
- About the LSE Impact Blog
- Comments Policy
- Popular Posts
- Recent Posts
- Subscribe to the Impact Blog
- Write for us
- LSE comment
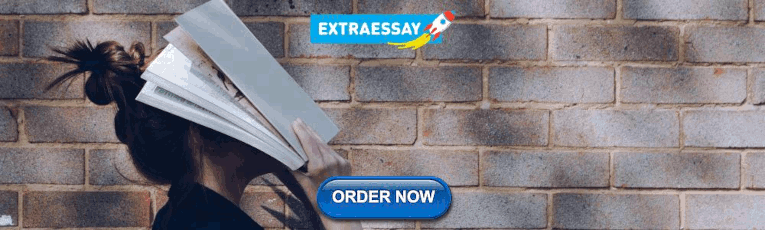
Alessandro Checco
Lorenzo bracciale, pierpaolo loreti, stephen pinfield, giuseppe bianchi, may 17th, 2021, can ai be used ethically to assist peer review.
3 comments | 194 shares
Estimated reading time: 7 minutes
As the rate and volume of academic publications has risen, so too has the pressure on journal editors to quickly find reviewers to assess the quality of academic work. In this context the potential of Artificial Intelligence (AI) to boost productivity and reduce workload has received significant attention. Drawing on evidence from an experiment utilising AI to learn and assess peer review outcomes, Alessandro Checco, Lorenzo Bracciale, Pierpaolo Loreti, Stephen Pinfield, and Giuseppe Bianchi, discuss the prospects for AI for assisting peer review and the potential ethical dilemmas its application might produce.
The scholarly communication process is under strain, particularly because of increasing demands on peer reviewers. Manuscript submissions to peer-review journals are growing roughly 6% annually. Every year, over 15 million hours are spent on reviewing manuscripts previously rejected and then resubmitted to other journals. Many of these could be avoided at the pre-peer review screening phase.

Fig.1 Stages of the Peer Review process.
Rather than more grandiose visions of replacing human decision-making entirely, we are interested in understanding the extent to which AI might assist reviewers and authors in dealing with this burden. Giving rise to the question: can we use AI as a rudimentary tool to model human reviewer decision making?
Experimenting with AI peer review
To test this proposition, we trained a neural network using a collection of submitted manuscripts of engineering conference papers, together with their associated peer review decisions.
The AI tool analysed the manuscripts using a set of features: the textual content, together with readability scores and formatting measures. Our analysis covers the parts of the quality assurance process of outputs where pre-peer-review screening and peer review itself overlap, covering aspects like formatting, and quality of expression.
Once the learning phase was completed, we evaluated how accurate the empirical rules were in predicting the peer review outcome of a previously unobserved manuscript. Finally, we asked “ Why has the AI tool marked papers as accepted or rejected?” ‘, as answering that question may give us insight into the human decision-making the tool was modelling.

Fig.2 Schematised AI peer review tool.
Opening up AI decision making
Explaining models depending on half a million parameters is practically impossible using standard tools. Outcomes from the model can be affected by a whole range of different issues, such as the presence of specific words or particular sentence structures. We used a technique known as LIME to help explain what the model was doing in the case of specific documents. The technique is based on slightly changing the content of a document and observing how the model predictions change.
In the Fig.3 an example of an explanation for an accepted paper is shown. In orange, the top features influencing the decision towards a positive outcome are represented, while the blue colour represents factors associated with a negative decision. The absence of the word “quadratic”, a low sentence count, and a high number of difficult/unusual words positively affects the model score, while a low number of pages, a small number of average syllables per word and a low text length affect the model score negatively. In some cases, explanations like this can expose potential biases or overfitting of the model: when the dataset is too small, the model could for example give too much importance to the presence/absence of a keyword.

Fig.3 Explanation for machine learning based peer review decision.
Perhaps surprisingly, even using only rather superficial metrics to perform the training, the machine learning system was often able to successfully predict the peer review outcome reached as a result of human reviewers’ recommendations. In other words, there was a strong correlation between word distribution, readability and formatting scores, and the outcome of the review process as a whole. Thus, if a manuscript was well written, used appropriate terminology and was well presented, it was more likely to be accepted.
One possible explanation for the success of this rather simplistic model is that if a paper is presented and reads badly, it is likely to be of lower quality in other, more substantial, ways, making these more superficial features proxy useful metrics for quality.
Tools of the kind we developed have the potential to be of direct benefit in assisting editors of journals and conference proceedings in decision making.
However, it may be that papers that score less well on these superficial features create a “first impression bias” on the part of peer reviewers, who then are more inclined to reject papers based on this negative first impression derived from what are arguably relatively superficial problems.
Reviewers may be unduly influenced by formatting or grammatical issues (or the use of methods that have been associated with rejected papers in the past) and become unconsciously influenced by this in their judgements of more substantive issues in the submission.
In that case, an AI tool which screens papers prior to peer review could be used to advise authors to rework their paper before it is sent on for peer review. This might be of particular benefit to authors for whom English is not a first language, for example, and whose work, therefore, may be likely to be adversely affected by first impression bias.
Opportunities and Shortcomings
Tools of the kind we developed have the potential to be of direct benefit in assisting editors of journals and conference proceedings in decision making. They have the potential to save the time of reviewers, when used as decision support systems. They could also be useful to authors, as we have suggested. In particular, they might:
Reduce desk rejects
By catching the ‘first impression’, the approach we have explored in this paper has the potential to detect superficial problems early, like formatting issues and quality of the figures. Authors could be made aware of such problems immediately without any further review, or the AI tool could be used to pre-empt/inform desk rejects.
Improve human decision making with data
By analysing review decisions via a data-driven predictor/classifier, it is possible to investigate the extent to which the complex reviewing process can be modelled at scale. An analysis of the human decision process through data analysis and AI replication could potentially expose biases and similar issues in the decision-making process.
Biases and Ethical issues
A number of potential ethical issues arise from this work. Machine learning techniques are inherently conservative, as they are trained with data from the past. This could lead to bias and other unintended consequences, when used to inform decision-making in the future. For example, papers with characteristics associated with countries historically under-represented in the scientific literature might have a higher rejection rate using AI methods, since automated reviews will reflect the biases of the previous human reviewers, and, for example, may not take account of rising quality of submissions from such sources over time. Biases might also be introduced by the fact that historically, editors have disproportionately selected reviewers from high-income regions of the world, while low-income regions are under-represented amongst reviewers, the tool may then reflect the biases of previous reviewers.
An author will not trust an automated review if there is no transparency on the rationale for the decision taken. This means that any tools developed to assist decision making in scholarly communication need to make what is going on under the bonnet as clear as possible. This is particularly the case since models are the result of a particular design path that has been selected following the values and goals of the designer. These values and goals will inevitably be “ frozen into the code ”.
An author will not trust an automated review if there is no transparency on the rationale for the decision taken
It is also worth noting that tools designed to assist reviewers can influence them in particular ways. Even using such tools only to signal potentially problematic papers, could affect the agency of reviewers by raising doubts in their minds about a paper’s quality. The way the model interprets the manuscript could propagate to the reviewer, potentially creating an unintended biased outcome.
All of these ethical concerns need to be considered carefully in the way AI tools are designed and deployed in practice, and in determining the role they play in decision-making. Continued research in these areas is crucial in helping to ensure that the role AI tools play processes like peer review is a positive one.
This post draws on the authors’ paper AI-assisted peer review , published in Humanities and Social Sciences Communication and is a collaboration between the University of Sheffield and the University of Rome “Tor Vergata”.
Note: This article gives the views of the author, and not the position of the Impact of Social Science blog, nor of the London School of Economics. Please review our Comments Policy if you have any concerns on posting a comment below.
Image Credit: In text images reproduced with permission of the authors, featured image LSE Impact Blog.

About the author

Alessandro Checco is a lecturer in Business Analytics at the Information School, University of Sheffield, and he is the project coordinator of the H2020 FashionBrain project. His main research interests are human computation, recommender systems, information retrieval, data privacy, societal and economic analysis of digital labour, and algorithmic bias.

Dr. Lorenzo Bracciale (M) is an Adjunct Professor and Research Scientist at the University of Rome "Tor Vergata" in the Department of Electronic Engineering. His research interests cover distributed systems, communication systems and privacy-preserving technologies.

Dr. Pierpaolo Loreti is a Researcher in Telecommunications at the University of Roma Tor Vergata. His research activity spans different topics in the areas of wireless and mobile networks, IoT systems and platforms, framework design, analytic modeling, performance evaluation through simulation and test-bedding.

Stephen Pinfield is Professor of Information Services Management at the University of Sheffield. His research interests include research communication, scholarly digital practice and science policy. He is Associate Director of the Research on Research Institute (RoRI), an international collaboration aiming to carry out research on the research ecosystem in a way that can inform policy and practice. He tweets @StephenPinfield.

Dr. Giuseppe Bianchi (M) is Full Professor of Networking at the University of Roma Tor Vergata since January 2007. His research activity includes IP networking, Wireless LANs, privacy and security, traffic monitoring, and is documented in about 180 peer-reviewed international journal and conference papers, accounting for more than 11 500 citations and a H-index of 29 (Scholar Google). He’s co-inventor in 7 filed patents. He’s editor for IEEE/ACM Transactions on Networking, area editor for IEEE Transactions on Wireless Communication and editor for Elsevier Computer Communication.
The first step here, as the authors point out, is to use AI as a way of making the human reviewer’s job easier. Once reviewers (and hopefully authors) have built confidence that a certain service performs certain checks well, then that part of the review process could potentially be left to AI and the reviewers can concentrate on aspects of a manuscript that don’t currently lend themselves to automated assessment. However, once the structure, language, and presence/absence of certain key criteria are able to assessed automatically, the next phase will be look to the *content* of the paper and whether experiment design is sound, appropriate statistical methods have been used, and whether the manuscripts describes anything novel.
My view is we are ready to trial the first phase of this – with appropriate oversight – but the real exciting stuff is when we can analyse content as well as structure. I am techno-optimist and appreciate not everyone will be as positive about this as I am.
But this also brings up another point about readership of these articles. If the manuscripts are being ‘refined’ by AI, and then subsequently ‘read’ by AI (for text-mining purposes), are we humans becoming secondary considerations?
- Pingback: Weekly digest (17-21 May 2021) - /usr/space
- Pingback: Can AI be used ethically to assist peer review? « K. N. Raj Library - Content Alerts
Leave a Comment Cancel reply
Your email address will not be published. Required fields are marked *
Notify me of follow-up comments by email.
Related Posts
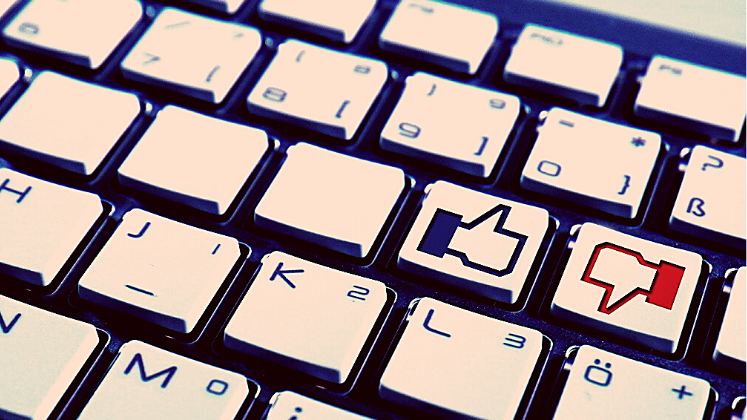
How the pandemic changed editorial peer review – and why we should wonder whether that’s desirable
February 10th, 2021.
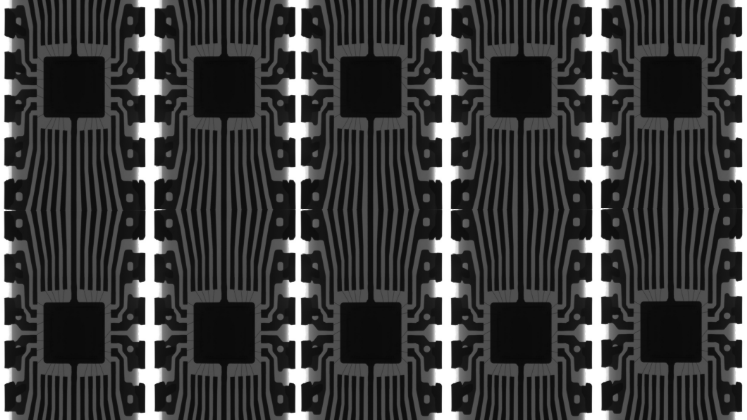
Podcast: Do algorithms have too much social power?
May 13th, 2021.

Reading Peer Review – What a dataset of peer review reports can teach us about changing research culture
March 31st, 2021.

Side-stepping safeguards – Data journalists are doing science now
April 26th, 2021.

Visit our sister blog LSE Review of Books
- Technical Support
- Find My Rep
You are here
- Using AI in peer review and publishing
Large Language Models (LLMs) and other AI models in Peer Review and Publishing
The opportunities and threats of using llms and ai in research writing.
At Sage, we recognize and champion new technology that facilitates conducting, writing and disseminating research. A multitude of tools and technology have been developed in recent years that can increase productivity and aid those perhaps writing in English as a second or third language.
We also recognize that the increasingly widespread use of generative AI/ LLMs blurs the lines between human generated and machine generated text, calling into question some of our usual assumptions and policies on authorship. This technology may be used by bad actors to create fabricated submissions and attempt to subvert the peer-review process for commercial gain. The currently available language models are not fully objective or factual. Authors using generative AI to write their research must make every effort to ensure that the output is factually correct, and the references provided reflect the claims made.
We’ve put together this guide for Editors around the use of LLMs and AI in scholarly publishing. As technology improves and we adapt to using these tools, we will likely develop this guidance further. Further resources that might be useful are listed at the bottom of the page. You could also have a look at our Sage Campus course, Introduction to Artificial Intelligence
If you have any questions or concerns about AI tools/LLMs do contact your Sage Editor in the first instance.
Sage policy on the use of LLMs in submissions
Potential biases in generative AI
The use of LLMs or AI tools in peer review
Combatting the use of ai to generate spurious content, detecting generative ai and llms, further resources.
Our policy on the use of LLMs in submissions can be found on our Publishing Policies pages: ChatGPT and Generative AI
Potential biases in generative AI
- The information being fed to the tool is curated by a human presence
- Some of these AI tools will be limited to using freely available resources and therefore based on a partial selection of the literature
- AI tools and LLMs are trained on sources that contain systemic biases and are likely to inadvertently replicate those biases in their outputs
- These tools may curate responses from multiple sources available on the internet which reduces accuracy and increases the chance of spurious information
Potentially false or misleading information and references
LLMs, like ChatGPT, have been seen to falsify references or insert incorrect ones within their essays or summaries. We have seen several instances where citations to publications that do not exist were provided by ChatGPT.
In addition, AI tools or LLMs may generate outputs that appear or sound plausible but cannot be validated or cannot be justified by its source data. This phenomenon, referred to as Hallucinations, can occur either directly from the source data or from the way the model is trained. Frequently occurring hallucinations are a considerable problem with this technology.
Image, data, and text fabrication in submissions
LLMs may be exploited by suspicious or bad actors to generate fabricated text, or text put together from various sources on the internet. While this may be appropriate for summarizing complex information for further study, it remains inappropriate for primary research articles that must contain critical new information, either with a new perspective or containing novel data. LLMs in primary research may only be detected using AI detection tools, but these tools cannot currently detect falsified references in text.
In addition, there are growing concerns that these tools may be used to generate images that are reported as primary data but were generated using AI. Before incorporating sources into your scholarly work, apply the CRAAP test to your responses to avoid sending out misinformation or spreading bias.
Use of LLMs for editors
The use of AI or LLMs for Editorial work presents confidentiality and copyright issues. As the tool or model will learn from what it receives over time and may use it to provide outputs to others, we ask Editors not to use these tools to triage manuscripts or create summaries. You should also not use these tools to summarize reviews and write decision letters due to concerns around confidentiality and copyright. You could use ChatGPT or other AI based tools to look for reviewers in the subject area. Due to concerns around spurious text generation, we ask that you verify their identity before inviting them to review a submission. Reviewer verification should typically involve checking their publication record and/or institutional profiles using a basic google/internet search.
Use of LLMs for reviewers
While LLMs can create a critical summary that would look like a review report, it is unlikely to be able to capture the reviewer’s experience as a researcher in the field, any local or contextual nuances of the study or indeed what impact the study may have on various populations. We ask that Editors ensure the reviewers invited are aware of the confidentiality issues presented by generating a review report using language models or generative AI. If an Editor is concerned about a review report that appears to be generated by ChatGPT or another tool, they should flag this to Sage for advice.
Vetted experts as reviewers
It continues to be important to use vetted reviewers who are experts in the field. Using reviewers who do not have specific expertise or those who cannot be verified increases the risk of machine written content to pass peer-review and masquerade as genuine human writing.
Reading the submission
Careful reading of text is crucial to understand if a submission was written by generative AI. As Editors, we rely on your subject level expertise to discern whether an article makes sense at the sentence level but also at the overall document level. If a sentence or paragraph does not make sense, or appears to be machine generated, please query it with authors, or raise it with Sage for advice. We recommend looking out for usual ChatGPT prompts such as “Regenerate response” in the text.
Qualitative indicators:
- Complexity of paragraph structure- humans are likely to have more sentences per paragraph in academic writing
- Diversity in sentence structures, length- humans are likely to have more words per paragraph and increased variability in length of consecutive sentences. For example, a short sentence followed by a very long sentence.
- Usage of punctuation unique to human academic writing- brackets, semi colons and colons
- Usage of equivocal words like “Although” or “However” or “but” and “because” are more commonly associated with human writing.
This is a constantly evolving landscape as LLMs are evolving fast and work into developing appropriate detection methods has been perceived as an arms race. We have identified some free tools that exist outside our submission system which will allow us to deepen our understanding of the AI generated content in our submissions and determine whether any currently available tools would help detection. We are undertaking a pilot on some journals to understand which tools may be useful for detection.
NB: many of the key differentiating traits between text generated by humans and AI-generated text—including the use of colloquial and emotional language—are not traits that academic scientists typically display in formal writing, so any differences or anomalies in this respect would not necessarily translate to academic writing.
WAME guidance on Chatbots, Generative AI and Scholarly Manuscripts
COPE guidance and news on Artificial Intelligence
ICMJE guidance on Artificial intelligence assisted technology
- Journal Author Gateway
- Increasing Citations and Improving Your Impact Factor
- Your role as Editor
- What you can expect from SAGE
- What SAGE expects from you
- Dignity at work
- Research ethics policies
- SAGE’s publication policy
- Publication ethics
- Types of publication misconduct
- Editor Guide to Peer Review Best Practice
- Open Access
- Working with Your Team
- Taking Action on Diversity
- Promote Your Journal
- Publishing Quality Content
- Publishing Special Issues
- Impact Factor & Ranking Results
- Editor Resources
- Peer Review and Sage Track Training Resources
- Journals Production
- New Journal Proposals
- Open Editor Positions
- Journal Editorial Board Members
- Journal Reviewer Gateway
- Ethics & Responsibility
- Sage Editorial Policies
- Publication Ethics Policies
- Sage Chinese Author Gateway 中国作者资源
- Open Resources & Current Initiatives
- Discipline Hubs
The May 2024 issue of IEEE Spectrum is here!
For IEEE Members
Ieee spectrum, follow ieee spectrum, support ieee spectrum, enjoy more free content and benefits by creating an account, saving articles to read later requires an ieee spectrum account, the institute content is only available for members, downloading full pdf issues is exclusive for ieee members, downloading this e-book is exclusive for ieee members, access to spectrum 's digital edition is exclusive for ieee members, following topics is a feature exclusive for ieee members, adding your response to an article requires an ieee spectrum account, create an account to access more content and features on ieee spectrum , including the ability to save articles to read later, download spectrum collections, and participate in conversations with readers and editors. for more exclusive content and features, consider joining ieee ., join the world’s largest professional organization devoted to engineering and applied sciences and get access to all of spectrum’s articles, archives, pdf downloads, and other benefits. learn more →, join the world’s largest professional organization devoted to engineering and applied sciences and get access to this e-book plus all of ieee spectrum’s articles, archives, pdf downloads, and other benefits. learn more →, access thousands of articles — completely free, create an account and get exclusive content and features: save articles, download collections, and talk to tech insiders — all free for full access and benefits, join ieee as a paying member., peer review of scholarly research gets an ai boost, open-access publisher's new artificial intelligence assistant, aira, can perform up to 20 recommendations in seconds.
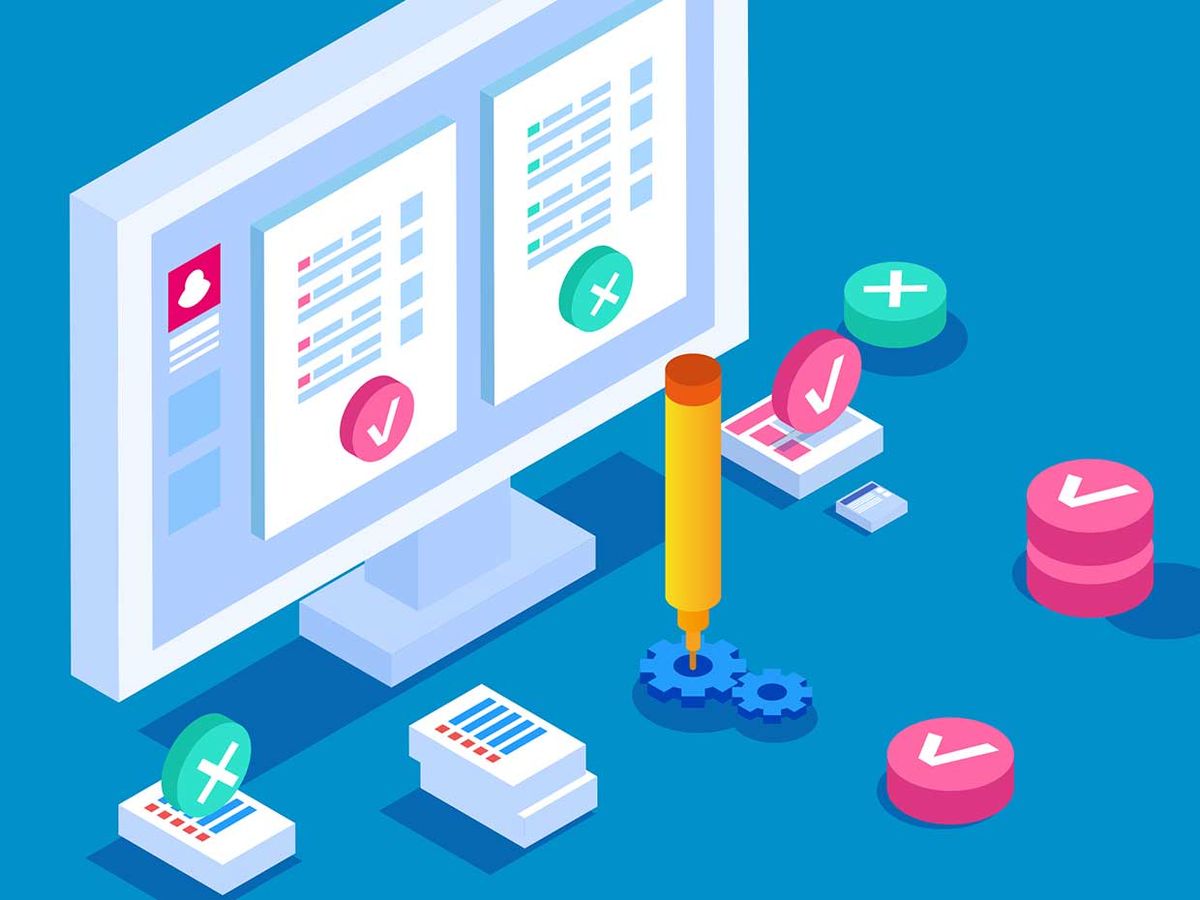
In the world of academics, peer review is considered the only credible validation of scholarly work. Although the process has its detractors , evaluation of academic research by a cohort of contemporaries has endured for over 350 years, with “ relatively minor changes .” However, peer review may be set to undergo its biggest revolution ever—the integration of artificial intelligence .
Open-access publisher Frontiers has debuted an AI tool called the Artificial Intelligence Review Assistant (AIRA), which purports to eliminate much of the grunt work associated with peer review. Since the beginning of June 2020, every one of the 11,000-plus submissions Frontiers received has been run through AIRA, which is integrated into its collaborative peer-review platform. This also makes it accessible to external users, accounting for some 100,000 editors, authors, and reviewers. Altogether, this helps “maximize the efficiency of the publishing process and make peer-review more objective,” says Kamila Markram , founder and CEO of Frontiers.
AIRA’s interactive online platform, which is a first of its kind in the industry, has been in development for three years.. It performs three broad functions, explains Daniel Petrariu , director of project management: assessing the quality of the manuscript, assessing quality of peer review, and recommending editors and reviewers. At the initial validation stage, the AI can make up to 20 recommendations and flag potential issues, including language quality, plagiarism, integrity of images, conflicts of interest, and so on. “This happens almost instantly and with [high] accuracy, far beyond the rate at which a human could be expected to complete a similar task,” Markram says.
“We have used a wide variety of machine-learning models for a diverse set of applications, including computer vision, natural language processing, and recommender systems,” says Markram. This includes simple bag-of-words models, as well as more sophisticated deep-learning ones. AIRA also leverages a large knowledge base of publications and authors.
Markram notes that, to address issues of possible AI bias, “We…[build] our own datasets and [design] our own algorithms. We make sure no statistical biases appear in the sampling of training and testing data. For example, when building a model to assess language quality, scientific fields are equally represented so the model isn’t biased toward any specific topic.” Machine- and deep-learning approaches, along with feedback from domain experts, including errors, are captured and used as additional training data. “By regularly re-training, we make sure our models improve in terms of accuracy and stay up-to-date.”
The AI’s job is to flag concerns; humans take the final decisions, says Petrariu. As an example, he cites image manipulation detection—something AI is super-efficient at but is nearly impossible for a human to perform with the same accuracy. “About 10 percent of our flagged images have some sort of problem,” he adds. “[In academic publishing] nobody has done this kind of comprehensive check [using AI] before,” says Petrariu. AIRA, he adds, facilitates Frontiers’ mission to make science open and knowledge accessible to all.
- Could AI Disrupt Peer Review? - IEEE Spectrum ›
Payal Dhar (she/they) is a freelance journalist on science, technology, and society. They write about AI, cybersecurity, surveillance, space, online communities, games, and any shiny new technology that catches their eye. You can find and DM Payal on Twitter (@payaldhar).
IEEE’s Honor Society Expands to More Countries
Video friday: loco-manipulation, smart antennas shape satellite internet tech to come, related stories, two natural-language ai algorithms walk into a bar..., are digital humans the next step in human-computer interaction, to learn to deal with uncertainty, this ai plays pong.
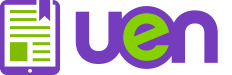
1 Navigating the New Frontier of Generative AI in Peer Review and Academic Writing
Chris Mayer
This chapter provides an overview of the current landscape and implications of Generative AI (GenAI) in higher education, particularly focusing on its role in academic writing and peer review. The emergence of GenAI tools such as ChatGPT is a transformative development in education, with widespread adoption at unprecedented speed. Large Language Models such as ChatGPT offer great potential for enhancing education and academic writing but also raise serious ethical concerns and tensions including access and usability, the perpetuation of Standard Academic English, and issues of linguistic justice. There is also the need for new approaches and pedagogies for how to ethically and effectively capitalize on this technology. Peer review has many benefits but can also be a source of anxiety and frustration for both teachers and students. The chapter discusses these issues and then offers an approach and lesson plans built around ChatGPT and John Bean’s hierarchy for error correction to guide in-class peer review in a first year writing class, as well as suggestions for how GenAI might be used for other disciplinary writing.
Keywords : Generative AI, academic writing, peer review, ethical considerations, pedagogical strategies
Introduction
The age of Skynet is on the horizon (or for the less cynical, the age of AI sentience). In less than two years, Generative AI has already begun reshaping the landscape of learning and evaluation. Sid Dobrin, a leading scholar on the intersections of writing and technology, writes that Generative AI (GenAI) is “now inextricably part of how students will write in the academy and beyond” (2023, p. 20). Indeed. In a survey of Canadian university students, more than half (52%) reported using GenAI in their schoolwork, and 87% agreed the quality of their work improved as a result of GenAI (KMPG, 2023). Another survey of 2000 US college students conducted in March and April of 2023 shows that 51% would continue using GenAI even if their schools or instructors prohibit their use (NeJame et al., 2023). This data strongly suggests GenAI adoption has already achieved critical mass. It is easy to see that “Higher education is beyond the point of no return” (NeJame et al., 2023).
As educators, we cannot ignore what is happening. Besides, universities are increasingly seen as preparing students for the workplace and AI will necessarily become part of that preparation. According to Stanford’s Artificial Intelligence Index Report, 34% of all industries were using AI for at least one function in their business in 2022 (Maslej et al., 2023). The number today is almost certainly higher.
In response to Dobrin’s question about whether GenAI heralds a “new phase of feedback to student writing processes” (2023, p. 21), I believe the answer is a resounding yes! It is widely known that feedback has a strong effect on student achievement and outcomes, including their motivation and self-efficacy (e.g., Hattie & Timperley, 2007; Wisniewski et al., 2019). However, providing feedback is time consuming and costly to provide whereas GenAI can do it nearly instantaneously. It has already been used for some years in computer science to provide formative feedback which students have found effective and useful (for instance, see Metz, 2021). Now with increasingly sophisticated language models, GenAI can be leveraged to give feedback to written essays outside the context of programming or simple (yet controversial) scoring of SAT essays.
In fact, GenAI even shows promise in providing formative feedback to instructors themselves. For instance, an AI tool called M-Powering Teachers was used to give teachers feedback on the feedback they gave students, specifically regarding the teachers’ uptake of student writing in their comments (uptake includes recasting a student’s idea and then framing a question aimed to generate more thinking, development, or perspectives). In their study, Demszky et al. (2023) found feedback offered by M-Powering Teachers increased the rate of uptake in instructors’ feedback to students. This increased the likelihood students would complete subsequent assignments and thus may be directly linked to student motivation.
GenAI will impact education on all fronts, from teacher to student, to pedagogy, approach, and more. Sooner rather than later, educators must adjust their pedagogies and policies in response to this transformative technology, for both their own good and their students’. To that end, this chapter introduces and discusses possibilities and tensions GenAI presents for academic writing and offers a two part lesson for using GenAI for in-class peer review.
Standard Academic English and GenAI
On the surface, GenAI tools like ChatGPT might seem like an easy answer to democratize access to linguistic resources. They have the potential to guide students in navigating Standard Academic English (SAE), especially for socio and linguistically marginalized students who may otherwise face penalties in traditional settings. However, it’s essential to approach the technology with caution. These tools can both empower and marginalize, a conundrum Warschauer et al. (2023) describe as the “rich get richer” contradiction. The digital divide remains real and for many underprivileged students, technological access and savviness remain barriers to entry (e.g., Dobrin, 2023; Horner et al., 2011). This speaks to the need for teachers to teach students how to use GenAI, including how to expertly prompt it to generate a particular kind and quality of content. Warschauer et al. (2023) compare using GenAI to search engines inputs, likening the prompt and results of poorly executed searches to “garbage in, garbage out” (p. 4). Therefore, as we integrate AI into our pedagogical toolkit, it is incumbent upon educators to not only teach students how to effectively utilize GenAI, but also to foster classroom environments where linguistic differences are celebrated, not just tolerated, and where every student’s voice finds a home.
In this light, tools like ChatGPT raise a critical question: do they merely replicate and perpetuate the dominance of SAE, or can they pave the way for a more inclusive and equitable pedagogical environment? Large Language Models (LLMs) like ChatGPT come with implicit biases which are ingrained from the data they are trained on. When tasked with academic writing, GenAI generates responses through replicating linguistic features characteristic of academic writing based on statistical likelihoods derived from their training data. In short, when prompted to produce or evaluate academic writing, it generates the most statistically “standard” version of academic writing from its training corpora and thus inadvertently reinforces SAE as the norm.
It is not the fault of GenAI, for academic institutions themselves and the peer-reviewed journals they oversee are the are arbiters and gatekeepers who determine what is worthy of being published, and they more or less continue to uphold SAE as the expected norm both locally and globally (e.g., Flowerdew, 2015). These biases, as Canagarajah (2013) notes, perpetuate the primacy of SAE and sideline the rich variety of Englishes that emerge from diverse racial and cultural milieus. This imposition of homogeneity of academic discourse is further complicated by Hyland (2003), who highlights its varied practices across disciplines.
However, the implications extend beyond linguistic practice. Writing is an embodied activity, deeply intertwined with our identities, emotions, motivations, and lived experiences. In his seminal work, Asao Inoue (2015) argues that traditional grading practices can inadvertently perpetuate linguistic racism, where students’ racial and linguistic identities can be marginalized or undervalued. This not only impacts students’ motivation and emotional well-being, but also brings to the fore issues of equity and fairness in academic assessment. To mitigate these challenges, Inoue suggests labor-based grading contracts, focusing on the effort and process of writing rather than strict adherence to normative linguistic standards like SAE.
As Inoue (2015) and Baker-Bell (2020) argue and show, writing is deeply embodied and personal. Traditional grading methods, often predicated on SAE linguistic standards, overlook these personal dimensions, rendering linguistic non-conformity as a deficit which writers may then project onto themselves and internalize. Thus, the emergence of alternative grading strategies, such as contract grading and labor-based grading, offer a more inclusive lens by emphasizing student evolution over fixed standards (Inoue, 2014).
SAE has long been a cornerstone of academic writing conventions, establishing a uniform standard against which student work is often judged. However, scholars such as Canagarajah (1997), Horner et al. (2011), and McIntosh et al. (2017) have argued for a translingual approach, emphasizing the value of multiple Englishes and the rich linguistic diversity they bring to academic discourses. Yet this creates tensions, particularly as students navigate the constraints of disciplinary writing conventions that are generally unaccommodating of linguistic diversity.
If that were not enough, peer review, particularly for first year writing students, involves navigating a complex emotional landscape and may be fraught with uncertainty about how to leave useful feedback (and indeed, about whether one is even capable of providing it). Students may also be skeptical of their peers’ expertise and ability to provide good feedback: some of the most frequent student complaints about peer review feedback are that it is too congratulatory, broad, and not specific and actionable enough to be of much help (Ferris, 2018; Ferris & Hedgcock, 2023). Others report that peer review exacerbates students’ fears and leaves teachers feeling drained after they see their students’ discomfort and rather limited and not particularly helpful comments after a peer review session (Shwetz & Assif, 2023). This is why many scholars, including Ferris and Hedgcock (2023) and Shwetz and Assif (2023) discuss the importance of framing, discussing, and modeling the peer review process, noting that it can help to defuse anxiety around the peer review process, even for students who are well acclimated to peer review.
In fact, this anxiety is not limited to students. Even teachers (especially new ones) face anxiety about providing feedback to their students. They may question their ability to offer constructive, clear and concise explanations and worry about being too directive and inadvertently appropriating their students’ texts (Ferris et al., 2011; Ferris & Hedgcock, 2023).
The emotional neutrality of a machine-based augmented peer review using ChatGPT could mitigate some of the anxiety-inducing elements of a human-to-human peer review, particularly in an academic context where one’s writing quality will be judged and grades are at stake. This could be particularly advantageous for introverted students and those who may feel intimidated by the peer review process, including non-native speakers and those who do not speak standard or mainstream varieties of English. This does not at all mean we should strip the human element out of peer review! The lack of emotional engagement in a ChatGPT aided peer review could also be a limitation because emotions play a crucial role in shaping the learning experience (Turner, 2023). Despite a lack of empirical evidence, it seems reasonable that using ChatGPT for peer review should be approached and taught as a complementary tool rather than a replacement for human interaction.
Taken together, leveraging GenAI alongside the use of alternative grading approaches – or at the least, with attention to the biases inherent in SAE – may help to reveal and allow us to decenter some of the SAE expectations of academia even as it perpetuates and reinforces those standards. LLMs like ChatGPT offer a potential solution to some of these challenges by assisting students in refining their writing or offering suggestions that align more closely with SAE, potentially leveling the playing field for socio and linguistically marginalized students. Conversely, it can draw students’ attention to linguistic and structural features of SAE so that they can make more informed decisions regarding the extent to which they choose to conform (or not) to SAE expectations.
Of course, we cannot blindly adopt these technologies as there may be insidious and powerful repercussions if they are used and accepted without caution and consideration. Thus, educators need to facilitate discussions on linguistic diversity in order to promote inclusive classroom environments, perhaps ideally through exploring alternative assessment methods that value and honor students’ varied linguistic backgrounds. It’s crucial to recognize that not all students have equal access to or familiarity with such technologies, which can further exacerbate disparities. That is why it is so important to teach students how to wield this potentially transformative technology with expertise. With that in mind, the final section of this chapter offers an approach and lesson plans to introduce ChatGPT into the classroom for in-class peer review.
Peer Review, GenAI, and Bean’s Hierarchy for Error Correction
The introduction of GenAI has profound implications for education, particularly those of ethics regarding labor and source attribution. For the purposes of doing peer review, this chapter assumes that students have produced their own writing or have appropriately attributed GenAI in their writing. This ameliorates many (but certainly not all!) ethical concerns with using AI in the classroom.
The appendices include Bean’s hierarchy for error correction (Appendix 1) and two sequential lessons: first, to introduce students to peer review augmented by ChatGPT (Appendix 2) and second, a lesson for conducting the actual peer review (Appendix 3). There are also language suggestions for how students can prompt the AI during the peer review (Appendix 4) and finally, there is a reflective writing prompt for after the peer review (Appendix 5).
The lessons approach peer review through Bean’s hierarchy for error correction (2011; see Appendix 1) to help structure and modulate an approach to editing and revising that places emphasis on higher order concerns over lower ones. Higher order concerns are foundational elements of a text like the main ideas, purpose, and overall organization while lower order concerns are generally more local in nature, such as grammar or spelling. Bean’s hierarchy categorizes errors into levels on a continuum from higher to lower:
- Higher Order Concerns (HOCs): These relate to fulfillment of the assignment itself, the paper’s main ideas, thesis, organization, and development.
- Middle Order Concerns (MOCs): Here, the focus is on issues like paragraph development, topic sentences, and clarity of ideas.
- Lower Order Concerns (LOCs): These are sentence-level errors, including grammar, punctuation, spelling, and syntax.
The idea is to address HOCs first, MOCs next, and finally, LOCs, ensuring that the author’s foundation is solid before digging into the nitty-gritty details. For a useful illustration of Bean’s hierarchy, see Appendix 1, taken directly from Concordia University.
The two lessons are designed to help students leverage GenAI (specifically ChatGPT 3.5, which is free and open access) during peer review using a generic “argument paper”, as it is one of the common “mutt genres” assigned to students in first year composition (Wardle, 2009). However, the lessons can be adapted to fit most, if not all, genres of writing assigned in FYC and for peer review in other courses.
The first lesson (Appendix 2) aims to introduce ChatGPT as a tool to augment traditional peer review methods. Students are taught how to use ChatGPT interfaces and apply tailored prompts based on Bean’s hierarchy to get feedback. The second lesson (Appendix 3) applies these newly acquired skills in a ChatGPT-assisted peer review session. By the end of these two sessions, students will not only be familiar with GenAI’s potential in reviewing and fine-tuning academic writing but will also be better equipped to offer constructive, nuanced feedback on their peers’ drafts. Critically, they will also get experience comparing their own thoughts and responses to what ChatGPT offers and reflecting on the process of using the technology (see Appendix 5).
How to Process and Share ChatGPT’s Feedback – Framing for the Classroom
When students offer feedback to peers, stress the importance of combining both human insights and ChatGPT’s analytical prowess – make it clear that while ChatGPT offers speed and consistency, it’s not a replacement for human intuition and understanding. Feedback should be specific, actionable, constructive, and kind. Encourage or even require students to not only identify issues but also offer solutions.
To help emphasize the subjectivity of writing and the possibility that what appears to be an error could be a stylistic choice, ask students to make note of instances where they disagree with the GenAI. Come prepared to class with your own examples to share. This is an excellent opportunity to discuss features of SAE as they relate to linguistic justice and notions of “right” and “wrong” in disciplinary academic writing.
Beyond First Year Writing: AI in Other Disciplines
While this chapter focuses on enhancing the peer-review process within first-year writing courses, the utility of GenAI extends to myriad academic disciplines. Consider the following examples:
Sciences: AI can assist in evaluating lab reports by scrutinizing the clarity and completeness of the hypothesis, methodology, and results sections. It can check for logical consistency, factual accuracy, and whether the conclusion aligns with the data presented.
Mathematics and Computer Science: It could be utilized to assess learners’ problem-solving techniques and the logic of underlying proofs. Gen AI can offer step-by-step feedback, providing students with insights into more efficient solutions to problems, and can even be set to take on a dialogic approach when offering feedback. The AI can flag errors in calculations, offer alternate approaches, and challenge students with extension questions to deepen their understanding.
Social Sciences : It could help evaluate the rigor of research methodologies, assess the validity and reliability of survey instruments and interview protocols, and help in coding qualitative data (including helping with cleaning up transcripts). It could also perform sentiment analysis of qualitative data or perform initial statistical analyses of quantitative data as well as write code for software such as R. Furthermore, the AI system can assist in reviewing academic papers for logical flow, coherence, and citation accuracy, thereby enhancing the quality of scholarly publications in the social sciences.
In general, if there are clearly defined evaluation criteria and the writing belongs to a well-established genre, GenAI can likely provide insightful, consistent, and rapid feedback.
GenAI heralds a transformative era in education. This chapter reviewed some of the relationships and emerging tensions between Large Language Models such as ChatGPT and Standard Academic English, particularly within the context of classroom peer review. This technology’s potential to help students with their academic writing is undeniable and it could even democratize access to linguistic resources. At the same time its use risks erasing and further marginalizing non-standard forms of English. It also heightens the need to pay heed to the digital divide and raises pedagogical and curricular questions about how to best equip and prepare students to use this technology effectively. Educators must approach this technology with care and caution, and foster discussion with students about the contradictory tensions inherent in using ChatGPT for peer review, writing, and more. In particular, students should be taught how to use it effectively and responsibly in a way that balances human insight and diversity with the technology’s capability.
Bean’s systematic approach to error correction offers a framework from which to approach the use of GenAI in peer review that could encourage more targeted, thorough, and effective feedback while also helping students (and the GenAI) keep the focus on fundamental, higher order concerns. GenAI augmented peer review holds great potential for more consistent, in-depth, and rapid peer review that cater to the individual needs of students while also addressing concerns and complaints students have about peer review, ranging from anxiety to feelings that the feedback is unhelpful. However, as we step into this new frontier, it is crucial for both instructors and students to proceed with a mix of enthusiasm and prudence to ensure we remain cognizant of, and appreciate, the rich tapestry of linguistic diversity in our classrooms.
Questions to Guide Reflection and Discussion
- How does generative AI reshape the dynamics of peer review in academic writing?
- Reflect on the ethical considerations involved in using AI for academic research and writing.
- Discuss the potential benefits and drawbacks of integrating AI tools in the peer review process.
- How can educators ensure the maintenance of academic integrity in an era of rapidly advancing AI technology?
- In what ways might AI influence the future of academic scholarship and publication standards?
Baker-Bell, A. (2020). Linguistic justice: Black language, literacy, identity, and pedagogy . Routledge.
Bean, J. (2011). Engaging ideas: The professor’s guide to integrating writing, critical thinking, and active learning in the classroom (2nd ed.). Jossey-Bass.
Canagarajah, S. (2013). Translingual practice: Global Englishes and cosmopolitan relations . Routledge.
Demszky, D., Liu, J., Hill, H. C., Jurafsky, D., & Piech, C. (2023). Can automated feedback improve teachers’ uptake of student ideas? Evidence from a randomized controlled trial in a large-scale online course. Educational Evaluation and Policy Analysis, 0 (0). https://doi.org/10.3102/01623737231169270
Dobrin, S. I. (2023). Talking about generative AI: A guide for educators (Version 1.0) [Ebook]. Creative Commons Attribution-NonCommercial-NoDerivatives 4.0 International License. https://files.broadviewpress.com/sites/uploads/sites/173/2023/05/Talking-about-Generative-AI-Sidney-I.-Dobrin-Version-1.0.pdf
Ferris, D. R. (2018). “They said I have a lot to learn”: How teacher feedback influences advanced university students’ views of writing. Journal of Response to Writing, 4 (2), 4-33. https://scholarsarchive.byu.edu/journalrw/vol4/iss2/2
Ferris, D. R., Brown, J., Liu, H., & Stine, M. E. A. (2011). Responding to L2 students in college writing classes: Teacher perspectives. TESOL Quarterly, 45 (2) , 207-234. https://www.jstor.org/stable/41307629
Ferris, D. R., & Hedgcock, J. S. (2023). Teaching L2 composition: Purpose, process, and practice (4th ed.). Routledge.
Flowerdew, J. (2015). Some thoughts on English for research publication purposes (ERPP) and related issues. Language Teaching, 48 (2), 250–262. https://doi.org/10.1017/S0261444812000523
Hattie, J., & Timperley, H. (2007). The power of feedback. Review of Educational Research , 77 (1), 81-112. https://doi.org/10.3102/003465430298487
Horner, B., Lu, M. Z., Royster, J. J., & Trimbur, J. (2011). Opinion: Language difference in writing: Toward a translingual approach. College English, 73 (3), 303-321.
Hyland, K. (2003). Second language writing . Cambridge University Press.
Inoue, A. B. (2014). Theorizing failure in U.S. writing assessments. Research in the Teaching of English, 48 (3), 330-352. https://www.jstor.org/stable/24398682
Inoue, A. B. (2015). Antiracist writing assessment ecologies: Teaching and assessing writing for a socially just future . WAC Clearinghouse. https://wac.colostate.edu/books/perspectives/inoue/
KPMG. (2023, August 30). Despite popularity, six in 10 students consider generative AI cheating. https://kpmg.com/ca/en/home/media/press-releases/2023/08/six-in-ten-students-consider-generative-ai-cheating.html
Maslej, N., Fattorini, L., Brynjolfsson, E., Etchemendy, J., Ligett, K., Lyons, T., Manyika, J., Ngo, H., Niebles, J. C., Parli, V., Shoham, Y., Wald, R., Clark, J., & Perrault, R. (2023). The AI index 2023 annual report . AI Index Steering Committee, Institute for Human-Centered AI, Stanford University. https://aiindex.stanford.edu/wp-content/uploads/2023/04/HAI_AI-Index-Report_2023.pdf
McIntosh, K., Connor, U., & Gokpinar-Shelton, E. (2017). What intercultural rhetoric can bring to EAP/ESP writing studies in an English as a lingua franca world. Journal of English for Academic Purposes, 29 , 12-20. https://doi.org/10.1016/j.jeap.2017.09.001
Metz, C. (2021, July 20). Can A.I. grade your next test? The New York Times .. https://www.nytimes.com/2021/07/20/technology/ai-education-neural-networks.html
NeJame, L., Bharadwaj, R., Shaw, C., & Fox, K. (2023, April 25). Generative AI in higher education: From fear to experimentation, embracing AI’s potential . Tyton Partners. https://tytonpartners.com/generative-ai-in-higher-education-from-fear-to-experimentation-embracing-ais-potential/
Shwetz, K., & Assif, M. (2023, February 28). Teaching peer feedback: How we can do better. Inside Higher Ed . https://www.insidehighered.com/advice/2023/03/01/student-peer-review-feedback-requires-guidance-and-structure-opinion
Turner, E. (2023). Peer review and the benefits of anxiety in the academic writing classroom. In P. Jackson & C. Weaver (Eds.), Rethinking peer review: Critical reflections on a pedagogical practice (pp. 147-167). WAC Clearinghouse. https://doi.org/10.37514/PER-B.2023.1961.2.07
Wardle, E. (2009). “Mutt genres” and the goal of FYC: Can we help students write the genres of the university? College Composition and Communication, 60 (4), 765-789.
Warschauer, M., Tseng, W., Yim, S., Webster, T., Jacob, S., Du, Q., & Tate, T. (2023). The affordances and contradictions of AI-generated text for writers of English as a second or foreign language. Journal of Second Language Writing , 62 . https://doi.org/10.1016/j.jslw.2023.101071
Wisniewski, B., Zierer, K., & Hattie, J. (2020). The power of feedback revisited: A meta-analysis of educational feedback research. Frontiers in Psychology , 10 . https://doi.org/10.3389/fpsyg.2019.03087
Appendix 1: Bean’s Hierarchy
Credit: Concordia University. Stable link: https://www.cui.edu/Portals/0/uploadedfiles/StudentLife/Writing%20Center/BeanHierarchyChart.pdf
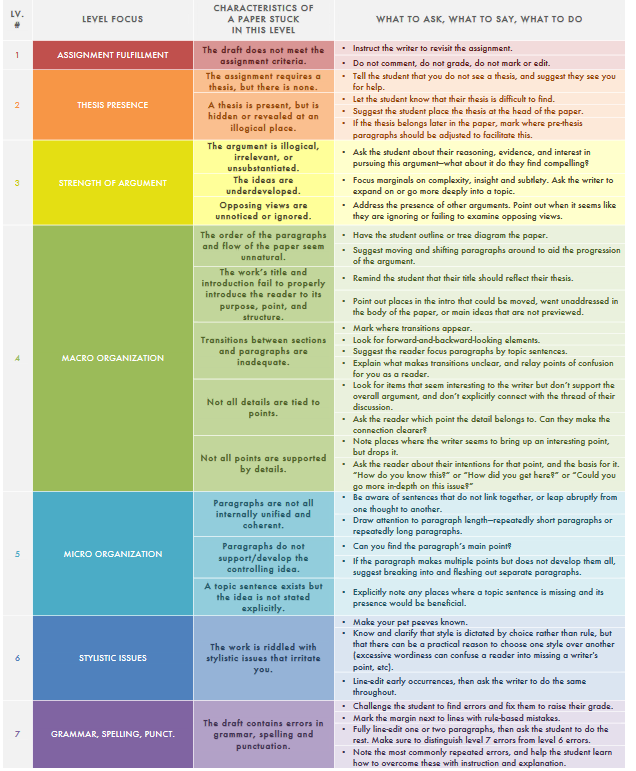
Appendix 2: First Lesson Plan Using Chat GPT for Peer Review
Lesson 1 Objective: Equip first-year composition students with the skills to use AI (specifically ChatGPT) to augment the peer review process of thesis-driven argument papers. By the end of the two sessions, students should be able to effectively incorporate AI feedback into their review process and offer constructive feedback to their peers.
Period 1: Introduction to AI and Preparing for Peer Review (45 minutes)
Materials Needed:
- Computers with internet access
- GPT interface or platform access
- Copies of Bean’s hierarchy for reference
- Prompts for ChatGPT (optional; see Appendix 4)
- Sample argument papers
- Peer review guidelines handout
Introduction (10 minutes)
- Discussion of Traditional Peer Review (5 minutes) : Discuss the benefits and challenges of the traditional peer review process. Elicit students’ prior knowledge and experiences.
- Introduction to AI and GPT (5 minutes) : Briefly explain what ChatGPT is and how it can be a complementary tool for peer review such as speed and lowering anxiety.
Instruction (20 minutes)
- Demonstration with GPT (10 minutes) : Using a sample argument essay, demonstrate how to input text into GPT and how to use specific prompts tailored to Bean’s hierarchy.
- Guided Practice (10 minutes) : In pairs, students practice inputting another sample essay into GPT and using Bean’s hierarchy prompts. They should jot down GPT’s feedback with the aim of sharing it with their peer.
Preparation for Peer Review (15 minutes)
- Overview of Peer Review Process (5 minutes) : Explain the process they will follow during the next class. Emphasize the importance of specific, constructive, and actionable feedback.
- Peer Review Guidelines (5 minutes) : Discuss guidelines, emphasizing what to look for and how to offer feedback (the particulars are up to the instructor here).
- Assignment (5 minutes) : Instruct students to bring two printed copies of their argument essay drafts to the next class for the peer review session. One copy will be for their peer, and the other will be for them to reference while receiving feedback.
Appendix 3: Second Lesson Plan Using Chat GPT for Peer Review
Lesson 2 Objective:
Students will integrate both human and AI-generated feedback into the peer review process for argumentative papers. By the end of the session, students should be proficient in using ChatGPT for feedback, able to compare it with their own initial reviews, and prepared to discuss and apply a comprehensive set of revisions to their drafts. The lesson also aims to stimulate critical thinking and reflection on the role of AI in academic writing.
Period 2: GPT-Assisted Peer Review Session (45 minutes)
- Prompts for ChatGPT (optional; see Appendix 2)
- Students’ draft argument papers, writing prompt, and rubric
- Peer review worksheets or digital forms (optional)
Setup (5 minutes)
- Pair up students. If there’s an odd number, create a group of three. Exchange one copy of their drafts with their peer.
Initial Review (10 minutes)
- Students read their peer’s argument paper without GPT, making preliminary notes on strengths, areas of improvement, clarity of argument, etc.
GPT-Assisted Review (20 minutes)
- Input into GPT (5 minutes) : Students input sections of their peer’s essay into GPT along with the assignment prompt and rubric.
- Review with GPT Prompts (10 minutes) : Using prompts and instructions based on Bean’s hierarchy, students take note of the feedback provided by GPT.
- Comparison (5 minutes) : Students briefly compare their initial impressions with GPT’s feedback.
Feedback Discussion (10 minutes)
- In pairs or groups, students discuss the feedback, pointing out areas of agreement and any discrepancies between their review and GPT’s feedback.
Conclusion (Wrap-Up and Homework Assignment – 5 minutes)
- Wrap-Up : Students should have a list of constructive feedback for their argument paper drafts, drawing from both personal insights and GPT’s suggestions.
- Homework 1 : Direct students to revise their drafts based on the feedback they’ve received. Encourage them to consider both human and AI feedback but to use their judgment in making revisions.
- Homework 2 : Assign a reflection essay that encourages students to consider the merits, drawbacks, and their feelings about using GenAI for peer review and/or academic work in general.
Appendix 4: Useful Prompts for ChatGPT
This can be distributed as a handout or projected on the screen, but before doing so I recommend having students look at Bean’s hierarchy and brainstorm questions or tasks they might ask the GenAI to do for each of Bean’s levels following a think-pair-share protocol.
1. Assignment Fulfillment
- Does the essay meet the criteria and requirements outlined in the assignment?
- Point out any sections that seem off-topic or irrelevant to the assignment.
- Are all the required sources or references included and properly cited?
- Which parts of the assignment criteria are strongly met, and which are weakly addressed?
- Highlight the areas that best align with the assignment’s objectives.
2. Thesis Presence
- Identify the thesis statement in this essay.
- Does the main argument of the essay come through clearly?
- Is the thesis statement arguable and not just a statement of fact?
- How effectively does the thesis set the tone for the entire essay?
- Point out any sections that might detract from or dilute the thesis.
3. Strength of Argument
- Analyze the strength and validity of the essay’s main argument.
- Are there enough evidence and examples to support the claims made?
- Highlight any assertions that seem unsupported or weak.
- Do the counterarguments strengthen the essay’s overall position? Why or why not?
- Identify the strongest and weakest points in the argument.
4. Macro Organization
- Analyze the essay’s overall structure and flow.
- Does the essay have a clear introduction, body, and conclusion?
- Highlight any transitions that effectively link the major sections.
- Identify areas where the progression of ideas might seem jumbled or out of place.
- Is there a logical sequence from the problem statement to the solution or conclusion?
5. Micro Organization
- Assess the development and coherence within individual paragraphs.
- Highlight the topic sentence of each paragraph.
- Point out any paragraphs that seem to diverge from their main idea.
- Are there smooth transitions between sentences?
- Identify any paragraph that might benefit from restructuring or reordering of sentences.
6. Stylistic Issues
- Point out any sentences or phrases that seem overly complex or convoluted.
- Are there any clichés, redundancies, or repetitive words/phrases?
- Highlight any passive voice constructions that could be more effective in the active voice.
- Assess the essay for its tone. Is it consistently formal/informal?
- Are there words or phrases that might be too jargony or technical for the intended audience?
- Identify any grammatical or punctuation errors in this essay.
- Highlight any subject-verb agreement mistakes.
- Point out any misuse of tenses.
- Are there any issues with pronoun reference or consistency?
- Highlight any sentences that seem fragmentary or run-on.
Appendix 5: Reflective Writing Prompt – Utilizing AI in Peer Review
In 300-500 words, reflect on your experience using ChatGPT for peer review. Consider some of the following guiding questions and ideas as you reflect and write your reflection:
- Initial Impressions : What were your expectations when you first heard about using GPT for peer review? Were they met or did something surprise you?
- Advantages : What strengths did you observe in GPT’s feedback? Were there moments when you felt that the AI caught something you might have overlooked or gave feedback that felt particularly insightful?
- Disadvantages : Were there instances where GPT’s feedback felt out of place or failed to capture the nuances of the text? Did you encounter moments where human intuition seemed more appropriate than AI analysis?
- Comfort Level : How comfortable were you with trusting an AI tool with providing feedback on writing, which is inherently a very human and personal endeavor? Did you feel the tool was impersonal, or were you relieved by its unbiased nature?
- Style and Personal Touches : Writing, especially in academia, often invites a blend of standard expectations and individual voice. Do you feel that using GPT might push writers too much towards a generic style? Or do you see it as a tool to refine one’s voice within academic guidelines?
- Standard Academic English Concerns : GPT is designed to understand and produce text based on vast data, often conforming to standard academic English. How do you feel about this? Were there moments where it challenged idiomatic or cultural expressions in your writing?
- Broader Implications : Can you think of other areas in academic life where GPT or similar AI tools might be beneficial? Conversely, are there spaces where you feel such tools should not venture?
- Personal Growth and Future Use : How has this experience shaped your view on the integration of AI in academic processes? Will you consider using GPT or similar tools in your future writing endeavors?
Remember, there’s no right or wrong reflection. This exercise is an opportunity to explore your thoughts, feelings, and predictions about the evolving landscape of academia with the introduction of Generative AI tools like ChatGPT.
About the author
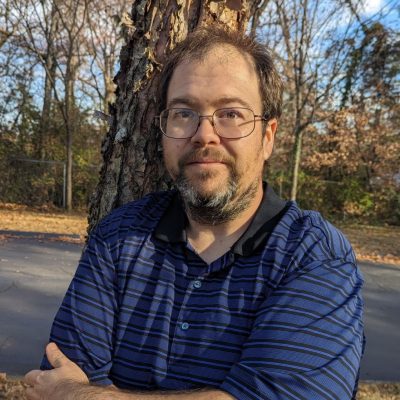
name: Chris Mayer
institution: University of Tennessee, Knoxville
Chris is a graduate student at the University of Tennessee, Knoxville. He is interested in writing pedagogies for both undergraduate and graduate learners.
Teaching and Generative AI Copyright © 2024 by Chris Mayer is licensed under a Creative Commons Attribution-NonCommercial-NoDerivatives 4.0 International License , except where otherwise noted.
BMC Series blog
Peer review week 2023: ai, peer-review, and the future of scientific publishing.
Samuel Brod & Anastasia Widyadari 25 Sep 2023
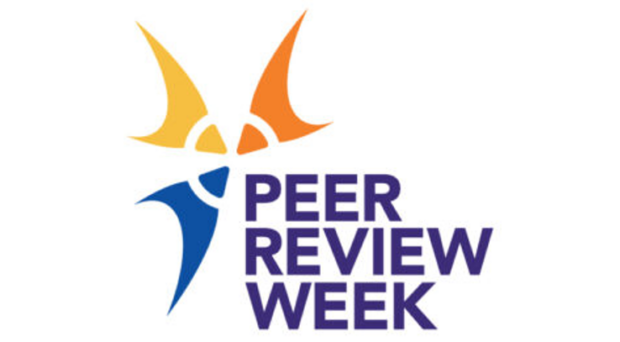
“AI will enhance the future of peer review by using automated systems to analyze and evaluate research articles. It will expedite the review process, improve accuracy, identify biases, and help in handling large volumes of submissions. However, human expertise and judgment will remain crucial in assessing the overall quality and impact of research.”
– ChatGPT-3.5
For many of us, conversations surrounding artificial intelligence (AI) and large language models (LLMs) seem inescapable. For some others, the thought of “let me ask ChatGPT about this” has become routine. Seemingly, AI has filtered into our everyday lives, a scientific innovation with an indiscriminate impact on the way we conduct scientific research. Natural Language Processing (NLP) is a branch of AI that deals with language processing and generation. Under this hierarchy, LLMs are a specific subset of NLP, which draws upon huge training datasets (of typically a billion parameters or more) to generate text, summarize and answer questions. Examples of commonly known LLMs include OpenAI’s ChatGPT-3.5 or -4, Microsoft’s Bing Chat and Google’s Bard.
As described in a recent Nature review, we are already seeing the multifaceted ways AI can be used to aid scientific discovery. From generating hypotheses to designing experiments, we have barely scratched the surface of harnessing this versatile tool. While much of the discussion has covered the potential benefits AI may bring, we are increasingly seeing concerns about the risks AI may carry. Echoing the plot of a dystopian sci-fi, some news outlets have gone so far as to state that this technology may pose an existential threat to humankind . Parallel with the rising use of LLMs, scientific publishing and academia are not absent from this nervous discourse, with much of the discussion focused around the use of AI tools in generating paper publications and peer review reports.
Computer-assistance is not a novel concept in scientific publishing. Automated systems have long been integrated into the process to scan references, inspect manuscripts for plagiarism and check their compliance with journal policies. However, the capacity of AI to expand the volume and type of tasks that can be automated poses several risks that could disrupt the peer review system:
- LLM-generated ‘peer review’ reports are at risk of error: its ability to conflate different sources of information can lead to inaccurate and misleading information (LLM developers refer to this phenomenon as ‘hallucination’).
- Although a powerful tool for processing and summarizing facts about the world, as their output is limited to the training data they are given, LLMs are not yet able to generate original information: this means that the produced reports may not necessarily be as critical as a human reviewer would be and are very rarely as insightful. They will also be subject to any biases inherent in the data they are fed.
- Developers and creators of LLMs are often not very transparent about how their models use and retain the prompts they receive, raising concerns about intellectual property and confidentiality.
- At a time when many academics already feel inundated with invitations to review a manuscript, LLMs are at risk of being misused by reviewers to rapidly generate poor quality, unhelpful reviews, thereby diminishing the value of the peer review process.
Such concerns have led some publishers and funding agencies such as the NIH to ban the use of AI in the peer review process . While these measures are expected and perhaps advisable as we come to better understand how to work with LLMs, we should not ignore their potential to help peer reviewers:
- LLMs have the potential to support reviewers as writing assistants in assembling constructive and easily readable reports
- With more than 90% of indexed scientific articles being published in English, English-speaking researchers are at an advantage in science communication, and the use of LLMs could help democratize peer review by allowing researchers who are not fluent English speakers to communicate science in English more effectively.
- While the risks of bias in LLM persist, the potential biases, judgments and subjectivity inherent in manual reviewing can be minimized by using these tools.
- A significant amount of research requires multidisciplinary scrutiny. LLMs could accelerate the assessment of aspects of papers that require significant time, energy and expertise (such as statistical validity)
- As a complementary tool to one’s own work, LLMs could expand on the existing human reviewers’ report by providing additional insights and context.
Scientific peer review has long been considered the gold standard for ensuring the quality and credibility of research. A way of replicating the research-focused discussions between academics that formed the foundation for the critical yet open-minded approach that defines modern scientific discovery. The use of LLMs in the scientific review process may, to some, feel like the removal of the ‘peer’ in peer review and, as such, a threat to science in its entirety. However, if used as a tool to assist the peer review process, rather than as a standalone “robot” reviewer, LLMs could enhance both the quality and efficiency of manuscript assessment.
Reaching this goal will require a concerted effort from all parties involved in the peer review process. Reviewers who use LLMs to assist with manuscript assessment must take responsibility for the accuracy and value of the reports they submit. Authors can use LLMs to help improve their work but should avoid the trap of assuming these imperfect tools can instantly create a complete manuscript. In turn, publishers must ensure that accountability across the peer review process remains in the hands of experienced human editors. Transparency and honesty about LLMs will be vital in shaping the policies that will help usher in their use.
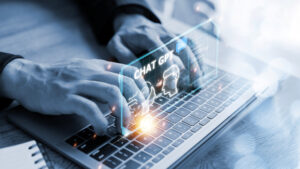
“AI can bring efficiency to peer review by identifying plagiarism, sorting submissions, and assessing the quality of research. However, it might also lead to job loss for human reviewers, lack of nuanced understanding, and the risk of overlooking innovative but unconventional studies. Additionally, reliance on AI may increase the risk of hacking and manipulation of review outcomes.”
– ChatGPT-4.0
The text at the top of the article was generated by ChatGPT3.5 in response to a prompt asking about the future of peer review. The text above, which provides a considerably more cautious perspective on AI, was created by the next iteration of ChatGPT. LLMs are a technology developing at an incredible pace, and opinions on its use are likely to shift just as rapidly. Science should strive to work with, rather than against or without, AI whilst recognizing the complexity of the challenges it proposes. Whatever these developments may bring, it is important that science maintains an attitude of critical open-mindedness and adaptability in the face of new tools and developments, like science always has.
The authors would like to acknowledge ChatGPT-3.5 and ChatGPT-4 for assistance in writing this piece.
View the latest posts on the BMC Series blog homepage
- Latest Posts
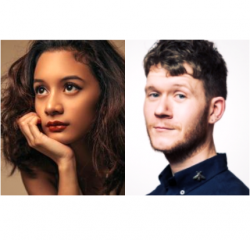
Samuel Brod & Anastasia Widyadari
Latest posts by samuel brod & anastasia widyadari ( see all ).
- Peer review week 2023: AI, peer-review, and the future of scientific publishing - 25th September 2023
Recommended posts
Popular bmc series blog tags.
- BMC Public Health
- BMC Evolutionary Biology
- BMC Ecology
- BMC Pregnancy and Childbirth
- BMC series highlights
- BMC Genomics
- BMC Psychiatry
- public health
- conferences
Popular posts
- Most shared
Sorry. No data so far.
Most Shared Posts
- Introducing BMC Bioinformatics’ Collection: Bioinformatics ethics and data privacy
- Highlights of the BMC Series – March 2024
- Introducing BMC Primary Care’s Collection: Trust and mistrust in primary care
- Introducing BMC Primary Care’s Collection: Diabetes in primary care
- May 2024 (1)
- April 2024 (3)
- March 2024 (2)
- February 2024 (3)
- January 2024 (3)
- December 2023 (6)
- November 2023 (2)
- October 2023 (1)
- September 2023 (7)
- August 2023 (4)
- July 2023 (3)
- June 2023 (3)
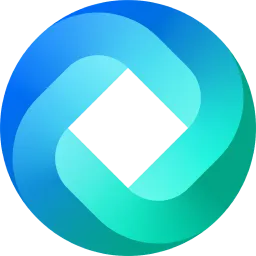
AI Essay Reviewer
Ai-powered essay analysis and feedback.
- Improve academic essays: Get detailed feedback on your essays to enhance your academic writing skills.
- Enhance professional writing: Use the tool to refine your professional writing and communicate more effectively in the workplace.
- Support teaching and learning: Teachers can use the tool to provide comprehensive feedback to students, while students can use it to understand their strengths and areas for improvement.
- Prepare for exams: Use the tool to prepare for exams that require essay writing, such as SAT, ACT, GRE, and more.
New & Trending Tools
Brand architecture and portfolio management tutor, brand identity and positioning tutor, introduction to brand management tutor.
Make your essay memorable to admissions
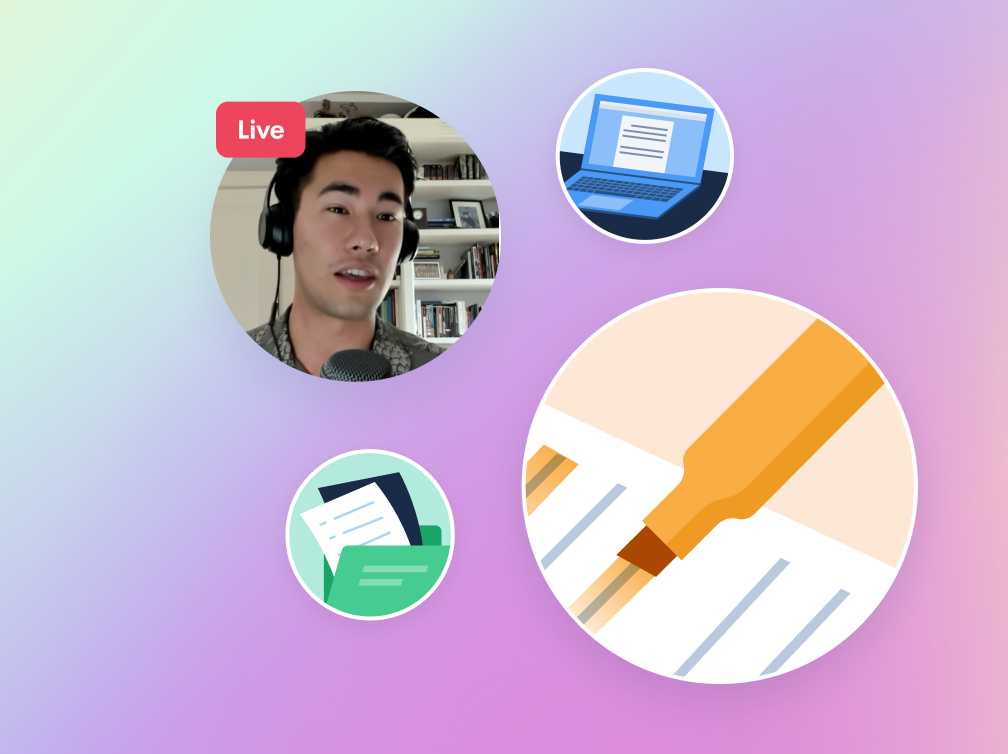
Submit an essay
Choose who you want an essay review from.
Peer review
Peer review community, expert review.
Daniel Berkowitz
Denise Haile
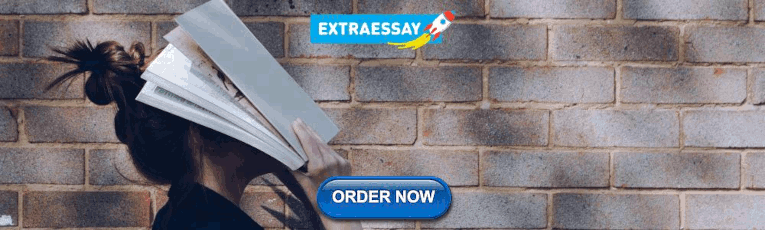
Chinaza Ruth Okonkwo
Craig Aimar
Denise Karp
Robbie Herbst
Christopher Kilner
Elias Miller
Veronica Prout
Sophie Alina
Robert Carlson
Adrian Russian
Subha Subramanian
Lori Zomback
Joseph Recupero
Priya Desai
Emily Kramer
Olga Ivleva
Kevin Dupont
Ethan Glasserman
Stephanie Vitanzo
Samara Watkins
Emily Brother
Gabe Henry Woody
Pascale Bradley
Abby Purfeerst
Shane Niesen
Review options
Specialties, what students are saying, peer review, college essay guy, how it works, differentiating yourself is more important than ever, review a peer essay.
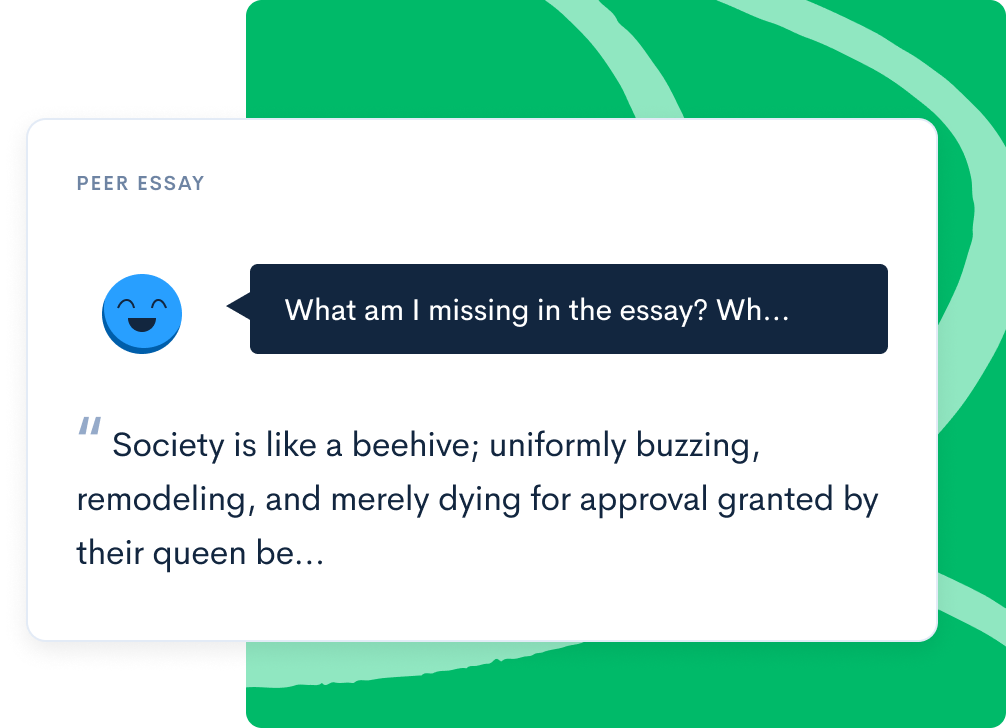

REGISTER INTEREST
EXHIBIT ENQUIRY
Peer Review Process 2024
Understanding the peer review process.
The process by which the Technical Papers Committee performs the peer review and editorial amendment of submitted synopses and complete papers has been arrived at over many years of refinement.
Technical Paper publication is the means by which new work is communicated and peer review is an important part of this process. Peer review is a vital part of the quality control mechanism that is used to determine what is presented/published, and what is not.
Back to Technical Papers Homepage
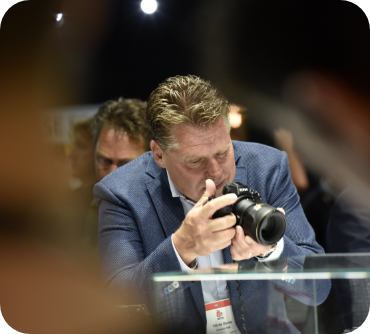
Peer Review Stages
View all stages.
Stage 1 – Synopsis submission
- Call for Papers Opens ( Jan )
- Call for Papers deadline – Synopses submitted ( Feb )
- Synopses Committee Peer Review Meeting ( March )
Stage 2 – Outcome of Stage 1
- Successful stage 1 authors invited to write a technical paper for further peer review for verbal presentation at the IBC conference (speaker slot) ( March )
- Unsuccessful stage 1 authors, where possible, offered an alternative route at IBC; details added to speaker database and sponsorship opportunities ( March )
Stage 3 – Full Paper submission
- Paper authors submit a technical paper for review ( May )
- Technical Paper Committee Peer Review Meeting ( June )
Stage 4 – Outcome of Stage 3
- Successful Stage 3 authors with straight paper accept are invited to present verbally at the IBC Conference and/or have their paper published on IBC365 ( June )
- Successful Stage 3 authors with a paper accepted with changes are requested to make the necessary improvements to their paper and resubmit ( June-July ) Authors are informed of their outcome and are invited to speak at IBC Conference and/or have their paper published in the Conference Proceedings.
- Unsuccessful Stage 3 authors informed their paper has not been accepted, and where possible, offered an alternative route at IBC ( June )
Stage 5 – Presentation at IBC
- Successful authors that been invited to present their technical paper at IBC are guided through the process and format of speaking slot ( July )
- IBC2024 Conference ( Sept )
Thank you for visiting nature.com. You are using a browser version with limited support for CSS. To obtain the best experience, we recommend you use a more up to date browser (or turn off compatibility mode in Internet Explorer). In the meantime, to ensure continued support, we are displaying the site without styles and JavaScript.
- View all journals
- Explore content
- About the journal
- Publish with us
- Sign up for alerts
- NEWS Q&A
- 01 September 2022
The researchers using AI to analyse peer review
- Richard Van Noorden
You can also search for this author in PubMed Google Scholar
Do more-highly cited journals have higher-quality peer review? Reviews are generally confidential and the definition of ‘quality’ is elusive, so this is a difficult question to answer. But researchers who used machine learning to study 10,000 peer-review reports in biomedical journals have tried. They invented proxy measures for quality, which they term thoroughness and helpfulness.
Access options
Access Nature and 54 other Nature Portfolio journals
Get Nature+, our best-value online-access subscription
24,99 € / 30 days
cancel any time
Subscribe to this journal
Receive 51 print issues and online access
185,98 € per year
only 3,65 € per issue
Rent or buy this article
Prices vary by article type
Prices may be subject to local taxes which are calculated during checkout
Nature 609 , 455 (2022)
doi: https://doi.org/10.1038/d41586-022-02787-5
This interview has been edited for length and clarity.
Severin, A. et al. Preprint at https://arxiv.org/abs/2207.09821 (2022).
van Rooyen, S., Black, N. & Godlee, F. J. Clin. Epidemiol. 52 , 625–629 (1999).
Article PubMed Google Scholar
Superchi, C. et al. BMJ Open 10 , e035604 (2020).
Buljan, I., Garcia-Costa, D., Grimaldo, F., Squazzoni, F. & Marušić, A. eLife 9 , e53249 (2020).
Squazzoni, F. et al. Sci. Adv. 7 , eabd0299 (2021).
Eve, M. P. et al. Reading Peer Review (Cambridge Univ. Press, 2021).
Google Scholar
Download references
Related Articles
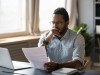
- Peer review
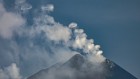
Mount Etna’s spectacular smoke rings and more — April’s best science images
News 03 MAY 24
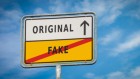
Plagiarism in peer-review reports could be the ‘tip of the iceberg’
Nature Index 01 MAY 24
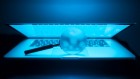
How reliable is this research? Tool flags papers discussed on PubPeer
News 29 APR 24
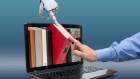
Algorithm ranks peer reviewers by reputation — but critics warn of bias
Nature Index 25 APR 24
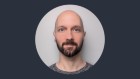
Structure peer review to make it more robust
World View 16 APR 24
Young talents in the fields of natural science and engineering technology
Apply for the 2024 Science Fund Program for Distinguished Young Scholars of the National Natural Science Foundation of China (Overseas).
Shenyang, Liaoning, China
Northeastern University, China
Calling for Application! Tsinghua Shenzhen International Graduate School Global Recruitment
To reshape graduate education as well as research and development to better serve local, national, regional, and global sustainable development.
Shenzhen, Guangdong, China
Tsinghua Shenzhen International Graduate School
Chief Editor, Physical Review X
The Chief Editor of PRX, you will build on this reputation and shape the journal’s scope and direction for the future.
United States (US) - Remote
American Physical Society
Assistant/Associate Professor, New York University Grossman School of Medicine
The Department of Biochemistry and Molecular Pharmacology at the NYUGSoM in Manhattan invite applications for tenure-track positions.
New York (US)
NYU Langone Health
Deputy Director. OSP
The NIH Office of Science Policy (OSP) is seeking an expert candidate to be its next Deputy Director. OSP is the agency’s central policy office an...
Bethesda, Maryland (US)
National Institutes of Health/Office of Science Policy (OSP)
Sign up for the Nature Briefing newsletter — what matters in science, free to your inbox daily.
Quick links
- Explore articles by subject
- Guide to authors
- Editorial policies
- Contributors
- Mission and Values
- Submissions
- The Regulatory Review In Depth
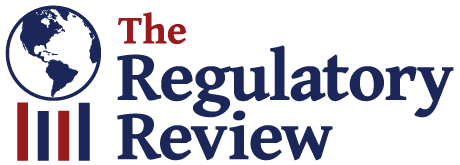
Is AI-Facilitated Gender-Based Violence the Next Pandemic?
Rangita de silva de alwis and elodie vialle.
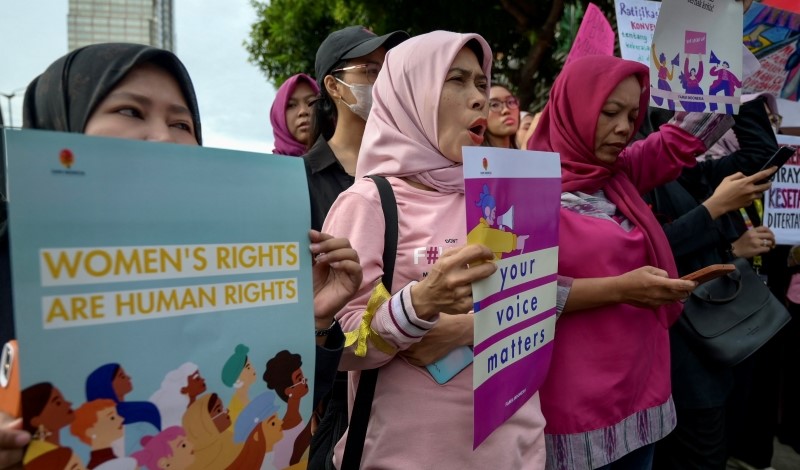
The rise of deep fakes and other AI-generated misinformation presents a direct threat to women’s freedom.
The rise of gender-based online violence amounts to a direct attack on women’s freedom of expression around the world, especially the freedom of women journalists and human rights defenders. The consequences? They include de-platforming women’s voices, undermining equal access to the digital public space, and creating a chilling effect on democratic deliberations with disproportionate impact on women journalists and women human rights defenders.
This year, with over 64 elections globally, it has never been so easy to produce false videos, audio, and text through content that deep-learning AI has generated and synthesized. During the last Slovak parliamentary election campaign, for example, deepfakes were used for the first time, spreading a fake video featuring journalist Monika Tódová and party chairman Michal Šimečka . Despite being fabricated, the video still reached thousands of social media users just two days before the election. This use of artificial intelligence technology to discredit a journalist and undermine election integrity—the first such instance in the European Union—offered a worrying example of the future use of so-called deepfakes.
If deepfakes pose a direct threat to information integrity, they also undermine women’s voices. The virality of deepfake images of Taylor Swift, seen over 45 million times on social media last January, revealed the potentially huge impact of new technology on women’s online safety and integrity. One study has found that 98 percent of deepfake videos online are pornographic and that 99 percent of those targeted are women or girls.
In addition, 73 percent of women journalists have faced online harassment, according to a 2020 UNESCO report . Among those targeted, 20 percent experienced online attacks in direct connection with their online harassment. Gender-based disinformation is a key component of these attacks aimed at discrediting these journalists, and such content often goes viral. MIT researchers concluded that “falsehood diffuses significantly farther, faster, deeper, and more broadly than the truth, in all categories of information, and in many cases by an order of magnitude.” They found specifically that falsehoods travels six times faster than truths.
Online gender-based violence is the flip side of digital authoritarianism. Indeed, one of the authors of this essay, an expert on the treaty body to the Convention on the Elimination of Discrimination against Women (CEDAW), has argued elsewhere that digitized violence is the “newest category of gender-based violence” and has called upon governments to address coded gender violence, especially in regard to the safety of women human rights defenders and women journalists, including online journalists. This rise of online gender-based violence is deeply intertwined with the rise of digital authoritarianism. Authoritarian anti-democratic states can employ anti-feminist narratives and policy measures to justify the oppression of marginalized groups.
In the Philippines, for example, Nobel Peace Prize winner Maria Ressa has decried online harassment as “ death by a thousand cuts,” stating that nothing had prepared her for the dehumanizing storm of gendered online violence directed at her over a half a decade. At one point, Ressa received more than 90 hate messages per hour on Facebook. Over the last few years, Ressa has focused on the responsibility of social media platforms, which monetize hate speech and misogyny, as documented by She Persisted .
In the face of an explosion of online violence against women, especially in the context of litigation, justice departments, including the U.S. Department of Justice need to address the panoply of digital attacks, including doxing, deepfakes, and other forms of misogynistic abuse that create intimidating tactics in the process of a fair trial.
In the last 10 years, there has been a shift toward better protections for victims of non-consensual pornography. When this problem first arose, those targeted had no legal protections.
So far, legislation has been focused on AI or gender. But it should also explore interconnections between both. The EU AI Act , for example, is the first global AI regulation, while the EU has also separately developed new rules to combat gender-based violence, criminalizing physical violence, as well as psychological, economic and sexual violence against women across the EU, both offline and online. These rules are an important part of a global gender equality strategy approach . But they should also be combined with legislation aimed specifically at AI-created abuses as well.
Tech companies must comply with international human rights standards. In a recent virtual summit on deepfake abuse, civil society organizations strategized responses, starting with the need to agree on a definition of deepfakes. One solution, for instance, would be to consider deepfakes as a violation of consent and to require developers to remove deepfake content from their data training. Search engines and AI developers could also put resources into mitigating the ability for users to access and distribute such content.
The Global Network Initiative , a stakeholder group convening civil society organizations and private tech companies, including Meta and Microsoft, has called for companies to respect and promote freedom of expression and comply with internationally recognized human rights, including the rights set out in the International Convention on Civil and Political Rights (ICCPR). Furthermore, the Initiative has stated that the scope of article 19(3) of the ICCPR must be read within the context of further interpretations issued by international human rights bodies, including the Human Rights Committee and the Special Rapporteur on the promotion and protection of the rights to freedom of opinion and expression.
But the ICCPR alone is not enough to challenge a gendered form of violence online. There is a need to enforce the women’s rights convention—the Convention on the Elimination of Discrimination against Women —together with the ICCPR. Protecting tech whistleblowers is another needed step toward addressing these digital attacks and holding big tech accountable, as stated by the Signals Network .
Online platforms also need to build AI resilience. Mitigating gender-based violence from the outset and implementing safety by design are necessary tools to build digital resilience. PEN America has developed concrete recommendations for social media platforms to mitigate the impact of online abuse without undermining freedom of expression.
Civil society organizations also recommend labeling deepfakes and red-teaming before launching any product. Reporters Without Borders calls on social media platforms to hire more information professionals to supervise during the training phase of large language models. Content generated by large language models in the training phase must be verified by media and information professionals instead of simply being evaluated on the basis of its plausibility. Reinforcement learning through human reviewers who can rate a language model’s output for accuracy, toxicity, or other attributes is another important mitigation tool.
Another solution is to implement crisis mechanisms at scale for journalists and human rights defenders. Today, when journalists and human rights defenders face severe online abuse, they often try to escalate their cases on social media platforms or with their employers or civil society organizations. But these escalation channels rely on personal connections, and recent tech platform staff turnover and reorganization make them unpredictable. Civil society organizations that support women’s rights need more reliable, efficient, timely, and structured escalation channels. Indeed, many of those organizations petitioned the UN for such reforms.
For women journalists, building digital awareness is a priority. Online content from creators such as the Digital Dada Podcast , which raises awareness on digital literacy and gender based violence in Kenya and across East Africa, should be scaled up among the community of journalists worldwide. Journalists could implement training, including on open-source intelligence methods and other specific measures to detect deepfakes or make photos harder to process for deepfake creation. For example, learning how to add noise to images or use filters that make slight edits can also prevent the use of deepfakes.
At the end of the day, policymakers need to raise awareness about the linkages between anti-feminism, democratic backsliding, and digital-authoritarianism. New developments in domestic and international norms must take into consideration these intertwined threats.
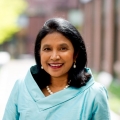
Rangita de Silva de Alwis is a faculty member at the University of Pennsylvania Carey Law School and an elected expert on the treaty body to the UN Convention on the Elimination of Discrimination against Women (CEDAW) .
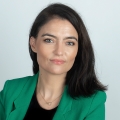
Elodie Vialle is a Senior Advisor to PEN America and a journalist working at the intersection of journalism, technology, and human rights.
Related Essays
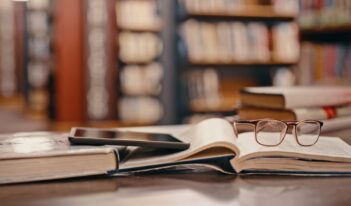
Why Metaphors Matter in AI Law and Policy
Scholar warns that figures of speech play an outsized role in shaping artificial intelligence regulation.
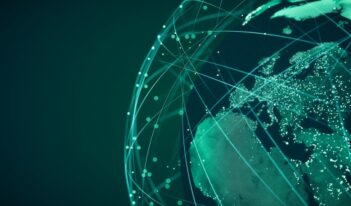
Harnessing AI to Combat Climate Change
At a Penn Program on Regulation workshop, Cass Sunstein explains how AI can help consumers make climate-friendly choices.
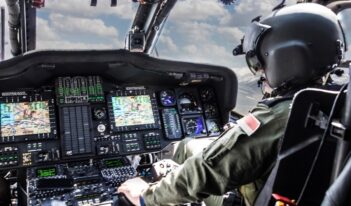
Regulating Wartime Artificial Intelligence
Scholar analyzes potential strategies to regulate wartime use of artificial intelligence.
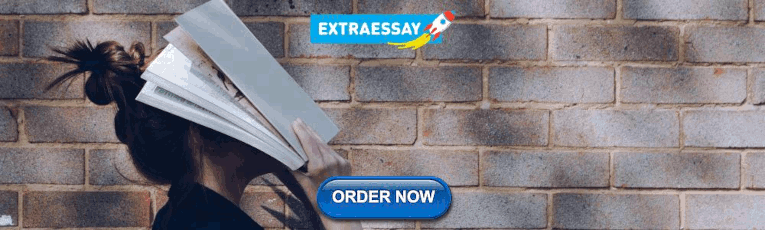
IMAGES
COMMENTS
If that was the case, it would be reasonable to use AI to screen papers before peer review process, using AI as a tool to desk reject papers based on these macroscopic features as part of the pre ...
Experience seamless content evaluation with our AI-powered Peer Review Generator. Improve your writing quality, receive unbiased feedback, and fast-track your path to publication. Harness the power of artificial intelligence to drive your academic and professional success. Try it today and elevate your writing to new heights!
The AI tool analysed the manuscripts using a set of features: the textual content, together with readability scores and formatting measures. Our analysis covers the parts of the quality assurance process of outputs where pre-peer-review screening and peer review itself overlap, covering aspects like formatting, and quality of expression.
For example, a study used 3,341 papers from three computing conferences (the 2018 IEEE Wireless Communications and Networking Conference and the 2018 and 2019 International Conference on Learning Representations) and assessed to what extent AI can predict human peer review decisions about papers (acceptance/rejection or average review score).
Large Language Models (LLMs) and other AI models in Peer Review and Publishing The opportunities and threats of using LLMs and AI in research writing At Sage, we recognize and champion new technology that facilitates conducting, writing and disseminating research. A multitude of tools and technology have been developed in recent years that can increase productivity and aid
Using artificial intelligence (AI) in peer review. At first sight, it might seem that AI and the peer review process have nothing in common. As a researcher, you want your paper to be reviewed as quickly as possible. Peer reviewers are human, and no machine has (yet) come up with the ability to read a paper and decide if it is worth publishing.
Open-access publisher's new artificial intelligence assistant, AIRA, can perform up to 20 recommendations in seconds. In the world of academics, peer review is considered the only credible ...
Students will integrate both human and AI-generated feedback into the peer review process for argumentative papers. By the end of the session, students should be proficient in using ChatGPT for feedback, able to compare it with their own initial reviews, and prepared to discuss and apply a comprehensive set of revisions to their drafts ...
Keywords including "artificial intelligence," "academic writing," and "research" were used to find articles published in English since 2019. This search focused on identifying peer-reviewed articles, review papers, and empirical studies that explored AI's application in academic writing and research.
ARTICLE. AI-assisted peer review. Alessandro Checco 1 , Lorenzo Bracciale2 , Pierpaolo Loreti2, Stephen Pinfield1 & Giuseppe Bianchi2. The scienti fic literature peer review workflow is under ...
An AI tool is designed and trained with 3300 papers from three conferences and the ability of the AI in predicting the review score of a new, unobserved manuscript is tested, showing that such techniques can reveal correlations between the decision process and other quality proxy measures, uncovering potential biases of the review process. The scientific literature peer review workflow is ...
Samuel Brod & Anastasia Widyadari 25 Sep 2023. "AI will enhance the future of peer review by using automated systems to analyze and evaluate research articles. It will expedite the review process, improve accuracy, identify biases, and help in handling large volumes of submissions. However, human expertise and judgment will remain crucial in ...
Such benefits continue to be debated alongside concerns that AI in peer review will simply reinforce existing biases (Checco et al. 2021; Derrick 2018) and the impact of using machine-learning in peer review or to guide research funding continues to be debated (Spezi et al. 2018; Weis and Jacobson 2021). There is some way to go before such ...
Our aim is to manage, fairly, both our editorial approach towards submitted papers that may use AI in research, and the peer-review of AI-informed submissions. In this editorial, we explain the thinking process that lies behind the new JMS policy regarding AI usage in terms of the authoring of papers - of all types - that are submitted to ...
The initial response to using AI in peer review has been guarded. Several journals and academic groups have already explicitly stated that the use of AI should be limited or banned in submissions. The National Institutes of Health banned the use of online generative AI tools like ChatGPT "for analyzing and formulating peer-review critiques.".
AI-powered essay analysis and feedback. HyperWrite's AI Essay Reviewer is an innovative tool that analyzes your essay and provides comprehensive feedback on its structure, grammar, coherence, and relevance. Leveraging the power of AI, it offers an overall rating and detailed feedback on each aspect of your essay, making it an invaluable tool ...
Expert and peer essay review Essay livestreams Get started. 10,000+ Essays reviewed | 25.6 hrs Avg. return time | 4.8/5 Avg.rating. 10,000+ Essays reviewed. 25.6 hrs Avg. return time. ... They *can* make great essays, but advisors relying on them are FAR more likely to give AI-created, recycled, or flat-out plagiarised advice/revisions. Every ...
Artificial intelligence (AI) is the ability of a machine, generally a computer or robot, to perform tasks normally associated with intelligent humans (for an extended discussion of the merits of calling AI 'intelligent', which is well outside the bounds of this perspective; see Searle, 1997). Current discussion mostly centres on forms of ...
Background: In recent years, artificial intelligence (AI) technology has emerged as a promising adjunctive tool for radiologists in detecting Bone metastasis (B ... Preprints available here are not Lancet publications or necessarily under review with a Lancet journal. These preprints are early stage research papers that have not been peer ...
Understanding the Peer Review Process. The process by which the Technical Papers Committee performs the peer review and editorial amendment of submitted synopses and complete papers has been arrived at over many years of refinement. Technical Paper publication is the means by which new work is communicated and peer review is an important part ...
Anna Severin and her team used artificial intelligence to analyse peer-review reports. ... Eve, M. P. et al. Reading Peer Review (Cambridge Univ. Press, 2021). The researchers using AI
fi fi. intelligence (AI) to support reviewing has not been clearly demonstrated. yet, however. Finally, whilst peer review text and scores can theoretically. have value for post-publication research assessment, it is not yet widely. enough available to be a practical evidence source for systematic automation.
A new tool called Writable, which uses ChatGPT to help grade student writing assignments, is being offered widely to teachers in grades 3-12. Why it matters: Teachers have quietly used ChatGPT to grade papers since it first came out — but now schools are sanctioning and encouraging its use. Driving the news: Writable, which is billed as a ...
The Army Health System must reduce the size and weight of contemporary equipment to keep pace with the kinetic, dispersed nature of future conflicts. It can accomplish this while also improving mobility for forward medical units supporting maneuver elements by integrating additive manufacturing technology. Troops watch activity ashore on Omaha ...
Maas defines analogies and metaphors as "communicated framings" that analogize a thing or issue to something else, and in doing so, suggest how to respond to the thing or issue. Such framings are fundamental to human reasoning and policymaking, Maas avers. Maas offers an "atlas" of 55 terms that policymakers and experts, as well as the ...
Rangita de Silva de Alwis and Elodie Vialle. The rise of deep fakes and other AI generated misinformation presents a to direct threat to women's freedom. The rise of gender-based online violence amounts to a direct attack on women's freedom of expression around the world, especially the freedom of women journalists and human rights defenders.