
An official website of the United States government
The .gov means it’s official. Federal government websites often end in .gov or .mil. Before sharing sensitive information, make sure you’re on a federal government site.
The site is secure. The https:// ensures that you are connecting to the official website and that any information you provide is encrypted and transmitted securely.
- Publications
- Account settings
Preview improvements coming to the PMC website in October 2024. Learn More or Try it out now .
- Advanced Search
- Journal List
- HHS Author Manuscripts

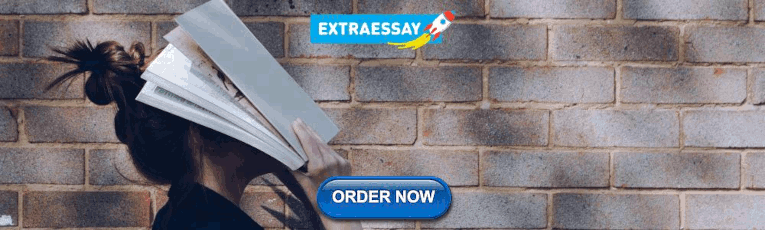
Overview: Cross-Sectional Studies
The conduct of research requires the selection of the appropriate method to evaluate the research problem or question. Due to some topics’ ethical nature or the need to understand the natural history (i.e., disease or condition), using an observational study design might be the best fit. The primary purposes of observational studies are to describe and examine the distributions of independent (predictor) and dependent (outcome) variables in a population (sample) and analyze the associations between them ( Cummings, 2013 ). Observational studies monitor study participants without providing study interventions. This paper describes the cross-sectional design, examines the strengths and weaknesses, and discusses some methods to report the results. Future articles will focus on other observational methods, the cohort, and case-control designs.
Cross-Sectional Design
Cross-sectional designs help determine the prevalence of a disease, phenomena, or opinion in a population, as represented by a study sample. Prevalence is the proportion of people in a population (sample) who have an attribute or condition at a specific time point ( Mann, 2012 ) regardless of when the attribute or condition first developed ( Wang & Cheng, 2020 ). Additionally, each study participant’s evaluation is completed at one time-point with no follow-ups ( Cummings, 2013 ), providing a ‘snapshot’ of the sample. Cross-sectional designs can be implemented as an interview or survey and may also collect physiological data and biological samples.
Cross-Sectional Design: Descriptive
Cross-sectional studies can be descriptive and analytic ( Alexander, 2015a ). Descriptive cross-sectional studies characterize the prevalence of health outcomes or phenomena under investigation. Prevalence is measured either at a one-time point ( point prevalence ), over a specified period ( period prevalence ) ( Alexander, 2015a ), or as a cross-sectional serial survey ( Cummings, 2013 ). The descriptive design starts by identifying the population of interest, collects the data, and classifies the participant, either as having the outcome or phenomena of interest or not ( Mann, 2012 ). For example, investigators want to determine the point prevalence of obesity among people with HIV. To conduct this study, investigators select several HIV primary care clinics in their region and obtain heights, weights, and measure waist circumference during one specified day at each clinic. For a period prevalence study, the investigators could visit each clinic at four-time points over 12 months to obtain body measurements to capture other patients visiting the clinics. Period prevalence and point prevalence are similar, except that the time-frame is broader since it can be difficult to evaluate or observe the entire population or sample at one time-point**.
For serial cross-sectional surveys, investigators collect data in the same population over a specified period. It uses a longitudinal time-frame. For example, every three years, investigators repeat the body measurements among HIV patients to draw inferences about the patterns over time about obesity( Cummings, 2013 ). However, new samples are selected each time; therefore, each participant’s changes cannot be evaluated. It is important to note that the results may be affected by “people entering or leaving the population due to births, deaths, and migration” ( Cummings, 2013 , p.88).
Method to Report Results: Descriptive Cross-Sectional Design
Prevalence is generally reported as a percentage (30% or 75 out of 250 HIV patients were obese). Knowing the prevalence of a condition in a population (sample) helps understand the disease burden in terms of services needed, morbidity, mortality, and quality of life ( Noordzij, Dekker, Zoccali, & Jager, 2010 ). For instance, if obesity is high among the participants, clinic visits could provide nutritional counseling and physical activity recommendations and regularly monitor body weight measurements to prevent the complications associated with obesity (i.e., knee osteoarthritis, type 2 diabetes mellitus).
Cross-Sectional Design: Analytic
Analytic cross-sectional studies can provide the groundwork to infer preliminary evidence for a causal relationship ( Mann, 2012 ). This design allows investigators to identify a population or sample and collect prevalence data to evaluate outcome differences between exposed and unexposed participants on a disease, phenomena, or opinion ( Wang & Cheng, 2020 ). This design compares the proportion of participants exposed to the disease or phenomena of interest with the proportion of participants non-exposed with the disease or phenomena of interest ( Alexander, 2015a ). However, determining which variable is the dependent and independent variable or cause and effect is difficult to determine. For example, the association between obesity and hours spent in sedentary behavior among HIV patients (see Table 1 ). Which came first? Did the participant become obese due to sedentary behavior, or was the participant inactive due to obesity? According to Cummings et al., 2013 , determining which variable to label as dependent or independent “depends on the cause-and-effect hypotheses of the investigator” (p. 85) or the biological plausibility rather than on the study design.
Calculation Example
- a = exposed participant and acquires the outcome of interest
- b = unexposed participant and acquires the outcome of interest
- c = exposed participant and does not acquire the outcome of interest
- d = unexposed participant and does not acquire the outcome of interest
- Prevalence of HIV participants who are obese and sedentary = a/(a + b) = 75/325 =. 23 × 100 = 23%
- Prevalence of HIV participants who are obese and not sedentary = c/(c + d) = 25/225 = .11 × 100 = 11.1%
- Prevalence of overall HIV participants who are obese = (a + c)/(a + b + c + d) = 100/550 = .182 × 100 = 18.2%
Interpretation of Prevalence Odds Ratio/Odds Ratio:
- OR = 1 Exposure did not effect the odds of the outcome
- OR > 1 Exposure is associated with the higher odds of outcome versus nonexposed group
- OR < 1 Exposure is associated with lower odds of outcome verus exposed group
- Upper 95 % CI = e ^ [ ln ( OR ) + 1.96 sqrt ( 1 / a + 1 / b + 1 / c + 1 / d ) ] = 1.4713
- Lower 95 % CI = e ^ [ ln ( OR ) − 1.96 sqrt ( 1 / a + 1 / b + 1 / c + 1 / d ) ] = 3.9150
Interpretation of Prevalence Ratio/Risk Ratio:
- RR = 1 Exposure did not prevent or harm the exposed and unexposed groups
- RR > 1 Exposure is harmful to the exposed group compared to the unexposed group
- RR < 1 Exposure is less harmful (protective) to the exposed group compared to the unexposed group
- Upper 95 % CI = e ^ [ ln ( RR ) − 1.96 sqrt ( 1 / a + 1 / c − 1 / a + b − 1 / c + d ) ] = 1.3653
- Lower 95 % CI = e ^ [ ln ( RR ) + 1.96 sqrt ( 1 / a + 1 / c − 1 / a + b − 1 / c + d ) ] = 3.159
References: Alexander, 2015a, Cummings, 2013, Tenny &Hoffman, 2019.
** https://www.medcalc.org/calc/odds_ratio.php (web-based confidence interval calculator of odds ratio)
*** https://www.medcalc.org/calc/relative_risk.php (web-based confidence interval calculator RR
Method to Report Results: Analytic Cross-Sectional Design
In continuing with the obesity and sedentary activity level among HIV participants, the example below (see Table 1 ) describes the methods for calculating and discussing the results for an analytic cross-sectional study. The prevalence odds ratio (POR) (calculated as [ ad/bc] ) and prevalence ratio (PR) (calculated as [a/(a + b)]/ [c/(c + d)]) are commonly used to report estimates of association between independent and dependent variables in cross-sectional studies ( Tamhane, Westfall, Burkholder, & Cutter, 2016 ).
Prevalence Odds Ratio/Odds Ratio
The POR is calculated similarly to the odds ratio (OR) ( Alexander, 2015b ) and referred to as POR when prevalence is used ( Tamhane et al., 2016 ). OR measures the association between exposure and outcome (see Table 1 ) and denotes the chances that an outcome happens with a specific exposure, compared to the chances of an outcome happening in the absence of the exposure (Szumilas, 2010). This information helps both clinicians and investigators determine if certain factors (i.e., clinical characteristics, medical history) are a risk for a particular outcome (i.e., disease, condition). Future studies or health policies can target methods to prevent or treat outcomes (i.e., disease, condition) identified in such studies.
For example, in Table 1 , using the formula and dataset below, the OR was 2.4. The result shows that the obese HIV participants (exposed) were two and a half times (2.5x) more likely to be sedentary than the non-obese participants (unexposed). If the OR for the dataset was equal to 1, then the exposure (obese) did not affect the outcome’s odds. In other words, the chance of being sedentary is the same in the exposed (obese) and the non-exposed (not obese) groups. Similarly, if the OR was less than 1, it implies that the exposed (obese) group, were less likely to be sedentary (outcome) compared to the non-obese group (unexposed) ( Tenny & Hoffman, 2019 ).
Prevalence Ratio/Risk Ratio and Excess Prevalence/Risk Difference
The PR is calculated similarly to the risk ratio (RR)( Alexander, 2015b ). The PR measures the prevalence of an outcome in the exposed group, divided by the unexposed group, and measures the association’s strength between the exposure and outcome (Alexander, 2015). Excess prevalence (EP) or the risk difference (RD) provides the difference in prevalence between the groups and indicates how much additional prevalence is due to the exposure of interest ( Alexander, 2015b ). From Table 1 , the PR/RR for the example equaled 2.07, with an EP of 11.9%. The results might conclude that obesity among the HIV participants was twice (2.07) as common and occurred almost 12% more often among HIV participants who were sedentary.
Similar to the OR interpretation, if the RR was equal to 1, exposure did not prevent or harm the exposed and unexposed groups. In other words, being obese did not affect the activity level (sedentary versus not sedentary). If the RR was less than 1, it implies that the exposure had a protective effect in that obese HIV participants were less likely to be sedentary than the unexposed group (not obese).
Considerations for use: Prevalence Odds Ratio versus Prevalence Ratio
The statistical literature has numerous articles discussing the pros and cons of using either the POR/OR or PR/RR for cross-sectional studies ( Tamhane et al., 2016 ). Consulting a statistician to discuss the best choice for each project is highly recommended. However, according to Alexander and colleagues (2015a) , the POR is preferred when the study topic is a chronic condition (i.e., hypertension, HIV), or the risk of developing the disease takes several months to develop. For studies evaluating acute conditions (i.e., the common cold), the PR is favored ( Alexander, 2015a ).
Furthermore, suppose the prevalence of a disease or phenomena is low, less than ten percent in the exposed and unexposed population (sample). In that case, the resulting POR and PR will be equal ( Alexander, 2015a ). Since cross-sectional studies are suitable for examining chronic diseases or conditions, the POR is generally the ideal measure of association to use ( Alexander, 2015a ).
Confidence Intervals
Confidence intervals (CI) measure the precision of the OR, RR, or the possible “variation in a point estimate (the mean value)” ( Alexander, 2015b , p 4). A narrower CI indicates a higher level of precision versus a wider CI suggesting a lower level of precision ( Cummings, 2013 ). The sample size also impacts the CI’s width, with larger sample sizes providing a more precise estimate. The approximate value of the point estimate is based on factors (i.e., characteristics like body weight, level of activity) such as the mean (average) of a population from a population’s random samples.
From Table 1 , the OR = 2.4 with a confidence interval of (95% CI (1.4713 – 3.9150)) might conclude that the obese HIV participants were two and a half times (2.5x) more likely to be sedentary than the non-obese participants. 2.4 is the point estimate obtained from this example; however, the entire population of obese HIV people was not included. If other samples of HIV participants were assessed, the point estimate would likely differ. Some samples might get the point estimate of less than or some greater than 2.4.
The 95% CI is the interval representing the (population) parameter value 95% of the time if an experiment or study is repeated, in that 95 out of 100 intervals would result in the intervals containing the true risk ratio or odds ratio value. For the sedentary and obesity study, the interpretation might conclude that a 2.4 point estimate could range from a low of 1.4713 to a high of 3.9150.
The main strength of the cross-sectional design is the ability to obtain results faster. Investigators do not need to wait for outcomes to occur. Participants either have the condition or attribute at the time of data collection or not. Furthermore, there are no participant follow-ups; therefore, losing study participants during the study is not an issue.
The design’s inherent nature makes it inexpensive to conduct and can yield multiple independent (predictor) and dependent (outcome) variables ( Cummings, 2013 ). The data collected can lead to additional studies to build upon the knowledge obtained. From the example, the investigators learned that obese HIV participants were more likely to be sedentary; the next study might develop a clinical trial to determine the methods to increase activity level in this population.
A significant limitation of using this design is the inability to measure the incidence of a disease or attribute ( Wang & Cheng, 2020 ). Incidence measures the proportion of participants that develop a disease or attribute over time ( Cummings, 2013 ). In other words, investigators need a follow-up phase to determine the incidence . In continuing with the example, if investigators continued to follow the HIV participants who were obese but not sedentary, would additional time (follow-up) result in increased sedentary behavior associated with conditions secondary to aging or worsening of immune status? Unfortunately, the cross-sectional design can not answer this question.
Additionally, the prevalence of a disease or attribute is influenced by the disease’s incidence and survival or disease duration ( Alexander, 2015a ). For example, participants who live longer with a disease will have a higher likelihood of being counted ( Prevalence = # of participants with the condition at the time point/ Total # of participants in the sample ) versus those who are short-term survivors. Moreover, if treatments for a disease or attribute are improved, or the survival time-frame decreases, the disease or attribute’s prevalence will reduce ( Alexander, 2015a ). New information presented to the lay public could also influence the prevalence of a disease or attribute through lifestyle changes (i.e., increasing physical activity, improving diet) or changing jobs if the profession is associated with an identified risk or disease. Therefore, this design does not allow investigators to ascertain the events’ sequence, which came first, obesity or sedentary behavior.
For investigators studying rare diseases or conditions, the cross-sectional design is not the best fit. Cross-sectional studies often draw samples from a large and heterogeneous study population ( Wang & Cheng, 2020 ). Participants with the rare condition of interest might not be identified in the study sample.
Reporting Recommendations
A reporting guideline for cross-sectional studies is available for investigators and consumers of research to use. A reporting guideline’s primary goal is to ensure that published clinical research studies provide transparency in reporting a study’s conduct (what was done) and results. The guideline is a tool investigators can use to develop their manuscripts and offers a checklist of inclusion items for a published paper (Equator.network). The recommended items will help ensure that a reader can understand the manuscript, follow the study’s planning and how the research was conducted, the findings, and the conclusions ( von Elm et al., 2014 ).
For cross-sectional studies, the guideline is titled Strengthening the Reporting of Observational Studies in Epidemiology (STROBE) ( von Elm et al., 2014 ). The STROBE g uideline is a 22-item checklist. The checklist provides essential information for a study to be replicated, useful for healthcare professionals to make clinical decisions, and give enough information for inclusion in a systematic review ( https://www.equator-network.org/reporting-guidelines/strobe/ ).
The cross-sectional design is an appropriate method to determine the prevalence of a disease, attribute, or phenomena in a study sample. The design provides a ‘snapshot” of the sample, and investigators can describe their study sample and review associations between the collected variables (independent and dependent). The observational nature makes it relatively quick to complete a study and provides data to support future studies that might lead to methods to treat or prevent diseases or conditions.
Acknowledgments
This manuscript is supported in part by grant # UL1TR001866 from the National Center for Advancing Translational Sciences (NCATS), National Institutes of Health (NIH) Clinical and Translational Science Award (CTSA) program.
- Alexander L, Lopes B, Richetti-Masterson K, Yeatts KR. (2015a) Cross-Sectional Studies. In: Vol. Second Edition. ERIC Notebook . Durham, NC: Department of Epidemiology at the UNC Gillings School of Global Public Health. [ Google Scholar ]
- Alexander L, Lopes B, Richetti-Masterson K, Yeatts KR. (2015b) Risk and Rate Measures in Cohort Studies. In: Vol. 2nd. ERIC Notebook . Durham, NC: Department of Epidemiology at the UNC Gillings School of Global Public Health. [ Google Scholar ]
- Cummings S B. H. S. R. (Ed.) (2013). Designing Clinical Research (4th ed.). Philadelphia, PA: Wolters Kluwer/Lippincott Williams & Wilkins. [ Google Scholar ]
- Mann CJ (2012). Observational research methods—Cohort studies, cross-sectional studies, and case-control studies . African Journal of Emergency Medicine , 2 ( 1 ), 38–46. doi: 10.1016/j.afjem.2011.12.004 [ CrossRef ] [ Google Scholar ]
- Noordzij M, Dekker FW, Zoccali C, & Jager KJ (2010). Measures of disease frequency: prevalence and incidence . Nephron Clin Pract , 115 ( 1 ), c17–20. doi: 10.1159/000286345 [ PubMed ] [ CrossRef ] [ Google Scholar ]
- Tamhane AR, Westfall AO, Burkholder GA, & Cutter GR (2016). Prevalence odds ratio versus prevalence ratio: choice comes with consequences . Stat Med , 35 ( 30 ), 5730–5735. doi: 10.1002/sim.7059 [ PMC free article ] [ PubMed ] [ CrossRef ] [ Google Scholar ]
- Tenny S, & Hoffman MR (2019). Odds Ratio (OR) : StatPearls Publishing, Treasure Island (FL). [ PubMed ] [ Google Scholar ]
- von Elm E, Altman DG, Egger M, Pocock SJ, Gøtzsche PC, & Vandenbroucke JP (2014). The Strengthening the Reporting of Observational Studies in Epidemiology (STROBE) Statement: guidelines for reporting observational studies . Int J Surg , 12 ( 12 ), 1495–1499. doi: 10.1016/j.ijsu.2014.07.013 [ PubMed ] [ CrossRef ] [ Google Scholar ]
- Wang X, & Cheng Z (2020). Cross-Sectional Studies: Strengths, Weaknesses, and Recommendations . Chest , 158 ( 1 ), S65–S71. doi: 10.1016/j.chest.2020.03.012 [ PubMed ] [ CrossRef ] [ Google Scholar ]
Have a language expert improve your writing
Run a free plagiarism check in 10 minutes, automatically generate references for free.
- Knowledge Base
- Methodology
- Cross-Sectional Study | Definitions, Uses & Examples
Cross-Sectional Study | Definitions, Uses & Examples
Published on 5 May 2022 by Lauren Thomas .
A cross-sectional study is a type of research design in which you collect data from many different individuals at a single point in time. In cross-sectional research, you observe variables without influencing them.
Researchers in economics, psychology, medicine, epidemiology, and the other social sciences all make use of cross-sectional studies in their work. For example, epidemiologists who are interested in the current prevalence of a disease in a certain subset of the population might use a cross-sectional design to gather and analyse the relevant data.
Table of contents
Cross-sectional vs longitudinal studies, when to use a cross-sectional design, how to perform a cross-sectional study, advantages and disadvantages of cross-sectional studies, frequently asked questions about cross-sectional studies.
The opposite of a cross-sectional study is a longitudinal study . While cross-sectional studies collect data from many subjects at a single point in time, longitudinal studies collect data repeatedly from the same subjects over time, often focusing on a smaller group of individuals connected by a common trait.
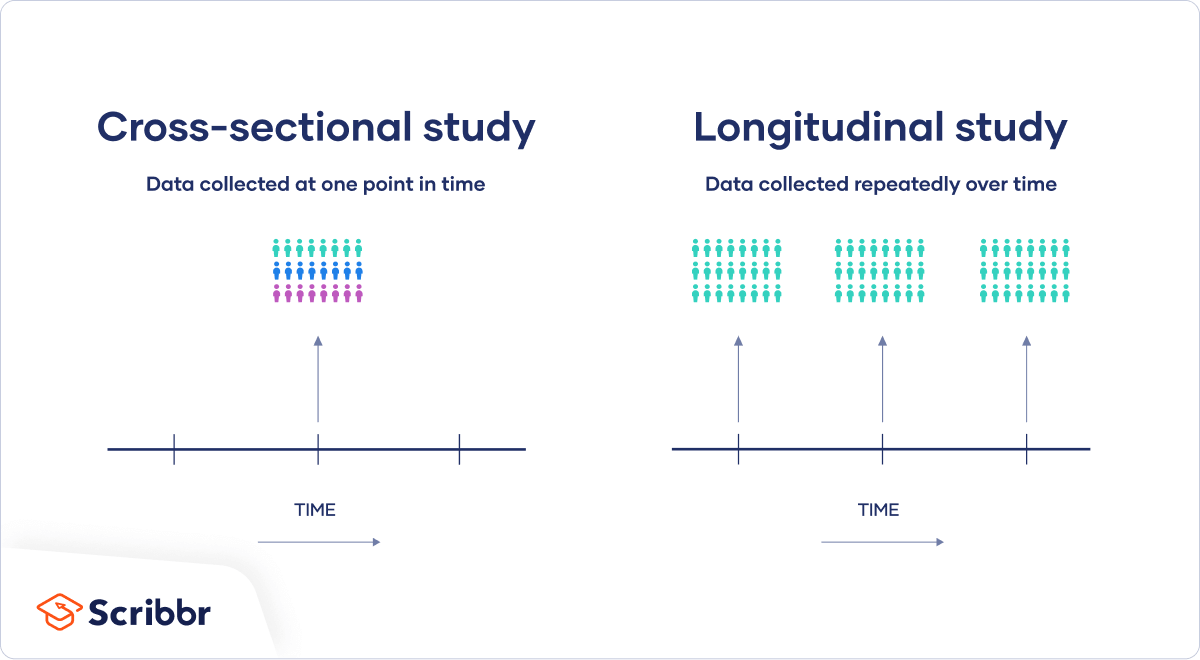
Both types are useful for answering different kinds of research questions . A cross-sectional study is a cheap and easy way to gather initial data and identify correlations that can then be investigated further in a longitudinal study.
Prevent plagiarism, run a free check.
When you want to examine the prevalence of some outcome at a certain moment in time, a cross-sectional study is the best choice.
Sometimes a cross-sectional study is the best choice for practical reasons – for instance, if you only have the time or money to collect cross-sectional data, or if the only data you can find to answer your research question were gathered at a single point in time.
As cross-sectional studies are cheaper and less time-consuming than many other types of study, they allow you to easily collect data that can be used as a basis for further research.
Descriptive vs analytical studies
Cross-sectional studies can be used for both analytical and descriptive purposes:
- An analytical study tries to answer how or why a certain outcome might occur.
- A descriptive study only summarises said outcome using descriptive statistics.
To implement a cross-sectional study, you can rely on data assembled by another source or collect your own. Governments often make cross-sectional datasets freely available online.
Prominent examples include the censuses of several countries like the US or France , which survey a cross-sectional snapshot of the country’s residents on important measures. International organisations like the World Health Organization or the World Bank also provide access to cross-sectional datasets on their websites.
However, these datasets are often aggregated to a regional level, which may prevent the investigation of certain research questions. You will also be restricted to whichever variables the original researchers decided to study.
If you want to choose the variables in your study and analyse your data on an individual level, you can collect your own data using research methods such as surveys . It’s important to carefully design your questions and choose your sample .
Like any research design , cross-sectional studies have various benefits and drawbacks.
- Because you only collect data at a single point in time, cross-sectional studies are relatively cheap and less time-consuming than other types of research.
- Cross-sectional studies allow you to collect data from a large pool of subjects and compare differences between groups.
- Cross-sectional studies capture a specific moment in time. National censuses, for instance, provide a snapshot of conditions in that country at that time.
Disadvantages
- It is difficult to establish cause-and-effect relationships using cross-sectional studies, since they only represent a one-time measurement of both the alleged cause and effect.
- Since cross-sectional studies only study a single moment in time, they cannot be used to analyse behavior over a period of time or establish long-term trends.
- The timing of the cross-sectional snapshot may be unrepresentative of behaviour of the group as a whole. For instance, imagine you are looking at the impact of psychotherapy on an illness like depression. If the depressed individuals in your sample began therapy shortly before the data collection, then it might appear that therapy causes depression even if it is effective in the long term.
Longitudinal studies and cross-sectional studies are two different types of research design . In a cross-sectional study you collect data from a population at a specific point in time; in a longitudinal study you repeatedly collect data from the same sample over an extended period of time.
Cross-sectional studies are less expensive and time-consuming than many other types of study. They can provide useful insights into a population’s characteristics and identify correlations for further research.
Sometimes only cross-sectional data are available for analysis; other times your research question may only require a cross-sectional study to answer it.
Cross-sectional studies cannot establish a cause-and-effect relationship or analyse behaviour over a period of time. To investigate cause and effect, you need to do a longitudinal study or an experimental study .
Cite this Scribbr article
If you want to cite this source, you can copy and paste the citation or click the ‘Cite this Scribbr article’ button to automatically add the citation to our free Reference Generator.
Thomas, L. (2022, May 05). Cross-Sectional Study | Definitions, Uses & Examples. Scribbr. Retrieved 29 April 2024, from https://www.scribbr.co.uk/research-methods/cross-sectional-design/
Is this article helpful?
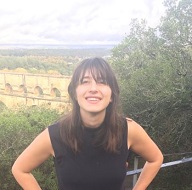
Lauren Thomas
Other students also liked, longitudinal study | definition, approaches & examples, descriptive research design | definition, methods & examples, correlational research | guide, design & examples.
- Bipolar Disorder
- Therapy Center
- When To See a Therapist
- Types of Therapy
- Best Online Therapy
- Best Couples Therapy
- Best Family Therapy
- Managing Stress
- Sleep and Dreaming
- Understanding Emotions
- Self-Improvement
- Healthy Relationships
- Student Resources
- Personality Types
- Guided Meditations
- Verywell Mind Insights
- 2023 Verywell Mind 25
- Mental Health in the Classroom
- Editorial Process
- Meet Our Review Board
- Crisis Support
How Do Cross-Sectional Studies Work?
Gathering Data From a Single Point in Time
Kendra Cherry, MS, is a psychosocial rehabilitation specialist, psychology educator, and author of the "Everything Psychology Book."
:max_bytes(150000):strip_icc():format(webp)/IMG_9791-89504ab694d54b66bbd72cb84ffb860e.jpg)
Steven Gans, MD is board-certified in psychiatry and is an active supervisor, teacher, and mentor at Massachusetts General Hospital.
:max_bytes(150000):strip_icc():format(webp)/steven-gans-1000-51582b7f23b6462f8713961deb74959f.jpg)
What Is a Cross-Sectional Study?
- Defining Characteristics
Advantages of Cross-Sectional Studies
Challenges of cross-sectional studies, cross-sectional vs. longitudinal studies.
Verywell / Jessica Olah
A cross-sectional study looks at data at a single point in time. The participants in this type of study are selected based on particular variables of interest. Cross-sectional studies are often used in developmental psychology , but this method is also used in many other areas, including social science and education.
Cross-sectional studies are observational in nature and are known as descriptive research, not causal or relational, meaning that you can't use them to determine the cause of something, such as a disease. Researchers record the information that is present in a population, but they do not manipulate variables .
This type of research can be used to describe characteristics that exist in a community, but not to determine cause-and-effect relationships between different variables. This method is often used to make inferences about possible relationships or to gather preliminary data to support further research and experimentation.
Example: Researchers studying developmental psychology might select groups of people who are different ages but investigate them at one point in time. By doing this, any differences among the age groups can be attributed to age differences rather than something that happened over time.
Defining Characteristics of Cross-Sectional Studies
Some of the key characteristics of a cross-sectional study include:
- The study takes place at a single point in time
- It does not involve manipulating variables
- It allows researchers to look at numerous characteristics at once (age, income, gender, etc.)
- It's often used to look at the prevailing characteristics in a given population
- It can provide information about what is happening in a current population
Think of a cross-sectional study as a snapshot of a particular group of people at a given point in time. Unlike longitudinal studies, which look at a group of people over an extended period, cross-sectional studies are used to describe what is happening at the present moment.This type of research is frequently used to determine the prevailing characteristics in a population at a certain point in time. For example, a cross-sectional study might be used to determine if exposure to specific risk factors might correlate with particular outcomes.
A researcher might collect cross-sectional data on past smoking habits and current diagnoses of lung cancer, for example. While this type of study cannot demonstrate cause and effect, it can provide a quick look at correlations that may exist at a particular point.
For example, researchers may find that people who reported engaging in certain health behaviors were also more likely to be diagnosed with specific ailments. While a cross-sectional study cannot prove for certain that these behaviors caused the condition, such studies can point to a relationship worth investigating further.
Cross-sectional studies are popular because they have several benefits that are useful to researchers.
Inexpensive and Fast
Cross-sectional studies typically allow researchers to collect a great deal of information quickly. Data is often obtained inexpensively using self-report surveys . Researchers are then able to amass large amounts of information from a large pool of participants.
For example, a university might post a short online survey about library usage habits among biology majors, and the responses would be recorded in a database automatically for later analysis. This is a simple, inexpensive way to encourage participation and gather data across a wide swath of individuals who fit certain criteria.
Can Assess Multiple Variables
Researchers can collect data on a few different variables to see how they affect a certain condition. For example, differences in sex, age, educational status, and income might correlate with voting tendencies or give market researchers clues about purchasing habits.
Might Prompt Further Study
Although researchers can't use cross-sectional studies to determine causal relationships, these studies can provide useful springboards to further research. For example, when looking at a public health issue, such as whether a particular behavior might be linked to a particular illness, researchers might utilize a cross-sectional study to look for clues that can spur further experimental studies.
For example, researchers might be interested in learning how exercise influences cognitive health as people age. They might collect data from different age groups on how much exercise they get and how well they perform on cognitive tests. Conducting such a study can give researchers clues about the types of exercise that might be most beneficial to the elderly and inspire further experimental research on the subject.
No method of research is perfect. Cross-sectional studies also have potential drawbacks.
Difficulties in Determining Causal Effects
Researchers can't always be sure that the conditions a cross-sectional study measures are the result of a particular factor's influence. In many cases, the differences among individuals could be attributed to variation among the study subjects. In this way, cause-and-effect relationships are more difficult to determine in a cross-sectional study than they are in a longitudinal study. This type of research simply doesn't allow for conclusions about causation.
For example, a study conducted some 20 years ago queried thousands of women about their consumption of diet soft drinks. The results of the study, published in the medical journal Stroke , associated diet soft drink intake with stroke risk that was greater than that of those who did not consume such beverages. In other words, those who drank lots of diet soda were more prone to strokes. However, correlation does not equal causation. The increased stroke risk might arise from any number of factors that tend to occur among those who drink diet beverages. For example, people who consume sugar-free drinks might be more likely to be overweight or diabetic than those who drink the regular versions. Therefore, they might be at greater risk of stroke—regardless of what they drink.
Cohort Differences
Groups can be affected by cohort differences that arise from the particular experiences of a group of people. For example, individuals born during the same period might witness the same important historical events, but their geographic regions, religious affiliations, political beliefs, and other factors might affect how they perceive such events.
Report Biases
Surveys and questionnaires about certain aspects of people's lives might not always result in accurate reporting. For example, respondents might not disclose certain behaviors or beliefs out of embarrassment, fear, or other limiting perception. Typically, no mechanism for verifying this information exists.
Cross-sectional research differs from longitudinal studies in several important ways. The key difference is that a cross-sectional study is designed to look at a variable at a particular point in time. A longitudinal study evaluates multiple measures over an extended period to detect trends and changes.
Evaluates variable at single point in time
Participants less likely to drop out
Uses new participant(s) with each study
Measures variable over time
Requires more resources
More expensive
Subject to selective attrition
Follows same participants over time
Longitudinal studies tend to require more resources; these are often more expensive than those used by cross-sectional studies. They are also more likely to be influenced by what is known as selective attrition , which means that some individuals are more likely to drop out of a study than others. Because a longitudinal study occurs over a span of time, researchers can lose track of subjects. Individuals might lose interest, move to another city, change their minds about participating, etc. This can influence the validity of the study.
One of the advantages of cross-sectional studies is that data is collected all at once, so participants are less likely to quit the study before data is fully collected.
A Word From Verywell
Cross-sectional studies can be useful research tools in many areas of health research. By learning about what is going on in a specific population, researchers can improve their understanding of relationships among certain variables and develop additional studies that explore these conditions in greater depth.
Levin KA. Study design III: Cross-sectional studies . Evid Based Dent . 2006;7(1):24-5. doi:10.1038/sj.ebd.6400375
Morin JF, Olsson C, Atikcan EO, eds. Research Methods in the Social Sciences: An A-Z of Key Concepts . Oxford University Press; 2021.
Abbasi J. Unpacking a recent study linking diet soda with stroke risks . JAMA . 2019;321(16):1554-1555. doi:10.1001/jama.2019.2123
Setia MS. Methodology series module 3: Cross-sectional studies . Indian J Dermatol . 2016;61(3):261-4. doi:10.4103/0019-5154.182410
By Kendra Cherry, MSEd Kendra Cherry, MS, is a psychosocial rehabilitation specialist, psychology educator, and author of the "Everything Psychology Book."
User Preferences
Content preview.
Arcu felis bibendum ut tristique et egestas quis:
- Ut enim ad minim veniam, quis nostrud exercitation ullamco laboris
- Duis aute irure dolor in reprehenderit in voluptate
- Excepteur sint occaecat cupidatat non proident
Keyboard Shortcuts
9.3 - example 9-1: population-based cohort or a cross-sectional studies, example 9-1 section .
Suppose you are interested in the question: "Does one group have a prevalence percentage that is different than other groups?" For example:
Baseline prevalence of smoking in a particular community is 30%. A clean indoor air policy goes into effect. What is the sample size required to detect a decrease in smoking prevalence of at least 2 percentage points? \(\alpha=0.05\); 90% power.
We are interested in testing the following hypothesis:
Null hypothesis:
\(H_0\colon \text{prevalence}_{(Before)}\le \text{prevalence}_{(After)}\)
Alternative hypothesis:
\(H_A\colon \text{prevalence}_{(Before)}- \text{prevalence}_{(After)}=\delta\)
Where \(\delta \gt 0\)
The resulting formula for the sample size for testing a difference in prevalence using a one-sided test is as follows:
and for this example, n can be calculated as:
\(n=\dfrac{1}{d^{2}}\left [ z_{\alpha }\sqrt{\pi_{0}(1-\pi_{0})}+z_{\beta }\sqrt{\pi_{1}(1-\pi_{1})} \right ]^{2}\)
Replace \(z_{\alpha }\) by \(z_{\alpha/2 }\) for a two-sided test
Take a moment to look at the table below for sample size requirements for testing the value of a single proportion with a one-sided test. Prevalence can be found along the top of the table and the percentage point difference vertically on the left. How many individuals do we need to include in our study in order to meet the above criteria?
(Tables from Woodward, M. Epidemiology Study Design and Analysis . Boca Raton: Chapman and Hall:, 1999 )
Table B.8. Sample size requirements for testing the value of a single proportion
Need a hint?
Try It! Section
- Prevalence increases (\(B_0\))? Does the sample size increase or decrease?
- What happens to the sample size as effect size decreases?
- What is the minimal detectable difference if you had funds for 1,500 subjects?
- The largest sample sizes occur with baseline prevalence at 0.5
- The smaller the effect size, the larger the sample size
- About 3.6% decrease in prevalence
13. Study design and choosing a statistical test
Sample size.

Repeated Cross-Sectional Data Analysis
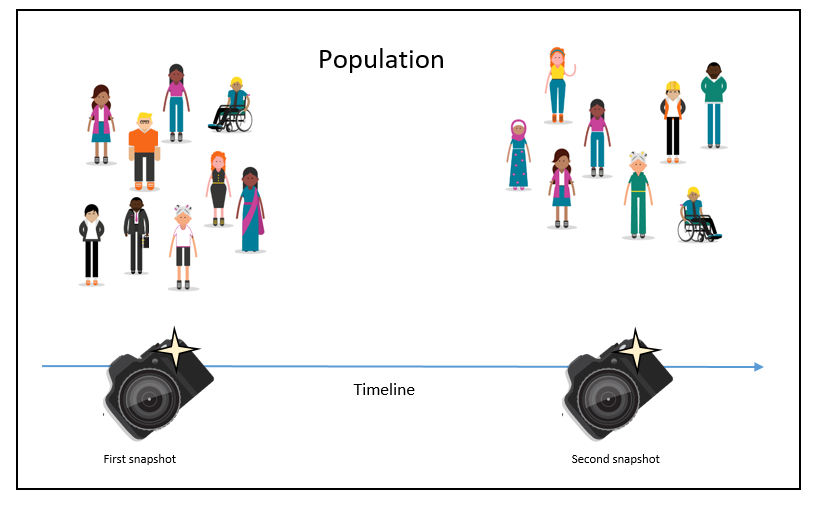
Which clinical questions does a Cross-Sectional study best answer?
Please note the Introduction , where there is a table under "Which study type will answer my clinical question?" . You may find that there are only one or two question types that your study answers – that’s ok.
Cross-sectional study designs are useful when:
- Answering questions about the incidence or prevalence of a condition, belief or situation.
- Establishing what the norm is for a specific demographic at a specific time. For example: what is the most common or normal age for students completing secondary education in Victoria?
- Justifying further research on a topic. Cross-sectional studies can infer a relationship or correlation but are not always sufficient to determine a direct cause. As a result, these studies often pave the way for other investigations.
What are the advantages and disadvantages to consider when using a Cross-Sectional study design?
What does a strong cross-sectional study look like.
- Appropriate recruitment of participants. The sample of participants must be an accurate representation of the population being measured.
- Sample size. As is the case for most study types a larger sample size gives greater power and is more ideal for a strong study design. Within a cross-sectional study a sample size of at least 60 participants is recommended, although this will depend on suitability to the research question and the variables being measured.
- A suitable number of variables. Cross-sectional studies ideally measure at least three variables in order to develop a well-rounded understanding of the potential relationships of the two key conditions being measured.
What are the pitfalls to look for?
Cross-sectional studies are at risk of participation bias, or low response rates from participants. If a large number of surveys are sent out and only a quarter are completed and returned then this becomes an issue as those who responded may not be a true representation of the overall population.
Critical appraisal tools
To assist with critically appraising cross-sectional studies there are some tools / checklists you can use.
- Axis Appraisal Tool for Cross Sectional Studies
- Critical Appraisal Tool for Cross- Sectional Studies (CAT-CSS)
- Critical Appraisal of a Cross-Sectional Study on Environmental Health
- Critical appraisal tool for cross-sectional studies using biomarker data (BIOCROSS)
- CEBM Critical Appraisal of a Cross-Sectional Study (Survey)
- JBI Critical Appraisal checklist for analytical cross-sectional studies
- Specialist Unit for Review Evidence (SURE) 2018. Questions to assist with the critical appraisal of cross sectional studies
- STROBE Checklist for cross-sectional studies
Real World Examples
The Australian National Survey of Mental Health and Wellbeing (NSMHWB)
https://www.abs.gov.au/statistics/health/mental-health/national-survey-mental-health-and-wellbeing-summary-results/2007
A widely known example of cross-sectional study design, the Australian National Survey of Mental Health and Wellbeing (NSMHWB). This study was a national epidemiological survey of mental disorders investigating the questions: How many people meet DSM-IV and ICD-10 diagnostic criteria for the major mental disorders? How disabled are they by their mental disorders? And, how many have seen a health professional for their mental disorder?
References and Further Reading
Australian Government Department of Health. (2003). The Australian National Survey of Mental Health and Wellbeing (NSMHWB). 2019, from https://www.abs.gov.au/statistics/health/mental-health/national-survey-mental-health-and-wellbeing-summary-results/2007
Bowers, D. a., Bewick, B., House, A., & Owens, D. (2013). Understanding clinical papers (Third edition. ed.): Wiley Blackwell.
Gravetter, F. J. a., & Forzano, L.-A. B. (2012). Research methods for the behavioral sciences (Fourth edition. ed.): Wadsworth Cengage Learning.
Greenhalgh, T. a. (2014). How to read a paper : the basics of evidence-based medicine (Fifth edition. ed.): John Wiley & Sons Inc.
Hoffmann, T. a., Bennett, S. P., & Mar, C. D. (2017). Evidence-Based Practice Across the Health Professions (Third edition. ed.): Elsevier.
Howitt, D., & Cramer, D. (2008). Introduction to research methods in psychology (Second edition. ed.): Prentice Hall.
Kelly, P. J., Kyngdon, F., Ingram, I., Deane, F. P., Baker, A. L., & Osborne, B. A. (2018). The Client Satisfaction Questionnaire‐8: Psychometric properties in a cross‐sectional survey of people attending residential substance abuse treatment. Drug and Alcohol Review, 37(1), 79-86. doi: 10.1111/dar.12522
Lawrence, D., Hancock, K. J., & Kisely, S. (2013). The gap in life expectancy from preventable physical illness in psychiatric patients in Western Australia: retrospective analysis of population based registers. BMJ: British Medical Journal, 346(7909), 13-13.
Nasir, B. F., Toombs, M. R., Kondalsamy-Chennakesavan, S., Kisely, S., Gill, N. S., Black, E., Ranmuthugala, G., Ostini, R., Nicholson, G. C., Hayman, N., & Beccaria, G.. (2018). Common mental disorders among Indigenous people living in regional, remote and metropolitan Australia: A cross-sectional study. BMJ Open , 8 (6). https://doi.org/10.1136/bmjopen-2017-020196
Robson, C., & McCartan, K. (2016). Real world research (Fourth Edition. ed.): Wiley.
Sedgwick, P. (2014). Cross sectional studies: advantages and disadvantages. BMJ : British Medical Journal, 348, g2276. doi: 10.1136/bmj.g2276
Setia, M. S. (2016). Methodology Series Module 3: Cross-sectional Studies. Indian journal of dermatology, 61(3), 261-264. doi: 10.4103/0019-5154.182410
Shafiei, T., Biggs, L. J., Small, R., McLachlan, H. L., & Forster, D. A. (2018). Characteristics of women calling the panda perinatal anxiety & depression australia national helpline: A cross-sectional study. Archives of Women's Mental Health. doi: 10.1007/s00737-018-0868-4
Van Heyningen, T., Honikman, S., Myer, L., Onah, M. N., Field, S., & Tomlinson, M. (2017). Prevalence and predictors of anxiety disorders amongst low-income pregnant women in urban South Africa: a cross-sectional study. Archives of Women's Mental Health(6), 765. doi: 10.1007/s00737-017-0768-z
Vogt, W. P. (2005). Dictionary of statistics & methodology : a nontechnical guide for the social sciences (Third edition. ed.): Sage Publications.
- << Previous: Case Control
- Next: Case Studies/ Case Report/ Case Series >>
- Last Updated: Feb 29, 2024 4:49 PM
- URL: https://deakin.libguides.com/quantitative-study-designs
- Open access
- Published: 23 April 2024
Effect of intravitreal injections due to neovascular age-related macular degeneration on retinal nerve fiber layer thickness and minimum rim width: a cross sectional study
- Agnes Boltz 1 , 2 ,
- Tanja Spöttl 1 , 2 ,
- Wolfgang Huf 3 , 4 ,
- Birgit Weingessel 1 , 2 &
- Veronika Pia Vécsei-Marlovits 1 , 2
BMC Ophthalmology volume 24 , Article number: 185 ( 2024 ) Cite this article
130 Accesses
Metrics details
The present study tested the hypothesis that repeated anti-VEGF injections are associated with reduced retinal nerve fiber layer (RNFL) and minimum rim width (MRW) of the optic nerve head.
Patients and methods
Sixty-six patients with a history of intravitreal injections due to neovascular age-related macular degeneration were included. RNFL and MRW were measured using optical coherence tomography (Spectralis OCT, Heidelberg Engineering, Heidelberg, Germany).
Mean global RNFL was 90.62 μm and both RNFL as well as MRW significantly decreased with advanced age ( p = 0.005 and p = 0.019, respectively). Correlating for the number of injections, no significant impact on RNFL was found globally ( p = 0.642) or in any of the sectors. In contrast, however, global MRW was significantly reduced with increasing numbers of intravitreal injections ( p = 0.012). The same holds true when adjusted for the confounding factor age (RNFL p = 0.566 and MRW p = 0.023).
Our study shows that repeated intravitreal injections due to choroidal neovascularization seem to have a deleterious effect on MRW but not on RNFL. This suggests that MRW is a more sensitive marker than RNFL for evaluating the effect of frequent intravitreal injections on the optic nerve head since it seems to be the first structure affected.
Peer Review reports
Around 15 years ago, the introduction and utilization of intravitreal injections of anti-vascular endothelial growth factor (anti-VEGF) antibodies has tremendously enhanced the treatment options and visual outcome for several eye diseases associated with macular edema, such as neovascular age-related macular degeneration (n-AMD), retinal vein occlusion, and diabetic retinopathy [ 1 , 2 , 3 , 4 , 5 , 6 , 7 , 8 , 9 ]. In general, anti-VEGF injections are considered safe and the benefits outweigh by far the possible ocular complications [ 10 ]. However, unlike laser therapy, patients usually have to undergo frequent reinjections each year to remain visually and morphologically stable. Subsequently, the risk of adverse events may be increased by the continuous long-term. As one would assume, the loading of additional fluid into the eyeball can raise intraocular pressure (IOP). Therefore, several studies were conducted investigating IOP following anti-VEGF injections [ 11 , 12 , 13 ]. A meta-analysis of 46 studies on this topic showed that IOP was significantly increased for all measured time-intervals of the day of injection, but slightly decreased on the day after injection and returned to normal thereafter [ 14 ]. These IOP fluctuations along with possible fluctuations in blood perfusion of the optic nerve head may lead to glaucoma over time.
The introduction of Optical Coherence Tomography (OCT) made it possible to detect early structural changes and at the same time deliver objective parameters in contrast to perimetry. In addition to a thinning of peripapillary retinal nerve fiber layer thickness (RNFL), another OCT parameter, i.e. Bruch’s membrane opening minimum rim width (MRW) has been established more recently for the assessment of optic discs. Hence, the present study tested the hypothesis that repeated anti-VEGF injections are associated with reduced RNFL and MRW of the optic nerve head.
Materials and methods
This cross sectional study was conducted after approval from the Ethics Committee of the City of Vienna had been obtained (EK 20-352-VK) and adhered to the tenets of the Declaration of Helsinki. Due to the retrospective character of the study, informed consent was waived in agreement with the positive vote of the above-mentioned Ethics Committee. Patients with a history of intravitreal injections due to neovascular AMD were included. Intravitreal injections with anti-VEGF were applied without paracentesis and according to the pro-re-nata regimen. RNFL and MRW were measured using optical coherence tomography (Spectralis OCT, Heidelberg Engineering, Heidelberg, Germany) and analyzed using the build in software (Heyex 2, Figs. 1 and 2 ) with included age-matched reference values. If necessary, manual corrections of retinal nerve fiber layer segmentation and Bruch’s membrane opening were undertaken by a trained physician. Exclusion criteria were presence of uncontrolled elevated IOP over 21mmHg prior to injections, a history of ischemic opticus atrophy, diagnosis of glaucoma prior to anti-VEGF treatment as well as presence of a clinically significant epiretinal membrane and high myopia. Statistical analysis and linear regression models were carried out in R (version 4.0.3, R Foundation for Statistical Computing, Vienna, Austria).
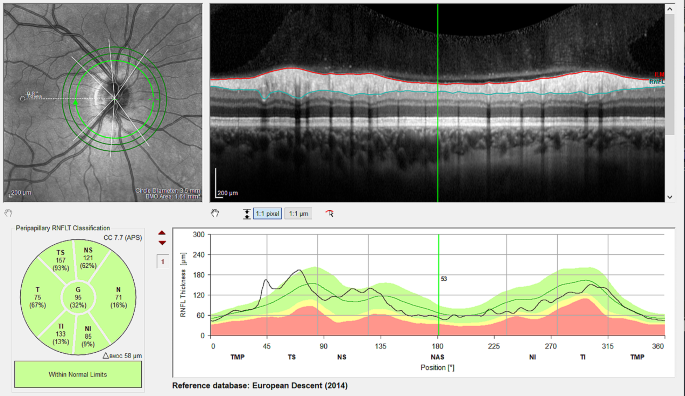
Exemplary retinal nerve fiber layer (RNFL) measurement
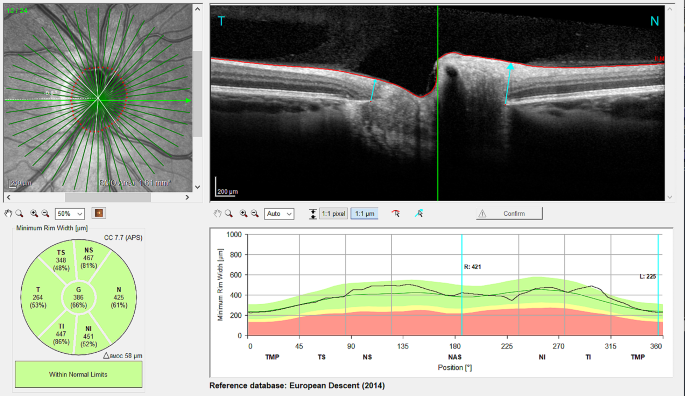
Exemplary minimum rim width (MRW) measurement
Sixty-six eyes of patients with CNV and a mean age of 83.4 years and an average of 12.58 prior injections were included (Fig. 3 ).
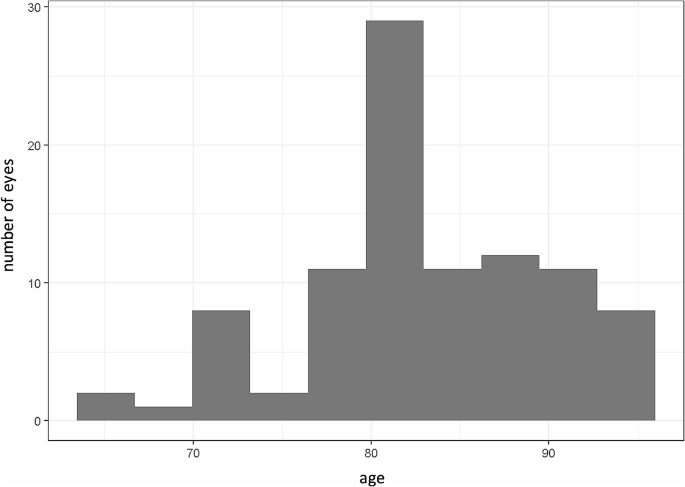
Age distribution and number of eyes
Mean global RNFL was 90.62 μm and significantly decreased with advanced age ( p = 0.005, Fig. 4 ).
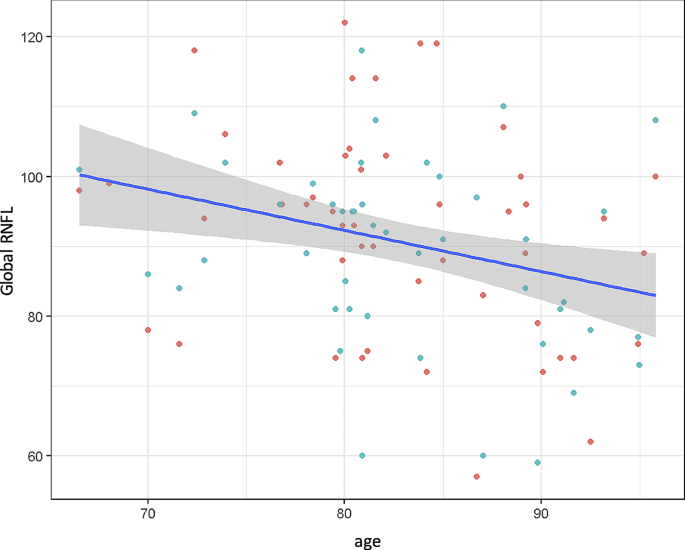
Global retinal nerve fiber layer (RNFL) by age. Green dots represent right eyes, red dots represent left eyes; p = 0.005
Similarly, RNFL of the nasal-superior ( p = 0.025), temporal-superior ( p < 0.001), temporal-inferior ( p = 0.004) sectors highly significantly correlated with age as well, whereas this did not hold true for the nasal ( p = 0.237), the temporal ( p = 0.216), and the nasal-inferior ( p = 0.862) sector. Advanced age was also significantly associated with lower global MRW ( p = 0.019, Fig. 5 ), nasal-superior ( p = 0.020), temporal-superior ( p = 0.014), temporal ( p = 0.004), and temporal-inferior ( p = 0.019) MRW, but not with nasal ( p = 0.272) and nasal-inferior ( p = 0.103) MRW.
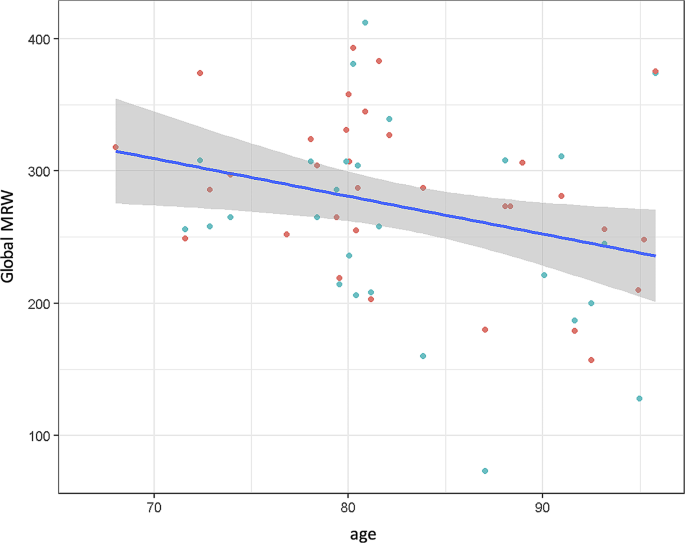
Global minimum rim width (MRW) by age. Green dots represent right eyes, red dots represent left eyes; p = 0.019
Correlating for the number of injections, no significant impact on RNFL was found globally ( p = 0.642, Fig. 6 ) or in any of the sectors.
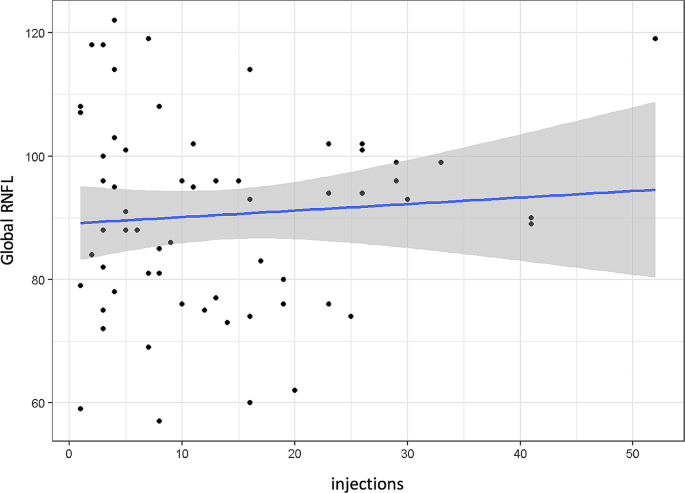
Global retinal nerve fiber layer (RNFL) by total number of intravitreal injections; p = 0.642
In contrast, however, global MRW was significantly reduced with increasing number of intravitreal injections ( p = 0.012, Fig. 7 ).
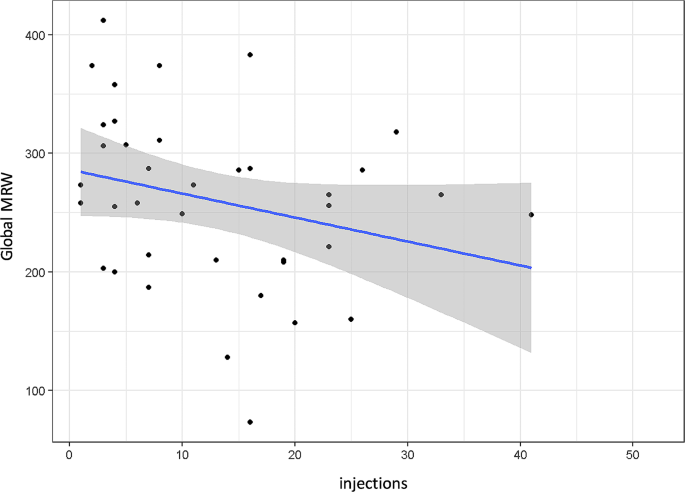
Global minimum rim width (MRW) by total number of intravitreal injections; p = 0.012
Adjusting for the confounding factor age, the level of significance neither changed for RNFL ( p = 0.566, Fig. 8 ) nor for MRW ( p = 0.023, Fig. 9 ).
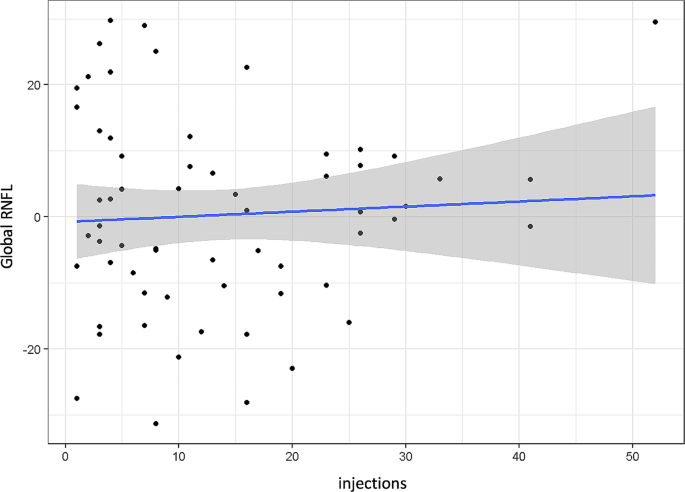
Global retinal nerve fiber layer (RNFL) adjusted for age (residuals) and grouped by total number of intravitreal injections; p = 0.566
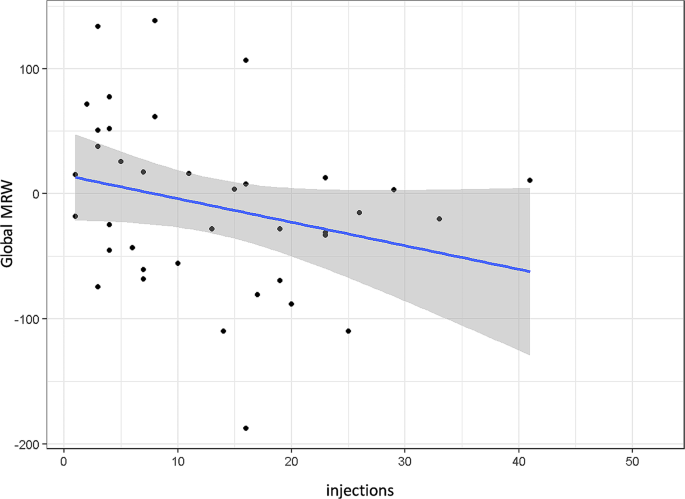
Global minimum rim width (MRW) adjusted for age (residuals) and grouped by total number of intravitreal injections; p = 0.023
Looking at the MRW sectors individually, the temporal ( p = 0.011), and the temporal-inferior ( p = 0.044) sector also significantly correlated with number of injections, whereas the other sectors did not (nasal p = 0.079; nasal-superior p = 0.099; temporal-superior p = 0.065; nasal-inferior p = 0.141).
Our study shows that repeated intravitreal injections due to choroidal neovascularization seem to have a deleterious effect on MRW but not on RNFL. The latter is in accordance with the majority of previous studies conducted on this topic [ 14 , 15 , 16 , 17 , 18 , 19 , 20 , 21 , 22 ]. However, two studies by Parlak et al. and Martinez-de-la-Casa et al. found a significant thinning of RNFL after administration of ranibizumab in patients with CNV [ 23 , 24 ]. The former study found a significant reduction in RNFL in both, the treatment arm as well as the untreated control arm with dry AMD at follow up, but no significant difference between both groups which questions the hypothesis that anti-VEGF injections caused this effect. The latter study only included treatment naïve patients, and as such they showed increased macular thickness, especially in the nasal quadrant, which was significantly reduced after treatment. One can speculate that the reduction of fluid in the nasal macula may also have an impact on RNFL measurements especially given that most reduction happened in the temporal sector of the optic nerve head as well as at first follow-up at 3 months and did not significantly change thereafter over the course of 12 months [ 24 ]. This effect may also explain the findings of another study that associated a thickening on the temporal RNFL quadrant to repeated anti-VEGF injections [ 25 ].
Limitations of our own study are the lack of axial eye length measurements which may have an effect on MRW analysis. We tried to minimize this by excluding patients with high myopia.
The lack of effect on RNFL in our study may be attributed to the average number of injections of 12.58 prior to inclusion, and RNFL thinning possibly presents itself just after a longer period of treatment which may also explain the results of the afore mentioned studies mainly in treatment naïve patients. Whether this can only be seen after a higher number of injections (> 30 and more) as suggested by a cross-sectional paper [ 26 ] remains to be seen in further studies.
To the best of our knowledge, only one other study investigated the effect of repeated intravitreal injections on other biomarkers of the optic nerve head, such as Bruch’s membrane opening (BMO) [ 27 ]. In the study conducted in 29 patients with CNV, diabetic edema, and retinal vein occlusion, a significant increase in BMO was found immediately, i.e. 5 min after each of the first 3 anti-VEGF injections, but this effect did not seem to persist after 12 months. In accordance with our paper, they found no negative effect on RNFL.
The mechanisms underlying the different response of MRW and RNFL to anti-VEGF injections are not clearly understood. However, recent papers have suggested that the rate of change in MRW is significantly greater than RNFL in patients with glaucoma over the course of disease and thus per se a more sensitive biomarker [ 28 , 29 ].
Since IOP fluctuations after intravitreal injections are only temporary, one also needs to take other potential pathways of optic nerve head damage into account. As such, blood perfusion has been shown to play a critical role in the pathogenesis of glaucoma [ 30 ]. VEGF can induce the release of nitric oxide [ 31 ] and thereby improve blood flow. Another mechanism may be that VEGF seems to have a neuroprotective effect [ 32 , 33 ]. Therefore, VEGF inhibition potentially leads to both lower perfusion of the optic nerve had and to limited neuroprotection.
In conclusion, our study suggests that MRW is a more sensitive marker than RNFL for evaluating the effect of frequent intravitreal injections on the optic nerve head since it seems to be the first structure affected. However, further longitudinal studies are warranted to widen our understanding of the potential role of anti-VEGF injections in the pathogenesis of glaucoma.
Data availability
The datasets used and/or analysed during the current study are available from the corresponding author on reasonable request.
Rosenfeld PJ, Brown DM, Heier JS, et al. Ranibizumab for neovascular age-related macular degeneration. N Engl J Med. 2006;355(14):1419–31.
Article CAS PubMed Google Scholar
Brown DM, Kaiser PK, Michels M, et al. Ranibizumab versus Verteporfin for neovascular age-related macular degeneration. N Engl J Med. 2006;355(14):1432–44.
CATT Research Group, Martin DF, Maguire MG, et al. Ranibizumab and Bevacizumab for neovascular age-related macular degeneration. N Engl J Med. 2011;364(20):1897–908.
Article Google Scholar
Heier JS, Brown DM, Chong V et al. Intravitreal aflibercept (VEGF trap-eye) in wet age-related macular degeneration [published correction appears in Ophthalmology. 2013;120(1):209– 10]. Ophthalmology . 2012;119(12):2537–2548.
Kornhauser T, Schwartz R, Goldstein M, Neudorfer M, Loewenstein A, Barak A. Bevacizumab treatment of macular edema in CRVO and BRVO: long-term follow-up. (BERVOLT study: Bevacizumab for RVO long-term follow-up). Graefes Arch Clin Exp Ophthalmol. 2016;254(5):835–44.
Tadayoni R, Waldstein SM, Boscia F, et al. Individualized stabilization criteria-driven ranibizumab versus laser in Branch Retinal vein occlusion: six-Month results of BRIGHTER. Ophthalmology. 2016;123(6):1332–44.
Article PubMed Google Scholar
Nguyen QD, Brown DM, Marcus DM, et al. Ranibizumab for diabetic macular edema: results from 2 phase III randomized trials: RISE and RIDE. Ophthalmology. 2012;119(4):789–801.
Clark WL, Boyer DS, Heier JS, et al. Intravitreal Aflibercept for Macular Edema following branch retinal vein occlusion: 52-Week results of the VIBRANT study. Ophthalmology. 2016;123(2):330–6.
Schmidt-Erfurth U, Garcia-Arumi J, Bandello F, et al. Guidelines for the management of Diabetic Macular Edema by the European Society of Retina Specialists (EURETINA). Ophthalmologica. 2017;237(4):185–222.
Falavarjani KG, Nguyen QD. Adverse events and complications associated with intravitreal injection of anti-VEGF agents: a review of literature. Eye (Lond). 2013;27(7):787–94.
Hollands H, Wong J, Bruen R, Campbell RJ, Sharma S, Gale J. Short-term intraocular pressure changes after intravitreal injection of bevacizumab. Can J Ophthalmol. 2007;42(6):807–11.
Lemos V, Cabugueira A, Noronha M, et al. Intraocular pressure in eyes receiving Intravitreal Antivascular endothelial growth factor injections. Ophthalmologica. 2015;233(3–4):162–8.
Lee JW, Park H, Choi JH, et al. Short-term changes of intraocular pressure and ocular perfusion pressure after intravitreal injection of bevacizumab or ranibizumab. BMC Ophthalmol. 2016;16:69. Published 2016 May 31.
Article PubMed PubMed Central Google Scholar
de Vries VA, Bassil FL, Ramdas WD. The effects of intravitreal injections on intraocular pressure and retinal nerve fiber layer: a systematic review and meta-analysis. Sci Rep. 2020;10(1):13248. Published 2020 Aug 6.
Demirel S, Batioğlu F, Özmert E, Erenler F. The effect of multiple injections of ranibizumab on retinal nerve fiber layer thickness in patients with age-related macular degeneration. Curr Eye Res. 2015;40(1):87–92.
Valverde-Megías A, Ruiz-Calvo A, Murciano-Cespedosa A, Hernández-Ruiz S, Martínez-de-la-Casa JM, García-Feijoo J. Long-term effect of intravitreal ranibizumab therapy on retinal nerve fiber layer in eyes with exudative age-related macular degeneration. Graefes Arch Clin Exp Ophthalmol. 2019;257(7):1459–66.
Sobacı G, Güngör R, Ozge G. Effects of multiple intravitreal anti-VEGF injections on retinal nerve fiber layer and intraocular pressure: a comparative clinical study. Int J Ophthalmol. 2013;6(2):211–5. Published 2013 Apr 18.
PubMed PubMed Central Google Scholar
Shin HJ, Shin KC, Chung H, Kim HC. Change of retinal nerve fiber layer thickness in various retinal diseases treated with multiple intravitreal antivascular endothelial growth factor. Invest Ophthalmol Vis Sci. 2014;55(4):2403–11. Published 2014 Apr 15.
Ahn J, Jang K, Sohn J, Park JI, Hwang DD. Effect of intravitreal ranibizumab and aflibercept injections on retinal nerve fiber layer thickness. Sci Rep. 2021;11(1):5010. Published 2021 Mar 3.
Article CAS PubMed PubMed Central Google Scholar
Zivkovic M, Radosavljevic A, Zlatanovic M, et al. Influence of multiple Anti-VEGF injections on retinal nerve Fiber layer and Ganglion Cell-Inner Plexiform Layer Thickness in patients with exudative age-related Macular Degeneration. Med (Kaunas). 2023;59(1):138. Published 2023 Jan 10.
Google Scholar
Jun SY, Hwang DD. Short-term effect of intravitreal brolucizumab injections in patients with neovascular age-related macular degeneration on retinal nerve fiber layer thickness. Sci Rep . 2023;13(1):6685. Published 2023 Apr 24. https://doi.org/10.1038/s41598-023-32024-6 .
Wichrowska M, Goździewska E, Kocięcki J. The safety of Anti-VEGF treatment, in the context of the retinal nerve Fibre Layer, in patients with wet age-related Macular Degeneration: a review. Front Biosci (Landmark Ed). 2023;28(9):222.
Parlak M, Oner FH, Saatci AO. The long-term effect of intravitreal ranibizumab on retinal nerve fiber layer thickness in exudative age-related macular degeneration. Int Ophthalmol. 2015;35(4):473–80.
Martinez-de-la-Casa JM, Ruiz-Calvo A, Saenz-Frances F, et al. Retinal nerve fiber layer thickness changes in patients with age-related macular degeneration treated with intravitreal ranibizumab. Invest Ophthalmol Vis Sci. 2012;53(10):6214–8. Published 2012 Sep 14.
Yau GL, Campbell RJ, Li C, Sharma S. Peripapillary RNFL thickness in nonexudative versus chronically treated exudative age-related macular degeneration. Can J Ophthalmol. 2015;50(5):345–9.
Wang L, Swaminathan SS, Yang J, et al. Dose-response relationship between Intravitreal injections and retinal nerve Fiber layer thinning in Age-Related Macular Degeneration. Ophthalmol Retina. 2021;5(7):648–54.
Gómez-Mariscal M, Puerto B, Muñoz-Negrete FJ, de Juan V, Rebolleda G. Acute and chronic optic nerve head biomechanics and intraocular pressure changes in patients receiving multiple intravitreal injections of anti-VEGF. Graefes Arch Clin Exp Ophthalmol. 2019;257(10):2221–31.
Cho HK, Kee C. Rate of change in Bruch’s membrane opening-minimum Rim Width and Peripapillary RNFL in early normal tension Glaucoma. J Clin Med. 2020;9(8):2321.
Choi HS, Joo CW, Park SP, Na KI. A decrease in Bruch’s membrane opening-minimum Rim Area precedes decreased retinal nerve Fiber layer thickness and visual field loss in Glaucoma. J Glaucoma. 2021;30(12):1033–8.
Flammer J, Orgül S, Costa VP, et al. The impact of ocular blood flow in glaucoma. Prog Retin Eye Res. 2002;21(4):359–93.
Hood JD, Meininger CJ, Ziche M, Granger HJ. VEGF upregulates ecNOS message, protein, and NO production in human endothelial cells. Am J Physiol. 1998;274(3):H1054–8.
CAS PubMed Google Scholar
Nishijima K, Ng YS, Zhong L, et al. Vascular endothelial growth factor-A is a survival factor for retinal neurons and a critical neuroprotectant during the adaptive response to ischemic injury. Am J Pathol. 2007;171(1):53–67.
Zachary I. Neuroprotective role of vascular endothelial growth factor: signalling mechanisms, biological function, and therapeutic potential. Neurosignals. 2005;14(5):207–21.
Download references
Acknowledgements
Not applicable.
The authors declare that no funds, grants, or other support were received during the preparation of this manuscript.
Author information
Authors and affiliations.
Department of Ophthalmology, Hietzing Hospital, Wolkersbergenstraße 1, Vienna, 1130, Austria
Agnes Boltz, Tanja Spöttl, Birgit Weingessel & Veronika Pia Vécsei-Marlovits
Karl-Landsteiner Institute for Process Optimization and Quality Management in Cataract Surgery, Vienna, Austria
Department of Laboratory Medicine, Hietzing Hospital, Vienna, Austria
Wolfgang Huf
Karl Landsteiner Institute for Clinical Risk Management, Vienna, Austria
You can also search for this author in PubMed Google Scholar
Contributions
A.B. was primary responsible for the study design, protocol and manuscript.T.S. was responsible for protocol and manuscript.W.H. was responsible for statistical analysis and figures.B.W. and V.V. provided general supervision and feedback and reviewed the manuscript.All authors reviewed and approved the final manuscript.
Corresponding author
Correspondence to Agnes Boltz .
Ethics declarations
Ethics approval and consent to participate.
This cross sectional study was conducted after approval from the Ethics Committee of the City of Vienna had been obtained (EK 20-352-VK) and adhered to the tenets of the Declaration of Helsinki. Due to the retrospective character of the study, informed consent was waived in agreement with the positive vote of the above-mentioned Ethics Committee.
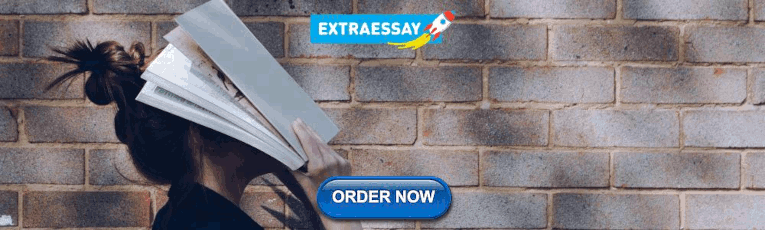
Consent for publication
Competing interests.
The authors declare no competing interests.
Additional information
Publisher’s note.
Springer Nature remains neutral with regard to jurisdictional claims in published maps and institutional affiliations.
Rights and permissions
Open Access This article is licensed under a Creative Commons Attribution 4.0 International License, which permits use, sharing, adaptation, distribution and reproduction in any medium or format, as long as you give appropriate credit to the original author(s) and the source, provide a link to the Creative Commons licence, and indicate if changes were made. The images or other third party material in this article are included in the article’s Creative Commons licence, unless indicated otherwise in a credit line to the material. If material is not included in the article’s Creative Commons licence and your intended use is not permitted by statutory regulation or exceeds the permitted use, you will need to obtain permission directly from the copyright holder. To view a copy of this licence, visit http://creativecommons.org/licenses/by/4.0/ . The Creative Commons Public Domain Dedication waiver ( http://creativecommons.org/publicdomain/zero/1.0/ ) applies to the data made available in this article, unless otherwise stated in a credit line to the data.
Reprints and permissions
About this article
Cite this article.
Boltz, A., Spöttl, T., Huf, W. et al. Effect of intravitreal injections due to neovascular age-related macular degeneration on retinal nerve fiber layer thickness and minimum rim width: a cross sectional study. BMC Ophthalmol 24 , 185 (2024). https://doi.org/10.1186/s12886-024-03453-2
Download citation
Received : 11 June 2023
Accepted : 12 April 2024
Published : 23 April 2024
DOI : https://doi.org/10.1186/s12886-024-03453-2
Share this article
Anyone you share the following link with will be able to read this content:
Sorry, a shareable link is not currently available for this article.
Provided by the Springer Nature SharedIt content-sharing initiative
- Retinal nerve fiber layer thickness
- Minimum rim width
- Intravitreal injections
BMC Ophthalmology
ISSN: 1471-2415
- General enquiries: [email protected]
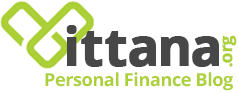
19 Advantages and Disadvantages of Cross Sectional Studies
A cross-sectional study involves the review of information from a population demographic at a specific point in time. The participants who get involved with this research are selected based on particular variables that researchers want to study. It is often used in developmental psychology, but this method is also useful in several other areas. Social sciences and educational processes benefit from this work.
Researchers who are following cross-sectional study techniques would study select groups of people in different age demographics. Their work would look at one investigatory point at a time. By taking this approach, any differences that exist between the demographics would be attributed to characteristics instead of something that happens.
These studies are observational in nature. They are sometimes described as descriptive research, but not causal or relational. That means researchers are unable to determine the cause of something, such as an illness, when using this method.
Several advantages and disadvantages are worth considering when looking at cross-sectional studies.
List of the Advantages of Cross-Sectional Studies
1. This study takes place during a specific moment in time. A cross-sectional study has defined characteristics that limit the size and scope of the work. Researchers look at specific relationships that happened during a particular moment in time. That means there are fewer risks to manage if tangents begin to develop in the data. The goal is to look for a meaningful result within an expected boundary.
2. No variable manipulation occurs with a cross-sectional study. Researchers directly observe the variables under study when using the cross-sectional technique. There is no reason to manipulate the environment because this is not an experimental technique. The data gathering process goes quickly because everything occurs within the scope of the research method. This advantage reduces the risk of having bias creep into the information being gathered.
3. It is an affordable way to conduct research. A cross-sectional study is much more affordable to complete when compared to the other options that are available to researchers today. No follow up work is necessary when taking this approach because once the information gets collected from the entire participant group, it can be analyzed immediately. This advantage as possible because only a single time reference is under consideration.
This approach allows for usable data to become available without the risk of a significant initial investment. Most of the data points collected using this method come from self-report surveys. Researchers can then collect a significant amount of information from a large pool of participants without a major time investment.
4. This study method provides excellent controls over the measurement process. Cross-sectional studies are only as good as the measurement processes that researchers used to collect data. Because there aren’t any long-term considerations involved with this specific approach, researchers have more control over the information acquisition process. Everything obtained during this work is quickly and easily measured and applied to the targeted demographics because the controls involved are straightforward to implement.
5. Researchers can look at several useful characteristics at once. Many researchers prefer the cross-sectional studies method because it allows them to look at numerous characteristics simultaneously. Instead of focusing on income, gender, age, or other separating factors, this method looks at each participant as an entire individual. That makes it possible for the work to include several useful characteristics that can each benefit from changing variables instead of using only one to determine an outcome.
This advantage is the reason why researchers often use cross-sectional studies to look at the prevailing characteristics in a given population. It is a process that lets different variables become the foundation of new correlations.
6. It provides relevant information in real-time updates. Cross-sectional studies provide us with a snapshot of a specific group of people at a particular point in time. Unlike other methods of research that look at demographics over an extended period, we use this information to look at what is happening in the present. That means the data researchers collect from this process is immediately relevant, giving us an opportunity to create real-time updates within specific population groups.
This process is how we can determine if there are specific risk factors that correlate to particular outcomes wit in that group. A cross-sectional study might look at a person’s past smoking and chewing habits to determine if there is a correlation with a recent lung cancer diagnosis. Although it won’t provide a cause-and-effect explanation, it does offer a fast look at potential correlations.
7. Cross-sectional studies miss fewer data points. The processes involved with cross-sectional studies reduce the risk of missing critical data points. Researchers have the ability to maximize their examination of the available information at any time because there are no time variables included in this work. That means a lower error rate typically occurs when using this method compared to the other approaches that are available to the scientific community.
8. It allows anyone to look at the data to determine a possible conclusion. The information that cross-sectional studies obtain is always suitable for secondary analysis. This advantage means that researchers can collect information for one set of purposes, and then use it to explore different variables that might exist in that specific demographic at the same time. That means an investment in this work can provide ongoing usefulness because it always applies to the people involved during that specific time. It is one of the easiest ways to maximize your research investment value.
9. Cross-sectional studies offer information that’s well-suited for descriptive analysis. If researchers want to develop a general hypothesis, then cross-sectional studies are the best way to generate specific situations that face a particular demographic. Each description of the critical data points creates the possibility of forwarding movement toward a future solution that may not have been considered previously.
Although this benefit doesn’t apply to causal relationships with this research method, the information collected from cross-sectional studies is a useful forward push toward additional research.
10. The focus of a cross-sectional study is to prove or disprove an assumption. A cross-sectional study is a research tool that is useful across various industries. The reason why it is such a generalized process that anyone can initiate is that the purpose of the work is to prove or disapprove and assumption or theory. Although health-related work tends to be the most popular industry that takes advantage of this approach, retail, education, social science, religion, and government industries can also benefit from this process.
The research that occurs allows each industry to learn more about the various demographics for the purpose of analyzing a target market. It creates data that’s useful when trying to determine what products or services to sell, or when it is necessary to look for specific patient outcomes.
List of the Disadvantages of Cross-Sectional Studies
1. It requires the entire population to be studied to create useful data. A correctly structured cross-sectional study must be representative of an entire demographic for it to provide useful information. If this representation is not possible, then the data collected from the participating individuals will have a built-in error rate that must come under consideration.
That’s why a complete generalization is not possible when using this approach. Environmental conditions, a person’s education, and several other factors can all change an individual’s perspective.
2. A researcher’s personal bias can influence the data from cross-sectional studies. Everyone has particular biases that influence their personality and general perspective on life. Many of these circumstances come from the conditioning that happens over the course of time. Even people who work hard to avoid showing bias in any situation can come under the influence of this disadvantage of cross-sectional studies.
Some demographics might include prison populations, the homeless, or people who are unable to leave their homes. If a researcher feels uncomfortable contacting individuals in these groups, then the final data points will not have as much relevance as they could.
3. The questions asked during cross-sectional studies may lead to specific results. If researchers want to achieve a specific result when performing a cross-sectional study, then they can ask questions in such a way that it leads participants to the desired answer. When there are surveys or questionnaires about specific aspects of a person’s life, then the answers received may not always result in an accurate report. Shared experiences can result in different perspectives.
We have seen this disadvantage play out numerous times throughout several generations. The people who were alive during the Vietnam war, the attack on Pearl Harbor, or the terrorist event in New York City on 9/11 have shared experiences that make them different from other age groups. The people who survived these events are another subgroup that can impact the quality of information gathered.
4. Large sample sizes are often necessary to generate usable information. A significant sample size is often necessary for a cross-sectional study to provide useful information. This disadvantage occurs because the entire population demographic must go through the research at once to prevent errors in the data. When a smaller sample size is the focus of the work, then the risk of errors entering into the information increases dramatically. There are more opportunities for coincidence or a chance to influence the results with a smaller research sample.
Although cross-sectional studies are often very affordable, the inclusion of an entire demographic pushes the cost of this work higher than it would be for other approaches.
5. Cross-sectional studies don’t offer any control over purpose or choice. When the data from a cross-sectional study is found useful for secondary data analysis, the bias of the researchers can influence the information without any future realization. The secondary approach has no control over how this work gets completed initially. That’s why an overview of the methods used and the purpose for collecting information in the first place are often included as part of the results of this work.
If these additional facts are not part of the final experience, and the usefulness of the information for future needs becomes questionable.
6. No information about causal relationships is possible with this approach. This research method does not provide information about causal relationships. The goal of this approach is to offer correlated data that is useful when drawing conclusions about a specific demographic. It can only let researchers see that a causal relationship exists without letting them know the reason behind its existence.
That’s why individualization is a disadvantage to this type of study. Researchers are wanting to see a generalized overview of a specific population sample instead of understanding why some people make particular choices.
7. Demographic definitions must be available to create a. successful result. The information collected during a cross-sectional study is not reliable unless there are specific definitions in place for a population sample that is large enough for generalization. If researchers want to look at a rare outcome or a unique event, then inappropriate conclusions could get gleaned from the collected data. Trying to force a specific question or result could encourage responses that are unnecessary within the study population.
The only way to avoid this disadvantage of a cross-sectional study is to create definitions that work specifically with the intended results.
8. Cross-sectional studies have no way to measure incidence. The goal of a cross-sectional study is to review the data that researchers collect as they study-specific variables. It does not take a look at the reason why the specific information points occur within the population demographics. This disadvantage limits the availability of an outcome for researchers in many situations because there is no determination available as to why the variables are present initially.
It only measures the existence and relationships that are present in that environment, not what triggers the variables.
9. It can be challenging to duplicate the results. Even though a large population sample is necessary to create an accurate dataset from a cross-sectional study, it is challenging to duplicate results from multiple efforts. This disadvantage occurs because work happens in real-time situations. What happens right now can create a very different result then what could happen in the future.
That’s why many institutions face some challenges when they attempt to put together a sampling pool. The variables that should be studied are available in complex ways that may be difficult to manage. This issue is so detrimental that the timing of a specific snapshot is never a 100% guarantee that it’s representative of the entire population group.
A cross-sectional study is a useful research tool in most areas of health and wellness. When we can learn more about what is happening within a specific population demographic, then researchers can better understand the relationships that could exist between particular variables. The information that comes out of this process allows us to develop further studies that can explore the results in greater depth.
Several other research study options are available when there is a need to collect information from a specific demographic. It is essential to compare the critical points from each approach to determine what the best possible solution will be for each situation.
These cross-sectional advantages and disadvantages show us that a massive undertaking of simultaneous data collection can provide unique results that can benefit an entire population group. Although there are some challenges to manage when taking this approach, it is one that most researchers find to be beneficial.
- Open access
- Published: 27 April 2024
Associations between trans fatty acids and systemic immune-inflammation index: a cross-sectional study
- Xiao-Feng Zhu 1 ,
- Yu-Qi Hu 2 ,
- Zhi-Cheng Dai 3 ,
- Xiu-Juan Li 2 &
- Jing Zhang 4
Lipids in Health and Disease volume 23 , Article number: 122 ( 2024 ) Cite this article
Metrics details
Previous studies have demonstrated that trans fatty acids (TFAs) intake was linked to an increased risk of chronic diseases. As a novel systemic inflammatory biomarker, the clinical value and efficacy of the systemic immune-inflammation index (SII) have been widely explored. However, the association between TFAs and SII is still unclear. Therefore, the study aims to investigate the connection between TFAs and SII in US adults.
The study retrieved data from the National Health and Nutrition Examination Survey (NHANES) for the years 1999–2000 and 2009–2010. Following the exclusion of ineligible participants, the study encompassed a total of 3047 individuals. The research employed a multivariate linear regression model to investigate the connection between circulating TFAs and SII. Furthermore, the restricted cubic spline (RCS) model was utilized to evaluate the potential nonlinear association. Subgroup analysis was also conducted to investigate the latent interactive factors.
In this investigation, participants exhibited a mean age of 47.40 years, with 53.91% of them being female. Utilizing a multivariate linear regression model, the independent positive associations between the log2-transformed palmitelaidic acid, the log2 transformed-vaccenic acid, the log2-transformed elaidic acid, the log2-transformed linolelaidic acid, and the log2-transformed-total sum of TFAs with the SII (all P < 0.05) were noted. In the RCS analysis, no nonlinear relationship was observed between the log2-transformed palmitelaidic acid, the log2 transformed-vaccenic acid, the log2-transformed elaidic acid, the log2-transformed linolelaidic acid, the log2-transformed-total sum of TFAs and the SII (all P for nonlinear > 0.05). For the stratified analysis, the relationship between the circulating TFAs and the SII differed by the obesity status and the smoking status.
Conclusions
A positive association was investigated between three types of TFA, the sum of TFAs, and the SII in the US population. Additional rigorously designed studies are needed to verify the results and explore the potential mechanism.
Introduction
Trans fatty acids (TFAs) are a specific type of unsaturated acids that are naturally occurring and artificially produced. In the U.S., dietary TFAs account for 2–3% of the energy intake, primarily from processed foods, including baked products and packaged snacks [ 1 ]. However, TFAs are not essential to the human body and are detrimental to health. Earlier investigations have established that the intake of TFAs is associated with an increase in lipid levels [ 2 , 3 ], which may lead to an increased prevalence of cardiovascular diseases [ 4 ]. Moreover, studies based on in vivo and in vitro models found that the TFAs could not only modulate the microbiome in the mice but also induce inflammation and oxidative stress [ 5 , 6 ], which are associated with the risk of some common chronic diseases [ 7 ].
It has been proposed that inflammation is a major factor in the development of diseases. To better evaluate the systematic inflammation of patients in clinical practice, a novel blood inflammation biomarker called the systematic immune-inflammation index (SII) has been proposed, which could be calculated based on three types of blood cells (lymphocytes, neutrophils, and platelets) [ 8 ]. As an easily accessible indicator, plenty of studies have investigated and confirmed its prognostic value in diabetes, lung cancer, and the general population [ 9 , 10 , 11 ]. A study based on 6003 Chinese adults discovered that the SII was significantly associated with hypertension over a long-term period [ 12 ]. In addition, recent studies have found that elevated SII may increase the risk of diabetic retinopathy and cognitive impairment, as well as the severity of carotid artery stenosis [ 13 , 14 , 15 ].
Some studies have reported that a few dietary factors, including dietary fiber, vitamin D and selenium, may influence systemic inflammation in humans [ 16 , 17 , 18 ]. However, information on the association between TFAs and systemic inflammation is limited. Given the widespread use of TFAs and the excellent efficacy of SII, exploring the relationship between circulating TFAs and SII may provide some novel insights into the adverse effects of TFAs on inflammation. Hence, National Health and Nutrition Examination Survey (NHANES) data collected during the years 1999–2000 and 2009–2010 were used in the study to explore the connections between plasma TFAs and SII among U.S. adults.
Study population
NHANES is a large database that could be freely accessed by researchers around the globe. The Centers for Disease Control and Prevention (CDC) conducted the NHANES project on a two-year cycle to evaluate the nutritional and medical status of non-institutionalized individuals living in the U.S. Approximately 5000 civilians living in the communities were selected by authorities across each cycle. The complex sampling and multi-stage methodology was utilized in the sample survey to generate nationally representative data.
The research selected participants’ data from two survey cycles of the database (1999–2000 and 2009–2010), for which the level of circulating TFAs was available. In this study, a total of 20,502 participants aged ≥ 20 years were first extracted. Then, we excluded 13,642 samples with missing data on TFAs in the second step and 29 samples with missing data on SII in the third step. Furthermore, 3784 participants with missing data on the covariates were also regarded as ineligible. Finally, 3047 eligible U.S. adults from the NHANES were included to conduct a cross-sectional study. The flowchart of the inclusion and exclusion criteria is shown in Fig. 1 . The protocol was approved by the Ethical Review Committee of the National Health Council, and each individual gave written informed consent.
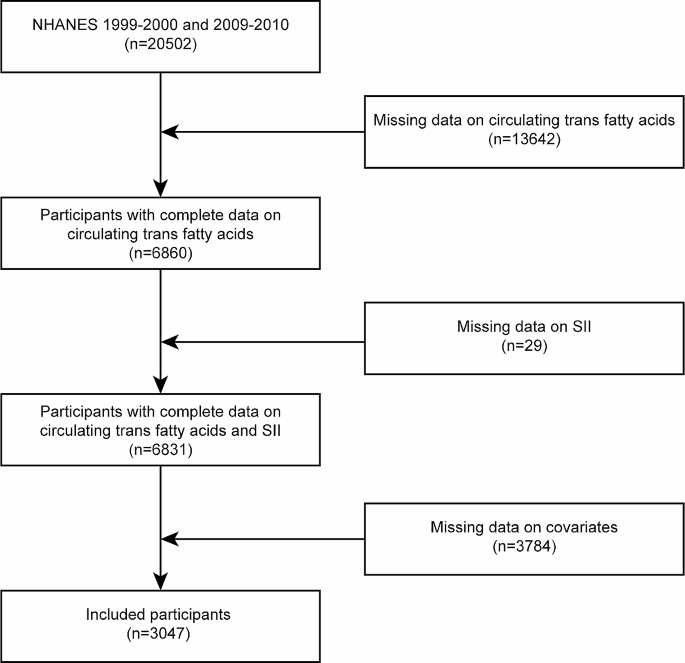
Flow chart of participant selection. Abbreviations: NHANES, National Health and Nutrition Examination Survey, SII, Systemic immune-inflammation index
Measurement of circulating TFA
Previous studies have reported detailed methods and approaches to evaluate the level of plasma TFA [ 19 , 20 ]. In brief, participants’ blood samples were obtained in the morning after a fasting period following the protocol outlined by the CDC. Subsequently, TFA isomers were identified by their chromatographic retention times and specific mass-to-charge ratios. Quantification of metabolites was conducted using established standard solutions, incorporating stable isotope-labeled fatty acids as internal standards. The total amount of TFAs was determined as follows: Sum TFAs = vaccenic acid + linoelaidic acid + palmitelaidic acid + elaidic acid.
Identification of SII
The study derived the SII by multiplying the number of neutrophils by the number of platelets, followed by dividing by the number of lymphocytes. The level of the complete blood cell count is expressed as ×103 cells/µl and was assessed by blood analysis equipment, which is conducted by professional laboratory staff.
Considering the clinical facts, the potential confounding factors were included in the study. Demographic factors, including age, gender, race, education, poverty income ratio (PIR), and marital status, were evaluated through a questionnaire conducted at the mobile examination center. Race was categorized into five groups: Mexican American, non-Hispanic Black, non-Hispanic White, other Hispanic, and other races. Marital status was categorized as married/living with a partner, widowed/divorced/separated, or never married. Smoking status was defined based on lifetime cigarette consumption, with categories for never smoked, ever smoked, and current smoker. Alcohol consumption was determined by the mean alcohol intake over a two-day diet obtained through dietary recall. Education level was stratified into three groups: less than high school, high school graduate, and more than high school. Trained medical personnel measured and calculated participants’ body mass index (BMI) during interviews. Information on cardiovascular disease (CVD), hypertension, cancer, and diabetes mellitus (DM) was collected through questionnaires. Specifically speaking, participants were considered CVD patients, based on the previous studies [ 21 , 22 , 23 ]. The direct immunoassay-related equipment was utilized for examining the level of the lipids in individuals. Serum uric acid levels were measured using the colorimetric method in laboratory tests, and the estimated glomerular filtration rate (eGFR) was calculated following established research protocols [ 24 ].
Statistical analysis
Based on the CDC guideline, all analyses involved in the study took clustering, multi-stage, and sample weights into consideration. Given the skewed distribution of TFAs, a log2 transformation was applied for the regression analysis. The baseline characteristics of participants were stratified by the tertiles of sum TFAs. Continuous variables were presented as mean ± standard error using weighted linear regression models, while categorical variables were expressed as percentages through the Rao-Scott chi-square test. Subsequently, the research employed the multivariate linear regression model to examine the relationship between TFAs and SII. The effect size (β) and 95% confidence intervals (CI) were calculated for statistical assessment. Model 1 was unadjusted, while Model 2 accounted for age, gender, and race. Model 3 was adjusted for the all latent confounders we included for the present investigation to verify the robustness of the results. Additionally, the restricted cubic spline (RCS) model was utilized to investigate potential non-linear associations involving four main types of TFAs, the sum TFAs, and SII. Furthermore, subgroup analysis and interactive P values were utilized to probe potential interaction effects among stratified variables. All analyses were conducted using R software (version 4.2.1).
Baseline characteristics of the study participants
Table 1 presents the weighted basic characteristics of 3047 individuals. In the study population, the average age was 47.40 years, and 53.91% were female. Additionally, the mean levels of the circulating palmitelaidic acid, vaccenic acid, elaidic acid and linolelaidic acid were 5.05 µmol/L, 25.87 µmol/L, 20.99 µmol/L, and 2.07 µmol/L, respectively. After classifying by sum TFAs tertiles, individuals with higher circulating TFAs were more likely to be older, non-Hispanic White, have lower educational attainment, married/living with a partner, current smokers, less alcohol consumption, lower eGFR, and higher SII. However, no statistically significant difference was shown in gender, PIR, uric acid, CVD, hypertension, DM, and cancer across the three groups. Interestingly, BMI was shown to be highest in the T2 group with an average of 29.24 kg/m2 and the population in the T2 group had the highest age with an average of 48.49 years.
Relationship between TFAs and SII
The multivariate linear regression model was performed and detailed results were shown in Table 2 . In the crude model (model 1), the four types of TFA and the sum of TFAs were significantly and positively related to SII. After adjusting for age, sex, and race (model 2), the relationship was weakened. After adjusting for the covariates that were included in the study in Model 3, the connection between the log2-transformed palmitelaidic acid (β = 56.84, 95% CI = 30.93, 82.74, P < 0.001), the log2-transformed vaccenic acid (β = 32.28, 95% CI = 14.99, 49.57, P = 0.002), the log2-transformed elaidic acid (β = 40.31, 95% CI = 23.09, 57.54, P < 0.001), the log2-transformed-linolelaidic acid (β = 27.04, 95% CI = 6.10, 47.97, P = 0.016), the log2-transformed sum TFAs (β = 40.33, 95% CI = 21.29, 59.38, P < 0.001) and SII remain robust. Compared to the T1 group, individuals in the T3 group of palmitelaidic acid (β = 75.19, 95% CI = 25.38, 125.00, P = 0.007), vaccenic acid (β = 62.02, 95% CI = 11.02, 113.02, P = 0.022), elaidic acid (β = 84.43, 95% CI = 34.80, 134.07, P = 0.003), and sum TFAs (β = 78.08, 95% CI = 31.74, 124.41, P = 0.003) were significantly had higher SII. However, the population in the T3 group of the linolelaidic acid was not observed to have a higher SII ( P > 0.05).
Furthermore, the study performed the RCS analysis for four main types of TFA and the sum of TFAs which was shown in Fig. 2 . Judging from the results, no significant nonlinear correlation was observed between four main types of TFAs, the sum TFAs and SII (all P for nonlinear > 0.05).
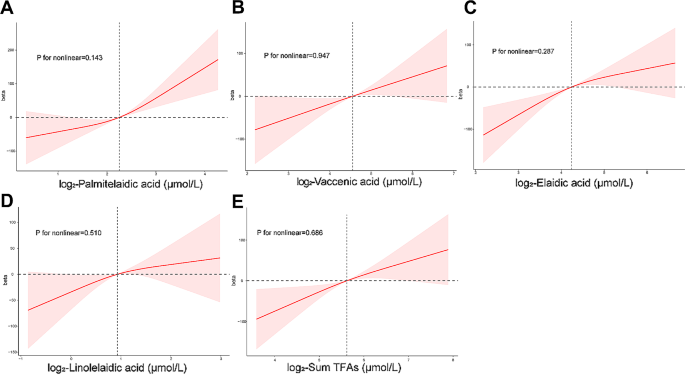
The restricted cubic splines analysis of the association between log2-Palmitelaidic acid ( A ), log2-Vaccenic acid ( B ), log2-Elaidic acid ( C ), log2-Linolelaidic acid ( D ), log2-Sum TFAs ( E ) and SII. Abbreviations: TFAs, trans fatty acids, SII, Systemic immune-inflammation index
Subgroup analysis
The stratified analysis was utilized to explore the potential interactive factors in the relationship between TFAs and SII. The results were shown in Tables 3 , 4 , 5 , 6 and 7 . For the circulating palmitelaidic acid, vaccenic acid, elaidic acid, and the sum TFAs, they were more pronounced in never smokers (all P for interaction < 0.05). Additionally, the linolelaidic acid was more positively related to the SII in individuals with lower BMI, and a history of never having smoked ( P for interaction < 0.05).
To our knowledge, there is currently limited research investigating the association between TFAs and SII. Therefore, we employed various advanced statistical models to comprehensively evaluate the influence of TFAs on SII levels. These findings revealed a positive correlation between palmitelaidic acid, vaccenic acid, elaidic acid, the total sum of TFAs, and SII in fully adjusted models. Notably, significant interactions were observed between smoking and certain TFAs.
SII is increasingly recognized as a potential biomarker for conditions such as gastrointestinal malignancies, prostate cancer, cardiovascular illnesses, and others [ 25 , 26 , 27 ]. In a cross-sectional study involving 730 healthy women from the Nurses’ Health Investigation I cohort, Lopez-Garcia et al. noted a positive correlation between TFAs intake and plasma concentrations of C-reactive protein (CRP), sE-selectin, sICAM-1, tumor necrosis factor-alpha receptors 2, and sVCAM-1 [ 28 ]. These findings were consistent with other interventional and observational studies that suggest consumption of TFAs could elevate inflammatory markers in the blood such as CRP, interleukin-1β, chemokine ligand 2 and interleukin-6 (IL-6) [ 27 , 29 , 30 ]. Further evidence from in vitro tests and animal models shows that TFAs can activate and accumulate macrophages, as well as activate NF-κB and enhance osteopontin production in the liver [ 31 , 32 , 33 , 34 ].
Another possible explanation for the correlation between TFAs and SII is the reduced proportion of gram-negative sulfate-reducing bacteria after a meal high in TFAs according to Ge et al. [ 35 ]. The bacteria’s subsequent overproduction of hydrogen sulfide (H 2 S) may be a factor in inflammatory bowel disease and bowel illnesses linked to inflammation [ 36 ]. By reducing the disulfide bonds in the mucus network, H 2 S promotes the breakdown of the mucus barrier and increases the permeability of the mucus layer [ 37 ]. When the mucus barrier is breached, germs and toxins can get in intimate contact with the colonic epithelium, which can lead to inflammation [ 37 ]. Owing to these inflammatory variables, a conceivable biological process that results in greater SII is excessive consumption of TFAs with pro-inflammatory properties.
The subgroup analysis and interaction tests conducted in this study revealed a noteworthy positive correlation between total TFAs and SII within subgroups categorized by smoking status, while the similar connection between the Linolelaidic acid and SII within subgroups categorized by BMI and smoking status. According to these findings, there was a higher positive association between SII scores and TFAs among nonsmokers. Previous studies have demonstrated that inflammation is frequently involved in the pathogenesis of illnesses associated with cigarette smoking [ 38 ]. The subgroup analysis’s findings further imply that the association between SII and TFAs varies according to BMI. Patients with a BMI under 30 kg/m² showed a greater correlation between TFAs and SII. Previous studies have connected TFA intake to higher BMI levels [ 39 ]. Studies suggest that BMI, a risk factor for various cancers, is associated with an elevation in SII [ 40 ]. Collectively, these results imply that those with high amounts of circulating TFAs should be closely detected for elevated SII, especially those without harmful lifestyle choices, which was consistent with previous findings [ 41 , 42 ]. Nevertheless, additional investigations are necessary to clarify the specific mechanisms involved.
Strengths and limitations
The research offers some fresh perspectives in this area. First, the study assessed the connection between TFAs and SII in U.S. adults for the first time. In addition, subgroup analyses were carried out to guarantee consistent results, and a wide range of potential confounding factors were taken into account in this study. Furthermore, after controlling for a wide range of potential confounders, the study discovered that the dose-response correlations of SII with all types of TFAs level and the sum TFAs were not nonlinear. However, some limitations of the investigation must be acknowledged. Initially, due to regulatory modifications in the past decade, the findings derived from data collected between 1999 and 2000 and 2009–2010 may not precisely depict the present scenario of TFAs intake among adults in the US. Furthermore, the results could not suggest the habits of the diet and lifestyle and the level of circulating trans fatty acids in the current Americans. Nevertheless, these results could establish a foundational reference point for subsequent analyses, given that they are grounded in the most recent data accessible for the entire adult US population. Second, even though the research employed the blood cell count-based comprehensive index as a biomarker of systemic immune inflammation, more research is necessary to determine the relationship between TFAs exposure and other biomarkers including CRP and IL-6. Thirdly, given the cross-sectional study design employed, the investigation is unable to establish causation from these findings. Consequently, even though variables were taken into account, measurement errors and uncontrolled confounders might have had an impact on the results.
In this cross-sectional study, the circulating TFAs were investigated to be positively associated with SII, and a nonlinear relationship was found. Notably, these associations could be more weakened or more pronounced in different subgroups. Briefly, the findings of the study emphasize the potential role of TFAs in systemic inflammation severity and provide new insights into controlling systemic inflammation levels in the US general population from a dietary health perspective. Nevertheless, additional research is essential to explore the cause-and-effect relationship and to elucidate the specific underlying mechanism.
Data availability
The study utilized data from the National Health and Nutrition Examination Survey (NHANES), which is publicly available in the NHANES repository, https://www.cdc.gov/nchs/nhanes .
Micha R, Mozaffarian D. Trans fatty acids: effects on cardiometabolic health and implications for policy. Prostaglandins Leukot Essent Fatty Acids. 2008;79:147–52.
Article CAS PubMed PubMed Central Google Scholar
Liska DJ, Cook CM, Wang DD, Gaine PC, Baer DJ. Trans fatty acids and cholesterol levels: an evidence map of the available science. Food Chem Toxicol. 2016;98:269–81.
Article CAS PubMed Google Scholar
Verneque BJF, Machado AM, de Abreu Silva L, Lopes ACS, Duarte CK. Ruminant and industrial trans-fatty acids consumption and cardiometabolic risk markers: a systematic review. Crit Rev Food Sci Nutr. 2020;62:2050–60.
Article PubMed Google Scholar
Mozaffarian D, Katan MB, Ascherio A, Stampfer MJ, Willett WC. Trans fatty acids and Cardiovascular Disease. N Engl J Med. 2006;354:1601–13.
Liu H, Nan B, Yang C, Li X, Yan H, Yuan Y. Elaidic acid induced NLRP3 inflammasome activation via ERS-MAPK signaling pathways in Kupffer cells. Biochim et Biophys Acta (BBA) - Mol Cell Biology Lipids 2022, 1867.
Mohammadi F, Green M, Tolsdorf E, Greffard K, Leclercq M, Bilodeau J-F, Droit A, Foster J, Bertrand N, Rudkowska I. Industrial and ruminant trans-fatty acids-enriched diets differentially modulate the Microbiome and Fecal metabolites in C57BL/6 mice. Nutrients 2023, 15.
Islam MA, Amin MN, Siddiqui SA, Hossain MP, Sultana F, Kabir MR. Trans fatty acids and lipid profile: a serious risk factor to cardiovascular disease, cancer and diabetes. Diabetes Metabolic Syndrome: Clin Res Reviews. 2019;13:1643–7.
Article Google Scholar
Hu B, Yang X-R, Xu Y, Sun Y-F, Sun C, Guo W, Zhang X, Wang W-M, Qiu S-J, Zhou J, Fan J. Systemic Immune-inflammation index predicts prognosis of patients after curative resection for Hepatocellular Carcinoma. Clin Cancer Res. 2014;20:6212–22.
Sun W, Zhang P, Ye B, Situ M-Y, Wang W, Yu Y. Systemic immune-inflammation index predicts survival in patients with resected lung invasive mucinous adenocarcinoma. Translational Oncol 2024, 40.
Wang H, Nie H, Bu G, Tong X, Bai X. Systemic immune-inflammation index (SII) and the risk of all-cause, cardiovascular, and cardio-cerebrovascular mortality in the general population. Eur J Med Res 2023, 28.
Yang C, Yang Q, Xie Z, Peng X, Liu H, Xie C. Association of systemic immune-inflammation-index with all-cause and cause-specific mortality among type 2 diabetes: a cohort study base on population. Endocrine 2023.
Ma L-L, Xiao H-B, Zhang J, Liu Y-H, Hu L-K, Chen N, Chu X, Dong J, Yan Y-X. Association between systemic immune inflammatory/inflammatory response index and hypertension: a cohort study of functional community. Nutr Metabolism Cardiovasc Dis 2023.
Kelesoglu S, Yilmaz Y, Elcik D, Bireciklioglu F, Ozdemir F, Balcı F, Tuncay A, Kalay N. Increased serum systemic Immune-inflammation index is independently Associated with severity of carotid artery stenosis. Angiology. 2022;74:790–7.
Li JQ, Zhang YR, Wang HF, Guo Y, Shen XN, Li MM, Song JH, Tan L, Xie AM, Yu JT. Exploring the links among peripheral immunity, biomarkers, cognition, and neuroimaging in Alzheimer’s disease. Alzheimer’s Dementia: Diagnosis Assess Disease Monit 2023, 15.
Wang S, Pan X, Jia B, Chen S. Exploring the correlation between the systemic Immune inflammation index (SII), systemic inflammatory response index (SIRI), and type 2 Diabetic Retinopathy. Diabetes Metabolic Syndrome Obes. 2023;16:3827–36.
Gonçalves de Carvalho CMR, Ribeiro SML. Aging, low-grade systemic inflammation and vitamin D: a mini-review. Eur J Clin Nutr. 2016;71:434–40.
Qi X, Li Y, Fang C, Jia Y, Chen M, Chen X, Jia J. The associations between dietary fibers intake and systemic immune and inflammatory biomarkers, a multi-cycle study of NHANES 2015–2020. Front Nutr 2023, 10.
Rayman MP. The importance of selenium to human health. Lancet. 2000;356:233–41.
Kuiper HC, Wei N, McGunigale SL, Vesper HW. Quantitation of trans-fatty acids in human blood via isotope dilution-gas chromatography-negative chemical ionization-mass spectrometry. J Chromatogr B. 2018;1076:35–43.
Article CAS Google Scholar
Vesper HW, Caudill SP, Kuiper HC, Yang Q, Ahluwalia N, Lacher DA, Pirkle JL. Plasma trans-fatty acid concentrations in fasting adults declined from NHANES 1999–2000 to 2009–2010. Am J Clin Nutr. 2017;105:1063–9.
Chen F, Song Y, Li W, Xu H, Dan H, Chen Q. Association between periodontitis and mortality of patients with cardiovascular diseases: a cohort study based on NHANES. J Periodontol. 2024;95:175–84.
Chen Y, Lin W, Fu L, Liu H, Jin S, Ye X, Pu S, Xue Y. Muscle quality index and cardiovascular disease among US population-findings from NHANES 2011–2014. BMC Public Health. 2023;23:2388.
Article PubMed PubMed Central Google Scholar
Dang K, Wang X, Hu J, Zhang Y, Cheng L, Qi X, Liu L, Ming Z, Tao X, Li Y. The association between triglyceride-glucose index and its combination with obesity indicators and cardiovascular disease: NHANES 2003–2018. Cardiovasc Diabetol. 2024;23:8.
Levey AS, Stevens LA, Schmid CH, Zhang Y, Castro AF, Feldman HI, Kusek JW, Eggers P, Van Lente F, Greene T, Coresh J. A New equation to Estimate glomerular filtration rate. Ann Intern Med 2009, 150.
Ye Z, Hu T, Wang J, Xiao R, Liao X, Liu M, Sun Z. Systemic immune-inflammation index as a potential biomarker of cardiovascular diseases: a systematic review and meta-analysis. Front Cardiovasc Med. 2022;9:933913.
Meng L, Yang Y, Hu X, Zhang R, Li X. Prognostic value of the pretreatment systemic immune-inflammation index in patients with prostate cancer: a systematic review and meta-analysis. J Translational Med. 2023;21:79.
Zhang Y, Lin S, Yang X, Wang R, Luo L. Prognostic value of pretreatment systemic immune-inflammation index in patients with gastrointestinal cancers. J Cell Physiol. 2019;234:5555–63.
Lopez-Garcia E, Schulze MB, Meigs JB, Manson JE, Rifai N, Stampfer MJ, Willett WC, Hu FB. Consumption of trans fatty acids is related to plasma biomarkers of inflammation and endothelial dysfunction. J Nutr. 2005;135:562–6.
Mozaffarian D, Pischon T, Hankinson SE, Rifai N, Joshipura K, Willett WC, Rimm EB. Dietary intake of trans fatty acids and systemic inflammation in women. Am J Clin Nutr. 2004;79:606–12.
Baer DJ, Judd JT, Clevidence BA, Tracy RP. Dietary fatty acids affect plasma markers of inflammation in healthy men fed controlled diets: a randomized crossover study. Am J Clin Nutr. 2004;79:969–73.
Machado RM, Nakandakare ER, Quintao ECR, Cazita PM, Koike MK, Nunes VS, Ferreira FD, Afonso MS, Bombo RPA, Machado-Lima A, et al. Omega-6 polyunsaturated fatty acids prevent atherosclerosis development in LDLr-KO mice, in spite of displaying a pro-inflammatory profile similar to trans fatty acids. Atherosclerosis. 2012;224:66–74.
Afonso MS, Lavrador MSF, Koike MK, Cintra DE, Ferreira FD, Nunes VS, Castilho G, Gioielli LA, Paula Bombo R, Catanozi S et al. Dietary interesterified fat enriched with palmitic acid induces atherosclerosis by impairing macrophage cholesterol efflux and eliciting inflammation. J Nutr Biochem 2016, 32.
Hu X, Tanaka N, Guo R, Lu Y, Nakajima T, Gonzalez FJ, Aoyama T. PPARα protects against trans-fatty-acid-containing diet-induced steatohepatitis. J Nutr Biochem. 2017;39:77–85.
Larner DP, Morgan SA, Gathercole LL, Doig CL, Guest P, Weston C, Hazeldine J, Tomlinson JW, Stewart PM, Lavery GG. Male 11β-HSD1 Knockout Mice Fed Trans-Fats and Fructose are not protected from metabolic syndrome or nonalcoholic fatty liver disease. Endocrinology. 2016;157:3493–504.
Ge Y, Liu W, Tao H, Zhang Y, Liu L, Liu Z, Qiu B, Xu T. Effect of industrial trans-fatty acids-enriched diet on gut microbiota of C57BL/6 mice. Eur J Nutr. 2019;58:2625–38.
Figliuolo VR, Dos Santos LM, Abalo A, Nanini H, Santos A, Brittes NM, Bernardazzi C, de Souza HSP, Vieira LQ, Coutinho-Silva R, Coutinho CMLM. Sulfate-reducing bacteria stimulate gut immune responses and contribute to inflammation in experimental colitis. Life Sci. 2017;189:29–38.
Ijssennagger N, van der Meer R, van Mil SWC. Sulfide as a mucus barrier-breaker in inflammatory bowel disease? Trends Mol Med. 2016;22:190–9.
Bhalla DK, Hirata F, Rishi AK, Gairola CG. Cigarette smoke, inflammation, and lung injury: a mechanistic perspective. J Toxicol Environ Health Part B Crit Reviews. 2009;12:45–64.
Hastert TA, Otto deO MC, L-S F, S BT, S LM, T MY, J DR, B A. Association of plasma phospholipid polyunsaturated and trans fatty acids with body mass index: results from the multi-ethnic study of atherosclerosis. Int J Obes. 2018;42:433–40.
Iyengar NM, Gucalp A, Dannenberg AJ, Hudis CA. Obesity and Cancer mechanisms: Tumor Microenvironment and inflammation. J Clin Oncology: Official J Am Soc Clin Oncol. 2016;34:4270–6.
Li H, Wu X, Bai Y, Wei W, Li G, Fu M, Jie J, Wang C, Guan X, Feng Y, et al. Physical activity attenuates the associations of systemic immune-inflammation index with total and cause-specific mortality among middle-aged and older populations. Sci Rep. 2021;11:12532.
You Y, Chen Y, Fang W, Li X, Wang R, Liu J, Ma X. The association between sedentary behavior, exercise, and sleep disturbance: a mediation analysis of inflammatory biomarkers. Front Immunol. 2022;13:1080782.
Download references
Acknowledgements
The authors appreciate the time and effort given by participants during the data collection phase of the NHANES project.
The study did not receive any funding.
Author information
Authors and affiliations.
Department of Clinical Medicine, The Nanshan College of Guangzhou Medical University, Guangzhou, 511436, China
Xiao-Feng Zhu
Department of Clinical Medicine, The Third Clinical School of Guangzhou Medical University, Guangzhou, 511436, China
Yu-Qi Hu & Xiu-Juan Li
Department of Orthopedics, Shanghai General Hospital, Shanghai Jiao Tong University School of Medicine, Shanghai, 201600, China
Zhi-Cheng Dai
Second Department of Infectious Disease, Shanghai Fifth People’s Hospital, Fudan University, Shanghai, 201100, China
You can also search for this author in PubMed Google Scholar
Contributions
ZXF contributed to data collection, analysis, study design, and manuscript writing. HYQ, DZC, and LXJ contributed to the writing of the manuscript, and ZJ contributed to the project design and administration. All authors have granted their approval for the manuscript.
Corresponding author
Correspondence to Jing Zhang .
Ethics declarations
Ethical approval and consent to participate.
The ethical review committee of the National Centre for Health Statistics approved all NHANES protocols and written informed consent was obtained from all participants. All the additional materials, including protocol numbers, are available at https://www.cdc.gov/nchs/nhanes/about_nhanes.htm . The authors confirmed that the whole procedure of the study was conducted under Protocol, which is available at https://www.cdc.gov/nchs/nhanes.htm .
Consent for publication
Not applicable.
Conflicts of interest
The authors declare no conflicts of interest.
Additional information
Publisher’s note.
Springer Nature remains neutral with regard to jurisdictional claims in published maps and institutional affiliations.
Electronic supplementary material
Below is the link to the electronic supplementary material.
Supplementary Material 1
Rights and permissions.
Open Access This article is licensed under a Creative Commons Attribution 4.0 International License, which permits use, sharing, adaptation, distribution and reproduction in any medium or format, as long as you give appropriate credit to the original author(s) and the source, provide a link to the Creative Commons licence, and indicate if changes were made. The images or other third party material in this article are included in the article’s Creative Commons licence, unless indicated otherwise in a credit line to the material. If material is not included in the article’s Creative Commons licence and your intended use is not permitted by statutory regulation or exceeds the permitted use, you will need to obtain permission directly from the copyright holder. To view a copy of this licence, visit http://creativecommons.org/licenses/by/4.0/ . The Creative Commons Public Domain Dedication waiver ( http://creativecommons.org/publicdomain/zero/1.0/ ) applies to the data made available in this article, unless otherwise stated in a credit line to the data.
Reprints and permissions
About this article
Cite this article.
Zhu, XF., Hu, YQ., Dai, ZC. et al. Associations between trans fatty acids and systemic immune-inflammation index: a cross-sectional study. Lipids Health Dis 23 , 122 (2024). https://doi.org/10.1186/s12944-024-02109-w
Download citation
Received : 22 January 2024
Accepted : 15 April 2024
Published : 27 April 2024
DOI : https://doi.org/10.1186/s12944-024-02109-w
Share this article
Anyone you share the following link with will be able to read this content:
Sorry, a shareable link is not currently available for this article.
Provided by the Springer Nature SharedIt content-sharing initiative
- Systemic immunity inflammation index
- Cross-sectional study
- National health and nutrition examination survey
- Trans fatty acids
Lipids in Health and Disease
ISSN: 1476-511X
- General enquiries: [email protected]
- Open access
- Published: 22 April 2024
Association of healthy eating index and self-rated health in adults living in Tehran: a cross-sectional study
- Bahareh Jabbarzadeh-Ganjeh 1 ,
- Kurosh Djafarian 2 &
- Sakineh Shab-Bidar 1 , 3
BMC Public Health volume 24 , Article number: 1106 ( 2024 ) Cite this article
205 Accesses
1 Altmetric
Metrics details
Self-rated health (SRH) has been identified in many studies as a valid predictor of mortality and healthcare utilization. There is limited research on SRH and dietary intake. This study aimed to investigate the association between healthy eating index (HEI) and SRH in adults living in Tehran.
This cross-sectional study was carried out among 850 adult men and women aged 20–59 years who visited health centers in Tehran from 2021 to 2022. Dietary intake was assessed using a validated and reliable semiquantitative food frequency questionnaire with 168 food items, and SRH was assessed with one question: “In general, how do you rate your health?“. We categorized SRH into excellent/very good, good, and fair/poor. In the descriptive statistics part, we used mean ± standard deviation or number (ratio) for quantitative and qualitative variables, respectively. The chi-squared test and one-way analysis of variance were used to calculate the percentage and mean for demographic characteristics across tertiles of SRH. An analysis of covariance was used to compare the means of energy, macronutrients, the HEI, and its component variables across the tertiles of SRH.
The final sample included 795 participants (68.2% female; mean ± standard deviation age: 44.81 ± 10.62 years) whose 40% reported excellent/very good SRH, and 30% reported good and fair/poor SRH separately. There was no association between body mass index, physical activity, education, health status, smoking, and sleep duration with SRH. After adjustment, the total HEI score and its component scores did not differ across the tertiles of SRH status. However, participants with good SRH had a higher intake of total energy (mean difference (MD): 180.33 Kcal, P value < 0.001), total fat (MD: 8.15 gr, P value = 0.002), and total carbohydrates (MD: 20.18 gr, P value = 0.004) than those with fair/poor SRH.
According to our findings, fair/poor SRH was associated with a lower consumption of total energy, total fat, and total carbohydrates in Iranian adults. Additional observational studies would be necessary to clarify these findings.
Peer Review reports
Introduction
Self-rated health (SRH) is one of the most commonly evaluated health conceptions in population-based and clinical studies [ 1 , 2 ]. SRH asks people to rate their general health qualitatively via a short question [ 3 ]. It has been identified in many studies as a strong predictor of mortality and might predict the incidence of diseases [ 4 ]. Some studies have shown that poor SRH status could predict greater healthcare utilization and diminish physical performance in the adult population [ 3 , 5 ]. The total cost of physical or psychological disorders and illnesses, including healthcare expenses and lost economic productivity, amounts to trillions [ 6 ]. Therefore, SRH as a screening tool [ 2 ] might be helpful to lower the healthcare budget. SRH affects the healthy behaviors of people. For older adults, SRH is a retrospective health history assessment and is, therefore, more indicative of their health status than many blood markers [ 7 ].
In the past, nutritional epidemiology mostly focused on the relationship between diseases and specific nutrients, such as vitamins, or specific food groups, such as vegetables and fruits. However, currently, more studies are focused on dietary patterns and evaluating the quality and variety of the whole diet [ 5 ]. Based on dietary guidelines, the diet plan should be low-fat, rich in fruits and vegetables, and generally have a high nutrient density [ 5 ]. There are different ways to assess diet quality, such as the food frequency questionnaire (FFQ), healthy eating index (HEI), alternative healthy eating index (AHEI), etc [ 5 , 8 , 9 ]... The HEI was created on dietary guidelines for Americans, and the scoring was based on adequacy components (foods to eat more of for good health) and moderation components (foods to limit for good health) [ 10 ]. Both HEI and AHEI scores ranged from 0 to 100, where a higher score presents a healthier diet [ 11 ]. A cross-sectional study in Tehran recently estimated the mean HEI score was 52.5, while it was 62.3 in 2001 [ 12 , 13 ]. A systematic review assessed the diet quality using AHEI, and the worldwide mean score was 40.3 among 185 countries from 1990 to 2018. Another systematic review of cohort studies found an association between higher scores of HEI and AHEI with lower risk of all-cause mortality (20%), cardiovascular disease (20%), cancer (14%), type 2 diabetes (19%), and neurodegenerative disease (18%) [ 14 ]. One study reported that those who consumed equal to or less than two servings of fruits and vegetables per day or consumed high-fat foods mostly had poor/fair SRH scores [ 15 ]. Another cross-sectional study revealed a significant and negative association between overweight and low physical activity with SRH [ 16 ].
To date, the status of SRH has not been investigated in Iran. Furthermore, studies exploring the relationship between SRH and the quality of diet, particularly HEI, are scarce. We wanted to determine whether having a better SRH can be associated with a better healthy diet. Conducting this study will help us to have a better understanding of SRH and self-assessment among Iranians. Therefore, this study aimed to determine the association between healthy eating index and self-rated health in adults living in Tehran.
Study design
The study was a cross-sectional population-based survey of those aged 20–59 years from 2021 to 2022. The data were collected from 850 healthy adult men and women through two-stage cluster sampling. In the first stage, a simple random sample was selected within 25 healthcare centers across five different geographic areas of Tehran, and a convenient sampling method was used for the second stage. People who visited health centers in Tehran and met the inclusion criteria were informed about the implementation and objectives of the study via informed consent forms. The research was approved by the Tehran University of Medical Science Human Research Ethics Committee (IR.TUMS.MEDICINE.REC.1401.604).
Data collection
A demographic questionnaire was used for general information through face-to-face interviews. It included age (year), sex (male/female), education level (illiterate/under diploma/diploma/university), occupation (employed/unemployed), marital status (single/married), smoking (yes/quit smoking/no), health status (healthy/one disease/comorbidity), and sleep duration.
Dietary intake assessment
We used a validated and reliable semiquantitative FFQ with 168 food items for each participant to assess their dietary intake. The nutritionist asked about FFQs from the participants through face-to-face interviews. The macro- and micronutrient intake were analyzed using Iranian-designed Nutrition IV Software (First Database, San Bruno, CA).
HEI was calculated based on predetermined criteria by the United States Department of Agriculture [ 17 ]. The 2015 version of this index has nine components related to adequacy and four related to moderation. The total score is the sum of the score of adequacy components (i.e. foods to eat more of for good health) and moderation components (i.e. foods to limit for good health). The HEI scores ranged from 0 to 100, where a higher score presents a healthier die [ 18 ]. The adequacy part includes the following: (1) Total fruit (includes fruit juice), (2) Whole fruits (all forms except fruit juice), (3) Total vegetables (includes any beans and peas), (4) Greens and beans (includes any beans and peas), (5) Whole grains, (6) Dairy (includes all milk products, such as fluid milk, yogurt, and cheese, and fortified soy beverages), (7) Total protein foods (beans and peas are included here (and not with vegetables) when the Total Protein Foods standard is otherwise not met), (8) Seafood and plant proteins (includes seafood, nuts, seeds, soy products (other than beverages) as well as beans and peas if they counted as Total Protein Foods), and (9) Fatty acids (ratio of poly- and monounsaturated fatty acids to saturated fatty acids). The moderation components consist of (1) refined grains, (2) sodium, (3) added sugars, and (4) saturated fats [ 18 ].
Self-rated health assessment
SRH was assessed by asking one question, “In general, how do you rate your health?“. The answers include excellent, very good, good, fair, and poor [ 19 ]. For this study, we combined the “excellent, very good” responses as one subgroup and “fair, poor” responses as another. Therefore, SRH responses were categorized into excellent/very good, good, and fair/poor. This method aligns with other studies that have used the SRH status question [ 2 , 20 ] and makes a better differentiation between positive and negative responses [ 21 ].
Physical activity
We used the short form of the international physical activity questionnaire, validated for the Iranian population [ 22 ]. Participants were questioned about the time spent on vigorous, moderate, and walking activities within the last seven days. The physical activity score was calculated based on the metabolic equivalent minutes per week (MET-minutes/week). At last, the physical activity level is categorized into low (< 600 MET-min/week), moderate (600–3000 MET-min/week), and high levels (> 3000 MET-min/week) [ 23 ].
Assessment of blood pressure
Blood pressure was measured twice by a digital sphygmomanometer (Beurer, BC 08, Germany) after at least 10–15 min of rest. An average of two blood pressures was reported for each person.
Anthropometric measurements
The participant’s height without shoes was measured using a wall stadiometer with a sensitivity of 0.1 cm (Seca, Germany). Weight was evaluated by a digital scale (808 Seca, Germany) with an accuracy of 0.1 kg with minimum clothes on. Body mass index (BMI) was calculated by dividing the weight (kg) by the square of the height (m) [ 24 ]. Based on the WHO, the BMI cut-off points for determining underweight, normal weight, overweight, and obesity are < 18.5, 18.5–24.9, 25-29.9, and ≥ 30, respectively [ 25 ]. Waist (WC) and hip (HC) circumferences were measured with a flexible nonelastic metric tape. WC was measured between the lowest rib and the Iliac crest during exhalation, while HC was at the point that yielded the maximum diameter over the buttocks [ 24 ]. The waist-to-hip ratio (WHR) was calculated by dividing the WC (cm) by HC (cm) [ 26 ]. The waist-to-height ratio (WHtR) was computed by dividing the WC (cm) by height (cm) [ 27 ]. We applied a single nutritionist performing all the measurements to reduce the odds of subjective errors.
Statistical analysis
The general characteristics of the participants are displayed as the mean and standard deviation or number and percent. We categorized SRH into excellent/very good, good, and fair/poor. The normality test of the data was through the Kolmogorov‒Smirnov test and the Q‒Q plot to determine the normal distribution of the data. The chi-squared test and one-way analysis of variance (ANOVA) were calculated as the percentage and mean for demographic characteristics across tertiles of SRH. To compare the means of energy, macronutrients, the HEI, and its component variables across the tertiles of SRH, we applied an analysis of covariance (ANCOVA), adjusting for age, sex, education, occupation, marital status, smoking status, health status, physical activity, and BMI. All analyses were performed with SPSS (SPSS Inc., version 26) software. A p-value less than 0.05 accounted for a significant difference.
Based on Table 1 , the mean ± standard deviation of the participant’s age was 44.81 ± 10.62 years old. Of 850 participants, 17 were excluded due to underreporting, extreme values for protein and total fat intake, and 38 due to lack of information. The final sample included 795 participants, and 542 were female. In total, 40% of the population reported excellent/very good SRH, and 30% reported good and fair/poor SRH separately.
Table 2 shows the frequency and the mean of some demographic characteristics across tertiles of SRH. There was no association between BMI, physical activity, education, health status, smoking, or sleep duration, and SRH.
Table 3 indicates the multivariate-adjusted means of the HEI and its component scores across tertiles of SRH status. The results from the Tukey post hoc test showed that participants with good SRH compared with fair/poor SRH had significant differences in total energy consumption (mean difference (MD): 180.33 Kcal, P value < 0.001), total carbohydrate (MD: 20.18 gr, P value = 0.004), and total fat intake (MD: 8.15 gr, P value = 0.002). Additionally, those with good SRH had lower scores for Total Vegetable (P value = 0.058), Greens and Beans (P value = 0.059), and Dairy (P value = 0.042) compared with participants with fair/poor SRH. However, after adjusting for confounders, the marginal and significant differences were all gone.
In this cross-sectional study, we aimed to investigate the association between a healthy eating index (HHEI) and self-rated health (SRH) in adults living in Tehran. Our study found that 40% (318 participants) reported excellent/very good SRH, 30.1% (239 participants) reported good SRH, and 29.9% (238 participants) reported fair/poor SRH. There were no statistically significant associations between BMI, physical activity, education, health status, smoking, or sleep duration, and SRH. After adjustment, the total HEI score and its component scores did not differ across the tertiles of SRH status. However, participants with good SRH had a higher intake of total energy, total fat, and total carbohydrates than those with fair/poor SRH.
Two studies involving younger populations reported similar findings. A cross-sectional study among 1504 US adolescents found no significant association between HEI score and SRH. However, further analysis revealed specific dietary differences: those with excellent-good SRH had a higher vegetable score, while those with fair/poor SRH had a higher total fat intake score [ 3 ]. A cohort study conducted from 2003 to 2012 on 953 German participants also found no significant association between SRH and those with high healthy nutrition scores and below-average scores in the physical activity and media use index [ 28 ]. The studies suggest this might be due to developmental differences and potentially limited awareness of healthy eating habits in younger individuals [ 3 , 28 ].
Several studies support the association between unhealthy lifestyle factors and poorer SRH. The Spanish DiSA-UMH study found that poorer SRH was linked to lower adherence to the Mediterranean diet, lower physical activity levels, excess weight, and smoking among university students [ 29 ]. Similarly, studies by Zarini et al. and Collins et al. linked fair/poor SRH to higher fat intake [ 5 , 15 ], lower fruit and vegetable intake, and lower physical activity, particularly among females [ 15 ]. These findings align with our null findings for BMI and smoking, as reported in another study conducted in a rural Greek population [ 2 ].
Some studies reported positive associations between HEI and SRH, contrasting with our findings. Vaudin et al. observed a link between better SRH and a more favorable HEI score in older adults [ 20 ]. Additionally, studies in rural populations found associations between healthier diets and better SRH [ 1 , 2 ], while lower education and chronic diseases were linked to poorer SRH [ 2 ]. A large survey [ 30 ] also reported associations between poor sleep, physical inactivity, and poor diet quality with higher odds of poor SRH. However, it’s important to note that the participants in this survey had reported “good” SRH earlier.
Possible explanations for these contrasting findings include:
Population differences: The studies with contrasting findings involved different age groups, health statuses, and potentially socioeconomic backgrounds compared to our study population.
Health awareness: Individuals with chronic diseases might have higher health awareness due to more frequent medical consultations, potentially leading to healthier dietary choices [ 15 ].
Confounding factors: Many variables beyond those we adjusted for in our study, such as socioeconomic status [ 31 , 32 ] and mental health [ 32 ], can influence SRH.
This study has some limitations. First, some confounders, such as social well-being, were not adjusted. Second, the cross-sectional design and the lack of significant associations between HEI and SRH might mirror low power due to the small sample size in this analysis. Our study also has some strengths. The strengths of the current observational study include a sample representative of Tehran’s general population, the first study in Iran around this field, using the latest version of the HEI, a gold standard tool for assessing usual food intake (FFQ), and the inclusion of a large number of covariates.
This is the first attempt to relate SRH status to HEI in healthy Iranian adults. The total HEI score did not vary by SRH status. In detail, those with good SRH had a higher intake of total energy, total fat, and total carbohydrates than those with fair/poor SRH. Additional observational studies are needed to clarify these findings.
Data availability
The datasets analyzed during the current study are available from the corresponding author on reasonable request.
Abbreviations
self-rated health
food frequency questionnaire
healthy eating index
Body mass index
Waist circumference
hip circumferences
waist-to-hip ratio
waist-to-height ratio
standard deviation
one-way analysis of variance
analysis of covariance
mean difference
An R, Xiang X, Liu J, Guan C. Diet and self-rated health among oldest-old Chinese. Arch Gerontol Geriatr. 2018;76:125–32.
Article PubMed Google Scholar
Darviri C, Fouka G, Gnardellis C, Artemiadis AK, Tigani X, Alexopoulos EC. Determinants of self-rated health in a representative sample of a rural population: a cross-sectional study in Greece. Int J Environ Res Public Health. 2012;9(3):943–54.
Article PubMed PubMed Central Google Scholar
Goodwin DK, Knol LL, Eddy JM, Fitzhugh EC, Kendrick OW, Donahue RE. The relationship between self-rated health status and the overall quality of dietary intake of US adolescents. J Am Diet Assoc. 2006;106(9):1450–3.
Loftfield E, Yi S, Immerwahr S, Eisenhower D. Construct validity of a single-item, self-rated question of diet quality. J Nutr Educ Behav. 2015;47(2):181–7.
Collins CE, Young AF, Hodge A. Diet quality is associated with higher nutrient intake and self-rated health in mid-aged women. J Am Coll Nutr. 2008;27(1):146–57.
Saklayen MG. The global epidemic of the metabolic syndrome. Curr Hypertens Rep. 2018;20(2):12.
Wang K, Zhang A, Sun F, Hu RX. Self-rated Health among older Chinese americans: the roles of Acculturation and Family Cohesion. J Appl Gerontol. 2021;40(4):387–94.
Guenther PM, Casavale KO, Reedy J, Kirkpatrick SI, Hiza HA, Kuczynski KJ, Kahle LL, Krebs-Smith SM. Update of the healthy eating index: HEI-2010. J Acad Nutr Diet. 2013;113(4):569–80.
Kennedy ET, Ohls J, Carlson S, Fleming K. The healthy eating index: design and applications. J Am Diet Assoc. 1995;95(10):1103–8.
Article CAS PubMed Google Scholar
Vahid F, Jalili M, Rahmani W, Nasiri Z, Bohn T. A higher healthy eating index is Associated with decreased markers of inflammation and lower odds for being Overweight/Obese based on a case-control study. Nutrients. 2022;14(23):5127.
Article CAS PubMed PubMed Central Google Scholar
Miller V, Webb P, Cudhea F, Shi P, Zhang J, Reedy J, Erndt-Marino J, Coates J, Mozaffarian D. Global dietary quality in 185 countries from 1990 to 2018 show wide differences by nation, age, education, and urbanicity. Nat Food. 2022;3(9):694–702.
Yazdani V, Zeraattalab-Motlagh S, Jayedi A, Majdi M, Mirrafiei A, Martami F, Djafarian K, Shab-Bidar S. The association between self-reported nocturnal sleep duration, irregularity in daily energy intake and diet quality in a sample of Iranian adults. Public Health Nutr. 2023;26(8):1609–16.
Diet quality indices and the risk of type 2 diabetes in the Tehran Lipid and Glucose Study. BMJ Open Diabetes Research & Care 2022, 10(5):e002818.
Morze J, Danielewicz A, Hoffmann G, Schwingshackl L. Diet Quality as assessed by the healthy eating index, alternate healthy eating Index, Dietary approaches to stop hypertension score, and Health outcomes: a second update of a systematic review and Meta-analysis of Cohort studies. J Acad Nutr Diet. 2020;120(12):1998–2031. e1915.
Zarini GG, Vaccaro JA, Canossa Terris MA, Exebio JC, Tokayer L, Antwi J, Ajabshir S, Cheema A, Huffman FG. Lifestyle behaviors and self-rated health: the living for health program. J Environ Public Health. 2014;2014:315042.
Aguilar-Palacio I, Gil-Lacruz AI, Sánchez-Recio R, Rabanaque MJ. Self-rated health in Europe and its determinants: does generation matter? Int J Public Health. 2018;63(2):223–32.
Guenther PM, Reedy J, Krebs-Smith SM, Reeve BB. Evaluation of the healthy eating Index-2005. J Am Diet Assoc. 2008;108(11):1854–64.
Krebs-Smith SM, Pannucci TE, Subar AF, Kirkpatrick SI, Lerman JL, Tooze JA, Wilson MM, Reedy J. Update of the healthy eating index: HEI-2015. J Acad Nutr Diet. 2018;118(9):1591–602.
Krause NM, Jay GM. What do global self-rated health items measure? Med Care. 1994;32(9):930–42.
Vaudin A, Wambogo E, Moshfegh A, Sahyoun NR. Awareness and use of nutrition information predict measured and self-rated diet quality of older adults in the USA. Public Health Nutr. 2021;24(7):1687–97.
Manderbacka K, Lundberg O. Examining points of reference of self-rated health among Swedish oldest old. Arch Gerontol Geriatr. 1996;23(1):47–60.
Vasheghani-Farahani A, Tahmasbi M, Asheri H, Ashraf H, Nedjat S, Kordi R. The Persian, last 7-day, long form of the International Physical Activity Questionnaire: translation and validation study. Asian J Sports Med. 2011;2(2):106–16.
Ainsworth B, Herrmann S, Meckes N, Bassett D, Tudor-Locke C, Greer J, Vezina J, Whitt-Glover M, Leon A. 2011 Compendium of Physical activities: a second update of codes and MET values. Med Sci Sports Exerc. 2011;43:1575–81.
Physical status. The use and interpretation of anthropometry. Report of a WHO Expert Committee. World Health Organ Tech Rep Ser. 1995;854:1–452.
Google Scholar
Barba C, Cavalli-Sforza T, Cutter J, Darnton-Hill I, Deurenberg P, Yap M, Gill T, James P, Ko G, Miu AH, et al. Appropriate body Mass Index for Asian populations and its implications for policy and intervention strategies. Lancet. 2004;363:157–63.
Article Google Scholar
World Health Organisation. Waist Circumference and Waist-hip Ratio: Report of a WHO Expert Consultation, [ http://www.who.int/nutrition/publications/obesity/WHO_report_waistcircumference_and_waisthip_ratio/en/ ].
Ashwell M, Gibson S. Waist-to-height ratio as an indicator of ‘early health risk’: simpler and more predictive than using a ‘matrix’ based on BMI and waist circumference. BMJ Open. 2016;6(3):e010159.
Spengler S, Mess F, Schmocker E, Woll A. Longitudinal associations of health-related behavior patterns in adolescence with change of weight status and self-rated health over a period of 6 years: results of the MoMo longitudinal study. BMC Pediatr. 2014;14:242.
Barrios-Vicedo R, Navarrete-Muñoz EM, García de la Hera M, González-Palacios S, Valera-Gran D, Checa-Sevilla JF, Gimenez-Monzo D, Vioque J. [A lower adherence to Mediterranean diet is associated with a poorer self-rated health in university population]. Nutr Hosp. 2014;31(2):785–92.
PubMed Google Scholar
Oftedal S, Rayward AT, Fenton S, Duncan MJ. Sleep, diet, activity, and incident poor self-rated health: a population-based cohort study. Health Psychol. 2021;40(4):252–62.
Cheng G, Yan Y. Sociodemographic, health-related, and social predictors of subjective well-being among Chinese oldest-old: a national community-based cohort study. BMC Geriatr. 2021;21(1):124.
Meyer OL, Castro-Schilo L, Aguilar-Gaxiola S. Determinants of mental health and self-rated health: a model of socioeconomic status, neighborhood safety, and physical activity. Am J Public Health. 2014;104(9):1734–41.
Download references
Acknowledgements
Special thanks go to all those who participated in this study.
This manuscript has been granted by the Tehran University of Medical Sciences (Grant No: 57728).
Author information
Authors and affiliations.
Department of Community Nutrition, School of Nutritional Sciences and Dietetics, Tehran University of Medical Sciences (TUMS), No 44, Hojjat-dost Alley, Naderi St., Keshavarz Blvd, 14167-53955, Tehran, 14155/6117, Iran
Bahareh Jabbarzadeh-Ganjeh & Sakineh Shab-Bidar
Department of Clinical Nutrition, School of Nutritional Sciences and Dietetics, Tehran University of Medical Sciences (TUMS), 14167-53955, Tehran, Iran
Kurosh Djafarian
Sports Medicine Research Center, Neuroscience Institute, Tehran University of Medical Sciences (TUMS), Tehran, Iran
Sakineh Shab-Bidar
You can also search for this author in PubMed Google Scholar
Contributions
B.J-G and S.S-B contributed to the conception/design of the research; B.J-G contributed to the analysis, interpretation of the data, and drafting the first version of the manuscript; S.S-B and K.DJ critically revised the manuscript; and S.S-B agree to be fully accountable for ensuring the integrity and accuracy of the work. All authors read and approved the final manuscript.
Corresponding author
Correspondence to Sakineh Shab-Bidar .
Ethics declarations
Ethics approval and consent to participate.
The sample collection was made possible by the coordination of the Health Bureau of the Municipality of Tehran and the cooperation of the health care centers of Tehran. The study was approved by the ethical committee of the Tehran University of Medical Sciences (Ethics No. IR.TUMS.MEDICINE.REC.1401.604). All participants signed an informed consent form to participate statement.
Consent for publication
Not applicable.
Competing interests
The authors declare no competing interests.
Additional information
Publisher’s note.
Springer Nature remains neutral with regard to jurisdictional claims in published maps and institutional affiliations.
Rights and permissions
Open Access This article is licensed under a Creative Commons Attribution 4.0 International License, which permits use, sharing, adaptation, distribution and reproduction in any medium or format, as long as you give appropriate credit to the original author(s) and the source, provide a link to the Creative Commons licence, and indicate if changes were made. The images or other third party material in this article are included in the article’s Creative Commons licence, unless indicated otherwise in a credit line to the material. If material is not included in the article’s Creative Commons licence and your intended use is not permitted by statutory regulation or exceeds the permitted use, you will need to obtain permission directly from the copyright holder. To view a copy of this licence, visit http://creativecommons.org/licenses/by/4.0/ . The Creative Commons Public Domain Dedication waiver ( http://creativecommons.org/publicdomain/zero/1.0/ ) applies to the data made available in this article, unless otherwise stated in a credit line to the data.
Reprints and permissions
About this article
Cite this article.
Jabbarzadeh-Ganjeh, B., Djafarian, K. & Shab-Bidar, S. Association of healthy eating index and self-rated health in adults living in Tehran: a cross-sectional study. BMC Public Health 24 , 1106 (2024). https://doi.org/10.1186/s12889-024-18568-w
Download citation
Received : 02 November 2023
Accepted : 11 April 2024
Published : 22 April 2024
DOI : https://doi.org/10.1186/s12889-024-18568-w
Share this article
Anyone you share the following link with will be able to read this content:
Sorry, a shareable link is not currently available for this article.
Provided by the Springer Nature SharedIt content-sharing initiative
- Self-assessment
- Self-rated health
BMC Public Health
ISSN: 1471-2458
- Submission enquiries: [email protected]
- General enquiries: [email protected]
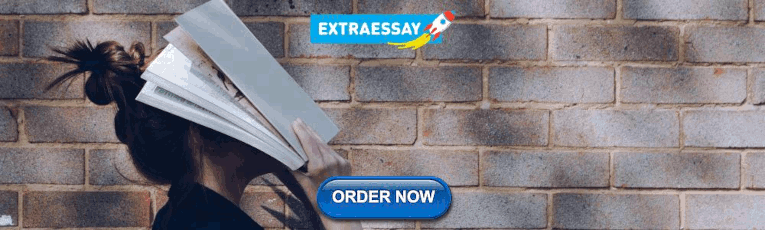
IMAGES
VIDEO
COMMENTS
Cross-Sectional Design. Cross-sectional designs help determine the prevalence of a disease, phenomena, or opinion in a population, as represented by a study sample.Prevalence is the proportion of people in a population (sample) who have an attribute or condition at a specific time point regardless of when the attribute or condition first developed (Wang & Cheng, 2020).
A cross-sectional study is a type of research design in which you collect data from many different individuals at a single point in time. In cross-sectional research, you observe variables without influencing them. Researchers in economics, psychology, medicine, epidemiology, and the other social sciences all make use of cross-sectional studies ...
A cross-sectional study is a cheap and easy way to gather initial data and identify correlations that can then be investigated further in a longitudinal study. Example: Cross-sectional vs longitudinal. You want to study the impact that a low-carb diet has on diabetes. You first conduct a cross-sectional study with a sample of diabetes patients ...
Cross-Sectional vs. Longitudinal. A cross-sectional study design is a type of observational study, or descriptive research, that involves analyzing information about a population at a specific point in time. This design measures the prevalence of an outcome of interest in a defined population. It provides a snapshot of the characteristics of ...
A cross-sectional study is an experimental design that analyzes data from a representative sample at a specific point in time. Researchers usually evaluate multiple attributes at once when using this design. Unlike longitudinal studies, these studies don't track changes over time. These studies are a snapshot of a moment in time.
Section 1.3 Effects of familial characteristics on dental fear: a cross-sectional study 24. In a 2019 cross-sectional study, Osama Felemban et al observed dental fear in children and how it may affect the child's dental treatment and behavior at the dental office. In this study, 16 middle schools in Jeddah, Saudi Arabia were randomly selected.
A cross-sectional study looks at data at a single point in time. The participants in this type of study are selected based on particular variables of interest. Cross-sectional studies are often used in developmental psychology, but this method is also used in many other areas, including social science and education.
In medical research, social science, and biology, a cross-sectional study (also known as a cross-sectional analysis, transverse study, prevalence study) is a type of observational study that analyzes data from a population, or a representative subset, at a specific point in time—that is, cross-sectional data. [definition needed]In economics, cross-sectional studies typically involve the use ...
3.1. Strengths: when to use cross-sectional data. The strengths of cross-sectional data help to explain their overuse in IS research. First, such studies can be conducted efficiently and inexpensively by distributing a survey to a convenient sample (e.g., the researcher's social network or students) (Compeau et al., 2012) or by using a crowdsourcing website (Lowry et al., 2016, Steelman et ...
In medical research, a cross-sectional study is a type of observational study design that involves looking at data from a population at one specific point in time. In a cross-sectional study, investigators measure outcomes and exposures of the study subjects at the same time. It is described as taking a "snapshot" of a group of individuals.
The resulting formula for the sample size for testing a difference in prevalence using a one-sided test is as follows: and for this example, n can be calculated as: n = 1 d 2 [ z α π 0 ( 1 − π 0) + z β π 1 ( 1 − π 1)] 2. Replace z α by z α / 2 for a two-sided test. Take a moment to look at the table below for sample size ...
Cross sectional studies can be done quickly and are the best way to determine prevalence; they are particularly useful for studying infectious diseases. ... It is usually possible to blind the subjects and observers to the study hypothesis by asking spurious questions. Observers can also be easily blinded to the case or control status of the ...
Cross-sectional Studies E R I C N O T E B O O K S E R I E S Like cohort studies, cross-sectional studies conceptually begin with a population base. But unlike cohort studies, in cross-sectional studies we do not follow individuals over time. Instead, we only look at the prevalence of disease and/or exposure at one moment in time.
Cross sectional studies are common and include surveys, laboratory experiments and studies to examine the prevalence of a disease. ... For example, in a prevalence study there is no hypothesis to test, and the size of the study is determined by how accurately the investigator wants to determine the prevalence. If there is no hypothesis, then ...
As is the case for most study types a larger sample size gives greater power and is more ideal for a strong study design. Within a cross-sectional study a sample size of at least 60 participants is recommended, although this will depend on suitability to the research question and the variables being measured. A suitable number of variables.
Deakin University. As we all know, Hypothesis is a tentative explanation for an observation. The hypothesis for the given research question could be stated as Null hypothesis (Ho) or Alternative ...
Design and setting A cross-sectional ecological study of patient life expectancy from 2015-2019. Method Selection of independent variables was based on conceptual frameworks describing general practice's influence on outcomes. ... increased numbers of practice staff (hypothesis 1); improved access and continuity (hypothesis 2); and. better ...
This cross sectional study was conducted after approval from the Ethics Committee of the City of Vienna had been obtained (EK 20-352-VK) and adhered to the tenets of the Declaration of Helsinki. Due to the retrospective character of the study, informed consent was waived in agreement with the positive vote of the above-mentioned Ethics Committee.
Cross-sectional studies offer information that's well-suited for descriptive analysis. If researchers want to develop a general hypothesis, then cross-sectional studies are the best way to generate specific situations that face a particular demographic. Each description of the critical data points creates the possibility of forwarding ...
In this cross-sectional study, the circulating TFAs were investigated to be positively associated with SII, and a nonlinear relationship was found. Notably, these associations could be more weakened or more pronounced in different subgroups. Briefly, the findings of the study emphasize the potential role of TFAs in systemic inflammation ...
Self-rated health (SRH) has been identified in many studies as a valid predictor of mortality and healthcare utilization. There is limited research on SRH and dietary intake. This study aimed to investigate the association between healthy eating index (HEI) and SRH in adults living in Tehran. This cross-sectional study was carried out among 850 adult men and women aged 20-59 years who ...
Background/Objectives: Cholesteatoma presents significant management challenges in otolaryngology. This study aimed to delineate the influence of demographic and clinical characteristics, preoperative imaging, and surgical approaches on treatment success in cholesteatoma management. Methods: A cross-sectional analytical study was conducted at the Otolaryngology Department of the University ...
Background: Long COVID presents a concern for collegiate athletes, potentially impacting sensorimotor processing and motor fitness. This study aimed to assess these effects. Methods: This cross-sectional study involved 60 athletes diagnosed with Long COVID and 60 controls. Sensorimotor processing and integration were evaluated using neurophysiological variables (N13, P14, N20, P27, and N30 ...
Consequently, this study's cross-sectional design restricts our ability to infer causal relationships and understand the mechanisms between myopia and age-related eye diseases. We focused on cataracts, glaucoma, and macular degeneration, which, while significant, limits our insight into the severity and progression of these conditions. ...
This cross-sectional retrospective study was approved by the ethics committee of Fırat University Faculty of Medicine (03.10.2019; approval number 15) and was conducted in accordance with the ethical standards of the 1975 Declaration of Helsinki (revised in 2000) and the National and Institutional Committee on Human Rights Watch. 17 As this ...