determine if they are significant in blended learning effectiveness and regression for predictors of blended learning effectiveness.
This study is based on an experiment in which learners participated during their study using face-to-face sessions and an on-line session of a blended learning design. A learning management system (Moodle) was used and learner characteristics/background and blended learning design features were measured in relation to learning effectiveness. It is therefore a planning evaluation research design as noted by Guskey ( 2000 ) since the outcomes are aimed at blended learning implementation at MMU. The plan under which the various variables were tested involved face-to-face study at the beginning of a 17 week semester which was followed by online teaching and learning in the second half of the semester. The last part of the semester was for another face-to-face to review work done during the online sessions and final semester examinations. A questionnaire with items on student characteristics, design features and learning outcomes was distributed among students from three schools and one directorate of postgraduate studies.
Participants
Cluster sampling was used to select a total of 238 learners to participate in this study. Out of the whole university population of students, three schools and one directorate were used. From these, one course unit was selected from each school and all the learners following the course unit were surveyed. In the school of Education ( n = 70) and Business and Management Studies ( n = 133), sophomore students were involved due to the fact that they have been introduced to ICT basics during their first year of study. Students of the third year were used from the department of technology in the School of Applied Sciences and Technology ( n = 18) since most of the year two courses had a lot of practical aspects that could not be used for the online learning part. From the Postgraduate Directorate ( n = 17), first and second year students were selected because learners attend a face-to-face session before they are given paper modules to study away from campus.
The study population comprised of 139 male students representing 58.4% and 99 females representing 41.6% with an average age of 24 years.
Instruments
The end of semester results were used to measure learner performance. The online self-regulated learning questionnaire (Barnard, Lan, To, Paton, & Lai, 2009 ) and the intrinsic motivation inventory (Deci & Ryan, 1982 ) were applied to measure the constructs on self regulation in the student characteristics and motivation in the learning outcome constructs. Other self-developed instruments were used for the other remaining variables of attitudes, computer competence, workload management, social and family support, satisfaction, knowledge construction, technology quality, interactions, learning management system tools and resources and face-to-face support.
Instrument reliability
Cronbach’s alpha was used to test reliability and the table below gives the results. All the scales and sub-scales had acceptable internal consistency reliabilities as shown in Table 1 below:
Data analysis
First, descriptive statistics was conducted. Shapiro-Wilk test was done to test normality of the data for it to qualify for parametric tests. The test results for normality of our data before the t- test resulted into significant levels (Male = .003, female = .000) thereby violating the normality assumption. We therefore used the skewness and curtosis results which were between −1.0 and +1.0 and assumed distribution to be sufficiently normal to qualify the data for a parametric test, (Pallant, 2010 ). An independent samples t -test was done to find out the differences in male and female performance to explain the gender characteristics in blended learning effectiveness. A one-way ANOVA between subjects was conducted to establish the differences in performance between age groups. Finally, multiple regression analysis was done between student variables and design elements with learning outcomes to determine the significant predictors for blended learning effectiveness.
Student characteristics, blended learning design features and learning outcomes ( RQ1 )
A t- test was carried out to establish the performance of male and female learners in the blended learning set up. This was aimed at finding out if male and female learners do perform equally well in blended learning given their different roles and responsibilities in society. It was found that male learners performed slightly better ( M = 62.5) than their female counterparts ( M = 61.1). An independent t -test revealed that the difference between the performances was not statistically significant ( t = 1.569, df = 228, p = 0.05, one tailed). The magnitude of the differences in the means is small with effect size ( d = 0.18). A one way between subjects ANOVA was conducted on the performance of different age groups to establish the performance of learners of young and middle aged age groups (20–30, young & and 31–39, middle aged). This revealed a significant difference in performance (F(1,236 = 8.498, p < . 001).
Average percentages of the items making up the self regulated learning scale are used to report the findings about all the sub-scales in the learner characteristics/background scale. Results show that learner self-regulation was good enough at 72.3% in all the sub-scales of goal setting, environment structuring, task strategies, time management, help-seeking and self-evaluation among learners. The least in the scoring was task strategies at 67.7% and the highest was learner environment structuring at 76.3%. Learner attitude towards blended learning environment is at 76% in the sub-scales of learner autonomy, quality of instructional materials, course structure, course interface and interactions. The least scored here is attitude to course structure at 66% and their attitudes were high on learner autonomy and course interface both at 82%. Results on the learners’ computer competences are summarized in percentages in the table below (Table 2 ):
It can be seen that learners are skilled in word processing at 91%, email at 63.5%, spreadsheets at 68%, web browsers at 70.2% and html tools at 45.4%. They are therefore good enough in word processing and web browsing. Their computer confidence levels are reported at 75.3% and specifically feel very confident when it comes to working with a computer (85.7%). Levels of family and social support for learners during blended learning experiences are at 60.5 and 75% respectively. There is however a low score on learners being assisted by family members in situations of computer setbacks (33.2%) as 53.4% of the learners reported no assistance in this regard. A higher percentage (85.3%) is reported on learners getting support from family regarding provision of essentials for learning such as tuition. A big percentage of learners spend two hours on study while at home (35.3%) followed by one hour (28.2%) while only 9.7% spend more than three hours on study at home. Peers showed great care during the blended learning experience (81%) and their experiences were appreciated by the society (66%). Workload management by learners vis-à-vis studying is good at 60%. Learners reported that their workmates stand in for them at workplaces to enable them do their study in blended learning while 61% are encouraged by their bosses to go and improve their skills through further education and training. On the time spent on other activities not related to study, majority of the learners spend three hours (35%) while 19% spend 6 hours. Sixty percent of the learners have to answer to someone when they are not attending to other activities outside study compared to the 39.9% who do not and can therefore do study or those other activities.
The usability of the online system, tools and resources was below average as shown in the table below in percentages (Table 3 ):
However, learners became skilled at navigating around the learning management system (79%) and it was easy for them to locate course content, tools and resources needed such as course works, news, discussions and journal materials. They effectively used the communication tools (60%) and to work with peers by making posts (57%). They reported that online resources were well organized, user friendly and easy to access (71%) as well as well structured in a clear and understandable manner (72%). They therefore recommended the use of online resources for other course units in future (78%) because they were satisfied with them (64.3%). On the whole, the online resources were fine for the learners (67.2%) and useful as a learning resource (80%). The learners’ perceived usefulness/satisfaction with online system, tools, and resources was at 81% as the LMS tools helped them to communicate, work with peers and reflect on their learning (74%). They reported that using moodle helped them to learn new concepts, information and gaining skills (85.3%) as well as sharing what they knew or learned (76.4%). They enjoyed the course units (78%) and improved their skills with technology (89%).
Learner interactions were seen from three angles of cognitivism, collaborative learning and student-teacher interactions. Collaborative learning was average at 50% with low percentages in learners posting challenges to colleagues’ ideas online (34%) and posting ideas for colleagues to read online (37%). They however met oftentimes online (60%) and organized how they would work together in study during the face-to-face meetings (69%). The common form of communication medium frequently used by learners during the blended learning experience was by phone (34.5%) followed by whatsapp (21.8%), face book (21%), discussion board (11.8%) and email (10.9%). At the cognitive level, learners interacted with content at 72% by reading the posted content (81%), exchanging knowledge via the LMS (58.4%), participating in discussions on the forum (62%) and got course objectives and structure introduced during the face-to-face sessions (86%). Student-teacher interaction was reported at 71% through instructors individually working with them online (57.2%) and being well guided towards learning goals (81%). They did receive suggestions from instructors about resources to use in their learning (75.3%) and instructors provided learning input for them to come up with their own answers (71%).
The technology quality during the blended learning intervention was rated at 69% with availability of 72%, quality of the resources was at 68% with learners reporting that discussion boards gave right content necessary for study (71%) and the email exchanges containing relevant and much needed information (63.4%) as well as chats comprising of essential information to aid the learning (69%). Internet reliability was rated at 66% with a speed considered averagely good to facilitate online activities (63%). They however reported that there was intermittent breakdown during online study (67%) though they could complete their internet program during connection (63.4%). Learners eventually found it easy to download necessary materials for study in their blended learning experiences (71%).
Learner extent of use of the learning management system features was as shown in the table below in percentage (Table 4 ):
From the table, very rarely used features include the blog and wiki while very often used ones include the email, forum, chat and calendar.
The effectiveness of the LMS was rated at 79% by learners reporting that they found it useful (89%) and using it makes their learning activities much easier (75.2%). Moodle has helped learners to accomplish their learning tasks more quickly (74%) and that as a LMS, it is effective in teaching and learning (88%) with overall satisfaction levels at 68%. However, learners note challenges in the use of the LMS regarding its performance as having been problematic to them (57%) and only 8% of the learners reported navigation while 16% reported access as challenges.
Learner attitudes towards Face-to-face support were reported at 88% showing that the sessions were enjoyable experiences (89%) with high quality class discussions (86%) and therefore recommended that the sessions should continue in blended learning (89%). The frequency of the face-to-face sessions is shown in the table below as preferred by learners (Table 5 ).
Learners preferred face-to-face sessions after every month in the semester (33.6%) and at the beginning of the blended learning session only (27.7%).
Learners reported high intrinsic motivation levels with interest and enjoyment of tasks at 83.7%, perceived competence at 70.2%, effort/importance sub-scale at 80%, pressure/tension reported at 54%. The pressure percentage of 54% arises from learners feeling nervous (39.2%) and a lot of anxiety (53%) while 44% felt a lot of pressure during the blended learning experiences. Learners however reported the value/usefulness of blended learning at 91% with majority believing that studying online and face-to-face had value for them (93.3%) and were therefore willing to take part in blended learning (91.2%). They showed that it is beneficial for them (94%) and that it was an important way of studying (84.3%).
Learner satisfaction was reported at 81% especially with instructors (85%) high percentage reported on encouraging learner participation during the course of study 93%, course content (83%) with the highest being satisfaction with the good relationship between the objectives of the course units and the content (90%), technology (71%) with a high percentage on the fact that the platform was adequate for the online part of the learning (76%), interactions (75%) with participation in class at 79%, and face-to-face sessions (91%) with learner satisfaction high on face-to-face sessions being good enough for interaction and giving an overview of the courses when objectives were introduced at 92%.
Learners’ knowledge construction was reported at 78% with initiation and discovery scales scoring 84% with 88% specifically for discovering the learning points in the course units. The accomplishment scale in knowledge construction scored 71% and specifically the fact that learners were able to work together with group members to accomplish learning tasks throughout the study of the course units (79%). Learners developed reports from activities (67%), submitted solutions to discussion questions (68%) and did critique peer arguments (69%). Generally, learners performed well in blended learning in the final examination with an average pass of 62% and standard deviation of 7.5.
Significant predictors of blended learning effectiveness ( RQ 2)
A standard multiple regression analysis was done taking learner characteristics/background and design features as predictor variables and learning outcomes as criterion variables. The data was first tested to check if it met the linear regression test assumptions and results showed the correlations between the independent variables and each of the dependent variables (highest 0.62 and lowest 0.22) as not being too high, which indicated that multicollinearity was not a problem in our model. From the coefficients table, the VIF values ranged from 1.0 to 2.4, well below the cut off value of 10 and indicating no possibility of multicollinearity. The normal probability plot was seen to lie as a reasonably straight diagonal from bottom left to top right indicating normality of our data. Linearity was found suitable from the scatter plot of the standardized residuals and was rectangular in distribution. Outliers were no cause for concern in our data since we had only 1% of all cases falling outside 3.0 thus proving the data as a normally distributed sample. Our R -square values was at 0.525 meaning that the independent variables explained about 53% of the variance in overall satisfaction, motivation and knowledge construction of the learners. All the models explaining the three dependent variables of learner satisfaction, intrinsic motivation and knowledge construction were significant at the 0.000 probability level (Table 6 ).
From the table above, design features (technology quality and online tools and resources), and learner characteristics (attitudes to blended learning, self-regulation) were significant predictors of learner satisfaction in blended learning. This means that good technology with the features involved and the learner positive attitudes with capacity to do blended learning with self drive led to their satisfaction. The design features (technology quality, interactions) and learner characteristics (self regulation and social support), were found to be significant predictors of learner knowledge construction. This implies that learners’ capacity to go on their work by themselves supported by peers and high levels of interaction using the quality technology led them to construct their own ideas in blended learning. Design features (technology quality, online tools and resources as well as learner interactions) and learner characteristics (self regulation), significantly predicted the learners’ intrinsic motivation in blended learning suggesting that good technology, tools and high interaction levels with independence in learning led to learners being highly motivated. Finally, none of the independent variables considered under this study were predictors of learning outcomes (grade).
In this study we have investigated learning outcomes as dependent variables to establish if particular learner characteristics/backgrounds and design features are related to the outcomes for blended learning effectiveness and if they predict learning outcomes in blended learning. We took students from three schools out of five and one directorate of post-graduate studies at a Ugandan University. The study suggests that the characteristics and design features examined are good drivers towards an effective blended learning environment though a few of them predicted learning outcomes in blended learning.
Student characteristics/background, blended learning design features and learning outcomes
The learner characteristics, design features investigated are potentially important for an effective blended learning environment. Performance by gender shows a balance with no statistical differences between male and female. There are statistically significant differences ( p < .005) in the performance between age groups with means of 62% for age group 20–30 and 67% for age group 31 –39. The indicators of self regulation exist as well as positive attitudes towards blended learning. Learners do well with word processing, e-mail, spreadsheets and web browsers but still lag below average in html tools. They show computer confidence at 75.3%; which gives prospects for an effective blended learning environment in regard to their computer competence and confidence. The levels of family and social support for learners stand at 61 and 75% respectively, indicating potential for blended learning to be effective. The learners’ balance between study and work is a drive factor towards blended learning effectiveness since their management of their workload vis a vis study time is at 60 and 61% of the learners are encouraged to go for study by their bosses. Learner satisfaction with the online system and its tools shows prospect for blended learning effectiveness but there are challenges in regard to locating course content and assignments, submitting their work and staying on a task during online study. Average collaborative, cognitive learning as well as learner-teacher interactions exist as important factors. Technology quality for effective blended learning is a potential for effectiveness though features like the blog and wiki are rarely used by learners. Face-to-face support is satisfactory and it should be conducted every month. There is high intrinsic motivation, satisfaction and knowledge construction as well as good performance in examinations ( M = 62%, SD = 7.5); which indicates potentiality for blended learning effectiveness.
Significant predictors of blended learning effectiveness
Among the design features, technology quality, online tools and face-to-face support are predictors of learner satisfaction while learner characteristics of self regulation and attitudes to blended learning are predictors of satisfaction. Technology quality and interactions are the only design features predicting learner knowledge construction, while social support, among the learner backgrounds, is a predictor of knowledge construction. Self regulation as a learner characteristic is a predictor of knowledge construction. Self regulation is the only learner characteristic predicting intrinsic motivation in blended learning while technology quality, online tools and interactions are the design features predicting intrinsic motivation. However, all the independent variables are not significant predictors of learning performance in blended learning.
The high computer competences and confidence is an antecedent factor for blended learning effectiveness as noted by Hadad ( 2007 ) and this study finds learners confident and competent enough for the effectiveness of blended learning. A lack in computer skills causes failure in e-learning and blended learning as noted by Shraim and Khlaif ( 2010 ). From our study findings, this is no threat for blended learning our case as noted by our results. Contrary to Cohen et al. ( 2012 ) findings that learners’ family responsibilities and hours of employment can impede their process of learning, it is not the case here since they are drivers to the blended learning process. Time conflict, as compounded by family, employment status and management support (Packham et al., 2004 ) were noted as causes of learner failure and drop out of online courses. Our results show, on the contrary, that these factors are drivers for blended learning effectiveness because learners have a good balance between work and study and are supported by bosses to study. In agreement with Selim ( 2007 ), learner positive attitudes towards e-and blended learning environments are success factors. In line with Coldwell et al. ( 2008 ), no statistically significant differences exist between age groups. We however note that Coldwel, et al dealt with young, middle-aged and old above 45 years whereas we dealt with young and middle aged only.
Learner interactions at all levels are good enough and contrary to Astleitner, ( 2000 ) that their absence makes learners withdraw, they are a drive factor here. In line with Loukis (2007) the LMS quality, reliability and ease of use lead to learning efficiency as technology quality, online tools are predictors of learner satisfaction and intrinsic motivation. Face-to-face sessions should continue on a monthly basis as noted here and is in agreement with Marriot et al. ( 2004 ) who noted learner preference for it for facilitating social interaction and communication skills. High learner intrinsic motivation leads to persistence in online courses as noted by Menager-Beeley, ( 2004 ) and is high enough in our study. This implies a possibility of an effectiveness blended learning environment. The causes of learner dissatisfaction noted by Islam ( 2014 ) such as incompetence in the use of the LMS are contrary to our results in our study, while the one noted by Hara and Kling, ( 2001 ) as resulting from technical difficulties and ambiguous course instruction are no threat from our findings. Student-teacher interaction showed a relation with satisfaction according to Swan ( 2001 ) but is not a predictor in our study. Initiating knowledge construction by learners for blended learning effectiveness is exhibited in our findings and agrees with Rahman, Yasin and Jusof ( 2011 ). Our study has not agreed with Eom et al. ( 2006 ) who found learner interactions as predictors of learner satisfaction but agrees with Naaj et al. ( 2012 ) regarding technology as a predictor of learner satisfaction.
Conclusion and recommendations
An effective blended learning environment is necessary in undertaking innovative pedagogical approaches through the use of technology in teaching and learning. An examination of learner characteristics/background, design features and learning outcomes as factors for effectiveness can help to inform the design of effective learning environments that involve face-to-face sessions and online aspects. Most of the student characteristics and blended learning design features dealt with in this study are important factors for blended learning effectiveness. None of the independent variables were identified as significant predictors of student performance. These gaps are open for further investigation in order to understand if they can be significant predictors of blended learning effectiveness in a similar or different learning setting.
In planning to design and implement blended learning, we are mindful of the implications raised by this study which is a planning evaluation research for the design and eventual implementation of blended learning. Universities should be mindful of the interplay between the learner characteristics, design features and learning outcomes which are indicators of blended learning effectiveness. From this research, learners manifest high potential to take on blended learning more especially in regard to learner self-regulation exhibited. Blended learning is meant to increase learners’ levels of knowledge construction in order to create analytical skills in them. Learner ability to assess and critically evaluate knowledge sources is hereby established in our findings. This can go a long way in producing skilled learners who can be innovative graduates enough to satisfy employment demands through creativity and innovativeness. Technology being less of a shock to students gives potential for blended learning design. Universities and other institutions of learning should continue to emphasize blended learning approaches through installation of learning management systems along with strong internet to enable effective learning through technology especially in the developing world.
Abubakar, D. & Adetimirin. (2015). Influence of computer literacy on post-graduates’ use of e-resources in Nigerian University Libraries. Library Philosophy and Practice. From http://digitalcommons.unl.edu/libphilprac/ . Retrieved 18 Aug 2015.
Ahmad, N., & Al-Khanjari, Z. (2011). Effect of Moodle on learning: An Oman perception. International Journal of Digital Information and Wireless Communications (IJDIWC), 1 (4), 746–752.
Google Scholar
Anderson, T. (2004). Theory and Practice of Online Learning . Canada: AU Press, Athabasca University.
Arbaugh, J. B. (2000). How classroom environment and student engagement affect learning in internet-basedMBAcourses. Business Communication Quarterly, 63 (4), 9–18.
Article Google Scholar
Askar, P. & Altun, A. (2008). Learner satisfaction on blended learning. E-Leader Krakow , 2008.
Astleitner, H. (2000) Dropout and distance education. A review of motivational and emotional strategies to reduce dropout in web-based distance education. In Neuwe Medien in Unterricht, Aus-und Weiterbildung Waxmann Munster, New York.
Barnard, L., Lan, W. Y., To, Y. M., Paton, V. O., & Lai, S. (2009). Measuring self regulation in online and blended learning environments’. Internet and Higher Education, 12 (1), 1–6.
Beard, L. A., Harper, C., & Riley, G. (2004). Online versus on-campus instruction: student attitudes & perceptions. TechTrends, 48 (6), 29–31.
Berenson, R., Boyles, G., & Weaver, A. (2008). Emotional intelligence as a predictor for success in online learning. International Review of Research in open & Distance Learning, 9 (2), 1–16.
Blocker, J. M., & Tucker, G. (2001). Using constructivist principles in designing and integrating online collaborative interactions. In F. Fuller & R. McBride (Eds.), Distance education. Proceedings of the Society for Information Technology & Teacher Education International Conference (pp. 32–36). ERIC Document Reproduction Service No. ED 457 822.
Cohen, K. E., Stage, F. K., Hammack, F. M., & Marcus, A. (2012). Persistence of master’s students in the United States: Developing and testing of a conceptual model . USA: PhD Dissertation, New York University.
Coldwell, J., Craig, A., Paterson, T., & Mustard, J. (2008). Online students: Relationships between participation, demographics and academic performance. The Electronic Journal of e-learning, 6 (1), 19–30.
Deci, E. L., & Ryan, R. M. (1982). Intrinsic Motivation Inventory. Available from selfdeterminationtheory.org/intrinsic-motivation-inventory/ . Accessed 2 Aug 2016.
Delone, W. H., & McLean, E. R. (2003). The Delone and McLean model of information systems success: A Ten-year update. Journal of Management Information Systems, 19 (4), 9–30.
Demirkol, M., & Kazu, I. Y. (2014). Effect of blended environment model on high school students’ academic achievement. The Turkish Online Journal of Educational Technology, 13 (1), 78–87.
Eom, S., Wen, H., & Ashill, N. (2006). The determinants of students’ perceived learning outcomes and satisfaction in university online education: an empirical investigation’. Decision Sciences Journal of Innovative Education, 4 (2), 215–235.
Garrison, D. R., & Kanuka, H. (2004). Blended learning: Uncovering its transformative potential in higher education. Internet and Higher Education, 7 (2), 95–105.
Goyal, E., & Tambe, S. (2015). Effectiveness of Moodle-enabled blended learning in private Indian Business School teaching NICHE programs. The Online Journal of New Horizons in Education, 5 (2), 14–22.
Green, J., Nelson, G., Martin, A. J., & Marsh, H. (2006). The causal ordering of self-concept and academic motivation and its effect on academic achievement. International Education Journal, 7 (4), 534–546.
Guskey, T. R. (2000). Evaluating Professional Development . Thousands Oaks: Corwin Press.
Hadad, W. (2007). ICT-in-education toolkit reference handbook . InfoDev. from http://www.infodev.org/en/Publication.301.html . Retrieved 04 Aug 2015.
Hara, N. & Kling, R. (2001). Student distress in web-based distance education. Educause Quarterly. 3 (2001).
Heinich, R., Molenda, M., Russell, J. D., & Smaldino, S. E. (2001). Instructional Media and Technologies for Learning (7th ed.). Englewood Cliffs: Prentice-Hall.
Hofmann, J. (2014). Solutions to the top 10 challenges of blended learning. Top 10 challenges of blended learning. Available on cedma-europe.org .
Islam, A. K. M. N. (2014). Sources of satisfaction and dissatisfaction with a learning management system in post-adoption stage: A critical incident technique approach. Computers in Human Behaviour, 30 , 249–261.
Kelley, D. H. & Gorham, J. (2009) Effects of immediacy on recall of information. Communication Education, 37 (3), 198–207.
Kenney, J., & Newcombe, E. (2011). Adopting a blended learning approach: Challenges, encountered and lessons learned in an action research study. Journal of Asynchronous Learning Networks, 15 (1), 45–57.
Kintu, M. J., & Zhu, C. (2016). Student characteristics and learning outcomes in a blended learning environment intervention in a Ugandan University. Electronic Journal of e-Learning, 14 (3), 181–195.
Kuo, Y., Walker, A. E., Belland, B. R., & Schroder, L. E. E. (2013). A predictive study of student satisfaction in online education programs. International Review of Research in Open and Distributed Learning, 14 (1), 16–39.
Kwak, D. W., Menezes, F. M., & Sherwood, C. (2013). Assessing the impact of blended learning on student performance. Educational Technology & Society, 15 (1), 127–136.
Lim, D. H., & Kim, H. J. (2003). Motivation and learner characteristics affecting online learning and learning application. Journal of Educational Technology Systems, 31 (4), 423–439.
Lim, D. H., & Morris, M. L. (2009). Learner and instructional factors influencing learner outcomes within a blended learning environment. Educational Technology & Society, 12 (4), 282–293.
Lin, B., & Vassar, J. A. (2009). Determinants for success in online learning communities. International Journal of Web-based Communities, 5 (3), 340–350.
Loukis, E., Georgiou, S. & Pazalo, K. (2007). A value flow model for the evaluation of an e-learning service. ECIS, 2007 Proceedings, paper 175.
Lynch, R., & Dembo, M. (2004). The relationship between self regulation and online learning in a blended learning context. The International Review of Research in Open and Distributed Learning, 5 (2), 1–16.
Marriot, N., Marriot, P., & Selwyn. (2004). Accounting undergraduates’ changing use of ICT and their views on using the internet in higher education-A Research note. Accounting Education, 13 (4), 117–130.
Menager-Beeley, R. (2004). Web-based distance learning in a community college: The influence of task values on task choice, retention and commitment. (Doctoral dissertation, University of Southern California). Dissertation Abstracts International, 64 (9-A), 3191.
Naaj, M. A., Nachouki, M., & Ankit, A. (2012). Evaluating student satisfaction with blended learning in a gender-segregated environment. Journal of Information Technology Education: Research, 11 , 185–200.
Nurmela, K., Palonen, T., Lehtinen, E. & Hakkarainen, K. (2003). Developing tools for analysing CSCL process. In Wasson, B. Ludvigsen, S. & Hoppe, V. (eds), Designing for change in networked learning environments (pp 333–342). Dordrecht, The Netherlands, Kluwer.
Osgerby, J. (2013). Students’ perceptions of the introduction of a blended learning environment: An exploratory case study. Accounting Education, 22 (1), 85–99.
Oxford Group, (2013). Blended learning-current use, challenges and best practices. From http://www.kineo.com/m/0/blended-learning-report-202013.pdf . Accessed on 17 Mar 2016.
Packham, G., Jones, P., Miller, C., & Thomas, B. (2004). E-learning and retention key factors influencing student withdrawal. Education and Training, 46 (6–7), 335–342.
Pallant, J. (2010). SPSS Survival Mannual (4th ed.). Maidenhead: OUP McGraw-Hill.
Park, J.-H., & Choi, H. J. (2009). Factors influencing adult learners’ decision to drop out or persist in online learning. Educational Technology & Society, 12 (4), 207–217.
Picciano, A., & Seaman, J. (2007). K-12 online learning: A survey of U.S. school district administrators . New York, USA: Sloan-C.
Piccoli, G., Ahmad, R., & Ives, B. (2001). Web-based virtual learning environments: a research framework and a preliminary assessment of effectiveness in basic IT skill training. MIS Quarterly, 25 (4), 401–426.
Pituch, K. A., & Lee, Y. K. (2006). The influence of system characteristics on e-learning use. Computers & Education, 47 (2), 222–244.
Rahman, S. et al, (2011). Knowledge construction process in online learning. Middle East Journal of Scientific Research, 8 (2), 488–492.
Rovai, A. P. (2003). In search of higher persistence rates in distance education online programs. Computers & Education, 6 (1), 1–16.
Sankaran, S., & Bui, T. (2001). Impact of learning strategies and motivation on performance: A study in Web-based instruction. Journal of Instructional Psychology, 28 (3), 191–198.
Selim, H. M. (2007). Critical success factors for e-learning acceptance: Confirmatory factor models. Computers & Education, 49 (2), 396–413.
Shraim, K., & Khlaif, Z. N. (2010). An e-learning approach to secondary education in Palestine: opportunities and challenges. Information Technology for Development, 16 (3), 159–173.
Shrain, K. (2012). Moving towards e-learning paradigm: Readiness of higher education instructors in Palestine. International Journal on E-Learning, 11 (4), 441–463.
Song, L., Singleton, E. S., Hill, J. R., & Koh, M. H. (2004). Improving online learning: student perceptions of useful and challenging characteristics’. Internet and Higher Education, 7 (1), 59–70.
Stacey, E., & Gerbic, P. (2007). Teaching for blended learning: research perspectives from on-campus and distance students. Education and Information Technologies, 12 , 165–174.
Swan, K. (2001). Virtual interactivity: design factors affecting student satisfaction and perceived learning in asynchronous online courses. Distance Education, 22 (2), 306–331.
Article MathSciNet Google Scholar
Thompson, E. (2004). Distance education drop-out: What can we do? In R. Pospisil & L. Willcoxson (Eds.), Learning Through Teaching (Proceedings of the 6th Annual Teaching Learning Forum, pp. 324–332). Perth, Australia: Murdoch University.
Tselios, N., Daskalakis, S., & Papadopoulou, M. (2011). Assessing the acceptance of a blended learning university course. Educational Technology & Society, 14 (2), 224–235.
Willging, P. A., & Johnson, S. D. (2009). Factors that influence students’ decision to drop-out of online courses. Journal of Asynchronous Learning Networks, 13 (3), 115–127.
Zhu, C. (2012). Student satisfaction, performance and knowledge construction in online collaborative learning. Educational Technology & Society, 15 (1), 127–137.
Zielinski, D. (2000). Can you keep learners online? Training, 37 (3), 64–75.
Download references
Authors’ contribution
MJK conceived the study idea, developed the conceptual framework, collected the data, analyzed it and wrote the article. CZ gave the technical advice concerning the write-up and advised on relevant corrections to be made before final submission. EK did the proof-reading of the article as well as language editing. All authors read and approved the final manuscript.
Competing interests
The authors declare that they have no competing interests.
Author information
Authors and affiliations.
Mountains of the Moon University, P.O. Box 837, Fort Portal, Uganda
Mugenyi Justice Kintu & Edmond Kagambe
Vrije Universiteit Brussel, Pleinlaan 2, Brussels, 1050, Ixelles, Belgium
Mugenyi Justice Kintu & Chang Zhu
You can also search for this author in PubMed Google Scholar
Corresponding author
Correspondence to Mugenyi Justice Kintu .
Rights and permissions
Open Access This article is distributed under the terms of the Creative Commons Attribution 4.0 International License ( http://creativecommons.org/licenses/by/4.0/ ), which permits unrestricted use, distribution, and reproduction in any medium, provided you give appropriate credit to the original author(s) and the source, provide a link to the Creative Commons license, and indicate if changes were made.
Reprints and permissions
About this article
Cite this article.
Kintu, M.J., Zhu, C. & Kagambe, E. Blended learning effectiveness: the relationship between student characteristics, design features and outcomes. Int J Educ Technol High Educ 14 , 7 (2017). https://doi.org/10.1186/s41239-017-0043-4
Download citation
Received : 13 July 2016
Accepted : 23 November 2016
Published : 06 February 2017
DOI : https://doi.org/10.1186/s41239-017-0043-4
Share this article
Anyone you share the following link with will be able to read this content:
Sorry, a shareable link is not currently available for this article.
Provided by the Springer Nature SharedIt content-sharing initiative
- Blended learning effectiveness
- Learner characteristics
- Design features
- Learning outcomes and significant predictors
Accessibility Links
- Skip to content
- Skip to search IOPscience
- Skip to Journals list
- Accessibility help
- Accessibility Help
Click here to close this panel.
Purpose-led Publishing is a coalition of three not-for-profit publishers in the field of physical sciences: AIP Publishing, the American Physical Society and IOP Publishing.
Together, as publishers that will always put purpose above profit, we have defined a set of industry standards that underpin high-quality, ethical scholarly communications.
We are proudly declaring that science is our only shareholder.
The Impact of Time Management on Students' Academic Achievement
S N A M Razali 1 , M S Rusiman 1 , W S Gan 1 and N Arbin 2
Published under licence by IOP Publishing Ltd Journal of Physics: Conference Series , Volume 995 , International Seminar on Mathematics and Physics in Sciences and Technology 2017 (ISMAP 2017) 28–29 October 2017, Hotel Katerina, Malaysia Citation S N A M Razali et al 2018 J. Phys.: Conf. Ser. 995 012042 DOI 10.1088/1742-6596/995/1/012042
Article metrics
111407 Total downloads
Share this article
Author affiliations.
1 Department of Mathematics and Statistic, Faculty of Applied Science and Technology University Tun Hussein Onn Malaysia, Batu Pahat, Johor, Malaysia.
2 Department of Mathematic, Faculty of Science and Mathematics, Universiti Pendidikan Sultan Idris, 35900 Perak, Malaysia.
Buy this article in print
Time management is very important and it may actually affect individual's overall performance and achievements. Students nowadays always commented that they do not have enough time to complete all the tasks assigned to them. In addition, a university environment's flexibility and freedom can derail students who have not mastered time management skills. Therefore, the aim of this study is to determine the relationship between the time management and academic achievement of the students. The factor analysis result showed three main factors associated with time management which can be classified as time planning, time attitudes and time wasting. The result also indicated that gender and races of students show no significant differences in time management behaviours. While year of study and faculty of students reveal the significant differences in the time management behaviours. Meanwhile, all the time management behaviours are significantly positively related to academic achievement of students although the relationship is weak. Time planning is the most significant correlated predictor.
Export citation and abstract BibTeX RIS
Content from this work may be used under the terms of the Creative Commons Attribution 3.0 licence . Any further distribution of this work must maintain attribution to the author(s) and the title of the work, journal citation and DOI.
Academia.edu no longer supports Internet Explorer.
To browse Academia.edu and the wider internet faster and more securely, please take a few seconds to upgrade your browser .
Enter the email address you signed up with and we'll email you a reset link.
- We're Hiring!
- Help Center
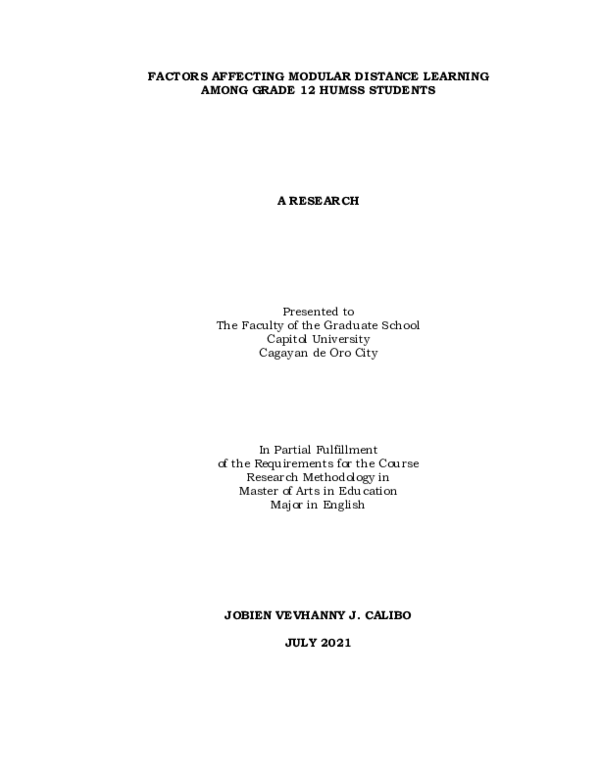
FACTORS AFFECTING MODULAR DISTANCE LEARNING AMONG GRADE 12 HUMSS STUDENTS

Related Papers
JPAIR Institutional Research
MARINO PAMOGAS
Modular Distance Learning is one of the learning modalities in the new normal using teacher-made modules with learning tasks based on the Most Essential Learning Competencies (MELCs). This online descriptive quantitative research investigated the extent of challenges faced by the Araling Panlipunan and Social Science teachers from the ten districts of the Schools Division Office of Surigao City for the school year 2020-2021, in which purposive sampling was used. Mean and standard deviation were the statistical tools used to treat the data. In a nutshell, the result reveals a moderate difficulty encountered by the teacher-respondents in facilitating modular distance learning, with an overall mean of 2.78 and a standard deviation of 0.71. These struggles manifest because handling modular classes during the pandemic was a surprise. The specific findings show that the Development of Self-Learning Modules (SLMs) and Quality Assurance topped the challenges of teachers. Other factors, such...
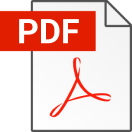
Proceedings of SOCIOINT 2021 8th International Conference on Education and Education of Social Sciences
Tarhata S . Guiamalon , SITTIE ALMIRAH ALON
To give consideration of the learners in rural areas where the internet inaccessible for online learning, Modular Learning modality is currently used by all public schools in the Philippines. A modular earning is a form of distance learning that uses Self-Learning Modules (SLM) and is one of the highly convenient for most of the typical Filipino students. It was also the most preferred learning system of the majority of parents/guardians for their children. The SLM is based on the most essential learning competencies (MELCS) provided by the Department of Education. The study was conducted to determine the issues and concerns on the use of Modular Distance Learning Modality among teachers. Of the ten different public elementary schools within the district of Buluan, Dvision of Maguindanao I, It found out that teachers are well-oriented and prepared to perform their tasks and functions on modular distance learning education in times of pandemic. They also have enough trainings and skill development necessary to effectively and efficiently do their job. Parents/guardian can able to support their children in the new learning modality but some of them are hampered because of incapability of facilitating and explaining the modules provided for their children. The study shows the elementary schools have given sufficient funds and resources and it is utilized in its proper allocation. These schools were located at Buluan where strategically located in the southern tip of the Maguindanao Province of Bangsamoro Autonomous Region in Muslim Mindanao (BARMM). It is composed of seven barangays occupying a total land area of 69,950 hectares. It has a substantial share of the famous Buluan Lake.
Challenges and Barriers Encountered by G10-Agoncillo Learners in the Implementation of Modular Distance Learning at Taal National High School
Waylie Niña De Claro
Modular Distance Learning offered a brand-new avenue in the education industry since the pandemic started. Amidst COVID-19 pandemic, DepEd ensured the continuity of learning through MDL-Self Learning Modules based on the Most Essential Learning Competencies. However, on the first few months of its implementation, several concerns where observed. Some of these were learners’ concerns. In order to address these concerns, this research was conducted to determine the challenges and barriers that G10 - Agoncillo learners from Taal NHS had encountered due to the implementation of Modular Distance Learning. The descriptive method of research was used. A research-made the questionnaire was used to gather data necessary for the study. The data collected were analyzed and statistically interpreted to support the identified questions. The researcher made use of frequency count, weighted mean, and ranking to explain the data gathered. The results of the study revealed that respondents considered “Poor Time Management” as one of their greatest challenges encountered. Moreover, the researcher found out that the respondents have series of solutions to the identified challenges and thus ranked them from the most relevant to least relevant. The study further revealed that a series of suggested activities may help learners address their challenges and barriers properly thus accomplishing their MDL-SLM properly. The researcher recommended that a.) learners should proceed with their suggested solutions to overcome the challenges they encountered, specifically on time management, self-motivation, and learning focus b) parents and guardians should actively support the school in the execution of MDL-SLM by facilitating learning at home c) content writers and teachers should always consider learners’ diversity and needs and d) identified challenges and barriers should be addressed properly to help improve learner’s performance.
Journal of Educational and Management Studies
GELLI A G U I L A R ABANDO
The present study aimed to have an in-depth phenomenological understanding of the various perceptions of the non-randomly selected five secondary English teachers in a public school in Paracale, Camarines Norte, Philippines towards the Modular Learning Modality being implemented by the Department of Education in terms of the following parameters; 1) demographic profile of the participants in terms of age, sex and location of residence; 2) the various perceptions of the participants based on their daily experiences as secondary English teachers towards the Modular Learning Modality in terms of school materials and devices navigation concerns and issues, financial aspect, and communicating with the learners and learner's parents and; 3.) practical recommendations by the participants to the learners, parents and school administrators in order to (if not to) lessen negative perceptions (difficulties) but to also improve the system implementation of Modular Learning Modality. This study was done by doing a face-to-face interview method (considering strict health protocols) with the participants in the data collection procedure. Thematic analysis approach specifically the coding of themes was done to filter and have an in-depth understanding of the different perceptions of the participants towards MLD in a public school in Paracale, Camarines Norte. Results revealed that most of the participants perceived that the MLD is difficult in terms of materials and devices navigation concerns and issues especially when the materials and devices needed for huge volume reproduction of modules for a large number of learners are insufficient and unstable. The participants also perceived that there are challenges in the financial aspect, communicating with both parents and learners when distributing, retrieving, and checking the modules, learners have low comprehension levels, have no (available) communication devices such as mobile phones (at least), internet connectivity and too far home location. The participants recommended that material supplies and devices must be made available on time, and conduct face-to-face sessions with less than the usual number of students in a class for a short span of time daily or at least weekly. There should be para-teachers assigned to remote and far-flung areas to assist low-performing students and students who do not have access to adult assistance. Provide Filipino or Tagalog translations for the instructions in the modules, especially for materials written in English, and consider risograph in reproducing modules.
AJHSSR Journal
This study seeks to help the Philippine Educational System reflect on the implementation of the Modular distance learning. The study aimed to indicate the assessment of Modular Distance Learning perceived by Social Studies teacher and School principals of public Secondary Schools in Zone 1 of the division of Zambales regarding module content, module printing, module retrieval and distribution. The teacher-respondent is a Social Studies major, BS degree with Master's units, Teacher I and had been in the service for almost a decade.The principal-respondent is a Social Studies major, masteral degree holders, Principal II and had been in the service for almost two decades. Both the teacher and principal respondent always observe the difficulty on Modular Learning implementation as to the retrieval of printed modules and answer sheets respectively.
Psychology and Education: A Multidisciplinary Journal
Psychology and Education , Mayline Atienza
The action research was a quantitative study that aims to know the effects of the Modular Distance Learning Approach on the academic performance of Intermediate Indigenous Learners particularly the "Dumagat-Remontado and to present accurate data and studies that will help the learners, parents, and teachers, especially in this time of the pandemic. From the results presented on the 52 respondents surveyed in Puray Elementary School of Rodriguez, Rizal. The findings of the overall academic performance of 65 indigenous peoples recipients in a modular approach have a stronger sense of responsibility in their study which may have a good effect on their academic performance. The findings of the study in the respondent's demographic profile, sex, age, Who among the household members can provide instructional support to the child's distance learning, devices available at home that the learner can use for learning, Internet connection, preferred distance learning modalities and challenges that may affect the child's learning process through distance education are in the significant relationship between the academic performance of indigenous peoples learners. Based on the findings of the study and conclusions drawn from the findings, the following recommendations are hereby offered: First, we recommend that future researchers should have complete information on the general average of the indigenous people learners to accurately get the relationship between the academic performance and effects of Modular Distance Learning Approach. Since the gathered data used summative test results as part of the academic performance of the pupils in this kind of approach. Secondly, the school should continue to implement special projects like "Project AKBAKAT" to help indigenous people learners with parents who can't assist them in working on their modules due to slow educational attainment. To our education department, modules should be reliable and can easily be understood by the pupils and parents/guardians even if there is no direct guidance from the teachers, to make the modular approach more effective. Finally, for the parents and guardians, we strongly recommend that they should encourage the pupils to work hard in their studies despite the pandemic and help them to understand their lessons because it has a great impact on academic performance in a modular approach.
Psychology and Education
This study investigated the limitations experienced by students, parents, and teachers in the implementation of Modular Distance Learning in Lagundi-CCL National High School during the school year 2021-2022. The researcher utilized the combination of quantitative and qualitative methods of research. An online research questionnaire utilizing Google Form was used to gather necessary information from the eighty (80) students, eighty (80) parents, and thirty-one (31) teachers who served as the respondents of this paper. Based on the results, the three major limitations experienced by students were: 1) insufficient knowledge of parents/ family members; 2) unavailability of gadgets; and 3) too many activities. In addition, parents' three major limitations were: 1) insufficient knowledge about the lessons; 2) difficulties in schedule of distribution and retrieval of modules; and 3) working parents. Furthermore, the identified limitations of teachers were: 1) too many additional tasks for teachers; 2) unavailability of self-learning modules; and 3) students who were lagging behind. From these limitations the respondents had given their suggestions. The students suggested that: 1) lessen the activities that are given to them; 2) conduct an online class even once a week; and 3) give additional time to answer the learning tasks. Meanwhile, parents' suggestions were: 1) enough information and examples in the modules should be given; 2) lessen the learning tasks; and 3) guide the parents on how to assist their children. Lastly, teachers' suggestions include: 1) proper dissemination of program, projects, and activities related to modular distance learning; 2) capacitate parents and students on MDL; and 3) distribution and retrieval should be done every other two weeks. The researchers crafted a process framework which may serve as basis in the modification of the implementation of modular distance learning which included seven (7) strategic dimensions.
Asian Journal of Education and Social Studies
Ruth Tumaneng
This study used a descriptive phenomenological approach to identify the problems encountered by teachers and students in implementing modular distance learning, as well as the possible solutions to the identified problems. The study was conducted among the teachers and students of Bachelor of Elementary Education Department (BEEd) of Apayao State College – Luna Campus. Four (4) BEEd program mainstay teachers and ten (10) BEEd students were approached to request their voluntary involvement as key participants to the study. The participants were chosen using convenience sampling and then interviewed individually via video conference to learn more about their experiences as a data-gathering tool. Data were analyzed using a thematic analysis approach to find any patterns in the participants’ responses by transcribing, organizing, and coding. Findings revealed that teachers identified four (4) themes of problems related to the preparation of printed learning materials; management in the...
United International Journal for Research & Technology
UIJRT | United International Journal for Research & Technology
Modular Learning Delivery is one of the prime modes of education delivery in the world today as imposed by the new normal trend in the society. Every aspect of learning is now faced with the fact that attending school via physical classroom today is not possible. Most especially, English teachers in the new normal setting face the new challenge of adapting and adopting different distance education teaching strategies to fit in today's education setting. This study determined the modular learning delivery of English teachers in distance learning landscape in Bulan, Sorsogon during the school year 2020-2021. It utilized a descriptivequalitative research designs. Audio-recorded interviews were transcribed and underwent conventional content analysis to identify categorical themes to achieve the goal of this research. It was found that some strategies used by English teachers to implement distance learning are modular teaching, home-based reading intervention, creating social media accounts, home visits, conducting limited face-to-face to low risk areas, training parents to be para-teachers and learning support system with subcategories, namely: scaffolding, feedback and counselling. The challenges they encountered include health conditions of the teachers, weather condition, issues on printed modules, home situations and parents' educational background, expenses on reproducing, distributing and retrieval of the printed modules, and teachers' lack of training in the conduct of the distance learning delivery modality. There are relevant and significant practices to apply different strategies to implement language learning amidst pandemic. Teachers have to be skilful in choosing the right strategies to use and consider some relevant factors will affect language learning. Challenges are likewise encountered and teachers may address them appropriately to overcome the burdens that they confront in conducting modular learning and ensure a success in the learning continuity that the DepEd is aiming for Filipino learners. Further researches on the different issues prevailing the education system in the country today may be conducted.
Asia Pacific Journal of Advanced Education and Technology
Asia Pacific Journal of Advanced Education and Technology (APJAET)
This study aimed to determine the performance of Grade 7 learners in TLE class specifically in cookery and the issues encountered in the implementation of modular distance learning. The descriptive method of research was utilized in this study. A researcher-made questionnaire was the main data-gathering instrument. Respondents were the 100 Grade 7 students of Dagatan Integrated National High School. The statistical tools that were used by the researchers throughout the study were simple frequency and percent counts, weighted mean, and Pearson Product-Moment Correlation Coefficient at a .05 level of significance. The respondents perceived issues encountered in using the learning module in terms of physical absence to great extent. While, the academic support, availability of instructional materials, learning process, learning space at home, feedback and monitoring, and assessment was perceived as moderate extent. The level of performance of respondents in terms of written works was at risk or below 75 while in terms of performance tasks they got outstanding grades. Based on the findings it was concluded that the hypothesis stating that there is no significant relationship between the perceived issues encountered in using learning modules and performance in TLE is not sustained with regards to the availability of instructional materials and feedback and monitoring of written works. Furthermore, the same conclusion was observed with regard to the relationship of the profile of the respondents in terms of gender to written works, and performance tasks. On the other hand, the rest of the variables under study were found not significantly related, hence the null hypothesis in this is reflected as sustained. Thus, it was recommended that the Department of Education may consider the findings of this study in the development of necessary academic materials. Similar study may be conducted focusing on other areas of TLE-Exploratory Course while addressing other problems and challenges met not only by the students but also by the teachers.
RELATED PAPERS
The European Physical Journal C
P. Malzacher
SSRN Electronic Journal
SF Qorina Fauzi
Juan José Bas
Amelia Silveira
Prehospital and Disaster Medicine
Jean luc Fortin
Physical Review D
Journal of nuclear medicine : official publication, Society of Nuclear Medicine
Helmut Maecke
Sharit valentina Cordoba Alarcón
Journal of Research in Science Teaching
Cathy Loving
New Microbes and New Infections
Claudia Barberis
Revista Ecuatoriana de Medicina y Ciencias Biológicas
Tjitte de Vries
Medicine & Science in Sports & Exercise
Motoyuki Iemitsu
Current Gastroenterology Reports
George Mazariegos
Jean Yoshii
Revista Veredas da História
Roberto Silva de Oliveira
Pérez García
Research Journal of Environmental Sciences
btissam Ennaffah
Revista Médica Electrónica
Maybet Perez
Journal of Agriculture, Food Systems, and Community Development
Isaac Leslie
Philippe Duchon
Journal of Safety Research
Teuvo Peltoniemi
Huisarts en Wetenschap
2015 14th IAPR International Conference on Machine Vision Applications (MVA)
Mohamed Jaward
Opeyemi Samuel
- We're Hiring!
- Help Center
- Find new research papers in:
- Health Sciences
- Earth Sciences
- Cognitive Science
- Mathematics
- Computer Science
- Academia ©2024
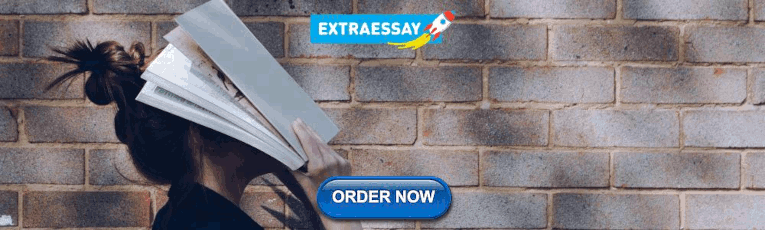
COMMENTS
The term "modular approach" refers to learning that takes the form of individualized instruction and allows students to use Self-Learning Modules (SLMs) in the print or advanced format/electronic ...
achievement r = .778 of distance learning students. It is concluded that both variables are associated with each other and time utilization skill affects students learning seriously. Students of web-based learning may more focus on proper time managing ability for better academic success. Keywords: Time management, academic achievement, distance
Time management can be defined as clusters of behavioral skills that are important in the organization of study and course load (Lay & Schouwenburg, 1993).Empirical evidence suggests that effective time management is associated with greater academic achievement (McKenzie & Gow, 2004; Trueman & Hartley, 1996) as students learn coping strategies that allow them to negotiate competing demands.
Despite its recognized importance for academic success, much of the research investigating time management has proceeded without regard to a comprehensive theoretical model for understanding its connections to students' engagement, learning, or achievement. Our central argument is that self-regulated learning provides the rich conceptual framework necessary for understanding college students ...
The purpose of this descriptive paper was to explore and synthesize literature related to understanding modular learning and how it can be implemented effectively so faculty members embrace its use. An in-depth review of literature addressed topics including, Educational Theories supporting modular learning, the development of modular learning,
The COVID-19 pandemic brought extraordinary challenges to K-12 students in using modular distance learning. According to Transactional Distance Theory (TDT), which is defined as understanding the effects of distance learning in the cognitive domain, the current study constructs a theoretical framework to measure student satisfaction and Bloom's Taxonomy Theory (BTT) to measure students ...
For STEMM students to enter, thrive, and be innovative in their future careers, they must possess excellent time management skills. We provide methods, resources, and strategies to help students better manage their time, which requires clear goal setting and proper time allocation to complete tasks. We begin by discussing goal setting followed ...
Achievement in science and mathematics is the highest among students with good time management. Procrastination negatively affects achievement. ... A. T. (2022). Impact of Modular Distance Learning on High School Students Mathematics Motivation, Interest/Attitude, Anxiety and Achievement during the COVID-19 Pandemic. ... Fentaw, Y., Moges, B. T ...
Improving online learning: student perceptions of useful and challenging characteristics'. Internet and Higher Education, 7(1), 59-70. Article Google Scholar Stacey, E., & Gerbic, P. (2007). Teaching for blended learning: research perspectives from on-campus and distance students.
The Impact of Time Management on Students' Academic Achievement. S N A M Razali 1, M S Rusiman 1, W S Gan 1 and N Arbin 2. Published under licence by IOP Publishing Ltd Journal of Physics: Conference Series, Volume 995, International Seminar on Mathematics and Physics in Sciences and Technology 2017 (ISMAP 2017) 28-29 October 2017, Hotel Katerina, Malaysia Citation S N A M Razali et al 2018 ...
Modular Distance Learning would help learners to be trained them on time management, setting their priorities, solve and answer the problems and questions given in their modules and helps learners mentally and it can be associated with developing self-regulation skill by being independent. ... 2477-8478 This research paper will help future ...
improving first year learning disabled students' academic time management and academic self efficacy. A total of 60 students identified with LD participated. The sample was divided into two groups; experimental ( n= 30 boys )and control ( n= 30 boys). ANCOVA and T .test were employed for data analysis. Findings from this study
This study aimed to determine the effectiveness of the modular learning approach to students' academic performance. The study was conducted in Sta. Cruz District in the province of Zambales, Philippines. A Descriptive Research Design was employed. A total of one hundred fifty-four (154) teacher-respondents were randomly selected.
Research Questions This study aimed to determine the learning challenges and opportunities experienced by students in modular distance learning during the pandemic. Specifically, it sought to answer the following questions: 1. What are the learning challenges faced by students on modular distance learning during the pandemic? 2.
Academia.edu is a platform for academics to share research papers. Procrastination Attitude of the Senior High School Students in Modular Distance Learning Modality ... First, students should have time management; (2) A healthy diet is a must; (3) Lastly, they should have motivations. Several students don't know how to manage their time ...
Notably, research reports that good time-management practices can extend beyond university study to significantly increase a person's quality of life (Wang et al., 2011). This paper explores the impact of providing a comparatively short training treatment of five time-management workshops on students' first-year study performance.
Time management for students includes setting realistic goals and deadlines for our self regarding major assignments. Make sure that we are not putting our self in ... RESEARCH PAPERS 40 i-manager's Journal on School Educational Technology, Vol. 10 l No. 3 l December 2014 - February 2015 (Best, 1982). The results of the analysis are presented in
Students in the Modular Distance Learning did not only learn topics but important values in the learning style of the learning modality which is Independent Learning. Ahmad et al (2019) note that there is a positive association between time management and the academic achievement of distance learning students.