Have a language expert improve your writing
Run a free plagiarism check in 10 minutes, generate accurate citations for free.
- Knowledge Base
- Nominal Data | Definition, Examples, Data Collection & Analysis
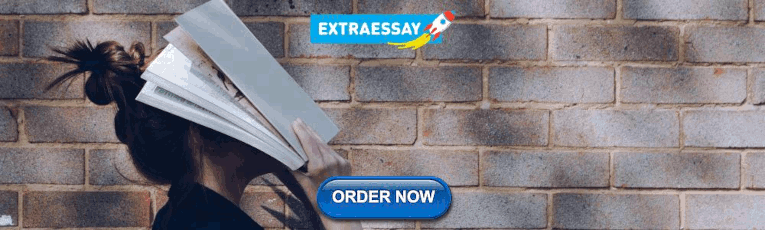
Nominal Data | Definition, Examples, Data Collection & Analysis
Published on August 7, 2020 by Pritha Bhandari . Revised on June 21, 2023.
Nominal data is labelled into mutually exclusive categories within a variable. These categories cannot be ordered in a meaningful way.
For example, pref erred mode of transportation is a nominal variable, because the data is sorted into categories: car, bus, train, tram, bicycle, etc.
Table of contents
Levels of measurement, examples of nominal data, how to collect nominal data, how to analyze nominal data, other interesting articles.
The level of measurement indicates how precisely data is recorded. There are 4 hierarchical levels: nominal, ordinal , interval , and ratio . The higher the level, the more complex the measurement.
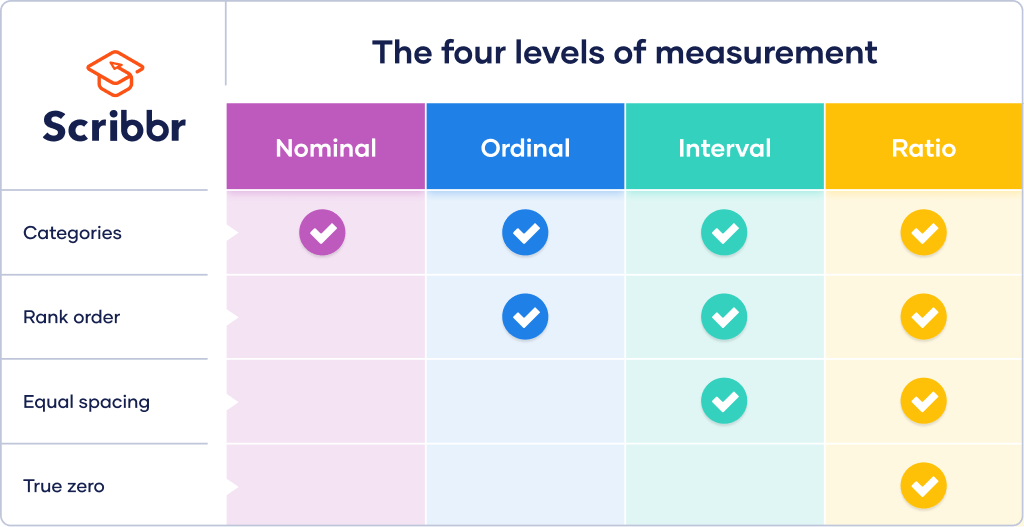
Nominal data is the least precise and complex level. The word nominal means “in name,” so this kind of data can only be labelled . It does not have a rank order, equal spacing between values, or a true zero value.
Here's why students love Scribbr's proofreading services
Discover proofreading & editing
At a nominal level, each response or observation fits only into one category.
Nominal data can be expressed in words or in numbers. But even if there are numerical labels for your data, you can’t order the labels in a meaningful way or perform arithmetic operations with them.
In social scientific research, nominal variables often include gender, ethnicity, political preferences or student identity number.
Variable types that can be coded in only 2 ways (e.g. yes/no or employed/unemployed) are called binary or dichotomous. Since the order of the labels within those variables doesn’t matter, they are types of nominal variable.
Nominal data can be collected through open- or closed-ended survey questions.
If the variable you are interested in has only a few possible labels that capture all of the data, use closed-ended questions.
If your variable of interest has many possible labels, or labels that you cannot generate a complete list for, use open-ended questions.
- What is your student ID number?
- What is your zip code?
- What is your native language?
To analyze nominal data, you can organize and visualize your data in tables and charts.
Then, you can gather some descriptive statistics about your data set. These help you assess the frequency distribution and find the central tendency of your data. But not all measures of central tendency or variability are applicable to nominal data.
Distribution
To organize this data set, you can create a frequency distribution table to show you the number of responses for each category of political preference.
- Simple frequency distribution
- Percentage frequency distribution
Using these tables, you can also visualize the distribution of your data set in graphs and charts.
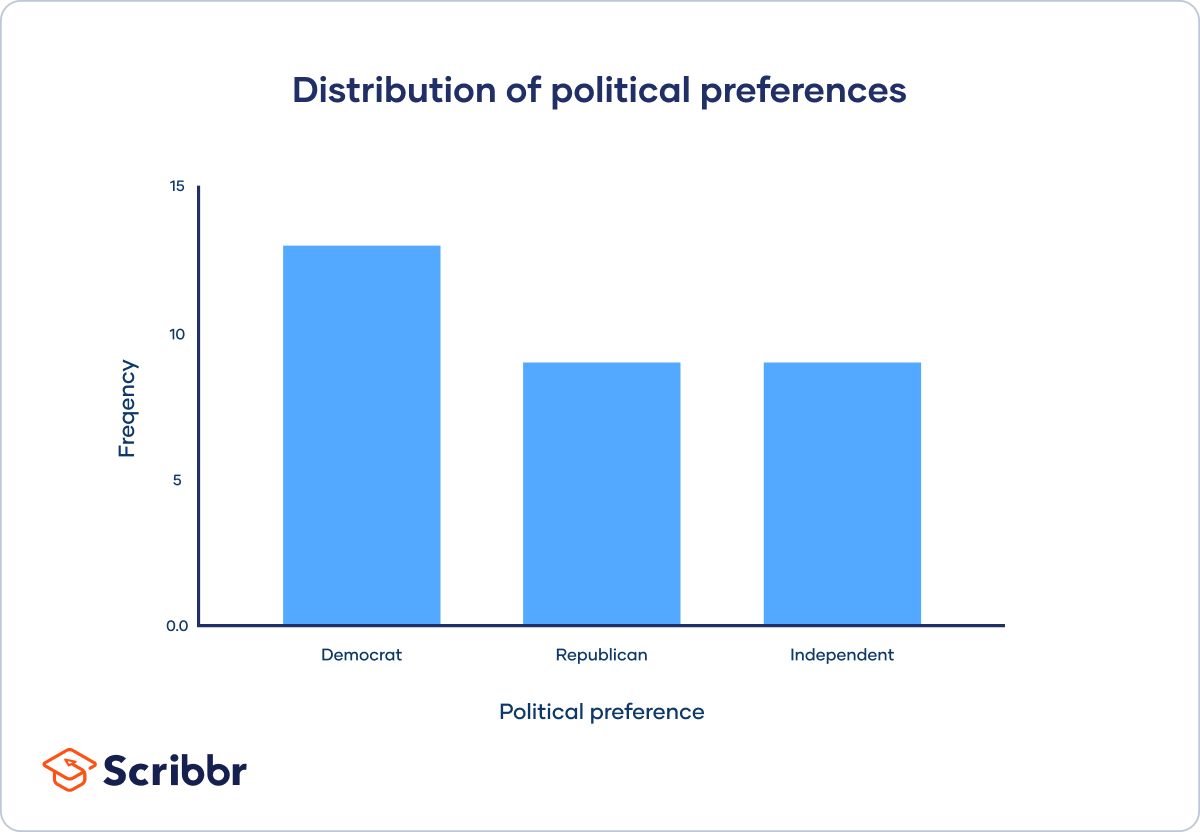
Central tendency
The central tendency of your data set tells you where most of your values lie.
The mode, mean, and median are three most commonly used measures of central tendency. However, only the mode can be used with nominal data.
To get the median of a data set, you have to be able to order values from low to high. For the mean , you need to be able to perform arithmetic operations like addition and division on the values in the data set. While nominal data can be grouped by category, it cannot be ordered nor summed up.
Therefore, the central tendency of nominal data can only be expressed by the mode – the most frequently recurring value.
Statistical tests for nominal data
Inferential statistics help you test scientific hypotheses about your data. Nonparametric statistical tests are used with nominal data.
While parametric tests assume certain characteristics about a data set, like a normal distribution of scores, these do not apply to nominal data because the data cannot be ordered in any meaningful way.
Chi-square tests are nonparametric statistical tests for categorical variables. The goodness of fit chi-square test can be used on a data set with one variable, while the chi-square test of independence is used on a data set with two variables.
The chi-square goodness of fit test is used when you have gathered data from a single population through random sampling. To measure how representative your sample is, you can use this test to assess whether the frequency distribution of your sample matches what you would expect from the broader population.
With the chi-square test of independence , you can find out whether a relationship between two categorical variables is statistically significant .
If you want to know more about statistics , methodology , or research bias , make sure to check out some of our other articles with explanations and examples.
- Student’s t -distribution
- Normal distribution
- Null and Alternative Hypotheses
- Chi square tests
- Confidence interval
Methodology
- Cluster sampling
- Stratified sampling
- Data cleansing
- Reproducibility vs Replicability
- Peer review
- Likert scale
Research bias
- Implicit bias
- Framing effect
- Cognitive bias
- Placebo effect
- Hawthorne effect
- Hindsight bias
- Affect heuristic
Cite this Scribbr article
If you want to cite this source, you can copy and paste the citation or click the “Cite this Scribbr article” button to automatically add the citation to our free Citation Generator.
Bhandari, P. (2023, June 21). Nominal Data | Definition, Examples, Data Collection & Analysis. Scribbr. Retrieved April 8, 2024, from https://www.scribbr.com/statistics/nominal-data/
Is this article helpful?
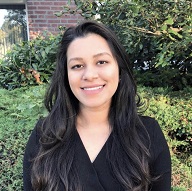
Pritha Bhandari
Other students also liked, levels of measurement | nominal, ordinal, interval and ratio, types of variables in research & statistics | examples, survey research | definition, examples & methods, what is your plagiarism score.

25 Nominal Variable Examples
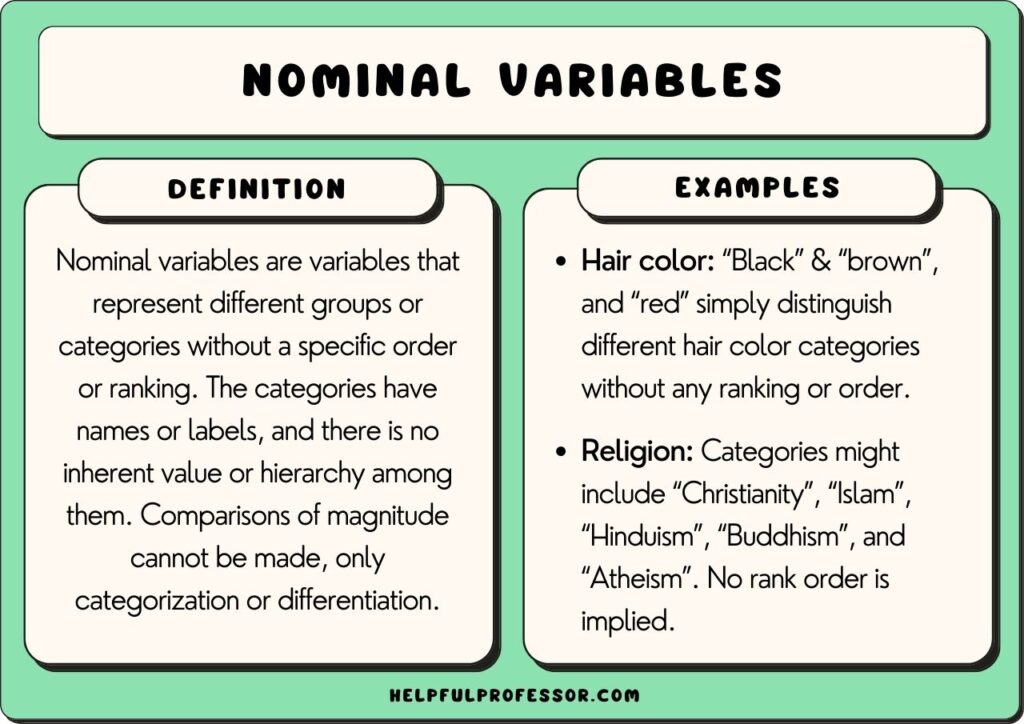
Nominal variables are variables that represent categories without any inherent order or ranking. They are simply used to distinguish different groups or categories without assigning any form of hierarchy or sequence to them (Babbie et al., 2007).
“Gender”, “marital status”, “nationality”, and “types of occupation” are typical nominal variables examples. These sorts of variables are commonly used in cross-sectional studies such as a population census. The critical distinction of nominal variables lies in their categorical nature and absence of systemic order (Katz, 2006b; Stockemer, 2018).

Nominal Variables Examples
1. Gender Gender, with categories typically including “male”, “female”, and “other”, is a primary example of a nominal variable. Unlike ordinal variables, these categories have no presumed order or ranking.
2. Marital Status Marital Status is a kind of nominal variable. The categories might be “single”, “married”, “divorced”, and “widowed”. No inherent hierarchy exists among these categories.
3. Nationality Nationality is a nominal variable. For instance, labels like “American”, “Canadian”, “Chinese”, and “Brazilian” are chiefly identifiers, and don’t denote any order.
4. Hair Color Hair color is another example of a nominal variable. Labels such as “black”, “brown”, “blonde” and “red” simply distinguish different hair color categories without implying any ranking or order.
5. Types of Pets One could amass nominal data by asking about a person’s pet type – “dog”, “cat”, “fish”, “bird”, etc. These categories bear no logical order.
6. Car Brands Car brands like “Toyota”, “Ford”, “Mercedes”, and “BMW” constitute another instance of a nominal variable. The names represent different brands without any inherent ranking or sequence.
7. Religion Religion is a nominal variable. Categories might include “Christianity”, “Islam”, “Hinduism”, “Buddhism”, and “Atheism”. Each label simply identifies a specific religious group, with no rank order implied.
8. Disciplines of Study The various disciplines of study — “Arts”, “Sciences”, “Commerce”, “Engineering” — are examples of nominal variables. These categories serve to label distinct branches of study, without any rank order.
9. Types of Houses The kinds of dwelling places – “apartment”, “bungalow”, “condo”, “duplex” – can be classified as a nominal variable.
10. Language Language is another nominal variable example. Categories such as “English”, “Spanish”, “French”, etc., are simply distinct labels without inherent hierarchy.
11. Blood Type Classification by blood type (A, B, AB, O) represents a nominal variable.
12. Occupations “Doctor”, “Engineer”, “Teacher”, “Artist” are types of occupations, hence a nominal variable, as there’s no inherent ranking or sequence among these professions.
13. Fast Food Chains Fast food chains like “McDonald’s”, “Burger King”, or “KFC” come under nominal variables.
14. Music Genre Music genres, such as “rock”, “jazz”, “classical”, “pop”, are examples of nominal variables. Here, the categories simply identify distinct types of music, with no rank order implied.
15. Eye Color Eye color, categorized as “black”, “blue”, “hazel”, “green”, is another example of a nominal variable.
16. Favorite Sport “Football”, “Basketball”, “Tennis”, “Cricket” are different sports, categorized as a nominal variable.
17. TV Show Genres Genres of TV shows, such as “comedy”, “drama”, “reality”, and “sitcom” classify as nominal variables.
18. Postal Codes Postal codes regularly classify as nominal variables. While they may contain numbers, the values don’t imply a specific order or ranking.
19. Patterns of Fabric References to “striped”, “floral”, “polka dot”, or “solid color” are nominal variables, used to classify different fabric patterns. These categories have no inherent order or ranking.
20. Types of Computers Categories such as “desktop”, “laptop”, and “tablet” can be classified as a nominal variable.
21. Types of Plants “Bushes”, “trees”, “flowers”, and “grasses” are different types of plants and a great example of a nominal variable.
22. Political Affiliations “Republican”, “Democrat”, “Independent”, and others are political affiliations. They classify as a nominal variable, as there’s no inherent ranking or sequence between them.
23. Patterns of Fabric References to “striped”, “floral”, “polka dot”, or “solid color” are nominal variables, used to classify different fabric patterns. These categories have no inherent order or ranking.
24. Forms of Payment Options like “credit card”, “debit card”, “cash”, or “cheque” are categorized as nominal variables.
25. Area of Residence The section of a city someone might live in, such as “North”, “South”, “East”, or “West”, serves as a nominal variable. These categories merely function as distinct identifiers, not suggesting any hierarchy or sequence.
Types of Variables (Compare and Contrast)
Nominal variables typically contrast with other types of variables including ordinal, interval, and ratio variables.
Here’s a short overview.
- Ordinal variables have categories that can be logically ordered or ranked. While ordinal variables provide a sense of order or ranking, the exact or consistent distance between different categories remains unknown or inconsistent. For instance, t-shirt sizes (“small”, “medium”, “large”) that presents an order but doesn’t clarify the actual extent of the difference between the categories (Katz, 2006a; Katz, 2006b).
- Interval variables , in contrast to nominal variables, have ordered categories with known and consistent distances. Temperature measurements such as Celsius or Fahrenheit are the classic examples of interval variables (Lewis-Beck, Bryman & Liao, 2004).
- Ratio variables resemble interval variables, but with a defined zero point. For instance, weight measurements (grams, kilograms) are examples of ratio variables (Katz, 2006a; Katz, 2006b).
- Nominal variables are variables with categories that don’t have a natural order or ranking (Wilson & Joye, 2016). Unlike ordinal and interval variables, nominal variables do not provide any sense of hierarchy or order among the variables.
Nominal variables serve an essential role in different types of academic research as a form of categorical data . They enable differentiating data into distinctive groups or labels with no order or sequence. While these variables provide clear distinctions between categories, the lack of any order often limits the kind of statistical tests that can be applied to them. Their propensity to identify and differentiate rather than measure places nominal variables as a common choice for demographical data and other forms of categorical information.
Babbie, E., Halley, F., & Zaino, J. (2007). Adventures in Social Research: Data Analysis Using SPSS 14.0 and 15.0 for Windows (6th ed.). New York: SAGE Publications.
De Vaus, D. A. (2001). Research Design in Social Research . New York: SAGE Publications.
Katz, M. (2006) . Study Design and Statistical Analysis: A Practical Guide for Clinicians . Cambridge: Cambridge University Press.
Katz, M. H. (2006). Multivariable analysis: A practical guide for clinicians . Cambridge: Cambridge University Press.
Lewis-Beck, M., Bryman, A. E., & Liao, T. F. (Eds.). (2004). The SAGE Encyclopedia of Social Science Research Methods (Vol. 1) . London: SAGE Publications.
Norman, G. R., & Streiner, D. L. (2008). Biostatistics: The Bare Essentials . New York: B.C. Decker.
Stockemer, D. (2018). Quantitative Methods for the Social Sciences: A Practical Introduction with Examples in SPSS and Stata . London: Springer International Publishing.
Wilson, J. H., & Joye, S. W. (2016). Research Methods and Statistics: An Integrated Approach . New York: SAGE Publications.
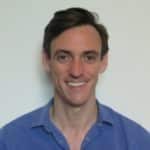
Chris Drew (PhD)
Dr. Chris Drew is the founder of the Helpful Professor. He holds a PhD in education and has published over 20 articles in scholarly journals. He is the former editor of the Journal of Learning Development in Higher Education. [Image Descriptor: Photo of Chris]
- Chris Drew (PhD) https://helpfulprofessor.com/author/chris-drew-phd/ 5 Top Tips for Succeeding at University
- Chris Drew (PhD) https://helpfulprofessor.com/author/chris-drew-phd/ 50 Durable Goods Examples
- Chris Drew (PhD) https://helpfulprofessor.com/author/chris-drew-phd/ 100 Consumer Goods Examples
- Chris Drew (PhD) https://helpfulprofessor.com/author/chris-drew-phd/ 30 Globalization Pros and Cons
Leave a Comment Cancel Reply
Your email address will not be published. Required fields are marked *

Statistics Made Easy
Levels of Measurement: Nominal, Ordinal, Interval and Ratio

In statistics, we use data to answer interesting questions. But not all data is created equal. There are actually four different data measurement scales that are used to categorize different types of data:
3. Interval
In this post, we define each measurement scale and provide examples of variables that can be used with each scale.
The simplest measurement scale we can use to label variables is a nominal scale .
Nominal scale: A scale used to label variables that have no quantitative values.
Some examples of variables that can be measured on a nominal scale include:
- Gender: Male, female
- Eye color: Blue, green, brown
- Hair color: Blonde, black, brown, grey, other
- Blood type: O-, O+, A-, A+, B-, B+, AB-, AB+
- Political Preference: Republican, Democrat, Independent
- Place you live: City, suburbs, rural
Variables that can be measured on a nominal scale have the following properties:
- They have no natural order. For example, we can’t arrange eye colors in order of worst to best or lowest to highest.
- Categories are mutually exclusive. For example, an individual can’t have both blue and brown eyes. Similarly, an individual can’t live both in the city and in a rural area.
- The only number we can calculate for these variables are counts . For example, we can count how many individuals have blonde hair, how many have black hair, how many have brown hair, etc.
- The only measure of central tendency we can calculate for these variables is the mode . The mode tells us which category had the most counts. For example, we could find which eye color occurred most frequently.
The most common way that nominal scale data is collected is through a survey. For example, a researcher might survey 100 people and ask each of them what type of place they live in.
Question: What type of area do you live in?
Possible Answers: City, Suburbs, Rural.
Using this data, the researcher can find out how many people live in each area, as well as which area is the most common to live in.
The next type of measurement scale that we can use to label variables is an ordinal scale .
Ordinal scale: A scale used to label variables that have a natural order , but no quantifiable difference between values.
Some examples of variables that can be measured on an ordinal scale include:
- Satisfaction: Very unsatisfied, unsatisfied, neutral, satisfied, very satisfied
- Socioeconomic status: Low income, medium income, high income
- Workplace status: Entry Analyst, Analyst I, Analyst II, Lead Analyst
- Degree of pain: Small amount of pain, medium amount of pain, high amount of pain
Variables that can be measured on an ordinal scale have the following properties:
- They have a natural order. For example, “very satisfied” is better than “satisfied,” which is better than “neutral,” etc.
- The difference between values can’t be evaluated. For example, we can’t exactly say that the difference between “very satisfied and “satisfied” is the same as the difference between “satisfied” and “neutral.”
- The two measures of central tendency we can calculate for these variables are the mode and the median . The mode tells us which category had the most counts and the median tells us the “middle” value.
Ordinal scale data is often collected by companies through surveys who are looking for feedback about their product or service. For example, a grocery store might survey 100 recent customers and ask them about their overall experience.
Question: How satisfied were you with your most recent visit to our store?
Possible Answers: Very unsatisfied, unsatisfied, neutral, satisfied, very satisfied.
Using this data, the grocery store can analyze the total number of responses for each category, identify which response was most common, and identify the median response.
The next type of measurement scale that we can use to label variables is an interval scale .
Interval scale: A scale used to label variables that have a natural order and a quantifiable difference between values, but no “true zero” value .
Some examples of variables that can be measured on an interval scale include:
- Temperature: Measured in Fahrenheit or Celsius
- Credit Scores: Measured from 300 to 850
- SAT Scores: Measured from 400 to 1,600
Variables that can be measured on an interval scale have the following properties:
- These variables have a natural order.
- We can measure the mean, median, mode, and standard deviation of these variables.
- These variables have an exact difference between values. Recall that ordinal variables have no exact difference between variables – we don’t know if the difference between “very satisfied” and “satisfied” is the same as the difference between “satisfied” and “neutral.” For variables on an interval scale, though, we know that the difference between a credit score of 850 and 800 is the exact same as the difference between 800 and 750.
- These variables have no “true zero” value. For example, it’s impossible to have a credit score of zero. It’s also impossible to have an SAT score of zero. And for temperatures, it’s possible to have negative values (e.g. -10° F) which means there isn’t a true zero value that values can’t go below.
The nice thing about interval scale data is that it can be analyzed in more ways than nominal or ordinal data. For example, researchers could gather data on the credit scores of residents in a certain county and calculate the following metrics:
- Median credit score (the “middle” credit score value)
- Mean credit score (the average credit score)
- Mode credit score (the credit score that occurs most often)
- Standard deviation of credit scores (a way to measure how spread out credit scores are)
The last type of measurement scale that we can use to label variables is a ratio scale .
Ratio scale: A scale used to label variables that have a natural order, a quantifiable difference between values, and a “true zero” value.
Some examples of variables that can be measured on a ratio scale include:
- Height: Can be measured in centimeters, inches, feet, etc. and cannot have a value below zero.
- Weight: Can be measured in kilograms, pounds, etc. and cannot have a value below zero.
- Length: Can be measured in centimeters, inches, feet, etc. and cannot have a value below zero.
Variables that can be measured on a ratio scale have the following properties:
- We can calculate the mean, median, mode, standard deviation, and a variety of other descriptive statistics for these variables.
- These variables have an exact difference between values.
- These variables have a “true zero” value. For example, length, weight, and height all have a minimum value (zero) that can’t be exceeded. It’s not possible for ratio variables to take on negative values. For this reason, the ratio between values can be calculated. For example, someone who weighs 200 lbs. can be said to weigh two times as much as someone who weights 100 lbs. Likewise someone who is 6 feet tall is 1.5 times taller than someone who is 4 feet tall.
Data that can be measured on a ratio scale can be analyzed in a variety of ways. For example, researchers could gather data about the height of individuals in a certain school and calculate the following metrics:
- Median height
- Mean height
- Mode height
- Standard deviation of heights
- Ratio of tallest height to smallest height
The following table provides a summary of the variables in each measurement scale:

Published by Zach
Leave a reply cancel reply.
Your email address will not be published. Required fields are marked *
What is Nominal Data? Definition, Characteristics and Examples
What is nominal data and what is it used for? How is it collected and analyzed? Learn everything you need to know in this guide.
There are many different industries and career paths that involve working with data—including psychology, marketing, and, of course, data analytics. If you’re working with data in any capacity, there are four main data types (or levels of measurement) to be aware of: nominal, ordinal, interval, and ratio. Here, we’ll focus on nominal data.
We’ll briefly introduce the four different types of data, before defining what nominal data is and providing some examples. We’ll then look at how nominal data can be collected and analyzed. If you want to skip ahead to a specific section, just use the clickable menu.
- An introduction to the four different types of data
- Nominal data definition
- Key characteristics of nominal data
- Nominal data examples
- How is nominal data collected and what is it used for?
- Nominal data analysis
- Key takeaways and next steps
Ready for a complete introduction to nominal data? Let’s go.
1. The four different types of data (or levels of measurement)
When we talk about the four different types of data, we’re actually referring to different levels of measurement. Levels (or scales) of measurement indicate how precisely a variable has been recorded. The level of measurement determines how and to what extent you can analyze the data.
The four levels of measurement are nominal , ordinal , interval , and ratio , with nominal being the least complex and precise measurement, and ratio being the most. In the hierarchy of measurement, each level builds upon the last. So:
- Nominal data denotes labels or categories (e.g. blonde hair, brown hair).
- Ordinal data refers to data that can be categorized and also ranked according to some kind of order or hierarchy (e.g. low income, medium income, high income). Learn more about ordinal data in this guide .
- Interval data can be categorized and ranked just like ordinal data, and there are equal, evenly spaced intervals between the categories (e.g. temperature in Fahrenheit). Learn more in this complete guide to interval data .
- Ratio data is just like interval data in that it can be categorized and ranked, and there are equal intervals between the data points. Additionally, ratio data has a true zero. Weight in kilograms is an example of ratio data; if something weighs zero kilograms, it truly weighs nothing. On the other hand, a temperature of zero degrees doesn’t mean there is “no temperature”—and that’s the difference between interval and ratio data. You’ll find a complete guide to ratio data here .
You can learn more in this comprehensive guide to the levels of measurement (with examples) .
What do the different levels of measurement tell you?
The various levels of measurement are important because they determine how you can analyze your data. When analyzing data, you’ll use descriptive statistics to describe or summarize the characteristics of your dataset, and inferential statistics to test different hypotheses. The descriptive and inferential methods you’re able to use will vary depending on whether the data are nominal, ordinal, interval, or ratio. You can learn more about the difference between descriptive and inferential statistics here .
So, before you start collecting data, it’s important to think about the levels of measurement you’ll use.
2. Nominal data definition
Nominal data is a type of qualitative data which groups variables into categories. You can think of these categories as nouns or labels; they are purely descriptive, they don’t have any quantitative or numeric value, and the various categories cannot be placed into any kind of meaningful order or hierarchy.
At this point, it’s important to note that nominal variables may be represented by numbers as well as words—however, these “number labels” don’t have any kind of numeric meaning. To illustrate this with an example, let’s imagine you’re collecting data on people’s hair color. You might use a numbering system to denote the different hair colors: say, 1 to represent brown hair, 2 to represent blonde hair, 3 for black hair, 4 for auburn hair, 5 for gray hair, and so on.
Although you are using numbers to label each category, these numbers do not represent any kind of value or hierarchy (e.g. gray hair as represented by the number 5 is not “greater than” or “better than” brown hair represented by the number 1, and vice versa).
As such, nominal data is the simplest, least precise level of measurement. You can identify nominal data according to the following characteristics.
3. Key characteristics of nominal data
- Nominal data are categorical, and the categories are mutually exclusive; there is no overlap between the categories.
- Nominal data are categorized according to labels which are purely descriptive—they don’t provide any quantitative or numeric value.
- Nominal data cannot be placed into any kind of meaningful order or hierarchy—no one category is greater than or “worth more” than another.
What’s the difference between nominal and ordinal data?
While nominal and ordinal data both count as categorical data (i.e. not numeric), there is one key difference. Nominal variables can be divided into categories, but there is no order or hierarchy to the categories. Ordinal variables, on the other hand, can be divided into categories that naturally follow some kind of order.
For example, the variable “hair color” is nominal as it can be divided into various categories (brown, blonde, gray, black, etc) but there is no hierarchy to the various hair colors. The variable “education level” is ordinal as it can be divided into categories (high school, bachelor’s degree, master’s degree, etc.) and there is a natural order to the categories; we know that a bachelor’s degree is a higher level of education than high school, and that a master’s degree is a higher level of education than a bachelor’s degree, and so on.
So, if there is no natural order to your data, you know that it’s nominal.
4. Nominal data examples
So what are some examples of nominal data that you might encounter? Let’s take a look.
- Hair color (blonde, gray, brown, black, etc.)
- Nationality (Kenyan, British, Chinese, etc.)
- Relationship status (married, cohabiting, single, etc.)
- Preferred mode of public transportation (bus, train, tram, etc.)
- Blood type (O negative, O positive, A negative, and so on)
- Political parties voted for (party X, party Y, party Z, etc.)
- Attachment style according to attachment theory (secure, anxious-preoccupied, dismissive-avoidant, fearful-avoidant)
- Personality type (introvert, extrovert, ambivert, for example)
- Employment status (employed, unemployed, retired, etc.)
As you can see, nominal data is really all about describing characteristics. With those examples in mind, let’s take a look at how nominal data is collected and what it’s used for.
5. How is nominal data collected and what is it used for?
Nominal data helps you to gain insight into a particular population or sample. This is useful in many different contexts, including marketing, psychology, healthcare, education, and business—essentially any scenario where you might benefit from learning more about your target demographic.
Nominal data is usually collected via surveys. Where the variables of interest can only be divided into two or a few categories, you can use closed questions. For example:
- Question: What’s your favorite mode of public transportation? Possible answers: Bus, tram, train
- Question: Are you over 30 years of age? Possible answers: Yes, no
If there are lots of different possible categories, you can use open questions where the respondent is required to write their answer. For example, “What is your native language?” or “What is your favorite genre of music?”
Once you’ve collected your nominal data, you can analyze it. We’ll look at how to analyze nominal data now.
6. Nominal data analysis
No matter what type of data you’re working with, there are some general steps you’ll take in order to analyze and make sense of it. These include gathering descriptive statistics to summarize the data, visualizing your data , and carrying out some statistical analysis .
So how do you analyze nominal data? Let’s take a look, starting with descriptive statistics.
Descriptive statistics for nominal data
Descriptive statistics help you to see how your data are distributed. Two useful descriptive statistics for nominal data are frequency distribution and central tendency (mode) .
Frequency distribution tables
Let’s imagine you’re investigating what mode of public transportation people living in London prefer. In its raw form, this data may appear quite disorganized and unstructured—a spreadsheet containing a column for “Preferred mode of public transport,” a column for “Location,” and a column for “Income,” with the values for each variable entered at random.
Note that, in this example dataset, the first two variables—“Preferred mode of transport” and “Location”—are nominal, but the third variable (“Income”) is ordinal as it follows some kind of hierarchy (high, medium, low).
At first glance, it’s not easy to see how your data are distributed. For example, it’s not immediately clear how many respondents answered “bus” versus “tram,” nor is it easy to see if there’s a clear winner in terms of preferred mode of transportation.
To bring some order to your nominal data, you can create a frequency distribution table. This allows you to see how many responses there were for each category. A simple way to do this in Microsoft Excel is to create a pivot table. You can learn how to create a pivot table in this step-by-step guide .
Here’s what a pivot table would look like for our transportation example:
You can also calculate the frequency distribution as a percentage, allowing you to see what proportion of your respondents prefer which mode of transport. Here’s what that would look like in our pivot table:
Measure of central tendency (mode)
As the name suggests, measures of central tendency help you to identify the “center point” of your dataset; that is, the value that is most representative of the entire dataset. Measures of central tendency include:
- The mode: The value that appears most frequently within a dataset
- The median: The middle value
- The mean: The average value
When it comes to nominal data, the only measure of central tendency you can use is the mode . To identify the mode, look for the value or category that appears most frequently in your distribution table. In the case of our example dataset, “bus” has the most responses (11 out of a total of 20, or 55%) and therefore constitutes the mode.
As you can see, descriptive statistics help you to gain an overall picture of your nominal dataset. Through your distribution tables, you can already glean insights as to which modes of transport people prefer.
Visualizing nominal data
Data visualization is all about presenting your data in a visual format. Just like the frequency distribution tables, visualizing your nominal data can help you to see more easily what the data may be telling you.
Some simple yet effective ways to visualize nominal data are through bar graphs and pie charts. You can do this in Microsoft Excel simply by clicking “Insert” and then selecting “Chart” from the dropdown menu.
(Non-parametric) statistical tests for nominal data
While descriptive statistics (and visualizations) merely summarize your nominal data, inferential statistics enable you to test a hypothesis and actually dig deeper into what the data are telling you.
There are two types of statistical tests to be aware of: parametric tests which are used for interval and ratio data, and non-parametric tests which are used for nominal and ordinal data. So, as we’re dealing with nominal data, we’re only concerned with non-parametric tests.
When analyzing a nominal dataset, you might run:
- A chi-square goodness of fit test, if you’re only looking at one variable
- A chi-square test of independence, if you’re looking at two variables
Chi-square goodness of fit test (for a dataset with one nominal variable)
The Chi-square goodness of fit test helps you to assess whether the sample data you’ve collected is representative of the whole population. In our earlier example, we gathered data on the public transport preferences of twenty Londoners. Let’s imagine that, prior to gathering this data, we looked at historical data published by Transport for London (TFL) and hypothesized that most Londoners will prefer to travel by train. However, according to the sample of data we collected ourselves, bus is the most popular way to travel.
Now we want to know how applicable our findings are to the whole population of people living in London. Of course, it’s not possible to gather data for every single person living in London; instead, we use the Chi-square goodness of fit test to see how much, or to what extent, our observations differ from what we expected or hypothesized. If you’re interested in carrying out a Chi-square goodness of fit test, you’ll find a comprehensive guide here .
Chi-square test of independence (for a dataset with two nominal variables)
If you want to explore the relationship between two nominal variables, you can use the Chi-square test of independence. In our public transport example, we also collected data on each respondent’s location (inner city or suburbs). Perhaps you want to see if there’s a significant correlation between people’s proximity to the city center and their preferred mode of transport.
In this case, you could carry out a Chi-square test of independence (otherwise known as a Chi-square association test). Essentially, the frequency of each category for one nominal variable (say, bus, train, and tram) is compared across the categories of the second nominal variable (inner city or suburbs). You can learn more about how to run a Chi-square test of independence here .
7. Key takeaways and next steps
In this guide, we answered the question: what is nominal data? We looked at:
- Introduced the four levels of data measurement: Nominal, ordinal, interval, and ratio.
- Defined nominal data as a type of qualitative data which groups variables into mutually exclusive, descriptive categories.
- Explained the difference between nominal and ordinal data: Both are divided into categories, but with nominal data, there is no hierarchy or order to the categories.
- Shared some examples of nominal data: Hair color, nationality, blood type, etc.
- Introduced descriptive statistics for nominal data: Frequency distribution tables and the measure of central tendency (the mode).
- Looked at how to visualize nominal data using bar graphs and pie charts.
- Introduced non-parametric statistical tests for analyzing nominal data: The Chi-square goodness of fit test (for one nominal variable) and the Chi-square test of independence (for exploring the relationship between two nominal variables).
If you’re exploring statistics as part of your journey into data analytics or data science, why not try a free introductory data analytics short course ? And, for further reading, check out the following:
- What is Bernoulli distribution? A beginner’s guide
- Quantitative vs. qualitative data: What’s the difference?
- An introduction to multivariate analysis
- How it works
A Comprehensive Guide on Nominal Data
Published by Owen Ingram at August 31st, 2021 , Revised On July 20, 2023
Almost every industry today involves data in one way or the other. And if you are dealing with data in any capacity, you must be familiar with the four data types, also known as levels of measurement. These are Nominal, Ordinal , Interval , and Ratio .
Though this blog will only reflect on what nominal data is, where it is used, and how to analyze it, we will briefly introduce the other three for a quick recap.
Introduction to Levels of Measurement
Whenever we say different types of data in statistics , know that we are actually referring to the levels of measurement . These various types of data show how precisely variables are recorded. The level of measurement can help you find how and to what extent the data can be evaluated.
Nominal Data:
Nominal data defines categories and labels, for instance, brown eyes, red hair.
Ordinal Data:
Ordinal data denotes data that can be ranked and categorized to form a hierarchy. An example would be low to higher grades.
Interval Data:
This level of measurement can also be categorized and ranked. Note that there are evenly spaced and equal intervals between the categories—for instance, the Fahrenheit temperature.
Ratio Data:
Ratio data , just like ordinal and interval data , can also be ranked and categorized. The intervals here are, likewise, equally spaced. The only thing that makes this one different from the rest is that ratio data has a true zero.
For example , if you measure something in kgs, it is ratio data. Now, if something weighs zero, then it means that it does not weigh anything or most likely does not even exist. However, if the temperature in Fahrenheit or any other temperature scale is zero, that does not imply ‘no temperature.’
Before we get to the actual topic, which is Nominal Data, let us also glance at what different levels of measurement or types of data tell us.
Significance of Levels of Measurement
The simplest answer to why these levels of measurement are significant in statistics and in our everyday lives is that they can help us analyze data. When we describe the characteristics of our dataset, we will use descriptive statistics , and when testing of various hypotheses is needed, inferential statistics would be required.
Now whether you should use inferential statistics or descriptive statistics will depend on the type of data you have at hand. So, before you get to the data collection process , make sure to be clear about which levels of measurement you might want to use.
What is Nominal Data?
It is a type of qualitative data that divides variables into groups and categories. Keep in mind that these variables are purely descriptive, meaning they do not have any quantitative nature or value. You can also not put these variables in the form of meaningful order.
Now, depending on the experiment under process, you can define these variables in the form of words or numbers. Were you surprised to hear the numbers? Well, yes, you can use numbers.
To illustrate this with an example, suppose you are collecting information on people’s eye color. What you can do here is, use a numbering system to denote different eye colors. Say, blue is numbered 1, brown 2, black 3, and so on.
In this way, you can use both numbers and words to label different categories.
Characteristics of Nominal Data
- Nominal data can be categorized and grouped into labels that are not numeric. They are purely descriptive!
- It is categorical, and the categories in nominal data are mutually exclusive. This means that there is no overlapping between the categories
- You cannot place nominal data in any hierarchy or order. One category is not more or less of value than the other
What are a Few Examples of Nominal Data?
Following are a few examples of nominal data for your understanding:
Eye Color: Black, Brown, Blue, Green, etc.
Blood Group: A negative, B positive, O negative, O positive, etc.
Religion: Christianity, Buddhism, Islam, etc.
Political Affiliation: XYZ, YZE, UIO, OPT, etc.
You might have noticed that in all these examples, the characteristics are descriptive and cannot be denoted in the form of numbers unless you label them yourself.
What data collection best suits your research?
- Find out by hiring an expert from ResearchProspect today!
- Despite how challenging the subject may be, we are here to help you.
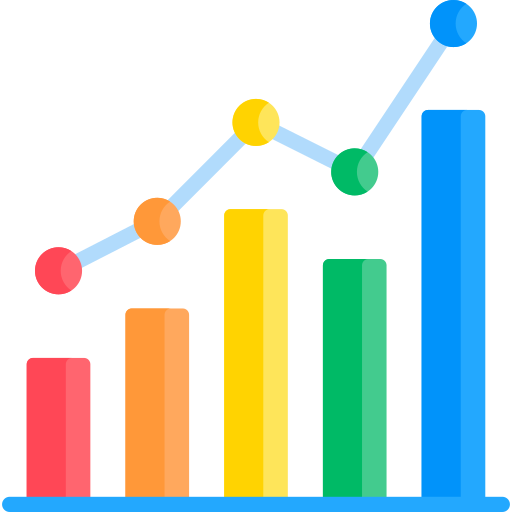
How do you Collect Nominal Data?
With the help of nominal data, you find out valuable important about a particular population or sample . Learning about your target demographic can benefit you in many ways, whether it is a finding you have been planning to do for years or a theory you believe can be proved right or rejected.
Nominal data can be collected through surveys , interviews, online questionnaires, and so on. You can either have close-ended questions for drawing certain conclusions, or have open-ended questions.
Examples of closed questions can be:
Are you over 18 years of age?
Possible answers: No, yes.
What is your hair color?
Possible answers: Black, Brown, and Ash Grey.
However, if there are lots of possible categories or groups, you can go with open-ended questions.
For instance:
What is your favorite movie genre?
What is your favorite sport?
What are some of the languages you can speak or understand?
It looks like we are done with collecting the data section, and now, it is our turn to analyze all the gathered data.
Is the Statistics assignment pressure too much to handle?
How about we handle it for you.
Put in the order right now in order to save yourself time, money, and nerves at the last minute.
How can you Analyze Nominal Data?
There are some general steps you must follow when assessing and evaluating data to form conclusions. These steps include collecting descriptive statistics , visualizing the data , and then carry out statistical analysis .
Descriptive Statistics
In order to see how data can be distributed, descriptive statistics can be used. The most common descriptive statistics for nominal data are central tendency and frequency distribution .
Frequency distribution in research is a graph or chart that shows the frequency of occurrence of each possible outcome of an event or process observed. You can bring some order to your nominal data by creating a frequency distribution table. Simply use Microsoft Excel for creating a pivot table, and it will help you deduce results swiftly.
The measure of central tendency identifies the center point of a dataset, which is the most representative of the entire dataset. You must come across mode , mean , and median in school. These are what the measures of central tendency include.
Here, the mode is the most frequent value , the median is the middle value, and the mean is the average. The names pretty much suggest what they do. The mode is the only measure of central tendency you can use in nominal data.
Visualizing Nominal Data
Presenting your gathered data in a visual format is called visualizing data. You can either use charts or graphs to see what your data is telling, just like we discussed in frequency distribution tables. Again, using Microsoft Excel for this would be convenient and quick.
Statistical Tests of Nominal Data
So, what comes after summarizing and visualizing data?
Yes, that’s right! Next up is testing the hypotheses so that you can actually dig deeper and see what the data is suggesting.
Two types of statistical tests you can use for testing your hypotheses are:
- Parameter Tests -used for ratio data and interval
- Non-parametric Tests -used for ordinal and nominal data
And that is a wrap for nominal data. If you have any queries or requests, please leave a comment in the comment section below.
FAQs About Nominal Data
What are the levels of measurements.
Levels of measurements or types of data show how precisely variables are recorded. The level of measurement can help you find how and to what extent the data can be evaluated. There are 4 levels of measurements, namely nominal, ordinal , interval , and ratio .
What is nominal data?
It is a type of qualitative data that divides variables into groups and categories.
How is nominal data collected?
Nominal data can be collected through surveys , interviews, online questionnaires, and so on. You can either have close-ended questions for drawing certain conclusions or have open-ended questions.
What is the difference between nominal data and ordinal data?
Though both levels of measurements are considered categorical, there is a slight difference between the two. There is no order or hierarchy in nominal data, while ordinal data can be grouped into categories following a meaningful order.
How can you analyze nominal data?
Presenting your data in a visual set-up is called visualizing data. You can either use charts or graphs to see what your data is telling, just like we discussed in frequency distribution tables. Again, using Microsoft Excel for this would be convenient and quick.
- Parameter Tests-used for ratio data and interval
- Non-parametric Tests-used for ordinal and nominal data
What is ratio data?
Ratio data , just like ordinal and interval data , can also be ranked and categorized. The intervals here are also equally spaced. The only thing that makes this one different from the rest is that ratio data has a true zero. For example, if you measure something in kgs, it is ratio data. Now, if something weighs zero, then it means that it does not weigh anything or most likely does not even exist. However, if the temperature in Fahrenheit or any other scale is zero, then that does not imply ‘no temperature.’
You May Also Like
In statistics, regression analysis is a technique used to study the relationship between an independent and dependent variable. In this method, one tries to ‘regress’ the value of ‘y,’ an dependent variable, with respect to ‘x,’ an independent variables.
A parameter in statistics implies a summary description of the characteristic of an entire population based on all the elements within it.
ANOVA is a statistical test for assessing how the levels of one or more categorical independent variables affect the changes in a quantitative dependent variable.
USEFUL LINKS
LEARNING RESOURCES
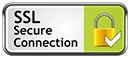
COMPANY DETAILS
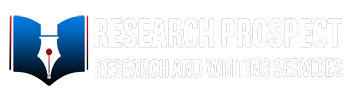
- How It Works
- Skip to main content
- Skip to primary sidebar
- Skip to footer
- QuestionPro

- Solutions Industries Gaming Automotive Sports and events Education Government Travel & Hospitality Financial Services Healthcare Cannabis Technology Use Case NPS+ Communities Audience Contactless surveys Mobile LivePolls Member Experience GDPR Positive People Science 360 Feedback Surveys
- Resources Blog eBooks Survey Templates Case Studies Training Help center

Home Market Research
Nominal Data: Definition, Characteristics, and Examples
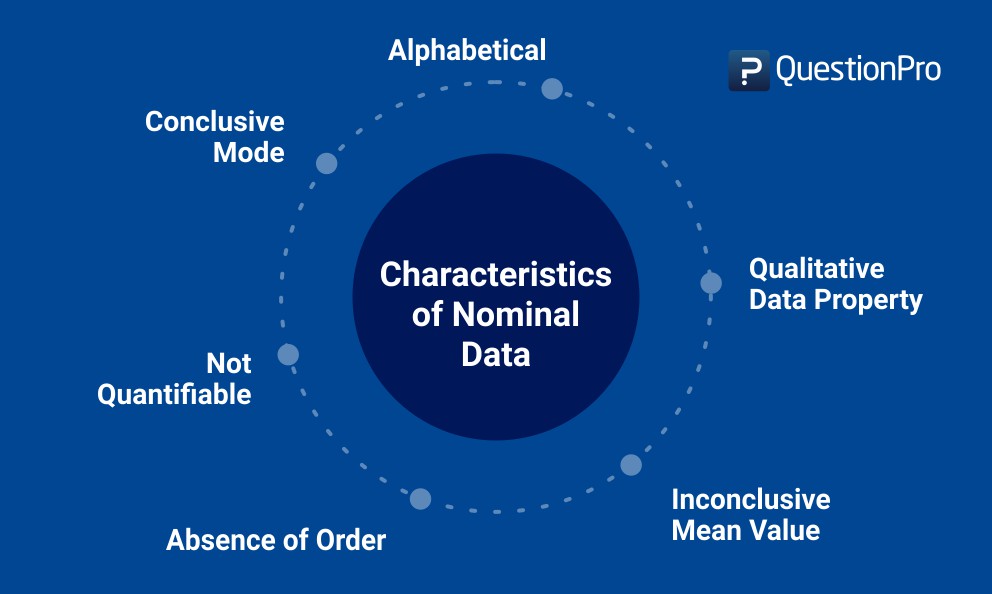
In statistical analysis , the level of measurement of variables is crucial since it influences the type of analysis possible. Nominal data provide the smallest amount of detail, while interval and ratio data provide the highest level of detail; these differences reflect the differences between the four primary levels of measurement (nominal, ordinal, interval, and ratio).
LEARN ABOUT: Level of Analysis
To have an understanding of the fundamentals of nominal data, this is the place to be. In this blog, we’ll go over the basics of this data analysis , including what it is, how to identify it, and some examples.
Content Index
What is Nominal Data?
Characteristics of nominal data, nominal data analysis, nominal data examples.
- Using QuestionPro Research Suite for Nominal Data Collection and Analysis
Nominal data is “labeled” or “named” data which can be divided into various groups that do not overlap. Data is not measured or evaluated in this case; it is just assigned to multiple groups. These groups are unique and have no common elements.
The order of the data collected can’t be established using nominal data; thus, if you change the order of data, the significance of the data will not be altered.
In Latin nomenclature, “Nomen” means – Name. Nominal data does present a similarity between the various items, but details regarding this similarity might not be disclosed. This is merely to make the data collection and analysis process easier for researchers. In some cases, it is also called “Categorical Data.”
If binary data represents “two-valued” data, this data represents “multi-valued” data, and it can’t be quantitative. It is considered to be discrete. For example, a dog can be a Labrador or not.
Learn about: Nominal Scale
Let’s discuss the characteristics of nominal data using this question:
- Central Asian
Now, its main characteristics are:
- Nominal data can never be quantified: It will always be in the form of nomenclature, i.e., a survey sent to Asian countries may include a question such as the one mentioned in this case. Here, statistical, logical, or numerical analysis of data is not possible, i.e., a researcher can’t add, subtract or multiply the collected data or conclude that variable 1 is greater than variable 2.
- Absence of order: Unlike ordinal data , nominal data can also never be assigned a definite order. In the above example, the order of answer options is irrelevant to the answers provided by the respondent.
- Qualitative property: Collected data will always have a qualitative property – answer options are highly likely to be qualitative in nature.
- Can’t calculate Mean: The mean of it can’t be established even if the data is arranged in alphabetical order. In the above-mentioned example, it is impossible for a researcher to calculate the mean of responses submitted for ethnicities because of the qualitative nature of options.
- Conclude a Mode: Asking a large sample of individuals to submit their preferences – the most common answer will be the mode. In the provided example, if Japanese is the answer submitted by a larger section of a sample, it will be the mode.
- Data is mostly alphabetical: In most cases, nominal data is alphabetical and not numerical – for example, in the mentioned case. Non-numerical data also can be categorized into various groups.
Learn more: Quantitative Data
Most nominal data is collected via questions that provide the respondent with a list of items to choose from, for example:
- Q1. Which state do you live in? ____ (followed by a drop-down list of states)
- Extra cheese
- Other (please specify) _______________
There are three ways that nominal data can be collected. In the first example, the respondent is given space to write in their home state. This is an open-ended question that will eventually be coded with each state being assigned a number. This information could also be provided to the respondent in the form of a list, where they would select one option.
The second example is in the form of multiple response questions where each category is coded 1 (if selected) and 0 if not selected. It also incorporates an open-end component allowing the respondent the option of writing in a category not included in the list. These ‘Other (please specify)’ responses’ will need coding if they are to be analyzed.
Nominal data is analyzed using percentages and the ‘mode,’ which represents the most common response(s). For a given question, there can be more than one modal response, for example, if olives and sausage were both selected the same number of times.
Multiple response questions, e.g., the pizza topping example listed above, allow researchers the ability to create a metric variable that can be used for additional analysis. In this scenario, the respondent can select any or all options providing you with a variable that ranges from zero (none selected) to the maximum number of categories. This becomes a useful tool for consumer behavioral segmentation .
Learn more: Market segmentation
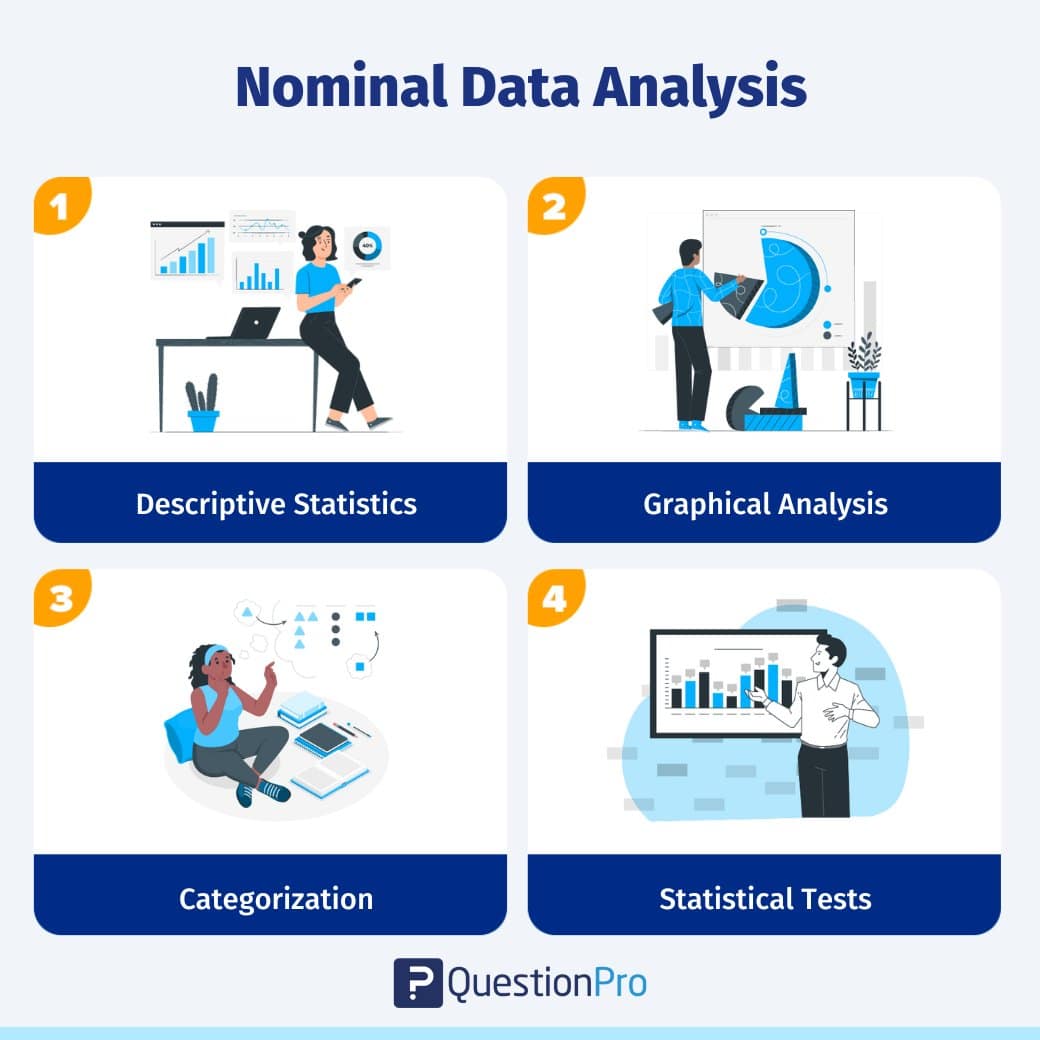
Descriptive Statistics
The distribution of the data can be determined using descriptive statistics. We can use two descriptive statistics methods for this data:
- Frequency distribution table: This is designed to organize nominal data in some order. This kind of table makes it easy to see how many responses there were for each category in the variable.
- Central tendency: This is commonly known as a mode. It serves as a measure of where the majority of values are. However, only one mode can be estimated for this data because it is only qualitative .
LEARN ABOUT: Descriptive Analysis
Graphical Analysis
The graphical analysis involves presenting the entire data in a visual format. Like descriptive statistics, visualizing your data helps you see what it is telling more easily. These methods can be used on the complete data set in the table and a sample taken from it.
- Bar Chart: The frequency of each response is graphically represented as a bar rising vertically from the horizontal axis in a bar chart, which is mostly utilized. Each bar’s height is inversely correlated with the frequency of the relevant answer.
- Pie Chart: The percentage frequency of each sample of the nominal dataset can be represented by a pie chart, which is also used.
The researcher typically uses a pie chart to represent percentages (or fractions), while a bar chart is typically used to represent distribution frequencies (mode).
Categorization of Nominal Data
Nominal data requires categorization based on similarities and differences to be properly analyzed. In this method, researchers can compare their research findings by matching them to a similar collection of data that has not been investigated.
- Matched Category: Samples from the same nominal data variable set are grouped together in the matched category. Improved statistical results are the primary goal of matching, which is accomplished by reducing the influence of confounding factors.
- Unmatched Category: Unmatched samples contain variables that are unconnected to one another. It’s a random selection from several different datasets with no commonalities.
Statistical Tests
Statistical tests allow you to test a hypothesis by delving deeper into the information that the data is revealing, whereas descriptive statistics, graphical analysis, and categorization only summarize the nominal data for straightforward analysis. In statistical analysis, distinguishing between categorical data and numerical data is essential, as categorical data involves distinct categories or labels, while numerical data consists of measurable quantities.
For nominal and ordinal data, non-parametric statistical tests are used. Therefore, you may do the popular Chi-square test when examining a nominal dataset:
- Chi-square goodness of fit test: This test determines if the sample of data is typical of the entire population of data. The test is applied when information is gathered via random sampling from a single population.
- Chi-square independence test: This examines the relationship between two nominal variables. Testing hypotheses enables determining the independence of two nominal variables from a single sample.
In each of the below-mentioned examples, there are labels associated with each of the answer options only for the purpose of labeling. For instance, in the first question, each dog breed is assigned numbers, while in the second question, both genders are assigned corresponding initials solely for convenience.
- Dalmatian – 1
- Doberman – 2
- Labrador – 3
- German Shepherd – 4
- Apartments – A
- Bungalows – B
Learn about: Types of Variable Measurement Scales
Using QuestionPro Research Suite for Nominal Data Collection and Analysis
QuestionPro Research Suite is a platform for surveys and research that may be used to examine nominal data. The platform provides numerous features and tools for data analysis, such as:
- Question Types: Question types, including single-select, multiple-select, and open-ended questions, are available in QuestionPro and can be used to gather nominal data.
- Data Collection: QuestionPro offers a variety of data collection options, including internet surveys, email invitations, and mobile surveys.
- Data Visualization: The platform offers interactive data visualization choices like pie charts and bar graphs.
- Data Analysis: The built-in data examination module in QuestionPro offers descriptive statistics for the analysis of nominal data, including frequency and percentage distribution.
- Segmentation: The platform has segmentation features that let users divide nominal data into groups based on various demographic, behavioral, or psychographic segmentations traits.
- Reports: QuestionPro offers customizable reports for summarizing and sharing findings with decision-makers.
Use QuestionPro Research Suite to collect and analyze nominal data to learn about your audience. Our platform lets you create and distribute online demographic surveys to collect age, gender, education, occupation, and more. Our data visualization tools and data analysis module will help you immediately interpret the results.
LEARN ABOUT: Average Order Value
Take this chance to improve your research skills and accomplish your objectives. Start your nominal data analysis journey right away with a free trial!
FREE TRIAL LEARN MORE
MORE LIKE THIS
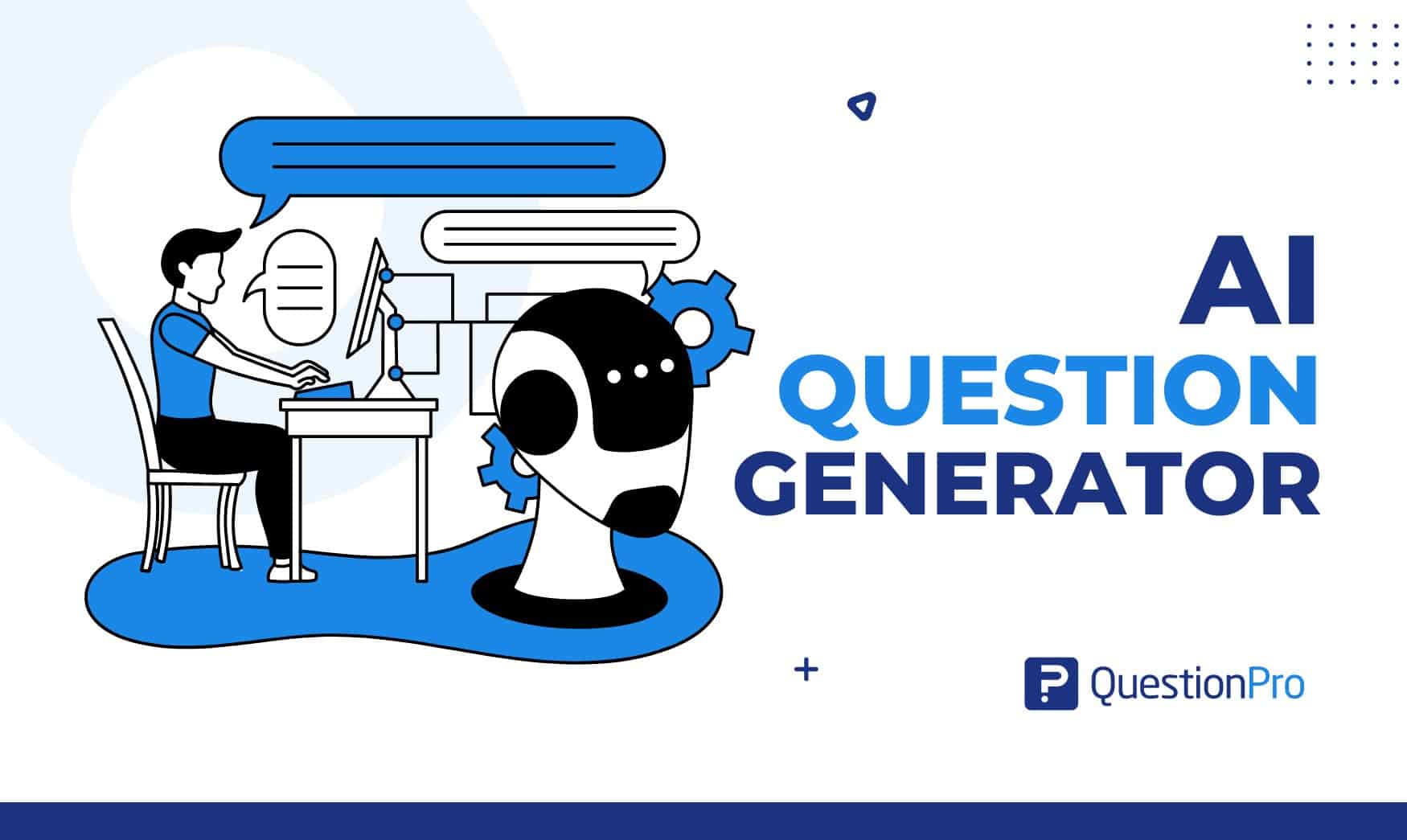
AI Question Generator: Create Easy + Accurate Tests and Surveys
Apr 6, 2024
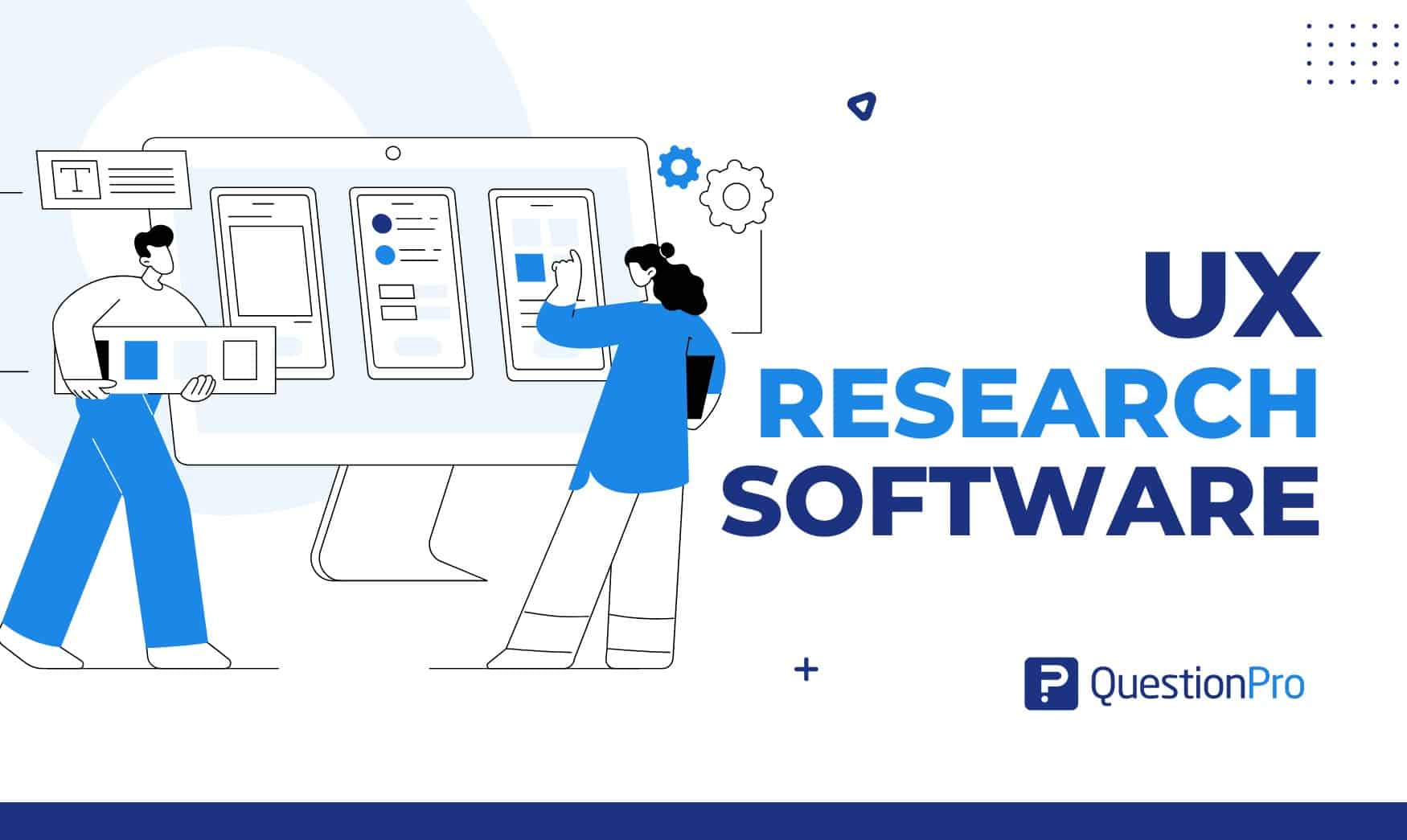
Top 17 UX Research Software for UX Design in 2024
Apr 5, 2024
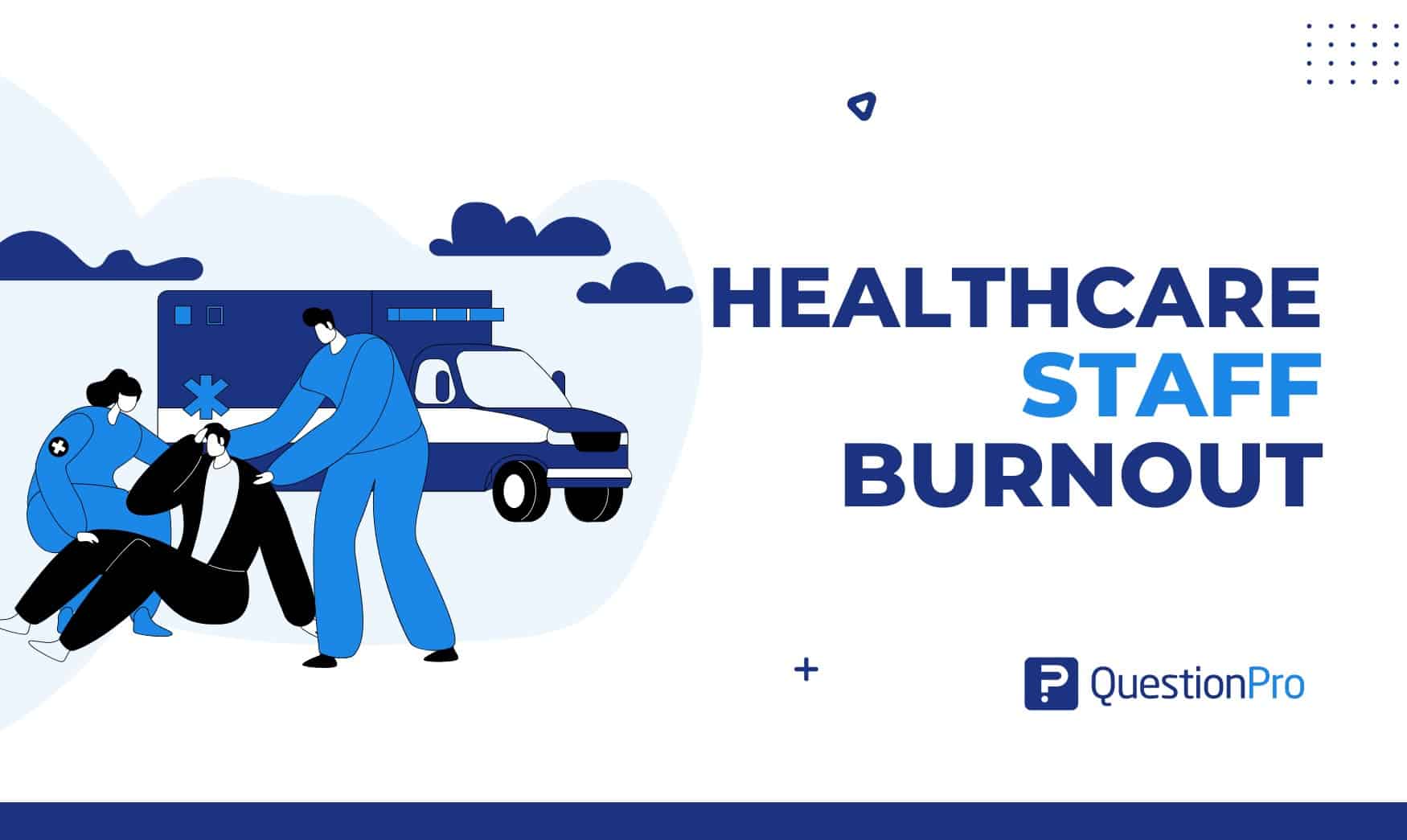
Healthcare Staff Burnout: What it Is + How To Manage It
Apr 4, 2024
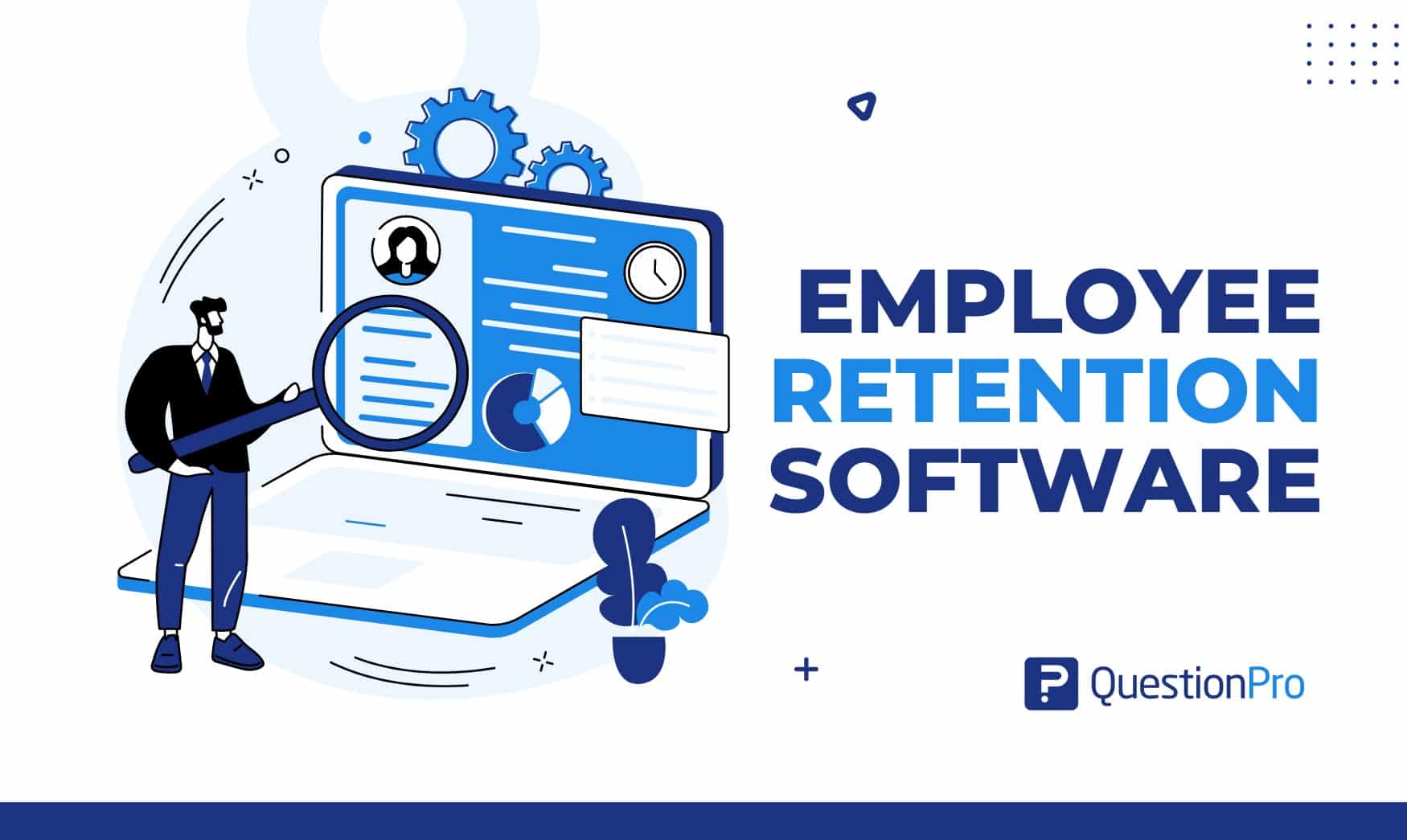
Top 15 Employee Retention Software in 2024
Other categories.
- Academic Research
- Artificial Intelligence
- Assessments
- Brand Awareness
- Case Studies
- Communities
- Consumer Insights
- Customer effort score
- Customer Engagement
- Customer Experience
- Customer Loyalty
- Customer Research
- Customer Satisfaction
- Employee Benefits
- Employee Engagement
- Employee Retention
- Friday Five
- General Data Protection Regulation
- Insights Hub
- Life@QuestionPro
- Market Research
- Mobile diaries
- Mobile Surveys
- New Features
- Online Communities
- Question Types
- Questionnaire
- QuestionPro Products
- Release Notes
- Research Tools and Apps
- Revenue at Risk
- Survey Templates
- Training Tips
- Uncategorized
- Video Learning Series
- What’s Coming Up
- Workforce Intelligence
What is Nominal Data? Definition, Characteristics, Examples
Appinio Research · 23.02.2024 · 32min read
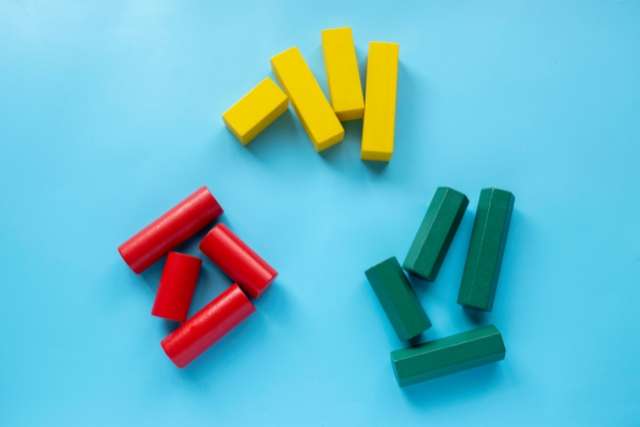
Ever wondered how researchers classify data into different categories? Nominal data holds the key to this classification puzzle. From demographic studies to market research, nominal data helps organize information into distinct groups or labels. But what exactly is nominal data, and why is it essential? In this guide, we'll explore the basics of nominal data, its characteristics, analysis techniques, and real-world applications. Whether you're a student, researcher, or data enthusiast, understanding nominal data is the first step towards unlocking valuable insights from your datasets.
What is Nominal Data?
Nominal data represents categories or labels that cannot be ordered or ranked in a meaningful way. Unlike numerical data, which can be quantified, nominal data is qualitative in nature, describing attributes rather than quantities.
Importance of Nominal Data
Nominal data holds significant importance across a wide range of disciplines and applications. Some key reasons for its importance include:
- Categorization : Nominal data allows for the classification of observations into distinct categories or groups, facilitating organization and analysis.
- Identification of Patterns : By categorizing data, nominal variables enable the identification of patterns , trends, and associations within datasets.
- Decision Making : Nominal data aids decision-making processes by providing insights into group compositions, preferences, and behaviors.
- Communication : Nominal data helps in effectively communicating information, such as demographic characteristics or group memberships, in a concise and understandable manner.
Nominal Data Characteristics and Properties
Nominal data exhibits specific characteristics and properties that distinguish it from other types of data. These characteristics include:
- Non-Numeric : Nominal data consists of categories or labels that cannot be expressed as numerical values.
- Discreteness : Each category in nominal data is discrete and mutually exclusive, with no overlap between categories.
- No Order : Unlike ordinal data, nominal data categories lack any inherent order or ranking.
- Qualitative : Nominal data describes qualitative attributes or characteristics rather than quantities or measurements.
Understanding these characteristics is essential for appropriately handling and analyzing nominal data in research and analysis.
Nominal Data Applications
Nominal data finds diverse applications across various fields and industries. Some common applications include:
- Demographic Analysis : Nominal data is frequently used in demographic studies to classify individuals based on characteristics such as age, gender, ethnicity, and education level.
- Market Research : In market research, nominal data is employed to segment consumers into distinct groups based on preferences, buying behaviors, or demographic profiles.
- Medical Diagnosis : Nominal data is used in medical diagnosis to classify patients into different diagnostic categories, such as disease types or severity levels.
- Sociological Studies : Sociologists utilize nominal data to examine social phenomena, such as group affiliations, cultural identities, or political affiliations.
These applications highlight the versatility and relevance of nominal data in understanding and interpreting various aspects of the world around us.
Understanding Nominal Variables
Nominal variables play a crucial role in data analysis, providing a framework for categorizing information into distinct groups or labels. Let's delve deeper into the concept of nominal variables, exploring their definition, examples, and how they differ from other data types.
What are Nominal Variables?
Nominal variables are categorical variables that represent distinct categories or labels within a dataset. These categories are used to classify data based on qualitative attributes rather than quantitative measurements. Unlike numerical variables, nominal variables do not have a numerical value associated with them. Instead, they serve as identifiers for different groups or characteristics.
For example, if we are conducting a survey on favorite movie genres and the categories include "Action," "Comedy," "Drama," and "Horror," each genre represents a nominal variable.
Examples of Nominal Variables
Nominal variables are prevalent in various fields and research studies. Here are some common examples:
- Gender : Male, Female, Other
- Marital Status : Single, Married, Divorced, Widowed
- Ethnicity : Caucasian, African American, Hispanic, Asian
- Educational Qualification : High School Diploma, Bachelor's Degree, Master's Degree, PhD
These examples illustrate how nominal variables are used to categorize individuals or entities based on non-quantitative attributes.
Differentiating Nominal Data from Other Data Types
Nominal data differs from other types of data, such as ordinal, interval, and ratio data, based on the level of measurement and the characteristics of the data.
- Ordinal Data : While nominal data categorizes information into distinct groups with no inherent order, ordinal data categorizes information into ordered categories where the relative position or ranking matters. For example, rating scales (e.g., "poor," "fair," "good") represent ordinal data.
- Interval Data : Interval data includes numerical values with equal intervals between them but lacks a true zero point. Unlike nominal data, interval data can be subjected to mathematical operations such as addition and subtraction. However, the zero point is arbitrary, as in the case of temperature measured in Celsius or Fahrenheit.
- Ratio Data : Ratio data possesses the properties of interval data with the addition of a true zero point, where zero represents the absence of the measured quantity. Ratio data allows for meaningful ratios and comparisons between values. Examples include height, weight, and income.
Understanding these distinctions is essential for selecting appropriate statistical methods and interpreting the results accurately in data analysis.
Data Collection Methods for Nominal Data
When it comes to collecting nominal data, researchers have several methods at their disposal, each suited to different research contexts and objectives. Let's explore these methods to understand how nominal data is gathered.
Surveys and Questionnaires
Surveys and questionnaires are popular tools for collecting nominal data, particularly in social sciences, market research, and public opinion studies. These instruments involve presenting respondents with a set of questions or statements, each accompanied by a list of predefined response options. Respondents select the option that best corresponds to their views, preferences , or characteristics.
Surveys and questionnaires offer several advantages for collecting nominal data:
- Scalability : Surveys can be administered to large samples of respondents, making them suitable for studying broad populations or demographic groups.
- Standardization : By using standardized questions and response options, researchers can ensure consistency and comparability across respondents.
- Anonymity : Respondents may feel more comfortable providing honest answers to sensitive questions when their responses are anonymous.
- Efficiency : Surveys can be conducted quickly and cost-effectively, allowing researchers to collect data efficiently.
However, surveys and questionnaires also pose some challenges, such as low response rates, response bias, and the potential to misinterpret questions.
Observational Studies
Observational studies involve systematically observing and recording behaviors, events, or phenomena in their natural settings without intervening or manipulating variables. Researchers collect nominal data by categorizing observed behaviors or characteristics into predefined categories or labels.
Observational studies offer several advantages for collecting nominal data:
- Naturalistic Setting : Observing behaviors in real-world settings allows researchers to capture authentic and spontaneous behavior.
- Flexibility : Observational studies can be adapted to various research contexts and objectives, making them suitable for studying diverse phenomena.
- Richness of Data : Observational data can provide rich, detailed insights into complex behaviors or interactions that may be challenging to capture using other methods.
However, observational studies also have limitations, including potential observer bias, lack of control over extraneous variables, and difficulties in generalizing findings to broader populations.
Experimental Studies
Experimental studies involve manipulating one or more independent variables to observe their effects on a dependent variable. While experimental studies are often associated with quantitative research, nominal data can also be collected within experimental designs by categorizing participants into groups or conditions based on qualitative attributes.
Experimental studies offer several advantages for collecting nominal data:
- Control Over Variables : Experimental designs allow researchers to manipulate variables systematically and control for confounding factors, enhancing the internal validity of the study.
- Causality : By manipulating independent variables and observing their effects on dependent variables, experimental studies can establish causal relationships.
- Replication : Experimental designs can be replicated or repeated to verify findings and ensure the reliability of results.
However, experimental studies also have limitations, including ethical constraints, potential artificiality of laboratory settings, and challenges in generalizing findings to real-world contexts.
Case Studies
Case studies involve in-depth examination and analysis of a single individual, group, organization, or event. Nominal data can be collected within case studies by categorizing attributes, characteristics, or outcomes into predefined categories or labels.
Case studies offer several advantages for collecting nominal data:
- Richness of Data : Case studies provide detailed, in-depth insights into specific cases, allowing researchers to explore complex phenomena in depth.
- Contextual Understanding : By examining individual cases within their broader contexts, case studies can provide a rich, nuanced understanding of real-world phenomena.
- Theory Development : Case studies can generate hypotheses or theories that can be further tested and refined in subsequent research.
However, case studies also have limitations, including potential bias in data collection and analysis, limited generalizability of findings, and challenges in establishing causality.
When considering the myriad of data collection methods for nominal data, it's imperative to weigh the pros and cons of each approach to ensure accurate and insightful results. Whether it's through surveys, observational studies, experimental designs, or case studies, the nuances of data collection can significantly impact the outcomes of your research endeavors.
Harnessing the power of tools like Appinio can streamline this process, allowing you to effortlessly gather real-time consumer insights and make informed decisions with confidence. Dive into the world of data-driven discovery with Appinio and revolutionize the way you conduct research. Schedule a demo today to experience the seamless integration of consumer insights into your decision-making journey!
Book a Demo
Data Representation and Measurement Scales
In data analysis, it's essential to understand different measurement scales and how they influence data representation and analysis. Let's explore the various measurement scales, including nominal, ordinal, interval, and ratio scales, and discuss their significance in data representation.
Nominal Scale
The nominal scale is the simplest form of measurement, where data is categorized into distinct labels or categories with no inherent order or ranking. Each category represents a unique attribute, but there is no quantitative significance to the labels. Nominal data is qualitative in nature and is often used to classify or categorize information.
In nominal scales:
- Categories are mutually exclusive : Each observation belongs to only one category.
- No inherent order : Categories have no natural order or hierarchy.
- Examples : Gender (male, female, other), marital status (single, married, divorced), and types of vehicles (car, truck, motorcycle).
Nominal data is typically represented using frequency counts or percentages within each category. Standard statistical analyses for nominal data include frequency distributions, chi-square tests, and cross-tabulations.
Ordinal Scale
The ordinal scale ranks data into ordered categories or levels, where the relative position or ranking of categories is meaningful. While the categories have a defined sequence, the intervals between them may not be equal or meaningful. Ordinal data retains the qualitative nature of nominal data but adds a degree of order or hierarchy.
In ordinal scales:
- Categories have a meaningful order : Categories are ranked or ordered based on their position.
- Unequal intervals : The differences between categories may not be equal or measurable.
- Examples : Educational attainment (high school diploma, bachelor's degree, master's degree), Likert scale responses (strongly agree, agree, neutral, disagree, strongly disagree).
Interval Scale
The interval scale measures data with equal intervals between consecutive points but lacks a true zero point. While the intervals between values are equal and meaningful, there is no absolute zero point that represents the absence of the measured quantity. Interval data allows for arithmetic operations such as addition and subtraction but not multiplication or division.
In interval scales:
- Equal intervals : The differences between consecutive values are equal and measurable.
- No true zero point : Zero does not represent the absence of the measured quantity.
- Examples : Temperature measured in Celsius or Fahrenheit, dates on the calendar.
Interval data is typically represented using numerical values, and common statistical analyses include mean calculations, standard deviation, and t-tests.
Ratio Scale
The ratio scale is the most informative measurement scale, featuring equal intervals between values and a true zero point where zero represents the absence of the measured quantity. Ratio data allows for meaningful ratios and comparisons between values, as well as all arithmetic operations.
In ratio scales:
- Equal intervals with a true zero point : Zero represents the absence of the measured quantity.
- Meaningful ratios : Ratios between values are meaningful and interpretable.
- Examples : Height, weight, age, income.
Ratio data is represented using numerical values, and common statistical analyses include mean calculations, standard deviation, correlations, and regression analysis.
Comparing Nominal Data with Other Measurement Scales
When comparing nominal data with other measurement scales, it's essential to recognize the qualitative nature of nominal data and its differences from ordinal, interval, and ratio scales.
- Qualitative vs. Quantitative : Nominal data represents qualitative attributes, while ordinal, interval, and ratio data represent quantitative measurements.
- Order and Hierarchy : Nominal data lacks order or hierarchy, while ordinal data has a meaningful order but unequal intervals.
- Arithmetic Operations : Unlike interval and ratio data, nominal and ordinal data cannot be subjected to arithmetic operations such as addition, subtraction, multiplication, or division.
- Statistical Analyses : Different measurement scales require different statistical analyses. Nominal data is often analyzed using non-parametric tests, while interval and ratio data can be analyzed using parametric tests.
Understanding these distinctions is crucial for selecting appropriate data representation techniques, statistical analyses, and interpretation methods in data analysis.
Data Analysis Techniques for Nominal Data
Analyzing nominal data involves various techniques to summarize, visualize, and interpret categorical information. Let's explore these techniques and understand how they contribute to gaining insights from nominal data.
Frequency Distribution
Frequency distribution is a fundamental technique for analyzing nominal data, providing a summary of the number of occurrences of each category within a dataset. It helps identify patterns, trends, and distributions within the data by counting the frequency of each category.
To Create a Frequency Distribution:
- Identify Categories : Determine the distinct categories or labels within the nominal dataset.
- Count Frequencies : Count the number of observations belonging to each category.
- Tabulate Data : Organize the frequencies into a table format, listing each category along with its corresponding frequency count.
- Visualize Data : Visualize the frequency distribution using bar charts or pie charts to enhance understanding and interpretation.
Frequency distributions provide valuable insights into the distribution of categorical variables, allowing researchers to identify dominant categories, outliers, and patterns of interest.
The mode is a measure of central tendency that represents the most frequently occurring category or value within a dataset. For nominal data, the mode is simply the category with the highest frequency count.
To Calculate the Mode:
- Identify Categories : Determine the distinct categories within the dataset.
- Count Frequencies : Calculate the frequency count for each category.
- Find the Mode : Identify the category with the highest frequency count.
The mode is particularly useful for identifying the most common or prevalent category within a dataset. It provides a simple and intuitive summary of the central tendency of nominal data.
Chi-square Test
The chi-square test is a statistical test used to determine whether there is a significant association between two categorical variables. It compares the observed frequencies of categories with the expected frequencies under the assumption of independence between the variables.
To Conduct a Chi-square Test:
- Formulate Hypotheses : Define the null hypothesis (no association) and alternative hypothesis (association) based on the research question.
- Calculate Expected Frequencies : Calculate the expected frequencies for each category under the assumption of independence.
- Compute Test Statistic : Calculate the chi-square statistic using the formula: χ^2 = ∑((Oi - Ei)^2 / Ei) Where Oi is the observed frequency, and Ei is the expected frequency for each category.
- Assess Significance : Compare the calculated chi-square statistic with the critical value from the chi-square distribution to determine statistical significance.
The chi-square test is widely used in various fields, including social sciences, market research, and epidemiology, to assess relationships between categorical variables.
Cross-tabulation
Cross-tabulation, also known as contingency table analysis, is a technique for examining the relationship between two or more categorical variables by organizing data into a table format. It allows researchers to compare the distribution of categories across different groups or conditions.
To Conduct Cross-tabulation:
- Identify Variables : Select the categorical variables of interest for cross-tabulation.
- Create Contingency Table : Construct a contingency table with rows representing one variable and columns representing another variable.
- Calculate Frequencies : Count the frequencies of observations for each combination of categories.
- Interpret Results : Analyze the patterns and associations observed in the contingency table to draw conclusions about the relationship between variables.
Cross-tabulation is a powerful tool for exploring interactions and dependencies between categorical variables, providing valuable insights into the underlying structure of the data.
Bar Charts and Pie Charts
Bar charts and pie charts are graphical representations of nominal data, visually displaying the distribution of categories within a dataset. These visualizations help researchers and stakeholders understand the relative frequencies of different categories and identify patterns or trends.
- Bar Charts : Bar charts represent categorical data using rectangular bars of varying lengths, with each bar corresponding to a category and its height proportional to the frequency count.
- Pie Charts : Pie charts display categorical data as a circular diagram divided into slices, with each slice representing a category and its size proportional to the frequency count.
Bar charts and pie charts are effective tools for communicating findings and presenting insights in a visually appealing format. They are widely used in reports, presentations, and publications to convey key messages derived from nominal data analysis.
Interpretation of Nominal Data Analysis
After analyzing nominal data, it's crucial to interpret the results accurately to draw meaningful conclusions and insights. Let's explore the interpretation of nominal data analysis in various contexts, including frequency distributions, cross-tabulations, chi-square test results, and effective communication of findings.
Drawing Conclusions from Frequency Distributions
Frequency distributions provide a summary of the number of occurrences of each category within a dataset. To draw conclusions from frequency distributions:
- Identify Dominant Categories : Determine which categories have the highest frequencies, indicating the most prevalent attributes within the dataset.
- Identify Outliers : Look for categories with unusually high or low frequencies compared to others, which may indicate unique or rare attributes.
- Identify Patterns : Analyze the distribution of categories to identify any patterns or trends, such as clustering or dispersion of data .
- Compare Subgroups : If applicable, compare frequency distributions across different subgroups or conditions to identify differences or similarities.
Interpreting frequency distributions allows researchers to gain insights into the distribution and prevalence of different attributes within the dataset, informing further analysis and decision-making.
Analyzing Patterns in Cross-tabulations
Cross-tabulations provide a means to examine the relationship between two or more categorical variables by organizing data into a table format. To analyze patterns in cross-tabulations:
- Examine Cell Counts : Review the frequencies of observations in each cell of the contingency table to identify patterns or associations.
- Calculate Percentages : Calculate row percentages, column percentages, or total percentages to compare the distribution of categories across different variables.
- Test for Independence : Use statistical tests, such as the chi-square test, to determine whether there is a significant association between variables.
Analyzing patterns in cross-tabulations helps identify relationships and dependencies between categorical variables, providing insights into the underlying structure of the data.
Interpreting Chi-square Test Results
The chi-square test is a statistical test used to determine whether there is a significant association between two categorical variables. To interpret chi-square test results:
- Compare Observed and Expected Frequencies : Review the calculated chi-square statistic and compare it to the critical value from the chi-square distribution.
- Assess Significance : Determine whether the chi-square statistic is statistically significant at a predetermined level of significance (e.g., p < 0.05).
- Interpret Effect Size : Consider the effect size measures, such as Cramer's V or Phi coefficient, to assess the strength of the association between variables.
- Examine Residuals : Analyze standardized residuals to identify specific cells contributing to the observed association.
Interpreting chi-square test results helps determine whether there is evidence of a significant association between categorical variables and provides insights into the nature and strength of the relationship.
Communicating Findings Effectively
Effective communication of findings is essential for conveying insights derived from nominal data analysis to stakeholders and decision-makers. To communicate findings effectively:
- Use Clear and Concise Language : Present findings in plain language, avoiding jargon or technical terms that may be unfamiliar to the audience.
- Use Visualizations : Utilize graphical representations, such as bar charts, pie charts, or tables, to visually illustrate key findings and trends.
- Provide Context : Offer context for the findings by explaining the significance of the results and their implications for decision-making or further research.
- Tailor Messaging to the Audience : Consider the needs and preferences of the audience when communicating findings, adapting the message to resonate with their interests and priorities.
Effective communication of findings ensures that insights derived from nominal data analysis are understood and utilized to inform decision-making and drive action.
Nominal Data Examples
Understanding nominal data is essential for various fields and industries. Let's delve into some detailed examples to grasp how nominal data manifests in different contexts.
Demographic Data
Demographic studies rely heavily on nominal data to classify individuals based on various attributes:
- Gender : Male, Female, Non-binary
- Education Level : High School Diploma, Bachelor's Degree, Master's Degree, PhD
Product Categories
In retail and market research, products are categorized into distinct groups:
- Apparel : Tops, Bottoms, Dresses, Accessories
- Food : Dairy, Produce, Meat, Frozen Foods
- Electronics : Smartphones, Laptops, Televisions, Headphones
Survey Responses
Survey data often involves nominal variables to categorize responses:
- Preferred Communication Method : Email, Phone, In-person
- Likert Scale Responses : Strongly Agree, Agree, Neutral, Disagree, Strongly Disagree
- Political Affiliation : Republican, Democrat, Independent, Other
Medical Diagnosis
Medical diagnoses are classified using nominal data to distinguish different conditions:
- Disease Status : Infected, Non-infected
- Cancer Subtypes : Breast Cancer, Lung Cancer, Prostate Cancer
- Severity Levels : Mild, Moderate, Severe
Geographic Regions
Geographic data is categorized into regions and zones:
- Continents : Africa, Asia, Europe, North America, South America
- Climate Zones : Tropical, Temperate, Polar
- Administrative Units : Countries, States, Provinces, Cities
Examining these examples gives you a deeper understanding of how nominal data is applied across various domains. Whether it's analyzing demographics, market segments, survey responses, medical conditions, or geographical regions, nominal data provides a versatile framework for classification and interpretation.
Nominal Data Challenges and Considerations
Working with nominal data presents several challenges and considerations that researchers must address to ensure accurate analysis and interpretation. These challenges include:
- Data Quality and Accuracy : Ensuring the quality and accuracy of nominal data is essential for reliable analysis. Common issues include missing values, misclassification, and data entry errors. Implementing data validation checks and cleaning procedures is vital to minimize errors and improve data quality.
- Handling Missing Values : Missing values can introduce bias and affect the validity of analysis results. Researchers must develop strategies for handling missing data, such as imputation techniques, deletion of incomplete cases, or sensitivity analysis to assess the impact of missingness on results.
- Dealing with Large Datasets : Large datasets present challenges in terms of processing power, storage, and analysis techniques. Researchers must develop strategies for efficiently managing and analyzing large volumes of nominal data, such as data sampling , parallel computing, or distributed computing frameworks.
- Addressing Bias and Confounding Factors : Bias and confounding factors can distort analysis results and lead to erroneous conclusions. Researchers must be vigilant in identifying and controlling for potential sources of bias , such as selection bias, measurement bias, or confounding variables. Strategies include randomization, blinding, and statistical adjustment techniques.
Best Practices for Handling Nominal Data
To ensure effective handling and analysis of nominal data, researchers should adhere to best practices throughout the data lifecycle. These best practices include:
- Data Cleaning and Preprocessing : Thoroughly clean and preprocess nominal data before analysis to address missing values, outliers, and inconsistencies. This may involve data validation, transformation, and normalization techniques to improve data quality and consistency.
- Choosing Appropriate Analysis Techniques : Select analysis techniques that are suitable for nominal data, such as frequency distributions, chi-square tests, or cross-tabulations. Consider the research question, data characteristics, and assumptions of the analysis techniques when choosing appropriate methods.
- Ensuring Data Privacy and Security : Protect the privacy and confidentiality of nominal data by implementing appropriate security measures, such as encryption, access controls, and anonymization techniques. Comply with data protection regulations and ethical guidelines to safeguard sensitive information.
- Documenting Data and Analysis Procedures : Maintain comprehensive documentation of nominal data and analysis procedures to ensure transparency, reproducibility, and auditability. Document data sources, variables, coding schemes, and analysis techniques to facilitate replication and validation of results.
Adhering to these best practices helps ensure the reliability, validity, and reproducibility of nominal data analysis, leading to more robust and trustworthy research outcomes.
Conclusion for Nominal Data
Nominal data plays a vital role in data analysis across various fields and industries. By categorizing information into distinct labels or categories, nominal data enables researchers to organize, analyze, and interpret complex datasets effectively. From demographic studies to market research and medical diagnosis, nominal data provides valuable insights into group characteristics, preferences , and behaviors. Understanding the basics of nominal data, including its definition, characteristics, and analysis techniques, empowers individuals to make informed decisions and draw meaningful conclusions from their data. In today's data-driven world, the importance of nominal data cannot be overstated. Whether you're conducting research, making business decisions, or simply exploring patterns in your data, nominal data serves as a fundamental building block for analysis and interpretation. By mastering the concepts and techniques outlined in this guide, you'll be equipped with the knowledge and skills needed to harness the power of nominal data and unlock actionable insights that drive success in your endeavors.
How to Collect Nominal Data in Minutes?
Discover the power of real-time consumer insights with Appinio , your go-to platform for collecting nominal data effortlessly. As a real-time market research platform, Appinio empowers businesses to make informed, data-driven decisions swiftly. With Appinio, conducting your own market research becomes a breeze, allowing you to gain valuable insights in minutes.
Here's why you should choose Appinio:
- From questions to insights in minutes: Appinio streamlines the research process, delivering actionable insights in record time.
- Intuitive platform for everyone: No need for a research PhD; our user-friendly interface ensures anyone can navigate and utilize the platform effectively.
- Extensive reach and targeting options: With access to over 90 countries and the ability to define target groups using 1200+ characteristics, Appinio ensures that you reach the right audience for your nominal data collection needs.
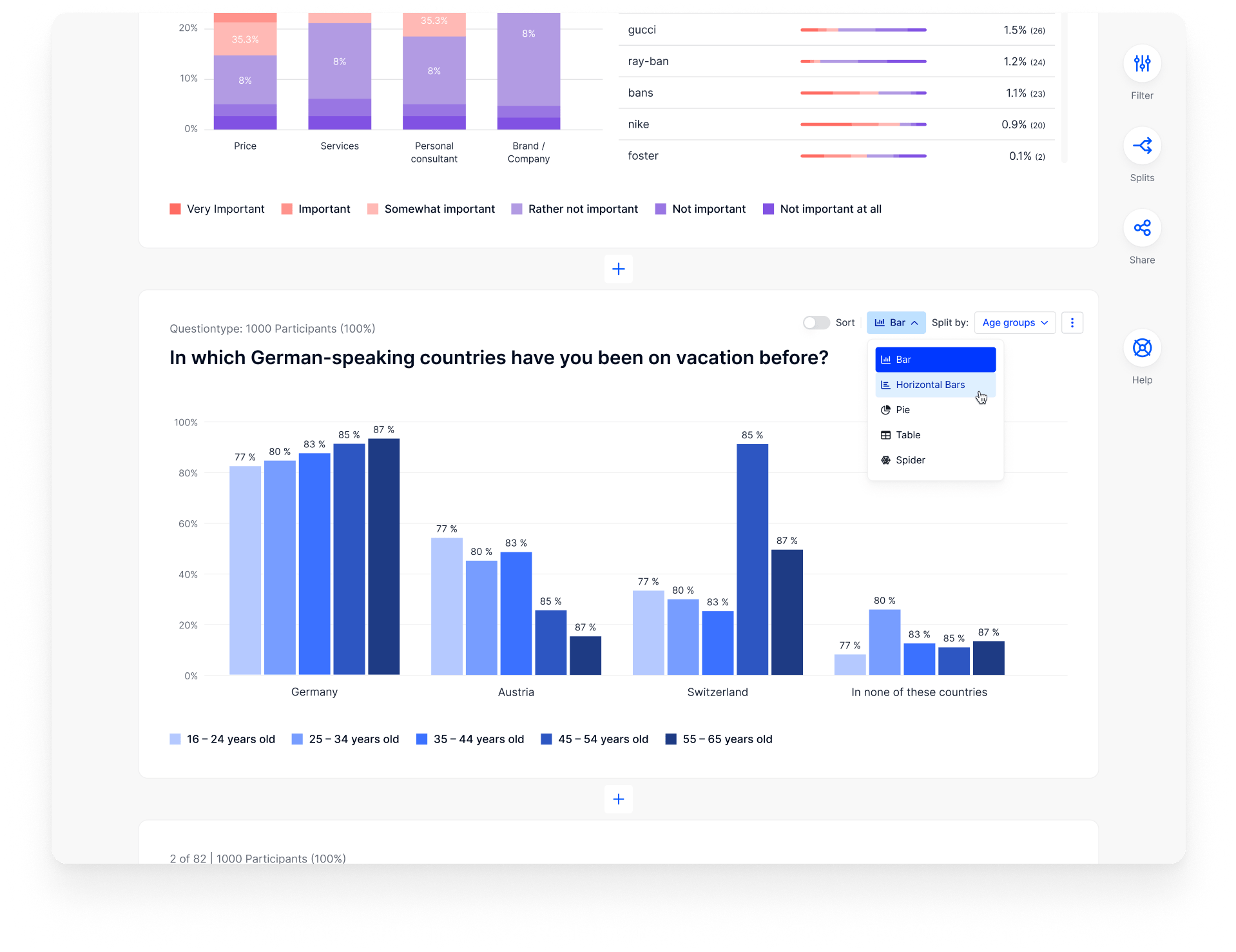
Get free access to the platform!
Join the loop 💌
Be the first to hear about new updates, product news, and data insights. We'll send it all straight to your inbox.
Get the latest market research news straight to your inbox! 💌
Wait, there's more
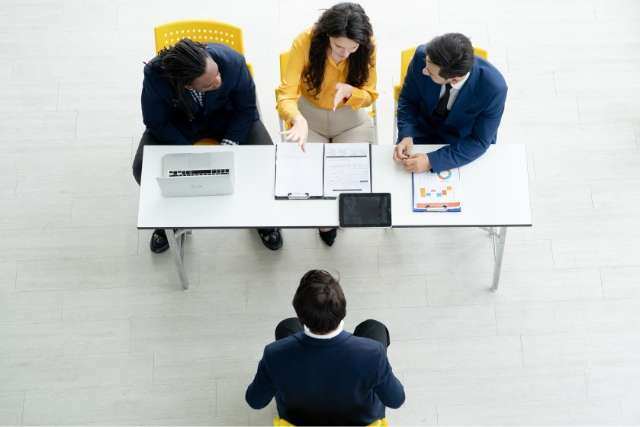
05.04.2024 | 27min read
What is Field Research? Definition, Types, Methods, Examples
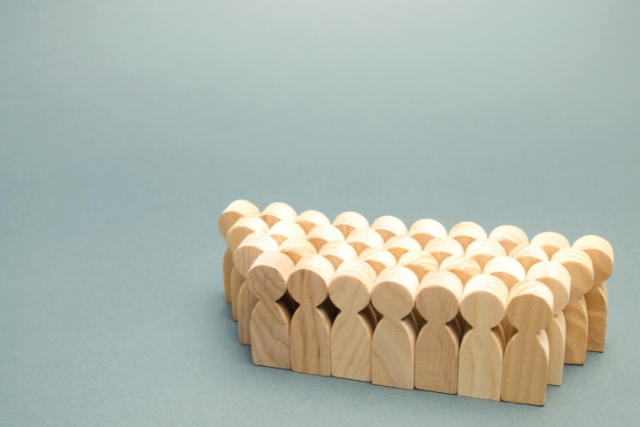
03.04.2024 | 29min read
What is Cluster Sampling? Definition, Methods, Examples
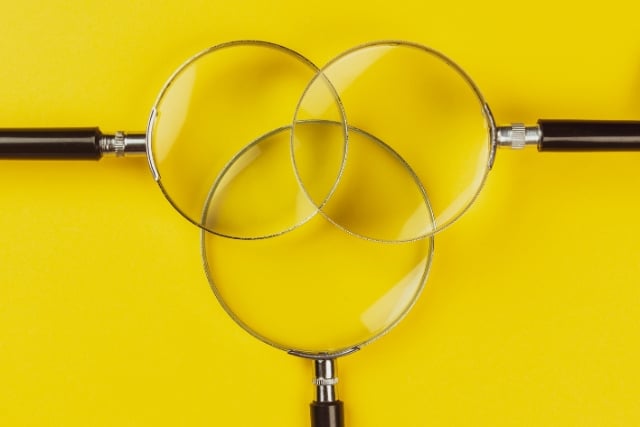
01.04.2024 | 26min read
Cross-Tabulation Analysis: A Full Guide (+ Examples)

Nominal, Ordinal, Interval & Ratio Data
Levels of measurement: explained simply (with examples).
By: Derek Jansen (MBA) | Expert Reviewed By Dr. Eunice Rautenbach | November 2020
If you’re new to the world of quantitative data analysis and statistics, you’ve most likely run into the four horsemen of levels of measurement : nominal, ordinal, interval and ratio . And if you’ve landed here, you’re probably a little confused or uncertain about them.
Don’t stress – in this post, we’ll explain nominal, ordinal, interval and ratio levels of measurement in simple terms , with loads of practical examples .
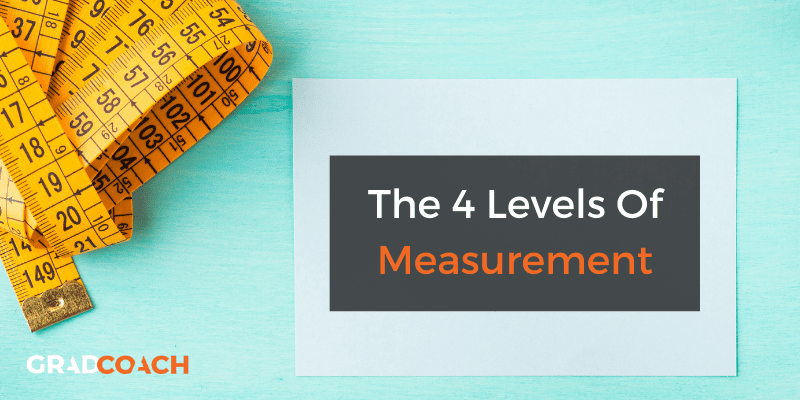
Overview: Levels of measurement
Here’s what we’ll be covering in this post. Click to skip directly to that section.
- What are levels of measurement in statistics?
- Nominal data
- Ordinal data
- Interval data
- Why does this matter?
- Recap & visual summary
Levels of Measurement 101
When you’re collecting survey data (or, really any kind of quantitative data) for your research project, you’re going to land up with two types of data – categorical and/or numerical . These reflect different levels of measurement.
Categorical data is data that reflect characteristics or categories (no big surprise there!). For example, categorical data could include variables such as gender, hair colour, ethnicity, coffee preference, etc. In other words, categorical data is essentially a way of assigning numbers to qualitative data (e.g. 1 for male, 2 for female, and so on).
Numerical data , on the other hand, reflects data that are inherently numbers-based and quantitative in nature. For example, age, height, weight. In other words, these are things that are naturally measured as numbers (i.e. they’re quantitative), as opposed to categorical data (which involves assigning numbers to qualitative characteristics or groups).
Within each of these two main categories, there are two levels of measurement:
- Categorical data – n ominal and o rdinal
- Numerical data – i nterval and r atio
Let’s take look at each of these, along with some practical examples.
Need a helping hand?
What is nominal data?
As we’ve discussed, nominal data is a categorical data type, so it describes qualitative characteristics or groups, with no order or rank between categories. Examples of nominal data include:
- Gender, ethnicity, eye colour, blood type
- Brand of refrigerator/motor vehicle/television owned
- Political candidate preference, shampoo preference, favourite meal
In all of these examples, the data options are categorical , and there’s no ranking or natural order . In other words, they all have the same value – one is not ranked above another. So, you can view nominal data as the most basic level of measurement , reflecting categories with no rank or order involved.
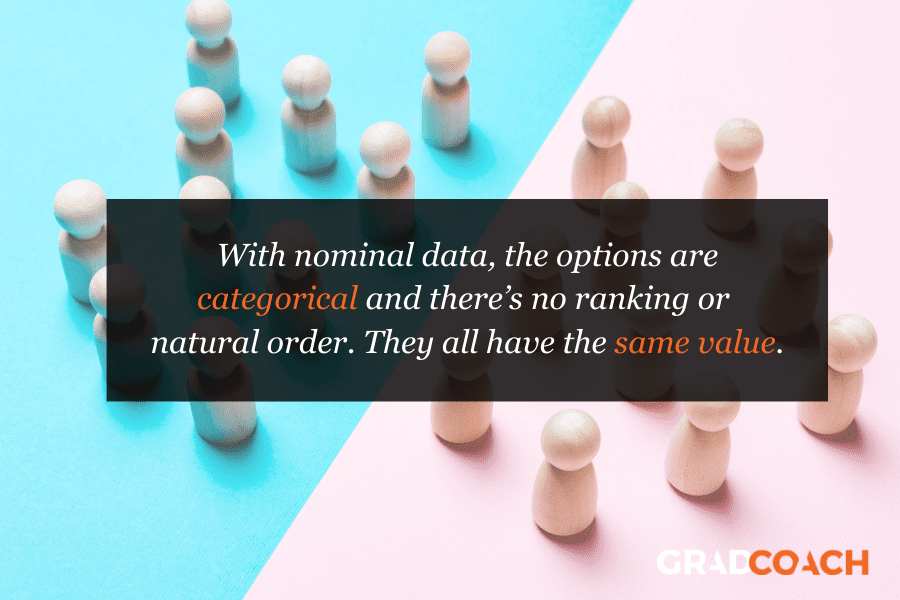
What is ordinal data?
Ordinal data kicks things up a notch. It’s the same as nominal data in that it’s looking at categories, but unlike nominal data, there is also a meaningful order or rank between the options. Here are some examples of ordinal data:
- Income level (e.g. low income, middle income, high income)
- Level of agreement (e.g. strongly disagree, disagree, neutral, agree, strongly agree)
- Political orientation (e.g. far left, left, centre, right, far right)
As you can see in these examples, all the options are still categories, but there is an ordering or ranking difference between the options . You can’t numerically measure the differences between the options (because they are categories, after all), but you can order and/or logically rank them. So, you can view ordinal as a slightly more sophisticated level of measurement than nominal.
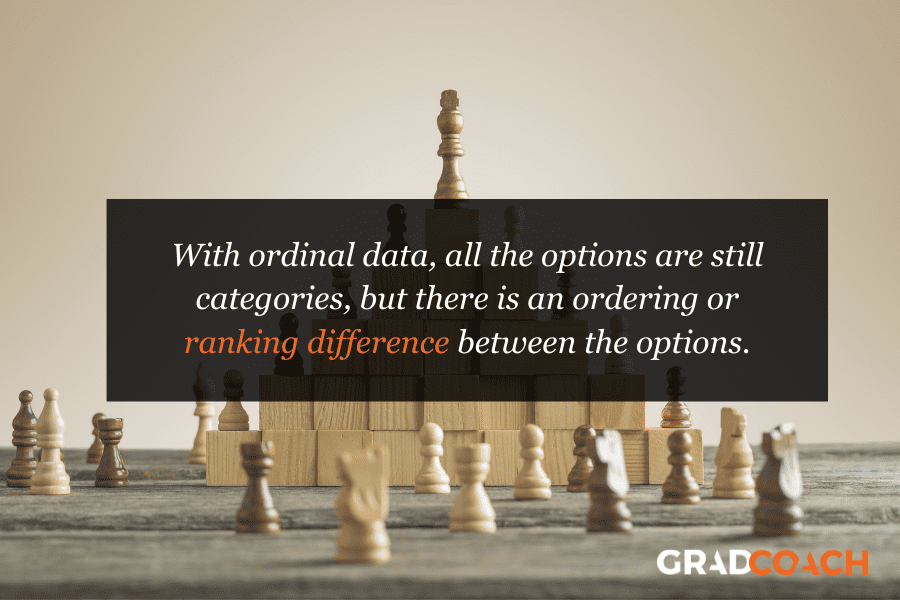
What is interval data?
As we discussed earlier, interval data are a numerical data type. In other words, it’s a level of measurement that involves data that’s naturally quantitative (is usually measured in numbers). Specifically, interval data has an order (like ordinal data), plus the spaces between measurement points are equal (unlike ordinal data).
Sounds a bit fluffy and conceptual? Let’s take a look at some examples of interval data:
- Credit scores (300 – 850)
- GMAT scores (200 – 800)
- The temperature in Fahrenheit
Importantly, in all of these examples of interval data, the data points are numerical , but the zero point is arbitrary . For example, a temperature of zero degrees Fahrenheit doesn’t mean that there is no temperature (or no heat at all) – it just means the temperature is 10 degrees less than 10. Similarly, you cannot achieve a zero credit score or GMAT score.
In other words, interval data is a level of measurement that’s numerical (and you can measure the distance between points), but that doesn’t have a meaningful zero point – the zero is arbitrary.
Long story short – interval-type data offers a more sophisticated level of measurement than nominal and ordinal data, but it’s still not perfect. Enter, ratio data…
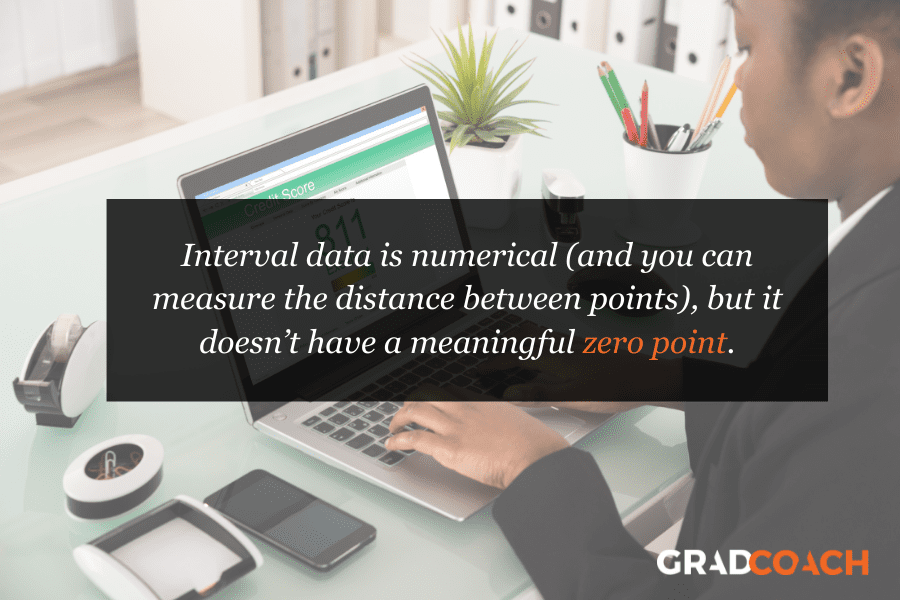
What is ratio data?
Ratio-type data is the most sophisticated level of measurement. Like interval data, it is ordered/ranked and the numerical distance between points is consistent (and can be measured). But what makes it the king of measurement is that the zero point reflects an absolute zero (unlike interval data’s arbitrary zero point). In other words, a measurement of zero means that there is nothing of that variable.
Here are some examples of ratio data:
- Weight, height, or length
- The temperature in Kelvin (since zero Kelvin means zero heat)
- Length of time/duration (e.g. seconds, minutes, hours)
In all of these examples, you can see that the zero point is absolute . For example, zero seconds quite literally means zero duration. Similarly, zero weight means weightless. It’s not some arbitrary number. This is what makes ratio-type data the most sophisticated level of measurement.
With ratio data, not only can you meaningfully measure distances between data points (i.e. add and subtract) – you can also meaningfully multiply and divide . For example, 20 minutes is indeed twice as much time as 10 minutes. You couldn’t do that with credit scores (i.e. interval data), as there’s no such thing as a zero credit score. This is why ratio data is king in the land of measurement levels.
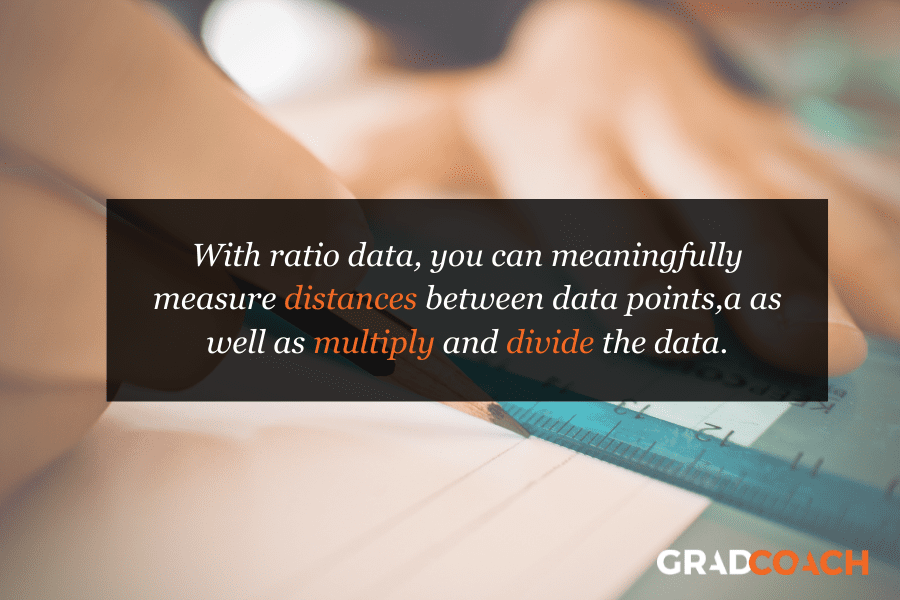
Why does it matter?
At this point, you’re probably thinking, “Well that’s some lovely nit-picking nerdery there, Derek – but why does it matter?”. That’s a good question. And there’s a good answer .
The reason it’s important to understand the levels of measurement in your data – nominal, ordinal, interval and ratio – is because they directly impact which statistical techniques you can use in your analysis. Each statistical test only works with certain types of data. Some techniques work with categorical data (i.e. nominal or ordinal data), while others work with numerical data (i.e. interval or ratio data) – and some work with a mix . While statistical software like SPSS or R might “let” you run the test with the wrong type of data, your results will be flawed at best , and meaningless at worst.
The takeaway – make sure you understand the differences between the various levels of measurement before you decide on your statistical analysis techniques. Even better, think about what type of data you want to collect at the survey design stage (and design your survey accordingly) so that you can run the most sophisticated statistical analyses once you’ve got your data.
Let’s recap.
In this post, we looked at the four levels of measurement – nominal, ordinal, interval and ratio . Here’s a visual summary of each.
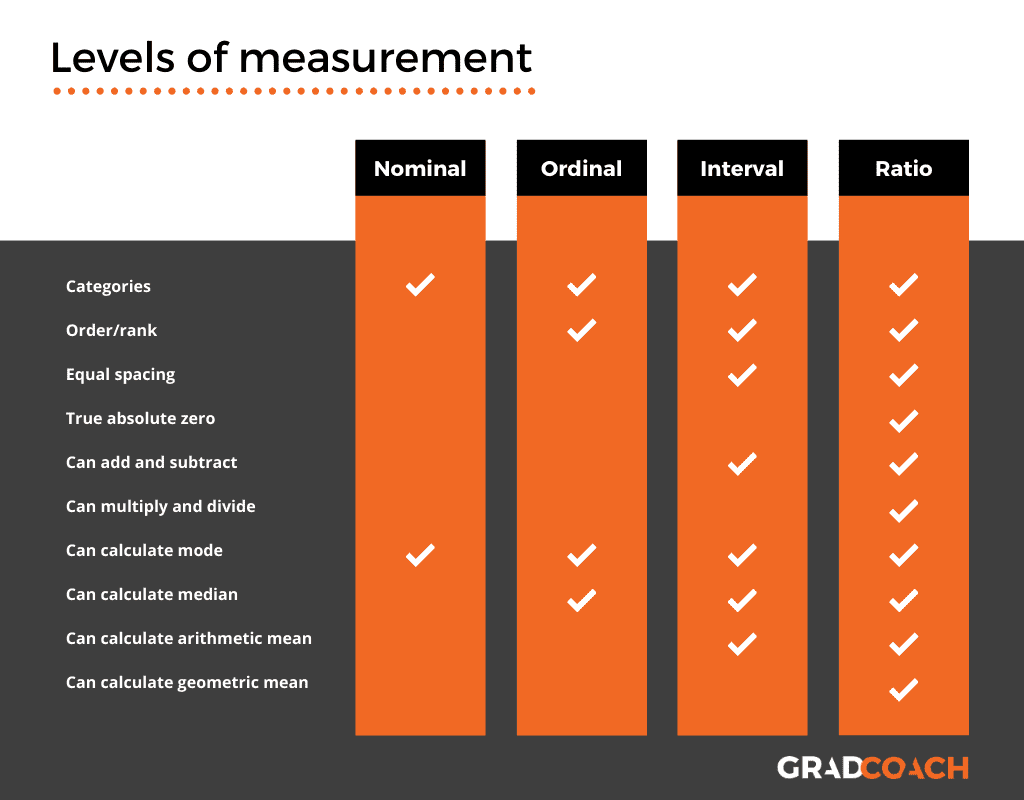
Psst… there’s more (for free)
This post is part of our dissertation mini-course, which covers everything you need to get started with your dissertation, thesis or research project.
You Might Also Like:
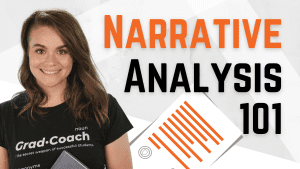
16 Comments
Clear, concise with examples. PLUS in your videos you include other names or terms that could apply to the topic you are reviewing. This is so important! You are fantastic! It has been many, many, many moons ago that these were learned. They are now necessary again as the nursing profession progresses deeper into evidence based practice.
Thanks for the feedback, Diana 🙂
Good explanation.. I came here after giving 5 marks for each question in a quiz n wondering that the data is not continuous and how to analyse it further.. Understood it is ratio and i can use mean/ median accordingly
Glad it helped!
Bloody good! You saved my homework (:
Happy to help 🙂
High quality of education stuff, thank you very much.
great knowledge shared here. I had problem understanding this at the undergraduate school but very clear now. Thanks to GRADCOACH
What type of data would age be? Ratio or interval?
It would be ratio. However, if you are using age ranges (e.g. 18 – 25, 26 – 35, etc.), this wouldn’t be the case.
What is age ranges considered?
What measurement scale is ideal to use when measuring “knowledge” and the acceptable responses are “yes,” “no,” or “not sure”? What kind of analytical test is suitable in this situation?
I watched your youtube tutorial on quantitative analysis and it was really informative.
However, I’m trying to navigate your blog to find the post that discusses the different inferential statistical methods and the data type they support.
Kindly forward this link to my email : [email protected]
Scores from a performance test are ratio data?
It is an excellent discussion about levels of measurement.
Pretty! This was an incredibly wonderful article. Thanks for providing this info.
Submit a Comment Cancel reply
Your email address will not be published. Required fields are marked *
Save my name, email, and website in this browser for the next time I comment.
- Print Friendly
Table of Contents
What is nominal data , characteristics of nominal data, nominal data analysis, examples of nominal data, nominal vs. ordinal data, frequently asked questions, choose the right program, what is nominal data definition, examples, variables & analysis.
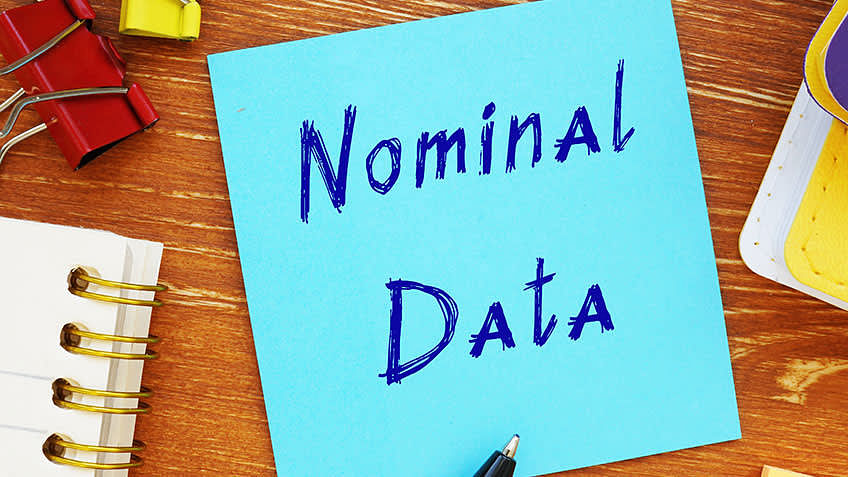
The level of measurement of variables is essential in statistical analysis because it determines how you can analyze your data. The four primary levels of measurement – nominal, ordinal, interval, and ratio provide different levels of detail – nominal provides minuscule detail, while interval and ratio give the maximum detail.
If you're interested in learning the basics of nominal data, this guide is for you. We'll define what nominal data is, look at the characteristics of nominal data, examples of nominal data, how to analyze nominal data, and nominal vs. ordinal data.
Nominal data is qualitative data used to name or label variables without providing numeric values. It is the most straightforward type of measurement scale. Nominal variables are labeled into categories that do not overlap. Unlike other data types, nominal data cannot be ordered or measured; it does not have equal spacing between values or a true zero value.
Nominal data is the foundation of statistical analysis and all other mathematical sciences. They comprise individual pieces of information recorded and used for analysis.
For instance, the preferred mode of transportation is a nominal variable since we can sort the data into mutually exclusive categories like a car, bus, train, bicycle, etc. Numbers and words may denote nominal variables, but the number labels do not have any numeric value.
The main characteristics of nominal data are:
- Nominal data are categorical, the categories being mutually exclusive without any overlap.
- The categories of nominal data are purely descriptive, that is, they do not possess any quantitative or numeric value. Nominal data can never be quantified
- Nominal data cannot be put into any definite order or hierarchy. None of the categories can be greater than or worth more than one another.
- The mean of nominal data cannot be calculated even if the data is arranged in alphabetical order.
- The mode is the only measure of central tendency for nominal data.
- In most cases, nominal data is alphabetical.
Most nominal data is collected through open or closed-ended survey questions that provide the respondent with a list of labels to choose from.
Close-ended questions are used if all data can be captured using a few possible labels.
On the other hand, if the variable selected has many possible labels, an open-ended question is preferred.
For example,
What is your ethnicity? __ (followed by a drop-down list of ethnicities)
Nominal data can be organized and visualized into tables and charts. Thereafter, you can get descriptive statistics about your data set to calculate your data's frequency distribution and central tendency.
The general steps to be taken to analyze nominal data include:
Descriptive Statistics
In this step, descriptive statistics will enable you to see how your data are distributed. The most common descriptive statistics methods for nominal data are
- Frequency Distribution – frequency distribution table is created to bring order to nominal data. Such a table clearly shows the number of responses for each category in the variable. Thus, you can use these tables to visualize data distribution through graphs and charts.
Central Tendency
it is a measure of where most of the values lie. The most commonly used measures of central tendency are the mean, median, and mode. However, since nominal data is purely qualitative, only one mode can be calculated for nominal data.
You can find the mode by identifying the most frequently appearing value in your frequency table.
Statistical Tests
Inferential statistics allow you to test scientific hypotheses about the data and dig deeper into what the data are conveying. Non-parametric tests are used for nominal data because the data cannot be ordered in any meaningful way.
Nonparametric tests used for nominal data are:
- Chi-square goodness of fit test – this test helps to assess if the sample data collected is representative of the whole data populace. The test is used when data is collected from a single population through random sampling.
- The Chi-square independence test explores the relationship between two nominal variables. Hypotheses testing allows testing whether two nominal variables from one sample are independent.
Become a Data Science & Business Analytics Professional
- 28% Annual Job Growth By 2026
- 11.5 M Expected New Jobs For Data Science By 2026
Data Analyst
- Industry-recognized Data Analyst Master’s certificate from Simplilearn
- Dedicated live sessions by faculty of industry experts
Post Graduate Program in Data Analytics
- Post Graduate Program certificate and Alumni Association membership
- Exclusive hackathons and Ask me Anything sessions by IBM
Here's what learners are saying regarding our programs:

Gayathri Ramesh
Associate data engineer , publicis sapient.
The course was well structured and curated. The live classes were extremely helpful. They made learning more productive and interactive. The program helped me change my domain from a data analyst to an Associate Data Engineer.
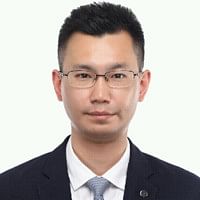
Felix Chong
Project manage , codethink.
After completing this course, I landed a new job & a salary hike of 30%. I now work with Zuhlke Group as a Project Manager.
Most nominal data is sorted into categories, where each response fits only into one category.
Some examples of nominal data are:
1. Which state do you live in? (Followed by a drop-down list of names of states)
2.Which among the following do you usually choose for pizza toppings?
- Extra Cheese
- Which is the most loved breed of dog?
- Doberman - 1
- Dalmatian - 2
- Labrador – 3
- German Shepherd – 4
3. Hair Color (black, brown, grey, blonde)
4. Preferred mode of Public Transport (bus, tram, train)
5. Employment Status (employed, unemployed, retired)
6. Literary Genre (comedy, tragedy, drama, epic, satire)
Ordinal data is a kind of qualitative data that groups variables into ordered categories. The categories have a natural order or rank based on some hierarchal scale.
The main differences between Nominal Data and Ordinal Data are:
While Nominal Data is classified without any intrinsic ordering or rank, Ordinal Data has some predetermined or natural order.
- Nominal data is qualitative or categorical data, while Ordinal data is considered “in-between” qualitative and quantitative data.
- Nominal data do not provide any quantitative value, and you cannot perform numeric operations with them or compare them with one another. However, Ordinal data provide sequence, and it is possible to assign numbers to the data. No numeric operations can be performed. But ordinal data makes it possible to compare one item with another in terms of ranking.
- Example of Nominal Data – Eye color, Gender; Example of Ordinal data – Customer Feedback, Economic Status
1. What is nominal or ordinal data?
There are four main data types or levels of measurement – nominal, ordinal, interval, and ratio. Nominal Data is qualitative data used to name or label variables without providing numeric values. It is the most straightforward form of a level of measurement.
Ordinal data is also qualitative data that groups variables into ordered categories. The categories have a natural order or rank based on some hierarchal scale, like from high to low.
2. What are nominal data statistics?
In statistics, Nominal data is qualitative data that groups variables into categories that do not overlap. Nominal data is the simplest measure level and are considered the foundation of statistical analysis and all other mathematical sciences. They are individual pieces of information recorded and used for analysis. Nominal data cannot be ordered and cannot be measured.
3. What are nominal and ordinal data examples?
1. Example of Nominal Data – Which state do you live in? (Followed by a drop-down list of names of states)
2. Example of Ordinal data – Rate education level according to:
- High School
- Post-graduate
4. What are the characteristics of nominal data?
5. which is an example of ordinal data.
An organization asks employees to rate how happy they are with their manager and peers according to the following scale:
- Extremely Happy – 1
- Unhappy – 4
- Extremely Unhappy – 5
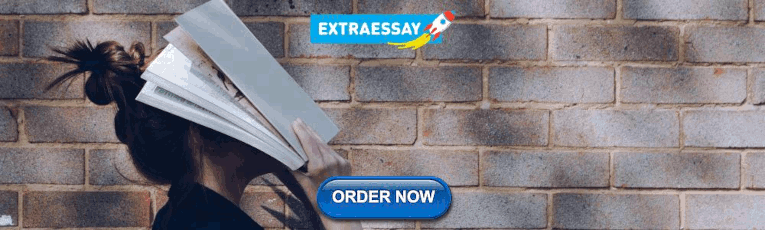
6. What is an example of nominal data?
Example of nominal data:
A real estate agent surveys to understand the answer to this question:
Which kind of houses are preferred by the residents of City X?
- Apartments -A
- Bungalows – B
Make an informed choice and accelerate your data science career by selecting the ideal program. We have meticulously curated a detailed comparison of our courses, enabling you to explore the specifics and discover the program that perfectly aligns with your goals and aspirations in the dynamic field of data science.
Program Name Data Scientist Master's Program Post Graduate Program In Data Science Post Graduate Program In Data Science Geo All Geos All Geos Not Applicable in US University Simplilearn Purdue Caltech Course Duration 11 Months 11 Months 11 Months Coding Experience Required Basic Basic No Skills You Will Learn 10+ skills including data structure, data manipulation, NumPy, Scikit-Learn, Tableau and more 8+ skills including Exploratory Data Analysis, Descriptive Statistics, Inferential Statistics, and more 8+ skills including Supervised & Unsupervised Learning Deep Learning Data Visualization, and more Additional Benefits Applied Learning via Capstone and 25+ Data Science Projects Purdue Alumni Association Membership Free IIMJobs Pro-Membership of 6 months Resume Building Assistance Upto 14 CEU Credits Caltech CTME Circle Membership Cost $$ $$$$ $$$$ Explore Program Explore Program Explore Program
This article discussed the basics of nominal data, its definition, examples, variables, and analysis. If you want to learn about these topics in more depth, our Caltech Post Graduate Program In Data Science is perfect for you. It’s also a great way to get certified by industry experts and take your career in data analytics or data science to the next level.
Data Science & Business Analytics Courses Duration and Fees
Data Science & Business Analytics programs typically range from a few weeks to several months, with fees varying based on program and institution.
Get Free Certifications with free video courses
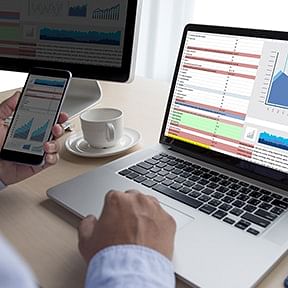
Data Science & Business Analytics
Introduction to Data Analytics Course
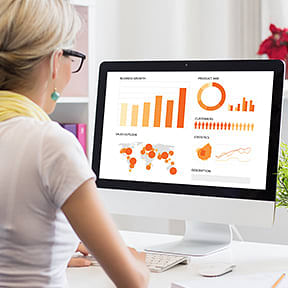
Introduction to Data Visualization
Learn from Industry Experts with free Masterclasses
Transform Your Career Path with AI & Data Science
Open Gates to a Successful Data Scientist Career in 2024 with Simplilearn Masters program
Kickstart Your Data Analytics Journey in 2024 with Caltech's Data Analytics Bootcamp
Recommended Reads
Data Science Career Guide: A Comprehensive Playbook To Becoming A Data Scientist
What Is Ordinal Data? Definition, Examples, Variables and Analysis
Data Analyst vs. Data Scientist: The Ultimate Comparison
Big Data Career Guide: A Comprehensive Playbook to Becoming a Big Data Engineer
Introduction to Data Imputation
Data Scientist vs Data Analyst vs Data Engineer: Job Role, Skills, and Salary
Get Affiliated Certifications with Live Class programs
- PMP, PMI, PMBOK, CAPM, PgMP, PfMP, ACP, PBA, RMP, SP, and OPM3 are registered marks of the Project Management Institute, Inc.
- Privacy Policy
Buy Me a Coffee

Home » Variables in Research – Definition, Types and Examples
Variables in Research – Definition, Types and Examples
Table of Contents

Variables in Research
Definition:
In Research, Variables refer to characteristics or attributes that can be measured, manipulated, or controlled. They are the factors that researchers observe or manipulate to understand the relationship between them and the outcomes of interest.
Types of Variables in Research
Types of Variables in Research are as follows:
Independent Variable
This is the variable that is manipulated by the researcher. It is also known as the predictor variable, as it is used to predict changes in the dependent variable. Examples of independent variables include age, gender, dosage, and treatment type.
Dependent Variable
This is the variable that is measured or observed to determine the effects of the independent variable. It is also known as the outcome variable, as it is the variable that is affected by the independent variable. Examples of dependent variables include blood pressure, test scores, and reaction time.
Confounding Variable
This is a variable that can affect the relationship between the independent variable and the dependent variable. It is a variable that is not being studied but could impact the results of the study. For example, in a study on the effects of a new drug on a disease, a confounding variable could be the patient’s age, as older patients may have more severe symptoms.
Mediating Variable
This is a variable that explains the relationship between the independent variable and the dependent variable. It is a variable that comes in between the independent and dependent variables and is affected by the independent variable, which then affects the dependent variable. For example, in a study on the relationship between exercise and weight loss, the mediating variable could be metabolism, as exercise can increase metabolism, which can then lead to weight loss.
Moderator Variable
This is a variable that affects the strength or direction of the relationship between the independent variable and the dependent variable. It is a variable that influences the effect of the independent variable on the dependent variable. For example, in a study on the effects of caffeine on cognitive performance, the moderator variable could be age, as older adults may be more sensitive to the effects of caffeine than younger adults.
Control Variable
This is a variable that is held constant or controlled by the researcher to ensure that it does not affect the relationship between the independent variable and the dependent variable. Control variables are important to ensure that any observed effects are due to the independent variable and not to other factors. For example, in a study on the effects of a new teaching method on student performance, the control variables could include class size, teacher experience, and student demographics.
Continuous Variable
This is a variable that can take on any value within a certain range. Continuous variables can be measured on a scale and are often used in statistical analyses. Examples of continuous variables include height, weight, and temperature.
Categorical Variable
This is a variable that can take on a limited number of values or categories. Categorical variables can be nominal or ordinal. Nominal variables have no inherent order, while ordinal variables have a natural order. Examples of categorical variables include gender, race, and educational level.
Discrete Variable
This is a variable that can only take on specific values. Discrete variables are often used in counting or frequency analyses. Examples of discrete variables include the number of siblings a person has, the number of times a person exercises in a week, and the number of students in a classroom.
Dummy Variable
This is a variable that takes on only two values, typically 0 and 1, and is used to represent categorical variables in statistical analyses. Dummy variables are often used when a categorical variable cannot be used directly in an analysis. For example, in a study on the effects of gender on income, a dummy variable could be created, with 0 representing female and 1 representing male.
Extraneous Variable
This is a variable that has no relationship with the independent or dependent variable but can affect the outcome of the study. Extraneous variables can lead to erroneous conclusions and can be controlled through random assignment or statistical techniques.
Latent Variable
This is a variable that cannot be directly observed or measured, but is inferred from other variables. Latent variables are often used in psychological or social research to represent constructs such as personality traits, attitudes, or beliefs.
Moderator-mediator Variable
This is a variable that acts both as a moderator and a mediator. It can moderate the relationship between the independent and dependent variables and also mediate the relationship between the independent and dependent variables. Moderator-mediator variables are often used in complex statistical analyses.
Variables Analysis Methods
There are different methods to analyze variables in research, including:
- Descriptive statistics: This involves analyzing and summarizing data using measures such as mean, median, mode, range, standard deviation, and frequency distribution. Descriptive statistics are useful for understanding the basic characteristics of a data set.
- Inferential statistics : This involves making inferences about a population based on sample data. Inferential statistics use techniques such as hypothesis testing, confidence intervals, and regression analysis to draw conclusions from data.
- Correlation analysis: This involves examining the relationship between two or more variables. Correlation analysis can determine the strength and direction of the relationship between variables, and can be used to make predictions about future outcomes.
- Regression analysis: This involves examining the relationship between an independent variable and a dependent variable. Regression analysis can be used to predict the value of the dependent variable based on the value of the independent variable, and can also determine the significance of the relationship between the two variables.
- Factor analysis: This involves identifying patterns and relationships among a large number of variables. Factor analysis can be used to reduce the complexity of a data set and identify underlying factors or dimensions.
- Cluster analysis: This involves grouping data into clusters based on similarities between variables. Cluster analysis can be used to identify patterns or segments within a data set, and can be useful for market segmentation or customer profiling.
- Multivariate analysis : This involves analyzing multiple variables simultaneously. Multivariate analysis can be used to understand complex relationships between variables, and can be useful in fields such as social science, finance, and marketing.
Examples of Variables
- Age : This is a continuous variable that represents the age of an individual in years.
- Gender : This is a categorical variable that represents the biological sex of an individual and can take on values such as male and female.
- Education level: This is a categorical variable that represents the level of education completed by an individual and can take on values such as high school, college, and graduate school.
- Income : This is a continuous variable that represents the amount of money earned by an individual in a year.
- Weight : This is a continuous variable that represents the weight of an individual in kilograms or pounds.
- Ethnicity : This is a categorical variable that represents the ethnic background of an individual and can take on values such as Hispanic, African American, and Asian.
- Time spent on social media : This is a continuous variable that represents the amount of time an individual spends on social media in minutes or hours per day.
- Marital status: This is a categorical variable that represents the marital status of an individual and can take on values such as married, divorced, and single.
- Blood pressure : This is a continuous variable that represents the force of blood against the walls of arteries in millimeters of mercury.
- Job satisfaction : This is a continuous variable that represents an individual’s level of satisfaction with their job and can be measured using a Likert scale.
Applications of Variables
Variables are used in many different applications across various fields. Here are some examples:
- Scientific research: Variables are used in scientific research to understand the relationships between different factors and to make predictions about future outcomes. For example, scientists may study the effects of different variables on plant growth or the impact of environmental factors on animal behavior.
- Business and marketing: Variables are used in business and marketing to understand customer behavior and to make decisions about product development and marketing strategies. For example, businesses may study variables such as consumer preferences, spending habits, and market trends to identify opportunities for growth.
- Healthcare : Variables are used in healthcare to monitor patient health and to make treatment decisions. For example, doctors may use variables such as blood pressure, heart rate, and cholesterol levels to diagnose and treat cardiovascular disease.
- Education : Variables are used in education to measure student performance and to evaluate the effectiveness of teaching strategies. For example, teachers may use variables such as test scores, attendance, and class participation to assess student learning.
- Social sciences : Variables are used in social sciences to study human behavior and to understand the factors that influence social interactions. For example, sociologists may study variables such as income, education level, and family structure to examine patterns of social inequality.
Purpose of Variables
Variables serve several purposes in research, including:
- To provide a way of measuring and quantifying concepts: Variables help researchers measure and quantify abstract concepts such as attitudes, behaviors, and perceptions. By assigning numerical values to these concepts, researchers can analyze and compare data to draw meaningful conclusions.
- To help explain relationships between different factors: Variables help researchers identify and explain relationships between different factors. By analyzing how changes in one variable affect another variable, researchers can gain insight into the complex interplay between different factors.
- To make predictions about future outcomes : Variables help researchers make predictions about future outcomes based on past observations. By analyzing patterns and relationships between different variables, researchers can make informed predictions about how different factors may affect future outcomes.
- To test hypotheses: Variables help researchers test hypotheses and theories. By collecting and analyzing data on different variables, researchers can test whether their predictions are accurate and whether their hypotheses are supported by the evidence.
Characteristics of Variables
Characteristics of Variables are as follows:
- Measurement : Variables can be measured using different scales, such as nominal, ordinal, interval, or ratio scales. The scale used to measure a variable can affect the type of statistical analysis that can be applied.
- Range : Variables have a range of values that they can take on. The range can be finite, such as the number of students in a class, or infinite, such as the range of possible values for a continuous variable like temperature.
- Variability : Variables can have different levels of variability, which refers to the degree to which the values of the variable differ from each other. Highly variable variables have a wide range of values, while low variability variables have values that are more similar to each other.
- Validity and reliability : Variables should be both valid and reliable to ensure accurate and consistent measurement. Validity refers to the extent to which a variable measures what it is intended to measure, while reliability refers to the consistency of the measurement over time.
- Directionality: Some variables have directionality, meaning that the relationship between the variables is not symmetrical. For example, in a study of the relationship between smoking and lung cancer, smoking is the independent variable and lung cancer is the dependent variable.
Advantages of Variables
Here are some of the advantages of using variables in research:
- Control : Variables allow researchers to control the effects of external factors that could influence the outcome of the study. By manipulating and controlling variables, researchers can isolate the effects of specific factors and measure their impact on the outcome.
- Replicability : Variables make it possible for other researchers to replicate the study and test its findings. By defining and measuring variables consistently, other researchers can conduct similar studies to validate the original findings.
- Accuracy : Variables make it possible to measure phenomena accurately and objectively. By defining and measuring variables precisely, researchers can reduce bias and increase the accuracy of their findings.
- Generalizability : Variables allow researchers to generalize their findings to larger populations. By selecting variables that are representative of the population, researchers can draw conclusions that are applicable to a broader range of individuals.
- Clarity : Variables help researchers to communicate their findings more clearly and effectively. By defining and categorizing variables, researchers can organize and present their findings in a way that is easily understandable to others.
Disadvantages of Variables
Here are some of the main disadvantages of using variables in research:
- Simplification : Variables may oversimplify the complexity of real-world phenomena. By breaking down a phenomenon into variables, researchers may lose important information and context, which can affect the accuracy and generalizability of their findings.
- Measurement error : Variables rely on accurate and precise measurement, and measurement error can affect the reliability and validity of research findings. The use of subjective or poorly defined variables can also introduce measurement error into the study.
- Confounding variables : Confounding variables are factors that are not measured but that affect the relationship between the variables of interest. If confounding variables are not accounted for, they can distort or obscure the relationship between the variables of interest.
- Limited scope: Variables are defined by the researcher, and the scope of the study is therefore limited by the researcher’s choice of variables. This can lead to a narrow focus that overlooks important aspects of the phenomenon being studied.
- Ethical concerns: The selection and measurement of variables may raise ethical concerns, especially in studies involving human subjects. For example, using variables that are related to sensitive topics, such as race or sexuality, may raise concerns about privacy and discrimination.
About the author
Muhammad Hassan
Researcher, Academic Writer, Web developer
You may also like

Control Variable – Definition, Types and Examples

Moderating Variable – Definition, Analysis...

Categorical Variable – Definition, Types and...

Independent Variable – Definition, Types and...

Ratio Variable – Definition, Purpose and Examples

Ordinal Variable – Definition, Purpose and...
- Nominal, Ordinal, Interval & Ratio Variable + [Examples]

- Data Collection
Measurement variables, or simply variables are commonly used in different physical science fields—including mathematics, computer science, and statistics. It has a different meaning and application in each of these fields.
In algebra, which is a common aspect of mathematics, a variable is simply referred to as an unknown value. This meaning is what is adopted in computer science, where it is used to define values when writing in various computer programming languages.
However, variables have a slightly different meaning and use in statistics. Although it also slightly intersects with algebraic meaning, its uses and definition differ greatly.
What is a Measurement Variable?
A measurement variable is an unknown attribute that measures a particular entity and can take one or more values. It is commonly used for scientific research purposes. Unlike in mathematics, measurement variables can not only take quantitative values but can also take qualitative values in statistics.
Statistical variables can be measured using measurement instruments, algorithms, or even human discretion.
How we measure variables are called scale of measurements, and it affects the type of analytical techniques that can be used on the data, and conclusions that can be drawn from it. Measurement variables are categorized into four types, namely; nominal , ordinal , interval and ratio variables.
Types of Measurement Variables
Nominal variable.
A nominal variable is a type of variable that is used to name, label or categorize particular attributes that are being measured. It takes qualitative values representing different categories, and there is no intrinsic ordering of these categories.
You can code nominal variables with numbers, but the order is arbitrary and arithmetic operations cannot be performed on the numbers. This is the case when a person’s phone number, National Identification Number postal code, etc. are being collected.
A nominal variable is one of the 2 types of categorical variables and is the simplest among all the measurement variables. Some examples of nominal variables include gender, Name, phone, etc.
Types of Nominal Variable
In statistics, there is no standard classification of nominal variables into types. However, we can classify them into different types based on some factors. We will be considering 2 factors in this case, namely; collection technique and numeric property.
Nominal Variable Classification Based on Collection Technique
There are different methods of collecting nominal variables, which may vary according to the purpose of collecting nominal data in the first place. Some of these methods include surveys, questionnaires, interviews, etc.
It doesn’t matter which method is used for data collection, one thing is however common to these methods—they are implemented using questions. The respondents are either asked, open-ended or closed-ended.
The open-ended technique gives respondents the freedom to respond the way they like. They are allowed to freely express their emotions.
This technique is used to collect detailed and descriptive information. For example, an organization who wants to receive feedback from its customers may ask, “How do you think we can improve our service?”—where the question asked is the nominal variable.
- Closed-ended
This technique restricts the kind of response a respondent can give to the questions asked. Questionnaires give predefined options for the respondent to choose from.
Unlike open-ended , this technique collects data from the questionnaire’s point of view, thereby limiting the respondent’s freedom. A closed-ended approach to the question asked above will be
How do you think we can improve our service?
- Better design
- Train chefs
- More attractive plating
Nominal Variable Classification Based on Numeric Property
Nominal variables are sometimes numeric but do not possess numerical characteristics. Some of thee numeric nominal variables are; phone numbers, student numbers, etc.
Therefore, a nominal variable can be classified as either numeric or not.
Characteristics of Nominal Variable
- The responses to a nominal variable can be divided into two or more categories. For example, gender is a nominal variable that can take responses male/female, which are the categories the nominal variable is divided into.
- A nominal variable is qualitative, which means numbers are used here only to categorize or identify objects. For example, the number at the back of a player’s jersey is used to identify the position he/she is playing.
- They can also take quantitative values. However, these quantitative values do not have numeric properties. That is, arithmetic operations cannot be performed on them.
Examples of Nominal Variable
- Personal Biodata: The variables included in a personal biodata is a nominal variable. This includes the name, date of birth, gender, etc. E.g
- Full Name _____
- Email address_____
- Customer Feedback: Organizations use this to get feedback about their product or service from customers. E.g.
How long have you been using our product?
- Less than 6 months
- What do you think about our mobile app?_____
Categories of Nominal Variable
There are 2 main categories of nominal variables, namely; the matched and unmatched categories.
- The Matched Category: In this category, all the values of the nominal variable are paired up or grouped so that each member of a group has similar characteristics except for the variable under investigation.
- The Unmatched Category: This is an independent sample of unrelated groups of data. Unlike in the matched category, the values in a group do not necessarily have similar characteristics.
Ordinal Variable
An ordinal variable is a type of measurement variable that takes values with an order or rank. It is the 2nd level of measurement and is an extension of the nominal variable.
They are built upon nominal scales by assigning numbers to objects to reflect a rank or ordering on an attribute. Also, there is no standard ordering in the ordinal variable scale .
In another sense, we could say the difference in the rank of an ordinal variable is not equal. It is mostly classified as one of the 2 types of categorical variables, while in some cases it is said to be a midpoint between categorical and numerical variables .
Types of Ordinal Variable
Similar to the nominal variable, there is no standard classification of ordinal variables into types. However, we will be classifying them according to the value assignment. I.e. Ordinal Variable type based on numerical and non numerical values.
What do we mean by value assignment?
The possible values of ordinal variables do have a rank or order, and a numeric value may be assigned to each rank for respondents to better understand them. In other cases, numeric values are not assigned to the ranks.
Below are examples of ordinal variable with and without numeric value.
Ordinal Variable With Numeric Value
How satisfied are you with our service tonight?
- Very satisfied
- Indifferent
- Dissatisfied
- Very dissatisfied
Ordinal Variable Without Numeric value
Characteristics of ordinal variable.
- It is an extension of nominal data.
- It has no standardized interval scale.
- It establishes a relative rank.
- It measures qualitative traits.
- The median and mode can be analyzed.
- It has a rank or order.
Examples of Ordinal Variable
Likert Scale: A Likert scale is a psychometric scale used by researchers to prepare questionnaires and get people’s opinions.
How satisfied are you with our service?
Interval Scale: each response in an interval scale is an interval on its own.
How old are you?
- 13-19 years
- 20-30 years
- 31-50 years
Categories of Ordinal Variable
There are also 2 main categories of ordinal variables, namely; the matched and unmatched category.
- The Matched Category: In the matched category, each member of a data sample is paired with similar members of every other sample concerning all other variables, aside from the one under consideration. This is done to obtain a better estimation of differences.
- The Unmatched Category: Unmatched category, also known as the independent category contains randomly selected samples with variables that do not depend on the values of other ordinal variables. Most researchers base their analysis on the assumption that the samples are independent, except in a few cases.
Differences Between Nominal and Ordinal Variable
- The ordinal variable has an intrinsic order while nominal variables do not have an order.
- It is only the mode of a nominal variable that can be analyzed while analysis like the median, mode, quantile, percentile, etc. can be performed on ordinal variables.
- The tests carried on nominal and ordinal variables are different.
Similarities Between Nominal and Ordinal Variable
- They are both types of categorical variables.
- They both have an inconclusive mean and a mode.
- They are both visualized using bar charts and pie charts.
Read Also: Nominal Vs Ordinal Data: 13 Key Differences & Similarities
Interval Variable
The interval variable is a measurement variable that is used to define values measured along a scale, with each point placed at an equal distance from one another. It is one of the 2 types of numerical variables and is an extension of the ordinal variable.
Unlike ordinal variables that take values with no standardized scale, every point in the interval scale is equidistant. Arithmetic operations can also be performed on the numerical values of the interval variable.
These arithmetic operations are, however, just limited to addition and subtraction. Examples of interval variables include; temperature measured in Celsius or Fahrenheit, time, generation age range, etc.
Characteristics of Interval Variable
- It is one of the 2 types of quantitative variables. It takes numeric values and may be classified as a continuous variable type.
- Arithmetic operations can be performed on interval variables. However, these operations are restricted to only addition and subtraction.
- The interval variable is an extension of the ordinal variable. In other words, we could say interval variables are built upon ordinary variables.
- The intervals on the scale are equal in an interval variable. The scale is equidistant.
- The variables are measured using an interval scale, which not only shows the order but also shows the exact difference in the value.
- It has no zero value.
Examples of Interval Variable
- Temperature: Temperature, when measured in Celsius or Fahrenheit is considered as an interval variable.
- Mark Grading: When grading test scores like the SAT, for example, we use numbers as a reference point.
- Time: Time, if measured using a 12-hour clock, or it is measured during the day is an example of interval data .
- IQ Test: An individual cannot have a zero IQ, therefore satisfying the no zero property of an interval variable. The level of an individual’s IQ will be determined, depending on which interval the score falls in.
- CGPA : This is an acronym for Cumulative Grade Point Average. It is used to determine a student’s class of degree, which depends on the interval a student’s point falls in.
- Test: When grading test scores like the SAT, for example, the numbers from 0 to 200 are not used when scaling the raw score to the section score. In this case, absolute zero is not used as a reference point. Therefore, it is an interval the score is an interval variable.
Categories of Interval Variable
There are 2 main categories of interval variables, namely; normal distribution and non-normal distributions.
- Normal Distribution: It is also called Gaussian distribution and is used to represent real-valued random variables with unknown distribution. This can be further divided into matched and unmatched samples
- Non-Normal Distribution: It can also be called the Non-Gaussian distribution, and is used to represent real-valued random variables with known distribution. It can also be further divided into matched and unmatched samples.
Ratio Variable
The ratio variable is one of the 2 types of continuous variables, where the interval variable is the 2nd. It is an extension of the interval variable and is also the peak of the measurement variable types.
The only difference between the ratio variable and interval variable is that the ratio variable already has a zero value. For example, temperature, when measured in Kelvin is an example of ratio variables.
The presence of a zero-point accommodates the measurement in Kelvin. Also, unlike the interval variable multiplication and division operations can be performed on the values of a ratio variable.
Characteristics of Ratio Variable
- Ratio variables have absolute zero characteristics. The zero point makes is what makes it possible to measure multiple values and perform multiplication and division operations. Therefore, we can say that an object is twice as big or as long as another.
- It has an intrinsic order with an equidistant scale. That is, all the levels in the ratio scale has an equal distance.
- Due to the absolute point characteristics of a ratio variable, it doesn’t have a negative number like an interval variable. Therefore, before measuring any object on a ratio scale, researchers need to first study if it satisfies all the properties of an interval variable and also the zero point characteristic.
- Ratio variable is the peak type of measurement variable in statistical analysis. It allows for the addition, interaction, multiplication, and division of variables.
Also, all statistical analysis including mean, mode, median, etc. can be calculated on the ratio scale.
Examples of Ratio Variable
Here are some examples of ratio variables according to their uses:
- Multiple Choice Questions
Multiple choice questions are mostly used for academic testing and ratio variables are sometimes used in this case. Especially for mathematics tests, or word problems we see many examples of ratio variables.
E.g. If Frank is 20 years old and Paul is twice as old as Frank. How old will Paul be in the next 10 years?
- Surveys/Questionnaires
Organizations use this tool whenever they want to get feedback about their product or service, perform market research, and competitive analysis. They use ratio variables to collect relevant data from respondents.
How much time do you spend on the internet daily?
- Less than 2 hours
- More than 6 hours
- Measurement
When registering for National passport, National ID Card, etc. there is always a need to profile applicants. As part of this profiling, a record of the applicant’s height, weight, etc. is usually taken.
What is your height in feet and inches?
- Less than 5ft
- 5ft 1inch – 5ft 4Inches
- 5ft 5Inches – 5ft 9Inches
- 6ft and above
E.g.2. What is your weight in kgs?
- Less than 50 kgs
- More than 110 kgs
Categories of Ratio Variable
The categories of ratio variables are the same as that of interval variables. Ratio variables are also classified into Gaussian and Non-Gaussian distributions.
They are both further divided into matched and unmatched samples.
The classification of variables according to their measurement type is very useful for researchers in concluding which analytical procedure should be used. It helps to determine the kind of data to be collected, how to collect it and which method of analysis should be used.
For a nominal variable, it is quite easy to collect data through open-ended or closed-ended questions . However, there is also a lot of downsides to this, as nominal data is the simplest data type and as such has limited capabilities.
Ratio variable, on the other hand, is the most complex of the measurement variables and as such can be used to perform the most complex analysis. Even at that, it may be unnecessarily complex times and one of the other variable types will be a better option.
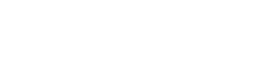
Connect to Formplus, Get Started Now - It's Free!
- examples of nominal variable
- interval variable examples
- interval variables
- measurement variable
- nominal ordinal interval ratio variable
- nominal variables
- ordinal variable
- ordinal variable example
- busayo.longe
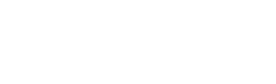
You may also like:
What is Nominal Data? + [Examples, Variables & Analysis]
This is a complete guide on nominal data, its examples, data collection techniques, category variables and analysis.

Categorical Data: Definition + [Examples, Variables & Analysis]
A simple guide on categorical data definitions, examples, category variables, collection tools and its disadvantages
What is Interval Data? + [Examples, Variables & Analysis]
An ultimate guide on interval data examples, category variables, analysis and interval scale of measurement
Brand vs Category Development Index: Formula & Template
In this article, we are going to break down the brand and category development index along with how it applies to all brands in the market.
Formplus - For Seamless Data Collection
Collect data the right way with a versatile data collection tool. try formplus and transform your work productivity today..
Nominal Variable
A nominal variable is a type of categorical variable that can have two or more categories. However, there is no ordering within these categories. A nominal variable does not have any numerical characteristics and is qualitative in nature. If a variable has a proper numerical ordering then it is known as an ordinal variable.
A nominal variable can be coded but arithmetic operations cannot be performed on them. In other words, nominal variables cannot be quantified. In this article, we will learn more about a nominal variable, a nominal scale and several associated examples.
What is a Nominal Variable?
A nominal variable along with a dichotomous and an ordinal variable form the three types of categorical variables. A dichotomous variable is a subtype of a nominal variable that can have only two levels or categories. An ordinal variable on the other hand can have two or more categories, however, these can be ranked or ordered. Apart from categorical variables, other types of variables such as interval and ratio variables are also used.
Nominal Variable Definition
A nominal variable can be defined as a categorical variable in which the categories cannot be ordered. A nominal variable might be numeric in nature but it cannot have any numerical properties. This type of variable is assigned to nominal data as such type of data is non-numerical.
Nominal Variable Examples
An example of a nominal variable is hair color. This is because hair can be of different colors such as blonde, black, brown, red, etc. These categories cannot be ordered and neither can any operations be performed.
An example of a nominal variable is a person being asked if she owns a Macbook. The answer can either be yes or no. Thus, Macbook ownership can be categorized as either yes or no.
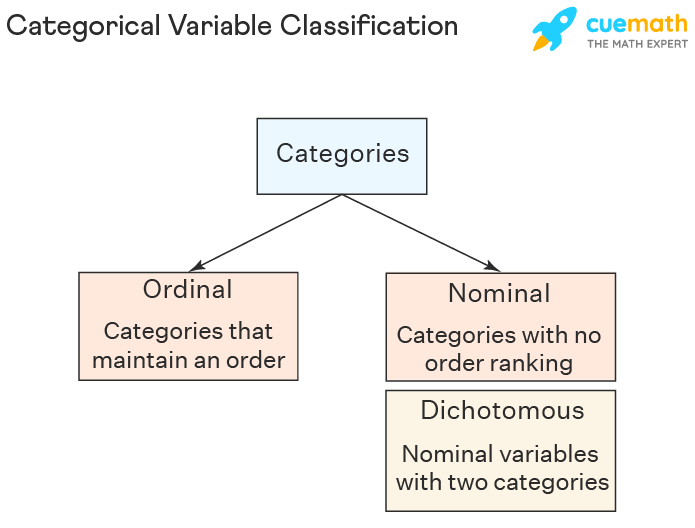
Nominal Scale
A nominal scale is a level of measurement where only qualitative variables are used. On such a scale, only tags or labels can classify objects. There are three other scales that are used for measurement levels - ordinal, interval, and ratio. A nominal variable is part of a nominal scale. In case a number is assigned to an object on a nominal scale there is a strict one-to-one correlation between the object and the corresponding numerical value. Thus, the variables in such a scale have no numeric property.
Nominal Scale Examples
An example of a nominal scale is categorizing dogs on the basis of their breeds (E.g. German shepherd, Husky, Samoyed, etc.).
Another example of a nominal scale is putting cities into states. (E.g. Seattle is in Washington).
Characteristics of Nominal Variable
The two main important characteristics of nominal variables are given as follows:
- Even though a nominal variable can take on numeric values, however, they cannot be quantified. In other words, arithmetic and logical operations cannot be performed on a nominal variable.
- The categories under nominal variables cannot be assigned a rank thereby, they cannot be ordered. Thus, a nominal variable is qualitative in nature.
Nominal Variable Types
A nominal variable can be classified either based on the collection technique or based on the numeric property. The nominal variable types are given as follows:
- Open-Ended Nominal Variable - When participants are asked open-ended questions such that they are free to respond in any way they like it is known as a nominal variable. For example, "How can a teacher improve his teaching methods?" is an open-ended nominal variable. Information collected using this variable is usually very detailed.
- Closed-Ended Nominal Variable - When the response of participants to a question has been restricted then such a question forms a closed-ended nominal variable. "How can a teacher improve his teaching methods? a) Acquiring better knowledge, b) Improving communication, c) Demonstrating flexibility. This is an example of a close-ended approach.
- Numeric and Non-Numeric Nominal Variable - A numeric nominal variable can take on a quantitative value but does not have any numeric property. For example, phone numbers. A non-numeric nominal variable neither takes on a numerical value nor does it have any numeric property. For example, an open-ended / closed-ended question.
Nominal Vs Ordinal Variable
A nominal and an ordinal variable are types of categorical variables. Both variables are qualitative in nature. The table given below highlights the main differences between nominal and ordinal variables.
Related Articles:
- Data Handling
- Data Collection
- Categorical Data
Important Notes on Nominal Variable
- A nominal variable is a categorical variable that does not have any intrinsic ordering or ranking.
- Such a variable is qualitative in nature and arithmetic or logical operations cannot be performed on it.
- A nominal variable follows a nominal scale of measurement.
- The types of nominal variables are open-ended, closed-ended, numeric, and non-numeric variables.
Examples on Nominal Variable
Example 1: How can a restaurant service be improved?
a) Improving menu
b) Changing the chef
c) Better Decor
What type of nominal variable is this?
Solution: As the question is in the form of multiple-choice thus, it is a closed-ended nominal variable. Furthermore, as there is no associated numeric value thus, it is a non-numeric nominal variable.
Answer: Close-ended non-numeric nominal variable.
Example 2: How satisfied are you with the course curriculum?
1. Dissatisfied
2. Satisfied
3. Very Satisfied
Is this a nominal variable?
Solution: As the replies to the question can be ranked hence, this is not a nominal variable. It is an ordinal variable.
Answer: Not a nominal variable
Example 3: Is a personal bio-data (name, gender, date of birth) a nominal variable?
Solution: Yes, because the categories cannot be ranked and do not possess numeric properties.
go to slide go to slide go to slide
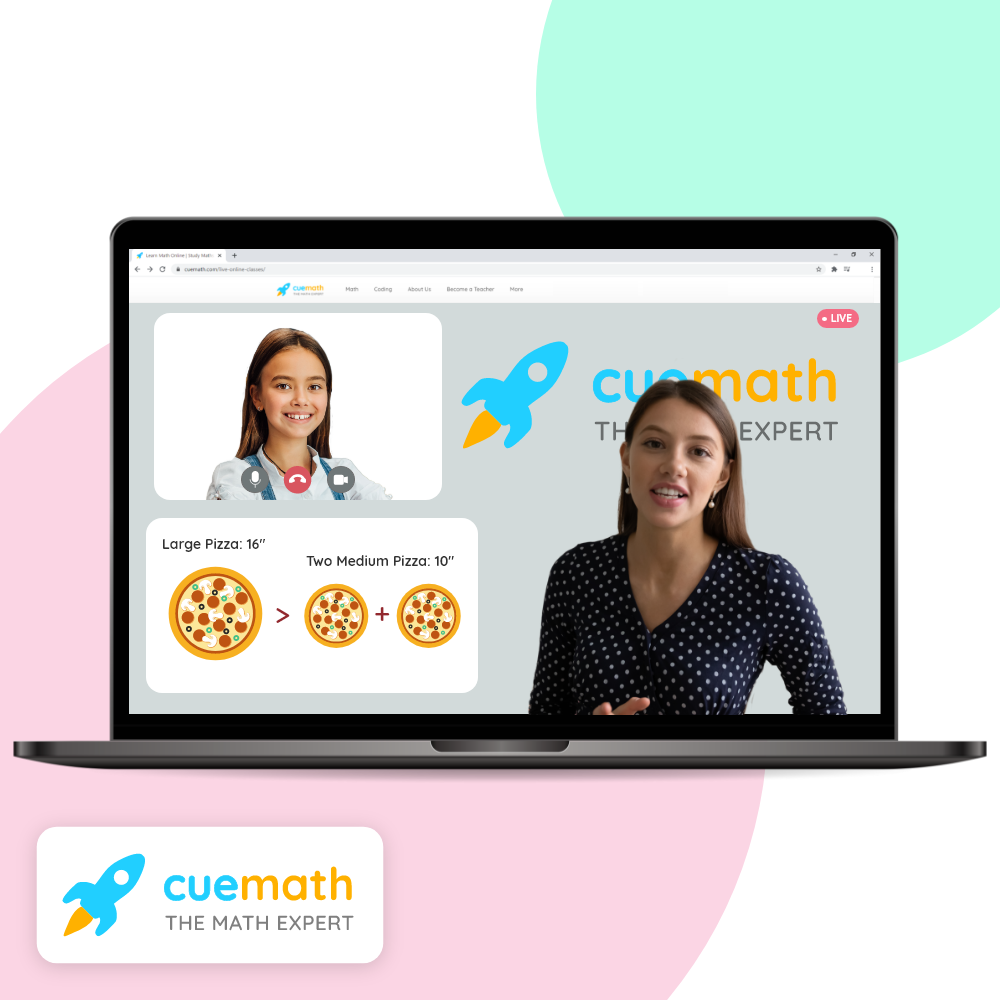
Book a Free Trial Class
FAQs on Nominal Variable
A variable consisting of categories that cannot be ranked or ordered is known as a nominal variable. A nominal variable cannot be quantitative.
Is a Nominal Variable a Type of Categorical Variable?
Yes, a nominal variable is a type of categorical variable. Other types of categorical variables are ordinal variables and dichotomous variables.
Is a Nominal Variable Qualitative in Nature?
Yes, a nominal variable is qualitative in nature. This means that arithmetic operations and logical operations cannot be performed on a nominal variable.
What Measurement Level is Followed by a Nominal Variable?
A nominal scale is the level of measurement used by a nominal variable. Such a scale is qualitative in nature and uses labels and tags to categorize data.
What is the Difference Between an Open-Ended and Closed-Ended Nominal Variable?
An open-ended nominal variable lets the participant respond freely while a closed-ended nominal variable is usually in the form of multiple-choice questions and restricts the participant's views.
Can a Nominal Variable Be Numeric?
Yes, a nominal variable can be in the form of a number however, it will not have any quantitative property. Thus, arithmetic operations cannot be performed on such a variable.
What is the Difference Between an Ordinal and a Nominal Variable?
In an ordinal variable the categories can be ranked and ordered however, in a nominal variable no ranking is possible.
Have a language expert improve your writing
Run a free plagiarism check in 10 minutes, automatically generate references for free.
- Knowledge Base
- Methodology
- Types of Variables in Research | Definitions & Examples
Types of Variables in Research | Definitions & Examples
Published on 19 September 2022 by Rebecca Bevans . Revised on 28 November 2022.
In statistical research, a variable is defined as an attribute of an object of study. Choosing which variables to measure is central to good experimental design .
You need to know which types of variables you are working with in order to choose appropriate statistical tests and interpret the results of your study.
You can usually identify the type of variable by asking two questions:
- What type of data does the variable contain?
- What part of the experiment does the variable represent?
Table of contents
Types of data: quantitative vs categorical variables, parts of the experiment: independent vs dependent variables, other common types of variables, frequently asked questions about variables.
Data is a specific measurement of a variable – it is the value you record in your data sheet. Data is generally divided into two categories:
- Quantitative data represents amounts.
- Categorical data represents groupings.
A variable that contains quantitative data is a quantitative variable ; a variable that contains categorical data is a categorical variable . Each of these types of variable can be broken down into further types.
Quantitative variables
When you collect quantitative data, the numbers you record represent real amounts that can be added, subtracted, divided, etc. There are two types of quantitative variables: discrete and continuous .
Categorical variables
Categorical variables represent groupings of some kind. They are sometimes recorded as numbers, but the numbers represent categories rather than actual amounts of things.
There are three types of categorical variables: binary , nominal , and ordinal variables.
*Note that sometimes a variable can work as more than one type! An ordinal variable can also be used as a quantitative variable if the scale is numeric and doesn’t need to be kept as discrete integers. For example, star ratings on product reviews are ordinal (1 to 5 stars), but the average star rating is quantitative.
Example data sheet
To keep track of your salt-tolerance experiment, you make a data sheet where you record information about the variables in the experiment, like salt addition and plant health.
To gather information about plant responses over time, you can fill out the same data sheet every few days until the end of the experiment. This example sheet is colour-coded according to the type of variable: nominal , continuous , ordinal , and binary .
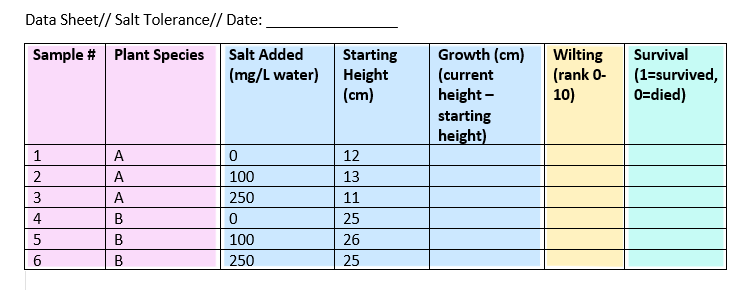
Prevent plagiarism, run a free check.
Experiments are usually designed to find out what effect one variable has on another – in our example, the effect of salt addition on plant growth.
You manipulate the independent variable (the one you think might be the cause ) and then measure the dependent variable (the one you think might be the effect ) to find out what this effect might be.
You will probably also have variables that you hold constant ( control variables ) in order to focus on your experimental treatment.
In this experiment, we have one independent and three dependent variables.
The other variables in the sheet can’t be classified as independent or dependent, but they do contain data that you will need in order to interpret your dependent and independent variables.
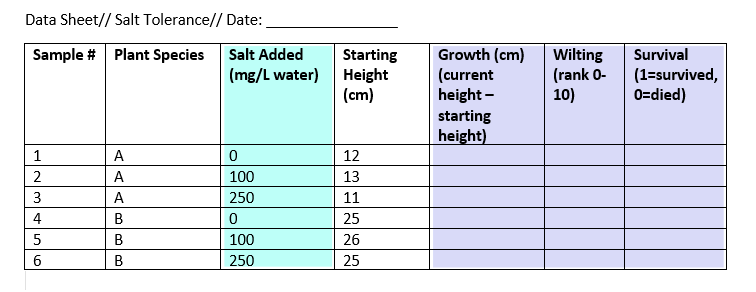
What about correlational research?
When you do correlational research , the terms ‘dependent’ and ‘independent’ don’t apply, because you are not trying to establish a cause-and-effect relationship.
However, there might be cases where one variable clearly precedes the other (for example, rainfall leads to mud, rather than the other way around). In these cases, you may call the preceding variable (i.e., the rainfall) the predictor variable and the following variable (i.e., the mud) the outcome variable .
Once you have defined your independent and dependent variables and determined whether they are categorical or quantitative, you will be able to choose the correct statistical test .
But there are many other ways of describing variables that help with interpreting your results. Some useful types of variable are listed below.
A confounding variable is closely related to both the independent and dependent variables in a study. An independent variable represents the supposed cause , while the dependent variable is the supposed effect . A confounding variable is a third variable that influences both the independent and dependent variables.
Failing to account for confounding variables can cause you to wrongly estimate the relationship between your independent and dependent variables.
Discrete and continuous variables are two types of quantitative variables :
- Discrete variables represent counts (e.g., the number of objects in a collection).
- Continuous variables represent measurable amounts (e.g., water volume or weight).
You can think of independent and dependent variables in terms of cause and effect: an independent variable is the variable you think is the cause , while a dependent variable is the effect .
In an experiment, you manipulate the independent variable and measure the outcome in the dependent variable. For example, in an experiment about the effect of nutrients on crop growth:
- The independent variable is the amount of nutrients added to the crop field.
- The dependent variable is the biomass of the crops at harvest time.
Defining your variables, and deciding how you will manipulate and measure them, is an important part of experimental design .
Cite this Scribbr article
If you want to cite this source, you can copy and paste the citation or click the ‘Cite this Scribbr article’ button to automatically add the citation to our free Reference Generator.
Bevans, R. (2022, November 28). Types of Variables in Research | Definitions & Examples. Scribbr. Retrieved 8 April 2024, from https://www.scribbr.co.uk/research-methods/variables-types/
Is this article helpful?
Rebecca Bevans
Other students also liked, a quick guide to experimental design | 5 steps & examples, quasi-experimental design | definition, types & examples, construct validity | definition, types, & examples.

An official website of the United States government
The .gov means it’s official. Federal government websites often end in .gov or .mil. Before sharing sensitive information, make sure you’re on a federal government site.
The site is secure. The https:// ensures that you are connecting to the official website and that any information you provide is encrypted and transmitted securely.
- Publications
- Account settings
Preview improvements coming to the PMC website in October 2024. Learn More or Try it out now .
- Advanced Search
- Journal List
- Indian Dermatol Online J
- v.10(1); Jan-Feb 2019
Types of Variables, Descriptive Statistics, and Sample Size
Feroze kaliyadan.
Department of Dermatology, King Faisal University, Al Hofuf, Saudi Arabia
Vinay Kulkarni
1 Department of Dermatology, Prayas Amrita Clinic, Pune, Maharashtra, India
This short “snippet” covers three important aspects related to statistics – the concept of variables , the importance, and practical aspects related to descriptive statistics and issues related to sampling – types of sampling and sample size estimation.
What is a variable?[ 1 , 2 ] To put it in very simple terms, a variable is an entity whose value varies. A variable is an essential component of any statistical data. It is a feature of a member of a given sample or population, which is unique, and can differ in quantity or quantity from another member of the same sample or population. Variables either are the primary quantities of interest or act as practical substitutes for the same. The importance of variables is that they help in operationalization of concepts for data collection. For example, if you want to do an experiment based on the severity of urticaria, one option would be to measure the severity using a scale to grade severity of itching. This becomes an operational variable. For a variable to be “good,” it needs to have some properties such as good reliability and validity, low bias, feasibility/practicality, low cost, objectivity, clarity, and acceptance. Variables can be classified into various ways as discussed below.
Quantitative vs qualitative
A variable can collect either qualitative or quantitative data. A variable differing in quantity is called a quantitative variable (e.g., weight of a group of patients), whereas a variable differing in quality is called a qualitative variable (e.g., the Fitzpatrick skin type)
A simple test which can be used to differentiate between qualitative and quantitative variables is the subtraction test. If you can subtract the value of one variable from the other to get a meaningful result, then you are dealing with a quantitative variable (this of course will not apply to rating scales/ranks).
Quantitative variables can be either discrete or continuous
Discrete variables are variables in which no values may be assumed between the two given values (e.g., number of lesions in each patient in a sample of patients with urticaria).
Continuous variables, on the other hand, can take any value in between the two given values (e.g., duration for which the weals last in the same sample of patients with urticaria). One way of differentiating between continuous and discrete variables is to use the “mid-way” test. If, for every pair of values of a variable, a value exactly mid-way between them is meaningful, the variable is continuous. For example, two values for the time taken for a weal to subside can be 10 and 13 min. The mid-way value would be 11.5 min which makes sense. However, for a number of weals, suppose you have a pair of values – 5 and 8 – the midway value would be 6.5 weals, which does not make sense.
Under the umbrella of qualitative variables, you can have nominal/categorical variables and ordinal variables
Nominal/categorical variables are, as the name suggests, variables which can be slotted into different categories (e.g., gender or type of psoriasis).
Ordinal variables or ranked variables are similar to categorical, but can be put into an order (e.g., a scale for severity of itching).
Dependent and independent variables
In the context of an experimental study, the dependent variable (also called outcome variable) is directly linked to the primary outcome of the study. For example, in a clinical trial on psoriasis, the PASI (psoriasis area severity index) would possibly be one dependent variable. The independent variable (sometime also called explanatory variable) is something which is not affected by the experiment itself but which can be manipulated to affect the dependent variable. Other terms sometimes used synonymously include blocking variable, covariate, or predictor variable. Confounding variables are extra variables, which can have an effect on the experiment. They are linked with dependent and independent variables and can cause spurious association. For example, in a clinical trial for a topical treatment in psoriasis, the concomitant use of moisturizers might be a confounding variable. A control variable is a variable that must be kept constant during the course of an experiment.
Descriptive Statistics
Statistics can be broadly divided into descriptive statistics and inferential statistics.[ 3 , 4 ] Descriptive statistics give a summary about the sample being studied without drawing any inferences based on probability theory. Even if the primary aim of a study involves inferential statistics, descriptive statistics are still used to give a general summary. When we describe the population using tools such as frequency distribution tables, percentages, and other measures of central tendency like the mean, for example, we are talking about descriptive statistics. When we use a specific statistical test (e.g., Mann–Whitney U-test) to compare the mean scores and express it in terms of statistical significance, we are talking about inferential statistics. Descriptive statistics can help in summarizing data in the form of simple quantitative measures such as percentages or means or in the form of visual summaries such as histograms and box plots.
Descriptive statistics can be used to describe a single variable (univariate analysis) or more than one variable (bivariate/multivariate analysis). In the case of more than one variable, descriptive statistics can help summarize relationships between variables using tools such as scatter plots.
Descriptive statistics can be broadly put under two categories:
- Sorting/grouping and illustration/visual displays
- Summary statistics.
Sorting and grouping
Sorting and grouping is most commonly done using frequency distribution tables. For continuous variables, it is generally better to use groups in the frequency table. Ideally, group sizes should be equal (except in extreme ends where open groups are used; e.g., age “greater than” or “less than”).
Another form of presenting frequency distributions is the “stem and leaf” diagram, which is considered to be a more accurate form of description.
Suppose the weight in kilograms of a group of 10 patients is as follows:
56, 34, 48, 43, 87, 78, 54, 62, 61, 59
The “stem” records the value of the “ten's” place (or higher) and the “leaf” records the value in the “one's” place [ Table 1 ].
Stem and leaf plot
Illustration/visual display of data
The most common tools used for visual display include frequency diagrams, bar charts (for noncontinuous variables) and histograms (for continuous variables). Composite bar charts can be used to compare variables. For example, the frequency distribution in a sample population of males and females can be illustrated as given in Figure 1 .

Composite bar chart
A pie chart helps show how a total quantity is divided among its constituent variables. Scatter diagrams can be used to illustrate the relationship between two variables. For example, global scores given for improvement in a condition like acne by the patient and the doctor [ Figure 2 ].

Scatter diagram
Summary statistics
The main tools used for summary statistics are broadly grouped into measures of central tendency (such as mean, median, and mode) and measures of dispersion or variation (such as range, standard deviation, and variance).
Imagine that the data below represent the weights of a sample of 15 pediatric patients arranged in ascending order:
30, 35, 37, 38, 38, 38, 42, 42, 44, 46, 47, 48, 51, 53, 86
Just having the raw data does not mean much to us, so we try to express it in terms of some values, which give a summary of the data.
The mean is basically the sum of all the values divided by the total number. In this case, we get a value of 45.
The problem is that some extreme values (outliers), like “'86,” in this case can skew the value of the mean. In this case, we consider other values like the median, which is the point that divides the distribution into two equal halves. It is also referred to as the 50 th percentile (50% of the values are above it and 50% are below it). In our previous example, since we have already arranged the values in ascending order we find that the point which divides it into two equal halves is the 8 th value – 42. In case of a total number of values being even, we choose the two middle points and take an average to reach the median.
The mode is the most common data point. In our example, this would be 38. The mode as in our case may not necessarily be in the center of the distribution.
The median is the best measure of central tendency from among the mean, median, and mode. In a “symmetric” distribution, all three are the same, whereas in skewed data the median and mean are not the same; lie more toward the skew, with the mean lying further to the skew compared with the median. For example, in Figure 3 , a right skewed distribution is seen (direction of skew is based on the tail); data values' distribution is longer on the right-hand (positive) side than on the left-hand side. The mean is typically greater than the median in such cases.

Location of mode, median, and mean
Measures of dispersion
The range gives the spread between the lowest and highest values. In our previous example, this will be 86-30 = 56.
A more valuable measure is the interquartile range. A quartile is one of the values which break the distribution into four equal parts. The 25 th percentile is the data point which divides the group between the first one-fourth and the last three-fourth of the data. The first one-fourth will form the first quartile. The 75 th percentile is the data point which divides the distribution into a first three-fourth and last one-fourth (the last one-fourth being the fourth quartile). The range between the 25 th percentile and 75 th percentile is called the interquartile range.
Variance is also a measure of dispersion. The larger the variance, the further the individual units are from the mean. Let us consider the same example we used for calculating the mean. The mean was 45.
For the first value (30), the deviation from the mean will be 15; for the last value (86), the deviation will be 41. Similarly we can calculate the deviations for all values in a sample. Adding these deviations and averaging will give a clue to the total dispersion, but the problem is that since the deviations are a mix of negative and positive values, the final total becomes zero. To calculate the variance, this problem is overcome by adding squares of the deviations. So variance would be the sum of squares of the variation divided by the total number in the population (for a sample we use “n − 1”). To get a more realistic value of the average dispersion, we take the square root of the variance, which is called the “standard deviation.”
The box plot
The box plot is a composite representation that portrays the mean, median, range, and the outliers [ Figure 4 ].

The concept of skewness and kurtosis
Skewness is a measure of the symmetry of distribution. Basically if the distribution curve is symmetric, it looks the same on either side of the central point. When this is not the case, it is said to be skewed. Kurtosis is a representation of outliers. Distributions with high kurtosis tend to have “heavy tails” indicating a larger number of outliers, whereas distributions with low kurtosis have light tails, indicating lesser outliers. There are formulas to calculate both skewness and kurtosis [Figures [Figures5 5 – 8 ].

Positive skew

High kurtosis (positive kurtosis – also called leptokurtic)

Negative skew

Low kurtosis (negative kurtosis – also called “Platykurtic”)
Sample Size
In an ideal study, we should be able to include all units of a particular population under study, something that is referred to as a census.[ 5 , 6 ] This would remove the chances of sampling error (difference between the outcome characteristics in a random sample when compared with the true population values – something that is virtually unavoidable when you take a random sample). However, it is obvious that this would not be feasible in most situations. Hence, we have to study a subset of the population to reach to our conclusions. This representative subset is a sample and we need to have sufficient numbers in this sample to make meaningful and accurate conclusions and reduce the effect of sampling error.
We also need to know that broadly sampling can be divided into two types – probability sampling and nonprobability sampling. Examples of probability sampling include methods such as simple random sampling (each member in a population has an equal chance of being selected), stratified random sampling (in nonhomogeneous populations, the population is divided into subgroups – followed be random sampling in each subgroup), systematic (sampling is based on a systematic technique – e.g., every third person is selected for a survey), and cluster sampling (similar to stratified sampling except that the clusters here are preexisting clusters unlike stratified sampling where the researcher decides on the stratification criteria), whereas nonprobability sampling, where every unit in the population does not have an equal chance of inclusion into the sample, includes methods such as convenience sampling (e.g., sample selected based on ease of access) and purposive sampling (where only people who meet specific criteria are included in the sample).
An accurate calculation of sample size is an essential aspect of good study design. It is important to calculate the sample size much in advance, rather than have to go for post hoc analysis. A sample size that is too less may make the study underpowered, whereas a sample size which is more than necessary might lead to a wastage of resources.
We will first go through the sample size calculation for a hypothesis-based design (like a randomized control trial).
The important factors to consider for sample size calculation include study design, type of statistical test, level of significance, power and effect size, variance (standard deviation for quantitative data), and expected proportions in the case of qualitative data. This is based on previous data, either based on previous studies or based on the clinicians' experience. In case the study is something being conducted for the first time, a pilot study might be conducted which helps generate these data for further studies based on a larger sample size). It is also important to know whether the data follow a normal distribution or not.
Two essential aspects we must understand are the concept of Type I and Type II errors. In a study that compares two groups, a null hypothesis assumes that there is no significant difference between the two groups, and any observed difference being due to sampling or experimental error. When we reject a null hypothesis, when it is true, we label it as a Type I error (also denoted as “alpha,” correlating with significance levels). In a Type II error (also denoted as “beta”), we fail to reject a null hypothesis, when the alternate hypothesis is actually true. Type II errors are usually expressed as “1- β,” correlating with the power of the test. While there are no absolute rules, the minimal levels accepted are 0.05 for α (corresponding to a significance level of 5%) and 0.20 for β (corresponding to a minimum recommended power of “1 − 0.20,” or 80%).
Effect size and minimal clinically relevant difference
For a clinical trial, the investigator will have to decide in advance what clinically detectable change is significant (for numerical data, this is could be the anticipated outcome means in the two groups, whereas for categorical data, it could correlate with the proportions of successful outcomes in two groups.). While we will not go into details of the formula for sample size calculation, some important points are as follows:
In the context where effect size is involved, the sample size is inversely proportional to the square of the effect size. What this means in effect is that reducing the effect size will lead to an increase in the required sample size.
Reducing the level of significance (alpha) or increasing power (1-β) will lead to an increase in the calculated sample size.
An increase in variance of the outcome leads to an increase in the calculated sample size.
A note is that for estimation type of studies/surveys, sample size calculation needs to consider some other factors too. This includes an idea about total population size (this generally does not make a major difference when population size is above 20,000, so in situations where population size is not known we can assume a population of 20,000 or more). The other factor is the “margin of error” – the amount of deviation which the investigators find acceptable in terms of percentages. Regarding confidence levels, ideally, a 95% confidence level is the minimum recommended for surveys too. Finally, we need an idea of the expected/crude prevalence – either based on previous studies or based on estimates.
Sample size calculation also needs to add corrections for patient drop-outs/lost-to-follow-up patients and missing records. An important point is that in some studies dealing with rare diseases, it may be difficult to achieve desired sample size. In these cases, the investigators might have to rework outcomes or maybe pool data from multiple centers. Although post hoc power can be analyzed, a better approach suggested is to calculate 95% confidence intervals for the outcome and interpret the study results based on this.
Financial support and sponsorship
Conflicts of interest.
There are no conflicts of interest.

Advertisement
The Independent Variable vs. Dependent Variable in Research
- Share Content on Facebook
- Share Content on LinkedIn
- Share Content on Flipboard
- Share Content on Reddit
- Share Content via Email
In any scientific research, there are typically two variables of interest: independent variables and dependent variables. In forming the backbone of scientific experiments , they help scientists understand relationships, predict outcomes and, in general, make sense of the factors that they're investigating.
Understanding the independent variable vs. dependent variable is so fundamental to scientific research that you need to have a good handle on both if you want to design your own research study or interpret others' findings.
To grasp the distinction between the two, let's delve into their definitions and roles.
What Is an Independent Variable?
What is a dependent variable, research study example, predictor variables vs. outcome variables, other variables, the relationship between independent and dependent variables.
The independent variable, often denoted as X, is the variable that is manipulated or controlled by the researcher intentionally. It's the factor that researchers believe may have a causal effect on the dependent variable.
In simpler terms, the independent variable is the variable you change or vary in an experiment so you can observe its impact on the dependent variable.
The dependent variable, often represented as Y, is the variable that is observed and measured to determine the outcome of the experiment.
In other words, the dependent variable is the variable that is affected by the changes in the independent variable. The values of the dependent variable always depend on the independent variable.
Let's consider an example to illustrate these concepts. Imagine you're conducting a research study aiming to investigate the effect of studying techniques on test scores among students.
In this scenario, the independent variable manipulated would be the studying technique, which you could vary by employing different methods, such as spaced repetition, summarization or practice testing.
The dependent variable, in this case, would be the test scores of the students. As the researcher following the scientific method , you would manipulate the independent variable (the studying technique) and then measure its impact on the dependent variable (the test scores).
You can also categorize variables as predictor variables or outcome variables. Sometimes a researcher will refer to the independent variable as the predictor variable since they use it to predict or explain changes in the dependent variable, which is also known as the outcome variable.
When conducting an experiment or study, it's crucial to acknowledge the presence of other variables, or extraneous variables, which may influence the outcome of the experiment but are not the focus of study.
These variables can potentially confound the results if they aren't controlled. In the example from above, other variables might include the students' prior knowledge, level of motivation, time spent studying and preferred learning style.
As a researcher, it would be your goal to control these extraneous variables to ensure you can attribute any observed differences in the dependent variable to changes in the independent variable. In practice, however, it's not always possible to control every variable.
The distinction between independent and dependent variables is essential for designing and conducting research studies and experiments effectively.
By manipulating the independent variable and measuring its impact on the dependent variable while controlling for other factors, researchers can gain insights into the factors that influence outcomes in their respective fields.
Whether investigating the effects of a new drug on blood pressure or studying the relationship between socioeconomic factors and academic performance, understanding the role of independent and dependent variables is essential for advancing knowledge and making informed decisions.
Correlation vs. Causation
Understanding the relationship between independent and dependent variables is essential for making sense of research findings. Depending on the nature of this relationship, researchers may identify correlations or infer causation between the variables.
Correlation implies that changes in one variable are associated with changes in another variable, while causation suggests that changes in the independent variable directly cause changes in the dependent variable.
Control and Intervention
In experimental research, the researcher has control over the independent variable, allowing them to manipulate it to observe its effects on the dependent variable. This controlled manipulation distinguishes experiments from other types of research designs.
For example, in observational studies, researchers merely observe variables without intervention, meaning they don't control or manipulate any variables.
Context and Analysis
Whether it's intentional or unintentional, independent, dependent and other variables can vary in different contexts, and their effects may differ based on various factors, such as age, characteristics of the participants, environmental influences and so on.
Researchers employ statistical analysis techniques to measure and analyze the relationships between these variables, helping them to draw meaningful conclusions from their data.
We created this article in conjunction with AI technology, then made sure it was fact-checked and edited by a HowStuffWorks editor.
Please copy/paste the following text to properly cite this HowStuffWorks.com article:
ORIGINAL RESEARCH article
Causal effects of gut microbiota on the prognosis of ischemic stroke: evidence from a bidirectional two-sample mendelian randomization study.
- Department of Tuina, Yueyang Hospital of Integrated Traditional Chinese and Western Medicine, Shanghai University of Traditional Chinese Medicine, Shanghai, China
Background: Increasing research has implicated the possible effect of gut microbiota (GM) on the prognosis of ischemic stroke (IS). However, the precise causal relationship between GM and functional outcomes after IS remains unestablished.
Methods: Data on 211 GM taxa from the MiBioGen consortium and data on prognosis of IS from the Genetics of Ischemic Stroke Functional Outcome (GISCOME) network were utilized as summary-level data of exposure and outcome. Four kinds of Mendelian randomization (MR) methods were carried out to ascertain the causal effect of GM on functional outcomes following IS. A reverse MR analysis was performed on the positive taxa identified in the forward MR analysis to determine the direction of causation. In addition, we conducted a comparative MR analysis without adjusting the baseline National Institute of Health Stroke Scale (NIHSS) of post-stroke functional outcomes to enhance confidence of the results obtained in the main analysis.
Results: Four taxa were identified to be related to stroke prognosis in both main and comparative analyses. Specifically, genus Ruminococcaceae UCG005 and the Eubacterium oxidoreducens group showed significantly negative effects on stroke prognosis, while the genus Lachnospiraceae NK4A136 group and Lachnospiraceae UCG004 showed protective effects against stroke prognosis. The reverse MR analysis did not support a causal role of stroke prognosis in GM. No evidence of heterogeneity, horizontal pleiotropy, and outliers was found.
Conclusion: This MR study provided evidence that genetically predicted GM had a causal link with post-stroke outcomes. Specific gut microbiota taxa associated with IS prognosis were identified, which may be helpful to clarify the pathogenesis of ischemic stroke and making treatment strategies.
Introduction
Stroke remains a severe health problem that causes death and long-term disability worldwide, resulting in increased economic and social burden ( Herpich and Rincon, 2020 ). More importantly, such negative impact of stroke will increase as people age across the globe ( Murray and Lopez, 2013 ). Stroke is usually classified into two types: ischemic and hemorrhagic. Ischemic stroke (IS) is the most prevalent one, accounting for approximately 70%−85% of all stroke cases globally ( Pluta et al., 2021 ; DeLong et al., 2022 ). At present, effective treatments targeting IS, such as thrombolytic therapy ( Li et al., 2021 ; Zhao et al., 2022 ), thrombectomy ( Winkelmeier et al., 2023 ), neuroprotective agents ( Paul and Candelario-Jalil, 2021 ), and early rehabilitation ( Gittler and Davis, 2018 ; Geng et al., 2022 ), seem to be related to a better functional outcome.
Recently, increasing evidence indicated that the outcome of IS can also be influenced by gut microbiota (GM). For example, animal model-based studies found that altering the gut microbiome of aged mice after experimental stroke through transplanting youthful microbiota can reverse the poor recovery in aged stroke mice ( Spychala et al., 2018 ; Lee et al., 2020 ). Another animal study also proved that the cerebral infarct size and post-stroke outcomes were impacted by transplantation of GM, which was associated with the trimethylamine-N-oxide (TMAO) pathway ( Zhu et al., 2021 ). Furthermore, some studies reported the relationship between the composition of GM and the prognosis of human stroke. An observational study reported that Christensenellaceae _R-7_group and norank_f_Ruminococcaceae were positively correlated with the modified Rankin scale (mRS) at 1 month, which was used to evaluate the stroke outcome, while genus Enterobacter was negatively correlated with the mRS ( Li et al., 2019 ). Another case–control study which defined the mRS score of ≥ 3 at 3 months as a poor functional outcome also analyzed the differences of microbiota composition between the outcome groups after stroke, and the results showed that the group with poor outcomes had a higher abundance of Ruminococcaceae and Prevotella and a lower abundance of Anaerococcus, Blautia, Dialister, Aerococcaceae, Propionibacterium, Microbacteriaceae , and Rothia compared with the group with good outcomes ( Chang et al., 2021 ). Although the above two research studies confirmed the association between GM and IS functional outcomes, the results did not seem to be entirely consistent, maybe because of the non-uniform functional outcome indicators. In addition, it is uncertain whether these associations are causal, given that the evidence obtained mainly from observational studies may result in potential bias in results due to reverse causation and residual confounding.
Mendelian randomization (MR) is an analytic method based on the summary data of genome-wide association study (GWAS) to establish the causality between exposure and outcome ( Sleiman and Grant, 2010 ; Sekula et al., 2016 ). Given that genetic variants, such as single-nucleotide polymorphisms (SNPs), are used as proxies for the modifiable environmental factors (exposure) under investigation, the MR method has the advantage of reducing potential bias from confounding factors and reverse causation because of the random assignment of alleles during human gamete formation and the perpetually immutable genotype determined before birth ( Sekula et al., 2016 ). Consequently, with the available GWAS data over the last decade, MR studies have been widely used to analyze the causal association between GM and diseases ( Smith and Ebrahim, 2003 ; Wang et al., 2018 ; Kurilshikov et al., 2021 ).
A newly published report has used MR to reveal the causal effect of GM on the risk of IS subtypes ( Meng et al., 2023 ). However, to date, MR studies about the causal inference between GM and IS prognosis remain unavailable. Furthermore, conducting large-scale longitudinal cohort studies or randomized controlled trials (RCTs) currently is unfeasible. In this context, we performed this MR study using the genetic variation associated with GM to assess their possible causal relationship with IS functional outcomes.
Study design
This two-sample MR study was designed to explore the causal relationship between genetically predicted GM and functional outcomes after IS. The flowchart of this study is shown in Figure 1 . The analysis results are presented in accordance with the Strengthening the Reporting of Observational Studies in Epidemiology-Mendelian Randomization (STROBE-MR) guidelines, which is recommended for this study type ( Skrivankova et al., 2021 ) ( Supplementary Table S7 ).
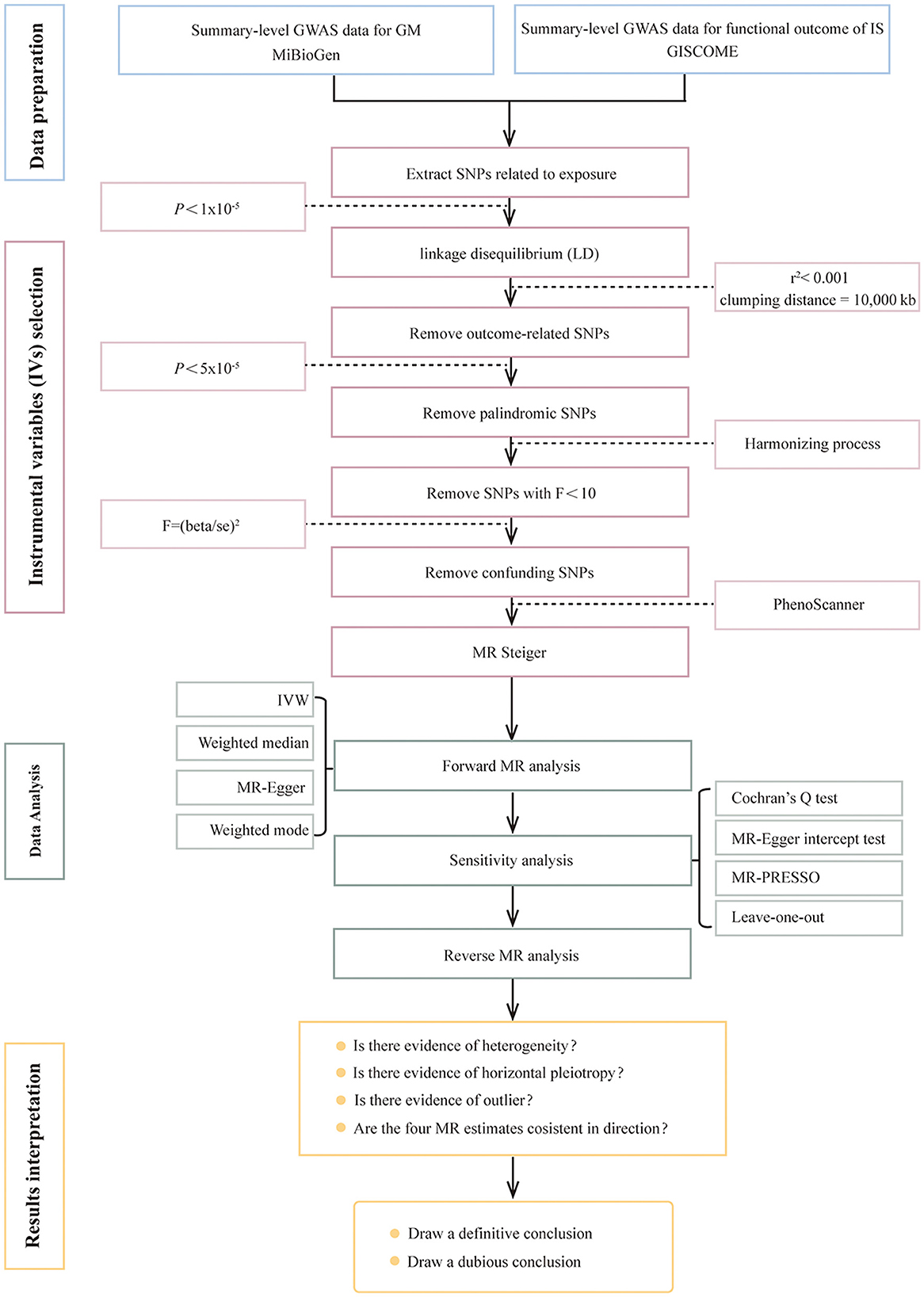
Figure 1 . Flowchart of Mendelian randomization analysis. GWAS, genome-wide association study; GM, gut microbiota; IS, ischemic stroke; GISCOME, Genetics of Ischemic Stroke Functional Outcome; IVs, instrumental variables; SNPs, single-nucleotide polymorphisms; LD, linkage disequilibrium; IVW, inverse variance weighted; MR, Mendelian randomization.
Data sources
GWAS data on GM and functional outcomes after IS were acquired from the MiBioGen consortium and the Genetics of Ischemic Stroke Functional Outcome (GISCOME) network, respectively. Notably, populations from both exposure and outcome cohorts were independent and non-overlapping. Moreover, most individuals involved were of European descent, contributing to decreased bias resulting from population stratification. The MiBioGen study was the largest-scale genome-wide meta-analysis of GM to date, identifying a total of 211 taxa from 18,340 individuals (13,266 of European ancestry) of 24 cohorts ( Wang et al., 2018 ). In this study, 196 taxa (comprising of 9 phyla, 16 classes, 20 orders, 32 families, and 119 genera) were ultimately retained and used owing to 15 unknown taxa being excluded. The GISCOME network included 6,021 IS individuals of mainly European ancestry from 12 population-based cohorts and defined mRS score at 3 months after IS as the primary outcome ( Soderholm et al., 2019 ). A dichotomized mRS at 3 months of 0–2 ( n = 3,741) indicates a better functional outcome and 3–6 ( n = 2,280) indicates a worse functional outcome. Data on IS prognosis we used in main MR analysis was adjusted for age, sex, ancestry, and baseline NIHSS. Besides, we conducted a comparative analysis without adjusting baseline NIHSS.
Instrumental variables selection
Choosing genetic variants meeting three key assumptions ( Figure 2 ) as valid IVs is fundamental to obtain a reliable and robust conclusion on causal inference. Valid IVs must be: (i) significantly associated with the exposure (the relevance assumption); (ii) independent of any confounding factors related to the exposure or the outcome (the independence assumption); (iii) associated with the outcome only through the exposure rather than any other ways (the exclusion restriction criteria) ( Davies et al., 2018 ; Carter et al., 2021 ). Rigorous criteria and steps ( Figure 1 ) were performed as below to obtain the optimal IVs. First, SNPs related to GM were identified as potential IVs under a significance level ( P < 1 × 10 −5 ) ( Li C. et al., 2022 ). Second, SNPs with the lowest p -value were eventually retained by performing a linkage disequilibrium (LD) analysis with r 2 < 0.001 and clumping distance = 10,000 kb based on the data of European samples from the 1,000 Genomes Project. Third, SNPs associated with the outcome under the significance level of P < 5 × 10 −5 were removed after extracting the corresponding information of the selected SNPs from the GWAS outcome data. Fourth, palindromic and ambiguous SNPs were removed during the harmonizing process. Fifth, weak IVs referring to SNPs with F-statistics < 10 were excluded. The F-statistics was calculated using the formula: F = (beta/se) 2 . Finally, SNPs significantly associated ( P < 5 × 10 −8 ) with confounding factors were checked through the PhenoScanner GWAS database and were manually removed. Meanwhile, the MR Steiger test was used to ensure the directional accuracy of the causality for each IV. Only SNPs that were retained through the above screening steps can be finally used for the subsequent MR analysis.
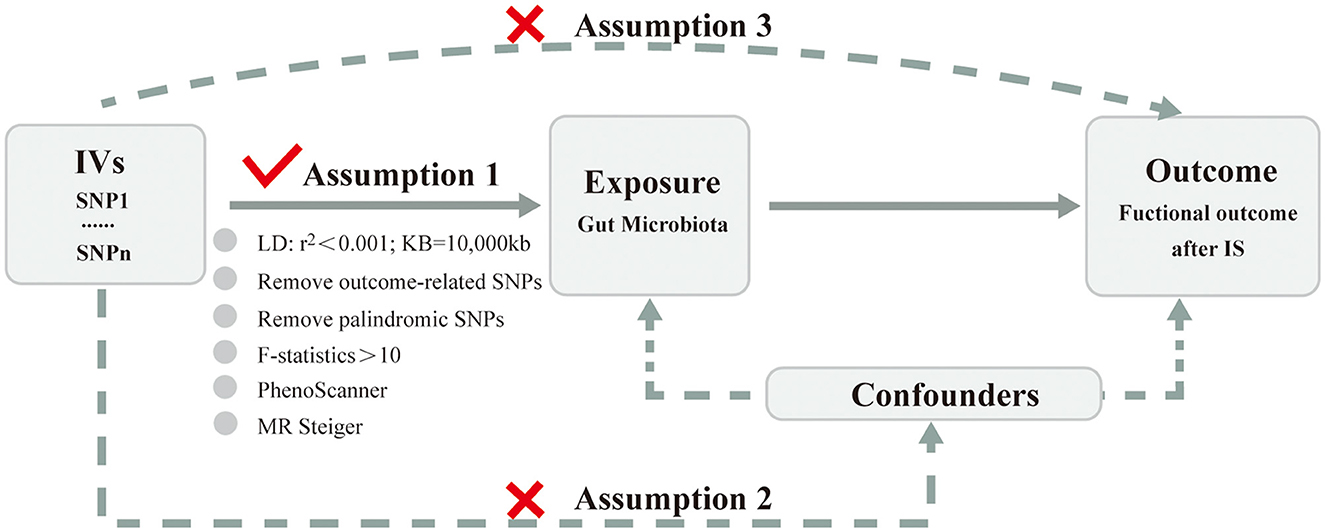
Figure 2 . Three key assumptions of Mendelian randomization analysis. IVs, instrumental variables; SNP, single-nucleotide polymorphism; LD, linkage disequilibrium; IS, ischemic stroke.
MR analysis
Four different methods were used for this bidirectional MR study to explore the causal relationship between GM and IS functional outcomes. Inverse variance weighted (IVW) was conducted as the primary analysis approach, complemented by the MR-Egger, weighted median, and weighted mode methods ( Long et al., 2023 ). IVW can provide unbiased causal results in the case of the balanced horizontal pleiotropy or no horizontal pleiotropy ( Hemani et al., 2018 ). Under the assumption that instrument strength is independent of direct effect (InSIDE), MR-Egger regression could provide evidence of no horizontal pleiotropy and a consistent result with IVW if the intercept equals zero ( Bowden et al., 2015 ). The efficiency of weighted median is similar to IVW if up to 50% of the weights is from valid IVs ( Bowden et al., 2016 ). The weighted mode method is proved to have fewer biases than MR-Egger regression if InSIDE assumption is falsified ( Li P. et al., 2022 ). As a whole, if the results of the above methods are inconsistent, the results of IVW will be given priority.
To address multiple comparisons (196 exposures), p -values of the IVW method have been adjusted by false discovery rate (FDR) correction. P FDR < 0.05 was considered to indicate a significant association ( Xu et al., 2021 ; Gu et al., 2023 ).
Sensitivity analysis
Sensitivity analysis for potential heterogeneity and horizontal pleiotropy have also been performed to detect the robustness of causal inference results. The Cochran's Q test with an insignificant P value ( P > 0.05) was defined as having no heterogeneity. The MR-Egger intercept test with an insignificant P value ( P > 0.05) indicated no horizontal pleiotropy. Furthermore, the MR-PRESSO test was performed to eliminate the effect of horizontal pleiotropy by re-analyzing it after removing pleiotropic SNPs. The leave-one-out analysis was applied to rule out potential pleiotropy driven by a single SNP by excluding one instrumental SNP each time and repeating the IVW analysis.
All statistical analyses, including the MR analysis and the sensitivity analysis, were performed using TwoSampleMR and MRPRESSO packages in R (version 4.2.1). In addition, ggplot2 package in R was used for data visualization.
IV selection
We first obtained 196 taxa at the phylum, class, order, family, and genus levels after excluding 15 unknown taxa ( Supplementary Table S1 ). Subsequently, several screening steps mentioned above were implemented. Confounding factors, such as smoking ( Zhang et al., 2022 ), migraine ( Wang et al., 2023 ), frailty ( Cai et al., 2023 ), depression ( Gill et al., 2019 ), diabetes ( Lau et al., 2019 ), body mass index (BMI), and insomnia ( Zhang et al., 2023 ), were determined by reviewing the literature. We manually removed 35 SNPs, and a total of 2038 SNPs from 196 taxa were eventually chosen as IVs ( Supplementary Table S2 ).
Causal effects of GM on functional outcomes after IS
The main IVW results of 196 GM taxa in the forward MR analysis were shown in the lollipop plot in Figure 3 . A total of 13 taxa which have the possibility of causal associations with the functional outcome after IS were initially selected. We further excluded class Verrucomicrobiae , family Verrucomicrobiaceae , genus Akkermansia , genus Lachnospiraceae ND3007 group, genus Ruminococcaceae UCG013 , order Verrucomicrobiales , and phylum Cyanobacteria from the analysis results because of the inconsistent direction of effect estimates produced by the four MR methods ( Supplementary Table S3 ). Finally, a total of six significant GM taxa were obtained. The Forest plot of four analyses is shown in Figure 4 . Based on the results of the IVW analysis, genus Ruminococcaceae UCG005 (odds ratio [OR] = 1.842, 95% confidence interval [CI] 1.210–2.804, P = 0.004, P FDR = 0.017) and the genus Eubacterium oxidoreducens group (OR = 1.771, 95% CI 1.105–2.837, P = 0.018, P FDR = 0.026) were demonstrated to have a positive correlation with worse functional outcomes after IS. Family Peptostreptococcaceae (OR = 0.635, 95% CI 0.413–0.975, P = 0.038, P FDR = 0.046), the genus Lachnospiraceae NK4A136 group (OR = 0.653, 95% CI 0.427–0.997, P = 0.048, P FDR = 0.048), genus Lachnospiraceae UCG004 (OR = 0.493, 95% CI 0.292–0.834, P = 0.008, P FDR = 0.017), and genus Odoribacter (OR = 0.399, 95% CI 0.208–0.766, P = 0.006, P FDR = 0.017) were negatively correlated with worse functional outcomes after IS. According to the MR estimates of the weighted median, genus Odoribacter (OR = 0.377, 95% CI, 0.154–0.920, P = 0.032) was considered as protective factors for functional outcomes after IS, whereas genus Ruminococcaceae UCG005 (OR = 1.895, 95% CI 1.068–3.362, P = 0.029) and the genus Eubacterium oxidoreducens group (OR = 1.809, 95% CI 1.068–3.270, P = 0.0499) were considered as risk factors ( Figure 4 ). The results of the sensitivity analysis for six significant taxa show no evidence of horizontal pleiotropy and heterogeneity ( Supplementary Table S4 ). The MR-PRESSO global test ( P > 0.05) indicated no outliers in the results. Furthermore, the leave-one-out analysis did not provide any evidence that one single SNP was responsible for the inferred causal relationship between GM and functional outcomes after IS ( Figure 5 ). The results of the above analysis confirmed the accuracy and robustness of causal inference of genetically predicted GM and functional outcomes after IS.
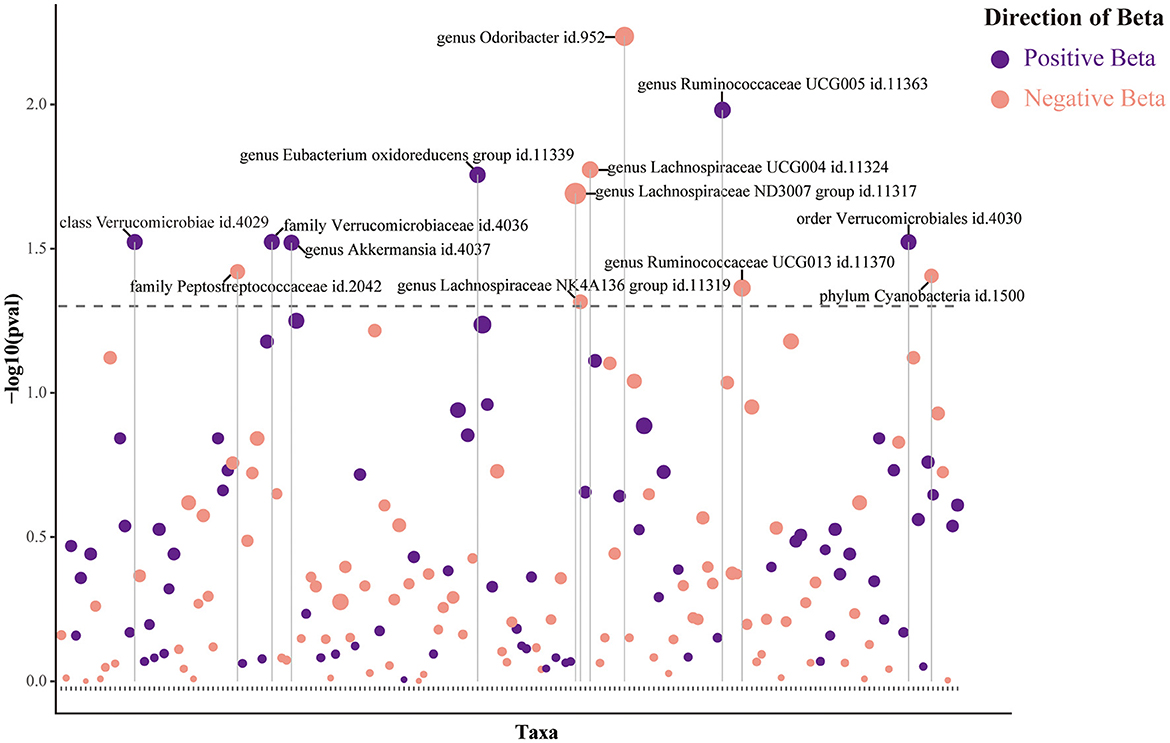
Figure 3 . Lollipop plot was constructed to illustrate the outcomes of the IVW analysis concerning the impact of 196 gut microbiota (GM) taxa on functional outcomes after ischemic stroke (IS). In this plot, positive beta values are represented in purple, while negative beta values are represented in pink. Dashed lines positioned above the plot indicate p -values below the 0.05 threshold. Taxa that achieved statistical significance are explicitly labeled in the plot.
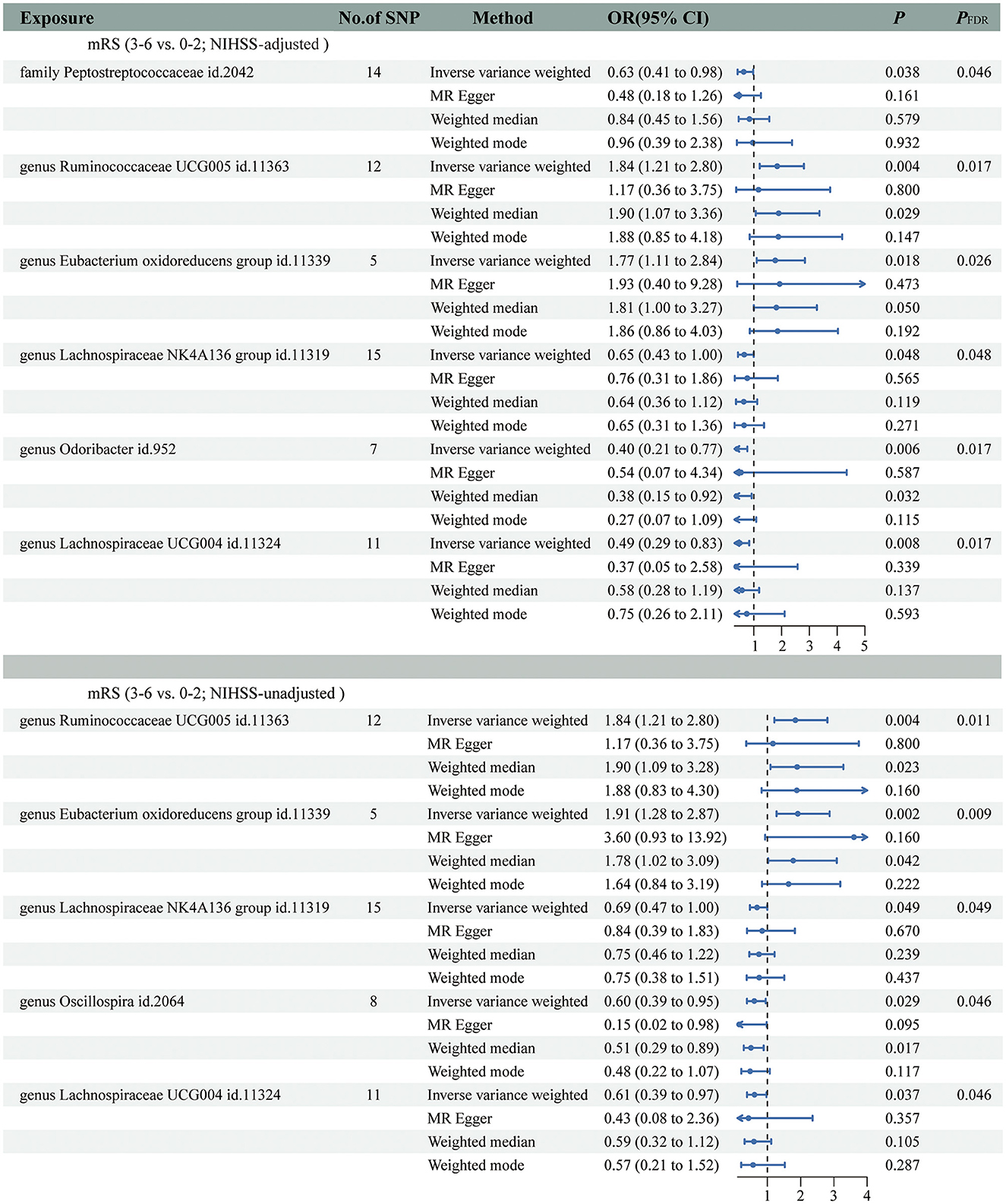
Figure 4 . Forest plot was used to present the results of four analyses on the genetic associations between gut microbiota (GM) and functional outcomes after ischemic stroke (IS).
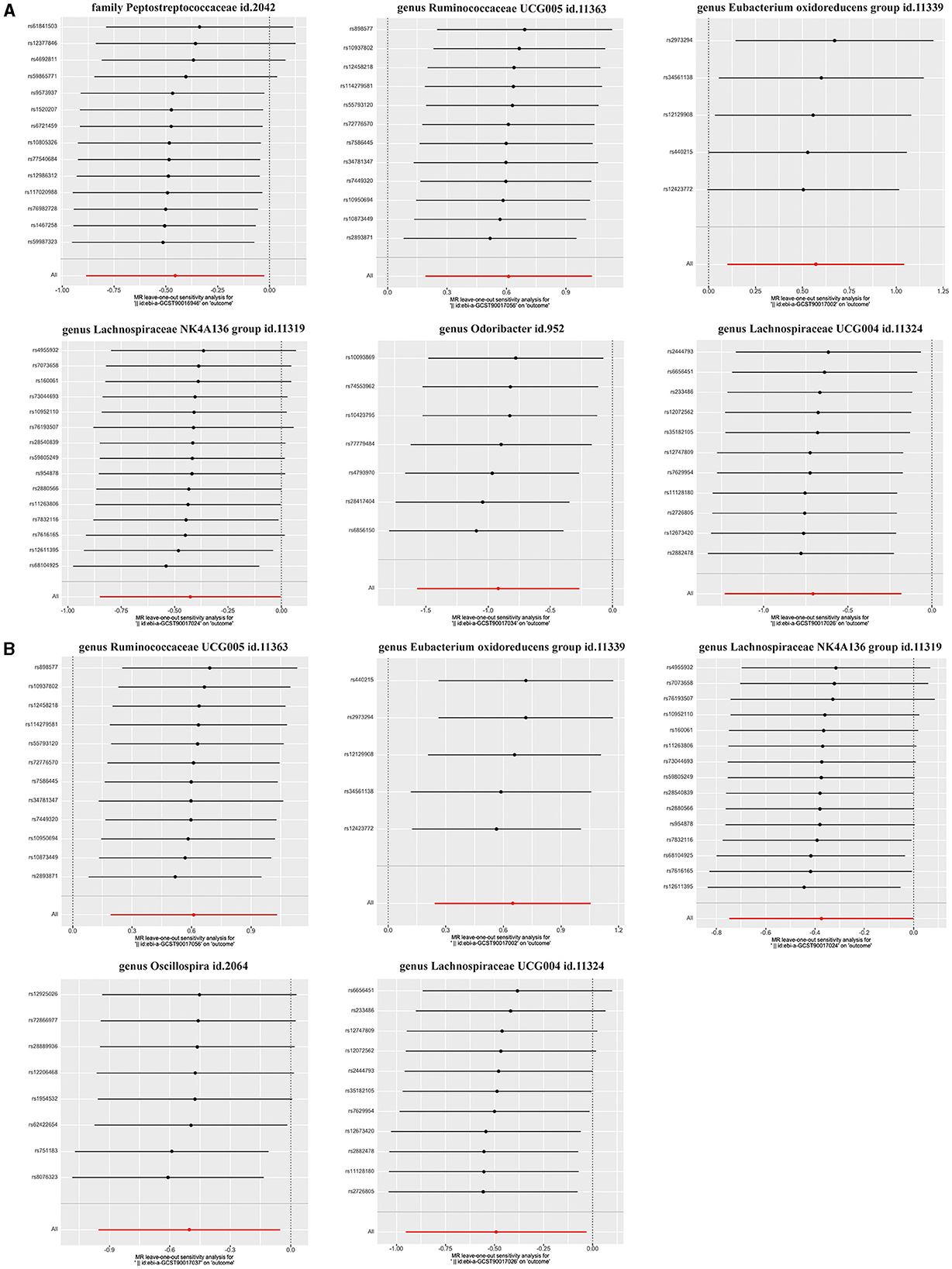
Figure 5 . Leave-one-out analysis for (A) six GM taxa in the main analysis, (B) five GM taxa in the comparative analysis.
In addition, the positive results of the analysis without adjustment for the baseline NIHSS were used as a comparison. Notably, we obtained five significant taxa ( Figure 4 ), four of which had similar results to the main analysis, and no evidence of pleiotropy, heterogeneity or outliers were found ( Supplementary Table S4 ). The results of leave-one-out analysis from the comparative analysis are presented in Figure 5 . Similar to the main IVW analysis, genus Ruminococcaceae UCG005 (OR = 1.842, 95% CI 1.210–2.804, P = 0.004, P FDR = 0.011) and the genus Eubacterium oxidoreducens group (OR = 1.913, 95% CI 1.275–2.871, P = 0.002, P FDR = 0.009) showed a positive correlation with the worse prognosis after IS, whereas genus Lachnospiraceae NK4A136 group (OR = 0.688, 95% CI 0.473–0.999, P = 0.049, P FDR = 0.049) and genus Lachnospiraceae UCG004 (OR = 0.612, 95% CI 0.386–0.970, P = 0.037, P FDR = 0.046) showed a negative correlation with worse prognosis after IS. Genus Oscillospira (OR = 0.605, 95% CI 0.385–0.949, P = 0.029, P FDR = 0.046) also showed a negative correlation with the poor outcome in the comparative analysis, which is different from Family Peptostreptococcaceae and genus Odoribacter in the main analysis. In terms of risk factors for functional outcomes after IS, the weighted median method produced results similar to the main analysis, further suggesting the confidence of genus Ruminococcaceae UCG005 (OR = 1.895, 95% CI 1.094–3.284, P = 0.023) and genus Eubacterium oxidoreducens group (OR = 1.776, 95% CI 1.022–3.086, P = 0.042) as risk factors. However, for protective factors, the weighted median method yielded a different result than the main analysis for the genus Oscillospira (OR = 0.506, 95% CI, 0.289–0.886, P = 0.017).
Causal effects of functional outcomes after IS on GM
We also performed a reverse MR analysis on seven positive taxa that were identified to be causally related to functional outcomes after IS in the forward MR analysis to explore the direction of causation. The reverse MR analysis showed no suggestive causal association between prognosis of IS and GM ( Supplementary Table S5 ). Additionally, the results of the sensitivity analysis did not provide any evidence of horizontal pleiotropy and heterogeneity ( Supplementary Table S6 ).
To our knowledge, this is the first MR analysis, overcoming environmental confounding and reverse causation, to investigate the causal effect of genetically determined gut microbiota on functional outcomes after ischemic stroke. Evidence from this study supported a causal association between the abundance of specific bacterial traits and the prognosis of IS. However, positive findings in this study were mainly at the genus level, and no causal associations between GM and IS prognosis at the level of the phylum, class, and order were found. Strikingly, genus Ruminococcaceae UCG005 and the genus Eubacterium oxidoreducens group showed significantly negative effects on stroke prognosis, while genus Lachnospiraceae NK4A136 group and genus Lachnospiraceae UCG004 showed protective effects against stroke prognosis in both main and comparative IVW analysis. Additionally, although the results of the main and comparative weighted median analyses were inconsistent in terms of protection factors, genus Ruminococcaceae UCG005 and genus Eubacterium oxidoreducens group as risk factors for poor prognosis of IS were indisputable. We also performed a reverse MR analysis of these positive taxa, and the results did not support a causal effect of post-stroke prognosis on these taxa. These findings may provide important implications for the discovery of novel biomarkers in future IS experiments and provide prevention and therapeutic strategies targeting dysbiosis of specific GM taxa.
Previous research found that Ruminococcaceae UCG005 increased in severe stroke patients who usually have a worse stroke outcome ( Li et al., 2019 ). This is consistent with our research. A network analysis suggested that Ruminococcaceae UCG005 was one of the genera driving the progress of the type 2 diabetes in a Mexican cohort ( Esquivel-Hernandez et al., 2023 ). An animal study reported a significantly negative correlation of Ruminococcaceae UCG005 with high-density lipoprotein cholesterol (HDL-C) and a positive correlation with body weight ( Qin et al., 2022 ). It is well known that abnormal glucose and lipid metabolism are the dominant causes of cerebrovascular diseases. Genus Ruminococcaceae UCG005 may influence the stroke prognosis by the pathway of glucose and lipid metabolism. Furthermore, ample evidence shows that imbalance in GM contributes to the neuroinflammation and worse stroke outcomes ( Huang et al., 2023 ; Park et al., 2023 ). An animal-based study found that maternal sleep deprivation (MSD) caused high expression of pro-inflammatory cytokines in offspring rats Moreover, pro-inflammatory cytokines were positively associated with Ruminococcaceae UCG005 ( Yao et al., 2022 ). Therefore, it is speculated that Ruminococcaceae UCG005 may also be involved in the inflammatory response after stroke, thereby adversely affecting the prognosis of stroke. These results may support the conclusion of Ruminococcaceae UCG005 as a risk factor for stroke prognosis.
For the Eubacterium oxidoreducens group, we failed to find some direct evidence of its role in stroke prognosis from existing research. Research on the role of the Eubacterium oxidoreducens group in other diseases is also rare. However, a previous study reported a significantly elevated relative abundance of E ubacterium oxidoreducens group which was positively correlated with the levels of serum and fecal lipopolysaccharide (LPS) in high-fat diet (HFD)-fed mice ( Zhang X. Y. et al., 2020 ). Increased LPS contents could impair the intestinal epithelial barrier (IEB) ( Guo et al., 2015 ) and blood–brain barrier (BBB) ( Peng et al., 2021 ). Recent studies have revealed that gut barrier integrity and BBB are involved in the influence of GM on IS ( Gwak and Chang, 2021 ; Zeng et al., 2023 ). A new study found that intraperitoneal injection of LPS after stroke exhibited intestinal morphology damage, decreased expression of tight-junction proteins associated with the BBB, and more neuronal loss, and these changes were consistent with stroke mice transplanted with gut microbiota associated with post-stroke cognitive impairment ( Wang et al., 2022 ). Therefore, we speculate that the Eubacterium oxidoreducens group, as a risk factor, may have adverse effects on stroke prognosis by affecting intestinal epithelial integrity and blood–brain barrier.
The results of another MR study evaluating the causal effect of GM on cardioembolic IS support the protective effect of the genus Lachnospiraceae NK4A136 group on IS prognosis in this MR analysis ( Dai et al., 2024 ). A previous study reported a significant negative association of the genus L achnospiraceae NK4A136 group with intestinal permeability and the plasma LPS level ( Ma et al., 2020 ), which means that genus Lachnospiraceae NK4A136 group is beneficial for protecting the intestinal barrier. The intestinal barrier acts as the first barrier to prevent harmful substances from penetrating the intestinal mucosa and damaging other tissues of the body. The disruption of IEB after stroke contributes to the microbial translocation which will increase the risk of post-stroke infections ( Zhao et al., 2023 ). Therefore, the protective effect of the genus Lachnospiraceae NK4A136 group on IEB may be helpful in IS prognosis.
High abundance of genus Lachnospiraceae UCG004 was also identified useful for IS prognosis. As probiotics in the body, it is beneficial for reducing obesity ( Xu et al., 2024 ). Evidence from a clinical study also showed a negative correlation between Lachnospiraceae UCG004 and cardiovascular disease risk factors ( Tindall et al., 2020 ). Another case–control study found a low abundance of Lachnospiraceae UCG004 in lacunar cerebral infarction patients compared with healthy controls ( Ma et al., 2023 ). It is worth noting that both the genus L achnospiraceae NK4A136 group and Lachnospiraceae UCG004 belong to family Lachnospiraceae which is recognized as short chain fatty acid (SCFA)-producer. In most studies, increased inflammation response and decreased SCFAs could be observed in stroke individuals and were significantly related to poor IS outcomes ( Spychala et al., 2018 ; Tan et al., 2021 ). Animal-based studies proved that transplantation of SCFAs-rich gut microbiota or SCFA supplementation in drinking water can effectively promote the recovery following ischemic stroke ( Chen et al., 2019 ; Lee et al., 2020 ; Sadler et al., 2020 ). Studies showed that SCFAs participate in the regulatory process of inflammatory responses, including promoting the anti-inflammatory cytokines and suppressing the pro-inflammatory cytokines ( Maslowski et al., 2009 ; Vinolo et al., 2011 ). This regulatory effect of SCFAs on inflammation may be one of the mechanisms by which GM affects the prognosis of stroke ( Iadecola and Anrather, 2011 ; Chamorro et al., 2012 ).
The underlying mechanisms involved in the influence of GM on outcomes following IS are multifaceted. In addition to the above-mentioned content, neurotransmitters and TMAO pathway ( Zhu et al., 2021 ), among others are involved in the regulation of GM on stroke. In conclusion, more research is needed to confirm whether and how the four taxa identified in this study are involved in these mechanisms.
To date, most studies evaluating the role of gut microbiome in stroke have focused on bacteria due to the overwhelming abundance of bacteria. Research on viruses, fungi, and archaea is scarce. However, these non-bacteria gut microbes play an important role in human health and diseases ( Goralska et al., 2018 ; Mukhopadhya et al., 2019 ; Coker, 2022 ; Ezzatpour et al., 2023 ). There is a growing body of evidence that suggests non-bacteria gut microbes are associated with neurological diseases, including stroke ( Forbes et al., 2018 ). A new study found that fecal viral taxa was altered significantly after stroke and dissimilar phage protein networks in mice ( Chelluboina et al., 2022 ). Phages are regarded as the most identified human gut virus components with significant roles. Phages can infect diverse bacterial phyla in the gut, such as Firmicutes, Bacteroidetes, Proteobacteria, and Actinobacteria ( Mirzaei and Maurice, 2017 ). When healthy people took phage dietary supplements orally, there was an increase in the butyrate-producing genus, Eubacterium ( Febvre et al., 2019 ). In addition to the viruses, some fungi housed in the gastrointestinal tract are considered to be pathogenic and can destroy CNS astrocytes, leading to BBB disruption and central infection. Colonizing the gut by archaea has also been demonstrated to decrease levels of trimethylamine ( Forbes et al., 2018 ), a compound produced by intestinal bacteria that is linked to an increased risk of atherosclerosis as well as cardiovascular and cerebrovascular diseases. Hence, the role of non-bacterial microorganisms in stroke is also one of the important directions of future stroke research. Unfortunately, there is no GWAS data for non-bacterial microorganisms, and the causal relationship between stroke and stroke prognosis cannot be further explored in this study. Nevertheless, we are eagerly looking forward to the improvement of the GWAS data that will enable us to delve deeper into this fascinating area of research in the future.
The MR analysis we designed is unlikely to be biased because of confounding factors and reverse causality. We also drew a relatively consistent and reliable conclusion from the data obtained from two sets of outcomes which were with or without adjustment for the baseline NIHSS. Although we have tried our best to make the conclusions accurate and robust, there are still some limiting factors that need to be considered in our research. First, the study's limitation in not being able to mine data below the genus level indeed restricts the depth of understanding of the potential links between the gut microbiota and outcomes of ischemic stroke. Second, a test about the associations between GM and post-stroke outcomes for different stroke subtypes was not performed because of the lack of available GWAS data in the GISCOME database. Different types, localization of stroke have a decisive influence on recovery in survivors ( Biffi et al., 2015 ; Zhang K. et al., 2020 ), which may contribute to some bias in our results. Third, we did not adequately control for age and sex among the patients included in the study. Thus, age and sex need to be taken into account in further investigations on the relationship between GM and post-stroke outcomes because the formation and shaping of the GM are easily influenced by sex and lifespan ( Snigdha et al., 2022 ). Fourth, a previous MR analysis has indicated that genetically determined GM was associated with the onset of ischemic stroke ( Meng et al., 2023 ), which made the collider bias a non-negligible issue in this MR investigation of IS prognosis ( Paternoster et al., 2017 ). Fifth, a small sample size of subjects ( n = 6,021) included in this study were only of European ancestry, which may restrict the generalizability of our findings to other populations. Thus, future studies with a larger sample size of other ancestries are needed to explore the associations between GM and ischemic stroke outcomes.
In summary, this MR analysis demonstrated that genetically predicted gut microbiota is causally associated with worse post-stroke outcomes. Our results suggest that interventions addressing particular GM taxa, such as Lachnospiraceae NK4A136, Lachnospiraceae UCG004, Ruminococcaceae UCG005 , and Eubacterium oxidoreducens groups, may provide new opportunities to improve recovery after ischemic stroke.
Data availability statement
The original contributions presented in the study are included in the article/ Supplementary material , further inquiries can be directed to the corresponding authors.
Author contributions
AZ: Conceptualization, Writing – review & editing. PL: Software, Writing – review & editing. YC: Software, Writing – review & editing. XW: Data curation, Writing – review & editing. JZ: Data curation, Writing – review & editing. LL: Methodology, Writing – review & editing. TZ: Conceptualization, Writing – original draft. JY: Funding acquisition, Writing – original draft.
The author(s) declare that financial support was received for the research, authorship, and/or publication of this article. This study was supported by the SMC Joint Project (Grant No. SMC 2013) and the National Natural Science Foundation of China (No. 82305425).
Acknowledgments
The GWAS data used in this MR study were from the MiBioGen consortium and the GISCOME network. We are grateful to the participants in the original study for their contributions, without whose efforts this research would not have been realized. We would especially like to thank Zhijia Zhou for his technical support on MR analysis.
Conflict of interest
The authors declare that the research was conducted in the absence of any commercial or financial relationships that could be construed as a potential conflict of interest.
Publisher's note
All claims expressed in this article are solely those of the authors and do not necessarily represent those of their affiliated organizations, or those of the publisher, the editors and the reviewers. Any product that may be evaluated in this article, or claim that may be made by its manufacturer, is not guaranteed or endorsed by the publisher.
Supplementary material
The Supplementary Material for this article can be found online at: https://www.frontiersin.org/articles/10.3389/fmicb.2024.1346371/full#supplementary-material
Abbreviations
IS, ischemic stroke; GM, gut microbiota; TMAO, trimethylamine-N-oxide; mRS, modified Rankin scale; MR, Mendelian randomization; NIHSS, National Institute of Health Stroke Scale; GWAS, genome-wide association study; RCT, randomized controlled trial; SNP, single-nucleotide polymorphism; IV, instrumental variable; GISCOME, Genetics of Ischemic Stroke Functional Outcome; LD, linkage disequilibrium; IVW, Inverse variance weighted; InSIDE, instrument strength is independent of direct effect; FDR, false discovery rate; BMI, body mass index; CI, confidence interval; OR, odds ratio; HDL-C, high-density lipoprotein cholesterol; MSD, maternal sleep deprivation; LPS, lipopolysaccharide; HFD, high-fat diet; IEB, intestinal epithelial barrier; BBB, blood–brain barrier; SCFA, short chain fatty acid; T 2 DM, type 2 diabetes mellitus.
Biffi, A., Anderson, C. D., Battey, T. W., Ayres, A. M., Greenberg, S. M., Viswanathan, A., et al. (2015). Association between blood pressure control and risk of recurrent intracerebral hemorrhage. JAMA . 314, 904–912. doi: 10.1001/jama.2015.10082
PubMed Abstract | Crossref Full Text | Google Scholar
Bowden, J., Davey Smith, G., and Burgess, S. (2015). Mendelian randomization with invalid instruments: effect estimation and bias detection through Egger regression. Int. J. Epidemiol . 44, 512–525. doi: 10.1093/ije/dyv080
Bowden, J., Davey Smith, G., Haycock, P. C., and Burgess, S. (2016). Consistent estimation in mendelian randomization with some invalid instruments using a weighted median estimator. Genet. Epidemiol . 40, 304–314. doi: 10.1002/gepi.21965
Cai, H., Zhang, H., Liang, J., Liu, Z., and Huang, G. (2023). Genetic liability to frailty in relation to functional outcome after ischemic stroke. Int. J. Stroke 19, 50–57. doi: 10.1177/17474930231194676
Carter, A. R., Sanderson, E., Hammerton, G., Richmond, R. C., Davey Smith, G., Heron, J., et al. (2021). Mendelian randomisation for mediation analysis: current methods and challenges for implementation. Eur. J. Epidemiol . 36, 465–478. doi: 10.1007/s10654-021-00757-1
Chamorro, A., Meisel, A., Planas, A. M., Urra, X., van de Beek, D., Veltkamp, R., et al. (2012). The immunology of acute stroke. Nat. Rev. Neurol . 8, 401–410. doi: 10.1038/nrneurol.2012.98
Crossref Full Text | Google Scholar
Chang, Y., Woo, H. G., Jeong, J. H., Kim, G. H., Park, K. D., Song, T. J., et al. (2021). Microbiota dysbiosis and functional outcome in acute ischemic stroke patients. Sci. Rep . 11:10977. doi: 10.1038/s41598-021-90463-5
Chelluboina, B., Kieft, K., Breister, A., Anantharaman, K., and Vemuganti, R. (2022). Gut virome dysbiosis following focal cerebral ischemia in mice. J. Cereb. Blood Flow Metab . 42, 1597–1602. doi: 10.1177/0271678X221107702
Chen, R., Xu, Y., Wu, P., Zhou, H., Lasanajak, Y., Fang, Y., et al. (2019). Transplantation of fecal microbiota rich in short chain fatty acids and butyric acid treat cerebral ischemic stroke by regulating gut microbiota. Pharmacol. Res . 148:104403. doi: 10.1016/j.phrs.2019.104403
Coker, O. O. (2022). Non-bacteria microbiome (virus, fungi, and archaea) in gastrointestinal cancer. J. Gastroenterol. Hepatol . 37, 256–262. doi: 10.1111/jgh.15738
Dai, X. C., Yu, Y., Zhou, S. Y., Yu, S., Xiang, M. X., Ma, H., et al. (2024). Assessment of the causal relationship between gut microbiota and cardiovascular diseases: a bidirectional Mendelian randomization analysis. BioData Min . 17:6. doi: 10.1186/s13040-024-00356-2
Davies, N. M., Holmes, M. V., and Davey Smith, G. (2018). Reading Mendelian randomisation studies: a guide, glossary, and checklist for clinicians. BMJ 362:k601. doi: 10.1136/bmj.k601
DeLong, J. H., Ohashi, S. N., O'Connor, K. C., and Sansing, L. H. (2022). Inflammatory responses after ischemic stroke. Semin. Immunopathol . 44, 625–648. doi: 10.1007/s00281-022-00943-7
Esquivel-Hernandez, D. A., Martinez-Lopez, Y. E., Sanchez-Castaneda, J. P., Neri-Rosario, D., Padron-Manrique, C., Giron-Villalobos, D., et al. (2023). A network perspective on the ecology of gut microbiota and progression of type 2 diabetes: linkages to keystone taxa in a Mexican cohort. Front. Endocrinol . 14:1128767. doi: 10.3389/fendo.2023.1128767
Ezzatpour, S., Mondragon Portocarrero, A. D. C., Cardelle-Cobas, A., Lamas, A., López-Santamarina, A., Miranda, J. M., et al. (2023). The human gut Virome and its relationship with nontransmissible chronic diseases. Nutrients 15:977. doi: 10.3390/nu15040977
Febvre, H. P., Rao, S., Gindin, M., Goodwin, N. D., Finer, E., Vivanco, J. S., et al. (2019). PHAGE study: effects of supplemental bacteriophage intake on inflammation and gut microbiota in healthy adults. Nutrients 11:666. doi: 10.3390/nu11030666
Forbes, J. D., Bernstein, C. N., Tremlett, H., Van Domselaar, G., and Knox, N. C. A. (2018). Fungal world: could the gut mycobiome be involved in neurological disease? Front. Microbiol . 9:3249. doi: 10.3389/fmicb.2018.03249
Geng, H., Li, M., Tang, J., Lv, Q., Li, R., Wang, L., et al. (2022). Early rehabilitation exercise after stroke improves neurological recovery through enhancing angiogenesis in patients and cerebral ischemia rat model. Int. J. Mol. Sci . 23:10508. doi: 10.3390/ijms231810508
Gill, D., James, N. E., Monori, G., Lorentzen, E., Fernandez-Cadenas, I., Lemmens, R., et al. (2019). Genetically determined risk of depression and functional outcome after ischemic stroke. Stroke 50, 2219–2222. doi: 10.1161/STROKEAHA.119.026089
Gittler, M., and Davis, A. M. (2018). Guidelines for adult stroke rehabilitation and recovery. JAMA 319, 820–821. doi: 10.1001/jama.2017.22036
Goralska, K., Blaszkowska, J., and Dzikowiec, M. (2018). Neuroinfections caused by fungi. Infection 46, 443–459. doi: 10.1007/s15010-018-1152-2
Gu, Y., Jin, Q., Hu, J., Wang, X., Yu, W., Wang, Z., et al. (2023). Causality of genetically determined metabolites and metabolic pathways on osteoarthritis: a two-sample mendelian randomization study. J. Transl. Med . 21:357. doi: 10.1186/s12967-023-04165-9
Guo, S., Nighot, M., Al-Sadi, R., Alhmoud, T., Nighot, P., Ma, T. Y., et al. (2015). Lipopolysaccharide regulation of intestinal tight junction permeability is mediated by TLR4 signal transduction pathway activation of FAK and MyD88. J. Immunol . 195, 4999–5010. doi: 10.4049/jimmunol.1402598
Gwak, M. G., and Chang, S. Y. (2021). Gut-brain connection: microbiome, gut barrier, and environmental sensors. Immune. Netw . 21:e20. doi: 10.4110/in.2021.21.e20
Hemani, G., Zheng, J., Elsworth, B., Wade, K. H., Haberland, V., Baird, D., et al. (2018). The MR-Base platform supports systematic causal inference across the human phenome. Elife 7:e34408. doi: 10.7554/eLife.34408
Herpich, F., and Rincon, F. (2020). Management of acute ischemic stroke. Crit. Care Med . 48, 1654–1663. doi: 10.1097/CCM.0000000000004597
Huang, A., Ji, L., Li, Y., Li, Y., and Yu, Q. (2023). Gut microbiome plays a vital role in post-stroke injury repair by mediating neuroinflammation. Int. Immunopharmacol . 118:110126. doi: 10.1016/j.intimp.2023.110126
Iadecola, C., and Anrather, J. (2011). The immunology of stroke: from mechanisms to translation. Nat. Med . 17, 796–808. doi: 10.1038/nm.2399
Kurilshikov, A., Medina-Gomez, C., Bacigalupe, R., Radjabzadeh, D., Wang, J., Demirkan, A., et al. (2021). Large-scale association analyses identify host factors influencing human gut microbiome composition. Nat. Genet . 53, 156–165. doi: 10.1038/s41588-020-00763-1
Lau, L. H., Lew, J., Borschmann, K., Thijs, V., and Ekinci, E. I. (2019). Prevalence of diabetes and its effects on stroke outcomes: a meta-analysis and literature review. J. Diab. Investig . 10, 780–792. doi: 10.1111/jdi.12932
Lee, J., d'Aigle, J., Atadja, L., Quaicoe, V., Honarpisheh, P., Ganesh, B. P., et al. (2020). Gut microbiota-derived short-chain fatty acids promote poststroke recovery in aged mice. Circ. Res . 127, 453–465. doi: 10.1161/CIRCRESAHA.119.316448
Li, C., Liu, C., and Li, N. (2022). Causal associations between gut microbiota and adverse pregnancy outcomes: a two-sample Mendelian randomization study. Front. Microbiol . 13:1059281. doi: 10.3389/fmicb.2022.1059281
Li, K. H. C., Jesuthasan, A., Kui, C., Davies, R., Tse, G., Lip, G. Y. H., et al. (2021). Acute ischemic stroke management: concepts and controversies: a narrative review. Expert. Rev. Neurother . 21, 65–79. doi: 10.1080/14737175.2021.1836963
Li, N., Wang, X., Sun, C., Wu, X., Lu, M., Si, Y., et al. (2019). Change of intestinal microbiota in cerebral ischemic stroke patients. BMC Microbiol . 19:191. doi: 10.1186/s12866-019-1552-1
Li, P., Wang, H., Guo, L., Gou, X., Chen, G., Lin, D., et al. (2022). Association between gut microbiota and preeclampsia-eclampsia: a two-sample Mendelian randomization study. BMC Med . 20:443. doi: 10.1186/s12916-022-02657-x
Long, Y., Tang, L., Zhou, Y., Zhao, S., and Zhu, H. (2023). Causal relationship between gut microbiota and cancers: a two-sample Mendelian randomisation study. BMC Med . 21:66. doi: 10.1186/s12916-023-02761-6
Ma, J., Xie, H., Yuan, C., Shen, J., Chen, J., Chen, Q., et al. (2023). The gut microbial signatures of patients with lacunar cerebral infarction. Nutr . Neurosci . 2023, 1–17. doi: 10.1080/1028415X.2023.2242121
Ma, L., Ni, Y., Wang, Z., Tu, W., Ni, L., Zhuge, F., et al. (2020). Spermidine improves gut barrier integrity and gut microbiota function in diet-induced obese mice. Gut Microbes . 12, 1–19. doi: 10.1080/19490976.2020.1832857
Maslowski, K. M., Vieira, A. T., Ng, A., Kranich, J., Sierro, F., Yu, D., et al. (2009). Regulation of inflammatory responses by gut microbiota and chemoattractant receptor GPR43. Nature 461, 1282–1286. doi: 10.1038/nature08530
Meng, C., Deng, P., Miao, R., Tang, H., Li, Y., Wang, J., et al. (2023). Gut microbiome and risk of ischaemic stroke: a comprehensive Mendelian randomization study. Eur. J. Prev. Cardiol . 30, 613–620. doi: 10.1093/eurjpc/zwad052
Mirzaei, M. K., and Maurice, C. F. (2017). Menage a trois in the human gut: interactions between host, bacteria and phages. Nat. Rev. Microbiol . 15, 397–408. doi: 10.1038/nrmicro.2017.30
Mukhopadhya, I., Segal, J. P., Carding, S. R., Hart, A. L., and Hold, G. L. (2019). The gut virome: the 'missing link' between gut bacteria and host immunity? Therap. Adv. Gastroenterol . 12:1756284819836620. doi: 10.1177/1756284819836620
Murray, C. J., and Lopez, A. D. (2013). Measuring the global burden of disease. N. Engl. J. Med . 369, 448–457. doi: 10.1056/NEJMra1201534
Park, S. Y., Lee, S. P., Kim, D., and Kim, W. J. (2023). Gut dysbiosis: a new avenue for stroke prevention and therapeutics. Biomedicines 11:2352. doi: 10.3390/biomedicines11092352
Paternoster, L., Tilling, K., and Davey Smith, G. (2017). Genetic epidemiology and Mendelian randomization for informing disease therapeutics: Conceptual and methodological challenges. PLoS Genet . 13:e1006944. doi: 10.1371/journal.pgen.1006944
Paul, S., and Candelario-Jalil, E. (2021). Emerging neuroprotective strategies for the treatment of ischemic stroke: an overview of clinical and preclinical studies. Exp. Neurol . 335:113518. doi: 10.1016/j.expneurol.2020.113518
Peng, X., Luo, Z., He, S., Zhang, L., and Li, Y. (2021). Blood-brain barrier disruption by lipopolysaccharide and sepsis-associated encephalopathy. Front. Cell Infect. Microbiol . 11:768108. doi: 10.3389/fcimb.2021.768108
Pluta, R., Januszewski, S., and Czuczwar, S. J. (2021). The role of gut microbiota in an ischemic stroke. Int. J. Mol. Sci . 22:915. doi: 10.3390/ijms22020915
Qin, S., He, Z., Wu, Y., Zeng, C., Zheng, Z., Zhang, H., et al. (2022). Instant dark tea alleviates hyperlipidaemia in high-fat diet-fed rat: from molecular evidence to redox balance and beyond. Front. Nutr . 9:819980. doi: 10.3389/fnut.2022.819980
Sadler, R., Cramer, J. V., Heindl, S., Kostidis, S., Betz, D., Zuurbier, K. R., et al. (2020). Short-chain fatty acids improve poststroke recovery via immunological mechanisms. J. Neurosci . 40, 1162–1173. doi: 10.1523/JNEUROSCI.1359-19.2019
Sekula, P., Del Greco, M. F., Pattaro, C., and Kottgen, A. (2016). Mendelian randomization as an approach to assess causality using observational data. J. Am. Soc. Nephrol . 27, 3253–3265. doi: 10.1681/ASN.2016010098
Skrivankova, V. W., Richmond, R. C., Woolf, B. A. R., Yarmolinsky, J., Davies, N. M., Swanson, S. A., et al. (2021). Strengthening the reporting of observational studies in epidemiology using mendelian randomization: the STROBE-MR statement. JAMA 326, 1614–1621. doi: 10.1001/jama.2021.18236
Sleiman, P. M., and Grant, S. F. (2010). Mendelian randomization in the era of genomewide association studies. Clin. Chem . 56, 723–728. doi: 10.1373/clinchem.2009.141564
Smith, G. D., and Ebrahim, S. (2003). 'Mendelian randomization': can genetic epidemiology contribute to understanding environmental determinants of disease? Int. J. Epidemiol . 32, 1–22. doi: 10.1093/ije/dyg070
Snigdha, S., Ha, K., Tsai, P., Dinan, T. G., Bartos, J. D., Shahid, M., et al. (2022). Probiotics: potential novel therapeutics for microbiota-gut-brain axis dysfunction across gender and lifespan. Pharmacol. Ther . 231:107978. doi: 10.1016/j.pharmthera.2021.107978
Soderholm, M., Pedersen, A., Lorentzen, E., Stanne, T. M., Bevan, S., Olsson, M., et al. (2019). Genome-wide association meta-analysis of functional outcome after ischemic stroke. Neurology 92, e1271–e83. doi: 10.1212/WNL.0000000000007138
Spychala, M. S., Venna, V. R., Jandzinski, M., Doran, S. J., Durgan, D. J., Ganesh, B. P., et al. (2018). Age-related changes in the gut microbiota influence systemic inflammation and stroke outcome. Ann. Neurol . 84, 23–36. doi: 10.1002/ana.25250
Tan, C., Wu, Q., Wang, H., Gao, X., Xu, R., Cui, Z., et al. (2021). Dysbiosis of gut microbiota and short-chain fatty acids in acute ischemic stroke and the subsequent risk for poor functional outcomes. JPEN J. Parenter. Enteral Nutr . 45, 518–529. doi: 10.1002/jpen.1861
Tindall, A. M., McLimans, C. J., Petersen, K. S., Kris-Etherton, P. M., and Lamendella, R. (2020). Walnuts and vegetable oils containing oleic acid differentially affect the gut microbiota and associations with cardiovascular risk factors: follow-up of a randomized, controlled, feeding trial in adults at risk for cardiovascular disease. J. Nutr . 150, 806–817. doi: 10.1093/jn/nxz289
Vinolo, M. A., Rodrigues, H. G., Nachbar, R. T., and Curi, R. (2011). Regulation of inflammation by short chain fatty acids. Nutrients 3, 858–876. doi: 10.3390/nu3100858
Wang, H., Zhang, M., Li, J., Liang, J., Yang, M., Xia, G., et al. (2022). Gut microbiota is causally associated with poststroke cognitive impairment through lipopolysaccharide and butyrate. J. Neuroinflam . 19:76. doi: 10.1186/s12974-022-02435-9
Wang, J., Kurilshikov, A., Radjabzadeh, D., Turpin, W., Croitoru, K., Bonder, M. J., et al. (2018). Meta-analysis of human genome-microbiome association studies: the MiBioGen consortium initiative. Microbiome 6:101. doi: 10.1186/s40168-018-0479-3
Wang, M., Daghlas, I., Zhang, Z., Ye, D., Li, S., Liu, D., et al. (2023). Genetic liability to migraine and functional outcome after ischemic stroke. Eur. Stroke J . 8, 517–521. doi: 10.1177/23969873231164728
Winkelmeier, L., Faizy, T. D., Broocks, G., Meyer, L., Heitkamp, C., Brekenfeld, C., et al. (2023). Association between recanalization attempts and functional outcome after thrombectomy for large ischemic stroke. Stroke 54, 2304–2312. doi: 10.1161/STROKEAHA.123.042794
Xu, L., Tang, Z., Herrera-Balandrano, D. D., Qiu, Z., Li, B., Yang, Y., et al. (2024). In vitro fermentation characteristics of blueberry anthocyanins and their impacts on gut microbiota from obese human. Food Res. Int . 176:113761. doi: 10.1016/j.foodres.2023.113761
Xu, Q., Ni, J. J., Han, B. X., Yan, S. S., Wei, X. T., Feng, G. J., et al. (2021). Causal relationship between gut microbiota and autoimmune diseases: a two-sample mendelian randomization study. Front. Immunol . 12:746998. doi: 10.3389/fimmu.2021.746998
Yao, Z. Y., Li, X. H., Zuo, L., Xiong, Q., He, W. T., Li, D. X., et al. (2022). Maternal sleep deprivation induces gut microbial dysbiosis and neuroinflammation in offspring rats. Zool Res . 43, 380–390. doi: 10.24272/j.issn.2095-8137.2022.023
Zeng, M., Peng, M., Liang, J., and Sun, H. (2023). The role of gut microbiota in blood-brain barrier disruption after stroke. Mol. Neurobiol . 2023, 1–17. doi: 10.1007/s12035-023-03512-7
Zhang, K., Li, T., Tian, J., Li, P., Fu, B., Yang, X., et al. (2020). Subtypes of anterior circulation large artery occlusions with acute brain ischemic stroke. Sci. Rep . 10:3442. doi: 10.1038/s41598-020-60399-3
Zhang, X. Y., Chen, J., Yi, K., Peng, L., Xie, J., Gou, X., et al. (2020). Phlorizin ameliorates obesity-associated endotoxemia and insulin resistance in high-fat diet-fed mice by targeting the gut microbiota and intestinal barrier integrity. Gut Microbes . 12, 1–18. doi: 10.1080/19490976.2020.1842990
Zhang, Z., Wang, M., Gill, D., and Liu, X. (2022). Genetically predicted smoking and alcohol consumption and functional outcome after ischemic stroke. Neurology 99, e2693–e2698. doi: 10.1212/WNL.0000000000201291
Zhang, Z., Wang, M., Gill, D., Zhu, W., and Liu, X. (2023). Genetically predicted sleep traits and functional outcome after ischemic stroke: a mendelian randomization study. Neurology 100, e1159–e65. doi: 10.1212/WNL.0000000000206745
Zhao, L., Xiao, J., Li, S., Guo, Y., Fu, R., Hua, S., et al. (2023). The interaction between intestinal microenvironment and stroke. CNS NeuroSci. Ther . 29, 185–199. doi: 10.1111/cns.14275
Zhao, Y., Zhang, X., Chen, X., and Wei, Y. (2022). Neuronal injuries in cerebral infarction and ischemic stroke: From mechanisms to treatment (Review). Int. J. Mol. Med . 49, 1–9. doi: 10.3892/ijmm.2021.5070
Zhu, W., Romano, K. A., Li, L., Buffa, J. A., Sangwan, N., Prakash, P., et al. (2021). Gut microbes impact stroke severity via the trimethylamine N-oxide pathway. Cell Host Microbe . 29, 1199–208.e5. doi: 10.1016/j.chom.2021.05.002
Keywords: gut microbiota, ischemic stroke, functional outcome, causal relationship, Mendelian randomization
Citation: Zhu A, Li P, Chu Y, Wei X, Zhao J, Luo L, Zhang T and Yan J (2024) Causal effects of gut microbiota on the prognosis of ischemic stroke: evidence from a bidirectional two-sample Mendelian randomization study. Front. Microbiol. 15:1346371. doi: 10.3389/fmicb.2024.1346371
Received: 19 December 2023; Accepted: 25 March 2024; Published: 08 April 2024.
Reviewed by:
Copyright © 2024 Zhu, Li, Chu, Wei, Zhao, Luo, Zhang and Yan. This is an open-access article distributed under the terms of the Creative Commons Attribution License (CC BY) . The use, distribution or reproduction in other forums is permitted, provided the original author(s) and the copyright owner(s) are credited and that the original publication in this journal is cited, in accordance with accepted academic practice. No use, distribution or reproduction is permitted which does not comply with these terms.
*Correspondence: Tao Zhang, tcm_zhang@126.com ; Juntao Yan, doctoryjt@163.com
† These authors have contributed equally to this work
Disclaimer: All claims expressed in this article are solely those of the authors and do not necessarily represent those of their affiliated organizations, or those of the publisher, the editors and the reviewers. Any product that may be evaluated in this article or claim that may be made by its manufacturer is not guaranteed or endorsed by the publisher.
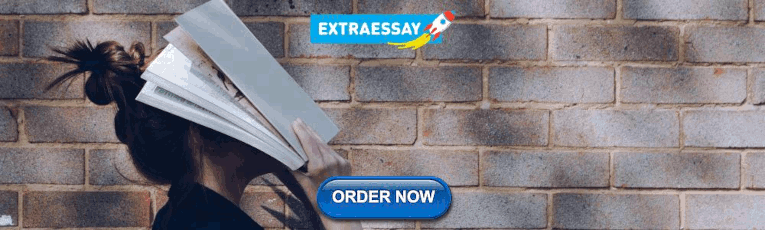
COMMENTS
Nominal data can be expressed in words or in numbers. But even if there are numerical labels for your data, you can't order the labels in a meaningful way or perform arithmetic operations with them. In social scientific research, nominal variables often include gender, ethnicity, political preferences or student identity number.
For example, data on the type of medication used, the stage of the disease, and patient demographic information can be analyzed using nominal variables. Education research: Nominal variables are used in education research to categorize data related to student demographics, academic performance, and other factors that may influence student success.
Nominal Variables Examples. 1. Gender. Gender, with categories typically including "male", "female", and "other", is a primary example of a nominal variable. Unlike ordinal variables, these categories have no presumed order or ranking. 2. Marital Status.
Nominal. 2. Ordinal. 3. Interval. 4. Ratio. In this post, we define each measurement scale and provide examples of variables that can be used with each scale. Nominal. The simplest measurement scale we can use to label variables is a nominal scale. Nominal scale: A scale used to label variables that have no quantitative values.
Nominal data is labelled into mutually exclusive categories within a variable. These categories cannot be ordered in a meaningful way. For example, pref erred mode of transportation is a nominal variable, because the data is sorted into categories: car, bus, train, tram, bicycle, etc.
Nominal data (also known as nominal scale) is a classification of categorical variables, that do not provide any quantitative value. Coined from the Latin nomenclature "Nomen" (meaning name), it is sometimes called "labeled" or "named" data. In some cases, nominal data may qualify as both quantitative and qualitative.
For example, literary genre is a nominal variable that can have the following categories: science fiction, drama, and comedy. Nominal Data Examples. For the following examples, remember that the nominal definition means "names." ... can't meaningfully order the groups. Social science research often collects a lot of nominal data in the ...
Nominal variables can be divided into categories, but there is no order or hierarchy to the categories. Ordinal variables, on the other hand, can be divided into categories that naturally follow some kind of order. For example, the variable "hair color" is nominal as it can be divided into various categories (brown, blonde, gray, black, etc ...
Nominal Data Definition. Nominal data is the simplest form of data, and is defined as data that is used for naming or labelling variables. Nominal data is the statistical data type that has the following characteristics: Nominal Data are observed, not measured, are unordered, non-equidistant and have no meaningful zero.
Following are a few examples of nominal data for your understanding: Eye Color: Black, Brown, Blue, Green, etc. Blood Group: A negative, B positive, O negative, O positive, etc. Religion: Christianity, Buddhism, Islam, etc. Political Affiliation: XYZ, YZE, UIO, OPT, etc. You might have noticed that in all these examples, the characteristics are ...
Testing hypotheses enables determining the independence of two nominal variables from a single sample. Nominal Data Examples. In each of the below-mentioned examples, there are labels associated with each of the answer options only for the purpose of labeling. ... Using QuestionPro Research Suite for Nominal Data Collection and Analysis .
For example, if we are conducting a survey on favorite movie genres and the categories include "Action," "Comedy," "Drama," and "Horror," each genre represents a nominal variable. Examples of Nominal Variables. Nominal variables are prevalent in various fields and research studies. Here are some common examples: Gender: Male, Female, Other ...
Binary variables are a type of nominal data. These data can have only two values. Statisticians also refer to binary data as indicator variables and dichotomous data. For example, male/female, pass/fail, and the presence/absence of an attribute are all binary data.
This post is part of our dissertation mini-course, which covers everything you need to get started with your dissertation, thesis or research project. Check out the free course. Learn about the 4 levels of measurement - nominal, ordinal, interval and ratio. Includes loads of practical examples and analogies.
In statistics, Nominal data is qualitative data that groups variables into categories that do not overlap. Nominal data is the simplest measure level and are considered the foundation of statistical analysis and all other mathematical sciences. They are individual pieces of information recorded and used for analysis.
Categorical Variable. This is a variable that can take on a limited number of values or categories. Categorical variables can be nominal or ordinal. Nominal variables have no inherent order, while ordinal variables have a natural order. Examples of categorical variables include gender, race, and educational level.
Data Collection. Nominal, Ordinal, Interval & Ratio Variable + [Examples] Measurement variables, or simply variables are commonly used in different physical science fields—including mathematics, computer science, and statistics. It has a different meaning and application in each of these fields. In algebra, which is a common aspect of ...
A nominal variable is a categorical variable that does not have any intrinsic ordering or ranking. Such a variable is qualitative in nature and arithmetic or logical operations cannot be performed on it. A nominal variable follows a nominal scale of measurement. The types of nominal variables are open-ended, closed-ended, numeric, and non ...
In statistical research, a variable is defined as an attribute of an object of study. ... Example: Variables If you want to test whether some plant species are more salt-tolerant than others, ... This example sheet is colour-coded according to the type of variable: nominal, continuous, ordinal, and binary. Prevent plagiarism, run a free check. ...
A variable is an essential component of any statistical data. It is a feature of a member of a given sample or population, which is unique, and can differ in quantity or quantity from another member of the same sample or population. Variables either are the primary quantities of interest or act as practical substitutes for the same.
The independent variable, often denoted as X, is the variable that is manipulated or controlled by the researcher intentionally. It's the factor that researchers believe may have a causal effect on the dependent variable. In simpler terms, the independent variable is the variable you change or vary in an experiment so you can observe its impact ...
In conclusion, more research is needed to confirm whether and how the four taxa identified in this study are involved in these mechanisms. To date, most studies evaluating the role of gut microbiome in stroke have focused on bacteria due to the overwhelming abundance of bacteria. Research on viruses, fungi, and archaea is scarce.