
- E-SUBMISSION
- Aims and scope
- About the journal
- Editorial board
- Best practice
- Open access
- Subscription information
- Journal catalog
- Accepted articles
- Current issue
- Article category
- Most download
- Funded articles
- JPMPH search
- Author index
- Instructions for authors
- E-submission
- Copyright transfer agreement
- Conflict of interest statement
- Article Processing Charge
- Subscription

- 14,160 Views
- 730 Download
- 19 Web of Science
- 24 Crossref
- Author information
- Article notes
- Copyright and License information
1 Department of Preventive Medicine, Ulsan University Hospital, University of Ulsan College of Medicine, Ulsan, Korea
2 Ulsan Metropolitan City Public Health Policy’s Institute, Ulsan, Korea
3 Department of Preventive Medicine, University of Ulsan College of Medicine, Seoul, Korea
Copyright © 2023 The Korean Society for Preventive Medicine
This is an Open Access article distributed under the terms of the Creative Commons Attribution Non-Commercial License (https://creativecommons.org/licenses/by-nc/4.0/) which permits unrestricted non-commercial use, distribution, and reproduction in any medium, provided the original work is properly cited.
- Full Article
- Figure & data

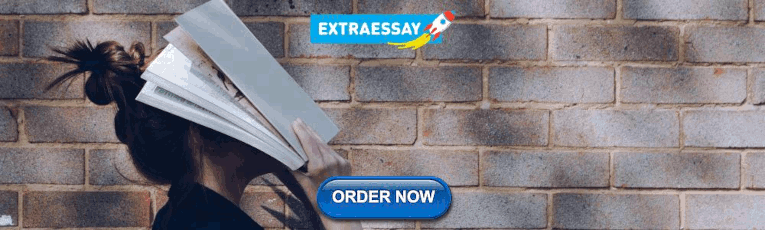
INTRODUCTION
In-depth review of qualitative research methods, validation of qualitative research, acknowledgements.
- Qualitative research methodology has been applied with increasing frequency in various fields, including in healthcare research, where quantitative research methodology has traditionally dominated, with an empirically driven approach involving statistical analysis. Drawing upon artifacts and verbal data collected from in-depth interviews or participatory observations, qualitative research examines the comprehensive experiences of research participants who have experienced salient yet unappreciated phenomena. In this study, we review 6 representative qualitative research methodologies in terms of their characteristics and analysis methods: consensual qualitative research, phenomenological research, qualitative case study, grounded theory, photovoice, and content analysis. We mainly focus on specific aspects of data analysis and the description of results, while also providing a brief overview of each methodology’s philosophical background. Furthermore, since quantitative researchers have criticized qualitative research methodology for its perceived lack of validity, we examine various validation methods of qualitative research. This review article intends to assist researchers in employing an ideal qualitative research methodology and in reviewing and evaluating qualitative research with proper standards and criteria.
- Keywords : Qualitative research , Methodology , Validation
CONFLICT OF INTEREST
The authors have no conflicts of interest associated with the material presented in this paper.
AUTHOR CONTRIBUTIONS
Conceptualization: Ock M. Literature review: Im D, Pyo J, Lee H, Jung H, Ock M. Funding acquisition: None. Writing – original draft: Im D, Pyo J, Lee H, Jung H, Ock M. Writing – review & editing: Im D, Pyo J, Lee H, Jung H, Ock M.
- 1. Chung J, Cho JJ. Use of qualitative research in the field of health. J Korean Acad Fam Med 2008;29(8):553-562. (Korean)
- 2. Pyo J, Lee W, Choi EY, Jang SG, Ock M. Qualitative research in healthcare: necessity and characteristics. J Prev Med Public Health 2023;56(1):12-20 Article PubMed PMC PDF
- 3. Hill CE, Thompson BJ, Williams EN. A guide to conducting consensual qualitative research. Couns Psychol 1997;25(4):517-572 Article PDF
- 4. Hill CE. Consensual qualitative research: a practical resource for investigating social science phenomena. 1st ed. Washington, D.C: American Psychological Association; 2012
- 5. Hill CE, Knox S, Thompson BJ, Williams EN, Hess SA, Ladany N. Consensual qualitative research: an update. J Couns Psychol 2005;52(2):196-205 Article
- 6. Ock M, Han YJ, Choi EY, Pyo J, Lee W. Perceptions of medical students regarding career counseling in Korea: a qualitative study. Int J Environ Res Public Health 2020;17(10):3486 Article PubMed PMC
- 7. Lee K, Lee SH, Park T, Lee JY. Stressors of Korean disaster relief team members during the Nepal Earthquake dispatch: a consensual qualitative research analysis. J Korean Med Sci 2017;32(3):507-513 Article PubMed PMC PDF
- 8. Van Manen M. Researching lived experience: human science for an action sensitive pedagogy. 2nd ed. New York: Routledge; 1997
- 9. Husserl E. Ideas: general introduction to pure phenomenology. 1st ed. London: Routledge; 2012
- 10. Lee N. Phenomenology and qualitative research method. Seoul: Hangilsa; 2014. (Korean)
- 11. Giorgi A. The descriptive phenomenological method in psychology: a modified Husserlian approach. Ann Arbor: Duquesne University Press; 2009
- 12. Edward KL, Welch T. The extension of Colaizzi’s method of phenomenological enquiry. Contemp Nurse 2011;39(2):163-171 Article PubMed
- 13. Colaizzi PF. Psychological research as the phenomenologist views it. In: Valle RS, King M, editors. Existential-phenomenological alternatives for psychology. New York: Oxford University Press; 1978
- 14. Benner P. From novice to expert: excellence and power in clinical nursing practice. Am J Nurs Sci 1984;84(12):1480 Article
- 15. Diekelmann N. Narrative pedagogy: Heideggerian hermeneutical analyses of lived experiences of students, teachers, and clinicians. ANS Adv Nurs Sci 2001;23(3):53-71 Article PubMed
- 16. Diekelmann N, Allen D. A hermeneutic analysis of the NLN criteria for the appraisal of baccalaureate programs. In: Diekelmann N, Allen D, Tanner CA, editors. The NLN criteria for appraisal of baccalaureate programs: a critical hermeneutic analysis. New York: National League for Nursing; 1989. p. 11-34
- 17. Van Kaam A. Existential foundations of psychology. Philos Phenomenol Res 1967;28(1):140-141 Article
- 18. Kim Y, Jung S. Qualitative research methods V: data analysis. Seoul: Academy Press; 2017. (Korean)
- 19. Castro De, Introduction A. to Giorgi’ s existential phenomenological research method. Psicol Caribe 2003;(11):45-56
- 20. Larraya FP. Drinking as a cultural pattern of the Chaco aborigins. Acta Psiquiatr Psicol Am Lat 1976;22(1):21-45. (Spanish) PubMed
- 21. Dhillon AZ, Doran T, Aggarwal VR. Perceptions of waterpipe smoking among young adults: a phenomenological study. Dent J (Basel) 2020;8(4):134 Article PubMed PMC
- 22. Bayked EM, Workneh BD, Kahissay MH. Thirst for information and needs reflections of type 2 diabetes patients receiving insulin treatment in north-east Ethiopia: a qualitative exploration. J Prev Med Public Health 2021;54(2):119-128 Article PubMed PMC PDF
- 23. Erol O, Unsar S, Yacan L, Pelin M, Kurt S, Erdogan B. Pain experiences of patients with advanced cancer: a qualitative descriptive study. Eur J Oncol Nurs 2018;33: 28-34 Article PubMed
- 24. Lane AS, Roberts C. Phenomenological study of medical interns reflecting on their experiences, of open disclosure communication after medication error: linking rationalisation to the conscious competency matrix. BMJ Open 2020;10(5):e035647 Article PubMed PMC
- 25. Kim Y, Lee H. Nurses’ experiences with disclosure of patient safety incidents: a qualitative study. Risk Manag Healthc Policy 2020;13: 453-464 PubMed PMC
- 26. Merriam SB. Qualitative research: a guide to design and implementation. 3rd ed. San Francisco: Jossey-Bass; 2009
- 27. Merriam SB. Qualitative research and case study applications in education. 2nd ed. San Francisco: Jossy-Bass; 1998
- 28. Stake RE. The art of case study research. Thousand Oaks: Sage; 1995
- 29. Yin RK. Discovering the future of the case study: method in evaluation research. Eval Pract 1994;15(3):283-290 Article PDF
- 30. Hays PA. Case study research. In: deMarrais KB, Lapan SD, editors. Foundations for research: methods of inquiry in education and the social sciences. Mahwah: Lawrence Erlbaum; 2004. p. 217-234
- 31. Creswell JW. Qualitative inquiry and research design: choosing among five approaches. Thousand Oaks: Sage Publications; 2007
- 32. Punch KF. Introduction to social research: quantitative and qualitative approaches. 2nd ed. Thousand Oaks: Sage; 2005
- 33. Yin RK. Case study research: design and methods. 4th ed. Thousand Oaks: Sage; 2009
- 34. Marshall DC, Rossman GB. Designing qualitative research. 5th ed. Newbury: Sage; 2011
- 35. Lincoln YS, Guba EG. Naturalistic inquiry. Thousand Oaks: Sage; 1985. p. 120
- 36. Woolston W, Connelly LM. Felty’s syndrome: a qualitative case study. Medsurg Nurs 2017;26(2):105-109. 118 PubMed
- 37. Pyo J, Ock M, Han YJ. Medical litigation experience of the victim of medical accident: a qualitative case study. Int J Qual Stud Health Well-being 2019;14(1):1595958 Article PubMed PMC PDF
- 38. Meyer Y, Pehlke-Milde J, Muntwyler FS, Fleming V. Integrative power in Swiss home-like childbirths: a qualitative multiple case study. Midwifery 2019;78: 97-103 Article PubMed
- 39. Beal CC, Millenbruch J. A qualitative case study of poststroke sexuality in a woman of childbearing age. J Obstet Gynecol Neonatal Nurs 2015;44(2):228-235 Article PubMed
- 40. Yoo K, Jung J, Kim Y, Kim H. Qualitative research methods. 2nd ed. Seoul: Park Young Story; 2018. (Korean)
- 41. Creswell JW. Educational research: planning, conducting, and evaluating quantitative and qualitative research. Upper Saddle River: Pearson Education; 2002
- 42. Morse JM. Situating grounded theory within qualitative inquiry. In: Schreiber RS, Stern PN, editors. Using grounded theory in nursing. New York: Springer; 2001
- 43. Charmaz K. Constructing grounded theory: a practical guide through qualitative analysis. Thousands Oaks: Sage; 2006
- 44. Strauss AL, Corbin JM. Basics of qualitative research: grounded theory procedures and techniques. Newbury Park: Sage; 1990
- 45. Kim JE. Changes in Strauss & Corbin’s grounded theory. J Korean Acad Nurs 2019;49(5):505-514. (Korean) Article PubMed PDF
- 46. Kim IS. Divergence of grounded theory: focused on the differences of Glaser and Strauss. Korean J Soc Welf Stud 2011;42(2):351-379. (Korean) Article
- 47. Choe KS. Grounded theory methodology – Strauss’ version vs Glaserian version-. J Korean Acad Psychiatr Ment Health Nurs 2005;14(1):82-90. (Korean) Article PDF
- 48. Kim B, Kim O. The disease management experience of patients with asthma: grounded theory approach. J Korean Acad Nurs 2020;50(5):714-726. (Korean) Article PubMed PDF
- 49. Choi HG, Yeom HA. Experiences of ego integrity recovery in elderly cancer patients: grounded theory approach. J Korean Acad Nurs 2019;49(3):349-360. (Korean) Article PubMed PDF
- 50. Waldboth V, Patch C, Mahrer-Imhof R, Metcalfe A. The family transition experience when living with childhood neuromuscular disease: a grounded theory study. J Adv Nurs 2021;77(4):1921-1933 Article PubMed PDF
- 51. Herron DL, Priest HM, Read S. Supporting people with an intellectual disability and dementia: a constructivist grounded theory study exploring care providers’ views and experiences in the UK. J Appl Res Intellect Disabil 2020;33(6):1405-1417 Article PubMed PDF
- 52. Wang C, Burris MA. Empowerment through photo novella: portraits of participation. Health Educ Q 1994;21(2):171-186 Article PubMed PDF
- 53. Latz AO, Mulvihill TM. Photovoice research in education and beyond: a practical guide from theory to exhibition. New York: Routledge; 2017
- 54. Wang CC. Youth participation in photovoice as a strategy for community change. J Community Pract 2006;14(1–2):147-161 Article
- 55. Wang C, Burris MA. Photovoice: concept, methodology, and use for participatory needs assessment. Health Educ Behav 1997;24(3):369-387 Article PubMed PDF
- 56. Wang CC. Photovoice: a participatory action research strategy applied to women’s health. J Womens Health 1999;8(2):185-192 Article PubMed
- 57. Graziano KJ. Oppression and resiliency in a post-apartheid South Africa: unheard voices of Black gay men and lesbians. Cultur Divers Ethnic Minor Psychol 2004;10(3):302-316 Article PubMed
- 58. Teti M, Murray C, Johnson L, Binson D. Photovoice as a community-based participatory research method among women living with HIV/AIDS: ethical opportunities and challenges. J Empir Res Hum Res Ethics 2012;7(4):34-43 Article PDF
- 59. Moffitt P, Vollman AR. Photovoice: picturing the health of Aboriginal women in a remote northern community. Can J Nurs Res 2004;36(4):189-201 PubMed
- 60. López ED, Eng E, Randall-David E, Robinson N. Quality-of-life concerns of African American breast cancer survivors within rural North Carolina: blending the techniques of photovoice and grounded theory. Qual Health Res 2005;15(1):99-115 Article PubMed PDF
- 61. Martin Romero MY, Jeitner EC, Francis LA. Visualizing perceived enablers of and barriers to healthy eating by youth in rural El Salvador. J Nutr Educ Behav 2019;51(3):348-356 Article PubMed
- 62. Weber RP. Basic content analysis. Beverly Hills: Sage; 1985
- 63. Choi S, Jeong J, Jung SW. Concept and procedure of qualitative content analysis. J Qual Inq 2016;2(1):127-155. (Korean)
- 64. Lee Y, Kim Y. Qualitative research in education. Seoul, Korea: Gyoyukgwahaksa; 1998. (Korean)
- 65. Riffe D, Lacy S, Fico FG. Analyzing media message: using quantitative content analysis in research. New York: Routledge; 1998
- 66. Krippendorff K. Content analysis: an introduction to its methodology. Beverly Hills: Sage; 2004
- 67. Hsieh HF, Shannon SE. Three approaches to qualitative content analysis. Qual Health Res 2005;15(9):1277-1288 Article PubMed PDF
- 68. Elo S, Kyngäs H. The qualitative content analysis process. J Adv Nurs 2008;62(1):107-115 Article PubMed
- 69. White MD, Marsh EE. Content analysis: a flexible methodology. Libr Trends 2006;55(1):22-45 Article
- 70. Lester P, Smith R. African-American photo coverage in life, Newsweek and Time, 1937–1988. Journal Mass Commun Q 1990;67(1):128-136 Article PDF
- 71. Benedikt T, Marlies B, Susanne G, Nikolaus R, Ewald S, Thomas N. Content analysis of suicide-related online portrayals: changes in contents retrieved with search engines in the United States and Austria from 2013 to 2018. J Affect Disord 2020;271: 300-309 Article PubMed
- 72. Huang X, Lee S, Hu Y, Gao H, O’Connor M. Talking about maternal breast cancer with young children: a content analysis of text in children’s books. J Pediatr Psychol 2015;40(6):609-621 Article PubMed
- 73. Rakic M, Escher M, Elger BS, Eckstein S, Pacurari N, Zwahlen S, et al. Feelings of burden in palliative care: a qualitative analysis of medical records. J Palliat Care 2018;33(1):32-38 Article PubMed PDF
- 74. Golafshani N. Understanding reliability and validity in qualitative research. Qual Rep 2003;8(4):597-607 Article
- 75. Guba EG, Lincoln YS. Fourth generation evaluation as an alternative. Educ Horiz 1985;63(4):139-141
- 76. Lincoln YS, Guba EG. But is it rigorous? Trustworthiness and authenticity in naturalistic evaluation. New Dir Program Eval 1986;(30):73-84 Article
- 77. Creswell JW, Miller DL. Determining validity in qualitative inquiry. Theory Pract 2000;39(3):124-130 Article
- 78. Korstjens I, Moser A. Series: practical guidance to qualitative research. Part 4: trustworthiness and publishing. Eur J Gen Pract 2018;24(1):120-124 Article PubMed PMC PDF
Figure & Data
- Development and validation of a competency evaluation index system for nurse endoscopists with different stages performing endoscopy nursing in China: A modified Delphi study Liangyu Fang, Bingbing Wu, Peipei Wang, Laijuan Chen, Yinchuan Xu Nurse Education Today .2025; 144: 106411. CrossRef
- Surgical De-escalation in Breast Cancer: Qualitative Research Introduces Hope for Patients and Illuminates a Blind Spot Within Blinded Studies Jose G. Bazan, Benjamin W. Corn International Journal of Radiation Oncology*Biology*Physics .2024; 118(2): 455. CrossRef
- Analyzing the prohibition of interfaith marriage in Indonesia: legal, religious, and human rights perspectives M. Thahir Maloko, Sippah Chotban, Muhammad Ikram Nur Fuady, Hasdiwanti Cogent Social Sciences .2024;[Epub] CrossRef
- Intervention in autism based on Early Start Denver Model in a multiethnic immigrant setting—experiences of preschool staff involved in its implementation Petra Linnsand, Gudrun Nygren, Jonas Hermansson, Christopher Gillberg, Emilia Carlsson Frontiers in Child and Adolescent Psychiatry .2024;[Epub] CrossRef
- Role of family medicine physicians in providing nutrition support to older patients admitted to orthopedics departments: a grounded theory approach Ryuichi Ohta, Tachiko Nitta, Akiko Shimizu, Chiaki Sano BMC Primary Care .2024;[Epub] CrossRef
- Diagnosing an overcrowded emergency department from its Electronic Health Records Luca Marzano, Adam S. Darwich, Raghothama Jayanth, Lethvall Sven, Nina Falk, Patrik Bodeby, Sebastiaan Meijer Scientific Reports .2024;[Epub] CrossRef
- Qualitative case study on the disability acceptance experiences of soldiers with disabilities Haneul Lee, Jeehee Pyo, Minsu Ock, Hyun Joo Kim International Journal of Qualitative Studies on Health and Well-being .2024;[Epub] CrossRef
- Qualitative Comparison of Perceptions Regarding Patient Engagement for Patient Safety by Physicians, Nurses, and Patients Seung Gyeong Jang, Minsu Ock, Soyoon Kim Patient Preference and Adherence .2024; Volume 18: 1065. CrossRef
- Coping Mechanisms Utilized by Individuals With Drug Addiction in Overcoming Challenges During the Recovery Process: A Qualitative Meta-synthesis Agus Setiawan, Junaiti Sahar, Budi Santoso, Muchtaruddin Mansyur, Syamikar Baridwan Syamsir Journal of Preventive Medicine and Public Health .2024; 57(3): 197. CrossRef
- Nurses and policymakers role in preparing adolescents with HIV for self-disclosure in Eswatini Baliwe P. Dlamini, Ntombifikile G. Mtshali African Journal of Primary Health Care & Family Medicine .2024;[Epub] CrossRef
- Exploring the economic toxicity experiences and coping styles of patients with colorectal cancer fistula in Jiangsu province, China: a qualitative study Weijing Zhao, Qin Xu, Xia Zhou, Chunmei Liu, Lingyu Ding BMJ Open .2024; 14(6): e082308. CrossRef
- Comprehensive Criteria for Reporting Qualitative Research (CCQR): Reporting Guideline for Global Health Qualitative Research Methods Priyanka Sinha, Binita Paudel, Tamara Mosimann, Hanan Ahmed, Gaotswake Patience Kovane, Miriam Moagi, Angel Phuti International Journal of Environmental Research and Public Health .2024; 21(8): 1005. CrossRef
- Evolution of the roles of family physicians through collaboration with rehabilitation therapists in rural community hospitals: a grounded theory approach Ryuichi Ohta, Kentaro Yoshioka, Chiaki Sano BMC Primary Care .2024;[Epub] CrossRef
- Barreras para la detección de tuberculosis infantil en el departamento del Cauca, Colombia Jhon Edwin Polanco-Pasaje, María Cristina Bolaños, Kelly Yoana Tello-Hoyos, Carlos Hernán Sierra-Torres Revista Facultad Nacional de Salud Pública .2024;[Epub] CrossRef
- Exploratory qualitative study of firearm storage practices among surgeons in the USA: contexts and motivations Shelbie D Waddle, Arielle Thomas, Brendan T Campbell, Marian E Betz, Julie K Johnson, Regina Royan, Alexander Ellyin, Angie Jang, Marie L Crandall, Anne M Stey Injury Prevention .2024; : ip-2023-045205. CrossRef
- What Impact Does the Diagnosis of Mild Cognitive Impairment Have on the Wellbeing, Everyday Behavior, and Healthcare Utilization of People and Their Carers? A Systematic Review Evie Margaret Connolly, Ríona Mc Ardle, Kweku Andrew Ampadu Bimpong, Sarah Slight Journal of Alzheimer's Disease .2024; 101(3): 715. CrossRef
- Traditional treatment for diastasis rectus abdominis in postpartum mothers: a phenomenological study Hemi Fitriani, Setyowati Setyowati, Yati Afiyanti, Raldi Artono Koestoer, Hidayat Arifin British Journal of Midwifery .2024; 32(9): 474. CrossRef
- Impact of Virtual Navigation on the Education and Access of Patients with Cancer: A National Mixed Methods Investigation Susan Haag, Joyce Schaffer SAGE Open Nursing .2024;[Epub] CrossRef
- Author Response: Students’ Positive Coping Strategies From Disaster: A Narrative Analysis Minsu Ock Journal of Preventive Medicine and Public Health .2023; 56(4): 390. CrossRef
- Letter to the Editor: Students’ Positive Coping Strategies From Disaster: A Narrative Analysis Allan M. Abiera, Jan Gresil Kahambing Journal of Preventive Medicine and Public Health .2023; 56(4): 388. CrossRef
- Knowledge, Attitude, and Practice of Qualitative Research Among Health Sciences Faculty Mohamud Mohamud, Alwaleed A Albarkani, Emad Masuadi, Abdullaziz A Alsahly, Abdulaziz I Alkudairy, Yazeed F Shalabi, Abdulrahman Faqih, Khaled A Alaukili, Saad J Alsahli Cureus .2023;[Epub] CrossRef
- Patients’ Reasons to Consider and Their Attitudes toward Complementary and Alternative Medicine Katharina Matjuschenko, Christian Keinki, Jutta Huebner, Pranshu Sahgal European Journal of Cancer Care .2023; 2023: 1. CrossRef
- The human role to guarantee an ethical AI in healthcare: a five-facts approach Raquel Iniesta AI and Ethics .2023;[Epub] CrossRef
- Consumer Perceptions of Home-Based Percussive Massage Therapy for Musculoskeletal Concerns: A Qualitative Analysis (Preprint) Saloni Butala, Pearl Valentine Galido, Benjamin K.P. Woo JMIR Rehabilitation and Assistive Technologies .2023;[Epub] CrossRef

- Qualitative Research in Healthcare: Necessity and Characteristics
Qualitative Research in Healthcare: Data Analysis
Affiliations.
- 1 Department of Preventive Medicine, Ulsan University Hospital, University of Ulsan College of Medicine, Ulsan, Korea.
- 2 Ulsan Metropolitan City Public Health Policy's Institute, Ulsan, Korea.
- 3 Department of Preventive Medicine, University of Ulsan College of Medicine, Seoul, Korea.
- PMID: 37055353
- PMCID: PMC10111102
- DOI: 10.3961/jpmph.22.471
Qualitative research methodology has been applied with increasing frequency in various fields, including in healthcare research, where quantitative research methodology has traditionally dominated, with an empirically driven approach involving statistical analysis. Drawing upon artifacts and verbal data collected from in-depth interviews or participatory observations, qualitative research examines the comprehensive experiences of research participants who have experienced salient yet unappreciated phenomena. In this study, we review 6 representative qualitative research methodologies in terms of their characteristics and analysis methods: consensual qualitative research, phenomenological research, qualitative case study, grounded theory, photovoice, and content analysis. We mainly focus on specific aspects of data analysis and the description of results, while also providing a brief overview of each methodology's philosophical background. Furthermore, since quantitative researchers have criticized qualitative research methodology for its perceived lack of validity, we examine various validation methods of qualitative research. This review article intends to assist researchers in employing an ideal qualitative research methodology and in reviewing and evaluating qualitative research with proper standards and criteria.
Keywords: Methodology; Qualitative research; Validation.
Publication types
- Data Analysis
- Delivery of Health Care*
- Grounded Theory
- Qualitative Research
- Research Design*
- - Google Chrome
Intended for healthcare professionals
- Access provided by Google Indexer
- My email alerts
- BMA member login
- Username * Password * Forgot your log in details? Need to activate BMA Member Log In Log in via OpenAthens Log in via your institution

Search form
- Advanced search
- Search responses
- Search blogs
- Practical thematic...
Practical thematic analysis: a guide for multidisciplinary health services research teams engaging in qualitative analysis
- Related content
- Peer review
- on behalf of the Coproduction Laboratory
- 1 Dartmouth Health, Lebanon, NH, USA
- 2 Dartmouth Institute for Health Policy and Clinical Practice, Geisel School of Medicine at Dartmouth College, Lebanon, NH, USA
- 3 Center for Primary Care and Public Health (Unisanté), Lausanne, Switzerland
- 4 Jönköping Academy for Improvement of Health and Welfare, School of Health and Welfare, Jönköping University, Jönköping, Sweden
- 5 Highland Park, NJ, USA
- 6 Division of Public Health Sciences, Department of Surgery, Washington University School of Medicine, St Louis, MO, USA
- Correspondence to: C H Saunders catherine.hylas.saunders{at}dartmouth.edu
- Accepted 26 April 2023
Qualitative research methods explore and provide deep contextual understanding of real world issues, including people’s beliefs, perspectives, and experiences. Whether through analysis of interviews, focus groups, structured observation, or multimedia data, qualitative methods offer unique insights in applied health services research that other approaches cannot deliver. However, many clinicians and researchers hesitate to use these methods, or might not use them effectively, which can leave relevant areas of inquiry inadequately explored. Thematic analysis is one of the most common and flexible methods to examine qualitative data collected in health services research. This article offers practical thematic analysis as a step-by-step approach to qualitative analysis for health services researchers, with a focus on accessibility for patients, care partners, clinicians, and others new to thematic analysis. Along with detailed instructions covering three steps of reading, coding, and theming, the article includes additional novel and practical guidance on how to draft effective codes, conduct a thematic analysis session, and develop meaningful themes. This approach aims to improve consistency and rigor in thematic analysis, while also making this method more accessible for multidisciplinary research teams.
Through qualitative methods, researchers can provide deep contextual understanding of real world issues, and generate new knowledge to inform hypotheses, theories, research, and clinical care. Approaches to data collection are varied, including interviews, focus groups, structured observation, and analysis of multimedia data, with qualitative research questions aimed at understanding the how and why of human experience. 1 2 Qualitative methods produce unique insights in applied health services research that other approaches cannot deliver. In particular, researchers acknowledge that thematic analysis is a flexible and powerful method of systematically generating robust qualitative research findings by identifying, analysing, and reporting patterns (themes) within data. 3 4 5 6 Although qualitative methods are increasingly valued for answering clinical research questions, many researchers are unsure how to apply them or consider them too time consuming to be useful in responding to practical challenges 7 or pressing situations such as public health emergencies. 8 Consequently, researchers might hesitate to use them, or use them improperly. 9 10 11
Although much has been written about how to perform thematic analysis, practical guidance for non-specialists is sparse. 3 5 6 12 13 In the multidisciplinary field of health services research, qualitative data analysis can confound experienced researchers and novices alike, which can stoke concerns about rigor, particularly for those more familiar with quantitative approaches. 14 Since qualitative methods are an area of specialisation, support from experts is beneficial. However, because non-specialist perspectives can enhance data interpretation and enrich findings, there is a case for making thematic analysis easier, more rapid, and more efficient, 8 particularly for patients, care partners, clinicians, and other stakeholders. A practical guide to thematic analysis might encourage those on the ground to use these methods in their work, unearthing insights that would otherwise remain undiscovered.
Given the need for more accessible qualitative analysis approaches, we present a simple, rigorous, and efficient three step guide for practical thematic analysis. We include new guidance on the mechanics of thematic analysis, including developing codes, constructing meaningful themes, and hosting a thematic analysis session. We also discuss common pitfalls in thematic analysis and how to avoid them.
Summary points
Qualitative methods are increasingly valued in applied health services research, but multidisciplinary research teams often lack accessible step-by-step guidance and might struggle to use these approaches
A newly developed approach, practical thematic analysis, uses three simple steps: reading, coding, and theming
Based on Braun and Clarke’s reflexive thematic analysis, our streamlined yet rigorous approach is designed for multidisciplinary health services research teams, including patients, care partners, and clinicians
This article also provides companion materials including a slide presentation for teaching practical thematic analysis to research teams, a sample thematic analysis session agenda, a theme coproduction template for use during the session, and guidance on using standardised reporting criteria for qualitative research
In their seminal work, Braun and Clarke developed a six phase approach to reflexive thematic analysis. 4 12 We built on their method to develop practical thematic analysis ( box 1 , fig 1 ), which is a simplified and instructive approach that retains the substantive elements of their six phases. Braun and Clarke’s phase 1 (familiarising yourself with the dataset) is represented in our first step of reading. Phase 2 (coding) remains as our second step of coding. Phases 3 (generating initial themes), 4 (developing and reviewing themes), and 5 (refining, defining, and naming themes) are represented in our third step of theming. Phase 6 (writing up) also occurs during this third step of theming, but after a thematic analysis session. 4 12
Key features and applications of practical thematic analysis
Step 1: reading.
All manuscript authors read the data
All manuscript authors write summary memos
Step 2: Coding
Coders perform both data management and early data analysis
Codes are complete thoughts or sentences, not categories
Step 3: Theming
Researchers host a thematic analysis session and share different perspectives
Themes are complete thoughts or sentences, not categories
Applications
For use by practicing clinicians, patients and care partners, students, interdisciplinary teams, and those new to qualitative research
When important insights from healthcare professionals are inaccessible because they do not have qualitative methods training
When time and resources are limited
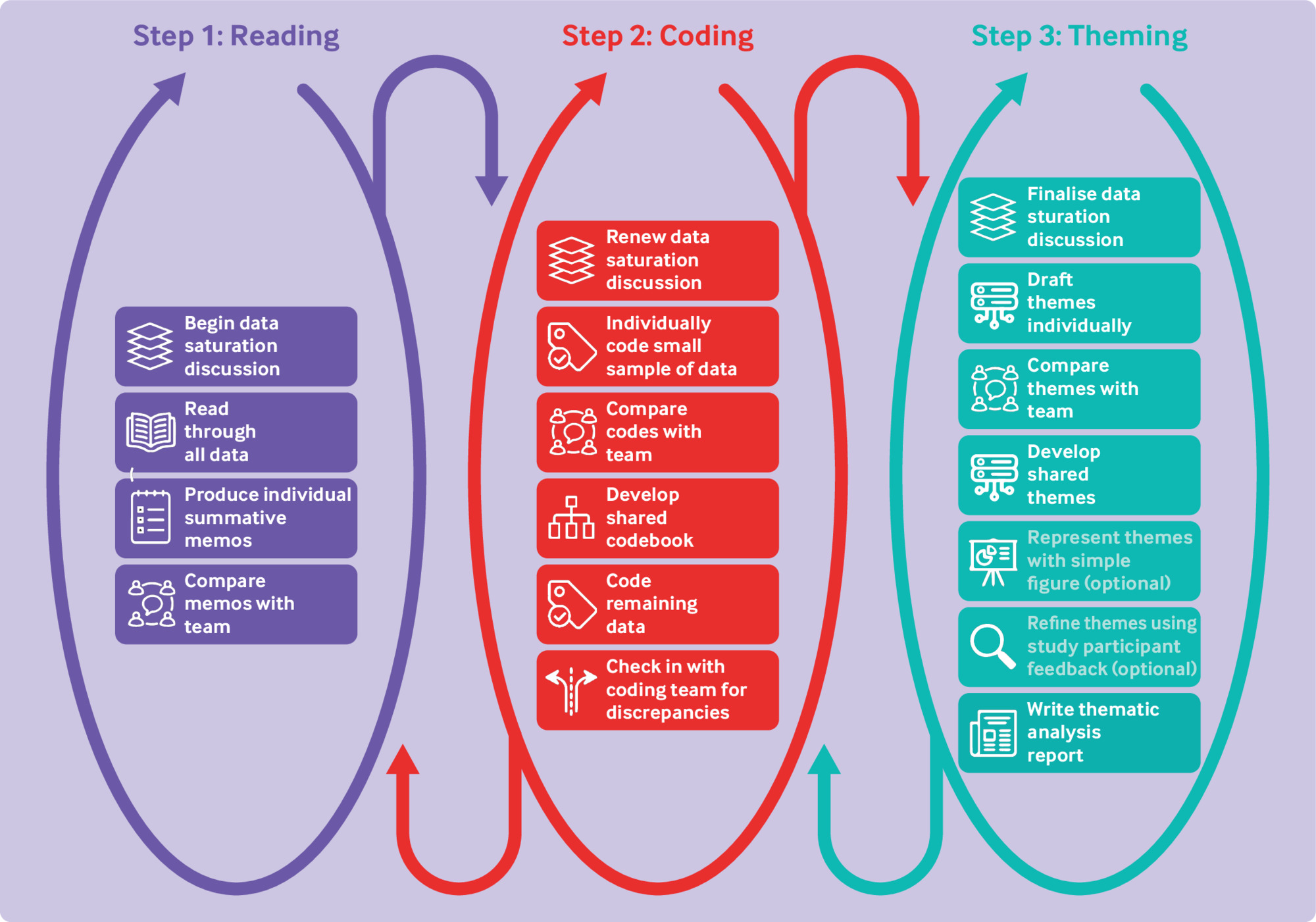
Steps in practical thematic analysis
- Download figure
- Open in new tab
- Download powerpoint
We present linear steps, but as qualitative research is usually iterative, so too is thematic analysis. 15 Qualitative researchers circle back to earlier work to check whether their interpretations still make sense in the light of additional insights, adapting as necessary. While we focus here on the practical application of thematic analysis in health services research, we recognise our approach exists in the context of the broader literature on thematic analysis and the theoretical underpinnings of qualitative methods as a whole. For a more detailed discussion of these theoretical points, as well as other methods widely used in health services research, we recommend reviewing the sources outlined in supplemental material 1. A strong and nuanced understanding of the context and underlying principles of thematic analysis will allow for higher quality research. 16
Practical thematic analysis is a highly flexible approach that can draw out valuable findings and generate new hypotheses, including in cases with a lack of previous research to build on. The approach can also be used with a variety of data, such as transcripts from interviews or focus groups, patient encounter transcripts, professional publications, observational field notes, and online activity logs. Importantly, successful practical thematic analysis is predicated on having high quality data collected with rigorous methods. We do not describe qualitative research design or data collection here. 11 17
In supplemental material 1, we summarise the foundational methods, concepts, and terminology in qualitative research. Along with our guide below, we include a companion slide presentation for teaching practical thematic analysis to research teams in supplemental material 2. We provide a theme coproduction template for teams to use during thematic analysis sessions in supplemental material 3. Our method aligns with the major qualitative reporting frameworks, including the Consolidated Criteria for Reporting Qualitative Research (COREQ). 18 We indicate the corresponding step in practical thematic analysis for each COREQ item in supplemental material 4.
Familiarisation and memoing
We encourage all manuscript authors to review the full dataset (eg, interview transcripts) to familiarise themselves with it. This task is most critical for those who will later be engaged in the coding and theming steps. Although time consuming, it is the best way to involve team members in the intellectual work of data interpretation, so that they can contribute to the analysis and contextualise the results. If this task is not feasible given time limitations or large quantities of data, the data can be divided across team members. In this case, each piece of data should be read by at least two individuals who ideally represent different professional roles or perspectives.
We recommend that researchers reflect on the data and independently write memos, defined as brief notes on thoughts and questions that arise during reading, and a summary of their impressions of the dataset. 2 19 Memoing is an opportunity to gain insights from varying perspectives, particularly from patients, care partners, clinicians, and others. It also gives researchers the opportunity to begin to scope which elements of and concepts in the dataset are relevant to the research question.
Data saturation
The concept of data saturation ( box 2 ) is a foundation of qualitative research. It is defined as the point in analysis at which new data tend to be redundant of data already collected. 21 Qualitative researchers are expected to report their approach to data saturation. 18 Because thematic analysis is iterative, the team should discuss saturation throughout the entire process, beginning with data collection and continuing through all steps of the analysis. 22 During step 1 (reading), team members might discuss data saturation in the context of summary memos. Conversations about saturation continue during step 2 (coding), with confirmation that saturation has been achieved during step 3 (theming). As a rule of thumb, researchers can often achieve saturation in 9-17 interviews or 4-8 focus groups, but this will vary depending on the specific characteristics of the study. 23
Data saturation in context
Braun and Clarke discourage the use of data saturation to determine sample size (eg, number of interviews), because it assumes that there is an objective truth to be captured in the data (sometimes known as a positivist perspective). 20 Qualitative researchers often try to avoid positivist approaches, arguing that there is no one true way of seeing the world, and will instead aim to gather multiple perspectives. 5 Although this theoretical debate with qualitative methods is important, we recognise that a priori estimates of saturation are often needed, particularly for investigators newer to qualitative research who might want a more pragmatic and applied approach. In addition, saturation based, sample size estimation can be particularly helpful in grant proposals. However, researchers should still follow a priori sample size estimation with a discussion to confirm saturation has been achieved.
Definition of coding
We describe codes as labels for concepts in the data that are directly relevant to the study objective. Historically, the purpose of coding was to distil the large amount of data collected into conceptually similar buckets so that researchers could review it in aggregate and identify key themes. 5 24 We advocate for a more analytical approach than is typical with thematic analysis. With our method, coding is both the foundation for and the beginning of thematic analysis—that is, early data analysis, management, and reduction occur simultaneously rather than as different steps. This approach moves the team more efficiently towards being able to describe themes.
Building the coding team
Coders are the research team members who directly assign codes to the data, reading all material and systematically labelling relevant data with appropriate codes. Ideally, at least two researchers would code every discrete data document, such as one interview transcript. 25 If this task is not possible, individual coders can each code a subset of the data that is carefully selected for key characteristics (sometimes known as purposive selection). 26 When using this approach, we recommend that at least 10% of data be coded by two or more coders to ensure consistency in codebook application. We also recommend coding teams of no more than four to five people, for practical reasons concerning maintaining consistency.
Clinicians, patients, and care partners bring unique perspectives to coding and enrich the analytical process. 27 Therefore, we recommend choosing coders with a mix of relevant experiences so that they can challenge and contextualise each other’s interpretations based on their own perspectives and opinions ( box 3 ). We recommend including both coders who collected the data and those who are naive to it, if possible, given their different perspectives. We also recommend all coders review the summary memos from the reading step so that key concepts identified by those not involved in coding can be integrated into the analytical process. In practice, this review means coding the memos themselves and discussing them during the code development process. This approach ensures that the team considers a diversity of perspectives.
Coding teams in context
The recommendation to use multiple coders is a departure from Braun and Clarke. 28 29 When the views, experiences, and training of each coder (sometimes known as positionality) 30 are carefully considered, having multiple coders can enhance interpretation and enrich findings. When these perspectives are combined in a team setting, researchers can create shared meaning from the data. Along with the practical consideration of distributing the workload, 31 inclusion of these multiple perspectives increases the overall quality of the analysis by mitigating the impact of any one coder’s perspective. 30
Coding tools
Qualitative analysis software facilitates coding and managing large datasets but does not perform the analytical work. The researchers must perform the analysis themselves. Most programs support queries and collaborative coding by multiple users. 32 Important factors to consider when choosing software can include accessibility, cost, interoperability, the look and feel of code reports, and the ease of colour coding and merging codes. Coders can also use low tech solutions, including highlighters, word processors, or spreadsheets.
Drafting effective codes
To draft effective codes, we recommend that the coders review each document line by line. 33 As they progress, they can assign codes to segments of data representing passages of interest. 34 Coders can also assign multiple codes to the same passage. Consensus among coders on what constitutes a minimum or maximum amount of text for assigning a code is helpful. As a general rule, meaningful segments of text for coding are shorter than one paragraph, but longer than a few words. Coders should keep the study objective in mind when determining which data are relevant ( box 4 ).
Code types in context
Similar to Braun and Clarke’s approach, practical thematic analysis does not specify whether codes are based on what is evident from the data (sometimes known as semantic) or whether they are based on what can be inferred at a deeper level from the data (sometimes known as latent). 4 12 35 It also does not specify whether they are derived from the data (sometimes known as inductive) or determined ahead of time (sometimes known as deductive). 11 35 Instead, it should be noted that health services researchers conducting qualitative studies often adopt all these approaches to coding (sometimes known as hybrid analysis). 3
In practical thematic analysis, codes should be more descriptive than general categorical labels that simply group data with shared characteristics. At a minimum, codes should form a complete (or full) thought. An easy way to conceptualise full thought codes is as complete sentences with subjects and verbs ( table 1 ), although full sentence coding is not always necessary. With full thought codes, researchers think about the data more deeply and capture this insight in the codes. This coding facilitates the entire analytical process and is especially valuable when moving from codes to broader themes. Experienced qualitative researchers often intuitively use full thought or sentence codes, but this practice has not been explicitly articulated as a path to higher quality coding elsewhere in the literature. 6
Example transcript with codes used in practical thematic analysis 36
- View inline
Depending on the nature of the data, codes might either fall into flat categories or be arranged hierarchically. Flat categories are most common when the data deal with topics on the same conceptual level. In other words, one topic is not a subset of another topic. By contrast, hierarchical codes are more appropriate for concepts that naturally fall above or below each other. Hierarchical coding can also be a useful form of data management and might be necessary when working with a large or complex dataset. 5 Codes grouped into these categories can also make it easier to naturally transition into generating themes from the initial codes. 5 These decisions between flat versus hierarchical coding are part of the work of the coding team. In both cases, coders should ensure that their code structures are guided by their research questions.
Developing the codebook
A codebook is a shared document that lists code labels and comprehensive descriptions for each code, as well as examples observed within the data. Good code descriptions are precise and specific so that coders can consistently assign the same codes to relevant data or articulate why another coder would do so. Codebook development is iterative and involves input from the entire coding team. However, as those closest to the data, coders must resist undue influence, real or perceived, from other team members with conflicting opinions—it is important to mitigate the risk that more senior researchers, like principal investigators, exert undue influence on the coders’ perspectives.
In practical thematic analysis, coders begin codebook development by independently coding a small portion of the data, such as two to three transcripts or other units of analysis. Coders then individually produce their initial codebooks. This task will require them to reflect on, organise, and clarify codes. The coders then meet to reconcile the draft codebooks, which can often be difficult, as some coders tend to lump several concepts together while others will split them into more specific codes. Discussing disagreements and negotiating consensus are necessary parts of early data analysis. Once the codebook is relatively stable, we recommend soliciting input on the codes from all manuscript authors. Yet, coders must ultimately be empowered to finalise the details so that they are comfortable working with the codebook across a large quantity of data.
Assigning codes to the data
After developing the codebook, coders will use it to assign codes to the remaining data. While the codebook’s overall structure should remain constant, coders might continue to add codes corresponding to any new concepts observed in the data. If new codes are added, coders should review the data they have already coded and determine whether the new codes apply. Qualitative data analysis software can be useful for editing or merging codes.
We recommend that coders periodically compare their code occurrences ( box 5 ), with more frequent check-ins if substantial disagreements occur. In the event of large discrepancies in the codes assigned, coders should revise the codebook to ensure that code descriptions are sufficiently clear and comprehensive to support coding alignment going forward. Because coding is an iterative process, the team can adjust the codebook as needed. 5 28 29
Quantitative coding in context
Researchers should generally avoid reporting code counts in thematic analysis. However, counts can be a useful proxy in maintaining alignment between coders on key concepts. 26 In practice, therefore, researchers should make sure that all coders working on the same piece of data assign the same codes with a similar pattern and that their memoing and overall assessment of the data are aligned. 37 However, the frequency of a code alone is not an indicator of its importance. It is more important that coders agree on the most salient points in the data; reviewing and discussing summary memos can be helpful here. 5
Researchers might disagree on whether or not to calculate and report inter-rater reliability. We note that quantitative tests for agreement, such as kappa statistics or intraclass correlation coefficients, can be distracting and might not provide meaningful results in qualitative analyses. Similarly, Braun and Clarke argue that expecting perfect alignment on coding is inconsistent with the goal of co-constructing meaning. 28 29 Overall consensus on codes’ salience and contributions to themes is the most important factor.
Definition of themes
Themes are meta-constructs that rise above codes and unite the dataset ( box 6 , fig 2 ). They should be clearly evident, repeated throughout the dataset, and relevant to the research questions. 38 While codes are often explicit descriptions of the content in the dataset, themes are usually more conceptual and knit the codes together. 39 Some researchers hypothesise that theme development is loosely described in the literature because qualitative researchers simply intuit themes during the analytical process. 39 In practical thematic analysis, we offer a concrete process that should make developing meaningful themes straightforward.
Themes in context
According to Braun and Clarke, a theme “captures something important about the data in relation to the research question and represents some level of patterned response or meaning within the data set.” 4 Similarly, Braun and Clarke advise against themes as domain summaries. While different approaches can draw out themes from codes, the process begins by identifying patterns. 28 35 Like Braun and Clarke and others, we recommend that researchers consider the salience of certain themes, their prevalence in the dataset, and their keyness (ie, how relevant the themes are to the overarching research questions). 4 12 34
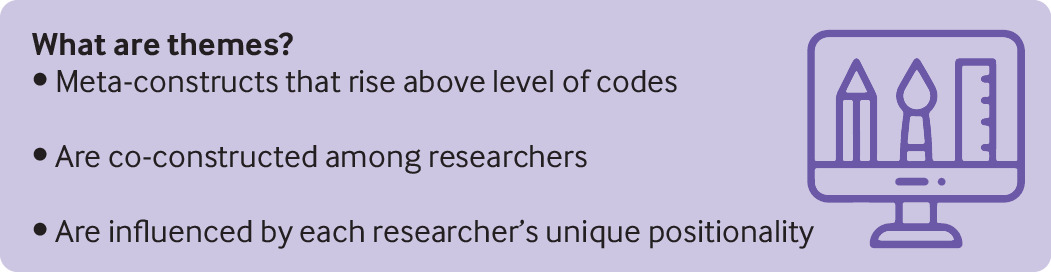
Use of themes in practical thematic analysis
Constructing meaningful themes
After coding all the data, each coder should independently reflect on the team’s summary memos (step 1), the codebook (step 2), and the coded data itself to develop draft themes (step 3). It can be illuminating for coders to review all excerpts associated with each code, so that they derive themes directly from the data. Researchers should remain focused on the research question during this step, so that themes have a clear relation with the overall project aim. Use of qualitative analysis software will make it easy to view each segment of data tagged with each code. Themes might neatly correspond to groups of codes. Or—more likely—they will unite codes and data in unexpected ways. A whiteboard or presentation slides might be helpful to organise, craft, and revise themes. We also provide a template for coproducing themes (supplemental material 3). As with codebook justification, team members will ideally produce individual drafts of the themes that they have identified in the data. They can then discuss these with the group and reach alignment or consensus on the final themes.
The team should ensure that all themes are salient, meaning that they are: supported by the data, relevant to the study objectives, and important. Similar to codes, themes are framed as complete thoughts or sentences, not categories. While codes and themes might appear to be similar to each other, the key distinction is that the themes represent a broader concept. Table 2 shows examples of codes and their corresponding themes from a previously published project that used practical thematic analysis. 36 Identifying three to four key themes that comprise a broader overarching theme is a useful approach. Themes can also have subthemes, if appropriate. 40 41 42 43 44
Example codes with themes in practical thematic analysis 36
Thematic analysis session
After each coder has independently produced draft themes, a carefully selected subset of the manuscript team meets for a thematic analysis session ( table 3 ). The purpose of this session is to discuss and reach alignment or consensus on the final themes. We recommend a session of three to five hours, either in-person or virtually.
Example agenda of thematic analysis session
The composition of the thematic analysis session team is important, as each person’s perspectives will shape the results. This group is usually a small subset of the broader research team, with three to seven individuals. We recommend that primary and senior authors work together to include people with diverse experiences related to the research topic. They should aim for a range of personalities and professional identities, particularly those of clinicians, trainees, patients, and care partners. At a minimum, all coders and primary and senior authors should participate in the thematic analysis session.
The session begins with each coder presenting their draft themes with supporting quotes from the data. 5 Through respectful and collaborative deliberation, the group will develop a shared set of final themes.
One team member facilitates the session. A firm, confident, and consistent facilitation style with good listening skills is critical. For practical reasons, this person is not usually one of the primary coders. Hierarchies in teams cannot be entirely flattened, but acknowledging them and appointing an external facilitator can reduce their impact. The facilitator can ensure that all voices are heard. For example, they might ask for perspectives from patient partners or more junior researchers, and follow up on comments from senior researchers to say, “We have heard your perspective and it is important; we want to make sure all perspectives in the room are equally considered.” Or, “I hear [senior person] is offering [x] idea, I’d like to hear other perspectives in the room.” The role of the facilitator is critical in the thematic analysis session. The facilitator might also privately discuss with more senior researchers, such as principal investigators and senior authors, the importance of being aware of their influence over others and respecting and eliciting the perspectives of more junior researchers, such as patients, care partners, and students.
To our knowledge, this discrete thematic analysis session is a novel contribution of practical thematic analysis. It helps efficiently incorporate diverse perspectives using the session agenda and theme coproduction template (supplemental material 3) and makes the process of constructing themes transparent to the entire research team.
Writing the report
We recommend beginning the results narrative with a summary of all relevant themes emerging from the analysis, followed by a subheading for each theme. Each subsection begins with a brief description of the theme and is illustrated with relevant quotes, which are contextualised and explained. The write-up should not simply be a list, but should contain meaningful analysis and insight from the researchers, including descriptions of how different stakeholders might have experienced a particular situation differently or unexpectedly.
In addition to weaving quotes into the results narrative, quotes can be presented in a table. This strategy is a particularly helpful when submitting to clinical journals with tight word count limitations. Quote tables might also be effective in illustrating areas of agreement and disagreement across stakeholder groups, with columns representing different groups and rows representing each theme or subtheme. Quotes should include an anonymous label for each participant and any relevant characteristics, such as role or gender. The aim is to produce rich descriptions. 5 We recommend against repeating quotations across multiple themes in the report, so as to avoid confusion. The template for coproducing themes (supplemental material 3) allows documentation of quotes supporting each theme, which might also be useful during report writing.
Visual illustrations such as a thematic map or figure of the findings can help communicate themes efficiently. 4 36 42 44 If a figure is not possible, a simple list can suffice. 36 Both must clearly present the main themes with subthemes. Thematic figures can facilitate confirmation that the researchers’ interpretations reflect the study populations’ perspectives (sometimes known as member checking), because authors can invite discussions about the figure and descriptions of findings and supporting quotes. 46 This process can enhance the validity of the results. 46
In supplemental material 4, we provide additional guidance on reporting thematic analysis consistent with COREQ. 18 Commonly used in health services research, COREQ outlines a standardised list of items to be included in qualitative research reports ( box 7 ).
Reporting in context
We note that use of COREQ or any other reporting guidelines does not in itself produce high quality work and should not be used as a substitute for general methodological rigor. Rather, researchers must consider rigor throughout the entire research process. As the issue of how to conceptualise and achieve rigorous qualitative research continues to be debated, 47 48 we encourage researchers to explicitly discuss how they have looked at methodological rigor in their reports. Specifically, we point researchers to Braun and Clarke’s 2021 tool for evaluating thematic analysis manuscripts for publication (“Twenty questions to guide assessment of TA [thematic analysis] research quality”). 16
Avoiding common pitfalls
Awareness of common mistakes can help researchers avoid improper use of qualitative methods. Improper use can, for example, prevent researchers from developing meaningful themes and can risk drawing inappropriate conclusions from the data. Braun and Clarke also warn of poor quality in qualitative research, noting that “coherence and integrity of published research does not always hold.” 16
Weak themes
An important distinction between high and low quality themes is that high quality themes are descriptive and complete thoughts. As such, they often contain subjects and verbs, and can be expressed as full sentences ( table 2 ). Themes that are simply descriptive categories or topics could fail to impart meaningful knowledge beyond categorisation. 16 49 50
Researchers will often move from coding directly to writing up themes, without performing the work of theming or hosting a thematic analysis session. Skipping concerted theming often results in themes that look more like categories than unifying threads across the data.
Unfocused analysis
Because data collection for qualitative research is often semi-structured (eg, interviews, focus groups), not all data will be directly relevant to the research question at hand. To avoid unfocused analysis and a correspondingly unfocused manuscript, we recommend that all team members keep the research objective in front of them at every stage, from reading to coding to theming. During the thematic analysis session, we recommend that the research question be written on a whiteboard so that all team members can refer back to it, and so that the facilitator can ensure that conversations about themes occur in the context of this question. Consistently focusing on the research question can help to ensure that the final report directly answers it, as opposed to the many other interesting insights that might emerge during the qualitative research process. Such insights can be picked up in a secondary analysis if desired.
Inappropriate quantification
Presenting findings quantitatively (eg, “We found 18 instances of participants mentioning safety concerns about the vaccines”) is generally undesirable in practical thematic analysis reporting. 51 Descriptive terms are more appropriate (eg, “participants had substantial concerns about the vaccines,” or “several participants were concerned about this”). This descriptive presentation is critical because qualitative data might not be consistently elicited across participants, meaning that some individuals might share certain information while others do not, simply based on how conversations evolve. Additionally, qualitative research does not aim to draw inferences outside its specific sample. Emphasising numbers in thematic analysis can lead to readers incorrectly generalising the findings. Although peer reviewers unfamiliar with thematic analysis often request this type of quantification, practitioners of practical thematic analysis can confidently defend their decision to avoid it. If quantification is methodologically important, we recommend simultaneously conducting a survey or incorporating standardised interview techniques into the interview guide. 11
Neglecting group dynamics
Researchers should concertedly consider group dynamics in the research team. Particular attention should be paid to power relations and the personality of team members, which can include aspects such as who most often speaks, who defines concepts, and who resolves disagreements that might arise within the group. 52
The perspectives of patient and care partners are particularly important to cultivate. Ideally, patient partners are meaningfully embedded in studies from start to finish, not just for practical thematic analysis. 53 Meaningful engagement can build trust, which makes it easier for patient partners to ask questions, request clarification, and share their perspectives. Professional team members should actively encourage patient partners by emphasising that their expertise is critically important and valued. Noting when a patient partner might be best positioned to offer their perspective can be particularly powerful.
Insufficient time allocation
Researchers must allocate enough time to complete thematic analysis. Working with qualitative data takes time, especially because it is often not a linear process. As the strength of thematic analysis lies in its ability to make use of the rich details and complexities of the data, we recommend careful planning for the time required to read and code each document.
Estimating the necessary time can be challenging. For step 1 (reading), researchers can roughly calculate the time required based on the time needed to read and reflect on one piece of data. For step 2 (coding), the total amount of time needed can be extrapolated from the time needed to code one document during codebook development. We also recommend three to five hours for the thematic analysis session itself, although coders will need to independently develop their draft themes beforehand. Although the time required for practical thematic analysis is variable, teams should be able to estimate their own required effort with these guidelines.
Practical thematic analysis builds on the foundational work of Braun and Clarke. 4 16 We have reframed their six phase process into three condensed steps of reading, coding, and theming. While we have maintained important elements of Braun and Clarke’s reflexive thematic analysis, we believe that practical thematic analysis is conceptually simpler and easier to teach to less experienced researchers and non-researcher stakeholders. For teams with different levels of familiarity with qualitative methods, this approach presents a clear roadmap to the reading, coding, and theming of qualitative data. Our practical thematic analysis approach promotes efficient learning by doing—experiential learning. 12 29 Practical thematic analysis avoids the risk of relying on complex descriptions of methods and theory and places more emphasis on obtaining meaningful insights from those close to real world clinical environments. Although practical thematic analysis can be used to perform intensive theory based analyses, it lends itself more readily to accelerated, pragmatic approaches.
Strengths and limitations
Our approach is designed to smooth the qualitative analysis process and yield high quality themes. Yet, researchers should note that poorly performed analyses will still produce low quality results. Practical thematic analysis is a qualitative analytical approach; it does not look at study design, data collection, or other important elements of qualitative research. It also might not be the right choice for every qualitative research project. We recommend it for applied health services research questions, where diverse perspectives and simplicity might be valuable.
We also urge researchers to improve internal validity through triangulation methods, such as member checking (supplemental material 1). 46 Member checking could include soliciting input on high level themes, theme definitions, and quotations from participants. This approach might increase rigor.
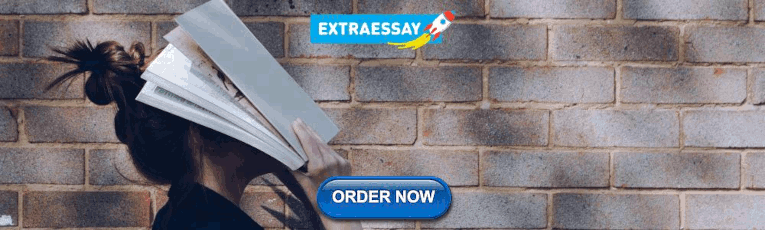
Implications
We hope that by providing clear and simple instructions for practical thematic analysis, a broader range of researchers will be more inclined to use these methods. Increased transparency and familiarity with qualitative approaches can enhance researchers’ ability to both interpret qualitative studies and offer up new findings themselves. In addition, it can have usefulness in training and reporting. A major strength of this approach is to facilitate meaningful inclusion of patient and care partner perspectives, because their lived experiences can be particularly valuable in data interpretation and the resulting findings. 11 30 As clinicians are especially pressed for time, they might also appreciate a practical set of instructions that can be immediately used to leverage their insights and access to patients and clinical settings, and increase the impact of qualitative research through timely results. 8
Practical thematic analysis is a simplified approach to performing thematic analysis in health services research, a field where the experiences of patients, care partners, and clinicians are of inherent interest. We hope that it will be accessible to those individuals new to qualitative methods, including patients, care partners, clinicians, and other health services researchers. We intend to empower multidisciplinary research teams to explore unanswered questions and make new, important, and rigorous contributions to our understanding of important clinical and health systems research.
Acknowledgments
All members of the Coproduction Laboratory provided input that shaped this manuscript during laboratory meetings. We acknowledge advice from Elizabeth Carpenter-Song, an expert in qualitative methods.
Coproduction Laboratory group contributors: Stephanie C Acquilano ( http://orcid.org/0000-0002-1215-5531 ), Julie Doherty ( http://orcid.org/0000-0002-5279-6536 ), Rachel C Forcino ( http://orcid.org/0000-0001-9938-4830 ), Tina Foster ( http://orcid.org/0000-0001-6239-4031 ), Megan Holthoff, Christopher R Jacobs ( http://orcid.org/0000-0001-5324-8657 ), Lisa C Johnson ( http://orcid.org/0000-0001-7448-4931 ), Elaine T Kiriakopoulos, Kathryn Kirkland ( http://orcid.org/0000-0002-9851-926X ), Meredith A MacMartin ( http://orcid.org/0000-0002-6614-6091 ), Emily A Morgan, Eugene Nelson, Elizabeth O’Donnell, Brant Oliver ( http://orcid.org/0000-0002-7399-622X ), Danielle Schubbe ( http://orcid.org/0000-0002-9858-1805 ), Gabrielle Stevens ( http://orcid.org/0000-0001-9001-178X ), Rachael P Thomeer ( http://orcid.org/0000-0002-5974-3840 ).
Contributors: Practical thematic analysis, an approach designed for multidisciplinary health services teams new to qualitative research, was based on CHS’s experiences teaching thematic analysis to clinical teams and students. We have drawn heavily from qualitative methods literature. CHS is the guarantor of the article. CHS, AS, CvP, AMK, JRK, and JAP contributed to drafting the manuscript. AS, JG, CMM, JAP, and RWY provided feedback on their experiences using practical thematic analysis. CvP, LCL, SLB, AVC, GE, and JKL advised on qualitative methods in health services research, given extensive experience. All authors meaningfully edited the manuscript content, including AVC and RKS. The corresponding author attests that all listed authors meet authorship criteria and that no others meeting the criteria have been omitted.
Funding: This manuscript did not receive any specific grant from funding agencies in the public, commercial, or not-for-profit sectors.
Competing interests: All authors have completed the ICMJE uniform disclosure form at https://www.icmje.org/disclosure-of-interest/ and declare: no support from any organisation for the submitted work; no financial relationships with any organisations that might have an interest in the submitted work in the previous three years; no other relationships or activities that could appear to have influenced the submitted work.
Provenance and peer review: Not commissioned; externally peer reviewed.
- Ziebland S ,
- ↵ A Hybrid Approach to Thematic Analysis in Qualitative Research: Using a Practical Example. 2018. https://methods.sagepub.com/case/hybrid-approach-thematic-analysis-qualitative-research-a-practical-example .
- Maguire M ,
- Vindrola-Padros C ,
- Vindrola-Padros B
- ↵ Vindrola-Padros C. Rapid Ethnographies: A Practical Guide . Cambridge University Press 2021. https://play.google.com/store/books/details?id=n80HEAAAQBAJ
- Schroter S ,
- Merino JG ,
- Barbeau A ,
- ↵ Padgett DK. Qualitative and Mixed Methods in Public Health . SAGE Publications 2011. https://play.google.com/store/books/details?id=LcYgAQAAQBAJ
- Scharp KM ,
- Korstjens I
- Barnett-Page E ,
- ↵ Guest G, Namey EE, Mitchell ML. Collecting Qualitative Data: A Field Manual for Applied Research . SAGE 2013. https://play.google.com/store/books/details?id=-3rmWYKtloC
- Sainsbury P ,
- Emerson RM ,
- Saunders B ,
- Kingstone T ,
- Hennink MM ,
- Kaiser BN ,
- Hennink M ,
- O’Connor C ,
- ↵ Yen RW, Schubbe D, Walling L, et al. Patient engagement in the What Matters Most trial: experiences and future implications for research. Poster presented at International Shared Decision Making conference, Quebec City, Canada. July 2019.
- ↵ Got questions about Thematic Analysis? We have prepared some answers to common ones. https://www.thematicanalysis.net/faqs/ (accessed 9 Nov 2022).
- ↵ Braun V, Clarke V. Thematic Analysis. SAGE Publications. 2022. https://uk.sagepub.com/en-gb/eur/thematic-analysis/book248481 .
- Kalpokas N ,
- Radivojevic I
- Campbell KA ,
- Durepos P ,
- ↵ Understanding Thematic Analysis. https://www.thematicanalysis.net/understanding-ta/ .
- Saunders CH ,
- Stevens G ,
- CONFIDENT Study Long-Term Care Partners
- MacQueen K ,
- Vaismoradi M ,
- Turunen H ,
- Schott SL ,
- Berkowitz J ,
- Carpenter-Song EA ,
- Goldwag JL ,
- Durand MA ,
- Goldwag J ,
- Saunders C ,
- Mishra MK ,
- Rodriguez HP ,
- Shortell SM ,
- Verdinelli S ,
- Scagnoli NI
- Campbell C ,
- Sparkes AC ,
- McGannon KR
- Sandelowski M ,
- Connelly LM ,
- O’Malley AJ ,
- Correspondence
- Open access
- Published: 18 September 2013
Using the framework method for the analysis of qualitative data in multi-disciplinary health research
- Nicola K Gale 1 ,
- Gemma Heath 2 ,
- Elaine Cameron 3 ,
- Sabina Rashid 4 &
- Sabi Redwood 2
BMC Medical Research Methodology volume 13 , Article number: 117 ( 2013 ) Cite this article
550k Accesses
5817 Citations
109 Altmetric
Metrics details
The Framework Method is becoming an increasingly popular approach to the management and analysis of qualitative data in health research. However, there is confusion about its potential application and limitations.
The article discusses when it is appropriate to adopt the Framework Method and explains the procedure for using it in multi-disciplinary health research teams, or those that involve clinicians, patients and lay people. The stages of the method are illustrated using examples from a published study.
Used effectively, with the leadership of an experienced qualitative researcher, the Framework Method is a systematic and flexible approach to analysing qualitative data and is appropriate for use in research teams even where not all members have previous experience of conducting qualitative research.
The Framework Method for the management and analysis of qualitative data has been used since the 1980s [ 1 ]. The method originated in large-scale social policy research but is becoming an increasingly popular approach in medical and health research; however, there is some confusion about its potential application and limitations. In this article we discuss when it is appropriate to use the Framework Method and how it compares to other qualitative analysis methods. In particular, we explore how it can be used in multi-disciplinary health research teams. Multi-disciplinary and mixed methods studies are becoming increasingly commonplace in applied health research. As well as disciplines familiar with qualitative research, such as nursing, psychology and sociology, teams often include epidemiologists, health economists, management scientists and others. Furthermore, applied health research often has clinical representation and, increasingly, patient and public involvement [ 2 ]. We argue that while leadership is undoubtedly required from an experienced qualitative methodologist, non-specialists from the wider team can and should be involved in the analysis process. We then present a step-by-step guide to the application of the Framework Method, illustrated using a worked example (See Additional File 1 ) from a published study [ 3 ] to illustrate the main stages of the process. Technical terms are included in the glossary (below). Finally, we discuss the strengths and limitations of the approach.
Glossary of key terms used in the Framework Method
Analytical framework: A set of codes organised into categories that have been jointly developed by researchers involved in analysis that can be used to manage and organise the data. The framework creates a new structure for the data (rather than the full original accounts given by participants) that is helpful to summarize/reduce the data in a way that can support answering the research questions.
Analytic memo: A written investigation of a particular concept, theme or problem, reflecting on emerging issues in the data that captures the analytic process (see Additional file 1 , Section 7).
Categories: During the analysis process, codes are grouped into clusters around similar and interrelated ideas or concepts. Categories and codes are usually arranged in a tree diagram structure in the analytical framework. While categories are closely and explicitly linked to the raw data, developing categories is a way to start the process of abstraction of the data (i.e. towards the general rather than the specific or anecdotal).
Charting: Entering summarized data into the Framework Method matrix (see Additional File 1 , Section 6).
Code: A descriptive or conceptual label that is assigned to excerpts of raw data in a process called ‘coding’ (see Additional File 1 , Section 3).
Data: Qualitative data usually needs to be in textual form before analysis. These texts can either be elicited texts (written specifically for the research, such as food diaries), or extant texts (pre-existing texts, such as meeting minutes, policy documents or weblogs), or can be produced by transcribing interview or focus group data, or creating ‘field’ notes while conducting participant-observation or observing objects or social situations.
Indexing: The systematic application of codes from the agreed analytical framework to the whole dataset (see Additional File 1 , Section 5).
Matrix: A spreadsheet contains numerous cells into which summarized data are entered by codes (columns) and cases (rows) (see Additional File 1 , Section 6).
Themes: Interpretive concepts or propositions that describe or explain aspects of the data, which are the final output of the analysis of the whole dataset. Themes are articulated and developed by interrogating data categories through comparison between and within cases. Usually a number of categories would fall under each theme or sub-theme [ 3 ].
Transcript: A written verbatim (word-for-word) account of a verbal interaction, such as an interview or conversation.
The Framework Method sits within a broad family of analysis methods often termed thematic analysis or qualitative content analysis. These approaches identify commonalities and differences in qualitative data, before focusing on relationships between different parts of the data, thereby seeking to draw descriptive and/or explanatory conclusions clustered around themes. The Framework Method was developed by researchers, Jane Ritchie and Liz Spencer, from the Qualitative Research Unit at the National Centre for Social Research in the United Kingdom in the late 1980s for use in large-scale policy research [ 1 ]. It is now used widely in other areas, including health research [ 3 – 12 ]. Its defining feature is the matrix output: rows (cases), columns (codes) and ‘cells’ of summarised data, providing a structure into which the researcher can systematically reduce the data, in order to analyse it by case and by code [ 1 ]. Most often a ‘case’ is an individual interviewee, but this can be adapted to other units of analysis, such as predefined groups or organisations. While in-depth analyses of key themes can take place across the whole data set, the views of each research participant remain connected to other aspects of their account within the matrix so that the context of the individual’s views is not lost. Comparing and contrasting data is vital to qualitative analysis and the ability to compare with ease data across cases as well as within individual cases is built into the structure and process of the Framework Method.
The Framework Method provides clear steps to follow and produces highly structured outputs of summarised data. It is therefore useful where multiple researchers are working on a project, particularly in multi-disciplinary research teams were not all members have experience of qualitative data analysis, and for managing large data sets where obtaining a holistic, descriptive overview of the entire data set is desirable. However, caution is recommended before selecting the method as it is not a suitable tool for analysing all types of qualitative data or for answering all qualitative research questions, nor is it an ‘easy’ version of qualitative research for quantitative researchers. Importantly, the Framework Method cannot accommodate highly heterogeneous data, i.e. data must cover similar topics or key issues so that it is possible to categorize it. Individual interviewees may, of course, have very different views or experiences in relation to each topic, which can then be compared and contrasted. The Framework Method is most commonly used for the thematic analysis of semi-structured interview transcripts, which is what we focus on in this article, although it could, in principle, be adapted for other types of textual data [ 13 ], including documents, such as meeting minutes or diaries [ 12 ], or field notes from observations [ 10 ].
For quantitative researchers working with qualitative colleagues or when exploring qualitative research for the first time, the nature of the Framework Method is seductive because its methodical processes and ‘spreadsheet’ approach seem more closely aligned to the quantitative paradigm [ 14 ]. Although the Framework Method is a highly systematic method of categorizing and organizing what may seem like unwieldy qualitative data, it is not a panacea for problematic issues commonly associated with qualitative data analysis such as how to make analytic choices and make interpretive strategies visible and auditable. Qualitative research skills are required to appropriately interpret the matrix, and facilitate the generation of descriptions, categories, explanations and typologies. Moreover, reflexivity, rigour and quality are issues that are requisite in the Framework Method just as they are in other qualitative methods. It is therefore essential that studies using the Framework Method for analysis are overseen by an experienced qualitative researcher, though this does not preclude those new to qualitative research from contributing to the analysis as part of a wider research team.
There are a number of approaches to qualitative data analysis, including those that pay close attention to language and how it is being used in social interaction such as discourse analysis [ 15 ] and ethnomethodology [ 16 ]; those that are concerned with experience, meaning and language such as phenomenology [ 17 , 18 ] and narrative methods [ 19 ]; and those that seek to develop theory derived from data through a set of procedures and interconnected stages such as Grounded Theory [ 20 , 21 ]. Many of these approaches are associated with specific disciplines and are underpinned by philosophical ideas which shape the process of analysis [ 22 ]. The Framework Method, however, is not aligned with a particular epistemological, philosophical, or theoretical approach. Rather it is a flexible tool that can be adapted for use with many qualitative approaches that aim to generate themes.
The development of themes is a common feature of qualitative data analysis, involving the systematic search for patterns to generate full descriptions capable of shedding light on the phenomenon under investigation. In particular, many qualitative approaches use the ‘constant comparative method’ , developed as part of Grounded Theory, which involves making systematic comparisons across cases to refine each theme [ 21 , 23 ]. Unlike Grounded Theory, the Framework Method is not necessarily concerned with generating social theory, but can greatly facilitate constant comparative techniques through the review of data across the matrix.
Perhaps because the Framework Method is so obviously systematic, it has often, as other commentators have noted, been conflated with a deductive approach to qualitative analysis [ 13 , 14 ]. However, the tool itself has no allegiance to either inductive or deductive thematic analysis; where the research sits along this inductive-deductive continuum depends on the research question. A question such as, ‘Can patients give an accurate biomedical account of the onset of their cardiovascular disease?’ is essentially a yes/no question (although it may be nuanced by the extent of their account or by appropriate use of terminology) and so requires a deductive approach to both data collection and analysis (e.g. structured or semi-structured interviews and directed qualitative content analysis [ 24 ]). Similarly, a deductive approach may be taken if basing analysis on a pre-existing theory, such as behaviour change theories, for example in the case of a research question such as ‘How does the Theory of Planned Behaviour help explain GP prescribing?’ [ 11 ]. However, a research question such as, ‘How do people construct accounts of the onset of their cardiovascular disease?’ would require a more inductive approach that allows for the unexpected, and permits more socially-located responses [ 25 ] from interviewees that may include matters of cultural beliefs, habits of food preparation, concepts of ‘fate’, or links to other important events in their lives, such as grief, which cannot be predicted by the researcher in advance (e.g. an interviewee-led open ended interview and grounded theory [ 20 ]). In all these cases, it may be appropriate to use the Framework Method to manage the data. The difference would become apparent in how themes are selected: in the deductive approach, themes and codes are pre-selected based on previous literature, previous theories or the specifics of the research question; whereas in the inductive approach, themes are generated from the data though open (unrestricted) coding, followed by refinement of themes. In many cases, a combined approach is appropriate when the project has some specific issues to explore, but also aims to leave space to discover other unexpected aspects of the participants’ experience or the way they assign meaning to phenomena. In sum, the Framework Method can be adapted for use with deductive, inductive, or combined types of qualitative analysis. However, there are some research questions where analysing data by case and theme is not appropriate and so the Framework Method should be avoided. For instance, depending on the research question, life history data might be better analysed using narrative analysis [ 19 ]; recorded consultations between patients and their healthcare practitioners using conversation analysis [ 26 ]; and documentary data, such as resources for pregnant women, using discourse analysis [ 27 ].
It is not within the scope of this paper to consider study design or data collection in any depth, but before moving on to describe the Framework Method analysis process, it is worth taking a step back to consider briefly what needs to happen before analysis begins. The selection of analysis method should have been considered at the proposal stage of the research and should fit with the research questions and overall aims of the study. Many qualitative studies, particularly ones using inductive analysis, are emergent in nature; this can be a challenge and the researchers can only provide an “imaginative rehearsal” of what is to come [ 28 ]. In mixed methods studies, the role of the qualitative component within the wider goals of the project must also be considered. In the data collection stage, resources must be allocated for properly trained researchers to conduct the qualitative interviewing because it is a highly skilled activity. In some cases, a research team may decide that they would like to use lay people, patients or peers to do the interviews [ 29 – 32 ] and in this case they must be properly trained and mentored which requires time and resources. At this early stage it is also useful to consider whether the team will use Computer Assisted Qualitative Data Analysis Software (CAQDAS), which can assist with data management and analysis.
As any form of qualitative or quantitative analysis is not a purely technical process, but influenced by the characteristics of the researchers and their disciplinary paradigms, critical reflection throughout the research process is paramount, including in the design of the study, the construction or collection of data, and the analysis. All members of the team should keep a research diary, where they record reflexive notes, impressions of the data and thoughts about analysis throughout the process. Experienced qualitative researchers become more skilled at sifting through data and analysing it in a rigorous and reflexive way. They cannot be too attached to certainty, but must remain flexible and adaptive throughout the research in order to generate rich and nuanced findings that embrace and explain the complexity of real social life and can be applied to complex social issues. It is important to remember when using the Framework Method that, unlike quantitative research where data collection and data analysis are strictly sequential and mutually exclusive stages of the research process, in qualitative analysis there is, to a greater or lesser extent depending on the project, ongoing interplay between data collection, analysis, and theory development. For example, new ideas or insights from participants may suggest potentially fruitful lines of enquiry, or close analysis might reveal subtle inconsistencies in an account which require further exploration.
Procedure for analysis
Stage 1: transcription.
A good quality audio recording and, ideally, a verbatim (word for word) transcription of the interview is needed. For Framework Method analysis, it is not necessarily important to include the conventions of dialogue transcriptions which can be difficult to read (e.g. pauses or two people talking simultaneously), because the content is what is of primary interest. Transcripts should have large margins and adequate line spacing for later coding and making notes. The process of transcription is a good opportunity to become immersed in the data and is to be strongly encouraged for new researchers. However, in some projects, the decision may be made that it is a better use of resources to outsource this task to a professional transcriber.
Stage 2: Familiarisation with the interview
Becoming familiar with the whole interview using the audio recording and/or transcript and any contextual or reflective notes that were recorded by the interviewer is a vital stage in interpretation. It can also be helpful to re-listen to all or parts of the audio recording. In multi-disciplinary or large research projects, those involved in analysing the data may be different from those who conducted or transcribed the interviews, which makes this stage particularly important. One margin can be used to record any analytical notes, thoughts or impressions.
Stage 3: Coding
After familiarization, the researcher carefully reads the transcript line by line, applying a paraphrase or label (a ‘code’) that describes what they have interpreted in the passage as important. In more inductive studies, at this stage ‘open coding’ takes place, i.e. coding anything that might be relevant from as many different perspectives as possible. Codes could refer to substantive things (e.g. particular behaviours, incidents or structures), values (e.g. those that inform or underpin certain statements, such as a belief in evidence-based medicine or in patient choice), emotions (e.g. sorrow, frustration, love) and more impressionistic/methodological elements (e.g. interviewee found something difficult to explain, interviewee became emotional, interviewer felt uncomfortable) [ 33 ]. In purely deductive studies, the codes may have been pre-defined (e.g. by an existing theory, or specific areas of interest to the project) so this stage may not be strictly necessary and you could just move straight onto indexing, although it is generally helpful even if you are taking a broadly deductive approach to do some open coding on at least a few of the transcripts to ensure important aspects of the data are not missed. Coding aims to classify all of the data so that it can be compared systematically with other parts of the data set. At least two researchers (or at least one from each discipline or speciality in a multi-disciplinary research team) should independently code the first few transcripts, if feasible. Patients, public involvement representatives or clinicians can also be productively involved at this stage, because they can offer alternative viewpoints thus ensuring that one particular perspective does not dominate. It is vital in inductive coding to look out for the unexpected and not to just code in a literal, descriptive way so the involvement of people from different perspectives can aid greatly in this. As well as getting a holistic impression of what was said, coding line-by-line can often alert the researcher to consider that which may ordinarily remain invisible because it is not clearly expressed or does not ‘fit’ with the rest of the account. In this way the developing analysis is challenged; to reconcile and explain anomalies in the data can make the analysis stronger. Coding can also be done digitally using CAQDAS, which is a useful way to keep track automatically of new codes. However, some researchers prefer to do the early stages of coding with a paper and pen, and only start to use CAQDAS once they reach Stage 5 (see below).
Stage 4: Developing a working analytical framework
After coding the first few transcripts, all researchers involved should meet to compare the labels they have applied and agree on a set of codes to apply to all subsequent transcripts. Codes can be grouped together into categories (using a tree diagram if helpful), which are then clearly defined. This forms a working analytical framework. It is likely that several iterations of the analytical framework will be required before no additional codes emerge. It is always worth having an ‘other’ code under each category to avoid ignoring data that does not fit; the analytical framework is never ‘final’ until the last transcript has been coded.
Stage 5: Applying the analytical framework
The working analytical framework is then applied by indexing subsequent transcripts using the existing categories and codes. Each code is usually assigned a number or abbreviation for easy identification (and so the full names of the codes do not have to be written out each time) and written directly onto the transcripts. Computer Assisted Qualitative Data Analysis Software (CAQDAS) is particularly useful at this stage because it can speed up the process and ensures that, at later stages, data is easily retrievable. It is worth noting that unlike software for statistical analyses, which actually carries out the calculations with the correct instruction, putting the data into a qualitative analysis software package does not analyse the data; it is simply an effective way of storing and organising the data so that they are accessible for the analysis process.
Stage 6: Charting data into the framework matrix
Qualitative data are voluminous (an hour of interview can generate 15–30 pages of text) and being able to manage and summarize (reduce) data is a vital aspect of the analysis process. A spreadsheet is used to generate a matrix and the data are ‘charted’ into the matrix. Charting involves summarizing the data by category from each transcript. Good charting requires an ability to strike a balance between reducing the data on the one hand and retaining the original meanings and ‘feel’ of the interviewees’ words on the other. The chart should include references to interesting or illustrative quotations. These can be tagged automatically if you are using CAQDAS to manage your data (N-Vivo version 9 onwards has the capability to generate framework matrices), or otherwise a capital ‘Q’, an (anonymized) transcript number, page and line reference will suffice. It is helpful in multi-disciplinary teams to compare and contrast styles of summarizing in the early stages of the analysis process to ensure consistency within the team. Any abbreviations used should be agreed by the team. Once members of the team are familiar with the analytical framework and well practised at coding and charting, on average, it will take about half a day per hour-long transcript to reach this stage. In the early stages, it takes much longer.
Stage 7: Interpreting the data
It is useful throughout the research to have a separate note book or computer file to note down impressions, ideas and early interpretations of the data. It may be worth breaking off at any stage to explore an interesting idea, concept or potential theme by writing an analytic memo [ 20 , 21 ] to then discuss with other members of the research team, including lay and clinical members. Gradually, characteristics of and differences between the data are identified, perhaps generating typologies, interrogating theoretical concepts (either prior concepts or ones emerging from the data) or mapping connections between categories to explore relationships and/or causality. If the data are rich enough, the findings generated through this process can go beyond description of particular cases to explanation of, for example, reasons for the emergence of a phenomena, predicting how an organisation or other social actor is likely to instigate or respond to a situation, or identifying areas that are not functioning well within an organisation or system. It is worth noting that this stage often takes longer than anticipated and that any project plan should ensure that sufficient time is allocated to meetings and individual researcher time to conduct interpretation and writing up of findings (see Additional file 1 , Section 7).
The Framework Method has been developed and used successfully in research for over 25 years, and has recently become a popular analysis method in qualitative health research. The issue of how to assess quality in qualitative research has been highly debated [ 20 , 34 – 40 ], but ensuring rigour and transparency in analysis is a vital component. There are, of course, many ways to do this but in the Framework Method the following are helpful:
Summarizing the data during charting, as well as being a practical way to reduce the data, means that all members of a multi-disciplinary team, including lay, clinical and (quantitative) academic members can engage with the data and offer their perspectives during the analysis process without necessarily needing to read all the transcripts or be involved in the more technical parts of analysis.
Charting also ensures that researchers pay close attention to describing the data using each participant’s own subjective frames and expressions in the first instance, before moving onto interpretation.
The summarized data is kept within the wider context of each case, thereby encouraging thick description that pays attention to complex layers of meaning and understanding [ 38 ].
The matrix structure is visually straightforward and can facilitate recognition of patterns in the data by any member of the research team, including through drawing attention to contradictory data, deviant cases or empty cells.
The systematic procedure (described in this article) makes it easy to follow, even for multi-disciplinary teams and/or with large data sets.
It is flexible enough that non-interview data (such as field notes taken during the interview or reflexive considerations) can be included in the matrix.
It is not aligned with a particular epistemological viewpoint or theoretical approach and therefore can be adapted for use in inductive or deductive analysis or a combination of the two (e.g. using pre-existing theoretical constructs deductively, then revising the theory with inductive aspects; or using an inductive approach to identify themes in the data, before returning to the literature and using theories deductively to help further explain certain themes).
It is easy to identify relevant data extracts to illustrate themes and to check whether there is sufficient evidence for a proposed theme.
Finally, there is a clear audit trail from original raw data to final themes, including the illustrative quotes.
There are also a number of potential pitfalls to this approach:
The systematic approach and matrix format, as we noted in the background, is intuitively appealing to those trained quantitatively but the ‘spreadsheet’ look perhaps further increases the temptation for those without an in-depth understanding of qualitative research to attempt to quantify qualitative data (e.g. “13 out of 20 participants said X). This kind of statement is clearly meaningless because the sampling in qualitative research is not designed to be representative of a wider population, but purposive to capture diversity around a phenomenon [ 41 ].
Like all qualitative analysis methods, the Framework Method is time consuming and resource-intensive. When involving multiple stakeholders and disciplines in the analysis and interpretation of the data, as is good practice in applied health research, the time needed is extended. This time needs to be factored into the project proposal at the pre-funding stage.
There is a high training component to successfully using the method in a new multi-disciplinary team. Depending on their role in the analysis, members of the research team may have to learn how to code, index, and chart data, to think reflexively about how their identities and experience affect the analysis process, and/or they may have to learn about the methods of generalisation (i.e. analytic generalisation and transferability, rather than statistical generalisation [ 41 ]) to help to interpret legitimately the meaning and significance of the data.
While the Framework Method is amenable to the participation of non-experts in data analysis, it is critical to the successful use of the method that an experienced qualitative researcher leads the project (even if the overall lead for a large mixed methods study is a different person). The qualitative lead would ideally be joined by other researchers with at least some prior training in or experience of qualitative analysis. The responsibilities of the lead qualitative researcher are: to contribute to study design, project timelines and resource planning; to mentor junior qualitative researchers; to train clinical, lay and other (non-qualitative) academics to contribute as appropriate to the analysis process; to facilitate analysis meetings in a way that encourages critical and reflexive engagement with the data and other team members; and finally to lead the write-up of the study.
We have argued that Framework Method studies can be conducted by multi-disciplinary research teams that include, for example, healthcare professionals, psychologists, sociologists, economists, and lay people/service users. The inclusion of so many different perspectives means that decision-making in the analysis process can be very time consuming and resource-intensive. It may require extensive, reflexive and critical dialogue about how the ideas expressed by interviewees and identified in the transcript are related to pre-existing concepts and theories from each discipline, and to the real ‘problems’ in the health system that the project is addressing. This kind of team effort is, however, an excellent forum for driving forward interdisciplinary collaboration, as well as clinical and lay involvement in research, to ensure that ‘the whole is greater than the sum of the parts’, by enhancing the credibility and relevance of the findings.
The Framework Method is appropriate for thematic analysis of textual data, particularly interview transcripts, where it is important to be able to compare and contrast data by themes across many cases, while also situating each perspective in context by retaining the connection to other aspects of each individual’s account. Experienced qualitative researchers should lead and facilitate all aspects of the analysis, although the Framework Method’s systematic approach makes it suitable for involving all members of a multi-disciplinary team. An open, critical and reflexive approach from all team members is essential for rigorous qualitative analysis.
Acceptance of the complexity of real life health systems and the existence of multiple perspectives on health issues is necessary to produce high quality qualitative research. If done well, qualitative studies can shed explanatory and predictive light on important phenomena, relate constructively to quantitative parts of a larger study, and contribute to the improvement of health services and development of health policy. The Framework Method, when selected and implemented appropriately, can be a suitable tool for achieving these aims through producing credible and relevant findings.
The Framework Method is an excellent tool for supporting thematic (qualitative content) analysis because it provides a systematic model for managing and mapping the data.
The Framework Method is most suitable for analysis of interview data, where it is desirable to generate themes by making comparisons within and between cases.
The management of large data sets is facilitated by the Framework Method as its matrix form provides an intuitively structured overview of summarised data.
The clear, step-by-step process of the Framework Method makes it is suitable for interdisciplinary and collaborative projects.
The use of the method should be led and facilitated by an experienced qualitative researcher.
Ritchie J, Lewis J: Qualitative research practice: a guide for social science students and researchers. 2003, London: Sage
Google Scholar
Ives J, Damery S, Redwod S: PPI, paradoxes and Plato: who's sailing the ship?. J Med Ethics. 2013, 39 (3): 181-185. 10.1136/medethics-2011-100150.
Article Google Scholar
Heath G, Cameron E, Cummins C, Greenfield S, Pattison H, Kelly D, Redwood S: Paediatric ‘care closer to home’: stake-holder views and barriers to implementation. Health Place. 2012, 18 (5): 1068-1073. 10.1016/j.healthplace.2012.05.003.
Elkington H, White P, Addington-Hall J, Higgs R, Petternari C: The last year of life of COPD: a qualitative study of symptoms and services. Respir Med. 2004, 98 (5): 439-445. 10.1016/j.rmed.2003.11.006.
Murtagh J, Dixey R, Rudolf M: A qualitative investigation into the levers and barriers to weight loss in children: opinions of obese children. Archives Dis Child. 2006, 91 (11): 920-923. 10.1136/adc.2005.085712.
Barnard M, Webster S, O’Connor W, Jones A, Donmall M: The drug treatment outcomes research study (DTORS): qualitative study. 2009, London: Home Office
Ayatollahi H, Bath PA, Goodacre S: Factors influencing the use of IT in the emergency department: a qualitative study. Health Inform J. 2010, 16 (3): 189-200. 10.1177/1460458210377480.
Sheard L, Prout H, Dowding D, Noble S, Watt I, Maraveyas A, Johnson M: Barriers to the diagnosis and treatment of venous thromboembolism in advanced cancer patients: a qualitative study. Palliative Med. 2012, 27 (2): 339-348.
Ellis J, Wagland R, Tishelman C, Williams ML, Bailey CD, Haines J, Caress A, Lorigan P, Smith JA, Booton R, et al: Considerations in developing and delivering a nonpharmacological intervention for symptom management in lung cancer: the views of patients and informal caregivers. J Pain Symptom Manag (0). 2012, 44 (6): 831-842. 10.1016/j.jpainsymman.2011.12.274.
Gale N, Sultan H: Telehealth as ‘peace of mind’: embodiment, emotions and the home as the primary health space for people with chronic obstructive pulmonary disorder. Health place. 2013, 21: 140-147.
Rashidian A, Eccles MP, Russell I: Falling on stony ground? A qualitative study of implementation of clinical guidelines’ prescribing recommendations in primary care. Health policy. 2008, 85 (2): 148-161. 10.1016/j.healthpol.2007.07.011.
Jones RK: The unsolicited diary as a qualitative research tool for advanced research capacity in the field of health and illness. Qualitative Health Res. 2000, 10 (4): 555-567. 10.1177/104973200129118543.
Pope C, Ziebland S, Mays N: Analysing qualitative data. British Med J. 2000, 320: 114-116. 10.1136/bmj.320.7227.114.
Pope C, Mays N: Critical reflections on the rise of qualitative research. British Med J. 2009, 339: 737-739.
Fairclough N: Critical discourse analysis: the critical study of language. 2010, London: Longman
Garfinkel H: Ethnomethodology’s program. Soc Psychol Quarter. 1996, 59 (1): 5-21. 10.2307/2787116.
Merleau-Ponty M: The phenomenology of perception. 1962, London: Routledge and Kegan Paul
Svenaeus F: The phenomenology of health and illness. Handbook of phenomenology and medicine. 2001, Netherlands: Springer, 87-108.
Reissmann CK: Narrative methods for the human sciences. 2008, London: Sage
Charmaz K: Constructing grounded theory: a practical guide through qualitative analysis. 2006, London: Sage
Glaser A, Strauss AL: The discovery of grounded theory. 1967, Chicago: Aldine
Crotty M: The foundations of social research: meaning and perspective in the research process. 1998, London: Sage
Boeije H: A purposeful approach to the constant comparative method in the analysis of qualitative interviews. Qual Quant. 2002, 36 (4): 391-409. 10.1023/A:1020909529486.
Hsieh H-F, Shannon SE: Three approaches to qualitative content analysis. Qual Health Res. 2005, 15 (9): 1277-1288. 10.1177/1049732305276687.
Redwood S, Gale NK, Greenfield S: ‘You give us rangoli, we give you talk’: using an art-based activity to elicit data from a seldom heard group. BMC Med Res Methodol. 2012, 12 (1): 7-10.1186/1471-2288-12-7.
Mishler EG: The struggle between the voice of medicine and the voice of the lifeworld. The sociology of health and illness: critical perspectives. Edited by: Conrad P, Kern R. 1990, New York: St Martins Press, Third
Hodges BD, Kuper A, Reeves S: Discourse analysis. British Med J. 2008, 337: 570-572. 10.1136/bmj.39370.701782.DE.
Sandelowski M, Barroso J: Writing the proposal for a qualitative research methodology project. Qual Health Res. 2003, 13 (6): 781-820. 10.1177/1049732303013006003.
Ellins J: It’s better together: involving older people in research. HSMC Newsletter Focus Serv Users Publ. 2010, 16 (1): 4-
Phillimore J, Goodson L, Hennessy D, Ergun E: Empowering Birmingham’s migrant and refugee community organisations: making a difference. 2009, York: Joseph Rowntree Foundation
Leamy M, Clough R: How older people became researchers. 2006, York: Joseph Rowntree Foundation
Glasby J, Miller R, Ellins J, Durose J, Davidson D, McIver S, Littlechild R, Tanner D, Snelling I, Spence K: Final report NIHR service delivery and organisation programme. Understanding and improving transitions of older people: a user and carer centred approach. 2012, London: The Stationery Office
Saldaña J: The coding manual for qualitative researchers. 2009, London: Sage
Lincoln YS: Emerging criteria for quality in qualitative and interpretive research. Qual Inquiry. 1995, 1 (3): 275-289. 10.1177/107780049500100301.
Mays N, Pope C: Qualitative research in health care: assessing quality in qualitative research. BMJ British Med J. 2000, 320 (7226): 50-10.1136/bmj.320.7226.50.
Seale C: Quality in qualitative research. Qual Inquiry. 1999, 5 (4): 465-478. 10.1177/107780049900500402.
Dingwall R, Murphy E, Watson P, Greatbatch D, Parker S: Catching goldfish: quality in qualitative research. J Health serv Res Policy. 1998, 3 (3): 167-172.
Popay J, Rogers A, Williams G: Rationale and standards for the systematic review of qualitative literature in health services research. Qual Health Res. 1998, 8 (3): 341-351. 10.1177/104973239800800305.
Morse JM, Barrett M, Mayan M, Olson K, Spiers J: Verification strategies for establishing reliability and validity in qualitative research. Int J Qual Methods. 2008, 1 (2): 13-22.
Smith JA: Reflecting on the development of interpretative phenomenological analysis and its contribution to qualitative research in psychology. Qual Res Psychol. 2004, 1 (1): 39-54.
Polit DF, Beck CT: Generalization in quantitative and qualitative research: Myths and strategies. Int J Nurs Studies. 2010, 47 (11): 1451-1458. 10.1016/j.ijnurstu.2010.06.004.
Pre-publication history
The pre-publication history for this paper can be accessed here: http://www.biomedcentral.com/1471-2288/13/117/prepub
Download references
Acknowledgments
All authors were funded by the National Institute for Health Research (NIHR) through the Collaborations for Leadership in Applied Health Research and Care for Birmingham and Black Country (CLAHRC-BBC) programme. The views in this publication expressed are those of the authors and not necessarily those of the NHS, the NIHR or the Department of Health.
Author information
Authors and affiliations.
Health Services Management Centre, University of Birmingham, Park House, 40 Edgbaston Park Road, Birmingham, B15 2RT, UK
Nicola K Gale
School of Health and Population Sciences, University of Birmingham, Edgbaston, Birmingham, B15 2TT, UK
Gemma Heath & Sabi Redwood
School of Life and Health Sciences, Aston University, Aston Triangle, Birmingham, B4 7ET, UK
Elaine Cameron
East and North Hertfordshire NHS Trust, Lister hospital, Coreys Mill Lane, Stevenage, SG1 4AB, UK
Sabina Rashid
You can also search for this author in PubMed Google Scholar
Corresponding author
Correspondence to Nicola K Gale .
Additional information
Competing interests.
The authors declare that they have no competing interests.
Authors’ contributions
All authors were involved in the development of the concept of the article and drafting the article. NG wrote the first draft of the article, GH and EC prepared the text and figures related to the illustrative example, SRa did the literature search to identify if there were any similar articles currently available and contributed to drafting of the article, and SRe contributed to drafting of the article and the illustrative example. All authors read and approved the final manuscript.
Electronic supplementary material
Additional file 1: illustrative example of the use of the framework method.(docx 167 kb), authors’ original submitted files for images.
Below are the links to the authors’ original submitted files for images.
Authors’ original file for figure 1
Authors’ original file for figure 2, authors’ original file for figure 3, authors’ original file for figure 4, authors’ original file for figure 5, authors’ original file for figure 6, rights and permissions.
This article is published under license to BioMed Central Ltd. This is an Open Access article distributed under the terms of the Creative Commons Attribution License ( http://creativecommons.org/licenses/by/2.0 ), which permits unrestricted use, distribution, and reproduction in any medium, provided the original work is properly cited.
Reprints and permissions
About this article
Cite this article.
Gale, N.K., Heath, G., Cameron, E. et al. Using the framework method for the analysis of qualitative data in multi-disciplinary health research. BMC Med Res Methodol 13 , 117 (2013). https://doi.org/10.1186/1471-2288-13-117
Download citation
Received : 17 December 2012
Accepted : 06 September 2013
Published : 18 September 2013
DOI : https://doi.org/10.1186/1471-2288-13-117
Share this article
Anyone you share the following link with will be able to read this content:
Sorry, a shareable link is not currently available for this article.
Provided by the Springer Nature SharedIt content-sharing initiative
- Qualitative research
- Qualitative content analysis
- Multi-disciplinary research
BMC Medical Research Methodology
ISSN: 1471-2288
- General enquiries: [email protected]

Official websites use .gov
A .gov website belongs to an official government organization in the United States.
Secure .gov websites use HTTPS
A lock ( ) or https:// means you've safely connected to the .gov website. Share sensitive information only on official, secure websites.

Collecting and Analyzing Qualitative Data
Brent Wolff, Frank Mahoney, Anna Leena Lohiniva, and Melissa Corkum
- Choosing When to Apply Qualitative Methods
- Commonly Used Qualitative Methods in Field Investigations
- Sampling and Recruitment for Qualitative Research
- Managing, Condensing, Displaying, and Interpreting Qualitative Data
- Coding and Analysis Requirements
Qualitative research methods are a key component of field epidemiologic investigations because they can provide insight into the perceptions, values, opinions, and community norms where investigations are being conducted ( 1,2 ). Open-ended inquiry methods, the mainstay of qualitative interview techniques, are essential in formative research for exploring contextual factors and rationales for risk behaviors that do not fit neatly into predefined categories. For example, during the 2014–2015 Ebola virus disease outbreaks in parts of West Africa, understanding the cultural implications of burial practices within different communities was crucial to designing and monitoring interventions for safe burials ( Box 10.1 ). In program evaluations, qualitative methods can assist the investigator in diagnosing what went right or wrong as part of a process evaluation or in troubleshooting why a program might not be working as well as expected. When designing an intervention, qualitative methods can be useful in exploring dimensions of acceptability to increase the chances of intervention acceptance and success. When performed in conjunction with quantitative studies, qualitative methods can help the investigator confirm, challenge, or deepen the validity of conclusions than either component might have yielded alone ( 1,2 ).
Qualitative research was used extensively in response to the Ebola virus disease outbreaks in parts of West Africa to understand burial practices and to design culturally appropriate strategies to ensure safe burials. Qualitative studies were also used to monitor key aspects of the response.
In October 2014, Liberia experienced an abrupt and steady decrease in case counts and deaths in contrast with predicted disease models of an increased case count. At the time, communities were resistant to entering Ebola treatment centers, raising the possibility that patients were not being referred for care and communities might be conducting occult burials.
To assess what was happening at the community level, the Liberian Emergency Operations Center recruited epidemiologists from the US Department of Health and Human Services/Centers for Disease Control and Prevention and the African Union to investigate the problem.
Teams conducted in-depth interviews and focus group discussions with community leaders, local funeral directors, and coffin makers and learned that communities were not conducting occult burials and that the overall number of burials was less than what they had experienced in previous years. Other key findings included the willingness of funeral directors to cooperate with disease response efforts, the need for training of funeral home workers, and considerable community resistance to cremation practices. These findings prompted the Emergency Operations Center to open a burial ground for Ebola decedents, support enhanced testing of burials in the private sector, and train private-sector funeral workers regarding safe burial practices.
Source: Melissa Corkum, personal communication.
Similar to quantitative approaches, qualitative research seeks answers to specific questions by using rigorous approaches to collecting and compiling information and producing findings that can be applicable beyond the study population. The fundamental difference in approaches lies in how they translate real-life complexities of initial observations into units of analysis. Data collected in qualitative studies typically are in the form of text or visual images, which provide rich sources of insight but also tend to be bulky and time-consuming to code and analyze. Practically speaking, qualitative study designs tend to favor small, purposively selected samples ideal for case studies or in-depth analysis ( 1 ). The combination of purposive sampling and open-ended question formats deprive qualitative study designs of the power to quantify and generalize conclusions, one of the key limitations of this approach.
Qualitative scientists might argue, however, that the generalizability and precision possible through probabilistic sampling and categorical outcomes are achieved at the cost of enhanced validity, nuance, and naturalism that less structured approaches offer ( 3 ). Open-ended techniques are particularly useful for understanding subjective meanings and motivations underlying behavior. They enable investigators to be equally adept at exploring factors observed and unobserved, intentions as well as actions, internal meanings as well as external consequences, options considered but not taken, and unmeasurable as well as measurable outcomes. These methods are important when the source of or solution to a public health problem is rooted in local perceptions rather than objectively measurable characteristics selected by outside observers ( 3 ). Ultimately, such approaches have the ability to go beyond quantifying questions of how much or how many to take on questions of how or why from the perspective and in the words of the study subjects themselves ( 1,2 ).
Another key advantage of qualitative methods for field investigations is their flexibility ( 4 ). Qualitative designs not only enable but also encourage flexibility in the content and flow of questions to challenge and probe for deeper meanings or follow new leads if they lead to deeper understanding of an issue (5). It is not uncommon for topic guides to be adjusted in the course of fieldwork to investigate emerging themes relevant to answering the original study question. As discussed herein, qualitative study designs allow flexibility in sample size to accommodate the need for more or fewer interviews among particular groups to determine the root cause of an issue (see the section on Sampling and Recruitment in Qualitative Research). In the context of field investigations, such methods can be extremely useful for investigating complex or fast-moving situations where the dimensions of analysis cannot be fully anticipated.
Ultimately, the decision whether to include qualitative research in a particular field investigation depends mainly on the nature of the research question itself. Certain types of research topics lend themselves more naturally to qualitative rather than other approaches ( Table 10.1 ). These include exploratory investigations when not enough is known about a problem to formulate a hypothesis or develop a fixed set of questions and answer codes. They include research questions where intentions matter as much as actions and “why?” or “why not?” questions matter as much as precise estimation of measured outcomes. Qualitative approaches also work well when contextual influences, subjective meanings, stigma, or strong social desirability biases lower faith in the validity of responses coming from a relatively impersonal survey questionnaire interview.
The availability of personnel with training and experience in qualitative interviewing or observation is critical for obtaining the best quality data but is not absolutely required for rapid assessment in field settings. Qualitative interviewing requires a broader set of skills than survey interviewing. It is not enough to follow a topic guide like a questionnaire, in order, from top to bottom. A qualitative interviewer must exercise judgment to decide when to probe and when to move on, when to encourage, challenge, or follow relevant leads even if they are not written in the topic guide. Ability to engage with informants, connect ideas during the interview, and think on one’s feet are common characteristics of good qualitative interviewers. By far the most important qualification in conducting qualitative fieldwork is a firm grasp of the research objectives; with this qualification, a member of the research team armed with curiosity and a topic guide can learn on the job with successful results.
Semi-Structured Interviews
Semi-structured interviews can be conducted with single participants (in-depth or individual key informants) or with groups (focus group discussions [FGDs] or key informant groups). These interviews follow a suggested topic guide rather than a fixed questionnaire format. Topic guides typically consist of a limited number ( 10– 15 ) of broad, open-ended questions followed by bulleted points to facilitate optional probing. The conversational back-and-forth nature of a semi-structured format puts the researcher and researched (the interview participants) on more equal footing than allowed by more structured formats. Respondents, the term used in the case of quantitative questionnaire interviews, become informants in the case of individual semi-structured in-depth interviews (IDIs) or participants in the case of FGDs. Freedom to probe beyond initial responses enables interviewers to actively engage with the interviewee to seek clarity, openness, and depth by challenging informants to reach below layers of self-presentation and social desirability. In this respect, interviewing is sometimes compared with peeling an onion, with the first version of events accessible to the public, including survey interviewers, and deeper inner layers accessible to those who invest the time and effort to build rapport and gain trust. (The theory of the active interview suggests that all interviews involve staged social encounters where the interviewee is constantly assessing interviewer intentions and adjusting his or her responses accordingly [ 1 ]. Consequently good rapport is important for any type of interview. Survey formats give interviewers less freedom to divert from the preset script of questions and formal probes.)
Individual In-Depth Interviews and Key-Informant Interviews
The most common forms of individual semi-structured interviews are IDIs and key informant interviews (KIIs). IDIs are conducted among informants typically selected for first-hand experience (e.g., service users, participants, survivors) relevant to the research topic. These are typically conducted as one-on-one face-to-face interviews (two-on-one if translators are needed) to maximize rapport-building and confidentiality. KIIs are similar to IDIs but focus on individual persons with special knowledge or influence (e.g., community leaders or health authorities) that give them broader perspective or deeper insight into the topic area ( Box 10.2 ). Whereas IDIs tend to focus on personal experiences, context, meaning, and implications for informants, KIIs tend to steer away from personal questions in favor of expert insights or community perspectives. IDIs enable flexible sampling strategies and represent the interviewing reference standard for confidentiality, rapport, richness, and contextual detail. However, IDIs are time-and labor-intensive to collect and analyze. Because confidentiality is not a concern in KIIs, these interviews might be conducted as individual or group interviews, as required for the topic area.
Focus Group Discussions and Group Key Informant Interviews
FGDs are semi-structured group interviews in which six to eight participants, homogeneous with respect to a shared experience, behavior, or demographic characteristic, are guided through a topic guide by a trained moderator ( 6 ). (Advice on ideal group interview size varies. The principle is to convene a group large enough to foster an open, lively discussion of the topic, and small enough to ensure all participants stay fully engaged in the process.) Over the course of discussion, the moderator is expected to pose questions, foster group participation, and probe for clarity and depth. Long a staple of market research, focus groups have become a widely used social science technique with broad applications in public health, and they are especially popular as a rapid method for assessing community norms and shared perceptions.
Focus groups have certain useful advantages during field investigations. They are highly adaptable, inexpensive to arrange and conduct, and often enjoyable for participants. Group dynamics effectively tap into collective knowledge and experience to serve as a proxy informant for the community as a whole. They are also capable of recreating a microcosm of social norms where social, moral, and emotional dimensions of topics are allowed to emerge. Skilled moderators can also exploit the tendency of small groups to seek consensus to bring out disagreements that the participants will work to resolve in a way that can lead to deeper understanding. There are also limitations on focus group methods. Lack of confidentiality during group interviews means they should not be used to explore personal experiences of a sensitive nature on ethical grounds. Participants may take it on themselves to volunteer such information, but moderators are generally encouraged to steer the conversation back to general observations to avoid putting pressure on other participants to disclose in a similar way. Similarly, FGDs are subject by design to strong social desirability biases. Qualitative study designs using focus groups sometimes add individual interviews precisely to enable participants to describe personal experiences or personal views that would be difficult or inappropriate to share in a group setting. Focus groups run the risk of producing broad but shallow analyses of issues if groups reach comfortable but superficial consensus around complex topics. This weakness can be countered by training moderators to probe effectively and challenge any consensus that sounds too simplistic or contradictory with prior knowledge. However, FGDs are surprisingly robust against the influence of strongly opinionated participants, highly adaptable, and well suited to application in study designs where systematic comparisons across different groups are called for.
Like FGDs, group KIIs rely on positive chemistry and the stimulating effects of group discussion but aim to gather expert knowledge or oversight on a particular topic rather than lived experience of embedded social actors. Group KIIs have no minimum size requirements and can involve as few as two or three participants.
Egypt’s National Infection Prevention and Control (IPC) program undertook qualitative research to gain an understanding of the contextual behaviors and motivations of healthcare workers in complying with IPC guidelines. The study was undertaken to guide the development of effective behavior change interventions in healthcare settings to improve IPC compliance.
Key informant interviews and focus group discussions were conducted in two governorates among cleaning staff, nursing staff, and physicians in different types of healthcare facilities. The findings highlighted social and cultural barriers to IPC compliance, enabling the IPC program to design responses. For example,
- Informants expressed difficulty in complying with IPC measures that forced them to act outside their normal roles in an ingrained hospital culture. Response: Role models and champions were introduced to help catalyze change.
- Informants described fatalistic attitudes that undermined energy and interest in modifying behavior. Response: Accordingly, interventions affirming institutional commitment to change while challenging fatalistic assumptions were developed.
- Informants did not perceive IPC as effective. Response: Trainings were amended to include scientific evidence justifying IPC practices.
- Informants perceived hygiene as something they took pride in and were judged on. Response: Public recognition of optimal IPC practice was introduced to tap into positive social desirability and professional pride in maintaining hygiene in the work environment.
Qualitative research identified sources of resistance to quality clinical practice in Egypt’s healthcare settings and culturally appropriate responses to overcome that resistance.
____________________ Source: Anna Leena Lohiniva, personal communication.
Visualization Methods
Visualization methods have been developed as a way to enhance participation and empower interviewees relative to researchers during group data collection ( 7 ). Visualization methods involve asking participants to engage in collective problem- solving of challenges expressed through group production of maps, diagrams, or other images. For example, participants from the community might be asked to sketch a map of their community and to highlight features of relevance to the research topic (e.g., access to health facilities or sites of risk concentrations). Body diagramming is another visualization tool in which community members are asked to depict how and where a health threat affects the human body as a way of understanding folk conceptions of health, disease, treatment, and prevention. Ensuing debate and dialogue regarding construction of images can be recorded and analyzed in conjunction with the visual image itself. Visualization exercises were initially designed to accommodate groups the size of entire communities, but they can work equally well with smaller groups corresponding to the size of FGDs or group KIIs.
Selecting a Sample of Study Participants
Fundamental differences between qualitative and quantitative approaches to research emerge most clearly in the practice of sampling and recruitment of study participants. Qualitative samples are typically small and purposive. In-depth interview informants are usually selected on the basis of unique characteristics or personal experiences that make them exemplary for the study, if not typical in other respects. Key informants are selected for their unique knowledge or influence in the study domain. Focus group mobilization often seeks participants who are typical with respect to others in the community having similar exposure or shared characteristics. Often, however, participants in qualitative studies are selected because they are exceptional rather than simply representative. Their value lies not in their generalizability but in their ability to generate insight into the key questions driving the study.
Determining Sample Size
Sample size determination for qualitative studies also follows a different logic than that used for probability sample surveys. For example, whereas some qualitative methods specify ideal ranges of participants that constitute a valid observation (e.g., focus groups), there are no rules on how many observations it takes to attain valid results. In theory, sample size in qualitative designs should be determined by the saturation principle , where interviews are conducted until additional interviews yield no additional insights into the topic of research ( 8 ). Practically speaking, designing a study with a range in number of interviews is advisable for providing a level of flexibility if additional interviews are needed to reach clear conclusions.
Recruiting Study Participants
Recruitment strategies for qualitative studies typically involve some degree of participant self-selection (e.g., advertising in public spaces for interested participants) and purposive selection (e.g., identification of key informants). Purposive selection in community settings often requires authorization from local authorities and assistance from local mobilizers before the informed consent process can begin. Clearly specifying eligibility criteria is crucial for minimizing the tendency of study mobilizers to apply their own filters regarding who reflects the community in the best light. In addition to formal eligibility criteria, character traits (e.g., articulate and interested in participating) and convenience (e.g., not too far away) are legitimate considerations for whom to include in the sample. Accommodations to personality and convenience help to ensure the small number of interviews in a typical qualitative design yields maximum value for minimum investment. This is one reason why random sampling of qualitative informants is not only unnecessary but also potentially counterproductive.
Analysis of qualitative data can be divided into four stages: data management, data condensation, data display, and drawing and verifying conclusions ( 9 ).
Managing Qualitative Data
From the outset, developing a clear organization system for qualitative data is important. Ideally, naming conventions for original data files and subsequent analysis should be recorded in a data dictionary file that includes dates, locations, defining individual or group characteristics, interviewer characteristics, and other defining features. Digital recordings of interviews or visualization products should be reviewed to ensure fidelity of analyzed data to original observations. If ethics agreements require that no names or identifying characteristics be recorded, all individual names must be removed from final transcriptions before analysis begins. If data are analyzed by using textual data analysis software, maintaining careful version control over the data files is crucial, especially when multiple coders are involved.
Condensing Qualitative Data
Condensing refers to the process of selecting, focusing, simplifying, and abstracting the data available at the time of the original observation, then transforming the condensed data into a data set that can be analyzed. In qualitative research, most of the time investment required to complete a study comes after the fieldwork is complete. A single hour of taped individual interview can take a full day to transcribe and additional time to translate if necessary. Group interviews can take even longer because of the difficulty of transcribing active group input. Each stage of data condensation involves multiple decisions that require clear rules and close supervision. A typical challenge is finding the right balance between fidelity to the rhythm and texture of original language and clarity of the translated version in the language of analysis. For example, discussions among groups with little or no education should not emerge after the transcription (and translation) process sounding like university graduates. Judgment must be exercised about which terms should be translated and which terms should be kept in vernacular because there is no appropriate term in English to capture the richness of its meaning.
Displaying Qualitative Data
After the initial condensation, qualitative analysis depends on how the data are displayed. Decisions regarding how data are summarized and laid out to facilitate comparison influence the depth and detail of the investigation’s conclusions. Displays might range from full verbatim transcripts of interviews to bulleted summaries or distilled summaries of interview notes. In a field setting, a useful and commonly used display format is an overview chart in which key themes or research questions are listed in rows in a word processer table or in a spreadsheet and individual informant or group entry characteristics are listed across columns. Overview charts are useful because they allow easy, systematic comparison of results.
Drawing and Verifying Conclusions
Analyzing qualitative data is an iterative and ideally interactive process that leads to rigorous and systematic interpretation of textual or visual data. At least four common steps are involved:
- Reading and rereading. The core of qualitative analysis is careful, systematic, and repeated reading of text to identify consistent themes and interconnections emerging from the data. The act of repeated reading inevitably yields new themes, connections, and deeper meanings from the first reading. Reading the full text of interviews multiple times before subdividing according to coded themes is key to appreciating the full context and flow of each interview before subdividing and extracting coded sections of text for separate analysis.
- Coding. A common technique in qualitative analysis involves developing codes for labeling sections of text for selective retrieval in later stages of analysis and verification. Different approaches can be used for textual coding. One approach, structural coding , follows the structure of the interview guide. Another approach, thematic coding , labels common themes that appear across interviews, whether by design of the topic guide or emerging themes assigned based on further analysis. To avoid the problem of shift and drift in codes across time or multiple coders, qualitative investigators should develop a standard codebook with written definitions and rules about when codes should start and stop. Coding is also an iterative process in which new codes that emerge from repeated reading are layered on top of existing codes. Development and refinement of the codebook is inseparably part of the analysis.
- Analyzing and writing memos. As codes are being developed and refined, answers to the original research question should begin to emerge. Coding can facilitate that process through selective text retrieval during which similarities within and between coding categories can be extracted and compared systematically. Because no p values can be derived in qualitative analyses to mark the transition from tentative to firm conclusions, standard practice is to write memos to record evolving insights and emerging patterns in the data and how they relate to the original research questions. Writing memos is intended to catalyze further thinking about the data, thus initiating new connections that can lead to further coding and deeper understanding.
- Verifying conclusions. Analysis rigor depends as much on the thoroughness of the cross-examination and attempt to find alternative conclusions as on the quality of original conclusions. Cross-examining conclusions can occur in different ways. One way is encouraging regular interaction between analysts to challenge conclusions and pose alternative explanations for the same data. Another way is quizzing the data (i.e., retrieving coded segments by using Boolean logic to systematically compare code contents where they overlap with other codes or informant characteristics). If alternative explanations for initial conclusions are more difficult to justify, confidence in those conclusions is strengthened.
Above all, qualitative data analysis requires sufficient time and immersion in the data. Computer textual software programs can facilitate selective text retrieval and quizzing the data, but discerning patterns and arriving at conclusions can be done only by the analysts. This requirement involves intensive reading and rereading, developing codebooks and coding, discussing and debating, revising codebooks, and recoding as needed until clear patterns emerge from the data. Although quality and depth of analysis is usually proportional to the time invested, a number of techniques, including some mentioned earlier, can be used to expedite analysis under field conditions.
- Detailed notes instead of full transcriptions. Assigning one or two note-takers to an interview can be considered where the time needed for full transcription and translation is not feasible. Even if plans are in place for full transcriptions after fieldwork, asking note-takers to submit organized summary notes is a useful technique for getting real-time feedback on interview content and making adjustments to topic guides or interviewer training as needed.
- Summary overview charts for thematic coding. (See discussion under “Displaying Data.”) If there is limited time for full transcription and/or systematic coding of text interviews using textual analysis software in the field, an overview chart is a useful technique for rapid manual coding.
- Thematic extract files. This is a slightly expanded version of manual thematic coding that is useful when full transcriptions of interviews are available. With use of a word processing program, files can be sectioned according to themes, or separate files can be created for each theme. Relevant extracts from transcripts or analyst notes can be copied and pasted into files or sections of files corresponding to each theme. This is particularly useful for storing appropriate quotes that can be used to illustrate thematic conclusions in final reports or manuscripts.
- Teamwork. Qualitative analysis can be performed by a single analyst, but it is usually beneficial to involve more than one. Qualitative conclusions involve subjective judgment calls. Having more than one coder or analyst working on a project enables more interactive discussion and debate before reaching consensus on conclusions.
- Systematic coding.
- Selective retrieval of coded segments.
- Verifying conclusions (“quizzing the data”).
- Working on larger data sets with multiple separate files.
- Working in teams with multiple coders to allow intercoder reliability to be measured and monitored.
The most widely used software packages (e.g., NVivo [QSR International Pty. Ltd., Melbourne, VIC, Australia] and ATLAS.ti [Scientific Software Development GmbH, Berlin, Germany]) evolved to include sophisticated analytic features covering a wide array of applications but are relatively expensive in terms of license cost and initial investment in time and training. A promising development is the advent of free or low-cost Web-based services (e.g., Dedoose [Sociocultural Research Consultants LLC, Manhattan Beach, CA]) that have many of the same analytic features on a more affordable subscription basis and that enable local research counterparts to remain engaged through the analysis phase (see Teamwork criteria). The start-up costs of computer-assisted analysis need to be weighed against their analytic benefits, which tend to decline with the volume and complexity of data to be analyzed. For rapid situational analyses or small scale qualitative studies (e.g. fewer than 30 observations as an informal rule of thumb), manual coding and analysis using word processing or spreadsheet programs is faster and sufficient to enable rigorous analysis and verification of conclusions.
Qualitative methods belong to a branch of social science inquiry that emphasizes the importance of context, subjective meanings, and motivations in understanding human behavior patterns. Qualitative approaches definitionally rely on open-ended, semistructured, non-numeric strategies for asking questions and recording responses. Conclusions are drawn from systematic visual or textual analysis involving repeated reading, coding, and organizing information into structured and emerging themes. Because textual analysis is relatively time-and skill-intensive, qualitative samples tend to be small and purposively selected to yield the maximum amount of information from the minimum amount of data collection. Although qualitative approaches cannot provide representative or generalizable findings in a statistical sense, they can offer an unparalleled level of detail, nuance, and naturalistic insight into the chosen subject of study. Qualitative methods enable investigators to “hear the voice” of the researched in a way that questionnaire methods, even with the occasional open-ended response option, cannot.
Whether or when to use qualitative methods in field epidemiology studies ultimately depends on the nature of the public health question to be answered. Qualitative approaches make sense when a study question about behavior patterns or program performance leads with why, why not , or how . Similarly, they are appropriate when the answer to the study question depends on understanding the problem from the perspective of social actors in real-life settings or when the object of study cannot be adequately captured, quantified, or categorized through a battery of closed-ended survey questions (e.g., stigma or the foundation of health beliefs). Another justification for qualitative methods occurs when the topic is especially sensitive or subject to strong social desirability biases that require developing trust with the informant and persistent probing to reach the truth. Finally, qualitative methods make sense when the study question is exploratory in nature, where this approach enables the investigator the freedom and flexibility to adjust topic guides and probe beyond the original topic guides.
Given that the conditions just described probably apply more often than not in everyday field epidemiology, it might be surprising that such approaches are not incorporated more routinely into standard epidemiologic training. Part of the answer might have to do with the subjective element in qualitative sampling and analysis that seems at odds with core scientific values of objectivity. Part of it might have to do with the skill requirements for good qualitative interviewing, which are generally more difficult to find than those required for routine survey interviewing.
For the field epidemiologist unfamiliar with qualitative study design, it is important to emphasize that obtaining important insights from applying basic approaches is possible, even without a seasoned team of qualitative researchers on hand to do the work. The flexibility of qualitative methods also tends to make them forgiving with practice and persistence. Beyond the required study approvals and ethical clearances, the basic essential requirements for collecting qualitative data in field settings start with an interviewer having a strong command of the research question, basic interactive and language skills, and a healthy sense of curiosity, armed with a simple open-ended topic guide and a tape recorder or note-taker to capture the key points of the discussion. Readily available manuals on qualitative study design, methods, and analysis can provide additional guidance to improve the quality of data collection and analysis.
- Patton MQ. Qualitative research and evaluation methods: integrating theory and practice . 4th ed. Thousand Oaks, CA: Sage; 2015.
- Hennink M, Hutter I, Bailey A. Qualitative research methods . Thousand Oaks, CA: Sage; 2010.
- Lincoln YS, Guba EG. The constructivist credo . Walnut Creek, CA: Left Coast Press; 2013.
- Mack N, Woodsong C, MacQueen KM, Guest G, Namey E. Qualitative research methods: a data collectors field guide. https://www.fhi360.org/sites/default/files/media/documents/Qualitative%20Research%20Methods%20-%20A%20Data%20Collector%27s%20Field%20Guide.pdf
- Kvale S, Brinkmann S. Interviews: learning the craft of qualitative research . Thousand Oaks, CA: Sage; 2009:230–43.
- Krueger RA, Casey MA. Focus groups: a practical guide for applied research . Thousand Oaks, CA: Sage; 2014.
- Margolis E, Pauwels L. The Sage handbook of visual research methods . Thousand Oaks, CA: Sage; 2011.
- Mason M. Sample size and saturation in PhD studies using qualitative interviews. Forum : Qualitative Social Research/Sozialforschung. 2010;11(3).
- Miles MB, Huberman AM, Saldana J. Qualitative data analysis: a methods sourcebook . 3rd ed. Thousand Oaks, CA: Sage; 2014.
- Silver C, Lewins A. Using software in qualitative research: a step-by-step guide . Thousand Oaks, CA; Sage: 2014.
< Previous Chapter 9: Optimizing Epidemiology– Laboratory Collaborations
Next Chapter 11: Developing Interventions >
The fellowship application period will be open March 3, 2025, through June 5, 2025.
The host site application period will be open December 4, 2024, through January 24, 2025.
For questions about the EIS program, please contact us directly at [email protected] .
- Laboratory Leadership Service (LLS)
- Fellowships and Training Opportunities
- Division of Workforce Development
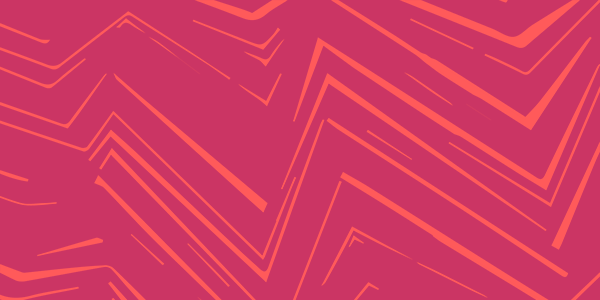
Economic and Social Research Council (ESRC)
ESRC is the UK’s largest funder of economic, social, behavioural and human data science.
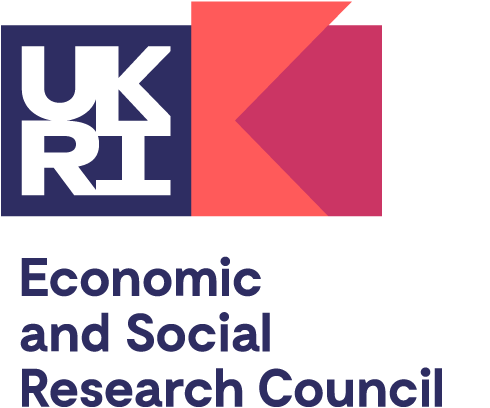
ESRC content
- Funding, assessment and award management
- Strategy, remit and programmes
- People, skills and fellowships
- Innovation and collaboration
- Investments, impacts and engagement
- Facilities and resources
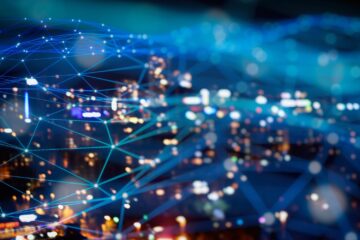
Transition to the new UKRI Funding Service
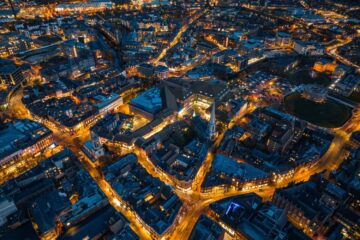
ESRC strategic delivery plan
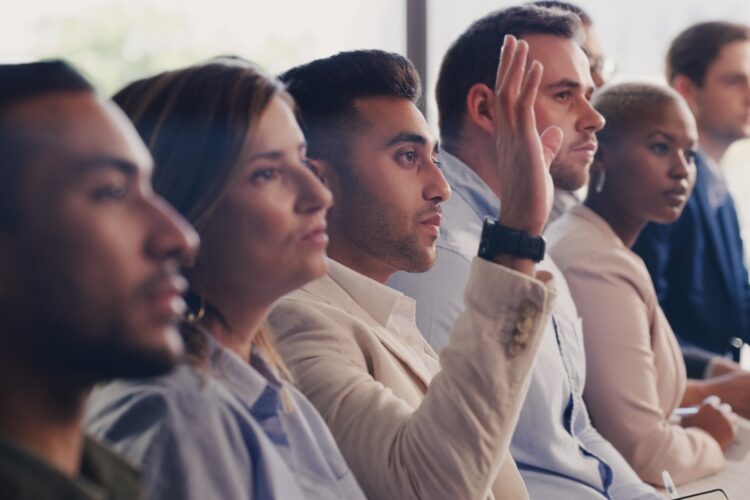
22 October 2024
UKRI seeks your views in our 2024 stakeholder perception survey
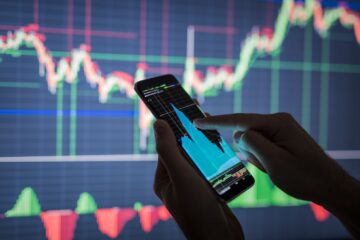
17 October 2024
£22 million investment in smart data services for UK research
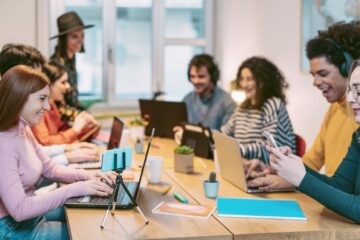
24 September 2024
22nd year of ESRC’s celebration of economics and social science
View all ESRC news
Funding opportunities
Pre-announcement: future leaders fellowships: round 10.
Funding to support ambitious research and innovation across UK Research and Innovation (UKRI)’s remit. You must be an early career researcher or innovator who is either:
- looking to establish or transition to independence
- developing your own original and ambitious plans within a commercial setting
UKRI Future Leaders Fellowships Renewal Meeting 11 (Invite only)
Future Leaders Fellowship (FLF) award holders have the opportunity to extend their four-year fellowship by up to a further three years. The renewal funding will continue to support the development of a fellow and further the fellow’s work to undertake adventurous, ambitious programmes that tackle difficult and novel challenges.
View all ESRC opportunities
Economic and Social Research Council (ESRC) Polaris House, North Star Avenue, Swindon, SN2 1UJ
Connect with ESRC
Subscribe to ukri emails.
Keep up to date with funding, news and events, and a weekly newsletter.
There are no upcoming ESRC events at this time.
View all events
This is the website for UKRI: our seven research councils, Research England and Innovate UK. Let us know if you have feedback or would like to help improve our online products and services .
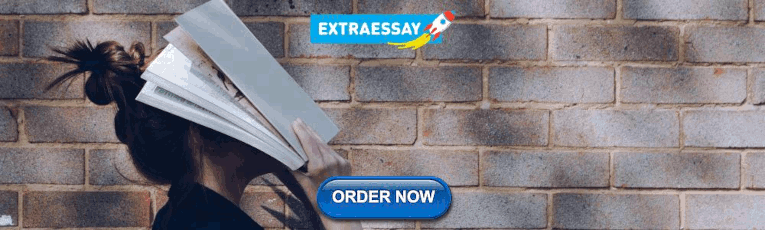
IMAGES
VIDEO
COMMENTS
Despite the growing recognition of qualitative research's utility for health equity, leading public health journals tend to publish more quantitative research (Stickley et al., 2022).Many researchers face barriers to applying qualitative methodologies, including the time and resources needed for data collection and analysis and limited or inadequate training experiences (Silverio, S., Hall ...
Nevertheless, some approaches to qualitative data analysis are useful in health services research. In this paper, we focus on strategies for analysis of qualitative data that are especially applicable in the generation of taxonomy, themes, and theory (Table 1).
In much qualitative research the analytical process begins during data collection as the data already gathered are analysed and shape the ongoing data collection. This sequential analysis 1 or interim analysis 2 has the advantage of allowing the researcher to go back and refine questions, develop hypotheses, and pursue emerging avenues of ...
This question is particularly relevant to researchers new to the field and practice of qualitative research and instructors and mentors who regularly introduce students to qualitative research practices. In this article, we seek to offer what we view as a useful starting point for learning how to do qualitative analysis. We begin by discussing ...
Data analysis is one of the most important, yet least understood, stages of the qualitative research process. Through rigorous analysis, data can illuminate the complexity of human behavior, inform interventions, and give voice to people's lived experiences. While significant progress has been made …
Process of data analysis. Qualitative data analysis can be both deductive and inductive. The deductive process, in which there is an attempt to establish causal relationships, is although associated with quantitative research, can be applied also in qualitative research as a deductive explanatory process or deductive category application. When ...
To enhance the transferability of qualitative research, researchers must describe the data collection and analysis processes in detail and provide thick data on the overall research process, including research participants and the context and culture of research [77,78]. Transferability can also be enhanced by checking whether the analysis ...
Qualitative inquiry can improve the description and explanation of complex, real-world phenomena pertinent to health services research. Greater understanding of the processes of qualitative data analysis can be helpful for health services researchers as they use these methods themselves or collabora …
Qualitative research methodology has been applied with increasing frequency in various fields, including in healthcare research, where quantitative research methodology has traditionally dominated, with an empirically driven approach involving statistical analysis. Drawing upon artifacts and verbal …
We draw on extant qualitative methodological literature to describe practical approaches to qualitative data analysis. Approaches to data analysis vary by discipline and analytic tradition; however, we focus on qualitative data analysis that has as a goal the generation of taxonomy, themes, and theory germane to health services research ...
Learn how to use thematic analysis, a flexible and powerful method of qualitative data analysis, in applied health services research. This article offers a step-by-step approach, practical tips, and companion materials for multidisciplinary teams, including patients and clinicians.
Data analysis is one of the most important, yet least understood, stages of the qualitative research process. Through rigorous analysis, data can illuminate the complexity of human behavior, inform interventions, and give voice to people's lived experiences. While significant progress has been made in advancing the rigor of qualitative ...
Learn how to use the Framework Method, a systematic and flexible approach to managing and analysing qualitative data in multi-disciplinary health research teams. The article explains the stages, strengths and limitations of the method, and provides a worked example from a published study.
A thematic analysis is a common method of analysing qualitative data and is frequently employed in health services research. 4 You can either transcribe the audio files containing the data to text yourself or use a professional transcription company. The next stage is to familiarise yourself with these data.
We draw on extant qualitative methodological literature to describe practical approaches to qualitative data analysis. Approaches to data analysis vary by discipline and analytic tradition; however, we focus on qualitative data analysis that has as a goal the generation of taxonomy, themes, and theory germane to health services research.
Qualitative data analysis in one of the most important steps in the qualitative research process (Leech & Onwuegbuzie, 2007) because it assists researchers to make sense of their qualitative data.
Data Analysis in Qualitative Study. Journal of . Preventive Medicine & Public Health. ... Most participants (214, 91%) agreed that qualitative research is important in health sciences. More than ...
The Qualitative Report Volume 27 Number 4 How To Article 9 4-5-2022 Qualitative Data Analysis for Health Research: A Step-by-Step Example of Phenomenological Interpretation Sue Monaro University of Sydney, Australia, [email protected] Janice Gullick University of Sydney, Australia, [email protected] Sandra West
To better understand how our field conducts and reports qualitative analysis, we reviewed qualitative articles published in Health Education & Behavior between 2000 and 2015. Two independent reviewers abstracted information in the following categories: data management software, coding approach, analytic approach, indicators of trustworthiness ...
Learn how qualitative methods can provide insight into perceptions, values, opinions, and community norms in field investigations. Find out when and how to apply qualitative research techniques, such as open-ended interviews, focus groups, and observation, in public health contexts.
ESRC supports research and training in economic, social, behavioural and human data science. Find out about ESRC's funding opportunities, news, events and strategic delivery plan.