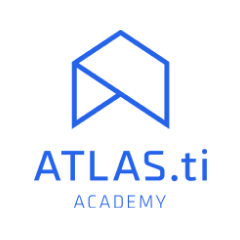
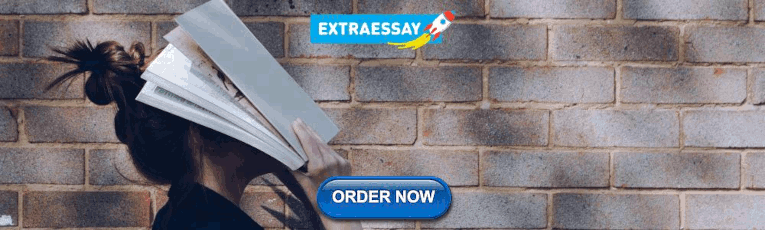
The Ultimate Guide to Qualitative Research - Part 1: The Basics
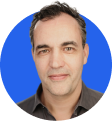
- Introduction and overview
- What is qualitative research?
- What is qualitative data?
- Examples of qualitative data
- Qualitative vs. quantitative research
- Mixed methods
- Qualitative research preparation
- Theoretical perspective
- Theoretical framework
- Literature reviews
- Research question
- Conceptual framework
- Conceptual vs. theoretical framework
- Data collection
- Qualitative research methods
- Focus groups
- Observational research
- Case studies
- Ethnographical research
- Ethical considerations
- Confidentiality and privacy
What is research bias?
Understanding unconscious bias, how to avoid bias in research, bias and subjectivity in research.
- Power dynamics
- Reflexivity
Bias in research
In a purely objective world, research bias would not exist because knowledge would be a fixed and unmovable resource; either one knows about a particular concept or phenomenon, or they don't. However, qualitative research and the social sciences both acknowledge that subjectivity and bias exist in every aspect of the social world, which naturally includes the research process too. This bias is manifest in the many different ways that knowledge is understood, constructed, and negotiated, both in and out of research.
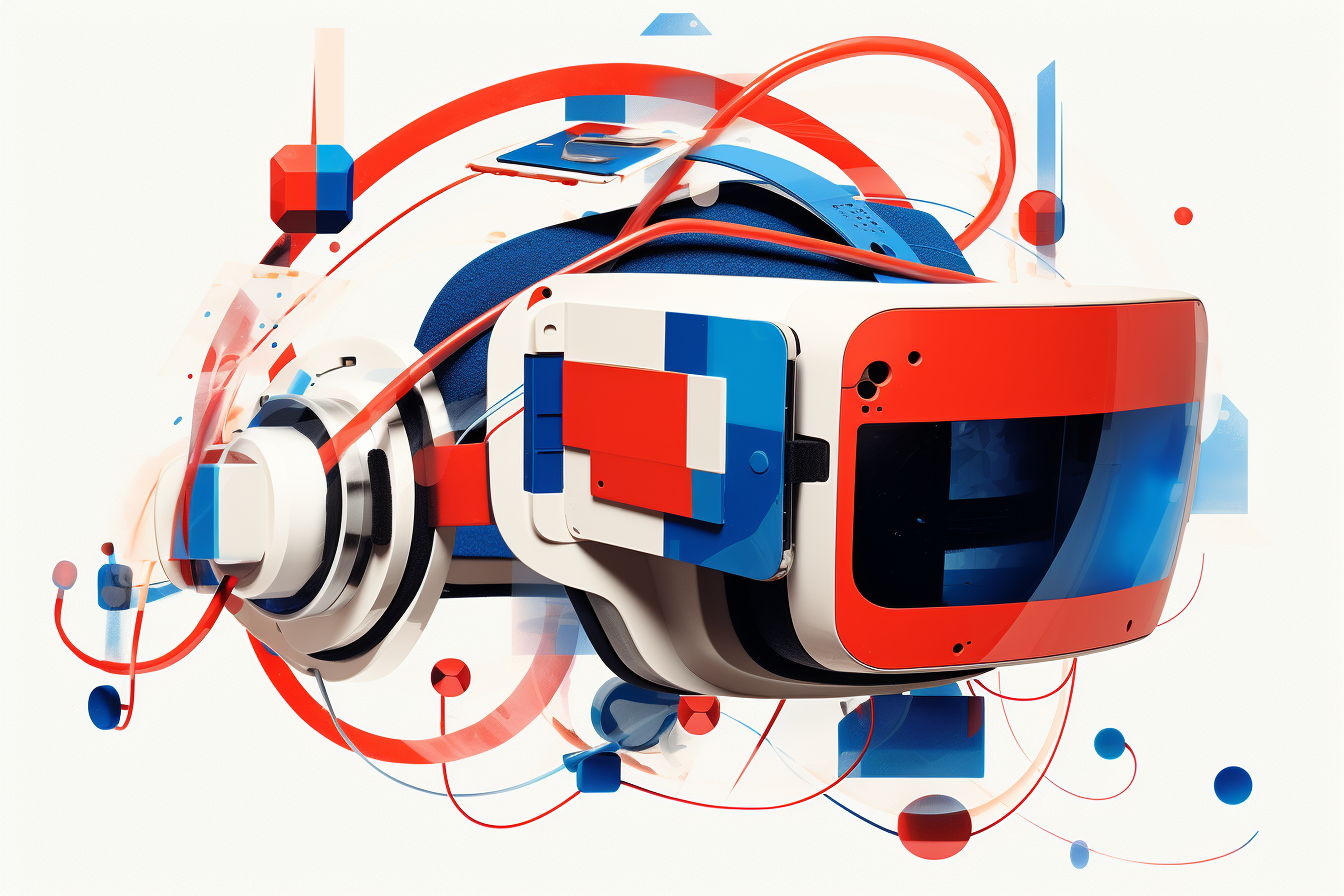
Understanding research bias has profound implications for data collection methods and data analysis , requiring researchers to take particular care of how to account for the insights generated from their data .
Research bias, often unavoidable, is a systematic error that can creep into any stage of the research process , skewing our understanding and interpretation of findings. From data collection to analysis, interpretation , and even publication , bias can distort the truth we seek to capture and communicate in our research.
It’s also important to distinguish between bias and subjectivity, especially when engaging in qualitative research . Most qualitative methodologies are based on epistemological and ontological assumptions that there is no such thing as a fixed or objective world that exists “out there” that can be empirically measured and understood through research. Rather, many qualitative researchers embrace the socially constructed nature of our reality and thus recognize that all data is produced within a particular context by participants with their own perspectives and interpretations. Moreover, the researcher’s own subjective experiences inevitably shape how they make sense of the data. These subjectivities are considered to be strengths, not limitations, of qualitative research approaches, because they open new avenues for knowledge generation. This is also why reflexivity is so important in qualitative research. When we refer to bias in this guide, on the other hand, we are referring to systematic errors that can negatively affect the research process but that can be mitigated through researchers’ careful efforts.
To fully grasp what research bias is, it's essential to understand the dual nature of bias. Bias is not inherently evil. It's simply a tendency, inclination, or prejudice for or against something. In our daily lives, we're subject to countless biases, many of which are unconscious. They help us navigate our world, make quick decisions, and understand complex situations. But when conducting research, these same biases can cause significant issues.
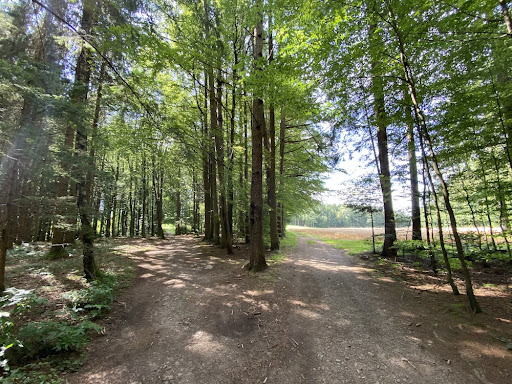
Research bias can affect the validity and credibility of research findings, leading to erroneous conclusions. It can emerge from the researcher's subconscious preferences or the methodological design of the study itself. For instance, if a researcher unconsciously favors a particular outcome of the study, this preference could affect how they interpret the results, leading to a type of bias known as confirmation bias.
Research bias can also arise due to the characteristics of study participants. If the researcher selectively recruits participants who are more likely to produce desired outcomes, this can result in selection bias.
Another form of bias can stem from data collection methods . If a survey question is phrased in a way that encourages a particular response, this can introduce response bias. Moreover, inappropriate survey questions can have a detrimental effect on future research if such studies are seen by the general population as biased toward particular outcomes depending on the preferences of the researcher.
Bias can also occur during data analysis . In qualitative research for instance, the researcher's preconceived notions and expectations can influence how they interpret and code qualitative data, a type of bias known as interpretation bias. It's also important to note that quantitative research is not free of bias either, as sampling bias and measurement bias can threaten the validity of any research findings.
Given these examples, it's clear that research bias is a complex issue that can take many forms and emerge at any stage in the research process. This section will delve deeper into specific types of research bias, provide examples, discuss why it's an issue, and provide strategies for identifying and mitigating bias in research.
What is an example of bias in research?
Bias can appear in numerous ways. One example is confirmation bias, where the researcher has a preconceived explanation for what is going on in their data, and any disconfirming evidence is (unconsciously) ignored. For instance, a researcher conducting a study on daily exercise habits might be inclined to conclude that meditation practices lead to greater engagement in exercise because that researcher has personally experienced these benefits. However, conducting rigorous research entails assessing all the data systematically and verifying one’s conclusions by checking for both supporting and refuting evidence.
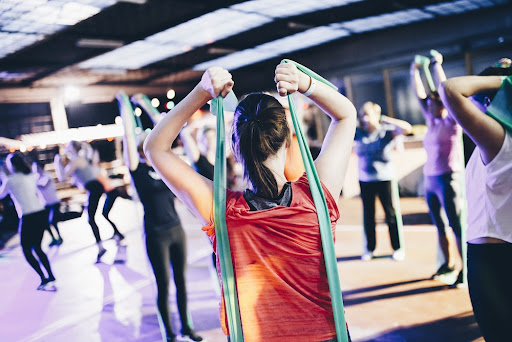
What is a common bias in research?
Confirmation bias is one of the most common forms of bias in research. It happens when researchers unconsciously focus on data that supports their ideas while ignoring or undervaluing data that contradicts their ideas. This bias can lead researchers to mistakenly confirm their theories, despite having insufficient or conflicting evidence.
What are the different types of bias?
There are several types of research bias, each presenting unique challenges. Some common types include:
Confirmation bias: As already mentioned, this happens when a researcher focuses on evidence supporting their theory while overlooking contradictory evidence.
Selection bias: This occurs when the researcher's method of choosing participants skews the sample in a particular direction.
Response bias: This happens when participants in a study respond inaccurately or falsely, often due to misleading or poorly worded questions.
Observer bias (or researcher bias): This occurs when the researcher unintentionally influences the results because of their expectations or preferences.
Publication bias: This type of bias arises when studies with positive results are more likely to get published, while studies with negative or null results are often ignored.
Analysis bias: This type of bias occurs when the data is manipulated or analyzed in a way that leads to a particular result, whether intentionally or unintentionally.
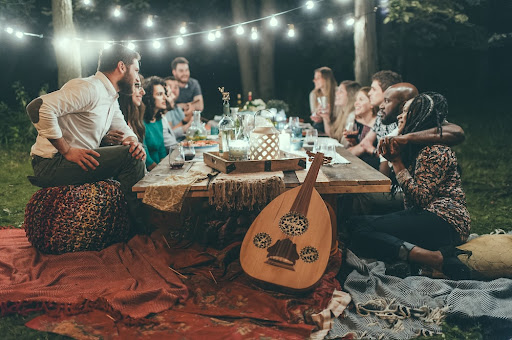
What is an example of researcher bias?
Researcher bias, also known as observer bias, can occur when a researcher's expectations or personal beliefs influence the results of a study. For instance, if a researcher believes that a particular therapy is effective, they might unconsciously interpret ambiguous results in a way that supports the efficacy of the therapy, even if the evidence is not strong enough.
Even quantitative research methodologies are not immune from bias from researchers. Market research surveys or clinical trial research, for example, may encounter bias when the researcher chooses a particular population or methodology to achieve a specific research outcome. Questions in customer feedback surveys whose data is employed in quantitative analysis can be structured in such a way as to bias survey respondents toward certain desired answers.
Turn your data into findings with ATLAS.ti
Key insights are at your fingertips with our powerful interface. See how with a free trial.
Identifying and avoiding bias in research
As we will remind you throughout this chapter, bias is not a phenomenon that can be removed altogether, nor should we think of it as something that should be eliminated. In a subjective world involving humans as researchers and research participants, bias is unavoidable and almost necessary for understanding social behavior. The section on reflexivity later in this guide will highlight how different perspectives among researchers and human subjects are addressed in qualitative research. That said, bias in excess can place the credibility of a study's findings into serious question. Scholars who read your research need to know what new knowledge you are generating, how it was generated, and why the knowledge you present should be considered persuasive. With that in mind, let's look at how bias can be identified and, where it interferes with research, minimized.
How do you identify bias in research?
Identifying bias involves a critical examination of your entire research study involving the formulation of the research question and hypothesis , the selection of study participants, the methods for data collection, and the analysis and interpretation of data. Researchers need to assess whether each stage has been influenced by bias that may have skewed the results. Tools such as bias checklists or guidelines, peer review , and reflexivity (reflecting on one's own biases) can be instrumental in identifying bias.
How do you identify research bias?
Identifying research bias often involves careful scrutiny of the research methodology and the researcher's interpretations. Was the sample of participants relevant to the research question ? Were the interview or survey questions leading? Were there any conflicts of interest that could have influenced the results? It also requires an understanding of the different types of bias and how they might manifest in a research context. Does the bias occur in the data collection process or when the researcher is analyzing data?
Research transparency requires a careful accounting of how the study was designed, conducted, and analyzed. In qualitative research involving human subjects, the researcher is responsible for documenting the characteristics of the research population and research context. With respect to research methods, the procedures and instruments used to collect and analyze data are described in as much detail as possible.
While describing study methodologies and research participants in painstaking detail may sound cumbersome, a clear and detailed description of the research design is necessary for good research. Without this level of detail, it is difficult for your research audience to identify whether bias exists, where bias occurs, and to what extent it may threaten the credibility of your findings.
How to recognize bias in a study?
Recognizing bias in a study requires a critical approach. The researcher should question every step of the research process: Was the sample of participants selected with care? Did the data collection methods encourage open and sincere responses? Did personal beliefs or expectations influence the interpretation of the results? External peer reviews can also be helpful in recognizing bias, as others might spot potential issues that the original researcher missed.
The subsequent sections of this chapter will delve into the impacts of research bias and strategies to avoid it. Through these discussions, researchers will be better equipped to handle bias in their work and contribute to building more credible knowledge.
Unconscious biases, also known as implicit biases, are attitudes or stereotypes that influence our understanding, actions, and decisions in an unconscious manner. These biases can inadvertently infiltrate the research process, skewing the results and conclusions. This section aims to delve deeper into understanding unconscious bias, its impact on research, and strategies to mitigate it.
What is unconscious bias?
Unconscious bias refers to prejudices or social stereotypes about certain groups that individuals form outside their conscious awareness. Everyone holds unconscious beliefs about various social and identity groups, and these biases stem from a tendency to organize social worlds into categories.
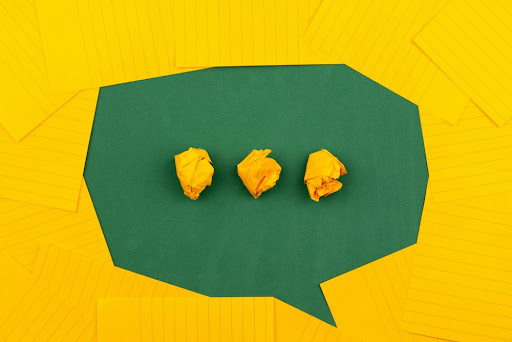
How does unconscious bias infiltrate research?
Unconscious bias can infiltrate research in several ways. It can affect how researchers formulate their research questions or hypotheses , how they interact with participants, their data collection methods, and how they interpret their data . For instance, a researcher might unknowingly favor participants who share similar characteristics with them, which could lead to biased results.
Implications of unconscious bias
The implications of unconscious research bias are far-reaching. It can compromise the validity of research findings , influence the choice of research topics, and affect peer review processes . Unconscious bias can also lead to a lack of diversity in research, which can severely limit the value and impact of the findings.
Strategies to mitigate unconscious research bias
While it's challenging to completely eliminate unconscious bias, several strategies can help mitigate its impact. These include being aware of potential unconscious biases, practicing reflexivity , seeking diverse perspectives for your study, and engaging in regular bias-checking activities, such as bias training and peer debriefing .
By understanding and acknowledging unconscious bias, researchers can take steps to limit its impact on their work, leading to more robust findings.
Why is researcher bias an issue?
Research bias is a pervasive issue that researchers must diligently consider and address. It can significantly impact the credibility of findings. Here, we break down the ramifications of bias into two key areas.
How bias affects validity
Research validity refers to the accuracy of the study findings, or the coherence between the researcher’s findings and the participants’ actual experiences. When bias sneaks into a study, it can distort findings and move them further away from the realities that were shared by the research participants. For example, if a researcher's personal beliefs influence their interpretation of data , the resulting conclusions may not reflect what the data show or what participants experienced.
The transferability problem
Transferability is the extent to which your study's findings can be applied beyond the specific context or sample studied. Applying knowledge from one context to a different context is how we can progress and make informed decisions. In quantitative research , the generalizability of a study is a key component that shapes the potential impact of the findings. In qualitative research , all data and knowledge that is produced is understood to be embedded within a particular context, so the notion of generalizability takes on a slightly different meaning. Rather than assuming that the study participants are statistically representative of the entire population, qualitative researchers can reflect on which aspects of their research context bear the most weight on their findings and how these findings may be transferable to other contexts that share key similarities.
How does bias affect research?
Research bias, if not identified and mitigated, can significantly impact research outcomes. The ripple effects of research bias extend beyond individual studies, impacting the body of knowledge in a field and influencing policy and practice. Here, we delve into three specific ways bias can affect research.
Distortion of research results
Bias can lead to a distortion of your study's findings. For instance, confirmation bias can cause a researcher to focus on data that supports their interpretation while disregarding data that contradicts it. This can skew the results and create a misleading picture of the phenomenon under study.
Undermining scientific progress
When research is influenced by bias, it not only misrepresents participants’ realities but can also impede scientific progress. Biased studies can lead researchers down the wrong path, resulting in wasted resources and efforts. Moreover, it could contribute to a body of literature that is skewed or inaccurate, misleading future research and theories.
Influencing policy and practice based on flawed findings
Research often informs policy and practice. If the research is biased, it can lead to the creation of policies or practices that are ineffective or even harmful. For example, a study with selection bias might conclude that a certain intervention is effective, leading to its broad implementation. However, suppose the transferability of the study's findings was not carefully considered. In that case, it may be risky to assume that the intervention will work as well in different populations, which could lead to ineffective or inequitable outcomes.
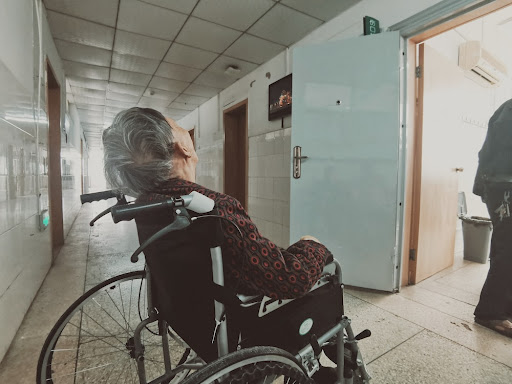
While it's almost impossible to eliminate bias in research entirely, it's crucial to mitigate its impact as much as possible. By employing thoughtful strategies at every stage of research, we can strive towards rigor and transparency , enhancing the quality of our findings. This section will delve into specific strategies for avoiding bias.
How do you know if your research is biased?
Determining whether your research is biased involves a careful review of your research design, data collection , analysis , and interpretation . It might require you to reflect critically on your own biases and expectations and how these might have influenced your research. External peer reviews can also be instrumental in spotting potential bias.
Strategies to mitigate bias
Minimizing bias involves careful planning and execution at all stages of a research study. These strategies could include formulating clear, unbiased research questions , ensuring that your sample meaningfully represents the research problem you are studying, crafting unbiased data collection instruments, and employing systematic data analysis techniques. Transparency and reflexivity throughout the process can also help minimize bias.
Mitigating bias in data collection
To mitigate bias in data collection, ensure your questions are clear, neutral, and not leading. Triangulation, or using multiple methods or data sources, can also help to reduce bias and increase the credibility of your findings.
Mitigating bias in data analysis
During data analysis , maintaining a high level of rigor is crucial. This might involve using systematic coding schemes in qualitative research or appropriate statistical tests in quantitative research . Regularly questioning your interpretations and considering alternative explanations can help reduce bias. Peer debriefing , where you discuss your analysis and interpretations with colleagues, can also be a valuable strategy.
By using these strategies, researchers can significantly reduce the impact of bias on their research, enhancing the quality and credibility of their findings and contributing to a more robust and meaningful body of knowledge.
Impact of cultural bias in research
Cultural bias is the tendency to interpret and judge phenomena by standards inherent to one's own culture. Given the increasingly multicultural and global nature of research, understanding and addressing cultural bias is paramount. This section will explore the concept of cultural bias, its impacts on research, and strategies to mitigate it.
What is cultural bias in research?
Cultural bias refers to the potential for a researcher's cultural background, experiences, and values to influence the research process and findings. This can occur consciously or unconsciously and can lead to misinterpretation of data, unfair representation of cultures, and biased conclusions.
How does cultural bias infiltrate research?
Cultural bias can infiltrate research at various stages. It can affect the framing of research questions , the design of the study, the methods of data collection , and the interpretation of results . For instance, a researcher might unintentionally design a study that does not consider the cultural context of the participants, leading to a biased understanding of the phenomenon being studied.
Implications of cultural bias
The implications of cultural bias are profound. Cultural bias can skew your findings, limit the transferability of results, and contribute to cultural misunderstandings and stereotypes. This can ultimately lead to inaccurate or ethnocentric conclusions, further perpetuating cultural bias and inequities.
As a result, many social science fields like sociology and anthropology have been critiqued for cultural biases in research. Some of the earliest research inquiries in anthropology, for example, have had the potential to reduce entire cultures to simplistic stereotypes when compared to mainstream norms. A contemporary researcher respecting ethical and cultural boundaries, on the other hand, should seek to properly place their understanding of social and cultural practices in sufficient context without inappropriately characterizing them.
Strategies to mitigate cultural bias
Mitigating cultural bias requires a concerted effort throughout the research study. These efforts could include educating oneself about other cultures, being aware of one's own cultural biases, incorporating culturally diverse perspectives into the research process, and being sensitive and respectful of cultural differences. It might also involve including team members with diverse cultural backgrounds or seeking external cultural consultants to challenge assumptions and provide alternative perspectives.
By acknowledging and addressing cultural bias, researchers can contribute to more culturally competent, equitable, and valid research. This not only enriches the scientific body of knowledge but also promotes cultural understanding and respect.
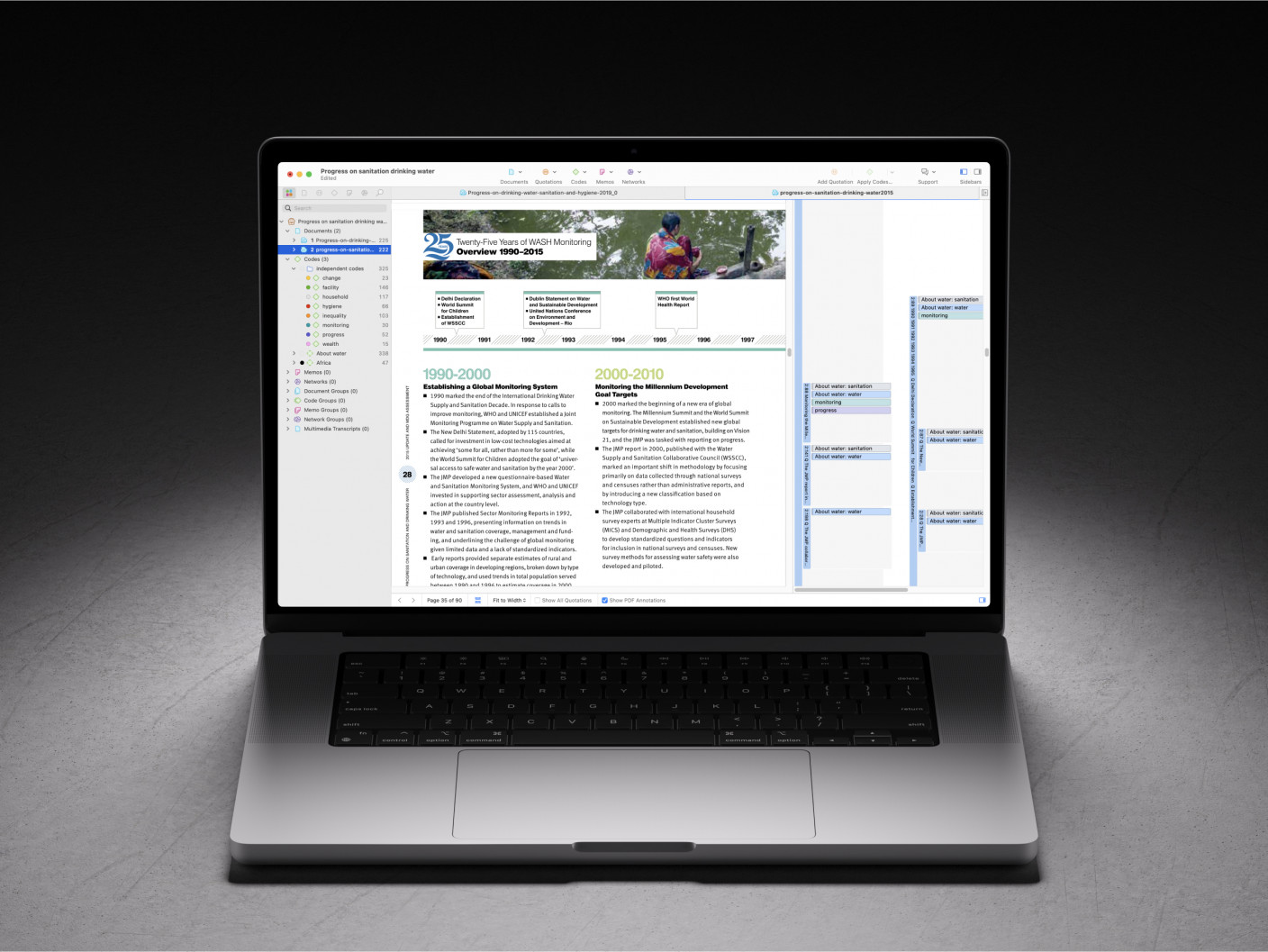
Ready to jumpstart your research with ATLAS.ti?
Conceptualize your research project with our intuitive data analysis interface. Download a free trial today.
Keep in mind that bias is a force to be mitigated, not a phenomenon that can be eliminated altogether, and the subjectivities of each person are what make our world so complex and interesting. As things are continuously changing and adapting, research knowledge is also continuously being updated as we further develop our understanding of the world around us.
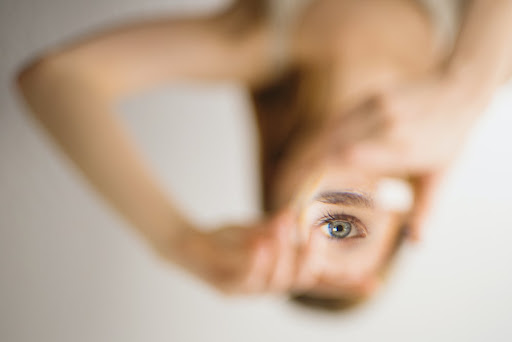
Ready to analyze your data with ATLAS.ti?
See how our intuitive software can draw key insights from your data with a free trial today.

How to Avoid Bias in Qualitative Research
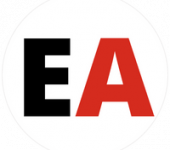
You can also listen to this article as an audio recording.
Research bias occurs when researchers try to influence the results of their work, in order to get the outcome they want. Often, researchers may not be aware they are doing this. Whether they are aware or not, such behavior clearly severely affects the impartiality of a study and greatly reduces the value of the results.
The Issues in Qualitative Research
Recently, I discussed the problem of bias with a researcher friend.
“I heard that research bias is a bigger problem for qualitative research than quantitative research.”
“Why is that?”
“Qualitative research relies more on the experience and judgment of the researcher. Also, the type of data collected is subjective and unique to the person or situation. So it is much harder to avoid bias than in quantitative research.”
“Are there ways to avoid bias ?”
“A good start is to recognize that bias exists in all research. We can then try to predict what type of bias we might have in our study, and try to avoid it as much as possible.”
Types of Bias in Research
“Are there different types of bias to watch out for?”
- There’s design bias , where the researcher does not consider bias in the design of the study. Factors like sample size , the range of participants, for example – all of these can cause bias.
- Next there’s also selection or sampling bias . For example, you might omit people of certain ages or ethnicities from your study. This is called omission bias. The other type, inclusive bias, is when you select a sample just because it is convenient. For example, if the people you select for your study are all college students, they are likely to share many characteristics.”
“Are there more?”
“Yes, there are lots of different types of bias.
- There’s procedural bias , where the way you carry out a study affects the results. For example, if you give people only a short time to answer questions, their responses will be rushed.
- There’s also measurement bias that can happen if the equipment you are using is faulty, or you are not using it correctly.”
“That’s a lot to think about.”
“I can think of three more.
- There’s interviewer bias , which is very hard to avoid. This is when an interviewer subconsciously influences the responses of the interviewee. Their body language might indicate their opinion, for example.
- Furthermore, there’s response bias , where someone tries to give the answers they think are “correct.”
- Finally, there’s reporting bias . This is often outside the researcher’s control. It means that research with positive, or exciting, results is far more likely to be reported, so can seem more critical.”
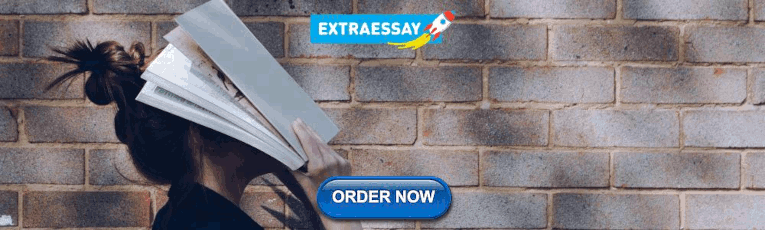
How to Avoid Bias in Research
“With so many types of bias, how can it be avoided?”
“There are a number of things the researcher can do to avoid bias.
- Read the guidelines : Check the guidelines of your institution or sponsor and make sure you follow them.
- Think about our objectives : Plan your study early. Be clear about what you want to achieve, and how. This will help to avoid bias when you start collecting data.”
“And next?”
- Maintain records : Keep detailed records. This reduces the chance of making mistakes.
- Be honest when reporting : Make sure you include all your results in your report. Even the results that don’t seem important. Finally, be honest about the limitations of your study in your report.”
Avoiding Participant Bias
“That explains what researchers can do. But what about participant bias?”
“Try asking indirect questions. People might change their answers to direct questions to make a good impression. But if you ask them what a friend or colleague might think, you might get a more honest response.”
“Are open-ended questions useful?”
“Yes. They allow information to flow more freely, by not forcing a limited set of answers. But even these should be used with caution . You should try to be impartial about all parts of the study, and avoid implying that there is a right answer. It might help to ask people to rate their responses on a scale of 1-5, for example, rather than agree/disagree.”
Reducing Researcher Bias
“All researchers should try to avoid confirmation bias. This is when you interpret your data in a way that supports your hypothesis. Secondly, you should make sure to analyze all your data, even if it doesn’t seem useful. Finally, always get an independent person to check your work, ideally several times during your study.”
Identifying and avoiding research bias in qualitative research is clearly tricky, with many different factors to consider. However, it is also vital. Biased research has little value; it is a waste of researchers’ valuable time and resources.
Learn even more about bias here . How did you overcome bias in your research? Share your experiences and thoughts in the comment section below.

Can you please tell me who is the author of this article: How to Avoid Bias in Qualitative Research Last updated May 3, 2019
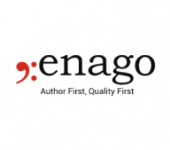
Hi Nuzhat, the author of this article is Enago Academy
Rate this article Cancel Reply
Your email address will not be published.
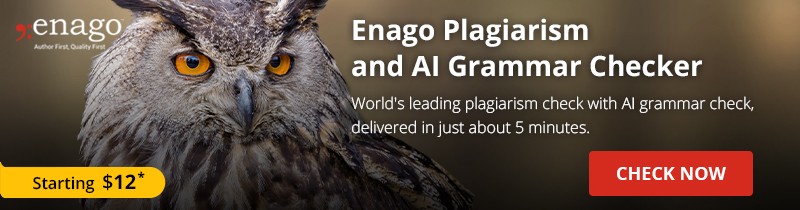
Enago Academy's Most Popular Articles
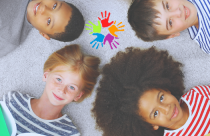
- Diversity and Inclusion
Need for Diversifying Academic Curricula: Embracing missing voices and marginalized perspectives
In classrooms worldwide, a single narrative often dominates, leaving many students feeling lost. These stories,…
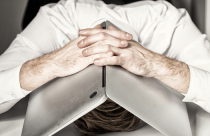
- Career Corner
- Trending Now
Recognizing the signs: A guide to overcoming academic burnout
As the sun set over the campus, casting long shadows through the library windows, Alex…
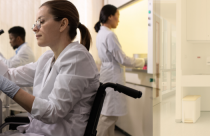
Reassessing the Lab Environment to Create an Equitable and Inclusive Space
The pursuit of scientific discovery has long been fueled by diverse minds and perspectives. Yet…
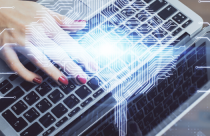
- AI in Academia
- Reporting Research
How to Improve Lab Report Writing: Best practices to follow with and without AI-assistance
Imagine you’re a scientist who just made a ground-breaking discovery! You want to share your…
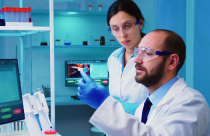
Achieving Research Excellence: Checklist for good research practices
Academia is built on the foundation of trustworthy and high-quality research, supported by the pillars…
7 Steps of Writing an Excellent Academic Book Chapter
When Your Thesis Advisor Asks You to Quit
Virtual Defense: Top 5 Online Thesis Defense Tips

Sign-up to read more
Subscribe for free to get unrestricted access to all our resources on research writing and academic publishing including:
- 2000+ blog articles
- 50+ Webinars
- 10+ Expert podcasts
- 50+ Infographics
- 10+ Checklists
- Research Guides
We hate spam too. We promise to protect your privacy and never spam you.
I am looking for Editing/ Proofreading services for my manuscript Tentative date of next journal submission:
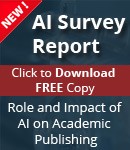
What should universities' stance be on AI tools in research and academic writing?

Research Bias 101: What You Need To Know
By: Derek Jansen (MBA) | Expert Reviewed By: Dr Eunice Rautenbach | September 2022
If you’re new to academic research, research bias (also sometimes called researcher bias) is one of the many things you need to understand to avoid compromising your study. If you’re not careful, research bias can ruin the credibility of your study.
In this post, we’ll unpack the thorny topic of research bias. We’ll explain what it is , look at some common types of research bias and share some tips to help you minimise the potential sources of bias in your research.
Overview: Research Bias 101
- What is research bias (or researcher bias)?
- Bias #1 – Selection bias
- Bias #2 – Analysis bias
- Bias #3 – Procedural (admin) bias
So, what is research bias?
Well, simply put, research bias is when the researcher – that’s you – intentionally or unintentionally skews the process of a systematic inquiry , which then of course skews the outcomes of the study . In other words, research bias is what happens when you affect the results of your research by influencing how you arrive at them.
For example, if you planned to research the effects of remote working arrangements across all levels of an organisation, but your sample consisted mostly of management-level respondents , you’d run into a form of research bias. In this case, excluding input from lower-level staff (in other words, not getting input from all levels of staff) means that the results of the study would be ‘biased’ in favour of a certain perspective – that of management.
Of course, if your research aims and research questions were only interested in the perspectives of managers, this sampling approach wouldn’t be a problem – but that’s not the case here, as there’s a misalignment between the research aims and the sample .
Now, it’s important to remember that research bias isn’t always deliberate or intended. Quite often, it’s just the result of a poorly designed study, or practical challenges in terms of getting a well-rounded, suitable sample. While perfect objectivity is the ideal, some level of bias is generally unavoidable when you’re undertaking a study. That said, as a savvy researcher, it’s your job to reduce potential sources of research bias as much as possible.
To minimize potential bias, you first need to know what to look for . So, next up, we’ll unpack three common types of research bias we see at Grad Coach when reviewing students’ projects . These include selection bias , analysis bias , and procedural bias . Keep in mind that there are many different forms of bias that can creep into your research, so don’t take this as a comprehensive list – it’s just a useful starting point.
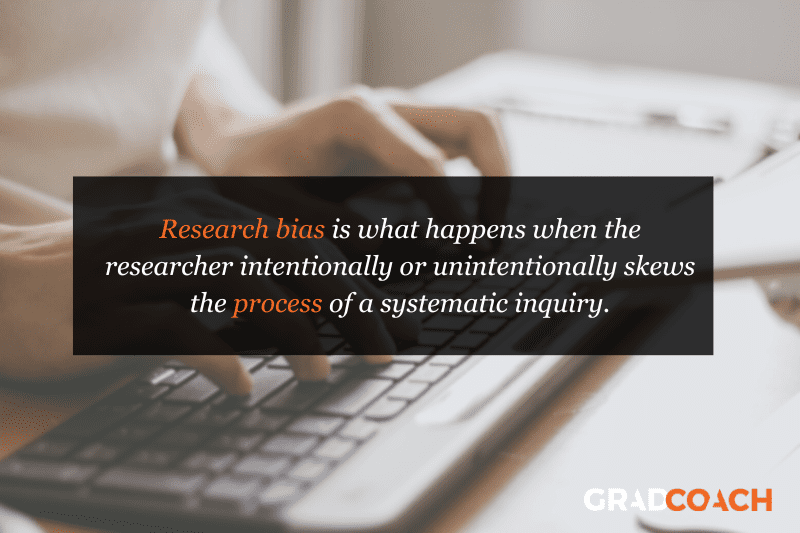
Bias #1 – Selection Bias
First up, we have selection bias . The example we looked at earlier (about only surveying management as opposed to all levels of employees) is a prime example of this type of research bias. In other words, selection bias occurs when your study’s design automatically excludes a relevant group from the research process and, therefore, negatively impacts the quality of the results.
With selection bias, the results of your study will be biased towards the group that it includes or favours, meaning that you’re likely to arrive at prejudiced results . For example, research into government policies that only includes participants who voted for a specific party is going to produce skewed results, as the views of those who voted for other parties will be excluded.
Selection bias commonly occurs in quantitative research , as the sampling strategy adopted can have a major impact on the statistical results . That said, selection bias does of course also come up in qualitative research as there’s still plenty room for skewed samples. So, it’s important to pay close attention to the makeup of your sample and make sure that you adopt a sampling strategy that aligns with your research aims. Of course, you’ll seldom achieve a perfect sample, and that okay. But, you need to be aware of how your sample may be skewed and factor this into your thinking when you analyse the resultant data.
Need a helping hand?
Bias #2 – Analysis Bias
Next up, we have analysis bias . Analysis bias occurs when the analysis itself emphasises or discounts certain data points , so as to favour a particular result (often the researcher’s own expected result or hypothesis). In other words, analysis bias happens when you prioritise the presentation of data that supports a certain idea or hypothesis , rather than presenting all the data indiscriminately .
For example, if your study was looking into consumer perceptions of a specific product, you might present more analysis of data that reflects positive sentiment toward the product, and give less real estate to the analysis that reflects negative sentiment. In other words, you’d cherry-pick the data that suits your desired outcomes and as a result, you’d create a bias in terms of the information conveyed by the study.
Although this kind of bias is common in quantitative research, it can just as easily occur in qualitative studies, given the amount of interpretive power the researcher has. This may not be intentional or even noticed by the researcher, given the inherent subjectivity in qualitative research. As humans, we naturally search for and interpret information in a way that confirms or supports our prior beliefs or values (in psychology, this is called “confirmation bias”). So, don’t make the mistake of thinking that analysis bias is always intentional and you don’t need to worry about it because you’re an honest researcher – it can creep up on anyone .
To reduce the risk of analysis bias, a good starting point is to determine your data analysis strategy in as much detail as possible, before you collect your data . In other words, decide, in advance, how you’ll prepare the data, which analysis method you’ll use, and be aware of how different analysis methods can favour different types of data. Also, take the time to reflect on your own pre-conceived notions and expectations regarding the analysis outcomes (in other words, what do you expect to find in the data), so that you’re fully aware of the potential influence you may have on the analysis – and therefore, hopefully, can minimize it.
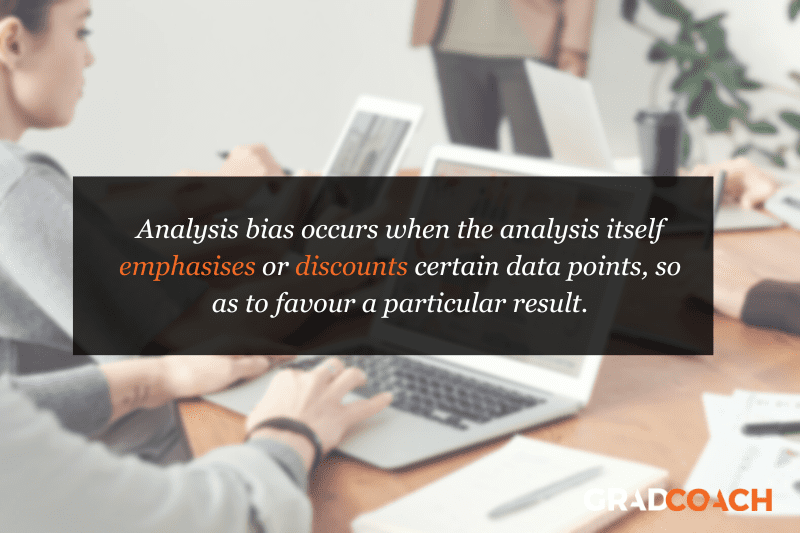
Bias #3 – Procedural Bias
Last but definitely not least, we have procedural bias , which is also sometimes referred to as administration bias . Procedural bias is easy to overlook, so it’s important to understand what it is and how to avoid it. This type of bias occurs when the administration of the study, especially the data collection aspect, has an impact on either who responds or how they respond.
A practical example of procedural bias would be when participants in a study are required to provide information under some form of constraint. For example, participants might be given insufficient time to complete a survey, resulting in incomplete or hastily-filled out forms that don’t necessarily reflect how they really feel. This can happen really easily, if, for example, you innocently ask your participants to fill out a survey during their lunch break.
Another form of procedural bias can happen when you improperly incentivise participation in a study. For example, offering a reward for completing a survey or interview might incline participants to provide false or inaccurate information just to get through the process as fast as possible and collect their reward. It could also potentially attract a particular type of respondent (a freebie seeker), resulting in a skewed sample that doesn’t really reflect your demographic of interest.
The format of your data collection method can also potentially contribute to procedural bias. If, for example, you decide to host your survey or interviews online, this could unintentionally exclude people who are not particularly tech-savvy, don’t have a suitable device or just don’t have a reliable internet connection. On the flip side, some people might find in-person interviews a bit intimidating (compared to online ones, at least), or they might find the physical environment in which they’re interviewed to be uncomfortable or awkward (maybe the boss is peering into the meeting room, for example). Either way, these factors all result in less useful data.
Although procedural bias is more common in qualitative research, it can come up in any form of fieldwork where you’re actively collecting data from study participants. So, it’s important to consider how your data is being collected and how this might impact respondents. Simply put, you need to take the respondent’s viewpoint and think about the challenges they might face, no matter how small or trivial these might seem. So, it’s always a good idea to have an informal discussion with a handful of potential respondents before you start collecting data and ask for their input regarding your proposed plan upfront.
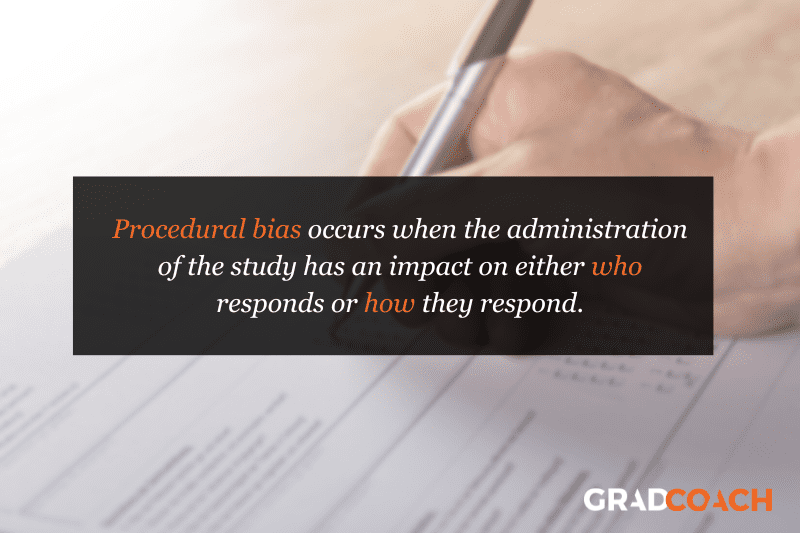
Let’s Recap
Ok, so let’s do a quick recap. Research bias refers to any instance where the researcher, or the research design , negatively influences the quality of a study’s results, whether intentionally or not.
The three common types of research bias we looked at are:
- Selection bias – where a skewed sample leads to skewed results
- Analysis bias – where the analysis method and/or approach leads to biased results – and,
- Procedural bias – where the administration of the study, especially the data collection aspect, has an impact on who responds and how they respond.
As I mentioned, there are many other forms of research bias, but we can only cover a handful here. So, be sure to familiarise yourself with as many potential sources of bias as possible to minimise the risk of research bias in your study.
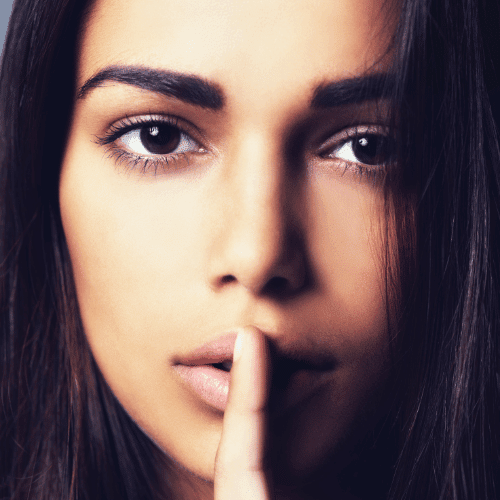
Psst... there’s more!
This post was based on one of our popular Research Bootcamps . If you're working on a research project, you'll definitely want to check this out ...
You Might Also Like:
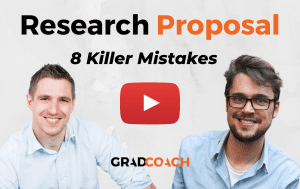
This is really educational and I really like the simplicity of the language in here, but i would like to know if there is also some guidance in regard to the problem statement and what it constitutes.
Do you have a blog or video that differentiates research assumptions, research propositions and research hypothesis?
Submit a Comment Cancel reply
Your email address will not be published. Required fields are marked *
Save my name, email, and website in this browser for the next time I comment.
- Print Friendly
Thank you for visiting nature.com. You are using a browser version with limited support for CSS. To obtain the best experience, we recommend you use a more up to date browser (or turn off compatibility mode in Internet Explorer). In the meantime, to ensure continued support, we are displaying the site without styles and JavaScript.
- View all journals
- Explore content
- About the journal
- Publish with us
- Sign up for alerts
- NATURE INDEX
- 25 April 2024
Algorithm ranks peer reviewers by reputation — but critics warn of bias
- Dalmeet Singh Chawla 0
Dalmeet Singh Chawla is a freelance science journalist based in London.
You can also search for this author in PubMed Google Scholar
An algorithm makes predictions on reviewers on the basis of previous papers’ performance. Credit: Oleh Stefaniak/Getty
An algorithm ranks the reputation of peer reviewers on the basis of how many citations the studies they have reviewed attracted.
The tool, outlined in a study published in February 1 , could help to identify which papers could become high impact during peer review, its creators say. They add that, during peer review, authors should put the most weight on the recommendations and feedback from reviewers of previous papers that have been highly cited.
The study authors extracted citation data from 308,243 papers published by journals of the American Physical Society (APS) between 1990 and 2010 that had accumulated more than 5 citations each. Information about the referees of these papers was not available, so the authors used an algorithm to create imaginary reviewers, which rated papers on the basis of an algorithm that was trained on citation data from the APS data set. Using the review scores that these papers received in real life (a score of 1 being poor and 5 being outstanding), the study authors compared how closely the imaginary reviewers’ scores correlated to the actual scores the papers received.
To rank the imaginary reviewers, the study authors tracked the citations accumulated by the papers published between 1990 and 2000 and checked the review scores they were given. Imaginary reviewers that gave high review scores to papers that went on to attract a high number of citations were given a high ranking.
The authors then tested how effective these reputation rankings were in predicting citation numbers of papers refereed by the same imaginary reviewers in the second decade of the data. The study found that the imaginary reviewers’ recommendations on the 2000–10 papers were in line with the actual citation counts of these papers over that time span, says study co-author An Zeng, an environmental scientist at Beijing Normal University. This suggests that the algorithm is good at predicting high-impact papers, he adds.
More eyes on peer reviewers
Previous attempts to quantify and predict the reach of studies have been widely criticized for relying too heavily on citation-based metrics , which, critics say, exacerbate existing biases in academia. A 2021 study 2 found that non-replicable papers are cited more than replicable studies, possibly because they have more ‘interesting’ results.
Zeng acknowledges the limitations of focusing on citation metrics, but says that it’s important to evaluate the work of peer reviewers. Solid studies are sometimes rejected because of one negative review, he notes, but there’s little attention given to how professional or reliable that reviewer is. “If this algorithm can identify reliable reviewers, it will give less weight to the reviewers who are not so reliable,” says Zeng.
Journal editors often use search tools to identify candidates to peer review papers, but they have to manually decide who to contact. If referee activities were ranked and quantified, this would make it easier for journal editors to choose, Zeng points out.
However, ranking reviewers on their reputation is likely to exacerbate the inequities and biases that exist in peer review, says Anita Bandrowski, an information scientist at the University of California, San Diego.
As previous data have shown , most of the responsibility of the peer-review process in science falls to a small subset of peer reviewers — typically men in senior positions in high-income nations that are geographically closer to most journal editors.
Bandrowski notes that the algorithm might favour those with a long history of reviewing, because they’ve had more time to accumulate citations on their refereed papers. “The oldest reviewers by this metric would be the best reviewers and yet the oldest reviewers are going to be retired or dead,” she says.
Zeng disagrees that his approach will make the selection of peer reviewers more inequitable than it is now. After implementing the reputation ranking, editors might find that some reviewers who are not frequently invited have high reputation scores — in some cases better than those who are inundated with referee requests, he says.
Capturing the nuance
Laura Feetham-Walker, a reviewer-engagement manager at the Institute of Physics Publishing in Bristol, UK, worries that the algorithm might not account for incremental studies , negative findings and replications of previous studies, all of which are crucial for science, albeit often not highly cited .
“Under their system, a reviewer who gave a favourable recommendation on an incremental study — for example, for a journal that does not have novelty as an editorial criterion — would go down in the reviewer reputation ranking, simply because that manuscript would be unlikely to accrue large numbers of citations when published,” she says.
Neither does the ranking account for researchers who have never reviewed before, Feetham-Walker adds, or at least those who have never reviewed for a particular publisher.
“We know that a reviewer’s ability to provide a helpful review is dependent not just on their expertise, but also their availability and interest in the subject matter. We also know that reviewers are human, and their reviewing behaviour can change over time depending on various factors,” Feetham-Walker says. “A nuanced algorithm that took all of this into account, as well as adding new reviewers to enrich the pool, would be of genuine value to publishers.”
doi: https://doi.org/10.1038/d41586-024-01235-w
Gao, F., Fenoaltea, E. M., Zhang, P. & Zeng, A. Expert Syst. Appl. 249 , 123551 (2024).
Article Google Scholar
Serra-Garcia, M. & Gneezy, U. Sci. Adv. 7 , eabd1705 (2021).
Article PubMed Google Scholar
Download references
Related Articles
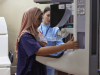
- Research management
- Peer review
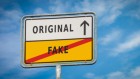
Plagiarism in peer-review reports could be the ‘tip of the iceberg’
Nature Index 01 MAY 24
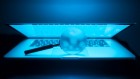
How reliable is this research? Tool flags papers discussed on PubPeer
News 29 APR 24
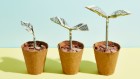
Scientists urged to collect royalties from the ‘magic money tree’
Career Feature 25 APR 24
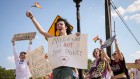
Why it’s essential to study sex and gender, even as tensions rise
Editorial 01 MAY 24
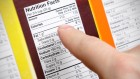
Researchers want a ‘nutrition label’ for academic-paper facts
Nature Index 17 APR 24
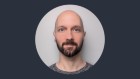
Structure peer review to make it more robust
World View 16 APR 24
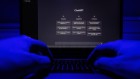
Is ChatGPT corrupting peer review? Telltale words hint at AI use
News 10 APR 24
W2 Professorship with tenure track to W3 in Animal Husbandry (f/m/d)
The Faculty of Agricultural Sciences at the University of Göttingen invites applications for a temporary professorship with civil servant status (g...
Göttingen (Stadt), Niedersachsen (DE)
Georg-August-Universität Göttingen
Postdoctoral Associate- Cardiovascular Research
Houston, Texas (US)
Baylor College of Medicine (BCM)
Faculty Positions & Postdocs at Institute of Physics (IOP), Chinese Academy of Sciences
IOP is the leading research institute in China in condensed matter physics and related fields. Through the steadfast efforts of generations of scie...
Beijing, China
Institute of Physics (IOP), Chinese Academy of Sciences (CAS)
Director, NLM
Vacancy Announcement Department of Health and Human Services National Institutes of Health DIRECTOR, NATIONAL LIBRARY OF MEDICINE THE POSITION:...
Bethesda, Maryland
National Library of Medicine - Office of the Director
Call for postdoctoral fellows in Molecular Medicine, Nordic EMBL Partnership for Molecular Medicine
The Nordic EMBL Partnership is seeking postdoctoral fellows for collaborative projects in molecular medicine through the first NORPOD call.
Helsinki, Finland
Nordic EMBL Partnership for Molecular Medicine
Sign up for the Nature Briefing newsletter — what matters in science, free to your inbox daily.
Quick links
- Explore articles by subject
- Guide to authors
- Editorial policies
OPINION article
Confirmation bias and quantitative approach in psychiatry: should ideological competing interests be declared.
- 1 ADDIPSY, Outpatient Addictology and Psychiatry Center, Santé Basque Développement Group, Lyon, France
- 2 Université Paris-Saclay, Université de Versailles Saint-Quentin-en-Yvelines (UVSQ), INSERM, Centre de Recherche en Epidémiologie et Santé des Populations Team “DevPsy”, Villejuif, France
- 3 Hôpital Femme-Mère-Enfant, Hospices Civils de Lyon, Lyon, France
1 Introduction
Humans have always sought to find the most accurate way to approach and understand reality. This assumption is particularly true in medical sciences, where two visions of knowledge have been opposed: a quantitative, rational, mathematical vision, embodied by fundamental sciences; and a spiritual, qualitative, philosophical vision, embodied by human sciences ( 1 ). This opposition mirrors the foundational sociological divide between Durkheim’s holism (“the whole explains the parts”) and Weber’s methodological individualism (“the parts explain the whole”) ( 2 ): quantitative approach may be compared to holism (by suggesting that knowledge should rely on evidence gathered from large groups), whereas qualitative approach may be compared to individualism (by suggesting that the observation of some individuals can help to build knowledge on the overall group). This opposition also has its corollary in the fields of psychology and psychiatry, especially in the second half of the 20 th century. On one side, the development and advent of the psychoanalytic theory of mental disorders in the first half of the century can be seen as an example of a rather qualitative understanding of mental disorders. On the other side, the second half of the century saw the development of a more quantitative approach of mental disorders, well represented by RCT-based psychopharmacology and quantitative psychology. Even though these two dominant approaches were not the only available (as emphasized, for instance, by the large number of innovations in the field of social psychiatry in 1960-1970), and even though this binary distinction can be considered as oversimplifying, this opposition between proponents of quantitative psychiatry (arguing for a more objective approach of mental health disorders) and advocates of a more qualitative psychiatry (seen as being more holistic and closer to human intimacy) has led to large debates that continue to unfold within research and clinical communities.
Confirmation bias can be defined as the tendency to conduct research, interpret data or recall information in a way that systematically impacts the possibility that the hypothesis is false, while neglecting information that could challenge or contradict that hypothesis ( 3 ). Such pitfalls have been described for qualitative approach of psychiatry, for which psychoanalysis can be used as an example ( 4 ). Moreover, many experts have also underlined the risk of such bias in quantitative medical research (e.g. ( 5 – 8 )), and the same is true for our fields (i.e., psychology and psychiatry). In his popular manifesto on the importance of reforming quantitative psychology, Chris Chambers ( 9 ) asks one of the most important questions concerning this field: “Are research psychologists just poorly paid lawyers?”. Indeed, quantitative research in psychology has been the subject of epistemological and methodological critics in the last decades. Alongside the lack of reproducibility and reliability, and the pressure for publications and citations attached to the academic world, Chambers described how, whether consciously or unconsciously, the researcher can bend the way in which statistical analyses are conducted, according to his or her preconceived opinions and/or what he or she needs the results to be. These concerns regarding the non-consideration of the potential influence of scientists’ perspectives on their results are also shared among methodologists ( 10 ). In our opinion, beyond the various academic warranties brought up by Chambers, it seems legitimate to also ask the question of the influence of the context in which the project, the analysis of results, or their interpretation is conducted.
Indeed, epistemology has told us that science, as a human practice, is profoundly influenced by the historical, sociological, economic and political context in which it is practiced ( 11 ). Because of the social utility it can have (in the sense that it can influence decision-making, whether medical or political), research can be subject to confirmation bias when its priorities are aligned with the social utility one wishes to confer to it ( 7 ). This consideration is also shared among epistemologists working on confirmation bias: while some experts see it as a pernicious tendency, others consider it adaptive, notably because of its usefulness to influence people and social structures to make them match our beliefs about them ( 12 ). This may be particularly relevant in psychiatry and psychology, because of the large impact these two domains can have on society ( 13 , 14 ). In this context, we believe that questioning the influence of the context on the outcomes of the research, and how to overcome such influence, is crucial if we want to provide more objective and less biased information to our readers.
2 Ideology and quantitative research
Many attempts have been developed to try to overcome the issue of confirmation bias in quantitative research and to reduce and/or disclose every element that may impact its conduction and conclusions. One example, on a public health perspective, is the disclosure of financial competing interests. This allows clinicians and researchers to have access to one possible source of bias (i.e. economic). According to the Haute Autorité de Santé in 2023 ( 15 ), the French regulatory agency, competing interests are defined as “ a situation in which a person’s links of interest are likely, by their nature or intensity, to raise question on his or her impartiality or independence in the exercise of his or her mission with regard to the case he or she was asked to work on ”. These links of interest may, of course, be financial, but they may also be intellectual. The intellectual form of such link is thus described as a “ benefit in terms of recognition, occasional or regular, in all its forms, notably for the promotion or defense of group interests, such as those of a school of thought, a discipline or a professional specialty ”. To note, the notion of intellectual competing interests is not considered only in France but also worldwide, as it can be found in the British Medical Association ( 16 ) and American Psychiatric Association ( 17 ) guidelines.
In our opinion, the latter aspect, and especially the promotion or defense of group interests (that will be referred to as “ ideology ” below), is often under-estimated, even though it may have an important impact on research conduction/results. Ideology can be defined as “ a set of ideas, beliefs and attitudes, consciously or unconsciously held, which reflects or shapes understandings or misconceptions of the social and political world ” ( 18 ). In terms of psychiatry and psychology, we believe that it can take different forms depending on the level of analysis. One example can be academic ideology, which can be defined as the influence of one’s school of thought on the conduction and/or outcomes of research. This example seems particularly relevant in our fields, where many schools of thoughts are at odds, especially when considering psychopathology and psychotherapy. In the latter domains, different schools co-exist (e.g., psychoanalysis, other psychodynamic psychotherapy or cognitive-behavioral therapy), and are sometimes opposed. Thus, it seems legitimate to assume that belonging to one school of thought may influence research conduction in favor of the latter. As an example, in 2008, two authors ( 19 ), the first being known for his extensive work on the efficacy of psychodynamic therapies, published a meta-analysis of the effects of long-term psychodynamic therapies, concluding that long-term psychodynamic psychotherapy produced significantly better results in terms of overall efficacy and personality functioning than shorter forms of psychotherapy. This study has been criticized by many authors (e.g., ( 20 , 21 )) including cognitive-behavioral therapy pioneer Aaron Beck, and the critical authors even published a re-analysis of the data, concluding on the absence of efficacy ( 22 ). In our opinion, this example, among others, illustrates well that, when facing the same data, different school of thought can be associated with opposite conclusions.
But academic ideology is just one example of ideological bias. Any topic associated with a significant polarization of the opinion (either medical, political or sociological ( 23 , 24 )) may be subject to this issue. Indeed, given the social utility research can have, and when the research results can lead to significant academical, societal, or political consequences, an important issue emerges: can we really be objective in studying a phenomenon that we are pre-committed to argue for/against it? This question may even raise concerns on the overall objectivity and actual relevance of quantitative approach in our fields, even more when considering that its safeguards such as methodological rigor, peer-reviewing, and the development of ethical guidelines (e.g., the recommendations of the International Committee of Medical Journal Editors (ICMJE, ( 25 )) may be subject to the same type of bias. For example, it has been shown that a reviewer’s political orientation can influence the likelihood of an article containing predominantly socially focused information being published ( 26 ) Such critic can also be made on the peer-review process ( 27 , 28 ). These examples, among others, make us believe that, if these safeguards are significantly helpful, they may be not sufficient to reduce ideological biases in quantitative research.
3 Are quantitative approach and scientific method useless in psychiatry?
At this point, one question can be asked: if quantitative approach exists in our fields to overcome human subjectivity, but if it is in fact also prone to the same type of bias than qualitative approach, then what’s its relevance?
Facing this question, we want to warn our readers on the risk of falling into nihilism regarding the utility of scientific method in psychiatry and psychology. Scientific approach has allowed considerable advances, ranging from the development of evidence-based treatments to the development of new understanding of mental health disorders (e.g., ( 29 , 30 )). The aforementioned safeguards, if imperfect, allowed to significantly reduce the risk of ideological biases, by allowing: i) the diffraction of the judgment process to different professionals with possibly different ideologies (via peer-reviewing), and ii) the pre- and post-publication analysis of protocols and statistical methodology through pre-registration and raw data diffusion (via the development of open science and ethical guidelines). Moreover, the growing emphasis on reproducibility (i.e., obtaining comparable results using the same data and the same methods, but under different analysis conditions) and replicability (i.e., obtaining comparable results in all studies designed to answer the same scientific question, each having obtained its own data but using the same methods) may also help to detect biased studies and to protect from long-term duration of ideological biases. Altogether, our aim here is not to dismiss quantitative approach, but more to contribute to ongoing debates regarding its improvement. Indeed, we believe that considering psychiatric quantitative science purely objective and free of biases is as problematic as considering it totally useless and subjective. Each approach has its own pitfalls, and qualitative approach shares many of them in terms of ideological influence (e.g., regarding socio-political ideologies and psychoanalysis in the French context ( 31 )). We believe it’s our role as researchers to shed light on the limitations of our practice to enhance the readers’ trust towards our results.
Thus, to move into this direction, we believe that quantitative research can learn from the rapidly expanding field of qualitative research, especially on how its researchers consider the risk of impact of preconceptions on their results. Indeed, the methodology requires authors to write a logbook describing why they became interested in a subject, what results they expect from their study, and what might surprise or challenge them ( 32 ). Accessible alongside the publication of their results, readers can thus make their own opinion on the risk of confirmation bias. This makes it possible, without making it disappear, to significantly reduce it, or at least make it visible. This kind of prior declaration seems central to implement in quantitative research, especially in fields with potential societal or political impact. Indeed, when looking at the definition of intellectual link of interest we presented earlier (“ benefits in terms of recognition [ … ] notably for the promotion or defense of group interests”) , and as it is mandatory to declare financial competing interests, it would seem logical that a declaration of ideological competing interest (whether academic, sociological, political or other) should be at least considered. Indeed, we believe that a fully transparent position should include a disclosure on from where the researcher is talking, as the latter may influence the objectivity of the results.
4 Reflections on ideological competing interests’ disclosure implementation challenges
We are aware that this proposition is provocative and may be difficult to implement in real life. Researchers have necessarily their own opinions, and the boundary is subtle between ideology and personal theoretical or political opinions. Thus, many practical challenges can be associated with the implementation of such a policy.
For example, there may be a risk of stigmatization of certain viewpoints leading to discouragement of researchers from exploring controversial topics. Indeed, if working on a “hot” and polarizing topic may include personal opinions’ disclosure, researchers having one opinion but evolving in a context favorable to another may start to withdraw from this specific topic exploration to not suffer any consequences (like rejection from social or academic spheres), which could in turn enhances the risk of publication bias (with only research matching the context’s opinion being conducted). However, this risk could be decreased by restricting the content of this disclosure only to the researchers’ preconceived opinions on the actual subject of the study, without compelling them to fully describe all their personal opinions outside of the research subject.
Also, there may be a risk of increasing the actual global mistrust in psychiatric science, both from public and practitioners [e.g., ( 33 )]. Indeed, if ideological interests are disclosed, and if the results found are in line with these interests, readers may be prone to over conclude that these results are inherently biased, especially when considering that these readers may also be prone to confirmation bias, and when considering the overall low level of knowledge on how research is conducted and statistical analyses work ( 34 , 35 ). If this risk should be carefully considered, we also believe that this mistrust partly relies on the actual non-disclosure of this type of competing interests that have long been the subject of concerns, and providing a way to address these concerns may thus be a way to enhance the readers’ trust.
Finally, given that the present paper can be seen as a practical example of confirmation bias and ideology, we wanted to lay the foundation stone of ideological competing interests’ disclosure. Indeed, the four authors have their own opinions regarding these topics, and notably on the risk of influence of ideologies on the conduction and production of science. Thus, the examples and the references we chose can be seen as subjective, partials, and selected to support our preconceived opinions. We described the latter in the Author’s Note section. Overall, we hope that this article will contribute to pursue the reflection on this important topic.
Author’s Note
Given our proposition to disclose ideological competing interests, we wanted to disclose our own regarding this paper. MB is a psychiatrist and psychotherapist specialized in the study and treatment of patients suffering from personality disorders. He received his MD in psychiatry in Lyon (France), a city where large academic conflicts took place in the last decades between psychoanalysts and biological psychiatrists, leading to important ideological consequences in terms of psychiatry training. His preconceived opinions are that quantitative and qualitative approaches can both be useful and should be considered, from psychoanalysis to biological psychiatry, notably because they all allowed great progress in the understanding and treatment of patients with personality disorders. Finally, he also has a great interest in the study of the link between society organization and psychiatry, especially regarding the development of personality disorders.
CG completed an engineering degree in computational sciences and a PhD in philosophy of medicine. As an adolescent psychiatrist who follows the French university curriculum quite classically, he is certainly interested in the discussions raised by such opinion articles at the border of methodology, philosophy and science studies, but he is undoubtedly and unconsciously moved by the implicit argument that such an article, in an indexed journal, brings him “points” for his institutional recognition by his peers.
PE is a child psychiatrist with a clinical practice in the field of psychotrauma and victimology. Before her practice of psychiatry, she completed a medicine-science curriculum and a master’s degree in neuroscience. She then started her training in psychiatry through institutional psychotherapy and psychoanalytic culture in Reims. She completed her internship in Lyon, where the neurobiological approach was predominant in academic teaching. She now tries to have a clinical practice as integrative as possible, with a growing interest in the socio-political aspects of psychotrauma. Finally, she belongs to scientific societies of the discipline (SFPEADA and API), and the union of hospital psychiatrists (SPH).
Finally, BF is a professor of biostatistics but is ambivalent about the dominant place of his discipline in the epistemology of contemporary biomedical research. As a child psychiatrist, he is critical of the dominant position of neuroscientific thought in this field and is interested in the human sciences and in psychoanalysis. He regrets the latter’s lack of openness to the experimental approach.
Author contributions
MB: Writing – review & editing, Writing – original draft. CG: Writing – review & editing, Supervision. PE: Writing – review & editing. BF: Writing – review & editing, Supervision.
The author(s) declare that no financial support was received for the research, authorship, and/or publication of this article.
Conflict of interest
The authors declare that the research was conducted in the absence of any commercial or financial relationships that could be construed as a potential conflict of interest.
Publisher’s note
All claims expressed in this article are solely those of the authors and do not necessarily represent those of their affiliated organizations, or those of the publisher, the editors and the reviewers. Any product that may be evaluated in this article, or claim that may be made by its manufacturer, is not guaranteed or endorsed by the publisher.
1. Falissard B, Révah A, Yang S, Fagot-Largeault A. The place of words and numbers in psychiatric research. Philosophy Ethics Humanities Med . (2013) 8:18. doi: 10.1186/1747-5341-8-18
CrossRef Full Text | Google Scholar
2. Zahle J, Collin F. Rethinking the individualism-holism debate. Essays Philosophy Soc Sci . (2014). doi: 10.1007/978-3-319-05344-8
3. Oswald ME, Grosjean S. Confirmation bias. Cogn Illusions: A Handb Fallacies Biases Thinking Judgement Memory . (2004) 79:79–96. doi: 10.13140/2.1.2068.0641
4. Lacewing M. The Science of Psychoanalysis. Philosophy Psychiatry Psychol . (2018) 25:95–111. doi: 10.1353/ppp.2018.0015
5. Schumm WR. Confirmation bias and methodology in social science: an editorial. Marriage Family Review . (2021) 57:285–93. doi: 10.1080/01494929.2021.1872859
6. Nickerson RS. Confirmation Bias: A Ubiquitous Phenomenon in Many Guises. Rev Gen Psychol . (1998) 2:175–220. doi: 10.1037//1089-2680.2.2.175
7. Braithwaite RS, Ban K, ER S, Caniglia EC. Rounding up the usual suspects: confirmation bias in epidemiological research. Int J Epidemiol . (2021) 50:1053–7. doi: 10.1093/ije/dyab091
8. Berger JO, Berry DA. Statistical Analysis and the Illusion of Objectivity. Am Scientist . (1988) 76:159–65.
Google Scholar
9. Chambers C. The Seven Deadly Sins of Psychology: A Manifesto for Reforming the Culture of Scientific Practice. In: The Seven Deadly Sins of Psychology . Princeton University Press, Princeton, NJ (2017). doi: 10.1515/9781400884940
10. Levitt HM, Surace FI, Wu MB, Chapin B, Hargrove JG, Herbitter C, et al. The meaning of scientific objectivity and subjectivity: From the perspective of methodologists. psychol Methods . (2022) 27:589–605. doi: 10.1037/met0000363
11. Charrier JP. Scientisme et Occident: essais d’épistémologie critique. Scientisme Occident . (2011), 1–400.
12. Peters U. What Is the Function of Confirmation Bias? Erkenn . (2022) 87:1351–76. doi: 10.1007/s10670-020-00252-1
13. Nasrallah H. Psychiatry’s social impact: Pervasive and multifaceted. Curr Psychiatry . (2019). 18(2):4, 6–7
14. Beeker T, Mills C, Bhugra D, te Meerman S, Thoma S, Heinze M, et al. Psychiatrization of Society: A Conceptual Framework and Call for Transdisciplinary Research. Front Psychiatry . (2021) 12. doi: 10.3389/fpsyt.2021.645556
15. Haute Autorité de Santé. Déclarations d’intérêts et gestion des conflits d’intérêts(2023). Available online at: https://www.has-sante.fr/upload/docs/application/pdf/guide_dpi.pdf .
16. British Medical Association. Transparency and doctors with competing interests – guidance from the BMA(2017). Available online at: https://www.bma.org.uk/ .
17. American Psychiatric Association. American Psychiatric Association Disclosure of Affiliations and Interests Policy . (2023).
18. Freeden M. Ideology. In: Routledge Encyclopedia of Philosophy , 1st. Routledge, London (2016). Available at: https://www.rep.routledge.com/articles/thematic/ideology/v-1 .
19. Leichsenring F, Rabung S. Effectiveness of long-term psychodynamic psychotherapy: a meta-analysis. JAMA . (2008) 300:1551–65. doi: 10.1001/jama.300.13.1551
20. Beck AT. Analyzing Effectiveness of Long-term Psychodynamic Psychotherapy. JAMA . (2009) 301:930. doi: 10.1001/jama.2009.179
21. Thombs BD. Analyzing Effectiveness of Long-term Psychodynamic Psychotherapy. JAMA . (2009) 301:930. doi: 10.1001/jama.2009.177
22. Bhar SS, Thombs BD, Pignotti M, Bassel M, Jewett L, Coyne JC, et al. Is Longer-Term Psychodynamic Psychotherapy More Effective than Shorter-Term Therapies? Review and Critique of the Evidence. Psychother Psychosom . (2010) 79:208–16. doi: 10.1159/000313689
23. Heinich N. Ce que le militantisme fait à la recherche. In: Ce que le militantisme fait à la recherche . Gallimard, Paris (2021). p. 1–48. Available at: https://www.cairn.info/tracts-n29-ce-que-le-militantisme-fait-a-la-recher–9782072955907-p-1.htm .
24. Block J. Gender dysphoria in young people is rising—and so is professional disagreement. BMJ . (2023) 380:p382. doi: 10.1136/bmj.p382
25. International Committee of Medical Journal Editors. Recommendations(2024). Available online at: https://www.icmje.org/recommendations/ .
26. Abramowitz SI, Gomes B, Abramowitz CV. Publish or Politic: Referee Bias in Manuscript Review1. J Appl Soc Psychol . (1975) 5:187–200. doi: 10.1111/j.1559-1816.1975.tb00675.x
27. Tennant JP, Ross-Hellauer T. The limitations to our understanding of peer review. Res Integr Peer Rev . (2020) 5:6. doi: 10.1186/s41073-020-00092-1
28. Lee CJ, Sugimoto CR, Zhang G, Cronin B. Bias in peer review. J Am Soc Inf Sci Technol . (2013) 64:2–17. doi: 10.1002/asi.22784
29. Caspi A, Houts RM, Belsky DW, Goldman-Mellor SJ, Harrington H, Israel S, et al. The p Factor: One General Psychopathology Factor in the Structure of Psychiatric Disorders? Clin Psychol Sci . (2014) 2:119–37. doi: 10.1177/2167702613497473
30. Sharp C, Wright AGC, Fowler JC, Frueh BC, Allen JG, Oldham J, et al. The structure of personality pathology: Both general (‘g’) and specific (‘s’) factors? J Abnormal Psychol . (2015) 124:387–98. doi: 10.1037/abn0000033
31. Durpoix A. Le rapport Inserm [2004] – Pourquoi cette évaluation des psychotherapies fut-elle si controversée? Faculté Des Sci Historiques/Université Strasbourg . (2021).
32. Skjott Linneberg M, Korsgaard S. Coding qualitative data: a synthesis guiding the novice. QRJ . (2019) 19:259–70. doi: 10.1108/QRJ-12-2018-0012
33. Haerlin B, Parr D. How to restore public trust in science. Nature . (1999) 400:499–9. doi: 10.1038/22867
34. Windish DM, Huot SJ, Green ML. Medicine residents’ understanding of the biostatistics and results in the medical literature. JAMA . (2007) 298:1010–22. doi: 10.1001/jama.298.9.1010
35. Nasr N. Overcoming the discourse of science mistrust: how science education can be used to develop competent consumers and communicators of science information. Cult Stud Sci Educ . (2021) 16:345–56. doi: 10.1007/s11422-021-10064-6
Keywords: quantitative approach, qualitative approach, ideology, confirmation bias, epistemology
Citation: Blay M, Gauld C, Espi P and Falissard B (2024) Confirmation bias and quantitative approach in psychiatry: should ideological competing interests be declared? Front. Psychiatry 15:1365733. doi: 10.3389/fpsyt.2024.1365733
Received: 04 January 2024; Accepted: 02 April 2024; Published: 24 April 2024.
Reviewed by:
Copyright © 2024 Blay, Gauld, Espi and Falissard. This is an open-access article distributed under the terms of the Creative Commons Attribution License (CC BY) . The use, distribution or reproduction in other forums is permitted, provided the original author(s) and the copyright owner(s) are credited and that the original publication in this journal is cited, in accordance with accepted academic practice. No use, distribution or reproduction is permitted which does not comply with these terms.
*Correspondence: Martin Blay, [email protected]
Disclaimer: All claims expressed in this article are solely those of the authors and do not necessarily represent those of their affiliated organizations, or those of the publisher, the editors and the reviewers. Any product that may be evaluated in this article or claim that may be made by its manufacturer is not guaranteed or endorsed by the publisher.
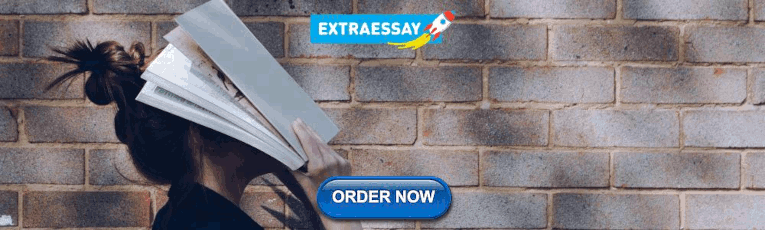
IMAGES
VIDEO
COMMENTS
Bias—commonly understood to be any influence that provides a distortion in the results of a study (Polit & Beck, 2014)—is a term drawn from the quantitative research paradigm.Most (though perhaps not all) of us would recognize the concept as being incompatible with the philosophical underpinnings of qualitative inquiry (Thorne, Stephens, & Truant, 2016).
Consider potential bias while constructing the interview and order the questions suitably. Ask general questions first, before moving to specific or sensitive questions. Leading questions and wording bias. Questions that lead or prompt the participants in the direction of probable outcomes may result in biased answers.
In qualitative research for instance, the researcher's preconceived notions and expectations can influence how they interpret and code qualitative data, a type of bias known as interpretation bias. It's also important to note that quantitative research is not free of bias either, as sampling bias and measurement bias can threaten the validity ...
Introduction. Bias, perhaps best described as 'any process at any stage of inference which tends to produce results or conclusions that differ systematically from the truth,' can pollute the entire spectrum of research, including its design, analysis, interpretation and reporting. 1 It can taint entire bodies of research as much as it can individual studies. 2 3 Given this extensive ...
Researcher reflexivity, essentially a researcher's insight into their own biases and rationale for decision-making as the study progresses, is critical to rigor. This article reviews common standards of rigor, quality scholarship criteria, and best practices for qualitative research from design through dissemination.
In other words, their subjective perspective (or "bias") is fundamentally intertwined with qualitative research processes. And while the researcher's perspective has many positive impacts, failure to attend to reflexivity can negatively impact the knowledge built via qualitative research and those connected to it.
There's interviewer bias, which is very hard to avoid. This is when an interviewer subconsciously influences the responses of the interviewee. Their body language might indicate their opinion, for example. Furthermore, there's response bias, where someone tries to give the answers they think are "correct.". Finally, there's reporting ...
Even if bias is not a primary concern of qualitative research (Galdas, 2017), selective sampling can be an issue (Miles et al., 2014;Tellis, 2017). I chose two strategies to help limit this impact ...
Abstract. This narrative review provides an overview on the topic of bias as part of Plastic and Reconstructive Surgery 's series of articles on evidence-based medicine. Bias can occur in the planning, data collection, analysis, and publication phases of research. Understanding research bias allows readers to critically and independently review ...
His account of validity in qualitative research is, at least in part, an attempt to uncover 'theory-in-use'. He distinguishes five types of validity: descriptive validity, interpretive validity, theoretical validity, generalisability and evaluative validity.[1] Maxwell notes that in experimental research threats to validity are "addressed ...
Revisiting Bias in Qualitative Research: Reflections on Its Relationship With Funding and Impact. Recognizing and understanding research bias is crucial for determining the utility of study results and an essential aspect of evidence-based decision-making in the health professions. Research proposals and manuscripts that do not provide satis ...
Qualitative research is frequently criticised for lacking scientific rigour with poor justification of the methods adopted, lack of transparency in the analytical procedures and the findings being merely a collection of personal opinions subject to researcher bias.2, 3 For the novice researcher, demonstrating rigour when undertaking qualitative ...
Qualitative research, like any research, starts with a systematic review of the literature to show that the topic being studied is significant and unresolved. Some forms of qualitative research focus on existing data, for example from archives or media re-ports. Our focus here is on interview studies. Both formats face the same problem, that of ...
Research bias can occur in both qualitative and quantitative research. Understanding research bias is important for several reasons. Bias exists in all research, across research designs, and is difficult to eliminate. ... Researcher bias occurs when the researcher's beliefs or expectations influence the research design or data collection process.
There is limited empirical research that has investigated strategies to reduce weight bias in qualitative research, so until the field expands in this area, this review provides practical strategies for researchers to apply and consider for research advancement on this topic. Future work will need to test the utility and effectiveness of these ...
Bias in research Joanna Smith,1 Helen Noble2 The aim of this article is to outline types of 'bias' across research designs, and consider strategies to minimise ... research cannot be applied to qualitative research. However, in the broadest context, these terms are applic-able, with validity referring to the integrity and applica- ...
Biases can exist in health research, in both quantitative and in qualitative research 1. Although it is not a new topic, the discussion about biases in qualitative research is still timid and demands greater attention and depth from researchers. According to Althubaiti 2, the problem of bias is still often ignored in practice.
Bias #2 - Analysis Bias. Next up, we have analysis bias.Analysis bias occurs when the analysis itself emphasises or discounts certain data points, so as to favour a particular result (often the researcher's own expected result or hypothesis).In other words, analysis bias happens when you prioritise the presentation of data that supports a certain idea or hypothesis, rather than presenting ...
There are questions about whether the tool, which could be used by editors to find and shortlist peer reviewers, would disadvantage inexperienced candidates or those in certain locations.
The analysis phase in qualitative research is where the real meaning behind the data comes to life. However, if coding bias is present, the analysis can become a reflection of the researcher's ...
Revisiting Bias in Qualitative Research: Reflections on Its Relationship With Funding and Impact. Recognizing and understanding research bias is crucial for determining the utility of study results and an essential aspect of evidence-based decision-making in the health professions. Research proposals and manuscripts that do not provide satis ...
Such pitfalls have been described for qualitative approach of psychiatry, for which psychoanalysis can be used as an example . Moreover, many experts have also underlined the risk of such bias in quantitative medical research (e.g. (5-8)), and the same is true for our fields
However, researcher biases can lead to questionable research practices in secondary data analysis, which can distort the evidence base. While pre-registration can help to protect against researcher biases, it presents challenges for secondary data analysis. In this article, we describe these challenges and propose novel solutions and ...
Revisiting Bias in Qualitative Research: Reflections on Its Relationship With Funding and Impact. Paul Galdas View all authors and affiliations. All Articles. ... The role of qualitative research within an evidence-based context: Can metasynthesis be the answer? International Journal of Nursing Studies, 46, 569-575. Crossref. PubMed. ISI ...
Artificial intelligence (AI)-based generative imaging systems such as DALL·E, Midjourney, Stable Diffusion, and Adobe Firefly, which work by transforming natural language descriptions into images, are revolutionizing computer vision. In this exploratory and qualitative research, we have replicated requests for images of women in different professions by comparing these representations in ...