
- Table of Contents
- Random Entry
- Chronological
- Editorial Information
- About the SEP
- Editorial Board
- How to Cite the SEP
- Special Characters
- Advanced Tools
- Support the SEP
- PDFs for SEP Friends
- Make a Donation
- SEPIA for Libraries
- Entry Contents
- Bibliography
- Academic Tools
- Friends PDF Preview
- Author and Citation Info
- Back to Top
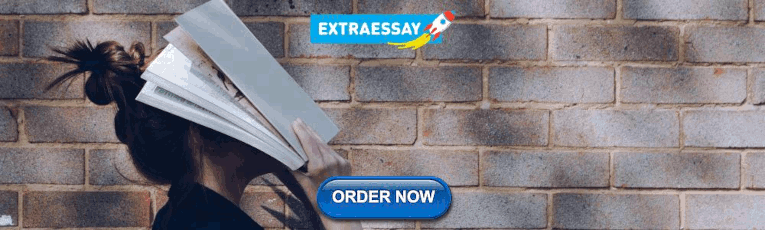
Measurement in Science
Measurement is an integral part of modern science as well as of engineering, commerce, and daily life. Measurement is often considered a hallmark of the scientific enterprise and a privileged source of knowledge relative to qualitative modes of inquiry. [ 1 ] Despite its ubiquity and importance, there is little consensus among philosophers as to how to define measurement, what sorts of things are measurable, or which conditions make measurement possible. Most (but not all) contemporary authors agree that measurement is an activity that involves interaction with a concrete system with the aim of representing aspects of that system in abstract terms (e.g., in terms of classes, numbers, vectors etc.) But this characterization also fits various kinds of perceptual and linguistic activities that are not usually considered measurements, and is therefore too broad to count as a definition of measurement. Moreover, if “concrete” implies “real”, this characterization is also too narrow, as measurement often involves the representation of ideal systems such as the average household or an electron at complete rest.
Philosophers have written on a variety of conceptual, metaphysical, semantic and epistemological issues related to measurement. This entry will survey the central philosophical standpoints on the nature of measurement, the notion of measurable quantity and related epistemological issues. It will refrain from elaborating on the many discipline-specific problems associated with measurement and focus on issues that have a general character.
1. Overview
2. quantity and magnitude: a brief history, 3.1 fundamental and derived measurement, 3.2 the classification of scales.
- 3.3 The measurability of sensation
- 3.4 Representational Theory of Measurement
- 4. Operationalism and Conventionalism
- 5. Realist Accounts of Measurement
- 6. Information-Theoretic Accounts of Measurement
- 7.1 The roles of models in measurement
- 7.2 Models and measurement in economics
- 7.3 Psychometric models and construct validity
- 8.1 Standardization and scientific progress
- 8.2 Theory-ladenness of measurement
- 8.3 Accuracy and precision
- Other Internet Resources
- Related Entries
Modern philosophical discussions about measurement—spanning from the late nineteenth century to the present day—may be divided into several strands of scholarship. These strands reflect different perspectives on the nature of measurement and the conditions that make measurement possible and reliable. The main strands are mathematical theories of measurement, operationalism, conventionalism, realism, information-theoretic accounts and model-based accounts. These strands of scholarship do not, for the most part, constitute directly competing views. Instead, they are best understood as highlighting different and complementary aspects of measurement. The following is a very rough overview of these perspectives:
- Mathematical theories of measurement view measurement as the mapping of qualitative empirical relations to relations among numbers (or other mathematical entities).
- Operationalists and conventionalists view measurement as a set of operations that shape the meaning and/or regulate the use of a quantity-term.
- Realists view measurement as the estimation of mind-independent properties and/or relations.
- Information-theoretic accounts view measurement as the gathering and interpretation of information about a system.
- Model-based accounts view measurement as the coherent assignment of values to parameters in a theoretical and/or statistical model of a process.
These perspectives are in principle consistent with each other. While mathematical theories of measurement deal with the mathematical foundations of measurement scales, operationalism and conventionalism are primarily concerned with the semantics of quantity terms, realism is concerned with the metaphysical status of measurable quantities, and information-theoretic and model-based accounts are concerned with the epistemological aspects of measuring. Nonetheless, the subject domain is not as neatly divided as the list above suggests. Issues concerning the metaphysics, epistemology, semantics and mathematical foundations of measurement are interconnected and often bear on one another. Hence, for example, operationalists and conventionalists have often adopted anti-realist views, and proponents of model-based accounts have argued against the prevailing empiricist interpretation of mathematical theories of measurement. These subtleties will become clear in the following discussion.
The list of strands of scholarship is neither exclusive nor exhaustive. It reflects the historical trajectory of the philosophical discussion thus far, rather than any principled distinction among different levels of analysis of measurement. Some philosophical works on measurement belong to more than one strand, while many other works do not squarely fit either. This is especially the case since the early 2000s, when measurement returned to the forefront of philosophical discussion after several decades of relative neglect. This recent body of scholarship is sometimes called “the epistemology of measurement”, and includes a rich array of works that cannot yet be classified into distinct schools of thought. The last section of this entry will be dedicated to surveying some of these developments.
Although the philosophy of measurement formed as a distinct area of inquiry only during the second half of the nineteenth century, fundamental concepts of measurement such as magnitude and quantity have been discussed since antiquity. According to Euclid’s Elements , a magnitude—such as a line, a surface or a solid—measures another when the latter is a whole multiple of the former (Book V, def. 1 & 2). Two magnitudes have a common measure when they are both whole multiples of some magnitude, and are incommensurable otherwise (Book X, def. 1). The discovery of incommensurable magnitudes allowed Euclid and his contemporaries to develop the notion of a ratio of magnitudes. Ratios can be either rational or irrational, and therefore the concept of ratio is more general than that of measure (Michell 2003, 2004a; Grattan-Guinness 1996).
Aristotle distinguished between quantities and qualities. Examples of quantities are numbers, lines, surfaces, bodies, time and place, whereas examples of qualities are justice, health, hotness and paleness ( Categories §6 and §8). According to Aristotle, quantities admit of equality and inequality but not of degrees, as “one thing is not more four-foot than another” (ibid. 6.6a19). Qualities, conversely, do not admit of equality or inequality but do admit of degrees, “for one thing is called more pale or less pale than another” (ibid. 8.10b26). Aristotle did not clearly specify whether degrees of qualities such as paleness correspond to distinct qualities, or whether the same quality, paleness, was capable of different intensities. This topic was at the center of an ongoing debate in the thirteenth and fourteenth centuries (Jung 2011). Duns Scotus supported the “addition theory”, according to which a change in the degree of a quality can be explained by the addition or subtraction of smaller degrees of that quality (2011: 553). This theory was later refined by Nicole Oresme, who used geometrical figures to represent changes in the intensity of qualities such as velocity (Clagett 1968; Sylla 1971). Oresme’s geometrical representations established a subset of qualities that were amenable to quantitative treatment, thereby challenging the strict Aristotelian dichotomy between quantities and qualities. These developments made possible the formulation of quantitative laws of motion during the sixteenth and seventeenth centuries (Grant 1996).
The concept of qualitative intensity was further developed by Leibniz and Kant. Leibniz’s “principle of continuity” stated that all natural change is produced by degrees. Leibniz argued that this principle applies not only to changes in extended magnitudes such as length and duration, but also to intensities of representational states of consciousness, such as sounds (Jorgensen 2009; Diehl 2012). Kant is thought to have relied on Leibniz’s principle of continuity to formulate his distinction between extensive and intensive magnitudes. According to Kant, extensive magnitudes are those “in which the representation of the parts makes possible the representation of the whole” (1787: A162/B203). An example is length: a line can only be mentally represented by a successive synthesis in which parts of the line join to form the whole. For Kant, the possibility of such synthesis was grounded in the forms of intuition, namely space and time. Intensive magnitudes, like warmth or colors, also come in continuous degrees, but their apprehension takes place in an instant rather than through a successive synthesis of parts. The degrees of intensive magnitudes “can only be represented through approximation to negation” (1787: A 168/B210), that is, by imagining their gradual diminution until their complete absence.
Scientific developments during the nineteenth century challenged the distinction between extensive and intensive magnitudes. Thermodynamics and wave optics showed that differences in temperature and hue corresponded to differences in spatio-temporal magnitudes such as velocity and wavelength. Electrical magnitudes such as resistance and conductance were shown to be capable of addition and division despite not being extensive in the Kantian sense, i.e., not synthesized from spatial or temporal parts. Moreover, early experiments in psychophysics suggested that intensities of sensation such as brightness and loudness could be represented as sums of “just noticeable differences” among stimuli, and could therefore be thought of as composed of parts (see Section 3.3 ). These findings, along with advances in the axiomatization of branches of mathematics, motivated some of the leading scientists of the late nineteenth century to attempt to clarify the mathematical foundations of measurement (Maxwell 1873; von Kries 1882; Helmholtz 1887; Mach 1896; Poincaré 1898; Hölder 1901; for historical surveys see Darrigol 2003; Michell 1993, 2003; Cantù and Schlaudt 2013; Biagioli 2016: Ch. 4, 2018). These works are viewed today as precursors to the body of scholarship known as “measurement theory”.
3. Mathematical Theories of Measurement (“Measurement Theory”)
Mathematical theories of measurement (often referred to collectively as “measurement theory”) concern the conditions under which relations among numbers (and other mathematical entities) can be used to express relations among objects. [ 2 ] In order to appreciate the need for mathematical theories of measurement, consider the fact that relations exhibited by numbers—such as equality, sum, difference and ratio—do not always correspond to relations among the objects measured by those numbers. For example, 60 is twice 30, but one would be mistaken in thinking that an object measured at 60 degrees Celsius is twice as hot as an object at 30 degrees Celsius. This is because the zero point of the Celsius scale is arbitrary and does not correspond to an absence of temperature. [ 3 ] Similarly, numerical intervals do not always carry empirical information. When subjects are asked to rank on a scale from 1 to 7 how strongly they agree with a given statement, there is no prima facie reason to think that the intervals between 5 and 6 and between 6 and 7 correspond to equal increments of strength of opinion. To provide a third example, equality among numbers is transitive [if (a=b & b=c) then a=c] but empirical comparisons among physical magnitudes reveal only approximate equality, which is not a transitive relation. These examples suggest that not all of the mathematical relations among numbers used in measurement are empirically significant, and that different kinds of measurement scale convey different kinds of empirically significant information.
The study of measurement scales and the empirical information they convey is the main concern of mathematical theories of measurement. In his seminal 1887 essay, “Counting and Measuring”, Hermann von Helmholtz phrased the key question of measurement theory as follows:
[W]hat is the objective meaning of expressing through denominate numbers the relations of real objects as magnitudes, and under what conditions can we do this? (1887: 4)
Broadly speaking, measurement theory sets out to (i) identify the assumptions underlying the use of various mathematical structures for describing aspects of the empirical world, and (ii) draw lessons about the adequacy and limits of using these mathematical structures for describing aspects of the empirical world. Following Otto Hölder (1901), measurement theorists often tackle these goals through formal proofs, with the assumptions in (i) serving as axioms and the lessons in (ii) following as theorems. A key insight of measurement theory is that the empirically significant aspects of a given mathematical structure are those that mirror relevant relations among the objects being measured. For example, the relation “bigger than” among numbers is empirically significant for measuring length insofar as it mirrors the relation “longer than” among objects. This mirroring, or mapping, of relations between objects and mathematical entities constitutes a measurement scale. As will be clarified below, measurement scales are usually thought of as isomorphisms or homomorphisms between objects and mathematical entities.
Other than these broad goals and claims, measurement theory is a highly heterogeneous body of scholarship. It includes works that span from the late nineteenth century to the present day and endorse a wide array of views on the ontology, epistemology and semantics of measurement. Two main differences among mathematical theories of measurement are especially worth mentioning. The first concerns the nature of the relata , or “objects”, whose relations numbers are supposed to mirror. These relata may be understood in at least four different ways: as concrete individual objects, as qualitative observations of concrete individual objects, as abstract representations of individual objects, or as universal properties of objects. Which interpretation is adopted depends in large part on the author’s metaphysical and epistemic commitments. This issue will be especially relevant to the discussion of realist accounts of measurement ( Section 5 ). Second, different measurement theorists have taken different stands on the kind of empirical evidence that is required to establish mappings between objects and numbers. As a result, measurement theorists have come to disagree about the necessary conditions for establishing the measurability of attributes, and specifically about whether psychological attributes are measurable. Debates about measurability have been highly fruitful for the development of measurement theory, and the following subsections will introduce some of these debates and the central concepts developed therein.
During the late nineteenth and early twentieth centuries several attempts were made to provide a universal definition of measurement. Although accounts of measurement varied, the consensus was that measurement is a method of assigning numbers to magnitudes . For example, Helmholtz (1887: 17) defined measurement as the procedure by which one finds the denominate number that expresses the value of a magnitude, where a “denominate number” is a number together with a unit, e.g., 5 meters, and a magnitude is a quality of objects that is amenable to ordering from smaller to greater, e.g., length. Bertrand Russell similarly stated that measurement is
any method by which a unique and reciprocal correspondence is established between all or some of the magnitudes of a kind and all or some of the numbers, integral, rational or real. (1903: 176)
Norman Campbell defined measurement simply as “the process of assigning numbers to represent qualities”, where a quality is a property that admits of non-arbitrary ordering (1920: 267).
Defining measurement as numerical assignment raises the question: which assignments are adequate, and under what conditions? Early measurement theorists like Helmholtz (1887), Hölder (1901) and Campbell (1920) argued that numbers are adequate for expressing magnitudes insofar as algebraic operations among numbers mirror empirical relations among magnitudes. For example, the qualitative relation “longer than” among rigid rods is (roughly) transitive and asymmetrical, and in this regard shares structural features with the relation “larger than” among numbers. Moreover, the end-to-end concatenation of rigid rods shares structural features—such as associativity and commutativity—with the mathematical operation of addition. A similar situation holds for the measurement of weight with an equal-arms balance. Here deflection of the arms provides ordering among weights and the heaping of weights on one pan constitutes concatenation.
Early measurement theorists formulated axioms that describe these qualitative empirical structures, and used these axioms to prove theorems about the adequacy of assigning numbers to magnitudes that exhibit such structures. Specifically, they proved that ordering and concatenation are together sufficient for the construction of an additive numerical representation of the relevant magnitudes. An additive representation is one in which addition is empirically meaningful, and hence also multiplication, division etc. Campbell called measurement procedures that satisfy the conditions of additivity “fundamental” because they do not involve the measurement of any other magnitude (1920: 277). Kinds of magnitudes for which a fundamental measurement procedure has been found—such as length, area, volume, duration, weight and electrical resistance—Campbell called “fundamental magnitudes”. A hallmark of such magnitudes is that it is possible to generate them by concatenating a standard sequence of equal units, as in the example of a series of equally spaced marks on a ruler.
Although they viewed additivity as the hallmark of measurement, most early measurement theorists acknowledged that additivity is not necessary for measuring. Other magnitudes exist that admit of ordering from smaller to greater, but whose ratios and/or differences cannot currently be determined except through their relations to other, fundamentally measurable magnitudes. Examples are temperature, which may be measured by determining the volume of a mercury column, and density, which may be measured as the ratio of mass and volume. Such indirect determination came to be called “derived” measurement and the relevant magnitudes “derived magnitudes” (Campbell 1920: 275–7).
At first glance, the distinction between fundamental and derived measurement may seem reminiscent of the distinction between extensive and intensive magnitudes, and indeed fundamental measurement is sometimes called “extensive”. Nonetheless, it is important to note that the two distinctions are based on significantly different criteria of measurability. As discussed in Section 2 , the extensive-intensive distinction focused on the intrinsic structure of the quantity in question, i.e., whether or not it is composed of spatio-temporal parts. The fundamental-derived distinction, by contrast, focuses on the properties of measurement operations . A fundamentally measurable magnitude is one for which a fundamental measurement operation has been found. Consequently, fundamentality is not an intrinsic property of a magnitude: a derived magnitude can become fundamental with the discovery of new operations for its measurement. Moreover, in fundamental measurement the numerical assignment need not mirror the structure of spatio-temporal parts. Electrical resistance, for example, can be fundamentally measured by connecting resistors in a series (Campbell 1920: 293). This is considered a fundamental measurement operation because it has a shared structure with numerical addition, even though objects with equal resistance are not generally equal in size.
The distinction between fundamental and derived measurement was revised by subsequent authors. Brian Ellis (1966: Ch. 5–8) distinguished among three types of measurement: fundamental, associative and derived. Fundamental measurement requires ordering and concatenation operations satisfying the same conditions specified by Campbell. Associative measurement procedures are based on a correlation of two ordering relationships, e.g., the correlation between the volume of a mercury column and its temperature. Derived measurement procedures consist in the determination of the value of a constant in a physical law. The constant may be local, as in the determination of the specific density of water from mass and volume, or universal, as in the determination of the Newtonian gravitational constant from force, mass and distance. Henry Kyburg (1984: Ch. 5–7) proposed a somewhat different threefold distinction among direct, indirect and systematic measurement, which does not completely overlap with that of Ellis. [ 4 ] A more radical revision of the distinction between fundamental and derived measurement was offered by R. Duncan Luce and John Tukey (1964) in their work on conjoint measurement, which will be discussed in Section 3.4 .

Want to create or adapt books like this? Learn more about how Pressbooks supports open publishing practices.
5.2 Reliability and Validity of Measurement
Learning objectives.
- Define reliability, including the different types and how they are assessed.
- Define validity, including the different types and how they are assessed.
- Describe the kinds of evidence that would be relevant to assessing the reliability and validity of a particular measure.
Again, measurement involves assigning scores to individuals so that they represent some characteristic of the individuals. But how do researchers know that the scores actually represent the characteristic, especially when it is a construct like intelligence, self-esteem, depression, or working memory capacity? The answer is that they conduct research using the measure to confirm that the scores make sense based on their understanding of the construct being measured. This is an extremely important point. Psychologists do not simply assume that their measures work. Instead, they collect data to demonstrate that they work. If their research does not demonstrate that a measure works, they stop using it.
As an informal example, imagine that you have been dieting for a month. Your clothes seem to be fitting more loosely, and several friends have asked if you have lost weight. If at this point your bathroom scale indicated that you had lost 10 pounds, this would make sense and you would continue to use the scale. But if it indicated that you had gained 10 pounds, you would rightly conclude that it was broken and either fix it or get rid of it. In evaluating a measurement method, psychologists consider two general dimensions: reliability and validity.
Reliability
Reliability refers to the consistency of a measure. Psychologists consider three types of consistency: over time (test-retest reliability), across items (internal consistency), and across different researchers (interrater reliability).
Test-Retest Reliability
When researchers measure a construct that they assume to be consistent across time, then the scores they obtain should also be consistent across time. Test-retest reliability is the extent to which this is actually the case. For example, intelligence is generally thought to be consistent across time. A person who is highly intelligent today will be highly intelligent next week. This means that any good measure of intelligence should produce roughly the same scores for this individual next week as it does today. Clearly, a measure that produces highly inconsistent scores over time cannot be a very good measure of a construct that is supposed to be consistent.
Assessing test-retest reliability requires using the measure on a group of people at one time, using it again on the same group of people at a later time, and then looking at test-retest correlation between the two sets of scores. This is typically done by graphing the data in a scatterplot and computing Pearson’s r . Figure 5.3 “Test-Retest Correlation Between Two Sets of Scores of Several College Students on the Rosenberg Self-Esteem Scale, Given Two Times a Week Apart” shows the correlation between two sets of scores of several college students on the Rosenberg Self-Esteem Scale, given two times a week apart. Pearson’s r for these data is +.95. In general, a test-retest correlation of +.80 or greater is considered to indicate good reliability.
Figure 5.3 Test-Retest Correlation Between Two Sets of Scores of Several College Students on the Rosenberg Self-Esteem Scale, Given Two Times a Week Apart
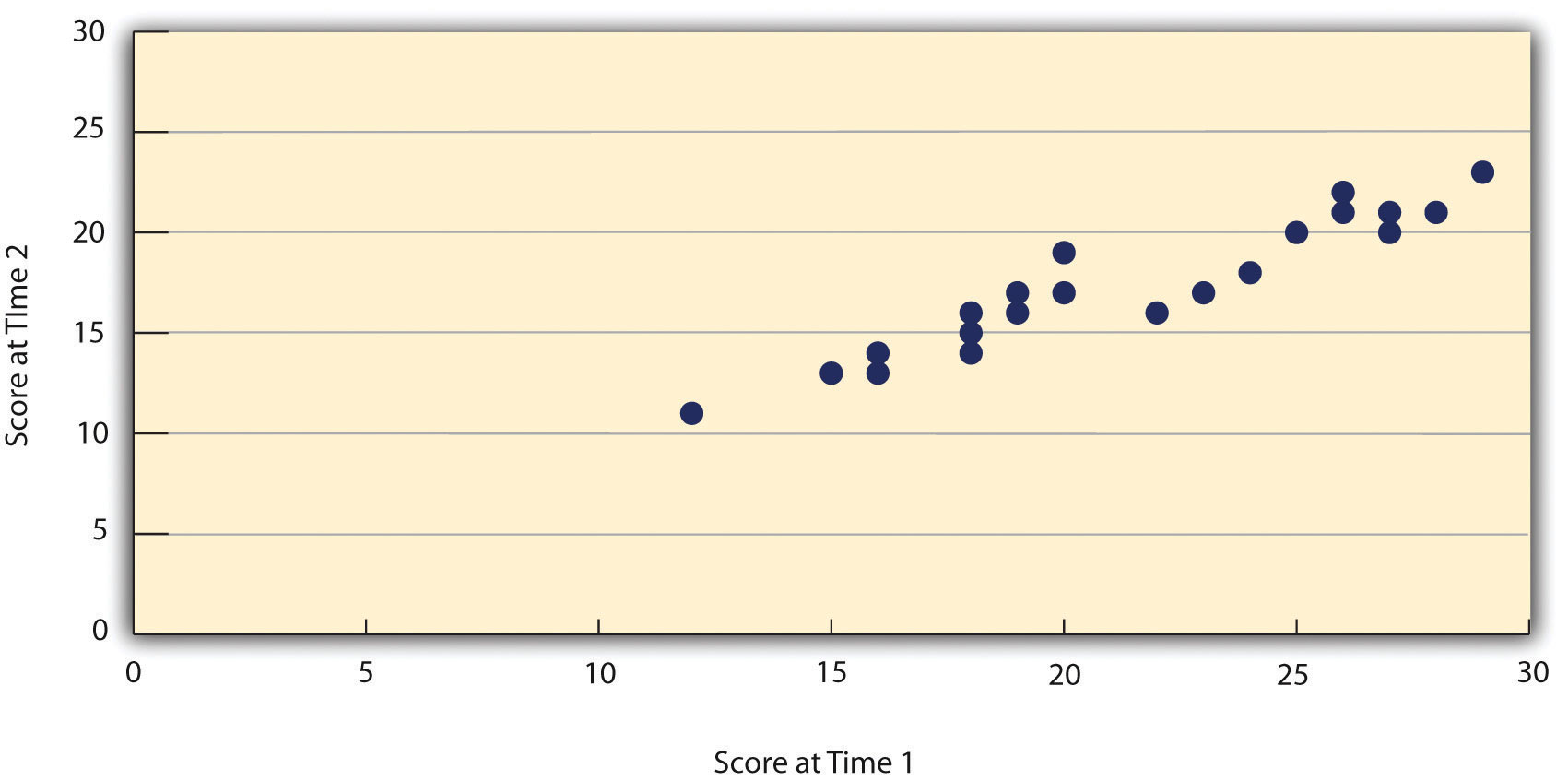
Again, high test-retest correlations make sense when the construct being measured is assumed to be consistent over time, which is the case for intelligence, self-esteem, and the Big Five personality dimensions. But other constructs are not assumed to be stable over time. The very nature of mood, for example, is that it changes. So a measure of mood that produced a low test-retest correlation over a period of a month would not be a cause for concern.
Internal Consistency
A second kind of reliability is internal consistency , which is the consistency of people’s responses across the items on a multiple-item measure. In general, all the items on such measures are supposed to reflect the same underlying construct, so people’s scores on those items should be correlated with each other. On the Rosenberg Self-Esteem Scale, people who agree that they are a person of worth should tend to agree that that they have a number of good qualities. If people’s responses to the different items are not correlated with each other, then it would no longer make sense to claim that they are all measuring the same underlying construct. This is as true for behavioral and physiological measures as for self-report measures. For example, people might make a series of bets in a simulated game of roulette as a measure of their level of risk seeking. This measure would be internally consistent to the extent that individual participants’ bets were consistently high or low across trials.
Like test-retest reliability, internal consistency can only be assessed by collecting and analyzing data. One approach is to look at a split-half correlation . This involves splitting the items into two sets, such as the first and second halves of the items or the even- and odd-numbered items. Then a score is computed for each set of items, and the relationship between the two sets of scores is examined. For example, Figure 5.4 “Split-Half Correlation Between Several College Students’ Scores on the Even-Numbered Items and Their Scores on the Odd-Numbered Items of the Rosenberg Self-Esteem Scale” shows the split-half correlation between several college students’ scores on the even-numbered items and their scores on the odd-numbered items of the Rosenberg Self-Esteem Scale. Pearson’s r for these data is +.88. A split-half correlation of +.80 or greater is generally considered good internal consistency.
Figure 5.4 Split-Half Correlation Between Several College Students’ Scores on the Even-Numbered Items and Their Scores on the Odd-Numbered Items of the Rosenberg Self-Esteem Scale
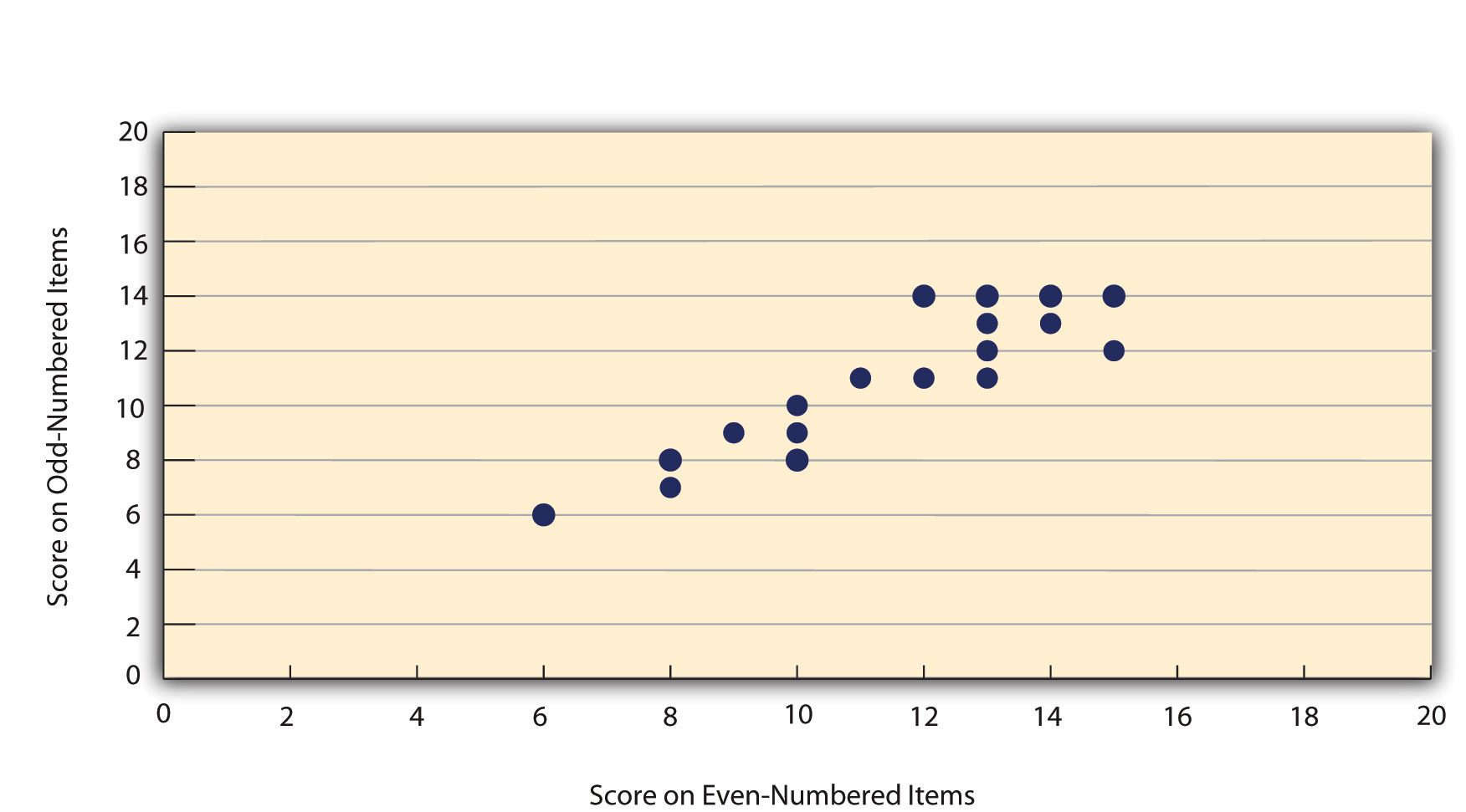
Perhaps the most common measure of internal consistency used by researchers in psychology is a statistic called Cronbach’s α (the Greek letter alpha). Conceptually, α is the mean of all possible split-half correlations for a set of items. For example, there are 252 ways to split a set of 10 items into two sets of five. Cronbach’s α would be the mean of the 252 split-half correlations. Note that this is not how α is actually computed, but it is a correct way of interpreting the meaning of this statistic. Again, a value of +.80 or greater is generally taken to indicate good internal consistency.
Interrater Reliability
Many behavioral measures involve significant judgment on the part of an observer or a rater. Interrater reliability is the extent to which different observers are consistent in their judgments. For example, if you were interested in measuring college students’ social skills, you could make video recordings of them as they interacted with another student whom they are meeting for the first time. Then you could have two or more observers watch the videos and rate each student’s level of social skills. To the extent that each participant does in fact have some level of social skills that can be detected by an attentive observer, different observers’ ratings should be highly correlated with each other. If they were not, then those ratings could not be an accurate representation of participants’ social skills. Interrater reliability is often assessed using Cronbach’s α when the judgments are quantitative or an analogous statistic called Cohen’s κ (the Greek letter kappa) when they are categorical.
Validity is the extent to which the scores from a measure represent the variable they are intended to. But how do researchers make this judgment? We have already considered one factor that they take into account—reliability. When a measure has good test-retest reliability and internal consistency, researchers should be more confident that the scores represent what they are supposed to. There has to be more to it, however, because a measure can be extremely reliable but have no validity whatsoever. As an absurd example, imagine someone who believes that people’s index finger length reflects their self-esteem and therefore tries to measure self-esteem by holding a ruler up to people’s index fingers. Although this measure would have extremely good test-retest reliability, it would have absolutely no validity. The fact that one person’s index finger is a centimeter longer than another’s would indicate nothing about which one had higher self-esteem.
Textbook presentations of validity usually divide it into several distinct “types.” But a good way to interpret these types is that they are other kinds of evidence—in addition to reliability—that should be taken into account when judging the validity of a measure. Here we consider four basic kinds: face validity, content validity, criterion validity, and discriminant validity.
Face Validity
Face validity is the extent to which a measurement method appears “on its face” to measure the construct of interest. Most people would expect a self-esteem questionnaire to include items about whether they see themselves as a person of worth and whether they think they have good qualities. So a questionnaire that included these kinds of items would have good face validity. The finger-length method of measuring self-esteem, on the other hand, seems to have nothing to do with self-esteem and therefore has poor face validity. Although face validity can be assessed quantitatively—for example, by having a large sample of people rate a measure in terms of whether it appears to measure what it is intended to—it is usually assessed informally.
Face validity is at best a very weak kind of evidence that a measurement method is measuring what it is supposed to. One reason is that it is based on people’s intuitions about human behavior, which are frequently wrong. It is also the case that many established measures in psychology work quite well despite lacking face validity. The Minnesota Multiphasic Personality Inventory (MMPI) measures many personality characteristics and disorders by having people decide whether each of over 567 different statements applies to them—where many of the statements do not have any obvious relationship to the construct that they measure. Another example is the Implicit Association Test, which measures prejudice in a way that is nonintuitive to most people (see Note 5.31 “How Prejudiced Are You?” ).
How Prejudiced Are You?
The Implicit Association Test (IAT) is used to measure people’s attitudes toward various social groups. The IAT is a behavioral measure designed to reveal negative attitudes that people might not admit to on a self-report measure. It focuses on how quickly people are able to categorize words and images representing two contrasting groups (e.g., gay and straight) along with other positive and negative stimuli (e.g., the words “wonderful” or “nasty”). The IAT has been used in dozens of published research studies, and there is strong evidence for both its reliability and its validity (Nosek, Greenwald, & Banaji, 2006). You can learn more about the IAT—and take several of them for yourself—at the following website: https://implicit.harvard.edu/implicit .
Content Validity
Content validity is the extent to which a measure “covers” the construct of interest. For example, if a researcher conceptually defines test anxiety as involving both sympathetic nervous system activation (leading to nervous feelings) and negative thoughts, then his measure of test anxiety should include items about both nervous feelings and negative thoughts. Or consider that attitudes are usually defined as involving thoughts, feelings, and actions toward something. By this conceptual definition, a person has a positive attitude toward exercise to the extent that he or she thinks positive thoughts about exercising, feels good about exercising, and actually exercises. So to have good content validity, a measure of people’s attitudes toward exercise would have to reflect all three of these aspects. Like face validity, content validity is not usually assessed quantitatively. Instead, it is assessed by carefully checking the measurement method against the conceptual definition of the construct.
Criterion Validity
Criterion validity is the extent to which people’s scores on a measure are correlated with other variables (known as criteria ) that one would expect them to be correlated with. For example, people’s scores on a new measure of test anxiety should be negatively correlated with their performance on an important school exam. If it were found that people’s scores were in fact negatively correlated with their exam performance, then this would be a piece of evidence that these scores really represent people’s test anxiety. But if it were found that people scored equally well on the exam regardless of their test anxiety scores, then this would cast doubt on the validity of the measure.
A criterion can be any variable that one has reason to think should be correlated with the construct being measured, and there will usually be many of them. For example, one would expect test anxiety scores to be negatively correlated with exam performance and course grades and positively correlated with general anxiety and with blood pressure during an exam. Or imagine that a researcher develops a new measure of physical risk taking. People’s scores on this measure should be correlated with their participation in “extreme” activities such as snowboarding and rock climbing, the number of speeding tickets they have received, and even the number of broken bones they have had over the years. Criteria can also include other measures of the same construct. For example, one would expect new measures of test anxiety or physical risk taking to be positively correlated with existing measures of the same constructs. So the use of converging operations is one way to examine criterion validity.
Assessing criterion validity requires collecting data using the measure. Researchers John Cacioppo and Richard Petty did this when they created their self-report Need for Cognition Scale to measure how much people value and engage in thinking (Cacioppo & Petty, 1982). In a series of studies, they showed that college faculty scored higher than assembly-line workers, that people’s scores were positively correlated with their scores on a standardized academic achievement test, and that their scores were negatively correlated with their scores on a measure of dogmatism (which represents a tendency toward obedience). In the years since it was created, the Need for Cognition Scale has been used in literally hundreds of studies and has been shown to be correlated with a wide variety of other variables, including the effectiveness of an advertisement, interest in politics, and juror decisions (Petty, Briñol, Loersch, & McCaslin, 2009).
Discriminant Validity
Discriminant validity is the extent to which scores on a measure are not correlated with measures of variables that are conceptually distinct. For example, self-esteem is a general attitude toward the self that is fairly stable over time. It is not the same as mood, which is how good or bad one happens to be feeling right now. So people’s scores on a new measure of self-esteem should not be very highly correlated with their moods. If the new measure of self-esteem were highly correlated with a measure of mood, it could be argued that the new measure is not really measuring self-esteem; it is measuring mood instead.
When they created the Need for Cognition Scale, Cacioppo and Petty also provided evidence of discriminant validity by showing that people’s scores were not correlated with certain other variables. For example, they found only a weak correlation between people’s need for cognition and a measure of their cognitive style—the extent to which they tend to think analytically by breaking ideas into smaller parts or holistically in terms of “the big picture.” They also found no correlation between people’s need for cognition and measures of their test anxiety and their tendency to respond in socially desirable ways. All these low correlations provide evidence that the measure is reflecting a conceptually distinct construct.
Key Takeaways
- Psychological researchers do not simply assume that their measures work. Instead, they conduct research to show that they work. If they cannot show that they work, they stop using them.
- There are two distinct criteria by which researchers evaluate their measures: reliability and validity. Reliability is consistency across time (test-retest reliability), across items (internal consistency), and across researchers (interrater reliability). Validity is the extent to which the scores actually represent the variable they are intended to.
- Validity is a judgment based on various types of evidence. The relevant evidence includes the measure’s reliability, whether it covers the construct of interest, and whether the scores it produces are correlated with other variables they are expected to be correlated with and not correlated with variables that are conceptually distinct.
- The reliability and validity of a measure is not established by any single study but by the pattern of results across multiple studies. The assessment of reliability and validity is an ongoing process.
- Practice: Ask several friends to complete the Rosenberg Self-Esteem Scale. Then assess its internal consistency by making a scatterplot to show the split-half correlation (even- vs. odd-numbered items). Compute Pearson’s r too if you know how.
- Discussion: Think back to the last college exam you took and think of the exam as a psychological measure. What construct do you think it was intended to measure? Comment on its face and content validity. What data could you collect to assess its reliability, criterion validity, and discriminant validity?
- Practice: Take an Implicit Association Test and then list as many ways to assess its criterion validity as you can think of.
Cacioppo, J. T., & Petty, R. E. (1982). The need for cognition. Journal of Personality and Social Psychology, 42 , 116–131.
Nosek, B. A., Greenwald, A. G., & Banaji, M. R. (2006). The Implicit Association Test at age 7: A methodological and conceptual review. In J. A. Bargh (Ed.), Social psychology and the unconscious: The automaticity of higher mental processes (pp. 265–292). London, England: Psychology Press.
Petty, R. E, Briñol, P., Loersch, C., & McCaslin, M. J. (2009). The need for cognition. In M. R. Leary & R. H. Hoyle (Eds.), Handbook of individual differences in social behavior (pp. 318–329). New York, NY: Guilford Press.
Research Methods in Psychology Copyright © 2016 by University of Minnesota is licensed under a Creative Commons Attribution-NonCommercial-ShareAlike 4.0 International License , except where otherwise noted.
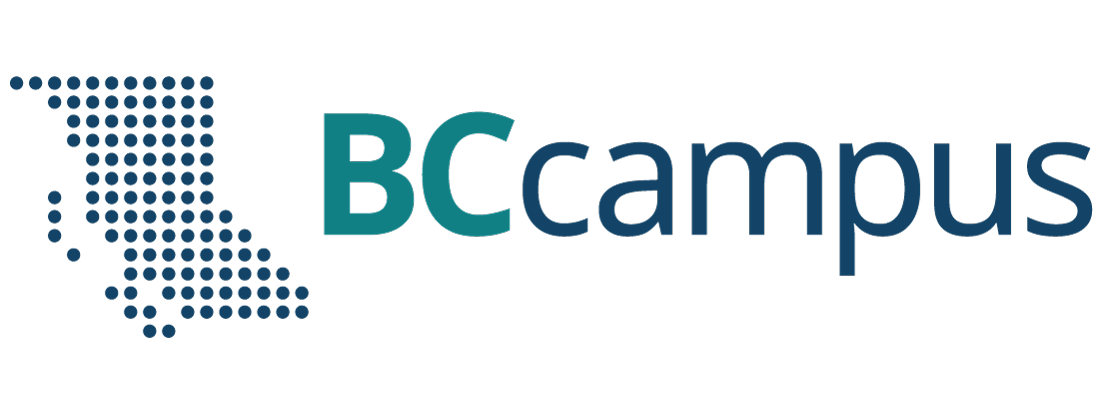
Want to create or adapt books like this? Learn more about how Pressbooks supports open publishing practices.
Chapter 5: Psychological Measurement
Reliability and Validity of Measurement
Learning Objectives
- Define reliability, including the different types and how they are assessed.
- Define validity, including the different types and how they are assessed.
- Describe the kinds of evidence that would be relevant to assessing the reliability and validity of a particular measure.
Again, measurement involves assigning scores to individuals so that they represent some characteristic of the individuals. But how do researchers know that the scores actually represent the characteristic, especially when it is a construct like intelligence, self-esteem, depression, or working memory capacity? The answer is that they conduct research using the measure to confirm that the scores make sense based on their understanding of the construct being measured. This is an extremely important point. Psychologists do not simply assume that their measures work. Instead, they collect data to demonstrate that they work. If their research does not demonstrate that a measure works, they stop using it.
As an informal example, imagine that you have been dieting for a month. Your clothes seem to be fitting more loosely, and several friends have asked if you have lost weight. If at this point your bathroom scale indicated that you had lost 10 pounds, this would make sense and you would continue to use the scale. But if it indicated that you had gained 10 pounds, you would rightly conclude that it was broken and either fix it or get rid of it. In evaluating a measurement method, psychologists consider two general dimensions: reliability and validity.
Reliability
Reliability refers to the consistency of a measure. Psychologists consider three types of consistency: over time (test-retest reliability), across items (internal consistency), and across different researchers (inter-rater reliability).
Test-Retest Reliability
When researchers measure a construct that they assume to be consistent across time, then the scores they obtain should also be consistent across time. Test-retest reliability is the extent to which this is actually the case. For example, intelligence is generally thought to be consistent across time. A person who is highly intelligent today will be highly intelligent next week. This means that any good measure of intelligence should produce roughly the same scores for this individual next week as it does today. Clearly, a measure that produces highly inconsistent scores over time cannot be a very good measure of a construct that is supposed to be consistent.
Assessing test-retest reliability requires using the measure on a group of people at one time, using it again on the same group of people at a later time, and then looking at test-retest correlation between the two sets of scores. This is typically done by graphing the data in a scatterplot and computing Pearson’s r . Figure 5.2 shows the correlation between two sets of scores of several university students on the Rosenberg Self-Esteem Scale, administered two times, a week apart. Pearson’s r for these data is +.95. In general, a test-retest correlation of +.80 or greater is considered to indicate good reliability.
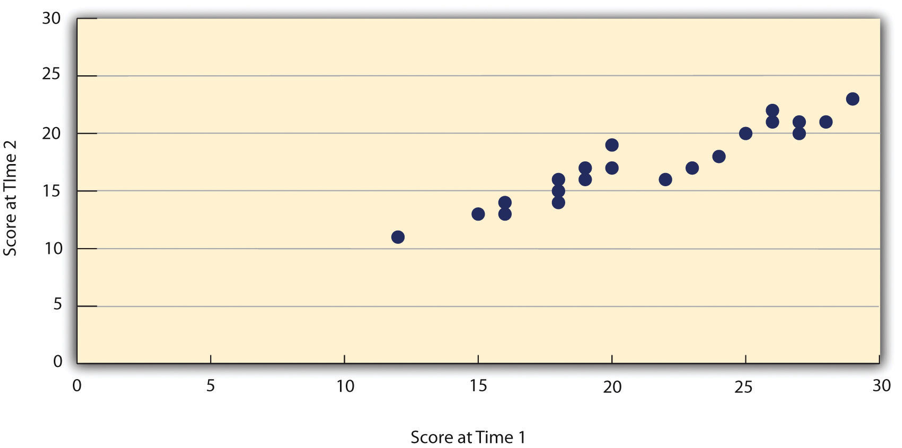
Again, high test-retest correlations make sense when the construct being measured is assumed to be consistent over time, which is the case for intelligence, self-esteem, and the Big Five personality dimensions. But other constructs are not assumed to be stable over time. The very nature of mood, for example, is that it changes. So a measure of mood that produced a low test-retest correlation over a period of a month would not be a cause for concern.
Internal Consistency
A second kind of reliability is internal consistency , which is the consistency of people’s responses across the items on a multiple-item measure. In general, all the items on such measures are supposed to reflect the same underlying construct, so people’s scores on those items should be correlated with each other. On the Rosenberg Self-Esteem Scale, people who agree that they are a person of worth should tend to agree that that they have a number of good qualities. If people’s responses to the different items are not correlated with each other, then it would no longer make sense to claim that they are all measuring the same underlying construct. This is as true for behavioural and physiological measures as for self-report measures. For example, people might make a series of bets in a simulated game of roulette as a measure of their level of risk seeking. This measure would be internally consistent to the extent that individual participants’ bets were consistently high or low across trials.
Like test-retest reliability, internal consistency can only be assessed by collecting and analyzing data. One approach is to look at a split-half correlation . This involves splitting the items into two sets, such as the first and second halves of the items or the even- and odd-numbered items. Then a score is computed for each set of items, and the relationship between the two sets of scores is examined. For example, Figure 5.3 shows the split-half correlation between several university students’ scores on the even-numbered items and their scores on the odd-numbered items of the Rosenberg Self-Esteem Scale. Pearson’s r for these data is +.88. A split-half correlation of +.80 or greater is generally considered good internal consistency.
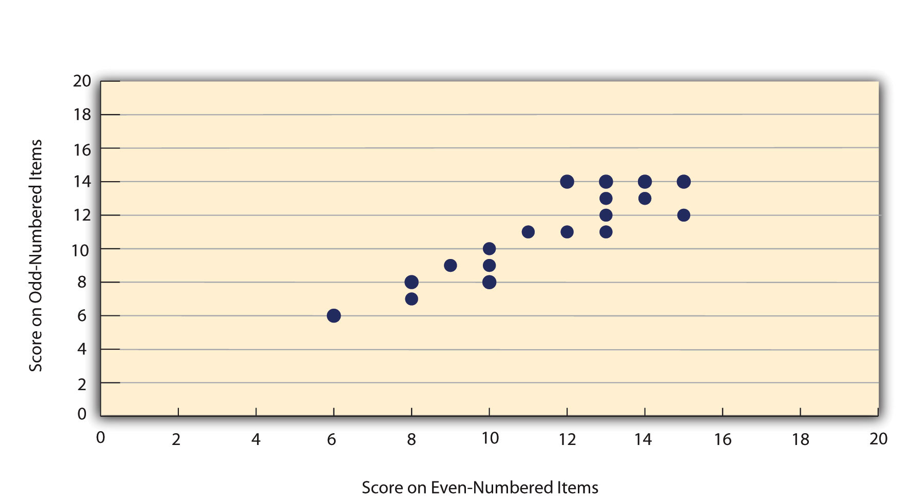
Perhaps the most common measure of internal consistency used by researchers in psychology is a statistic called Cronbach’s α (the Greek letter alpha). Conceptually, α is the mean of all possible split-half correlations for a set of items. For example, there are 252 ways to split a set of 10 items into two sets of five. Cronbach’s α would be the mean of the 252 split-half correlations. Note that this is not how α is actually computed, but it is a correct way of interpreting the meaning of this statistic. Again, a value of +.80 or greater is generally taken to indicate good internal consistency.
Interrater Reliability
Many behavioural measures involve significant judgment on the part of an observer or a rater. Inter-rater reliability is the extent to which different observers are consistent in their judgments. For example, if you were interested in measuring university students’ social skills, you could make video recordings of them as they interacted with another student whom they are meeting for the first time. Then you could have two or more observers watch the videos and rate each student’s level of social skills. To the extent that each participant does in fact have some level of social skills that can be detected by an attentive observer, different observers’ ratings should be highly correlated with each other. Inter-rater reliability would also have been measured in Bandura’s Bobo doll study. In this case, the observers’ ratings of how many acts of aggression a particular child committed while playing with the Bobo doll should have been highly positively correlated. Interrater reliability is often assessed using Cronbach’s α when the judgments are quantitative or an analogous statistic called Cohen’s κ (the Greek letter kappa) when they are categorical.
Validity is the extent to which the scores from a measure represent the variable they are intended to. But how do researchers make this judgment? We have already considered one factor that they take into account—reliability. When a measure has good test-retest reliability and internal consistency, researchers should be more confident that the scores represent what they are supposed to. There has to be more to it, however, because a measure can be extremely reliable but have no validity whatsoever. As an absurd example, imagine someone who believes that people’s index finger length reflects their self-esteem and therefore tries to measure self-esteem by holding a ruler up to people’s index fingers. Although this measure would have extremely good test-retest reliability, it would have absolutely no validity. The fact that one person’s index finger is a centimetre longer than another’s would indicate nothing about which one had higher self-esteem.
Discussions of validity usually divide it into several distinct “types.” But a good way to interpret these types is that they are other kinds of evidence—in addition to reliability—that should be taken into account when judging the validity of a measure. Here we consider three basic kinds: face validity, content validity, and criterion validity.
Face Validity
Face validity is the extent to which a measurement method appears “on its face” to measure the construct of interest. Most people would expect a self-esteem questionnaire to include items about whether they see themselves as a person of worth and whether they think they have good qualities. So a questionnaire that included these kinds of items would have good face validity. The finger-length method of measuring self-esteem, on the other hand, seems to have nothing to do with self-esteem and therefore has poor face validity. Although face validity can be assessed quantitatively—for example, by having a large sample of people rate a measure in terms of whether it appears to measure what it is intended to—it is usually assessed informally.
Face validity is at best a very weak kind of evidence that a measurement method is measuring what it is supposed to. One reason is that it is based on people’s intuitions about human behaviour, which are frequently wrong. It is also the case that many established measures in psychology work quite well despite lacking face validity. The Minnesota Multiphasic Personality Inventory-2 (MMPI-2) measures many personality characteristics and disorders by having people decide whether each of over 567 different statements applies to them—where many of the statements do not have any obvious relationship to the construct that they measure. For example, the items “I enjoy detective or mystery stories” and “The sight of blood doesn’t frighten me or make me sick” both measure the suppression of aggression. In this case, it is not the participants’ literal answers to these questions that are of interest, but rather whether the pattern of the participants’ responses to a series of questions matches those of individuals who tend to suppress their aggression.
Content Validity
Content validity is the extent to which a measure “covers” the construct of interest. For example, if a researcher conceptually defines test anxiety as involving both sympathetic nervous system activation (leading to nervous feelings) and negative thoughts, then his measure of test anxiety should include items about both nervous feelings and negative thoughts. Or consider that attitudes are usually defined as involving thoughts, feelings, and actions toward something. By this conceptual definition, a person has a positive attitude toward exercise to the extent that he or she thinks positive thoughts about exercising, feels good about exercising, and actually exercises. So to have good content validity, a measure of people’s attitudes toward exercise would have to reflect all three of these aspects. Like face validity, content validity is not usually assessed quantitatively. Instead, it is assessed by carefully checking the measurement method against the conceptual definition of the construct.
Criterion Validity
Criterion validity is the extent to which people’s scores on a measure are correlated with other variables (known as criteria ) that one would expect them to be correlated with. For example, people’s scores on a new measure of test anxiety should be negatively correlated with their performance on an important school exam. If it were found that people’s scores were in fact negatively correlated with their exam performance, then this would be a piece of evidence that these scores really represent people’s test anxiety. But if it were found that people scored equally well on the exam regardless of their test anxiety scores, then this would cast doubt on the validity of the measure.
A criterion can be any variable that one has reason to think should be correlated with the construct being measured, and there will usually be many of them. For example, one would expect test anxiety scores to be negatively correlated with exam performance and course grades and positively correlated with general anxiety and with blood pressure during an exam. Or imagine that a researcher develops a new measure of physical risk taking. People’s scores on this measure should be correlated with their participation in “extreme” activities such as snowboarding and rock climbing, the number of speeding tickets they have received, and even the number of broken bones they have had over the years. When the criterion is measured at the same time as the construct, criterion validity is referred to as concurrent validity ; however, when the criterion is measured at some point in the future (after the construct has been measured), it is referred to as predictive validity (because scores on the measure have “predicted” a future outcome).
Criteria can also include other measures of the same construct. For example, one would expect new measures of test anxiety or physical risk taking to be positively correlated with existing measures of the same constructs. This is known as convergent validity .
Assessing convergent validity requires collecting data using the measure. Researchers John Cacioppo and Richard Petty did this when they created their self-report Need for Cognition Scale to measure how much people value and engage in thinking (Cacioppo & Petty, 1982) [1] . In a series of studies, they showed that people’s scores were positively correlated with their scores on a standardized academic achievement test, and that their scores were negatively correlated with their scores on a measure of dogmatism (which represents a tendency toward obedience). In the years since it was created, the Need for Cognition Scale has been used in literally hundreds of studies and has been shown to be correlated with a wide variety of other variables, including the effectiveness of an advertisement, interest in politics, and juror decisions (Petty, Briñol, Loersch, & McCaslin, 2009) [2] .
Discriminant Validity
Discriminant validity , on the other hand, is the extent to which scores on a measure are not correlated with measures of variables that are conceptually distinct. For example, self-esteem is a general attitude toward the self that is fairly stable over time. It is not the same as mood, which is how good or bad one happens to be feeling right now. So people’s scores on a new measure of self-esteem should not be very highly correlated with their moods. If the new measure of self-esteem were highly correlated with a measure of mood, it could be argued that the new measure is not really measuring self-esteem; it is measuring mood instead.
When they created the Need for Cognition Scale, Cacioppo and Petty also provided evidence of discriminant validity by showing that people’s scores were not correlated with certain other variables. For example, they found only a weak correlation between people’s need for cognition and a measure of their cognitive style—the extent to which they tend to think analytically by breaking ideas into smaller parts or holistically in terms of “the big picture.” They also found no correlation between people’s need for cognition and measures of their test anxiety and their tendency to respond in socially desirable ways. All these low correlations provide evidence that the measure is reflecting a conceptually distinct construct.
Key Takeaways
- Psychological researchers do not simply assume that their measures work. Instead, they conduct research to show that they work. If they cannot show that they work, they stop using them.
- There are two distinct criteria by which researchers evaluate their measures: reliability and validity. Reliability is consistency across time (test-retest reliability), across items (internal consistency), and across researchers (interrater reliability). Validity is the extent to which the scores actually represent the variable they are intended to.
- Validity is a judgment based on various types of evidence. The relevant evidence includes the measure’s reliability, whether it covers the construct of interest, and whether the scores it produces are correlated with other variables they are expected to be correlated with and not correlated with variables that are conceptually distinct.
- The reliability and validity of a measure is not established by any single study but by the pattern of results across multiple studies. The assessment of reliability and validity is an ongoing process.
- Practice: Ask several friends to complete the Rosenberg Self-Esteem Scale. Then assess its internal consistency by making a scatterplot to show the split-half correlation (even- vs. odd-numbered items). Compute Pearson’s r too if you know how.
- Discussion: Think back to the last college exam you took and think of the exam as a psychological measure. What construct do you think it was intended to measure? Comment on its face and content validity. What data could you collect to assess its reliability and criterion validity?
- Cacioppo, J. T., & Petty, R. E. (1982). The need for cognition. Journal of Personality and Social Psychology, 42 , 116–131. ↵
- Petty, R. E, Briñol, P., Loersch, C., & McCaslin, M. J. (2009). The need for cognition. In M. R. Leary & R. H. Hoyle (Eds.), Handbook of individual differences in social behaviour (pp. 318–329). New York, NY: Guilford Press. ↵
The consistency of a measure.
The consistency of a measure over time.
The consistency of a measure on the same group of people at different times.
Consistency of people’s responses across the items on a multiple-item measure.
Method of assessing internal consistency through splitting the items into two sets and examining the relationship between them.
A statistic in which α is the mean of all possible split-half correlations for a set of items.
The extent to which different observers are consistent in their judgments.
The extent to which the scores from a measure represent the variable they are intended to.
The extent to which a measurement method appears to measure the construct of interest.
The extent to which a measure “covers” the construct of interest.
The extent to which people’s scores on a measure are correlated with other variables that one would expect them to be correlated with.
In reference to criterion validity, variables that one would expect to be correlated with the measure.
When the criterion is measured at the same time as the construct.
when the criterion is measured at some point in the future (after the construct has been measured).
When new measures positively correlate with existing measures of the same constructs.
The extent to which scores on a measure are not correlated with measures of variables that are conceptually distinct.
Research Methods in Psychology - 2nd Canadian Edition Copyright © 2015 by Paul C. Price, Rajiv Jhangiani, & I-Chant A. Chiang is licensed under a Creative Commons Attribution-NonCommercial-ShareAlike 4.0 International License , except where otherwise noted.
Share This Book
Popular searches
- How to Get Participants For Your Study
- How to Do Segmentation?
- Conjoint Preference Share Simulator
- MaxDiff Analysis
- Likert Scales
- Reliability & Validity
Request consultation
Do you need support in running a pricing or product study? We can help you with agile consumer research and conjoint analysis.
Looking for an online survey platform?
Conjointly offers a great survey tool with multiple question types, randomisation blocks, and multilingual support. The Basic tier is always free.
Research Methods Knowledge Base
- Navigating the Knowledge Base
- Foundations
- Construct Validity
- Reliability
- Levels of Measurement
- Survey Research
- Scaling in Measurement
- Qualitative Measures
- Unobtrusive Measures
- Research Design
- Table of Contents
Fully-functional online survey tool with various question types, logic, randomisation, and reporting for unlimited number of surveys.
Completely free for academics and students .
Measurement
Measurement is the process of observing and recording the observations that are collected as part of a research effort. There are two major issues that will be considered here.
First, you have to understand the fundamental ideas involved in measuring. Here we consider two of major measurement concepts. In Levels of Measurement , I explain the meaning of the four major levels of measurement: nominal, ordinal, interval and ratio. Then we move on to the reliability of measurement, including consideration of true score theory and a variety of reliability estimators.
Second, you have to understand the different types of measures that you might use in social research. We consider four broad categories of measurements. Survey research includes the design and implementation of interviews and questionnaires. Scaling involves consideration of the major methods of developing and implementing a scale. Qualitative research provides an overview of the broad range of non-numerical measurement approaches. And unobtrusive measures presents a variety of measurement methods that don’t intrude on or interfere with the context of the research.
Cookie Consent
Conjointly uses essential cookies to make our site work. We also use additional cookies in order to understand the usage of the site, gather audience analytics, and for remarketing purposes.
For more information on Conjointly's use of cookies, please read our Cookie Policy .
Which one are you?
I am new to conjointly, i am already using conjointly.
Measurements in quantitative research: how to select and report on research instruments
Affiliation.
- 1 Department of Acute and Tertiary Care in the School of Nursing, University of Pittsburgh in Pennsylvania.
- PMID: 24969252
- DOI: 10.1188/14.ONF.431-433
Measures exist to numerically represent degrees of attributes. Quantitative research is based on measurement and is conducted in a systematic, controlled manner. These measures enable researchers to perform statistical tests, analyze differences between groups, and determine the effectiveness of treatments. If something is not measurable, it cannot be tested.
Keywords: measurements; quantitative research; reliability; validity.
- Clinical Nursing Research / methods*
- Clinical Nursing Research / standards
- Fatigue / nursing*
- Neoplasms / nursing*
- Oncology Nursing*
- Quality of Life*
- Reproducibility of Results
- Member Benefits
- Communities
- Grants and Scholarships
- Student Nurse Resources
- Member Directory
- Course Login
- Professional Development
- Institutions Hub
- ONS Course Catalog
- ONS Book Catalog
- ONS Oncology Nurse Orientation Program™
- Account Settings
- Help Center
- Print Membership Card
- Print NCPD Certificate
- Verify Cardholder or Certificate Status
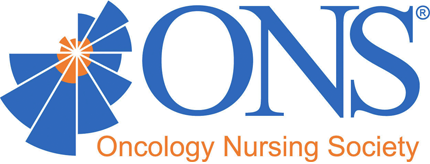
- Trouble finding what you need?
- Check our search tips.
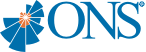
- Oncology Nursing Forum
- Number 4 / July 2014
Measurements in Quantitative Research: How to Select and Report on Research Instruments
Teresa L. Hagan
Measures exist to numerically represent degrees of attributes. Quantitative research is based on measurement and is conducted in a systematic, controlled manner. These measures enable researchers to perform statistical tests, analyze differences between groups, and determine the effectiveness of treatments. If something is not measurable, it cannot be tested.
Jump to a section
Related articles, systematic reviews, case study research methodology in nursing research, preferred reporting items for systematic reviews and meta-analyses.
info This is a space for the teal alert bar.
notifications This is a space for the yellow alert bar.

Research Process
- Brainstorming
- Explore Google This link opens in a new window
- Explore Web Resources
- Explore Background Information
- Explore Books
- Explore Scholarly Articles
- Narrowing a Topic
- Primary and Secondary Resources
- Academic, Popular & Trade Publications
- Scholarly and Peer-Reviewed Journals
- Grey Literature
- Clinical Trials
- Evidence Based Treatment
- Scholarly Research
- Database Research Log
- Search Limits
- Keyword Searching
- Boolean Operators
- Phrase Searching
- Truncation & Wildcard Symbols
- Proximity Searching
- Field Codes
- Subject Terms and Database Thesauri
- Reading a Scientific Article
- Website Evaluation
- Article Keywords and Subject Terms
- Cited References
- Citing Articles
- Related Results
- Search Within Publication
- Database Alerts & RSS Feeds
- Personal Database Accounts
- Persistent URLs
- Literature Gap and Future Research
- Web of Knowledge
- Annual Reviews
- Systematic Reviews & Meta-Analyses
- Finding Seminal Works
- Exhausting the Literature
- Finding Dissertations
- Researching Theoretical Frameworks
- Research Methodology & Design
Tests and Measurements
- Organizing Research & Citations This link opens in a new window
- Scholarly Publication
- Learn the Library This link opens in a new window
Tests & Measurements FAQs
- Ask a Librarian Search all of the FAQs here.
NU Dissertation Center
If you are looking for a document in the Dissertation Center or Applied Doctoral Center and can't find it please contact your Chair or The Center for Teaching and Learning at [email protected]
- NCU Dissertation Center Find valuable resources and support materials to help you through your doctoral journey.
- Applied Doctoral Center Collection of resources to support students in completing their project/dissertation-in-practice as part of the Applied Doctoral Experience (ADE).
If you are doing dissertation level research, you will also be collecting your own data using a test or measure designed to address the variables present in your research. Finding the right test or measure can sometimes be difficult. In some cases, tests are copyrighted and must be purchased from commercial publishers. In other cases instruments can be obtained for free directly from the authors or can be found within published articles (in the methods section or as an appendix). The Library can help you with obtaining publisher or author information along with test reviews, if they are available.
One important decision you will eventually face in the dissertation process is whether to use an existing instrument, to modify an instrument, or to create your own instrument from scratch. The latter two will require extensive testing and are not generally recommended. Whichever decision you make should be thought over carefully and discussed with your mentor or dissertation chair committee.
You will need to either purchase the test from a publisher or contact author(s) to obtain the test along with copyright permissions to use it in your research. When contacting an author for copyright permissions you will often send a permission letter. Examples of permission letters are included in the Permission Letters section below.
Want a video introduction? See the Introduction to Tests and Measurements Workshop below.
Want to learn via a week's worth of daily lessons ? Sign up for our Tests & Measures - Learn in a Week series.
Introduction to Tests & Measurements Workshop
This workshop provides an introduction to library resources which can be used to locate tests and measurements for dissertation research.
- Introduction to Tests and Measurements Workshop Outline
Searching for Tests and Measurements
When conducting a search, remember that different keywords yield different results. Consider these terms and add them to your search string when trying to locate tests or measurements:
- Survey
- Instrument
- Questionnaire
- Measure
- Measurement
- Assessment
NavigatorSearch Search
Searching in NavigatorSearch
The simplest way to discover instruments relevant to your dissertation research is to carefully read the "Methods" section in peer-reviewed journal articles. A dissertation will build on a field of study and you will be well served by understanding how the constructs you are interested in have been measured. For example, while exploring the topic of depression, read articles and take note of which depression inventories are used and why.
- Start by conducting a keyword search on your topic using NavigatorSearch , the central search box found on the Library's homepage. NavigatorSearch searches most of our Library's database content, so it is a great starting point for any research topic.
- Use advanced search techniques covered in Searching 101 like subject searching, truncation, and Boolean operators to make your search more precise. You may also read about these search techniques by referring to the Preparing to Search section of our Research Process guide.
Library Databases
- APA PsycArticles & APA PsycInfo
- APA PsycTests
- ETS Test Link
- Health and Psychosocial Instruments (HAPI)
- MMY with Tests in Print
- ScienceDirect
- ProQuest Dissertations & Theses

Content: APA database that offers full-text for journals published by APA, the Canadian Psychological Association, Hogrefe Publishing Group and APA's Educational Publishing Foundation. View the APA PsycArticles Journal History for a complete coverage list.
Purpose: Important database for psychology, counseling, and education students.
Special Features: The database is updated bi-weekly all content is available in PDF and HTML formats.
Help using this database.

Content: Journal article database from the American Psychological Association that indexes over 2,500 journals along with book chapters and dissertations.
Purpose: Provides a single source of vetted, authoritative research for users across the behavioral and social sciences.
Special Features: citations in APA Style®, updated bi-weekly, spans 600 years of content
Searching in APA PsycArticles and APA PsycInfo
To locate tests and measurements in APA PsycArticles or APA PsycInfo, follow the below steps:
Content: Psychological tests and measures designed for use with social and behavioral science research
Purpose: Allows students and researchers to find and download instruments for research and/or teaching. Focused primarily on unpublished tests, this database was designed to save researchers time from having to reproduce tests when conducting research on previously measured constructs.
Special Features: Records include summary of the construct, and users can find information on reliability, validity, and factor analysis when that data is reported in the source document.
Searching in APA PsycTests
To locate tests and measurements in APA PsycTests, follow the below steps:
Content: EBSCO’s nursing database covering biomedicine, alternative/complementary medicine, consumer health, and allied health disciplines.
Purpose: Database for research in nursing, medicine, and consumer health.
Special Features: Strong qualitative studies. Filter studies by nurse as author, evidence-based practice, and type of study. Includes MESH indexing, PICO search functionality, text-to-speech feature for some articles, and a tool for discovering citing articles.
Searching in CINAHL
To search for tests or measurements in CINAHL, follow the below steps:

Additional Search Strategies for Locating Tests and Measurements in CINAHL
Content: Government (Department of Education) database focusing on education research and information.
Purpose: Excellent database to use for all topics in education.
Special Features: After an initial search, filter by audience, grade level, survey used, and main topic. Includes a thesaurus to aid in the discovery process.
This federally subsidized database indexes both journals and other resources important to educators. ERIC Journals (EJ): journal articles ERIC Documents (ED): all non-journal materials (some books, unpublished reports, and presentations) Some faculty limit use of EDs
Searching in ERIC
To search for tests or measurements in ERIC, follow the below steps:
- On the search results page, use the filters on the left-hand side to limit your results. Select Tests/Questionnaires under Publication Type .

In addition, the ERIC thesaurus entries list descriptors of tests and scales which may be used to construct a search. Select a broad category and continue narrowing down to your desired term. Click on Search collection using this descriptor to begin your search, as shown below.

For additional information, see the following quick tutorial video:
- ERIC Quick Tutorial Video
Content: A tests and measurements database containing standardized tests and research instruments.
Purpose: Allows users to search for tests and measurements, generally in the education field.
Special Features: A simple keyword strategy reveals many useful tests and measurements.
Searching in ETS TestLink
To locate tests and measurements for education in ETS TestLink, follow the below steps:

- ETS TestLink Quick Tutorial Video
Content: EBSCO database of test instruments found within articles.
Purpose: Provides users with instruments and measurements used in health and behavioral sciences and education
Special Features: Can be used along with APA PsycTests, ETS, and Mental Measurements to learn about instruments in the education and behavioral and health sciences.
A comprehensive bibliographic database providing information about behavioral measurement instruments. Information in the database is abstracted from hundreds of leading journals covering health sciences and psychosocial sciences.
HaPI provides free assistance to students in optimizing searches and locating hard copies and scoring instructions of specific assessment tools.
You can reach HaPI measurement staff either by phone (412-687-6850) or by email ( [email protected] ).
Searching in Health and Psychosocial Instruments (HAPI)
To locate tests and measurements in HAPI, follow the below steps:
Content: Contains reviews of test instruments and measures
Purpose: Users may learn about the strengths and weaknesses of particular test instruments.
Special Features: Includes automatic translation software
Searching in Mental Measurements Yearbook with Tests in Print
Mental Measurements Yearbook with Tests in Print (MMY with TiP) offers test reviews that are written by experts and contain descriptions of tests and commentary on their psychometric adequacy (Cone & Foster, 2006, pg. 170). You can use MMY with TiP to (1) obtain contact information for an author or publisher, and (2) read descriptive information on the measure of interest. Note that you will need to either purchase the test from the publisher directly, or contact author(s) to obtain the test along with copyright permissions to use it in your research.
To locate tests and measurements in Mental Measurements Yearbook with Tests in Print, follow the below steps:
- Click on a search result to obtain relevant information about the test or measurement, including Publisher Information, Purpose, Population, Time for completion, and Price Data among other details. A detailed review and summary of the test or measurement will also be provided.
Content: Includes citations to millions of biomedical journal articles, as well as some books, book chapters, and reports.
Purpose: An essential database for biomedical and health topics
Special Features: Includes MeSH search functionality
Searching in PubMed
To locate tests and measurements in PubMed, use the following strategies:
- Add any of the following MESH subject headings to your topic search string to locate relevant tests or measurements:
- "Research Design"[Mesh]
- "Surveys and Questionnaires"[Mesh]
- "Personality Inventory"[Mesh]
- "Test Anxiety Scale"[Mesh]
- "Health Care Surveys"[Mesh]
- "Nutrition Surveys"[Mesh]
- "Health Surveys"[Mesh]
- "Dental Health Surveys"[Mesh]
- "Diet Surveys"[Mesh]
- "Behavior Rating Scale"[Mesh]
- "Patient Health Questionnaire"[Mesh]
Below are example search strings incorporating the use of these MESH subject headings:
- "eating disorder" AND "Surveys and Questionnaires"[Mesh]
- depression AND "Patient Health Questionnaire"[Mesh]
- anxiety AND "Personality Inventory"[Mesh]
For additional information, see the following training videos:
- PubMed Online Training
Content: Elsevier’s science database covering computer science, health science, and social sciences. Contains peer-reviewed and open-access journal articles and book chapters.
Purpose: A great resource that covers foundational science to new and novel research.
Special Features: Covers theoretical and practical aspects of physical, life, health, and social sciences.
Searching in ScienceDirect
Use ScienceDirect to locate tests and measurements used in studies and published articles relevant to your topic. Add any of the following keywords to your search string:
For additional information, see the following video:
- ScienceDirect Quick Tutorial Video
Content: Citations and articles in multi-disciplines not found through a NavigatorSearch.
Purpose: Used to conduct topic searches as well as find additional resources that have cited a specific resource (citation network).
Searching in Web of Knowledge
Use Web of Knowledge to locate tests and measurements used in studies and published articles relevant to your topic. Add any of the following keywords to your search string:
For additional information, visit the following website:
- Web of Science Training Portal
Content: Global student dissertations and literature reviews.
Purpose: Use for foundational research, to locate test instruments and data, and more.
Special Features: Search by advisor (chair), degree, degree level, or department. Includes a read-aloud feature
The ProQuest Dissertations & Theses database (PQDT) is the world's most comprehensive collection of dissertations and theses. It is the database of record for graduate research, with over 2.3 million dissertations and theses included from around the world.
Content: National University & NCU student dissertations and literature reviews.
Special Features: Search by advisor (chair), degree, degree level, or department. Includes a read-aloud feature.
Searching in ProQuest Dissertations & Theses
Locate tests and measurements in ProQuest Dissertations & Theses by using the following strategies:
Search for related graduate and doctoral-level research that has already been conducted on your topic. Similar studies may have employed a relevant test or measurement.
- ProQuest Dissertations & Theses Quick Tutorial Video
Internet Search
Lastly, you might try searching for a test or measurement or information about them on the Internet. Google is an excellent search engine for finding information on test instruments. To find information about a particular test or measurement on Google, type the name of the test or measurement into the empty search field and place it in quotes:

Permissions
Unless your test instrument is commercially available (i.e., available for purchase), you will likely need to seek permission to use a test instrument in your dissertation. An exception may be instruments retrieved from the APA PsycTests database. The majority of tests within this database can be used without seeking additional permission. However, the instrument must explicitly state May use for Research/Teaching in the permissions field.
Also note that obtaining permission to use an instrument is not the same as obtaining permission to reproduce the instrument in its entirety in your dissertation appendix. It is important that you ask for separate permissions to do that.
First, you will need to identify who owns the copyright. The copyright holder is usually the author/creator of the work. Often, the author’s email address appears within the published journal article from which the instrument originated. If you need help tracking down the original article, please contact the Library.
If an email address is not readily available or seems to be outdated, you will need to search for the author’s contact information online. Try using quotation marks around the name or adding an associated institution to narrow your results. Again, if you need assistance with the step, the Library can recommend search techniques. However, the Library will not contact authors on your behalf.

Once you have located the contact information, prepare to introduce yourself and explain why are seeking permission. State clearly who you are, your institutional affiliation (e.g., Northcentral University), and the general nature of your thesis/dissertation research. Also discuss whether you are modifying the instrument, or if you are reproducing the instrument in your appendix. Typically, an email exchange is best, but some authors may prefer mail correspondence or a phone call. There are many sample permissions letters available online, including some examples linked below.
In some cases, authors transfer copyright to another entity, such as a journal publisher or an organization. Publishers often have website forms or letter templates that you can use to submit your request. See an example from Wiley here .
Remember, you will need to document permissions in your dissertation appendix. Make sure to save a copy of the correspondence and the agreement. Documentation allows you to demonstrate to your Chair and others that you have the legal right to use the owner's work.
In some cases, authors or publishers may either not respond to requests or refuse to grant permission to use their work. Therefore, it is important to select a few potential tests or measurements. The Library can certainly assist with searching for alternate test instruments.
For additional information about copyright and permission guidelines, see sections 12.14 - 12.18 in the APA Manual, 7th edition.
- Columbia University: Reprinting into a New Work Model Letter
- Copyright and Your Dissertation or Theses
- St Mary's University: Sample Permission Letter for a Thesis or Dissertation
- University of Pittsburgh: Sample Permission Letter for Dissertations
- University of Michigan: Obtaining Copyright Permissions
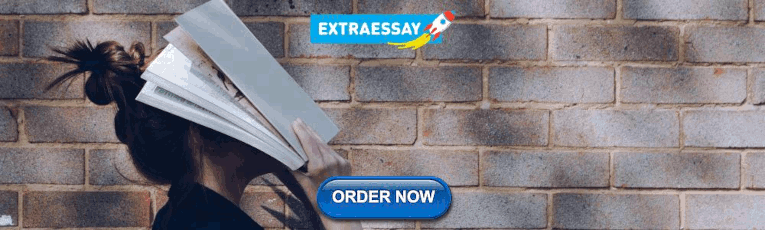
Selected Resources
- Open Access
- Tutorials & Guides
- Additional Resources
- Applied Measurement in Education
- Applied Psychological Measurement
- Assessment and Evaluation in Higher Education
- Assessment for Effective Intervention
- Assessment in Education: Principles, Policy& Practice
- Assessment Update
- Educational Assessment
- Educational Assessment, Evaluation andAccountability
- Educationaland Psychological Measurement
- Educational Evaluation and Policy Analysis
- Educational Measurement Issues and Practice
- European Journal of Psychological Assessment
- FairTest Examiner
- International Journal of Educational and Psychological Assessment
- International Journal of Selection and Assessment
- Journal of Educational Measurement
- Journal of Methods and Measurement in the Social Sciences
- Journalof Personality Assessment
- Journal of Psychoeducational Assessment
- Journal of Psychopathology and Behavioral Assessment
- Large-Scale Assessments in Education
- Measurement and Evaluation in Counseling and Development
- Practical Assessment, Research & Evaluation
- Psychological Assessment
- Psychological Test and Assessment Modeling
- Research & Practice in Assessment
- Social Science Research
- Sociological Methods & Research

Content: Books, reference works, journal articles, and instructional videos on research methods and design.
Purpose: Use to learn more about qualitative, quantitative, and mixed methods research.
Special Features: Includes a methods map, project planner, and "which stats" test
- Measuring Intimate Partner Violence Victimization and Perpetration: A Compendium of Assessment Tools Includes more than 20 scales for measuring the self-reported incidence and prevalence of Intimate Partner Violence victimization and perpetration.
- Measuring Violence-Related Attitudes, Beliefs, and Behaviors Among Youths: A Compendium of Assessment Tools Contains more than 100 measures designed to assess violence-related beliefs, behaviors, and influences, as well as to evaluate programs to prevent youth violence.
- Practitioner's Guide to Empirically Based Measures of School Behavior Contains descriptions of instruments in Chapter 6.
- Taking the Measure of Work: A Guide to Validated Scales for Organizational Research and Diagnosis Measures included in the book are those that can be completed as part of a questionnaire or survey, or administered as part of an interview.
- Alcohol & Drug Abuse Institute (ADAI) Screening and Assessment Instruments Database Provides instruments on alcohol and other drug use from all relevant disciplines. Some instruments are in the public domain and can be freely downloaded from the web.
- Association of Test Publishers Represents providers of tests and assessment tools and/or services related to assessment, selection, screening, certification, licensing, educational or clinical uses. Includes links to test publishers.
- Atlas of Integrated Behavioral Health Care Quality Measures Provides a list of existing measures relevant to integrated behavioral health care.
- British Psychological Society's Psychological Testing Center Provides test reviews in the areas of Counselling, Education, General Health, Life and Well-being, Occupational and Work, Psychology and more.
- Center for Equity and Excellence in Education Test Database Collection of abstracts and descriptions of almost 200 tests commonly used with Limited English Proficient students.
- Center for HIV Identification, Prevention and Treatment Services Directory of instruments including Abuse Assessment Screen, Depression (Thai Version), Emotional Social Support, and Quality of Life, HIV.
- Center for Outcome Measurement in Brain Injury Provides scales relating to rehabilitation, disability, cognitive functioning, life satisfaction, and more.
- Child Care and Early Education: Datasets, Instruments and Tools for Analysis Search for instruments by keyword or author, or browse by topic.
- Childhood Anxiety Screening Tool Instruments/Rating Scales Includes screening tool instruments and rating scales for supporting children and adolescents experiencing general anxiety disorder and/or post traumatic stress disorder.
- Compendium of Assessment and Research Tools Database that provides information on instruments that measure attributes associated with youth development programs.
- Directory of Tests with Links to Publishers Links will re-direct you to the publishers' sites for price and ordering information. Test topics include ability, achievement, neuropsychology, personality, psychopathology, and more.
- DMOZ Open Directory Project listing of tests and testing links.
- Ericae.net Online gateway to ERIC's resources on assessment and evaluation.
- Health Services and Sciences Research Resources Provides information about research datasets and instruments/indices that are used in Health Services Research, Behavioral and Social Sciences, and Public Health.
- Instrument Wizard Site will help you identify and learn more about screening, diagnostic, research, evaluation, and needs assessment instruments designed to measure substance use and related topics. Requires membership fee.
- International Personality Item Pool Provides access to measures of individual differences, all in the public domain.
- Mental Health Instruments in Non-English Languages Provides links to a range of scales, resources, research and other related information.
- National Information Center on Health Services Research and Health Care Technology Information about research datasets and instruments/indices employed in Health Services Research, Behavioral and Social Sciences and Public Health with links to PubMed.
- National Quality Measures Clearinghouse Provides information on specific evidence-based health care quality measures and measure sets.
- Online Evaluation Resource Directory Collection of sound education plans, reports, and instruments from past and current project evaluations in several content areas.
- Patient-Reported Outcome and Quality of Life Instruments Database (PROQOLID) Offers free, but limited public access to their database. For each instrument in the database, you will find 14 categories of basic information (e.g., author, objective, mode of administration, original language, existing translations, pathology, number of items, etc.). Requires registration.
- Pearson Assessments Commercial site that offers assessments for clinical and psychological use. Tests are clearly explained for functionality and implementation. Tests can be purchased from this site.
- Positive Psychology Questionnaires Information about the positive psychology questionnaires, some of which can be downloaded from the site.
- Psychological Tests for Student Use List of copyrighted tests that students have permission to use (and so don't have to go through the permission inquiry process), from York University.
- Psychosocial Measures for Review (PhenX) List of psychosocial measures with background information, full text of the measure, and scoring instructions.
- RAND Health - Surveys & Tools Surveys include topics such as Aging and Health, Mental Health, & Quality of Life. All of the surveys from RAND Health are public documents, available without charge.
- Registry of Scales and Measures Psychological tests, scales, questionnaires, and checklists can be searched by several parameters, including author, title, year of publication, and topic, as well as by scale and item characteristics.
- Research Instruments Developed, Adapted or Used by the Stanford Patient Education Research Center Includes scales for research subjects with chronic diseases in English and Spanish. You may use any of these scales at no cost without permission.
- SDSU Test Finder San Diego State University librarians have developed a searchable database of tests, instruments, rating scales, and measures available in books.
- SDSU Test Finder for Journal Articles Another tool from San Diego State University allows you to search for complete psychosocial tests, instruments, rating scales, and measures found in the journal literature.
- Self-Report Measures From the University of Miami Department of Psychology, a listing of scales made available for use in research and teaching applications. All are available without charge and without any need for permission.
- Social-Personality Psychology Questionnaire Instrument Compendium (QIC) Directory of public domain tests.
- Statistics Solutions Directory of Survey Instruments Each survey instrument's page includes a description, references, and a link to purchase the instrument directly from the author. Individual authorization from the author is required in order to administer any of the surveys.
- Substance Use Screening & Assessment Instruments Database Instruments used for screening and assessment of substance use and substance use disorders. Some instruments are in the public domain and can be freely downloaded from the web; others can only be obtained from the copyright holder.
- Test Reviews Online Buros Center for Testing site provides reviews for over 4,000 commercially available tests. Over 2,000 of the tests have been reviewed in Mental Measurements. Reviews require purchase.
- Tests and Measures in the Social Sciences An index to 139 print compilations, web sites and other resources for test instruments with nearly 14,000 tests and measurements compiled from 1967 - 2014.
- USF Test & Measures Collection Indexes instruments, questionnaires, surveys, or tests that are contained in various books held in libraries. Click "full record" for the test, you will find annotated details about the instrument.
- Index to Tests in Journal Articles San Diego State University
- Searching for Test Instruments Lister Hill Library of the Health Sciences
- Tests and Measures in the Social Sciences University of Texas at Arlington
- APA: FAQ/Finding Information About Psychological Tests
- ERIC: Questions To Ask When Evaluating Tests
- APA PsycTests on EBSCOhost
Was this resource helpful?
- << Previous: Research Methodology & Design
- Next: Organizing Research & Citations >>
- Last Updated: May 1, 2024 12:51 PM
- URL: https://resources.nu.edu/researchprocess

© Copyright 2024 National University. All Rights Reserved.
Privacy Policy | Consumer Information
Thank you for visiting nature.com. You are using a browser version with limited support for CSS. To obtain the best experience, we recommend you use a more up to date browser (or turn off compatibility mode in Internet Explorer). In the meantime, to ensure continued support, we are displaying the site without styles and JavaScript.
- View all journals
- My Account Login
- Explore content
- About the journal
- Publish with us
- Sign up for alerts
- Data Descriptor
- Open access
- Published: 03 May 2024
A dataset for measuring the impact of research data and their curation
- Libby Hemphill ORCID: orcid.org/0000-0002-3793-7281 1 , 2 ,
- Andrea Thomer 3 ,
- Sara Lafia 1 ,
- Lizhou Fan 2 ,
- David Bleckley ORCID: orcid.org/0000-0001-7715-4348 1 &
- Elizabeth Moss 1
Scientific Data volume 11 , Article number: 442 ( 2024 ) Cite this article
576 Accesses
8 Altmetric
Metrics details
- Research data
- Social sciences
Science funders, publishers, and data archives make decisions about how to responsibly allocate resources to maximize the reuse potential of research data. This paper introduces a dataset developed to measure the impact of archival and data curation decisions on data reuse. The dataset describes 10,605 social science research datasets, their curation histories, and reuse contexts in 94,755 publications that cover 59 years from 1963 to 2022. The dataset was constructed from study-level metadata, citing publications, and curation records available through the Inter-university Consortium for Political and Social Research (ICPSR) at the University of Michigan. The dataset includes information about study-level attributes (e.g., PIs, funders, subject terms); usage statistics (e.g., downloads, citations); archiving decisions (e.g., curation activities, data transformations); and bibliometric attributes (e.g., journals, authors) for citing publications. This dataset provides information on factors that contribute to long-term data reuse, which can inform the design of effective evidence-based recommendations to support high-impact research data curation decisions.
Similar content being viewed by others
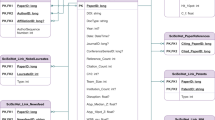
SciSciNet: A large-scale open data lake for the science of science research
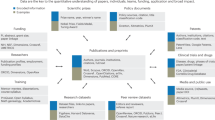
Data, measurement and empirical methods in the science of science
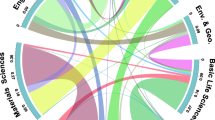
Interdisciplinarity revisited: evidence for research impact and dynamism
Background & summary.
Recent policy changes in funding agencies and academic journals have increased data sharing among researchers and between researchers and the public. Data sharing advances science and provides the transparency necessary for evaluating, replicating, and verifying results. However, many data-sharing policies do not explain what constitutes an appropriate dataset for archiving or how to determine the value of datasets to secondary users 1 , 2 , 3 . Questions about how to allocate data-sharing resources efficiently and responsibly have gone unanswered 4 , 5 , 6 . For instance, data-sharing policies recognize that not all data should be curated and preserved, but they do not articulate metrics or guidelines for determining what data are most worthy of investment.
Despite the potential for innovation and advancement that data sharing holds, the best strategies to prioritize datasets for preparation and archiving are often unclear. Some datasets are likely to have more downstream potential than others, and data curation policies and workflows should prioritize high-value data instead of being one-size-fits-all. Though prior research in library and information science has shown that the “analytic potential” of a dataset is key to its reuse value 7 , work is needed to implement conceptual data reuse frameworks 8 , 9 , 10 , 11 , 12 , 13 , 14 . In addition, publishers and data archives need guidance to develop metrics and evaluation strategies to assess the impact of datasets.
Several existing resources have been compiled to study the relationship between the reuse of scholarly products, such as datasets (Table 1 ); however, none of these resources include explicit information on how curation processes are applied to data to increase their value, maximize their accessibility, and ensure their long-term preservation. The CCex (Curation Costs Exchange) provides models of curation services along with cost-related datasets shared by contributors but does not make explicit connections between them or include reuse information 15 . Analyses on platforms such as DataCite 16 have focused on metadata completeness and record usage, but have not included related curation-level information. Analyses of GenBank 17 and FigShare 18 , 19 citation networks do not include curation information. Related studies of Github repository reuse 20 and Softcite software citation 21 reveal significant factors that impact the reuse of secondary research products but do not focus on research data. RD-Switchboard 22 and DSKG 23 are scholarly knowledge graphs linking research data to articles, patents, and grants, but largely omit social science research data and do not include curation-level factors. To our knowledge, other studies of curation work in organizations similar to ICPSR – such as GESIS 24 , Dataverse 25 , and DANS 26 – have not made their underlying data available for analysis.
This paper describes a dataset 27 compiled for the MICA project (Measuring the Impact of Curation Actions) led by investigators at ICPSR, a large social science data archive at the University of Michigan. The dataset was originally developed to study the impacts of data curation and archiving on data reuse. The MICA dataset has supported several previous publications investigating the intensity of data curation actions 28 , the relationship between data curation actions and data reuse 29 , and the structures of research communities in a data citation network 30 . Collectively, these studies help explain the return on various types of curatorial investments. The dataset that we introduce in this paper, which we refer to as the MICA dataset, has the potential to address research questions in the areas of science (e.g., knowledge production), library and information science (e.g., scholarly communication), and data archiving (e.g., reproducible workflows).
We constructed the MICA dataset 27 using records available at ICPSR, a large social science data archive at the University of Michigan. Data set creation involved: collecting and enriching metadata for articles indexed in the ICPSR Bibliography of Data-related Literature against the Dimensions AI bibliometric database; gathering usage statistics for studies from ICPSR’s administrative database; processing data curation work logs from ICPSR’s project tracking platform, Jira; and linking data in social science studies and series to citing analysis papers (Fig. 1 ).
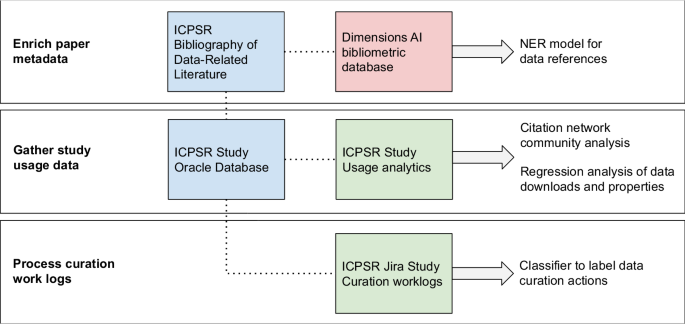
Steps to prepare MICA dataset for analysis - external sources are red, primary internal sources are blue, and internal linked sources are green.
Enrich paper metadata
The ICPSR Bibliography of Data-related Literature is a growing database of literature in which data from ICPSR studies have been used. Its creation was funded by the National Science Foundation (Award 9977984), and for the past 20 years it has been supported by ICPSR membership and multiple US federally-funded and foundation-funded topical archives at ICPSR. The Bibliography was originally launched in the year 2000 to aid in data discovery by providing a searchable database linking publications to the study data used in them. The Bibliography collects the universe of output based on the data shared in each study through, which is made available through each ICPSR study’s webpage. The Bibliography contains both peer-reviewed and grey literature, which provides evidence for measuring the impact of research data. For an item to be included in the ICPSR Bibliography, it must contain an analysis of data archived by ICPSR or contain a discussion or critique of the data collection process, study design, or methodology 31 . The Bibliography is manually curated by a team of librarians and information specialists at ICPSR who enter and validate entries. Some publications are supplied to the Bibliography by data depositors, and some citations are submitted to the Bibliography by authors who abide by ICPSR’s terms of use requiring them to submit citations to works in which they analyzed data retrieved from ICPSR. Most of the Bibliography is populated by Bibliography team members, who create custom queries for ICPSR studies performed across numerous sources, including Google Scholar, ProQuest, SSRN, and others. Each record in the Bibliography is one publication that has used one or more ICPSR studies. The version we used was captured on 2021-11-16 and included 94,755 publications.
To expand the coverage of the ICPSR Bibliography, we searched exhaustively for all ICPSR study names, unique numbers assigned to ICPSR studies, and DOIs 32 using a full-text index available through the Dimensions AI database 33 . We accessed Dimensions through a license agreement with the University of Michigan. ICPSR Bibliography librarians and information specialists manually reviewed and validated new entries that matched one or more search criteria. We then used Dimensions to gather enriched metadata and full-text links for items in the Bibliography with DOIs. We matched 43% of the items in the Bibliography to enriched Dimensions metadata including abstracts, field of research codes, concepts, and authors’ institutional information; we also obtained links to full text for 16% of Bibliography items. Based on licensing agreements, we included Dimensions identifiers and links to full text so that users with valid publisher and database access can construct an enriched publication dataset.
Gather study usage data
ICPSR maintains a relational administrative database, DBInfo, that organizes study-level metadata and information on data reuse across separate tables. Studies at ICPSR consist of one or more files collected at a single time or for a single purpose; studies in which the same variables are observed over time are grouped into series. Each study at ICPSR is assigned a DOI, and its metadata are stored in DBInfo. Study metadata follows the Data Documentation Initiative (DDI) Codebook 2.5 standard. DDI elements included in our dataset are title, ICPSR study identification number, DOI, authoring entities, description (abstract), funding agencies, subject terms assigned to the study during curation, and geographic coverage. We also created variables based on DDI elements: total variable count, the presence of survey question text in the metadata, the number of author entities, and whether an author entity was an institution. We gathered metadata for ICPSR’s 10,605 unrestricted public-use studies available as of 2021-11-16 ( https://www.icpsr.umich.edu/web/pages/membership/or/metadata/oai.html ).
To link study usage data with study-level metadata records, we joined study metadata from DBinfo on study usage information, which included total study downloads (data and documentation), individual data file downloads, and cumulative citations from the ICPSR Bibliography. We also gathered descriptive metadata for each study and its variables, which allowed us to summarize and append recoded fields onto the study-level metadata such as curation level, number and type of principle investigators, total variable count, and binary variables indicating whether the study data were made available for online analysis, whether survey question text was made searchable online, and whether the study variables were indexed for search. These characteristics describe aspects of the discoverability of the data to compare with other characteristics of the study. We used the study and series numbers included in the ICPSR Bibliography as unique identifiers to link papers to metadata and analyze the community structure of dataset co-citations in the ICPSR Bibliography 32 .
Process curation work logs
Researchers deposit data at ICPSR for curation and long-term preservation. Between 2016 and 2020, more than 3,000 research studies were deposited with ICPSR. Since 2017, ICPSR has organized curation work into a central unit that provides varied levels of curation that vary in the intensity and complexity of data enhancement that they provide. While the levels of curation are standardized as to effort (level one = less effort, level three = most effort), the specific curatorial actions undertaken for each dataset vary. The specific curation actions are captured in Jira, a work tracking program, which data curators at ICPSR use to collaborate and communicate their progress through tickets. We obtained access to a corpus of 669 completed Jira tickets corresponding to the curation of 566 unique studies between February 2017 and December 2019 28 .
To process the tickets, we focused only on their work log portions, which contained free text descriptions of work that data curators had performed on a deposited study, along with the curators’ identifiers, and timestamps. To protect the confidentiality of the data curators and the processing steps they performed, we collaborated with ICPSR’s curation unit to propose a classification scheme, which we used to train a Naive Bayes classifier and label curation actions in each work log sentence. The eight curation action labels we proposed 28 were: (1) initial review and planning, (2) data transformation, (3) metadata, (4) documentation, (5) quality checks, (6) communication, (7) other, and (8) non-curation work. We note that these categories of curation work are very specific to the curatorial processes and types of data stored at ICPSR, and may not match the curation activities at other repositories. After applying the classifier to the work log sentences, we obtained summary-level curation actions for a subset of all ICPSR studies (5%), along with the total number of hours spent on data curation for each study, and the proportion of time associated with each action during curation.
Data Records
The MICA dataset 27 connects records for each of ICPSR’s archived research studies to the research publications that use them and related curation activities available for a subset of studies (Fig. 2 ). Each of the three tables published in the dataset is available as a study archived at ICPSR. The data tables are distributed as statistical files available for use in SAS, SPSS, Stata, and R as well as delimited and ASCII text files. The dataset is organized around studies and papers as primary entities. The studies table lists ICPSR studies, their metadata attributes, and usage information; the papers table was constructed using the ICPSR Bibliography and Dimensions database; and the curation logs table summarizes the data curation steps performed on a subset of ICPSR studies.
Studies (“ICPSR_STUDIES”): 10,605 social science research datasets available through ICPSR up to 2021-11-16 with variables for ICPSR study number, digital object identifier, study name, series number, series title, authoring entities, full-text description, release date, funding agency, geographic coverage, subject terms, topical archive, curation level, single principal investigator (PI), institutional PI, the total number of PIs, total variables in data files, question text availability, study variable indexing, level of restriction, total unique users downloading study data files and codebooks, total unique users downloading data only, and total unique papers citing data through November 2021. Studies map to the papers and curation logs table through ICPSR study numbers as “STUDY”. However, not every study in this table will have records in the papers and curation logs tables.
Papers (“ICPSR_PAPERS”): 94,755 publications collected from 2000-08-11 to 2021-11-16 in the ICPSR Bibliography and enriched with metadata from the Dimensions database with variables for paper number, identifier, title, authors, publication venue, item type, publication date, input date, ICPSR series numbers used in the paper, ICPSR study numbers used in the paper, the Dimension identifier, and the Dimensions link to the publication’s full text. Papers map to the studies table through ICPSR study numbers in the “STUDY_NUMS” field. Each record represents a single publication, and because a researcher can use multiple datasets when creating a publication, each record may list multiple studies or series.
Curation logs (“ICPSR_CURATION_LOGS”): 649 curation logs for 563 ICPSR studies (although most studies in the subset had one curation log, some studies were associated with multiple logs, with a maximum of 10) curated between February 2017 and December 2019 with variables for study number, action labels assigned to work description sentences using a classifier trained on ICPSR curation logs, hours of work associated with a single log entry, and total hours of work logged for the curation ticket. Curation logs map to the study and paper tables through ICPSR study numbers as “STUDY”. Each record represents a single logged action, and future users may wish to aggregate actions to the study level before joining tables.
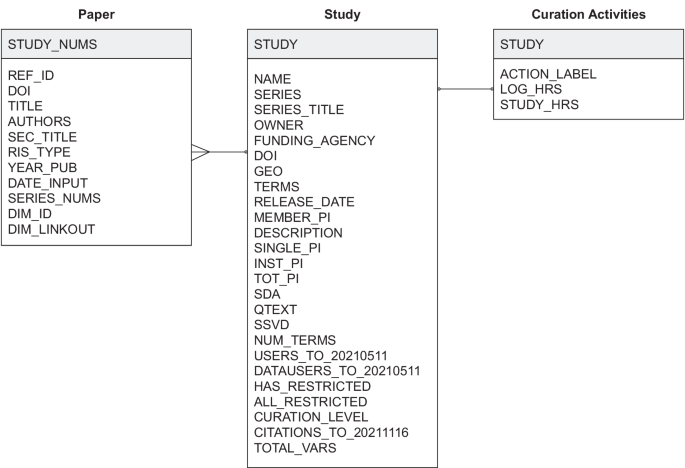
Entity-relation diagram.
Technical Validation
We report on the reliability of the dataset’s metadata in the following subsections. To support future reuse of the dataset, curation services provided through ICPSR improved data quality by checking for missing values, adding variable labels, and creating a codebook.
All 10,605 studies available through ICPSR have a DOI and a full-text description summarizing what the study is about, the purpose of the study, the main topics covered, and the questions the PIs attempted to answer when they conducted the study. Personal names (i.e., principal investigators) and organizational names (i.e., funding agencies) are standardized against an authority list maintained by ICPSR; geographic names and subject terms are also standardized and hierarchically indexed in the ICPSR Thesaurus 34 . Many of ICPSR’s studies (63%) are in a series and are distributed through the ICPSR General Archive (56%), a non-topical archive that accepts any social or behavioral science data. While study data have been available through ICPSR since 1962, the earliest digital release date recorded for a study was 1984-03-18, when ICPSR’s database was first employed, and the most recent date is 2021-10-28 when the dataset was collected.
Curation level information was recorded starting in 2017 and is available for 1,125 studies (11%); approximately 80% of studies with assigned curation levels received curation services, equally distributed between Levels 1 (least intensive), 2 (moderately intensive), and 3 (most intensive) (Fig. 3 ). Detailed descriptions of ICPSR’s curation levels are available online 35 . Additional metadata are available for a subset of 421 studies (4%), including information about whether the study has a single PI, an institutional PI, the total number of PIs involved, total variables recorded is available for online analysis, has searchable question text, has variables that are indexed for search, contains one or more restricted files, and whether the study is completely restricted. We provided additional metadata for this subset of ICPSR studies because they were released within the past five years and detailed curation and usage information were available for them. Usage statistics including total downloads and data file downloads are available for this subset of studies as well; citation statistics are available for 8,030 studies (76%). Most ICPSR studies have fewer than 500 users, as indicated by total downloads, or citations (Fig. 4 ).
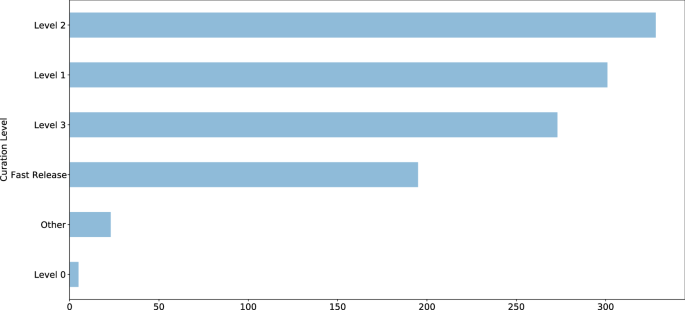
ICPSR study curation levels.
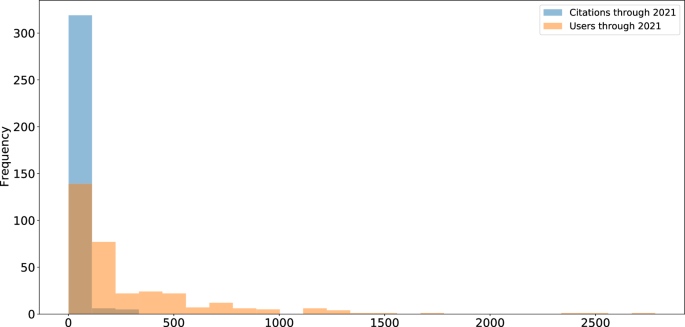
ICPSR study usage.
A subset of 43,102 publications (45%) available in the ICPSR Bibliography had a DOI. Author metadata were entered as free text, meaning that variations may exist and require additional normalization and pre-processing prior to analysis. While author information is standardized for each publication, individual names may appear in different sort orders (e.g., “Earls, Felton J.” and “Stephen W. Raudenbush”). Most of the items in the ICPSR Bibliography as of 2021-11-16 were journal articles (59%), reports (14%), conference presentations (9%), or theses (8%) (Fig. 5 ). The number of publications collected in the Bibliography has increased each decade since the inception of ICPSR in 1962 (Fig. 6 ). Most ICPSR studies (76%) have one or more citations in a publication.
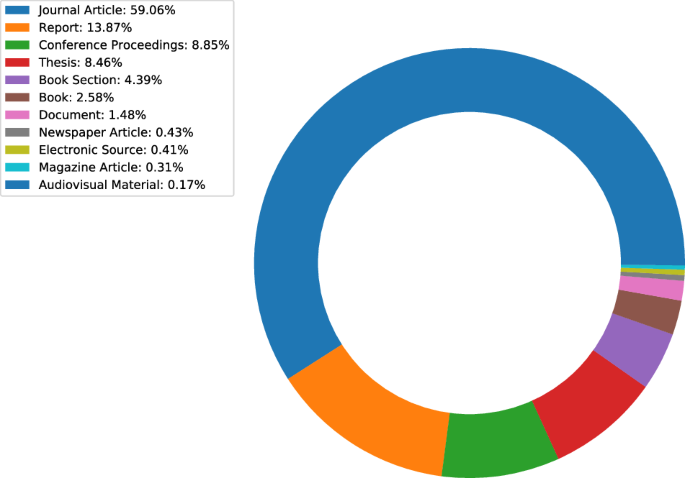
ICPSR Bibliography citation types.
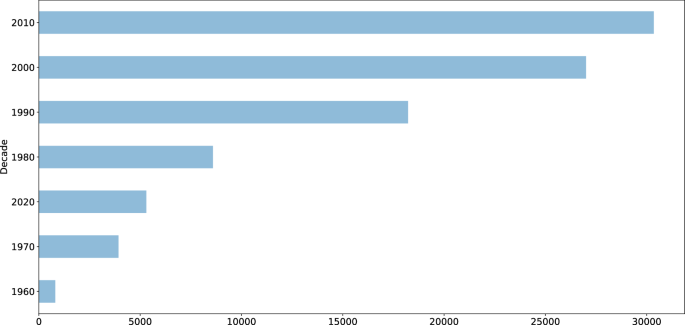
ICPSR citations by decade.
Usage Notes
The dataset consists of three tables that can be joined using the “STUDY” key as shown in Fig. 2 . The “ICPSR_PAPERS” table contains one row per paper with one or more cited studies in the “STUDY_NUMS” column. We manipulated and analyzed the tables as CSV files with the Pandas library 36 in Python and the Tidyverse packages 37 in R.
The present MICA dataset can be used independently to study the relationship between curation decisions and data reuse. Evidence of reuse for specific studies is available in several forms: usage information, including downloads and citation counts; and citation contexts within papers that cite data. Analysis may also be performed on the citation network formed between datasets and papers that use them. Finally, curation actions can be associated with properties of studies and usage histories.
This dataset has several limitations of which users should be aware. First, Jira tickets can only be used to represent the intensiveness of curation for activities undertaken since 2017, when ICPSR started using both Curation Levels and Jira. Studies published before 2017 were all curated, but documentation of the extent of that curation was not standardized and therefore could not be included in these analyses. Second, the measure of publications relies upon the authors’ clarity of data citation and the ICPSR Bibliography staff’s ability to discover citations with varying formality and clarity. Thus, there is always a chance that some secondary-data-citing publications have been left out of the bibliography. Finally, there may be some cases in which a paper in the ICSPSR bibliography did not actually obtain data from ICPSR. For example, PIs have often written about or even distributed their data prior to their archival in ICSPR. Therefore, those publications would not have cited ICPSR but they are still collected in the Bibliography as being directly related to the data that were eventually deposited at ICPSR.
In summary, the MICA dataset contains relationships between two main types of entities – papers and studies – which can be mined. The tables in the MICA dataset have supported network analysis (community structure and clique detection) 30 ; natural language processing (NER for dataset reference detection) 32 ; visualizing citation networks (to search for datasets) 38 ; and regression analysis (on curation decisions and data downloads) 29 . The data are currently being used to develop research metrics and recommendation systems for research data. Given that DOIs are provided for ICPSR studies and articles in the ICPSR Bibliography, the MICA dataset can also be used with other bibliometric databases, including DataCite, Crossref, OpenAlex, and related indexes. Subscription-based services, such as Dimensions AI, are also compatible with the MICA dataset. In some cases, these services provide abstracts or full text for papers from which data citation contexts can be extracted for semantic content analysis.
Code availability
The code 27 used to produce the MICA project dataset is available on GitHub at https://github.com/ICPSR/mica-data-descriptor and through Zenodo with the identifier https://doi.org/10.5281/zenodo.8432666 . Data manipulation and pre-processing were performed in Python. Data curation for distribution was performed in SPSS.
He, L. & Han, Z. Do usage counts of scientific data make sense? An investigation of the Dryad repository. Library Hi Tech 35 , 332–342 (2017).
Article Google Scholar
Brickley, D., Burgess, M. & Noy, N. Google dataset search: Building a search engine for datasets in an open web ecosystem. In The World Wide Web Conference - WWW ‘19 , 1365–1375 (ACM Press, San Francisco, CA, USA, 2019).
Buneman, P., Dosso, D., Lissandrini, M. & Silvello, G. Data citation and the citation graph. Quantitative Science Studies 2 , 1399–1422 (2022).
Chao, T. C. Disciplinary reach: Investigating the impact of dataset reuse in the earth sciences. Proceedings of the American Society for Information Science and Technology 48 , 1–8 (2011).
Article ADS Google Scholar
Parr, C. et al . A discussion of value metrics for data repositories in earth and environmental sciences. Data Science Journal 18 , 58 (2019).
Eschenfelder, K. R., Shankar, K. & Downey, G. The financial maintenance of social science data archives: Four case studies of long–term infrastructure work. J. Assoc. Inf. Sci. Technol. 73 , 1723–1740 (2022).
Palmer, C. L., Weber, N. M. & Cragin, M. H. The analytic potential of scientific data: Understanding re-use value. Proceedings of the American Society for Information Science and Technology 48 , 1–10 (2011).
Zimmerman, A. S. New knowledge from old data: The role of standards in the sharing and reuse of ecological data. Sci. Technol. Human Values 33 , 631–652 (2008).
Cragin, M. H., Palmer, C. L., Carlson, J. R. & Witt, M. Data sharing, small science and institutional repositories. Philosophical Transactions of the Royal Society A: Mathematical, Physical and Engineering Sciences 368 , 4023–4038 (2010).
Article ADS CAS Google Scholar
Fear, K. M. Measuring and Anticipating the Impact of Data Reuse . Ph.D. thesis, University of Michigan (2013).
Borgman, C. L., Van de Sompel, H., Scharnhorst, A., van den Berg, H. & Treloar, A. Who uses the digital data archive? An exploratory study of DANS. Proceedings of the Association for Information Science and Technology 52 , 1–4 (2015).
Pasquetto, I. V., Borgman, C. L. & Wofford, M. F. Uses and reuses of scientific data: The data creators’ advantage. Harvard Data Science Review 1 (2019).
Gregory, K., Groth, P., Scharnhorst, A. & Wyatt, S. Lost or found? Discovering data needed for research. Harvard Data Science Review (2020).
York, J. Seeking equilibrium in data reuse: A study of knowledge satisficing . Ph.D. thesis, University of Michigan (2022).
Kilbride, W. & Norris, S. Collaborating to clarify the cost of curation. New Review of Information Networking 19 , 44–48 (2014).
Robinson-Garcia, N., Mongeon, P., Jeng, W. & Costas, R. DataCite as a novel bibliometric source: Coverage, strengths and limitations. Journal of Informetrics 11 , 841–854 (2017).
Qin, J., Hemsley, J. & Bratt, S. E. The structural shift and collaboration capacity in GenBank networks: A longitudinal study. Quantitative Science Studies 3 , 174–193 (2022).
Article PubMed PubMed Central Google Scholar
Acuna, D. E., Yi, Z., Liang, L. & Zhuang, H. Predicting the usage of scientific datasets based on article, author, institution, and journal bibliometrics. In Smits, M. (ed.) Information for a Better World: Shaping the Global Future. iConference 2022 ., 42–52 (Springer International Publishing, Cham, 2022).
Zeng, T., Wu, L., Bratt, S. & Acuna, D. E. Assigning credit to scientific datasets using article citation networks. Journal of Informetrics 14 , 101013 (2020).
Koesten, L., Vougiouklis, P., Simperl, E. & Groth, P. Dataset reuse: Toward translating principles to practice. Patterns 1 , 100136 (2020).
Du, C., Cohoon, J., Lopez, P. & Howison, J. Softcite dataset: A dataset of software mentions in biomedical and economic research publications. J. Assoc. Inf. Sci. Technol. 72 , 870–884 (2021).
Aryani, A. et al . A research graph dataset for connecting research data repositories using RD-Switchboard. Sci Data 5 , 180099 (2018).
Färber, M. & Lamprecht, D. The data set knowledge graph: Creating a linked open data source for data sets. Quantitative Science Studies 2 , 1324–1355 (2021).
Perry, A. & Netscher, S. Measuring the time spent on data curation. Journal of Documentation 78 , 282–304 (2022).
Trisovic, A. et al . Advancing computational reproducibility in the Dataverse data repository platform. In Proceedings of the 3rd International Workshop on Practical Reproducible Evaluation of Computer Systems , P-RECS ‘20, 15–20, https://doi.org/10.1145/3391800.3398173 (Association for Computing Machinery, New York, NY, USA, 2020).
Borgman, C. L., Scharnhorst, A. & Golshan, M. S. Digital data archives as knowledge infrastructures: Mediating data sharing and reuse. Journal of the Association for Information Science and Technology 70 , 888–904, https://doi.org/10.1002/asi.24172 (2019).
Lafia, S. et al . MICA Data Descriptor. Zenodo https://doi.org/10.5281/zenodo.8432666 (2023).
Lafia, S., Thomer, A., Bleckley, D., Akmon, D. & Hemphill, L. Leveraging machine learning to detect data curation activities. In 2021 IEEE 17th International Conference on eScience (eScience) , 149–158, https://doi.org/10.1109/eScience51609.2021.00025 (2021).
Hemphill, L., Pienta, A., Lafia, S., Akmon, D. & Bleckley, D. How do properties of data, their curation, and their funding relate to reuse? J. Assoc. Inf. Sci. Technol. 73 , 1432–44, https://doi.org/10.1002/asi.24646 (2021).
Lafia, S., Fan, L., Thomer, A. & Hemphill, L. Subdivisions and crossroads: Identifying hidden community structures in a data archive’s citation network. Quantitative Science Studies 3 , 694–714, https://doi.org/10.1162/qss_a_00209 (2022).
ICPSR. ICPSR Bibliography of Data-related Literature: Collection Criteria. https://www.icpsr.umich.edu/web/pages/ICPSR/citations/collection-criteria.html (2023).
Lafia, S., Fan, L. & Hemphill, L. A natural language processing pipeline for detecting informal data references in academic literature. Proc. Assoc. Inf. Sci. Technol. 59 , 169–178, https://doi.org/10.1002/pra2.614 (2022).
Hook, D. W., Porter, S. J. & Herzog, C. Dimensions: Building context for search and evaluation. Frontiers in Research Metrics and Analytics 3 , 23, https://doi.org/10.3389/frma.2018.00023 (2018).
https://www.icpsr.umich.edu/web/ICPSR/thesaurus (2002). ICPSR. ICPSR Thesaurus.
https://www.icpsr.umich.edu/files/datamanagement/icpsr-curation-levels.pdf (2020). ICPSR. ICPSR Curation Levels.
McKinney, W. Data Structures for Statistical Computing in Python. In van der Walt, S. & Millman, J. (eds.) Proceedings of the 9th Python in Science Conference , 56–61 (2010).
Wickham, H. et al . Welcome to the Tidyverse. Journal of Open Source Software 4 , 1686 (2019).
Fan, L., Lafia, S., Li, L., Yang, F. & Hemphill, L. DataChat: Prototyping a conversational agent for dataset search and visualization. Proc. Assoc. Inf. Sci. Technol. 60 , 586–591 (2023).
Download references
Acknowledgements
We thank the ICPSR Bibliography staff, the ICPSR Data Curation Unit, and the ICPSR Data Stewardship Committee for their support of this research. This material is based upon work supported by the National Science Foundation under grant 1930645. This project was made possible in part by the Institute of Museum and Library Services LG-37-19-0134-19.
Author information
Authors and affiliations.
Inter-university Consortium for Political and Social Research, University of Michigan, Ann Arbor, MI, 48104, USA
Libby Hemphill, Sara Lafia, David Bleckley & Elizabeth Moss
School of Information, University of Michigan, Ann Arbor, MI, 48104, USA
Libby Hemphill & Lizhou Fan
School of Information, University of Arizona, Tucson, AZ, 85721, USA
Andrea Thomer
You can also search for this author in PubMed Google Scholar
Contributions
L.H. and A.T. conceptualized the study design, D.B., E.M., and S.L. prepared the data, S.L., L.F., and L.H. analyzed the data, and D.B. validated the data. All authors reviewed and edited the manuscript.
Corresponding author
Correspondence to Libby Hemphill .
Ethics declarations
Competing interests.
The authors declare no competing interests.
Additional information
Publisher’s note Springer Nature remains neutral with regard to jurisdictional claims in published maps and institutional affiliations.
Rights and permissions
Open Access This article is licensed under a Creative Commons Attribution 4.0 International License, which permits use, sharing, adaptation, distribution and reproduction in any medium or format, as long as you give appropriate credit to the original author(s) and the source, provide a link to the Creative Commons licence, and indicate if changes were made. The images or other third party material in this article are included in the article’s Creative Commons licence, unless indicated otherwise in a credit line to the material. If material is not included in the article’s Creative Commons licence and your intended use is not permitted by statutory regulation or exceeds the permitted use, you will need to obtain permission directly from the copyright holder. To view a copy of this licence, visit http://creativecommons.org/licenses/by/4.0/ .
Reprints and permissions
About this article
Cite this article.
Hemphill, L., Thomer, A., Lafia, S. et al. A dataset for measuring the impact of research data and their curation. Sci Data 11 , 442 (2024). https://doi.org/10.1038/s41597-024-03303-2
Download citation
Received : 16 November 2023
Accepted : 24 April 2024
Published : 03 May 2024
DOI : https://doi.org/10.1038/s41597-024-03303-2
Share this article
Anyone you share the following link with will be able to read this content:
Sorry, a shareable link is not currently available for this article.
Provided by the Springer Nature SharedIt content-sharing initiative
Quick links
- Explore articles by subject
- Guide to authors
- Editorial policies
Sign up for the Nature Briefing newsletter — what matters in science, free to your inbox daily.

- Skip to main content
- Keyboard shortcuts for audio player
Scientists at Berkeley develope a tool to help cities measure carbon emissions
Kevin Stark
Scientists at U.C. Berkeley are using a network of C02 sensors to more accurately monitor emissions. It's a model that is being used in some cities, and could eventually become a national program.
Copyright © 2024 NPR. All rights reserved. Visit our website terms of use and permissions pages at www.npr.org for further information.
NPR transcripts are created on a rush deadline by an NPR contractor. This text may not be in its final form and may be updated or revised in the future. Accuracy and availability may vary. The authoritative record of NPR’s programming is the audio record.
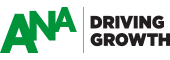
Your company may already be a member. View our member list to find out, or create a new account .
Forgot Password?
Content Library
You can search our content library for case studies, research, industry insights, and more.
You can search our website for events, press releases, blog posts, and more.
Pursuing Equitability in Representation of and Measurement for Women
May 3, 2024
A panel session held during the 2024 ANA/SeeHer Gender Equality Conference convened experts to speak on the perils and promise of pursuing equitability for women in media representation and in the ways in which they are measured as an audience.
This Is an ANA Member Exclusive
Access to this item is reserved for ANA members only.
Already have an account? The industry's best insights and resources await:
No Account?
Use your business email address to create your free account ; if you're a member through your company, we'll know.
Members can access their benefits as soon as they sign up and log in.
Not a Member?
You can still create a free account to access the latest from our online publication, ANA Magazine , receive content and special event offers through our newsletters, get breaking industry updates, and so much more.
The content you're trying to see is available to:
- ANA Client-Side Marketer Tier Members
- Platinum Tier Members
- Gold Tier Members
- Silver Tier Members
- Individual Members
Discover everything the ANA can do to help drive growth for your organization. Connect with our membership team.
Research on internal quality testing method of dry longan based on terahertz imaging detection technology
- Original Paper
- Published: 11 May 2024
Cite this article
- Jun Hu ORCID: orcid.org/0000-0003-0027-7993 1 ,
- Hao Wang 1 ,
- Yongqi Zhou 1 ,
- Shimin Yang 1 ,
- Haohao Lv 1 &
- Liang Yang 1
Longan is a kind of nut with rich nutritional value and homologous function of medicine and food. The quality of longan directly affects its curative effect, and its fullness is the key index to evaluate its quality. However, the internal information of longan cannot be obtained from the outside. Therefore, rapid non-destructive testing of internal quality of dry longan is of great significance. In this paper, rapid non-destructive testing of longan internal fullness based on terahertz transmission imaging technology was carried out. This study takes longan as the research object. Firstly, the terahertz transmission images of longans with different fullness were collected, and the terahertz spectral signals of different regions of interest were extracted for analysis. Then, three qualitative discriminant models, support vector machine (SVM), Random forest (RF) and linear discriminant analysis (LDA), were established to explore the optimal discriminant model and realize the distinction of different regional categories of longan. Finally, the collected longan terahertz transmission image is processed, and the number of white pixels in the connected domain is calculated by using Otsu threshold segmentation and image inversion. The fullness of longan can be achieved by calculating the ratio of core and pulp to the pixel of the shell. The LDA discriminant model had the best prediction effect. It could not only identify the spectral data of background region, shell region, core region, but also reach 98.57% accuracy for the spectral data of pulp region. The maximum error between the measured fullness and the actual fullness of the terahertz image processed by Otsu threshold segmentation is less than 3.11%. Terahertz imaging technique can realize rapid non-destructive detection of longan fullness and recognition of different regions. This study provides an effective scheme for selecting the quality of longan.
Graphical abstract
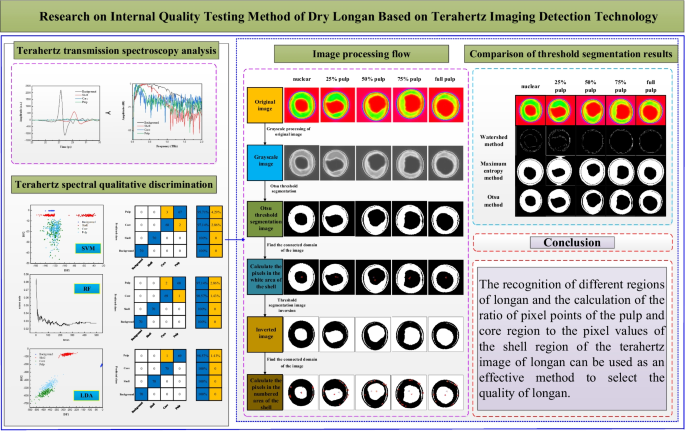
This is a preview of subscription content, log in via an institution to check access.
Access this article
Price includes VAT (Russian Federation)
Instant access to the full article PDF.
Rent this article via DeepDyve
Institutional subscriptions
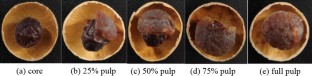
Data availability
Data underlying the results presented in this paper are not publicly available at this time but may be obtained from the authors upon reasonable request.
X. Zhang, S. Guo, C.T. Ho et al., Phytochemical constituents and biological activities of longan ( Dimocarpus longan Lour.) fruit: a review. Food Sci. Hum. Wellness 9 (2), 95–102 (2020)
Article Google Scholar
H. Lim, J. Lee, S. Lee et al., Low-density foreign body detection in food products using single-shot grid-based dark-field X-ray imaging. J. Food Eng. 335 , 111189 (2022)
Article CAS Google Scholar
D. Mery, I. Lillo, H. Loebel et al., Automated fish bone detection using X-ray imaging. J. Food Eng. 105 (3), 485–492 (2011)
I. Orina, M. Manley, S. Kucheryavskiy et al., Application of image texture analysis for evaluation of X-ray images of fungal-infected maize kernels. Food Anal. Methods 11 , 2799–2815 (2018)
X. Cheng, M. Zhang, B. Adhikari et al., Effect of power ultrasound and pulsed vacuum treatments on the dehydration kinetics, distribution, and status of water in osmotically dehydrated strawberry: a combined NMR and DSC study. Food Bioprocess Technol. 7 , 2782–2792 (2014)
S. Baek, J. Lim, J.G. Lee et al., Investigation of the maturity changes of cherry tomato using magnetic resonance imaging. Appl. Sci. 10 (15), 5188 (2020)
M.S. Razavi, A. Asghari, M. Azadbakh et al., Analyzing the pear bruised volume after static loading by magnetic resonance imaging (MRI). Sci. Hortic. 229 , 33–39 (2018)
F. Wang, C. Zhao, H. Yang et al., Non-destructive and in-site estimation of apple quality and maturity by hyperspectral imaging. Comput. Electron. Agric. 195 , 106843 (2022)
P. Zhang, H. Wang, H. Ji et al., Hyperspectral imaging-based early damage degree representation of apple: a method of correlation coefficient. Postharvest Biol. Technol. 199 , 112309 (2023)
J. Sun, K. Tang, X. Wu et al., Nondestructive identification of green tea varieties based on hyperspectral imaging technology. J. Food Process Eng 41 (5), e12800 (2018)
SY M Y. Terahertz Pulsed Imaging in Reflection Geometry[D]. The Chinese University of Hong Kong, 2011.
Z. Zhu, J. Zhang, Y. Song et al., Broadband terahertz signatures and vibrations of dopamine. Analyst 145 (18), 6006–6013 (2020)
Article CAS PubMed Google Scholar
J. El Haddad, F. de Miollis, J. Bou Sleiman et al., Chemometrics applied to quantitative analysis of ternary mixtures by terahertz spectroscopy. Anal. Chem. 86 (10), 4927–4933 (2014)
Article PubMed Google Scholar
C. Wang, R. Zhou, Y. Huang et al., Terahertz spectroscopic imaging with discriminant analysis for detecting foreign materials among sausages. Food Control 97 , 100–104 (2019)
Y. Shen, Y. Yin, B. Li et al., Detection of impurities in wheat using terahertz spectral imaging and convolutional neural networks. Comput. Electron. Agric. 181 , 105931 (2021)
X. Sun, J. Li, Y. Shen et al., Non-destructive detection of insect foreign bodies in finishing tea product based on terahertz spectrum and image. Front. Nutr. 8 , 757491 (2021)
Article PubMed PubMed Central Google Scholar
J. Hu, H. Shi, C. Zhan et al., Study on the identification and detection of walnut quality based on terahrtz imaging. Foods 11 (21), 3498 (2022)
B. Li, D. Zhang, Y. Shen, Study on terahertz spectrum analysis and recognition modeling of common agricultural diseases. Spectrochim. Acta Part A Mol. Biomol. Spectrosc. 243 , 118820 (2020)
W. Liu, C. Liu, X. Hu et al., Application of terahertz spectroscopy imaging for discrimination of transgenic rice seeds with chemometrics. Food Chem. 210 , 415–421 (2016)
X. Sun, J. Liu, Measurement of plumpness for intact sunflower seed using Terahertz transmittance imaging. J. Infrared Millimeter Terahertz Waves 41 (3), 307–321 (2020)
D.J. Jwo, W.Y. Chang, I.H. Wu, Windowing techniques, the welch method for improvement of power spectrum estimation. Comput. Mater. Contin 67 , 3983–4003 (2021)
Google Scholar
S. Pan, H. Zhang, Z. Li et al., Classification of Ginseng with different growth ages based on terahertz spectroscopy and machine learning algorithm. Optik 236 , 166322 (2021)
X. Wei, D. Kong, S. Zhu et al., Rapid identification of soybean varieties by terahertz frequency-domain spectroscopy and grey wolf optimizer-support vector machine. Front. Plant Sci. 13 , 823865 (2022)
H. Zhang, Z. Li, T. Chen et al., Discrimination of traditional herbal medicines based on terahertz spectroscopy. Optik 138 , 95–102 (2017)
Y. Cao, J. Chen, G. Zhang et al., Characterization and discrimination of human colorectal cancer cells using terahertz spectroscopy. Spectrochim. Acta Part A Mol. Biomol. Spectrosc. 256 , 119713 (2021)
S. Yang, C. Li, Y. Mei et al., Determination of the geographical origin of coffee beans using terahertz spectroscopy combined with machine learning methods. Front. Nutr. 8 , 680627 (2021)
J. Liu, H. Xie, B. Zha et al., Detection of genetically modified sugarcane by using terahertz spectroscopy and chemometrics. J. Appl. Spectrosc. 85 , 119–125 (2018)
J. Li, W. Luo, Z. Wang et al., Early detection of decay on apples using hyperspectral reflectance imaging combining both principal component analysis and improved watershed segmentation method. Postharvest Biol. Technol. 149 , 235–246 (2019)
J. Hu, P. Qiao, L. Yang et al., Research on nondestructive detection of pine nut quality based on terahertz imaging. Infrared Phys. Technol. 134 , 104798 (2023)
Wang Y T, Li Q. A segmentation algorithm on terahertz digital holographic image[C]//14th National Conference on Laser Technology and Optoelectronics (LTO 2019). SPIE, 2019, 11170: 310–315.
H. Li, J.X. Wang, Z.N. Xing et al., Influence of improved Kennard/Stone algorithm on the calibration transfer in near-infrared spectroscopy. Spectrosc Spect Anal 31 (2), 362–365 (2011)
Download references
Acknowledgements
National Youth Natural Science Foundation of China (32302261); Jiangxi Ganpo Talented Support Plan -Young science and technology talent Lift Project (2023QT04); Jiangxi Provincial Youth Science Fund Project (20224BAB215042); National Key R&D Program of China (2022YFD2001805).
Author information
Authors and affiliations.
School of Mechatronics & Vehicle Engineering, East China Jiaotong University, Nanchang, 330013, Jiangxi, China
Jun Hu, Hao Wang, Yongqi Zhou, Shimin Yang, Haohao Lv & Liang Yang
You can also search for this author in PubMed Google Scholar
Contributions
Jun Hu: Investigation, Writing-review and editing, Experimental scheme design, Formal analysis. Hao Wang: Writing-original draft, Formal analysis. Yongqi Zhou: Experiment. Shimin Yang, Haohao Lv: Review and editing. Liang Yang: Formal analysis.
Corresponding author
Correspondence to Jun Hu .
Ethics declarations
Competing interests.
We declare that we have no financial and personal relationships with other people or organizations that can inappropriately influence our work, there is no professional or other personal interest of any nature or kind in any product, service and/or company that could be construed as influencing the position presented in, or the review of, the manuscript entitled. Jun Hu, Hao Wang, Yongqi Zhou, Shimin Yang, Haohao Lv, Liang Yang declare that they have no conflict of interest.
Additional information
Publisher's note.
Springer Nature remains neutral with regard to jurisdictional claims in published maps and institutional affiliations.
Rights and permissions
Springer Nature or its licensor (e.g. a society or other partner) holds exclusive rights to this article under a publishing agreement with the author(s) or other rightsholder(s); author self-archiving of the accepted manuscript version of this article is solely governed by the terms of such publishing agreement and applicable law.
Reprints and permissions
About this article
Hu, J., Wang, H., Zhou, Y. et al. Research on internal quality testing method of dry longan based on terahertz imaging detection technology. Food Measure (2024). https://doi.org/10.1007/s11694-024-02583-x
Download citation
Received : 29 December 2023
Accepted : 18 April 2024
Published : 11 May 2024
DOI : https://doi.org/10.1007/s11694-024-02583-x
Share this article
Anyone you share the following link with will be able to read this content:
Sorry, a shareable link is not currently available for this article.
Provided by the Springer Nature SharedIt content-sharing initiative
- Longan fullness
- Terahertz imaging technology
- Otsu threshold segmentation
- Linear discriminant analysis (LDA)
- Find a journal
- Publish with us
- Track your research
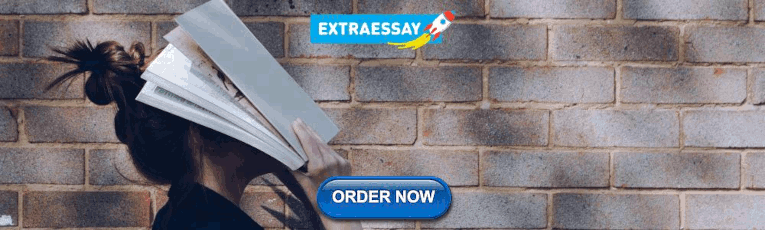
IMAGES
VIDEO
COMMENTS
The importance of measurement in research and technology is indisputable. Measurement is the fundamental mechanism of scientific study and development, and it allows to describe the different phenomena of the universe through the exact and general language of mathematics, without which it would be challenging to define practical or theoretical approaches from scientific investigation.
be done to measure something, whether measuring brain activity, attitude toward an object, organizational emphasis on research and development, or stock market performance. Therefore, these rules include a range of things that occur during the data collection process, such as how questions are worded and how a measure is administered.
The same is true of research instru-ments in psychology and the social sciences. Instead of making music, in research you are asking questions about psychology or other social science concepts. It is critical that you select the best instrument to measure each concept you study. You will have many choices to make about the research instruments
Quantitative research is based on measurement and is conducted in a systematic, controlled manner. These measures enable researchers to perform statistical tests, analyze differences between ...
Measurement in Science. Measurement is an integral part of modern science as well as of engineering, commerce, and daily life. Measurement is often considered a hallmark of the scientific enterprise and a privileged source of knowledge relative to qualitative modes of inquiry. [ 1] Despite its ubiquity and importance, there is little consensus ...
Reliability is consistency across time (test-retest reliability), across items (internal consistency), and across researchers (interrater reliability). Validity is the extent to which the scores actually represent the variable they are intended to. Validity is a judgment based on various types of evidence.
Reliability is consistency across time (test-retest reliability), across items (internal consistency), and across researchers (interrater reliability). Validity is the extent to which the scores actually represent the variable they are intended to. Validity is a judgment based on various types of evidence.
Measurement tools are instruments used by researchers and practitioners to aid in the assessment or evaluation of subjects, clients or patients. The instruments are used to measure or collect data on a variety of variables ranging from physical functioning to psychosocial wellbeing. Types of measurement tools include scales, indexes, surveys ...
Measurement is the process of observing and recording the observations that are collected as part of a research effort. There are two major issues that will be considered here. First, you have to understand the fundamental ideas involved in measuring. Here we consider two of major measurement concepts. In Levels of Measurement, I explain the ...
RESEARCH SCENARIOS AND INSTRUMENT DEVELOPMENT OR ADAPTATION. Epidemiological studies require well-defined and socially relevant research questions, which, in turn, demand reliable and accurate measurements of the phenomena and concepts needed to answer them 8.Berry et al. 9 discuss three perspectives that are particularly relevant for the issues at hand.
Quantitative research is based on measurement and is conducted in a systematic, controlled manner. These measures enable researchers to perform statistical tests, analyze differences between groups, and determine the effectiveness of treatments. If something is not measurable, it cannot be tested. Keywords: measurements; quantitative research ...
Ratio level data provide the final and most robust level of measurement. Ratio level data are measured continuously, with equal spacing between intervals and with a true zero. Examples include height, weight, heart rate, and serum laboratory values. A zero value is interpreted as the absence of the characteristic.
The journal Measurement is receiving an increasing number of papers in the area of machine learning/neural networks and other techniques based on artificial intelligence. These submissions will be desk rejected unless they: prove that the described research advances the state-of-the-art in measurement science and is not just an application of ...
A research design is a strategy for answering your research question using empirical data. Creating a research design means making decisions about: Your overall research objectives and approach. Whether you'll rely on primary research or secondary research. Your sampling methods or criteria for selecting subjects. Your data collection methods.
Research methods are specific procedures for collecting and analyzing data. Developing your research methods is an integral part of your research design. When planning your methods, there are two key decisions you will make. First, decide how you will collect data. Your methods depend on what type of data you need to answer your research question:
Levels of measurement, also called scales of measurement, tell you how precisely variables are recorded. In scientific research, a variable is anything that can take on different values across your data set (e.g., height or test scores). There are 4 levels of measurement: Nominal: the data can only be categorized
DOI: 10.1188/14.ONF.431-433. Measures exist to numerically represent degrees of attributes. Quantitative research is based on measurement and is conducted in a systematic, controlled manner. These measures enable researchers to perform statistical tests, analyze differences between groups, and determine the effectiveness of treatments.
Tests and Measurements. If you are doing dissertation level research, you will also be collecting your own data using a test or measure designed to address the variables present in your research. Finding the right test or measure can sometimes be difficult. In some cases, tests are copyrighted and must be purchased from commercial publishers.
Knowledge of statistics is highly important for research scholars, as they are expected to submit a thesis based on original research as part of a PhD program. As statistics play a major role in the analysis and interpretation of scientific data, intensive training at the beginning of a PhD programme is essential. PhD coursework is mandatory in universities and higher education institutes in ...
This paper introduces a dataset developed to measure the impact of archival and data curation decisions on data reuse. The dataset describes 10,605 social science research datasets, their curation ...
The research, published in the scientific journal RNA, marks the first time that a direct measurement of the interaction between immune cells and cancer cells from a patient's biopsy has been ...
Tornadoes are dramatic and deadly natural disasters. Over 1,200 tornadoes touch down in the U.S. every year — causing dozens of deaths. Until recently, a common perception among structural engineers was that tornadoes were too intense to design buildings to withstand them. But thanks to decades of research at NIST, that misconception has changed.
Reliability is a key concept in research that measures how consistent and trustworthy the results are. In this article, you will learn about the four types of reliability in research: test-retest, inter-rater, parallel forms, and internal consistency. You will also find definitions and examples of each type, as well as tips on how to improve reliability in your own research.
RESEARCH ARTICLE. A systematic study on linear thermal expansion coefficient of metals based on interferometric measurement with Fresnel bimirror. Sifan Lu, ... The measurement results obtained via the finite-method simulation demonstrate the feasibility and reliability of the system. Overall, this study provides a new idea for measuring the ...
Scientists at U.C. Berkeley are using a network of C02 sensors to more accurately monitor emissions. It's a model that is being used in some cities, and could eventually become a national program.
Validity refers to how accurately a method measures what it is intended to measure. If research has high validity, that means it produces results that correspond to real properties, characteristics, and variations in the physical or social world. High reliability is one indicator that a measurement is valid.
Pursuing Equitability in Representation of and Measurement for Women. May 3, 2024 . A panel session held during the 2024 ANA/SeeHer Gender Equality Conference convened experts to speak on the perils and promise of pursuing equitability for women in media representation and in the ways in which they are measured as an audience.
Journal of Food Measurement and Characterization - Longan is a kind of nut with rich nutritional value and homologous function of medicine and food. ... Secondly, the visual research of longan fullness is carried out, transmission imaging is performed on longan samples with different fullness degrees, and gray level processing and Otsu ...